Click through the PLOS taxonomy to find articles in your field.
For more information about PLOS Subject Areas, click here .
Loading metrics
Open Access
Peer-reviewed
Research Article
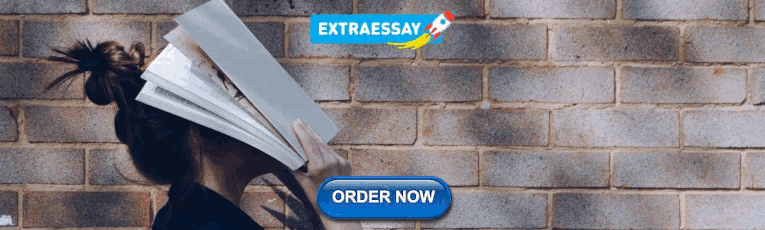
Multi-Reader Multi-Case Studies Using the Area under the Receiver Operator Characteristic Curve as a Measure of Diagnostic Accuracy: Systematic Review with a Focus on Quality of Data Reporting
Affiliation Department of Radiology, Prince of Songkla University, Hat Yai, Thailand
Affiliation Centre for Medical Imaging, University College London, London, United Kingdom
* E-mail: [email protected]
Affiliation Nuffield Department of Primary Care Health Sciences, Oxford University, Oxford, United Kingdom
Affiliation Centre for Statistics in Medicine, Wolfson College, Oxford University, Oxford, United Kingdom
- Thaworn Dendumrongsup,
- Andrew A. Plumb,
- Steve Halligan,
- Thomas R. Fanshawe,
- Douglas G. Altman,
- Susan Mallett
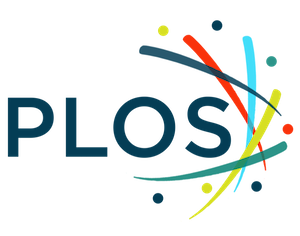
- Published: December 26, 2014
- https://doi.org/10.1371/journal.pone.0116018
- Reader Comments
Introduction
We examined the design, analysis and reporting in multi-reader multi-case (MRMC) research studies using the area under the receiver-operating curve (ROC AUC) as a measure of diagnostic performance.
We performed a systematic literature review from 2005 to 2013 inclusive to identify a minimum 50 studies. Articles of diagnostic test accuracy in humans were identified via their citation of key methodological articles dealing with MRMC ROC AUC. Two researchers in consensus then extracted information from primary articles relating to study characteristics and design, methods for reporting study outcomes, model fitting, model assumptions, presentation of results, and interpretation of findings. Results were summarized and presented with a descriptive analysis.
Sixty-four full papers were retrieved from 475 identified citations and ultimately 49 articles describing 51 studies were reviewed and extracted. Radiological imaging was the index test in all. Most studies focused on lesion detection vs. characterization and used less than 10 readers. Only 6 (12%) studies trained readers in advance to use the confidence scale used to build the ROC curve. Overall, description of confidence scores, the ROC curve and its analysis was often incomplete. For example, 21 (41%) studies presented no ROC curve and only 3 (6%) described the distribution of confidence scores. Of 30 studies presenting curves, only 4 (13%) presented the data points underlying the curve, thereby allowing assessment of extrapolation. The mean change in AUC was 0.05 (−0.05 to 0.28). Non-significant change in AUC was attributed to underpowering rather than the diagnostic test failing to improve diagnostic accuracy.
Conclusions
Data reporting in MRMC studies using ROC AUC as an outcome measure is frequently incomplete, hampering understanding of methods and the reliability of results and study conclusions. Authors using this analysis should be encouraged to provide a full description of their methods and results.
Citation: Dendumrongsup T, Plumb AA, Halligan S, Fanshawe TR, Altman DG, Mallett S (2014) Multi-Reader Multi-Case Studies Using the Area under the Receiver Operator Characteristic Curve as a Measure of Diagnostic Accuracy: Systematic Review with a Focus on Quality of Data Reporting. PLoS ONE 9(12): e116018. https://doi.org/10.1371/journal.pone.0116018
Editor: Delphine Sophie Courvoisier, University of Geneva, Switzerland
Received: September 23, 2014; Accepted: December 2, 2014; Published: December 26, 2014
Copyright: © 2014 Dendumrongsup et al. This is an open-access article distributed under the terms of the Creative Commons Attribution License , which permits unrestricted use, distribution, and reproduction in any medium, provided the original author and source are credited.
Data Availability: The authors confirm that all data underlying the findings are fully available without restriction. All relevant data are within the paper and its Supporting Information files.
Funding: This work was supported by the UK National Institute for Health (NIHR) Research under its Programme Grants for Applied Research funding scheme (RP-PG-0407-10338). The funder had no role in the design, execution, analysis, reporting, or decision to submit for publication.
Competing interests: The authors have declared that no competing interests exist.
The receiver operator characteristic (ROC) curve describes a plot of sensitivity versus 1-specificity for a diagnostic test, across the whole range of possible diagnostic thresholds [1] . The area under the ROC curve (ROC AUC) is a well-recognised single measure that is often used to combine elements of both sensitivity and specificity, sometimes replacing these two measures. ROC AUC is often used to describe the diagnostic performance of radiological tests, either to compare the performance of different tests or the same test under different circumstances [2] , [3] . Radiological tests must be interpreted by human observers and a common study design uses multiple readers to interpret multiple image cases; the multi-reader multi-case (MRMC) design [4] . The MRMC design is popular because once a radiologist has viewed 20 cases there is less information to be gained by asking him to view a further 20 than by asking a different radiologist to view the same 20. This procedure enhances the generalisability of study results and having multiple readers interpret multiple cases enhances statistical power. Because multiple radiologists view the same cases, “clustering” occurs. For example, small lesions are generally seen less frequently than larger lesions, i.e. reader observations are clustered within cases. Similarly, more experienced readers are likely to perform better across a series of cases than less experienced readers, i.e. results are correlated within readers. Bootstrap resampling and multilevel modeling can account for clustering, linking results from the same observers and cases, so that 95% confidence intervals are not too narrow. MRMC studies using ROC AUC as the primary outcome are often required by regulatory bodies for the licensing of new radiological devices [5] .
We attempted to use ROC AUC as the primary outcome measure in a prior MRMC study of computer-assisted detection (CAD) for CT colonography [6] . However, we encountered several difficulties when trying to implement this approach, described in detail elsewhere [7] . Many of these difficulties were related to issues implementing confidence scores in a transparent and reliable fashion, which led ultimately to a flawed analysis. We considered, therefore, that for ROC AUC to be a valid measure there are methodological components that need addressing in study design, data collection and analysis, and interpretation. Based on our attempts to implement the MRMC ROC AUC analysis, we were interested in whether other researchers have encountered similar hurdles and, if so, how these issues were tackled.
In order to investigate how often other studies have addressed and reported on methodological issues with implementing ROC AUC, we performed a systematic review of MRMC studies using ROC AUC an outcome measure. We searched and investigated the available literature with the objective to describe the statistical methods used, the completeness of data presentation, and investigate whether any problems with analysis were encountered and reported.
Ethics statement
Ethical approval is not required by our institutions for research studies of published data.
Search strategy, inclusion and exclusion criteria
This systematic review was performed guided by the Preferred Reporting Items for Systematic Reviews and Meta-Analyses (PRISMA), an evidence-based minimum set of items for reporting in systematic reviews and meta-analyses [8] . We developed an extraction sheet for the systematic review, broken down into different sections (used as subheadings for the Results section of this report), with notes relating to each individual item extracted ( S1 File ). In consensus we considered approximately 50 articles would provide a sufficiently representative overview of current reporting practice. Based on our prior experience of performing systematic reviews we believed that searching for additional articles beyond 50 would be unlikely to yield valuable additional data (i.e. we believed we would reach “saturation” by 50 articles) yet would present a very considerable extraction burden.
In order to achieve this, potentially eligible primary articles published between 2005 and February 2013 inclusive were identified by a radiologist researcher (TD) using PUBMED via their citation of one or more of 8 key methodological articles relating to MRMC ROC AUC analysis [9] – [16] . To achieve this the Authors' names (combined using “AND”) were entered in the PUBMED search field and the specific article identified and clicked in the results list. The abstract was then accessed and the “Cited By # PubMed Central Articles” link and “Related Citations” link used to identify those articles in the PubMed Central database that have cited the original article. There was no language restriction. Online abstracts were examined in reverse chronological order, the full text of potentially eligible papers then retrieved, and selection stopped once the threshold of 50 studies fulfilling inclusion criteria had been passed.
To be eligible, primary studies had to be diagnostic test accuracy studies of human observers interpreting medical image data from real patients, and attempting to use a MRMC ROC AUC analysis as a study outcome based on the following methodological approaches [9] – [16] ; Reviews, solely methodological papers, and those using simulated imaging data were excluded.
Data extraction
An initial pilot sample of 5 full-paper articles were extracted and the data checked by a subgroup of investigators in consensus, to both confirm the process was feasible and to identify potential problems. These papers were extracted by TD using the search strategy described in the previous section. A further 10 full-papers were extracted by two radiologist researchers again using the same search strategy and working independently (TD, AP) to check agreement further. The remaining articles included in the review were extracted predominantly by TD, who discussed any concerns/uncertainty with AP. Any disagreement following their discussion was arbitrated by SH and/or SM where necessary. These discussions took place during two meetings when the authors met to discuss progress of the review; multiple papers and issues were discussed on each occasion.
The extraction covered the following broad topics: Study characteristics, methods to record study outcomes, model assumptions, model fitting, data presentation ( S1 File ).
We extracted data relating to the organ and disease studied, the nature of the diagnostic task (e.g. characterization vs. localization vs. presence/absence), test methods, patient source and characteristics, study design (e.g. prospective/retrospective, secondary analysis, single/multicenter) and reference standard. We extracted the number of readers, their prior experience, specific interpretation training for the study (e.g. use of CAD software), blinding to clinical data and/or reference results, the number of times they read each case and the presence of any washout period to diminish recall bias, case ordering, and whether all readers read all cases (i.e. a fully-crossed design). We extracted the unit of analysis (e.g. patient vs. organ vs. segment), and sample size for patients with and without pathology.
We noted whether study imaging reflected normal daily clinical practice or was modified for study purposes (e.g. restricted to limited images). We noted the confidence scores used for the ROC curve and their scale, and whether training was provided for scoring. We noted if there were multiple lesions per unit of analysis. We noted if scoring differed for positive and negative patient cases, whether score distribution was reported, and whether transformation to a normal distribution was performed.
We extracted if ROC cures were presented in the published article and, if so, whether for individual readers, whether the curve was smoothed, and if underlying data points were shown. We defined unreasonable extrapolation as an absence of data in the right-hand 25% of the plot space. We noted the method for curve fitting and whether any problems with fitting were reported, and the method used to compare AUC or pAUC. We extracted the primary outcome, the accuracy measures reported, and whether these were overall or for individual readers. We noted the size of any change in AUC, whether this was significant, and made a subjective assessment of whether significance could be attributed to a single reader or case. We noted how the study authors interpreted change in AUC, if any, and whether any change was reported in terms of effect on individual patients. We also noted if a ROC researcher was named as an author or acknowledged, defined as an individual who had published indexed research papers dealing with ROC methodology.
Data were summarized in an Excel worksheet (Excel For Mac 14.3.9, Microsoft Corporation) with additional cells for explanatory free text. A radiologist researcher (SH) then compiled the data and extracted frequencies, consulting the two radiologists who performed the extraction for clarification when necessary. The investigator group discussed the implication of the data subsequently, to guide interpretation.
Four hundred and seventy five citations of the 8 key methodological papers were identified and 64 full papers retrieved subsequently. Fifteen [17] – [31] of these were rejected after reading the full text (the papers and reason for rejection are shown in Table 1 ) leaving 49 [32] – [80] for extraction and analysis that were published between 2010 and 2012 inclusive; these are detailed in Table 1 . Two papers [61] , [75] contributed two separate studies each, meaning that 51 studies were extracted in total. The PRISMA checklist [8] is detailed in Fig. 1 . The raw extracted data are available in S2 File .
- PPT PowerPoint slide
- PNG larger image
- TIFF original image
https://doi.org/10.1371/journal.pone.0116018.g001
https://doi.org/10.1371/journal.pone.0116018.t001
Study characteristics
The index test was imaging in all studies. Breast was the commonest organ studied (20 studies), followed by lung (11 studies) and brain (7 studies). Mammography (15 studies) was the commonest individual modality investigated, followed by plain film (12 studies), CT and MRI (11 studies each), tomosynthesis (six studies), ultrasound (two studies) and PET (one study); 9 studies investigated multiple modalities. In most studies (28 studies) the prime interpretation task was lesion detection. Eleven studies focused on lesion characterization and 12 combined detection and characterization. Forty-one studies compared 2 tests/conditions (i.e. a single test but used in different ways) to a reference standard (41 studies), while 2 studies compared 1 test/condition, 7 studies compared 3 tests/conditions, and 1 study compared 4 tests/conditions. Twenty-five studies combined data to create a reference standard while the reference was a single finding in 24 (14 imaging, 5 histology, 5 other – e.g. endoscopy). The reference method was unclear in 2 studies [54] , [55] .
Twenty-four studies were single center, 12 multicenter, with the number of centers unclear in 15 (29%) studies. Nine studies recruited symptomatic patients, 8 asymptomatic, and 7 a combination, but the majority (53%; 27 studies) did not state whether patients were symptomatic or not. 42 (82%) studies described the origin of patients with half of these stating a precise geographical region or hospital name. However, 9 (18%) studies did not sufficiently describe the source of patients and 21 (41%) did not describe patients' age and/or gender distribution.
Study design
Extracted data relating to study design and readers are presented graphically in Fig. 2 . Most studies (29; 57%) used patient data collected retrospectively. Fourteen (28%) were prospective while 2 used an existing database. Whether prospective/retrospective data was used was unstated/unclear in a further 6 (12%). While 13 studies (26%) used cases unselected other than for the disease in question, the majority (34; 67%) applied further criteria, for example to preselect “difficult” cases (11 studies), or to enrich disease prevalence (4 studies). How this selection bias was applied was stated explicitly in 18 (53%) of these 34. Whether selection bias was used was unclear in 4 studies.
https://doi.org/10.1371/journal.pone.0116018.g002
The number of readers per study ranged from 2 [56] to 258 [76] . The mean number was 13, median 6. The large majority of studies (35; 69%) used fewer than 10 readers. Reader experience was described in 40 (78%) studies but not in 11. Specific reader training for image interpretation was described in 31 (61%) studies. Readers were not trained specifically in 14 studies and in 6 it was unclear whether readers were trained specifically or not. Readers were blind to clinical information for individual patients in 37 (73%) studies, unblind in 3, and this information was unrecorded or uncertain in 11 (22%). Readers were blind to prevalence in the dataset in 21 (41%) studies, unblind in 2, but this information was unsure/unrecorded or uncertain in the majority (28, 55%).
Observers read the same patient case on more than one occasion in 50 studies; this information was unclear in the single further study [70] . A fully crossed design (i.e. all readers read all patients with all modalities) was used in 47 (92%) studies, but not stated explicitly in 23 of these. A single study [72] did not use a fully crossed design and the design was unclear or unrecorded in 3 [34] , [70] , [76] . Case ordering was randomised (either a different random order across all readers or a different random order for each individual reader) between consecutive readings in 31 (61%) studies, unchanged in 6, and unclear/unrecorded in 14 (27%). The ordering of the index test being compared varied between consecutive readings in 20 (39%) studies, was unchanged in 17 (33%), and was unclear/unrecorded in 14 (27%). 26 (51%) studies employed a time interval between readings that ranged from 3 hours [50] to 2 months [63] , with a median of 4 weeks. There was no interval (i.e. reading of cases in all conditions occurred at the same sitting) in 17 (33%) studies, and time interval was unclear/unrecorded in 8 (16%).
Methods of reporting study outcomes
The unit of analysis for the ROC AUC analysis was the patient in 23 (45%) studies, an organ in 5, an organ segment in 5, a lesion in 11 (22%), other in 2, and unclear or unrecorded in 6 (12%); one study [34] examined both organ and lesion so there were 52 extractions for this item. Analysis was based on multiple images in 33 (65%) studies, a single image in 16 (31%), multiple modalities in a single study [40] , and unclear in a single study [57] ; no study used videos.
The number of disease positive patients per study ranged between 10 [79] and 100 [53] (mean 42, median 48) in 46 studies, and was unclear/unrecorded in 5 studies. The number of disease positive units of outcome for the primary ROC AUC analysis ranged between 10 [79] and 240 [41] (mean 59, median 50) in 43 studies, and was unclear/unrecorded in 8 studies. The number of disease negative patients per study ranged between 3 [69] and 352 [34] (mean 66, median 38) in 44 studies, was zero in 1 study [80] , and was unclear/unrecorded in 6 studies. The number of disease negative units of analysis for the primary outcome for the ROC AUC analysis ranged between 10 [51] and 535 [39] (mean 99, median 68) in 42 studies, and was unclear/unrecorded in the remaining 9 studies. The large majority of studies (41, 80%) presented readers with an image or set of images reflecting normal clinical practice whereas 10 presented specific lesions or regions of interest to readers.
Calculation of ROC AUC requires the use of confidence scores, where readers rate their confidence in the presence of a lesion or its characterization. In our previous study [6] we identified the assignment of confidence scores to be potentially on separate scales for disease positive and negative cases [7] . For rating scores used to calculate ROC AUC, 25 (49%) studies used a relatively small number of categories (defined as up to 10) and 25 (49%) used larger scales or a continuous measurement (e.g. visual analogue scale). One study did not specify the scale used [76] . Only 6 (12%) studies stated explicitly that readers were trained in advance to use the scoring system, for example being encouraged to use the full range available. In 15 (29%) studies there was the potential for multiple abnormalities in each unit of analysis (stated explicitly by 12 of these). This situation was dealt with by asking readers to assess the most advanced or largest lesion (e.g. [43] ), by an analysis using the highest score attributed (e.g. [42] ), or by adopting a per-lesion analysis (e.g. [52] ). For 23 studies only a single abnormality per unit of analysis was possible, whereas this issue was unclear in 13 studies.
Model assumptions
The majority of studies (41, 80%) asked readers to ascribe the same scoring system to both disease-positive and disease-negative patients. Another 9 studies asked that different scoring systems be used, depending on whether the case was perceived as positive or negative (e.g. [61] ), or depending on the nature of the lesion perceived (e.g. [66] ). Scoring was unclear in a single study [76] . No study stated that two types of true-negative classifications were possible (i.e. where a lesion was seen but misclassified vs. not being seen at all), a situation that potentially applied to 22 (43%) of the 51 studies. Another concern occurs when more than one observation for each patient is included in the analysis, violating the assumption that data are independent. This could occur if multiple diseased segments were analysed for each patient without using a statistical method that treats these as clustered data. An even more flawed approach occurs when analysis includes one segment for patients without disease but multiple segments for patients with disease.
When publically available DBM MRMC software [81] is used for ROC AUC modeling, this requires assumptions of normality for confidence scores or their transformations if the standard parametric ROC curve fitting methods are used. When scores are not normally distributed, even if non parametric approaches are used to estimate ROC AUC, this lack of normality may indicate additional problems with obtaining reliable estimates of ROC AUC [82] – [86] . While 17 studies stated explicitly that the data fulfilled the assumptions necessary for modeling, none described whether confidence scores were transformed to a normal distribution for analysis. Indeed, only 3 studies [54] , [73] , [76] described the distribution of confidence scores, which was non-normal in each case.
Model fitting
Thirty (59%) studies presented ROC curves based on confidence scores; i.e. 21 (41%) studies showed no ROC curve. Of the 30 with curves, only 5 presented a curve for each reader whereas 24 presented curves averaged over all readers; a further study presented both. Of the 30 studies presenting ROC curves, 26 (87%) showed only smoothed curves, with the data points underlying the ROC curve presented in only 4 (13%) [43] , [51] , [63] , [78] . Thus, a ROC curve with underlying data points was presented in only 4 of 51 (8%) studies overall. The degree of extrapolation is critical in understanding the reliability of the ROC AUC result [7] . However, extrapolation could only be assessed in these four articles, with unreasonable extrapolation, by our definition, occurring in two [43] , [63] .
The majority of studies (31, 61%) did not specify the method used for curve fitting. Of the 20 that did, 7 used non-parametric methods (Trapezoidal/Wilcoxon), 8 used parametric methods (7 of which used Proproc), 3 used other methods, and 2 used a combination. Previous research [7] , [84] has demonstrated considerable problems fitting ROC curves due to degenerate data where the fitted ROC curve corresponds to vertical and horizontal lines, e.g there are no FP data. Only 2 articles described problems with curve fitting [55] , [61] . Two studies stated that data was degenerate: Subhas and co-workers [66] stated that, “data were not well dispersed over the five confidence level scores”. Moin and co-workers [53] stated that, “If we were to recode categories 1 and 2, and discard BI-RADS 0 in the ROC analysis, it would yield degenerative results because the total number of cases collected would not be adequate”. While all studies used MRMC AUC methods to compare AUC outcomes, 5 studies also used other methods (e.g. t-testing) [37] , [52] , [60] , [67] , [77] . Only 3 studies described using a partial AUC [42] , [55] , [77] . Forty-four studies additionally reported non-AUC outcomes (e.g. McNemar's test to compare test performance at a specified diagnostic threshold [58] , Wilcoxon signed rank test to compare changes in patient management decisions [64] ). Eight (16%) of the studies included a ROC researcher as an author [39] , [47] , [48] , [54] , [60] , [65] , [66] , [72] .
Presentation of results
Extracted data relating to the presentation of individual study results is presented graphically in Fig. 3 . All studies presented ROC AUC as an accuracy measure with 49 (96%) presenting the change in AUC for the conditions tested. Thirty-five (69%) studies presented additional measures such as change in sensitivity/specificity (24 studies), positive/negative predictive values (5 studies), or other measures (e.g. changes in clinical management decisions [64] , intraobserver agreement [36] ). Change in AUC was the primary outcome in 45 (88%) studies. Others used sensitivity [34] , [40] , accuracy [35] , [69] , the absolute AUC [44] or JAFROC figure of merit [68] . All studies presented an average of the primary outcome over all readers, with individual reader results presented in 38 (75%) studies but not in 13 (25%). The mean change/difference in AUC was 0.051 (range −0.052 to 0.280) across the extracted studies and was stated as “significant” in 31 and “non-significant” in the remaining 20. No study failed to comment on significance of the stated change/difference in AUC. In 22 studies we considered that a significant change in AUC was unlikely to be due to results from a single reader/patient but we could not determine whether this was possible in 11 studies, and judged this not-applicable in a further 18 studies. One study appeared to report an advantage for a test when the AUC increased, but not significantly [65] . There were 5 (10%) studies where there appeared to be discrepancies between the data presented in the abstract/text/ROC curve [36] , [38] , [69] , [77] , [80] .
https://doi.org/10.1371/journal.pone.0116018.g003
While the majority of studies (42, 82%) did not present an interpretation of their data framed in terms of changes to individual patient diagnoses, 9 (18%) did so, using outcomes in addition to ROC AUC: For example, as a false-positive to true-positive ratio [35] or the proportion of additional biopsies precipitated and disease detected [64] , or effect on callback rate [43] . The change in AUC was non-significant in 22 studies and in 12 of these the authors speculated why, for example stating that the number of cases was likely to be inadequate [65] , [70] , that the observer task was insufficiently taxing [36] , or that the difference was too subtle to be resolved [45] . For studies where a non-significant change in AUC was observed, authors sometimes framed this as demonstrating equivalence (16 studies, e.g. [55] , [74] ), stated that there were other benefits (3 studies), or adopted other interpretations. For example, one study stated that there were “beneficial” effects on many cases despite a non-significant change in AUC [54] and one study stated that the intervention “improved visibility” of microcalcifications noting that the lack of any statistically significant difference warranted further investigation [65] .
