Thesis Helpers

Find the best tips and advice to improve your writing. Or, have a top expert write your paper.
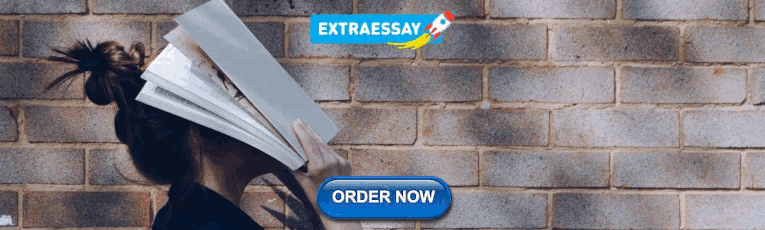
156 Hot Agriculture Research Topics For High Scoring Thesis
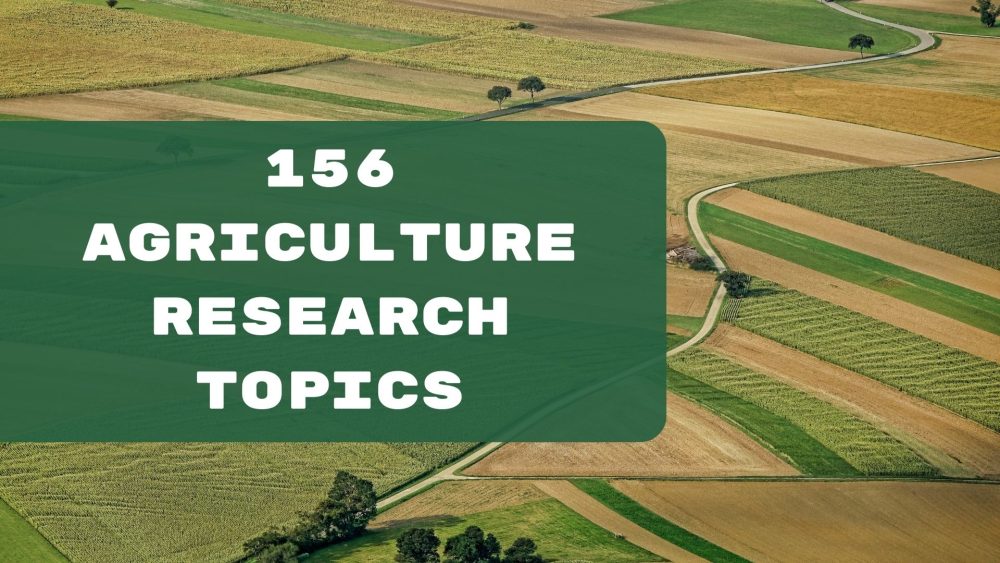
Are you preparing an agriculture research paper or dissertation on agriculture but stuck trying to pick the right topic? The title is very important because it determines how easy or otherwise the process of writing the thesis will be. However, this is never easy for many students, but you should not give up because we are here to offer some assistance. This post is a comprehensive list of the best 156 topics for agriculture projects for students. We will also outline what every part of a thesis should include. Keep reading and identify an interesting agriculture topic to use for your thesis paper. You can use the topics on agriculture as they are or change them a bit to suit your project preference.
What Is Agriculture?
Also referred to as farming, agriculture is the practice of growing crops and raising livestock. Agriculture extends to processing plants and animal products, their distribution and use. It is an essential part of local and global economies because it helps to feed people and supply raw materials for different industries.
The concept of agriculture is evolving pretty fast, with modern agronomy extending to complex technology. For example, plant breeding, agrochemicals, genetics, and relationship to emerging disasters, such as global warming, are also part of agriculture. For students studying agriculture, the diversity of the subject is a good thing, but it can also make selecting the right research paper, thesis, or dissertation topics a big challenge.
How To Write A Great Thesis: What Should You Include In Each Section?
If you are working on a thesis, it is prudent to start by understanding the main structure. In some cases, your college/ university professor or the department might provide a structure for it, but if it doesn’t, here is an outline:
- Thesis Topic This is the title of your paper, and it is important to pick something that is interesting. It should also have ample material for research.
- Introduction This takes the first chapter of a thesis paper, and you should use it to set the stage for the rest of the paper. This is the place to bring out the objective of the study, justification, and research problem. You also have to bring out your thesis statement.
- Literature Review This is the second chapter of a thesis statement and is used to demonstrate that you have comprehensively looked at what other scholars have done. You have to survey different resources, from books to journals and policy papers, on the topic under consideration.
- Methodology This chapter requires you to explain the methodology that was used for the study. It is crucial because the reader wants to know how you arrived at the results. You can opt to use qualitative, quantitative, or both methods.
- Results This chapter presents the results that you got after doing your study. Make sure to use different strategies, such as tables and graphs, to make it easy for readers to understand.
- Discussion This chapter evaluates the results gathered from the study. It helps the researcher to answer the main questions that he/she outlined in the first chapter. In some cases, the discussion can be merged with the results chapter.
- Conclusion This is the summary of the research paper. It demonstrates what the thesis contributed to the field of study. It also helps to approve or nullify the thesis adopted at the start of the paper.
Interesting Agriculture Related Topics
This list includes all the interesting topics in agriculture. You can take any topic and get it free:
- Food safety: Why is it a major policy issue for agriculture on the planet today?
- European agriculture in the period 1800-1900.
- What are the main food safety issues in modern agriculture? A case study of Asia.
- Comparing agri-related problems between Latin America and the United States.
- A closer look at the freedom in the countryside and impact on agriculture: A case study of Texas, United States.
- What are the impacts of globalisation on sustainable agriculture on the planet?
- European colonisation and impact on agriculture in Asia and Africa.
- A review of the top five agriculture technologies used in Israel to increase production.
- Water saving strategies and their impacts on agriculture.
- Homeland security: How is it related to agriculture in the United States?
- The impact of good agricultural practices on the health of a community.
- What are the main benefits of biotechnology?
- The Mayan society resilience: what was the role of agriculture?
Sustainable Agricultural Research Topics For Research
The list of topics for sustainable agriculture essays has been compiled by our editors and writers. This will impress any professor. Start writing now by choosing one of these topics:
- Cover cropping and its impact on agriculture.
- Agritourism in modern agriculture.
- review of the application of agroforestry in Europe.
- Comparing the impact of traditional agricultural practices on human health.
- Comparing equity in agriculture: A case study of Asia and Africa.
- What are the humane methods employed in pest management in Europe?
- A review of water management methods used in sustainable agriculture.
- Are the current methods used in agricultural production sufficient to feed the rapidly growing population?
- A review of crop rotation and its effects in countering pests in farming.
- Using sustainable agriculture to reduce soil erosion in agricultural fields.
- Comparing the use of organic and biological pesticides in increasing agricultural productivity.
- Transforming deserts into agricultural lands: A case study of Israel.
- The importance of maintaining healthy ecosystems in raising crop productivity.
- The role of agriculture in countering the problem of climate change.
Unique Agriculture Research Topics For Students
If students want to receive a high grade, they should choose topics with a more complicated nature.This list contains a variety of unique topics that can be used. You can choose from one of these options right now:
- Why large-scale farming is shifting to organic agriculture.
- What are the implications of groundwater pollution on agriculture?
- What are the pros and cons of raising factory farm chickens?
- Is it possible to optimise food production without using organic fertilisers?
- A review of the causes of declining agricultural productivity in African fields.
- The role of small-scale farming in promoting food sufficiency.
- The best eco-strategies for improving the productivity of land in Asia.
- Emerging concerns about agricultural production.
- The importance of insurance in countering crop failure in modern agriculture.
- Comparing agricultural policies for sustainable agriculture in China and India.
- Is agricultural technology advancing rapidly enough to feed the rapidly growing population?
- Reviewing the impact of culture on agricultural production: A case study of rice farming in Bangladesh.
Fun Agricultural Topics For Your Essay
This list has all the agricultural topics you won’t find anywhere else. It contains fun ideas for essay topics on agriculture that professors may find fascinating:
- Managing farm dams to support modern agriculture: What are the best practices?
- Native Americans’ history and agriculture.
- Agricultural methods used in Abu Dhabi.
- The history of agriculture: A closer look at the American West.
- What impacts do antibiotics have on farm animals?
- Should we promote organic food to increase food production?
- Analysing the impact of fish farming on agriculture: A case study of Japan.
- Smart farming in Germany: The impact of using drones in crop management.
- Comparing the farming regulations in California and Texas.
- Economics of pig farming for country farmers in the United States.
- Using solar energy in farming to reduce carbon footprint.
- Analysing the effectiveness of standards used to confine farm animals.
Technology And Agricultural Related Topics
As you can see, technology plays a significant role in agriculture today.You can now write about any of these technology-related topics in agriculture:
- A review of technology transformation in modern agriculture.
- Why digital technology is a game changer in agriculture.
- The impact of automation in modern agriculture.
- Data analysis and biology application in modern agriculture.
- Opportunities and challenges in food processing.
- Should artificial intelligence be made mandatory in all farms?
- Advanced food processing technologies in agriculture.
- What is the future of genetic engineering of agricultural crops?
- Is fertiliser a must-have for success in farming?
- Agricultural robots offer new hope for enhanced productivity.
- Gene editing in agriculture: Is it a benefit or harmful?
- Identify and trace the history of a specific technology and its application in agriculture today.
- What transformations were prompted by COVID-19 in the agricultural sector?
- Reviewing the best practices for pest management in agriculture.
- Analysing the impacts of different standards and policies for pest management in two countries of your choice on the globe.
Easy Agriculture Research Paper Topics
You may not want to spend too much time writing the paper. You have other things to accomplish. Look at this list of topics that are easy to write about in agriculture:
- Agricultural modernization and its impacts in third world countries.
- The role of human development in agriculture today.
- The use of foreign aid and its impacts on agriculture in Mozambique.
- The effect of hydroponics in agriculture.
- Comparing agriculture in the 20th and 21st centuries.
- Is it possible to engage in farming without water?
- Livestock owners should use farming methods that will not destroy forests.
- Subsistence farming versus commercial farming.
- Comparing the pros and cons of sustainable and organic agriculture.
- Is intensive farming the same as sustainable agriculture?
- A review of the leading agricultural practices in Latin America.
- Mechanisation of agriculture in Eastern Europe: A case study of Ukraine.
- Challenges facing livestock farming in Australia.
- Looking ahead: What is the future of livestock production for protein supply?
Emerging Agriculture Essay Topics
Emerging agriculture is an important part of modern life. Why not write an essay or research paper about one of these emerging agriculture topics?
- Does agriculture help in addressing inequality in society?
- Agricultural electric tractors: Is this a good idea?
- What ways can be employed to help Africa improve its agricultural productivity?
- Is education related to productivity in small-scale farming?
- Genome editing in agriculture: Discuss the pros and cons.
- Is group affiliation important in raising productivity in Centre Europe? A case study of Ukraine.
- The use of Agri-Nutrition programs to change gender norms.
- Mega-Farms: Are they the future of agriculture?
- Changes in agriculture in the next ten years: What should we anticipate?
- A review of the application of DNA fingerprinting in agriculture.
- Global market of agricultural products: Are non-exporters locked out of foreign markets for low productivity?
- Are production technologies related to agri-environmental programs more eco-efficient?
- Can agriculture support greenhouse mitigation?
Controversial Agricultural Project For Students
Our team of experts has searched for the most controversial topics in agriculture to write a thesis on. These topics are all original, so you’re already on your way towards getting bonus points from professors. However, the process of writing is sometimes not as easy as it seems, so dissertation writers for hire will help you to solve all the problems.
- Comparing the mechanisms of US and China agricultural markets: Which is better?
- Should we ban GMO in agriculture?
- Is vivisection a good application or a necessary evil?
- Agriculture is the backbone of modern Egypt.
- Should the use of harmful chemicals in agriculture be considered biological terror?
- How the health of our planet impacts the food supply networks.
- People should buy food that is only produced using sustainable methods.
- What are the benefits of using subsidies in agriculture? A case study of the United States.
- The agrarian protests: What were the main causes and impacts?
- What impact would a policy requiring 2/3 of a country to invest in agriculture have?
- Analysing the changes in agriculture over time: Why is feeding the world population today a challenge?
Persuasive Agriculture Project Topics
If you have difficulty writing a persuasive agricultural project and don’t know where to start, we can help. Here are some topics that will convince you to do a persuasive project on agriculture:
- What is the extent of the problem of soil degradation in the US?
- Comparing the rates of soil degradation in the United States and Africa.
- Employment in the agricultural sector: Can it be a major employer as the population grows?
- The process of genetic improvement for seeds: A case study of agriculture in Germany.
- The importance of potatoes in people’s diet today.
- Comparing sweet potato production in the US to China.
- What is the impact of corn production for ethanol production on food supply chains?
- A review of sustainable grazing methods used in the United States.
- Does urban proximity help improve efficiency in agriculture?
- Does agriculture create economic spillovers for local economies?
- Analysing the use of sprinkle drones in agriculture.
- The impact of e-commerce development on agriculture.
- Reviewing the agricultural policy in Italy.
- Climate change: What does it mean for agriculture in developed nations?
Advanced Agriculture Project Topics
A more difficult topic can help you impress your professor. It can earn you bonus points. Check out the latest list of advanced agricultural project topics:
- Analysing agricultural exposure to toxic metals: The case study of arsenic.
- Identifying the main areas for reforms in agriculture in the United States.
- Are developed countries obligated to help starving countries with food?
- World trade adjustments to emerging agricultural dynamics and climate change.
- Weather tracking and impacts on agriculture.
- Pesticides ban by EU and its impacts on agriculture in Asia and Africa.
- Traditional farming methods used to feed communities in winter: A case study of Mongolia.
- Comparing the agricultural policy of the EU to that of China.
- China grew faster after shifting from an agro to an industrial-based economy: Should more countries move away from agriculture to grow?
- What methods can be used to make agriculture more profitable in Africa?
- A comprehensive comparison of migratory and non-migratory crops.
- What are the impacts of mechanical weeding on soil structure and fertility?
- A review of the best strategies for restoring lost soil fertility in agricultural farmlands: A case study of Germany.
Engaging Agriculture Related Research Topics
When it comes to agriculture’s importance, there is so much to discuss. These engaging topics can help you get started in your research on agriculture:
- Agronomy versus horticultural crops: What are the main differences?
- Analysing the impact of climate change on the food supply networks.
- Meat processing laws in Germany.
- Plant parasites and their impacts in agri-production: A case study of India.
- Milk processing laws in Brazil.
- What is the extent of post-harvest losses on farming profits?
- Agri-supply chains and local food production: What is the relationship?
- Can insects help improve agriculture instead of harming it?
- The application of terraculture in agriculture: What are the main benefits?
- Vertical indoor farms.
- Should we be worried about the declining population of bees?
- Is organic food better than standard food?
- What are the benefits of taking fresh fruits and veggies?
- The impacts of over-farming on sustainability and soil quality.
Persuasive Research Topics in Agriculture
Do you need to write a paper on agriculture? Perfect! Here are the absolute best persuasive research topics in agriculture:
- Buying coffee produced by poor farmers to support them.
- The latest advances in drip irrigation application.
- GMO corn in North America.
- Global economic crises and impact on agriculture.
- Analysis of controversies on the use of chemical fertilisers.
- What challenges are facing modern agriculture in France?
- What are the negative impacts of cattle farms?
- A closer look at the economics behind sheep farming in New Zealand.
- The changing price of energy: How important is it for the local farms in the UK?
- A review of the changing demand for quality food in Europe.
- Wages for people working in agriculture.
Work With Experts To Get High Quality Thesis Paper
Once you pick the preferred topic of research, it is time to get down and start working on your thesis paper. If writing the paper is a challenge, do not hesitate to seek thesis help from our experts. We work with ENL writers who are educated in top universities. Therefore, you can trust them to carry out comprehensive research on your paper and deliver quality work to impress your supervisor. Students who come to us for assistance give a high rating to our writers after scoring top grades or emerging top in class. Our trustworthy experts can also help with other school assignments, thesis editing, and proofreading. We have simplified the process of placing orders so that every student can get assistance quickly and affordably. You only need to navigate to the ordering page to buy a custom thesis paper online.
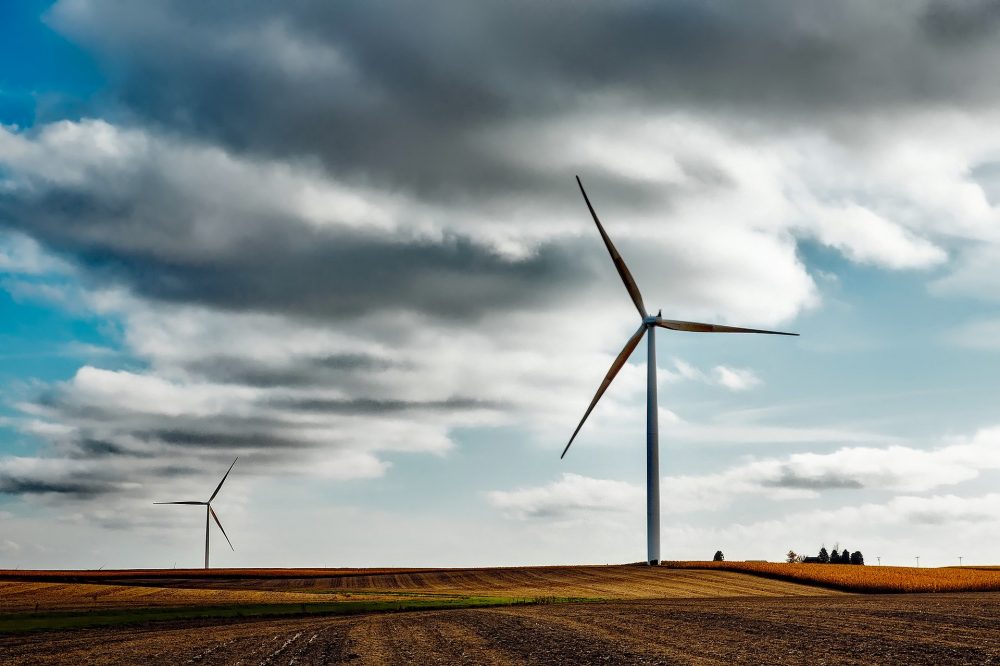
Make PhD experience your own
Leave a Reply Cancel reply
Your email address will not be published. Required fields are marked *
- Interesting
- Scholarships
- UGC-CARE Journals
45 Research Project Ideas in Agriculture – Innovative Approaches to Sustainable Farming
Explore 45 research project ideas in agriculture for sustainable farming.
Agriculture is a vast and dynamic field that plays a critical role in feeding the world’s population. As the global population continues to grow, the demand for food production is also increasing, making agriculture one of the most important sectors for ensuring food security and sustainable development. However, the challenges facing the agriculture industry today are numerous, ranging from climate change, soil degradation, water scarcity, and pest infestation to biodiversity loss and food waste.
To tackle these issues and promote sustainable agriculture, researchers and professionals in the field are continuously exploring new and innovative ways to improve agricultural practices, increase productivity, and reduce environmental impact. In this article, we will present 45 research project ideas in agriculture that can help address some of the most pressing issues facing the industry today.
These research projects cover a wide range of topics, from soil health and crop yields to livestock farming, aquaculture, and food systems, providing a comprehensive overview of the latest trends and innovations in agricultural research.
Whether you are a student, researcher, or professional in the field, these research project ideas can help guide your work and contribute to a more sustainable and resilient agriculture industry.
- Evaluating the effectiveness of natural pest control methods in agriculture.
- Investigating the effects of climate change on crop yields and food security.
- Studying the impact of soil quality on plant growth and crop yields.
- Analyzing the potential of precision agriculture techniques to increase yields and reduce costs.
- Assessing the feasibility of vertical farming as a sustainable solution to food production.
- Investigating the impact of sustainable agriculture practices on soil health and ecosystem services.
- Exploring the potential of agroforestry to improve soil fertility and crop yields.
- Developing strategies to mitigate the effects of drought on crop production.
- Analyzing the impact of irrigation management techniques on crop yields and water use efficiency.
- Studying the potential of biochar as a soil amendment to improve crop productivity.
- Investigating the effects of soil compaction on crop yields and soil health.
- Evaluating the impact of soil erosion on agriculture and ecosystem services.
- Developing integrated pest management strategies for organic agriculture.
- Assessing the potential of cover crops to improve soil health and reduce erosion.
- Studying the effects of biofertilizers on crop yields and soil health.
- Investigating the potential of phytoremediation to mitigate soil pollution in agriculture.
- Developing sustainable practices for livestock farming and manure management.
- Studying the effects of climate change on animal health and productivity.
- Analyzing the impact of animal feeding practices on meat quality and safety.
- Investigating the potential of aquaponics to increase food production and reduce environmental impact.
- Developing strategies to reduce food waste and loss in agriculture.
- Studying the effects of nutrient management practices on crop yields and environmental impact.
- Evaluating the potential of organic agriculture to improve soil health and reduce environmental impact.
- Investigating the effects of land use change on agriculture and biodiversity.
- Developing strategies to reduce greenhouse gas emissions from agriculture.
- Analyzing the impact of agricultural policies on food security and sustainability.
- Studying the potential of precision livestock farming to improve animal welfare and productivity.
- Investigating the impact of agrochemicals on soil health and biodiversity.
- Developing sustainable practices for fisheries and aquaculture.
- Studying the potential of bioremediation to mitigate pollution in aquaculture.
- Investigating the effects of climate change on fisheries and aquaculture.
- Developing strategies to reduce water pollution from agriculture and aquaculture.
- Studying the impact of land use change on water resources and aquatic ecosystems.
- Evaluating the potential of agroecology to promote sustainable agriculture and food systems.
- Investigating the impact of climate-smart agriculture practices on food security and resilience.
- Studying the potential of agrobiodiversity to improve crop productivity and resilience.
- Analyzing the impact of agricultural trade on food security and sustainability.
- Investigating the effects of urbanization on agriculture and food systems.
- Developing strategies to promote gender equity in agriculture and food systems.
- Studying the potential of agroforestry to promote biodiversity and ecosystem services.
- Analyzing the impact of food systems on public health and nutrition.
- Investigating the effects of climate change on pollination and crop yields.
- Developing strategies to promote agrotourism and rural development.
- Studying the potential of agroforestry to promote carbon sequestration and mitigate climate change.
- Analyzing the impact of agricultural subsidies on food security and sustainability.
I hope this article would help you to know the new project topics and research ideas in Agricultural.
- agriculture research
- crop yields
- food systems
- livestock farming
- Project Topics
- Research Ideas
- soil health
- sustainable farming
How to Write a Research Paper? A Complete Guide
14 websites to download research paper for free – 2024, 42 digital signal processing project ideas to explore, most popular, phd in india 2024 – cost, duration, and eligibility for admission, 100 connective words for research paper writing, phd supervisors – unsung heroes of doctoral students, india-canada collaborative industrial r&d grant, call for mobility plus project proposal – india and the czech republic, effective tips on how to read research paper, iitm & birmingham – joint master program, anna’s archive – download research papers for free, fulbright-kalam climate fellowship: fostering us-india collaboration, fulbright specialist program 2024-25, best for you, 24 best free plagiarism checkers in 2024, what is phd, popular posts, how to check scopus indexed journals 2024, 480 ugc-care list of journals – science – 2024, popular category.
- POSTDOC 317
- Interesting 258
- Journals 234
- Fellowship 128
- Research Methodology 102
- All Scopus Indexed Journals 92
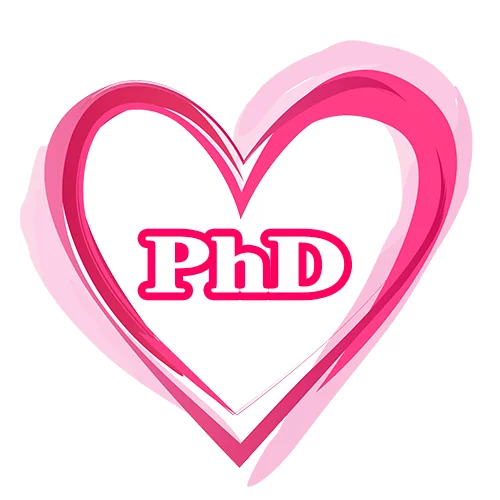
iLovePhD is a research education website to know updated research-related information. It helps researchers to find top journals for publishing research articles and get an easy manual for research tools. The main aim of this website is to help Ph.D. scholars who are working in various domains to get more valuable ideas to carry out their research. Learn the current groundbreaking research activities around the world, love the process of getting a Ph.D.
Contact us: [email protected]
Google News
Copyright © 2024 iLovePhD. All rights reserved
- Artificial intelligence

