The relationship between junk food consumption, healthy nutrition, and obesity among children aged 7 to 8 years in Mersin, Turkey
Affiliations.
- 1 Department of Community Health Nursing, Faculty of Nursing, Mersin University, Mersin, Turkey. Electronic address: [email protected].
- 2 Department of Community Health Nursing, Faculty of Nursing, Mersin University, Mersin, Turkey. Electronic address: [email protected].
- PMID: 35429797
- DOI: 10.1016/j.nutres.2022.03.004
Increasing junk food (JF) consumption (JFC) among school-aged children is a public health issue that may affect the quality of their diet. The purpose of this study is to identify the factors associated with high-energy intake from JFC in children aged 7 to 8 years with different socioeconomic backgrounds and examine the relationship among JFC, healthy food consumption, and obesity. The hypothesis of the study is that JFC index is related to consumption of staple foods and body mass index. This cross-sectional study was conducted between October 2018 and April 2019 with the participation of 524 children and their parents with low or high socioeconomic status. The data collected with "Healthy Food and JFC Frequency Parents" "Views Form" and "24-Hour Dietary Recall Child Form" were analyzed using multiple analysis methods with P ≤ .05 significance level. Among the children, 53.1% were female, 14.1% were children with obesity, and 18.5% consumed at least one of the JF groups daily. A difference was observed between the calorie intake from JF in children with obesity (292.90 kcal/d) and children without obesity (395.76 kcal/d). The consumption of salty food is associated with low-income levels and consumption of fast food is associated with high-income levels. According to the variables of usually having snacks at home, not receiving pocket money every day, and consuming dairy products at the recommended level, usually having JF at home (odds ratio [OR] = 3.90; 95% confidence interval [CI], 1.40-10.86), receiving pocket money (OR = 2.96; 95% CI, 1.97-4.48), and consuming dairy products at a the recommended level (OR = 1.60; 95% CI, 1.03-2.49) were found to be better predictors of high level of calorie intake from JF (P < .05). Consumption of fruits, dairy products, and protein-rich foods is associated with JFC (P < .05). Regardless of obesity status, a significant portion of the daily calorie needs of children from any socioeconomic level is met with JFC. Those who consume JF frequently and in large amounts also consume a lot of dairy products and protein-rich foods and very little fruit. The long-term effects of JFC on those who consume staple foods at an adequate level should also be investigated.
Keywords: Calorie intake; Healthy nutrition; Junk food consumption; Obesity; School-age children.
Copyright © 2022 Elsevier Inc. All rights reserved.
- Cross-Sectional Studies
- Diet, Healthy*
- Energy Intake*
- Skip to main content
- Keyboard shortcuts for audio player
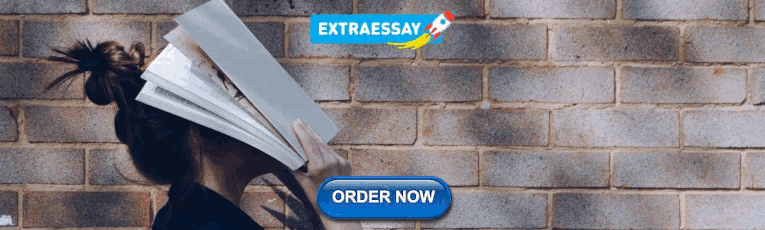
Children's Health
If you think kids are eating mostly junk food, a new study finds you're right.
Xcaret Nuñez

Researchers found that 67% of calories consumed by children and adolescents in the U.S. came from ultra-processed foods in 2018, a jump from 61% in 1999. The nationwide study analyzed the diets of 33,795 children and adolescents. Drazen Stader / EyeEm/Getty Images hide caption
Researchers found that 67% of calories consumed by children and adolescents in the U.S. came from ultra-processed foods in 2018, a jump from 61% in 1999. The nationwide study analyzed the diets of 33,795 children and adolescents.
Kids and teens in the U.S. get the majority of their calories from ultra-processed foods like frozen pizza, microwavable meals, chips and cookies, a new study has found.
Two-thirds — or 67% — of calories consumed by children and adolescents in 2018 came from ultra-processed foods, a jump from 61% in 1999, according to a peer-reviewed study published in the medical journal JAMA . The research, which analyzed the diets of 33,795 youths ages 2 to 19 across the U.S., noted the "overall poorer nutrient profile" of the ultra-processed foods.
"This is particularly worrisome for children and adolescents because they are at a critical life stage to form dietary habits that can persist into adulthood," says Fang Fang Zhang , the study's senior author and a nutrition and cancer epidemiologist at Tufts University's Friedman School of Nutrition Science and policy. "A diet high in ultra-processed foods may negatively influence children's dietary quality and contribute to adverse health outcomes in the long term."
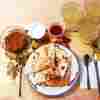
It's Not Just Salt, Sugar, Fat: Study Finds Ultra-Processed Foods Drive Weight Gain
One reason for the increase may be the convenience of ultra-processed foods, Zhang says. Industrial processing, such as changing the physical structure and chemical composition of foods, not only gives them a longer shelf life but also a more appetizing taste.
"Things like sugar, corn syrup, some hemp oil and other ingredients that we usually don't usually use in our kitchen, that are extracted from foods and synthesized in the laboratory, those are being added in the final product of ultra-processed foods," Zhang said. "A purpose of doing this is to make them highly palatable. So kids will like those foods that somehow make it hard to resist."

Shots - Health News
Cheap, legal and everywhere: how food companies get us 'hooked' on junk.
During the same two-decade period when the study data was collected, the consumption of unprocessed or minimally processed foods decreased to 23.5% from 28.8%, the study found.
The greatest increase in calories came from ready-to-eat or ready-to-heat meals such as pizza, sandwiches and hamburgers, rising to 11.2% of calories from 2.2%. Packaged sweet snacks and treats such as cakes and ice cream were a runner-up, which made up 12.9% of calorie consumption in 2018, compared with 10.6% in 1999.
When broken down by race and ethnicity, the growth in consumption of ultra-processed foods was significantly higher for Black, non-Hispanic youth, compared to white, non-Hispanic youths. The study also noted that Mexican American youths consumed ultra-processed foods at a persistently lower rate, which the researchers said may indicate more home cooking by Hispanic families.
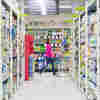
Opinion: Why Ditching Processed Foods Won't Be Easy — Barriers To Cooking From Scratch
The study also found that the education levels of parents or family income didn't affect consumption of ultra-processed foods, suggesting that these types of foods are common in many households.
But the responsibility for tackling this problem shouldn't fall only on parents, Zhang says.
While she would encourage parents and children to consider "replacing ultra-processed foods with minimally and unprocessed foods," Zhang says changes at the policy level are needed "to achieve a broader and more sustainable impact."
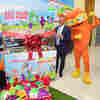
Want Kids To Eat More Veggies? Market Them With Cartoons
Take, for instance, consumption of soda. The consumption of sugar-sweetened beverages dropped to 5.3% from 10.8% of overall calories. The study's researchers noted that the decline could be related to efforts such as soda taxes and raising awareness about the effects sugar has on youth health .
"We may have won this battle, at least partially, for some sugary beverages," Zhang says, "but we haven't yet against ultra-processed foods."
This widespread reliance on junk food is an increasing public health concern as the obesity rate has been rising steadily among U.S. youths for the past two decades.
While the study's authors said that the relationship between childhood obesity and ultra-processed foods is complex, they acknowledge that "cohort studies provide consistent evidence suggesting high intake of ultra-processed foods contributes to obesity in children and young adults."
Indeed, a 2019 study by researchers at the National Institutes of Health found that a diet filled with ultra-processed foods encourages people to overeat and gain weigh t compared to diets that consist of whole or minimally processed foods.
- child nutrition
- food and nutrition
- American diet
Ultra-Processed Food Addiction: A Research Update
- Open access
- Published: 18 May 2024
Cite this article
You have full access to this open access article
- Erica M. LaFata 1 ,
- Kelly C. Allison 2 ,
- Janet Audrain-McGovern 3 &
- Evan M. Forman 1 , 4
893 Accesses
22 Altmetric
Explore all metrics
Purpose of Review
Detail recent advancements in the science on ultra-processed food (UPF) addiction, focusing on estimated prevalence rates and emerging health disparities; progress towards identifying biological underpinnings and behavioral mechanisms; and implications for weight management.
Recent Findings
Notable developments in the field have included: (1) estimating the global prevalence of UPF addiction at 14% of adults and 15% of youths; (2) revealing health disparities for persons of color and those with food insecurity; (3) observing altered functioning across the brain-gut-microbiome axis; (4) providing early evidence for UPF withdrawal; and (5) elucidating poorer weight management outcomes among persons with UPF addiction.
The breadth of recent work on UPF addiction illustrates continued scientific and public interest in the construct and its implications for understanding and treating overeating behaviors and obesity. One pressing gap is the lack of targeted interventions for UPF addiction, which may result in more optimal clinical outcomes for this underserved population.
Similar content being viewed by others
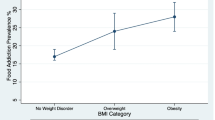
Clinical Considerations of Ultra-processed Food Addiction Across Weight Classes: an Eating Disorder Treatment and Care Perspective
How to Treat Food Addiction from a Nutritional Perspective: Consideration of Diet and Abstinence
Avoidant/Restrictive Food Intake Disorder: Comorbidities and Longitudinal Course
Avoid common mistakes on your manuscript.
Introduction
Over the past 40 years, global rates of overweight and obesity have increased substantially [ 1 ], leading researchers to explore contributing mechanisms. While numerous biological (e.g., genetic, hormonal) and behavioral (e.g., sedentary lifestyle) factors are known to be associated with obesity [ 2 ], one of the key systemic changes in our environment that has paralleled the timeline of rising obesity rates has been the exponential increase of ultra-processed foods (UPFs) in our food system since the 1980s [ 3 , 4 ]. UPFs are not found in nature and have been intentionally created to be hyper-palatable through the addition of added fats, refined carbohydrates (e.g., white flour, sugar), and/or salt [ 5 , 6 ]. Examples of UPFs include pastries, candy, packaged snacks, fast foods, and sugar-sweetened beverages [ 6 ]. Rigorous, epidemiological research has soundly implicated UPFs in not only obesity but also in an array of physical health conditions. As detailed in a recent review [ 7 ], four prospective cohort studies ( n s = 11,898-44,551; mean follow-up = 6.6–19 years) have consistently observed that persons reporting the highest versus lowest quartile of UPF intake at baseline had a 25–62% increased hazard for all-cause mortality, and, relatedly, numerous prospective and cross-sectional studies have linked increased UPF intake with elevated rates and/or complications of diet-related conditions (e.g., cardiovascular disease, type 2 diabetes).
While it has long been possible to create homemade versions of popular UPFs (e.g., homemade chocolate chip cookies), some researchers have noted that the uptick in rates of obesity and diet-related diseases have occurred on a similar timeline as industry-driven changes in the global food system [ 8 ]. One such change was the increased development and distribution of UPFs that followed after Kraft Foods and General Foods were acquired by Phillip Morris – one of the largest tobacco companies worldwide [ 9 •, 10 ]. Released industry documents have revealed that the same tobacco industry leaders admitted to applying the techniques used to enhance the addictive properties of tobacco products to the development of UPFs (e.g., use of additives to enhance flavor, texture, and visual appeal) in order to maximize profits [ 9 •]. As such, the direct role of UPFs in driving the obesity epidemic may be at least partially explained by the tobacco industry’s involvement in designing UPFs to be highly reinforcing, using practices like those used for maximizing the addictive potential of tobacco products.
Notably, preclinical and human studies have demonstrated that repeated intake of UPFs triggers addiction-like biological (e.g., dopaminergic sensitization) and behavioral (e.g., withdrawal, use despite consequences) responses, whereas consumption of naturally occurring foods like fruits, vegetables, and meats have demonstrated limited or no associations with indicators of addiction (for a review of the evidence for the addictive properties of UPFs, see [ 11 ••]). This differentiation has been attributed to UPFs sharing pharmacokinetic properties with addictive substances, namely containing artificially high doses of rewarding ingredients (e.g., added sugars) that are rapidly absorbed by the system [ 12 ]. Strikingly, researchers recently argued that UPFs meet the scientific criteria used to define tobacco products as addictive [ 13 ].
Evidence for the addictive potentials of UPFs has been coupled with empirical investigations of the plausibility of UPF addiction as a novel clinical presentation, which has been most commonly operationalized by adapting the diagnostic criteria for substance-use disorders (SUDs) to the intake of UPFs (for a review, see [ 14 ••]). While individuals can experience problematic eating behaviors with a wide array of foods, the presentation of UPF addiction is specific to exhibiting diagnostic features of SUDs specifically with UPFs (akin to alcohol-use disorder being specific to experiencing diagnostic indicators of SUDs with alcoholic beverages) [ 14 ••]. The focus on UPFs reflects the strong science that UPFs trigger addictive responses in a manner that other foods do not and importantly addresses a main critique of the construct (distinguishing UPF addiction from the homeostatic need to eat) [ 11 ••]. Evaluating the evidence for UPF addiction also requires acknowledgement of the key limitations of the literature to date, such as the lack of research exploring the addictive ingredient/attribute of UPFs (refined carbohydrates vs. added fats vs. both) [ 15 , 16 ] and the limited studies clarifying the shared and distinct features of UPF addiction versus existing eating disorders [ 17 , 18 ]. In addition, UPF addiction is not a recognized clinical diagnosis in the DSM-5, and further investigation on this topic is needed to understand if this presentation may warrant consideration as a novel diagnostic category in the future.
As such, while this this review details recent advancements in the empirical understanding of UPF addiction, future research directions are also highlighted to underscore gaps in the existing literature. Specifically, the authors will describe: (1) global population prevalence estimates of UPF addiction and health disparities; (2) recent research on the biological underpinnings and behavioral features of UPF addiction; (3) implications of UPF addiction for weight management; and (4) key next steps for clinical research and treatment to understand UPF addiction both within the context of obesity and as a distinct clinical presentation.
Current Prevalence Estimates of UPF Addiction and Evidence for Health Disparities
The Yale Food Addiction Scale (YFAS) measures remain the most common empirical approach for operationalizing UPF addiction [ 19 ]. The current version of the YFAS (YFAS 2.0) parallels the Diagnostic and Statistical Manual of Mental Disorders (5th edition; DSM-5) approach for diagnosing SUDs [ 20 ]. Specifically, individuals can meet for UPF addiction by endorsing at least two of the 11 symptoms of SUDs with respect to their intake of UPFs (e.g., use despite negative consequences, persistent unsuccessful attempts to cut down, withdrawal), plus clinically significant impairment (e.g., interference with role obligations) or psychological distress [ 20 ]. Two recent systematic reviews of 281 studies across 36 countries estimated the prevalence of UPF addiction in the general population as 14% in adults [ 21 ]• and 15% in youths [ 22 •]. The rate of UPF addiction among adults is highly similar to the prevalence of SUDs where the addictive substance is legal, such as alcohol-use disorder (14%) and tobacco-use disorder (18%) [ 23 , 24 ]. However, the prevalence of UPF addiction in youths is striking and unprecedented [ 25 ••]. Across the lifespan, individuals with obesity seem to be at higher risk for UPF addiction, with prevalence estimated at 28% of adults and 19% of youths with obesity [ 21 •, 22 •]. While UPF addiction may be more common among persons with obesity, the observed degree of overlap demonstrates that the two conditions are not synonymous and should not be conflated.
Progress towards understanding the demographic correlates of UPF addiction has revealed significant health disparities [ 25 ••]. A nationally representative sample of the United States revealed that the prevalence of UPF addiction was substantially higher among individuals who identified with an underrepresented racial or ethnic group (Black: 16.8%; Hispanic: 32.3%) compared to those who identified as non-Hispanic and White (11.7%) [ 26 ]. Further, UPF addiction was more strongly associated with obesity for individuals who reported lower versus higher household incomes [ 26 ]. In addition, food insecurity has been associated with a 21–56% greater risk of meeting criteria for UPF addiction [ 27 , 28 ] and persons with lower household incomes are more likely to have obesity if they meet criteria for UPF addiction [ 26 ]. These health disparities may be largely driven by two key factors. First, food industry documents have revealed that UPFs are disproportionately marketed to persons of color using exploitative techniques taken from the playbook of Big Tobacco [ 29 ••]. Second, the accessibility and affordability of rewarding, calorie-dense UPFs in our environment makes them, understandably, a highly enticing choice for individuals with limited time and/or financial resources or those experiencing prolonged stress.
Recent Research on the Biological Underpinnings of UPF Addiction: A Focus on Alterations in the Brain-Gut-Microbiome Axis
The foundation of biological evidence for the addictive potentials of UPFs comes from prior neuroimaging studies that have observed similar reward-related neural responses associated with UPFs and addictive substances [ 30 , 31 ]. Further, individuals with elevated symptoms or who meet criteria for UPF addiction exhibit neural responses to UPFs similar to those observed among those with a SUD for their addictive substance of choice (e.g., greater anticipatory reward, reduced consummatory reward, enhanced functional connectivity across reward processing regions) [ 32 , 33 , 34 ]. A 2021 review provides a detailed account of the neurobiological research supporting the plausibility of UPF addiction [ 35 ]. Further, a 2023 review summarizes 16 studies that have observed associations between UPF addiction and blood biomarkers (e.g., glucose, insulin, leptin, ghrelin) and critically raises methodological limitations that require attention in future work (e.g., small sample sizes, variation in comorbidities) [ 36 ]. Thus, the current section will focus on another emerging area of studies exploring the biological underpinnings of UPF addiction—alterations in the brain-gut-microbiome axis implicated in chronic UPF intake and UPF addiction.
Preclinical models can examine the causal consequences of UPF intake on the brain-gut-microbiome axis by introducing UPFs to rats without previous exposure. In rats prone to obesity, repeated intake of UPFs triggers a rapid upregulation in calcium-permeable AMPA receptors in the nucleus accumbens, which is characteristic of addictive substances and associated with increased cue-induced craving and drug-seeking behavior [ 37 , 38 , 39 , 40 ]. This UPF-induced alteration to the reward system notably preceded the onset of obesity [ 37 , 39 , 40 ]. Prolonged consumption of UPFs among obesity-prone rats has also been found to cause reduced excitability of nucleus accumbens core neurons, which is suggestive of altered dopaminergic reward responses and has been similarly demonstrated with chronic cocaine exposure [ 41 , 42 ]. Another study demonstrated that prolonged UPF consumption alters the gut microbiome in a manner that creates an abundance of microbes implicated in obesity and metabolic disease [ 43 ]. Across these studies, addiction-like alterations were not found to occur when obesity-prone rats consumed their nutritionally balanced chow [ 38 , 39 , 40 , 41 , 42 , 43 ].
Collectively, this animal research provides convincing support for the direct and unique role that UPFs have in promoting obesity via their ability to alter the brain-gut-microbiome axis in a manner that increases craving and motivates continued UPF intake. However, unlike studies of rats, in which UPFs can be introduced for the first time and the causal effects on the brain-gut-microbiome axis can be observed, the potential to observe such effects in humans is limited by the high rate of typical UPF intake in the population. As such, preclinical models lay the groundwork for the translational research that can be feasible explored in humans.
Relatedly, human research has identified numerous alterations across the brain-gut-microbiome axis among individuals with UPF addiction. Chronically high levels of UPF intake among persons with UPF addiction has been associated with disrupted dopaminergic signaling (increased hedonic drive for UPFs), dysregulated hunger/satiety hormones (increased hunger, reduced satiety), and alterations to the gut microbiome related to obesity risk [ 44 ]. Similarly, women with versus without UPF addiction were more likely to exhibit gut dysbiosis, marked by an overabundance of microbes implicated in insulin resistance and greater propensity for obesity, and the presence of gut dysbiosis was also associated with increased neural connectivity in reward motivation regions [ 45 ]. Further, one study observed that individuals with UPF addiction and overweight/obesity were more likely to exhibit gut metabolomic patterns associated with high-risk traits for addictive disorders (e.g., emotion dysregulation, increased reward seeking), and the presence of this gut microbial signature mediated the positive relationship between UPF addiction and body mass index (BMI) [ 46 ].
Overall, there is emerging evidence for altered functioning across the brain-gut-microbiome axis among individuals with UPF addiction, which appear to be closely implicated in perpetuating compulsive eating behavior and/or increasing one’s risk for obesity.
Recent Research on the Behavioral Features of UPF Addiction
UPF addiction has been most consistently operationalized by adapting the 11 DSM-5 behavioral criteria for diagnosing SUDs to the addictive-like intake of UPFs [ 14 ••]. One theme of recent studies and scientific reviews has been to consider if and how the behavioral features of UPF addiction represent a distinct clinical presentation from existing eating disorders [ 47 , 48 , 49 ]. Importantly, overlap exists between several of the 11 DSM-5 diagnostic indicators of SUDs and problematic overeating behaviors, such as consuming more than intended, wanting to cut down but being unable to do so, and experiencing cravings. In binge-type eating disorders, overlapping behavioral features with addictive disorders can be more substantial, extending to symptoms like continued consumption despite negative physical/psychological consequences, interference with role obligations, and significant time spent obtaining, consuming, and recovering from the effects of the food/substance. As may be expected, the prevalence of UPF addiction among individuals with binge-type eating disorders is high (48–55% in a global meta-analysis [ 21 •]).
Yet, most individuals with UPF addiction do not have an eating disorder, as defined by the DSM-5, as the population prevalence rate of UPF addiction is 14% [ 21 •] and of a binge-type eating disorder is 1.0-2.8% [ 50 ]. The increased prevalence of UPF addiction compared to binge-type eating disorders may reflect the wider range of problematic behaviors encompassed by an addiction framework. While binge consumption is one presentation of addiction (e.g., binge drinking, chain smoking), many individuals meet criteria for a SUD by using their substance of choice in a grazing pattern (e.g., smoking cigarettes throughout the day). In parallel, while binge-type eating disorders are characterized by 2-hour episodes where an objectively large amount of food is consumed, UPF addiction may present as binge episodes and/or grazing (e.g., eating UPFs frequently throughout the day in response to persistent cravings). Importantly, research is needed to characterize common presentations of UPF addiction, as no data exist to speak to the frequency of addictive-like UPF intake occurring in binge episodes versus grazing patterns.
Two key differences in the presentation of UPF addiction, relative to binge-type eating disorders, are (1) the specificity of the addictive-like eating behaviors occurring with UPFs; and (2) the absence of a defined threshold for the quantity of food that must be consumed. Importantly, while no SUDs have a set threshold for how much of an addictive substance must be taken to meet diagnostic criteria, clinical judgment is used to ensure that individuals are using addictive substances to the extent that their use leads to impairment and/or distress. Similarly, while no set quantity of UPF intake is required to meet criteria for UPF addiction, the clinical presentation is intended to operationalize problematic overeating patterns with UPFs.
Research is needed to examine if the quantity of UPF intake has clinical relevance among individuals with UPF addiction. Similar consideration has been given to whether binge episodes (in the context of binge-type eating disorders) need to involve an objectively large amount of food, in light of prior studies finding stronger associations between increased symptoms of disordered eating and one’s experience of loss of control overeating versus the size of the binge [ 51 , 52 ]. In addition, following bariatric surgery, loss of control eating can re-emerge, but less commonly are patients able to consume objectively large amounts of food. Akin to ongoing discussions of whether this post-operative, loss of control eating should be classified as a binge-type eating disorder [ 53 ], research is warranted to inform the assessment of addictive-like UPF intake among individuals with conditions that physically limit their food intake. Further, although individuals with anorexia nervosa endorse high levels of UPF addiction on the self-report YFAS measures [ 54 , 55 ], these estimates have been recently discussed as likely false positives [ 14 ••, 56 •]. Presently, a clinician-guided tool for diagnosing UPF addiction is in development, which will likely clarify UPF addiction rates among persons with restrictive-type eating disorders.
In a similar vein of evaluating UPF addiction as a distinct clinical presentation, a second emerging trend in behavioral research is the evaluation of whether withdrawal can occur with UPFs [ 57 , 58 ]. Withdrawal is a core diagnostic indicator of SUDs that is not acknowledged by theoretical perspectives of obesity or eating disorders. As such, researchers have suggested that scientific evidence for the plausibility of UPF withdrawal would also support the clinical utility of UPF addiction as a unique presentation of problematic overeating [ 58 ]. While tolerance is also a unique feature of SUDs, less research has systematically assessed tolerance to UPFs, given the high levels of UPF consumption in the population, and thus withdrawal has been the mechanism of focus (for a review of neurobiological evidence for tolerance to UPFs, see [ 57 ]).
Early evidence for the plausibility for UPF withdrawal came from preclinical models, which observed that rats exhibited withdrawal-like symptoms (e.g., anxiety, teeth chattering) when UPFs or sugar (an ingredient often added to UPFs) were removed from their diets [ 59 ]. These findings sparked investigations of UPF withdrawal in humans, and though all human studies to date have relied on retrospective self-report, the preliminary data is similarly suggestive of its plausibility [ 58 ]. For instance, qualitative studies have revealed that both adults and youths describe experiencing physical (e.g., headaches, fatigue) and psychological (e.g., irritability, preoccupation with cravings) symptoms when trying to reduce UPFs that parallel features of nicotine withdrawal [ 60 , 61 , 62 ]. Further, the withdrawal symptom on the YFAS measures has been endorsed by 18.5–29.7% of adults and 18.9% of youths in general population samples [ 20 , 26 , 63 ].
Systematic investigations of UPF withdrawal in humans has been facilitated by the recent development of the Highly Processed Food Withdrawal Scale (ProWS) [ 64 ] and its adaptation designed for parents to report on their children’s UPF withdrawal symptoms (ProWS-C) [ 65 ]. (In this scale, “highly processed food” is synonymous with UPF.) The validation studies for both the ProWS and ProWS-C measures demonstrated that adults and youths, respectively, reported physical (e.g., headaches) and psychological (e.g., irritability, preoccupation) symptoms reminiscent of tobacco withdrawal during their most recent attempt to cut down on UPFs [ 64 , 65 ]. Both samples indicated that these withdrawal-like symptoms were most intense 2–5 days after reducing UPFs and decreased in intensity over the following 7–14 days, which parallels the time course of withdrawal syndromes for addictive substances [ 64 , 65 ]. Further, in both adults and youths, increased UPF withdrawal symptoms were associated with self-reported history of weight cycling and successful dietary adherence above and beyond BMI. Critically, methodologically rigorous, prospective techniques (e.g., randomized controlled trials, ecological momentary assessment) are needed to validate the presence, features, and time course of UPF withdrawal. Nonetheless, evidence to date from preclinical and human studies supports the plausibility of UPF withdrawal and suggests its clinical relevance to weight management and adherence to dietary recommendations.
Emerging Evidence for the Clinical Utility of UPF Addiction within Overweight or Obesity
In the past 5 years, evidence has grown for the clinical relevance of UPF addiction among individuals with overweight or obesity. The most compelling finding to date resulted from a large, randomized controlled trial of 609 adults who participated in a 12-month behavioral weight loss intervention [ 66 ••]. Strikingly, of the 41 demographic and psychosocial factors examined, symptoms of UPF addiction at baseline was the single strongest psychosocial predictor of both treatment dropout and lower weight loss [ 66 ••]. Other baseline characteristics that were found to be less predictive of these outcomes than symptoms of UPF addiction (or not significant at all) included self-efficacy for dietary change, emotional eating, body dissatisfaction, social support for eating habits, perceived stress, quality of life, dietary restraint, depression, sleep quality, age, education, and race [ 66 ••]. Three other related studies yielded mixed findings on the association of UPF addiction with outcomes in obesity treatments [ 67 , 68 , 69 ], though null findings may have been partially attributable to limitations in sample size, treatment duration, and/or intervention approach [ 66 ••]. While replication is warranted, the recent findings from Fielding-Singh and colleagues [ 66 ••] convincingly illustrate that UPF addiction warrants assessment and intervention among persons seeking behavioral weight loss treatment.
The relevance of UPF addiction to weight management was further elucidated by Schulte and colleagues [ 70 ], who observed that adults with versus without UPF addiction reported gaining six times more weight during the first year of the COVID-19 pandemic (12.42 vs. 2.14 pounds, respectively). Further, those with UPF addiction reported consuming greater quantities of UPFs both before and during COVID-19 and endorsed greater distress related to their eating habits [ 70 ]. Though this study was cross-sectional, the findings are suggestive of the potential for UPF addiction to be a risk factor for weight gain, UPF overconsumption, and psychologically distressing eating behaviors.
Speaking to another facet of the relationship between UPF addiction and weight management, Leary and colleagues [ 71 ] reviewed eight studies that assessed the efficacy of pharmacological (naltrexone/bupropion, pexacerfont), bariatric surgery, and lifestyle (individual or group-based behavioral counseling, calorie restriction, and/or physical activity promotion) interventions for obesity for improving UPF addiction. Five of the eight studies observed improvements in UPF addiction among adults with obesity, in which the interventions included medications (naltrexone/bupropion and pexacerfont), bariatric surgery, and a behavioral weight loss intervention involving meal replacements [ 71 ]. Interestingly, the medications that led to improvements in UPF addiction have also demonstrated efficacy for decreasing cravings for addictive substances and treating SUDs (though naltrexone and bupropion are prescribed separately for those with an addiction) [ 72 , 73 ].
From the small number of studies included in Leary and colleagues’ review [ 71 ], it is apparent that more research is needed to explore how existing interventions for overweight and obesity may have secondary benefits for improving UPF addiction. However, it is also evident from Fielding-Singh and colleagues’ [ 66 ••] data that exhibiting UPF addiction may substantially interfere with one’s ability to engage in weight management treatments or achieve weight loss. The complex, bidirectional relationship between UPF addiction and obesity treatments warrants attention in future research. One pressing and unexplored focus may be the identification of risk and resilience factors among those with UPF addiction that differentially predict who does versus does not achieve clinically meaningful weight loss through existing treatments.
Next Steps for the Treatment of UPF Addiction
Table 1 summarizes the future directions for advancing the science on UPF stated earlier in this review, as well as the ideas for treating UPF addiction described in this section. At present, no evidence-based interventions have been specifically developed to treat UPF addiction. Yet, there are online self-help and community-based resources for individuals who self-identify as having a “food addiction,” which suggests there is an unaddressed need for scientifically informed clinical assessments and interventions for persons who exhibit addictive-like food intake. McKenna and colleagues [ 74 ] found that nearly all online support options aligned with the 12-step abstinence framework of community-based SUD treatments (e.g., Alcoholics Anonymous), which has been adapted as Overeaters Anonymous (OA). While OA does not use the term UPFs, the food plans and abstinence guidelines of OA emphasize abstinence from foods with sugar and refined carbohydrates, which would be classified as UPFs.
Given the anonymous nature of OA, no randomized controlled trials have explored the program’s efficacy. The limited data on OA stems from case series and qualitative research, though these studies suggest benefits for binge-type eating disorders and obesity (for a review, see [ 75 ]). Potential benefits of OA’s abstinence-based approach to treating UPF addiction may be gleaned from a recent pilot trial of 103 adults, which demonstrated that a 10-to-14-week, group-based intervention emphasizing abstinence from UPFs led to significant reductions in symptoms of UPF addiction [ 76 ]. In addition, a case series and pilot trial have shown that the ketogenic diet, which severely limits sugars and refined carbohydrates, may also lead to reductions in symptoms of UPF addiction [ 77 , 78 ]. Rigorous, randomized controlled trials are needed to evaluate if abstaining from UPFs is an appropriate dietary recommendation for the treatment of UPF addiction.
Some have questioned the long-term feasibility of abstinent approaches, and not all evidence-based treatments for SUDs prescribe abstinence. For example, harm reduction is an efficacious treatment for alcohol-use disorder that nuances being abstinent from high-risk use patterns (e.g., hard liquor, drinking when alone or in a negative mood state) and engaging in low-risk use patterns (e.g., having one glass of wine at a restaurant with friends) [ 79 ]. This approach has been particularly effective at preventing relapse among individuals who perceive alcohol to be integrated into their social circles in normative ways and can identify that their problematic drinking behaviors occur primarily in specific contexts [ 80 ]. The social acceptability of and abundant access to UPFs in our society may necessitate prioritization on evidence-based interventions that do not require abstinence from all UPFs (e.g., splitting a dessert with friends at a restaurant but not keeping pastries or packaged sweets in the home). Research is needed to determine whether a harm reduction approach is effective for treating UPF addiction, and if so, how it compares to recommendations to abstain from UPFs in terms of adherence and outcomes.
The notable finding that UPF addiction was the strongest psychosocial predictor of poorer engagement and outcomes in a behavioral weight loss program [ 66 ••] raises concern about how existing behavioral interventions may overlook, or even worsen, symptoms of UPF addiction among individuals with overweight or obesity. Commonly, behavioral weight loss treatments are based on the Diabetes Prevention Program, emphasizing dietary modification, increased physical activity, self-monitoring, and psychological influences on lifestyle changes (e.g., stimulus control, negative thought patterns) [ 81 ]. While the program recommends limiting high-calorie foods (often UPFs), dietary changes are centered on meeting a calorie goal that promotes weight loss, which allows for all foods to be consumed in moderation. The program acknowledges that individuals may have momentary lapses and longer-lasting relapses in meeting their calorie target [ 81 ], though no connections are made to how these setbacks may, for some people, stem from the intake of UPFs.
From an addiction standpoint, the intermittent intake of an addictive substance may increase the reinforcement that a person experiences, particularly for individuals with a SUD [ 82 ]. Similarly, for individuals with UPF addiction, the common behavioral weight loss principle of eating all foods in moderation, especially within a calorie target, may inadvertently promote UPF cravings and subsequent UPF intake (a lapse/relapse). In addition, features of addiction, such as withdrawal, may interfere with early dietary adherence and motivate treatment dropout for persons with UPF addiction. Future research is needed to understand whether tailored dietary modification goals and addiction-focused strategies (e.g., coping with withdrawal) would improve outcomes in behavioral interventions for obesity among individuals with UPF addiction, as well as identify individual characteristics that may inform whether an abstinence-based or harm reduction approach may be more optimal.
The breadth of recent research on UPF addiction illustrates continued scientific and public interest in the construct and its implications for understanding and treating overeating behaviors and obesity. Topical developments have included: (1) estimating the global prevalence of UPF addiction at 14% of adults and 15% of youths (28% and 19% among samples with obesity, respectively); (2) revealing health disparities in rates of UPF addiction for persons of color and those with food insecurity; (3) observing altered functioning across the brain-gut-microbiome axis among individuals with UPF addiction; (4) providing early evidence for the plausibility of experiencing a withdrawal syndrome when cutting down on UPFs; and (5) elucidating poorer weight management outcomes among persons with UPF addiction. There are abundant next steps in this line of research, though a particularly pressing gap in the literature is the lack of targeted interventions for UPF addiction. Developing and demonstrating the efficacy of novel, addiction-based treatments for UPF addiction may result in more optimal clinical outcomes for this underserved population.
Data Availability
No datasets were generated or analysed during the current study.
Papers of particular interest, published recently, have been highlighted as: • Of importance •• Of major importance
Haththotuwa RN, Wijeyaratne CN. Worldwide epidemic of obesity. In: Obesity and Obstetrics. Elsevier; 2020. p. 3–8.
Chapter Google Scholar
Masood B, Moorthy M. Causes of obesity: a review. Clin Med (Lond). 2023;23(4):284–91.
Article PubMed Google Scholar
Harb AA, Shechter A, Koch PA, St-Onge MP. Ultra-processed foods and the development of obesity in adults. Eur J Clin Nutr. 2023;77(6):619–27.
Hall KD. Did the food environment cause the obesity epidemic? Obesity (Silver Spring). 2018;26(1):11–3.
Monteiro CA, Cannon G, Lawrence M, Costa Louzada M, Pereira Machado P. Ultra-processed foods, diet quality, and health using the NOVA classification system. Rome: FAO; 2019. p. 49.
Google Scholar
Monteiro CA, Cannon G, Levy RB, Moubarac J, Louzada MLC, Rauber F, et al. Ultra-processed foods: what they are and how to identify them. Public Health Nutr. 2019;22(5):936–41.
Article PubMed PubMed Central Google Scholar
Chen X, Zhang Z, Yang H, Qiu P, Wang H, Wang F, et al. Consumption of ultra-processed foods and health outcomes: a systematic review of epidemiological studies. Nutr J. 2020;19(1):86.
Swinburn BA, Sacks G, Hall KD, McPherson K, Finegood DT, Moodie ML, et al. The global obesity pandemic: shaped by global drivers and local environments. Lancet. 2011;378(9793):804–14.
• Fazzino TL, Jun D, Chollet-Hinton L, Bjorlie K. US tobacco companies selectively disseminated hyper-palatable foods into the US food system: empirical evidence and current implications. Addiction. 2024;119(1):62–71. This study reviewed primary industry documents and found that food brands owned by tobacco companies were more likely to formulate ultra-processed foods compared to brands not owned by tobacco industry leaders .
Demeke S, Rohde K, Chollet-Hinton L, Sutton C, Kong KL, Fazzino TL. Change in hyper-palatable food availability in the US food system over 30 years: 1988–2018. Public Health Nutr. 2023;26(1):182–9.
•• Gearhardt AN, Schulte EM. Is food addictive? A review of the science. Annu Rev Nutr. 2021;41:387–410. This review comprehensively describes the evidence for the addictive potentials of ultra-processed foods and which attributes of these foods may be most reinforcing .
Article CAS PubMed Google Scholar
Schulte EM, Avena NM, Gearhardt AN. Which foods may be addictive? The roles of processing, fat content, and glycemic load. PLoS ONE. 2015;10(2):e0117959.
Gearhardt AN, DiFeliceantonio AG. Highly processed foods can be considered addictive substances based on established scientific criteria. Addiction. 2023;118(4):589–98.
•• LaFata EM, Gearhardt AN. Ultra-processed food addiction: an epidemic? Psychother Psychosom. 2022;91(6):363–72. This editorial describes the empirical evidence for ultra-processed food addiction as a distinct clinical presentation .
Hebebrand J, Gearhardt AN. The concept of food addiction helps inform the understanding of overeating and obesity: NO. Am J Clin Nutr. 2021;113(2):268–73.
Mestre-Bach G, Jiménez-Murcia S, Fernández-Aranda F, Potenza MN. Addressing controversies surrounding food addiction. Compulsive Eating Behavior and Food Addiction. Elsevier. 2019. pp. 419–48.
Vasiliu O. Current status of evidence for a new diagnosis: food addiction-a literature review. Front Psychiatry. 2021;12:824936.
Ratkovic D, Knezevic V, Dickov A, Fedrigolli E, Comic M. Comparison of binge-eating disorder and food addiction. J Int Med Res. 2023;51(4):3000605231171016.
Meule A, Gearhardt AN. Ten years of the yale food addiction scale: a review of version 2.0. Curr Addict Rep. 2019;6(3):218–28.
Article Google Scholar
Gearhardt AN, Corbin WR, Brownell KD. Development of the yale food addiction scale version 2.0. Psychol Addict Behav. 2016;30(1):113–21.
• Praxedes DRS, Silva-Junior AE, Macena ML, Oliveira AD, Cardoso KS, Nunes LO, et al. Prevalence of food addiction determined by the yale food addiction scale and associated factors: a systematic review with meta-analysis. Eur Eat Disord Rev. 2022;30(2):85–95. This systematic review estimated the prevalence of ultra-processed food addiction at 14% of adults in the general population and 28% of adults with obesity .
• Yekaninejad MS, Badrooj N, Vosoughi F, Lin CY, Potenza MN, Pakpour AH. Prevalence of food addiction in children and adolescents: a systematic review and meta-analysis. Obes Rev. 2021;22(6):e13183. This systematic review estimated the prevalence of ultra-processed food addiction at 15% of youths in the general population and 19% of youths with overweight or obesity .
World Health Organization. WHO report on the global tobacco epidemic 2019. In: Offer help to quit tobacco use. World Health Organization; 2019.
World Health Organization. Global status report on alcohol and health 2018. World Health Organization; 2019.
•• Gearhardt AN, Bueno NB, DiFeliceantonio AG, Roberto CA, Jimenez-Murcia S, Fernandez-Aranda F. Social, clinical, and policy implications of ultra-processed food addiction. BMJ. 2023;383:e075354. This article outlines the substantial social justice, clinical, and public policy implications that will likely follow as evidence for ultra-processed food addiction continues to grow .
Schulte EM, Gearhardt AN. Associations of food addiction in a sample recruited to be nationally representative of the United States. Eur Eat Disord Rev. 2018;26(2):112–9.
Parnarouskis L, Gearhardt AN, Mason AE, Adler NE, Laraia BA, Epel ES, et al. Association of food insecurity and food addiction symptoms: a secondary analysis of two samples of low-income female adults. J Acad Nutr Diet. 2022;122(10):1885–92.
Leung CW, Fulay AP, Parnarouskis L, Martinez-Steele E, Gearhardt AN, Wolfson JA. Food insecurity and ultra-processed food consumption: the modifying role of participation in the Supplemental Nutrition Assistance Program (SNAP). Am J Clin Nutr. 2022;116(1):197–205.
•• Nguyen KH, Glantz SA, Palmer CN, Schmidt LA. ransferring racial/ethnic marketing strategies from tobacco to food corporations: Philip Morris and kraft general foods. Am J Public Health. 2020;110(3):329–36. This study examined internal industry documents and revealed that after Phillip Morris (a tobacco company) purchased General Foods and Kraft Foods, a marketing program was initiated to target underrepresented racial and ethnic groups in campaigns promoting ultra-processed foods .
Makaronidis JM, Batterham RL. Obesity, body weight regulation and the brain: insights from fMRI. Br J Radiol. 2018;91(1089):20170910.
Tang DW, Fellows LK, Small DM, Dagher A. Food and drug cues activate similar brain regions: a meta-analysis of functional MRI studies. Physiol Behav. 2012;106(3):317–24.
