Scientific Research and Methodology : An introduction to quantitative research and statistics
10 collecting data.
So far, you have learnt to ask a RQ and design the study. In this chapter , you will learn how to:
- record the important steps in data collection.
- describe study protocols.
- ask survey questions.

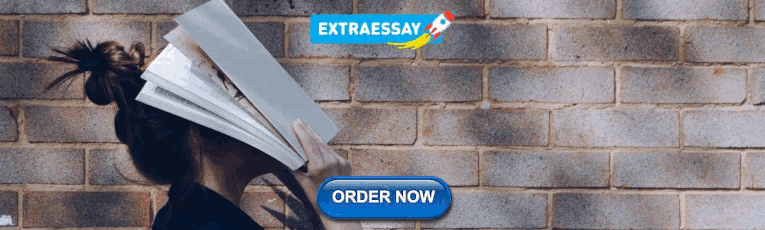
10.1 Protocols
If the RQ is well-constructed, terms are clearly defined, and the study is well designed and explained, then the process for collecting the data should be easy to describe. Data collection is often time-consuming, tedious and expensive, so collecting the data correctly first time is important.
Before collecting the data, a plan should be established and documented that explains exactly how the data will be obtained, which will include operational definitions (Sect. 2.10 ). This plan is called a protocol .
Definition 10.1 (Protocol) A protocol is a procedure documenting the details of the design and implementation of studies, and for data collection.
Unforeseen complications are not unusual, so often a pilot study (or a practice run ) is conducted before the real data collection, to:
- determine the feasibility of the data collection protocol.
- identify unforeseen challenges.
- obtain data to determine appropriate sample sizes (Sect. 30 ).
- potentially save time and money.
The pilot study may suggest changes to the protocol.
Definition 10.2 (Pilot study) A pilot study is a small test run of the study protocol used to check that the protocol is appropriate and practical, and to identify (and hence fix) possible problems with the research design or protocol.
A pilot study allows the researcher

The data can be collected once the protocol has been finalised. Protocols ensure studies are repeatable (Sect. 4.3 ) so others can confirm or compare results, and others can understand exactly what was done, and how. Protocols should indicate how design aspects (such as blinding the individuals, random allocation of treatments, etc.) will happen. The final protocol , without pedantic detail, should be reported. Diagrams can be useful to support explanations. All studies should have a well-established protocol for describing how the study was done.
A protocol usually has at least three components that describe:
- how individuals are chosen from the population (i.e., external validity).
- how information is collected from the individuals (i.e., internal validity).
- the analyses and software (including version) used.
Example 10.1 (Protocol) Romanchik-Cerpovicz, Jeffords, and Onyenwoke ( 2018 ) made cookies using pureed green peas in place of margarine (to increase the nutritional value of cookies). They assessed the acceptance of these cookies to college students.
The protocol discussed how the individuals were chosen (p. 4):
...through advertisement across campus from students attending a university in the southeastern United States.
This voluntary sample comprised \(80.6\) % women, a higher percentage of women than in the general population, or the college population. (Other extraneous variables were also recorded.)
Exclusion criteria were also applied, excluding people "with an allergy or sensitivity to an ingredient used in the preparation of the cookies" (p. 5). The researchers also described how the data was obtained (p. 5):
During the testing session, panelists were seated at individual tables. Each cookie was presented one at a time on a disposable white plate. Samples were previously coded and randomized. The presentation order for all samples was \(25\) %, \(0\) %, \(50\) %, \(100\) % and \(75\) % substitution of fat with puree of canned green peas. To maintain standard procedures for sensory analysis [...], panelists cleansed their palates between cookie samples with distilled water ( \(25^\circ\) C) [...] characteristics of color, smell, moistness, flavor, aftertaste, and overall acceptability, for each sample of cookies [was recorded]...
Thus, internal validity was managed using random allocation, blinding individuals, and washouts. Details are also given of how the cookies were prepared, and how objective measurements (such as moisture content) were determined.
The analyses and software used were also given.
Consider this partial protocol, which shows honesty in describing a protocol:
Fresh cow dung was obtained from free-ranging, grass fed, and antibiotic-free Milking Shorthorn cows ( Bos taurus ) in the Tilden Regional Park in Berkeley, CA. Resting cows were approached with caution and startled by loud shouting, whereupon the cows rapidly stood up, defecated, and moved away from the source of the annoyance. Dung was collected in ZipLoc bags ( \(1\) gallon), snap-frozen and stored at \(-80\) C. --- Hare et al. ( 2008 ) , p. 10
10.2 Collecting data using questionnaires
10.2.1 writing questions.
Collecting data using questionnaires is common for both observational and experimental studies. Questionnaires are very difficult to do well: question wording is crucial, and surprisingly difficult to get right ( Fink 1995 ) . Pilot testing questionnaires is crucial!
Definition 10.3 (Questionnaire) A questionnaire is a set of questions for respondents to answer.