While many studies have used ROC AUC as an outcome measure, very little research has investigated how these studies are conducted, analysed and presented. We could find only a single existing systematic review that has investigated this question [87] . The authors stated in their Introduction, “we are not aware of any attempt to provide an overview of the kinds of ROC analyses that have been most commonly published in radiologic research.” They investigated articles published in the journal “Radiology” between 1997 and 2006, identifying 295 studies [87] . The authors concluded that “ROC analysis is widely used in radiologic research, confirming its fundamental role in assessing diagnostic performance”. For the present review, we wished to focus on MRMC studies specifically, since these are most complex and are often used as the basis for technology licensing. We also wished to broaden our search criteria beyond a single journal. Our systematic review found that the quality of data reporting in MRMC studies using ROC AUC as an outcome measure was frequently incomplete and who would therefore agree with the conclusions of Shiraishi et al. who stated that studies, “were not always adequate to support clear and clinically relevant conclusions” [87] .
Many omissions we identified were those related to general study design and execution, and are well-covered by the STARD initiative [88] as factors that should be reported in studies of diagnostic test accuracy in general. For example, we found that the number of participating research centres was unclear in approximately one-third of studies, that most studies did not describe whether patients were symptomatic or asymptomatic, that criteria applied to case selection were sometimes unclear, and that observer blinding was not mentioned in one-fifth of studies. Regarding statistical methods, STARD states that studies should, “describe methods for calculating or comparing measures of diagnostic accuracy” [88] ; this systematic review aimed to focus on description of methods for MRMC studies using ROC AUC as an outcome measure.
The large majority of studies used less than 10 observers, some did not describe reader experience, and the majority did not mention whether observers were aware of prevalence of abnormality, a factor that may influence diagnostic vigilance. Most studies required readers to detect lesions while a minority asked for characterization, and others were a combination of the two. We believe it is important for readers to understand the precise nature of the interpretative task since this will influence the rating scale used to build the ROC curve. A variety of units of analysis were adopted, with just under half being the patient case. We were surprised that some studies failed to record the number of disease-positive and disease-negative patients in their dataset. Concerning the confidence scales used to construct the ROC curve, only a small minority (12%) of studies stated that readers were trained to use these in advance of scoring. We believe such training is important so that readers can appreciate exactly how the interpretative task relates to the scale; there is evidence that radiologists score in different ways when asked to perform the same scoring task because of differences in how they interpret the task [89] . For example, readers should appreciate how the scale reflects lesion detection and/or characterization, especially if both are required, and how multiple abnormalities per unit of analysis are handled. Encouragement to use the full range of the scale is required for normal rating distributions. Whether readers must use the same scale for patients with and without pathology is also important to know.
Despite their importance for understanding the validity of study results, we found that description of the confidence scores, the ROC curve and its analysis was often incomplete. Strikingly, only three studies described the distribution of confidence scores and none stated whether transformation to a normal distribution was needed. When publically available DBM MRMC software (ref DBM) is used for ROC AUC modeling, this requires assumptions of normality for confidence scores or their transformations when ROC curve fitting methods are used. Where confidence scores are not normally distributed these software methods are not recommended [84] – [86] , [90] . Although Hanley shows that ROC curves can be reasonable under some distributions of non normal data [91] , concerns have been raised particularly in imaging detection studies measuring clinically useful tests with good performance to distinguish well defined abnormalities. In tests with good performance two factors make estimation of ROC AUC unreliable. Firstly readers' scores are by definition often at the ends of the confidence scale so that the confidence score distributions for normal and abnormal cases have very little overlap [82] – [86] . Secondly tests with good performance also have few false positives making ROC AUC estimation highly dependent on confidence scores assigned to possibly fewer than 5% or 10% of cases in the study [86] .
Most studies did not describe the method used for curve fitting. Over 40% of studies presented no ROC curve in the published article. When present, the large majority were smoothed and averaged over all readers. Only four articles presented data points underlying the curve meaning that the degree of any extrapolation could not be assessed despite this being an important factor regarding interpretation of results [92] . While, by definition, all studies used MRMC AUC methods, most reported additional non-AUC outcomes. Approximately one-quarter of studies did not present AUC data for individual readers. Because of this, variability between readers and/or the effect of individual readers on the ultimate statistical analysis could not be assessed.
Interpretation of study results was variable. Notably, when no significant change in AUC was demonstrated, authors stated that the number of cases was either insufficient or that the difference could not be resolved by the study, appearing to claim that their studies were underpowered rather than that the intervention was ineffective when required to improve diagnostic accuracy. Indeed some studies claimed an advantage for a new test in the face of a non-significant increase in AUC, or turned to other outcomes as proof of benefit. Some interpreted no significant difference in AUC as implying equivalence.
Our review does have limitations. Indexing of the statistical methods used to analyse studies is not common so we used a proxy to identify studies; their citation of “key” references related to MRMC ROC methodology. While it is possible we missed some studies, our aim was not to identify all studies using such analyses. Rather, we aimed to gather a representative sample that would provide a generalizable picture of how such studies are reported. It is also possible that by their citation of methodological papers (and on occasion including a ROC researcher as an author), our review was biased towards papers likely to be of higher methodological quality than average. This systematic review was cross-disciplinary and two radiological researchers performed the bulk of the extraction rather than statisticians. This proved challenging since the depth of statistical knowledge required was demanding, especially when details of the analysis was being considered. We anticipated this and piloted extraction on a sample of five papers to determine if the process was feasible, deciding that it was. Advice from experienced statisticians was also available when uncertainty arose.
In summary, via systematic review we found that MRMC studies using ROC AUC as the primary outcome measure often omit important information from both the study design and analysis, and presentation of results is frequently not comprehensive. Authors using MRMC ROC analyses should be encouraged to provide a full description of their methods and results so as to increase interpretability.
Supporting Information
Extraction sheet used for the systematic review.
https://doi.org/10.1371/journal.pone.0116018.s001
Raw data extracted for the systematic review.
https://doi.org/10.1371/journal.pone.0116018.s002
S1 PRISMA Checklist.
https://doi.org/10.1371/journal.pone.0116018.s003
Author Contributions
Conceived and designed the experiments: TD AAP SH TRF DGA SM. Performed the experiments: TD AAP SH TRF DGA SM. Analyzed the data: TD AAP SH TRF DGA SM. Contributed reagents/materials/analysis tools: TD AAP SH TRF DGA SM. Wrote the paper: TD AAP SH TRF DGA SM.
- View Article
- Google Scholar
- 7. Mallett S, Halligan S, Collins GS, Altman DG (2014) Exploration of analysis methods for diagnostic imaging tests: Problems woth ROC AUC and confidence scores in CT colonography. PLoS One (in press).
- 81. v2.1 DMs. Available: http://www-radiology.uchicago.edu/krl/KRL_ROC/software_index6.htm .
- 85. Zhou XH, Obuchowski N, McClish DK (2002) Statistical methods in diagnostic medicine. New York NY: Wiley.
- Skip to main content
- Skip to FDA Search
- Skip to in this section menu
- Skip to footer links

The .gov means it’s official. Federal government websites often end in .gov or .mil. Before sharing sensitive information, make sure you're on a federal government site.
The site is secure. The https:// ensures that you are connecting to the official website and that any information you provide is encrypted and transmitted securely.
U.S. Food and Drug Administration
- Search
- Menu
- Medical Devices
- Science and Research | Medical Devices
iMRMC: Software to do Multi-reader Multi-case Statistical Analysis of Reader Studies
Catalog of Regulatory Science Tools to Help Assess New Medical Devices
Technical Description
The primary objective of the iMRMC statistical software is to assist investigators with analyzing and sizing multi-reader multi-case (MRMC) reader studies that compare the difference in the area under Receiver Operating Characteristic curves (AUCs) from two modalities. The iMRMC application is a software package that includes simulation tools to characterize bias and variance of the MRMC variance estimates.
The core elements of this application include the ability to perform MRMC variance analysis and the ability to size an MRMC trial.
- The core iMRMC application is a stand-alone, precompiled, license-free Java applications and the source code. It can be used in GUI mode or on the command line.
- There is also an R package that utilizes the core Java application. Examples for using the programs can be found in the R help files.
- Additional functionality of the GitHub package includes an example to guide users on how to perform a noninferiority study using the iMRMC R package.
The software treats arbitrary study designs that are not "fully-crossed."
Intended Purpose
The iMRMC package analyzes data from Multiple Readers and Multiple Cases (MRMC) studies, which are often imaging studies where clinicians (readers) evaluate patient images (cases). The MRMC methods apply to any scenario in which clinicians interpret data to make clinical decisions. The iMRMC package calculates the reader-averaged area under the receiver operating characteristic curve: the AUC of the ROC curve. AUC is a diagnostic performance measure. Additional functions analyze other endpoints (binary performance and score differences). This package also estimates variances, confidence intervals and p-values. These uncertainty characteristics are needed for hypothesis tests to size and assess the efficacy of diagnostic imaging devices and computer aids (artificial intelligence).
The analysis is important because imaging studies are designed so that every reader reads every case in all modalities, a fully-crossed study. In this case, the data is cross-correlated, and the readers and cases are considered to be cross-correlated random effects. An MRMC analysis accounts for the variability and correlations from the readers and cases when estimating variances, confidence intervals, and p-values. The functions in this package can treat arbitrary study designs and studies with missing data, not just fully-crossed study designs.
The methods in the iMRMC package are not standard. The package permits industry statisticians to use a validated statistical analysis method without having to develop and validate it themselves.
Related FDA Product Codes
The FDA product codes this tool is applicable to include, but are not limited to:
- KPS: System, Tomography, Computed, Emission
- LLZ: System, Image Processing, Radiological
- PAA: Automated Breast Ultrasound
- POK: Computer-Assisted Diagnostic Software For Lesions Suspicious For Cancer
- QDQ: Radiological Computer Assisted Detection/Diagnosis Software For Lesions Suspicious For Cancer
- QPN: Software Algorithm Device To Assist Users In Digital Pathology
- QNP: Gastrointestinal lesion software detection system
The tool has been characterized through simulations (bias and variance of the estimates) and has been compared with other methods as appropriate for the task.
The following peer-reviewed research includes the detailed verification methods and results
- Desc: Study that uses the software and related research methods and study designs in a large study. Supplementary materials include data and scripts to reproduce study results.
- Desc: Original description of method and validation with simulations. Results comparable to jackknife resampling technique.
- Generalize method to binary performance measures.
- Provide framework for understanding method and comparing to other methods analytically and with simulations.
- Gallas, B. D., & Brown, D. G. (2008). Reader studies for validation of CAD systems. Neural Networks Special Conference Issue, 21 (2), 387–397. https://doi.org/10.1016/j.neunet.2007.12.013
Limitations
Currently, the tool can produce negative variance estimates if the relevant dataset is small.
Supporting Documentation
Tool websites:.
- Primary: https://github.com/DIDSR/iMRMC
- Secondary: https://cran.r-project.org/web/packages/iMRMC/index.html
User manual for java app
- http://didsr.github.io/iMRMC/000_iMRMC/userManualPDF/iMRMCuserManual.pdf
User manual for R package
- https://cran.r-project.org/web/packages/iMRMC/iMRMC.pdf
- https://github.com/DIDSR/iMRMC/wiki/iMRMC-FAQ
Supplementary materials
- Data and scripts to reproduce results for manuscripts that use iMRMC
- https://github.com/DIDSR/iMRMC/wiki/iMRMC-Datasets
Related Work
- Chen, W., Gong, Q., Gallas, B.D. (2018). Paired split-plot designs of multireader multicase studies. Journal of Medical Imaging 5, 031410. https://doi.org/10.1117/1.JMI.5.3.031410
- Obuchowski, N.A., Gallas, B.D., Hillis, S.L. (2012). Multi-Reader ROC studies with Split-Plot Designs: A Comparison of Statistical Methods. Acad Radiol 19, 1508– 1517. https://doi.org/10.1016/j.acra.2012.09.012
- Gallas, B.D., Chan, H.-P., D’Orsi, C.J., Dodd, L.E., Giger, M.L., Gur, D., Krupinski,
- E.A., Metz, C.E., Myers, K.J., Obuchowski, N.A., Sahiner, B., Toledano, A.Y., Zuley, M.L. (2012). Evaluating imaging and computer-aided detection and diagnosis devices at the FDA. Acad Radiol 19, 463–477. https://doi.org/10.1016/j.acra.2011.12.016
- Obuchowski, N. A., Gallas, B. D., & Hillis, S. L. (2012). Multi-Reader ROC studies with Split-Plot Designs: A Comparison of Statistical Methods. Academic Radiology, 19 (12), 1508–1517. https://doi.org/10.1016/j.acra.2012.09.012
- Gallas, B. D., & Hillis, S. L. (2014). Generalized Roe and Metz ROC model: Analytic link between simulated decision scores and empirical AUC variances and covariances. J Med Img, 1 (3), 031006. https://doi.org/doi:10.1117/1.JMI.1.3.031006
Tool Reference
In addition to citing relevant publications please reference the use of this tool using DOI: 10.5281/zenodo.8383591
For more information
- Catalog of Regulatory Science Tools to Help Assess New Medical Devices
Advertisement
Impact of artificial intelligence support on accuracy and reading time in breast tomosynthesis image interpretation: a multi-reader multi-case study
- Open access
- Published: 04 May 2021
- Volume 31 , pages 8682–8691, ( 2021 )
Cite this article
You have full access to this open access article
- Suzanne L. van Winkel ORCID: orcid.org/0000-0001-7273-4386 1 ,
- Alejandro Rodríguez-Ruiz 2 ,
- Linda Appelman 1 ,
- Albert Gubern-Mérida 2 ,
- Nico Karssemeijer 1 , 2 ,
- Jonas Teuwen 1 , 3 ,
- Alexander J. T. Wanders 4 ,
- Ioannis Sechopoulos 1 , 5 &
- Ritse M. Mann 1 , 6
40 Citations
55 Altmetric
Explore all metrics
Digital breast tomosynthesis (DBT) increases sensitivity of mammography and is increasingly implemented in breast cancer screening. However, the large volume of images increases the risk of reading errors and reading time. This study aims to investigate whether the accuracy of breast radiologists reading wide-angle DBT increases with the aid of an artificial intelligence (AI) support system. Also, the impact on reading time was assessed and the stand-alone performance of the AI system in the detection of malignancies was compared to the average radiologist.
A multi-reader multi-case study was performed with 240 bilateral DBT exams (71 breasts with cancer lesions, 70 breasts with benign findings, 339 normal breasts). Exams were interpreted by 18 radiologists, with and without AI support, providing cancer suspicion scores per breast. Using AI support, radiologists were shown examination-based and region-based cancer likelihood scores. Area under the receiver operating characteristic curve (AUC) and reading time per exam were compared between reading conditions using mixed-models analysis of variance.
On average, the AUC was higher using AI support (0.863 vs 0.833; p = 0.0025). Using AI support, reading time per DBT exam was reduced ( p < 0.001) from 41 (95% CI = 39–42 s) to 36 s (95% CI = 35– 37 s). The AUC of the stand-alone AI system was non-inferior to the AUC of the average radiologist (+0.007, p = 0.8115).
Conclusions
Radiologists improved their cancer detection and reduced reading time when evaluating DBT examinations using an AI reading support system.
• Radiologists improved their cancer detection accuracy in digital breast tomosynthesis (DBT) when using an AI system for support, while simultaneously reducing reading time.
• The stand-alone breast cancer detection performance of an AI system is non-inferior to the average performance of radiologists for reading digital breast tomosynthesis exams.
• The use of an AI support system could make advanced and more reliable imaging techniques more accessible and could allow for more cost-effective breast screening programs with DBT.
Similar content being viewed by others
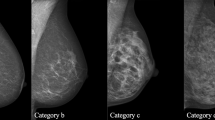
ESR Essentials: screening for breast cancer - general recommendations by EUSOBI
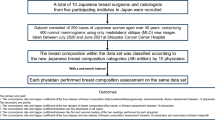
Inter- and intra-observer variability of qualitative visual breast-composition assessment in mammography among Japanese physicians: a first multi-institutional observer performance study in Japan
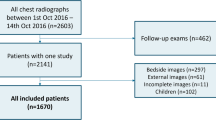
Performance of AI to exclude normal chest radiographs to reduce radiologists’ workload
Avoid common mistakes on your manuscript.
Introduction
In recent years, several clinical trials have demonstrated how using digital breast tomosynthesis (DBT) as a breast cancer screening modality may improve screening results compared to 2D mammography, leading to increased cancer detection and a reduction of recalls [ 1 , 2 , 3 ]. Albeit a reduction in the frequency of interval cancers has not yet been shown [ 4 ], the improved detection is possible because DBT generates a pseudo-3D volume of the breast which partially overcomes one of the main limitations of any 2D imaging technique: tissue superposition [ 5 ]. However, the introduction of DBT as a screening modality still faces difficulties. The interpretation of DBT screening exams takes significantly longer compared to interpreting 2D mammography images [ 6 , 7 ]. Particularly in settings where exams are double-read, like most European screening programs, the increasing lack of specialized breast radiologists [ 8 ] reduces the potential of DBT introduction. Deep learning–based artificial intelligence (AI) systems are quickly gaining attention in the field of radiology, particular in breast imaging [ 9 ]. The current stand-alone performance of AI systems for mammography is approaching, if not already exceeding, the performance of radiologists [ 10 , 11 , 12 , 13 ]. This may result in tools that sustain current mammography-based breast cancer screening programs with less human interaction or even improve the overall quality of screening [ 14 , 15 ]. AI support in screening with DBT could improve cost-efficiency by increasing radiologists’ breast cancer detection performance, allowing radiologists to read DBT exams faster [ 16 , 17 ], or triaging the studies [ 15 ].
The first studies investigating the impact of using AI during DBT interpretation use narrow-angle DBT examinations (with scan angle of 20° or lower) [ 16 ]. However, technical specifications of DBT are highly variable across vendors of DBT systems, leading to more substantial differences in the resulting images compared to mammography [ 18 ]. This is mainly due to differences in the angular range of the various machine models, the reconstruction, and other post-processing algorithms. Technically, a wider angle provides a higher depth resolution [ 18 ] and may enable better separation of lesions from superimposed fibroglandular tissue, but may lead to a poorer calcification depiction [ 5 ].
This study evaluates the impact of an AI support system in wide-angle DBT, previously validated for 2D mammograms [ 10 , 11 , 15 ], on radiologists’ accuracy and reading time. It was hypothesized that radiologists’ average performance in the detection of malignancies using AI support is superior to reading unaided. In addition, the aim was to demonstrate whether AI support could improve radiologists’ average reading time while maintaining or improving sensitivity and specificity and to compare the stand-alone detection performance of the AI system to the average radiologist.
Materials and methods
A HIPAA-compliant fully-crossed fully-randomized multi-reader multi-case (MRMC) study was performed with 18 radiologists, reading a series of wide-angle DBT exams twice, with and without AI support.
Study population
Case collection.
This study included 360 cases: 110 biopsy-proven cancer cases, 104 benign cases (proven by biopsy or at least 6-month follow-up), and 146 randomly selected negative cases (at least 1-year follow-up). Cases were collected from a dataset of a previous, IRB-approved, clinical trial registered with protocol number NCT01373671 [ 19 , 20 ]. Data was collected between May 2011 and February 2014 from seven US clinical sites, representative of women undergoing screening and diagnostic DBT exams in the USA. The mean age was 56.3 ± 9.8 (standard deviation) years. Each case consists of a bilateral two-view (cranio-caudal/mediolateral oblique CC/MLO) DBT exam acquired using standard exposure settings with a Mammomat Inspiration (Siemens Healthineers)) and reconstructed with the latest algorithm (EMPIRE), also generating the corresponding synthetic mammography (SM) images [ 21 ]. The DBT system has a wide 50° scanning angle. This data was not used for the development of the AI support system.
Case selection protocol
The case selection was aimed at obtaining a challenging and representative set for the observer evaluation. Exclusion criteria were as follows: breast implants, sub-optimal quality (judged by a radiologist and a radiographer with respectively 14 and 38 years of breast imaging experience), missing image data, or missing truth data. After exclusion and performing a power analysis [ 22 ], to achieve a power of at least 0.8 (80%) to test the primary hypothesis of the study, we target to select 110 negative cases, 65 benign cases, and 65 malignant cases. Negative and benign cases were randomly selected to avoid selection bias. The aim for the malignant case selection was to include all cases categorized as “subtle”, and as many “moderately subtle” cases as available while including at least a random selection of five “obvious” cases. To reach the targeted sample size of 65 malignant cases, a subtlety score (1, “subtle”; 2, “moderately subtle”; 3, “obvious”) was independently determined by three breast radiologists (respectively 14, 39, and 5 years mammography experience; 5, 5, and 5 years DBT experience), with the third acting as an arbiter in case of disagreement.
Reference standard
For every case, per breast, the reference standard based on pathology and imaging reports was available in electronic format and reviewed by the radiologists participating in the case selection process (not participating in the observer study), including location and radiological characterization of cancers, location of benign lesions, or confirmed normal status.
AI support system
The AI support system used during the observer evaluation was Transpara™ 1.6.0, (ScreenPoint Medical BV). This system is based on deep convolutional neural networks [ 23 , 24 ] and automatically detects lesions suspicious of breast cancer in 2D and DBT mammograms from different vendors. The results are shown to radiologists in two distinct ways:
A score from 1 to 10, indicating the increasing likelihood that a visible cancer is present at the mammogram. In a screening setting, approximately 10% of mammograms are assigned each score.
The most suspicious findings are marked and a scored with the level of suspicion (LOS) for cancer (1–100).