Gearhardt AN, Yokum S, Orr PT, Stice E, Corbin WR, Brownell KD. Neural correlates of food addiction. Arch Gen Psychiatry. 2011;68(8):808–16.
Schulte EM, Yokum S, Jahn A, Gearhardt AN. Food cue reactivity in food addiction: a functional magnetic resonance imaging study. Physiol Behav. 2019;208:112574.
Article CAS PubMed PubMed Central Google Scholar
Ravichandran S, Bhatt RR, Pandit B, Osadchiy V, Alaverdyan A, Vora P, et al. Alterations in reward network functional connectivity are associated with increased food addiction in obese individuals. Sci Rep. 2021;11(1):3386.
Schulte EM, Chao AM, Allison KC. Advances in the neurobiology of food addiction. Curr Behav Neurosci Rep. 2021;8:103–12.
Romer SS, Bliokas V, Teo JT, Thomas SJ. Food addiction, hormones and blood biomarkers in humans: a systematic literature review. Appetite. 2023;183:106475.
Oginsky MF, Goforth PB, Nobile CW, Lopez-Santiago LF, Ferrario CR. Eating ‘junk-food’ produces rapid and long-lasting increases in NAc CP-AMPA receptors: implications for enhanced cue-induced motivation and food addiction. Neuropsychopharmacology. 2016;41(13):2977–86.
Brown RM, Kupchik YM, Spencer S, Garcia-Keller C, Spanswick DC, Lawrence AJ, et al. Addiction-like synaptic impairments in diet-induced obesity. Biol Psychiatry. 2017;81(9):797–806.
Alonso-Caraballo Y, Fetterly TL, Jorgensen ET, Nieto AM, Brown TE, Ferrario CR. Sex specific effects of junk-food diet on calcium permeable AMPA receptors and silent synapses in the nucleus accumbens core. Neuropsychopharmacology. 2021;46(3):569–78.
Fetterly TL, Catalfio AM, Ferrario CR. Effects of junk-food on food-motivated behavior and nucleus accumbens glutamate plasticity; insights into the mechanism of calcium-permeable AMPA receptor recruitment. Neuropharmacology. 2024;242:109772.
Oginsky MF, Ferrario CR. Eating junk food has opposite effects on intrinsic excitability of nucleus accumbens core neurons in obesity-susceptible versus -resistant rats. J Neurophysiol. 2019;122(3):1264–73.
Nieto AM, Catalfio AM, Papacostas Quintanilla H, Alonso-Caraballo Y, Ferrario CR. Transient effects of junk food on NAc core MSN excitability and glutamatergic transmission in obesity-prone female rats. Obes (Silver Spring). 2023;31(2):434–45.
Article CAS Google Scholar
Kendig MD, Hasebe K, McCague R, Lee F, Leigh SJ, Arnold R, et al. Adolescent exposure to a solid high-fat, high-sugar ‘cafeteria’ diet leads to more pronounced changes in metabolic measures and gut microbiome composition than liquid sugar in female rats. Appetite. 2022;172:105973.
Gupta A, Osadchiy V, Mayer EA. Brain-gut-microbiome interactions in obesity and food addiction. Nat Rev Gastroenterol Hepatol. 2020;17(11):655–72.
Dong TS, Mayer EA, Osadchiy V, Chang C, Katzka W, Lagishetty V, et al. A distinct brain-gut-microbiome profile exists for females with obesity and food addiction. Obes (Silver Spring). 2020;28(8):1477–86.
Zhang X, Bhatt RR, Todorov S, Gupta A. Brain-gut microbiome profile of neuroticism predicts food addiction in obesity: a transdiagnostic approach. Prog Neuropsychopharmacol Biol Psychiatry. 2023;125:110768.
di Giacomo E, Aliberti F, Pescatore F, Santorelli M, Pessina R, Placenti V, et al. Disentangling binge eating disorder and food addiction: a systematic review and meta-analysis. Eat Weight Disord. 2022;27(6):1963–70.
Schulte EM, Wadden TA, Allison KC. An evaluation of food addiction as a distinct psychiatric disorder. Int J Eat Disord. 2020;53(10):1610–22.
Wiss D. Clinical considerations of ultra-processed food addiction across weight classes: an eating disorder treatment and care perspective. Curr Addict Rep. 2022;9(4):255–67.
Hudson JI, Hiripi E, Pope HG Jr, Kessler RC. The prevalence and correlates of eating disorders in the national comorbidity survey replication. Biol Psychiatry. 2007;61(3):348–58.
Bruzas MB, Tronieri JS, Chao AM, Jones E, McAllister C, Gruber K, et al. Binge size and loss of control as correlates of eating behavior and psychopathology among individuals with binge eating disorder and higher weight. J Behav Med. 2022;45(4):603–12.
Brownstone LM, Bardone-Cone AM. Subjective binge eating: a marker of disordered eating and broader psychological distress. Eat Weight Disord. 2021;26(7):2201–9.
Ivezaj V, Lydecker JA, Wiedemann AA, Duffy AJ, Grilo CM. Does bariatric binge-eating size matter? Conceptual model and empirical support. Obes (Silver Spring). 2020;28(9):1645–51.
Sanchez I, Lucas I, Munguia L, Camacho-Barcia L, Gimenez M, Sanchez-Gonzalez J, et al. Food addiction in anorexia nervosa: implications for the understanding of crossover diagnosis. Eur Eat Disord Rev. 2022;30(3):278–88.
Tran H, Poinsot P, Guillaume S, Delaunay D, Bernetiere M, Begin C, et al. Food addiction as a proxy for anorexia nervosa severity: new data based on the yale food addiction scale 2.0. Psychiatry Res. 2020;293:113472.
• Wiss D, Brewerton T. Separating the signal from the noise: How psychiatric diagnoses can help discern food addiction from dietary restraint. Nutrients. 2020;12(10):2937. This article provides interpretation on high endorsement rates of ultra-processed food addiction on the Yale Food Addiction Scale measures among individuals with restrictive-type eating disorders, raising that high false positive rates may have resulted from self-report assessments .
Parnarouskis L, Gearhardt AN. Preliminary evidence that tolerance and withdrawal occur in response to ultra-processed foods. Curr Addict Rep. 2022;9(4):282–9.
Parnarouskis L, Leventhal AM, Ferguson SG, Gearhardt AN, Withdrawal. A key consideration in evaluating whether highly processed foods are addictive. Obes Rev. 2022;23(11):e13507.
Avena NM. The study of food addiction using animal models of binge eating. Appetite. 2010;55(3):734–7.
Rodda SN, Booth N, Brittain M, McKean J, Thornley S. I was truly addicted to sugar: a consumer-focused classification system of behaviour change strategies for sugar reduction. Appetite. 2020;144:104456.
Schiestl ET, Wolfson JA, Gearhardt AN. The qualitative evaluation of the Yale Food Addiction Scale 2.0. Appetite. 2022;175:106077.
Pretlow RA. Addiction to highly pleasurable food as a cause of the childhood obesity epidemic: a qualitative internet study. Eat Disord. 2011;19(4):295–307.
Gearhardt AN, Roberto CA, Seamans MJ, Corbin WR, Brownell KD. Preliminary validation of the yale food addiction scale for children. Eat Behav. 2013;14(4):508–12.
Schulte EM, Smeal JK, Lewis J, Gearhardt AN. Development of the highly processed food withdrawal scale. Appetite. 2018;131:148–54.
Parnarouskis L, Schulte EM, Lumeng JC, Gearhardt AN. Development of the highly processed food withdrawal scale for children. Appetite. 2020;147:104553.
•• Fielding-Singh P, Patel ML, King AC, Gardner CD. Baseline psychosocial and demographic factors associated with study attrition and 12-month weight gain in the DIETFITS trial. Obesity (Silver Spring). 2019;27(12):1997–2004. This large, randomized controlled trial of 609 adults enrolled in a 12-month behavioral weight loss program found that increased symptoms of ultra-processed food addiction at baseline was the single strongest predictor of treatment dropout and lesser weight loss .
Burmeister JM, Hinman N, Koball A, Hoffmann DA, Carels RA. Food addiction in adults seeking weight loss treatment. Implications for psychosocial health and weight loss. Appetite. 2013;60(1):103–10.
Chao AM, Wadden TA, Tronieri JS, Pearl RL, Alamuddin N, Bakizada ZM, et al. Effects of addictive-like eating behaviors on weight loss with behavioral obesity treatment. J Behav Med. 2019;42(2):246–55.
Lent MR, Eichen DM, Goldbacher E, Wadden TA, Foster GD. Relationship of food addiction to weight loss and attrition during obesity treatment. Obes (Silver Spring). 2014;22(1):52–5.
Schulte EM, Kral TVE, Allison KC. A cross-sectional examination of reported changes to weight, eating, and activity behaviors during the COVID-19 pandemic among United States adults with food addiction. Appetite. 2022;168:105740.
Leary M, Pursey KM, Verdejo-Garcia A, Burrows TL. Current intervention treatments for food addiction: a systematic review. Behav Sci (Basel). 2021;11(6):80.
Potenza MN, Sofuoglu M, Carroll KM, Rounsaville BJ. Neuroscience of behavioral and pharmacological treatments for addictions. Neuron. 2011;69(4):695–712.
Morabbi MJ, Razaghi E, Moazen-Zadeh E, Safi-Aghdam H, Zarrindast MR, Vousoghi N, et al. Pexacerfont as a CRF1 antagonist for the treatment of withdrawal symptoms in men with heroin/methamphetamine dependence: a randomized, double-blind, placebo-controlled clinical trial. Int Clin Psychopharmacol. 2018;33(2):111–9.
McKenna RA, Rollo ME, Skinner JA, Burrows TL. Food addiction support: website content analysis. JMIR Cardio. 2018;2(1):e10.
Bray B, Rodriguez-Martin BC, Wiss DA, Bray CE, Zwickey H. Overeaters Anonymous: an overlooked intervention for binge eating disorder. Int J Environ Res Public Health. 2021;18(14):7303.
Unwin J, Delon C, Giaever H, Kennedy C, Painschab M, Sandin F, et al. Low carbohydrate and psychoeducational programs show promise for the treatment of ultra-processed food addiction. Front Psychiatry. 2022;13:1005523.
Rostanzo E, Marchetti M, Casini I, Aloisi AM. Very-low-calorie ketogenic diet: a potential treatment for binge eating and food addiction symptoms in women. A pilot study. Int J Environ Res Public Health. 2021;18(23):12802.
Carmen M, Safer DL, Saslow LR, Kalayjian T, Mason AE, Westman EC, et al. Treating binge eating and food addiction symptoms with low-carbohydrate ketogenic diets: a case series. J Eat Disord. 2020;8:2.
Marlatt GA, Witkiewitz K. Harm reduction approaches to alcohol use: health promotion, prevention, and treatment. Addict Behav. 2002;27(6):867–86.
Marlatt GA, Donovan DM. Relapse prevention: maintenance strategies in the treatment of addictive behaviors. Guilford Press; 2005.
Diabetes Prevention Program Research G. The Diabetes Prevention Program (DPP). Description of lifestyle intervention. Diabetes Care. 2002;25(12):2165–71.
Kawa AB, Bentzley BS, Robinson TE. Less is more: prolonged intermittent access cocaine self-administration produces incentive-sensitization and addiction-like behavior. Psychopharmacology. 2016;233(19–20):3587–602.
Download references
Author information
Authors and affiliations.
Center for Weight, Eating, and Lifestyle Science, Drexel University, 3201 Chestnut Street, Philadelphia, PA, 19104, United States
Erica M. LaFata & Evan M. Forman
Center for Weight and Eating Disorders, Department of Psychiatry, Perelman School of Medicine, University of Pennsylvania, 3535 Market Street, Philadelphia, PA, 19104, United States
Kelly C. Allison
Department of Psychiatry, Perelman School of Medicine, University of Pennsylvania, 3535 Market Street, Philadelphia, PA, 19104, United States
Janet Audrain-McGovern
Department of Psychological and Brain Sciences, Drexel University, 3201 Chestnut Street, Philadelphia, PA, 19104, United States
Evan M. Forman
You can also search for this author in PubMed Google Scholar
Contributions
E.M.L. drafted the manuscript. All authors participated in editing the manuscript and approved the final version.
Corresponding author
Correspondence to Erica M. LaFata .
Ethics declarations
Conflict of interests.
The authors declare no competing interests.
Human and Animal Rights and Informed Consent
This article does not contain any studies with human or animal subjects performed by any of the authors.
Additional information
Publisher’s note.
Springer Nature remains neutral with regard to jurisdictional claims in published maps and institutional affiliations.
Rights and permissions
Open Access This article is licensed under a Creative Commons Attribution 4.0 International License, which permits use, sharing, adaptation, distribution and reproduction in any medium or format, as long as you give appropriate credit to the original author(s) and the source, provide a link to the Creative Commons licence, and indicate if changes were made. The images or other third party material in this article are included in the article's Creative Commons licence, unless indicated otherwise in a credit line to the material. If material is not included in the article's Creative Commons licence and your intended use is not permitted by statutory regulation or exceeds the permitted use, you will need to obtain permission directly from the copyright holder. To view a copy of this licence, visit http://creativecommons.org/licenses/by/4.0/ .
Reprints and permissions
About this article
LaFata, E.M., Allison, K.C., Audrain-McGovern, J. et al. Ultra-Processed Food Addiction: A Research Update. Curr Obes Rep (2024). https://doi.org/10.1007/s13679-024-00569-w
Download citation
Accepted : 22 April 2024
Published : 18 May 2024
DOI : https://doi.org/10.1007/s13679-024-00569-w
Share this article
Anyone you share the following link with will be able to read this content:
Sorry, a shareable link is not currently available for this article.
Provided by the Springer Nature SharedIt content-sharing initiative
- Ultra-processed foods
- Ultra-processed food addiction
- Compulsive overeating
- Obesity treatment outcomes
Advertisement
- Find a journal
- Publish with us
- Track your research
Study confirms that processed foods key to rising obesity
‘Protein hunger’ drives overeating, large-scale population study shows
The modern palate's preference for highly processed and refined foods over a protein-rich diet is a key contributor to the high obesity rates in the Western world.
A year-long study of the dietary habits of 9,341 Australians has backed growing evidence that highly processed and refined foods are the leading contributor of rising obesity rates in the Western world.
The new study , in the latest issue of the journal Obesity conducted by the University of Sydney’s Charles Perkins Centre (CPC), was based on a national nutrition and physical activity survey undertaken by the Australian Bureau of Statistics (ABS), and further backs the ‘Protein Leverage Hypothesis’.
First put forward in 2005 by professors Raubenheimer and Stephen Simpson, the Protein Leverage Hypothesis argues that people overeat fats and carbohydrates because of the body’s strong appetite for protein, which the body actively favours over everything else. Because so much of modern diets consist of highly processed and refined foods – which are low in protein – people are driven to consume more energy-dense foods until they satisfy their protein demand.
Processed foods lack protein and drive craving
“As people consume more junk foods or highly processed and refined foods, they dilute their dietary protein and increase their risk of being overweight and obese, which we know increases the risk of chronic disease,” said lead author Dr Amanda Grech , a Postdoctoral Research Fellow at the CPC and the university’s School of Life and Environmental Sciences.
“It’s increasingly clear that our bodies eat to satisfy a protein target,” added Professor David Raubenheimer , the Leonard Ullmann Chair in Nutritional Ecology at the School of Life and Environmental Sciences.
“But the problem is that the food in Western diets contains increasingly less protein. So, you have to consume more of it to reach your protein target, which effectively elevates your daily energy intake.
“Humans, like many other species, have a stronger appetite for protein than for the main energy-providing nutrients of fats and carbohydrates. That means that if the protein in our diet is diluted with fats and carbohydrates, we will eat more energy to get the protein that our bodies crave.”
Protein essential to good health
Proteins are the building blocks of life: every cell in the body contains them, and they are used to repair cells or make new ones; and it’s estimated that over a million forms of protein are needed to allow a human body to function. Protein sources include meats, milk, fish, eggs, soy, legumes, beans, and some grains such as wheat germ and quinoa.
The University of Sydney scientists analysed data from a cross-sectional survey of nutrition and physical activity in 9,341 adults, known as the National Nutrition and Physical Activity Survey which was conducted from May 2011 to June 2012, with a mean age of 46.3 years. They found the population’s mean energy intake was 8,671 kilojoules (kJ), with the mean percentage of energy from protein being just 18.4 percent, compared with 43.5 percent from carbohydrates and from 30.9 percent from fat, and just 2.2 percent from fibre and 4.3 percent from alcohol.
They then plotted energy intake versus the time of consumption and found that the pattern matched that predicted by the Protein Leverage Hypothesis. Those who consumed lower amounts of protein in their first meal of the day went on to increase their overall food intake in subsequent meals, whereas those who received the recommended amount of protein did not – and, in fact, declined their food intake throughout the day.
‘Protein hunger’ found to drive overeating
They also found a statistically significant difference between groups by the third meal of the day: those with a higher proportion of energy from protein at the start of the day had much lower total energy intake for the day. Meanwhile, those who consumed foods low in protein at the start of the day proceeded to increase consumption, indicating they were seeking to compensate with a higher consumption of overall energy. This is despite the fact the first meal was the smallest for both groups, with the least amount of energy and food consumed, whereas the last meal was the largest.
Participants with a lower proportion of protein than recommended at the first meal consumed more discretionary foods – energy-dense foods high in saturated fats, sugars, salt, or alcohol – throughout the day, and less of the recommended five food groups (grains; vegetables/legumes; fruit; dairy and meats). Consequently, they had an overall poorer diet at each mealtime, with their percentage of protein energy decreasing even as their discretionary food intake rose – an effect the scientists call ‘protein dilution’.
Explanation for obesity
“The results support an integrated ecological and mechanistic explanation for obesity, in which low-protein, highly processed foods lead to higher energy intake in response to a nutrient imbalance driven by a dominant appetite for protein,” said Professor Raubenheimer. “It supports a central role for protein in the obesity epidemic, with significant implications for global health.”
Seeking to understand how protein drives human nutrition has also sought taken Professor Raubenheimer to study the diets of people in some of the most remote places, from the Congo to the Himalayas. “The protein mechanism in appetite is a revolutionary insight,” he said. “Obesity, diabetes, cardiovascular disease - they’re all driven by diet, and we have to use what we’re learning to bring them under control.”
The CPC team’s paper was chosen by the editors of Obesity as one of the year’s top five papers, with the study’s leader, Professor Raubenheimer, invited to speak at the annual Obesity Journal Symposium in San Diego on November 4.
Wilson da Silva
- +61 421 616 103
- [email protected]
Related articles
Put down the protein shake: variety of protein better for health, taking a diet break can help weight loss.
Could indulging in chocolate over the Easter season actually be good for you? Dr Nicholas Fuller explains why taking a diet break can help with long-term weight loss goals.
National supplies of protein, carbs and fats may predict your lifespan
Frontiers for Young Minds
- Download PDF
The Impacts of Junk Food on Health
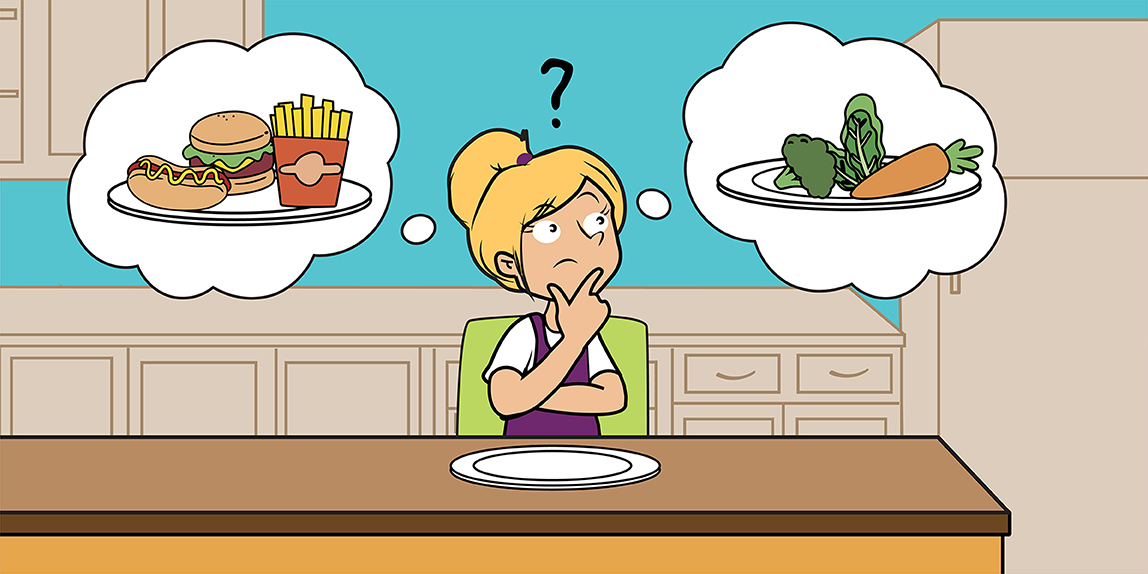
Energy-dense, nutrient-poor foods, otherwise known as junk foods, have never been more accessible and available. Young people are bombarded with unhealthy junk-food choices daily, and this can lead to life-long dietary habits that are difficult to undo. In this article, we explore the scientific evidence behind both the short-term and long-term impacts of junk food consumption on our health.
Introduction
The world is currently facing an obesity epidemic, which puts people at risk for chronic diseases like heart disease and diabetes. Junk food can contribute to obesity and yet it is becoming a part of our everyday lives because of our fast-paced lifestyles. Life can be jam-packed when you are juggling school, sport, and hanging with friends and family! Junk food companies make food convenient, tasty, and affordable, so it has largely replaced preparing and eating healthy homemade meals. Junk foods include foods like burgers, fried chicken, and pizza from fast-food restaurants, as well as packaged foods like chips, biscuits, and ice-cream, sugar-sweetened beverages like soda, fatty meats like bacon, sugary cereals, and frozen ready meals like lasagne. These are typically highly processed foods , meaning several steps were involved in making the food, with a focus on making them tasty and thus easy to overeat. Unfortunately, junk foods provide lots of calories and energy, but little of the vital nutrients our bodies need to grow and be healthy, like proteins, vitamins, minerals, and fiber. Australian teenagers aged 14–18 years get more than 40% of their daily energy from these types of foods, which is concerning [ 1 ]. Junk foods are also known as discretionary foods , which means they are “not needed to meet nutrient requirements and do not belong to the five food groups” [ 2 ]. According to the dietary guidelines of Australian and many other countries, these five food groups are grains and cereals, vegetables and legumes, fruits, dairy and dairy alternatives, and meat and meat alternatives.
Young people are often the targets of sneaky advertising tactics by junk food companies, which show our heroes and icons promoting junk foods. In Australia, cricket, one of our favorite sports, is sponsored by a big fast-food brand. Elite athletes like cricket players are not fuelling their bodies with fried chicken, burgers, and fries! A study showed that adolescents aged 12–17 years view over 14.4 million food advertisements in a single year on popular websites, with cakes, cookies, and ice cream being the most frequently advertised products [ 3 ]. Another study examining YouTube videos popular amongst children reported that 38% of all ads involved a food or beverage and 56% of those food ads were for junk foods [ 4 ].
What Happens to Our Bodies Shortly After We Eat Junk Foods?
Food is made up of three major nutrients: carbohydrates, proteins, and fats. There are also vitamins and minerals in food that support good health, growth, and development. Getting the proper nutrition is very important during our teenage years. However, when we eat junk foods, we are consuming high amounts of carbohydrates, proteins, and fats, which are quickly absorbed by the body.
Let us take the example of eating a hamburger. A burger typically contains carbohydrates from the bun, proteins and fats from the beef patty, and fats from the cheese and sauce. On average, a burger from a fast-food chain contains 36–40% of your daily energy needs and this does not account for any chips or drinks consumed with it ( Figure 1 ). This is a large amount of food for the body to digest—not good if you are about to hit the cricket pitch!
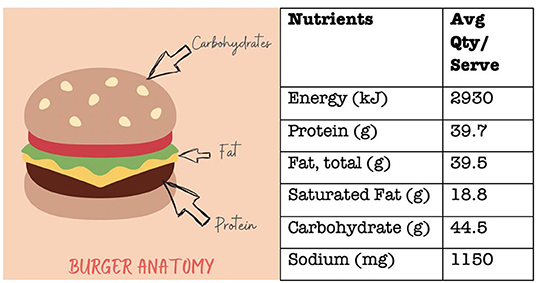
- Figure 1 - The nutritional composition of a popular burger from a famous fast-food restaurant, detailing the average quantity per serving and per 100 g.
- The carbohydrates of a burger are mainly from the bun, while the protein comes from the beef patty. Large amounts of fat come from the cheese and sauce. Based on the Australian dietary guidelines, just one burger can be 36% of the recommended daily energy intake for teenage boys aged 12–15 years and 40% of the recommendations for teenage girls 12–15 years.
A few hours to a few days after eating rich, heavy foods such as a burger, unpleasant symptoms like tiredness, poor sleep, and even hunger can result ( Figure 2 ). Rather than providing an energy boost, junk foods can lead to a lack of energy. For a short time, sugar (a type of carbohydrate) makes people feel energized, happy, and upbeat as it is used by the body for energy. However, refined sugar , which is the type of sugar commonly found in junk foods, leads to a quick drop in blood sugar levels because it is digested quickly by the body. This can lead tiredness and cravings [ 5 ].
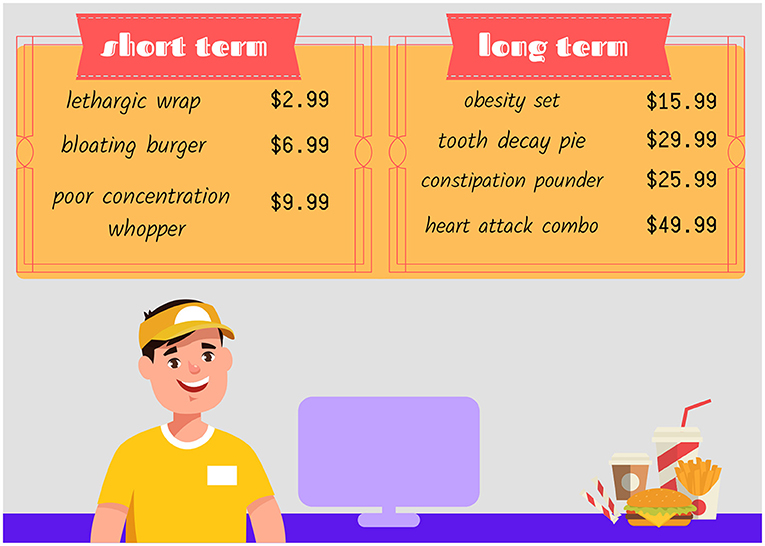
- Figure 2 - The short- and long-term impacts of junk food consumption.
- In the short-term, junk foods can make you feel tired, bloated, and unable to concentrate. Long-term, junk foods can lead to tooth decay and poor bowel habits. Junk foods can also lead to obesity and associated diseases such as heart disease. When junk foods are regularly consumed over long periods of time, the damages and complications to health are increasingly costly.
Fiber is a good carbohydrate commonly found in vegetables, fruits, barley, legumes, nuts, and seeds—foods from the five food groups. Fiber not only keeps the digestive system healthy, but also slows the stomach’s emptying process, keeping us feeling full for longer. Junk foods tend to lack fiber, so when we eat them, we notice decreasing energy and increasing hunger sooner.
Foods such as walnuts, berries, tuna, and green veggies can boost concentration levels. This is particularly important for young minds who are doing lots of schoolwork. These foods are what most elite athletes are eating! On the other hand, eating junk foods can lead to poor concentration. Eating junk foods can lead to swelling in the part of the brain that has a major role in memory. A study performed in humans showed that eating an unhealthy breakfast high in fat and sugar for 4 days in a row caused disruptions to the learning and memory parts of the brain [ 6 ].
Long-Term Impacts of Junk Foods
If we eat mostly junk foods over many weeks, months, or years, there can be several long-term impacts on health ( Figure 2 ). For example, high saturated fat intake is strongly linked with high levels of bad cholesterol in the blood, which can be a sign of heart disease. Respected research studies found that young people who eat only small amounts of saturated fat have lower total cholesterol levels [ 7 ].
Frequent consumption of junk foods can also increase the risk of diseases such as hypertension and stroke. Hypertension is also known as high blood pressure and a stroke is damage to the brain from reduced blood supply, which prevents the brain from receiving the oxygen and nutrients it needs to survive. Hypertension and stroke can occur because of the high amounts of cholesterol and salt in junk foods.
Furthermore, junk foods can trigger the “happy hormone,” dopamine , to be released in the brain, making us feel good when we eat these foods. This can lead us to wanting more junk food to get that same happy feeling again [ 8 ]. Other long-term effects of eating too much junk food include tooth decay and constipation. Soft drinks, for instance, can cause tooth decay due to high amounts of sugar and acid that can wear down the protective tooth enamel. Junk foods are typically low in fiber too, which has negative consequences for gut health in the long term. Fiber forms the bulk of our poop and without it, it can be hard to poop!
Tips for Being Healthy
One way to figure out whether a food is a junk food is to think about how processed it is. When we think of foods in their whole and original forms, like a fresh tomato, a grain of rice, or milk squeezed from a cow, we can then start to imagine how many steps are involved to transform that whole food into something that is ready-to-eat, tasty, convenient, and has a long shelf life.
For teenagers 13–14 years old, the recommended daily energy intake is 8,200–9,900 kJ/day or 1,960 kcal-2,370 kcal/day for boys and 7,400–8,200 kJ/day or 1,770–1,960 kcal for girls, according to the Australian dietary guidelines. Of course, the more physically active you are, the higher your energy needs. Remember that junk foods are okay to eat occasionally, but they should not make up more than 10% of your daily energy intake. In a day, this may be a simple treat such as a small muffin or a few squares of chocolate. On a weekly basis, this might mean no more than two fast-food meals per week. The remaining 90% of food eaten should be from the five food groups.
In conclusion, we know that junk foods are tasty, affordable, and convenient. This makes it hard to limit the amount of junk food we eat. However, if junk foods become a staple of our diets, there can be negative impacts on our health. We should aim for high-fiber foods such as whole grains, vegetables, and fruits; meals that have moderate amounts of sugar and salt; and calcium-rich and iron-rich foods. Healthy foods help to build strong bodies and brains. Limiting junk food intake can happen on an individual level, based on our food choices, or through government policies and health-promotion strategies. We need governments to stop junk food companies from advertising to young people, and we need their help to replace junk food restaurants with more healthy options. Researchers can focus on education and health promotion around healthy food options and can work with young people to develop solutions. If we all work together, we can help young people across the world to make food choices that will improve their short and long-term health.
Obesity : ↑ A disorder where too much body fat increases the risk of health problems.
Processed Food : ↑ A raw agricultural food that has undergone processes to be washed, ground, cleaned and/or cooked further.
Discretionary Food : ↑ Foods and drinks not necessary to provide the nutrients the body needs but that may add variety to a person’s diet (according to the Australian dietary guidelines).
Refined Sugar : ↑ Sugar that has been processed from raw sources such as sugar cane, sugar beets or corn.
Saturated Fat : ↑ A type of fat commonly eaten from animal sources such as beef, chicken and pork, which typically promotes the production of “bad” cholesterol in the body.
Dopamine : ↑ A hormone that is released when the brain is expecting a reward and is associated with activities that generate pleasure, such as eating or shopping.
Conflict of Interest
The authors declare that the research was conducted in the absence of any commercial or financial relationships that could be construed as a potential conflict of interest.
[1] ↑ Australian Bureau of Statistics. 2013. 4324.0.55.002 - Microdata: Australian Health Survey: Nutrition and Physical Activity, 2011-12 . Australian Bureau of Statistics. Available online at: http://bit.ly/2jkRRZO (accessed December 13, 2019).
[2] ↑ National Health and Medical Research Council. 2013. Australian Dietary Guidelines Summary . Canberra, ACT: National Health and Medical Research Council.
[3] ↑ Potvin Kent, M., and Pauzé, E. 2018. The frequency and healthfulness of food and beverages advertised on adolescents’ preferred web sites in Canada. J. Adolesc. Health. 63:102–7. doi: 10.1016/j.jadohealth.2018.01.007
[4] ↑ Tan, L., Ng, S. H., Omar, A., and Karupaiah, T. 2018. What’s on YouTube? A case study on food and beverage advertising in videos targeted at children on social media. Child Obes. 14:280–90. doi: 10.1089/chi.2018.0037
[5] ↑ Gómez-Pinilla, F. 2008. Brain foods: the effects of nutrients on brain function. Nat. Rev. Neurosci. 9, 568–78. doi: 10.1038/nrn2421
[6] ↑ Attuquayefio, T., Stevenson, R. J., Oaten, M. J., and Francis, H. M. 2017. A four-day western-style dietary intervention causes reductions in hippocampal-dependent learning and memory and interoceptive sensitivity. PLoS ONE . 12:e0172645. doi: 10.1371/journal.pone.0172645
[7] ↑ Te Morenga, L., and Montez, J. 2017. Health effects of saturated and trans-fatty acid intake in children and adolescents: systematic review and meta-analysis. PLoS ONE. 12:e0186672. doi: 10.1371/journal.pone.0186672
[8] ↑ Reichelt, A. C. 2016. Adolescent maturational transitions in the prefrontal cortex and dopamine signaling as a risk factor for the development of obesity and high fat/high sugar diet induced cognitive deficits. Front. Behav. Neurosci. 10. doi: 10.3389/fnbeh.2016.00189
Log in using your username and password
- Search More Search for this keyword Advanced search
- Latest content
- BMJ NPH Collections
- BMJ Journals More You are viewing from: Google Indexer
You are here
- Online First
- Food environment and obesity: a systematic review and meta-analysis
- Article Text
- Article info
- Citation Tools
- Rapid Responses
- Article metrics

- http://orcid.org/0000-0002-5210-1538 Elisa Pineda 1 , 2 ,
- Jemima Stockton 3 ,
- Shaun Scholes 3 ,
- Camille Lassale 4 , 5 and
- Jennifer S Mindell 3
- 1 The George Institute for Global Health UK , Imperial College London , London , UK
- 2 School of Public Health , Imperial College London , London , UK
- 3 Research Department of Epidemiology and Public Health , University College London , London , UK
- 4 Barcelona Institute for Global Health (ISGlobal) , Barcelona , Spain
- 5 CIBER Physiopathology of Obesity and Nutrition (CIBEROBN) , Carlos III Health Institute (ISCIII) , Madrid , Spain
- Correspondence to Dr Elisa Pineda; e.pineda{at}imperial.ac.uk
Background Obesity is influenced by a complex, multifaceted system of determinants, including the food environment. Governments need evidence to act on improving the food environment. The aim of this study was to review the evidence from spatial environmental analyses and to conduct the first series of meta-analyses to assess the impact of the retail food environment on obesity.
Methods We performed a systematic review and random-effects meta-analyses, focusing on geographical–statistical methods to assess the associations between food outlet availability and obesity. We searched OvidSP-Medline, Scielo, Scopus and Google Scholar databases up to January 2022. The search terms included spatial analysis, obesity and the retail food environment. Effect sizes were pooled by random-effects meta-analyses separately according to food outlet type and geographical and statistical measures.
Findings Of the 4118 retrieved papers, we included 103 studies. Density (n=52, 50%) and linear and logistic regressions (n=68, 66%) were the main measures used to assess the association of the food environment with obesity. Multilevel or autocorrelation analyses were used in 35 (34%) studies. Fast-food outlet proximity was positively and significantly associated with obesity (OR: 1.15, 95% CI: 1.02 to 1.30, p=0.02). Fresh fruit and vegetable outlet density and supermarket proximity were inversely associated with obesity (OR: 0.93, 95% CI: 0.90 to 0.96, p<0.001; OR: 0.90, 95% CI: 0.82 to 0.98, p=0.02). No significant associations were found for restaurants, convenience stores or any of the body mass index measures.
Conclusions Food outlets which sell mostly unhealthy and ultra-processed foods were associated with higher levels of obesity, while fruit and vegetable availability and supermarket accessibility, which enable healthier food access, were related to lower levels of obesity. The regulation of food outlets through zoning laws may not be enough to tackle the burden of obesity. Regulations that focus on increasing the availability of healthy food within stores and ensure overall healthy food environments require further attention.
PROSPERO registration number CRD42018111652.
- Malnutrition
Data availability statement
All data relevant to the study are included in the article or uploaded as supplementary information.
This is an open access article distributed in accordance with the Creative Commons Attribution 4.0 Unported (CC BY 4.0) license, which permits others to copy, redistribute, remix, transform and build upon this work for any purpose, provided the original work is properly cited, a link to the licence is given, and indication of whether changes were made. See: https://creativecommons.org/licenses/by/4.0/ .
https://doi.org/10.1136/bmjnph-2023-000663
Statistics from Altmetric.com
Request permissions.
If you wish to reuse any or all of this article please use the link below which will take you to the Copyright Clearance Center’s RightsLink service. You will be able to get a quick price and instant permission to reuse the content in many different ways.
WHAT IS ALREADY KNOWN ON THIS TOPIC
The food environment is a recognised key determinant for the prevention of obesity and other diet-related non-communicable diseases (NCDs). Multiple studies have identified inconsistent findings regarding the association between elements of the retail food environment and obesity. Variability in geographical and analytical methods has been pointed out as a potential cause for these discrepancies.
WHAT THIS STUDY ADDS
This systematic literature review and meta-analyses consolidates all the evidence and effect sizes to determine which elements of the retail food environment have the greatest impact on obesity. It stratigically considers elements of the retail food environment, along with geographical and statistical methods to provide increased statistical power, accuracy, and a comprehensive summary of findings regarding the association of the food environment with obesity.
HOW THIS STUDY MIGHT AFFECT RESEARCH, PRACTICE OR POLICY
The evidence generated from this systematic review and meta-analyses can serve as a foundational tool for policymakers and researchers in developing programmes and interventions for the prevention of obesity and other diet-related NCDs. This study offers a quantitative and visual guide for identifying the retail food environment elements that require greater focus in strategies aimed at tackling obesity.
Introduction
The retail food environment and obesity.
Obesity, a critical risk factor for non-communicable diseases (NCDs), is prevalent in countries across all income levels, including low-, middle- and high-income nations. 1 2 Its prevalence is shaped by a complex array of determinants, notably the retail food environment and advertising landscapes. 3 Modern food environments are marked by the widespread availability and promotion of energy-dense, nutrient-poor foods. 4 For instance, the increase in food retailers has contributed to a significant rise in calorie availability, facilitating greater access to a wide array of food choices. 5 To combat structural overconsumption and curb the obesity epidemic, policy interventions must be enacted, even in the face of commercial interests. However, the specific influence of food environments on obesity, as distinct from individual behaviour, remains poorly defined. 6 7 There is a scarcity of evidence identifying the exact elements of food environments that contribute to obesity and could be targeted for change. 3 4 8 This review aims to enhance understanding of the analytical methods required to dissect the various components of the modern retail food environment in relation to obesity and to assess the impact of retail food environments on obesity levels.
Analysing the retail food environment
Spatial analysis, leveraging Geographic Information Systems (GIS), has become instrumental in exploring the interplay between the environment and health outcomes. It particularly aids in investigating the food environment by mapping the locations of food stores, examining their spatial distribution and assessing their impact on obesity and population health. This approach enables the study of how the proximity and density of food outlets relative to residential areas influence access to healthy versus unhealthy food options, thereby identifying key environmental factors and protective measures against obesity through spatial patterns. 9–12
Previous literature reviews
Previous literature reviews on the relationship between the retail food environment and obesity have underscored methodological issues that may affect the analysis and interpretation of how food environments influence health and dietary outcomes. There is a recognised need for precise, comprehensive evaluations, including standardised and validated measurement techniques and diverse approaches to assessing the retail food environment, as current methods exhibit considerable variability. 12–14 Essential aspects of retail food environment research involve confirming the location and type of food outlets through store audits (ground truthing), 13 considering the confounding effects of socioeconomic status 14 15 and using longitudinal studies to observe changes in the retail food environment and dietary choices over time. 15 16
Despite numerous studies investigating the retail food environment’s impact on obesity, systematic reviews and meta-analyses are scarce. 17–20 Previous analyses have often been restricted to specific regions or populations, with limited attention to the methodologies for measuring the retail food environment. 17–20 This paper undertakes a systematic review and meta-analyses to synthesise available evidence on the retail food environment’s role in obesity and diet-related NCDs, aiming to pinpoint elements that could be targeted by policy interventions. Furthermore, it critically assesses the methodological strategies used to study the global impact of the retail food environment on obesity.
Obesity and the food environment
The food environment encompasses physical, economic, political and sociocultural factors affecting dietary choices. 21 Glanz et al. ’s 22 model suggests that dietary intake is shaped by policy, environment, individual and behavioural factors. This includes the community nutrition environment (types of food stores, locations, and availability), which in this study we refer to as the 'retail food environment'; organisational settings (neighbourhood, school, workplace); and consumer aspects (food availability, placement, pricing, promotions, nutrition labelling). Key attributes defining the food environment are geographical access, availability, affordability and advertising. 23–25 While various factors contribute to obesity, environmental and policy measures can significantly improve the food environment, leading to widespread dietary changes and reduced obesity and disease rates. 26
We performed a systematic review and meta-analyses to assess the association of the retail food environment with adult obesity and to evaluate the geographical and statistical methods used. PRISMA (Preferred Reporting Items for Systematic Reviews and Meta-Analyses) guidelines were followed ( online supplemental figure S1 ). Search results were screened by two reviewers for eligibility. The review was registered in PROSPERO as CRD42018111652.
Supplemental material
Literature search strategy.