A questionnaire is a set of question to obtain information from individuals. A survey is an entire methodology, that includes gathering data using a questionnaire, finding a sample, and other components.
Questions in a questionnaire may be open-ended (respondents can write their own answers) or closed (respondents select from a small number of possible answers, as in multiple-choice questions). Open and closed questions both have advantages and disadvantages. Answers to open questions more easily lend themselves to qualitative analysis. This section briefly discusses writing questions.
Example 10.2 (Open and closed questions) Raab and Bogner ( 2021 ) asked German students a series of questions about microplastics, including:
- Name sources of microplastics in the household.
- In which ecosystems are microplastics in Germany? Tick the answer (multiple ticks are possible). Options : (a) sea; (b) rivers; (c) lakes; (d) groundwater.
- Assess the potential danger posed by microplastics. Options : (a) very dangerous; (b) dangerous; (c) hardly dangerous; (d) not dangerous.
The first question is an open : respondents could provide their own answers. The second question is closed , where multiple options can be selected. The third question is closed , where only one option can be selected
Important advice for writing questionnaire questions include:
- Avoid leading questions , which may lead respondents to answer a certain way. Imprecise question wording is the usual reason for leading questions.
- Avoid ambiguity : avoid unfamiliar terms and unclear questions.
- Avoid asking the uninformed : avoid asking respondents about issues they don't know about. Many people will give a response even if they do not understand (such responses are worthless). For example, people may give directions to places that do not even exist ( Collett and O’Shea 1976 ) .
- Avoid complex and double-barrelled questions , which are hard to understand.
- Avoid problems with ethics : avoid questions about people breaking laws, or revealing confidential or private information. In special cases and with justification, ethics committees may allow such questions.
- Ensure clarity in question wording.
- Ensure options are mutually exhaustive , so answers fit into only one category.
- Ensure options are exhaustive , so that the categories cover all options.
Example 10.3 (Poor question wording) Consider a questionnaire asking these questions:
- Because bottles from bottled water create enormous amounts of non-biodegradable landfill and hence threaten native wildlife, do you support banning bottled water?
- Do you drink more water now?
- Are you more concerned about Coagulase-negative Staphylococcus or Neisseria pharyngis in bottled water?
- Do you drink water in plastic and glass bottles?
- Do you have a water tank installed illegally, without permission?
- Do you avoid purchasing water in plastic bottles unless it is carbonated, unless the bottles are plastic but not necessarily if the lid is recyclable?
Question 1 is leading because the expected response is obvious.
Question 2 is ambiguous : it is unclear what 'more water now' is being compared to.
Question 3 is unlikely to give sensible answers, as most people will be uninformed . Many people will still give an opinion, but the data will be effectively useless (though the researcher may not realise).
Question 4 is double-barrelled , and would be better asked as two separate questions (one asking about plastic bottles, and one about glass bottles).
Question 5 is unlikely to be given ethical approval or to obtain truthful answers, as respondents are unlikely to admit to breaking rules.
Question 6 is unclear , since knowing what a yes or no answer means is confusing.
Example 10.4 (Question wording) Question wording can be important. In the 2014 General Social Survey ( https://gss.norc.org ), when white Americans were asked for their opinion of the amount America spends on welfare , \(58\) % of respondents answered 'Too much' ( Jardina 2018 ) .
However, when white Americans were asked for their opinion of the amount America spends on assistance to the poor , only \(16\) % of respondents answered 'Too much'.
Example 10.5 (Leading question) Consider this question:
Do you like this new orthotic?
This question is leading , since liking is the only option presented. Better would be:
Do you like or dislike this new orthotic?
Example 10.6 (Mutually exclusive options) In a study to determine the time doctors spent on patients (from Chan et al. ( 2008 ) ), doctors were given the options:
- \(0\) -- \(5\) mins;
- \(5\) -- \(10\) mins; or
- more than \(10\) mins.
This is a poor question, because a respondent does not know which option to select for an answer of ' \(5\) minutes'. The options are not mutually exclusive .
The following (humourous) video shows how questions can be manipulated by those not wanting to be ethical:
10.2.2 Challenges using questionnaires
Using questionnaires presents myriad challenges.
- Non-response bias (Sect. 5.11 ): Non-response bias is common with questionnaires, as they are often used with voluntary-response samples. The people who do not respond to the survey may be different than those who do respond.
- Response bias (Sect. 5.11 ): People do not always answer truthfully; for example, what people say may not correspond with what people do (Example 9.6 ). Sometimes this is unintentional (e.g., poor questions wording), due to embarrassment or because questions are controversial. Sometimes, respondents repeatedly provide the same answer to a series of multichoice questions.
- Recall bias : People may not be able to accurately recall past events clearly, or recall when they happened.
- Question order : The order of the questions can influence the responses.
- Interpretation : Phrases and words such as 'Sometimes' and 'Somewhat disagree' may mean different things to different people.
Many of these can be managed with careful questionnaire design, but discussing the methods are beyond the scope of this book.