The system has been validated for 2D mammograms in previously performed clinical studies with independent datasets [ 10 , 11 , 15 ]. It has been trained and tested using a proprietary database containing over 1,000,000 2D mammography and DBT images (over 20,000 with cancer), acquired with machines from five different mammography vendors at a dozen institutions (academic and private health centers) across 10 countries in Europe, America, and Asia.
Each selected DBT mammogram was processed by the AI system. The results of this analysis were shown during the observer evaluation. Radiologists could concurrently use the AI system with or without the corresponding SM and interactive navigation support. Interactive navigation support consists of automatic access to the DBT plane where the AI algorithm detected abnormalities, with a single click on a mark shown on the SM.
Observer evaluation
Sessions and training.
The observer evaluation consisted of two parts. Exams were read twice, with and without AI support, separated by a wash-out period of at least 4 weeks. The case order and the availability of AI support were randomized for each radiologist.
During the evaluation of the cases with the AI support available, two reading protocols were tested. Half of the radiologists (readers 1–9) read the exams with access to the corresponding SM and interactive navigation support, while the other half (readers 10–18) read exams without these functionalities, showing AI findings only in the 3D DBT stack.
To get familiar with the AI system and workstation before participating in the study, all radiologists were trained by evaluating a set of 50 DBT exams (not included in the study). Radiologists were blinded to patient history and any other information not visible in the included DBT imaging exams.
The radiologists used a reading workstation for DBT exams and a 12MP DBT-certified diagnostic color display (Coronis Uniti, Barco) calibrated to the DICOM Grayscale Standard Display Function. The workstation tracked the reader actions in the interface with timestamps.
For every case, radiologists were instructed to the following:
Mark the 3D location of findings in every view visible
Assign a LOS to each finding
Provide a BI-RADS category (1, 2, 3, 4a, 4b, 4c, or 5) per breast.
All radiologists were American Board of Radiology–certified, qualified to interpret mammograms under the Mammography Quality Standard Act (MQSA) and active in reading DBT exams in clinical practice. Half of the readers devoted less than 75% of their professional time to breast imaging for the last 3 years while the other half devoted more time. The median experience with MQSA qualification of the readers was 9 years (range 2–23 years) and the median volume of 2D or DBT mammograms read per year was 4200 (1000-18,000). All the readers were at the time of the study reading DBT exams in clinical practice.
Endpoints and statistical analysis
Primary hypothesis.
The primary hypothesis was that radiologists’ average breast-level area under the receiver operating characteristic (ROC) curve (AUC) for detection of malignancies in DBT using AI reading support is superior to reading unaided. This was tested against the null hypothesis: radiologists’ average breast-level AUC with AI support being equivalent to their average AUC unaided. p < 0.05 indicated a statistically significant difference between both reading conditions.
AUC superiority analysis was performed using the statistical package developed by Tabata et al [ 25 ], using the Obuchowski and Rockette method adapted to consider clustered data when calculating reader by modality covariances [ 26 , 27 ].
ROC curves were built using the LOS assigned to each breast. Standard errors (SE) and 95% confidence intervals (CI) were computed.
Secondary hypotheses
If the primary hypothesis was met, four secondary hypotheses were evaluated in a (hierarchical) fixed sequence to control type I error rate at significance level alpha = 0.05.
Radiologists’ average reading time per DBT exam using AI support is superior to (shorter than) the average reading time per DBT exam unaided.
Average reading times per DBT exam were compared between reading conditions by using a generalized linear mixed-effects (GLME) model, taking repeated measures by multiple readers into account [ 28 ].
Radiologists’ average sensitivity reading DBT exams with AI support is non-inferior/superior compared to reading DBT exams unaided, at a pre-specified non-inferiority margin delta of 0.05.
Radiologists’ average specificity reading DBT exams with AI support is non-inferior/superior compared to reading DBT exams unaided, at a pre-specified non-inferiority margin delta of 0.05.
The analysis was performed following the analysis described in the primary hypothesis for AUC comparisons, formatting the input data accordingly. A breast was considered positive if the radiologist assigned a BI-RADS score ≥ 3.
The stand-alone AI system AUC is non-inferior to the radiologists’ average breast-level AUC reading DBT exams unaided at a pre-specified non-inferiority margin delta of 0.05.
The public domain iMRMC software (version 4.0.3, Division of Imaging, Diagnostics, and Software Reliability, OSEL/CDRH/FDA) was used, which can also handle single reader data (the AI system) [ 29 ].
Figure 1 shows the case selection flowchart. The characteristics of the selected sample are detailed in Tables 1 and 2 . No protocol deviations were found during data selection.

Flow of women through the study, from data collection until data selection for the observer evaluation
Impact on breast cancer detection accuracy
All readers completed the reading sessions as planned; 8640 case reports were received (240 × 18 × 2), and there was no missing data.
Radiologists significantly improved their DBT detection performance using AI support. The average AUC increased from 0.833 (95% CI = 0.799–0.867) to 0.863 (95% CI = 0.829–0.898), p = 0.0025 (difference + 0.030, 95% CI = 0.011–0.049). The average ROC curves are presented in Fig. 2 . Differences per reader are shown in Table 3 . Sixteen out of 18 readers (89%) had a higher AUC using AI support; improvements ranged from + 0.010 to + 0.088.
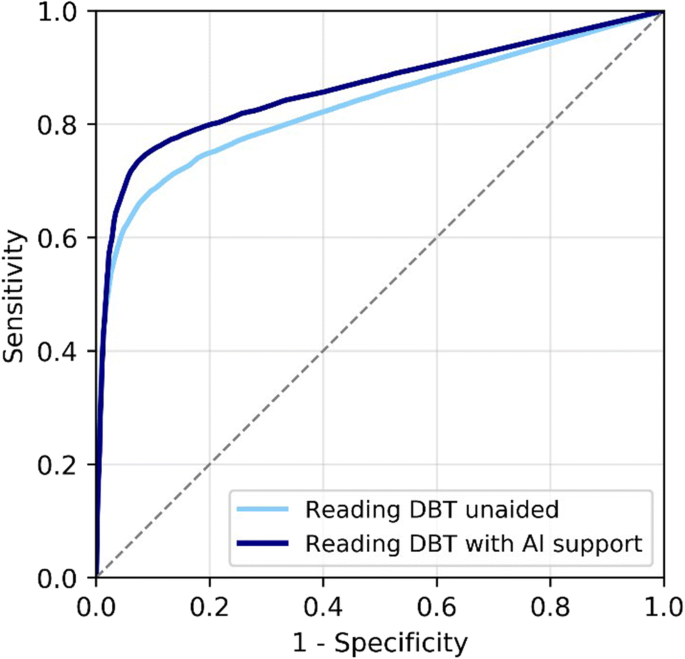
Average receiver operating characteristic curves (ROC) of the radiologists reading breast tomosynthesis (DBT) unaided and reading DBT exams with AI support concurrently. The difference in ROC area under the curve was significant, + 0.03, p = 0.0025
Descriptive analysis showed AUC improvements when using AI support were present in all subgroups:
Lesion type (+ 0.022 [95% CI = −0.005, 0.049] for cases with soft tissue lesions, + 0.046 [95% CI = 0.015, 0.077] for cases with calcifications)
Reading protocol (+ 0.041 [95% CI = 0.019, 0.063] for radiologists using SM and interactive navigation, + 0.020 [95% CI = −0.004, 0.044] for radiologists reading DBT alone)
Radiologists’ specialization (+0.020 [95% CI = −0.007, 0.047] for radiologists who dedicated > 75% of their professional time to breast imaging in the last 3 years, +0.038 [95% CI = 0.022, 0.054] for the rest).
Impact on reading time
The average reading time per DBT exam was significantly shorter using AI support (36 s, 95% CI = 35–37s) compared to reading unaided (41 s, 95% CI = 39–42s); resulting in a difference of −11% (95% CI = −8%, −13%), p < 0.001 (Table 3 ). Descriptively, reading time was shorter using AI support, regardless of breast density (low breast density: −13% (95% CI = −10%, −16%); high breast density: −10% (95% CI = −7%, −13%)) or reading protocol. However, the reduction was larger using 2D SM images and interactive navigation (shorter for 7/9 radiologists, decreasing from 39 to 32s, a difference of −19%, 95% CI = −16%, −22%), than without these tools (shorter for 5/9 radiologists (56%), decreasing from 42 to 40s, a difference of −4%, 95% CI = −1%, −7%).
The reading time reduction using AI support was correlated with the exam-level score assigned by the AI system: reductions were stronger for the lowest exam-level scores (−30%) (see Fig. 3 ). Figure 4 shows an example where radiologists consistently read the exam faster when using AI support.
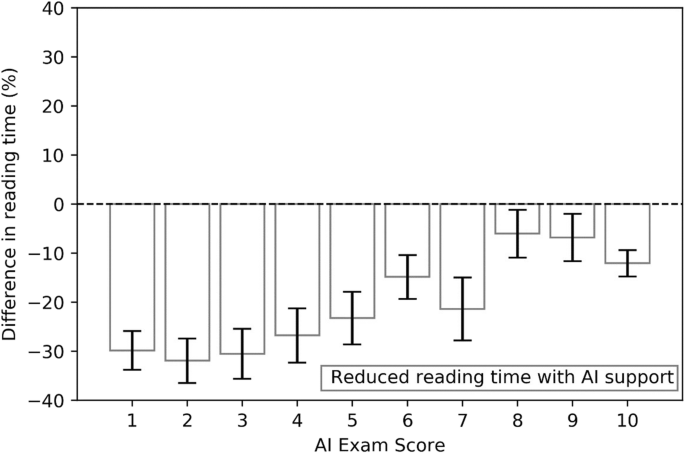
Average differences in reading time (%) across radiologists using synthetic mammograms and interactive navigation features between reading breast tomosynthesis exams unaided or reading with AI support, as a function of the exam-level score assigned by the AI system
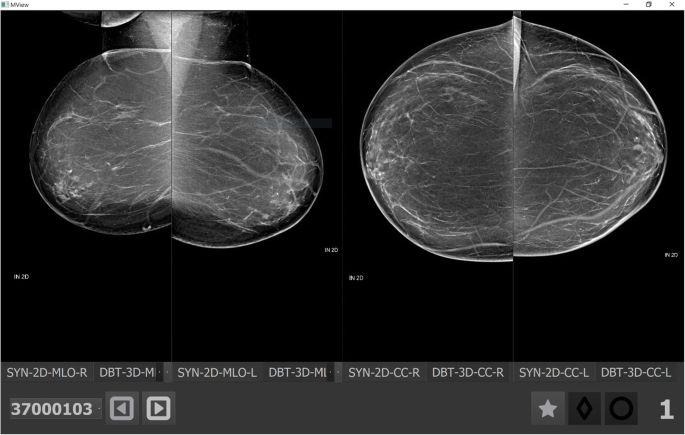
Breast tomosynthesis exam (the synthetic image) of a woman without cancer and an exam-level cancer likelihood score of 1 (lowest) by the AI system. When reading the case aided, 17/18 (94%) radiologists read the exam faster, with an average reduction of reading time of −54% (from 36 to 19 s)
Impact on sensitivity and specificity
Using AI support, radiologists significantly improved their cancer detection sensitivity. The average sensitivity increased from 74.6 (95% CI = 68.3–80.8%) to 79.2% (95% CI = 73.3–85.1%), a relative difference of +6.2% (95% CI = 1.3–11.1%), p = 0.016. Specificity was maintained: a relative difference of + 1.1% (95% CI = −1.3%, 3.5%), p = 0.380. Figure 5 shows an example where radiologists consistently improved sensitivity when using AI support.
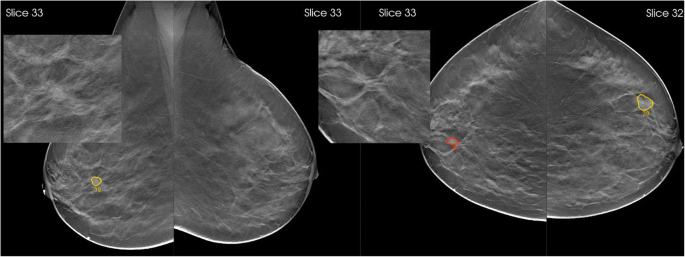
Breast tomosynthesis exam of a woman with an architectural distortion in the right breast, proven to be a 15-mm invasive ductal carcinoma (zoomed). The AI system marked the regions and assigned region-scores of 76 and 39 on cranio-caudal and mediolateral oblique views, respectively, and an exam-level cancer likelihood score of 10, the highest category. When reading the case unaided, 8/18 (44%) radiologists would have recalled the woman, a proportion that increased to 15/18 (83%) radiologists when reading the case with AI support
Stand-alone AI detection performance
The stand-alone AUC of the AI system was 0.840 (SE = 0.034), +0.007 higher (95% CI = −0.048, 0.062) compared to the average unaided radiologist AUC. This performance was statistically non-inferior ( p = 0.8115). Descriptively, the stand-alone AUC was higher compared to the AUC of 10/18 radiologists, reading DBT unaided. The stand-alone ROC curve of the AI system compared to radiologists’ performance is depicted in Fig. 6 .
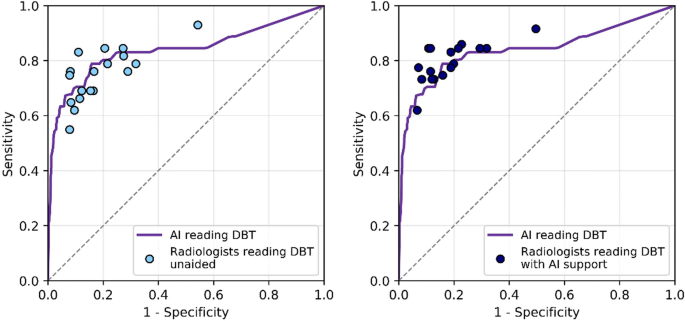
Stand-alone receiver operating characteristic curve of the AI support system, together with the operating points of the 18 individual radiologists reading breast tomosynthesis (DBT) unaided (left) or with AI support (right)
This study shows that a deep learning–based AI system for DBT enables radiologists to increase their breast cancer detection performance in terms of overall accuracy and sensitivity at similar specificity, while reducing reading time. The observed improvement in accuracy is comparable to what has been reported with an earlier version of this AI program for mammography evaluation and another AI program for evaluation of narrow-angle DBT [ 10 , 16 ]. The sensitivity improvement could help to reduce the number of DBT screening false negatives, i.e. lesions being overlooked or misdiagnosed [ 3 ], while the reduced reading time might enable the implementation of DBT for screening in sites where DBT is currently considered too time-intensive. Furthermore, the improvement in AUC was observed for all radiologists regardless of the time they dedicate to breast imaging in clinical practice.
The reduction in reading time per DBT exam when concurrently using an AI system for reading support is similar to those from other studies [ 16 , 17 ]. Nevertheless, reading times heavily rely on the specific functionality of the viewing application used for interpretation, as well as the exact viewing protocol used to evaluate the DBT exams, which prevents direct comparisons among the available studies. For example, the average unaided reading time in the study by Conant et al [ 16 ] using another AI software was almost twice as long as the one found in our study, but interestingly, the AI-assisted reading times were similar in both studies; approximately 30 s per four-view DBT exam with 2D synthetic images. Also, observing the largest reading time reduction in this study for the readers presented with an SM for navigation suggests that functionality of the reading environment is an important factor.
Similar to results in a previous 2D mammography study with this AI system [ 10 ], a strong dependency of the reading time reduction on the exam-based AI scores was found (Fig. 3 ). This suggests that the biggest reading time reduction can be achieved for the lowest exam-based AI scores, indicating the readers were confident enough to spend less time on exams categorized as most likely normal, despite the relatively short time to get familiar with the AI system. The resulting reading times per category may be used to estimate the potential of AI support in a representative series of screening exams. Provided that readers will be using 2D SM images and interactive navigation, the reading time reduction in a screening population would be approximately −20% (95% CI = −16%, −25%).
The increased accuracy and decreased reading time were observed using cases from a previously, prospectively collected dataset, consisting of DBT exams obtained with a large acquisition angle. Since a previous study [ 10 ] showed similar improvements for 2D mammography, it is likely that the AI induced improvements hold true for the whole spectrum of mammographic techniques, which is corroborated by the fact that the stand-alone performances of the AI system equal the radiologists for both wide-angle DBT and 2D mammography.
As the stand-alone performance of the AI system was equivalent to the performance of the radiologists, it may be feasible to explore implementation strategies beyond the concurrent reading of DBT exams with AI support. Like in 2D mammography, it might be feasible to use AI for efficient triaging of the screening workload [ 14 , 15 ]. By identifying a large group of normal exams with a high negative predictive value, alternative strategies such as single-reading when double-reading, or even exclusion from radiologist evaluation, could be explored.
A limitation of this study is the use of a cancer-enriched dataset instead of a consecutively collected sample of screening mammograms from a clinical setting. This was to allow a multi-reader evaluation with sufficient findings to draw useful conclusions. Consequently, this may not be fully representative of a real screening situation. To what extent this difference affects the results is unknown. The effectiveness of AI support in screening with DBT still needs to be assessed: results from this study should serve as a starting point for prospective studies focusing on the impact of using AI in DBT mammography in clinical and screening environments. In conclusion, radiologists improved their cancer detection in DBT examinations when using an AI support system, while simultaneously reducing reading time. Using an AI reading support system could allow for more cost-effective screening programs with DBT.
Abbreviations
Artificial intelligence
Area under the ROC curve
Cranio-caudal
Confidence interval
Digital breast tomosynthesis
Generalized linear mixed-effects
Mediolateral oblique
Multi-reader multi-case
Receiver operating characteristic
Synthetic mammography
Rafferty EA, Durand MA, Conant EF et al (2016) Breast cancer screening using tomosynthesis and digital mammography in dense and nondense breasts. JAMA. 315(16):1784–1786
Article Google Scholar
Friedewald SM, Rafferty EA, Rose SL et al (2014) Breast cancer screening using tomosynthesis in combination with digital mammography. JAMA. 311(24):2499–2507
Article CAS Google Scholar
Zackrisson S, Lång K, Rosso A et al (2018) One-view breast tomosynthesis versus two-view mammography in the Malmö Breast Tomosynthesis Screening Trial (MBTST): a prospective, population-based, diagnostic accuracy study. Lancet Oncol 19(11):1493–1503
Bernardi D, Gentilini MA, De Nisi M et al (2019) Effect of implementing digital breast tomosynthesis (DBT) instead of mammography on population screening outcomes including interval cancer rates: Results of the Trento DBT pilot evaluation. Breast 50:135–140
Sechopoulos I (2013) A review of breast tomosynthesis. Part I. The image acquisition process. Med Phys 40(1):014301
Dang PA, Freer PE, Humphrey KL, Halpern EF, Rafferty EA (2014) Addition of tomosynthesis to conventional digital mammography: effect on image interpretation time of screening examinations. Radiology. 270(1):49–56
Rodriguez-Ruiz A, Gubern-Merida A, Imhof-Tas M et al (2017) One-view digital breast tomosynthesis as a stand-alone modality for breast cancer detection: do we need more? Eur Radiol 28:1938–1948
Rimmer A (2017) Radiologist shortage leaves patient care at risk, warns royal college. BMJ 359. https://doi.org/10.1136/bmj.j4683
Litjens G, Kooi T, Bejnordi BE et al (2017) A survey on deep learning in medical image analysis. Med Image Anal 42:60–88
Rodríguez-Ruiz A, Krupinski E, Mordang J-J et al (2018) Detection of breast cancer with mammography: effect of an artificial intelligence support system. Radiology. 00:1–10
Google Scholar
Rodriguez-Ruiz A, Lång K, Gubern-Merida A, Broeders M et al (2019) Stand-alone artificial intelligence for breast cancer detection in mammography: comparison with 101 radiologists. J Natl Cancer Inst 111(9)
Wu N, Phang J, Park J et al (2019) Deep neural networks improve radiologists’ performance in breast cancer screening. IEEE Trans Med Imaging 39(4):1184–1194
McKinney SM, Sieniek M, Godbole V et al (2020) International evaluation of an AI system for breast cancer screening. Nature. 577(7788):89–94
Yala A, Schuster T, Miles R, Barzilay R, Lehman C (2019) A deep learning model to triage screening mammograms: a simulation study. Radiology. 293:38–46
Rodriguez-Ruiz A, Lång K, Gubern-Merida A, Teuwen J et al (2019) Can we reduce the workload of mammographic screening by automatic identification of normal exams with artificial intelligence? A feasibility study. Eur Radiol 29(9):4825–4832
Conant EF, Toledano AY, Periaswamy S et al (2019) Improving accuracy and efficiency with concurrent use of artificial intelligence for digital breast tomosynthesis. Radiol Artif Intell 1(4):e180096
Chae EY, Kim HH, Jeong J-w, Chae S-H, Lee S, Choi Y-W (2018) Decrease in interpretation time for both novice and experienced readers using a concurrent computer-aided detection system for digital breast tomosynthesis. Eur Radiol 29:2518–2525
Rodriguez-Ruiz A, Castillo M, Garayoa J, Chevalier M (2016) Evaluation of the technical performance of three different commercial digital breast tomosynthesis systems in the clinical environment. Phys Med 32(6):767–777
Georgian-Smith D, Obuchowski NA, Lo JY et al (2019) Can digital breast tomosynthesis replace full-field digital mammography? A multireader, multicase study of wide-angle tomosynthesis. AJR Am J Roentgenol. 212(6):1393–1399
Siemens Medical Solutions USA Inc. (2015) FDA application: mammomat inspiration with digital breast tomosynthesis. https://www.accessdata.fda.gov/cdrh_docs/pdf14/P140011b.pdf
Rodriguez-Ruiz A, Teuwen J, Vreemann S, Bouwman RW et al (2017) New reconstruction algorithm for digital breast tomosynthesis: better image quality for humans and computers. Acta Radiol 284185117748487
Hillis SL, Obuchowski NA, Berbaum KS (2011) Power estimation for multireader ROC methods: an updated and unified approach. Academic Radiology. 18(2):129–142
Kooi T, Litjens G, van Ginneken B et al (2017) Large scale deep learning for computer aided detection of mammographic lesions. Med Image Anal 35:303–312
Mordang J-J, Janssen T, Bria A, Kooi T, Gubern-Mérida A, Karssemeijer N (2016) Automatic microcalcification detection in multi-vendor mammography using convolutional neural networks. International Workshop on Digital Mammography. Springer. 9699:35–42
Tabata K, Uraoka N, Benhamida J et al (2019) Validation of mitotic cell quantification via microscopy and multiple whole-slide scanners. Diagn Pathol. 14(1):65
Obuchowski NA (1997) Nonparametric analysis of clustered ROC curve data. Biometrics 567-78
Obuchowski NA (1995) Multireader, multimodality receiver operating characteristic curve studies: hypothesis testing and sample size estimation using an analysis of variance approach with dependent observations. Acad Radiol 2(Suppl 1):S22–S29 discussion S57-64, S70-1 pas
PubMed Google Scholar
McCullagh P (2019) Generalized linear models. Routledge
Gallas B (2017) iMRMC v4.0: Application for analyzing and sizing MRMC reader studies. https://github.com/DIDSR/iMRMC/releases , https://cran.r-project.org/web/packages/iMRMC/index.html
Download references
This study has received funding from ScreenPoint Medical (Nijmegen, The Netherlands), the company that develops and commercializes the investigated AI support system (Transpara™).