We conducted a literature search on 31 January 2022, spanning papers published from 1946 onwards, to identify studies focusing on the impact of the retail food environment on obesity through spatial analysis. Using OvidSP-Medline, Scopus and Google Scholar databases, we structured the search around three primary themes: the retail food environment, obesity and spatial analysis. Initially, each theme was explored individually, and subsequently, we employed the ‘AND’ operator to search them concurrently. Using the Population, Intervention, Control, Outcome (PICO) framework ( online supplemental table S1 ) for eligibility assessment, 27 we considered publications examining the influence of the retail food environment on adult obesity or body mass index (BMI) for inclusion in our systematic literature review and meta-analyses.
Our literature search strategy involved MeSH words, Boolean search terms and proximity searching characters ($, *, W, #) on Medline (OvidSP, 1946–current: 31 January 2022). The terms covered diverse aspects such as buffer, chain, convenience, density variations (denoted by densit*), desert, distance, eating habits (indicated by eat$), environmental factors, farmers’ markets, fast food, geography, geolocation, geospatial analysis, GIS (geographic information systems), global, grocery stores, increase, index, location, markets, access, provision, proximity, restaurants, retail, spatial considerations, stores, supermarkets, supply, BMI (body mass index), body mass, nutrition, obesity, overweight, positional factors, weight gain and overeating. Additionally, the search extended to Scopus and Google Scholar using the query “(ALL (obesity) AND ALL (food environment OR convenience store OR food retail) AND ALL (GIS OR spatial analysis OR geographic information systems))” as of 31 January 2022.
Risk of bias and quality assessment criteria
Risk of bias and quality were evaluated using a weighted quality score derived from the Cochrane risk-of-bias tool, the systematic review data collection procedures from The Guide to Community Preventive Services 28 and the food environment quality assessment by Williams et al . 29 Nine criteria were assessed: population representativeness, outcome validity, exposure representativeness, exposure source, retail food environment assessment method, physical activity assessment, study design, statistical methods and data temporality. Studies received one point for each criterion met ( online supplemental table S2 ).
Spatial and statistical methods and study design appraisal
Study design, statistical methods and models were explored and assessed according to their consideration of spatial clustering, 30 and according to their inclusion of confounders.
Meta-analysis
We performed random-effect meta-analyses to explore the link between the retail food environment and obesity, analysing data from various outlets including fast-food restaurants, convenience stores, supermarkets and farmers’ markets. We evaluated the retail food environment using density, proximity and the Retail Food Environment Index (RFEI)—the ratio of unhealthy to healthy food outlets. Our analyses focused on ORs for categorical outcomes and beta-coefficients (β) for continuous variables, combining similar measures for meta-analyses. We assessed the impact of the retail food environment on adult BMI (β) and obesity prevalence (ORs), selecting the most relevant estimate from studies providing multiple results to ensure observations remained independent. Only models adjusted for confounders were included. For comparability, we considered data within 1 mile buffers or equivalent, representing walkable distances. In longitudinal studies, the most recent data were used. When results were stratified by sex and socioeconomic position (SEP), we chose observations based on the largest sample size or prioritised women and low-income groups if sizes were equal. We reported effect sizes and 95% CIs for each study, using Stata V.16.0 for all statistical analyses. 31
We retrieved 4118 studies, and after applying inclusion and exclusion criteria, retained 103 articles yielding 526 data points ( online supplemental figure S1 ). These were categorised by statistical measure, geographical measure and food outlet type, with 437 data points used in meta-analyses and meta-regression. The analysis covered 16 countries, with 90% of the studies from high-income countries: 1 from Africa, 5 each from Asia, Latin America and Australia, 14 from Europe and 74 from North America, spanning from 2004 to 2021, predominantly between 2011 and 2017 (n=54, 52%) ( online supplemental table S3 ).
In terms of retail food environment measures, 52 (50%) studies evaluated density, 21 (20%) proximity, 3 (3%) both, 4 (4%) the RFEI or variants and 15 (15%) other measures like ratio and diversity. Most studies (n=77, 75%) assessed one geographical measure, 20 (19%) evaluated two and six (6%) assessed up to three. From the 526 data points that were extracted from all studies, fast-food outlets were the most examined (n=166, 32%), followed by supermarkets (n=102, 19%), restaurants (n=101, 19%) and convenience stores (n=61, 12%), fresh fruit and vegetable stores (n=17, 3%), grocery stores (n=14, 3%), specialty stores (n=8, 2%), supercentres (n=5, 1%), and farmers’ markets (n=4, 1%). A majority of the studies, 61% (n=63), accounted for walkability or physical activity as a confounder ( online supplemental table S4 ).
Associations varied by geographical area, underscoring the need for representative geographical selection. For example, Fan et al 32 found different associations between restaurants and obesity for men at the census tract level and for women at the block level. However, 64% (n=66) of studies did not perform ground truthing or verify retail food environment data ( online supplemental table S4 ).
Statistical and geographical methods
Of the studies analysed, 68 (66%) applied linear or logistic regression, while 35 (34%) used multilevel modelling or methods accounting for spatial factors and clustering ( online supplemental table S3 ). In terms of data sources for food outlet locations, 39 (38%) used government databases, 27 (26%) commercial databases, 14 (14%) conducted ground truthing, 23 (22%) employed various methods and 1 (1%) did not disclose their source. Among the studies employing multilevel modelling or spatial considerations, 26 (74%) identified positive correlations between the presence of food retailers selling foods high in fat, sugar and salt (HFSS) and obesity rates ( online supplemental table S3 ).
Study design
Of the 89 cross-sectional studies analysed, 59 (66%) discovered a correlation between obesity and food retailers specialising in unhealthy foods and beverages, such as convenience stores and fast-food outlets. Among the 14 longitudinal studies, half revealed a significant link between the presence of unhealthy food outlets and obesity (refer to online supplemental tables S3 and S4 for detailed findings).
Quality and bias assessment of studies
The mean quality score of the studies was low, at 4 out of 9 points, with the highest being 7. 33 34 Key limitations included the reliance on cross-sectional designs, the failure to account for clustering or to apply spatial methods in 30 (29%) studies, reliance on self-reported height and weight data in 34 (33%) studies and the use of inappropriate statistical methods in 43 (42%) studies ( online supplemental table S5 ). Studies deemed to have a high risk of bias were excluded from the meta-analyses.
In the meta-analyses conducted, significant heterogeneity was observed across the studies, stemming from variations in statistical methods, study designs, stratification by gender and ethnicity, geographical measures of the retail food environment, classifications of food outlets and the definitions used to measure or define obesity, thereby limiting the robustness of the pooled analyses. Despite these variances, the majority of the studies used BMI, derived from measured height and weight, as a primary indicator, reporting it either as a continuous variable (kg/m 2 ) or in categorical terms (overweight or obesity). However, there was a notable scarcity of studies disaggregating outcome data by critical demographic factors such as age group, gender, ethnicity or SEP, which is pivotal considering the diverse exposure to retail food environments experienced by these groups. 35 Results of the meta-analyses are presented below by measure of the retail food environment (ie, density and proximity) and statistical measures (ORs and Beta-coefficients─in the supplemental material).
The findings revealed that the density of fast-food outlets did not significantly influence obesity rates (OR: 1.01, 95% CI: 0.99 to 1.04, p=0.18), in contrast to proximity to fast-food outlets, which showed a significant association with obesity (OR: 1.15, 95% CI: 1.02 to 1.30, p=0.02) ( figure 1 ). Restaurant density’s correlation with obesity was marginally significant (OR: 0.92, 95% CI: 0.85 to 1.00, p=0.05), yet the literature lacked sufficient data to evaluate the impact of restaurant proximity ( figure 2 ). No significant relationship was identified between the density of convenience stores and obesity (OR: 1.02, 95% CI: 0.95 to 1.10, p=0.64), and a similar non-significant trend was observed for proximity to convenience stores (OR: 1.04, 95% CI: 0.97 to 1.11, p=0.31) ( figure 3 ).
- Download figure
- Open in new tab
- Download powerpoint
Fast-food outlet density and proximity and its association with obesity. REML, Restricted Maximum Likelihood.
Restaurant density and its association with obesity. REML, Restricted Maximum Likelihood.
Convenience store density and proximity and its association with obesity. REML, Restricted Maximum Likelihood.
Furthermore, supermarket density did not show a significant relationship with obesity (OR: 0.98, 95% CI: 0.92 to 1.05, p=0.53), whereas a significant inverse relationship was evident between supermarket proximity and obesity (OR: 0.90, 95% CI: 0.82 to 0.98, p=0.02) ( figure 4 ). An inverse association was also noted between the density of fresh fruit and vegetable stores and obesity (OR: 0.93, 95% CI: 0.90 to 0.96, p<0.001) ( figure 5 ), though data were insufficient to assess the impact of proximity to these outlets. The RFEI did not reveal any significant associations with obesity (OR: 1.00, 95% CI: 0.99 to 1.01, p=0.99) ( figure 6 ), and BMI as a continuous variable showed no association with any type of food outlet, indicating a nuanced and complex relationship between the retail food environment and obesity ( online supplemental figures S2–S7 ).
Supermarket density and proximity and its association with obesity. REML, Restricted Maximum Likelihood.
Fruit and vegetable store density and its association with obesity. REML, Restricted Maximum Likelihood.
Retail Food Environment Index (RFEI) and its association with obesity. REML, Restricted Maximum Likelihood.
The results of our systematic review and meta-analyses indicate a nuanced relationship between the retail food environment and obesity. Results for the association between the retail food environment and obesity varied significantly by type of food outlet, statistical measure and geographical measure. However, the pooled effect sizes show that proximity of fast-food outlets was associated with a higher risk of obesity, while proximity of supermarkets and fresh fruit and vegetable stores was associated with a lower risk of obesity.
Previous research highlights the crucial role of fruit and vegetable availability and affordability in fostering healthy eating habits and preventing obesity and chronic diseases. 36 Conversely, fast-food outlets predominantly offer ultra-processed foods—industrially processed items rich in fat, salt and/or sugar—whose consumption is associated with increased risks of obesity and chronic conditions. 37
The observed phenomenon can be attributed to the ease of access to different types of food outlets and their impact on dietary choices. Fast-food outlets, often closer to residential areas or on the pathways from school or the office to home, provide convenient access to high-calorie, processed foods, which can contribute to higher obesity rates among nearby residents. 14 Conversely, supermarkets, which are sometimes located further from residential areas, offer a broader range of healthier food options. When supermarkets are closer, it encourages the purchase and consumption of healthier foods, potentially reducing obesity risk. 38 This highlights the significant role of the retail food environment accessibility in influencing dietary behaviours and obesity prevalence.
In addition, socioeconomic area level may play a critical role in this context by influencing both access to and choices within the retail food environment. 39 Individuals living in lower socioeconomic areas may have more limited access to supermarkets offering a variety of healthy options due to cost or proximity, leading to a reliance on closer, often less expensive fast-food outlets. 39 This disparity can result in dietary patterns that contribute to higher obesity rates in these populations, underscoring the need for targeted interventions to improve access to healthy food options across all socioeconomic groups.
Importantly, while geographical measures such as proximity and density provide insights into the retail food environment or built food environment, they do not capture the complexities within food outlets that influence consumer choices. The 'in-store food environment', encompassing product placement, promotion strategies and food layout, plays a pivotal role in shaping dietary habits. Studies have demonstrated that strategic placement of healthy food options at eye level or in prominent store locations can significantly influence consumer purchases towards healthier choices. 40–43
A comprehensive approach, addressing both the proximity of various food outlet types and the intricate details of the in-store food environment, is essential for devising effective public health interventions aimed at reducing obesity. Future research and policy efforts should consider these dimensions of the food environment to develop more nuanced and impactful strategies for obesity prevention.
The UK is a pioneer in regulating the food environment, having introduced legislation to restrict the promotion and placement of HFSS foods within retail settings, both online and physical. 44 This legislation targets the influence of food retailers on consumer choices, particularly aiming to reduce the impact of price promotions on children’s food preferences by limiting promotions and strategic placement of HFSS products. This is a crucial step in promoting healthier eating habits and combating obesity and related health issues.
Additionally, in high-income countries, zoning powers allow local authorities to regulate food outlets’ location, and healthy food carts have been effectively deployed in urban areas to increase access to nutritious food. 18
Studies on the food environment can inform the creation of improved land use and public health policies, mitigating the negative effects of local food and nutrition environments on population health 45 Effective obesity reduction efforts should include policies or regulations to limit the availability of low-quality food in neighbourhoods, schools and other sensitive areas. However, the relationship between food outlets and obesity has shown inconsistent results, underscoring the need for solid evidence to guide government actions on enhancing the food environment.
This research significantly advances the evidence 18–20 by integrating a systematic review with meta-analyses to explore the retail food environment’s influence on obesity and BMI. This dual approach, not previously used for this topic, integrates geographical and statistical analyses and offers a comprehensive analysis of the relationship between food outlet types, BMI and obesity. Furthermore, this study is distinct as it includes analyses that employ spatial methodologies to explore the retail food environment’s components and their correlation with obesity, providing a comprehensive evidence base for policy formulation aimed at enhancing public health.
Implications for policymakers and urban planners
The observed association between fast-food outlet proximity and increased obesity risk emphasises the need for zoning regulations to manage their density in residential areas, schools and communal spaces. This strategic intervention becomes crucial in mitigating the obesity crisis. Our study discerns variations in associations among different food outlet types. While proximity of fast-food outlets correlates positively with obesity, proximity of supermarkets and fresh produce stores demonstrates an inverse relationship. Urban planners can influence health outcomes by strategically placing health-promoting outlets in residential areas, aligning with the concept of fostering a ‘healthy food environment’.
Beyond reaffirming existing knowledge, our study introduces novel insights into nuanced relationships between specific food outlets and obesity risk. Policymakers and urban planners can leverage this information to refine existing zoning laws based on prevalent food outlet types.
Our analysis also reveals a gap in the assessment of in-store food environments. Policymakers should focus on internal dynamics, implementing regulations targeting the arrangement and promotion of food items within stores to encourage healthier choices. Moreover, they should engage with town planners, health professionals and community representatives to develop comprehensive strategies. Collaborative efforts can lead to urban spaces that limit the impact of detrimental food outlets and food choices while promoting health and well-being. This aligns with the broader goal of fostering healthier communities, emphasising the importance of continued research and dialogue between academia and policymakers.
Strengths and limitations
This study’s primary strength lies in its comprehensive systematic search strategy, which involved querying multiple databases, imposing no publication date restrictions and conducting searches in two languages. Additionally, it uniquely explored and assessed geographical measures and statistical methods within a systematic literature review context and conducted a risk-of-bias assessment to objectively evaluate the reviewed literature.
By incorporating spatial analysis, this study addressed gaps in previous literature by elucidating the impact of food outlets’ geographical distribution on obesity rates. This approach enabled the identification of spatial patterns and correlations potentially overlooked in traditional epidemiological studies, thereby providing insight into the obesogenic environment.
Spatial analysis also enhanced the meta-analyses by facilitating the integration and comparison of findings from studies across different geographical scales and settings, thereby bolstering the robustness of our conclusions. This rigour in methodology supported evidence synthesis, offering a detailed overview of the retail food environment’s role in obesity.
Through a detailed spatial analysis, our study not only corroborates the significance of geographical factors in obesity prevalence but also underscores the need for targeted public health interventions. By pinpointing areas with high concentrations of unhealthy food outlets relative to healthy ones, policymakers and urban planners can devise more effective strategies aimed at improving the food environment and, subsequently, public health.
However, the study has limitations. The review focused on obesity in the adult population because of the diverse reviews already focused on children, and because of the important role that adults play in food outlet selection within a family setting. Focusing on adult populations is critical for chronic disease prevention and successful ageing. Only studies based on neighbourhood, rural or urban environments were considered. Studies that did not include an objective measure of obesity such as BMI via measured height and weight were excluded. However, many studies that used BMI and other measures of diet and obesity were considered. The identified exposures, measures and outcomes included in this study were the most reported in the literature. Although this may exclude other important obesity-related outcomes (eg, adiposity, fat mass, diet), focusing on BMI and obesity allowed a wider comparison between studies and could facilitate translation into policies and actions to regulate and improve the food environment.
Despite significant methodological diversity among the studies reviewed, the literature consistently identifies the food environment as a crucial factor in preventing obesity. Regions characterised by abundant fast-food outlets, limited supermarket access and scarce fresh fruit and vegetable stores tend to have higher obesity rates. While regulating access to healthier food options is necessary, it may not suffice to combat obesity on its own. Comprehensive strategies are also needed, including regulation of the in-store availability of unhealthy foods and the promotion of a food environment that supports healthy and affordable diets.
Ethics statements
Patient consent for publication.
Not applicable.
Ethics approval
Acknowledgments.
The authors wish to acknowledge Dr Clare Llewelyn and Professor Eric Brunner for their guidance and support on this study.
- Boutayeb A ,
- Butland B ,
- Kopelman P , et al
- Geiger BM , et al
- Ritchie H ,
- Dubowitz T ,
- Bird CE , et al
- Jones NRV ,
- Conklin AI ,
- Suhrcke M , et al
- Cromley EK ,
- McLafferty SL
- Penney TL ,
- Rainham DGC ,
- Dummer TJB , et al
- Thornton LE ,
- Pearce JR ,
- Kavanagh AM
- Charreire H ,
- Salze P , et al
- Sorensen G ,
- Subramanian SV , et al
- Fraser LK ,
- Edwards KL ,
- Cade J , et al
- Dzewaltowski DA
- Rangan A , et al
- Westbury S ,
- Jones HM , et al
- Fuentes Pacheco A ,
- Carrillo Balam G ,
- Archibald D , et al
- Osei-Assibey G ,
- Macdiarmid J , et al
- Swinburn B ,
- Vandevijvere S , et al
- Sallis JF ,
- Saelens BE , et al
- Office of Nutrition Policy and Promotion HC
- Hommel B , et al
- Excellence NIfHaC
- Wright-De Agüero LK ,
- Briss PA , et al
- Williams J ,
- Scarborough P ,
- Matthews A , et al
- Gleditsch KS
- Hanson HA ,
- Zick CD , et al
- Carroll SJ ,
- Taylor AW , et al
- Brunner EJ ,
- Llewellyn CH , et al
- Patton GC ,
- Sawyer SM ,
- Santelli JS , et al
- Alae-Carew C , et al
- Monteiro C ,
- Lawrence M , et al
- Michimi A ,
- Wimberly MC
- Ohri-Vachaspati P ,
- DeWeese RS ,
- Acciai F , et al
- Liberato SC ,
- Brimblecombe J
- McGowan CC ,
- Zenk SN , et al
- Morales KH , et al
- Department of Health & Social care
- Cardinal BJ , et al
Supplementary materials
Supplementary data.
This web only file has been produced by the BMJ Publishing Group from an electronic file supplied by the author(s) and has not been edited for content.
- Data supplement 1
X @elisap_ana
Contributors EP designed the study, collected and analysed the data, drafted the manuscript, and was responsible for the overall content as the guarantor. JS, SS, CL and JSM drafted and revised the draft and provided statistical advice.
Funding This study was funded by CONACYT, the National Council on Science and Technology in Mexico.
Competing interests None declared.
Provenance and peer review Not commissioned; externally peer reviewed.
Supplemental material This content has been supplied by the author(s). It has not been vetted by BMJ Publishing Group Limited (BMJ) and may not have been peer-reviewed. Any opinions or recommendations discussed are solely those of the author(s) and are not endorsed by BMJ. BMJ disclaims all liability and responsibility arising from any reliance placed on the content. Where the content includes any translated material, BMJ does not warrant the accuracy and reliability of the translations (including but not limited to local regulations, clinical guidelines, terminology, drug names and drug dosages), and is not responsible for any error and/or omissions arising from translation and adaptation or otherwise.
Read the full text or download the PDF:
Food and Diet
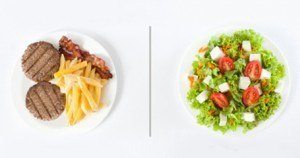
Beyond Willpower: Diet Quality and Quantity Matter
It’s no secret that the amount of calories people eat and drink has a direct impact on their weight: Consume the same number of calories that the body burns over time, and weight stays stable. Consume more than the body burns, weight goes up. Less, weight goes down. But what about the type of calories: Does it matter whether they come from specific nutrients-fat, protein, or carbohydrate? Specific foods-whole grains or potato chips? Specific diets-the Mediterranean diet or the “Twinkie” diet? And what about when or where people consume their calories: Does eating breakfast make it easier to control weight? Does eating at fast-food restaurants make it harder?
There’s ample research on foods and diet patterns that protect against heart disease, stroke, diabetes, and other chronic conditions. The good news is that many of the foods that help prevent disease also seem to help with weight control-foods like whole grains, vegetables, fruits, and nuts. And many of the foods that increase disease risk-chief among them, refined grains and sugary drinks-are also factors in weight gain.Conventional wisdom says that since a calorie is a calorie, regardless of its source, the best advice for weight control is simply to eat less and exercise more. Yet emerging research suggests that some foods and eating patterns may make it easier to keep calories in check, while others may make people more likely to overeat.
This article briefly reviews the research on dietary intake and weight control, highlighting diet strategies that also help prevent chronic disease.
Macronutrients and Weight: Do Carbs, Protein, or Fat Matter?
When people eat controlled diets in laboratory studies, the percentage of calories from fat, protein, and carbohydrate do not seem to matter for weight loss. In studies where people can freely choose what they eat, there may be some benefits to a higher protein, lower carbohydrate approach. For chronic disease prevention, though, the quality and food sources of these nutrients matters more than their relative quantity in the diet. And the latest research suggests that the same diet quality message applies for weight control.
Dietary Fat and Weight
Low-fat diets have long been touted as the key to a healthy weight and to good health. But the evidence just isn’t there: Over the past 30 years in the U.S., the percentage of calories from fat in people’s diets has gone down, but obesity rates have skyrocketed. ( 1 , 2 ) Carefully conducted clinical trials have found that following a low-fat diet does not make it any easier to lose weight than following a moderate- or high-fat diet. In fact, study volunteers who follow moderate- or high-fat diets lose just as much weight, and in some studies a bit more, as those who follow low-fat diets. ( 3 , 4 ) And when it comes to disease prevention, low-fat diets don’t appear to offer any special benefits. ( 5 )
Part of the problem with low-fat diets is that they are often high in carbohydrate, especially from rapidly digested sources, such as white bread and white rice. And diets high in such foods increase the risk of weight gain, diabetes, and heart disease. (See Carbohydrates and Weight , below.)
For good health, the type of fat people eat is far more important that the amount (see box), and there’s some evidence that the same may be true for weight control. ( 6 – 9 ) In the Nurses’ Health Study, for example, which followed 42,000 middle-age and older women for eight years, increased consumption of unhealthy fats-trans fats, especially, but also saturated fats-was linked to weight gain, but increased consumption of healthy fats-monounsaturated and polyunsaturated fat-was not. ( 6 )
Protein and Weight
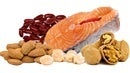
Higher protein diets seem to have some advantages for weight loss, though more so in short-term trials; in longer term studies, high-protein diets seem to perform equally well as other types of diets. ( 3 , 4 ) High-protein diets tend to be low in carbohydrate and high in fat, so it is difficult to tease apart the benefits of eating lots of protein from those of eating more fat or less carbohydrate. But there are a few reasons why eating a higher percentage of calories from protein may help with weight control:
- More satiety: People tend to feel fuller, on fewer calories, after eating protein than they do after eating carbohydrate or fat. ( 10 )
- Greater thermic effect: It takes more energy to metabolize and store protein than other macronutrients, and this may help people increase the energy they burn each day. ( 10 , 11 )
- Improved body composition: Protein seems to help people hang on to lean muscle during weight loss, and this, too, can help boost the energy-burned side of the energy balance equation. ( 11 )
Higher protein, lower carbohydrate diets improve blood lipid profiles and other metabolic markers, so they may help prevent heart disease and diabetes. ( 4 , 12 , 13 ) But some high-protein foods are healthier than others: High intakes of red meat and processed meat are associated with an increased risk of heart disease, diabetes, and colon cancer. ( 14 – 16 )
Replacing red and processed meat with nuts, beans, fish, or poultry seems to lower the risk of heart disease and diabetes. ( 14 , 16 ) And this diet strategy may help with weight control, too, according to a recent study from the Harvard School of Public Health. Researchers tracked the diet and lifestyle habits of 120,000 men and women for up to 20 years, looking at how small changes contributed to weight gain over time. ( 9 ) People who ate more red and processed meat over the course of the study gained more weight-about a pound extra every four years. People who ate more nuts over the course of the study gained less weight-about a half pound less every four years.
Carbohydrates and Weight
Lower carbohydrate, higher protein diets may have some weight loss advantages in the short term. ( 3 , 4 ) Yet when it comes to preventing weight gain and chronic disease, carbohydrate quality is much more important than carbohydrate quantity.
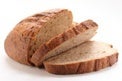
Read more about carbohydrates on The Nutrition Source
Milled, refined grains and the foods made with them-white rice, white bread, white pasta, processed breakfast cereals, and the like-are rich in rapidly digested carbohydrate. So are potatoes and sugary drinks. The scientific term for this is that they have a high glycemic index and glycemic load. Such foods cause fast and furious increases in blood sugar and insulin that, in the short term, can cause hunger to spike and can lead to overeating-and over the long term, increase the risk of weight gain, diabetes, and heart disease. ( 17 – 19 )
For example, in the diet and lifestyle change study, people who increased their consumption of French fries, potatoes and potato chips, sugary drinks, and refined grains gained more weight over time-an extra 3.4, 1.3, 1.0, and 0.6 pounds every four years, respectively. ( 9 ) People who decreased their intake of these foods gained less weight.
Specific Foods that Make It Easier or Harder to Control Weight
There’s growing evidence that specific food choices may help with weight control. The good news is that many of the foods that are beneficial for weight control also help prevent heart disease, diabetes, and other chronic diseases. Conversely, foods and drinks that contribute to weight gain—chief among them, refined grains and sugary drinks—also contribute to chronic disease.
Whole Grains, Fruits and Vegetables, and Weight
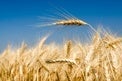
Read more about whole grains on The Nutrition Source
Whole grains-whole wheat, brown rice, barley, and the like, especially in their less-processed forms-are digested more slowly than refined grains. So they have a gentler effect on blood sugar and insulin, which may help keep hunger at bay. The same is true for most vegetables and fruits. These “slow carb” foods have bountiful benefits for disease prevention, and there’s also evidence that they can help prevent weight gain.
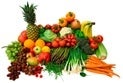
Read more about vegetables and fruits on The Nutrition Source
The weight control evidence is stronger for whole grains than it is for fruits and vegetables. ( 20 – 22 ) The most recent support comes from the Harvard School of Public Health diet and lifestyle change study: People who increased their intake of whole grains, whole fruits (not fruit juice), and vegetables over the course of the 20-year study gained less weight-0.4, 0.5, and 0.2 pounds less every four years, respectively. ( 9 )
Of course, the calories from whole grains, whole fruits, and vegetables don’t disappear. What’s likely happening is that when people increase their intake of these foods, they cut back on calories from other foods. Fiber may be responsible for these foods’ weight control benefits, since fiber slows digestion, helping to curb hunger. Fruits and vegetables are also high in water, which may help people feel fuller on fewer calories.
Nuts and Weight
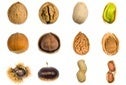
Read more about nuts on The Nutrition Source
Nuts pack a lot of calories into a small package and are high in fat, so they were once considered taboo for dieters. As it turns out, studies find that eating nuts does not lead to weight gain and may instead help with weight control, perhaps because nuts are rich in protein and fiber, both of which may help people feel fuller and less hungry. ( 9 , 23 – 25 ) People who regularly eat nuts are less likely to have heart attacks or die from heart disease than those who rarely eat them, which is another reason to include nuts in a healthy diet. ( 19 )
Dairy and Weight
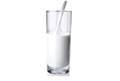
Read more about calcium and milk on The Nutrition Source
The U.S. dairy industry has aggressively promoted the weight-loss benefits of milk and other dairy products, based largely on findings from short-term studies it has funded. ( 26 , 27 ) But a recent review of nearly 50 randomized trials finds little evidence that high dairy or calcium intakes help with weight loss. ( 28 ) Similarly, most long-term follow-up studies have not found that dairy or calcium protect against weight gain, ( 29 – 32 ) and one study in adolescents found high milk intakes to be associated with increased body mass index. ( 33 )
One exception is the recent dietary and lifestyle change study from the Harvard School of Public Health, which found that people who increased their yogurt intake gained less weight; increases in milk and cheese intake, however, did not appear to promote weight loss or gain. ( 9 ) It’s possible that the beneficial bacteria in yogurt may influence weight control, but more research is needed.
Sugar-Sweetened Beverages and Weight
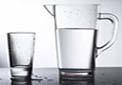
Read more about healthy drinks on The Nutrition Source
There’s convincing evidence that sugary drinks increase the risk of weight gain, obesity, and diabetes: ( 34 – 36 ) A systematic review and meta-analysis of 88 studies found “clear associations of soft drink intake with increased caloric intake and body weight.” ( 34 ) In children and adolescents, a more recent meta analysis estimates that for every additional 12-ounce serving of sugary beverage consumed each day, body mass index increases by 0.08 units. ( 35 ) Another meta analysis finds that adults who regularly drink sugared beverages have a 26 percent higher risk of developing type 2 diabetes than people who rarely drink sugared beverages. ( 36 ) Emerging evidence also suggests that high sugary beverage intake increases the risk of heart disease. ( 37 )
Like refined grains and potatoes, sugary beverages are high in rapidly-digested carbohydrate. (See Carbohydrates and Weight , above.) Research suggests that when that carbohydrate is delivered in liquid form, rather than solid form, it is not as satiating, and people don’t eat less to compensate for the extra calories. ( 38 )
These findings on sugary drinks are alarming, given that children and adults are drinking ever-larger quantities of them: In the U.S., sugared beverages made up about 4 percent of daily calorie intake in the 1970s, but by 2001, represented about 9 percent of calories. ( 36 ) The most recent data find that on any given day, half of Americans consume some type of sugared beverage, 25 percent consume at least 200 calories from sugared drinks, and 5 percent of consume at least 567 calories-the equivalent of four cans of sugary soda. ( 39 )
The good news is that studies in children and adults have also shown that cutting back on sugary drinks can lead to weight loss. ( 40 , 41 ) Sugary drinks have become an important target for obesity prevention efforts, prompting discussions of policy initiatives such as taxing soda. ( 42 )
Fruit Juice and Weight
It’s important to note that fruit juices are not a better option for weight control than sugar-sweetened beverages. Ounce for ounce, fruit juices-even those that are 100 percent fruit juice, with no added sugar- are as high in sugar and calories as sugary sodas. So it’s no surprise that a recent Harvard School of Public Health study, which tracked the diet and lifestyle habits of 120,000 men and women for up to 20 years, found that people who increased their intake of fruit juice gained more weight over time than people who did not. ( 9 ) Pediatricians and public health advocates recommend that children and adults limit fruit juice to just a small glass a day, if they consume it at all.
Alcohol and Weight
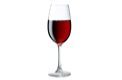
Read more about alcohol on The Nutrition Source
Even though most alcoholic beverages have more calories per ounce than sugar-sweetened beverages, there’s no clear-cut evidence that moderate drinking contributes to weight gain. While the recent diet and lifestyle change study found that people who increased their alcohol intake gained more weight over time, the findings varied by type of alcohol. ( 9 ) In most previous prospective studies, there was no difference in weight gain over time between light-to-moderate drinkers and nondrinkers, or the light-to-moderate drinkers gained less weight than nondrinkers. ( 43 – 47 )
Diet Patterns, Portion Size, and Weight
People don’t eat nutrients or foods in isolation. They eat meals that fall into an overall eating pattern, and researchers have begun exploring whether particular diet or meal patterns help with weight control or contribute to weight gain. Portion sizes have also increased dramatically over the past three decades, as has consumption of fast food-U.S. children, for example, consume a greater percentage of calories from fast food than they do from school food ( 48 )-and these trends are also thought to be contributors to the obesity epidemic.
Dietary Patterns and Weight
So-called “prudent” dietary patterns-diets that feature whole grains, vegetables, and fruits-seem to protect against weight gain, whereas “Western-style” dietary patterns-with more red meat or processed meat, sugared drinks, sweets, refined carbohydrates, or potatoes-have been linked to obesity. ( 49 – 52 ) The Western-style dietary pattern is also linked to increased risk of heart disease, diabetes, and other chronic conditions.
Following a Mediterranean-style diet, well-documented to protect against chronic disease, ( 53 ) appears to be promising for weight control, too. The traditional Mediterranean-style diet is higher in fat (about 40 percent of calories) than the typical American diet (34 percent of calories ( 54 )), but most of the fat comes from olive oil and other plant sources. The diet is also rich in fruits, vegetables, nuts, beans, and fish. A 2008 systematic review found that in most (but not all) studies, people who followed a Mediterranean-style diet had lower rates of obesity or more weight loss. ( 55 ) There is no single “Mediterranean” diet, however, and studies often use different definitions, so more research is needed.
Breakfast, Meal Frequency, Snacking, and Weight
There is some evidence that skipping breakfast increases the risk of weight gain and obesity, though the evidence is stronger in children, especially teens, than it is in adults. ( 56 ) Meal frequency and snacking have increased over the past 30 years in the U.S. ( 57 )-on average, children get 27 percent of their daily calories from snacks, primarily from desserts and sugary drinks, and increasingly from salty snacks and candy. But there have been conflicting findings on the relationship between meal frequency, snacking, and weight control, and more research is needed. ( 56 )
Portion Sizes and Weight
Since the 1970s, portion sizes have increased both for food eaten at home and for food eaten away from home, in adults and children. ( 58 , 59 ) Short-term studies clearly demonstrate that when people are served larger portions, they eat more. One study, for example, gave moviegoers containers of stale popcorn in either large or medium-sized buckets; people reported that they did not like the taste of the popcorn-and even so, those who received large containers ate about 30 percent more popcorn than those who received medium-sized containers. ( 60 ) Another study showed that people given larger beverages tended to drink significantly more, but did not decrease their subsequent food consumption . ( 67 ) An additional study provided evidence that when provided with larger portion sizes, people tended to eat more, with no decrease in later food intake. ( 68 ) There is an intuitive appeal to the idea that portion sizes increase obesity, but long-term prospective studies would help to strengthen this hypothesis.
Fast Food and Weight
Fast food is known for its large portions, low prices, high palatability, and high sugar content, and there’s evidence from studies in teens and adults that frequent fast-food consumption contributes to overeating and weight gain. ( 61 – 66 ) The CARDIA study, for example, followed 3,000 young adults for 13 years. People who had higher fast-food-intake levels at the start of the study weighed an average of about 13 pounds more than people who had the lowest fast-food-intake levels. They also had larger waist circumferences and greater increases in triglycercides, and double the odds of developing metabolic syndrome. ( 62 ) More research is needed to tease apart the effect of eating fast food itself from the effect of the neighborhood people live in, or other individual traits that may make people more likely to eat fast food.
The Bottom Line: Healthy Diet Can Prevent Weight Gain and Chronic Disease
Weight gain in adulthood is often gradual, about a pound a year ( 9 )-too slow of a gain for most people to notice, but one that can add up, over time, to a weighty personal and public health problem. There’s increasing evidence that the same healthful food choices and diet patterns that help prevent heart disease, diabetes, and other chronic conditions may also help to prevent weight gain:
- Choose minimally processed, whole foods-whole grains, vegetables, fruits, nuts, healthful sources of protein (fish, poultry, beans), and plant oils.
- Limit sugared beverages, refined grains, potatoes, red and processed meats, and other highly processed foods, such as fast food.
Though the contribution of any one diet change to weight control may be small, together, the changes could add up to a considerable effect, over time and across the whole society. ( 9 ) Since people’s food choices are shaped by their surroundings, it’s imperative for governments to promote policy and environmental changes that make healthy foods more accessible and decrease the availability and marketing of unhealthful foods.
1. Willett WC, Leibel RL. Dietary fat is not a major determinant of body fat. Am J Med . 2002;113 Suppl 9B:47S-59S.
2. Melanson EL, Astrup A, Donahoo WT. The relationship between dietary fat and fatty acid intake and body weight, diabetes, and the metabolic syndrome. Ann Nutr Metab . 2009;55:229-43.
3. Sacks FM, Bray GA, Carey VJ, et al. Comparison of weight-loss diets with different compositions of fat, protein, and carbohydrates. N Engl J Med . 2009;360:859-73.
4. Shai I, Schwarzfuchs D, Henkin Y, et al. Weight loss with a low-carbohydrate, Mediterranean, or low-fat diet. N Engl J Med . 2008;359:229-41.
5. Howard BV, Manson JE, Stefanick ML, et al. Low-fat dietary pattern and weight change over 7 years: the Women’s Health Initiative Dietary Modification Trial. JAMA . 2006;295:39-49.
6. Field AE, Willett WC, Lissner L, Colditz GA. Dietary fat and weight gain among women in the Nurses’ Health Study. Obesity (Silver Spring) . 2007;15:967-76.
7. Koh-Banerjee P, Chu NF, Spiegelman D, et al. Prospective study of the association of changes in dietary intake, physical activity, alcohol consumption, and smoking with 9-y gain in waist circumference among 16 587 US men. Am J Clin Nutr . 2003;78:719-27.
8. Thompson AK, Minihane AM, Williams CM. Trans fatty acids and weight gain. Int J Obes (Lond) . 2011;35:315-24.
9. Mozaffarian D, Hao T, Rimm EB, Willett WC, Hu FB. Changes in diet and lifestyle and long-term weight gain in women and men. N Engl J Med . 2011;364:2392-404.
10. Halton TL, Hu FB. The effects of high protein diets on thermogenesis, satiety and weight loss: a critical review. J Am Coll Nutr . 2004;23:373-85.
11. Westerterp-Plantenga MS, Nieuwenhuizen A, Tome D, Soenen S, Westerterp KR. Dietary protein, weight loss, and weight maintenance. Annu Rev Nutr . 2009;29:21-41.
12. Furtado JD, Campos H, Appel LJ, et al. Effect of protein, unsaturated fat, and carbohydrate intakes on plasma apolipoprotein B and VLDL and LDL containing apolipoprotein C-III: results from the OmniHeart Trial. Am J Clin Nutr . 2008;87:1623-30.
13. Appel LJ, Sacks FM, Carey VJ, et al. Effects of protein, monounsaturated fat, and carbohydrate intake on blood pressure and serum lipids: results of the OmniHeart randomized trial. JAMA . 2005;294:2455-64.
14. Bernstein AM, Sun Q, Hu FB, Stampfer MJ, Manson JE, Willett WC. Major dietary protein sources and risk of coronary heart disease in women. Circulation . 2010;122:876-83.
15. Aune D, Ursin G, Veierod MB. Meat consumption and the risk of type 2 diabetes: a systematic review and meta-analysis of cohort studies. Diabetologia . 2009;52:2277-87.
16. Pan A, Sun Q, Bernstein AM, et al. Red meat consumption and risk of type 2 diabetes: 3 cohorts of US adults and an updated meta-analysis. Am J Clin Nutr . 2011;94(4):1088-96.
17. Abete I, Astrup A, Martinez JA, Thorsdottir I, Zulet MA. Obesity and the metabolic syndrome: role of different dietary macronutrient distribution patterns and specific nutritional components on weight loss and maintenance. Nutr Rev . 2010;68:214-31.
18. Barclay AW, Petocz P, McMillan-Price J, et al. Glycemic index, glycemic load, and chronic disease risk—a meta-analysis of observational studies. Am J Clin Nutr . 2008;87:627-37.
19. Mente A, de Koning L, Shannon HS, Anand SS. A systematic review of the evidence supporting a causal link between dietary factors and coronary heart disease. Arch Intern Med . 2009;169:659-69.
20. Koh-Banerjee P, Franz M, Sampson L, et al. Changes in whole-grain, bran, and cereal fiber consumption in relation to 8-y weight gain among men. Am J Clin Nutr . 2004;80:1237-45.
21. Liu S, Willett WC, Manson JE, Hu FB, Rosner B, Colditz G. Relation between changes in intakes of dietary fiber and grain products and changes in weight and development of obesity among middle-aged women. Am J Clin Nutr . 2003;78:920-7.
22. Ledoux TA, Hingle MD, Baranowski T. Relationship of fruit and vegetable intake with adiposity: a systematic review. Obes Rev . 2011;12:e143-50.
23. Mattes RD, Kris-Etherton PM, Foster GD. Impact of peanuts and tree nuts on body weight and healthy weight loss in adults. J Nutr . 2008;138:1741S-5S.
24. Bes-Rastrollo M, Sabate J, Gomez-Gracia E, Alonso A, Martinez JA, Martinez-Gonzalez MA. Nut consumption and weight gain in a Mediterranean cohort: The SUN study. Obesity (Silver Spring) . 2007;15:107-16.
25. Bes-Rastrollo M, Wedick NM, Martinez-Gonzalez MA, Li TY, Sampson L, Hu FB. Prospective study of nut consumption, long-term weight change, and obesity risk in women. Am J Clin Nutr . 2009;89:1913-19.
26. Zemel MB, Shi H, Greer B, Dirienzo D, Zemel PC. Regulation of adiposity by dietary calcium. FASEB J . 2000;14:1132-8.
27. Zemel MB, Thompson W, Milstead A, Morris K, Campbell P. Calcium and dairy acceleration of weight and fat loss during energy restriction in obese adults. Obes Res . 2004;12:582-90.
28. Lanou AJ, Barnard ND. Dairy and weight loss hypothesis: an evaluation of the clinical trials. Nutr Rev . 2008;66:272-9.
29. Phillips SM, Bandini LG, Cyr H, Colclough-Douglas S, Naumova E, Must A. Dairy food consumption and body weight and fatness studied longitudinally over the adolescent period. Int J Obes Relat Metab Disord . 2003;27:1106-13.