10.3 Chapter summary
Having a detailed procedure for collecting the data (the protocol ) is important. Using a pilot study to trial the protocol an often reveal unexpected changes necessary for a good protocol. Creating good questionnaires questions is difficult, but important.
10.4 Quick review questions
What is the biggest problem with this question: 'Do you have bromodosis?'
What is the biggest problem with this question: 'Do you spend too much time connected to the internet?'
What is the biggest problem with this question: 'Do you eat fruits and vegetables?'
Which of these are reasons for producing a well-defined protocol?
- It allows the researchers to make the study externally valid. TRUE FALSE
- It ensures that others know exactly what was done. TRUE FALSE
- It ensures that the study is repeatable for others. TRUE FALSE
Which of the following questionnaire questions likely to be leading questions?
- Do you, or do you not, believe that permeable pavements are a viable alternative to traditional pavements? TRUE FALSE
- Do you support a ban on bottled water? TRUE FALSE
- Do you believe that double-gloving by paramedics reduces the risk of infection, increases the risk of infection, or makes no difference to the risk of infection? TRUE FALSE
- Should Ireland ban breakfast cereals with unhealthy sugar levels? TRUE FALSE
10.5 Exercises
Answers to odd-numbered exercises are available in App. E .
Exercise 10.1 What is the problem with this question?
What is your age? (Select one option) Under \(18\) Over \(18\)
Exercise 10.2 What is the problem with this question?
How many children do you have? (Select one option) None 1 or 2 2 or 3 More than 4
Exercise 10.3 Which of these questionnaire questions is better? Why?
- Should concerned cat owners vaccinate their pets?
- Should domestic cats be required to be vaccinated or not?
- Do you agree that pet-owners should have their cats vaccinated?
Exercise 10.4 Which of these questionnaire questions is better? Why?
- Do you own an environmentally-friendly electric vehicle?
- Do you own an electric vehicle?
- Do you own or do you not own an electric vehicle?
Exercise 10.5 Falk and Anderson ( 2013 ) studied sunscreen use, and asked participants questions, including these:
- How often do you sun bathe with the intention to tan during the summer in Sweden? (Possible answers: never, seldom, sometimes, often, always).
- How long do you usually stay in the sun between \(11\) am and \(3\) pm, during a typical day-off in the summer (June--August)? (Possible answers: \(<30\) min, \(30\) min-- \(1\) h, \(1\) -- \(2\) h, \(2\) -- \(3\) h, \(>3\) h).
Critique these questions. What biases may be present?
Exercise 10.6 Morón-Monge, Hamed, and Morón Monge ( 2021 ) studied primary-school children's knowledge of their natural environment. They were asked three questions:
- No, I don’t like parks.
- No, I don’t usually visit it.
- Yes, once per week.
- Yes, more than once a week
- Two to three times
- More than three times
- Write a story
- Draw a picture
Which questions are open and which are closed ? Critique the questions.

Write an Error-free Research Protocol As Recommended by WHO: 21 Elements You Shouldn’t Miss!
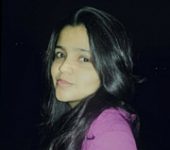
Principal Investigator: Did you draft the research protocol?
Student: Not yet. I have too many questions about it. Why is it important to write a research protocol? Is it similar to research proposal? What should I include in it? How should I structure it? Is there a specific format?
Researchers at an early stage fall short in understanding the purpose and importance of some supplementary documents, let alone how to write them. Let’s better your understanding of writing an acceptance-worthy research protocol.
Table of Contents
What Is Research Protocol?
The research protocol is a document that describes the background, rationale, objective(s), design, methodology, statistical considerations and organization of a clinical trial. It is a document that outlines the clinical research study plan. Furthermore, the research protocol should be designed to provide a satisfactory answer to the research question. The protocol in effect is the cookbook for conducting your study
Why Is Research Protocol Important?
In clinical research, the research protocol is of paramount importance. It forms the basis of a clinical investigation. It ensures the safety of the clinical trial subjects and integrity of the data collected. Serving as a binding document, the research protocol states what you are—and you are not—allowed to study as part of the trial. Furthermore, it is also considered to be the most important document in your application with your Institution’s Review Board (IRB).
It is written with the contributions and inputs from a medical expert, a statistician, pharmacokinetics expert, the clinical research coordinator, and the project manager to ensure all aspects of the study are covered in the final document.
Is Research Protocol Same As Research Proposal?
Often misinterpreted, research protocol is not similar to research proposal. Here are some significant points of difference between a research protocol and a research proposal:
What Are the Elements/Sections of a Research Protocol?