Author information
Authors and affiliations.
Department of Medical Imaging, Radboud University Medical Center, PO Box 9101, 6500 HB Nijmegen, Geert Grooteplein 10, 6525 GA, Post 766, Nijmegen, The Netherlands
Suzanne L. van Winkel, Linda Appelman, Nico Karssemeijer, Jonas Teuwen, Ioannis Sechopoulos & Ritse M. Mann
ScreenPoint Medical BV, Toernooiveld 300, 6525 EC, Nijmegen, The Netherlands
Alejandro Rodríguez-Ruiz, Albert Gubern-Mérida & Nico Karssemeijer
Department of Radiation Oncology, Netherlands Cancer Institute (NKI), Plesmanlaan 121, 1066 CX, Amsterdam, The Netherlands
Jonas Teuwen
Bevolkingsonderzoek Zuid-West Borstkanker, Laan 20, 2512 GB, Den Haag, The Netherlands
Alexander J. T. Wanders
Dutch Expert Centre for Screening (LRCB), Wijchenseweg 101, 6538 SW, Nijmegen, The Netherlands
Ioannis Sechopoulos
Department of Radiology, Netherlands Cancer Institute (NKI), Plesmanlaan 121, 1066 CX, Amsterdam, The Netherlands
Ritse M. Mann
You can also search for this author in PubMed Google Scholar
Corresponding authors
Correspondence to Suzanne L. van Winkel or Ritse M. Mann .
Ethics declarations
The scientific guarantor of this publication is Ritse M. Mann, PhD, MD.
Conflict of interest
The authors of this manuscript declare relationships with the following companies:
The AI support system under investigation (Transpara™) in this study was developed by ScreenPoint Medical (Nijmegen, The Netherlands), a spin-off company of the Department of Medical Imaging, Radboud University Medical Center. Several authors are employees of this company (Alejandro Rodriguez-Ruiz, PhD; Albert Gubern-Merida, PhD; Nico Karssemeijer, PhD). The content of this study was also used for FDA approval. All data was generated by a fully independent clinical research organization (Radboudumc; Radboud University Medical Center, Nijmegen, The Netherlands). Readers were not affiliated with ScreenPoint Medical in any way. Data was handled and controlled at all times by the non-ScreenPoint employee authors.
Statistics and biometry
One of the authors has significant statistical expertise.
The tomosynthesis image files are owned by a third company party (Siemens Medical Solutions, USA) and were made available for research purposes to ScreenPoint Medical (Nijmegen, The Netherlands) only. Without the DBT image data, the observer study data alone is of limited value and challenging to interpret. However, if needed, the observer study data could be made available for auditing purposes.
Informed consent
Written informed consent was not required for this study because the completely anonymized cases were collected from a library of images collected prospectively for a previously performed, IRB-approved, clinical trial registered with protocol number NCT01373671.
Ethical approval
Institutional Review Board approval was obtained.
The conducted observer evaluation study was HIPAA-compliant. Cases were collected from a library of images collected prospectively for a previously performed, IRB-approved, clinical trial registered with protocol number NCT01373671.
Methodology
• Prospective
• Diagnostic or prognostic study
• Multicenter study
Additional information
Publisher’s note.
Springer Nature remains neutral with regard to jurisdictional claims in published maps and institutional affiliations.
Rights and permissions
Open Access This article is licensed under a Creative Commons Attribution 4.0 International License, which permits use, sharing, adaptation, distribution and reproduction in any medium or format, as long as you give appropriate credit to the original author(s) and the source, provide a link to the Creative Commons licence, and indicate if changes were made. The images or other third party material in this article are included in the article's Creative Commons licence, unless indicated otherwise in a credit line to the material. If material is not included in the article's Creative Commons licence and your intended use is not permitted by statutory regulation or exceeds the permitted use, you will need to obtain permission directly from the copyright holder. To view a copy of this licence, visit http://creativecommons.org/licenses/by/4.0/ .
Reprints and permissions
About this article
van Winkel, S.L., Rodríguez-Ruiz, A., Appelman, L. et al. Impact of artificial intelligence support on accuracy and reading time in breast tomosynthesis image interpretation: a multi-reader multi-case study. Eur Radiol 31 , 8682–8691 (2021). https://doi.org/10.1007/s00330-021-07992-w
Download citation
Received : 19 October 2020
Revised : 16 March 2021
Accepted : 09 April 2021
Published : 04 May 2021
Issue Date : November 2021
DOI : https://doi.org/10.1007/s00330-021-07992-w
Share this article
Anyone you share the following link with will be able to read this content:
Sorry, a shareable link is not currently available for this article.
Provided by the Springer Nature SharedIt content-sharing initiative
- Digital breast tomosynthesis (DBT)
- Artificial intelligence (AI)
- Breast cancer
- Mammography
- Mass screening
- Find a journal
- Publish with us
- Track your research

An official website of the United States government
The .gov means it’s official. Federal government websites often end in .gov or .mil. Before sharing sensitive information, make sure you’re on a federal government site.
The site is secure. The https:// ensures that you are connecting to the official website and that any information you provide is encrypted and transmitted securely.
- Publications
- Account settings
Preview improvements coming to the PMC website in October 2024. Learn More or Try it out now .
- Advanced Search
- Journal List

Multi-Reader Multi-Case Studies Using the Area under the Receiver Operator Characteristic Curve as a Measure of Diagnostic Accuracy: Systematic Review with a Focus on Quality of Data Reporting
Thaworn dendumrongsup.
1 Department of Radiology, Prince of Songkla University, Hat Yai, Thailand
Andrew A. Plumb
2 Centre for Medical Imaging, University College London, London, United Kingdom
Steve Halligan
Thomas r. fanshawe.
3 Nuffield Department of Primary Care Health Sciences, Oxford University, Oxford, United Kingdom
Douglas G. Altman
4 Centre for Statistics in Medicine, Wolfson College, Oxford University, Oxford, United Kingdom
Susan Mallett
Conceived and designed the experiments: TD AAP SH TRF DGA SM. Performed the experiments: TD AAP SH TRF DGA SM. Analyzed the data: TD AAP SH TRF DGA SM. Contributed reagents/materials/analysis tools: TD AAP SH TRF DGA SM. Wrote the paper: TD AAP SH TRF DGA SM.
Associated Data
The authors confirm that all data underlying the findings are fully available without restriction. All relevant data are within the paper and its Supporting Information files.
Introduction
We examined the design, analysis and reporting in multi-reader multi-case (MRMC) research studies using the area under the receiver-operating curve (ROC AUC) as a measure of diagnostic performance.
We performed a systematic literature review from 2005 to 2013 inclusive to identify a minimum 50 studies. Articles of diagnostic test accuracy in humans were identified via their citation of key methodological articles dealing with MRMC ROC AUC. Two researchers in consensus then extracted information from primary articles relating to study characteristics and design, methods for reporting study outcomes, model fitting, model assumptions, presentation of results, and interpretation of findings. Results were summarized and presented with a descriptive analysis.
Sixty-four full papers were retrieved from 475 identified citations and ultimately 49 articles describing 51 studies were reviewed and extracted. Radiological imaging was the index test in all. Most studies focused on lesion detection vs. characterization and used less than 10 readers. Only 6 (12%) studies trained readers in advance to use the confidence scale used to build the ROC curve. Overall, description of confidence scores, the ROC curve and its analysis was often incomplete. For example, 21 (41%) studies presented no ROC curve and only 3 (6%) described the distribution of confidence scores. Of 30 studies presenting curves, only 4 (13%) presented the data points underlying the curve, thereby allowing assessment of extrapolation. The mean change in AUC was 0.05 (−0.05 to 0.28). Non-significant change in AUC was attributed to underpowering rather than the diagnostic test failing to improve diagnostic accuracy.
Conclusions
Data reporting in MRMC studies using ROC AUC as an outcome measure is frequently incomplete, hampering understanding of methods and the reliability of results and study conclusions. Authors using this analysis should be encouraged to provide a full description of their methods and results.
The receiver operator characteristic (ROC) curve describes a plot of sensitivity versus 1-specificity for a diagnostic test, across the whole range of possible diagnostic thresholds [1] . The area under the ROC curve (ROC AUC) is a well-recognised single measure that is often used to combine elements of both sensitivity and specificity, sometimes replacing these two measures. ROC AUC is often used to describe the diagnostic performance of radiological tests, either to compare the performance of different tests or the same test under different circumstances [2] , [3] . Radiological tests must be interpreted by human observers and a common study design uses multiple readers to interpret multiple image cases; the multi-reader multi-case (MRMC) design [4] . The MRMC design is popular because once a radiologist has viewed 20 cases there is less information to be gained by asking him to view a further 20 than by asking a different radiologist to view the same 20. This procedure enhances the generalisability of study results and having multiple readers interpret multiple cases enhances statistical power. Because multiple radiologists view the same cases, “clustering” occurs. For example, small lesions are generally seen less frequently than larger lesions, i.e. reader observations are clustered within cases. Similarly, more experienced readers are likely to perform better across a series of cases than less experienced readers, i.e. results are correlated within readers. Bootstrap resampling and multilevel modeling can account for clustering, linking results from the same observers and cases, so that 95% confidence intervals are not too narrow. MRMC studies using ROC AUC as the primary outcome are often required by regulatory bodies for the licensing of new radiological devices [5] .
We attempted to use ROC AUC as the primary outcome measure in a prior MRMC study of computer-assisted detection (CAD) for CT colonography [6] . However, we encountered several difficulties when trying to implement this approach, described in detail elsewhere [7] . Many of these difficulties were related to issues implementing confidence scores in a transparent and reliable fashion, which led ultimately to a flawed analysis. We considered, therefore, that for ROC AUC to be a valid measure there are methodological components that need addressing in study design, data collection and analysis, and interpretation. Based on our attempts to implement the MRMC ROC AUC analysis, we were interested in whether other researchers have encountered similar hurdles and, if so, how these issues were tackled.
In order to investigate how often other studies have addressed and reported on methodological issues with implementing ROC AUC, we performed a systematic review of MRMC studies using ROC AUC an outcome measure. We searched and investigated the available literature with the objective to describe the statistical methods used, the completeness of data presentation, and investigate whether any problems with analysis were encountered and reported.
Ethics statement
Ethical approval is not required by our institutions for research studies of published data.
Search strategy, inclusion and exclusion criteria
This systematic review was performed guided by the Preferred Reporting Items for Systematic Reviews and Meta-Analyses (PRISMA), an evidence-based minimum set of items for reporting in systematic reviews and meta-analyses [8] . We developed an extraction sheet for the systematic review, broken down into different sections (used as subheadings for the Results section of this report), with notes relating to each individual item extracted ( S1 File ). In consensus we considered approximately 50 articles would provide a sufficiently representative overview of current reporting practice. Based on our prior experience of performing systematic reviews we believed that searching for additional articles beyond 50 would be unlikely to yield valuable additional data (i.e. we believed we would reach “saturation” by 50 articles) yet would present a very considerable extraction burden.
In order to achieve this, potentially eligible primary articles published between 2005 and February 2013 inclusive were identified by a radiologist researcher (TD) using PUBMED via their citation of one or more of 8 key methodological articles relating to MRMC ROC AUC analysis [9] – [16] . To achieve this the Authors' names (combined using “AND”) were entered in the PUBMED search field and the specific article identified and clicked in the results list. The abstract was then accessed and the “Cited By # PubMed Central Articles” link and “Related Citations” link used to identify those articles in the PubMed Central database that have cited the original article. There was no language restriction. Online abstracts were examined in reverse chronological order, the full text of potentially eligible papers then retrieved, and selection stopped once the threshold of 50 studies fulfilling inclusion criteria had been passed.
To be eligible, primary studies had to be diagnostic test accuracy studies of human observers interpreting medical image data from real patients, and attempting to use a MRMC ROC AUC analysis as a study outcome based on the following methodological approaches [9] – [16] ; Reviews, solely methodological papers, and those using simulated imaging data were excluded.
Data extraction
An initial pilot sample of 5 full-paper articles were extracted and the data checked by a subgroup of investigators in consensus, to both confirm the process was feasible and to identify potential problems. These papers were extracted by TD using the search strategy described in the previous section. A further 10 full-papers were extracted by two radiologist researchers again using the same search strategy and working independently (TD, AP) to check agreement further. The remaining articles included in the review were extracted predominantly by TD, who discussed any concerns/uncertainty with AP. Any disagreement following their discussion was arbitrated by SH and/or SM where necessary. These discussions took place during two meetings when the authors met to discuss progress of the review; multiple papers and issues were discussed on each occasion.
The extraction covered the following broad topics: Study characteristics, methods to record study outcomes, model assumptions, model fitting, data presentation ( S1 File ).
We extracted data relating to the organ and disease studied, the nature of the diagnostic task (e.g. characterization vs. localization vs. presence/absence), test methods, patient source and characteristics, study design (e.g. prospective/retrospective, secondary analysis, single/multicenter) and reference standard. We extracted the number of readers, their prior experience, specific interpretation training for the study (e.g. use of CAD software), blinding to clinical data and/or reference results, the number of times they read each case and the presence of any washout period to diminish recall bias, case ordering, and whether all readers read all cases (i.e. a fully-crossed design). We extracted the unit of analysis (e.g. patient vs. organ vs. segment), and sample size for patients with and without pathology.
We noted whether study imaging reflected normal daily clinical practice or was modified for study purposes (e.g. restricted to limited images). We noted the confidence scores used for the ROC curve and their scale, and whether training was provided for scoring. We noted if there were multiple lesions per unit of analysis. We noted if scoring differed for positive and negative patient cases, whether score distribution was reported, and whether transformation to a normal distribution was performed.
We extracted if ROC cures were presented in the published article and, if so, whether for individual readers, whether the curve was smoothed, and if underlying data points were shown. We defined unreasonable extrapolation as an absence of data in the right-hand 25% of the plot space. We noted the method for curve fitting and whether any problems with fitting were reported, and the method used to compare AUC or pAUC. We extracted the primary outcome, the accuracy measures reported, and whether these were overall or for individual readers. We noted the size of any change in AUC, whether this was significant, and made a subjective assessment of whether significance could be attributed to a single reader or case. We noted how the study authors interpreted change in AUC, if any, and whether any change was reported in terms of effect on individual patients. We also noted if a ROC researcher was named as an author or acknowledged, defined as an individual who had published indexed research papers dealing with ROC methodology.
Data were summarized in an Excel worksheet (Excel For Mac 14.3.9, Microsoft Corporation) with additional cells for explanatory free text. A radiologist researcher (SH) then compiled the data and extracted frequencies, consulting the two radiologists who performed the extraction for clarification when necessary. The investigator group discussed the implication of the data subsequently, to guide interpretation.
Four hundred and seventy five citations of the 8 key methodological papers were identified and 64 full papers retrieved subsequently. Fifteen [17] – [31] of these were rejected after reading the full text (the papers and reason for rejection are shown in Table 1 ) leaving 49 [32] – [80] for extraction and analysis that were published between 2010 and 2012 inclusive; these are detailed in Table 1 . Two papers [61] , [75] contributed two separate studies each, meaning that 51 studies were extracted in total. The PRISMA checklist [8] is detailed in Fig. 1 . The raw extracted data are available in S2 File .

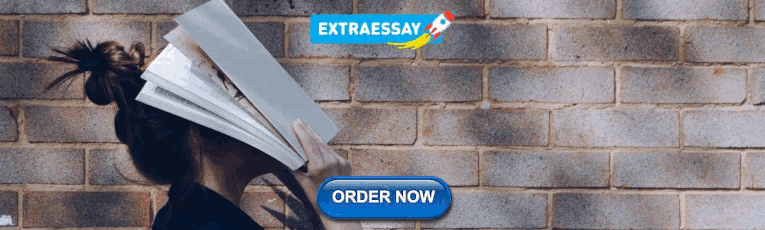
Study characteristics
The index test was imaging in all studies. Breast was the commonest organ studied (20 studies), followed by lung (11 studies) and brain (7 studies). Mammography (15 studies) was the commonest individual modality investigated, followed by plain film (12 studies), CT and MRI (11 studies each), tomosynthesis (six studies), ultrasound (two studies) and PET (one study); 9 studies investigated multiple modalities. In most studies (28 studies) the prime interpretation task was lesion detection. Eleven studies focused on lesion characterization and 12 combined detection and characterization. Forty-one studies compared 2 tests/conditions (i.e. a single test but used in different ways) to a reference standard (41 studies), while 2 studies compared 1 test/condition, 7 studies compared 3 tests/conditions, and 1 study compared 4 tests/conditions. Twenty-five studies combined data to create a reference standard while the reference was a single finding in 24 (14 imaging, 5 histology, 5 other – e.g. endoscopy). The reference method was unclear in 2 studies [54] , [55] .
Twenty-four studies were single center, 12 multicenter, with the number of centers unclear in 15 (29%) studies. Nine studies recruited symptomatic patients, 8 asymptomatic, and 7 a combination, but the majority (53%; 27 studies) did not state whether patients were symptomatic or not. 42 (82%) studies described the origin of patients with half of these stating a precise geographical region or hospital name. However, 9 (18%) studies did not sufficiently describe the source of patients and 21 (41%) did not describe patients' age and/or gender distribution.
Study design
Extracted data relating to study design and readers are presented graphically in Fig. 2 . Most studies (29; 57%) used patient data collected retrospectively. Fourteen (28%) were prospective while 2 used an existing database. Whether prospective/retrospective data was used was unstated/unclear in a further 6 (12%). While 13 studies (26%) used cases unselected other than for the disease in question, the majority (34; 67%) applied further criteria, for example to preselect “difficult” cases (11 studies), or to enrich disease prevalence (4 studies). How this selection bias was applied was stated explicitly in 18 (53%) of these 34. Whether selection bias was used was unclear in 4 studies.

The number of readers per study ranged from 2 [56] to 258 [76] . The mean number was 13, median 6. The large majority of studies (35; 69%) used fewer than 10 readers. Reader experience was described in 40 (78%) studies but not in 11. Specific reader training for image interpretation was described in 31 (61%) studies. Readers were not trained specifically in 14 studies and in 6 it was unclear whether readers were trained specifically or not. Readers were blind to clinical information for individual patients in 37 (73%) studies, unblind in 3, and this information was unrecorded or uncertain in 11 (22%). Readers were blind to prevalence in the dataset in 21 (41%) studies, unblind in 2, but this information was unsure/unrecorded or uncertain in the majority (28, 55%).
Observers read the same patient case on more than one occasion in 50 studies; this information was unclear in the single further study [70] . A fully crossed design (i.e. all readers read all patients with all modalities) was used in 47 (92%) studies, but not stated explicitly in 23 of these. A single study [72] did not use a fully crossed design and the design was unclear or unrecorded in 3 [34] , [70] , [76] . Case ordering was randomised (either a different random order across all readers or a different random order for each individual reader) between consecutive readings in 31 (61%) studies, unchanged in 6, and unclear/unrecorded in 14 (27%). The ordering of the index test being compared varied between consecutive readings in 20 (39%) studies, was unchanged in 17 (33%), and was unclear/unrecorded in 14 (27%). 26 (51%) studies employed a time interval between readings that ranged from 3 hours [50] to 2 months [63] , with a median of 4 weeks. There was no interval (i.e. reading of cases in all conditions occurred at the same sitting) in 17 (33%) studies, and time interval was unclear/unrecorded in 8 (16%).
Methods of reporting study outcomes
The unit of analysis for the ROC AUC analysis was the patient in 23 (45%) studies, an organ in 5, an organ segment in 5, a lesion in 11 (22%), other in 2, and unclear or unrecorded in 6 (12%); one study [34] examined both organ and lesion so there were 52 extractions for this item. Analysis was based on multiple images in 33 (65%) studies, a single image in 16 (31%), multiple modalities in a single study [40] , and unclear in a single study [57] ; no study used videos.
The number of disease positive patients per study ranged between 10 [79] and 100 [53] (mean 42, median 48) in 46 studies, and was unclear/unrecorded in 5 studies. The number of disease positive units of outcome for the primary ROC AUC analysis ranged between 10 [79] and 240 [41] (mean 59, median 50) in 43 studies, and was unclear/unrecorded in 8 studies. The number of disease negative patients per study ranged between 3 [69] and 352 [34] (mean 66, median 38) in 44 studies, was zero in 1 study [80] , and was unclear/unrecorded in 6 studies. The number of disease negative units of analysis for the primary outcome for the ROC AUC analysis ranged between 10 [51] and 535 [39] (mean 99, median 68) in 42 studies, and was unclear/unrecorded in the remaining 9 studies. The large majority of studies (41, 80%) presented readers with an image or set of images reflecting normal clinical practice whereas 10 presented specific lesions or regions of interest to readers.
Calculation of ROC AUC requires the use of confidence scores, where readers rate their confidence in the presence of a lesion or its characterization. In our previous study [6] we identified the assignment of confidence scores to be potentially on separate scales for disease positive and negative cases [7] . For rating scores used to calculate ROC AUC, 25 (49%) studies used a relatively small number of categories (defined as up to 10) and 25 (49%) used larger scales or a continuous measurement (e.g. visual analogue scale). One study did not specify the scale used [76] . Only 6 (12%) studies stated explicitly that readers were trained in advance to use the scoring system, for example being encouraged to use the full range available. In 15 (29%) studies there was the potential for multiple abnormalities in each unit of analysis (stated explicitly by 12 of these). This situation was dealt with by asking readers to assess the most advanced or largest lesion (e.g. [43] ), by an analysis using the highest score attributed (e.g. [42] ), or by adopting a per-lesion analysis (e.g. [52] ). For 23 studies only a single abnormality per unit of analysis was possible, whereas this issue was unclear in 13 studies.
Model assumptions
The majority of studies (41, 80%) asked readers to ascribe the same scoring system to both disease-positive and disease-negative patients. Another 9 studies asked that different scoring systems be used, depending on whether the case was perceived as positive or negative (e.g. [61] ), or depending on the nature of the lesion perceived (e.g. [66] ). Scoring was unclear in a single study [76] . No study stated that two types of true-negative classifications were possible (i.e. where a lesion was seen but misclassified vs. not being seen at all), a situation that potentially applied to 22 (43%) of the 51 studies. Another concern occurs when more than one observation for each patient is included in the analysis, violating the assumption that data are independent. This could occur if multiple diseased segments were analysed for each patient without using a statistical method that treats these as clustered data. An even more flawed approach occurs when analysis includes one segment for patients without disease but multiple segments for patients with disease.