Thank you for visiting nature.com. You are using a browser version with limited support for CSS. To obtain the best experience, we recommend you use a more up to date browser (or turn off compatibility mode in Internet Explorer). In the meantime, to ensure continued support, we are displaying the site without styles and JavaScript.
- View all journals
- Explore content
- About the journal
- Publish with us
- Sign up for alerts
- Perspective
- Published: 23 December 2021
On-Farm Experimentation to transform global agriculture
- Myrtille Lacoste ORCID: orcid.org/0000-0001-6557-1865 1 , 2 ,
- Simon Cook ORCID: orcid.org/0000-0003-0902-1476 1 , 3 ,
- Matthew McNee 4 ,
- Danielle Gale ORCID: orcid.org/0000-0003-3733-025X 1 ,
- Julie Ingram ORCID: orcid.org/0000-0003-0712-4789 5 ,
- Véronique Bellon-Maurel 6 , 7 ,
- Tom MacMillan ORCID: orcid.org/0000-0002-2893-6981 8 ,
- Roger Sylvester-Bradley 9 ,
- Daniel Kindred ORCID: orcid.org/0000-0001-7910-7676 9 ,
- Rob Bramley ORCID: orcid.org/0000-0003-0643-7409 10 ,
- Nicolas Tremblay ORCID: orcid.org/0000-0003-1409-4442 11 ,
- Louis Longchamps ORCID: orcid.org/0000-0002-4761-6094 12 ,
- Laura Thompson ORCID: orcid.org/0000-0001-5751-7869 13 ,
- Julie Ruiz ORCID: orcid.org/0000-0001-5672-2705 14 ,
- Fernando Oscar García ORCID: orcid.org/0000-0001-6681-0135 15 , 16 ,
- Bruce Maxwell 17 ,
- Terry Griffin ORCID: orcid.org/0000-0001-5664-484X 18 ,
- Thomas Oberthür ORCID: orcid.org/0000-0002-6050-9832 19 , 20 ,
- Christian Huyghe 21 ,
- Weifeng Zhang 22 ,
- John McNamara 23 &
- Andrew Hall ORCID: orcid.org/0000-0002-8580-6569 24
Nature Food volume 3 , pages 11–18 ( 2022 ) Cite this article
3840 Accesses
74 Citations
141 Altmetric
Metrics details
- Agriculture
- Science, technology and society
- Scientific community
- Sustainability
Restructuring farmer–researcher relationships and addressing complexity and uncertainty through joint exploration are at the heart of On-Farm Experimentation (OFE). OFE describes new approaches to agricultural research and innovation that are embedded in real-world farm management, and reflects new demands for decentralized and inclusive research that bridges sources of knowledge and fosters open innovation. Here we propose that OFE research could help to transform agriculture globally. We highlight the role of digitalization, which motivates and enables OFE by dramatically increasing scales and complexity when investigating agricultural challenges.
This is a preview of subscription content, access via your institution
Access options
Access Nature and 54 other Nature Portfolio journals
Get Nature+, our best-value online-access subscription
24,99 € / 30 days
cancel any time
Subscribe to this journal
Receive 12 digital issues and online access to articles
111,21 € per year
only 9,27 € per issue
Buy this article
- Purchase on Springer Link
- Instant access to full article PDF
Prices may be subject to local taxes which are calculated during checkout
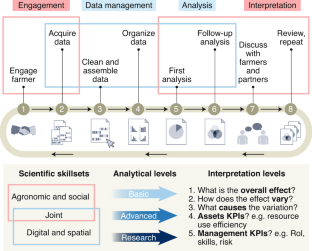
Similar content being viewed by others
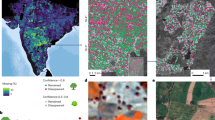
Severe decline in large farmland trees in India over the past decade
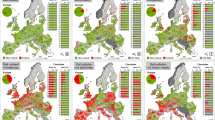
A unifying modelling of multiple land degradation pathways in Europe
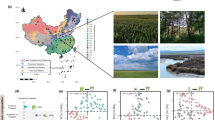
Land conversion to agriculture induces taxonomic homogenization of soil microbial communities globally
Data availability.
The authors declare that the data supporting the findings of this study are available within the paper and its Supplementary Information (sources of Figs. 1 – 3 ).
Whitfield, S., Challinor, A. J. & Rees, R. M. Frontiers in climate smart food systems: outlining the research space. Front. Sustain. Food Syst. 2 , https://doi.org/10.3389/fsufs.2018.00002 (2018).
Scoones, I. & Thompson, J. (eds) Farmer First Revisited: Innovation for Agricultural Research and Development 1st edn (Practical Action Publishing, 2009).
Stone, G. D. Towards a general theory of agricultural knowledge production: environmental, social, and didactic learning. Cult. Agric. Food Environ. 38 , 5–17 (2016).
Hansson, S. O. Farmers’ experiments and scientific methodology. Euro. J. Phil. Sci. 9 , 32 (2019).
Maat, H. & Glover, D. in Contested Agronomy: Agricultural Research in a Changing World (eds Sumberg, J. & Thompson, J.) 131–145 (Routledge, 2012).
Šūmane, S. et al. Local and farmers’ knowledge matters! How integrating informal and formal knowledge enhances sustainable and resilient agriculture. J. Rural Stud. 59 , 232–241 (2018).
Article Google Scholar
de Janvry, A., Sadoulet, E. & Rao, M. Adjusting Extension Models to the Way Farmers Learn Policy Brief No. 159 (FERDI, 2016).
Cross, R. & Ampt, P. Exploring agroecological sustainability: unearthing innovators and documenting a community of practice in Southeast Australia. Soc. Nat. Resour. 30 , 585–600 (2016).
Rickards, L., Alexandra, J., Jolley, C., Farhey, K. & Frewer, T. Review of Agricultural Extension (ACIAR, 2019).
MacMillan, T. & Benton, T. G. Engage farmers in research. Nature 509 , 25–27 (2014).
Article ADS CAS Google Scholar
Waters-Bayer, A. et al. Exploring the impact of farmer-led research supported by civil society organisations. Agric. Food Secur. 4 , 4 (2015).
Berthet, E. T. A., Barnaud, C., Girard, N., Labatut, J. & Martin, G. How to foster agroecological innovations? A comparison of participatory design methods. J. Environ. Plan. Manage. 59 , 280–301 (2015).
Cook, S. et al. An on-farm experimental philosophy for farmer-centric digital innovation. In 14th International Conference on Precision Agriculture (ISPA, 2018).
Cook, S. E., Cock, J., Oberthür, T. & Fisher, M. On-farm experimentation. Better Crops 97 , 17–20 (2013).
Google Scholar
Richardson, M. et al. Farmer research networks in principle and practice. Int. J. Agric. Sustain. https://doi.org/10.1080/14735903.2021.1930954 (2021).
Thompson, L. J. et al. Farmers as researchers: in‐depth interviews to discern participant motivation and impact. Agron. J. 111 , 2670–2680 (2019).
Sewell, A. M. et al. Hatching new ideas about herb pastures: learning together in a community of New Zealand farmers and agricultural scientists. Agric. Syst. 125 , 63–73 (2014).
Bramley, R. G. V., Lawes, R. & Cook, S. in Precision Agriculture for Sustainability and Environmental Protection (eds Oliver, M. A., Bishop, T. F. A. & Marchant, B. M.) 205–218 (Routledge, 2013).
Marchant, B. et al. Establishing the precision and robustness of farmers’ crop experiments. Field Crops Res. 230 , 31–45 (2019).
Briggs, J. Indigenous knowledge: a false dawn for development theory and practice? Progr. Dev. Stud. 13 , 231–243 (2013).
Caron, P., Biénabe, E. & Hainzelin, E. Making transition towards ecological intensification of agriculture a reality: the gaps in and the role of scientific knowledge. Curr. Opin. Environ. Sustain. 8 , 44–52 (2014).
Kool, H., Andersson, J. A. & Giller, K. E. Reproducibility and external validity of on-farm experimental research in Africa. Exp. Agric . 56 , 587–607 (2020).
de Roo, N., Andersson, J. A. & Krupnik, T. J. On-farm trials for development impact? The organisation of research and the scaling of agricultural technologies. Exp. Agric. 55 , 163–184 (2019).
Möhring, N. et al. Pathways for advancing pesticide policies. Nat. Food 1 , 535–540 (2020).
Sylvester-Bradley, R. et al. Agronōmics: transforming crop science through digital technologies. Adv. Anim. Biosci. 8 , 728–733 (2017).
Ruiz, J., Dumont, A. & Zingraff, V. in Penser le Gouvernement des Ressources Naturelles (eds Busca, D. & Lew, N.) 293–330 (Presses de l’Université Laval, 2019).
Fabregas, R., Kremer, M. & Schilbach, F. Realizing the potential of digital development: the case of agricultural advice. Science https://doi.org/10.1126/science.aay3038 (2019).
Dowd, A.-M. et al. The role of networks in transforming Australian agriculture. Nat. Clim. Change 4 , 558–563 (2014).
Article ADS Google Scholar
Klerkx, L., van Mierlo, B. & Leeuwis, C. in Farming Systems Research into the 21st Century: The New Dynamic (eds Darnhofer, I., Gibbon, D. & Dedieu, B.) 457–483 (Springer, 2012).
Ingram, J., Gaskell, P., Mills, J. & Dwyer, J. How do we enact co-innovation with stakeholders in agricultural research projects? Managing the complex interplay between contextual and facilitation processes. J. Rural Stud. 78 , 65–77 (2020).
Jackson, L. et al. Biodiversity and agricultural sustainagility: from assessment to adaptive management. Curr. Opin. Environ. Sustain. 2 , 80–87 (2010).
Laurent, A., Kyveryga, P., Makowski, D. & Miguez, F. A framework for visualization and analysis of agronomic field trials from on‐farm research networks. Agron. J. 111 , 2712–2723 (2019).
Kyveryga, P. M. On‐farm research: experimental approaches, analytical frameworks, case studies, and impact. Agron. J. 111 , 2633–2635 (2019).
Tremblay, N. in Precision Agriculture for Sustainability (ed. Stafford, J.) 145–168 (Burleigh Dodds Science Limited, 2019); https://doi.org/10.1201/9781351114592
Bullock, D. S. et al. The data‐intensive farm management project: changing agronomic research through on‐farm precision experimentation. Agron. J. 111 , 2736–2746 (2019).
Wyatt, J., Brown, T. & Carey, S. The next chapter in design for social innovation. Stanford Soc. Innov. Rev. 19 , 40–47 (2021).
Griffin, T. W., Fitzgerald, G. J., Lowenberg‐DeBoer, J. & Barnes, E. M. Modeling local and global spatial correlation in field‐scale experiments. Agron. J . https://doi.org/10.1002/agj2.20266 (2020).
Coudel, E., Tonneau, J.-P. & Rey-Valette, H. Diverse approaches to learning in rural and development studies: review of the literature from the perspective of action learning. Knowl. Manage. Res. Pract. 9 , 120–135 (2017).
Browning, D. M. et al. Emerging technological and cultural shifts advancing drylands research and management. Front. Ecol. Environ. 13 , 52–60 (2015).
Maxwell, B. et al. Can optimization associated with on-farm experimentation using site-specific technologies improve producer management decisions? In 14th International Conference on Precision Agriculture (2018).
Kindred, D. et al. Supporting and analysing on-farm nitrogen tramline trials so farmers, industry, agronomists and scientists can learn together. In 14th International Conference on Precision Agriculture (2018).
Oberthür, T. et al. Plantation intelligence applied oil palm operations: unlocking value by analysing commercial data. Planter 93 , 339–351 (2017).
Jin, H., Shuvo Bakar, K., Henderson, B. L., Bramley, R. G. V. & Gobbett, D. L. An efficient geostatistical analysis tool for on-farm experiments targeted at localised treatment. Biosys. Eng. 205 , 121–136 (2021).
Berthet, E. T., Hickey, G. M. & Klerkx, L. Opening design and innovation processes in agriculture: insights from design and management sciences and future directions. Agric. Syst. 165 , 111–115 (2018).
Curley, M. Twelve principles for open innovation 2.0. Nature 533 , 315–316 (2016).
Ryan, S. F. et al. The role of citizen science in addressing grand challenges in food and agriculture research. Proc. Biol. Sci . 285 , 20181977 (2018).
Herrero, M. et al. Innovation can accelerate the transition towards a sustainable food system. Nat. Food 1 , 266–272 (2020).
Fielke, S. J. et al. Conceptualising the DAIS: implications of the ‘digitalisation of agricultural innovation systems’ on technology and policy at multiple levels. NJAS 90–91 , 100296 (2019).
Cook, S., Jackson, E. L., Fisher, M. J., Baker, D. & Diepeveen, D. Embedding digital agriculture into sustainable Australian food systems: pathways and pitfalls to value creation. Int. J. Agric. Sustain. https://doi.org/10.1080/14735903.2021.1937881 (2021).
van Etten, J. et al. Crop variety management for climate adaptation supported by citizen science. Proc. Natl Acad. Sci. USA 116 , 4194–4199 (2019).
Ingram, J. & Maye, D. What are the implications of digitalisation for agricultural knowledge? Front. Sustain. Food Syst. 4 , https://doi.org/10.3389/fsufs.2020.00066 (2020).
McNee, M. Government Support for Farmer-Based Research in the Falkland Islands AAC Agenda 07.11.2019, Item 10 (Agricultural Advisory Committee, Falkland Islands Government, 2019).
Zhang, W. et al. Closing yield gaps in China by empowering smallholder farmers. Nature 537 , 671–674 (2016).
Lechenet, M., Dessaint, F., Py, G., Makowski, D. & Munier-Jolain, N. Reducing pesticide use while preserving crop productivity and profitability on arable farms. Nat. Plants 3 , 17008 (2017).
García, F. et al. La Red de Nutrición de la Región Crea Sur de Santa Fe: Resultados y Conclusiones de los Primeros Diez Años 2000-2009 (AACREA, 2010).
Posner, S. M., McKenzie, E. & Ricketts, T. H. Policy impacts of ecosystem services knowledge. Proc. Natl. Acad Sci. USA 113 , 1760–1765 (2016).
Moore, M.-L., Riddell, D. & Vocisano, D. Scaling out, scaling up, scaling deep. Strategies of non-profits in advancing systemic social innovation. J. Corp. Citizenship 58 , 67–84 (2015).
Payan, J.‐C. & Pichon, L. ApeX‐Vigne, Version 2020: Une Application Mobile Gratuite pour Faciliter le Suivi de la Croissance des Vignes et Estimer la Contrainte Hydrique (Institut Français de la Vigne et du Vin and Institut Agro, Montpellier SupAgro, 2020).
Samberg, L. H. A collaboration worth its weight in grain. Nature 537 , 624–625 (2016).
Download references
Acknowledgements
This study was funded by the Premier’s Agriculture and Food Fellowship Program of Western Australia. This Fellowship is a collaboration between Curtin and Murdoch Universities and the State Government. The Fellowship is the centrepiece of the Science and Agribusiness Connect initiative, made possible by the State Government’s Royalties for Regions program. Additional support was provided by the MAK’IT-FIAS Fellowship programme (Montpellier Advanced Knowledge Institute on Transitions – French Institutes for Advanced Study) co-funded by the University of Montpellier and the European Union’s Horizon 2020 Marie Skłodowska-Curie Actions (co-fund grant agreement no. 945408), the Digital Agriculture Convergence Lab #DigitAg (grant no. ANR-16-CONV-0004) supported by ANR/PIA, and the Elizabeth Creak Charitable Trust. Contributions toward enabling workshops were made by the USDA (USDA AFRI FACT Los Angeles 2017), the International Society for Precision Agriculture (ICPA Montreal 2018 OFE-C, On-Farm Experimentation Community), the National Key Research and Development Program of China (2016YFD0201303) and ADAS (Cambridge 2018), the European Conference for Precision Agriculture (ECPA Montpellier 2019) and the OECD Co-operative Research Program for ‘Biological resource management for sustainable agricultural systems – Transformational technologies and innovation’ towards ‘#OFE2021, the first Conference on farmer-centric On-Farm Experimentation – Digital Tools for a Scalable Transformative Pathway’. L. Tresh assisted with the design and preparation of Figs. 2 and 3. Members of the #OFE2021 Working Groups also contributed their experiences and insights.
Author information
Authors and affiliations.
Centre for Digital Agriculture, Curtin University, Perth, Western Australia, Australia
Myrtille Lacoste, Simon Cook & Danielle Gale
Montpellier Advanced Knowledge Institute on Transitions (MAK’IT), University of Montpellier, Montpellier, France
Myrtille Lacoste
Centre for Digital Agriculture, Murdoch University, Perth, Western Australia, Australia
Department of Agriculture, Falkland Islands Government, Stanley, Falkland Islands
Matthew McNee
Countryside and Community Research Institute, University of Gloucestershire, Cheltenham, UK
Julie Ingram
Technologies and methods for the agricultures of tomorrow (ITAP), University of Montpellier–National Research Institute for Agriculture, Food and Environment (INRAE)–L’Institut Agro, Montpellier, France
Véronique Bellon-Maurel
Digital Agriculture Convergence Lab (#DigitAg), National Research Institute for Agriculture, Food and Environment (INRAE), Montpellier, France
Centre for Effective Innovation in Agriculture, Royal Agricultural University, Cirencester, UK
Tom MacMillan
ADAS, Cambridge, UK
Roger Sylvester-Bradley & Daniel Kindred
Commonwealth Scientific and Industrial Research Organisation (CSIRO), Adelaide, South Australia, Australia
Rob Bramley
Saint-Jean-sur-Richelieu Research and Development Centre, Agriculture and Agri-Food Canada (AAFC), St-Jean-sur-Richelieu, Quebec, Canada
Nicolas Tremblay
School of Integrative Plant Science, Cornell University, Ithaca, NY, USA
Louis Longchamps
Institute of Agriculture and Natural Resources, University of Nebraska-Lincoln, Falls City, NE, USA
Laura Thompson
Watershed and Aquatic Ecosystem Interactions Research Centre (RIVE), Université du Québec à Trois-Rivières, Trois-Rivières, Québec, Canada
Latin America Southern Cone Group, International Plant Nutrition Institute (IPNI), Buenos Aires, Argentina
Fernando Oscar García
Faculty of Agricultural Sciences, National University of Mar del Plata, Balcarce, Argentina
Montana Institute on Ecosystems, Montana State University, Bozeman, MT, USA
Bruce Maxwell
Department of Agricultural Economics, Kansas State University, Manhattan, KS, USA
Terry Griffin
Southeast Asia Group, International Plant Nutrition Institute (IPNI), Penang, Malaysia
Thomas Oberthür
Business and Partnership Development, African Plant Nutrition Institute (APNI), Benguérir, Morocco
Scientific Direction of Agriculture, National Research Institute for Agriculture, Food and Environment (INRAE), Paris, France
Christian Huyghe
College of Resources and Environmental Sciences and National Academy of Agriculture Green Development, China Agricultural University, Beijing, China
Weifeng Zhang
National Animal Nutrition Program (NANP), United States Department of Agriculture (USDA), Pullman, WA, USA
John McNamara
Commonwealth Scientific and Industrial Research Organisation (CSIRO), Canberra, Australian Capital Territory, Australia
Andrew Hall
You can also search for this author in PubMed Google Scholar
Contributions
M.L. and S.C. developed the study concept. M.M., D.G., J.I., V.B.-M., T.M., R.S.-B. and A.H. contributed additional concept development. M.L. and D.G. obtained the data and prepared the results. M.L., M.M., L.T., D.K., F.O.G., B.M., V.B.-M., J.R., C.H. and W.Z. contributed data. M.L. wrote the manuscript with input from all other authors.
Corresponding author
Correspondence to Myrtille Lacoste .
Ethics declarations
Competing interests.
The authors declare no competing interests.
Additional information
Peer review information Nature Food thanks Carol Shennan, Petro Kyveryga, Nicolas Martin and the other, anonymous, reviewer(s) for their contribution to the peer review of this work.
Publisher’s note Springer Nature remains neutral with regard to jurisdictional claims in published maps and institutional affiliations.
Supplementary information
Supplementary information.
Sources for Figs. 1–3.
Rights and permissions
Reprints and permissions
About this article
Cite this article.
Lacoste, M., Cook, S., McNee, M. et al. On-Farm Experimentation to transform global agriculture. Nat Food 3 , 11–18 (2022). https://doi.org/10.1038/s43016-021-00424-4
Download citation
Received : 13 August 2020
Accepted : 05 November 2021
Published : 23 December 2021
Issue Date : January 2022
DOI : https://doi.org/10.1038/s43016-021-00424-4
Share this article
Anyone you share the following link with will be able to read this content:
Sorry, a shareable link is not currently available for this article.
Provided by the Springer Nature SharedIt content-sharing initiative
This article is cited by
Can machine learning models provide accurate fertilizer recommendations.
- Takashi S. T. Tanaka
- Gerard B. M. Heuvelink
- David S. Bullock
Precision Agriculture (2024)
Digital strategies for nitrogen management in grain production systems: lessons from multi-method assessment using on-farm experimentation
- A. F. Colaço
- B. M. Whelan
- R. A. Lawes
Analytic transparency is key for reproducibility of agricultural research
- Gudeta W. Sileshi
CABI Agriculture and Bioscience (2023)
Pesticide reduction amidst food and feed security concerns in Europe
- Kevin Schneider
- Jesus Barreiro-Hurle
- Emilio Rodriguez-Cerezo
Nature Food (2023)
Nutrient use efficiency has decreased in southwest China since 2009 with increasing risk of nutrient excess
- Guitang Liao
- Yongdong Wang
Communications Earth & Environment (2023)
Quick links
- Explore articles by subject
- Guide to authors
- Editorial policies
Sign up for the Nature Briefing: Anthropocene newsletter — what matters in anthropocene research, free to your inbox weekly.


An official website of the United States government
Here's how you know
The .gov means it’s official. Federal government websites often end in .gov or .mil. Before sharing sensitive information, make sure you’re on a federal government site.
The site is secure. The https:// ensures that you are connecting to the official website and that any information you provide is encrypted and transmitted securely.
About Grants
The lifecycle of grants and cooperative agreements consists of four phases: Pre-Award, Award, Post-Award, and Close Out.
Access to Data
The National Institute of Food and Agriculture is committed to serving its stakeholders, Congress, and the public by using new technologies to advance greater openness.
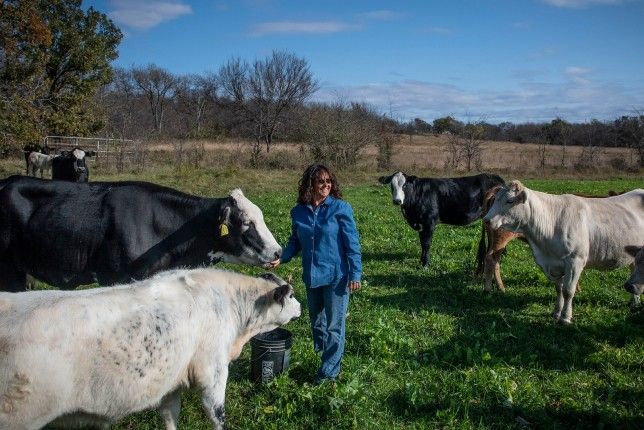
Access Data Gateway
The Data Gateway enables users to find funding data, metrics, and information about research, education, and extension projects that have received grant awards from NIFA.
View Resources Page
This website houses a large volume of supporting materials. In this section, you can search the wide range of documents, videos, and other resources.
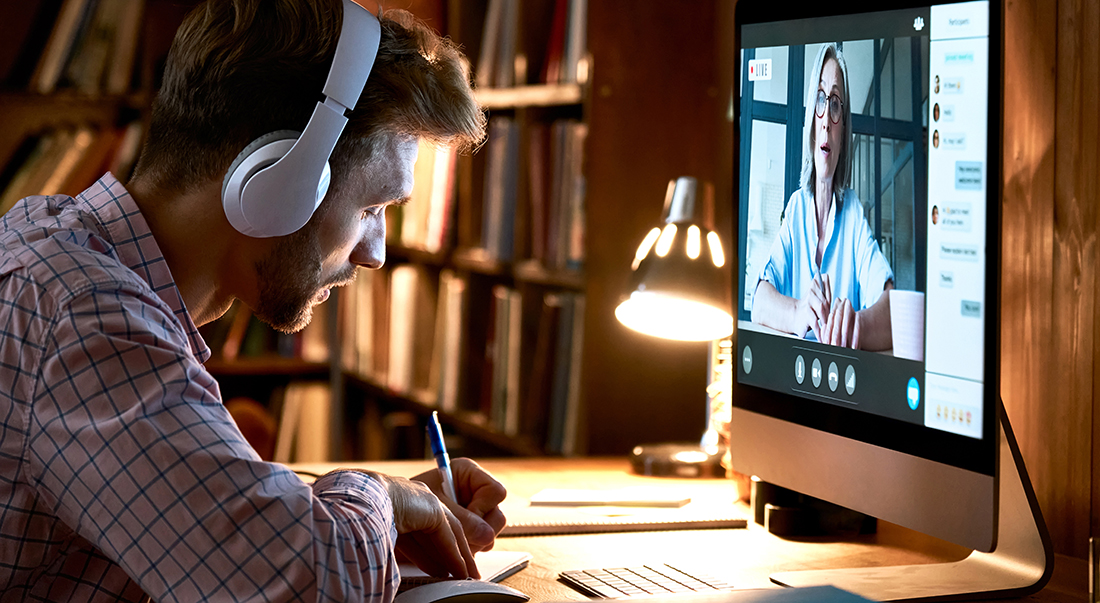
Featured Webinar
Second annual virtual grants support technical assistance workshop.
Check out this five-day workshop in March 2024 workshop, designed to help you learn about NIFA grants and resources for grants development and management.
The National Institute of Food and Agriculture provides leadership and funding for programs that advance agriculture-related sciences.