According to Good Clinical Practice guidelines laid by WHO, a research protocol should include the following:
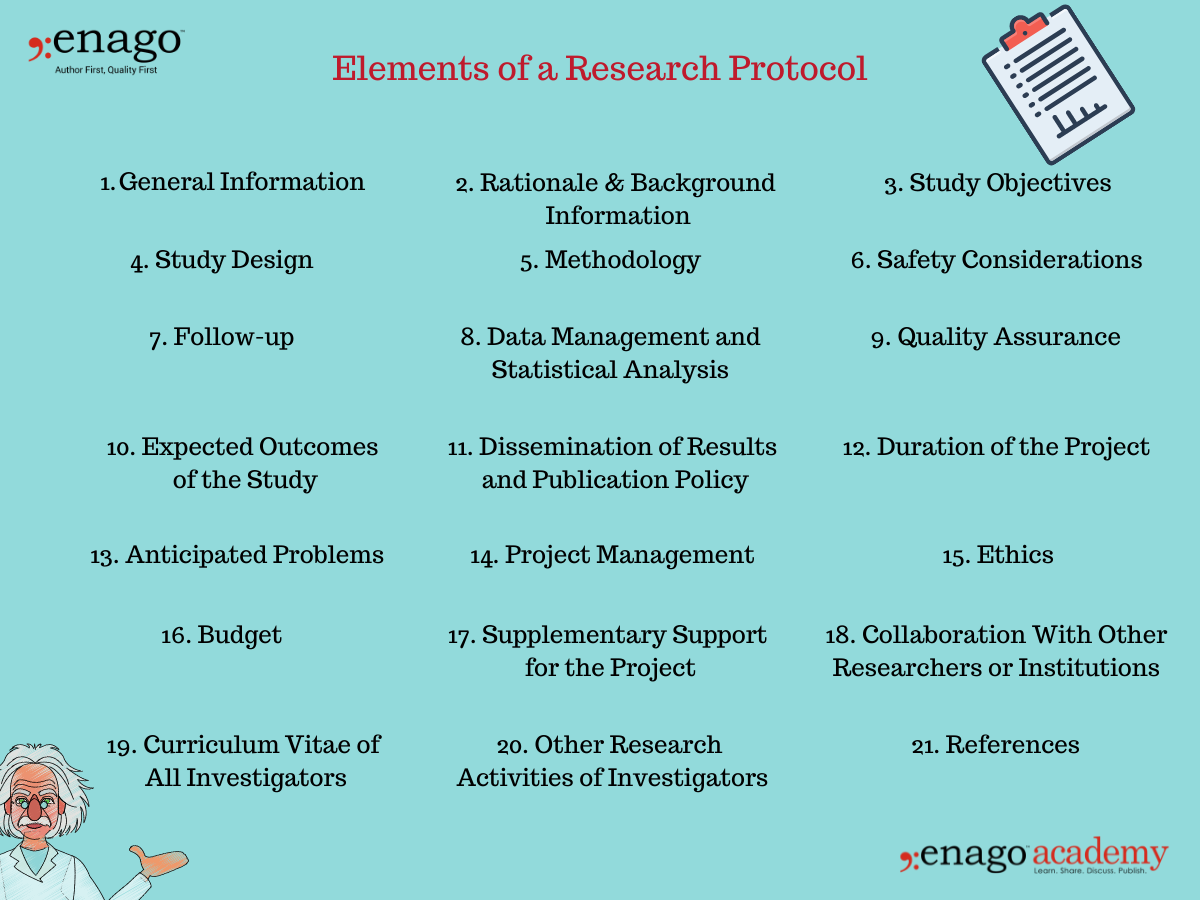
1. General Information
- Protocol title, protocol identifying number (if any), and date.
- Name and address of the funder.
- Name(s) and contact details of the investigator(s) responsible for conducting the research, the research site(s).
- Responsibilities of each investigator.
- Name(s) and address(es) of the clinical laboratory(ies), other medical and/or technical department(s) and/or institutions involved in the research.
2. Rationale & Background Information
- The rationale and background information provides specific reasons for conducting the research in light of pertinent knowledge about the research topic.
- It is a statement that includes the problem that is the basis of the project, the cause of the research problem, and its possible solutions.
- It should be supported with a brief description of the most relevant literatures published on the research topic.
3. Study Objectives
- The study objectives mentioned in the research proposal states what the investigators hope to accomplish. The research is planned based on this section.
- The research proposal objectives should be simple, clear, specific, and stated prior to conducting the research.
- It could be divided into primary and secondary objectives based on their relativity to the research problem and its solution.
4. Study Design
- The study design justifies the scientific integrity and credibility of the research study.
- The study design should include information on the type of study, the research population or the sampling frame, participation criteria (inclusion, exclusion, and withdrawal), and the expected duration of the study.
5. Methodology
- The methodology section is the most critical section of the research protocol.
- It should include detailed information on the interventions to be made, procedures to be used, measurements to be taken, observations to be made, laboratory investigations to be done, etc.
- The methodology should be standardized and clearly defined if multiple sites are engaged in a specified protocol.
6. Safety Considerations
- The safety of participants is a top-tier priority while conducting clinical research .
- Safety aspects of the research should be scrutinized and provided in the research protocol.
7. Follow-up
- The research protocol clearly indicate of what follow up will be provided to the participating subjects.
- It must also include the duration of the follow-up.