When publically available DBM MRMC software [81] is used for ROC AUC modeling, this requires assumptions of normality for confidence scores or their transformations if the standard parametric ROC curve fitting methods are used. When scores are not normally distributed, even if non parametric approaches are used to estimate ROC AUC, this lack of normality may indicate additional problems with obtaining reliable estimates of ROC AUC [82] – [86] . While 17 studies stated explicitly that the data fulfilled the assumptions necessary for modeling, none described whether confidence scores were transformed to a normal distribution for analysis. Indeed, only 3 studies [54] , [73] , [76] described the distribution of confidence scores, which was non-normal in each case.
Model fitting
Thirty (59%) studies presented ROC curves based on confidence scores; i.e. 21 (41%) studies showed no ROC curve. Of the 30 with curves, only 5 presented a curve for each reader whereas 24 presented curves averaged over all readers; a further study presented both. Of the 30 studies presenting ROC curves, 26 (87%) showed only smoothed curves, with the data points underlying the ROC curve presented in only 4 (13%) [43] , [51] , [63] , [78] . Thus, a ROC curve with underlying data points was presented in only 4 of 51 (8%) studies overall. The degree of extrapolation is critical in understanding the reliability of the ROC AUC result [7] . However, extrapolation could only be assessed in these four articles, with unreasonable extrapolation, by our definition, occurring in two [43] , [63] .
The majority of studies (31, 61%) did not specify the method used for curve fitting. Of the 20 that did, 7 used non-parametric methods (Trapezoidal/Wilcoxon), 8 used parametric methods (7 of which used Proproc), 3 used other methods, and 2 used a combination. Previous research [7] , [84] has demonstrated considerable problems fitting ROC curves due to degenerate data where the fitted ROC curve corresponds to vertical and horizontal lines, e.g there are no FP data. Only 2 articles described problems with curve fitting [55] , [61] . Two studies stated that data was degenerate: Subhas and co-workers [66] stated that, “data were not well dispersed over the five confidence level scores”. Moin and co-workers [53] stated that, “If we were to recode categories 1 and 2, and discard BI-RADS 0 in the ROC analysis, it would yield degenerative results because the total number of cases collected would not be adequate”. While all studies used MRMC AUC methods to compare AUC outcomes, 5 studies also used other methods (e.g. t-testing) [37] , [52] , [60] , [67] , [77] . Only 3 studies described using a partial AUC [42] , [55] , [77] . Forty-four studies additionally reported non-AUC outcomes (e.g. McNemar's test to compare test performance at a specified diagnostic threshold [58] , Wilcoxon signed rank test to compare changes in patient management decisions [64] ). Eight (16%) of the studies included a ROC researcher as an author [39] , [47] , [48] , [54] , [60] , [65] , [66] , [72] .
Presentation of results
Extracted data relating to the presentation of individual study results is presented graphically in Fig. 3 . All studies presented ROC AUC as an accuracy measure with 49 (96%) presenting the change in AUC for the conditions tested. Thirty-five (69%) studies presented additional measures such as change in sensitivity/specificity (24 studies), positive/negative predictive values (5 studies), or other measures (e.g. changes in clinical management decisions [64] , intraobserver agreement [36] ). Change in AUC was the primary outcome in 45 (88%) studies. Others used sensitivity [34] , [40] , accuracy [35] , [69] , the absolute AUC [44] or JAFROC figure of merit [68] . All studies presented an average of the primary outcome over all readers, with individual reader results presented in 38 (75%) studies but not in 13 (25%). The mean change/difference in AUC was 0.051 (range −0.052 to 0.280) across the extracted studies and was stated as “significant” in 31 and “non-significant” in the remaining 20. No study failed to comment on significance of the stated change/difference in AUC. In 22 studies we considered that a significant change in AUC was unlikely to be due to results from a single reader/patient but we could not determine whether this was possible in 11 studies, and judged this not-applicable in a further 18 studies. One study appeared to report an advantage for a test when the AUC increased, but not significantly [65] . There were 5 (10%) studies where there appeared to be discrepancies between the data presented in the abstract/text/ROC curve [36] , [38] , [69] , [77] , [80] .

While the majority of studies (42, 82%) did not present an interpretation of their data framed in terms of changes to individual patient diagnoses, 9 (18%) did so, using outcomes in addition to ROC AUC: For example, as a false-positive to true-positive ratio [35] or the proportion of additional biopsies precipitated and disease detected [64] , or effect on callback rate [43] . The change in AUC was non-significant in 22 studies and in 12 of these the authors speculated why, for example stating that the number of cases was likely to be inadequate [65] , [70] , that the observer task was insufficiently taxing [36] , or that the difference was too subtle to be resolved [45] . For studies where a non-significant change in AUC was observed, authors sometimes framed this as demonstrating equivalence (16 studies, e.g. [55] , [74] ), stated that there were other benefits (3 studies), or adopted other interpretations. For example, one study stated that there were “beneficial” effects on many cases despite a non-significant change in AUC [54] and one study stated that the intervention “improved visibility” of microcalcifications noting that the lack of any statistically significant difference warranted further investigation [65] .
While many studies have used ROC AUC as an outcome measure, very little research has investigated how these studies are conducted, analysed and presented. We could find only a single existing systematic review that has investigated this question [87] . The authors stated in their Introduction, “we are not aware of any attempt to provide an overview of the kinds of ROC analyses that have been most commonly published in radiologic research.” They investigated articles published in the journal “Radiology” between 1997 and 2006, identifying 295 studies [87] . The authors concluded that “ROC analysis is widely used in radiologic research, confirming its fundamental role in assessing diagnostic performance”. For the present review, we wished to focus on MRMC studies specifically, since these are most complex and are often used as the basis for technology licensing. We also wished to broaden our search criteria beyond a single journal. Our systematic review found that the quality of data reporting in MRMC studies using ROC AUC as an outcome measure was frequently incomplete and who would therefore agree with the conclusions of Shiraishi et al. who stated that studies, “were not always adequate to support clear and clinically relevant conclusions” [87] .
Many omissions we identified were those related to general study design and execution, and are well-covered by the STARD initiative [88] as factors that should be reported in studies of diagnostic test accuracy in general. For example, we found that the number of participating research centres was unclear in approximately one-third of studies, that most studies did not describe whether patients were symptomatic or asymptomatic, that criteria applied to case selection were sometimes unclear, and that observer blinding was not mentioned in one-fifth of studies. Regarding statistical methods, STARD states that studies should, “describe methods for calculating or comparing measures of diagnostic accuracy” [88] ; this systematic review aimed to focus on description of methods for MRMC studies using ROC AUC as an outcome measure.
The large majority of studies used less than 10 observers, some did not describe reader experience, and the majority did not mention whether observers were aware of prevalence of abnormality, a factor that may influence diagnostic vigilance. Most studies required readers to detect lesions while a minority asked for characterization, and others were a combination of the two. We believe it is important for readers to understand the precise nature of the interpretative task since this will influence the rating scale used to build the ROC curve. A variety of units of analysis were adopted, with just under half being the patient case. We were surprised that some studies failed to record the number of disease-positive and disease-negative patients in their dataset. Concerning the confidence scales used to construct the ROC curve, only a small minority (12%) of studies stated that readers were trained to use these in advance of scoring. We believe such training is important so that readers can appreciate exactly how the interpretative task relates to the scale; there is evidence that radiologists score in different ways when asked to perform the same scoring task because of differences in how they interpret the task [89] . For example, readers should appreciate how the scale reflects lesion detection and/or characterization, especially if both are required, and how multiple abnormalities per unit of analysis are handled. Encouragement to use the full range of the scale is required for normal rating distributions. Whether readers must use the same scale for patients with and without pathology is also important to know.
Despite their importance for understanding the validity of study results, we found that description of the confidence scores, the ROC curve and its analysis was often incomplete. Strikingly, only three studies described the distribution of confidence scores and none stated whether transformation to a normal distribution was needed. When publically available DBM MRMC software (ref DBM) is used for ROC AUC modeling, this requires assumptions of normality for confidence scores or their transformations when ROC curve fitting methods are used. Where confidence scores are not normally distributed these software methods are not recommended [84] – [86] , [90] . Although Hanley shows that ROC curves can be reasonable under some distributions of non normal data [91] , concerns have been raised particularly in imaging detection studies measuring clinically useful tests with good performance to distinguish well defined abnormalities. In tests with good performance two factors make estimation of ROC AUC unreliable. Firstly readers' scores are by definition often at the ends of the confidence scale so that the confidence score distributions for normal and abnormal cases have very little overlap [82] – [86] . Secondly tests with good performance also have few false positives making ROC AUC estimation highly dependent on confidence scores assigned to possibly fewer than 5% or 10% of cases in the study [86] .
Most studies did not describe the method used for curve fitting. Over 40% of studies presented no ROC curve in the published article. When present, the large majority were smoothed and averaged over all readers. Only four articles presented data points underlying the curve meaning that the degree of any extrapolation could not be assessed despite this being an important factor regarding interpretation of results [92] . While, by definition, all studies used MRMC AUC methods, most reported additional non-AUC outcomes. Approximately one-quarter of studies did not present AUC data for individual readers. Because of this, variability between readers and/or the effect of individual readers on the ultimate statistical analysis could not be assessed.
Interpretation of study results was variable. Notably, when no significant change in AUC was demonstrated, authors stated that the number of cases was either insufficient or that the difference could not be resolved by the study, appearing to claim that their studies were underpowered rather than that the intervention was ineffective when required to improve diagnostic accuracy. Indeed some studies claimed an advantage for a new test in the face of a non-significant increase in AUC, or turned to other outcomes as proof of benefit. Some interpreted no significant difference in AUC as implying equivalence.
Our review does have limitations. Indexing of the statistical methods used to analyse studies is not common so we used a proxy to identify studies; their citation of “key” references related to MRMC ROC methodology. While it is possible we missed some studies, our aim was not to identify all studies using such analyses. Rather, we aimed to gather a representative sample that would provide a generalizable picture of how such studies are reported. It is also possible that by their citation of methodological papers (and on occasion including a ROC researcher as an author), our review was biased towards papers likely to be of higher methodological quality than average. This systematic review was cross-disciplinary and two radiological researchers performed the bulk of the extraction rather than statisticians. This proved challenging since the depth of statistical knowledge required was demanding, especially when details of the analysis was being considered. We anticipated this and piloted extraction on a sample of five papers to determine if the process was feasible, deciding that it was. Advice from experienced statisticians was also available when uncertainty arose.
In summary, via systematic review we found that MRMC studies using ROC AUC as the primary outcome measure often omit important information from both the study design and analysis, and presentation of results is frequently not comprehensive. Authors using MRMC ROC analyses should be encouraged to provide a full description of their methods and results so as to increase interpretability.
Supporting Information
Extraction sheet used for the systematic review.
Raw data extracted for the systematic review.
S1 PRISMA Checklist
Funding statement.
This work was supported by the UK National Institute for Health (NIHR) Research under its Programme Grants for Applied Research funding scheme (RP-PG-0407-10338). The funder had no role in the design, execution, analysis, reporting, or decision to submit for publication.
Data Availability
iMRMC Multi-Reader, Multi-Case Analysis Methods (ROC, Agreement, and Other Metrics)
- convertDF: Convert MRMC data frames
- convertDFtoDesignMatrix: Convert an MRMC data frame to a design matrix
- convertDFtoScoreMatrix: Convert an MRMC data frame to a score matrix
- createGroups: Assign a group label to items in a vector
- createIMRMCdf: Convert a data frame with all needed factors to doIMRMC...
- doIMRMC: MRMC analysis of the area under the ROC curve
- extractPairedComparisonsBRBM: Extract between-reader between-modality pairs of scores
- extractPairedComparisonsWRBM: Extract within-reader between-modality pairs of scores
- getBRBM: Get between-reader, between-modality paired data from an MRMC...
- getMRMCscore: Get a score from an MRMC data frame
- getWRBM: Get within-reader, between-modality paired data from an MRMC...
- init.lecuyerRNG: Initialize the l'Ecuyer random number generator
- laBRBM: MRMC analysis of between-reader between-modality limits of...
- laWRBM: MRMC analysis of within-reader between-modality limits of...
- renameCol: Rename a data frame column name or a list object name
- roc2binary: Convert ROC data formatted for doIMRMC to TPF and FPF data...
- roeMetzConfigs: roeMetzConfigs
- sim.gRoeMetz: Simulate an MRMC data set of an ROC experiment comparing two...
- sim.gRoeMetz.config: Create a configuration object for the sim.gRoeMetz program
- simMRMC: Simulate an MRMC data set
- simRoeMetz.example: Simulates a sample MRMC ROC experiment
- successDFtoROCdf: Convert an MRMC data frame of successes to one formatted for...
- undoIMRMCdf: Convert a doIMRMC formatted data frame to a standard data...
- uStat11: Analysis of U-statistics degree 1,1
- uStat11.diff: Create the kernel and design matrices for uStat11
- uStat11.identity: Create the kernel and design matrices for uStat11
- Browse all...
iMRMC: Multi-Reader, Multi-Case Analysis Methods (ROC, Agreement, and Other Metrics)
Do Multi-Reader, Multi-Case (MRMC) analyses of data from imaging studies where clinicians (readers) evaluate patient images (cases). What does this mean? ... Many imaging studies are designed so that every reader reads every case in all modalities, a fully-crossed study. In this case, the data is cross-correlated, and we consider the readers and cases to be cross-correlated random effects. An MRMC analysis accounts for the variability and correlations from the readers and cases when estimating variances, confidence intervals, and p-values. The functions in this package can treat arbitrary study designs and studies with missing data, not just fully-crossed study designs. The initial package analyzes the reader-average area under the receiver operating characteristic (ROC) curve with U-statistics according to Gallas, Bandos, Samuelson, and Wagner 2009 <doi:10.1080/03610920802610084>. Additional functions analyze other endpoints with U-statistics (binary performance and score differences) following the work by Gallas, Pennello, and Myers 2007 <doi:10.1364/JOSAA.24.000B70>. Package development and documentation is at <https://github.com/DIDSR/iMRMC/tree/master>.
Getting started
Browse package contents, try the imrmc package in your browser.
Any scripts or data that you put into this service are public.
R Package Documentation
Browse r packages, we want your feedback.

Add the following code to your website.
REMOVE THIS Copy to clipboard
For more information on customizing the embed code, read Embedding Snippets .
Europe PMC requires Javascript to function effectively.
Either your web browser doesn't support Javascript or it is currently turned off. In the latter case, please turn on Javascript support in your web browser and reload this page.
Search life-sciences literature (44,128,147 articles, preprints and more)
- Free full text
- Citations & impact
- Similar Articles
Multi-reader multi-case studies using the area under the receiver operator characteristic curve as a measure of diagnostic accuracy: systematic review with a focus on quality of data reporting.
Author information, affiliations.
- Dendumrongsup T 1
- Halligan S 2
- Fanshawe TR 3
- Mallett S 3
- Altman DG 4
ORCIDs linked to this article
- Mallett S | 0000-0002-0596-8200
- Fanshawe TR | 0000-0002-9928-8934
- Halligan S | 0000-0003-0632-5108
Plos one , 26 Dec 2014 , 9(12): e116018 https://doi.org/10.1371/journal.pone.0116018 PMID: 25541977 PMCID: PMC4277459
Abstract
- Introduction
Conclusions
Free full text .

Multi-Reader Multi-Case Studies Using the Area under the Receiver Operator Characteristic Curve as a Measure of Diagnostic Accuracy: Systematic Review with a Focus on Quality of Data Reporting
Thaworn dendumrongsup.
1 Department of Radiology, Prince of Songkla University, Hat Yai, Thailand,
Andrew A. Plumb
2 Centre for Medical Imaging, University College London, London, United Kingdom,
Steve Halligan
Thomas r. fanshawe.
3 Nuffield Department of Primary Care Health Sciences, Oxford University, Oxford, United Kingdom,
Douglas G. Altman
4 Centre for Statistics in Medicine, Wolfson College, Oxford University, Oxford, United Kingdom,
Susan Mallett
Conceived and designed the experiments: TD AAP SH TRF DGA SM. Performed the experiments: TD AAP SH TRF DGA SM. Analyzed the data: TD AAP SH TRF DGA SM. Contributed reagents/materials/analysis tools: TD AAP SH TRF DGA SM. Wrote the paper: TD AAP SH TRF DGA SM.
- Associated Data
The authors confirm that all data underlying the findings are fully available without restriction. All relevant data are within the paper and its Supporting Information files.
We examined the design, analysis and reporting in multi-reader multi-case (MRMC) research studies using the area under the receiver-operating curve (ROC AUC) as a measure of diagnostic performance.
We performed a systematic literature review from 2005 to 2013 inclusive to identify a minimum 50 studies. Articles of diagnostic test accuracy in humans were identified via their citation of key methodological articles dealing with MRMC ROC AUC. Two researchers in consensus then extracted information from primary articles relating to study characteristics and design, methods for reporting study outcomes, model fitting, model assumptions, presentation of results, and interpretation of findings. Results were summarized and presented with a descriptive analysis.
Sixty-four full papers were retrieved from 475 identified citations and ultimately 49 articles describing 51 studies were reviewed and extracted. Radiological imaging was the index test in all. Most studies focused on lesion detection vs. characterization and used less than 10 readers. Only 6 (12%) studies trained readers in advance to use the confidence scale used to build the ROC curve. Overall, description of confidence scores, the ROC curve and its analysis was often incomplete. For example, 21 (41%) studies presented no ROC curve and only 3 (6%) described the distribution of confidence scores. Of 30 studies presenting curves, only 4 (13%) presented the data points underlying the curve, thereby allowing assessment of extrapolation. The mean change in AUC was 0.05 (−0.05 to 0.28). Non-significant change in AUC was attributed to underpowering rather than the diagnostic test failing to improve diagnostic accuracy.
Data reporting in MRMC studies using ROC AUC as an outcome measure is frequently incomplete, hampering understanding of methods and the reliability of results and study conclusions. Authors using this analysis should be encouraged to provide a full description of their methods and results.
The receiver operator characteristic (ROC) curve describes a plot of sensitivity versus 1-specificity for a diagnostic test, across the whole range of possible diagnostic thresholds [1] . The area under the ROC curve (ROC AUC) is a well-recognised single measure that is often used to combine elements of both sensitivity and specificity, sometimes replacing these two measures. ROC AUC is often used to describe the diagnostic performance of radiological tests, either to compare the performance of different tests or the same test under different circumstances [2] , [3] . Radiological tests must be interpreted by human observers and a common study design uses multiple readers to interpret multiple image cases; the multi-reader multi-case (MRMC) design [4] . The MRMC design is popular because once a radiologist has viewed 20 cases there is less information to be gained by asking him to view a further 20 than by asking a different radiologist to view the same 20. This procedure enhances the generalisability of study results and having multiple readers interpret multiple cases enhances statistical power. Because multiple radiologists view the same cases, “clustering” occurs. For example, small lesions are generally seen less frequently than larger lesions, i.e. reader observations are clustered within cases. Similarly, more experienced readers are likely to perform better across a series of cases than less experienced readers, i.e. results are correlated within readers. Bootstrap resampling and multilevel modeling can account for clustering, linking results from the same observers and cases, so that 95% confidence intervals are not too narrow. MRMC studies using ROC AUC as the primary outcome are often required by regulatory bodies for the licensing of new radiological devices [5] .
We attempted to use ROC AUC as the primary outcome measure in a prior MRMC study of computer-assisted detection (CAD) for CT colonography [6] . However, we encountered several difficulties when trying to implement this approach, described in detail elsewhere [7] . Many of these difficulties were related to issues implementing confidence scores in a transparent and reliable fashion, which led ultimately to a flawed analysis. We considered, therefore, that for ROC AUC to be a valid measure there are methodological components that need addressing in study design, data collection and analysis, and interpretation. Based on our attempts to implement the MRMC ROC AUC analysis, we were interested in whether other researchers have encountered similar hurdles and, if so, how these issues were tackled.
In order to investigate how often other studies have addressed and reported on methodological issues with implementing ROC AUC, we performed a systematic review of MRMC studies using ROC AUC an outcome measure. We searched and investigated the available literature with the objective to describe the statistical methods used, the completeness of data presentation, and investigate whether any problems with analysis were encountered and reported.
Ethics statement
Ethical approval is not required by our institutions for research studies of published data.
Search strategy, inclusion and exclusion criteria
This systematic review was performed guided by the Preferred Reporting Items for Systematic Reviews and Meta-Analyses (PRISMA), an evidence-based minimum set of items for reporting in systematic reviews and meta-analyses [8] . We developed an extraction sheet for the systematic review, broken down into different sections (used as subheadings for the Results section of this report), with notes relating to each individual item extracted ( S1 File ). In consensus we considered approximately 50 articles would provide a sufficiently representative overview of current reporting practice. Based on our prior experience of performing systematic reviews we believed that searching for additional articles beyond 50 would be unlikely to yield valuable additional data (i.e. we believed we would reach “saturation” by 50 articles) yet would present a very considerable extraction burden.
In order to achieve this, potentially eligible primary articles published between 2005 and February 2013 inclusive were identified by a radiologist researcher (TD) using PUBMED via their citation of one or more of 8 key methodological articles relating to MRMC ROC AUC analysis [9] – [16] . To achieve this the Authors' names (combined using “AND”) were entered in the PUBMED search field and the specific article identified and clicked in the results list. The abstract was then accessed and the “Cited By # PubMed Central Articles” link and “Related Citations” link used to identify those articles in the PubMed Central database that have cited the original article. There was no language restriction. Online abstracts were examined in reverse chronological order, the full text of potentially eligible papers then retrieved, and selection stopped once the threshold of 50 studies fulfilling inclusion criteria had been passed.
To be eligible, primary studies had to be diagnostic test accuracy studies of human observers interpreting medical image data from real patients, and attempting to use a MRMC ROC AUC analysis as a study outcome based on the following methodological approaches [9] – [16] ; Reviews, solely methodological papers, and those using simulated imaging data were excluded.
Data extraction
An initial pilot sample of 5 full-paper articles were extracted and the data checked by a subgroup of investigators in consensus, to both confirm the process was feasible and to identify potential problems. These papers were extracted by TD using the search strategy described in the previous section. A further 10 full-papers were extracted by two radiologist researchers again using the same search strategy and working independently (TD, AP) to check agreement further. The remaining articles included in the review were extracted predominantly by TD, who discussed any concerns/uncertainty with AP. Any disagreement following their discussion was arbitrated by SH and/or SM where necessary. These discussions took place during two meetings when the authors met to discuss progress of the review; multiple papers and issues were discussed on each occasion.