30. Rajpathak SN, Rimm EB, Rosner B, Willett WC, Hu FB. Calcium and dairy intakes in relation to long-term weight gain in US men. Am J Clin Nutr . 2006;83:559-66.
31. Snijder MB, van Dam RM, Stehouwer CD, Hiddink GJ, Heine RJ, Dekker JM. A prospective study of dairy consumption in relation to changes in metabolic risk factors: the Hoorn Study. Obesity (Silver Spring) . 2008;16:706-9.
32. Boon N, Koppes LL, Saris WH, Van Mechelen W. The relation between calcium intake and body composition in a Dutch population: The Amsterdam Growth and Health Longitudinal Study. Am J Epidemiol . 2005;162:27-32.
33. Berkey CS, Rockett HR, Willett WC, Colditz GA. Milk, dairy fat, dietary calcium, and weight gain: a longitudinal study of adolescents. Arch Pediatr Adolesc Med . 2005;159:543-50.
34. Vartanian LR, Schwartz MB, Brownell KD. Effects of soft drink consumption on nutrition and health: a systematic review and meta-analysis. Am J Public Health . 2007;97:667-75.
35. Malik VS, Willett WC, Hu FB. Sugar-sweetened beverages and BMI in children and adolescents: reanalyses of a meta-analysis. Am J Clin Nutr . 2009;89:438-9; author reply 9-40.
36. Hu FB, Malik VS. Sugar-sweetened beverages and risk of obesity and type 2 diabetes: epidemiologic evidence. Physiol Behav . 2010;100:47-54.
37. Malik VS, Popkin BM, Bray GA, Despres JP, Willett WC, Hu FB. Sugar-sweetened beverages and risk of metabolic syndrome and type 2 diabetes: a meta-analysis. Diabetes Care . 2010;33:2477-83.
38. Pan A, Hu FB. Effects of carbohydrates on satiety: differences between liquid and solid food. Curr Opin Clin Nutr Metab Care . 2011;14:385-90.
39. Ogden CL KB, Carroll MD, Park S. Consumption of sugar drinks in the United States , 2005-2008. Hyattsville, MD: National Center for Health Statistics; 2011.
40. Chen L, Appel LJ, Loria C, et al. Reduction in consumption of sugar-sweetened beverages is associated with weight loss: the PREMIER trial. Am J Clin Nutr . 2009;89:1299-306.
41. Ebbeling CB, Feldman HA, Osganian SK, Chomitz VR, Ellenbogen SJ, Ludwig DS. Effects of decreasing sugar-sweetened beverage consumption on body weight in adolescents: a randomized, controlled pilot study. Pediatrics . 2006;117:673-80.
42. Brownell KD, Farley T, Willett WC, et al. The public health and economic benefits of taxing sugar-sweetened beverages. N Engl J Med . 2009;361:1599-605.
43. Wang L, Lee IM, Manson JE, Buring JE, Sesso HD. Alcohol consumption, weight gain, and risk of becoming overweight in middle-aged and older women. Arch Intern Med . 2010;170:453-61.
44. Liu S, Serdula MK, Williamson DF, Mokdad AH, Byers T. A prospective study of alcohol intake and change in body weight among US adults. Am J Epidemiol . 1994;140:912-20.
45. Wannamethee SG, Field AE, Colditz GA, Rimm EB. Alcohol intake and 8-year weight gain in women: a prospective study. Obes Res . 2004;12:1386-96.
46. Lewis CE, Smith DE, Wallace DD, Williams OD, Bild DE, Jacobs DR, Jr. Seven-year trends in body weight and associations with lifestyle and behavioral characteristics in black and white young adults: the CARDIA study. Am J Public Health . 1997;87:635-42.
47. Bes-Rastrollo M, Sanchez-Villegas A, Gomez-Gracia E, Martinez JA, Pajares RM, Martinez-Gonzalez MA. Predictors of weight gain in a Mediterranean cohort: the Seguimiento Universidad de Navarra Study 1. Am J Clin Nutr . 2006;83:362-70; quiz 94-5.
48. Poti JM, Popkin BM. Trends in Energy Intake among US Children by Eating Location and Food Source, 1977-2006. J Am Diet Assoc . 2011;111:1156-64.
49. Schulze MB, Fung TT, Manson JE, Willett WC, Hu FB. Dietary patterns and changes in body weight in women. Obesity (Silver Spring) . 2006;14:1444-53.
50. Newby PK, Muller D, Hallfrisch J, Andres R, Tucker KL. Food patterns measured by factor analysis and anthropometric changes in adults. Am J Clin Nutr . 2004;80:504-13.
51. Schulz M, Nothlings U, Hoffmann K, Bergmann MM, Boeing H. Identification of a food pattern characterized by high-fiber and low-fat food choices associated with low prospective weight change in the EPIC-Potsdam cohort. J Nutr . 2005;135:1183-9.
52. Newby PK, Muller D, Hallfrisch J, Qiao N, Andres R, Tucker KL. Dietary patterns and changes in body mass index and waist circumference in adults. Am J Clin Nutr . 2003;77:1417-25.
53. Sofi F, Abbate R, Gensini GF, Casini A. Accruing evidence on benefits of adherence to the Mediterranean diet on health: an updated systematic review and meta-analysis. Am J Clin Nutr . 2010;92:1189-96.
54. Wright JD WC-Y. Trends in intake of energy and macronutrients in adults from 1999-2000 through 2007-2008 . Hyattsville, MD: National Center for Health Statistics; 2010.
55. Buckland G, Bach A, Serra-Majem L. Obesity and the Mediterranean diet: a systematic review of observational and intervention studies. Obes Rev . 2008;9:582-93.
56. Dietary Guidelines for Americans Advisory Committee. Report of the DGAC on the Dietary Guidelines for Americans , 2010 ; 2010.
57. Popkin BM, Duffey KJ. Does hunger and satiety drive eating anymore? Increasing eating occasions and decreasing time between eating occasions in the United States. Am J Clin Nutr . 2010;91:1342-7.
58. Nielsen SJ, Popkin BM. Patterns and trends in food portion sizes, 1977-1998. JAMA . 2003;289:450-3.
59. Piernas C, Popkin BM. Food portion patterns and trends among U.S. children and the relationship to total eating occasion size, 1977-2006. J Nutr . 2011;141:1159-64.
60. Wansink B, Kim J. Bad popcorn in big buckets: portion size can influence intake as much as taste. J Nutr Educ Behav . 2005;37:242-5.
61. Duffey KJ, Gordon-Larsen P, Jacobs DR, Jr., Williams OD, Popkin BM. Differential associations of fast food and restaurant food consumption with 3-y change in body mass index: the Coronary Artery Risk Development in Young Adults Study. Am J Clin Nutr . 2007;85:201-8.
62. Duffey KJ, Gordon-Larsen P, Steffen LM, Jacobs DR, Jr., Popkin BM. Regular consumption from fast food establishments relative to other restaurants is differentially associated with metabolic outcomes in young adults. J Nutr . 2009;139:2113-8.
63. Taveras EM, Berkey CS, Rifas-Shiman SL, et al. Association of consumption of fried food away from home with body mass index and diet quality in older children and adolescents. Pediatrics . 2005;116:e518-24.
64. French SA, Harnack L, Jeffery RW. Fast food restaurant use among women in the Pound of Prevention study: dietary, behavioral and demographic correlates. Int J Obes Relat Metab Disord . 2000;24:1353-9.
65. Pereira MA, Kartashov AI, Ebbeling CB, et al. Fast-food habits, weight gain, and insulin resistance (the CARDIA study): 15-year prospective analysis. Lancet . 2005;365:36-42.
66. Rosenheck R. Fast food consumption and increased caloric intake: a systematic review of a trajectory towards weight gain and obesity risk. Obes Rev . 2008;9:535-47.
67. Rolls, B. J., L. S. Roe, et al. (2007). “The effect of large portion sizes on energy intake is sustained for 11 days.” Obesity (Silver Spring) 15(6): 1535-1543.
68. Ello-Martin, J. A., J. H. Ledikwe, et al. (2005). “The influence of food portion size and energy density on energy intake: implications for weight management.” Am J Clin Nutr 82(1 Suppl): 236S-241S.
- U.S. Department of Health & Human Services

- Virtual Tour
- Staff Directory
- En Español
You are here
Nih research matters.
January 28, 2013
Genes, Junk Food and Weight

Researchers gained new insight into how genetics may influence obesity by studying how the mouse equivalent of a fast-food diet affects different mouse strains. The findings may help explain why some people gain weight more easily than others.
Excess weight can raise your risk for type 2 and gestational diabetes, heart disease, cancer and other health problems. But maintaining weight is difficult for many people. Body weight reflects the balance between the amount of energy consumed and the amount the body uses. But the body’s metabolism can change as you lose weight and alter your exercise habits. These changes may differ significantly among people, depending on genetics, age and other factors. Recent evidence also suggests that gut microbes play a role in obesity.
Dr. Brian Parks and Dr. Aldons J. Lusis at the University of California, Los Angeles, set out to explore the factors affecting the body's response to a high-calorie diet in mice. They fed about 100 inbred strains of mice a normal chow diet until 8 weeks of age. For the following 8 weeks, they gave the mice a diet designed to represent a typical fast food diet, with 32% of calories from fat and 25% from sugar. The study was supported in part by NIH's National Heart, Lung and Blood Institute (NHLBI) and National Institute of Diabetes and Digestive and Kidney Diseases (NIDDK). Results appeared on January 8, 2013, in Cell Metabolism .
The team saw a wide range of body fat across the mouse strains even during the normal chow feeding stage. The response to 8 weeks of a high-fat, high-sugar diet also varied widely. Mice eating the “junk food” diet had increases in body fat ranging from none to more than 600% higher than mice who continued to eat a normal diet.
The researchers found that food intake correlated with body weight and lean mass. However, intake levels didn't account for the body fat changes seen with the high-fat, high-sugar diet. The investigators estimated that more than 70% of these body fat differences could be attributed to genetics.
To identify specific regions associated with obesity, the scientists performed a genome-wide analysis of about 100,000 genetic variations, or single nucleotide polymorphisms (SNPs). They found 11 regions that were associated with obesity. The regions contain several genes with known links to fat biology and metabolism in mice. Some have been linked to obesity in humans as well.
The researchers also analyzed gut microbe populations. They found that some mouse strains had large microbial shifts after eating the high-fat, high-sugar diet. Other strains of mice, however, showed little fluctuation. This finding shows that genetics strongly influences changes in gut microbes in response to diet.
“Our research demonstrates that body fat responses to high-fat, high-sugar diets have a very strong genetic component, and we have identified several genetic factors potentially regulating these responses,” Parks says. “Overall, our work has broad implications concerning the genetic nature of obesity and weight gain.”
The researchers now plan to explore the specific roles these genetic factors play in the interactions between diet and body weight.
— by Harrison Wein, Ph.D.
Related Links
- Predicting How Diet and Exercise Affect Weight
- Certain Foods Linked to Long-term Weight Gain
- Gut Microbiomes Differ Between Obese and Lean People
- Weight-control Information Network
- Overweight and Obesity
- Aim for a Healthy Weight
- Energy Balance: Weight and Obesity, Physical Activity, Diet
References: Cell Metab. 2013 Jan 8;17(1):141-52. doi: 10.1016/j.cmet.2012.12.007. PMID: 23312289.
Connect with Us
- More Social Media from NIH
Thank you for visiting nature.com. You are using a browser version with limited support for CSS. To obtain the best experience, we recommend you use a more up to date browser (or turn off compatibility mode in Internet Explorer). In the meantime, to ensure continued support, we are displaying the site without styles and JavaScript.
- View all journals
- My Account Login
- Explore content
- About the journal
- Publish with us
- Sign up for alerts
- Open access
- Published: 15 May 2024
GLP-1-directed NMDA receptor antagonism for obesity treatment
- Jonas Petersen ORCID: orcid.org/0000-0002-6219-0271 1 , 2 ,
- Mette Q. Ludwig ORCID: orcid.org/0000-0002-7814-3859 1 ,
- Vaida Juozaityte 1 ,
- Pablo Ranea-Robles ORCID: orcid.org/0000-0001-6478-3815 1 ,
- Charlotte Svendsen ORCID: orcid.org/0000-0002-8329-319X 1 ,
- Eunsang Hwang 3 ,
- Amalie W. Kristensen 2 ,
- Nicole Fadahunsi 1 ,
- Jens Lund ORCID: orcid.org/0000-0003-2338-6033 1 ,
- Alberte W. Breum ORCID: orcid.org/0000-0002-8399-4308 1 ,
- Cecilie V. Mathiesen ORCID: orcid.org/0000-0002-8325-5898 1 ,
- Luisa Sachs 1 ,
- Roger Moreno-Justicia ORCID: orcid.org/0000-0002-8587-4772 1 ,
- Rebecca Rohlfs 4 ,
- James C. Ford 4 ,
- Jonathan D. Douros 4 ,
- Brian Finan ORCID: orcid.org/0000-0002-6467-0447 4 ,
- Bryan Portillo 3 ,
- Kyle Grose 3 ,
- Jacob E. Petersen 1 ,
- Mette Trauelsen ORCID: orcid.org/0000-0002-6908-106X 1 ,
- Annette Feuchtinger 5 ,
- Richard D. DiMarchi ORCID: orcid.org/0000-0003-0220-4085 6 ,
- Thue W. Schwartz ORCID: orcid.org/0000-0002-0261-6904 1 ,
- Atul S. Deshmukh ORCID: orcid.org/0000-0002-2278-1843 1 ,
- Morten B. Thomsen 7 ,
- Kristi A. Kohlmeier ORCID: orcid.org/0000-0003-0183-3816 2 ,
- Kevin W. Williams 3 ,
- Tune H. Pers ORCID: orcid.org/0000-0003-0207-4831 1 ,
- Bente Frølund 2 ,
- Kristian Strømgaard 2 ,
- Anders B. Klein ORCID: orcid.org/0000-0002-0236-3089 1 &
- Christoffer Clemmensen ORCID: orcid.org/0000-0003-2456-9667 1
Nature ( 2024 ) Cite this article
34k Accesses
2 Citations
403 Altmetric
Metrics details
- Drug discovery and development
The N -methyl- d -aspartate (NMDA) receptor is a glutamate-activated cation channel that is critical to many processes in the brain. Genome-wide association studies suggest that glutamatergic neurotransmission and NMDA receptor-mediated synaptic plasticity are important for body weight homeostasis 1 . Here we report the engineering and preclinical development of a bimodal molecule that integrates NMDA receptor antagonism with glucagon-like peptide-1 (GLP-1) receptor agonism to effectively reverse obesity, hyperglycaemia and dyslipidaemia in rodent models of metabolic disease. GLP-1-directed delivery of the NMDA receptor antagonist MK-801 affects neuroplasticity in the hypothalamus and brainstem. Importantly, targeting of MK-801 to GLP-1 receptor-expressing brain regions circumvents adverse physiological and behavioural effects associated with MK-801 monotherapy. In summary, our approach demonstrates the feasibility of using peptide-mediated targeting to achieve cell-specific ionotropic receptor modulation and highlights the therapeutic potential of unimolecular mixed GLP-1 receptor agonism and NMDA receptor antagonism for safe and effective obesity treatment.
Similar content being viewed by others
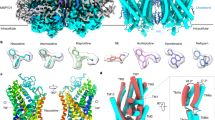
Dimerization and antidepressant recognition at noradrenaline transporter
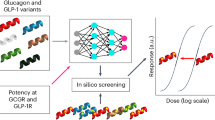
Machine learning designs new GCGR/GLP-1R dual agonists with enhanced biological potency
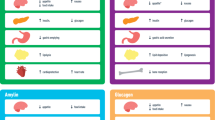
What is the pipeline for future medications for obesity?
Non-competitive, open-channel NMDA receptor blockers are used clinically for the management of Alzheimer’s disease and treatment-resistant depression 2 , 3 . It is believed that this class of small-molecule drugs improves brain disorders through mechanisms involving neurostructural changes and synaptic plasticity 4 . Notably, genome-wide association study (GWAS) analyses for body mass index (BMI) have linked glutamatergic signalling and NMDA receptor-related neuroplasticity to regulation of body weight and obesity 1 , 5 . In rodents, disparate effects of NMDA receptor antagonism on food intake have been observed. Specifically, antagonizing NMDA receptors in the brainstem has been associated with an increase in short-term food intake 6 , 7 ; by contrast, antagonizing NMDA receptors in the hypothalamus has been linked to a reduction in food intake and a decrease in body weight 8 . In the context of prolonged systemic administration, NMDA receptor antagonists, such as memantine and MK-801 (also known as dizocilpine), induce anorexia and weight loss in rodents 9 , 10 , 11 . Furthermore, these antagonists are reported to diminish palatable food preferences in rodents 12 and non-human primates 13 and to reduce binge-eating episodes in humans 14 .
Here we affirm the pharmacological potential of NMDA receptor channel blockers for obesity treatment (Extended Data Fig. 1a–p ). Once-daily subcutaneous (s.c.) injections of the potent NMDA receptor antagonist MK-801 in diet-induced obese (DIO) mice led to a dose-dependent decrease in food intake and body weight (Extended Data Fig. 1a–f ). MK-801 is widely used experimentally, but its clinical application is hampered by severe adverse effects. For example, chronic treatment with MK-801 promotes hyperthermia and hyperlocomotion 15 , 16 , 17 . Consistent with this, we demonstrate that treatment with MK-801, even at doses that do not evoke weight loss, elicits pronounced hyperthermia in DIO mice (Extended Data Fig. 1g–l ), emphasizing the unsuitability of MK-801 monotherapy for obesity treatment.
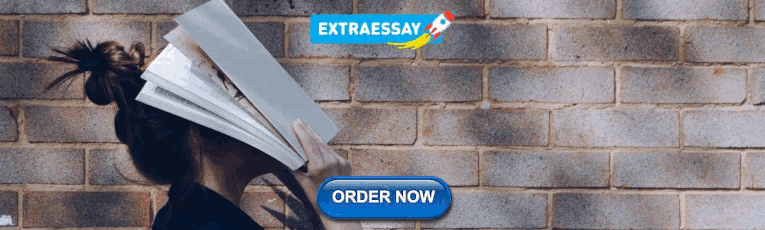
Development of GLP-1–MK-801
To safely harness the weight-lowering properties of NMDA receptor antagonism, we developed a targeting approach based on the hypothesis that conjugation of MK-801 to a GLP-1 analogue through a chemically cleavable disulfide linker would enhance the therapeutic potential of NMDA receptor antagonism in appetite-regulating brain regions enriched for GLP-1 receptors, while mitigating the adverse effects related to the non-restricted actions of MK-801. These efforts involve chemically masking the secondary amine of MK-801 to render it inactive until the linker is cleaved, by the higher concentration of thiol-containing compounds in the intracellular compartment, resulting in liberation of MK-801 18 . Through iterative chemical synthesis and structural refinement, we developed a stabilized conjugate equipped with a C-terminal l -penicillamine residue and a self-immolative disulfide linker (GLP-1–MK-801) (Extended Data Fig. 2a ). The plasma stability of GLP-1–MK-801 was optimized through incorporation of different cysteine homologues to the C terminus of the peptide to facilitate disulfide conjugation to the linker (Extended Data Fig. 2b–d ). Moreover, we confirmed that the conjugate is degraded in vitro by incubation under high concentrations of glutathione (Extended Data Fig. 2e ). We observed that GLP-1–MK-801 has similar receptor signalling properties at the GLP-1 receptor compared to the parent GLP-1 analogue as well as to the pharmacokinetically optimized GLP-1 receptor agonists semaglutide and liraglutide (Extended Data Fig. 2f ). Furthermore, we confirmed GLP-1–MK-801-mediated target engagement with NMDA receptors in GLP-1-receptor-positive neurons in the arcuate nucleus using electrophysiological recordings of isolated NMDA receptor currents. Specifically, we demonstrated that GLP-1–MK-801, but not the parent GLP-1 analogue, suppressed NMDA-induced inward currents (Extended Data Fig. 2g,h ). In agreement with previous research on GLP-1 receptor agonists, GLP-1–MK-801 increased the excitability of around 35% of POMC-expressing neurons 19 (POMC neurons; Extended Data Fig. 2i–m ). These observations were corroborated by single-cell calcium imaging studies in slices of arcuate nuclei, demonstrating that GLP-1–MK-801 inhibits the NMDA-induced intracellular calcium surge relative to GLP-1 alone (Extended Data Fig. 2n–r ).
Metabolic phenotyping of GLP-1–MK-801
After the dose-determination studies (Extended Data Fig. 2s–v ), we assessed the in vivo metabolic effects in DIO mice by comparing GLP-1–MK-801 with the parent GLP-1 analogue, MK-801 and vehicle treatments (Fig. 1a ). Over a 14-day treatment period, GLP-1–MK-801 synergistically lowered body weight compared with the dose-matched monotherapies and produced a vehicle-corrected weight loss of 23.2% (Fig. 1b and Extended Data Fig. 3a,b,d,e ). The potent weight loss induced by GLP-1–MK-801 was linked to a potentiated decrease in food intake in mice treated with GLP-1–MK-801 compared to mice treated with vehicle or GLP-1 or MK-801 monotherapies (Fig. 1c and Extended Data Fig. 3c ). GLP-1–MK-801 produced a vehicle-corrected reduction in body fat mass of 45%, accompanied by an 8% loss in lean mass (Fig. 1d,e and Extended Data Fig. 3f,g ). In comparison, GLP-1 induced a 22% decrease in body fat mass and a 4% reduction in lean mass. Mice treated with GLP-1–MK-801 for 14 days exhibited lower plasma insulin levels compared with both vehicle-treated mice and those treated with MK-801 alone (Fig. 1f ). Moreover, mice receiving GLP-1 monotherapy displayed significantly lower insulin levels compared with mice that were treated with MK-801 alone. Treatment with GLP-1–MK-801 led to a decrease in plasma cholesterol levels compared with vehicle treatment and treatment with either GLP-1 or MK-801 (Fig. 1g ). Furthermore, both GLP-1 monotherapy and GLP-1–MK-801 treatment resulted in decreased plasma triglyceride levels compared with the vehicle treatment (Fig. 1h ). Notably, GLP-1–MK-801 also resulted in lower plasma triglycerides compared with MK-801 monotherapy. The effectiveness of GLP-1–MK-801 in regulating energy balance was further validated by its ability to normalize body weight and fat mass relative to age-matched control animals maintained on chow diet (Extended Data Fig. 3h–m ). GLP-1–MK-801 also displayed superior weight loss efficacy compared with co-administration of GLP-1 and MK-801, despite having similar effects on food intake (Extended Data Fig. 3a–c ). This implies that GLP-1–MK-801 reduces body weight through effects on both energy intake and energy expenditure. This notion was confirmed using metabolic cages, revealing that GLP-1–MK-801 counteracted the decrease in energy expenditure that is engaged by calorie-restricted mammals as an adaptive ‘starvation response’ to protect the organism from an excessive loss of body weight and fat mass (Fig. 1i–p and Extended Data Fig. 3n ). Accordingly, despite losing 25% of their body mass, GLP-1–MK-801-treated mice maintained an energy expenditure that was similar to that of vehicle-treated control mice and was therefore also significantly higher than the gradually decreasing energy expenditure observed in mice that were calorie-restricted to match the weight loss trajectory of GLP-1–MK-801-treated mice. A subsequent study supported this finding by demonstrating that the energy expenditure of mice treated with the conjugate resembles that of the heavier control animals undergoing monotherapy treatment with GLP-1 and MK-801 (Extended Data Fig. 3o–s ). This study also showed that GLP-1 and the GLP-1–MK-801 conjugate promote enhanced whole-body lipid oxidation, evident by a decrease in respiratory exchange ratio (RER) compared with vehicle or MK-801 treatment (Extended Data Fig. 3q,r ).
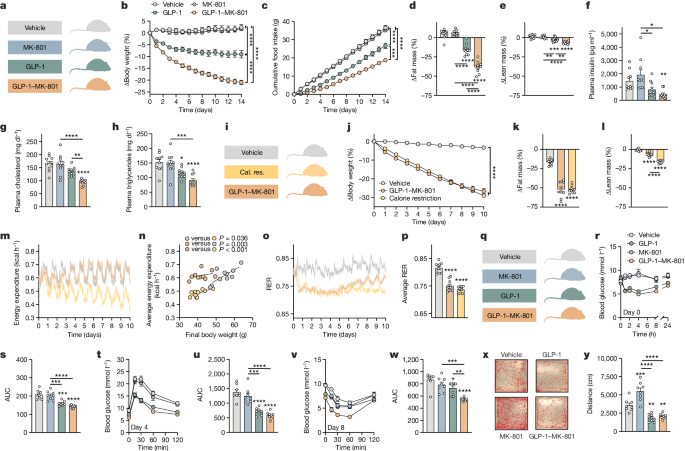
a – h , DIO mice were treated once-daily with s.c. injections of MK-801, GLP-1, GLP-1–MK-801 or vehicle for 14 days. n = 10 mice per group. 100 nmol kg −1 dose. a , Schematic. b , Change in body weight. c , Cumulative food intake. d , Change in fat mass. e , Change in lean mass. f , Plasma insulin. g , Plasma cholesterol. h , Plasma triglycerides. i – p , DIO mice were treated once-daily with s.c. injections of GLP-1–MK-801 (100 nmol kg −1 ), calorie restriction (cal. res.) to match the weight loss of the GLP-1–MK-801 group or vehicle for 10 days. n = 9–10 mice per group. i , Schematic. j , Change in body weight. k , Change in fat mass. l , Change in lean mass. m , Energy expenditure. n , Average energy expenditure relative to final body weight. o , RER. One mouse in the calorie-restriction group was excluded due to a CO 2 -sensor-related deviation. p , Average RER. q – w , DIO mice were treated once-daily with s.c. injections of MK-801, GLP-1, GLP-1–MK-801 or vehicle for 8 days. n = 8 mice per group. 100 nmol kg −1 dose. q , Schematic. r , Compound tolerance test on day 0. s , Area under the curve (AUC) of data in r . t , Glucose tolerance test on day 4. u , AUC of data in t . v , Insulin tolerance test on day 8. w , AUC of data in v . x , y , Open-field test after a single s.c. injection of MK-801, GLP-1, GLP-1–MK-801 or vehicle. n = 8 mice per group. 300 nmol kg −1 dose. x , Representative traces. y , Distance travelled. Data were analysed using one-way analysis of variance (ANOVA) with Bonferroni post hoc multiple-comparison test ( d – h , k , l , p , s , u , w and y ), two-way repeated-measures ANOVA to assess main effects of treatment ( b , c and j ) or analysis of covariance (ANCOVA) computed with calR using body weight as a covariate ( n ). Data are mean ± s.e.m. * P < 0.05, ** P < 0.01, *** P < 0.001, **** P < 0.0001. Detailed statistics are provided in Supplementary Table 1 . The diagrams in a , i and q were created using BioRender.
Source Data
Both MK-801 and GLP-1 have been linked to improvements in glucose homeostasis in mice 20 , 21 . Here we show that a single administration of GLP-1 or GLP-1–MK-801 elicits comparable reductions in the blood glucose levels in DIO mice, whereas equimolar MK-801 monotherapy does not acutely affect glycaemia (Fig. 1q–s and Extended Data Fig. 4a–c ). The decrease in hyperglycaemia after administration of GLP-1 or GLP-1–MK-801 was not associated with changes in plasma insulin levels (Extended Data Fig. 4d, e ). However, in comparison to vehicle-treated mice, mice treated with GLP-1–MK-801 demonstrated a slight decrease in plasma glucagon levels (Extended Data Fig. 4f,g ). After 4 days of treatment, GLP-1 monotherapy and GLP-1–MK-801 displayed comparable benefits on glucose tolerance relative to vehicle treatment or treatment with MK-801 (Fig. 1t,u ). In a follow-up study, a calorie-restricted weight-matched group was introduced alongside the vehicle, GLP-1, MK-801 and GLP-1–MK-801 groups (Extended Data Fig. 4h–j ). In this study, the GLP-1–MK-801-treated cohort exhibited significantly enhanced glucose tolerance compared with all of the other groups (Extended Data Fig. 4k,l ). Insulin levels during the glucose tolerance test were substantially lowered in the calorie-restricted mice and in mice treated with GLP-1–MK-801 (Extended Data Fig. 4m,n ). However, only the animals that were administered with the incretin-based compounds (GLP-1 and GLP-1–MK-801) displayed increased baseline-corrected glucose-stimulated insulin secretion (Extended Data Fig. 4o,p ). Mice that were treated with GLP-1–MK-801 for 8 days displayed improved insulin sensitivity as assessed by an insulin tolerance test compared with mice that were treated with either monotherapy or vehicle (Fig. 1v,w and Extended Data Fig. 4q ). The improved insulin sensitivity after GLP-1–MK-801 treatment was confirmed in a 2-week study that, in addition to the pharmacological groups, included a calorie-restricted group weight-matched to the GLP-1–MK-801 group (Extended Data Fig. 4r–t ). In this study, both the GLP-1–MK-801-treated mice and the calorie-restricted mice exhibited improved insulin sensitivity relative to the vehicle treatment and both monotherapy control groups (Extended Data Fig. 4u–x ). After 2 weeks of treatment, mice treated with GLP-1–MK-801, as well as those subjected to calorie restriction or GLP-1 monotherapy, demonstrated enhanced glucose tolerance compared with mice that were treated with the vehicle or MK-801 monotherapy (Extended Data Fig. 4y,z ). In summary, the favourable effects of GLP-1–MK-801 on glucose homeostasis seem to stem from both its incretin action and its weight-reducing effect.
Safety profiling of GLP-1–MK-801
To evaluate the cardiometabolic safety of the conjugate, key markers of liver damage and cardiovascular health were assessed. Whereas GLP-1 monotherapy slightly reduced plasma aspartate aminotransferase (AST) levels compared with those in MK-801-treated mice, GLP-1–MK-801 treatment did not affect plasma AST or alanine transaminase (ALT) levels (Extended Data Fig. 5a,b ). In DIO mice that were treated for 14 days with vehicle, MK-801, GLP-1 or GLP-1–MK-801, heart weights remained unaffected (Extended Data Fig. 5c ). Subsequent haemodynamic assessments did not show any adverse effects of any of the treatments on heart rate or arterial blood pressure in lean mice receiving treatment for 14 days (Extended Data Fig. 5d–j ).
To examine whether the chemical conjugation of MK-801 to GLP-1 mitigates the adverse hyperthermic and hyperlocomotive effects of MK-801, mice were subjected to body temperature measurements and open-field behavioural tests after chronic and acute drug administration, respectively. These experiments demonstrated that the relatively low dose of MK-801, required for achieving a synergistic weight loss by the conjugate, does not affect the body temperature after 14 days of repeated dosing, as none of the treatment groups displayed different core temperature compared with the vehicle-treated mice (Extended Data Fig. 5k ). Finally, we demonstrated that the coupling of MK-801 to GLP-1 effectively eliminates the unfavourable hyperlocomotive reaction to MK-801 in an open-field test (Fig. 1x,y ). Conversely, animals administered either GLP-1 or GLP-1–MK-801 exhibited diminished locomotion compared with both MK-801-treated and vehicle-treated mice.
Pharmacological characterization
To examine whether the potent weight loss observed in vivo after GLP-1–MK-801 treatment is attributable to pharmacological synergy between GLP-1 and MK-801 or consequential to altered pharmacokinetic properties of the parent GLP-1 analogue, we designed and synthesized a conjugate comprising an inactive MK-801 surrogate 22 (Supplementary Fig. 1 ). The inactivated conjugate displayed comparable plasma stability and human serum albumin (HSA) binding properties to GLP-1–MK-801 (Fig. 2a–d ). These findings were corroborated by in vitro experiments showing similar GLP-1 receptor signalling properties of GLP-1–MK-801 and GLP-1–inactive MK-801 compared with lipidated analogues of GLP-1, semaglutide and liraglutide, and the parent GLP-1 analogue (Fig. 2e ). However, in the presence of 20% human plasma, a 100-fold rightward curve shift was observed for the lipidated compounds, but not the conjugates and the GLP-1 analogue, indicating that MK-801 and inactive MK-801 do not associate strongly with HSA (Fig. 2f ). Having demonstrated that GLP-1–MK-801 and GLP-1–inactive MK-801 display comparable pharmacokinetic properties in vitro, we next assessed their respective in vivo efficacies against the parent GLP-1 analogue in DIO mice. Treatment with GLP-1–inactive MK-801 produced no additional weight loss efficacy relative to GLP-1 monotherapy (Fig. 2g–i ), indicating that the pronounced weight loss induced by GLP-1–MK-801 is driven by concerted and site-directed pharmacological GLP-1 receptor agonism and NMDA receptor antagonism. Finally, using liquid chromatography coupled with mass spectrometry (LC–MS), we quantified the plasma concentrations of GLP-1, GLP-1–inactive MK-801 and GLP-1–MK-801 after s.c. administration and observed a half-life of 1.9 h for GLP-1–MK-801, which is longer than the parent GLP-1 analogue, but comparable to that of the GLP-1–inactive MK-801 conjugate (Fig. 2j ).
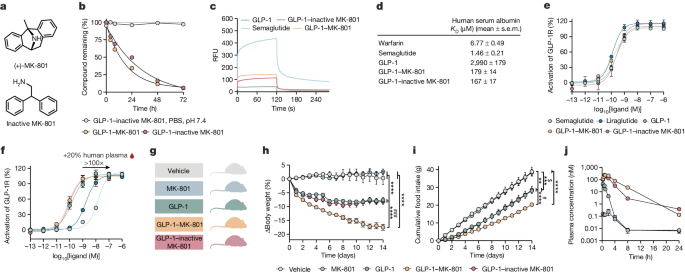
a , The chemical structures of (+)-MK-801 and 2,2-diphenylethan-1-amine (inactive MK-801). b , In vitro stability assay of GLP-1–MK-801 ( n = 3) and GLP-1–inactive MK-801 ( n = 3) incubated in human plasma at 37 °C and GLP-1–MK-801 ( n = 1) incubated in PBS buffer, pH 7.4 at 37 °C. c , d , The interactions with human serum albumin of GLP-1, GLP-1–MK-801, GLP-1–inactive MK-801, semaglutide and warfarin were analysed using surface plasmon resonance ( n = 3 per compound). c , Sensorgrams measured at 25 µM. RFU, relative fluorescence units. d , Dissociation constants were derived using a multi-site fit model. e , f , Dose–response curves for in vitro GLP-1 receptor activation of GLP-1, GLP-1–MK-801, GLP-1–inactive MK-801, liraglutide and semaglutide ( n = 3 per compound). e , Dose–response curves. f , Dose–response curves in the presence of 20% human plasma. g – i , DIO mice were treated once-daily with s.c. injections of MK-801, GLP-1, GLP-1–inactive MK-801 or vehicle for 14 days. n = 8 mice. 100 nmol kg −1 dose. g , Schematic. h , Change in body weight. i , Cumulative food intake. j , The plasma concentration of MK-801, GLP-1, GLP-1–inactive MK-801 and GLP-1–MK-801 in chow-fed male C57BL/6J mice. n = 4 mice per group. 100 nmol kg −1 dose. Data were analysed using two-way repeated-measures ANOVA to assess main effects of treatment ( h – j ). Dissociation constants were determined using a multi-site fit model ( d ). Data are mean ± s.e.m. * P < 0.05, ** P < 0.01, *** P < 0.001, **** P < 0.0001. # denotes comparison between GLP-1–MK-801 and GLP-1–inactive MK-801. $ denotes comparison between vehicle and GLP-1–inactive MK-801. Detailed statistics are provided in Supplementary Table 1 . The diagram in g was created using BioRender.
To further examine the possibility that MK-801 could convey protraction, we synthesized a series of conjugates comprising MK-801 in combination with other peptide analogues, peptide YY (PYY), glucose insulinotropic peptide (GIP) as well as a GIP/GLP-1 co-agonist, for which half-life extension has been shown to enhance weight loss efficacy 23 , 24 , 25 , 26 . Treatment of DIO mice with PYY–MK-801 and GIP–MK-801 did not affect body weight or food intake beyond that of the parent peptide analogues (Extended Data Fig. 6a–g and Supplementary Fig. 1 ). GIP/GLP-1 co-agonists, developed by merging the respective peptide sequences, potently lower body weight in rodents and humans 27 , 28 . Chemical synthesis and subsequent in vivo evaluation of a GIP/GLP-1–MK-801 co-agonist conjugate revealed enhanced weight loss in DIO mice compared with mice treated with the GIP/GLP-1 co-agonist alone (Extended Data Fig. 6h–k ). These experiments support the notion that MK-801 does not enhance the pharmacokinetics of gut peptides and that the additional metabolic benefits and synergistic weight loss effects brought about by MK-801 are dependent on NMDA receptor antagonism in GLP-1-receptor-positive neurons.
Neuronal effects of GLP-1–MK-801
To understand the biological underpinnings of the metabolic benefits of GLP-1–MK-801, we performed RNA sequencing (RNA-seq) and MS-based proteomics analyses on hypothalami from DIO mice treated with vehicle, GLP-1, MK-801 or the GLP-1–MK-801 conjugate for 5 days (Fig. 3a–c and Extended Data Fig. 8a,b ). A pronounced overlap in transcriptional signatures between GLP-1–MK-801 and each of GLP-1 and MK-801 was observed (Fig. 3d ), suggesting that GLP-1–MK-801 engages signalling pathways that are related to both receptor systems in the hypothalamus. Notably, 1,568 transcripts were uniquely regulated in response to GLP-1–MK-801 treatment, emphasizing that coordinated activity between the two receptor targets in vivo potently influences hypothalamic transcription. Volcano plot visualization of GLP-1–MK-801 relative to vehicle revealed transcripts associated with glutamatergic signalling, such as Grin2a , Grin2b , Shisa6 and Slc17a7 among the most differentially upregulated genes (Fig. 3e ). The targeted delivery of MK-801 was further emphasized by analysis of the top 20 differentially expressed genes derived from the top five enriched functional terms showing a similar but stronger transcriptional regulation for GLP-1–MK-801 in comparison to MK-801 (Fig. 3f ). The transcriptional signatures identified in GLP-1–MK-801-treated animals were enriched for functional terms related to synaptic transmission, such as postsynaptic density and glutamatergic synapse (Fig. 3g and Extended Data Fig. 7a–g ). These findings were substantiated by MS-based proteomics analyses in which we observed a more pronounced response to treatment with GLP-1–MK-801 compared with either monotherapy (Fig. 3h,i and Extended Data Fig. 8a,b ). Moreover, the alterations induced by the conjugate in the hypothalamic proteome primarily highlight shifts in functional terms associated with cellular processes and synaptic function (Extended Data Fig. 8c–g ). Collectively, these observations underscore that the pronounced weight loss caused by GLP-1–MK-801 coincides with changes in hypothalamic neuroplasticity and glutamatergic signalling. Thus, the conjugate seemingly harnesses the dual pharmacological benefits of hypothalamic GLP-1 receptor agonism and NMDA receptor antagonism while mitigating adverse behavioural effects linked to the latter. To probe the translational potential of GLP-1–MK-801, we assessed whether the differentially expressed genes regulated in response to treatment overlapped with genes located in obesity-associated GWAS loci (Fig. 3j ). Using two independent bioinformatic tools 29 , 30 , we found a significant overlap with transcripts associated with genetic susceptibility to obesity in humans, suggesting that GLP-1–MK-801 targets biological pathways implicated in common polygenic forms of human obesity 1 , 31 (Fig. 3k ).
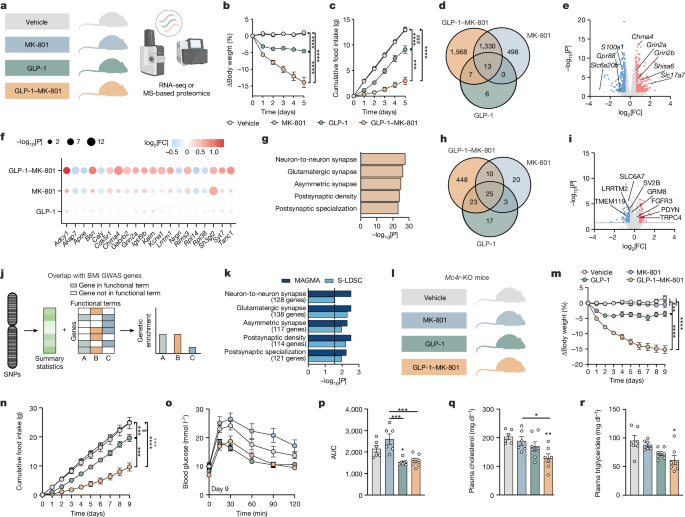
a – g , j , k , RNA-seq analysis of hypothalami from DIO mice treated once daily with s.c. injections of MK-801, GLP-1, GLP-1–MK-801 or vehicle for 5 days. n = 8 mice per group. 100 nmol kg −1 dose. a , Schematic. b , Change in body weight. c , Cumulative food intake. d , Venn diagram of differentially expressed genes. e , Volcano plot of differentially expressed genes in response to GLP-1–MK-801. FC, fold change. f , The top 20 differentially expressed genes found in the top five functional terms in g . g , The top five functional terms in response to GLP-1–MK-801 in the hypothalamus. h , i , MS-based proteomic analyses of hypothalami from DIO mice treated with once-daily s.c. injections of MK-801, GLP-1, GLP-1–MK-801 or vehicle for 5 days. n = 8 mice per group. 100 nmol kg −1 dose. h , Venn diagram of differentially expressed proteins. i , Volcano plot of proteins regulated in response to GLP-1–MK-801. j , Schematic of BMI GWAS integration. Differentially expressed proteins found in the top five functional terms were integrated with human BMI GWAS data. SNP, single-nucleotide polymorphism. k , Overlap analyses using the MAGMA and S-LDSC tools to compute BMI GWAS integration. l – r , Treatment of Mc4r -KO mice with once-daily s.c. administration of MK-801, GLP-1, GLP-1–MK-801 or vehicle for 9 days. n = 6–7 mice. 100 nmol kg −1 dose. One mouse in the MK-801 group had to be euthanized after the intraperitoneal glucose tolerance test. l , Schematic. m , Change in body weight. n , Cumulative food intake. o , Glucose tolerance test on day 9. p , AUC of data in o . q , Plasma cholesterol. r , Plasma triglycerides. Data were analysed using one-way ANOVA with Bonferroni post hoc multiple-comparison test ( p – r ) and two-way repeated-measures ANOVA to assess main effects of treatment ( b , c , m and n ). Data are mean ± s.e.m. * P < 0.05, ** P < 0.01, *** P < 0.001, **** P < 0.0001. # denotes comparison between MK-801 and GLP-1. $ denotes comparison between vehicle and GLP-1. + denotes comparison between vehicle and GLP-1–MK-801. Detailed statistics are provided in Supplementary Table 1 . The diagrams in a , d , h , j and l were created using BioRender.