NIFA supports research, educational, and extension efforts in a wide range of scientific fields related to agricultural and behavioral sciences. In all of these areas, you will find NIFA working in pursuit of our vision. To address contemporary agricultural challenges, we seek to catalyze transformative discoveries and enhance education and engagement.

Advanced Technologies

Business and Economics

Environment

Farming and Ranching

Food and Nutrition Security
Human sciences.

International

Natural Resources

The Science of Food
Your feedback is important to us..
- Our Program Divisions
- Our Three Academies
- Government Affairs
- Statement on Diversity and Inclusion
- Our Study Process
- Conflict of Interest Policies and Procedures
- Project Comments and Information
- Read Our Expert Reports and Published Proceedings
- Explore PNAS, the Flagship Scientific Journal of NAS
- Access Transportation Research Board Publications
- Coronavirus Disease 2019 (COVID-19)
- Diversity, Equity, and Inclusion
- Economic Recovery
- Fellowships and Grants
- Publications by Division
- Division of Behavioral and Social Sciences and Education
- Division on Earth and Life Studies
- Division on Engineering and Physical Sciences
- Gulf Research Program
- Health and Medicine Division
- Policy and Global Affairs Division
- Transportation Research Board
- National Academy of Sciences
- National Academy of Engineering
- National Academy of Medicine
- Publications by Topic
- Agriculture
- Behavioral and Social Sciences
- Biography and Autobiography
- Biology and Life Sciences
- Computers and Information Technology
- Conflict and Security Issues
- Earth Sciences
- Energy and Energy Conservation
- Engineering and Technology
- Environment and Environmental Studies
- Food and Nutrition
- Health and Medicine
- Industry and Labor
- Math, Chemistry, and Physics
- Policy for Science and Technology
- Space and Aeronautics
- Surveys and Statistics
- Transportation and Infrastructure
- Searchable Collections
- New Releases
VIEW LARGER COVER
Science Breakthroughs to Advance Food and Agricultural Research by 2030
For nearly a century, scientific advances have fueled progress in U.S. agriculture to enable American producers to deliver safe and abundant food domestically and provide a trade surplus in bulk and high-value agricultural commodities and foods. Today, the U.S. food and agricultural enterprise faces formidable challenges that will test its long-term sustainability, competitiveness, and resilience. On its current path, future productivity in the U.S. agricultural system is likely to come with trade-offs. The success of agriculture is tied to natural systems, and these systems are showing signs of stress, even more so with the change in climate.
More than a third of the food produced is unconsumed, an unacceptable loss of food and nutrients at a time of heightened global food demand. Increased food animal production to meet greater demand will generate more greenhouse gas emissions and excess animal waste. The U.S. food supply is generally secure, but is not immune to the costly and deadly shocks of continuing outbreaks of food-borne illness or to the constant threat of pests and pathogens to crops, livestock, and poultry. U.S. farmers and producers are at the front lines and will need more tools to manage the pressures they face.
Science Breakthroughs to Advance Food and Agricultural Research by 2030 identifies innovative, emerging scientific advances for making the U.S. food and agricultural system more efficient, resilient, and sustainable. This report explores the availability of relatively new scientific developments across all disciplines that could accelerate progress toward these goals. It identifies the most promising scientific breakthroughs that could have the greatest positive impact on food and agriculture, and that are possible to achieve in the next decade (by 2030).
RESOURCES AT A GLANCE
- Press Release
- Report Highlights
- Interactive Overview of Breakthrough Opportunities
- Agriculture — Policy, Reviews and Evaluations
- Food and Nutrition — Policy, Reviews and Evaluations
- Earth Sciences — Policy, Reviews and Evaluations
- Environment and Environmental Studies — Policy, Reviews and Evaluations
Suggested Citation
National Academies of Sciences, Engineering, and Medicine. 2019. Science Breakthroughs to Advance Food and Agricultural Research by 2030 . Washington, DC: The National Academies Press. https://doi.org/10.17226/25059. Import this citation to: Bibtex EndNote Reference Manager
Publication Info
- Paperback: 978-0-309-47392-7
- Ebook: 978-0-309-47395-8
What is skim?
The Chapter Skim search tool presents what we've algorithmically identified as the most significant single chunk of text within every page in the chapter. You may select key terms to highlight them within pages of each chapter.
- Interactive Overview of Breakthrough Opportunities Read Description: This study identified five convergent breakthrough opportunities. Some are in early stages of development, while others are on the cusp of widespread application.
This brief video outlines some of the key background info and major conclusions of this report.
Report Release Webinar
The National Academies of Science, Engineering, and Medicine held a public release webinar for the report on Wednesday, July 18. The webinar featured a presentation and live Q&A by the co-chairs and two committee members of the report’s authoring committee:– Susan R. Wessler, NAS, University of California, Riverside (co-chair)– John D. Floros, New Mexico State University (co-chair)– Corrie Brown, University of Georgia– Gregory V. Lowry, Carnegie Mellon University
Copyright Information
The National Academies Press (NAP) has partnered with Copyright Clearance Center's Marketplace service to offer you a variety of options for reusing NAP content. Through Marketplace, you may request permission to reprint NAP content in another publication, course pack, secure website, or other media. Marketplace allows you to instantly obtain permission, pay related fees, and print a license directly from the NAP website. The complete terms and conditions of your reuse license can be found in the license agreement that will be made available to you during the online order process. To request permission through Marketplace you are required to create an account by filling out a simple online form. The following list describes license reuses offered by the NAP through Marketplace:
- Republish text, tables, figures, or images in print
- Post on a secure Intranet/Extranet website
- Use in a PowerPoint Presentation
- Distribute via CD-ROM
Click here to obtain permission for the above reuses. If you have questions or comments concerning the Marketplace service, please contact:
Marketplace Support International +1.978.646.2600 US Toll Free +1.855.239.3415 E-mail: [email protected] marketplace.copyright.com
To request permission to distribute a PDF, please contact our Customer Service Department at [email protected] .
What is a prepublication?
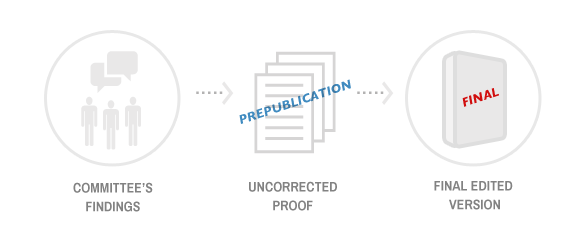
An uncorrected copy, or prepublication, is an uncorrected proof of the book. We publish prepublications to facilitate timely access to the committee's findings.
What happens when I pre-order?
The final version of this book has not been published yet. You can pre-order a copy of the book and we will send it to you when it becomes available. We will not charge you for the book until it ships. Pricing for a pre-ordered book is estimated and subject to change. All backorders will be released at the final established price. As a courtesy, if the price increases by more than $3.00 we will notify you. If the price decreases, we will simply charge the lower price. Applicable discounts will be extended.
Downloading and Using eBooks from NAP
What is an ebook.
An ebook is one of two file formats that are intended to be used with e-reader devices and apps such as Amazon Kindle or Apple iBooks.
Why is an eBook better than a PDF?
A PDF is a digital representation of the print book, so while it can be loaded into most e-reader programs, it doesn't allow for resizable text or advanced, interactive functionality. The eBook is optimized for e-reader devices and apps, which means that it offers a much better digital reading experience than a PDF, including resizable text and interactive features (when available).
Where do I get eBook files?
eBook files are now available for a large number of reports on the NAP.edu website. If an eBook is available, you'll see the option to purchase it on the book page.
View more FAQ's about Ebooks
Types of Publications
Consensus Study Report: Consensus Study Reports published by the National Academies of Sciences, Engineering, and Medicine document the evidence-based consensus on the study’s statement of task by an authoring committee of experts. Reports typically include findings, conclusions, and recommendations based on information gathered by the committee and the committee’s deliberations. Each report has been subjected to a rigorous and independent peer-review process and it represents the position of the National Academies on the statement of task.
- Email Alert
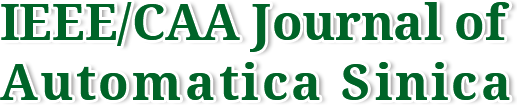
论文 全文 图 表 新闻
- Abstracting/Indexing
- Journal Metrics
- Current Editorial Board
- Early Career Advisory Board
- Previous Editor-in-Chief
- Past Issues
- Current Issue
- Special Issues
- Early Access
- Online Submission
- Information for Authors
- Share facebook twitter google linkedin
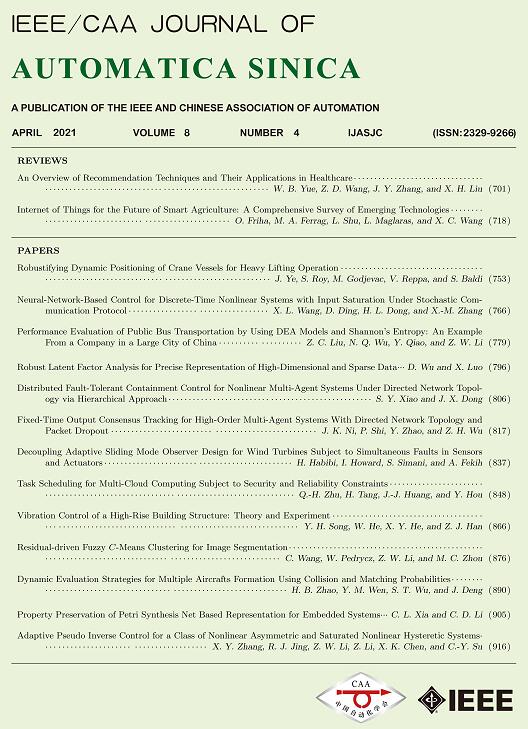
IEEE/CAA Journal of Automatica Sinica
- JCR Impact Factor: 11.8 , Top 4% (SCI Q1) CiteScore: 17.6 , Top 3% (Q1) Google Scholar h5-index: 77, TOP 5
Internet of Things for the Future of Smart Agriculture: A Comprehensive Survey of Emerging Technologies
Doi: 10.1109/jas.2021.1003925.
- Othmane Friha 1 , ,
- Mohamed Amine Ferrag 2 , ,
- Lei Shu 3, 4 , , ,
- Leandros Maglaras 5 , ,
- Xiaochan Wang 6 ,
Networks and Systems Laboratory, University of Badji Mokhtar-Annaba, Annaba 23000, Algeria
Department of Computer Science, Guelma University, Gulema 24000, Algeria
College of Engineering, Nanjing Agricultural University, Nanjing 210095, China
School of Engineering, University of Lincoln, Lincoln LN67TS, UK
School of Computer Science and Informatics, De Montfort University, Leicester LE1 9BH, UK
Department of Electrical Engineering, Nanjing Agricultural University, Nanjing 210095, China
Othmane Friha received the master degree in computer science from Badji Mokhtar-Annaba University, Algeria, in 2018. He is currently working toward the Ph.D. degree in the University of Badji Mokhtar-Annaba, Algeria. His current research interests include network and computer security, internet of things (IoT), and applied cryptography
Mohamed Amine Ferrag received the bachelor degree (June, 2008), master degree (June, 2010), Ph.D. degree (June, 2014), HDR degree (April, 2019) from Badji Mokhtar-Annaba University, Algeria, all in computer science. Since October 2014, he is a Senior Lecturer at the Department of Computer Science, Guelma University, Algeria. Since July 2019, he is a Visiting Senior Researcher, NAULincoln Joint Research Center of Intelligent Engineering, Nanjing Agricultural University. His research interests include wireless network security, network coding security, and applied cryptography. He is featured in Stanford University’s list of the world’s Top 2% Scientists for the year 2019. He has been conducting several research projects with international collaborations on these topics. He has published more than 60 papers in international journals and conferences in the above areas. Some of his research findings are published in top-cited journals, such as the IEEE Communications Surveys and Tutorials , IEEE Internet of Things Journal , IEEE Transactions on Engineering Management , IEEE Access , Journal of Information Security and Applications (Elsevier), Transactions on Emerging Telecommunications Technologies (Wiley), Telecommunication Systems (Springer), International Journal of Communication Systems (Wiley), Sustainable Cities and Society (Elsevier), Security and Communication Networks (Wiley), and Journal of Network and Computer Applications (Elsevier). He has participated in many international conferences worldwide, and has been granted short-term research visitor internships to many renowned universities including, De Montfort University, UK, and Istanbul Technical University, Turkey. He is currently serving on various editorial positions such as Editorial Board Member in Journals (Indexed SCI and Scopus) such as, IET Networks and International Journal of Internet Technology and Secured Transactions (Inderscience Publishers)
Lei Shu (M’07–SM’15) received the B.S. degree in computer science from South Central University for Nationalities in 2002, and the M.S. degree in computer engineering from Kyung Hee University, South Korea, in 2005, and the Ph.D. degree from the Digital Enterprise Research Institute, National University of Ireland, Ireland, in 2010. Until 2012, he was a Specially Assigned Researcher with the Department of Multimedia Engineering, Graduate School of Information Science and Technology, Osaka University, Japan. He is currently a Distinguished Professor with Nanjing Agricultural University and a Lincoln Professor with the University of Lincoln, U.K. He is also the Director of the NAU-Lincoln Joint Research Center of Intelligent Engineering. He has published over 400 papers in related conferences, journals, and books in the areas of sensor networks and internet of things (IoT). His current H-index is 54 and i10-index is 197 in Google Scholar Citation. His current research interests include wireless sensor networks and IoT. He has also served as a TPC Member for more than 150 conferences, such as ICDCS, DCOSS, MASS, ICC, GLOBECOM, ICCCN, WCNC, and ISCC. He was a Recipient of the 2014 Top Level Talents in Sailing Plan of Guangdong Province, China, the 2015 Outstanding Young Professor of Guangdong Province, and the GLOBECOM 2010, ICC 2013, ComManTel 2014, WICON 2016, SigTelCom 2017 Best Paper Awards, the 2017 and 2018 IEEE Systems Journal Best Paper Awards, the 2017 Journal of Network and Computer Applications Best Research Paper Award, and the Outstanding Associate Editor Award of 2017, and the 2018 IEEE ACCESS. He has also served over 50 various Co-Chair for international conferences/workshops, such as IWCMC, ICC, ISCC, ICNC, Chinacom, especially the Symposium Co-Chair for IWCMC 2012, ICC 2012, the General Co-Chair for Chinacom 2014, Qshine 2015, Collaboratecom 2017, DependSys 2018, and SCI 2019, the TPC Chair for InisCom 2015, NCCA 2015, WICON 2016, NCCA 2016, Chinacom 2017, InisCom 2017, WMNC 2017, and NCCA 2018
Leandros Maglaras (SM’15) received the B.Sc. degree from Aristotle University of Thessaloniki, Greece, in 1998, M.Sc. in industrial production and management from University of Thessaly in 2004, and M.Sc. and Ph.D. degrees in electrical & computer engineering from University of Volos in 2008 and 2014, respectively. He is the Head of the National Cyber Security Authority of Greece and a Visiting Lecturer in the School of Computer Science and Informatics at the De Montfort University, U.K. He serves on the Editorial Board of several International peer-reviewed journals such as IEEE Access , Wiley Journal on Security & Communication Networks , EAI Transactions on e-Learning and EAI Transactions on Industrial Networks and Intelligent Systems . He is an author of more than 80 papers in scientific magazines and conferences and is a Senior Member of IEEE. His research interests include wireless sensor networks and vehicular ad hoc networks
Xiaochan Wang is currently a Professor in the Department of Electrical Engineering at Nanjing Agricultural University. His main research fields include intelligent equipment for horticulture and intelligent measurement and control. He is an ASABE Member, and the Vice Director of CSAM (Chinese Society for Agricultural Machinery), and also the Senior Member of Chinese Society of Agricultural Engineering. He was awarded the Second Prize of Science and Technology Invention by the Ministry of Education (2016) and the Advanced Worker for Chinese Society of Agricultural Engineering (2012), and he also gotten the “Blue Project” in Jiangsu province young and middle-aged academic leaders (2010)
- Corresponding author: Lei Shu, e-mail: [email protected]
- Revised Date: 2020-11-25
- Accepted Date: 2020-12-30
- Agricultural internet of things (IoT) ,
- internet of things (IoT) ,
- smart agriculture ,
- smart farming ,
- sustainable agriculture
Proportional views
通讯作者: 陈斌, [email protected].
沈阳化工大学材料科学与工程学院 沈阳 110142
Figures( 12 ) / Tables( 9 )
Article Metrics
- PDF Downloads( 2235 )
- Abstract views( 11904 )
- HTML views( 1407 )
- We review the emerging technologies used by the Internet of Things for the future of smart agriculture.
- We provide a classification of IoT applications for smart agriculture into seven categories, including, smart monitoring, smart water management, agrochemicals applications, disease management, smart harvesting, supply chain management, and smart agricultural practices.
- We provide a taxonomy and a side-by-side comparison of the state-of-the-art methods toward supply chain management based on the blockchain technology for agricultural IoTs.
- We highlight open research challenges and discuss possible future research directions for agricultural IoTs.
- Copyright © 2022 IEEE/CAA Journal of Automatica Sinica
- 京ICP备14019135号-24
- E-mail: [email protected] Tel: +86-10-82544459, 10-82544746
- Address: 95 Zhongguancun East Road, Handian District, Beijing 100190, China
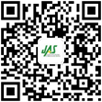
Export File

- Figure 1. The four agricultural revolutions
- Figure 2. Survey structure
- Figure 3. IoT-connected smart agriculture sensors enable the IoT
- Figure 4. The architecture of a typical IoT sensor node
- Figure 5. Fog computing-based agricultural IoT
- Figure 6. SDN/NFV architecture for smart agriculture
- Figure 7. Classification of IoT applications for smart agriculture
- Figure 8. Greenhouse system [ 101 ]
- Figure 9. Aerial-ground robotics system [ 67 ]
- Figure 10. Photovoltaic agri-IoT schematic diagram [ 251 ]
- Figure 11. Smart dairy farming system [ 254 ]
- Figure 12. IoT-based solar insecticidal lamp [ 256 ], [ 257 ]
Agriculture Research Paper Topics
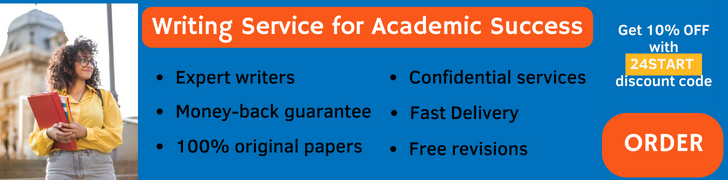
- Agrochemical
- Aquaculture
- Biotechnology
- DDT (dichlorodiphenyltrichloroethane)
- Genetic engineering
- Organic farming
- Slash-and-burn agriculture
Four stages of agricultural development
Agriculture advanced in four major stages that were closely linked with other key historical periods. The first, the Neolithic or New Stone Age, marks the beginning of sedentary farming. Although much of this history is lost in antiquity, dating back 10,000 years or more, anthropologists believe farming arose because of increasing population. The major technological development of this ancient time was the plow. Appearing in Mesopotamia (an ancient region in southwest Asia) around 4000 B.C., the plow allowed farmers to plant crops in rows, saving time and increasing food production.
Academic Writing, Editing, Proofreading, And Problem Solving Services
Get 10% off with 24start discount code.
The second major advance came as a result of Spanish explorer Christopher Columbus’s voyages to the New World in the late fifteenth century. The connecting of the New World and the Old World saw the exchange of farming products and methods. From the New World came maize (Indian corn), beans, the “Irish” potato, squash, peanuts, tomatoes, and tobacco. From the Old World came wheat, rice, coffee, cattle, horses, sheep, and goats. Several Native American tribes adopted new lifestyles, notably the Navajo as sheepherders and the Cheyenne as nomads (wanderers) who used the horse to hunt buffalo. In the twentieth century, maize is a staple food in Africa.
The Industrial Revolution of the eighteenth and nineteenth centuries both contributed to and was supported by agriculture. The greatest agricultural advances came in transportation, where canals, railroads, and then steamships made possible the shipment of food. This in turn increased productivity, but most important, it reduced the threat of starvation. Without these massive increases in food shipments, the exploding populations could not have been fed and the greatly increased demand for labor by emerging industries could not have been met.
As a consequence, the Industrial Revolution introduced major advances in farm technology, such as the cotton gin, mechanical reaper, threshing machine, mowing machine, improved plows, and, in the twentieth century, tractors and trucks. These advances enabled fewer and fewer farmers to feed larger and larger populations, freeing workers to fill demands for factory labor and the growing service industries.
Finally, scientific advances of the twentieth century—the refrigeration of meat, the development of hybrid crops, research into genetics— have greatly benefitted agriculture. Great potential exists for the development of crop and animal varieties with greatly improved dietary characteristics, such as higher protein or reduced fat.
Drawbacks to the rise of agriculture
The agricultural revolution is also associated with some of humankind’s darker moments. In the tropical and subtropical climates of the New World, slave labor was used extensively in farm fields in the eighteenth and nineteenth centuries. In the late twentieth century, the mass production of animals, especially in close quarters, has been extremely controversial. While farmers view new breeding practices as useful means to producing more food, animal rights activists protest them as showing a disregard for animals’ comfort and welfare. Additionally, the widespread use of fertilizers, pesticides, and other chemicals in agriculture have led to serious pollution crises in many areas of the world.
Famine throughout history shows mankind’s desperate dependence on agriculture. Advances in farming, especially in the last few centuries, have led to increases in population. Growing populations—made possible by food surpluses—have forced agricultural expansion onto less and less desirable lands. Because agriculture drastically simplifies ecosystems (communities of plants and animals) and greatly increases soil erosion, many areas such as the Mediterranean basin and tropical forestlands have severely deteriorated.
The future of agriculture
Some argue that the agricultural revolution masks the growing hazards of an overpopulated, increasingly contaminated planet. In the nineteenth and twentieth centuries, agriculture more than compensated for the population explosion. Through scientific advances in areas such as genetic engineering, there is hope that the trend will continue. However, the environmental effects of the agricultural progress could soon undermine any advances if they are not taken seriously.
Back to Science Research Paper Topics .
ORDER HIGH QUALITY CUSTOM PAPER

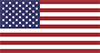
Official websites use .gov A .gov website belongs to an official government organization in the United States.
Secure .gov websites use HTTPS A lock ( ) or https:// means you've safely connected to the .gov website. Share sensitive information only on official, secure websites.
Animal Health and Welfare
Selected resources on humane animal care (e.g., proper nutrition, housing, and environment, as well as, prevention of pain, suffering, disease, and disability), laws and regulations and certificate programs.
Farms and Agricultural Production Systems
Information on sustainable and organic farming, hydroponics, aquaculture, irrigation and urban agriculture, as well as farm ownership and heirs' property.
Human Nutrition and Food Safety
Information on various nutrition and food safety topics including food security, nutrient composition, food defense, and local food systems.
Natural Resources, Conservation, and Environment
Topics relating to the environment, including, weather and climate change, conservation practices, environmental justice, invasive species and soil.
Plant Production and Gardening
Community and container gardening, raised beds, seeds and plants, specialty and cover crops, growing vegetables, medicinal herbs and more.
Rural Development and Communities
Resources on community development; environmental justice, rural funding, sustainable rural communities, and links to past and present USDA rural development collections.
Economics, Business, and Trade
Information about agricultural subsidies, funding for farms and businesses, trade policy, food waste and more with these agricultural marketing and trade resources.
Stanford University