The extraction covered the following broad topics: Study characteristics, methods to record study outcomes, model assumptions, model fitting, data presentation ( S1 File ).
We extracted data relating to the organ and disease studied, the nature of the diagnostic task (e.g. characterization vs. localization vs. presence/absence), test methods, patient source and characteristics, study design (e.g. prospective/retrospective, secondary analysis, single/multicenter) and reference standard. We extracted the number of readers, their prior experience, specific interpretation training for the study (e.g. use of CAD software), blinding to clinical data and/or reference results, the number of times they read each case and the presence of any washout period to diminish recall bias, case ordering, and whether all readers read all cases (i.e. a fully-crossed design). We extracted the unit of analysis (e.g. patient vs. organ vs. segment), and sample size for patients with and without pathology.
We noted whether study imaging reflected normal daily clinical practice or was modified for study purposes (e.g. restricted to limited images). We noted the confidence scores used for the ROC curve and their scale, and whether training was provided for scoring. We noted if there were multiple lesions per unit of analysis. We noted if scoring differed for positive and negative patient cases, whether score distribution was reported, and whether transformation to a normal distribution was performed.
We extracted if ROC cures were presented in the published article and, if so, whether for individual readers, whether the curve was smoothed, and if underlying data points were shown. We defined unreasonable extrapolation as an absence of data in the right-hand 25% of the plot space. We noted the method for curve fitting and whether any problems with fitting were reported, and the method used to compare AUC or pAUC. We extracted the primary outcome, the accuracy measures reported, and whether these were overall or for individual readers. We noted the size of any change in AUC, whether this was significant, and made a subjective assessment of whether significance could be attributed to a single reader or case. We noted how the study authors interpreted change in AUC, if any, and whether any change was reported in terms of effect on individual patients. We also noted if a ROC researcher was named as an author or acknowledged, defined as an individual who had published indexed research papers dealing with ROC methodology.
Data were summarized in an Excel worksheet (Excel For Mac 14.3.9, Microsoft Corporation) with additional cells for explanatory free text. A radiologist researcher (SH) then compiled the data and extracted frequencies, consulting the two radiologists who performed the extraction for clarification when necessary. The investigator group discussed the implication of the data subsequently, to guide interpretation.
Four hundred and seventy five citations of the 8 key methodological papers were identified and 64 full papers retrieved subsequently. Fifteen [17] – [31] of these were rejected after reading the full text (the papers and reason for rejection are shown in Table 1 ) leaving 49 [32] – [80] for extraction and analysis that were published between 2010 and 2012 inclusive; these are detailed in Table 1 . Two papers [61] , [75] contributed two separate studies each, meaning that 51 studies were extracted in total. The PRISMA checklist [8] is detailed in Fig. 1 . The raw extracted data are available in S2 File .

Study characteristics
The index test was imaging in all studies. Breast was the commonest organ studied (20 studies), followed by lung (11 studies) and brain (7 studies). Mammography (15 studies) was the commonest individual modality investigated, followed by plain film (12 studies), CT and MRI (11 studies each), tomosynthesis (six studies), ultrasound (two studies) and PET (one study); 9 studies investigated multiple modalities. In most studies (28 studies) the prime interpretation task was lesion detection. Eleven studies focused on lesion characterization and 12 combined detection and characterization. Forty-one studies compared 2 tests/conditions (i.e. a single test but used in different ways) to a reference standard (41 studies), while 2 studies compared 1 test/condition, 7 studies compared 3 tests/conditions, and 1 study compared 4 tests/conditions. Twenty-five studies combined data to create a reference standard while the reference was a single finding in 24 (14 imaging, 5 histology, 5 other – e.g. endoscopy). The reference method was unclear in 2 studies [54] , [55] .
Twenty-four studies were single center, 12 multicenter, with the number of centers unclear in 15 (29%) studies. Nine studies recruited symptomatic patients, 8 asymptomatic, and 7 a combination, but the majority (53%; 27 studies) did not state whether patients were symptomatic or not. 42 (82%) studies described the origin of patients with half of these stating a precise geographical region or hospital name. However, 9 (18%) studies did not sufficiently describe the source of patients and 21 (41%) did not describe patients' age and/or gender distribution.
Study design
Extracted data relating to study design and readers are presented graphically in Fig. 2 . Most studies (29; 57%) used patient data collected retrospectively. Fourteen (28%) were prospective while 2 used an existing database. Whether prospective/retrospective data was used was unstated/unclear in a further 6 (12%). While 13 studies (26%) used cases unselected other than for the disease in question, the majority (34; 67%) applied further criteria, for example to preselect “difficult” cases (11 studies), or to enrich disease prevalence (4 studies). How this selection bias was applied was stated explicitly in 18 (53%) of these 34. Whether selection bias was used was unclear in 4 studies.

The number of readers per study ranged from 2 [56] to 258 [76] . The mean number was 13, median 6. The large majority of studies (35; 69%) used fewer than 10 readers. Reader experience was described in 40 (78%) studies but not in 11. Specific reader training for image interpretation was described in 31 (61%) studies. Readers were not trained specifically in 14 studies and in 6 it was unclear whether readers were trained specifically or not. Readers were blind to clinical information for individual patients in 37 (73%) studies, unblind in 3, and this information was unrecorded or uncertain in 11 (22%). Readers were blind to prevalence in the dataset in 21 (41%) studies, unblind in 2, but this information was unsure/unrecorded or uncertain in the majority (28, 55%).
Observers read the same patient case on more than one occasion in 50 studies; this information was unclear in the single further study [70] . A fully crossed design (i.e. all readers read all patients with all modalities) was used in 47 (92%) studies, but not stated explicitly in 23 of these. A single study [72] did not use a fully crossed design and the design was unclear or unrecorded in 3 [34] , [70] , [76] . Case ordering was randomised (either a different random order across all readers or a different random order for each individual reader) between consecutive readings in 31 (61%) studies, unchanged in 6, and unclear/unrecorded in 14 (27%). The ordering of the index test being compared varied between consecutive readings in 20 (39%) studies, was unchanged in 17 (33%), and was unclear/unrecorded in 14 (27%). 26 (51%) studies employed a time interval between readings that ranged from 3 hours [50] to 2 months [63] , with a median of 4 weeks. There was no interval (i.e. reading of cases in all conditions occurred at the same sitting) in 17 (33%) studies, and time interval was unclear/unrecorded in 8 (16%).
Methods of reporting study outcomes
The unit of analysis for the ROC AUC analysis was the patient in 23 (45%) studies, an organ in 5, an organ segment in 5, a lesion in 11 (22%), other in 2, and unclear or unrecorded in 6 (12%); one study [34] examined both organ and lesion so there were 52 extractions for this item. Analysis was based on multiple images in 33 (65%) studies, a single image in 16 (31%), multiple modalities in a single study [40] , and unclear in a single study [57] ; no study used videos.
The number of disease positive patients per study ranged between 10 [79] and 100 [53] (mean 42, median 48) in 46 studies, and was unclear/unrecorded in 5 studies. The number of disease positive units of outcome for the primary ROC AUC analysis ranged between 10 [79] and 240 [41] (mean 59, median 50) in 43 studies, and was unclear/unrecorded in 8 studies. The number of disease negative patients per study ranged between 3 [69] and 352 [34] (mean 66, median 38) in 44 studies, was zero in 1 study [80] , and was unclear/unrecorded in 6 studies. The number of disease negative units of analysis for the primary outcome for the ROC AUC analysis ranged between 10 [51] and 535 [39] (mean 99, median 68) in 42 studies, and was unclear/unrecorded in the remaining 9 studies. The large majority of studies (41, 80%) presented readers with an image or set of images reflecting normal clinical practice whereas 10 presented specific lesions or regions of interest to readers.
Calculation of ROC AUC requires the use of confidence scores, where readers rate their confidence in the presence of a lesion or its characterization. In our previous study [6] we identified the assignment of confidence scores to be potentially on separate scales for disease positive and negative cases [7] . For rating scores used to calculate ROC AUC, 25 (49%) studies used a relatively small number of categories (defined as up to 10) and 25 (49%) used larger scales or a continuous measurement (e.g. visual analogue scale). One study did not specify the scale used [76] . Only 6 (12%) studies stated explicitly that readers were trained in advance to use the scoring system, for example being encouraged to use the full range available. In 15 (29%) studies there was the potential for multiple abnormalities in each unit of analysis (stated explicitly by 12 of these). This situation was dealt with by asking readers to assess the most advanced or largest lesion (e.g. [43] ), by an analysis using the highest score attributed (e.g. [42] ), or by adopting a per-lesion analysis (e.g. [52] ). For 23 studies only a single abnormality per unit of analysis was possible, whereas this issue was unclear in 13 studies.
Model assumptions
The majority of studies (41, 80%) asked readers to ascribe the same scoring system to both disease-positive and disease-negative patients. Another 9 studies asked that different scoring systems be used, depending on whether the case was perceived as positive or negative (e.g. [61] ), or depending on the nature of the lesion perceived (e.g. [66] ). Scoring was unclear in a single study [76] . No study stated that two types of true-negative classifications were possible (i.e. where a lesion was seen but misclassified vs. not being seen at all), a situation that potentially applied to 22 (43%) of the 51 studies. Another concern occurs when more than one observation for each patient is included in the analysis, violating the assumption that data are independent. This could occur if multiple diseased segments were analysed for each patient without using a statistical method that treats these as clustered data. An even more flawed approach occurs when analysis includes one segment for patients without disease but multiple segments for patients with disease.
When publically available DBM MRMC software [81] is used for ROC AUC modeling, this requires assumptions of normality for confidence scores or their transformations if the standard parametric ROC curve fitting methods are used. When scores are not normally distributed, even if non parametric approaches are used to estimate ROC AUC, this lack of normality may indicate additional problems with obtaining reliable estimates of ROC AUC [82] – [86] . While 17 studies stated explicitly that the data fulfilled the assumptions necessary for modeling, none described whether confidence scores were transformed to a normal distribution for analysis. Indeed, only 3 studies [54] , [73] , [76] described the distribution of confidence scores, which was non-normal in each case.
Model fitting
Thirty (59%) studies presented ROC curves based on confidence scores; i.e. 21 (41%) studies showed no ROC curve. Of the 30 with curves, only 5 presented a curve for each reader whereas 24 presented curves averaged over all readers; a further study presented both. Of the 30 studies presenting ROC curves, 26 (87%) showed only smoothed curves, with the data points underlying the ROC curve presented in only 4 (13%) [43] , [51] , [63] , [78] . Thus, a ROC curve with underlying data points was presented in only 4 of 51 (8%) studies overall. The degree of extrapolation is critical in understanding the reliability of the ROC AUC result [7] . However, extrapolation could only be assessed in these four articles, with unreasonable extrapolation, by our definition, occurring in two [43] , [63] .
The majority of studies (31, 61%) did not specify the method used for curve fitting. Of the 20 that did, 7 used non-parametric methods (Trapezoidal/Wilcoxon), 8 used parametric methods (7 of which used Proproc), 3 used other methods, and 2 used a combination. Previous research [7] , [84] has demonstrated considerable problems fitting ROC curves due to degenerate data where the fitted ROC curve corresponds to vertical and horizontal lines, e.g there are no FP data. Only 2 articles described problems with curve fitting [55] , [61] . Two studies stated that data was degenerate: Subhas and co-workers [66] stated that, “data were not well dispersed over the five confidence level scores”. Moin and co-workers [53] stated that, “If we were to recode categories 1 and 2, and discard BI-RADS 0 in the ROC analysis, it would yield degenerative results because the total number of cases collected would not be adequate”. While all studies used MRMC AUC methods to compare AUC outcomes, 5 studies also used other methods (e.g. t-testing) [37] , [52] , [60] , [67] , [77] . Only 3 studies described using a partial AUC [42] , [55] , [77] . Forty-four studies additionally reported non-AUC outcomes (e.g. McNemar's test to compare test performance at a specified diagnostic threshold [58] , Wilcoxon signed rank test to compare changes in patient management decisions [64] ). Eight (16%) of the studies included a ROC researcher as an author [39] , [47] , [48] , [54] , [60] , [65] , [66] , [72] .
Presentation of results
Extracted data relating to the presentation of individual study results is presented graphically in Fig. 3 . All studies presented ROC AUC as an accuracy measure with 49 (96%) presenting the change in AUC for the conditions tested. Thirty-five (69%) studies presented additional measures such as change in sensitivity/specificity (24 studies), positive/negative predictive values (5 studies), or other measures (e.g. changes in clinical management decisions [64] , intraobserver agreement [36] ). Change in AUC was the primary outcome in 45 (88%) studies. Others used sensitivity [34] , [40] , accuracy [35] , [69] , the absolute AUC [44] or JAFROC figure of merit [68] . All studies presented an average of the primary outcome over all readers, with individual reader results presented in 38 (75%) studies but not in 13 (25%). The mean change/difference in AUC was 0.051 (range −0.052 to 0.280) across the extracted studies and was stated as “significant” in 31 and “non-significant” in the remaining 20. No study failed to comment on significance of the stated change/difference in AUC. In 22 studies we considered that a significant change in AUC was unlikely to be due to results from a single reader/patient but we could not determine whether this was possible in 11 studies, and judged this not-applicable in a further 18 studies. One study appeared to report an advantage for a test when the AUC increased, but not significantly [65] . There were 5 (10%) studies where there appeared to be discrepancies between the data presented in the abstract/text/ROC curve [36] , [38] , [69] , [77] , [80] .

While the majority of studies (42, 82%) did not present an interpretation of their data framed in terms of changes to individual patient diagnoses, 9 (18%) did so, using outcomes in addition to ROC AUC: For example, as a false-positive to true-positive ratio [35] or the proportion of additional biopsies precipitated and disease detected [64] , or effect on callback rate [43] . The change in AUC was non-significant in 22 studies and in 12 of these the authors speculated why, for example stating that the number of cases was likely to be inadequate [65] , [70] , that the observer task was insufficiently taxing [36] , or that the difference was too subtle to be resolved [45] . For studies where a non-significant change in AUC was observed, authors sometimes framed this as demonstrating equivalence (16 studies, e.g. [55] , [74] ), stated that there were other benefits (3 studies), or adopted other interpretations. For example, one study stated that there were “beneficial” effects on many cases despite a non-significant change in AUC [54] and one study stated that the intervention “improved visibility” of microcalcifications noting that the lack of any statistically significant difference warranted further investigation [65] .
While many studies have used ROC AUC as an outcome measure, very little research has investigated how these studies are conducted, analysed and presented. We could find only a single existing systematic review that has investigated this question [87] . The authors stated in their Introduction, “we are not aware of any attempt to provide an overview of the kinds of ROC analyses that have been most commonly published in radiologic research.” They investigated articles published in the journal “Radiology” between 1997 and 2006, identifying 295 studies [87] . The authors concluded that “ROC analysis is widely used in radiologic research, confirming its fundamental role in assessing diagnostic performance”. For the present review, we wished to focus on MRMC studies specifically, since these are most complex and are often used as the basis for technology licensing. We also wished to broaden our search criteria beyond a single journal. Our systematic review found that the quality of data reporting in MRMC studies using ROC AUC as an outcome measure was frequently incomplete and who would therefore agree with the conclusions of Shiraishi et al. who stated that studies, “were not always adequate to support clear and clinically relevant conclusions” [87] .
Many omissions we identified were those related to general study design and execution, and are well-covered by the STARD initiative [88] as factors that should be reported in studies of diagnostic test accuracy in general. For example, we found that the number of participating research centres was unclear in approximately one-third of studies, that most studies did not describe whether patients were symptomatic or asymptomatic, that criteria applied to case selection were sometimes unclear, and that observer blinding was not mentioned in one-fifth of studies. Regarding statistical methods, STARD states that studies should, “describe methods for calculating or comparing measures of diagnostic accuracy” [88] ; this systematic review aimed to focus on description of methods for MRMC studies using ROC AUC as an outcome measure.
The large majority of studies used less than 10 observers, some did not describe reader experience, and the majority did not mention whether observers were aware of prevalence of abnormality, a factor that may influence diagnostic vigilance. Most studies required readers to detect lesions while a minority asked for characterization, and others were a combination of the two. We believe it is important for readers to understand the precise nature of the interpretative task since this will influence the rating scale used to build the ROC curve. A variety of units of analysis were adopted, with just under half being the patient case. We were surprised that some studies failed to record the number of disease-positive and disease-negative patients in their dataset. Concerning the confidence scales used to construct the ROC curve, only a small minority (12%) of studies stated that readers were trained to use these in advance of scoring. We believe such training is important so that readers can appreciate exactly how the interpretative task relates to the scale; there is evidence that radiologists score in different ways when asked to perform the same scoring task because of differences in how they interpret the task [89] . For example, readers should appreciate how the scale reflects lesion detection and/or characterization, especially if both are required, and how multiple abnormalities per unit of analysis are handled. Encouragement to use the full range of the scale is required for normal rating distributions. Whether readers must use the same scale for patients with and without pathology is also important to know.
Despite their importance for understanding the validity of study results, we found that description of the confidence scores, the ROC curve and its analysis was often incomplete. Strikingly, only three studies described the distribution of confidence scores and none stated whether transformation to a normal distribution was needed. When publically available DBM MRMC software (ref DBM) is used for ROC AUC modeling, this requires assumptions of normality for confidence scores or their transformations when ROC curve fitting methods are used. Where confidence scores are not normally distributed these software methods are not recommended [84] – [86] , [90] . Although Hanley shows that ROC curves can be reasonable under some distributions of non normal data [91] , concerns have been raised particularly in imaging detection studies measuring clinically useful tests with good performance to distinguish well defined abnormalities. In tests with good performance two factors make estimation of ROC AUC unreliable. Firstly readers' scores are by definition often at the ends of the confidence scale so that the confidence score distributions for normal and abnormal cases have very little overlap [82] – [86] . Secondly tests with good performance also have few false positives making ROC AUC estimation highly dependent on confidence scores assigned to possibly fewer than 5% or 10% of cases in the study [86] .
Most studies did not describe the method used for curve fitting. Over 40% of studies presented no ROC curve in the published article. When present, the large majority were smoothed and averaged over all readers. Only four articles presented data points underlying the curve meaning that the degree of any extrapolation could not be assessed despite this being an important factor regarding interpretation of results [92] . While, by definition, all studies used MRMC AUC methods, most reported additional non-AUC outcomes. Approximately one-quarter of studies did not present AUC data for individual readers. Because of this, variability between readers and/or the effect of individual readers on the ultimate statistical analysis could not be assessed.
Interpretation of study results was variable. Notably, when no significant change in AUC was demonstrated, authors stated that the number of cases was either insufficient or that the difference could not be resolved by the study, appearing to claim that their studies were underpowered rather than that the intervention was ineffective when required to improve diagnostic accuracy. Indeed some studies claimed an advantage for a new test in the face of a non-significant increase in AUC, or turned to other outcomes as proof of benefit. Some interpreted no significant difference in AUC as implying equivalence.
Our review does have limitations. Indexing of the statistical methods used to analyse studies is not common so we used a proxy to identify studies; their citation of “key” references related to MRMC ROC methodology. While it is possible we missed some studies, our aim was not to identify all studies using such analyses. Rather, we aimed to gather a representative sample that would provide a generalizable picture of how such studies are reported. It is also possible that by their citation of methodological papers (and on occasion including a ROC researcher as an author), our review was biased towards papers likely to be of higher methodological quality than average. This systematic review was cross-disciplinary and two radiological researchers performed the bulk of the extraction rather than statisticians. This proved challenging since the depth of statistical knowledge required was demanding, especially when details of the analysis was being considered. We anticipated this and piloted extraction on a sample of five papers to determine if the process was feasible, deciding that it was. Advice from experienced statisticians was also available when uncertainty arose.
In summary, via systematic review we found that MRMC studies using ROC AUC as the primary outcome measure often omit important information from both the study design and analysis, and presentation of results is frequently not comprehensive. Authors using MRMC ROC analyses should be encouraged to provide a full description of their methods and results so as to increase interpretability.
- Supporting Information
Extraction sheet used for the systematic review.
Raw data extracted for the systematic review.
S1 PRISMA Checklist
- Funding Statement
This work was supported by the UK National Institute for Health (NIHR) Research under its Programme Grants for Applied Research funding scheme (RP-PG-0407-10338). The funder had no role in the design, execution, analysis, reporting, or decision to submit for publication.
- Data Availability
Full text links
Read article at publisher's site: https://doi.org/10.1371/journal.pone.0116018
Citations & impact
Impact metrics, citations of article over time, alternative metrics.

Article citations
Artificial intelligence applications in cardiovascular magnetic resonance imaging: are we on the path to avoiding the administration of contrast media.
Cau R , Pisu F , Suri JS , Mannelli L , Scaglione M , Masala S , Saba L
Diagnostics (Basel) , 13(12):2061, 14 Jun 2023
Cited by: 2 articles | PMID: 37370956 | PMCID: PMC10297403
Diagnostic performance of deep learning-based vessel extraction and stenosis detection on coronary computed tomography angiography for coronary artery disease: a multi-reader multi-case study.
Yang W , Chen C , Yang Y , Chen L , Yang C , Gong L , Wang J , Shi F , Wu D , Yan F
Radiol Med , 128(3):307-315, 17 Feb 2023
Cited by: 4 articles | PMID: 36800112
Deep Learning System Boosts Radiologist Detection of Intracranial Hemorrhage.
Warman R , Warman A , Warman P , Degnan A , Blickman J , Chowdhary V , Dash D , Sangal R , Vadhan J , Bueso T , Windisch T , Neves G
Cureus , 14(10):e30264, 13 Oct 2022
Cited by: 1 article | PMID: 36381767 | PMCID: PMC9653089
Assessing Detection Accuracy of Computerized Sonographic Features and Computer-Assisted Reading Performance in Differentiating Thyroid Cancers.
Tai HC , Chen KY , Wu MH , Chang KJ , Chen CN , Chen A
Biomedicines , 10(7):1513, 26 Jun 2022
Cited by: 0 articles | PMID: 35884818 | PMCID: PMC9313277
A review of explainable and interpretable AI with applications in COVID-19 imaging.
Fuhrman JD , Gorre N , Hu Q , Li H , El Naqa I , Giger ML
Med Phys , 49(1):1-14, 07 Dec 2021
Cited by: 23 articles | PMID: 34796530 | PMCID: PMC8646613
Data behind the article
This data has been text mined from the article, or deposited into data resources.
BioStudies: supplemental material and supporting data
- http://www.ebi.ac.uk/biostudies/studies/S-EPMC4277459?xr=true
Similar Articles
To arrive at the top five similar articles we use a word-weighted algorithm to compare words from the Title and Abstract of each citation.