Weight loss drugs targeting the hypothalamus often converge on the leptin–melanocortin pathway. To assess whether the anti-obesity properties of GLP-1–MK-801 are dependent on this key energy balance-governing pathway, we tested the weight loss efficacy in DIO Mc4r -KO (also known as melanocortin-4 receptor) mice. We observed a pronounced vehicle-corrected weight loss of 15.2% in the group treated with GLP-1–MK-801 compared with 3.5% in the group treated with the parent GLP-1 analogue after 9 days of treatment, underscoring that the weight-lowering efficacy of the conjugate is intact in the absence of functional MC4R signalling (Fig. 3l,m ). The weight loss was accompanied by a decrease in food intake in comparison to mice treated with GLP-1 or MK-801 monotherapy or vehicle (Fig. 3n ). Despite the large differences in weight loss between GLP-1 and GLP-1–MK-801, the two compounds exhibited similar benefits on glucose tolerance in Mc4r -KO mice. While only the GLP-1-treated group achieved statistical significance relative to the vehicle group, both the GLP-1 and GLP-1–MK-801 groups exhibited significantly enhanced glucose tolerance in comparison to animals treated with MK-801 (Fig. 3o,p ). By contrast, only GLP-1–MK-801 treatment lowered plasma cholesterol and plasma triglycerides as compared to vehicle or MK-801 treatment (Fig. 3q,r ). These findings emphasize that targeted antagonism of NMDA receptor signalling lowers body weight independently of MC4R, indicating that this preclinical drug candidate might not only be effective in treating polygenic obesity but also holds potential as an effective weight loss strategy for patients with loss-of-function mutations in MC4R , the most common form of monogenic obesity 32 .
GLP-1–MK-801 versus semaglutide
To further scrutinize the translational potential of GLP-1–MK-801, we benchmarked it against semaglutide, a pharmacokinetically optimized GLP-1 receptor agonist approved for treatment of obesity and clinically available as a once-weekly injectable 33 . To directly evaluate the head-to-head weight-lowering potential of semaglutide versus GLP-1–MK-801, we performed single intracerebroventricular (i.c.v.) infusions of equimolar concentrations of GLP-1–MK-801 or semaglutide (Fig. 4a ). This study revealed a superior vehicle-corrected weight loss of 9.5% in response to the GLP-1–MK-801 infusion relative to a weight loss of 4.5% after semaglutide infusion (Fig. 4b ). Notably, over the subsequent 6 days, we observed a sustained body-weight-lowering effect after infusion of GLP-1–MK-801, but not semaglutide, suggesting that the targeted inhibition of NMDA receptors in GLP-1 receptor-positive neurons might promote sustained changes to the defended level of adiposity. The enhanced weight loss observed in response to GLP-1–MK-801 coincided with a more pronounced decrease in food intake within the first 48 h after the infusion relative to semaglutide-treated mice (Fig. 4c ). Together these data support a stronger brain dependence of the body-weight-lowering effects of GLP-1–MK-801 compared with semaglutide. At the same time, the data imply that a medicinal chemistry campaign focusing on engineering pharmacokinetically optimized GLP-1–MK-801 conjugates might give rise to drugs that could outperform the current incretin-based treatments for obesity, such as semaglutide and tirzepatide.
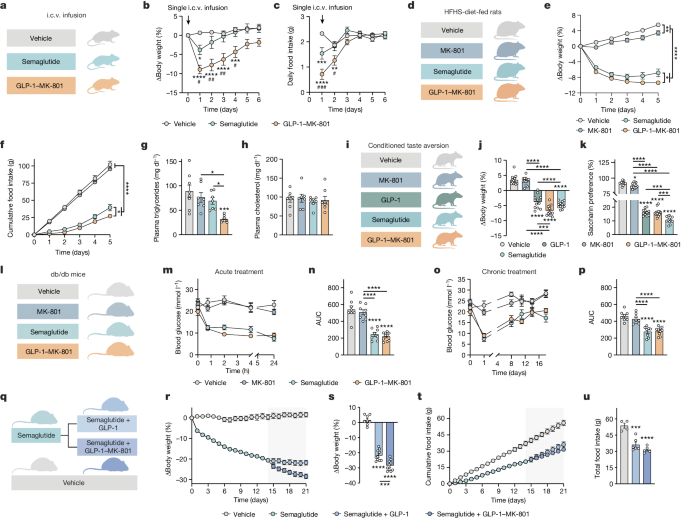
a – c , i.c.v. infusions of 0.22 nmol semaglutide ( n = 15 mice), 0.22 nmol GLP-1–MK-801 ( n = 15 mice) or vehicle ( n = 12 mice) in high-fat high-sucrose (HFHS)-fed mice. a , Schematic. b , Change in body weight. c , Daily food intake. One datapoint was excluded for one vehicle mouse owing to a measurement error. d – h , Once-daily s.c. treatment of Sprague-Dawley rats maintained on an HFHS diet for 4 weeks with MK-801 (100 nmol kg −1 ), semaglutide (10 nmol kg −1 ), GLP-1–MK-801 (100 nmol kg −1 ) or vehicle. n = 7–8 rats. d , Schematic. e , Change in body weight. f , Cumulative food intake. g , Plasma triglycerides. One plasma sample was lost in the semaglutide group. h , Plasma cholesterol. i – k , CTA analysis of chow-fed Wistar rats after treatment with MK-801 (100 nmol kg −1 ), GLP-1 (100 nmol kg −1 ), GLP-1–MK-801 (100 nmol kg −1 ), semaglutide (10 nmol kg −1 ) or vehicle. n = 12 rats per group. i , Schematic. j , Change in body weight 24 h after dosing. k , Saccharin preference. Saccharin intake data for one mouse in the GLP-1 group was not collected due to a sensor malfunction. l – p , Treatment of db/db mice with once-daily s.c. injections of MK-801 (100 nmol kg −1 ), semaglutide (10 nmol kg −1 ), GLP-1–MK-801 (100 nmol kg −1 ) or vehicle for 18 days. n = 8–10 mice per group. l , Schematic. m , Compound tolerance test on day 0. n , Area under the curve of data in m . o , Basal blood glucose. p , Area under the curve of data in o . q – u , DIO mice were treated once-daily with s.c. injections of semaglutide ( n = 20 mice, 10 nmol kg −1 ) or vehicle ( n = 8 mice). After 14 days of treatment, semaglutide-treated mice were randomized to receive semaglutide and GLP-1 (100 nmol kg −1 ) as co-administration or semaglutide and GLP-1–MK-801 (100 nmol kg −1 ) as co-administration. q , Schematic. r , Change in body weight. s , Change in body weight after 21 days. t , Cumulative food intake. u , Total food intake at day 21. Data were analysed using one-way ANOVA with Bonferroni post hoc multiple-comparison test ( g , h , j , k , n , p , s and u ), two-way repeated-measures ANOVA to assess the main effects of treatment ( e and f ) and two-way ANOVA with Bonferroni post hoc multiple-comparison test ( b and c ). Data are mean ± s.e.m. * P < 0.05, ** P < 0.01, *** P < 0.001, **** P < 0.0001. # P < 0.05, ## P < 0.01, ### P < 0.001 denotes comparison between GLP-1–MK-801 and semaglutide. Detailed statistics are provided in Supplementary Table 1 . The diagrams in a , d , i , l and q were created using BioRender.
To substantiate the weight-lowering efficacy in another rodent species, Sprague-Dawley rats fed a high-fat high-sugar diet for 4 weeks were treated with once-daily s.c. injections of vehicle, MK-801, GLP-1–MK-801 or semaglutide at a dose that has previously been demonstrated to induce maximal body weight loss in rodents 34 (Fig. 4d ). After 5 days of treatment, rats treated with MK-801, semaglutide or GLP-1–MK-801 had significantly decreased body weight compared with the vehicle-treated rats. Semaglutide-treated rats exhibited a greater weight loss compared with MK-801-treated rats, and rats that were treated with GLP-1–MK-801 had significantly reduced body weight compared with rats that were treated with either MK-801 or semaglutide (Fig. 4e ). Semaglutide and GLP-1–MK-801, but not MK-801 monotherapy, lowered food intake in rats, with GLP-1–MK-801 treatment resulting in a more pronounced reduction compared with semaglutide (Fig. 4f ). Moreover, rats treated with GLP-1–MK-801 had reduced levels of circulating triglycerides compared with rats that were treated with either semaglutide or MK-801. No changes in plasma cholesterol levels were observed for any of the treatments (Fig. 4g,h ).
To further assess the adverse profile of GLP-1–MK-801, we performed a series of complementary studies in mice and rats. A conditioned-taste aversion (CTA) experiment in male chow-fed Wistar rats affirmed a potent weight loss in response to GLP-1, semaglutide and GLP-1–MK-801 compared with MK-801 and vehicle treatment. Rats that were treated with GLP-1–MK-801 exhibited a lower body weight compared with those that were treated with GLP-1 (Fig. 4i,j ). Notably, although semaglutide resulted in a slightly less pronounced weight loss, it elicited a significantly greater aversive response compared with GLP-1–MK-801 (Fig. 4k ). None of the tested treatments, including GLP-1–MK-801, triggered pica behaviour in Wistar rats, indicated by the low consumption of the non-nutritive substance kaolin (Extended Data Fig. 9a–d ). However, in Sprague Dawley rats, GLP-1 monotherapy significantly increased kaolin intake compared with vehicle-treated rats and rats treated with MK-801 (Extended Data Fig. 9e–h ). GLP-1–MK-801 did not elicit pica behaviour in Sprague Dawley rats. Together, these rat studies suggest that the weight-lowering potential of GLP-1–MK-801 is not associated with exacerbated nausea. Exercise avoidance behaviour is a sensitive indicator of substance-induced malaise in mice 35 . Here we demonstrate that mice treated with either semaglutide or GLP-1–MK-801 exhibit a similar yet transient effect on exercise avoidance (Extended Data Fig. 9i–m ). This further underlines that the potent weight-reducing benefits of the bimodal compound are not linked to an aggravated adverse profile when compared to marketed incretin-based drugs such as semaglutide.
As NMDA receptors have previously been associated with regulation of glucose metabolism 20 , we evaluated the glucometabolic properties of GLP-1–MK-801 in the diabetic db / db mouse model. We observed that GLP-1–MK-801 and semaglutide elicited comparable glucose-lowering effects compared with vehicle and MK-801 monotherapy (Fig. 4l–p ). Finally, to expand on the opportunity for NMDA receptor antagonism as a complementary pharmacological partner to GLP-1 receptor agonism, we pretreated DIO mice with semaglutide for 14 days and subsequently randomized the mice to stay on semaglutide in combination with either GLP-1–MK-801 or the GLP-1 monotherapy (Fig. 4q ). Mice that received GLP-1–MK-801 on top of semaglutide displayed potentiated weight loss compared with mice that were co-treated with semaglutide and GLP-1 (Fig. 4r–u ). Collectively, these data demonstrate that GLP-1–MK-801 corrects hypertriglyceridaemia and hyperglycaemia with improved or similar efficacy to semaglutide in animal models of obesity and type 2 diabetes. Moreover, the observation that adding GLP-1–MK-801 treatment on top of semaglutide leads to an additional weight loss of 7% in mice that have reached a weight loss plateau with semaglutide provides additional support for integrating NMDA receptor antagonism in next-generation weight loss therapeutics.
Brain activity profiling of GLP-1–MK-801
Encouraged by the transcriptomic data indicating that the weight loss is evoked through a mechanism of action involving the combined pharmacological actions of NMDA receptor antagonism and GLP-1 receptor agonism in the hypothalamus, we performed a comprehensive comparison between GLP-1–MK-801 and the long-acting GLP-1 receptor agonist, semaglutide. The substantial difference in weight loss between GLP-1–MK-801 treatment and the parent GLP-1 analogue could potentially confound the interpretation of differences in transcriptional regulation. To correct for this, we performed RNA-seq analysis of hypothalami obtained from animals treated with daily injections of either GLP-1–MK-801 or a dose of semaglutide that was equipotent in terms of weight-lowering efficacy over 5 days of treatment, that is, mice had achieved a comparable weight loss and reduction in food intake as that of GLP-1–MK-801-treated mice (Extended Data Fig. 10a–c ). Similar to our previous RNA-seq analysis, we demonstrate that treatment with GLP-1–MK-801 significantly regulates around 150 times the number of hypothalamic transcripts relative to the weight-matched semaglutide-treated group, emphasizing a mode of action for GLP-1–MK-801 that involves biological pathways independent of the GLP-1 receptor signalling pathway (Fig. 5a,b ). Further analyses of differentially expressed genes between GLP-1–MK-801 and semaglutide treatment groups support this notion by showing enrichment of functional terms pertaining to glutamatergic signalling and synaptic plasticity among the most upregulated genes. These observations were further supported by pathway analyses confirming the strong annotations related to NMDA receptor signalling (Fig. 5c–e and Extended Data Fig. 10d–i ).
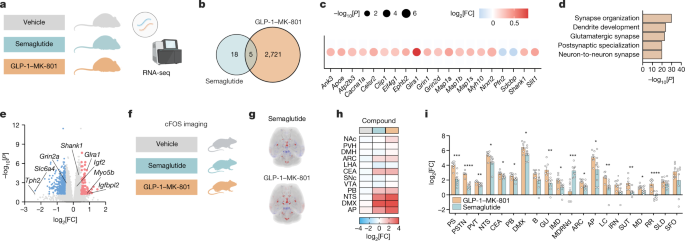
a – e , RNA-seq analysis of hypothalami from DIO mice treated once-daily with s.c. injections of semaglutide (10 nmol kg −1 ), GLP-1–MK-801 (100 nmol kg −1 ) or vehicle for 5 days. n = 6 mice. a , Schematic. b , Venn diagram of differentially expressed genes. c , The top 20 most differentially expressed genes found in the top 5 functional terms in d . d , The top 5 functional terms between GLP-1–MK-801 and semaglutide treatments. e , Volcano plot of differentially expressed genes in response to GLP-1–MK-801 relative to semaglutide. f – i , Whole-brain 3D mapping and quantification of cFOS responses to a single s.c. injection with semaglutide (10 nmol kg −1 ), GLP-1–MK-801 (100 nmol kg −1 ) or vehicle in lean mice. n = 7–8 mice. One mouse in the GLP-1–MK-801 group was excluded due to sample-processing deviation. f , Schematic. g , Mouse brain images showing a heat map of the averaged changes in cFOS expression in response to treatments. h , Heat map of cFOS activity in brain regions associated with appetite regulation. i , Comparison of changes in cFOS expression in the top 20 most significantly regulated brain regions. Data were analysed using an unpaired two-tailed t -test ( i ). Data are mean ± s.e.m. * P < 0.05, ** P < 0.01, *** P < 0.001, **** P < 0.0001. Definitions of brain region abbreviations are provided in the ‘cFOS whole-brain imaging’ section of the Methods . Detailed statistics are provided in Supplementary Table 1 . The diagrams in a , b and f were created using BioRender.
GLP-1 and NMDA receptors are expressed throughout the mammalian brain and, although reports indicate that GLP-1 analogues engage central appetite circuits through distinct subpopulations of neurons (most notably in the brainstem and the hypothalamus) 36 , feedforward neural pathways modulated by inhibition of NMDA receptors specifically in GLP-1-receptor-positive neurons may differ from those engaged by exclusive activation of GLP-1 receptors. To understand whether the GLP-1–MK-801 conjugate activates distinct neural circuitries, we used light-sheet microscopy and cFOS immunohistochemistry to obtain quantitative 3D whole-brain activity signatures in response to GLP-1–MK-801 and semaglutide dosing (Fig. 5f,g ). A large overlap in neuronal activity patterns was observed between the two treatments and, in agreement with previous research 37 , strong signals were detected in the arcuate nucleus, in the central amygdala and in subregions of the brainstem, including the area postrema, the nucleus of the solitary tract and the dorsal motor nucleus of the vagus nerve (Fig. 5h,i ). The most noticeable difference in whole-brain activity signatures between GLP-1–MK-801 and semaglutide was the significantly higher number of cFOS + cells in the nucleus accumbens in response to GLP-1–MK-801 compared with semaglutide and vehicle treatment. However, this might be consequential to the fatty acid sidechain 38 and not related to NMDA receptor modulation (Extended Data Fig. 11a–j ). Subsequent subregion analyses showed that the ventral tegmental area as well as subregions of the amygdala displayed enhanced activity patterns after GLP-1–MK-801 treatment (Extended Data Fig. 11k–o ). Collectively, these analyses suggest that the mesolimbic reward system may be more potently engaged with GLP-1–MK-801 than semaglutide treatment. To gain deeper insights into the impact of the conjugate on both the brainstem and the mesolimbic reward system, we conducted complementary transcriptomics analyses (Extended Data Fig. 8a,b ). Similar to the transcriptomic changes observed in the hypothalamus, we found that the conjugate prompted transcriptional patterns enriched for functional terms associated with synaptic transmission, including postsynaptic specialization and neuron-to-neuron synapse in the brainstem (Extended Data Fig. 12a–k ). By contrast, substantial transcriptional alterations were not induced by the conjugate in the nucleus accumbens (Extended Data Fig. 12l–n ). Together, these findings necessitate further investigations to ascertain whether coordinated inhibition of NMDA receptors in GLP-1 receptor neurons modulates neuronal activity beyond canonical feeding regions, such as the mesolimbic dopaminergic reward pathway.
Here we demonstrate the efficacy of peptide-targeted NMDA receptor inhibition for obesity treatment. To bypass the pharmacological challenges associated with unspecific blocking of NMDA receptors, we designed a peptide–drug conjugate comprising the NMDA receptor antagonist MK-801 and a GLP-1 peptide analogue. By using a reducible disulfide linker, we built in a redox-sensitive release mechanism to facilitate intracellular release of the unmodified NMDA receptor antagonist, enabling the combined cellular actions of GLP-1 receptor agonism and NMDA receptor antagonism.
Treatment with the GLP-1–MK-801 conjugate potently reverses obesity, diabetes and dyslipidaemia in a wide range of rodent models of obesity and metabolic disease. Although the exact mechanisms by which the coordinated actions of GLP-1 receptor agonism and NMDA receptor antagonism correct metabolic diseases await further elucidation, the extensive alterations in transcriptomic and proteomic responses within the hypothalamus, linked to glutamatergic signalling and synaptic plasticity, imply that the conjugate might elicit neurostructural changes in GLP-1-receptor-expressing neurons. This observation aligns with previous work showing that pharmacological inhibition or genetic ablation of NMDA receptors in agouti-related-protein (AgRP)-expressing neurons alters synaptic adaptation to fasting 39 , 40 . Moreover, previous consideration of NMDA receptor antagonism as potential treatment for binge-eating disorder suggests that the weight-lowering benefits linked to GLP-1–MK-801 could be the result of a combined impact on both energy homeostasis and disordered eating behaviours 10 , 14 , 41 .
Although our data align with the notion that inhibiting NMDA receptor signalling in the hypothalamus decreases food intake and body weight 8 , 40 , earlier studies have demonstrated that NMDA receptor antagonism in the brainstem attenuates vagus-mediated meal-related satiety 6 , 7 , 42 . The bidirectional effects of NMDA receptor antagonism on feeding could potentially diminish the weight-lowering efficacy of systemic exposure to NMDA receptor antagonists. Supported by the substantial weight-loss efficacy of the GLP-1–MK-801 conjugate and the potentiated hypothalamic changes in transcripts and proteins related to NMDA receptor-linked neuroplasticity, the alteration in MK-801 biodistribution governed by GLP-1-mediated targeting may effectively bypass the delivery of MK-801 to vagal afferent target neurons in the nucleus of the solitary tract. Accordingly, this mechanism would circumvent the blocking of distinct NMDA receptors in the brainstem that might otherwise increase food intake in response to MK-801. Supporting this hypothesis, we demonstrate that GLP-1 receptor-mediated targeting of MK-801 effectively avoids other adverse effects associated with MK-801 monotherapy, including hyperthermia and hyperlocomotion.
Multimodal weight loss compounds that integrate agonism at two or more receptor systems are emerging 27 , 43 , 44 , 45 . Here we report a unimolecular compound composed of a small-molecule antagonist and a peptide agonist for obesity treatment. Whereas previous research has demonstrated the feasibility of using peptides to target nuclear hormone receptors 46 , 47 , to our knowledge, this is the first single molecule that uses a G-protein-coupled-receptor targeting approach to deliver a small-molecule modulator of an ionotropic receptor. We provide evidence that this targeting concept can be used to antagonize NMDA receptors specifically in GLP-1-receptor-positive neurons to reverse obesity and correct symptoms of cardiometabolic diseases in preclinical obesity models. While the clinical evaluation of GLP-1–MK-801 for weight loss awaits, efforts to broaden the scope of developing peptide-mediated targeting of ion channels are encouraged and should not be restricted to metabolic diseases.
Peptide synthesis
All peptides were prepared using ChemMatrix Rink amide resin and Fmoc-based automated peptide synthesis on a Prelude X peptide synthesizer (Gyros Protein Technologies) with induction heating and oscillation mixing. All of the solutions were freshly prepared immediately before synthesis as stock solutions in DMF: Fmoc-protected amino acid (0.2 M), HCTU (0.5 M), DIPEA (1.0 M) and piperidine (20% v/v). Peptide elongation was achieved through consecutive cycles of Fmoc deprotection and coupling reactions. Fmoc deprotection was achieved with 20% piperidine in DMF (twice for 2 min, room temperature, 300 rpm shaking) and peptide couplings were performed as double or triple couplings (twice for 5 min, 75 °C, 300 rpm shaking, except for Arg and His, for which twice for 5 min, 50 °C, 300 rpm shaking) consisting of AA/HCTU/DIPEA (ratio, 1:1.25:2.5) in fivefold excess compared to the resin. Extensive DMF washes were performed after each deprotection or coupling reaction.
Peptide cleavage and purification
Dried peptide-containing resin was suspended in 1.5 ml cleavage cocktail (2.5% DODT, 2.5% H 2 O, 2.5% TIPS in TFA) per 100 mg resin and agitated for 2 h. The peptide-containing solution was collected by filtration, reduced under a stream of nitrogen and precipitated with ice-cold ether. The crude peptide pellet was isolated by centrifugation at 3,600 g for 10 min at 4 °C, redissolved in MeCN:H 2 O (1:1) and lyophilized. Ultraperformance LC (UPLC) and electrospray ionization LC–MS (ESI-LC–MS) analysis were conducted for the crude peptide. Purifications were conducted by preparative RP-HPLC, eluting with a linear gradient (20 ml min −1 ) and using a binary solvent system consisting of H 2 O:MeCN:TFA (buffer A, 95:5:0.1; buffer B: 5:95:0.1). The collected fractions were analysed by UPLC and ESI-LC–MS. Fractions with a purity of greater than 95% were pooled and lyophilized. All peptides and peptide drug conjugates were desalted by three consecutive cycles of redissolving the peptide in 0.001 M aqueous HCl followed by lyophilization. All peptides and conjugates used for in vitro and in vivo experiments were of >95% purity.
Synthesis of disulfide linker functionalized (+)-MK-801 and inactive MK-801
2-Mercaptoethanol (Sigma-Aldrich) was treated with 2,2-dipyridyl disulfide (3 equivalents) (Sigma-Aldrich) in dry methanol for 2 h. After completion as monitored by UPLC–MS, the reaction was concentrated in vacuo. Purification by silica gel flash chromatography (EtOAc:CH 2 Cl 2 , 2:8) afforded intermediate 2-(pyridine-2-yldisulfaneyl)ethan-1-ol (> 95%). The compound was dissolved in dry CH 2 Cl 2 under an N 2 atmosphere and reacted with 4-nitrophenyl chloroformate (1.2 equivalents) for 4 h. The reaction was worked up by extraction, washing with water (×3) and brine. The organic layer was dried over anhydrous MgSO 4 , filtered and concentrated in vacuo. The crude residue was purified by silica gel flash column chromatography ( n -heptane:EtOAc, 2:1) yielding 4-nitrophenyl (2-(pyridin-2-yldisulfaneyl)ethyl) carbonate (89%). The intermediate was finally reacted with the appropriate amine-containing drug (+)-MK-801 (2,2-diphenyl-1-amine; 1.5 equivalent) in dry DMF with addition of dry Et 3 N (3.0 equivalents) under an N 2 atmosphere for 18 h. Purification by preparative HPLC afforded the disulfide linker functionalized (+)-MK-801 (70%) and inactive MK-801 (70%). Conjugation to the corresponding peptides was conducted using a general disulfide conjugation protocol.
Disulfide conjugation protocol
The pure thiol-containing peptide and thiopyridyl-activated linker-functionalized MK-801 were dissolved in DMF (2 ml) and buffer consisting of 6 M guanidine and 1.5 M imidazole in H 2 O at pH 8 (200 µl) and agitated for at least 2 h. After completion, as monitored by UPLC and ESI-LC–MS, the reaction mixture was diluted with buffer A, filtered and purified directly by preparative RP-HPLC, eluting with a linear gradient (see the ‘Peptide cleavage and purification’ section).
In vitro human plasma stability
Human plasma was preheated at 37 °C for 15 min before being spiked with a final concentration of 0.25 mM of the peptide or conjugate. The samples were collected at timepoints [0, 1, 2, 4, 8, 24 h], [0, 2, 4, 8, 24, 48 h] or [0, 4, 8, 24, 48, 72 h] depending on stability. The samples were processed by pretreatment with 6 M urea for 30 min at 0 °C followed by 20% trichloroacetic acid in acetone (wt./v%) at −20 °C overnight. The samples were centrifuged (13,400 rpm) for 30 min, and the supernatant was collected and filtered (0.2 µm syringe filter) into an LC–MS vial. The samples were analysed by reversed-phase UPLC (RP-UPLC) at 214 nm and LC–MS. The area under the curve was determined and normalized to the first timepoint. Regression lines were computed with GraphPad Prism 9.0 using the one-phase decay equation.
In vitro stability assay with high glutathione concentration
GLP-1–MK-801 was dissolved in dimethyl sulfoxide at a concentration of 0.25 mM and diluted with a solution of 200 mM glutathione in PBS, pH 7, such that the final concentration of GLP-1–MK-801 in the assay was 100 µM. The solution was incubated at 37 °C and samples collected at timepoints [0, 1, 2, 4, 8, 12 h]. The samples were analysed using RP-UPLC at 214 nm and LC–MS. The data were normalized to the first timepoint. Regression lines were computed with GraphPad Prism 9.0 using the one-phase decay equation.
GLP-1 receptor activation
GLP-1 receptor activation was determined using an in vitro bioluminescence resonance energy transfer (BRET)-based assay that measures changes in intracellular cyclic-AMP levels. HEK293 cells were transiently transfected with GLP-1 receptor and cyclic-AMP sensor using YFP-Epac-RLuc (CAMYEL). Cells were cultured at 37 °C in Dulbecco’s modified Eagle’s medium (DMEM) + GlutaMax 1965 (Gibco) supplemented with 10% fetal bovine serum and 1% penicillin–streptomycin in a humidified 10% CO 2 incubator.
Cells were seeded in 96-well plates at a density of 35,000 cells per well and transiently transfected using Lipofectamine 2000 according to the manufacturer’s protocol. On the day of the assay, plates were removed from the incubator and each well was washed twice with 100 μl HBSS (Gibco, Life Technologies) and pre-incubated for 30 min at 37 °C with 85 μl HBSS per well. Luciferase substrate coelenterazine (5 μM, Thermo Fisher Scientific, C6780) was added and a baseline was measured after a 5 min incubation. The ligand mixture was added and measurements were recorded every minute for 30 min on a CLARIOstar Plus plate reader (BMG labtech). Dose–response curves were generated at equilibrium (10 min) and EC 50 values were calculated from this.
SPR biosensing of human serum albumin binding affinity
Interaction of peptide–drug conjugates with surface immobilized human serum albumin (HSA) (Sigma-Aldrich) was determined using surface plasmon resonance (SPR). The samples were acquired at 25 °C according to literature procedure using a Biacore X100 instrument equipped with a CM5 sensor chip (GE Healthcare Biosciences) 48 .
The system was equilibrated with 10 mM PBS at a flow rate of 20 µl min −1 to achieve a stable baseline. HSA was immobilized to the surface by pre-activation (1.0 EDC, 1.0 M NHS in PBS, pH 7.4, 7 min) followed by injection of HSA to flow cell 1 (30 µg ml −1 in 10 mM NaOAc buffer, pH 5.0, twice for 7 min). Unreacted sites were capped with ethanolamine (1.0 M ethanolamine in 10 mM PBS, pH 8.1, 7 min). Residual unreacted HSA was removed by three consecutive pulses of 9 s with 25 mM NaOH (3 µl), resulting in a surface of 14,360 RFU.
Interactions between immobilized HSA and the experimental compounds were determined using a running buffer of 10 mM PBS, 3% dimethyl sulfoxide (DMSO), pH 7.4 and a flow rate of 30 µl min −1 . Peptides were dissolved in running buffer and the stock concentrations determined using the NanoDrop 2000 spectrophotometer (Thermo Fischer Scientific) at a wavelength of 280 nm. The samples were measured as triplicates by the same researcher, going from low to high concentrations. Compounds were injected for 150 s, and the corresponding dissociation constants were measured for 300 s. The surface was washed between samples with 25 mM NaOH for 4 s followed by equilibration with running buffer. Blanks were acquired after each triplicate measure.
Mouse studies
All in vivo experiments were conducted according to international principles of animal care and under the approval of the Danish Ethical Committee for Animal Research and the Danish Animal Experimentation Inspectorate. Experiments were conducted using DIO male C57BL/6J mice (Janvier Labs) kept on a HFHS diet (58 kcal% fat, D12331i, Research Diets) from 8 weeks of age. Mice were maintained on the HFHS diet for a minimum of 16 weeks and had an average body weight of >45 g, before initiation of pharmacological studies. The mice were either single-housed or doubled-housed and maintained on a 12 h–12 h dark–light cycle (06:00–18:00) at 21–23 °C. Mice received once-daily sham injections with isotonic saline from 3 days before study start and were randomized to treatments on the basis of body weight at the day of study start. All compounds were administered as once-daily s.c. injections (between 15:00 and 18:00) with concomitant measurements of body weight and food intake. Vehicle was isotonic saline, which was also used for dissolving the compounds. Compounds were administered at the indicated doses at a volume of 5 µl g −1 . For studies with a calorie-restricted and body-weight-loss-matched control group, HFHS diet (58 kcal% fat, D12331i, Research Diets) was weighed and provided as one separate pellet per mouse at the time of injection. The pellets were placed in each side of the cage for double-housed mice and the mice were treated with isotonic saline.
db/db mice (The Jackson Laboratory, 000697) were kept on a chow diet (Brogaarden, Altromin, 1310) and experiments were conducted on 9-week-old male mice. Grouping was based on blood glucose level (using a minimum of 11.1 mM) and body weight. Male Mc4r- KO mice were kept on a HFHS diet for 9 weeks and the mice were 17 weeks old at study start (The Jackson Laboratory, 032518). For electrophysiological studies, male pathogen-free mice at an age of 6–18 weeks were used for all experiments. All of the mice were housed under standard laboratory conditions (12 h–12 h on–off; lights on at 07:00) and a temperature-controlled environment with food and water available ad libitum. All experiments were performed in accordance with the guidelines established by the National Institute of Health Guide for the Care and Use of Laboratory Animals and approved by the University of Texas Institutional Animal Care and Use Committee. To identify POMC neurons and GLP-1 receptor-positive neurons, POMC humanized Renilla green fluorescent protein (hrGFP) (The Jackson Laboratory, 006421) and GLP-1 receptor cre::tdTomato (The Jackson Laboratory, 029283) mice were used. For calcium imaging studies, male Naval Medical Research Institute (NMRI) mice (Taconic Biosciences, BomTac:NMRI) were used (aged 18–28 days). Mice were bred and housed in the animal facility at the Department of Drug Design and Pharmacology, University of Copenhagen and studies were conducted under the approval of the Danish Ethical Committee for Animal Research and the Danish Animal Experimentation Inspectorate. The mice were housed in ventilated cages in a humidity- and temperature-controlled room (temperature, 22 ± 2 °C; humidity, 36–58%) with a 12 h–12 h light–dark cycle (lights on at 07:00) with ad libitum access to chow diet and tap water.
Rat studies
Double-housed male Sprague-Dawley rats (Janvier Labs, RjHan:SD) were kept on a HFHS diet for 4 weeks from 8–10 weeks of age and had an average body weight of >500 g before initiation of pharmacological studies. The rats were housed in ventilated cages in a humidity- and temperature-controlled room (temperature, 22 ± 2 °C; humidity, 36–58%) under a 12 h–12 h light–dark cycle (lights on at 07:00) with ad libitum access to chow diet and tap water. The rats were administered once-daily s.c. injections (between 15:00 and 17:00) of 100 nmol kg −1 MK-801, 10 nmol kg −1 semaglutide, 100 nmol kg −1 GLP-1–MK-801 or vehicle, with concomitant measurements of body weight and food intake. Vehicle was isotonic saline and compounds were administered at a volume of 1 µl g −1 . For the kaolin-intake study in Sprague-Dawley rats, 8-week-old double-housed male rats were used.
For CTA and kaolin-intake studies, male Wistar rats (Janvier Labs, WistarRjHan:WI) were used. The rats were 8 weeks old at the initiation of the studies with a body weight of 250–325 g. The rats were group-housed (3–4 rats per cage) in ventilated cages in a humidity- and temperature-controlled room (temperature, 21 ± 2 °C; humidity, 50 ± 10%) under a 12 h–12 h light–dark cycle (light, 01:00 to 13:00) with ad libitum access to chow diet (Brogaarden, Altromin, 1324) and tap water. The rats were dosed by s.c. injections of 100 nmol kg −1 MK-801, 100 nmol kg −1 GLP-1, 10 nmol kg −1 semaglutide, 100 nmol kg −1 GLP-1–MK-801 or vehicle immediately before onset of the dark cycle at a volume of 2 ml kg −1 .
Tissue collection and processing
Mice or rats were euthanized by decapitation for collection of trunk blood and tissues. Blood was collected in EDTA-coated microvette tubes, chilled on ice and centrifuged at 3,000 g and 4 °C for 10 min. The plasma was aliquoted and stored at −80 °C until analysis. Tissues were collected by dissection, frozen on dry-ice and stored at −80 °C until further processing.
Body composition measurements
Body composition measurements were performed using quantitative nuclear magnetic resonance imaging (EchoMRI).
Glucose tolerance, compound tolerance and insulin tolerance tests
Mice were fasted for 5 h before being challenged with an intraperitoneal injection of 1.75 g kg −1 of glucose dissolved in isotonic saline. Tail vein blood glucose concentrations were measured using a handheld glucometer (Contour XT, Bayers) at 0, 15, 30, 60 and 120 min after injection (for the Mc4r KO study a 90 min timepoint was also included). Compound tolerance was assessed by s.c. injection of the experimental compound followed by measurements of tail vein blood glucose concentrations using a handheld glucometer (Contour XT, Bayers) at various timepoints over the following 24 hours. For studies with measurements of plasma insulin and glucagon concentrations, plasma was collected by tail vein bleeding at timepoints 0, 60, 120 and 240 min. For insulin tolerance testing, the mice were fasted for 5 h before being challenged with an intraperitoneal injection of 0.75 U kg −1 of human insulin (Actrapid). Tail vein blood glucose concentrations were measured using a handheld glucometer (Contour XT, Bayers) at 0, 15, 30, 60 and 120 min after injection. For glucose-stimulated insulin secretion testing, the mice were fasted for 4 h before being challenged with intraperitoneal injections of 1.75 g kg −1 of glucose dissolved in isotonic saline. Tail vein blood glucose concentrations were measured using a handheld glucometer (Contour XT, Bayers) at 0, 15, 30, 60 and 120 min after injection. Plasma was collected at timepoints 0, 15 and 60 min by tail vein blood sampling and insulin concentrations were measured by enzyme-linked immunosorbent assay (ELISA; Crystal Chem Ultra Sensitive Mouse Insulin Eisa Kit, 90080).
Rectal body temperature
Conscious mice were restrained and a high-precision thermometer (BIO-TK8851, Bioseblab) was carefully inserted half-way into the rectum. Temperature measurements of each mouse were performed by the same researcher on day 7 at timepoints 0, 20, 45 and 90 min in response to s.c. injection with 200 nmol kg −1 MK-801, 600 nmol kg −1 MK-801 or vehicle, and for measurement of rectal temperature on day 14 of mice receiving 14 days of once-daily s.c. dosing with 100 nmol kg −1 MK-801, 100 nmol kg −1 GLP-1, 100 nmol kg −1 GLP-1–MK-801 or vehicle.
Plasma parameters
Plasma was sampled from a non-fasted state 2 h after the final compound administration. Plasma insulin levels were quantified using the Crystal Chem Ultra Sensitive Mouse Insulin ELISA kit (Crystal Chem Ultra Sensitive Mouse Insulin Eisa Kit, 90080). Plasma glucagon levels were quantified using the Mercodia Glucagon ELISA kit (10-1281-01, Mercodia). Plasma total cholesterol (Thermo Fisher Scientific, Infinity Total Cholesterol Reagent, TR13421), triglycerides (total glycerol and triglycerides) (Thermo Fisher Scientific, Infinity Total Triglycerides Reagent, TT22421), non-esterified fatty acids (NEFA) (Invitrogen, non-esterified free fatty acids (NEFA/FFA) Colorimetric Assay Kit, Thermo Fisher Scientific, EEA017), AST (Thermo Fisher Scientific, EEA003) and ALT (Thermo Fisher Scientific, EEA001) were quantified using enzymatic kits according to the manufacturer’s protocols.
In vivo pharmacokinetic measurements
The pharmacokinetic assessment was conducted using a total of eight male DIO C57BL/6J mice ( n = 4 per subgroup). Mice were administered single s.c. injections with a dose of 100 nmol kg −1 of the experimental compounds. Each subgroup was bled at the following timepoints: subgroup A at 15 min, 45 min, 2 h and 8 h; subgroup B at 30 min, 1 h, 4 h and 24 h.
Mouse plasma containing test substance was crashed in 96-well non-binding plates using liquid–liquid extraction with ethanol containing internal standard. The samples were then centrifuged, and the supernatants were transferred to new wells and diluted with water. The prepared mouse plasma was analysed for the test substance using LC–MS. The system consisted of a TSQ Quantis Triple Quad mass spectrometer (Thermo Fisher Scientific) equipped with a Vanquish Horizon UPLC (Thermo Fisher Scientific). RP-UPLC separation was performed on the Acquity UPLC system (Waters, column: BEH C18 1.7 µm, 2.1 × 50 mm). Mobile phase A was composed of 0.1% formic acid in water and mobile phase B was composed of 0.1% formic acid in acetonitrile. The UPLC flow rate was set to 0.3 ml min −1 at 60 °C using a gradient elution from 10 to 65% B over the course of 4.0 min. The gradient was then ramped from 65% B to 99% B for 0.1 min and held at 99% for 0.9 min. The mass spectrometer was operated in positive-ionization SRM mode.
Intracerebroventricular administration study
The intracerebroventricular study was performed in HFHS-fed C57BL/6J mice that were single-housed in open cages. Mice were pretreated with lidocaine (Accord Healthcare) at the site of incision, anaesthetized with isoflurane and fixed in a stereotaxic instrument. The skin of the head was incised, a hole was drilled into the skull and a guide cannula was subsequently implanted into the lateral ventricle (26GA; PlasticOne; C2315GS-4/SPC) using stereotaxic coordinates (−0.3 mm posterior to bregma; ±1.0 mm lateral to bregma) (David Kopf Instruments). The guide cannula was held in place using UV-cured cement (G-bond and G-aenial Universal Flo, GC), and a dummy cannula (PlasticsOne, C315DCS-4/Spc, 2.5 mm) was inserted into the guide cannula to keep the cannulation closed until and in between compound infusions. Post-operatively, mice were administered s.c. injections of carprofen (5 mg kg −1 , Pfizer) for 3 days and allowed to recover for a minimum of 7 days with daily monitoring of food intake and body weight.