The Center on Food Security and the Environment is a joint effort of the Freeman Spogli Institute for International Studies and the Stanford Woods Institute for the Environment .
Climate and Agriculture: Models, Impacts, and Adaptation Strategies
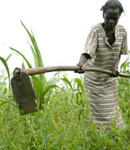
Researchers
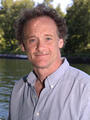
There is little disagreement now that the climate is changing, and that such changes could fundamentally affect humanity's collective ability to feed itself. However, there is little systematic knowledge of where climate effects will hit hardest and how agriculture might adapt to a rapidly changing climate.
This research area examines the potential effects of climate change on regional and global food security, and how to adapt agriculture to a warmer world. Research topics include: past and future effects of climate variability and climate change on agricultural productivity; assessments of gains from different possible adaptation strategies, with the goal of prioritizing agricultural adaptation options in sub-Saharan Africa; the impact of food prices spikes in a warming world; and the nexus of climate, energy, food and health.
Publications
What works for climate adaptation testing and targeting strategies in crop development.
- David Lobell
Impacts of climate change on agriculture and opportunities for detection and attribution
Climate change and agricultural adaptation.
- David Lobell ,
- Fatima Denton
Food Security, Food Prices, and Climate Change
Climate variability, rice production, and food policy in indonesia.
- Rosamond Naylor
Towards Food Security in a Warmer World: Understanding Crop Responses to Climate
Climate and agriculture in the context of copenhagen.
- Gerald Nelson
Climate Extremes and Crop Adaptation
5 Key Issues in Agriculture in 2021
Photo Credit: Maria Fleischmann/World Bank
As 2021 ends, we take a retrospective look at five topics that were covered in our analytical work this year. These issues represent just a fragment of the Bank's work, but they are key to reducing poverty and hunger while slowing climate change.
Food Security
Like the previous year, news in agriculture and food in 2021 was dominated by deteriorating food security. Approximately 30 percent of the world’s population lacked access to adequate food in 2020 and into 2021.
The World Bank took action to fight food insecurity around the world, providing immediate aid to vulnerable households and more long-term support to farmers in the form of seeds, fertilizer, and other agricultural inputs.
COVID-19 also pushed more people into poverty and made the poor poorer around the world. This, along with supply chain interruptions and rising prices had a major impact on hunger. To learn more about these impacts, read this brief compiled by the Bank and updated at least once a month.
Our new podcast series, Table for 10 Billion , launched in March examined the causes of growing hunger and offered some surprising answers.
Farming Insects for Food and Feed
This December, the Bank released a ground-breaking report looking at the valuable role farming insects can play in both food security and climate-smart agriculture, Insect and Hydroponic Farming in Africa: The New Circular Food Economy .
While two billion people regularly eat insects harvested in the wild, farming them for food at scale is new. The report found that African insect farming could generate crude protein worth up to US$2.6 billion and biofertilizers worth up to US$19.4 billion. That is enough protein meal to meet up to 14% of the crude protein needed to rear all the pigs, goats, fish, and chickens in Africa.
Key benefits of insect farming include:
- Insects can be farmed without arable land.
- Insects can be grown within a couple of weeks.
- The food they need to grow comes from food waste.
- Insect waste can be used as fertilizer.
- Insects can be used as animal feed, cutting farmer expenses, and reducing greenhouse gases generated from farming and transporting other feed such as soybeans.
- People can eat insects or sell them for income, increasing food security.
For more on farming insects and the role they can play in the food system, take a listen to this episode of the Table for 10 Billion podcast .
Fixing Food Finance to Build a Greener Future
While agriculture currently accounts for about 25% of greenhouse gas emissions, it also offers opportunities to both fight climate change and feed more people as the world’s population grows to 10 billion people by 2050. One of the keys will be changing the way agriculture is financed and incentivized .
A report from the Bank, published in September, explains the US$12 trillion in annual hidden social, economic, and environmental costs generated by our current food system and offers recommendations to break the cycle by implementing five food finance imperatives that will help implement climate-smart agriculture .
The report, Food Finance Architecture: Financing a Healthy, Equitable and Sustainable Food System , was written in conjunction with the Food and Land Use Coalition, and the International Food Policy Research Institute, and released to coincide with the UN Food Systems Summit.
A feature story and episode of the Table for 10 Billion podcast examine transforming the food system to adapt to climate change.
Digital Agriculture and the Path to the Future
Agriculture continued its march to the digital future in 2021, both with new techniques being developed for growing food and technology that better links the world’s 570 million farmers and 8 billion consumers.
In March, the Bank released the report What’s Cooking: Digital Transformation of the Agrifood System , which explores how digital technologies are improving the food system and provides a roadmap for countries to scale up their own digital agriculture. The report also provides a framework to evaluate policy proposals that can make the food system more efficient, equitable, and environmentally sustainable.
A live event was held in conjunction with the report’s release and the Table for 10 Billion podcast also looked forward to the digital future of food.
Feeding Growing Cities
In March, the Bank looked at the fast-growing cities of Asia and how they have integrated food systems into their planning. The resulting report, RICH Food, Smart City , demonstrated that more needs to be done at the planning stages to ensure a plentiful and safe supply of food to residents now and in the future.
Only 8% of the 170 emerging Asian cities surveyed by the Bank and FAO were deemed to be “food-smart”–working proactively to ensure strong food systems.
As was made clear by the disruptions caused by the COVID-19 pandemic, it is key for cities to pursue “smarter” food policy in order to foster reliable, inclusive, competitive, and healthy (“RICH”) food systems better aligned with their challenges and aspirations.
The authors of the book sat down to answer questions on what needs to be done and how to do it.
This site uses cookies to optimize functionality and give you the best possible experience. If you continue to navigate this website beyond this page, cookies will be placed on your browser. To learn more about cookies, click here .

An official website of the United States government
The .gov means it’s official. Federal government websites often end in .gov or .mil. Before sharing sensitive information, make sure you’re on a federal government site.
The site is secure. The https:// ensures that you are connecting to the official website and that any information you provide is encrypted and transmitted securely.
- Publications
- Account settings
Preview improvements coming to the PMC website in October 2024. Learn More or Try it out now .
- Advanced Search
- Journal List
- Sensors (Basel)

Machine Learning in Agriculture: A Comprehensive Updated Review
Lefteris benos.
1 Centre of Research and Technology-Hellas (CERTH), Institute for Bio-Economy and Agri-Technology (IBO), 6th km Charilaou-Thermi Rd, GR 57001 Thessaloniki, Greece; [email protected] (L.B.); [email protected] (A.C.T.); [email protected] (G.D.); [email protected] (D.K.)
Aristotelis C. Tagarakis
Georgios dolias, remigio berruto.
2 Department of Agriculture, Forestry and Food Science (DISAFA), University of Turin, Largo Braccini 2, 10095 Grugliasco, Italy; [email protected]
Dimitrios Kateris
Dionysis bochtis.
3 FarmB Digital Agriculture P.C., Doiranis 17, GR 54639 Thessaloniki, Greece
The digital transformation of agriculture has evolved various aspects of management into artificial intelligent systems for the sake of making value from the ever-increasing data originated from numerous sources. A subset of artificial intelligence, namely machine learning, has a considerable potential to handle numerous challenges in the establishment of knowledge-based farming systems. The present study aims at shedding light on machine learning in agriculture by thoroughly reviewing the recent scholarly literature based on keywords’ combinations of “machine learning” along with “crop management”, “water management”, “soil management”, and “livestock management”, and in accordance with PRISMA guidelines. Only journal papers were considered eligible that were published within 2018–2020. The results indicated that this topic pertains to different disciplines that favour convergence research at the international level. Furthermore, crop management was observed to be at the centre of attention. A plethora of machine learning algorithms were used, with those belonging to Artificial Neural Networks being more efficient. In addition, maize and wheat as well as cattle and sheep were the most investigated crops and animals, respectively. Finally, a variety of sensors, attached on satellites and unmanned ground and aerial vehicles, have been utilized as a means of getting reliable input data for the data analyses. It is anticipated that this study will constitute a beneficial guide to all stakeholders towards enhancing awareness of the potential advantages of using machine learning in agriculture and contributing to a more systematic research on this topic.
1. Introduction
1.1. general context of machine learning in agriculture.
Modern agriculture has to cope with several challenges, including the increasing call for food, as a consequence of the global explosion of earth’s population, climate changes [ 1 ], natural resources depletion [ 2 ], alteration of dietary choices [ 3 ], as well as safety and health concerns [ 4 ]. As a means of addressing the above issues, placing pressure on the agricultural sector, there exists an urgent necessity for optimizing the effectiveness of agricultural practices by, simultaneously, lessening the environmental burden. In particular, these two essentials have driven the transformation of agriculture into precision agriculture. This modernization of farming has a great potential to assure sustainability, maximal productivity, and a safe environment [ 5 ]. In general, smart farming is based on four key pillars in order to deal with the increasing needs; (a) optimal natural resources’ management, (b) conservation of the ecosystem, (c) development of adequate services, and (d) utilization of modern technologies [ 6 ]. An essential prerequisite of modern agriculture is, definitely, the adoption of Information and Communication Technology (ICT), which is promoted by policy-makers around the world. ICT can indicatively include farm management information systems, humidity and soil sensors, accelerometers, wireless sensor networks, cameras, drones, low-cost satellites, online services, and automated guided vehicles [ 7 ].
The large volume of data, which is produced by digital technologies and usually referred to as “big data”, needs large storage capabilities in addition to editing, analyzing, and interpreting. The latter has a considerable potential to add value for society, environment, and decision-makers [ 8 ]. Nevertheless, big data encompass challenges on account of their so-called “5-V” requirements; (a) Volume, (b) Variety, (c) Velocity, (d) Veracity, and (e) Value [ 9 ]. The conventional data processing techniques are incapable of meeting the constantly growing demands in the new era of smart farming, which is an important obstacle for extracting valuable information from field data [ 10 ]. To that end, Machine Learning (ML) has emerged, which is a subset of artificial intelligence [ 11 ], by taking advantage of the exponential computational power capacity growth.
There is a plethora of applications of ML in agriculture. According to the recent literature survey by Liakos et al. [ 12 ], regarding the time period of 2004 to 2018, four generic categories were identified ( Figure 1 ). These categories refer to crop, water, soil, and livestock management. In particular, as far as crop management is concerned, it represented the majority of the articles amongst all categories (61% of the total articles) and was further sub-divided into:
- Yield prediction;
- Disease detection;
- Weed detection;
- Crop recognition;
- Crop quality.

The four generic categories in agriculture exploiting machine learning techniques, as presented in [ 12 ].
The generic categories dealing with the management of water and soil were found to be less investigated, corresponding cumulatively to 20% of the total number of papers (10% for each category).
Finally, two main sub-categories were identified for the livestock-related applications corresponding to a total 19% of journal papers:
- Livestock production;
- Animal welfare.
1.2. Open Problems Associated with Machine Learning in Agriculture
Due to the broad range of applications of ML in agriculture, several reviews have been published in this research field. The majority of these review studies have been dedicated to crop disease detection [ 13 , 14 , 15 , 16 ], weed detection [ 17 , 18 ], yield prediction [ 19 , 20 ], crop recognition [ 21 , 22 ], water management [ 23 , 24 ], animal welfare [ 25 , 26 ], and livestock production [ 27 , 28 ]. Furthermore, other studies were concerned with the implementation of ML methods regarding the main grain crops by investigating different aspects including quality and disease detection [ 29 ]. Finally, focus has been paid on big data analysis using ML, aiming at finding out real-life problems that originated from smart farming [ 30 ], or dealing with methods to analyze hyperspectral and multispectral data [ 31 ].
Although ML in agriculture has made considerable progress, several open problems remain, which have some common points of reference, despite the fact that the topic covers a variety of sub-fields. According to [ 23 , 24 , 28 , 32 ], the main problems are associated with the implementation of sensors on farms for numerous reasons, including high costs of ICT, traditional practices, and lack of information. In addition, the majority of the available datasets do not reflect realistic cases, since they are normally generated by a few people getting images or specimens in a short time period and from a limited area [ 15 , 21 , 22 , 23 ]. Consequently, more practical datasets coming from fields are required [ 18 , 20 ]. Moreover, the need for more efficient ML algorithms and scalable computational architectures has been pointed out, which can lead to rapid information processing [ 18 , 22 , 23 , 31 ]. The challenging background, when it comes to obtaining images, video, or audio recordings, has also been mentioned owing to changes in lighting [ 16 , 29 ], blind spots of cameras, environmental noise, and simultaneous vocalizations [ 25 ]. Another important open problem is that the vast majority of farmers are non-experts in ML and, thus, they cannot fully comprehend the underlying patterns obtained by ML algorithms. For this reason, more user-friendly systems should be developed. In particular, simple systems, being easy to understand and operate, would be valuable, as for example a visualization tool with a user-friendly interface for the correct presentation and manipulation of data [ 25 , 30 , 31 ]. Taking into account that farmers are getting more and more familiar with smartphones, specific smartphone applications have been proposed as a possible solution to address the above challenge [ 15 , 16 , 21 ]. Last but not least, the development of efficient ML techniques by incorporating expert knowledge from different stakeholders should be fostered, particularly regarding computing science, agriculture, and the private sector, as a means of designing realistic solutions [ 19 , 22 , 24 , 33 ]. As stated in [ 12 ], currently, all of the efforts pertain to individual solutions, which are not always connected with the process of decision-making, as seen for example in other domains.
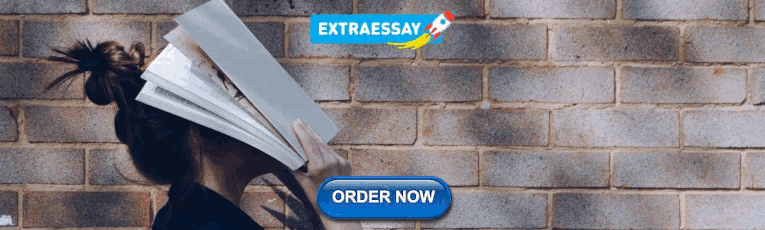
1.3. Aim of the Present Study
As pointed out above, because of the multiple applications of ML in agriculture, several review studies have been published recently. However, these studies usually concentrate purely on one sub-field of agricultural production. Motivated by the current tremendous progress in ML, the increasing interest worldwide, and its impact in various do-mains of agriculture, a systematic bibliographic survey is presented on the range of the categories proposed in [ 12 ], which were summarized in Figure 1 . In particular, we focus on reviewing the relevant literature of the last three years (2018–2020) for the intention of providing an updated view of ML applications in agricultural systems. In fact, this work is an updated continuation of the work presented at [ 12 ]; following, consequently, exactly the same framework and inclusion criteria. As a consequence, the scholarly literature was screened in order to cover a broad spectrum of important features for capturing the current progress and trends, including the identification of: (a) the research areas which are interested mostly in ML in agriculture along with the geographical distribution of the contributing organizations, (b) the most efficient ML models, (c) the most investigated crops and animals, and (d) the most implemented features and technologies.
As will be discussed next, overall, a 745% increase in the number of journal papers took place in the last three years as compared to [ 12 ], thus justifying the need for a new updated review on the specific topic. Moreover, crop management remained as the most investigated topic, with a number of ML algorithms having been exploited as a means of tackling the heterogeneous data that originated from agricultural fields. As compared to [ 12 ], more crop and animal species have been investigated by using an extensive range of input parameters coming mainly from remote sensing, such as satellites and drones. In addition, people from different research fields have dealt with ML in agriculture, hence, contributing to the remarkable advancement in this field.
1.4. Outline of the Paper
The remainder of this paper is structured as follows. The second section briefly describes the fundamentals of ML along with the subject of the four generic categories for the sake of better comprehension of the scope of the present study. The implemented methodology, along with the inclusive criteria and the search engines, is analyzed in the third section. The main performance metrics, which were used in the selected articles, are also presented in this section. The main results are shown in the fourth section in the form of bar and pie charts, while in the fifth section, the main conclusions are drawn by also discussing the results from a broader perspective. Finally, all the selected journal papers are summarized in Table A1 , Table A2 , Table A3 , Table A4 , Table A5 , Table A6 , Table A7 , Table A8 and Table A9 , in accordance with their field of application, and presented in the Appendix A , together with Table A10 and Table A11 that contain commonly used abbreviations, with the intention of not disrupting the flow of the main text.
2. Background
2.1. fundamentals of machine learning: a brief overview.
In general, the objective of ML algorithms is to optimize the performance of a task, via exploiting examples or past experience. In particular, ML can generate efficient relationships regarding data inputs and reconstruct a knowledge scheme. In this data-driven methodology, the more data are used, the better ML works. This is similar to how well a human being performs a particular task by gaining more experience [ 34 ]. The central outcome of ML is a measure of generalizability; the degree to which the ML algorithm has the ability to provide correct predictions, when new data are presented, on the basis of learned rules originated from preceding exposure to similar data [ 35 ]. More specifically, data involve a set of examples, which are described by a group of characteristics, usually called features. Broadly speaking, ML systems operate at two processes, namely the learning (used for training) and testing. In order to facilitate the former process, these features commonly form a feature vector that can be binary, numeric, ordinal, or nominal [ 36 ]. This vector is utilized as an input within the learning phase. In brief, by relying on training data, within the learning phase, the machine learns to perform the task from experience. Once the learning performance reaches a satisfactory point (expressed through mathematical and statistical relationships), it ends. Subsequently, the model that was developed through the training process can be used to classify, cluster, or predict.
An overview of a typical ML system is illustrated in Figure 2 . With the intention of forming the derived complex raw data into a suitable state, a pre-processing effort is required. This usually includes: (a) data cleaning for removing inconsistent or missing items and noise, (b) data integration, when many data sources exist and (c) data transformation, such as normalization and discretization [ 37 ]. The extraction/selection feature aims at creating or/and identifying the most informative subset of features in which, subsequently, the learning model is going to be implemented throughout the training phase [ 38 ]. Regarding the feedback loop, which is depicted in Figure 2 , it serves for adjustments pertaining to the feature extraction/selection unit as well as the pre-processing one that further improves the overall learning model’s performance. During the phase of testing, previously unseen samples are imported to the trained model, which are usually represented as feature vectors. Finally, an appropriate decision is made by the model (for example, classification or regression) in reliance of the features existing in each sample. Deep learning, a subfield of ML, utilizes an alternative architecture via shifting the process of converting raw data to features (feature engineering) to the corresponding learning system. Consequently, the feature extraction/selection unit is absent, resulting in a fully trainable system; it starts from a raw input and ends with the desired output [ 39 , 40 ].

A graphical illustration of a typical machine learning system.
Based on the learning type, ML can be classified according to the relative literature [ 41 , 42 ] as:
- Supervised learning: The input and output are known and the machine tries to find the optimal way to reach an output given an input;
- Unsupervised learning: No labels are provided, leaving the learning algorithm itself to generate structure within its input;
- Semi-supervised learning: Input data constitute a mixture of labeled and non-labeled data;
- Reinforcement learning: Decisions are made towards finding out actions that can lead to the more positive outcome, while it is solely determined by trial and error method and delayed outcome.
Nowadays, ML is used in facilitating several management aspects in agriculture [ 12 ] and in a plethora of other applications, such as image recognition [ 43 ], speech recognition [ 44 ], autonomous driving [ 45 ], credit card fraud detection [ 46 ], stock market forecasting [ 47 ], fluid mechanics [ 48 ], email, spam and malware filtering [ 49 ], medical diagnosis [ 40 ], contamination detection in urban water networks [ 50 ], and activity recognition [ 51 ], to mention but a few.
2.2. Brief Description of the Four Generic Categories
2.2.1. crop management.
The crop management category involves versatile aspects that originated from the combination of farming techniques in the direction of managing the biological, chemical and physical crop environment with the aim of reaching both quantitative and qualitative targets [ 52 ]. Using advanced approaches to manage crops, such as yield prediction, disease detection, weed detection, crop recognition, and crop quality, contributes to the increase of productivity and, consequently, the financial income. The above aspects constitute key goals of precision agriculture.
Yield Prediction
In general, yield prediction is one of the most important and challenging topics in modern agriculture. An accurate model can help, for instance, the farm owners to take informed management decisions on what to grow towards matching the crop to the existing market’s demands [ 20 ]. However, this is not a trivial task; it consists of various steps. Yield prediction can be determined by several factors such as environment, management practices, crop genotypic and phenotypic characteristics, and their interactions. Hence, it necessitates a fundamental comprehension of the relationship between these interactive factors and yield. In turn, identifying such kinds of relationships mandates comprehensive datasets along with powerful algorithms such as ML techniques [ 53 ].
Disease Detection
Crop diseases constitute a major threat in agricultural production systems that deteriorate yield quality and quantity at production, storage, and transportation level. At farm level, reports on yield losses, due to plant diseases, are very common [ 54 ]. Furthermore, crop diseases pose significant risks to food security at a global scale. Timely identification of plant diseases is a key aspect for efficient management. Plant diseases may be provoked by various kinds of bacteria, fungi, pests, viruses, and other agents. Disease symptoms, namely the physical evidence of the presence of pathogens and the changes in the plants’ phenotype, may consist of leaf and fruit spots, wilting and color change [ 55 ], curling of leaves, etc. Historically, disease detection was conducted by expert agronomists, by performing field scouting. However, this process is time-consuming and solely based on visual inspection. Recent technological advances have made commercially available sensing systems able to identify diseased plants before the symptoms become visible. Furthermore, in the past few years, computer vision, especially by employing deep learning, has made remarkable progress. As highlighted by Zhang et al. [ 56 ], who focused on identifying cucumber leaf diseases by utilizing deep learning, due to the complex environmental background, it is beneficial to eliminate background before model training. Moreover, accurate image classifiers for disease diagnosis need a large dataset of both healthy and diseased plant images. In reference to large-scale cultivations, such kinds of automated processes can be combined with autonomous vehicles, to timely identify phytopathological problems by implementing regular inspections. Furthermore, maps of the spatial distribution of the plant disease can be created, depicting the zones in the farm where the infection has been spread [ 57 ].
Weed Detection
As a result of their prolific seed production and longevity, weeds usually grow and spread invasively over large parts of the field very fast, competing with crops for the resources, including space, sunlight, nutrients, and water availability. Besides, weeds frequently arise sooner than crops without having to face natural enemies, a fact that adversely affects crop growth [ 18 ]. In order to prevent crop yield reduction, weed control is an important management task by either mechanical treatment or application of herbicides. Mechanical treatment is, in most cases, difficult to be performed and ineffective if not properly performed, making herbicide application the most widely used operation. Using large quantities of herbicides, however, turns out to be both costly and detrimental for the environment, especially in the case of uniform application without taking into account the spatial distribution of the weeds. Remarkably, long-term herbicide use is very likely to make weeds more resistant, thus, resulting in more demanding and expensive weed control. In recent years, considerable achievements have been made pertaining to the differentiation of weeds from crops on the basis of smart agriculture. This discrimination can be accomplished by using remote or proximal sensing with sensors attached on satellites, aerial, and ground vehicles, as well as unmanned vehicles (both ground (UGV) and aerial (UAV)). The transformation of data gathered by UAVs into meaningful information is, however, still a challenging task, since both data collection and classification need painstaking effort [ 58 ]. ML algorithms coupled with imaging technologies or non-imaging spectroscopy can allow for real-time differentiation and localization of target weeds, enabling precise application of herbicides to specific zones, instead of spraying the entire fields [ 59 ] and planning of the shortest weeding path [ 60 ].
Crop Recognition
Automatic recognition of crops has gained considerable attention in several scientific fields, such as plant taxonomy, botanical gardens, and new species discovery. Plant species can be recognized and classified via analysis of various organs, including leaves, stems, fruits, flowers, roots, and seeds [ 61 , 62 ]. Using leaf-based plant recognition seems to be the most common approach by examining specific leaf’s characteristics like color, shape, and texture [ 63 ]. With the broader use of satellites and aerial vehicles as means of sensing crop properties, crop classification through remote sensing has become particularly popular. As in the above sub-categories, the advancement on computer software and image processing devices combined with ML has led to the automatic recognition and classification of crops.
Crop Quality
Crop quality is very consequential for the market and, in general, is related to soil and climate conditions, cultivation practices and crop characteristics, to name a few. High quality agricultural products are typically sold at better prices, hence, offering larger earnings to farmers. For instance, as regards fruit quality, flesh firmness, soluble solids content, and skin color are among the most ordinary maturity indices utilized for harvesting [ 64 ]. The timing of harvesting greatly affects the quality characteristics of the harvested products in both high value crops (tree crops, grapes, vegetables, herbs, etc.) and arable crops. Therefore, developing decision support systems can aid farmers in taking appropriate management decisions for increased quality of production. For example, selective harvesting is a management practice that may considerably increase quality. Furthermore, crop quality is closely linked with food waste, an additional challenge that modern agriculture has to cope with, since if the crop deviates from the desired shape, color, or size, it may be thrown away. Similarly to the above sub-section, ML algorithms combined with imaging technologies can provide encouraging results.
2.2.2. Water Management
The agricultural sector constitutes the main consumer of available fresh water on a global scale, as plant growth largely relies on water availability. Taking into account the rapid depletion rate of a lot of aquifers with negligible recharge, more effective water management is needed for the purpose of better conserving water in terms of accomplishing a sustainable crop production [ 65 ]. Effective water management can also lead to the improvement of water quality as well as reduction of pollution and health risks [ 66 ]. Recent research on precision agriculture offers the potential of variable rate irrigation so as to attain water savings. This can be realized by implementing irrigation at rates, which vary according to field variability on the basis of specific water requirements of separate management zones, instead of using a uniform rate in the entire field. The effectiveness and feasibility of the variable rate irrigation approach depend on agronomic factors, including topography, soil properties, and their effect on soil water in order to accomplish both water savings and yield optimization [ 67 ]. Carefully monitoring the status of soil water, crop growth conditions, and temporal and spatial patterns in combination with weather conditions monitoring and forecasting, can help in irrigation programming and efficient management of water. Among the utilized ICTs, remote sensing can provide images with spatial and temporal variability associated with the soil moisture status and crop growth parameters for precision water management. Interestingly, water management is challenging enough in arid areas, where groundwater sources are used for irrigation, with the precipitation providing only part of the total crop evapotranspiration (ET) demands [ 68 ].
2.2.3. Soil Management
Soil, a heterogeneous natural resource, involves mechanisms and processes that are very complex. Precise information regarding soil on a regional scale is vital, as it contributes towards better soil management consistent with land potential and, in general, sustainable agriculture [ 5 ]. Better management of soil is also of great interest owing to issues like land degradation (loss of the biological productivity), soil-nutrient imbalance (due to fertilizers overuse), and soil erosion (as a result of vegetation overcutting, improper crop rotations rather than balanced ones, livestock overgrazing, and unsustainable fallow periods) [ 69 ]. Useful soil properties can entail texture, organic matter, and nutrients content, to mention but a few. Traditional soil assessment methods include soil sampling and laboratory analysis, which are normally expensive and take considerable time and effort. However, remote sensing and soil mapping sensors can provide low-cost and effortless solution for the study of soil spatial variability. Data fusion and handling of such heterogeneous “big data” may be important drawbacks, when traditional data analysis methods are used. ML techniques can serve as a trustworthy, low-cost solution for such a task.
2.2.4. Livestock Management
It is widely accepted that livestock production systems have been intensified in the context of productivity per animal. This intensification involves social concerns that can influence consumer perception of food safety, security, and sustainability, based on animal welfare and human health. In particular, monitoring both the welfare of animals and overall production is a key aspect so as to improve production systems [ 70 ]. The above fields take place in the framework of precision livestock farming, aiming at applying engineering techniques to monitor animal health in real time and recognizing warning messages, as well as improving the production at the initial stages. The role of precision livestock farming is getting more and more significant by supporting the decision-making processes of livestock owners and changing their role. It can also facilitate the products’ traceability, in addition to monitoring their quality and the living conditions of animals, as required by policy-makers [ 71 ]. Precision livestock farming relies on non-invasive sensors, such as cameras, accelerometers, gyroscopes, radio-frequency identification systems, pedometers, and optical and temperature sensors [ 25 ]. IoT sensors leverage variable physical quantities (VPQs) as a means of sensing temperature, sound, humidity, etc. For instance, IoT sensors can warn if a VPQ falls out of regular limits in real-time, giving valuable information regarding individual animals. As a result, the cost of repetitively and arduously checking each animal can be reduced [ 72 ]. In order to take advantage of the large amounts of data, ML methodologies have become an integral part of modern livestock farming. Models can be developed that have the capability of defining the manner a biological system operates, relying on causal relationships and exploiting this biological awareness towards generating predictions and suggestions.
Animal Welfare
There is an ongoing concern for animal welfare, since the health of animals is strongly associated with product quality and, as a consequence, predominantly with the health of consumers and, secondarily, with the improvement of economic efficiency [ 73 ]. There exist several indexes for animal welfare evaluation, including physiological stress and behavioral indicators. The most commonly used indicator is animal behavior, which can be affected by diseases, emotions, and living conditions, which have the potential to demonstrate physiological conditions [ 25 ]. Sensors, commonly used to detect behavioral changes (for example, changes in water or food consumption, reduced animal activity), include microphone systems, cameras, accelerometers, etc.
Livestock Production
The use of sensor technology, along with advanced ML techniques, can increase livestock production efficiency. Given the impact of practices of animal management on productive elements, livestock owners are getting cautious of their asset. However, as the livestock holdings get larger, the proper consideration of every single animal is very difficult. From this perspective, the support to farmers via precision livestock farming, mentioned above, is an auspicious step for aspects associated with economic efficiency and establishment of sustainable workplaces with reduced environmental footprint [ 74 ]. Generally, several models have been used in animal production, with their intentions normally revolving around growing and feeding animals in the best way. However, the large volumes of data being involved, again, call for ML approaches.
3.1. Screening of the Relative Literature
In order to identify the relevant studies concerning ML in respect to different aspects of management in agriculture, the search engines of Scopus, Google Scholar, ScienceDirect, PubMed, Web of Science, and MDPI were utilized. In addition, keywords’ combinations of “machine learning” in conjunction with each of the following: “crop management”, “water management”, “soil management”, and “livestock management” were used. Our intention was to filter the literature on the same framework as [ 12 ]; however, focusing solely within the period 2018–2020. Once a relevant study was being identified, the references of the paper at hand were being scanned to find studies that had not been found throughout the initial searching procedure. This process was being iterated until no relevant studies occurred. In this stage, only journal papers were considered eligible. Thus, non-English studies, conferences papers, chapters, reviews, as well as Master and Doctoral Theses were excluded. The latest search was conducted on 15 December 2020. Subsequently, the abstract of each paper was being reviewed, while, at a next stage, the full text was being read to decide its appropriateness. After a discussion between all co-authors with reference to the appropriateness of the selected papers, some of them were excluded, in the case they did not meet the two main inclusion criteria, namely: (a) the paper was published within 2018–2020 and (b) the paper referred to one of the categories and sub-categories, which were summarized in Figure 1 . Finally, the papers were classified in these sub-categories. Overall, 338 journal papers were identified. The flowchart of the present review methodology is depicted in Figure 3 , based on the PRISMA guidelines [ 75 ], along with information about at which stage each exclusive criterion was imposed similarly to recent systematic review studies such as [ 72 , 76 , 77 , 78 ].