Folic acid supplementation and malaria susceptibility and severity among people taking antifolate antimalarial drugs in endemic areas.
Crider K , Williams J , Qi YP , Gutman J , Yeung L , Mai C , Finkelstain J , Mehta S , Pons-Duran C , Menéndez C , Moraleda C , Rogers L , Daniels K , Green P
Cochrane Database Syst Rev , 2(2022), 01 Feb 2022
Cited by: 6 articles | PMID: 36321557 | PMCID: PMC8805585
Review Free full text in Europe PMC
Disadvantages of using the area under the receiver operating characteristic curve to assess imaging tests: a discussion and proposal for an alternative approach.
Halligan S , Altman DG , Mallett S
Eur Radiol , 25(4):932-939, 20 Jan 2015
Cited by: 83 articles | PMID: 25599932 | PMCID: PMC4356897
Exploration of analysis methods for diagnostic imaging tests: problems with ROC AUC and confidence scores in CT colonography.
Mallett S , Halligan S , Collins GS , Altman DG
PLoS One , 9(10):e107633, 29 Oct 2014
Cited by: 4 articles | PMID: 25353643 | PMCID: PMC4212964
Diagnostic test accuracy of nutritional tools used to identify undernutrition in patients with colorectal cancer: a systematic review.
Håkonsen SJ , Pedersen PU , Bath-Hextall F , Kirkpatrick P
JBI Database System Rev Implement Rep , 13(4):141-187, 15 May 2015
Cited by: 18 articles | PMID: 26447079
Accuracy of diagnostic tests read with and without clinical information: a systematic review.
Loy CT , Irwig L
JAMA , 292(13):1602-1609, 01 Oct 2004
Cited by: 110 articles | PMID: 15467063
Funding
Funders who supported this work.
Cancer Research UK (1)
Medical statistics group.
Professor Gary Collins, University of Oxford
Grant ID: 16895
358 publication s
National Institute for Health Research (NIHR) (1)
Imaging diagnosis of colorectal cancer: interventions for efficient and acceptable diagnosis in symptomatic and screening populations..
Professor Steve Halligan, University College London Hospitals NHS Foundation Trust
Grant ID: RP-PG-0407-10338
38 publication s
Europe PMC is part of the ELIXIR infrastructure
- Case Report
- Open access
- Published: 27 May 2024
A complex case study: coexistence of multi-drug-resistant pulmonary tuberculosis, HBV-related liver failure, and disseminated cryptococcal infection in an AIDS patient
- Wei Fu 1 , 2 na1 ,
- Zi Wei Deng 3 na1 ,
- Pei Wang 1 ,
- Zhen Wang Zhu 1 ,
- Zhi Bing Xie 1 ,
- Yong Zhong Li 1 &
- Hong Ying Yu 1
BMC Infectious Diseases volume 24 , Article number: 533 ( 2024 ) Cite this article
1 Altmetric
Metrics details
Hepatitis B virus (HBV) infection can cause liver failure, while individuals with Acquired Immunodeficiency Virus Disease (AIDS) are highly susceptible to various opportunistic infections, which can occur concurrently. The treatment process is further complicated by the potential occurrence of immune reconstitution inflammatory syndrome (IRIS), which presents significant challenges and contributes to elevated mortality rates.
Case presentation
The 50-year-old male with a history of chronic hepatitis B and untreated human immunodeficiency virus (HIV) infection presented to the hospital with a mild cough and expectoration, revealing multi-drug resistant pulmonary tuberculosis (MDR-PTB), which was confirmed by XpertMTB/RIF PCR testing and tuberculosis culture of bronchoalveolar lavage fluid (BALF). The patient was treated with a regimen consisting of linezolid, moxifloxacin, cycloserine, pyrazinamide, and ethambutol for tuberculosis, as well as a combination of bictegravir/tenofovir alafenamide/emtricitabine (BIC/TAF/FTC) for HBV and HIV viral suppression. After three months of treatment, the patient discontinued all medications, leading to hepatitis B virus reactivation and subsequent liver failure. During the subsequent treatment for AIDS, HBV, and drug-resistant tuberculosis, the patient developed disseminated cryptococcal disease. The patient’s condition worsened during treatment with liposomal amphotericin B and fluconazole, which was ultimately attributed to IRIS. Fortunately, the patient achieved successful recovery after appropriate management.
Enhancing medical compliance is crucial for AIDS patients, particularly those co-infected with HBV, to prevent HBV reactivation and subsequent liver failure. Furthermore, conducting a comprehensive assessment of potential infections in patients before resuming antiviral therapy is essential to prevent the occurrence of IRIS. Early intervention plays a pivotal role in improving survival rates.
Peer Review reports
HIV infection remains a significant global public health concern, with a cumulative death toll of 40 million individuals [ 1 ]. In 2021 alone, there were 650,000 deaths worldwide attributed to AIDS-related causes. As of the end of 2021, approximately 38 million individuals were living with HIV, and there were 1.5 million new HIV infections reported annually on a global scale [ 2 ]. Co-infection with HBV and HIV is prevalent due to their similar transmission routes, affecting around 8% of HIV-infected individuals worldwide who also have chronic HBV infection [ 3 ]. Compared to those with HBV infection alone, individuals co-infected with HIV/HBV exhibit higher HBV DNA levels and a greater risk of reactivation [ 4 ]. Opportunistic infections, such as Pneumocystis jirovecii pneumonia, Toxoplasma encephalitis, cytomegalovirus retinitis, cryptococcal meningitis (CM), tuberculosis, disseminated Mycobacterium avium complex disease, pneumococcal pneumonia, Kaposi’s sarcoma, and central nervous system lymphoma, are commonly observed due to HIV-induced immunodeficiency [ 5 ]. Tuberculosis not only contributes to the overall mortality rate in HIV-infected individuals but also leads to a rise in the number of drug-resistant tuberculosis cases and transmission of drug-resistant strains. Disseminated cryptococcal infection is a severe opportunistic infection in AIDS patients [ 6 ], and compared to other opportunistic infections, there is a higher incidence of IRIS in patients with cryptococcal infection following antiviral and antifungal therapy [ 7 ]. This article presents a rare case of an HIV/HBV co-infected patient who presented with MDR-PTB and discontinued all medications during the initial treatment for HIV, HBV, and tuberculosis. During the subsequent re-anti-HBV/HIV treatment, the patient experienced two episodes of IRIS associated with cryptococcal infection. One episode was classified as “unmasking” IRIS, where previously subclinical cryptococcal infection became apparent with immune improvement. The other episode was categorized as “paradoxical” IRIS, characterized by the worsening of pre-existing cryptococcal infection despite immune restoration [ 8 ]. Fortunately, both episodes were effectively treated.
A 50-year-old male patient, who is self-employed, presented to our hospital in January 2022 with a chief complaint of a persistent cough for the past 2 months, without significant shortness of breath, palpitations, or fever. His medical history revealed a previous hepatitis B infection, which resulted in hepatic failure 10 years ago. Additionally, he was diagnosed with HIV infection. However, he ceased taking antiviral treatment with the medications provided free of charge by the Chinese government for a period of three years. During this hospital visit, his CD4 + T-cell count was found to be 26/μL (normal range: 500–1612/μL), HIV-1 RNA was 1.1 × 10 5 copies/ml, and HBV-DNA was negative. Chest computed tomography (CT) scan revealed nodular and patchy lung lesions (Fig. 1 ). The BALF shows positive acid-fast staining. Further assessment of the BALF using XpertMTB/RIF PCR revealed resistance to rifampicin, and the tuberculosis drug susceptibility test of the BALF (liquid culture, medium MGIT 960) indicated resistance to rifampicin, isoniazid, and streptomycin. Considering the World Health Organization (WHO) guidelines for drug-resistant tuberculosis, the patient’s drug susceptibility results, and the co-infection of HIV and HBV, an individualized treatment plan was tailored for him. The treatment plan included BIC/TAF/FTC (50 mg/25 mg/200 mg per day) for HBV and HIV antiviral therapy, as well as linezolid (0.6 g/day), cycloserine (0.5 g/day), moxifloxacin (0.4 g/day), pyrazinamide (1.5 g/day), and ethambutol (0.75 g/day) for anti-tuberculosis treatment, along with supportive care.
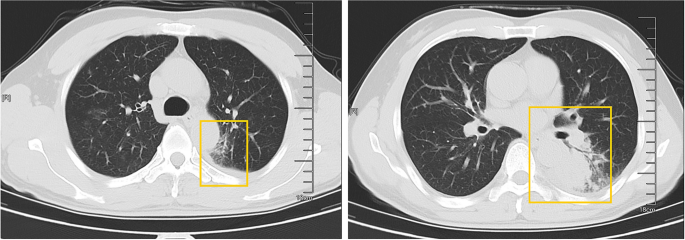
The patient’s pulmonary CT scan shows patchy and nodular lesions accompanied by a small amount of pleural effusion, later confirmed to be MDR-PTB
Unfortunately, after 3 months of follow-up, the patient discontinued all medications due to inaccessibility of the drugs. He returned to our hospital (Nov 12, 2022, day 0) after discontinuing medication for six months, with a complaint of poor appetite for the past 10 days. Elevated liver enzymes were observed, with an alanine aminotransferase level of 295 IU/L (normal range: 0–40 IU/L) and a total bilirubin(TBIL) level of 1.8 mg/dL (normal range: 0–1 mg/dL). His HBV viral load increased to 5.5 × 10 9 copies/ml. Considering the liver impairment, elevated HBV-DNA and the incomplete anti-tuberculosis treatment regimen (Fig. 2 A), we discontinued pyrazinamide and initiated treatment with linezolid, cycloserine, levofloxacin, and ethambutol for anti-tuberculosis therapy, along with BIC/TAF/FTC for HIV and HBV antiviral treatment. Additionally, enhanced liver protection and supportive management were provided, involving hepatoprotective effects of medications such as glutathione, magnesium isoglycyrrhizinate, and bicyclol. However, the patient’s TBIL levels continued to rise progressively, reaching 4.4 mg/dL on day 10 (Fig. 3 B). Suspecting drug-related factors, we discontinued all anti-tuberculosis medications while maintaining BIC/TAF/FTC for antiviral therapy, the patient’s TBIL levels continued to rise persistently. We ruled out other viral hepatitis and found no significant evidence of obstructive lesions on magnetic resonance cholangiopancreatography. Starting from the day 19, due to the patient’s elevated TBIL levels of 12.5 mg/dL, a decrease in prothrombin activity (PTA) to 52% (Fig. 3 D), and the emergence of evident symptoms such as abdominal distension and poor appetite, we initiated aggressive treatment methods. Unfortunately, on day 38, his hemoglobin level dropped to 65 g/L (normal range: 120–170 g/L, Fig. 3 A), and his platelet count decreased to 23 × 10 9 /L (normal range: 125–300 × 10 9 /L, Fig. 3 C). Based on a score of 7 on the Naranjo Scale, it was highly suspected that “Linezolid” was the cause of these hematological abnormalities. Therefore, we had to discontinue Linezolid for the anti-tuberculosis treatment. Subsequently, on day 50, the patient developed recurrent fever, a follow-up chest CT scan revealed enlarged nodules in the lungs (Fig. 2 B). The patient also reported mild dizziness and a worsening cough. On day 61, the previous blood culture results reported the growth of Cryptococcus. A lumbar puncture was performed on the same day, and the cerebrospinal fluid (CSF) opening pressure was measured at 130 mmH 2 O. India ink staining of the CSF showed typical encapsulated yeast cells suggestive of Cryptococcus. Other CSF results indicated mild leukocytosis and mildly elevated protein levels, while chloride and glucose levels were within normal limits. Subsequently, the patient received a fungal treatment regimen consisting of liposomal amphotericin B (3 mg/kg·d −1 ) in combination with fluconazole(600 mg/d). After 5 days of antifungal therapy, the patient’s fever symptoms were well controlled. Despite experiencing bone marrow suppression, including thrombocytopenia and worsening anemia, during this period, proactive symptom management, such as the use of erythropoietin, granulocyte colony-stimulating factor, and thrombopoietin, along with high-calorie dietary management, even reducing the dosage of liposomal amphotericin B to 2 mg/kg/day for 10 days at the peak of severity, successfully controlled the bone marrow suppression. However, within the following week, the patient experienced fever again, accompanied by a worsened cough, increased sputum production, and dyspnea. Nevertheless, the bilirubin levels did not show a significant increase. On day 78 the patient’s lung CT revealed patchy infiltrates and an increased amount of pleural effusion (Fig. 2 C). The CD4 + T-cell count was 89/μL (normal range: 500–700/μL), indicating a significant improvement in immune function compared to the previous stage, and C-reactive protein was significantly elevated, reflecting the inflammatory state, other inflammatory markers such as IL-6 and γ-IFN were also significantly elevated. On day 84, Considering the possibility of IRIS, the patient began taking methylprednisolone 30 mg once a day as part of an effort to control his excessive inflammation. Following the administration of methylprednisolone, the man experienced an immediate improvement in his fever. Additionally, symptoms such as cough, sputum production, dyspnea, and poor appetite gradually subsided over time. A follow-up lung CT showed significant improvement, indicating a positive response to the treatment. After 28 days of treatment with liposomal amphotericin B in combination with fluconazole, liposomal amphotericin B was discontinued, and the patient continued with fluconazole to consolidate the antifungal therapy for Cryptococcus. Considering the patient’s ongoing immunodeficiency, the dosage of methylprednisolone was gradually reduced by 4 mg every week. After improvement in liver function, the patient’s anti-tuberculosis treatment regimen was adjusted to include bedaquiline, contezolid, cycloserine, moxifloxacin, and ethambutol. The patient’s condition was well controlled, and a follow-up lung CT on day 117 indicated a significant improvement in lung lesions (Fig. 2 D).
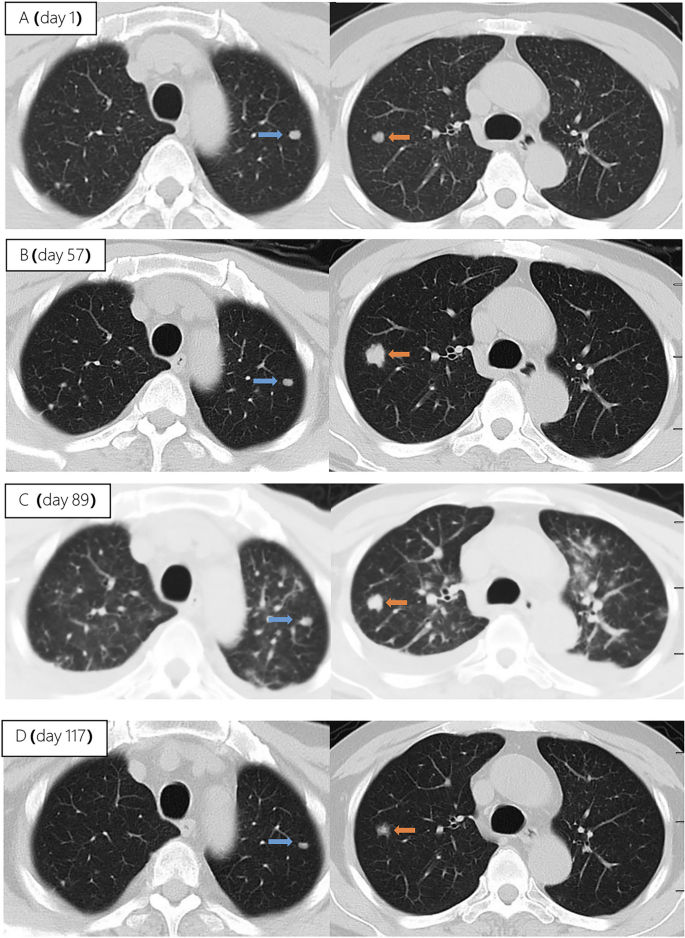
Upon second hospitalization admission ( A ), nodular lesions were already present in the lungs, and their size gradually increased after the initiation of ART ( B , C ). Notably, the lung lesions became more pronounced following the commencement of anti-cryptococcal therapy, coinciding with the occurrence of pleural effusion ( C ). However, with the continuation of antifungal treatment and the addition of glucocorticoids, there was a significant absorption and reduction of both the pleural effusion and pulmonary nodules ( D )
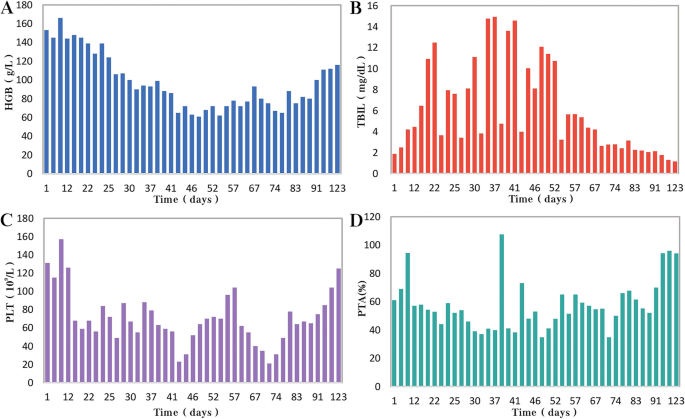
During the patient's second hospitalization, as the anti-tuberculosis treatment progressed and liver failure developed, the patient’s HGB levels gradually decreased ( A ), while TBIL levels increased ( B ). Additionally, there was a gradual decrease in PLT count ( C ) and a reduction in prothrombin activity (PTA) ( D ), indicating impaired clotting function. Moreover, myelosuppression was observed during the anti-cryptococcal treatment ( C )
People living with HIV/AIDS are susceptible to various opportunistic infections, which pose the greatest threat to their survival [ 5 ]. Pulmonary tuberculosis and disseminated cryptococcosis remain opportunistic infections with high mortality rates among AIDS patients [ 9 , 10 ]. These infections occurring on the basis of liver failure not only increase diagnostic difficulty but also present challenges in treatment. Furthermore, as the patient’s immune function and liver function recover, the occurrence of IRIS seems inevitable.
HIV and HBV co-infected patients are at a higher risk of HBV reactivation following the discontinuation of antiviral drugs
In this case, the patient presented with both HIV and HBV infections. Although the HBV DNA test was negative upon admission. However, due to the patient’s self-discontinuation of antiretroviral therapy (ART), HBV virologic and immunologic reactivation occurred six months later, leading to a rapid increase in viral load and subsequent hepatic failure. Charles Hannoun et al. also reported similar cases in 2001, where two HIV-infected patients with positive HBsAg experienced HBV reactivation and a rapid increase in HBV DNA levels after discontinuing antiretroviral and antiviral therapy, ultimately resulting in severe liver failure [ 11 ]. The European AIDS Clinical Society (EACS) also emphasize that abrupt discontinuation of antiviral therapy in patients co-infected with HBV and HIV can trigger HBV reactivation, which, although rare, can potentially result in liver failure [ 12 ].
Diagnosing disseminated Cryptococcus becomes more challenging in AIDS patients with liver failure, and the selection of antifungal medications is significantly restricted
In HIV-infected individuals, cryptococcal disease typically manifests as subacute meningitis or meningoencephalitis, often accompanied by fever, headache, and neck stiffness. The onset of symptoms usually occurs approximately two weeks after infection, with typical signs and symptoms including meningeal signs such as neck stiffness and photophobia. Some patients may also experience encephalopathy symptoms like somnolence, mental changes, personality changes, and memory loss, which are often associated with increased intracranial pressure (ICP) [ 13 ]. The presentation of cryptococcal disease in this patient was atypical, as there were no prominent symptoms such as high fever or rigors, nor were there any signs of increased ICP such as somnolence, headache, or vomiting. The presence of pre-existing pulmonary tuberculosis further complicated the early diagnosis, potentially leading to the clinical oversight of recognizing the presence of cryptococcus. In addition to the diagnostic challenges, treating a patient with underlying liver disease, multidrug-resistant tuberculosis, and concurrent cryptococcal infection poses significant challenges. It requires considering both the hepatotoxicity of antifungal agents and potential drug interactions. EACS and global guideline for the diagnosis and management of cryptococcosis suggest that liposomal amphotericin B (3 mg/kg·d −1 ) in combination with flucytosine (100 mg/kg·d −1 ) or fluconazole (800 mg/d) is the preferred induction therapy for CM for 14 days [ 12 , 14 ]. Flucytosine has hepatotoxicity and myelosuppressive effects, and it is contraindicated in patients with severe liver dysfunction. The antiviral drug bictegravir is a substrate for hepatic metabolism by CYP3A and UGT1A1 enzymes [ 15 ], while fluconazole inhibits hepatic enzymes CYP3A4 and CYP2C9 [ 16 ]. Due to the patient's liver failure and bone marrow suppression, we reduced the dosage of liposomal amphotericin B and fluconazole during the induction period. Considering the hepatotoxicity of fluconazole and its interaction with bictegravir, we decreased the dosage of fluconazole to 600 mg/d, while extending the duration of induction therapy to 28 days.
During re-antiviral treatment, maintaining vigilance for the development of IRIS remains crucial
IRIS refers to a series of inflammatory diseases that occur in HIV-infected individuals after initiating ART. It is associated with the paradoxical worsening of pre-existing infections, which may have been previously diagnosed and treated or may have been subclinical but become apparent due to the host regaining the ability to mount an inflammatory response. Currently, there is no universally accepted definition of IRIS. However, the following conditions are generally considered necessary for diagnosing IRIS: worsening of a diagnosed or previously unrecognized pre-existing infection with immune improvement (referred to as “paradoxical” IRIS) or the unmasking of a previously subclinical infection (referred to as “unmasking” IRIS) [ 8 ]. It is estimated that 10% to 30% of HIV-infected individuals with CM will develop IRIS after initiating or restarting effective ART [ 7 , 17 ]. In the guidelines of the WHO and EACS, it is recommended to delay the initiation of antiviral treatment for patients with CM for a minimum of 4 weeks to reduce the incidence of IRIS. Since we accurately identified the presence of multidrug-resistant pulmonary tuberculosis in the patient during the early stage, we promptly initiated antiretroviral and anti-hepatitis B virus treatment during the second hospitalization. However, subsequent treatment revealed that the patient experienced at least two episodes of IRIS. The first episode was classified as “unmasking” IRIS, as supported by the enlargement of pulmonary nodules observed on the chest CT scan following the initiation of ART (Fig. 2 A). Considering the morphological changes of the nodules on the chest CT before antifungal therapy, the subsequent emergence of disseminated cryptococcal infection, and the subsequent reduction in the size of the lung nodules after antifungal treatment, although there is no definitive microbiological evidence, we believe that the initial enlargement of the lung nodules was caused by cryptococcal pneumonia. As ART treatment progressed, the patient experienced disseminated cryptococcosis involving the blood and central nervous system, representing the first episode. Following the initiation of antifungal therapy for cryptococcosis, the patient encountered a second episode characterized by fever and worsening pulmonary lesions. Given the upward trend in CD4 + T-cell count, we attributed this to the second episode of IRIS, the “paradoxical” type. The patient exhibited a prompt response to low-dose corticosteroids, further supporting our hypothesis. Additionally, the occurrence of cryptococcal IRIS in the lungs, rather than the central nervous system, is relatively uncommon among HIV patients [ 17 ].