After full recovery, correct cannulation was tested by observing water drinking responsiveness after infusion of 1 µl human angiotensin II (Sigma-Aldrich, A9525) at a concentration of 24 µM in artificial cerebrospinal fluid (distilled water with 125 mM NaCl, 2.5 mM KCl, 2.6 mM NaHCO 3 , 1.25 mM NaH 2 PO 4 ·2H 2 O, 25 mM d -glucose monohydrate, 1 mM MgCl 2 , 2 mM CaCl 2 ). Mice that did not drink within 15 min of the infusion were excluded from the study. In the afternoon on the experimental day, semaglutide (Novo Nordisk) and GLP-1–MK-801 were dissolved in isotonic saline at a concentration of 0.11 nmol µl −1 . Before infusion, mice were relocated to new cages with fresh bedding to ensure that no remnant food pellets remained in the cage. Mice were placed onto a cage hopper and infused with semaglutide, GLP-1–MK-801 or vehicle (isotonic saline) over 60 s at a total infusion volume of 2 µl through a Hamilton syringe mounted onto an automated syringe pump (Harvard Apparatus) and a 33-gauge internal cannula (PlasticsOne, C315IS-4/SPC, 2.5 mm). The injector was kept in the guide cannula for 30–60 s after infusion stop to ensure complete infusions and to avoid backflow. Body weight and food intake were subsequently monitored daily in the afternoon. At day 6 after the first infusion, the semaglutide-treated and GLP-1–MK-801-treated mice were crossed-over in terms of treatment and administered with another single infusion of either GLP-1–MK-801 (0.22 nmol, 2 µl) and semaglutide (0.22 nmol, 2 µl), respectively, while vehicle-treated mice received vehicle treatment once again. Again, body weight and food intake were monitored once daily in the afternoon.
Metabolic phenotyping and indirect calorimetry
Single-housed male DIO C57BL/6J mice were acclimatized to metabolic cages (16-channel Promethion, Sable Systems International) for 1 week before the start of the study. Oxygen consumption (VO 2 ), carbon dioxide production (VCO 2 ), RER, energy expenditure (kcal h −1 ) and locomotor activity (cm s −1 ) were recorded and collected in 15 min bins. Water and HFHS food were available ad libitum throughout the study period. New food was provided every second day. For study 1 (weight loss study of GLP-1–MK-801 relative to monotherapies), mice were randomly divided into four experimental groups ( n = 8 mice per group) with similar mean body weights and assigned to receive once-daily s.c. injections of 100 nmol kg −1 MK-801, 100 nmol kg −1 GLP-1, 100 nmol kg −1 GLP-1–MK-801 or vehicle for 14 days. For study 2, mice were randomly divided into three experimental groups ( n = 10 mice per group) such that each group had a similar mean body weight and was assigned to receive once-daily s.c. injections with 100 nmol kg −1 GLP-1–MK-801 or vehicle, or calorie restriction to match the weight loss trajectory of the GLP-1–MK-801 group for 10 days. In both studies, body weights and food intake were measured manually each day at the time of injection and dosing was performed at a volume of 5 µl g − 1 . Mice assigned to the different experimental groups were randomly distributed across two systems. Raw data for each individual mouse were analysed using the online tool CalR (CalR, v.1.3; www.calrapp.org ) and visualized using GraphPad Prism. ANCOVA analyses for statistical comparison of regression lines in body weight versus average energy expenditure plots were computed using CalR.
Open-field test
Locomotor activity was evaluated using an open-field test. Mice were acclimatized in the procedure room for 7 days before the experiments. The experiments were conducted by placing the mice in one of four 50 × 50 × 50 cm arenas immediately after compound administration, allowing their locomotion to be monitored by a ceiling-mounted Logitech C920 Pro camera (1,080 × 1,080 px, 30 fps, Logitech software). DIO mice were divided into four groups such that each group had the same average mean body weight ( n = 8 mice), and their movements were recorded for a period of 20 min. Each run was conducted with one mouse from each treatment group and with run-to-run alternation so that treatments were equally distributed across all four arenas. Movement traces and quantification of locomotor activity (velocity and distance travelled) were obtained using Noldus EthoVision XT software (Noldus).
A week before the study start (day −7), rats were single-housed with ad libitum access to chow diet and one bottle of water (food and water were placed in the cage lid so that there was room for two water bottles). The position of the water bottle was alternated daily between sides to avoid the development of a side preference. The animals were weighed and handled daily from day −3. On day −3, the rats were exposed to a bottle containing 0.1% saccharin-flavoured water with high palatability followed by s.c. administration of the experimental compounds (MK-801, GLP-1, GLP-1–MK-801 (all at doses of 100 nmol kg −1 ), semaglutide (10 nmol kg −1 ) or isotonic saline as vehicle). Then, 3 days after the first dosing, the rats were exposed to a two-bottle taste preference test, that is, a voluntary choice between tap-water or the 0.1% saccharin solution. Saccharin and water intake was monitored for 24 h followed by preference determination. These experiments were conducted at Gubra.
Kaolin intake
For the Wistar pica study, rats were group-housed (3–4 rats per cage) and allowed to acclimatize to the HM-2 system (MBRose) for 7 days before the study start. At the initiation of the experiment, the rats were randomized such that each group had the same average body weight and was then assigned to receive once-daily s.c. injections of 100 nmol kg −1 MK-801, 100 nmol kg −1 GLP-1, 100 nmol kg −1 GLP-1–MK-801 or vehicle (isotonic saline). Food intake data were collected on a continuous basis from 24 h before dosing (allowing for replenishment of food as well as dosing time) and throughout the study. After the study start, food pellets were placed in one food channel of the HM-2 system and kaolin pellets in the other channel. The position of the food and kaolin was alternated every day to correct for side preference. These experiments were conducted at Gubra. For the Sprague Dawley study, rats were single housed and had ad libitum access to a chow diet, kaolin pellets (K50001, Research Diets) and tap water. The rats were habituated to kaolin for 5 days before pharmacological testing. At the initiation of the experiment, the rats were randomized such that each group had the same average body weight and was then assigned to receive once-daily s.c. injections of 100 nmol kg −1 MK-801, 100 nmol kg −1 GLP-1, 100 nmol kg −1 GLP-1–MK-801 or vehicle (isotonic saline) for 3 days. Body weight, chow intake and kaolin intake were measured daily, and the cage was carefully assessed for any remnant leftover food and kaolin.
Voluntary running
Experiments were conducted using 8-week-old male C57BL/6J mice (Janvier Labs) kept on a chow diet. Mice were single-housed in cages equipped with a running wheel (23 cm in diameter, Techniplast). The amount of bedding material was reduced to avoid blockade of the running wheels. Running distance was monitored using a Sigma Pure 1 Topline 2016 computer (Sigma Sports) and, after 1 week of habituation, daily running distance was monitored for 3 days. The effect of experimental compounds on voluntary wheel running was monitored by randomizing mice to receive once-daily s.c. injections of 100 nmol kg −1 GLP-1–MK-801, 10 nmol kg −1 semaglutide or vehicle (isotonic saline) based on baseline running wheel distance measurements, such that each group had similar average running wheel distances at the day of study initiation. Running distance, food intake and body weight were measured daily before dosing of experimental compounds.
Heart rate and blood pressure assessment
Blood pressure and electrocardiogram (ECG) were recorded in anaesthetized, lean mice. Chow-fed male C57BL/6J mice at 18 weeks of age were randomly assigned to receive once-daily s.c. injections of 100 nmol kg −1 MK-801 ( n = 10), 100 nmol kg −1 GLP-1 ( n = 10), 100 nmol kg −1 GLP-1–MK-801 ( n = 10) or vehicle (isotonic saline, n = 10) for 14 days. Body weight and food intake were measured manually each day at the time of injections. The morning after the final injections, mice were anaesthetized using 2% isoflurane in a mix of O 2 and N 2 (30:70). The mice received an intraperitoneal administration of isotonic saline (0.5 ml) to compensate for potential fluid loss. Body temperature was monitored and kept at 36–37 °C throughout the procedure.
For heart rate measurements, a surface ECG was recorded using custom-made needle electrodes placed on each limb. For blood pressure measurements, a 0.8F pressure catheter (SPR-1000, Millar, 8410001) connected to a bioamplifier unit was inserted in the right carotid artery. The catheter tip was placed at the level of the aortic arch. Evaluation of blood pressure pulse profile was used to confirm correct positioning of the catheter tip. A PowerLab unit (PowerLab 16/35, AD Instruments) was used to record ECG and blood pressure at a sampling rate of 4 kHz. The ECG was recorded for 5 min after the mouse was anaesthetized and then again for 10 min once the blood pressure catheter was placed.
Electrophysiology
Brain slices were prepared from adult Glp1r-cre::tdTomato or Pomc-hrGFP male mice (aged 6–18 weeks) as previously described 49 , 50 , 51 . In brief, male mice were deeply anaesthetized with an intraperitoneal injection of 7% chloral hydrate and transcardially perfused with a modified ice-cold artificial cerebrospinal fluid (aCSF) (described below). The mice were then decapitated and the entire brain was removed and immediately submerged in ice-cold, carbogen-saturated (95% O 2 and 5% CO 2 ) aCSF (126 mM NaCl, 2.8 mM KCl, 1.2 mM MgCl 2 , 2.5 mM CaCl 2 , 1.25 mM NaH 2 PO 4 , 26 mM NaHCO 3 and 5 mM glucose). Coronal sections (250 mm) were cut using the Leica VT1000S Vibratome and then incubated in oxygenated aCSF (32–34 °C) for at least 1 h before recordings. The slices were bathed in oxygenated aCSF (32–34 °C) at a flow rate of ~2 ml min −1 . All electrophysiology recordings were performed at ambient temperature.
The pipette solution for whole-cell recordings was modified to include an intracellular dye (Alexa Fluor 350 hydrazide dye) and contained: 120 mM K-gluconate, 10 mM KCl, 10 mM HEPES, 5 mM EGTA, 1 mM CaCl 2 , 1 mM MgCl 2 and 2 mM MgATP, and 0.03 mM Alexa Fluor 350 hydrazide dye (pH 7.3). Epifluorescence was briefly used to target fluorescent cells, at which time the light source was switched to infrared differential interference contrast imaging to obtain the whole-cell recordings (Zeiss Axioskop FS2 Plus equipped with a fixed stage and a QuantEM:512SC electron-multiplying charge-coupled device camera). Electrophysiological signals were recorded using the Axopatch 700B amplifier (Molecular Devices), low-pass filtered at 2–5 kHz, and analysed offline on a PC with pCLAMP programs (Molecular Devices). To measure NMDA-induced inward current from GLP-1-receptor-positive neurons, we used magnesium-free aCSF (126 mM NaCl, 2.8 mM KCl, 2.5 mM CaCl 2 , 1.25 mM NaH 2 PO 4 , 26 mM NaHCO 3 and 5 mM glucose) containing 10 μM CNQX and 100 μM picrotoxin. Membrane potentials and firing rates were measured from POMC neurons in brain slices. Recording electrodes had resistances of 2.5–5 MΩ when filled with the K-gluconate internal solution. The frequency and peak amplitude of excitatory neurons were analysed using the Easy electrophysiology program (Easy Electrophysiology).
Drug working concentrations and stock preparation were as follows: GLP-1–MK-801 and MK-801 (both 50 μM, dissolved in aCSF or magnesium-free aCSF), NMDA (100 μM, dissolved in magnesium free aCSF), CNQX (10 μM, dissolved in DMSO, Alomone Labs), picrotoxin (100 μM, dissolved in DMSO). The final concentration of DMSO applied to the slices was <0.05%.
A change in membrane potential was required to be at least 2 mV in amplitude in response to drug application. Membrane potential values were not compensated to account for junction potential (−8 mV). Effects of GLP-1–MK-801 on frequency (over 0.5 Hz) and synaptic activity before and during acute GLP-1–MK-801 bath application were analysed within a recording using the Kolmogorov–Smirnov test (a nonparametric, distribution-free goodness-of-fit test for probability distributions).
Calcium imaging
Brain slices were prepared from adult NMRI male mice (aged 18–28 days). After deep anaesthesia with isoflurane (Attane Vet, 1,000 mg g −1 , ScanVet, Piramal Critical Care) mice were decapitated and the brain was removed and submerged in ice-cold aCSF (124 mM NaCl, 5 mM KCl, 1.2 mM Na 2 HPO 4 ·2H 2 O, 2.7 mM CaCl 2 ·2H 2 O, 1.2 mM MgSO 4 (anhydrous)), 10 mM dextrose, 26 mM NaHCO 3 was adjusted to pH 7.4 and an osmolarity of 298–302 mOsm kg −1 after saturation with carbogen (95% O 2 /5% CO 2 ). A vibratome (Leica VT1200S, Leica Biosystems) was used to obtain 250 μm thin acute brain slices containing Arc, identified by well-known landmarks. After incubation for 15 min in a 32 °C water bath and 1 h at ambient temperature, the slices were loaded with Fura-2-AM (4 mM; Hello Bio) under carbogen exposure in a 32 °C water bath for 10 min + 1 min for each PND. The slices were rinsed and placed into a chamber embedded in the stage of an Olympus BX51WI microscope (Olympus) coupled to a 12-bit CCD fluorescent camera (SensiCam, PCO imaging). A monochromator (Polychrome V, TILL Photonics, FEI) combined with a xenon light bulb provided fluorescent illumination. Protocols for fluorescence exposure of slices were controlled by software (Live Acquisition, TillVision), and analyses were conducted using Offline Analysis (TillVision).
Regions of interest were drawn around Fura-2-AM-loaded cells under ×40 magnification as well as around one region of the field devoid of cells, which was to be used as the background. The entire field of view was exposed to excitation wavelengths of 340 nm (exposure time, 50 ms) and 380 nm (exposure time, 40 ms). Each frame pair (340 nm:380 nm was collected at an interval of 4 s for 10 min of recording in total. GLP-1 or GLP-1–MK-801 (1 µM in aCSF) was bath-applied for 25 min, with recording only being conducted during the first 10 min, at which point a maximum and stable change in fluorescence was achieved. Fifteen additional minutes of application of GLP-1 or GLP-1–MK-801 were conducted without recording to minimize exposure to fluorescent light to reduce bleaching of the fluorescent indicator. Then, a 10 min recording with excitation was started, and after establishment of a baseline consisting of 10 frame pairs, NMDA (50 µM, Tocris) + GLP-1 or GLP-1–MK-801 was bath-applied.
The fluorescence intensity within each region of interest was binned at 2 × 2 pixels and averaged. A ratio of fluorescence intensities measured at 340 nm and 380 nm was calculated minus the background fluorescence. The peak amplitude of a change in fluorescence induced by GLP-1, GLP-1–MK-801 or NMDA was calculated by taking an average of ten datapoints from the baseline ( F 0 ) and subtracting this from an average of ten data points from the peak fluorescence (Δ F : average amplitude peak − average amplitude baseline ), which was normalized by dividing by F 0 . Graphic plots were converted to a percentage defined as %Δ F / F 0 .
RNA-seq analysis
mRNA-seq was performed by the Single-Cell Omics platform at the Novo Nordisk Foundation Center for Basic Metabolic Research. Libraries were prepared using the Universal Plus mRNA-seq protocol (Tecan) according to the manufacturer’s protocol. Libraries were quantified with NuQuant, quality checked using a TapeStation instrument (Agilent Technologies) and subjected to 52 bp paired-end sequencing on the NovaSeq 6000 system (Illumina). For differential expression testing, the R package DESeq2 (v.1.30.1) was used to identify differentially expressed genes. P values were adjusted for multiple testing using the Benjamini–Hochberg post hoc method. For functional enrichment analysis, the R package gprofiler2 (v.0.2.0) was used to identify enriched functional terms (GO:MF, GO:BP, GO:CC, KEGG pathways and REACTOME pathways). The gene-set enrichment analysis was performed with the parameters ‘exclude_iea’ set to true and ‘correction method’ set to Benjamini-Hochberg. SynGO enrichment analyses were conducted using the online tool https://syngoportal.org/ with the background set to brain expressed and using differentially expressed genes ( P < 0.05). The following transcripts are not depicted in Fig. 3e : gh , s carna13 and CT010467.1.
MS-based proteomics
Hypothalamic tissue was powdered and lysed (lysis buffer 50 mM Tris, 4% SDS buffer, pH 8.5) using BeatBox homogenizer (PreOmics). Protein lysates were boiled at 95 °C for 10 min on a thermomixer (Thermo Fisher Scientific) and sonicated on the Bioruptor (Diagenode) system. Proteins were digested into peptides using a high-throughput automated version of the protein aggregation capture workflow 52 on the KingFisher Flex Purification System (Thermo Fisher Scientific). Proteins were on-bead digested overnight in a solution containing LysC and trypsin at 37 °C. The resulting tryptic peptides were desalted using in-house-crafted SDB-RPS StageTips and 200 ng of peptides were loaded in Evotips (Evosep) according to the manufacturer’s instructions.
Desalted peptides were separated on the Pepsep (15 cm, 150 μM inner diameter) column packed with C18 beads (1.9 μm; Bruker) on the Evosep ONE HPLC system using the ‘30 samples per day’ method, then injected through a CaptiveSpray ion source and 20 μm emitter into a timsTOF Pro 2 mass spectrometer (Bruker) operated in diaPASEF mode. The resulting MS raw files were processed with the DIA-NN software v.1.876 in a library-free manner, using a Mus Musculus FASTA file from UniProt (December 2023). Proteotypic peptides were used for protein group quantification, under double-pass mode neural network configuration. ‘Robust LC (high accuracy)’ was chosen as the quantification strategy and the match between runs options was enabled. The rest of the parameters were set as the default, which included precursor FDR set to 1% and peptide length of 7–30 amino acids. For differential expression analysis, the R package limma (v.3.54.2) was used to identify differentially expressed proteins. P values were adjusted for multiple testing using the Benjamini–Hochberg post hoc method. For functional enrichment analysis, enriched gene sets were determined by applying the same workflow used for RNA, using the R package gprofiler2 (v.0.2.0). SynGO enrichment analyses were conducted using the online tool https://syngoportal.org/ with the background set to brain expressed and using differentially expressed proteins ( P < 0.05).
cFOS whole-brain imaging
Lean male C57BL/6J mice (aged 8 weeks) maintained on a chow diet (Brogaarden, Altrumin, 1310) were randomized 4 days before the study start and treated with once-daily s.c. mock dosing with isotonic saline. Study 1 was conducted during the light phase. All compounds were prepared as solutions in isotonic saline and dosed as s.c. injections of 10 nmol kg −1 semaglutide ( n = 8 mice), 100 nmol kg −1 GLP-1–MK-801 ( n = 8 mice) or vehicle (isotonic saline, n = 8 mice) at a volume of 5 µl g −1 . Study 2 was conducted during the light phase. All of the compounds were prepared as solutions in isotonic saline and dosed as s.c. injections of 100 nmol kg −1 MK-801 ( n = 8 mice), 100 nmol kg −1 GLP-1 ( n = 8 mice), 100 nmol kg −1 GLP-1–MK-801 ( n = 8 mice) or vehicle (isotonic saline, n = 8 mice) at a volume of 5 µl g −1 . One outlier was removed from the vehicle, MK-801 and GLP-1–MK-801 groups due to deviation related to tissue processing. Tissue processing and quantification of cFOS was conducted as previously described 37 . These experiments were conducted at Gubra.
Brain abbreviations are as follows: NAc, nucleus accumbens; PVH, paraventricular hypothalamic nucleus; DMH, dorsomedial nucleus of the hypothalamus; ARC, arcuate nucleus of the hypothalamus; LHA, lateral hypothalamic area; CEA, central amygdala nucleus; SNc, substantia nigra, compact part; VTA, ventral tegmental area; PB, parabrachial nucleus; NTS, nucleus of the solitary tract; DMX, dorsal motor nucleus of the vagus nerve; AP, area postrema; IMD, intermediodorsal nucleus of thalamus; PG, pontine gray; DG, dentate gyrus; MS, medial septal nucleus; LS, lateral septal nucleus; SFO, subfornical organ; PH, posterior hypothalamic nucleus; SLD, sublaterodorsal nucleus; TRN, tegmental reticular nucleus; PSTN, parasubthalamic nucleus; PS, parastrial nucleus; B, Barrington’s nucleus; PVT, paraventricular nucleus of the thalamus; RR, midbrain reticular nucleus, retrorubral area; MD, mediodoral nucleus of thalamus; SUT, supratrigmental nucleus; IRN, intermediate reticular nucleus; LC, locus ceruleus; MDRNd, medullary reticular nucleus, dorsal part; GU, gustatoty areas.
Quantification and statistical analysis
Statistical analyses were performed using GraphPad Prism 10.1.1 (GraphPad) and figures were generated using either GraphPad Prism or CorelDraw X8 (Corel). For comparison of multiple groups, one-way ANOVA or two-way repeated measures ANOVA were used. Two-way ANOVA main effects are reported and Bonferroni post hoc multiple-comparison analyses applied when relevant for interpretation. Regression plot ANCOVA analyses of indirect calorimetry data were computed using the online tool calR ( www.calR.org ). For comparison of two groups, unpaired two-tailed Students t -tests were used. Data were evaluated for distribution patterns using tests including Shapiro-Wilk and Kolmogorov–Smirnov tests and by visual inspection of the distribution residuals. Data from designated brain regions from the cFOS 3D brain imaging study were analysed using one-way ANOVA with Dunnet’s post hoc multiple-comparison test relative to the vehicle, using a negative binomial generalized linear model to control for Gaussian distribution. However, the top 20 most statistically significantly regulated brain regions in response to treatment were analysed as previously described 37 . No statistical methods were applied to predetermine the sample size for in vivo pharmacology experiments. Data represent mean ± s.e.m.
Reporting summary
Further information on research design is available in the Nature Portfolio Reporting Summary linked to this article.
Data availability
All data necessary for the conclusions of the study are provided with the Article. Genetic data generated for the bulk RNA-seq analysis of GLP-1–MK-801 versus monotherapies of nuclei from the brainstem and the nucleus accumbens are available at the Gene Expression Omnibus under SuperSeries accession number GSE245728 . The MS proteomics data have been deposited to the ProteomeXchange Consortium through the PRIDE partner repository under dataset identifier PXD045816 . Source data are provided with this paper.
Code availability
The scripts used to analyse the RNA-seq data are available at GitHub ( https://github.com/perslab/Petersen-Nature-2024 ).
Locke, A. E. et al. Genetic studies of body mass index yield new insights for obesity biology. Nature 518 , 197–206 (2015).
Article CAS PubMed PubMed Central Google Scholar
Kim, J., Farchione, T., Potter, A., Chen, Q. & Temple, R. Esketamine for treatment-resistant depression—first FDA-approved antidepressant in a new class. N. Engl. J. Med. 381 , 1–4 (2019).
Article PubMed Google Scholar
Robinson, D. M. & Keating, G. M. Memantine: a review of its use in Alzheimer’s disease. Drugs 66 , 1515–1534 (2006).
Article CAS PubMed Google Scholar
Zanos, P. & Gould, T. D. Mechanisms of ketamine action as an antidepressant. Mol. Psychiatry 23 , 801–811 (2018).
Turcot, V. et al. Protein-altering variants associated with body mass index implicate pathways that control energy intake and expenditure in obesity. Nat. Genet. 50 , 26–41 (2018).
Burns, G. A. & Ritter, R. C. The non-competitive NMDA antagonist MK-801 increases food intake in rats. Pharmacol. Biochem. Behav. 56 , 145–149 (1997).
Zheng, H., Kelly, L., Patterson, L. M. & Berthoud, H. R. Effect of brain stem NMDA-receptor blockade by MK-801 on behavioral and fos responses to vagal satiety signals. Am. J. Physiol. 277 , R1104–R1111 (1999).
CAS PubMed Google Scholar
Stanley, B. G., Willett, V. L. 3rd, Donias, H. W., Dee, M. G. 2nd & Duva, M. A. Lateral hypothalamic NMDA receptors and glutamate as physiological mediators of eating and weight control. Am. J. Physiol. 270 , R443–R449 (1996).
Wessinger, W. D. Tolerance to and dependence on MK-801 (dizocilpine) in rats. Pharmacol. Biochem. Behav. 49 , 1049–1056 (1994).
Deng, S. N. et al. Long-term NMDAR antagonism correlates weight loss with less eating. Front. Psychiatry 10 , 15 (2019).
Article PubMed PubMed Central Google Scholar
Huang, X. T. et al. Activation of N -methyl- d -aspartate receptor regulates insulin sensitivity and lipid metabolism. Theranostics 11 , 2247–2262 (2021).
Popik, P., Kos, T., Zhang, Y. & Bisaga, A. Memantine reduces consumption of highly palatable food in a rat model of binge eating. Amino Acids 40 , 477–485 (2011).
Foltin, R. W., Danysz, W. & Bisaga, A. A novel procedure for assessing the effects of drugs on satiation in baboons: effects of memantine and dexfenfluramine. Psychopharmacology 199 , 583–592 (2008).
Brennan, B. P. et al. Memantine in the treatment of binge eating disorder: an open-label, prospective trial. Int. J. Eat. Disord. 41 , 520–526 (2008).
Pechnick, R. N. & Hiramatsu, M. The effects of MK-801 on body temperature and behavior in the rat: cross-sensitization and cross-tolerance with phencyclidine. Eur. J. Pharmacol. 252 , 35–42 (1994).
Pechnick, R. N. et al. Comparison of the effects of the acute administration of dexoxadrol, levoxadrol, MK-801 and phencyclidine on body temperature in the rat. Neuropharmacology 28 , 829–835 (1989).
Danysz, W., Essmann, U., Bresink, I. & Wilke, R. Glutamate antagonists have different effects on spontaneous locomotor activity in rats. Pharmacol. Biochem. Behav. 48 , 111–118 (1994).
Bargh, J. D., Isidro-Llobet, A., Parker, J. S. & Spring, D. R. Cleavable linkers in antibody-drug conjugates. Chem. Soc. Rev. 48 , 4361–4374 (2019).
Dong, Y. et al. Time and metabolic state-dependent effects of GLP-1R agonists on NPY/AgRP and POMC neuronal activity in vivo. Mol. Metab. 54 , 101352 (2021).
Marquard, J. et al. Characterization of pancreatic NMDA receptors as possible drug targets for diabetes treatment. Nat. Med. 21 , 363–372 (2015).
Smith, E. P. et al. The role of beta cell glucagon-like peptide-1 signaling in glucose regulation and response to diabetes drugs. Cell Metab. 19 , 1050–1057 (2014).
Bolshakov, K. V. et al. Design of antagonists for NMDA and AMPA receptors. Neuropharmacology 49 , 144–155 (2005).
Zhang, Q. et al. The glucose-dependent insulinotropic polypeptide (GIP) regulates body weight and food intake via CNS-GIPR signaling. Cell Metab. 33 , 833–844 (2021).
Mroz, P. A. et al. Optimized GIP analogs promote body weight lowering in mice through GIPR agonism not antagonism. Mol Metab 20 , 51–62 (2019).
Rangwala, S. M. et al. A long-acting PYY 3-36 analog mediates robust anorectic efficacy with minimal emesis in nonhuman primates. Cell Metab 29 , 837–843 (2019).
Ostergaard, S. et al. Design of Y 2 receptor selective and proteolytically stable PYY 3-36 analogues. J. Med. Chem. 61 , 10519–10530 (2018).
Jastreboff, A. M. et al. Tirzepatide once weekly for the treatment of obesity. N. Engl. J. Med. 387 , 205–216 (2022).
Finan, B. et al. Unimolecular dual incretins maximize metabolic benefits in rodents, monkeys, and humans. Sci. Transl. Med. 5 , 209ra151 (2013).
Finucane, H. K. et al. Partitioning heritability by functional annotation using genome-wide association summary statistics. Nat. Genet. 47 , 1228–1235 (2015).
de Leeuw, C. A., Mooij, J. M., Heskes, T. & Posthuma, D. MAGMA: generalized gene-set analysis of GWAS data. PLoS Comput. Biol. 11 , e1004219 (2015).
Ludwig, M. Q. et al. A genetic map of the mouse dorsal vagal complex and its role in obesity. Nat. Metab. 3 , 530–545 (2021).
Wade, K. H. et al. Loss-of-function mutations in the melanocortin 4 receptor in a UK birth cohort. Nat. Med. 27 , 1088–1096 (2021).
FDA Approves New Drug Treatment for Chronic Weight Management, First Since 2014 (FDA, 2021); www.fda.gov/news-events/press-announcements/fda-approves-new-drug-treatment-chronic-weight-management-first-2014 .
Gabery, S. et al. Semaglutide lowers body weight in rodents via distributed neural pathways. JCI Insight 5 , e133429 (2020).
Klein, A. B. et al. Pharmacological but not physiological GDF15 suppresses feeding and the motivation to exercise. Nat. Commun. 12 , 1041 (2021).
Article ADS CAS PubMed PubMed Central Google Scholar
Secher, A. et al. The arcuate nucleus mediates GLP-1 receptor agonist liraglutide-dependent weight loss. J. Clin. Invest. 124 , 4473–4488 (2014).
Hansen, H. H. et al. Whole-brain activation signatures of weight-lowering drugs. Mol. Metab. 47 , 101171 (2021).
Skovbjerg, G. et al. Uncovering CNS access of lipidated exendin-4 analogues by quantitative whole-brain 3D light sheet imaging. Neuropharmacology 238 , 109637 (2023).
Liu, T. et al. Fasting activation of AgRP neurons requires NMDA receptors and involves spinogenesis and increased excitatory tone. Neuron 73 , 511–522 (2012).
Grzelka, K. et al. A synaptic amplifier of hunger for regaining body weight in the hypothalamus. Cell Metab. 35 , 770–785 (2023).
Bisaga, A., Danysz, W. & Foltin, R. W. Antagonism of glutamatergic NMDA and mGluR5 receptors decreases consumption of food in baboon model of binge-eating disorder. Eur. Neuropsychopharmacol. 18 , 794–802 (2008).
Burns, G. A., Fleischmann, L. G. & Ritter, R. C. MK-801 interferes with nutrient-related signals for satiation. Appetite 30 , 1–12 (1998).
Muller, T. D., Bluher, M., Tschop, M. H. & DiMarchi, R. D. Anti-obesity drug discovery: advances and challenges. Nat. Rev. Drug Discov. 21 , 201–223 (2022).
Jastreboff, A. M. et al. Triple-hormone-receptor agonist retatrutide for obesity—a phase 2 trial. N. Engl. J. Med. 389 , 514–526 (2023).
Zimmermann, T. et al. BI 456906: discovery and preclinical pharmacology of a novel GCGR/GLP-1R dual agonist with robust anti-obesity efficacy. Mol. Metab. 66 , 101633 (2022).
Quarta, C. et al. Molecular integration of incretin and glucocorticoid action reverses immunometabolic dysfunction and obesity. Cell Metab. 26 , 620–632 (2017).
Finan, B. et al. Targeted estrogen delivery reverses the metabolic syndrome. Nat. Med. 18 , 1847–1856 (2012).
Bech, E. M. et al. Peptide half-life extension: divalent, small-molecule albumin interactions direct the systemic properties of glucagon-like peptide 1 (GLP-1) analogues. J. Med. Chem. 60 , 7434–7446 (2017).
Hwang, E. et al. Sustained inhibition of NPY/AgRP neuronal activity by FGF1. JCI Insight 7 , e160891 (2022).
Gao, Y. et al. TrpC5 mediates acute leptin and serotonin effects via pomc neurons. Cell Rep. 18 , 583–592 (2017).
Williams, E. K. et al. Sensory neurons that detect stretch and nutrients in the digestive system. Cell 166 , 209–221 (2016).
Batth, T. S. et al. Protein aggregation capture on microparticles enables multipurpose proteomics sample preparation. Mol. Cell. Proteom. 18 , 1027–1035 (2019).
Article CAS Google Scholar
Download references
Acknowledgements
We thank H. Liu and P. Mehrwarz for experimental and technical assistance; L. L. Larsen, C. Zhang, U. Roostalu, A. N. Madsen, T. Porsgaard, N. Sonne and D. Dencker Thorbek for technical support; the previous and current members of the Clemmensen Group for technical assistance and scientific discussions; the staff at the Single-Cell Omics platform and the Rodent Metabolic Phenotyping Platform at the Novo Nordisk Foundation Center for Basic Metabolic Research (CBMR) for technical expertise and support; and the staff at the Biophysics Platform, Protein Structure and Function Program from The Novo Nordisk Foundation Center for Protein Research, University of Copenhagen for performing the human serum albumin SPR binding measurements. Cartoon images were created using BioRender. This work was supported by the Lundbeck Foundation (fellowship R238-2016-2859), the BioInnovation Institute (grant number NNF20OC0063538) and the Novo Nordisk Foundation (grant numbers NNF17OC0026114 and NNF22OC0073778); and grants to E.H. (National Research Foundation of Korea, NRF 2021R1A6A3A14044733) and K.W.W. (R01 DK119169 and DK119130–5830). MS analyses were performed by the Proteomics Research Infrastructure (PRI) at the University of Copenhagen (UCPH), supported by the Novo Nordisk Foundation (NNF) (grant number NNF19SA0059305). Blood pressure recordings were performed by the Telemetry Unit for Cardiovascular Phenotyping at the University of Copenhagen, supported by the Novo Nordisk Foundation (grant number NNF18OC0032728 to M.B.T.). The Novo Nordisk Foundation Center for Protein Research is supported financially by the Novo Nordisk Foundation (grant number NNF14CC0001). The Novo Nordisk Foundation Center for Basic Metabolic Research is an independent Research Center, based at the University of Copenhagen, Denmark, and is partially funded by an unconditional donation from the Novo Nordisk Foundation ( www.cbmr.ku.dk ) (grant numbers NNF18CC0034900 and NNF23SA0084103).
Author information
Authors and affiliations.
Novo Nordisk Foundation Center for Basic Metabolic Research, Faculty of Health and Medical Sciences, University of Copenhagen, Copenhagen, Denmark
Jonas Petersen, Mette Q. Ludwig, Vaida Juozaityte, Pablo Ranea-Robles, Charlotte Svendsen, Nicole Fadahunsi, Jens Lund, Alberte W. Breum, Cecilie V. Mathiesen, Luisa Sachs, Roger Moreno-Justicia, Jacob E. Petersen, Mette Trauelsen, Thue W. Schwartz, Atul S. Deshmukh, Tune H. Pers, Anders B. Klein & Christoffer Clemmensen
Department of Drug Design and Pharmacology, Faculty of Health and Medical Sciences, University of Copenhagen, Copenhagen, Denmark
Jonas Petersen, Amalie W. Kristensen, Kristi A. Kohlmeier, Bente Frølund & Kristian Strømgaard
Center for Hypothalamic Research, the University of Texas Southwestern Medical Center at Dallas, Dallas, TX, USA
Eunsang Hwang, Bryan Portillo, Kyle Grose & Kevin W. Williams
Novo Nordisk Research Center Indianapolis, Indianapolis, IN, USA
Rebecca Rohlfs, James C. Ford, Jonathan D. Douros & Brian Finan
Core Facility Pathology & Tissue Analytics, Helmholtz Munich, Neuherberg, Germany
Annette Feuchtinger
Department of Chemistry, Indiana University, Bloomington, IN, USA
Richard D. DiMarchi
Department of Biomedical Sciences, University of Copenhagen, Copenhagen, Denmark
Morten B. Thomsen
You can also search for this author in PubMed Google Scholar
Contributions
Conceptualization of the project: J.P., A.B.K. and C.C. Chemistry: J.P., K.S. and B. Frølund. Rodent studies: J.P., C.S., L.S., V.J., N.F., J.L., A.F., A.B.K., A.W.B. and C.V.M. Cardiovascular assessment: P.R.-R. and M.B.T. Electrophysiology: E.H., B.P., K.G. and K.W.W. Calcium imaging: A.W.K. and K.A.K. In vivo pharmacokinetic study: R.R., J.C.F., J.D.D. and B. Finan. Cell studies: J.E.P. and M.T. RNA-seq studies: M.Q.L., J.P., C.V.M., C.C. and T.H.P. Proteomics: J.P., C.S., R.M.-J., C.C. and A.S.D. All of the authors contributed to data analysis and interpretation. J.P. and C.C. wrote the manuscript. All of the authors provided comments on and approved the final manuscript.
Corresponding author
Correspondence to Christoffer Clemmensen .
Ethics declarations
Competing interests.
J.P., A.B.K. and C.C. are co-founders of Ousia Pharma, a biotech company developing therapeutics for treatment of obesity. T.W.S. is a co-founder of Embark Laboratories and SOLID therapeutics. B. Frølund is a co-founder of Ceremedy. K.S. is a co-founder and employee of Avilex Pharma. R.R., J.C.F., J.D.D. and B. Finan are employees of Novo Nordisk. R.D.D. is a co-inventor on intellectual property owned by Indiana University and licensed to Novo Nordisk; and was previously employed at Novo Nordisk. The other authors declare no competing interests.
Peer review
Peer review information.
Nature thanks Darleen Sandoval and the other, anonymous, reviewer(s) for their contributions to the peer review of this work.
Additional information
Publisher’s note Springer Nature remains neutral with regard to jurisdictional claims in published maps and institutional affiliations.
Extended data figures and tables
Extended data fig. 1 pharmacological nmda receptor antagonism dose-dependently lowers body weight but induces hyperthermia..
a - f , Treatment of C57BL/6 J DIO mice with once-daily subcutaneous (s.c.) injections of 900 nmol kg −1 MK-801 (n = 8 mice and n = 4 cages), 1800 nmol kg −1 MK-801 (n = 6 mice and n = 3 cages) or vehicle (isotonic saline, n = 7 mice and n = 3 cages) over 7 days. One mouse and cage were excluded from vehicle group due to the development of constipation. a , Schematic. b , Change in body weight. c , Daily food intake. d , Cumulative food intake. e , Change in fat mass. f , Change in lean mass. g - l , Treatment of C57BL/6 J DIO mice with once-daily s.c. injections of 200 nmol kg −1 MK-801 (n = 8 mice and n = 4 cages), 600 nmol kg −1 MK-801 (n = 7 mice and n = 4 cages) or vehicle (isotonic saline, n = 7 mice and n = 4 cages) for 7 days. g , Schematic. h , Change in body weight. i , Cumulative food intake. j , Change in rectal temperature in response to treatment on day 7. k , Area under curve (AUC) of j . l , Baseline rectal temperature on day 7. m - p , Treatment of C57BL/6 J DIO mice with once-daily s.c. injections of 50 µmol kg −1 memantine (n = 6 mice and n = 3 cages), 125 µmol kg −1 memantine (n = 6 mice and n = 3 cages) or vehicle (isotonic saline, n = 6 mice and n = 3 cages) over 7 days. m , Schematic. n , Change in body weight. o , Daily food intake. p , Cumulative food intake. Data analysed by one-way ANOVA, multiple comparison, Bonferroni post hoc test ( e , f , k and l ) and two-way repeated measures ANOVA to assess main effects of treatment ( b , d , h - j , n and p ). Data represents mean ± SEM; * P < 0.05, ** P < 0.01, *** P < 0.001, **** P < 0.0001. Detailed statistics are in Supplementary Table 1 . The diagrams in a , g and m were created using BioRender.
Extended Data Fig. 2 Pharmacological characterization of GLP-1–MK-801.
a , Chemical synthesis of GLP-1–MK-801 conjugates with different cysteine homologues. b , In vitro stability assay in human plasma. The assay was performed as biological replicates of GLP-1–MK-801 (Cys-linked) ( n = 3), GLP-1–MK-801 (hCys-linked) ( n = 2), GLP-1–MK-801 (Pen-linked) ( n = 3). c , Change in body weight of DIO mice treated with once-daily s.c. injections of 100 nmol kg −1 MK-801 ( n = 8 mice), 100 nmol kg −1 GLP-1 ( n = 8 mice), 100 nmol kg −1 GLP-1–MK-801 (Cys-linked) ( n = 8 mice) or vehicle (isotonic saline, n = 8 mice) for 5 days. d , Change in body weight of DIO mice treated with once-daily s.c. injections of 100 nmol kg −1 GLP-1 ( n = 6 mice), 100 nmol kg −1 GLP-1–MK-801 (Pen-linked) ( n = 6 mice) or vehicle (isotonic saline, n = 6 mice) for 7 days. e , Degradation assay. GLP-1–MK-801 was incubated in PBS with 200 mM glutathione at pH 7.0. The assay was performed as triplicates ( n = 3). f , Murine GLP-1 receptor activation of GLP-1, GLP-1–MK-801, liraglutide and semaglutide in transiently transfected HEK293 cells. The data represents dose-response at 2 min and is normalized to the maximal GLP-1 response (100%). The assay was performed as duplicates ( n = 2). g , h , Electrophysiological recordings of GLP-1 receptor-positive neurons, which were identified using a tdtomato reporter, being stimulated with NMDA after 30 min of bath application of GLP-1 or GLP-1–MK-801. g , Current responses at a holding potential of −70 mV elicited by NMDA bath application in GLP-1 receptor-positive neurons of the arcuate nucleus (ARC) with bath application of 50 µM GLP-1–MK-801, 50 µM GLP-1, 50 µM MK-801 or artificial cerebrospinal fluid (aCSF). h , Bar graph summarizing the effect of NMDA-induced inward current after control (aCSF, n = 5 neurons), GLP-1 (50 μM, n = 5 neurons), MK-801 (50 μM, n = 5 neurons) or GLP-1–MK-801 (50 μM, n = 5 neurons). Bath application normalized to the first bath application of NMDA (100 μM). i - m , Electrophysiological recordings of POMC neurons stimulated with GLP-1–MK-801. i , Representative trace showing that 50 μM GLP-1–MK-801 acute bath application induces a depolarization of POMC neurons in ARC ( n = 6 of 17 neurons). Bar graphs summarizing the acute effect of 50 μM GLP-1–MK-801 on POMC neurons. j , Resting membrane potential, k , Action potential frequency n . l , Excitatory postsynaptic current (EPSC) frequency. m , EPSC amplitude of POMC neurons that were depolarized in response to GLP-1–MK-801 bath application. n - r , Calcium imaging of ARC brain slices. n , Representative image of Fura-2AM loaded cells in an ARC brain slice. Scalebar is 20 µm. o , Application of GLP-1 and GLP-1–MK-801 induced changes in fluorescence indicative of rises in intracellular calcium (%ΔF/F 0 ) as shown by these representative fluorescent responses of two different Fura 2-AM loaded cells to application of GLP-1 (1 µM) or GLP-1–MK-801 (1 µM). p , The amplitude of the change in fluorescence (%ΔF/F 0 ) elicited by GLP-1 (1 µM, n = 20 neurons) or GLP-1–MK-801 (1 µM, n = 38 neurons). q , Representative traces of intracellular calcium levels in response to application of NMDA (50 µM) following bath application of GLP-1 (1 µM) or GLP-1–MK-801 (1 µM). r , Quantification of NMDA-induced (50 µM) intracellular calcium rises (%ΔF/F 0 ) following bath application of GLP-1 (1 µM, n = 20 neurons) or GLP-1–MK-801 (1 µM, n = 38 neurons). s , t , Treatment of DIO mice with once-daily s.c. injections of 50 nmol kg −1 GLP-1–MK-801 ( n = 6 mice and n = 3 cages), 100 nmol kg −1 GLP-1–MK-801 ( n = 6 mice and n = 3 cages) or vehicle (isotonic saline, n = 6 mice and n = 3 cages) for 6 days. s , Change in body weight. t , Cumulative food intake. u , v , Treatment of C57BL/6 J DIO mice with once-daily s.c. injections of 100 nmol kg −1 GLP-1–MK-801 ( n = 8 mice and n = 4 cages), 300 nmol kg −1 GLP-1–MK-801 ( n = 8 mice and n = 4 cages) or vehicle (isotonic saline, n = 8 mice and n = 4 cages) for 14 days. u , Change in body weight. v , Cumulative food intake. Data analysed by paired two-tailed Student’s t-test ( j - m ), unpaired two-tailed Student’s t-test ( p and r ), one-way ANOVA, multiple comparison, Bonferroni post hoc test ( h ) and two-way repeated measures ANOVA to assess main effects of treatment ( c , d and s - v ). Data represents mean ± SEM; * P < 0.05, ** P < 0.01, *** P < 0.001, **** P < 0.0001. Detailed statistics are in Supplementary Table 1 .