The flowchart of the methodology of the present systematic review along with the flow of information regarding the exclusive criteria, based on PRISMA guidelines [ 75 ].
3.2. Definition of the Performance Metrics Commonly Used in the Reviewed Studies
In this subsection, the most commonly used performance metrics of the reviewed papers are briefly described. In general, these metrics are utilized in an effort to provide a common measure to evaluate the ML algorithms. The selection of the appropriate metrics is very important, since: (a) how the algorithm’s performance is measured relies on these metrics and (b) the metric itself can influence the way the significance of several characteristics is weighted.
Confusion matrix constitutes one of the most intuitive metrics towards finding the correctness of a model. It is used for classification problems, where the result can be of at least two types of classes. Let us consider a simple example, by giving a label to a target variable: for example, “1” when a plant has been infected with a disease and “0” otherwise. In this simplified case, the confusion matrix ( Figure 4 ) is a 2 × 2 table having two dimensions, namely “Actual” and “Predicted”, while its dimensions have the outcome of the comparison between the predictions with the actual class label. Concerning the above simplified example, this outcome can acquire the following values:
- True Positive (TP): The plant has a disease (1) and the model classifies this case as diseased (1);
- True Negative (TN): The plant does not have a disease (0) and the model classifies this case as a healthy plant (0);
- False Positive (FP): The plant does not have a disease (0), but the model classifies this case as diseased (1);
- False Negative (FN): The plant has a disease (1), but the model classifies this case as a healthy plant (0).

Representative illustration of a simplified confusion matrix.
As can be shown in Table 1 , the aforementioned values can be implemented in order to estimate the performance metrics, typically observed in classification problems [ 79 ].
Summary of the most commonly used evaluation metrics of the reviewed studies.
Other common evaluation metrics were the coefficient of correlation ( R ), coefficient of determination ( R 2 ; basically, the square of the correlation coefficient), Mean Absolute Error (MAE), Mean Absolute Percentage Error (MAPE), and Mean Squared Error (MSE), which can be given via the following relationships [ 80 , 81 ]:
where X t and Z t correspond to the predicted and real value, respectively, t stands for the iteration at each point, while T for the testing records number. Accordingly, low values of MAE, MAPE, and MSE values denote a small error and, hence, better performance. In contrast, R 2 near 1 is desired, which demonstrates better model performance and also that the regression curve efficiently fits the data.
4.1. Preliminary Data Visualization Analysis
Graphical representation of data related to the reviewed studies, by using maps, bar or pie charts, for example, can provide an efficient approach to demonstrate and interpret the patterns of data. The data visualization analysis, as it usually refers to, can be vital in the context of analyzing large amounts of data and has gained remarkable attention in the past few years, including review studies. Indicatively, significant results can be deduced in an effort to identify: (a) the most contributing authors and organizations, (b) the most contributing international journals (or equivalently which research fields are interested in this topic), and (c) the current trends in this field [ 82 ].
4.1.1. Classification of the Studies in Terms of Application Domain
As can be seen in the flowchart of the present methodology ( Figure 3 ), the literature survey on ML in agriculture resulted in 338 journal papers. Subsequently, these studies were classified into the four generic categories as well as into their sub-categories, as already mentioned above. Figure 5 depicts the aforementioned papers’ distribution. In particular, the majority of the studies were intended for crop management (68%), while soil management (10%), water management (10%), and livestock management (12% in total; animal welfare: 7% and livestock production: 5%) had almost equal contribution in the present bibliographic survey. Focusing on crop management, the most contributing sub-categories were yield prediction (20%) and disease detection (19%). The former research field arises as a consequence of the increasing interest of farmers in taking decisions based on efficient management that can lead to the desired yield. Disease detection, on the other hand, is also very important, as diseases constitute a primary menace for food security and quality assurance. Equal percentages (13%) were observed for weed detection and crop recognition, both of which are essential in crop management at farm and agricultural policy making level. Finally, examination of crop quality was relatively scarce corresponding to 3% of all studies. This can be attributed to the complexity of monitoring and modeling the quality-related parameters.

The classification of the reviewed studies according to the field of application.
In this fashion, it should be mentioned again that all the selected journal papers are summarized in Table A1 , Table A2 , Table A3 , Table A4 , Table A5 , Table A6 , Table A7 , Table A8 and Table A9 , depending on their field of application, and presented in the Appendix A . The columns of the tables correspond (from left to right) to the “Reference number” (Ref), “Input Data”, “Functionality”, “Models/Algorithms”, and “Best Output”. One additional column exists for the sub-categories belonging in crop management, namely “Crop”, whereas the corresponding column in the sub-categories pertaining to livestock management refers to “Animal”. The present systematic review deals with a plethora of different ML models and algorithms. For the sake of brevity, the commonly used abbreviations are used instead of the entire names, which are summarized in Table A10 and Table A11 (presented also in the Appendix A ). The list of the aforementioned Tables, along with their content, is listed in Table 2 .
List of the tables appearing in the Appendix A related to: (a) the categories and sub-categories of the machine learning applications in agriculture ( Table A1 , Table A2 , Table A3 , Table A4 , Table A5 , Table A6 , Table A7 , Table A8 and Table A9 ) and (b) the abbreviations of machine learning models and algorithms ( Table A10 and Table A11 , respectively).
4.1.2. Geographical Distribution of the Contributing Organizations
The subject of this sub-section is to find out the geographical distribution of all the contributing organizations in ML applications in agriculture. To that end, the author’s affiliation was taken into account. In case a paper included more than one author, which was the most frequent scenario, each country could contribute only once in the final map chart ( Figure 6 ), similarly to [ 83 , 84 ]. As can be gleaned from Figure 6 , investigating ML in agriculture is distributed worldwide, including both developed and developing economies. Remarkably, out of the 55 contributing countries, the least contribution originated from African countries (3%), whereas the major contribution came from Asian countries (55%). The latter result is attributed mainly to the considerable contribution of Chinese (24.9%) as well as Indian organizations (10.1%). USA appeared to be the second most contributing country with 20.7% percentage, while Australia (9.5%), Spain (6.8%), Germany (5.9%), Brazil, UK, and Iran (5.62%) seem to be particularly interested in ML in agriculture. It should be stressed that livestock management, which is a relatively different sub-field comparing to crop, water, and soil management, was primary examined from studies coming from Australia, USA, China, and UK, while all the papers regarding Ireland were focused on animals. Finally, another noteworthy observation is that a large number of articles were a result of international collaboration, with the synergy of China and USA standing out.

Geographical distribution of the contribution of each country to the research field focusing on machine learning in agriculture.
4.1.3. Distribution of the Most Contributing Journal Papers
For the purpose of identifying the research areas that are mostly interested in ML in agriculture, the most frequently appeared international journal papers are depicted in Figure 7 . In total, there were 129 relevant journals. However, in this bar chart, only the journals contributing with at least 4 papers are presented for brevity. As a general remark, remote sensing was of particular importance, since reliable data from satellites and UAV, for instance, constitute valuable input data for the ML algorithms. In addition, smart farming, environment, and agricultural sustainability were of central interest. Journals associated with computational techniques were also presented with considerable frequency. A typical example of such type of journals, which was presented in the majority of the studies with a percentage of 19.8%, was “ Computers and Electronics in Agriculture ”. This journal aims at providing the advances in relation to the application of computers and electronic systems for solving problems in plant and animal production.

Distribution of the most contributing international journals (published at least four articles) concerning applications of machine learning in agriculture.
The “ Remote Sensing ” and “ Sensors ” journals followed with approximately 11.8% and 6.5% of the total number of publications, respectively. These are cross-sectoral journals that are concentrated on applications of science and sensing technologies in various fields, including agriculture. Other journals, covering this research field, were also “ IEEE Access ” and “ International Journal of Remote Sensing ” with approximately 2.1% and 1.2% contribution, respectively. Moreover, agriculture-oriented journals were also presented in Figure 7 , including “ Precision Agriculture ”, “ Frontiers in Plant Science ”, “ Agricultural and Forest Meteorology ”, and “ Agricultural Water Management ” with 1–3% percentage. These journals deal with several aspects of agriculture ranging from management strategies (so as to incorporate spatial and temporal data as a means of optimizing productivity, resource use efficiency, sustainability and profitability of agricultural production) up to crop molecular genetics and plant pathogens. An interdisciplinary journal concentrating on soil functions and processes also appeared with 2.1%, namely “ Geoderma ”, plausibly covering the soil management generic category. Finally, several journals focusing on physics and applied natural sciences, such as “ Applied Sciences ” (2.7%), “ Scientific Reports ” (1.8%), “ Biosystems Engineering ” (1.5%), and “ PLOS ONE ” (1.5%), had a notable contribution to ML studies. As a consequence, ML in agriculture concerns several disciplines and constitutes a fundamental area for developing various techniques, which can be beneficial to other fields as well.
4.2. Synopsis of the Main Features Associated with the Relative Literature
4.2.1. machine learning models providing the best results.
A wide range of ML algorithms was implemented in the selected studies; their abbreviations are given in Table A11 . The ML algorithms that were used by each study as well as those that provided the best output have been listed in the last two columns of Table A1 , Table A2 , Table A3 , Table A4 , Table A5 , Table A6 , Table A7 , Table A8 and Table A9 . These algorithms can be classified into the eight broad families of ML models, which are summarized in Table A10 . Figure 8 focuses on the best performed ML models as a means of capturing a broad picture of the current situation and demonstrating advancement similarly to [ 12 ].

Machine Learning models giving the best output.
As can be demonstrated in Figure 8 , the most frequent ML model providing the best output was, by far, Artificial Neural Networks (ANNs), which appeared in almost half of the reviewed studies (namely, 51.8%). More specifically, ANN models provided the best results in the majority of the studies concerning all sub-categories. ANNs have been inspired by the biological neural networks that comprise human brains [ 85 ], while they allow for learning via examples from representative data describing a physical phenomenon. A distinct characteristic of ANNs is that they can develop relationships between dependent and independent variables, and thus extract useful information from representative datasets. ANN models can offer several benefits, such as their ability to handle noisy data [ 86 ], a situation that is very common in agricultural measurements. Among the most popular ANNs are the Deep Neural Networks (DNNs), which utilize multiple hidden layers between input and output layers. DNNs can be unsupervised, semi-supervised, or supervised. A usual kind of DNNs are the Convolutional Neural Networks (CNNs), whose layers, unlike common neural networks, can set up neurons in three dimensions [ 87 ]. In fact, CNNs were presented as the algorithms that provide the best output in all sub-categories, with an almost 50% of the individual percentage of ANNs. As stressed in recent studies, such as that of Yang et al. [ 88 ], CNNs are receiving more and more attention because of their efficient results when it comes to detection through images’ processing.
Recurrent Neural Networks (RNNs) followed, representing approximately 10% of ANNs, with Long Short-Term Memory (LSTM) standing out. They are called “recurrent” as they carry out the same process for every element, with the previous computations determining the current output, while they have a “memory” that stores information pertaining to what has been calculated so far. RNNs can face problems concerning vanishing gradients and inability to “memorize” many sequential data. Towards addressing these issues, the cell structures of LSTM can control which part of information will be either stored in long memory or discarded, resulting in optimization of the memorizing process [ 51 ]. Moreover, Multi-Layer Perceptron (MLP), Fully Convolutional Networks (FCNs), and Radial Basis Function Networks (RBFNs) appeared to have the best performance in almost 3–5% of ANNs. Finally, ML algorithms, belonging to ANNs with low frequency, were Back-Propagation Neural Networks (BPNNs), Modular Artificial Neural Networks (MANNs), Deep Belief Networks (DBNs), Adaptive-Neuro Fuzzy Inference System (ANFIS), Subtractive Clustering Fuzzy Inference System (SCFIS), Takagi-Sugeno Fuzzy Neural Networks (TS-FNN), and Feed Forward Neural Networks (FFNNs).
The second most accurate ML model was Ensemble Learning (EL), contributing to the ML models used in agricultural systems with approximately 22.2%. EL is a concise term for methods that integrate multiple inducers for the purpose of making a decision, normally in supervised ML tasks. An inducer is an algorithm, which gets as an input a number of labeled examples and creates a model that can generalize these examples. Thus, predictions can be made for a set of new unlabeled examples. The key feature of EL is that via combining various models, the errors coming from a single inducer is likely to be compensated from other inducers. Accordingly, the prediction of the overall performance would be superior comparing to a single inducer [ 89 ]. This type of ML model was presented in all sub-categories, apart from crop quality, perhaps owing to the small number of papers belonging in this subcategory. Support Vector Machine (SVM) followed, contributing in approximately 11.5% of the studies. The strength of the SVM stems from its capability to accurately learn data patterns while showing reproducibility. Despite the fact that it can also be applied for regression applications, SVM is a commonly used methodology for classification extending across numerous data science settings [ 90 ], including agricultural research.
Decision Trees (DT) and Regression models came next with equal percentage, namely 4.7%. Both these ML models were presented in all generic categories. As far as DT are concerned, they are either regression or classification models structured in a tree-like architecture. Interestingly, handling missing data in DT is a well-established problem. By implementing DT, the dataset can be gradually organized into smaller subsets, whereas, in parallel, a tree graph is created. In particular, each tree’s node denotes a dissimilar pairwise comparison regarding a certain feature, while each branch corresponds to the result of this comparison. As regards leaf nodes, they stand for the final decision/prediction provided after following a certain rule [ 91 , 92 ]. As for Regression, it is used for supervised learning models intending to model a target value on the basis of independent predictors. In particular, the output can be any number based on what it predicts. Regression is typically applied for time series modeling, prediction, and defining the relationships between the variables.
Finally, the ML models, leading to optimal performance (although with lower contribution to literature), were those of Instance Based Models (IBM) (2.7%), Dimensionality Reduction (DR) (1.5%), Bayesian Models (BM) (0.9%), and Clustering (0.3%). IBM appeared only in crop, water, and livestock management, whereas BM only in crop and soil management. On the other hand, DR and Clustering appeared as the best solution only in crop management. In brief, IBM are memory-based ML models that can learn through comparison of the new instances with examples within the training database. DR can be executed both in unsupervised and supervised learning types, while it is typically carried out in advance of classification/regression so as to prevent dimensionality effects. Concerning the case of BM, they are a family of probabilistic models whose analysis is performed within the Bayesian inference framework. BM can be implemented in both classification and regression problems and belong to the broad category of supervised learning. Finally, Clustering belongs to unsupervised ML models. It contains automatically discovering of natural grouping of data [ 12 ].
4.2.2. Most Studied Crops and Animals
In this sub-section, the most examined crops and animals that were used in the ML models are discussed as a result of our searching within the four sub-categories of crop management similarly to [ 12 ]. These sub-categories refer to yield prediction, disease detection, crop recognition, and crop quality. Overall, approximately 80 different crop species were investigated. The 10 most utilized crops are summarized in Figure 9 . Specifically, the remarkable interest on maize (also known as corn) can be attributed to the fact that it is cultivated in many parts across the globe as well as its versatile usage (for example, direct consumption by humans, animal feed, producing ethanol, and other biofuels). Wheat and rice follow, which are two of the most widely consumed cereal grains. According to the Food and Agriculture Organization (FAO) [ 93 ], the trade in wheat worldwide is more than the summation of all other crops. Concerning rice, it is the cereal grain with the third-highest production and constitutes the most consumed staple food in Asia [ 94 ]. The large contribution of Asian countries presented in Figure 6 , like China and India, justifies the interest in this crop. In the same vein, soybeans, which are broadly distributed in East Asia, USA, Africa, and Australia [ 95 ], were presented in many studies. Finally, tomato, grape, canola/rapeseed (cultivated primarily for its oil-rich seed), potato, cotton, and barley complete the top 10 examined crops. All these species are widely cultivated all over the world. Some other indicative species, which were investigated at least five times in the present reviewed studies, were also alfalfa, citrus, sunflower, pepper, pea, apple, squash, sugarcane, and rye.

The 10 most investigated crops using machine learning models; the results refer to crop management.
As far as livestock management is concerned, the examined animal species can be classified, in descending order of frequency, into the categories of cattle (58.5%), sheep and goats (26.8%), swine (14.6%), poultry (4.9%), and sheepdog (2.4%). As can be depicted in Figure 10 , the last animal, which is historically utilized with regard to the raising of sheep, was investigated only in one study belonging to animal welfare, whereas all the other animals were examined in both categories of livestock management. In particular, the most investigated animal in both animal welfare and livestock production was cattle. Sheep and goats came next, which included nine studies for sheep and two studies for goats. Cattles are usually raised as livestock aimed at meat, milk, and hide used for leather. Similarly, sheep are raised for meat and milk as well as fleece. Finally, swine (often called domestic pigs) and poultry (for example, chicken, turkey, and duck), which are used mainly for their meat or eggs (poultry), had equal contribution from the two livestock sub-categories.

Frequency of animal species in studies concerning livestock management by using machine learning models.
4.2.3. Most Studied Features and Technologies
As mentioned in the beginning of this study, modern agriculture has to incorporate large amounts of heterogeneous data, which have originated from a variety of sensors over large areas at various spatial scale and resolution. Subsequently, such data are used as input into ML algorithms for their iterative learning up until modeling of the process in the most effective way possible. Figure 11 shows the features and technologies that were used in the reviewed studies, separately for each category, for the sake of better comprehending the results of the analysis.

Distribution of the most usual features implemented as input data in the machine learning algorithms for each category/sub-category.
Data coming from remote sensing were the most common in the yield prediction sub-category. Remote sensing, in turn, was primarily based on data derived from satellites (40.6% of the total studies published in this sub-category) and, secondarily, from UAVs (23.2% of the total studies published in this sub-category). A remarkable observation is the rapid increase of the usage of UAVs versus satellites from the year 2018 towards 2020, as UAVs seem to be a reliable alternative that can give faster and cheaper results, usually in higher resolution and independent of the weather conditions. Therefore, UAVs allow for discriminating details of localized circumscribed regions that the satellites’ lowest resolution may miss, especially under cloudy conditions. This explosion in the use of UAV systems in agriculture is a result of the developing market of drones and sensing solutions attached to them, rendering them economically affordable. In addition, the establishment of formal regulations for UAV operations and the simplification and automatization of the operational and analysis processes had a significant contribution on the increasing popularity of these systems. Data pertaining to the weather conditions of the investigated area were also of great importance as well as soil parameters of the farm at hand. An additional way of getting the data was via in situ manual measurements, involving measurements such as crop height, plant growth, and crop maturity. Finally, data concerning topographic, irrigation, and fertilization aspects were presented with approximately equal frequency.
As far as disease detection is concerned, Red-Green-Blue (RGB) images appear to be the most usual input data for the ML algorithms (in 62% of the publications). Normally, deep learning methods like CNNs are implemented with the intention of training a classifier to discriminate images depicting healthy leaves, for example, from infected ones. CNNs use some particular operations to transform the RGB images so that the desired features are enhanced. Subsequently, higher weights are given to the images having the most suitable features. This characteristic constitutes a significant advantage of CNNs as compared to other ML algorithms, when it comes to image classification [ 79 ]. The second most common input data came from either multispectral or hyperspectral measurements originated from spectroradiometers, UAVs, and satellites. Concerning the investigated diseases, fungal diseases were the most common ones with diseases from bacteria following, as is illustrated in Figure 12 a. This kind of disease can cause major problems in agriculture with detrimental economic consequences [ 96 ]. Other examined origins of crop diseases were, in descending order of frequency, pests, viruses, toxicity, and deficiencies.