Conclusions
From the initial case of AIDS combined with chronic hepatitis B, through the diagnosis and treatment of multidrug-resistant tuberculosis, the development of liver failure and disseminated cryptococcosis, and ultimately the concurrent occurrence of IRIS, the entire process was tortuous but ultimately resulted in a good outcome (Fig. 4 ). Treatment challenges arose due to drug interactions, myelosuppression, and the need to manage both infectious and inflammatory conditions. Despite these hurdles, a tailored treatment regimen involving antifungal and antiretroviral therapies, along with corticosteroids, led to significant clinical improvement. While CM is relatively common among immunocompromised individuals, especially those with acquired immunodeficiency syndrome (AIDS) [ 13 ], reports of disseminated cryptococcal infection on the background of AIDS complicated with liver failure are extremely rare, with a very high mortality rate.
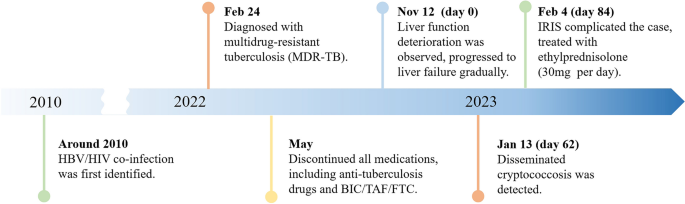
A brief timeline of the patient's medical condition progression and evolution
Through managing this patient, we have also gained valuable insights. (1) Swift and accurate diagnosis, along with timely and effective treatment, can improve prognosis, reduce mortality, and lower disability rates. Whether it's the discovery and early intervention of liver failure, the identification and treatment of disseminated cryptococcosis, or the detection and management of IRIS, all these interventions are crucially timely. They are essential for the successful treatment of such complex and critically ill patients.
(2) Patients who exhibit significant drug reactions, reducing the dosage of relevant medications and prolonging the treatment duration can improve treatment success rates with fewer side effects. In this case, the dosages of liposomal amphotericin B and fluconazole are lower than the recommended dosages by the World Health Organization and EACS guidelines. Fortunately, after 28 days of induction therapy, repeat CSF cultures showed negative results for Cryptococcus, and the improvement of related symptoms also indicates that the patient has achieved satisfactory treatment outcomes. (3) When cryptococcal infection in the bloodstream or lungs is detected, prompt lumbar puncture should be performed to screen for central nervous system cryptococcal infection. Despite the absence of neurological symptoms, the presence of Cryptococcus neoformans in the cerebrospinal fluid detected through lumbar puncture suggests the possibility of subclinical or latent CM, especially in late-stage HIV-infected patients.
We also encountered several challenges and identified certain issues that deserve attention. Limitations: (1) The withdrawal of antiviral drugs is a critical factor in the occurrence and progression of subsequent diseases in patients. Improved medical education is needed to raise awareness and prevent catastrophic consequences. (2) Prior to re-initiating antiviral therapy, a thorough evaluation of possible infections in the patient is necessary. Caution should be exercised, particularly in the case of diseases prone to IRIS, such as cryptococcal infection. (3) There is limited evidence on the use of reduced fluconazole dosage (600 mg daily) during antifungal therapy, and the potential interactions between daily fluconazole (600 mg) and the antiviral drug bictegravir and other tuberculosis medications have not been extensively studied. (4) Further observation is needed to assess the impact of early-stage limitations in the selection of anti-tuberculosis drugs on the treatment outcome of tuberculosis in this patient, considering the presence of liver failure.
In conclusion, managing opportunistic infections in HIV patients remains a complex and challenging task, particularly when multiple opportunistic infections are compounded by underlying liver failure. Further research efforts are needed in this area.
Availability of data and materials
All data generated or analyzed during this study are included in this published article.
Abbreviations
Hepatitis B virus
Acquired immunodeficiency virus disease
Immune reconstitution inflammatory syndrome
Human immunodeficiency virus
Multi-drug resistant pulmonary tuberculosis
Bronchoalveolar lavage fluid
Bictegravir/tenofovir alafenamide/emtricitabine
Cryptococcal meningitis
World Health Organization
Computed tomography
Total bilirubin
Cerebrospinal fluid
European AIDS Clinical Society
Intracranial pressure
Antiretroviral therapy
Prothrombin activity
Bekker L-G, Beyrer C, Mgodi N, Lewin SR, Delany-Moretlwe S, Taiwo B, et al. HIV infection. Nat Rev Dis Primer. 2023;9:1–21.
Google Scholar
Data on the size of the HIV epidemic. https://www.who.int/data/gho/data/themes/topics/topic-details/GHO/data-on-the-size-of-the-hiv-aids-epidemic?lang=en . Accessed 3 May 2023.
Leumi S, Bigna JJ, Amougou MA, Ngouo A, Nyaga UF, Noubiap JJ. Global burden of hepatitis B infection in people living with human immunodeficiency virus: a systematic review and meta-analysis. Clin Infect Dis Off Publ Infect Dis Soc Am. 2020;71:2799–806.
Article Google Scholar
McGovern BH. The epidemiology, natural history and prevention of hepatitis B: implications of HIV coinfection. Antivir Ther. 2007;12(Suppl 3):H3-13.
Article CAS PubMed Google Scholar
Kaplan JE, Masur H, Holmes KK, Wilfert CM, Sperling R, Baker SA, et al. USPHS/IDSA guidelines for the prevention of opportunistic infections in persons infected with human immunodeficiency virus: an overview. USPHS/IDSA Prevention of Opportunistic Infections Working Group. Clin Infect Dis Off Publ Infect Dis Soc Am. 1995;21 Suppl 1:S12-31.
Article CAS Google Scholar
Bamba S, Lortholary O, Sawadogo A, Millogo A, Guiguemdé RT, Bretagne S. Decreasing incidence of cryptococcal meningitis in West Africa in the era of highly active antiretroviral therapy. AIDS Lond Engl. 2012;26:1039–41.
Müller M, Wandel S, Colebunders R, Attia S, Furrer H, Egger M, et al. Immune reconstitution inflammatory syndrome in patients starting antiretroviral therapy for HIV infection: a systematic review and meta-analysis. Lancet Infect Dis. 2010;10:251–61.
Article PubMed PubMed Central Google Scholar
Haddow LJ, Easterbrook PJ, Mosam A, Khanyile NG, Parboosing R, Moodley P, et al. Defining immune reconstitution inflammatory syndrome: evaluation of expert opinion versus 2 case definitions in a South African cohort. Clin Infect Dis Off Publ Infect Dis Soc Am. 2009;49:1424–32.
Obeagu E, Onuoha E. Tuberculosis among HIV patients: a review of Prevalence and Associated Factors. Int J Adv Res Biol Sci. 2023;10:128–34.
Rajasingham R, Govender NP, Jordan A, Loyse A, Shroufi A, Denning DW, et al. The global burden of HIV-associated cryptococcal infection in adults in 2020: a modelling analysis. Lancet Infect Dis. 2022;22:1748–55.
Manegold C, Hannoun C, Wywiol A, Dietrich M, Polywka S, Chiwakata CB, et al. Reactivation of hepatitis B virus replication accompanied by acute hepatitis in patients receiving highly active antiretroviral therapy. Clin Infect Dis Off Publ Infect Dis Soc Am. 2001;32:144–8.
EACS Guidelines | EACSociety. https://www.eacsociety.org/guidelines/eacs-guidelines/ . Accessed 7 May 2023.
Cryptococcosis | NIH. 2021. https://clinicalinfo.hiv.gov/en/guidelines/hiv-clinical-guidelines-adult-and-adolescent-opportunistic-infections/cryptococcosis . Accessed 6 May 2023.
Chang CC, Harrison TS, Bicanic TA, Chayakulkeeree M, Sorrell TC, Warris A, et al. Global guideline for the diagnosis and management of cryptococcosis: an initiative of the ECMM and ISHAM in cooperation with the ASM. Lancet Infect Dis. 2024;10:S1473-3099(23)00731-4.
Deeks ED. Bictegravir/emtricitabine/tenofovir alafenamide: a review in HIV-1 infection. Drugs. 2018;78:1817–28.
Article CAS PubMed PubMed Central Google Scholar
Bellmann R, Smuszkiewicz P. Pharmacokinetics of antifungal drugs: practical implications for optimized treatment of patients. Infection. 2017;45:737–79.
Shelburne SA, Darcourt J, White AC, Greenberg SB, Hamill RJ, Atmar RL, et al. The role of immune reconstitution inflammatory syndrome in AIDS-related Cryptococcus neoformans disease in the era of highly active antiretroviral therapy. Clin Infect Dis Off Publ Infect Dis Soc Am. 2005;40:1049–52.
Download references
Acknowledgements
We express our sincere gratitude for the unwavering trust bestowed upon our medical team by the patient throughout the entire treatment process.
This work was supported by the Scientific Research Project of Hunan Public Health Alliance with the approval No. ky2022-002.
Author information
Wei Fu and Zi Wei Deng contributed equally to this work.
Authors and Affiliations
Center for Infectious Diseases, Hunan University of Medicine General Hospital, Huaihua, Hunan, China
Wei Fu, Pei Wang, Zhen Wang Zhu, Ye Pu, Zhi Bing Xie, Yong Zhong Li & Hong Ying Yu
Department of Tuberculosis, The First Affiliated Hospital of Xinxiang Medical University, XinXiang, Henan, China
Department of Clinical Pharmacy, Hunan University of Medicine General Hospital, Huaihua, Hunan, China
Zi Wei Deng
You can also search for this author in PubMed Google Scholar
Contributions
WF and ZWD integrated the data and wrote the manuscript, YHY contributed the revision of the manuscript, PW and YP provided necessary assistance and provided key suggestions, ZWZ, YZL and ZBX contributed data acquisition and interpretation for etiological diagnosis. All authors reviewed and approved the final manuscript.
Corresponding author
Correspondence to Hong Ying Yu .
Ethics declarations
Ethics approval and consent to participate.
The study was approved by the Ethics Committee of the Hunan University of Medicine General Hospital (HYZY-EC-202306-C1), and with the informed consent of the patient.
Consent for publication
Written informed consent was obtained from the patient for the publication of this case report and any accompanying images.
Competing interests
The authors declare no competing interests.
Additional information
Publisher’s note.
Springer Nature remains neutral with regard to jurisdictional claims in published maps and institutional affiliations.
Rights and permissions
Open Access This article is licensed under a Creative Commons Attribution 4.0 International License, which permits use, sharing, adaptation, distribution and reproduction in any medium or format, as long as you give appropriate credit to the original author(s) and the source, provide a link to the Creative Commons licence, and indicate if changes were made. The images or other third party material in this article are included in the article's Creative Commons licence, unless indicated otherwise in a credit line to the material. If material is not included in the article's Creative Commons licence and your intended use is not permitted by statutory regulation or exceeds the permitted use, you will need to obtain permission directly from the copyright holder. To view a copy of this licence, visit http://creativecommons.org/licenses/by/4.0/ . The Creative Commons Public Domain Dedication waiver ( http://creativecommons.org/publicdomain/zero/1.0/ ) applies to the data made available in this article, unless otherwise stated in a credit line to the data.
Reprints and permissions
About this article
Cite this article.
Fu, W., Deng, Z.W., Wang, P. et al. A complex case study: coexistence of multi-drug-resistant pulmonary tuberculosis, HBV-related liver failure, and disseminated cryptococcal infection in an AIDS patient. BMC Infect Dis 24 , 533 (2024). https://doi.org/10.1186/s12879-024-09431-9
Download citation
Received : 30 June 2023
Accepted : 24 May 2024
Published : 27 May 2024
DOI : https://doi.org/10.1186/s12879-024-09431-9
Share this article
Anyone you share the following link with will be able to read this content:
Sorry, a shareable link is not currently available for this article.
Provided by the Springer Nature SharedIt content-sharing initiative
- Liver failure
- Disseminated cryptococcal disease
BMC Infectious Diseases
ISSN: 1471-2334
- General enquiries: [email protected]
Multi-Reader Multi-Case Study for Performance Evaluation of High-Risk Thyroid Ultrasound with Computer-Aided Detection
Affiliations.
- 1 Department of Surgery, National Taiwan University Hospital, Taipei 10002, Taiwan.
- 2 Department of Internal Medicine, National Taiwan University, Taipei 10002, Taiwan.
- 3 Graduate Institute of Industrial Engineering, National Taiwan University, Taipei 10617, Taiwan.
- PMID: 32041119
- PMCID: PMC7072687
- DOI: 10.3390/cancers12020373
Physicians use sonographic characteristics as a reference for the possible diagnosis of thyroid cancers. The purpose of this study was to investigate whether physicians were more effective in their tentative diagnosis based on the information provided by a computer-aided detection (CAD) system. A computer compared software-defined and physician-adjusted tumor loci. A multicenter, multireader, and multicase (MRMC) study was designed to compare clinician performance without and with the use of CAD. Interobserver variability was also analyzed. Excellent, satisfactory, and poor segmentations were observed in 25.3%, 58.9%, and 15.8% of nodules, respectively. There were 200 patients with 265 nodules in the study set. Nineteen physicians scored the malignancy potential of the nodules. The average area under the curve (AUC) of all readers was 0.728 without CAD and significantly increased to 0.792 with CAD. The average standard deviation of the malignant potential score significantly decreased from 18.97 to 16.29. The mean malignant potential score significantly decreased from 35.01 to 31.24 for benign cases. With the CAD system, an additional 7.6% of malignant nodules would be suggested for further evaluation, and biopsy would not be recommended for an additional 10.8% of benign nodules. The results demonstrated that applying a CAD system would improve clinicians' interpretations and lessen the variability in diagnosis. However, more studies are needed to explore the use of the CAD system in an actual ultrasound diagnostic situation where much more benign thyroid nodules would be seen.
Keywords: computer-aided detection; thyroid cancer; thyroid nodule; ultrasonography.
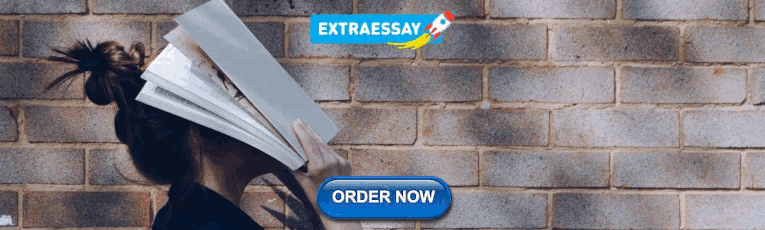
IMAGES
VIDEO
COMMENTS
Introduction We examined the design, analysis and reporting in multi-reader multi-case (MRMC) research studies using the area under the receiver-operating curve (ROC AUC) as a measure of diagnostic performance. Methods We performed a systematic literature review from 2005 to 2013 inclusive to identify a minimum 50 studies. Articles of diagnostic test accuracy in humans were identified via ...
Technical Description. The primary objective of the iMRMC statistical software is to assist investigators with analyzing and sizing multi-reader multi-case (MRMC) reader studies that compare the ...
Degree of Reader aggressiveness - controlled for by ROC (and related paradigms) - Range of difficulty of patient Cases - Range of Reader skill - Correlation of Patients across Modalities - Correlation of Readers across Modalities - Reader "jitter" - inconsistency w/i a reader, etc. Full analysis requires Multiple-Reader, Multiple-Case
Multi-reader multi-case studies using the area under the receiver operator characteristic curve as a measure of diagnostic accuracy: systematic review with a focus on quality of data reporting. PLoS One 2014;9(12):e116018. Crossref, Medline, Google Scholar; 2. Zhou XH, Obuchowski NA, McClish DL. Statistical Methods in Diagnostic Medicine. 2nd ed.
In this study, the fundamentals of MRMC study design and analysis are reviewed. The goal is to provide investigators with a guide to the fundamentals of MRMC design and analysis, with references to more detailed discus-sions. In addition, readers are updated on newer areas of research, including correction for studies with multiple diagnostic ...
MRMCaov performs multi-reader multi-case analysis of variance for the performance comparison of imaging modalities. This software is the first R implementation of Dr. Hillis unified methodology and builds upon his OR-DBM MRMC SAS software. 8 It is designed to be user friendly, integrate with the R statistical computing and graphics environment ...
Evaluation of medical imaging devices often involves clinical studies where multiple readers (MR) read images of multiple cases (MC) for a clinical task, which are often called MRMC studies. ... Adaptive designs in multi-reader multi-case clinical trials of imaging devices Stat Methods Med Res. 2020 Jun;29(6):1592-1611. doi: 10.1177 ...
Corresponding analyses can be conducted in a multi-reader multi-case (MRMC) f... In the radiology field, whether magnetic resonance imaging (MRI) is better than mammograms is a problem of interest. Hypothesis testing methods for multi-reader multi-case studies: Biostatistics & Epidemiology: Vol 7 , No 1 - Get Access
Abstract. Diagnostic tests are often compared in multi-reader multi-case (MRMC) studies in which a number of cases (subjects with or without the disease in question) are examined by several readers using all tests to be compared. One of the commonly used methods for analyzing MRMC data is the Obuchowski-Rockette (OR) method, which assumes that ...
A multi-reader multi-case study was performed with 240 bilateral DBT exams (71 breasts with cancer lesions, 70 breasts with benign findings, 339 normal breasts). Exams were interpreted by 18 radiologists, with and without AI support, providing cancer suspicion scores per breast. Using AI support, radiologists were shown examination-based and ...
In this multi-reader multi-case (MRMC) study, we examined retrospective data from two clinical trials conducted at five sites. All subjects received FFDM and QT scans within 90 days. Data were analyzed in a reader study with full factorial design involving 22 radiologists and 108 breast cases (42 normal, 39 pathology-confirmed benign, and 27 ...
The first step in conducting a multi-reader, multi-case analysis with the package is an R command-line call to the function mrmc to specify the data inputs, performance metric of interest, and covariance estimation method. Below is a summary of the function arguments. Study design is automatically inferred from the case identifiers.
Radiological tests must be interpreted by human observers and a common study design uses multiple readers to interpret multiple image cases; the multi-reader multi-case (MRMC) design . The MRMC design is popular because once a radiologist has viewed 20 cases there is less information to be gained by asking him to view a further 20 than by ...
Improving Prostate Cancer Detection With MRI: A Multi-Reader, Multi-Case Study Using Computer-Aided Detection (CAD) Author links open overlay panel Mark A. Anderson MD 1 2 #, Sarah Mercaldo PhD 3 #, Ryan Chung MD 1 2, ... Based upon PI-RADS scores, multi-reader multi-case (MRMC) analysis was used to compare the reader performance without and ...
Introduction: We examined the design, analysis and reporting in multi-reader multi-case (MRMC) research studies using the area under the receiver-operating curve (ROC AUC) as a measure of diagnostic performance. Methods: We performed a systematic literature review from 2005 to 2013 inclusive to identify a minimum 50 studies. Articles of diagnostic test accuracy in humans were identified via ...
Do Multi-Reader, Multi-Case (MRMC) analyses of data from imaging studies where clinicians (readers) evaluate patient images (cases). What does this mean? ... Many imaging studies are designed so that every reader reads every case in all modalities, a fully-crossed study. In this case, the data is cross-correlated, and we consider the readers and cases to be cross-correlated random effects. An ...
Diagnostic tests are often compared in multi-reader multi-case (MRMC) studies in which a number of cases (subjects with or without the disease in question) are examined by several readers using all tests to be compared. One of the commonly used methods for analyzing MRMC data is the Obuchowski-Rockette (OR) method, which assumes that the true ...
Multi-reader multi-case studies using the area under the receiver operator characteristic curve as a measure of diagnostic accuracy: systematic review with a focus on quality of data reporting. Sign in | Create an account. https://orcid.org. Europe PMC ...
A common study design for comparing the diagnostic performance of imaging modalities is to obtain modality-specific ratings from multiple readers of multiple cases whose true statuses are known. Typically, there is overlap between the modalities, readers, and/or cases for which special analytical me … Multi-reader multi-case analysis of ...
Physicians use sonographic characteristics as a reference for the possible diagnosis of thyroid cancers. The purpose of this study was to investigate whether physicians were more effective in their tentative diagnosis based on the information provided by a computer-aided detection (CAD) system. A computer compared software-defined and physician-adjusted tumor loci. A multicenter, multireader ...
Sea level monitoring is an essential foundational project for studying global climate change and the rise in sea levels. Satellite radar altimeters, which can sometimes provide inaccurate sea surface height data near the coast, are affected by both the instrument itself and geophysical factors. Buoys equipped with GNSS receivers offer a relatively flexible deployment at sea, allowing for long ...
The study was approved by the Ethics Committee of the Hunan University of Medicine General Hospital (HYZY-EC-202306-C1), and with the informed consent of the patient. Consent for publication. Written informed consent was obtained from the patient for the publication of this case report and any accompanying images. Competing interests
This study proposes the Periodic Transformer Encoder (PTE) as a solution for estimating future travel times from past traffic data along specific road segments. The proposed architecture is illustrated in Figure 1. Fundamental to our approach is the construction of a transformation function for each road segment r.
Methods: A multi-reader multi-case study was performed with 240 bilateral DBT exams (71 breasts with cancer lesions, 70 breasts with benign findings, 339 normal breasts). Exams were interpreted by 18 radiologists, with and without AI support, providing cancer suspicion scores per breast. Using AI support, radiologists were shown examination ...
The MDP includes the policies used for dispatch and storage operation, which are represented by linear programming as a part of the simulation model. The effectiveness of our proposed method is demonstrated with a case study, where decisions over multiple decades are considered along with various uncertainties of multi-timescales.
AMA Style. Sudžum R, Nestić S, Komatina N, Kraišnik M. An Intuitionistic Fuzzy Multi-Criteria Approach for Prioritizing Failures That Cause Overproduction: A Case Study in Process Manufacturing.
A multicenter, multireader, and multicase (MRMC) study was designed to compare clinician performance without and with the use of CAD. Interobserver variability was also analyzed. Excellent, satisfactory, and poor segmentations were observed in 25.3%, 58.9%, and 15.8% of nodules, respectively. There were 200 patients with 265 nodules in the ...
and a common study design uses multiple readers to interpret multiple image cases; the multi-reader multi-case (MRMC) design [4]. The MRMC design is popular because once a radiologist has viewed 20 cases there is less information to be gained by asking him to view a further 20 than by asking a different radiologist to view the same 20.