Extended Data Fig. 3 Supplementary metabolic phenotyping of GLP-1–MK-801.
a - c , Treatment of DIO mice with once-daily s.c. injections of 100 nmol kg −1 MK-801 ( n = 7 mice and n = 4 cages), 100 nmol kg −1 GLP-1 ( n = 6 mice and n = 3 cages), 100 nmol kg −1 , GLP-1–MK-801 ( n = 6 mice and n = 3 cages), co-administration of 100 nmol kg −1 GLP-1 and 100 nmol kg −1 MK-801 ( n = 6 mice and n = 3 cages) or vehicle (isotonic saline, n = 7 mice and n = 4 cages) for 14 days. a , Schematic. b , Change in body weight. c , Cumulative food intake. d - g and o - s , Treatment of DIO mice in metabolic cages with once-daily s.c. injections of 100 nmol kg −1 MK-801 ( n = 7-8 mice), 100 nmol kg −1 GLP-1 ( n = 8 mice), 100 nmol kg −1 GLP-1–MK-801 ( n = 8 mice) or vehicle (isotonic saline, n = 8 mice) for 14 days. d , Schematic. e , Change in body weight. f , Change in fat mass. For MRI, two values were not registered from MK-801 group, two values were not registered from GLP-1 group and two values were not registered from GLP-1–MK-801 group due to MRI instrumental error. g , Change in lean mass. h - m , GLP-1–MK-801 corrects body weight and fat mass relative to age-matched chow-fed control mice. Treatment of DIO mice with once-daily s.c. injections of 100 nmol kg −1 GLP-1–MK-801 ( n = 8 mice and n = 4 cages), 300 nmol kg −1 GLP-1–MK-801 ( n = 8 mice and n = 4 cages) or vehicle (isotonic saline, n = 7 mice and n = 4 cages) in comparison with vehicle-treated age-matched chow-fed C57BL/6 J mice ( n = 16 mice and n = 8-11 cages) for 14 days. Three double-housed cages with age-matched control animals were split on day 7 of the experiment due to fighting. One mouse was found dead in vehicle group. h , Schematic. i , Change in body weight. j , Body weight on day 14. k , Cumulative food intake. l , Fat mass on day 14. m , Lean mass on day 14. n , Total locomotor activity of mice from indirect calorimetry study in Fig. 1i–p . o , Energy expenditure. One mouse in MK-801 group excluded due to sensor related deviation. p , Regression-based analysis of energy expenditure relative to body weight on day 14. q , Respiratory exchange ratio (RER). r , Average respiratory exchange ratio. s , Total locomotor activity. Data analysed by one-way ANOVA, multiple comparison, Bonferroni post hoc test ( f , g , j , l - n , r and s ), two-way repeated measures ANOVA to assess main effects of treatment ( b , c , e and k ), and ANCOVA computed with calR using body weight as a covariate ( p ). Data represents mean ± SEM; * P < 0.05, ** P < 0.01, *** P < 0.001, **** P < 0.0001. Detailed statistics are in Supplementary Table 1 . The diagrams in a , d and h were created using BioRender.
Extended Data Fig. 4 Evaluation of glucometabolic effects of GLP-1–MK-801.
a - g , Compound tolerance test in response to a single s.c. injection of 100 nmol kg −1 MK-801 ( n = 8 mice), 100 nmol kg −1 GLP-1 ( n = 8 mice), 100 nmol kg −1 , GLP-1–MK-801 ( n = 8 mice), or vehicle (isotonic saline, n = 8 mice) in DIO mice. a , Schematic. b , Compound tolerance test on day 0. c , AUC of b . d , Plasma insulin levels during compound tolerance test. One mouse and one datapoint in vehicle group was omitted from analysis as they had insulin levels outside the assay range. e , AUC of d . f , Plasma glucagon levels during compound tolerance test. One mouse in vehicle group had supraphysiological glucagon levels outside the assay range and was omitted from analysis. g , AUC of f . h - p , Glucose-stimulated insulin secretion. Treatment of DIO mice with once-daily s.c. injections of 100 nmol kg −1 MK-801 ( n = 8 mice and n = 4 cages), 100 nmol kg −1 GLP-1 ( n = 8 mice and n = 4 cages), 100 nmol kg −1 GLP-1–MK-801 ( n = 8 mice and n = 4 cages), calorie restriction (Cal. Res.) to match the weight loss of GLP-1–MK-801 ( n = 8 mice and n = 5 cages) or vehicle (isotonic saline, n = 8 mice and n = 5 cages) for 4 days. On day 4, an intraperitoneal glucose tolerance test was conducted with collection of tail blood at timepoints 0, 15 and 60 min. h , Schematic. i , Change in body weight. j , Cumulative food intake. k , Glucose tolerance test on day 4. l , AUC of k . m , Glucose-stimulated insulin secretion. One datapoint in vehicle group, one datapoint in MK-801 group and one mouse and one datapoint in GLP-1–MK-801 group were outside assay range and omitted from analysis. n , AUC of m . o , Percentage to baseline of m . p , AUC of o . q , Percentage to baseline of insulin tolerance test in Fig. 1v . r - z , Insulin and glucose tolerance compared to mice undergoing calorie restriction to match the weight loss trajectory of GLP-1–MK-801. Treatment of DIO mice with once daily s.c. injections of 100 nmol kg −1 MK-801 ( n = 8 mice and n = 4 cages), 100 nmol kg −1 GLP-1 ( n = 8 mice and n = 4 cages), 100 nmol kg −1 GLP-1–MK-801 ( n = 8 mice and n = 4 cages), calorie restriction (Cal. Res.) to match the weight loss of GLP-1–MK-801 ( n = 8 mice and n = 4 cages) or vehicle (isotonic saline, n = 8 mice and n = 3-4 cages) for 14 days. On day 8, an intraperitoneal insulin tolerance test was conducted and on day 14 an intraperitoneal glucose tolerance test was conducted. r , Schematic. s , Change in body weight. t , Cumulative food intake. Four datapoints were excluded for one cage in vehicle group due to food spillage on day 11-14. u , Insulin tolerance test on day 8. v , AUC of u . w , Percentage to baseline of u . x , AUC of w . y , Glucose tolerance test on day 14. z , AUC of y . Data analysed by one-way ANOVA, multiple comparison, Bonferroni post hoc test ( c , e , g , l , n , p , v , x and z ) and two-way repeated measures ANOVA to assess main effects of treatment ( i , j , q , s and t ). Data represents mean ± SEM; * P < 0.05, ** P < 0.01, *** P < 0.001, **** P < 0.0001. Detailed statistics are in Supplementary Table 1 . The diagrams in a , h and r were created using BioRender.
Extended Data Fig. 5 GLP-1–MK-801 is devoid of hallmark MK-801-induced adverse effects.
a , b , Supporting data to study in Fig. 1a–h (vehicle (isotonic saline), n = 10 mice, 100 nmol kg −1 MK-801 n = 10 mice, 100 nmol kg −1 GLP-1 n = 10 mice, 100 nmol kg −1 GLP-1–MK-801 n = 10 mice). a , Plasma alanine transaminase (ALT) activity. b , Plasma aspartate aminotransferase (AST) activity. Two samples were removed from vehicle group, i.e., one sample was non-detectable and for another sample the activity was a significant outlier (Grubbs test). c , Heart weights of mice in Extended Data Figs. 3d–g and 3o–s (vehicle n = 8 mice, 100 nmol kg −1 MK-801 n = 8 mice, 100 nmol kg −1 GLP-1 n = 8 mice, 100 nmol kg −1 GLP-1–MK-801 n = 8 mice). d - j , Assessment of cardiovascular safety following chronic treatment of chow-fed lean C57BL/6 J mice with once-daily s.c. injections of 100 nmol kg −1 MK-801 ( n = 9 mice and 4 cages), 100 nmol kg −1 GLP-1 ( n = 10 mice and 4 cages), 100 nmol kg −1 GLP-1–MK-801 ( n = 10 mice and 4 cages), or vehicle (isotonic saline, n = 10 mice and 4 cages) for 14 days. On day 14, mice were anesthetized, and blood pressure and echocardiograms were recorded. One mouse was euthanized on day 12 in MK-801 group due to sickness. d , Schematic. e , Change in body weight. f , Cumulative food intake. g , Heart rate in beats per minute (bpm). h , Mean arterial pressure. i , Systolic blood pressure. j , Diastolic blood pressure. k , Rectal temperature on day 14 of mice from study in Fig. 1a–h . Data analysed by one-way ANOVA, multiple comparison, Bonferroni post hoc test ( a - c and g - k ) and two-way repeated measures ANOVA to assess main effects of treatment ( e and f ). Data represents mean ± SEM; * P < 0.05, ** P < 0.01, **** P < 0.0001. Detailed statistics are in Supplementary Table 1 . The diagram in d was created using BioRender.
Extended Data Fig. 6 Assessment of MK-801 as a half-life extender of gut peptide hormones.
a - c , Treatment of DIO mice with once-daily s.c. injections of 100 nmol kg −1 GIP analogue ( n = 8 mice and n = 4 cages), 100 nmol kg −1 GIP–MK-801 ( n = 8 mice and n = 4 cages) or vehicle (isotonic saline, n = 8 mice and n = 4 cages) for 7 days. a , Schematic. b , Change in body weight. c , Cumulative food intake. d , In vitro stability assay of GIP–MK-801 ( n = 2) incubated in human plasma or PBS, pH = 7.4 ( n = 1). e - g , Treatment of DIO mice with once daily s.c. injections of 100 nmol kg −1 PYY analogue ( n = 7 mice and n = 6 cages), 100 nmol kg −1 PYY–MK-801 ( n = 7 mice and n = 4 cages), 100 nmol kg −1 GLP-1–MK-801 ( n = 7 mice and n = 5 cages) or vehicle (isotonic saline, n = 7 mice and n = 4 cages) for 6 days. The dose of PYY-based compounds was escalated at day 4 from 100 nmol kg −1 to 500 nmol kg −1 . e , Schematic. f , Change in body weight. g , Cumulative food intake. h - j , Treatment of DIO mice with once daily s.c. injections of 50 nmol kg −1 GIP/GLP-1 ( n = 6 mice and n = 3 cages), 50 nmol kg −1 GIP/GLP-1–MK-801 ( n = 6 mice and n = 3 cages) or vehicle (isotonic saline, n = 6 mice and n = 3 cages) for 5 days. h , Schematic. i , Change in body weight. j , Cumulative food intake. k , In vitro stability assay of GIP/GLP-1–MK-801 ( n = 3) incubated in human plasma. Data analysed by two-way repeated measures ANOVA to assess main effects of treatment ( b , c , f , g , i and j ). Data represents mean ± SEM; * P < 0.05, **** P < 0.0001. Detailed statistics are in Supplementary Table 1 and chemical structures are in Supplementary Fig. 1 . The diagrams in a , e and h were created using BioRender.
Extended Data Fig. 7 Bulk hypothalamic RNA sequencing data.
a - g , Supplementary analyses of RNA sequencing data shown in Fig. 3a–g, j, k . a , Top 5 most enriched functional terms for the 1417 differentially expressed genes that were downregulated following GLP-1–MK-801 treatment. b , Top 5 enriched functional terms for the 1501 differentially expressed genes that were upregulated following GLP-1–MK-801 treatment. c , Top four cellular component and top nine biological process functional terms identified using gene set enrichment (GSEA) analysis. d , Venn diagram showing the overlap between the SynGO database and the 2918 uniquely differentially expressed genes in response to treatment with GLP-1–MK-801. e , Sunburst plot of differentially expressed genes belonging to cellular component functional terms computed with SynGO. Enrichment is colour coded by Q value for the functional terms. All level terms identified have been labelled. f , Sunburst plot of differentially expressed genes belonging to biological process functional terms computed with SynGO. Enrichment is colour coded by Q value for the functional terms. All level terms identified have been labelled. g , Top seven enriched functional terms for cellular component and top 7 functional terms enriched for biological process identified by SynGO. Benjamini-Hochberg adjusted P values were used for all analyses ( a – g ). The diagram in d was created using BioRender.
Extended Data Fig. 8 Mass spectrometry-based proteomics analyses of hypothalamic nuclei in response to GLP-1–MK-801.
a - g , Proteomics data from study in Fig. 3h, i of mice treated with 100 nmol kg −1 MK-801 ( n = 8 mice and n = 4 cages), 100 nmol kg −1 GLP-1 ( n = 8 mice and n = 4 cages), 100 nmol kg −1 GLP-1–MK-801 ( n = 8 mice and n = 4 cages) or vehicle (isotonic saline, n = 8 mice and n = 4 cages) for 5 days. a , Change in body weight. b , Cumulative food intake. c , Top twelve cellular component and top nine biological process ontologies identified using gene set enrichment (GSEA) analysis. d , Venn diagram showing the overlap between the SynGO database and the 506 uniquely differentially expressed proteins in response to GLP-1–MK-801. e , Sunburst plot of differentially expressed proteins belonging to cellular component functional terms computed with SynGO. Enrichment is colour coded by Q value for the functional terms. All level terms identified have been labelled. f , Sunburst plot of differentially expressed proteins belonging to biological process functional terms computed with SynGO. Enrichment is colour coded by Q value for the functional terms. All level terms identified have been labelled. g , Top ten SynGO enriched functional terms for cellular component and biological process. Data analysed by two-way repeated measures ANOVA to assess main effects of treatment ( a and b ). Benjamini-Hochberg corrected P ( c - g ) Data represents mean ± SEM; **** P < 0.0001. Detailed statistics are in Supplementary Table 1 . The diagram in d was created using BioRender.
Extended Data Fig. 9 Assessment of aversion in rodents.
a - d , Chow-fed Wistar rats were dosed once-daily with s.c. injections of 100 nmol kg −1 MK-801 ( n = 12 rats), 100 nmol kg −1 GLP-1 ( n = 12 rats), 100 nmol kg −1 GLP-1–MK-801 ( n = 12 rats) or vehicle (isotonic saline, n = 13 rats) for three consecutive days while being offered the voluntary choice between chow and kaolin (a non-food product). a , Schematic. b , Change in body weight. c , Cumulative food intake. One food monitor did not work for one mouse in vehicle group. d , Time-resolved kaolin intake. One datapoint in GLP-1–MK-801 was omitted due to extensive food spillage. e - h , Chow-fed Sprague-Dawley rats were dosed once-daily with s.c. injections of 100 nmol kg −1 MK-801 ( n = 8 rats), 100 nmol kg −1 GLP-1 ( n = 8 rats), 100 nmol kg −1 GLP-1–MK-801 ( n = 8 rats) or vehicle (isotonic saline, n = 8 rats) for three consecutive days while being offered the choice between chow and kaolin. e , Schematic. f , Change in body weight. g , Cumulative food intake. h , Cumulative kaolin intake. Two data points were omitted from analysis in vehicle group due to extensive food spillage. i - m , Wheel running study investigating aversive behaviour in response to treatment. Lean chow-fed male C57BL/6 J mice were single-housed in cages equipped with a running wheel and allowed 3 days of habituation before being treated with once-daily s.c. injections of 100 nmol kg −1 GLP-1–MK-801 ( n = 8 mice), 10 nmol kg −1 semaglutide ( n = 8 mice) or vehicle (isotonic saline, n = 7 mice) for 5 days with daily measurements of running distance at the time of injection. One mouse in vehicle group was excluded from analysis due to sickness. i , Schematic. j , Change in body weight. k , Cumulative food intake. l , Daily running wheel distance during habituation and treatment period. One datapoint in vehicle group was excluded due to the running wheel got stuck between day -1 to day 0. m , Cumulative running wheel distance during treatment period. Data analysed by two-way repeated measures ANOVA to assess main effect effects of treatment ( b , c , f , g , j , k and m ) and two-way ANOVA, multiple comparison, Bonferroni post hoc test ( h ). Data represents mean ± SEM; * P < 0.05, ** P < 0.01, *** P < 0.001, **** P < 0.0001. Detailed statistics are in Supplementary Table 1 . The diagrams in a , e and i were created using BioRender.
Extended Data Fig. 10 Bulk RNA-sequencing of hypothalamic nuclei comparing GLP-1–MK-801 and semaglutide treatments.
a - i , Supporting data for study in Fig. 5a–e . Treatment of DIO mice with once-daily s.c. injections of 10 nmol kg −1 semaglutide ( n = 6 mice and n = 3 cages), 100 nmol kg −1 GLP-1–MK-801 ( n = 6 mice and n = 3 cages) or vehicle (isotonic saline, n = 6 mice and n = 3 cages) for 5 days. a , Schematic. b , Change in body weight. c , Cumulative food intake. d , Top five most enriched functional terms of the 2176 differentially expressed genes that were downregulated following GLP-1–MK-801 treatment relative to semaglutide. e , Top five most enriched functional terms for the 1866 differentially expressed genes that were upregulated following GLP-1–MK-801 treatment relative to semaglutide. f , Venn diagram showing the overlap between the SynGO database and the 4042 differentially expressed genes in response to GLP-1–MK-801 relative to semaglutide. g , Sunburst plot of differentially expressed genes belonging to cellular component functional terms computed with SynGO. Enrichment is colour coded by Q value for the functional terms. All level terms identified have been labelled. h , Sunburst plot of differentially expressed genes belonging to biological process functional terms computed with SynGO. Enrichment is colour coded by Q value for the functional terms. All level terms identified have been labelled. i , Top seven SynGO enriched functional terms for cellular component and biological process. Data analysed by two-way repeated measures ANOVA to assess main effects of treatment ( b and c ). Benjamini-Hochberg adjusted P ( d - i ). Data represents mean ± SEM; *** P < 0.001, **** P < 0.0001. Detailed statistics are in Supplementary Table 1 . The diagrams in a and f were created using BioRender.
Extended Data Fig. 11 Whole brain cFos expression in response to semaglutide, MK-801, GLP-1 and GLP-1–MK-801.
a - j , Whole-brain 3D mapping and quantification of cFOS responses to treatment single s.c. injection with 100 nmol kg −1 MK-801 ( n = 7 mice), 100 nmol kg −1 GLP-1 ( n = 8 mice), 100 nmol kg −1 GLP-1–MK-801 ( n = 7 mice) or vehicle (isotonic saline, n = 8 mice) in lean mice. One mouse in MK-801 group, GLP-1–MK-801 group and vehicle group were excluded from analysis due to sample processing related deviation. a , Schematic. b , Mouse brain images showing heatmap of averaged changes in cFOS expression in response to treatments relative to vehicle. Red areas indicate increased cFOS activity and blue areas indicate decreased cFOS activity. c , Heatmap of cFOS activity in brain regions involved in body weight regulation. Values exceeding the colour scale are presented with the numeric values. d , Volcano plot of brain regions regulated in response to MK-801 relative to vehicle. Selected regions are labelled. e , Volcano plot of brain regions regulated in response to GLP-1 relative to vehicle. Selected regions are labelled. f , Volcano plot of brain regions regulated in response to GLP-1–MK-801 relative to vehicle. Selected regions are labelled. g , Quantification of cFOS + cells in dorsal motor nucleus X (DMX). h , Quantification of cFOS + cells in nucleus of the solitary tract (NTS). i , Quantification of cFOS + cells in rostral linear nucleus raphe (RR). j , Quantification of cFOS + cells in Edinger-Westphal nucleus (EW). k - o , cFOS + whole-brain imaging data came from mice in Fig. 5f–i . One mouse in GLP-1–MK-801 group excluded due to sample processing related deviation. k , cFOS + cells in nucleus accumbens (NAc). l , cFOS + cells in ventral tegmental area (VTA). m , cFOS + cells in basolateral amygdala nucleus (BLA). n , cFOS + cells in central amygdala (CEA). o , cFOS + cells in agranular insular area (AI). Data analysed by one-way ANOVA, multiple comparison, Bonferroni post hoc test ( g - o ). Data analysed by one-way ANOVA, multiple comparison, Bonferroni post hoc test ( g - o ). Benjamini-Hochberg adjusted P ( d - f ). Data represents mean ± SEM; ** P < 0.01, *** P < 0.001, **** P < 0.0001. For brain region abbreviations please refer to ‘cFos whole-brain imaging’ in the methods section. Detailed statistics are in Supplementary Table 1 . The diagram in a was created using BioRender.
Extended Data Fig. 12 Bulk RNA sequencing of brainstem and nucleus accumbens after treatment with GLP-1–MK-801.
a - n , Brain nuclei for bulk RNA sequencing of the brainstem came from mice in Fig. 3h, i and Extended Data Fig. 8 treated with 100 nmol kg −1 MK-801 ( n = 8 mice), 100 nmol kg −1 GLP-1 ( n = 8 mice), 100 nmol kg −1 GLP-1–MK-801 ( n = 8 mice) or vehicle (isotonic saline, n = 8 mice) for 5 days. a , Schematic. b , Schematic highlighting the brainstem. c , Venn diagram of differentially expressed genes in response to treatments. d , Volcano plot for differentially expressed genes in response to GLP-1–MK-801. e , Top five most enriched functional terms for the 3594 differentially expressed genes between GLP-1–MK-801 and vehicle. f , Top five most enriched functional terms for the 2076 differentially expressed genes that were upregulated in response to GLP-1–MK-801 treatment relative to vehicle. g , Top five most enriched functional terms for the 1518 differentially expressed genes that were downregulated following GLP-1–MK-801 treatment relative to vehicle. h , Top eleven cellular component and top three biological process functional terms identified using gene set enrichment (GSEA) analysis. i , Venn diagram showing the overlap with the SynGO database and the 3594 differentially expressed genes in response to GLP-1–MK-801. j , Sunburst plot of differentially expressed genes belonging to cellular component functional terms computed with SynGO. Enrichment is colour coded by Q value for the functional terms. All level terms identified have been labelled. k , Sunburst plot of differentially expressed genes belonging to biological process functional terms computed with SynGO. Enrichment is colour coded by Q value for the functional terms. All level terms identified have been labelled. l , Schematic highlighting the nucleus accumbens. m , Venn diagram of differentially expressed genes in response to treatments. n , Volcano plot for differentially expressed genes of GLP-1–MK-801. Benjamini-Hochberg adjusted P ( c - n ). Detailed statistics are in Supplementary Table 1 . The diagrams in a - c , i , l and m were created using BioRender.
Supplementary information
Supplementary fig. 1, reporting summary, supplementary table 1.
Sample sizes ( n ) and statistical significance values ( P values) for all data panels in the study.
Source data
Source data fig. 1, source data fig. 2, source data fig. 3, source data fig. 4, source data fig. 5, source data extended data fig. 1, source data extended data fig. 2, source data extended data fig. 3, source data extended data fig. 4, source data extended data fig. 5, source data extended data fig. 6, source data extended data fig. 7, source data extended data fig. 8, source data extended data fig. 9, source data extended data fig. 10, source data extended data fig. 11, source data extended data fig. 12, rights and permissions.
Open Access This article is licensed under a Creative Commons Attribution 4.0 International License, which permits use, sharing, adaptation, distribution and reproduction in any medium or format, as long as you give appropriate credit to the original author(s) and the source, provide a link to the Creative Commons licence, and indicate if changes were made. The images or other third party material in this article are included in the article’s Creative Commons licence, unless indicated otherwise in a credit line to the material. If material is not included in the article’s Creative Commons licence and your intended use is not permitted by statutory regulation or exceeds the permitted use, you will need to obtain permission directly from the copyright holder. To view a copy of this licence, visit http://creativecommons.org/licenses/by/4.0/ .
Reprints and permissions
About this article
Cite this article.
Petersen, J., Ludwig, M.Q., Juozaityte, V. et al. GLP-1-directed NMDA receptor antagonism for obesity treatment. Nature (2024). https://doi.org/10.1038/s41586-024-07419-8
Download citation
Received : 23 November 2022
Accepted : 12 April 2024
Published : 15 May 2024
DOI : https://doi.org/10.1038/s41586-024-07419-8
Share this article
Anyone you share the following link with will be able to read this content:
Sorry, a shareable link is not currently available for this article.
Provided by the Springer Nature SharedIt content-sharing initiative
This article is cited by
Experimental obesity drug packs double punch to reduce weight.
- Asher Mullard
Nature (2024)
Dual-action obesity drug rewires brain circuits for appetite
- Tyler M. Cook
- Darleen Sandoval
By submitting a comment you agree to abide by our Terms and Community Guidelines . If you find something abusive or that does not comply with our terms or guidelines please flag it as inappropriate.
Quick links
- Explore articles by subject
- Guide to authors
- Editorial policies
Sign up for the Nature Briefing newsletter — what matters in science, free to your inbox daily.

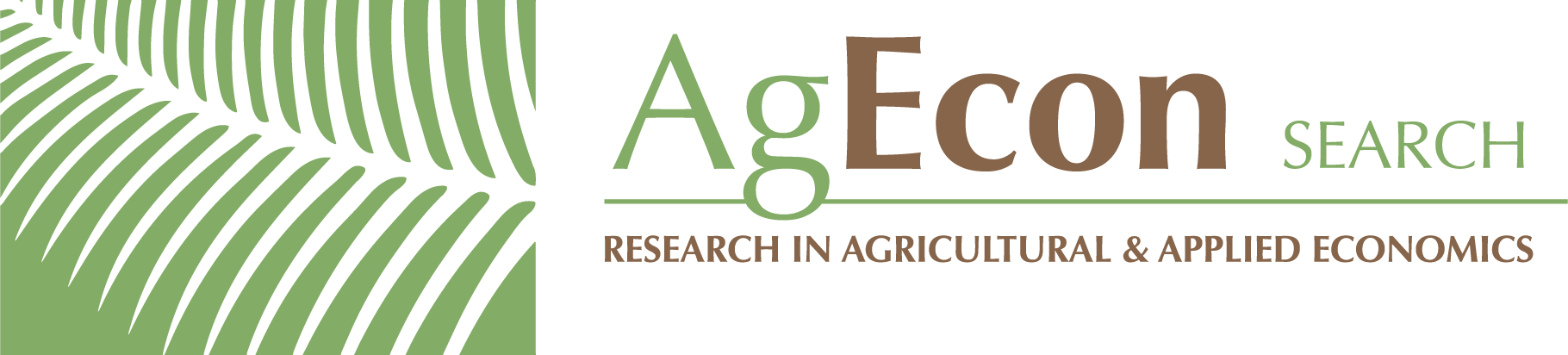
The obesity epidemic in America: Costly in food and lives
T he obesity epidemic is a global catastrophe attacking the world in slow motion — moving fast enough to wreak havoc on health, but not rapidly enough for people to always recognize its devastating effects.
America — and the rest of the world — is getting heavier every year. Experts say food addiction is this generation’s tobacco addiction, with the same harmful results.
In the United States, nearly 2 in 5 adults are now considered obese, and 1 in 11 have severe obesity, according to the National Health and Nutrition Examination Survey . The number of individuals who qualify as overweight is harder to calculate.
The World Obesity Organization estimates that by 2035, more than 4 billion people — over half of the world’s population — will be overweight or obese, using a body mass index calculator.
The growing obesity epidemic raises some important questions in the U.S. and abroad, like should the federal government change its food and tax policy to make unhealthy food less accessible? And how does shame about weight play into a person’s decisions about nutrition?
There is clear evidence about what factors are driving the epidemic, including a person’s environment, whether they have affordable access to healthy foods, poor gut health and the too-slow pace of progress of prevention efforts, among other factors.
Calculating weight
Body mass index, or BMI, is determined using a somewhat complicated calculation using a person’s height and weight. Online calculators exist to help people determine their BMI.
If the BMI calculation is below 18.5, the person is considered underweight. The number considered a healthy weight is between 18.5 and 24.9, while the calculation above 25 is considered overweight. Anything 30 or above indicates obesity.
Excess weight has been linked to heart disease, sleep apnea, certain cancers, Type 2 diabetes, hypertension and a higher risk of stroke.
Food environment
As of March 2022, the World Health Organization reported that “more than 1 billion people worldwide are obese — 650 million adults, 340 million adolescents and 39 million children.”
The change has been fast: The World Health Organization separately said that in just the five years between 1995 and 2000 the number of obese adults worldwide increased by 100 million, to 300 million.
Dr. Tom Rifa i, founder of health consulting company Reality Meets Science , told the Deseret News that the environment you live in is “arguably the strongest factor” in influencing your food choices. He describes himself as a “flexitarian” lifestyle medicine expert. A flexitarian diet is flexible, but emphasizes plant-based foods and beverages. His Flex5 Lifestyle based eating pattern emphasizes minimally processed plant-predominant foods.
The European Public Health Alliance said one’s food environment includes the physical, economic, political and sociocultural context of the area where a person buys and eats food.
An “obesogenic” environment — one that promotes weight gain — is one with a lot of fast food restaurants and that encourages people to use their car over walking, according to the Obesity Evidence Hub.
Rifai said part of the problem in the obesity and overall health epidemic is the lack of access to healthy foods, while it’s easier to access foods that are calorie-rich, refined and highly processed — which he calls CRRAHP foods , a trademarked acronym.
Processed foods dominate most Americans’ plates, especially within the last 20 years.
A study by Northeastern University’s Network Science Institute found that 73% of the food supply in the United States is considered “ultra-processed.” These foods are cheaper, full of additives and are linked to diseases such as obesity, metabolic syndrome, heart disease and sleep apnea.
A senior research scientist involved in the study, Giulia Menichetti, said the results shocked her and her staff.
“It surprised me how a considerable amount of highly processed food is mistakenly considered healthy because the public narrative still focuses on one nutrient at a time, instead of evaluating food as a whole,” she said.
Menichetti and her team are among researchers working to inform people about America’s food industry and the lack of guidelines on healthy eating.
Affordable food
The Supplemental Nutrition Assistance Program, or SNAP, is the U.S.’s biggest food and nutrition assistance program, and is managed by the U.S. Department of Agriculture. SNAP provides a monthly benefit to eligible households to buy food, the amount based on a family’s income and family size.
As of 2019, 38 million people were enrolled in the program, which is about 12% of the country’s population.
Although the program’s goal is to combat food insecurity with nutritious food, it does not necessarily contribute to good nutrition.
Angela Rachidi , a senior fellow and the Rowe scholar in poverty studies at the American Enterprise Institute, said she respects the argument that people of all income levels should have the autonomy to purchase whatever food they want. But since SNAP (formerly food stamps) is a tax-funded program, she believes food purchased with the benefit should have nutrition standards to encourage people enrolled in the program to have healthier eating habits.
She also believes that federal food programs need to challenge the food industry by promoting healthy food. “There needs to be a counterweight to this intense marketing around food and what is healthy and what is not healthy,” she said. “So if you have a niche, your largest nutrition assistance program is funding all of these purchases of foods that are not nutritious. That sends a very clear message suggesting that these foods are okay.”
Rachidi cited statistics from the 2016 Department of Agriculture report in an article she wrote for the institute: “SNAP households spend almost 25% of their food budgets on sweetened beverages, frozen prepared foods and prepared desserts alone.”
Diet culture and body image
In 2022, close to half of Americans’ New Year's resolutions were fitness-related and 40% were weight-loss goals, according to reporting by NPR . The U.S. spends an estimated $30 billion on the health and diet industry every year.
Body image researcher Nadia Craddock told NPR that diet culture is a group of social expectations “telling us that there’s one way to be and one way to look and one way to eat and that we are a better person, we’re a more worthy person if our bodies are a certain way.”
Michelle Lewis, a Salt Lake City weight counselor and licensed clinical social worker, said there is correlation between increasing obesity and diet culture — a pattern in history that when diet culture is popular, obesity numbers rise.
The reason, she said, is that unhealthy habits are promoted by diet culture. People will either lose weight unhealthily or ignore their weight in defiance, creating two extremes.
“When we feel bad about ourselves and our bodies, shame is a huge contributor to emotional eating. If you have decided not to eat something like sugar, but then do, you beat yourself up and feel shame. This is a big trigger for eating more to avoid feeling the shame,” Lewis said.
The shame that comes with weight stigma may mire a person in an endless cycle of weight loss and gain. Stigmas hinder progress for those who are overweight or obese and fail to address real solutions that can help people maintain a healthy weight, she said.
One of Sweden’s leading obesity researchers, Erik Hemmingsson, told the Deseret News, “Personally, I don’t think we will ever find solutions for the obesity epidemic until we get rid of obesity stigma. ... We need to find a balance in promoting a healthy body weight, but not at the expense of people’s relations with their bodies or other aspects of their mental health.”
Gender differences
Diet culture disproportionately affects women compared to men, starting at younger ages.
Larry Tucker , a professor of exercise science at Brigham Young University, said a big factor for weight gain in young women is childbirth. “Pregnancy usually carries with it weight gain — significant weight gain — and it takes an awful lot of effort to regain a lean body after,” he said.
A study of almost 14,000 randomly selected U.S. adults conducted by Tucker and Kayla Parker found that “women gained around twice as much as men over 10 years, on average 12 pounds for women and six for men. Including race in the analysis found that Black women had the greatest average weight gain over the 10-year study period, averaging 19.4 pounds. Conversely, Asian men experienced the least weight gain, at 2.9 pounds.”
“One of the best strategies to achieve a healthy weight is to focus on eating low-energy-dense foods, like fruits and nonstarchy vegetables,” Tucker said. “Foods, such as whole grains, with lots of fiber, are also good choices. Because most adults do not like the taste of non-starchy vegetables as much as sweet or high-fat foods, they should eat their vegetables first as a pre-load, which will help them feel full and consume fewer calories.”
- Will half the world be overweight by 2035 — and what will that mean for health and its cost?
- Your loaf of bread might be saltier than your bag of chips
- Want to improve your overall health? Look at these 5 areas of your life
Research has shown that people who immigrate to the U.S. often struggle with health issues related to weight gain.
Our stomachs contain about 300 trillion different microbes, which are bacteria designed to carry out proper functions and contribute to overall health. In a TED Talk on gut health, Dan Knight , who runs the Knights Lab at University of Minnesota, said his research found immigrants and refugees who reach the U.S. with healthy metabolic gut health quickly become at risk for obesity and other diseases due to processed foods in American diets.
“What we found is that when people come to the USA from these groups, they lose a large fraction of their microbiome, somewhere around 20%, and those who come to the USA and become obese lose about a third of their microbes. ... So we know that moving to the USA is sufficient to cause a dramatic change in your microbiome, probably not for the better,” he said.
According to the Centers for Disease Control and Prevention , certain minority communities have significantly higher obesity prevalence.
The CDC website lists the number of states with obesity rates higher than 35% among different races:
- 10 states had an obesity prevalence of 35% or higher among white adults.
- 27 states had an obesity prevalence of 35% or higher among Hispanic adults.
- 31 states for American Indian or Alaska Native adults.
- 36 states and the District of Columbia for Black adults.
“Racial and ethnic disparities in obesity underscore the need to address social determinants of health such as poverty, education, housing, health care access and to remove barriers to health,” per the CDC.
Social adversity, according to the National Institutes of Health , is an umbrella term that describes negative living situations like poverty, violent crime and poor parenting.
Hemmingsson was the lead author of an Obesity Reviews article that showed a correlation between social adversities, genetic makeup and obesity. He told the Deseret News by email, “There is no one magic bullet for stopping obesity. It will require multiple and prolonged changes to our societies to have any lasting impact. One of the major changes will need to be the promotion of greater socioeconomic equality (for example by helping families living in deprived areas) and some type of regulation of cheap ‘junk’ calories.”
Prevention efforts
A study in the National Library of Medicine found that “physicians with greater knowledge in obesity management were more likely to treat patients with weight-loss medications or bariatric surgery,” which is surgery on your digestive system to promote weight loss.
“Bariatric surgery remains the most durable option for people to lose weight and keep it off, particularly if someone has a significant amount of weight to lose or certain medical conditions. However, weight-loss surgery is not magic,” Elizabeth Hanna, a doctor of nursing practice and advanced practice registered nurse at St. Mark’s Weight Treatment Center, told the Deseret News.
A news release earlier this year by the American Academy of Pediatrics recommends health care providers’ treatment for teens with obesity include, “nutrition support, physical activity treatment, behavioral therapy, pharmacotherapy and metabolic and bariatric surgery.”
Hanna said maintaining a healthy lifestyle after surgery is challenging in an environment full of hyper-palatable foods.
Rifai said by “incentivizing and subsidizing healthier foods” to the public, the government can help reduce obesity numbers.
Peter Lurie, president of the Center for Science in the Public Interest , said in an interview with the Deseret News that the Washington, D.C.-based center pays special attention to helping design healthier food environments, especially for children.
Engineering retail spaces to place healthier food items in the open with high visibility, along with pushing restaurants to offer healthier food and beverage options for children, are two efforts in which Lurie’s team has made progress.
A study in the National Library of Medicine said that 52% of 448 women surveyed felt that their weight prevented them from getting appropriate health care, due to discrimination. To lower this percentage, the study said health care providers should try to communicate in a respectful and compassionate manner the seriousness of the disease to their patients who struggle with obesity. People who suffered from internalized weight stigma struggled more with binge eating and were much less likely to diet.
Lewis said addressing trauma is an important factor in tackling obesity.
“The biggest takeaway I hope your readers have is that weight and eating are so intrinsically tied to our emotional health that behavior is difficult, if not impossible to change without addressing the underlying emotional components that created their relationship with food or body.”

An official website of the United States government
The .gov means it’s official. Federal government websites often end in .gov or .mil. Before sharing sensitive information, make sure you’re on a federal government site.
The site is secure. The https:// ensures that you are connecting to the official website and that any information you provide is encrypted and transmitted securely.
- Publications
- Account settings
Preview improvements coming to the PMC website in October 2024. Learn More or Try it out now .
- Advanced Search
- Journal List
- HHS Author Manuscripts

Food Cravings and Body Weight: A Conditioning Response
Corresponding Author: John W. Apolzan, Pennington Biomedical Research Center, Louisiana State University System, 6400 Perkins Rd, Baton Rouge, LA 70808; [email protected] , Office Phone: 225-763-2827; Fax: 225-763-3022
Purpose of review:
To provide current information on interventions that alter food cravings. Specifically, dietary, physical activity, pharmacologic, and bariatric surgery interventions are examined. Lastly, the effects of social determinants and current controversies on food cravings are outlined.
Recent findings:
Dietary, pharmacologic, and bariatric surgery weight loss interventions decrease food cravings. Physical activity interventions also decrease cravings. There is potential to see differential responses in food cravings in different demographic and socioeconomic groups, but more research is needed.
Food cravings influence body weight. Food craving reductions are due to reductions in the frequency of consuming craved foods, independent of energy content, but further research is warranted. Most findings continue to support the conditioning model of food cravings.
Introduction:
Food craving is typically defined as frequent, intense desires to consume a particular type of food. Until recently, there was a debate about whether food cravings are reduced following energy restriction and if the conditioning model of cravings is valid. The conditioning model of food cravings states that cravings can develop from pairing consumption of certain foods with external (e.g., watching television) or internal (e.g. feeling sad) stimuli. Food cravings are believed to account for up to 11% of the variance in eating behavior and weight gain ( 1* ) and are positively associated with BMI ( 2 ). Thus, food cravings have the potential to be a modifiable predictor of body weight. This review will summarize and critique the most relevant areas of current food craving research.
Recently, the manuscript authored by Apolzan and colleagues utilized food intake and Food Craving Inventory (FCI) data from The POUNDS Lost multi-site weight loss randomized controlled trial (RCT) ( 3 ) to examine the association between change in FCI food item consumption and change in food cravings. Decreasing the frequency of consuming food items was associated with decreases in cravings for those items during weight loss ( 4** ). To the authors’ knowledge, this was the first time that it was demonstrated that food cravings are reduced following a reduction in individual FCI food item consumption during a RCT. Furthermore, the amount of energy consumed per FCI food item did not affect results. Additionally, while food cravings were positively associated with body weight changes during weight loss, the same was not found during a period of weight maintenance. These findings suggest that during weight loss interventions participants should decrease the frequency of consuming craved foods instead of lessening the amount consumed. However, the authors concluded that this hypothesis should be directly tested.