8. Data Management and Statistical Analysis
- The research protocol should include information on how the data will be managed, including data handling and coding for computer analysis, monitoring and verification.
- It should clearly outline the statistical methods proposed to be used for the analysis of data.
- For qualitative approaches, specify in detail how the data will be analysed.
9. Quality Assurance
- The research protocol should clearly describe the quality control and quality assurance system.
- These include GCP, follow up by clinical monitors, DSMB, data management, etc.
10. Expected Outcomes of the Study
- This section indicates how the study will contribute to the advancement of current knowledge, how the results will be utilized beyond publications.
- It must mention how the study will affect health care, health systems, or health policies.
11. Dissemination of Results and Publication Policy
- The research protocol should specify not only how the results will be disseminated in the scientific media, but also to the community and/or the participants, the policy makers, etc.
- The publication policy should be clearly discussed as to who will be mentioned as contributors, who will be acknowledged, etc.
12. Duration of the Project
- The protocol should clearly mention the time likely to be taken for completion of each phase of the project.
- Furthermore a detailed timeline for each activity to be undertaken should also be provided.
13. Anticipated Problems
- The investigators may face some difficulties while conducting the clinical research. This section must include all anticipated problems in successfully completing their projects.
- Furthermore, it should also provide possible solutions to deal with these difficulties.
14. Project Management
- This section includes detailed specifications of the role and responsibility of each investigator of the team.
- Everyone involved in the research project must be mentioned here along with the specific duties they have performed in completing the research.
- The research protocol should also describe the ethical considerations relating to the study.
- It should not only be limited to providing ethics approval, but also the issues that are likely to raise ethical concerns.
- Additionally, the ethics section must also describe how the investigator(s) plan to obtain informed consent from the research participants.
- This section should include a detailed commodity-wise and service-wise breakdown of the requested funds.
- It should also include justification of utilization of each listed item.
17. Supplementary Support for the Project
- This section should include information about the received funding and other anticipated funding for the specific project.
18. Collaboration With Other Researchers or Institutions
- Every researcher or institute that has been a part of the research project must be mentioned in detail in this section of the research protocol.
19. Curriculum Vitae of All Investigators
- The CVs of the principal investigator along with all the co-investigators should be attached with the research protocol.
- Ideally, each CV should be limited to one page only, unless a full-length CV is requested.
20. Other Research Activities of Investigators
- A list of all current research projects being conducted by all investigators must be listed here.
21. References
- All relevant references should be mentioned and cited accurately in this section to avoid plagiarism.
How Do You Write a Research Protocol? (Research Protocol Example)
Main Investigator
Number of Involved Centers (for multi-centric studies)
Indicate the reference center
Title of the Study
Protocol ID (acronym)
Keywords (up to 7 specific keywords)
Study Design
Mono-centric/multi-centric
Perspective/retrospective
Controlled/uncontrolled
Open-label/single-blinded or double-blinded
Randomized/non-randomized
n parallel branches/n overlapped branches
Experimental/observational
Endpoints (main primary and secondary endpoints to be listed)
Expected Results
Analyzed Criteria
Main variables/endpoints of the primary analysis
Main variables/endpoints of the secondary analysis
Safety variables
Health Economy (if applicable)
Visits and Examinations
Therapeutic plan and goals
Visits/controls schedule (also with graphics)
Comparison to treatment products (if applicable)
Dose and dosage for the study duration (if applicable)
Formulation and power of the studied drugs (if applicable)
Method of administration of the studied drugs (if applicable)
Informed Consent
Study Population
Short description of the main inclusion, exclusion, and withdrawal criteria
Sample Size
Estimated Duration of the Study
Safety Advisory
Classification Needed
Requested Funds
Additional Features (based on study objectives)
Click Here to Download the Research Protocol Example/Template
Be prepared to conduct your clinical research by writing a detailed research protocol. It is as easy as mentioned in this article. Follow the aforementioned path and write an impactful research protocol. All the best!

Clear as template! Please, I need your help to shape me an authentic PROTOCOL RESEARCH on this theme: Using the competency-based approach to foster EFL post beginner learners’ writing ability: the case of Benin context. I’m about to start studies for a master degree. Please help! Thanks for your collaboration. God bless.
Rate this article Cancel Reply
Your email address will not be published.