Distribution of the most usual output features of the machine learning algorithms regarding: ( a ) Disease detection and ( b ) Crop quality.
Images were also the most used input data for weed detection purposes. These images were RGB images that originated mainly from in situ measurements as well as from UGVs and UAVs and, secondarily, multispectral images from the aforementioned sources. Finally, other parameters that were observed, although with lower frequency, were satellite multispectral images, mainly due to the considerably low resolution they provide, video recordings, and hyperspectral and greyscale images. Concerning crop recognition, the majority of the studies used data coming mostly from satellites and, secondarily, from in situ manual measurements. This is attributed to the fact that most of the studies in this category concern crop classification, a sector where satellite imaging is the most widely used data source owing to its potential for analysis of time series of extremely large surfaces of cultivated land. Laboratory measurements followed, while RGB and greyscale images as well as hyperspectral and multispectral measurements from UAVs were observed with lower incidence.
The input data pertaining to crop quality consisted mainly of RGB images, while X-ray images were also utilized (for seed germination monitoring). Additionally, quality parameters, such as color, mass, and flesh firmness, were used. There were also two studies using spectral data either from satellites or spectroradiometers. In general, the studies belonging in this sub-category dealt with either crop quality (80%) or seed germination potential (20%) ( Figure 12 b). The latter refers to the seed quality assessment that is essential for the seed production industry. Two studies were found about germination that both combined X-ray images analysis and ML.
Concerning soil management, various soil properties were taken into account in 65.7% of the studies. These properties included salinity, organic matter content, and electrical conductivity of soil and soil organic carbon. Usage of weather data was also very common (in 48.6% of the studies), while topographic and data pertaining to the soil moisture content (namely the ratio of the water mass over the dry soil) and crop properties were presented with lower frequency. Additionally, remote sensing, including satellite and UAV multispectral and hyperspectral data, as well as proximal sensing, to a lesser extent, were very frequent choices (in 40% of the studies). Finally, properties associated with soil temperature, land type, land cover, root microbial dynamics, and groundwater salinity make up the rest of data, which are labeled as “other” in the corresponding graph of Figure 11 .
In water management, weather data stood for the most common input data (appeared in the 75% of the studies), with ET being used in the vast majority of them. In many cases, accurate estimation of ET (the summation of the transpiration via the plant canopy and the evaporation from plant, soil, and open water surface) is among the most central elements of hydrologic cycle for optimal management of water resources [ 97 ]. Data from remote sensors and measurements of soil water content were also broadly used in this category. Soil water availability has a central impact on crops’ root growth by affecting soil aeration and nutrient availability [ 98 ]. Stem water potential, appearing in three studies, is actually a measure of water tension within the xylem of the plant, therefore functioning as an indicator of the crop’s water status. Furthermore, in situ measurements, soil, and other parameters related to cumulative water infiltration, soil and water quality, field topography, and crop yield were also used, as can be seen in Figure 11 .
Finally, in what concerns livestock management, motion capture sensors, including accelerometers, gyroscopes, and pedometers, were the most common devices giving information about the daily activities of animals. This kind of sensors was used solely in the studies investigating animal welfare. Images, audio, and video recordings came next, however, appearing in both animal welfare and livestock production sub-categories. Physical and growth characteristics followed, with slightly less incidence, by appearing mainly in livestock production sub-category. These characteristics included the animal’s weight, gender, age, metabolites, biometric traits, backfat and muscle thickness, and heat stress. The final characteristic may have detrimental consequences in livestock health and product quality [ 99 ], while through the measurement of backfat and muscle thickness, estimations of the carcass lean yield can be made [ 100 ].
5. Discussion and Main Conclusions
The present systematic review study deals with ML in agriculture, an ever-increasing topic worldwide. To that end, a comprehensive analysis of the present status was conducted concerning the four generic categories that had been identified in the previous review by Liakos et al. [ 12 ]. These categories pertain to crop, water, soil, and livestock management. Thus, by reviewing the relative literature of the last three years (2018–2020), several aspects were analyzed on the basis of an integrated approach. In summary, the following main conclusions can be drawn:
- The majority of the journal papers focused on crop management, whereas the other three generic categories contributed almost with equal percentage. Considering the review paper of [ 12 ] as a reference study, it can be deduced that the above picture remains, more or less, the same, with the only difference being the decrease of the percentage of the articles regarding livestock from 19% to 12% in favor of those referring to crop management. Nonetheless, this reveals just one side of the coin. Taking into account the tremendous increase in the number of relative papers published within the last three years (in particular, 40 articles were identified in [ 12 ] comparing to the 338 of the present literature survey), approximately 400% more publications were found on livestock management. Another important finding was the increasing research interest on crop recognition.
- Several ML algorithms have been developed for the purpose of handling the heterogeneous data coming from agricultural fields. These algorithms can be classified in families of ML models. Similar to [ 12 ], the most efficient ML models proved to be ANNs. Nevertheless, in contrast to [ 12 ], the interest also been shifted towards EL, which can combine the predictions that originated from more than one model. SVM completes the group with the three most accurate ML models in agriculture, due to some advantages, such as its high performance when it works with image data [ 101 ].
- As far as the most investigated crops are concerned, mainly maize and, secondarily, wheat, rice, and soybean were widely studied by using ML. In livestock management, cattle along with sheep and goats stood out constituting almost 85% of the studies. Comparing to [ 12 ], more species have been included, while wheat and rice as well as cattle, remain important specimens for ML applications.
- A very important result of the present review study was the demonstration of the input data used in the ML algorithms and the corresponding sensors. RGB images constituted the most common choice, thus, justifying the broad usage of CNNs due to their ability to handle this type of data more efficiently. Moreover, a wide range of parameters pertaining to weather as well as soil, water, and crop quality was used. The most common means of acquiring measurements for ML applications was remote sensing, including imaging from satellites, UAVs and UGVs, while in situ and laboratory measurements were also used. As highlighted above, UAVs are constantly gaining ground against satellites mainly because of their flexibility and ability to provide images with high resolution under any weather conditions. Satellites, on the other hand, can supply time-series over large areas [ 102 ]. Finally, animal welfare-related studies used mainly devices such as accelerometers for activity recognition, whereas those ones referring to livestock production utilized primary physical and growth characteristics of the animal.
As can be inferred from the geographical distribution (illustrated in Figure 6 ) in tandem with the broad spectrum of research fields, ML applications for facilitating various aspects of management in the agricultural sector is an important issue on an international scale. As a matter of fact, its versatile nature favors convergence research. Convergence research is a relatively recently introduced approach that is based on shared knowledge between different research fields and can have a positive impact on the society. This can refer to several aspects, including improvement of the environmental footprint and assuring human’s health. Towards this direction, ML in agriculture has a considerable potential to create value.
Another noteworthy finding of the present analysis is the capturing of the increasing interest on topics concerning ML analyses in agricultural applications. More specifically, as can be shown in Figure 13 , an approximately 26% increase was presented in the total number of the relevant studies, if a comparison is made between 2018 and 2019. The next year (i.e., 2020), the corresponding increase jumped to 109% against 2019 findings; thus, resulting in an overall 164% rise comparing with 2018. The accelerating rate of the research interest on ML in agriculture is a consequence of various factors, following the considerable advancements of ICT systems in agriculture. Moreover, there exists a vital need for increasing the efficiency of agricultural practices while reducing the environmental burden. This calls for both reliable measurements and handling of large volumes of data as a means of providing a wide overview of the processes taking place in agriculture. The currently observed technological outbreak has a great potential to strengthen agriculture in the direction of enhancing food security and responding to the rising consumers’ demands.