Secondly, a meta-analysis by Kahathuduwa et al. found that with energy restriction overall food cravings, as well as the sweets, high-fats, carbohydrates/starches, and fast food subscales, decrease in weight loss studies over 12 weeks ( 5** ). It should be noted that the majority of the participants were female, and 10 out of 13 studies included in the meta-analysis utilized the FCI opposed to other validated measures of food cravings. The results are important as they further validate that food cravings are due to a conditioned association between consumption of a specific type of food with a stimulus, further suggesting cravings are a result of classical conditioning.
Recently, Richard et al. tested associations similar to those found by Apolzan and colleagues. Persons with high and low trait chocolate cravings were instructed to abstain from chocolate consumption during a period of weight maintenance. After two weeks, the high cravers, but not the low cravers, reduced cravings ( 6** ). A similar response was found with snacking by the same group ( 7 ). However, even after the reduction the high cravers had double the score on the State version of the Food Craving Questionnaire (FCQ-State), thus floor effects were likely seen in low trait chocolate cravers ( 6** ). Thus, persons must be ‘cravers’ in order to have a reduction in cravings following a reduction in craving item consumption. This study again confirms classical conditioning of food cravings in those that regularly consume chocolate, but further suggests conditioning may also extend to periods of weight maintenance.
It is unclear whether results that support the conditioning model of food cravings are found in commercial weight management programs. An online questionnaire including the Control of Eating Questionnaire (CoEQ) was provided to persons attending a group based weight management program ( 8* ). Craving control and craving for sweet was lower in those dieting compared to those watching what they ate ( 8* ). Persons not dieting had higher craving control, craving for savory, and craving for sweet foods compared to the dieting and watching what they were eating groups. These results align well with the conditioning model of food cravings and the frequency of consumption manuscript ( 4** ). After controlling for age and BMI, nearly 5% of weight change variance was attributed to food cravings with the CoEQ. In participants no longer dieting, an association between cravings and body weight was no longer demonstrated. During weight maintenance in the POUNDS Lost manuscript, a similar result was found suggesting food cravings tend to be more tightly correlated with change in body weight during active weight loss. While this study suggests the conclusions of the conditioning theory are pragmatic, further research is needed to confirm the results that utilizes an RCT design.
Physical Activity/Inactivity:
With lifestyle interventions, participants are told to restrict energy consumption and to perform physical activity. It is clear that weight loss results in decreased food cravings but more investigation is needed into physical activity. It is thought that physical activity results in less weight loss than expected due to increased energy intake ( 9 ), which may lead to increased food cravings.
Bergouignan et al. found that walking 5 minutes out of every hour reduced food cravings before lunch compared to the continuous sitting intervention using the FCQ ( 10* ). Using data from the Energy Balance study, Drenowatz et al. discovered that meeting the physical activity recommendations was associated with less frequent craving for savory foods ( 11 ). Self-report data suggest that a decrease in the amount of physical activity was associated with an increase in cravings for sweet foods using the CoEQ. However, more resistance training was associated with a reduced likelihood to eat ( 11 ). Rocha et al. found that after 12 weeks of supervised aerobic exercise total cravings, and cravings of high-fat foods, fast food fats, and carbohydrates/starches decreased ( 12* ). Thus, food cravings are not increased with exercise and most studies demonstrate a reduction.
Pharmacotherapy:
Since food cravings are associated with body weight, several obesity drugs have been examined to understand their effect on food cravings. During a weight loss study that incorporated Medifast® meal replacements, participants consuming phentermine lost more weight (~3.3%) with a greater reduction in the fat and sweets subcategories of the FCI ( 13* ). Lorcaserin (Belviq) (10 mg 2x/daily) with and without phentermine (15 g 1 or 2x/daily) improved food cravings in a dose dependent manner ( 14* ). Recently semaglutide, a GLP-1 analogue, that is similar to liraglutide was investigated ( 15* ). It was provided for 12 weeks and decreased hunger and food cravings based on the CoEQ. Liraglutide (Saxenda) and naltrexone and bupropion (Contrave) have demonstrated similar reductions in cravings, thus they may be viable therapeutic targets ( 16 ). Importantly, pharmacologic therapies have been shown to decrease cravings.
Recently, Dalton et al. examined the effects of food craving in persons undergoing pharmacotherapy for weight loss ( 17** ). Using the CoEQ, early predictors of weight loss were tested. Craving control was the most significant predictor of BMI slope. Furthermore, craving control was examined by responder and non-responders. By 8 weeks (the first time point tested) there was a significant difference in weight loss between the groups. This continued for 56 weeks. While speculative, craving control may be a potential modifier for those that are less successful with weight loss during the initial weight loss period ( 17** ). Many obesity drugs reduce cravings, thus are a viable target for those with high cravings trying to lose or maintain weight. However, understanding how the conditioning theory of food cravings and the mechanism(s) of action for food craving reduction with these drugs needs to be further elucidated. Likely, persons are reducing frequency of consumption of craved foods, but this is clearly a critical area of future exploration.
Bariatric Surgery:
Currently, sleeve gastrectomy (SG) and Roux-en-Y-gastic bypass (RYGB) are the most common forms of weight loss surgery. Nance et al. recently examined cravings in patients undergoing SG and RYGB procedures ( 18* ). They found that both groups had decreased cravings on the high-fat, carbohydrates/starches, sweets, and fast food subscales of the FCI. There were only 8 patients in the SG group so the study was underpowered to examine differences in procedures, but results indicate that both procedures decreased cravings with energy restriction. Cushing et al. performed an elegant statistical analysis on a sample of adolescents that underwent RYGB surgery ( 19** ). Cravings were reduced in a nonlinear trajectory over 24 months. After 18 months, the trajectory flattened. The first 6 months saw the greatest reduction in cravings. Surprisingly, Sudan et al. did not find a similar reduction in cravings ( 2 ). There was no change in the FCI. A reduction was found 2 weeks after surgery, but cravings returned to baseline thereafter. This is the only recent study where the conditioning model of food cravings is not validated. This could be an anomaly or it could suggest that surgery evokes different mechanisms of food craving reductions than other targets (i.e. diet).
Social Determinants:
Most food craving research has been concentrated in weight loss studies with relatively homogeneous samples in regard to demographic and socioeconomic characteristics, which is not uncommon among weight loss studies. As reported for POUNDS Lost, the study sample was largely white (79%) and married (70%), had high educational attainment (90% some college or college graduate or beyond) and an annual household income over $50,000 (75%) ( 3 ). These descriptives highlight a sample primarily composed of non-minority, well-educated, high-resource individuals. Given this, secondary analyses of the POUNDS Lost study ( 4** , 20 ) provide a somewhat restricted examination of food cravings that largely focuses on socioeconomically advantaged individuals to the exclusion of those who obtain dissimilar demographic and socioeconomic characteristics, i.e., less advantaged and socially vulnerable groups with low income, less education, and fewer resources.
Surveying recent food craving studies does produce some empirical work that has more broadly focused on diverse samples outside of weight loss interventions. Malika and colleagues undertook a qualitative study that focused on the perception and conceptualization of food craving in low-income women, specifically those with less than 4 years of college and who were eligible for federal medical and social assistance ( 21* ). They concluded that food craving was not viewed aversively in their sample of low-income women and that popular food craving questionnaires and obesity interventions may miss the target with underserved populations. Standard assessments that frame food craving as problematic and subsequent weight loss treatments that target the resistance of food cravings may not be particularly effective for low-income women.
Chao and colleagues have carried out a number of cross-sectional and prospective analyses using community-based samples to investigate associations between food cravings and multiple nutrition and health indicators, such as body weight, stress, and appetite-related hormones ( 22 – 26** ). Among their results, compared to those who never smoked, current smokers reported more frequent cravings for high-fat foods and fast-food fats using the FCI, as well as greater consumption of these craved foods ( 24** ). This finding is particularly relevant given the higher prevalence of smoking found in populations with lower socioeconomic status. These studies highlight a need for further research that targets samples of low resource and disadvantaged populations to better understand how food cravings present in these populations and potential associations with diet, body weight, and other health behaviors.
When considering the importance of food craving to diet and weight status, an emergent social determinant of health is food insecurity, which is the lack of sufficient food in both quantity and quality. As noted by Apolzan and colleagues in their analysis of POUNDS Lost data, consumption of food items on a craving inventory is associated with cravings. This finding has specific implications for those who report being food insecure given that food insecurity is often cyclical with episodes of food adequacy and food shortage. Often associated with the receipt of monthly federal food assistance, food insecure persons are thought to experience a ‘feast/famine cycle,’ which is posited to be associated with increased body weight. Thus, food insecure persons with intermittent access to highly palatable, energy dense foods (i.e. increased frequency of consumption) could experience increased food cravings. Moreover, research indicates that food insecure persons report higher levels of binge eating and eating disorder pathology ( 27 ), which is important as Chao and colleagues ( 23** ) found that food cravings were associated with binge eating behavior and global eating disorder psychopathology. This confluence of potentially higher food cravings and disordered eating could perturb appetite regulation and body weight maintenance in food insecure individuals.
The implications of the conditioning model of food cravings are far reaching. Specifically, as socioeconomically disadvantaged populations with fewer resources suffer from poorer dietary intake, data is needed to understand the implications for conditioning of food cravings to findings drawn from traditional weight loss samples. More research is warranted to establish how cravings present in low-income and food insecure populations, if the traditional model of conditioning is corroborated in these groups, as well as potential perturbations of food craving and associated disordered eating.
Controversies:
The FCI, which may have the most robust food craving findings, was developed and validated in the southern United States (US). While foods used in this questionnaire are fairly standard, it is unknown if the results translate uniformly across the US. Others have suggested this hypothesis ( 25 ), and it is supported in the recent analysis by Apolzan and colleagues using the POUNDS Lost study, which found that Baton Rouge, LA, had a higher change in food craving with the change in frequency of FCI food item consumption compared with Boston, MA ( 4 ). In this case, it is unknown if geographic location or study site could potentially act as a proxy for other important confounding or unmeasured covariates, such as geographic differences in social determinants (e.g., education, income, etc.).
Another issue in the field of food cravings is the lack of consistency among findings. This may be due to the use of different questionnaires, including the FCI, FCQ-S, FCQ-T, and the CoEQ. The NIH has recently made a push for consistency with self-report instruments due to similar reasons and concerns. These aforementioned questionnaires examine different craving constructs, such as state vs. trait cravings, and therefore could produce different results. Standardizing craving questionnaire(s) for trait and state measures would be a positive step in future studies.
Conclusion:
Food cravings account for 7–11% ( 1* , 8* ) of variance in body weight, which is far more than genetics currently explains ( 28 ). Food cravings are important to consider when examining body weight outcomes and more research is needed to understand the mechanism(s) underlying this association. Stepwise designs could be utilized to examine early changes in food cravings and body weight and based on these assessments a more targeted study design could be developed to improve weight loss outcomes. Also, increased and /or decreased craved items should be directly consumed to determine if cravings are truly independent of energy content and follow the conditioning model of food cravings. Similar studies should be performed with dietary, pharmacologic, and physical activity interventions to ensure the theory is supported. Lastly, current research has convincingly set the stage for further studies to better elaborate relationships between various social determinants of health (e.g., socioeconomic position and food insecurity) and food craving. Since the field is still in a state of relative infancy, there are a number of topics to be elucidated, but the conditioning model of cravings continues to be supported.
Key Points:
- Food cravings influence eating behavior and body weight.
- Food cravings decline during periods of energy restriction, regardless of treatment.
- Little is currently known about potential differences in food cravings owing to social determinants.
- Recent findings suggest that the conditioning model of food cravings continues to be supported.
Acknowledgments:
Financial Support and Sponsorship: Supported in part by U54 GM104940 from the National Institute of General Medical Sciences of the National Institutes of Health, which funds the Louisiana Clinical and Translational Science Center (CAM), and a NORC Center Grant # P30DK072476 entitled “Nutrition and Metabolic Health through the Lifespan” sponsored by NIDDK (CKM). The content is solely the responsibility of the authors and does not necessarily represent the official views of the National Institutes of Health.
Conflicts of interest: There are no conflicts of interest.
References:
Papers of particular interest, published within the annual period of review, have been highlighted as:
* of special interest
** of outstanding interest

A .gov website belongs to an official government organization in the United States.
A lock ( ) or https:// means you've safely connected to the .gov website. Share sensitive information only on official, secure websites.
- Risk Factors
- Health Equity
- Cancer Data and Statistics
- Cancer Research
- Resource Library
- Types of Cancer
- HPV and Cancer
- Health Care Provider Resources
- Evidence-Based Interventions
Obesity and Cancer
- Being overweight or having obesity increases your risk of getting cancer.
- The risk of cancer increases with the more excess weight a person gains and the longer a person is overweight.
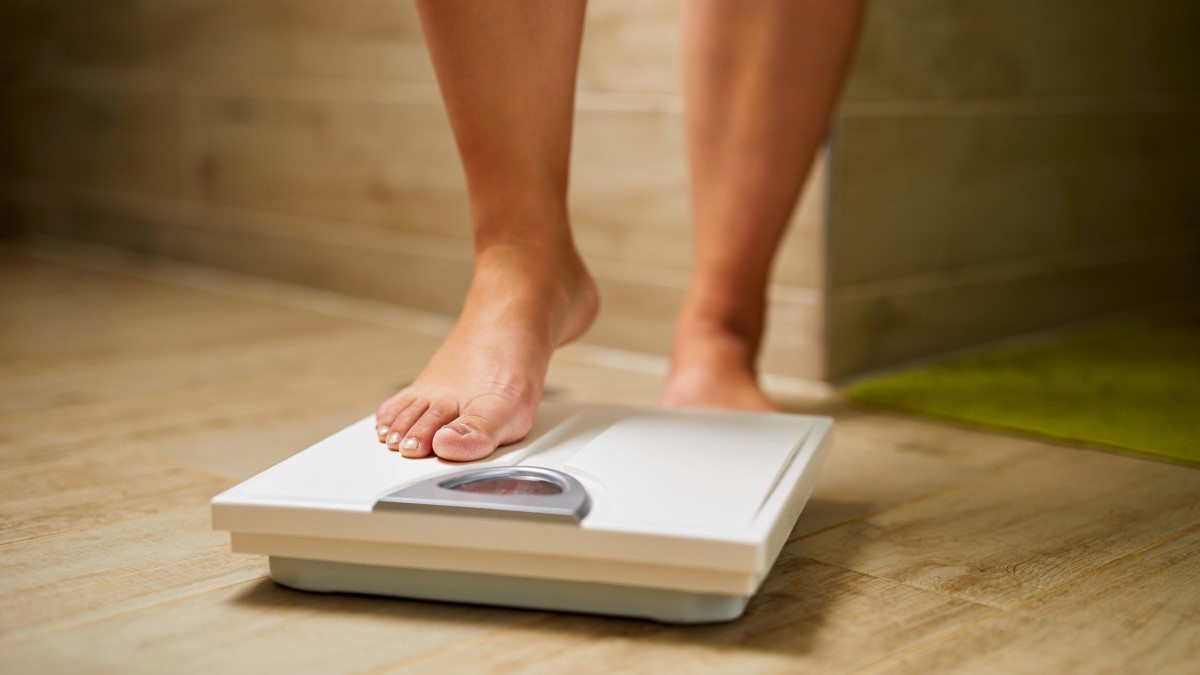
You may be surprised to learn that being overweight or having obesity are linked with a higher risk of getting 13 types of cancer. These cancers make up 40% of all cancers diagnosed in the United States each year.
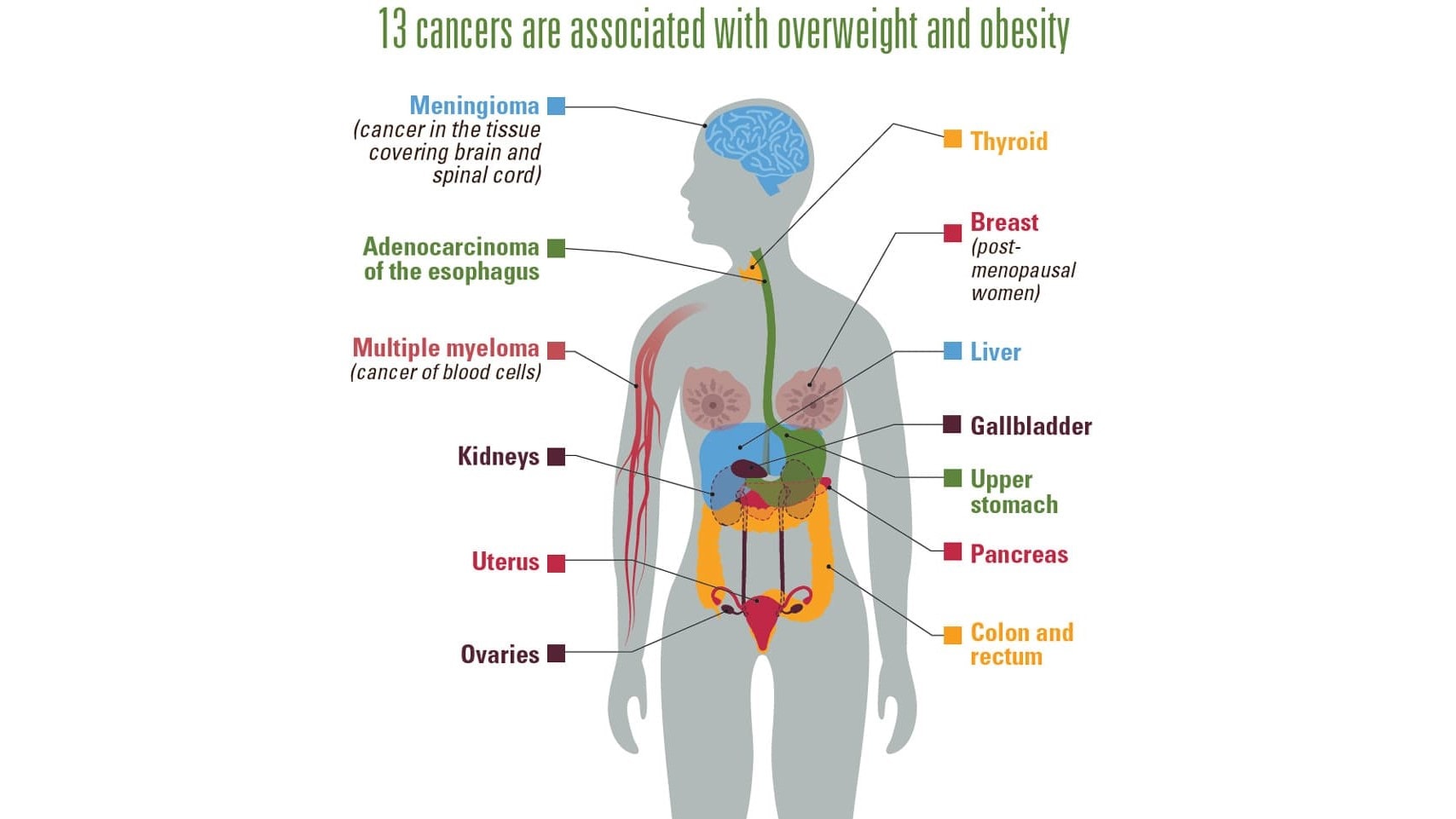
Many things are associated with cancer, but avoiding tobacco use and keeping a healthy weight are two of the most important steps you can take to lower your risk of getting cancer.
Being overweight or having obesity doesn’t mean that someone will definitely get cancer. But it does mean that they are more likely to get cancer than if they kept a healthy weight.
Which cancers are related to obesity?
Being overweight or having obesity is linked with a higher risk of getting 13 kinds of cancer:
- Adenocarcinoma of the esophagus.
- Breast (in women who have gone through menopause).
- Colon and rectum.
- Gallbladder.
- Upper stomach.
- Meningioma (a type of brain cancer).
- Multiple myeloma.
What is overweight and obesity?
Weight that is higher than what is considered a healthy weight for a given height is called overweight or obesity.
Body mass index (BMI) is used as a screening tool for overweight and obesity. For adults, a person with a BMI from 25.0 to 29.9 is considered overweight. A person with a BMI that is 30.0 or higher has obesity.
For children and teens, BMI is calculated the same way, but interpreted in a different way. BMI for children is often compared to the average BMI of other children in the same age range.
How can overweight and obesity lead to cancer?
Overweight and obesity can cause changes in the body including long-lasting inflammation and higher than normal levels of insulin, insulin-like growth factor, and sex hormones. These changes may lead to cancer. The risk of cancer increases with the more excess weight a person gains and the longer a person is overweight.
How can I lower my risk?
You can achieve a healthy weight by making healthy lifestyle choices. You can reduce your risk of obesity-related cancer by following a healthy eating plan and getting regular physical activity .
"Talk to Someone" Simulation
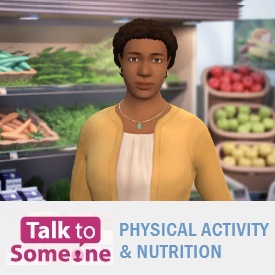
Talk to Someone: Physical Activity and Nutrition gives tips for cancer survivors to improve physical activity and healthy eating.
How can doctors help their patients keep a healthy weight?
Health care providers can help patients keep a healthy weight in several ways. For example, they can:
- Measure patients' weight, height, and BMI.
- Explain that keeping a healthy weight can lower a person's cancer risk.
- Connect patients and families with community services that provide healthy food and ways to be active.
- Encourage adult patients to participate in behavioral interventions recommended by the US Preventive Services Task Force. These interventions are designed to help patients lose 5% or more of their weight through dietary changes and increased physical activity.
- Screen for obesity in children and teens who are 6 years old or older. If needed, refer them to behavioral interventions to help them lose weight.
How many people have obesity or cancers linked to obesity?
Many people in the United States have obesity. Recent surveys have found that about 42% of adults and about 20% of children and adolescents have obesity. Adults with obesity have a higher risk of many serious diseases and health conditions, including cancer, than people with a healthy weight.
From 2005 to 2014, most cancers associated with overweight and obesity increased in the United States, while cancers associated with other factors decreased. During this time, the rate of new cancers associated with overweight and obesity (except colorectal cancer) increased 7%, while the rate of new cancers not associated with overweight and obesity dropped 13%. (The rate of new cases of colorectal cancer dropped 23% during this time. Screening tests can prevent this cancer.)
CDC's Data Visualizations tool provides data on the rates of new obesity-associated cancers among males and females in the United States. For example:
- More than 684,000 obesity-associated cancers occur in the United States each year, including more than 210,000 among men and 470,000 among women.
- Breast cancer after menopause is the most common obesity-associated cancer among women. Colorectal cancer is the most common obesity-associated cancer among men.
- More than 90% of new obesity-related cancers occur in men and women who are 50 or older.
- Obesity and Cancer (National Cancer Institute)
- Does Body Weight Affect Cancer Risk? (American Cancer Society)
Learn how to lower your cancer risk and what CDC is doing to prevent and control cancer.
For Everyone
Health care providers, public health.
- Share full article

Fast Food Forever: How McHaters Lost the Culture War
“Super Size Me” helped lead a backlash against McDonald’s. Twenty years on, the industry is bigger than ever.
Credit... Ben Wiseman
Supported by
By Brian Gallagher
- Published May 12, 2024 Updated May 24, 2024
The camera zooms in on a large woman, sitting on a cooler at the beach. It cuts to a shirtless man, also quite large, his face blurred out. The next shot shows another overweight man, sitting on a beach towel with plastic grocery bags arrayed in front of him.
“America has now become the fattest nation in the world. Congratulations,” a voice narrates. “Nearly 100 million Americans are today either overweight or obese.” At the end of this soliloquy, the opening credits roll — accompanied by Queen’s “Fat Bottomed Girls.”
So begins “ Super Size Me ,” which was released 20 years ago this month.
Directed by and starring Morgan Spurlock, the bootstrapped, lo-fi documentary was a smash hit, grossing more than $22 million on a $65,000 budget. Following Mr. Spurlock as he ate nothing but McDonald’s for 30 days — and the ill effects that diet had on his health — the film became the high-water mark in a tide of sentiment against fast food. McDonald’s, specifically, became a symbol for the glossy hegemony of American capitalism both at home and abroad.

“McJobs” became a term for low-paying, dead-end positions, “McMansions” for garish, oversize houses. In 1992, the political theorist Benjamin Barber used the term “McWorld” as shorthand for emergent neoliberal dominance; seven years later, protesters against the World Trade Organization seemed to agree, launching a newspaper box through a McDonald’s window during the “Battle of Seattle” marches.
Two years after that, Eric Schlosser’s “ Fast Food Nation ” was published. A broad indictment of the entire fast-food industry, the best seller accused the industry of being bad for the environment, rife with labor issues, culturally flattening and culinarily fattening.
That last point was the primary focus for Mr. Spurlock’s stunt. Awareness was raised, alarms were sounded and nightly news segments ensued. Six weeks after the film’s release, McDonald’s discontinued its Super Size menu, though a company spokesman said at the time that the film had “nothing to do with that whatsoever.”
It would have been easy to call the cultural moment a brand crisis for fast food.
But two decades later, not only is McDonald’s bigger than ever, with nearly 42,000 global locations, but fast food in general has boomed. There are now some 40 chains with more than 500 locations in the United States. Fast food is the second-largest private employment sector in the country, after hospitals, and about 36 percent of Americans — more than 115 million people — eat fast food on any given day. The three major appeals of fast food remain intact: It’s cheap, it’s convenient and people like the way it tastes.
“I used to own shares of McDonald’s,” said Jay Zagorsky , a professor at Boston University’s Questrom School of Business who has studied fast food in America. “Around the time of ‘Super Size Me,’ I sold off the shares, and now I’m saying to myself why? That was one of the greatest stocks.”
He’s right. The stock price of McDonald’s hit an all-time high in January, and has gone up nearly 1,000 percent since “Super Size Me” came out — nearly twice the return of the S&P 500.
While the sector’s financial performance was largely unaffected, there was a very real image problem, to the point that fast-food companies were compared to Big Tobacco . A big part of that problem had to do with children, who were seen not as informed consumers but rather as victims of their parents’ choices, the industry’s predatory advertising, or both. In fact, the inspiration for “Super Size Me” was a lawsuit filed by two New York City parents against McDonald’s, claiming that the company’s food had made their children severely obese.
In the end, the chains handled the brand crisis with the very tool — their most powerful — that had caused the problem in the first place: marketing.
‘Stop Listening to the Haters’
Historically, fast-food companies have been very astute about marketing to children, realizing decades ago that creating customers early means creating customers for life. At the peak of his fame in the 1980s, Ronald McDonald was in some countries more recognizable to children than Mickey Mouse. In 2000, 90 percent of children ages 6 to 9 visited a McDonald’s in a given month.
But as Frances Fleming-Milici, the director of marketing initiatives at the UConn Rudd Center for Food Policy and Health, put it, “If it’s marketed to children, it’s probably bad for you.”
That became increasingly clear in the mid-2000s. Childhood obesity rates had nearly tripled in 25 years, and the public outcry was growing more urgent. A consortium of large food brands, including McDonald’s, Burger King, PepsiCo and Coca-Cola, tried to get out in front of the problem. They formed the Children’s Food and Beverage Advertising Initiative , and the participating corporations self-imposed limits on advertising to children under 13 (later 12).
In place of that marketing to children, though, the big fast-food chains have found something arguably more potent, with McDonald’s, as ever, leading the way.
“They’re hyperfocusing on what they call fan-favorite moments, trying to essentially identify how we emotionally connect to McDonald’s,” said Kaitlin Ceckowski, who researches fast-food marketing strategies at Mintel, a market research agency. “What ‘human truths’ exist around their brand?”
That “human truths” idea — essentially, the genuine emotional resonance of eating McDonald’s — originated in part from Wieden+Kennedy and the Narrative Group, the two creative agencies that the chain hired in 2019 and 2020.
As W+K New York’s co-chief creative officer, Brandon Henderson, explained to AdAge in March, “When we first started with McDonald’s, they were hesitant to be themselves and had been listening to the haters since the ‘Super Size Me’ documentary. I think the big shift we gave them was to stop listening to the haters and listen to the fans.”
For the agencies, the lodestar of that strategy was the idea that “No matter who you are, everyone has a McDonald’s order.”
A Universal Experience
It turns out that years of saturating American childhood with fast food has paid real dividends. The 6-to-9-year-olds in that 2000 statistic are now younger millennials, among the group with the highest rate of fast-food consumption today. They have a lifetime of memories that connect them to fast-food brands, and to McDonald’s in particular.
All that needed to be done was to connect the power of that comfort and nostalgia to the power of celebrity. Fast food isn’t just cheap, accessible calories; it’s a universal experience. You’re eating the same fries as your idols.
That idea animated a 2020 Super Bowl ad that showed the McDonald’s orders of famous people both real (Kim Kardashian) and not (Dracula). That spot led, in turn, to a phenomenally successful campaign designed around the preferred orders of celebrities. The first of these, the Travis Scott menu, featured the go-to meal of the Houston rapper and doubled sales of Quarter Pounders in the first week. As a result, the market capitalization of McDonald’s went up by $10 billion.
Other chains have followed suit, with partnerships between Megan Thee Stallion and Popeyes, Ice Spice and Dunkin’, Justin Bieber and Tim Hortons, and Lil Nas X and Taco Bell, which named the pop star its “chief impact officer.”
“It’s not directly targeting children, but let’s be clear: The celebrity meals are for BTS, Travis Scott, Cardi B and J Balvin,” said Ms. Ceckowski. “These are people who resonate with younger audiences.”
They are also celebrities who resonate in particular with younger audiences of color, who tend to have higher rates of fast-food consumption than white consumers.
So while the vast majority of fast-food marketing is no longer aimed at children, per se — the ad budget expressly for kids’ meals and healthy menu items represents just 2 percent of the total spending — that only means that children are now going after the menu items they are seeing advertised. According to a Rudd Center study , this means they are simply ordering from the adult menu at a younger age.
In that same study, 20 percent of parents reported buying additional items for their children, which at Wendy’s could mean an order of fries to round out a meal that comes with apple slices, or at McDonald’s a soda to accompany a Happy Meal that now features only milk.
“If you look at where they put their ad dollars, it’s really just the highest-calorie items,” Ms. Fleming-Milici said. “These healthier menu items appear to be a bit of a public relations effort.”
In the age of social media, brands don’t even have to advertise expressly to children anyway, in the way they might have in the past, by buying a slot during Saturday-morning cartoons or on Nickelodeon. On TikTok and Instagram, kids of all ages see the same content we all do.
Younger people are also making content of their own, getting in on the marketing campaigns with thousands of videos of themselves ordering, unwrapping, eating — a sort of advertising Amway .
‘A Form of Civic Participation’
We may be living in a new era of social-media-driven viral marketing in the palms of millennial hands, but what hasn’t really changed is the food.
The Wendy’s Baconator, for instance, was introduced in 2007, three years after “Super Size Me” came out, and it remains one of the chain’s most popular items. A protein conglomeration of a half-pound of beef, six pieces of bacon and two slices of cheese, each burger delivers 1,010 calories and 67 grams of fat.
Burger King offers a triple Whopper, which carries similar nutritional values, even without the optional bacon and cheese. And at Chipotle, a brand often held up as evidence of healthier fast-food tastes, a standard chicken burrito can easily contain 1,100 calories. The classic Big Mac remains basically intact, at a relatively tame 590 calories.
There are still efforts to steer Americans, particularly American children, away from these options. In April, Senators Bernie Sanders, Cory Booker and Peter Welch introduced the Childhood Diabetes Reduction Act , which would ban advertising junk food to children and require stronger health and nutrition warning labels. The law “would take on the greed of the food and beverage industry and address the growing diabetes and obesity epidemics negatively impacting millions of American children and families across the country,” according to a news release from Mr. Sanders.
Fast food may be a tough habit to legislate away, though. In 2016, 91 percent of parents reported buying lunch or dinner for their child in the past week from one of the four biggest chains — a significant increase compared with the 79 percent who did in 2010 and the 83 percent in 2013.
The problem may be that while we are often scolded for eating at these restaurants, we are more often encouraged. There is a vast network of enticement — from huge marketing budgets, to family traditions, to just the tastiness of the meals — that pushes diners toward the drive-through.
In its harsh depiction of American obesity, “Super Size Me” seemed to judge individuals for their failure to resist that machine. But according to Virgie Tovar , who has written books about weight discrimination, that’s an unfair indictment — especially when applied to consumers for whom a trip to McDonald’s might well offer the most accessible version of the American dream.
“People in my generation, and certainly Gen Z, probably aren’t going to be homeowners,” Ms. Tovar said. “Job insecurity is really high. All these markers of what it means to be a successful American are increasingly inaccessible to these younger generations. And I think about the things that are : They’re these cheaper consumer goods, and some of them are food.”
Eating McDonald’s, she said, should be seen as “a form of civic participation — whether we want to admit it or not.”
Follow New York Times Cooking on Instagram , Facebook , YouTube , TikTok and Pinterest . Get regular updates from New York Times Cooking, with recipe suggestions, cooking tips and shopping advice .
An earlier version of this article misstated the number of Americans who eat fast food on a given day. It is more than 115 million, not 84 million.
How we handle corrections
More on Food and Dining
Keep tabs on dining trends, restaurant reviews and recipes..
Are American omakase restaurants the new steakhouses? They are fast becoming the preferred venues for young men of means to commune around expensive protein.
Making food for just yourself can feel like a burden night after night, but there are ways to make it less of a chore. Here’s how .
Snacking between meals isn’t really a thing in France. Unless, it’s “apéro.” The writer Rebekah Peppler shines a spotlight on a beloved French tradition that you can try at home.
How long does chicken last in the fridge? Here are answers to all your meat expiration date questions.
Eating in New York City
Mads Refslund’s creative ideas at the Brooklyn restaurant ILIS can be dreamily seductive, even if the execution isn’t always as compelling , Pete Wells, our dining critic, writes .
A Times food editor documented the high, the low and the mid from a week’s worth of TikTok restaurant suggestions.
We asked, you answered: Here are the restaurants our dining-obsessed readers would rank the best in the city.
Wells has unveiled his annual ranking of the 100 best restaurants in New York City .
Advertisement
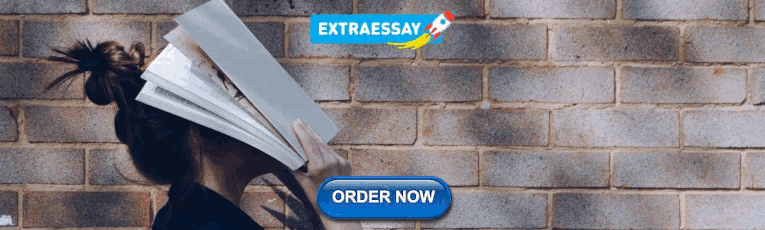
IMAGES
VIDEO
COMMENTS
Junk food consumption enhances the risk of both general and abdominal obesity. Open in a separate window. Additionally, junk food was found to increase NAc CP-AMPAR function in obesity-susceptible rats. AMPA upregulation occurred more promptly in obesity-susceptible rats and preceded the development of obesity.
The fourth hypothesis was about eating junk food behaviors which lead to obesity was supported by many researchers of the past with the reasoning of development of such behavior would resultantly set on the obesity (Huang et al., 2003). This is established from decades that behavior of eating unhealthy or the junk food leads to the obesity. so ...
In the fight against obesity, junk foods have become public enemy No. 1. But some people question whether certain foods are really the culprits. Two new studies come to very different conclusions.
Abstract. Despite limited empirical evidence, there is growing concern that junk food availability in schools has contributed to the childhood obesity epidemic. In this paper, we estimate the effects of junk food availability on BMI, obesity, and related outcomes among a national sample of fifth-graders. Unlike previous studies, we address the ...
To test the hypothesis that fast food consumers eat differently from nonconsumers for the remainder of diet outside of the fast food restaurant, we compared mean dietary intake, excluding fast foods, among fast food nonconsumers, low-consumers, and high-consumers by using t tests with Bonferroni correction for multiple comparisons. Food groups ...
The prevalence of obesity in the group that consumes junk food is 12%, while in the group that does not consume junk food is 6.3%. There was a significant relationship between consumption of junk food and the prevalence of obesity (x2 = 4,65; p=0,031) with Odds Ratio 2.56 and 95% Trust Interval 1.15-5.72. After being controlled by the parents ...
The moderating role of product knowledge hiding has also been measured on the relationship of junk food eating and obesity. Structural equation modeling is used using the software Smart-PLS for measuring the hypothesis with a sample size of 228 selected through purposive sampling. The sample consisted of kids and adolescents who were reached on ...
Increasing junk food (JF) consumption (JFC) among school-aged children is a public health issue that may affect the quality of their diet. The purpose of this study is to identify the factors associated with high-energy intake from JFC in children aged 7 to 8 years with different socioeconomic backgrounds and examine the relationship among JFC, healthy food consumption, and obesity.
or the junk food leads to the obesity. so, our hypothesis results were in accordance to such p revious researches. e h, sixth and seventh hypothesis responded to the media ting role of
Increasing junk food (JF) consumption (JFC) among school-aged children is a public health issue that may affect the quality of their diet. The purpose of this study is to identify the factors associated with high-energy intake from JFC in children aged 7 to 8 years with different socioeconomic backgrounds and examine the relationship among JFC, healthy food consumption, and obesity.
This widespread reliance on junk food is an increasing public health concern as the obesity rate has been rising steadily among U.S. youths for the past two decades.
Prevalence of food addiction in children and adolescents: a systematic review and meta-analysis. Obes Rev. 2021;22 (6):e13183. This systematic review estimated the prevalence of ultra-processed food addiction at 15% of youths in the general population and 19% of youths with overweight or obesity. Article PubMed PubMed Central Google Scholar.
A year-long study of the dietary habits of 9,341 Australians has backed growing evidence that highly processed and refined foods are the leading contributor of rising obesity rates in the Western world. The new study, in the latest issue of the journal Obesity conducted by the University of Sydney's Charles Perkins Centre (CPC), was based on ...
Figure 2 - The short- and long-term impacts of junk food consumption. In the short-term, junk foods can make you feel tired, bloated, and unable to concentrate. Long-term, junk foods can lead to tooth decay and poor bowel habits. Junk foods can also lead to obesity and associated diseases such as heart disease.
Background Obesity is influenced by a complex, multifaceted system of determinants, including the food environment. Governments need evidence to act on improving the food environment. The aim of this study was to review the evidence from spatial environmental analyses and to conduct the first series of meta-analyses to assess the impact of the retail food environment on obesity. Methods We ...
There is an intuitive appeal to the idea that portion sizes increase obesity, but long-term prospective studies would help to strengthen this hypothesis. Fast Food and Weight Fast food is known for its large portions, low prices, high palatability, and high sugar content, and there's evidence from studies in teens and adults that frequent ...
Junk Food and its Link to Childhood Obesity Erik Keough Johnson & Wales University - Providence, ... The second hypothesis is that if the child participates in a lower amount of physical activity outside of school then there is a higher chance that the participant may be obese.
Obesity may affect the normal body metabolism which may lead to many complications like Type II diabetes, cardiovascular disorders etc. • Consuming junk food, lack of physical activity, and psychological stress stand out as major causes for overweight in children which also supresses their immunity which is a menace during the spread of corona virus.
Genes, Junk Food and Weight. Researchers gained new insight into how genetics may influence obesity by studying how the mouse equivalent of a fast-food diet affects different mouse strains. The findings may help explain why some people gain weight more easily than others. Excess weight can raise your risk for type 2 and gestational diabetes ...
that "junk food" is the cause of obesity has little a priori plausibility, these articles in the popular press present a testable hypothesis that, in spite of some measurem ent impossibilities ...
1. Introduction. A person's health is often influenced by diet and the surrounding environment (Hoek et al., Citation 2017a).Based on the scaling up nutrition in 2021, a total of 68 million (35.54%) Indonesian adults were obese due to diet and inadequate activity (Republika.Co.Id, Citation 2021).In previous decades, FAO and WHO have reportedly campaigned intensively for a healthy lifestyle ...
Fast food consumption is strongly associated with weight gain and obesity. Fast food consumption could increase the risk of obesity and obesity-related diseases as a major public health issue [9, 10]. Obesity and overweight are the most important factors of non-communicable diseases related to years of life lost in cardiovascular diseases [11, 12].
GLP-1-directed delivery of the NMDA receptor antagonist MK-801 affects neuroplasticity in the hypothalamus and brainstem. Importantly, targeting of MK-801 to GLP-1 receptor-expressing brain ...
The popular press has triumphantly announced that the cause of the obesity epidemic is "junk food." After a moment's reflection, however, it seems likely that the true causal structure of the obesity epidemic can be neither single-equation nor univariate. Therefore, while the hypothesis that "junk food" is the cause of obesity has little a priori plausibility, these articles in the ...
Experts say food addiction is this generation's tobacco addiction, with the same harmful results. In the United States, nearly 2 in 5 adults are now considered obese, and 1 in 11 have severe ...
Food cravings influence body weight. Food craving reductions are due to reductions in the frequency of consuming craved foods, independent of energy content, but further research is warranted. Most findings continue to support the conditioning model of food cravings. Keywords: food craving, eating behavior, body weight, obesity, social ...
CDC's Data Visualizations tool provides data on the rates of new obesity-associated cancers among males and females in the United States. For example: More than 684,000 obesity-associated cancers occur in the United States each year, including more than 210,000 among men and 470,000 among women. Breast cancer after menopause is the most common ...
In 2000, 90 percent of children ages 6 to 9 visited a McDonald's in a given month. But as Frances Fleming-Milici, the director of marketing initiatives at the UConn Rudd Center for Food Policy ...
Junk food advertising, the prevalence of takeaways and the volume of processed foods available in supermarkets are all blamed for fuelling the crisis. ... Obesity increases demand on healthcare ...