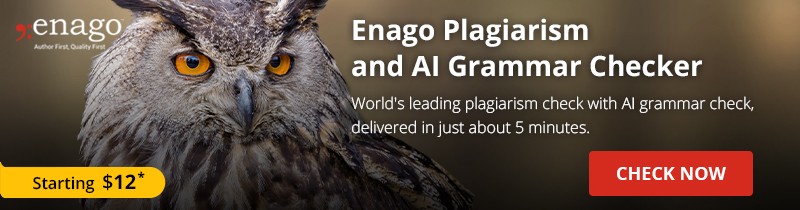
Enago Academy's Most Popular Articles
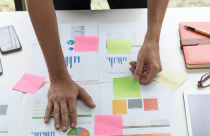
- Publishing Research
- Reporting Research
How to Optimize Your Research Process: A step-by-step guide
For researchers across disciplines, the path to uncovering novel findings and insights is often filled…
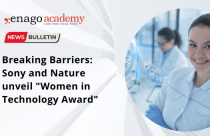
- Industry News
- Trending Now
Breaking Barriers: Sony and Nature unveil “Women in Technology Award”
Sony Group Corporation and the prestigious scientific journal Nature have collaborated to launch the inaugural…
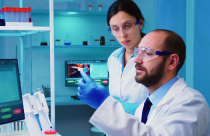
Achieving Research Excellence: Checklist for good research practices
Academia is built on the foundation of trustworthy and high-quality research, supported by the pillars…
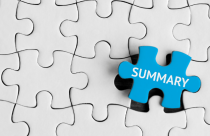
- Promoting Research
Plain Language Summary — Communicating your research to bridge the academic-lay gap
Science can be complex, but does that mean it should not be accessible to the…
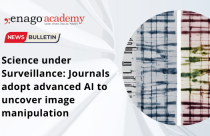
Science under Surveillance: Journals adopt advanced AI to uncover image manipulation
Journals are increasingly turning to cutting-edge AI tools to uncover deceitful images published in manuscripts.…
Choosing the Right Analytical Approach: Thematic analysis vs. content analysis for…
Research Recommendations – Guiding policy-makers for evidence-based decision making
Demystifying the Role of Confounding Variables in Research

Sign-up to read more
Subscribe for free to get unrestricted access to all our resources on research writing and academic publishing including:
- 2000+ blog articles
- 50+ Webinars
- 10+ Expert podcasts
- 50+ Infographics
- 10+ Checklists
- Research Guides
We hate spam too. We promise to protect your privacy and never spam you.
I am looking for Editing/ Proofreading services for my manuscript Tentative date of next journal submission:
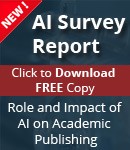
What should universities' stance be on AI tools in research and academic writing?
Data collection in research: Your complete guide
Last updated
31 January 2023
Reviewed by
Cathy Heath
In the late 16th century, Francis Bacon coined the phrase "knowledge is power," which implies that knowledge is a powerful force, like physical strength. In the 21st century, knowledge in the form of data is unquestionably powerful.
But data isn't something you just have - you need to collect it. This means utilizing a data collection process and turning the collected data into knowledge that you can leverage into a successful strategy for your business or organization.
Believe it or not, there's more to data collection than just conducting a Google search. In this complete guide, we shine a spotlight on data collection, outlining what it is, types of data collection methods, common challenges in data collection, data collection techniques, and the steps involved in data collection.
Analyze all your data in one place
Uncover hidden nuggets in all types of qualitative data when you analyze it in Dovetail
- What is data collection?
There are two specific data collection techniques: primary and secondary data collection. Primary data collection is the process of gathering data directly from sources. It's often considered the most reliable data collection method, as researchers can collect information directly from respondents.
Secondary data collection is data that has already been collected by someone else and is readily available. This data is usually less expensive and quicker to obtain than primary data.
- What are the different methods of data collection?
There are several data collection methods, which can be either manual or automated. Manual data collection involves collecting data manually, typically with pen and paper, while computerized data collection involves using software to collect data from online sources, such as social media, website data, transaction data, etc.
Here are the five most popular methods of data collection:
Surveys are a very popular method of data collection that organizations can use to gather information from many people. Researchers can conduct multi-mode surveys that reach respondents in different ways, including in person, by mail, over the phone, or online.
As a method of data collection, surveys have several advantages. For instance, they are relatively quick and easy to administer, you can be flexible in what you ask, and they can be tailored to collect data on various topics or from certain demographics.
However, surveys also have several disadvantages. For instance, they can be expensive to administer, and the results may not represent the population as a whole. Additionally, survey data can be challenging to interpret. It may also be subject to bias if the questions are not well-designed or if the sample of people surveyed is not representative of the population of interest.
Interviews are a common method of collecting data in social science research. You can conduct interviews in person, over the phone, or even via email or online chat.
Interviews are a great way to collect qualitative and quantitative data . Qualitative interviews are likely your best option if you need to collect detailed information about your subjects' experiences or opinions. If you need to collect more generalized data about your subjects' demographics or attitudes, then quantitative interviews may be a better option.
Interviews are relatively quick and very flexible, allowing you to ask follow-up questions and explore topics in more depth. The downside is that interviews can be time-consuming and expensive due to the amount of information to be analyzed. They are also prone to bias, as both the interviewer and the respondent may have certain expectations or preconceptions that may influence the data.
Direct observation
Observation is a direct way of collecting data. It can be structured (with a specific protocol to follow) or unstructured (simply observing without a particular plan).
Organizations and businesses use observation as a data collection method to gather information about their target market, customers, or competition. Businesses can learn about consumer behavior, preferences, and trends by observing people using their products or service.