Temporal distribution of the reviewed studies focusing on machine learning in agriculture, which were published within 2018–2020.
In a nutshell, ICT in combination with ML, seem to constitute one of our best hopes to meet the emerging challenges. Taking into account the rate of today’s data accumulation along with the advancement of various technologies, farms will certainly need to advance their management practices by adopting Decision Support Systems (DSSs) tailored to the needs of each cultivation system. These DSSs use algorithms, which have the ability to work on a wider set of cases by considering a vast amount of data and parameters that the farmers would be impossible to handle. However, the majority of ICT necessitates upfront costs to be paid, namely the high infrastructure investment costs that frequently prevent farmers from adopting these technologies. This is going to be a pressing issue, mainly in developing economies, where agriculture is an essential economic factor. Nevertheless, having a tangible impact is a long-haul game. A different mentality is required by all stakeholders so as to learn new skills, be aware of the potential profits of handling big data, and assert sufficient funding. Overall, considering the constantly increasing recognition of the value of artificial intelligence in agriculture, ML will definitely become a behind-the-scenes enabler for the establishment of a sustainable and more productive agriculture. It is anticipated that the present systematic effort is going to constitute a beneficial guide to researchers, manufacturers, engineers, ICT system developers, policymakers, and farmers and, consequently, contribute towards a more systematic research on ML in agriculture.
In this section, the reviewed articles are summarized within the corresponding Tables as described in Table 2 .
Crop Management: Yield Prediction.
Acc: Accuracy: CA: Conservation Agriculture; CI: Crop Indices; CEC: Cation Exchange Capacity; CCC: Concordance Correlation Coefficient; DOY: Day Of Year; EC: Electrical Conductivity; HD: Heading Date; HDM: Heading Date to Maturity; K: Potassium; Mg: Magnesium; N: Nitrogen; OLI: Operational Land Imager; P: Phosphorus; RGB: Red-Green-Blue; S: Sulphur; SOM: Soil Organic Matter; SPAD: Soil and Plant Analyzer Development; STI: Soil Texture Information; STD: Standard Deviation; UAV: Unmanned Aerial Vehicle; UGV: Unmanned Ground Vehicle.
Crop Management: Disease Detection.
Acc: Accuracy; AUC: Area Under Curve; CR: Cedar Rust; ExGR: Excess Green Minus Excess Red; FS: Frogeye Spot; H: Healthy; mAP: mean Average Precision; RGB: Red-Green-Blue; S: Scab; TYLC: Tomato Yellow Leaf Curl; UAV: Unmanned Aerial Vehicle; VddNet: Vine Disease Detection Network.
Crop Management: Weed Detection.
Acc: Accuracy; AUC: Area under Curve; IoU: Intersection over Union; mAP: mean Average Precision; RGB: Red-Green-Blue; UAV: Unmanned Aerial Vehicle; UGV: Unmanned Ground Vehicle.
Crop Management: Crop Recognition.
Acc: Accuracy; IoU: Intersection over Union; RGB: Red-Green-Blue; UAV: Unmanned Aerial Vehicle.
Crop Management: Crop Quality.
Acc: Accuracy; DSM: Detection and Segmentation Module; EDG: Estimated Dimensions Geometry; IVTD: In Vitro True Digestibility; RGB; Red-Green-Blue; MMD: Manually Measured Dimensions; mAP: mean Average Precision; PSO: Particle Swarm Optimization; RGB; Red-Green-Blue; SAE: Stacked AutoEncoder; VI: Vegetation Indices; WF: Wavelet Features.
Water management.
Acc: Accuracy; CC: Coefficient of Correlation; ET: Evapotranspiration; ET o : reference EvapoTranspiration; ROC: Receiver Operating Characteristic; ME: Model Efficiency; NSE: Nash-Sutcliffe model efficiency Coefficient; POD: Probability Of Detection.
Soil management.
ACCA: Aminoyclopropane-1-carboxylate; AUC: Area Under Curve; BP: Bacterial Population; CC: Coefficient of Correlation; CCC: Concordance Correlation Coefficient; CCE: Calcium Carbonate Equivalent; ET: EvaporoTransporation; MIR: Mid InfraRed; NSE: Nash-Sutcliffe model efficiency Coefficient; NIR: Near-InfraRed; PS: Phosphate Solubilization; PWP: Permanent Wilting Point; RPIQ: Ratio of Performance to Interquartile Range; RPD: Relative Percent Deviation; SOC: Soil Organic Carbon; WI: Willmott’s Index.
Livestock Management: Animal Welfare.
AUC: Area Under Curve; Cont: Contagious; DE: Digestible Energy; ED: Energy Digestibility; ENV: Environmental; DWT: Discrete Wavelet Transform; MFCCs: Mel-Frequency Cepstral Coefficients; NIR: Near InfraRed; NPV: Negative Predictive Value; PTZ: Pan-Tilt-Zoom; PPV: Positive Predictive Value; RGB: Red-Green-Blue; RR: Respiration Rate; ST: Skin Temperature.
Livestock Management: Livestock Production.
ACFW: Adult Clean Fleece Weight; ADG: Average Daily Gain; AFD: Adult Fibre Diameter; AGFW: Adult Greasy Fleece Weight; ASL: Adult Staple Length; ASS: Adult Staple Strength; BBFT: Bacon/BackFat Thickness; BCS: Body Condition Score; CCW: Cold Carcass Weights; CTLEAN: Computed Tomography Lean Meat Yield; DBT: Deep Body Temperature; EMA: Eye Muscle Area; GWAS: Genome-Wide Association Studies; GRFAT: Greville Rule Fat Depth; HER: Human Error Range; IMF: IntraMuscular Fat; HCW: Hot Carcass Weight; LW: Loin Weight; MS: Marbling Score; MT: Muscle Thickness; REIMS: Rapid Evaporative Ionization Mass Spectrometry; RGB: Red-Green-Blue; SMY: Saleable Meat Yield.
Abbreviations for machine learning models.
Abbreviations for machine learning algorithms.
Author Contributions
Conceptualization, D.B.; methodology, L.B., G.D., R.B., D.K. and A.C.T.; investigation, L.B. and G.D.; writing—original draft preparation, L.B. and A.C.T.; writing—review and editing, L.B., G.D., D.K., A.C.T., R.B. and D.B.; visualization, L.B.; supervision, D.B. All authors have read and agreed to the published version of the manuscript.
This work has been partly supported by the Project “BioCircular: Bio-production System for Circular Precision Farming” (project code: T1EDK- 03987) co-financed by the European Union and the Greek national funds through the Operational Programme Competitiveness, Entrepreneurship and Innovation, under the call RESEARCH—CREATE—INNOVATE.
Conflicts of Interest
The authors declare no conflict of interest.
Publisher’s Note: MDPI stays neutral with regard to jurisdictional claims in published maps and institutional affiliations.
- Paper writing help
- Buy an Essay
- Pay for essay
- Buy Research Paper
- Write My Research Paper
- Research Paper Help
- Custom Research Paper
- Custom Dissertation
- Dissertation Help
- Buy Dissertation
- Dissertation Writer
- Write my Dissertation
- How it works
130 Agriculture Research Topics To Write An Excellent Paper
The preparation of an agriculture research paper involves several nuances and complexities. The first aspect is technical requirements, such as text formatting, structure, and source list. It's also important to choose those agriculture topics that you can analyze and find expert material. Any research paper is based on theses and statements, which are supported by evidence and factual information.
This is especially important when you tend to choose agricultural controversial topics. Then you need to find studies with verified information and prepare arguments for your paper. The whole process of work requires meticulous data collection and analysis of alternative sources. Then choosing any agricultural essay topics won't seem like a heady decision.
Your academic paper may relate to environmental factors, the economic feasibility of starting a farm, or the nuances of breeding. The main plus is that you can choose any of the agricultural related topics for research preparation. Here are 130 options for you.
Fisheries And Aquaculture
Such agricultural research paper topics allow revealing the topic of fishery and agricultural procurement. Students can concentrate on many aspects of the payback of farms and fisheries. The topics are quite extensive, and you can find a lot of research on the Internet for choosing trust sources.
- Trout breeding in freshwaters.
- Effect of algae on oxygen levels in fish rates.
- Seasonal spawning of oceanic fish.
- Prohibited fishing waters in the United States.
- Exploration of the Pacific Ocean.
- The impact of cyclones on fishing.
- Poisonous fish and the reasons for their breeding in North America.
- Seasonal diseases of trout.
- Sea horse: A case study.
- Risk analysis of water quality in aquaculture.
Plant Science And Crop Production
Crop Production agricultural research topics and plant science are not the easiest, but they contain a ton of information on the Internet. It is not a problem to find research by leading scientists and create your own research paper based on their statistics. The plus is that you don't have to start from scratch.
- Innovative plant breeding.
- Reclamation as a method of increasing yields.
- Hybrid plants of Montana.
- Citrus growing methods.
- Technical cannabis and plantations in the USA.
- Analysis of the yield of leguminous crops.
- Method for creating genetically modified plants.
- Field analysis of wheat for pesticides.
- New plants and methods of growing them.
- Hybrids and cold-resistant plants.
Topics in Agricultural Science
Agriculture essay topics like this allow you to select a specific aspect to research. You can concentrate on vegetation breeding or high tech greenhouse methodology. A large amount of research is a definite plus because you can build your theses on the basis of available data, criticizing or supporting research by scientists.
- Harvesting robots.
- Methodology for improving agricultural performance.
- The influence of technology on the growth of grain crops.
- How important is the timely irrigation of fields?
- Climatic changes and impact on yield.
- Breeding earthworms.
- Hydroponic gardening.
- Genetically modified organisms and their distribution.
- Starting a garden.
- How can we make medicine from plants?
Topics in Agronomy
Agronomy agriculture projects for students allow you to consider the aspects of growing crops in conditions with a specific soil type and natural characteristics. You can base your claims on statistics with the ability to draw on facts from other research. For example, this is relevant for papers examining the fertility of the topsoil.
- Choosing the type of soil for the cornfield.
- Innovative land reclamation.
- New branches in agronomy.
- Phosphate-free fertilizers.
- Hydroponics and greenhouses.
- Hybrid yield analysis.
- Methodology for assessing agronomic losses.
- Stages of preparing a field for harvesting.
- The role of GMOs in the fight against insect pests.
- Cultivation of technical hemp and soil fertilization methods.
Topics in Animal Breeding And Genetics
Agriculture related topics are interesting because you can touch on aspects of genetics and breeding. Students can concentrate on specific aspects of species modification and animal rearing. The research paper will look more convincing when there are references to real scientific papers with statistics and experimental results.
- Breeding new types of sheep.
- Breeding bulls and genetic engineering.
- The influence of selection on the growth of the animal population.
- Proper nutrition for livestock in winter.
- Vitamin complexes for animals.
- Genetic changes in chickens for resistance to cold.
- Nuances of animal genetic modifications.
- Stages of caring for newborn kittens.
- What is a negative selection?
- Basic methods of genetic experiments on animals.
Topics in Animal Production And Health
Such agriculture research paper topics are especially interesting because you can write about farming aspects in the context of raising animals, vegetables, and various crops. It is broad enough, so you will not be limited by narrow boundaries and will be able to consider many aspects of your research paper.
- Environmental threats to the oversupply of the sheep population.
- The role of livestock in marginal areas.
- Livestock digitalization.
- Animal selection for meat preparation.
- Analysis of livestock farms.
- Animal production evaluation technique.
- Cow health during calving.
- The importance of animal vaccination.
- Technical aspects of the medical treatment of animals.
- Environmental aspects of animal husbandry.
Topics in Ecotourism And Wildlife
Ecotourism is gaining momentum all over the world. The new trend is aimed at bringing people closer to nature and exploring the beauty of different countries. This issue will be of interest to those who want to talk about wildlife and nature reserves. The topic is quite extensive, so students will not have problems with preparing a research paper.
- Minnesota and Eco-Tourism.
- The influence of wolves on the formation of the local ecosystem.
- Recreational tourism in the USA.
- Methods for preparing resorts for eco-tourism.
- Lakes and environmental factors.
- A technique for preserving wildlife in its original form.
- Classic models of eco-tourism.
- Stages of creating ecological reserves.
- The role of tourism in the restoration of the ecological environment.
- The main factors of wildlife conservation.
- The legislative framework for wildlife protection.
- The nuances of creating a farm in reserve.
- Consolidation of resources for the development of a livestock farm.
Topics in Farm Management
Managing a farm can be a complex and multifaceted process. Many students may choose this topic to talk about aspects of breeding and breeding pets or crops. The topic is quite extensive and allows you to touch on any aspect of the farmer's activities related to the production and sale of products.
- Farm methods to improve performance.
- Stages of creating a livestock farm.
- Farm success analysis forms.
- Management of the process of planting crops.
- The role of modern equipment in cow milking.
- Farm reporting and profitability analysis.
- Breeding exotic animals.
- Rabbit population management.
- Statistical methodology for farm control.
- Stages of the animal population control on the farm.
Topics in Fisheries And Aquaculture
A similar topic is associated with fish farming, introductory aquaculture, and general aquaculture. Quite a few students can prepare a good research paper if they turn to other people's research and use it as a basis to prove or disprove their own claims and theories. It is also a good opportunity to select food related research topics as you can touch upon the aspect of fish farming and marketing.
- Creation and management of a fish rate.
- Sturgeon breeding and distribution.
- Methods for improving the ecological state of water bodies.
- Planting plants in reservoirs for liquid purification.
- Fish spawning control.
- The aquaculture aspect and social trends.
- Methods for increasing fish resources.
- Breeding in the fishing industry.
- Methods for creating a fish farm.
- River resource monitoring and digitalization.
Topics in Agric Business And Financial Management
Control of a livestock or vegetable enterprise depends on many factors, so such a topic's choice will be extremely relevant. The student's most important task is to bring only proven facts and arguments of his own judgments. These agriculture topics for students include an overview of many business processes and farm management.
- The farm cost reduction methodology.
- US agricultural financing sector.
- Agricultural business practices.
- Data analysis and farming development.
- Financial management of small livestock farms.
- Impact of drought on yield.
- Cost and payback of farms.
- Selecting a region for creating a farm.
- A method for analyzing animal resources on a farm.
- Management of automated farming enterprises.
- Local farming business.
- Key factors of farm management.
- Farm reports and breeding work.
Topics in Agric Meteorology And Water Management
Meteorological aspects are very important for the management of a company or agricultural enterprises. Another aspect of this topic is water management, which may also be interesting for those who are going to reveal the nuances of fish farming in local waters. The topic will be especially interesting for those who want to connect their lives with agronomy and a similar field.
- Cattle breeding methodology.
- Pig breeding methods.
- Water management to maximize profits.
- The choice of a reservoir for growing fish.
- Analysis of the ecological situation in water bodies.
- Farm equipment management techniques.
- Water supply for farm households.
- Analysis and selection of a farm development methodology.
- Finding the right methods for creating protected reservoirs.
- Stages of development of a water farm.
Other Agric Topics
Sometimes choosing a specific topic can be difficult. This is because students are not quite sure which study to base their paper on. You can take a neutral topic that has no specific relation to breeding, meteorology, or farming aspects in such cases.
- Innovative farming methods.
- Choosing the right water farm management model.
- The nuances of trout breeding.
- Population control and livestock farm development plan.
- Financial analytics and purchase of farm animals.
- The self-sufficiency period of the fish farm.
- How to create fish spawning tanks?
- Selection of breeds of cows for farming.
- Methodology for calculating farm risks.
- Time management and selection of plants for the plantation.
- Features of the legal registration of a farm household.
- Modern agricultural drones.
- The difference between Ayn Rand's anthem and George Orwell's animal farm.
- Animal rights vs. animal welfare.
How to Write a Good Agriculture Research Paper?
One of the main life hacks for getting a high mark is choosing controversial agricultural topics. Choosing this option allows students to consider an interesting statement and back it up with real facts. A paper-based on real statistics with proof of student work is valued above all else.
But even when choosing a good topic, you still need to prepare the right outline for writing your research paper. The introduction should be of the highest quality as well as the final paragraph since these are the main parts that affect the assessment. Real facts and statistics must support all the statements above if you are talking about specific figures. Many colleges and universities have their own paper requirements as well as the nuances of the design of research work. You must consider each parameter in order to get the best result.
If it is difficult to find controversial topics in agriculture and write a high-quality research paper, we can help you with this issue. Our best essay writing service has been in operation for many years and provides writing assistance for many types of essays, research papers, and theses. We will help you synchronize your preparation process and create an expert paper that gets high marks. You can switch to other tasks and get the opportunity to free up some time to study other disciplines.
An Inspiration List:
- Agricultural Research
- Current Agriculture Research Journal
- Agricultural Research & Technology
- Journal of Agriculture and Food Research
- Advances in Plants & Agriculture Research
- Journal of Bioscience and Agriculture Research
- Middle East Journal of Agriculture Research
186 Agriculture Essay Topics & Research Questions + Examples
Are you looking for the best agriculture topics to write about? You’re at the right place! StudyCorgi has prepared a list of important agriculture research topics. On this page, any student can find essay questions and project ideas on various agricultural issues, such as food safety, genetically engineered crops, and sustainable farming practices.
👨🌾 TOP 7 Agriculture Research Topics – 2024
🏆 best essay topics on agriculture, 🎓 most interesting agriculture topics for college students, 👍 good agriculture research topics & essay examples, 💡 cool agricultural research topics for high school students, ❓ research questions about agriculture, 🔎 current agriculture research paper topics, 📝 agriculture argumentative essay topics, 🗣️ agriculture topics for speech.
- Globalization Impact on Sustainable Agriculture
- Agriculture and Its Role in Economic Development
- Agricultural Biotechnology and Its Pros and Cons
- Food Safety Issues in Modern Agriculture
- Agriculture: Personal Field Visit
- Commercial Agriculture, Its Role and Definition
- In Support of Robotics Use in Agriculture
- The Impact of Pesticides’ Use on Agriculture Pesticides are mostly known for their adverse effects and, therefore, have a mostly negative connotation when discussed among general audiences.
- Agricultural Influences on the Developing Civil Society Agriculture had a significant influence on developing societies, ranging from creating trade to bringing industrialization, education, and social classes.
- Agriculture and Food in Ancient Greece The paper states that agricultural practices and goods from Greece extended to neighboring countries in the Mediterranean as the dominance increased.
- Industry and Agriculture: Use of Technology Industry and agriculture are among the areas that have experienced a vast rise in effectiveness and performance quality due to the integration of new types of technology into them.
- Soil: The Essential Aspect of Agriculture Soil is an integral part of human life as it determines one’s quality of life. The health of the soil is reduced by erosion and degradation due to human activities.
- Agricultural Role in African Development Diao et al. attempt to determine the role of agriculture in overcoming the challenge of poverty in rural areas of Africa compared to alternative theories of economic growth.
- Population Growth and Agriculture in the Future The current industrial agriculture needs to be advanced and developed in combination with sustainable agricultural practices.
- Hunting and Gathering Versus Agricultural Society The hunting and gathering society is considered the most equitable of all seven types, while the agricultural community gives rise to the development of civilization.
- Agriculture the Backbone of Ancient Egypt’s Economy In pre-industrial societies, agriculture was the backbone of most economies. This is true in ancient times and very much evident in ancient Egypt.
- Improving Stress Resistance in Agricultural Crops The essay suggests that stress-resistant crops are needed to ensure yield stability under stress conditions and to minimize the environmental impacts of crop production.
- Virtual Water Trade and Savings in Agriculture This essay discusses the savings associated with virtual water trade in agriculture and touches on the effects of a shift to local agricultural production on global water savings.
- Repeasantization: Impact on Agriculture The repeasantization led to fundamental changes that created a new system of agriculture that is still relevant today.
- History of Agricultural Technology Development Agricultural technologies were majorly developed during the Medieval period to ensure sufficient product yields for growing populations around the world.
- The Big History of Civilizations – Origins of Agriculture: Video Analysis This paper aims to analyze the origins of agriculture – what was a foraging economy and way of life like, as well as compare foragers and farmers.
- Agriculture: Application of Information Technology IT application in agriculture has contributed to food security in most modern communities. Farming has become easier than before as new inventions are made.
- Food and Agriculture of Ancient Greece The concepts of agriculture and cuisine both have a deep connection to Greek history, culture, development, and social trends.
- The Neolithic Era: Architecture and Agriculture The improvements to agriculture, society, architecture, and culture made during the Neolithic period had an undeniable impact on aspects of the world.
- Sustainable Agriculture Against Food Insecurity The paper argues sustainable agriculture is one way to reduce food insecurity without harming the planet because the number of resources is currently decreasing.
- Agricultural Technology Implementation by Medieval Europeans and West Africans The paper examines how West Africans and Medieval Europeans were affected by their corresponding climates and why their methods were unique to their respective locations.
- Agricultural Traditions of Canadians In Canada there is a very good agricultural education, so young people can get higher education in agriculture and use it on their own farms.
- Climate Changes Impact on Agriculture and Livestock The project evaluates the influences of climate changes on agriculture and livestock in different areas in the Kingdom of Saudi Arabia.
- Food Safety: A Policy Issue in Agriculture Today Food safety constitutes proper preparation, storage and preservation of all foods. Markets are increasingly calling for improvement in the quality and safety standards of food crops.
- Colonialism and Economic Development of Africa Through Agriculture The colonial period is characterized by the exploitation of the agricultural sector in Africa to make a profit and provide Western countries with raw materials.
- Agriculture Development and Related Theories There are two main domestication models used to describe the development of agriculture: unconscious and conscious.
- Agriculture in Honduras: Existing Challenges and Possible Solutions This paper tackles the issue of existing challenges and possible solutions to the problems of agriculture in Honduras.
- Market Revolution: Agriculture and Global Trade In the era of traders, the vast land area and rich natural resources created many economic opportunities. Most people lived in rural areas and were engaged in agriculture.
- Agriculture and Food Production in the Old Kingdom
- Agriculture and the Transition to the Market in Asia
- Agrarian Reform and Subsistence Agriculture in Russia
- Agriculture, Nutrition, and the Green Revolution in Bangladesh
- Agriculture Business and Management
- Agriculture, Horticulture, and Ancient Egypt
- Agriculture and Food Production in the Old Kingdom of Egypt
- Administrative and Transaction-Related Costs of Subsidising Agriculture
- Agriculture and Economic Growth in Argentina, 1913-84
- Agriculture and Economic Development in Brazil, 1960-1995
- Agriculture and Greenhouse Gas Cap-And-Trade
- Croatian Agriculture Towards World Market Liberalization
- Adapting Credit Risk Models to Agriculture
- Agriculture and European Union Enlargement
- Agriculture and Food Security in Pakistan
- Cash Flows and Financing in Texas Agriculture
- Current Problems With Indian Agriculture
- Agriculture and Its Drain on California
- Agriculture and the Economic Life of India
- Agriculture and Global Climate Stabilization
- Achieving Regional Growth Dynamics in African Agriculture
- Agriculture and Non-agricultural Liberalization in the Millennium Round
- Corporate Agriculture and Modern Times
- Agriculture and Rural Employment Agricultural in Bolivia
- Climatic Fluctuations and the DI¤Usion of Agriculture
- Agriculture Global Market Briefing
- Agriculture and the Industrial Revolution of the Late 1700s
- Agriculture and Animal Husbandry in Ecuador
- Biofuels, Agriculture, and Climate Change
- Aggregate Technical Efficiency and Water Use in U.S. Agriculture
- Agriculture, Water, and Food Security in Tanzania This paper evaluates the strategies applicable to the development and further maintenance of agriculture, water, and food security in Tanzania.
- The Australian Agriculture Company’s Financial Analysis The Australian Agriculture Company shows a positive sign for investment due to its financial analysis indicating company resilience and strong prospects of growth.
- Governmental Price Control in Agricultural Sector The consequences of real-life governmental price control are the evolutionary nature of transformations in the agricultural sector.
- The Agriculture Industry’s Digital Transformation This study seeks to explore the dynamics of digital technology in agriculture over the past two decades, focusing on the perspectives and perceptions of the farmers.
- Aspects of Pesticide Use in Agriculture This paper investigates socio-environmental factors connected with pesticide use in agriculture and food production. It has a destructive impact on the environment
- Agriculture-Led Food Crops and Cash Crops in Tanzania This paper aims to explore the contributions of the agriculture sector in Tanzania to the country’s industrialization process by using recent data about its food and cash crops.
- Cuisine and Agriculture of Ancient Greece There are many reasons for modern students to investigate the development of cuisine and agriculture in Ancient Greece.
- Agriculture and Food Safety in the United States Agriculture in the United States has grown progressively centralized. The shortcomings in the 2018 U.S. farm legislation resulted in multiple challenges in the food system.
- Sustainable Agriculture and Future Perspectives Sustainable agriculture is essential to the earth’s environment. When farmers take care of their land and crops, they are taking care of environmental sustainability.
- Agricultural Adaptation to Changing Environments The paper discusses the impact of climate change on agriculture in Canada. This phenomenon is real and has affected the industry over at least the last three decades.
- Trade Peculiarities in Food and Agriculture Food trading is a peculiar area, as food is the basis for surviving the population. The one who controls food production and trading routes, also controls all populations.
- Multinational Agricultural Manufacturing Companies’ Standardization & Adaptation The most popular approaches that multinational companies use to serve their customers from various countries are standardization and adaptation.
- Impacts of Climate Change on Agriculture and Food This paper will examine four aspects of climate change: variation in the rainfall pattern, water levels, drought, temperature, and heatwaves.
- Canadian Laws Regarding Agricultural Sector The unions in Canada are the concept over which there has been an excessive dispute involving court proceedings and questioning the constitutional rights of citizens.
- Sharecropping. History of Racial Agriculture Sharecropping became a variation of racialized agriculture, that which has negative impact on the capabilities of the black population to generate and pass down wealth.
- Food Additives Use in Agriculture in the United States Food additives in agriculture become a debatable issue because their benefits do not always prevail over such shortages like health issues and environmental concerns.
- Radio-Frequency Identification in Healthcare and Agriculture Specifically, radio-frequency identification (RFID) has gained traction due to its ability to transmit data over distance.
- Mechanism of US Agricultural Market The fact that lower interest rates increased the number of potential customers for real estate in the 2000s shows that housing prices should have increased.
- A Biological Terror Attack in Agriculture The United States is highly vulnerable to terror attacks of biological nature in agriculture yet such an occurrence can cripple the economy.
- The Economics of Race, Agriculture and Environment This research paper is going to answer the question; do public policies reduce or enhance racial inequality in agricultural and environmental affairs?
- Impact of Bioterrorism on the U.S Agriculture System The paper describes that the term bioterrorism has several definitions depending upon the origin of the attack but in general terms, it refers to any form of terrorist attack.
- Impacts of Genetic Engineering of Agricultural Crops In present days the importance of genetic engineering grew due to the innovations in biotechnologies and Sciences.
- The Effects of Genetic Modification of Agricultural Products Discussion of the threat to the health of the global population of genetically modified food in the works of Such authors as Jane Brody and David Ehrenfeld.
- Climate Change and Its Potential Impact on Agriculture and Food Supply The global food supply chain has been greatly affected by the impact of global climate change. There are, however, benefits as well as drawbacks to crop production.
- Agriculture and Mayan Society Resilience The Yucatan peninsula had a vast landscape which was good for agriculture thus making agriculture to be the main economic base for the Mayans.
- Homeland Security in Agriculture and Health Sectors Lack of attention to the security and protection of the agricultural sector in the U.S. economy can create a serious threat to the health and safety of the population.
- Water Savings and Virtual Trade in Agriculture Water trade in agriculture is not a practice that is unique to the modern generation. The practice was common long before the emergence of the Egyptian Empire.
- Virtual Water Trade of Agricultural Products Virtual water trade is a concept associated with globalization and the global economy. Its rise was motivated by growing water scarcity in arid areas around the world.
- Virtual Water Savings and Trade in Agriculture The idea of virtual water was initially created as a method for assessing how water-rare nations could offer food, clothing, and other water-intensive products to their residents.
- European Invasion and Agriculture in the Caribbean The early invasion of the Europeans in the Caribbean did not prompt the employment of the slave trade in the agricultural activities until the development of the sugar plantations.
- Freedom in American Countryside and Agriculture This paper portrays how freedom has been eliminated in the countryside by the state agriculture department, and whether the farmer has a moral right to do his farming practices.
- Agricultural Problems in Venezuela Agriculture has been greatly underdeveloped in Venezuela, yet it is a country that has vital minerals and resources required for the global economy.
- America’s Agriculture in the Period of 1865-1938 This paper analyzes America’s contribution in prevention of natural calamities, decline of soil quality, promotion of production outlay and provision of sufficient food.
- Capital Taxes and Agriculture
- Canadian Trade With the Chinese Agriculture Market
- Agriculture and Its Impact on Economic Development
- Bacteriocins From the Rhizosphere Microbiome From an Agriculture Perspective
- Agriculture and Its Impact on Financial Institutions
- Agriculture, Fisheries, and Food in the Irish Economy
- Adoption and Economic Impact of Site-Specific Technologies in U.S. Agriculture
- Cash Rents and Land Values in U.S. Agriculture
- Crises and Structural Change in Australian Agriculture
- Biotechnology and Its Application in Agriculture
- Alternative Policies for Agriculture in Europe
- Agriculture and Food Security in Asia by 2030
- Agriculture and Coping Climate Change in Nepal
- Agriculture and Ethiopia’s Economic Transformation
- Culture: Agriculture and Egalitarian Social
- Adaptation, Climate Change, Agriculture, and Water
- Agriculture and the Literati in Colonial Bengal, 1870 to 1940
- Agriculture and Barley Farming Taro
- Agriculture and Agricultural Inputs Markets
- Agriculture and Environmental Challenges
- Challenges for Sustainable Agriculture in India
- Agriculture and German Reunification
- Agriculture and Tourism Relationship in Malaysia Tourism
- 21st Century Rural America: New Horizons for U.S. Agriculture
- Canadian Agriculture and the Canadian Agricultural Industry
- California Agriculture Dimensions and Issues
- Advancements and the Development of Agriculture in Ancient Greece and Rome
- Agriculture and Early Industrial Revolution
- Aztec: Agriculture and Habersham County
- Agriculture and Current Deforestation Practices
- How Has Agriculture Changed From Early Egypt, Greece, and Rome to the Present?
- What Are the Advantages of Using Pesticides on Agriculture?
- Are Digital Technologies for the Future of Agriculture?
- How Did Agriculture Change Our Society?
- Does Agriculture Help Poverty and Inequality Reduction?
- Can Agriculture Prosper Without Increased Social Capital?
- Are Mega-Farms the Future of Global Agriculture?
- How Can African Agriculture Adapt to Climate Change?
- Does Agriculture Really Matter for Economic Growth in Developing Countries?
- Can Conservation Agriculture Save Tropical Forests?
- How Can Sustainable Agriculture Be Better for Americans?
- Are U.S. and European Union Agriculture Policies Becoming More Similar?
- Should Pollution Reductions Count as Productivity Gains for Agriculture?
- Can Market Access Help African Agriculture?
- How Does Genetic Engineering Affect Agriculture?
- Does Individualization Help Productivity of Transition Agriculture?
- Can Spot and Contract Markets Co-Exist in Agriculture?
- How Has Biotechnology Changed Agriculture Throughout the Years?
- Does Trade Policy Impact Food and Agriculture Global Value Chain Participation of Sub-Saharan African Countries?
- Can Sustainable Agriculture Feed Africa?
- How Can Multifunctional Agriculture Support a Transition to a Green Economy in Africa?
- Does Urban Agriculture Enhance Dietary Diversity?
- How Did Government Policy, Technology, and Economic Conditions Affect Agriculture?
- Can the Small Dairy Farm Remain Competitive in US Agriculture?
- What Are the Main Changes in French Agriculture Since 1945 and What Challenges Does It Face Today?
- How Can Marketing Theory Be Applied to Policy Design to Deliver Sustainable Agriculture in England?
- Will African Agriculture Survive Climate Change?
- How Has Agriculture Changed Civilizations?
- Does Urban Agriculture Improve Food Security?
- Can US and Great Plains Agriculture Compete in the World Market?
- The effect of climate change on crop yields and food security.
- Sustainable agricultural practices for soil health.
- Precision agriculture techniques and applications.
- The impact of genetically engineered organisms on crop yields and safety.
- The benefits of agroforestry systems for the environment.
- Current challenges in water management in agriculture.
- The environmental impact of organic farming.
- The potential of urban agriculture to address food insecurity.
- Food waste in the agricultural supply chain.
- Comparing the effectiveness of aquaponic and hydroponic systems.
- Organic vs. conventional farming.
- Can regenerative agriculture combat climate change?
- Agricultural subsidies: pros and cons.
- Should harmful pesticides be banned to protect pollinators?
- Should arable land be used for biofuels or food production?
- Do patent protections of seeds hinder agricultural innovation?
- Agricultural robots: increased efficiency or displaced rural labor?
- Should GMO labeling be mandatory?
- Do the benefits of pesticides outweigh their potential health harms?
- Is it unsustainable to grow water-intensive crops in arid regions?
- The economics of organic farming.
- The need for climate-adaptive crops.
- The role of bees in agriculture and threats to their survival.
- Smart agriculture: transforming farming with data and connectivity.
- The journey of food in modern agricultural supply chains.
- The role of agri-tech startups in agricultural innovation.
- Youth in agriculture: inspiring the next generation of farmers.
- Why should we shift to plant-based meat alternatives?
- The importance of preserving indigenous agricultural practices.
- Smart irrigation systems: optimizing water use in agriculture.
Cite this post
- Chicago (N-B)
- Chicago (A-D)
StudyCorgi. (2022, March 1). 186 Agriculture Essay Topics & Research Questions + Examples. https://studycorgi.com/ideas/agriculture-essay-topics/
"186 Agriculture Essay Topics & Research Questions + Examples." StudyCorgi , 1 Mar. 2022, studycorgi.com/ideas/agriculture-essay-topics/.
StudyCorgi . (2022) '186 Agriculture Essay Topics & Research Questions + Examples'. 1 March.
1. StudyCorgi . "186 Agriculture Essay Topics & Research Questions + Examples." March 1, 2022. https://studycorgi.com/ideas/agriculture-essay-topics/.
Bibliography
StudyCorgi . "186 Agriculture Essay Topics & Research Questions + Examples." March 1, 2022. https://studycorgi.com/ideas/agriculture-essay-topics/.
StudyCorgi . 2022. "186 Agriculture Essay Topics & Research Questions + Examples." March 1, 2022. https://studycorgi.com/ideas/agriculture-essay-topics/.
These essay examples and topics on Agriculture were carefully selected by the StudyCorgi editorial team. They meet our highest standards in terms of grammar, punctuation, style, and fact accuracy. Please ensure you properly reference the materials if you’re using them to write your assignment.
This essay topic collection was updated on January 21, 2024 .
The Ohio State University
- BuckeyeLink
- Find People
- Search Ohio State
CFAES COVID-19 Resources: Safe and Healthy Buckeyes | COVID-19 Hub | CFAES Calendar

Ohio State University Extension
Search form
Explore on-farm research reports, breadcrumb menu.

You can now find our most recent on-farm research reports at https://agcrops.osu.edu/on-farm-research . Explore new and past studies using our search tool, or filter reports by county with the clickable map of Ohio.
Reports provide in-depth summaries of projects conducted on farms and research branch stations by Ohio State University Extension professionals. All studies are replicated, randomized trials and reports are peer reviewed by two specialists.
In the past review cycle, there were 15 total reports published from studies conducted in 9 different counties. See below for a summary of reports and direct link to their respective PDF reports:
If you are interested in conducting on-farm research, the OSU eFields program provides the opportunity to work and learn together with OSU Extension professionals. Reach out to your local OSU Extension office or the eFields team at [email protected] to learn how to get involved.
Crop Observation and Recommendation Network
C.O.R.N. Newsletter is a summary of crop observations, related information, and appropriate recommendations for Ohio crop producers and industry. C.O.R.N. Newsletter is produced by the Ohio State University Extension Agronomy Team, state specialists at The Ohio State University and the Ohio Agricultural Research and Development Center (OARDC). C.O.R.N. Newsletter questions are directed to Extension and OARDC state specialists and associates at Ohio State.
- Browse Works
Agriculture
Browse agriculture topics/papers by subfields, agriculture research papers/topics, determining the profitability of different npsb and nitrogen fertilizer rates on yield and yield attributes of sweet potato (ipomoea batatas l.) in the midland areas of guji southern oromia,.
The activity was proposed with the objective of determining the combined application rate of inorganic NPSB and N fertilizer that maximizes the yield and yield components of sweet potato. Sweet Potato variety Naspot-13, was used for the experiment. The experiment was arranged in factorial combination of four level of NPSB (0, 50, 100 and 150 kg ha-1) with four level of N2 (0, 23, 46 and 69 kg ha-1) in RCBD with 3 replications. The analysis of variance showed that the combination of 100 kg ha-...
Diet selection, intake and weight gain of dorper sheep fed on selected grasses in a cafeteria system in Machakos County, Kenya
Abstract: Livestock is a key asset and a primary livelihood resource for rural households in most parts of the world and accounts for nearly 95 per cent of family income in the Arid and Semi-Arid Lands (ASALs) in Kenya. Despite high economic importance of livestock, inadequate nutrition results to low livestock productivity in Kenya. Ruminant feeding in the ASALs is mainly based on the exploitation of range feed resources. Grazing ruminants forage on different plant species with varying leve...
Effect of transportation on welfare of indigenous chicken in Machakos County, Kenya
Abstract: The aim of this study was to investigate effect of transportation on welfare of indigenous chicken. A sample of 8 hens were randomly selected from the target population. Each treatment had an equal number of mature indigenous chicken hens weighing between 1.25 and 2.4 kg. The first batch of 4 birds were tied together and loaded on to an open vehicle roof top. The second batch of 4 birds was loaded into traditional transport cages and the cage loaded on top of the transport vehicle....
Effects of spacing and Negarim micro catchment on the growth of two provenances of Moringa (Moringa oleifera) in Kitui county, south eastern Kenya
Abstract: There has been an increased human population in arid and semi-arid areas. However, these areas are characterized by harsh climatic conditions hence low agricultural productivity, environmental degradation and over exploitation of natural resources. There's need to understand the best agronomic crop requirements for high value trees and shrubs like Moringa oleifera (Lamark) through climate smart agriculture. Limited studies on Moringa oleifera provenance trials, use of micro-catchme...
Comparative differences of whitefly-transmitted diseases between local and hybrid bean varieties in Kitui County, Kenya
Abstract: The typical dry bean, Phaseolus vulgaris, is the essential food legume for direct human consumption. They play a significant role in food security and nutrition. Despite their nutritional importance, its production growth rates have declined in Kenya due to diseases, insect pests, plant nutritional deficiencies, and drought. Therefore, this study's main objective was to determine whether there are differences in bean varieties' tolerance to whitefly transmitted viral diseases. The ...
Factors influencing smallholder dairy cattle productivity in Tigania East sub-county, Meru County
Abstract: Dairy farming complements both food and cash crop farming in Kenya. Due to limited land sizes, smallholder dairy farming is popular and dominates the dairy sector in terms of milk production. Low milk production is a major constraint in Tigania East SubCounty despite the fact that the surrounding Sub-Counties produce high amounts of milk. A survey was carried out in Tigania East Sub-County involving 156 smallholder farmers randomly selected across three agro-ecological zones (AEZ1,...
Assessment of rain water harvesting technologies for improved food security in Kauwi sub-location, Kitui County
Abstract: Water is an essential natural resource, vital for any development to take place. However, not more than one percent of the water is freely available for human needs including agricultural production in the entire world. Arid and semi-arid lands globally are facing water scarcity challenges. Rain- fed agricultural system is the major farming method in these areas, but this has been challenged greatly by aridity and climatic uncertainty. Kitui County is an ASAL where farmers are expe...
Women participation in agroforestry technologies enhances climate change adaptation in Nguumo and Makindu locations, Makindu sub county, Makueni county, Kenya
Abstract: Agroforestry presents a promising option to sustainable agricultural productivity by providing a buffer to climate variability through permanent tree cover and varied ecological niches. Thus, agroforestry can be used as a strategy to adapt to climate change and variability challenges for smallholder farmers. Success of this strategy in adapting to climate change calls for active participation of men and women in agroforestry technologies. This study aimed to establish roles women p...
Identification and validation of African indigenous knowledge practices on management of crop pests in Kitui West sub-county
Abstract: Agriculture in Sub-Saharan Africa is predominantly subsistence and perennial food deficits, cyclic famines characterize it, and poverty is prompted largely by erratic rainfall patterns, declining soil fertility, and pests and diseases. In Kitui County, farmers are largely small-scale and face various challenges: from poor soil fertility to erratic rainfall. The farmers, too, have not been spared by the pest menace. Consequently, they have resorted to unwarranted and unregulated app...
Factors influencing adoption of giant bamboo (dendrocalamus asper {Schult} Backer) for agroforestry in selected subcounties of Nyandarua county, Kenya
Abstract: Giant bamboo (Dendrocalamus asper) is a very fast growing plant in the family of Poaceae (Gramineae) with great potential in environmental conservation and poverty alleviation. It has over 1500 documented uses. However, with the reducing sizes and productivity of land in Kenya especially in high potential areas where bamboo is grown, there is a need to identify options of cultivating bamboo as an agroforestry crop. Therefore, the main objective of the study was to establish factors...
Growth, nodulation and yield of selected legumes under drought conditions in Kitui county, Kenya
Abstract: Loss of fertility in soil is the main limiting factor that affects production of crops in Kenya, especially in the Arid and Semi-arid regions. In the lower parts of Eastern Kenya, unreliable and low rainfall has led to the low yields in crop production. Lack of the use of commercial fertilizers is also a contributing factor to low crop yields. Amongst new solutions that can assist farmers facing this challenge of low yields includes the emerged potential role of rhizobia in crop pe...
Assessment of fish farmer’s vulnerability to climate variability and extreme climate events in selected parts of Kitui county, Kenya
Abstract: Fish farmers are a vulnerable group to climate variability and extreme climate events effects as their production heavily relies on precipitation and temperature. However, previous studies on vulnerability of fish farmers to climate variability and extreme events have been done on global, regional and national scales, thus failing to capture the local realities on spatial variability. The current study was carried out to assess the householdlevel vulnerability of fish farmers to cl...
Evaluation of farmers’ vulnerability to climate variability and extreme events in selected agro-ecological zones in Kitui county, Kenya
Abstract: Climate variability and extreme events are some of the most pressing environmental challenges occurring in the contemporary world. Farming communities in Sub-Saharan Africa, particularly in Kenya are more vulnerable to climate variability and extreme events due to high dependence on weather patterns in their farming activities. There is little understanding of the vulnerability to climate variability and extreme events among farmers in Kitui County based on the agro-ecological zone...
Adoption of modern dairy technologies and its impact on milk production in Nzaui sub-county, Makueni county
Abstract: Dairy production is a biologically efficient system that converts large quantities of roughage in the tropics to milk. Milk production levels are determined by the levels of technologies applied to the dairy enterprise. However, information on levels of adoption of dairy technologies especially in the arid and semi-arid areas (ASALs) of Kenya is scanty. This study thus sought to evaluate the extent of adoption of modern dairy technologies and its impact on milk production in Nzaui ...
An evaluation of the use of a microcomputer on a livestock farm
Abstract: The purpose of this study was to investigate the potential of a microcomputer as a tool of information handling. Specifically, this involved developing software packages for use on an Apple II Plus microcomputer. The Willow Bend Demonstrational Farm in Union, West Virginia, was chosen. A farm records and budgets model was developed. Receipts and expenses from the Electronic Farm Accounting records were used to test the model for use by the farm operators in West Virginia. Enterpris...
Agriculture is the cultivation of land and breeding of animals (livestock), plants and fungi to produce food, feed, fiber and many other desired products to sustain and enhance life. The study of agriculture can lead to a variety of careers, including those associated with consulting, farming, management and research. Afribary publishes latest agriculture topics for students. Browse through Agriculture projects, agriculture project topics, Agriculture thesis, seminars, research papers etc. All papers and research works in agriculture and its sub-fields.
Privacy Policy | Refund Policy | Terms | Copyright | © 2024, Afribary Limited. All rights reserved.

More efficient bioethanol production might be possible using persimmon tannin to help yeast thrive
Naturally derived antioxidants improve growth of yeast strain in presence of ethanol.
While ethanol in alcoholic beverages impairs drinkers' motor functions, it is that same substance that can power motor vehicles in a cleaner, more sustainable manner. What is necessary for the production of ethanol is yeast, but ethanol is among the environmental factors that add stress to yeasts, hindering their growth. To promote efficient bioethanol production, scientists have been searching for substances that can help yeasts better withstand ethanol, but few effective ones have been found.
An Osaka Metropolitan University research team, including graduate student Ilhamzah and Professor Ken-ichi Fujita of the Graduate School of Science and Professor Akira Ogita of the Research Center for Urban Health and Sports, has found that tannin from persimmons improves the growth of the yeast strain Saccharomyces cerevisiae in the presence of ethanol.
"In this study, yeast cultures grown in a medium containing ethanol and persimmon tannin showed an 8.9-fold increase in cell number compared to cultures grown in an ethanol medium without persimmon tannin," stated Professor Fujita.
The researchers explored persimmon tannin because it is known for its antioxidative properties.
"Persimmon tannin reduced ethanol-induced oxidative stress," Fujita added. "However, persimmon tannin did not prevent ethanol-induced cell membrane damage. This indicates the potential of persimmon tannin as a protective agent to enhance the yeast's tolerance to ethanol stress by limiting oxidative damage, rather than limiting damage to the yeast's cell membranes."
The findings were published inthe Journal of the Science of Food and Agriculture .
- Agriculture and Food
- Microbiology
- Alternative Fuels
- Energy Technology
- Renewable Energy
- Energy and the Environment
- Sustainability
- Polyphenol antioxidant
- Ethanol fuel
- Common ethanol fuel mixtures
- Hydroponics
- Alternative fuel vehicle
Story Source:
Materials provided by Osaka Metropolitan University . Note: Content may be edited for style and length.
Journal Reference :
- Ilhamzah, Yuka Tsukuda, Yoshihiro Yamaguchi, Akira Ogita, Ken‐ichi Fujita. Persimmon tannin promotes the growth of Saccharomyces cerevisiae under ethanol stress . Journal of the Science of Food and Agriculture , 2024; DOI: 10.1002/jsfa.13439
Cite This Page :
Explore More
- Charge Your Laptop in a Minute?
- Caterpillars Detect Predators by Electricity
- 'Electronic Spider Silk' Printed On Human Skin
- Engineered Surfaces Made to Shed Heat
- Innovative Material for Sustainable Building
- Human Brain: New Gene Transcripts
- Epstein-Barr Virus and Resulting Diseases
- Origins of the Proton's Spin
- Symbiotic Bacteria Communicate With Plants
- Birdsong and Human Voice: Same Genetic Blueprint
Trending Topics
Strange & offbeat.
- Frontiers in Plant Science
- Sustainable and Intelligent Phytoprotection
- Research Topics
Advanced Methods, Equipment and Platforms in Precision Field Crops Protection, Volume II
Total Downloads
Total Views and Downloads
About this Research Topic
Diseases, pests and weeds have severely affected the production of crops. Traditional agriculture solves the above problems by large-scale chemical spraying, but excessive pesticides can also seriously affect the growth of crops and pollute the environment. Therefore, it is necessary to use modern technologies to protect field crops accurately. Collect and analyze the characteristics and location information of diseases, pests and weeds as early as possible with equipment and methods, and use various platforms to complete the precise protection of field crops. This Reaserch Topic welcomes submissions that address precision crop protection research and demonstrate how smart control strategies, sensor technology, and operational platforms can be integrated into actual crop protection. We welcome submissions of original research articles, reviews, and methods, including (but not limited to) research on the following sub-themes: 1. Real-time monitoring and detection sensors for diseases, pests and weeds; 2. Intelligent crop protection methods based on artificial intelligence; 3. Phenotypic parameter analysis of weeds and affected crops; 4. Precision variable-rate equipment and system for crop protection; 5. Digital twin models, novel IoT technologies and cloud platform for crop protection; 6. Advances, innovations, and new trends in modern technologies for crop protection and development.
Keywords : Crop Protection, Agricultural Sensors, Variable-rate Control, Phenotypic Parameter Analysis, Intelligent Perception
Important Note : All contributions to this Research Topic must be within the scope of the section and journal to which they are submitted, as defined in their mission statements. Frontiers reserves the right to guide an out-of-scope manuscript to a more suitable section or journal at any stage of peer review.
Topic Editors
Topic coordinators, submission deadlines, participating journals.
Manuscripts can be submitted to this Research Topic via the following journals:
total views
- Demographics
No records found
total views article views downloads topic views
Top countries
Top referring sites, about frontiers research topics.
With their unique mixes of varied contributions from Original Research to Review Articles, Research Topics unify the most influential researchers, the latest key findings and historical advances in a hot research area! Find out more on how to host your own Frontiers Research Topic or contribute to one as an author.
share this!
May 23, 2024
This article has been reviewed according to Science X's editorial process and policies . Editors have highlighted the following attributes while ensuring the content's credibility:
fact-checked
peer-reviewed publication
trusted source
Climate change will reduce streamflow in the upper Colorado river basin as groundwater levels fall, study finds
by Desert Research Institute
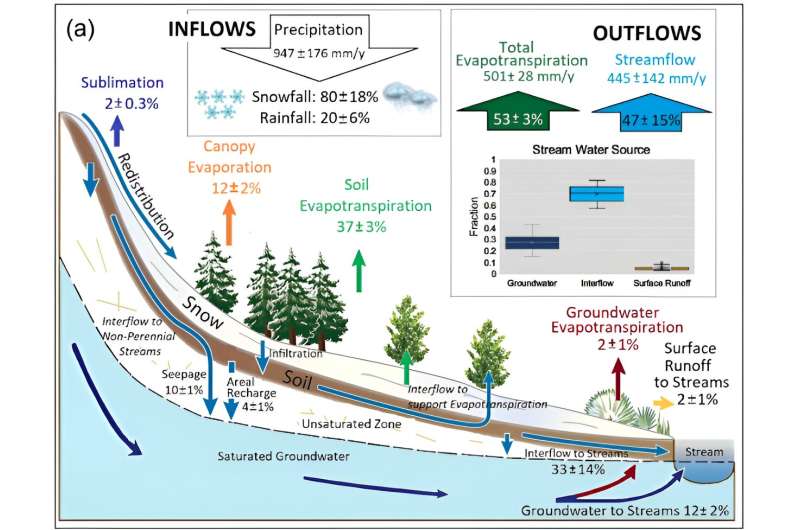
The Colorado River makes life possible in many Western cities and supports agriculture that sustains people throughout the country. Most of the river's water begins as snowmelt from the mountainous watersheds of Colorado, Utah, and Wyoming, and a warming climate will drastically reduce these streamflows, new research finds.
Researchers from Desert Research Institute (DRI), USGS, and Lawrence Berkeley National Laboratory teamed up for the new study , published May 23 in Nature Water .
By applying warming to historical conditions for the East River in Colorado and using computer simulations to observe the impact on streamflow and groundwater levels , the scientists found that groundwater storage would fall to the lowest known levels after the first extremely dry year and fail to recover even after multiple wet periods. When groundwater levels fall, streamflows are drawn into the water table instead of contributing to Colorado River flows.
"We found that groundwater matters a lot," says Rosemary Carroll, Ph.D., DRI research professor of hydrology and lead author of the study. "Even with historically observed wet periods in the model, the groundwater can't come back from a single dry water year under end-of-century warming."
The Colorado River has been in a drought state for decades, creating tensions around water rights throughout the Southwest. Scientists have been perplexed by falling river levels even in relatively wet years—in 2021, the Upper Colorado River Basin reached 80% normal snowpack but delivered only 30% of average streamflow to the river.
The study authors wanted to examine how warming in mountain basins may be contributing to this phenomenon. Increased temperatures alter the balance between snowfall and water availability in a number of ways, including more snow evaporation (known as sublimation), more precipitation falling as rain instead of snow, and more frequent melting throughout the winter.
Past research has largely overlooked the role of groundwater and how it may buffer, or intensify, streamflow loss from climate change.
"I'm very interested in the relationship of groundwater contributions to streamflow—that has been a running theme in my research for a long time," Carroll says. "A lot of studies indicate that in the short term, groundwater release to streamflow will help buffer drought impacts, but before this study, we didn't have any knowledge on what that would mean over the long haul."
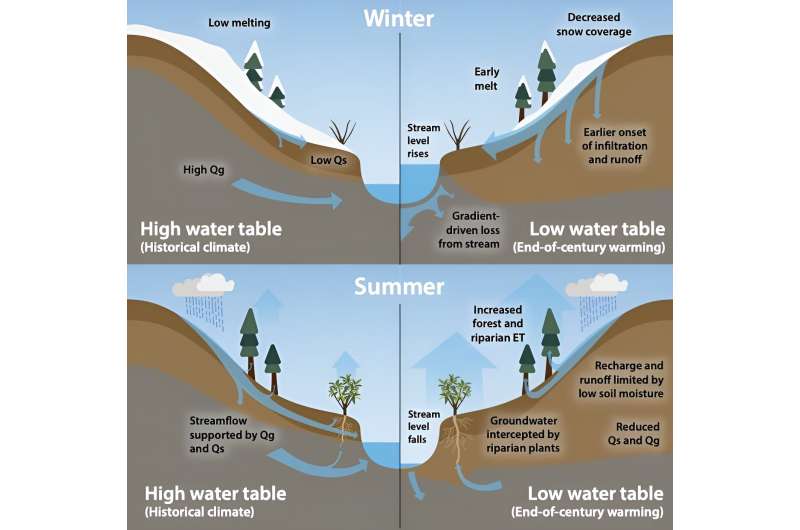
The study authors used the East River as the focus for the research because it is representative of the headwaters of the Colorado River, as well as the significant weather and water monitoring infrastructure available. Ground observations and airborne mapping measure snowpack depth and density, streamflow, plant cover, and groundwater levels, among many other measurements throughout the watershed.
Historical data from 1987 to 2022 was used to create the computer model. The research team then applied 4 degrees Celsius of warming to this time period in two ways: for one simulation, warming was applied constantly across time, while in subsequent simulations, warming was applied to each season independently.
The approach allowed the scientists to examine the differing impacts of warming between seasons. Four degrees Celsius is representative of the projected climate at the end of the century based on observed warming of approximately 0.4 degrees Celsius per decade in the watershed. The simulation doesn't account for likely changes in vegetation over the same timeframe.
The study showed that consistently warmer temperatures resulted in sharp declines in groundwater levels that were unable to recover to historical average levels during wet periods. Isolating the warming seasonally demonstrated the strong impact of warmer summers on water table declines, as the atmosphere increases evaporation rates, plants increase their water uptake, and soils dry out. The largest declines in water table elevations occur in the subalpine forests where conifer forests are most dense.
"As the groundwater level drops, you lose more streamflow to the water table," Carroll says. "When precipitation is low, the East River stops flowing for a portion of the summer. Of course, this would have dramatic effects on ecological health and agricultural irrigation."
By including declining water table levels in the analysis, the study found that streamflow reductions nearly double when compared to simulations that examined the impacts of climate change in the region without accounting for groundwater declines. This is because of the multiple impacts occurring simultaneously: less water flows to streams from the aquifers, while more stream water drains into the soil.
The research demonstrates the need to jointly manage forest and groundwater in the Upper Colorado River Basin, as well as account for the exchange of surface and groundwater in mountain basins, to minimize streamflow declines under climate change, Carroll says.
"I think of groundwater as your savings account," she adds. "Snowpack is like your checking account; it changes from year to year. Groundwater is a longer-term investment—it can smooth out the really wet and dry years. But if you start consistently reducing that groundwater year after year, then you can no longer modulate those extremes."
Journal information: Nature Water
Provided by Desert Research Institute
Explore further
Feedback to editors

NASA launches ground-breaking climate change satellite
8 hours ago

Dyson spheres: Astronomers report potential candidates for alien structures, and evidence against their existence
10 hours ago

You leave a 'microbe fingerprint' on every piece of clothing you wear—and it could help forensic scientists solve crimes
13 hours ago

Saturday Citations: The cheapness horizon of electric batteries; the battle-worthiness of ancient armor; scared animals
16 hours ago

Cosmic leap: NASA Swift satellite and AI unravel the distance of the farthest gamma-ray bursts
19 hours ago

Scientists discover CO₂ and CO ices in outskirts of solar system

Charge your laptop in a minute? Supercapacitors can help; new research offers clues

New study discovers tiny target on RNA to short-circuit inflammation

Researchers develop organic photoredox catalysts with enhanced stability and recyclability
May 24, 2024

Theory and experiment combine to shine a new light on proton spin
Relevant physicsforums posts, adirondack mountains and earthquakes, the secrets of prof. verschure's rosetta stones, mt. vesuvius 1944 eruption light show -- static electricity.
May 22, 2024
Can a glass of water be filled to its edge?
May 21, 2024
Pyramids built along dry river bed
May 18, 2024
A very puzzling rock or a pallasite / mesmosiderite or a nothing burger
May 8, 2024
More from Earth Sciences
Related Stories

Researching the spread of drought and its potential negative impacts
Mar 25, 2024

As climate warms, summer monsoons to produce less streamflow
Feb 2, 2021

Precipitation may brighten Colorado River's future, says modeling study
May 1, 2024

New research predicts peak groundwater extraction for key basins around the globe
Apr 25, 2024

Climate change is already impacting stream flows across the US
Apr 27, 2023

Study: Increase in cannabis cultivation or residential development could impact water resources
Nov 22, 2019
Recommended for you

Heavy water: How melting ice sheets and pumped groundwater can lower local sea levels—and boost them elsewhere

Ambitious targets are needed to end ocean plastic pollution by 2100, analysis finds

Drought in the Brazil's Cerrado is the worst for at least seven centuries, study shows

Study: While most of the world trusts climate scientists, a skeptical minority can lead to climate inaction

Dangerous brew: Ocean heat and La Nina combo likely mean more Atlantic hurricanes this summer
Let us know if there is a problem with our content.
Use this form if you have come across a typo, inaccuracy or would like to send an edit request for the content on this page. For general inquiries, please use our contact form . For general feedback, use the public comments section below (please adhere to guidelines ).
Please select the most appropriate category to facilitate processing of your request
Thank you for taking time to provide your feedback to the editors.
Your feedback is important to us. However, we do not guarantee individual replies due to the high volume of messages.
E-mail the story
Your email address is used only to let the recipient know who sent the email. Neither your address nor the recipient's address will be used for any other purpose. The information you enter will appear in your e-mail message and is not retained by Phys.org in any form.
Newsletter sign up
Get weekly and/or daily updates delivered to your inbox. You can unsubscribe at any time and we'll never share your details to third parties.
More information Privacy policy
Donate and enjoy an ad-free experience
We keep our content available to everyone. Consider supporting Science X's mission by getting a premium account.
E-mail newsletter
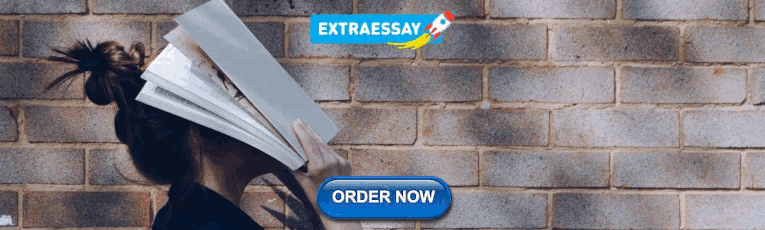
IMAGES
VIDEO
COMMENTS
Analysing the impact of fish farming on agriculture: A case study of Japan. Smart farming in Germany: The impact of using drones in crop management. Comparing the farming regulations in California and Texas. Economics of pig farming for country farmers in the United States.
In this article, we will present 45 research project ideas in agriculture that can help address some of the most pressing issues facing the industry today. These research projects cover a wide range of topics, from soil health and crop yields to livestock farming, aquaculture, and food systems, providing a comprehensive overview of the latest ...
Agriculture is the cultivation of plants, animals, and some other organisms, such as fungi, for the production of food, fibre, fuel, and medicines used by society. A sweet gel from the endocarp of ...
A previous paper about the top 100 questions of importance to the future of global agriculture was published almost a decade ago, with contributors primarily comprising experts and representatives from agricultural organizations. 4 Our collection was intended for a broad community, including scientists, engineers, farmers, entrepreneurs ...
Modeling and Artificial Intelligence (AI) in Pest Management. José Bruno Malaquias. Michael Caprio. 565 views. This exciting journal investigates how agronomy will develop in the 21st century as we address climate change, focus on food systems and find ways to produce enough, waste less, and recycle more.
Abstract. Restructuring farmer-researcher relationships and addressing complexity and uncertainty through joint exploration are at the heart of On-Farm Experimentation (OFE). OFE describes new ...
Topics. NIFA supports research, educational, and extension efforts in a wide range of scientific fields related to agricultural and behavioral sciences. In all of these areas, you will find NIFA working in pursuit of our vision. To address contemporary agricultural challenges, we seek to catalyze transformative discoveries and enhance education ...
1,795 Research Topics Guest edit your own article collection Suggest a topic. Submission. null. Submission ... Exploring Agricultural Efforts and Development of Bio Inputs for Crop Management To Reduce Greenhouse Gas Emissions. Virgilio Gavicho Uarrota; Marcelo Maraschin;
It identifies the most promising scientific breakthroughs that could have the greatest positive impact on food and agriculture, and that are possible to achieve in the next decade (by 2030). ... Topics. Agriculture — Policy, ... Science Breakthroughs to Advance Food and Agricultural Research by 2030. Washington, DC: The National Academies ...
This paper presents a comprehensive review of emerging technologies for the internet of things (IoT)-based smart agriculture. We begin by summarizing the existing surveys and describing emergent technologies for the agricultural IoT, such as unmanned aerial vehicles, wireless technologies, open-source IoT platforms, software defined networking (SDN), network function virtualization (NFV ...
See our list of agriculture research paper topics. The development of agriculture—the raising of crops and animals for food—has been fundamental to the development of civilization. Farming brought about the settlement of farm communities, which grew into towns and city-states. Farming also made possible sedentary (settled) lifestyles, which ...
Robotics in agriculture explores the potential of robotics and artificial intelligence to revolutionize the way farming is done. It looks at the possibilities for automation in crop production and livestock farming, as well as the implications for farming and rural communities. It examines the ways in which robotics could reduce costs, increase yields, and improve safety and sustainability. It ...
Natural Resources, Conservation, and Environment. Topics relating to the environment, including, weather and climate change, conservation practices, environmental justice, invasive species and soil.
This research area examines the potential effects of climate change on regional and global food security, and how to adapt agriculture to a warmer world. Research topics include: past and future effects of climate variability and climate change on agricultural productivity; assessments of gains from different possible adaptation strategies ...
2 answers. Jul 2, 2023. 1- "I, Dr. Amir Afzal, am currently affiliated with the prestigious Barani Agricultural Research Institute in Chakwal, Pakistan. My published works primarily focus on the ...
5 Key Issues in Agriculture in 2021. As 2021 ends, we take a retrospective look at five topics that were covered in our analytical work this year. These issues represent just a fragment of the Bank's work, but they are key to reducing poverty and hunger while slowing climate change. Food Security.
1.1. General Context of Machine Learning in Agriculture. Modern agriculture has to cope with several challenges, including the increasing call for food, as a consequence of the global explosion of earth's population, climate changes [], natural resources depletion [], alteration of dietary choices [], as well as safety and health concerns [].As a means of addressing the above issues, placing ...
In light of this, this Special Issue will comprise original research, reviews, and case studies outlining recent progress and exploring scenarios in the abovementioned research areas. Potential topics include, but are not limited to: aquaponics, hydroponic and aeroponic systems; agricultural automation and farm machinery; agricultural robotics ...
Such agricultural research paper topics allow revealing the topic of fishery and agricultural procurement. Students can concentrate on many aspects of the payback of farms and fisheries. The topics are quite extensive, and you can find a lot of research on the Internet for choosing trust sources. Trout breeding in freshwaters.
This Research Topic will help to (1) identify factors responsible for environmental risks of agricultural land use and evaluate its impacts; (2) highlight the costs and risks associated with policies and solutions to environmental problems; and (3) provide examples, concepts, and pathways of sustainable agricultural land-use transition.
StudyCorgi has prepared a list of important agriculture research topics. On this page, any student can find essay questions and project ideas on various agricultural issues, such as food safety, genetically engineered crops, and sustainable farming practices. ... This paper tackles the issue of existing challenges and possible solutions to the ...
C.O.R.N. Newsletter is a summary of crop observations, related information, and appropriate recommendations for Ohio crop producers and industry. C.O.R.N. Newsletter is produced by the Ohio State University Extension Agronomy Team, state specialists at The Ohio State University and the Ohio Agricultural Research and Development Center (OARDC).
The four major international organizations related to agriculture and environment (i.e., WHO—World Health Organization, FAO—Food and Agriculture Organization, UNEP—UN Environment Programme, and WOAH—World Organisation for Animal Health, have launched the "One Health" 2022-2026 action plan [] to preserve the health of people, animals, plants and the environment.
The study of agriculture can lead to a variety of careers, including those associated with consulting, farming, management and research. Afribary publishes latest agriculture topics for students. Browse through Agriculture projects, agriculture project topics, Agriculture thesis, seminars, research papers etc.
Twenty agricultural education experts identified research topics and categories, which were then rated by 34 research experts (92 percent) and 49 department heads (79 percent). Highest ratings went to biotechnology , high technology, and agribusiness; agricultural education curriculum; and long-term impact and cost effectiveness of agricultural…
More efficient bioethanol production might be possible using persimmon tannin to help yeast thrive. ScienceDaily . Retrieved May 22, 2024 from www.sciencedaily.com / releases / 2024 / 05 ...
Diseases, pests and weeds have severely affected the production of crops. Traditional agriculture solves the above problems by large-scale chemical spraying, but excessive pesticides can also seriously affect the growth of crops and pollute the environment. Therefore, it is necessary to use modern technologies to protect field crops accurately. Collect and analyze the characteristics and ...
The Colorado River makes life possible in many Western cities and supports agriculture that sustains people throughout the country. Most of the river's water begins as snowmelt from the ...