There are two types of observation: participatory and non-participatory. In participatory observation, the researcher is actively involved in the observed activities. This type of observation is used in ethnographic research , where the researcher wants to understand a group's culture and social norms. Non-participatory observation is when researchers observe from a distance and do not interact with the people or environment they are studying.
There are several advantages to using observation as a data collection method. It can provide insights that may not be apparent through other methods, such as surveys or interviews. Researchers can also observe behavior in a natural setting, which can provide a more accurate picture of what people do and how and why they behave in a certain context.
There are some disadvantages to using observation as a method of data collection. It can be time-consuming, intrusive, and expensive to observe people for extended periods. Observations can also be tainted if the researcher is not careful to avoid personal biases or preconceptions.
Automated data collection
Business applications and websites are increasingly collecting data electronically to improve the user experience or for marketing purposes.
There are a few different ways that organizations can collect data automatically. One way is through cookies, which are small pieces of data stored on a user's computer. They track a user's browsing history and activity on a site, measuring levels of engagement with a business’s products or services, for example.
Another way organizations can collect data automatically is through web beacons. Web beacons are small images embedded on a web page to track a user's activity.
Finally, organizations can also collect data through mobile apps, which can track user location, device information, and app usage. This data can be used to improve the user experience and for marketing purposes.
Automated data collection is a valuable tool for businesses, helping improve the user experience or target marketing efforts. Businesses should aim to be transparent about how they collect and use this data.
Sourcing data through information service providers
Organizations need to be able to collect data from a variety of sources, including social media, weblogs, and sensors. The process to do this and then use the data for action needs to be efficient, targeted, and meaningful.
In the era of big data, organizations are increasingly turning to information service providers (ISPs) and other external data sources to help them collect data to make crucial decisions.
Information service providers help organizations collect data by offering personalized services that suit the specific needs of the organizations. These services can include data collection, analysis, management, and reporting. By partnering with an ISP, organizations can gain access to the newest technology and tools to help them to gather and manage data more effectively.
There are also several tools and techniques that organizations can use to collect data from external sources, such as web scraping, which collects data from websites, and data mining, which involves using algorithms to extract data from large data sets.
Organizations can also use APIs (application programming interface) to collect data from external sources. APIs allow organizations to access data stored in another system and share and integrate it into their own systems.
Finally, organizations can also use manual methods to collect data from external sources. This can involve contacting companies or individuals directly to request data, by using the right tools and methods to get the insights they need.
- What are common challenges in data collection?
There are many challenges that researchers face when collecting data. Here are five common examples:
Big data environments
Data collection can be a challenge in big data environments for several reasons. It can be located in different places, such as archives, libraries, or online. The sheer volume of data can also make it difficult to identify the most relevant data sets.
Second, the complexity of data sets can make it challenging to extract the desired information. Third, the distributed nature of big data environments can make it difficult to collect data promptly and efficiently.
Therefore it is important to have a well-designed data collection strategy to consider the specific needs of the organization and what data sets are the most relevant. Alongside this, consideration should be made regarding the tools and resources available to support data collection and protect it from unintended use.
Data bias is a common challenge in data collection. It occurs when data is collected from a sample that is not representative of the population of interest.
There are different types of data bias, but some common ones include selection bias, self-selection bias, and response bias. Selection bias can occur when the collected data does not represent the population being studied. For example, if a study only includes data from people who volunteer to participate, that data may not represent the general population.
Self-selection bias can also occur when people self-select into a study, such as by taking part only if they think they will benefit from it. Response bias happens when people respond in a way that is not honest or accurate, such as by only answering questions that make them look good.
These types of data bias present a challenge because they can lead to inaccurate results and conclusions about behaviors, perceptions, and trends. Data bias can be avoided by identifying potential sources or themes of bias and setting guidelines for eliminating them.
Lack of quality assurance processes
One of the biggest challenges in data collection is the lack of quality assurance processes. This can lead to several problems, including incorrect data, missing data, and inconsistencies between data sets.
Quality assurance is important because there are many data sources, and each source may have different levels of quality or corruption. There are also different ways of collecting data, and data quality may vary depending on the method used.
There are several ways to improve quality assurance in data collection. These include developing clear and consistent goals and guidelines for data collection, implementing quality control measures, using standardized procedures, and employing data validation techniques. By taking these steps, you can ensure that your data is of adequate quality to inform decision-making.
Limited access to data
Another challenge in data collection is limited access to data. This can be due to several reasons, including privacy concerns, the sensitive nature of the data, security concerns, or simply the fact that data is not readily available.
Legal and compliance regulations
Most countries have regulations governing how data can be collected, used, and stored. In some cases, data collected in one country may not be used in another. This means gaining a global perspective can be a challenge.
For example, if a company is required to comply with the EU General Data Protection Regulation (GDPR), it may not be able to collect data from individuals in the EU without their explicit consent. This can make it difficult to collect data from a target audience.
Legal and compliance regulations can be complex, and it's important to ensure that all data collected is done so in a way that complies with the relevant regulations.
- What are the key steps in the data collection process?
There are five steps involved in the data collection process. They are:
1. Decide what data you want to gather
Have a clear understanding of the questions you are asking, and then consider where the answers might lie and how you might obtain them. This saves time and resources by avoiding the collection of irrelevant data, and helps maintain the quality of your datasets.
2. Establish a deadline for data collection
Establishing a deadline for data collection helps you avoid collecting too much data, which can be costly and time-consuming to analyze. It also allows you to plan for data analysis and prompt interpretation. Finally, it helps you meet your research goals and objectives and allows you to move forward.
3. Select a data collection approach
The data collection approach you choose will depend on different factors, including the type of data you need, available resources, and the project timeline. For instance, if you need qualitative data, you might choose a focus group or interview methodology. If you need quantitative data , then a survey or observational study may be the most appropriate form of collection.
4. Gather information
When collecting data for your business, identify your business goals first. Once you know what you want to achieve, you can start collecting data to reach those goals. The most important thing is to ensure that the data you collect is reliable and valid. Otherwise, any decisions you make using the data could result in a negative outcome for your business.
5. Examine the information and apply your findings
As a researcher, it's important to examine the data you're collecting and analyzing before you apply your findings. This is because data can be misleading, leading to inaccurate conclusions. Ask yourself whether it is what you are expecting? Is it similar to other datasets you have looked at?
There are many scientific ways to examine data, but some common methods include:
looking at the distribution of data points
examining the relationships between variables
looking for outliers
By taking the time to examine your data and noticing any patterns, strange or otherwise, you can avoid making mistakes that could invalidate your research.
- How qualitative analysis software streamlines the data collection process
Knowledge derived from data does indeed carry power. However, if you don't convert the knowledge into action, it will remain a resource of unexploited energy and wasted potential.
Luckily, data collection tools enable organizations to streamline their data collection and analysis processes and leverage the derived knowledge to grow their businesses. For instance, qualitative analysis software can be highly advantageous in data collection by streamlining the process, making it more efficient and less time-consuming.
Secondly, qualitative analysis software provides a structure for data collection and analysis, ensuring that data is of high quality. It can also help to uncover patterns and relationships that would otherwise be difficult to discern. Moreover, you can use it to replace more expensive data collection methods, such as focus groups or surveys.
Overall, qualitative analysis software can be valuable for any researcher looking to collect and analyze data. By increasing efficiency, improving data quality, and providing greater insights, qualitative software can help to make the research process much more efficient and effective.
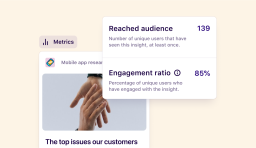
Learn more about qualitative research data analysis software
Get started today.
Go from raw data to valuable insights with a flexible research platform
Editor’s picks
Last updated: 21 December 2023
Last updated: 16 December 2023
Last updated: 6 October 2023
Last updated: 5 March 2024
Last updated: 25 November 2023
Last updated: 15 February 2024
Last updated: 11 March 2024
Last updated: 12 December 2023
Last updated: 6 March 2024
Last updated: 10 April 2023
Last updated: 20 December 2023
Latest articles
Related topics, log in or sign up.
Get started for free
Protocols for Data Collection, Management and Treatment
- First Online: 03 August 2016
Cite this chapter
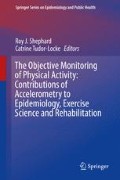
- Catrine Tudor-Locke 5
Part of the book series: Springer Series on Epidemiology and Public Health ((SSEH))
1175 Accesses
1 Citations
Epidemiologists and other researchers must plan study protocols that incorporate objective monitoring of physical activity and/or sedentary behaviours by systematically considering all of the complex logistics associated with data collection, management and treatment. With regard to data collection, instrument choice is a foremost consideration, largely shaped by the researcher’s questions and available resources. Instrument-specific features may provide greater analytical capacity, but can also greatly complicate planning and must be accommodated. Data collection decisions also include the duration of monitoring required to establish stable estimates of behaviour, without overburdening participants. Data management concerns include systematic processes for quality control, data cleaning, data organization and storage. Data treatment includes decision rules that further shape the accumulated information, including computation of derived variables (as catalogued in Chap. 3 ) in anticipation of subsequent data analyses.
This is a preview of subscription content, log in via an institution to check access.
Access this chapter
- Available as PDF
- Read on any device
- Instant download
- Own it forever
- Available as EPUB and PDF
- Compact, lightweight edition
- Dispatched in 3 to 5 business days
- Free shipping worldwide - see info
- Durable hardcover edition
Tax calculation will be finalised at checkout
Purchases are for personal use only
Institutional subscriptions
Tudor-Locke C, Barreira TV, Schuna JM, et al. Improving wear time compliance with a 24-hour waist-worn accelerometer protocol in the International Study of Childhood Obesity, Lifestyle and the Environment (ISCOLE). Int J Behv Nutr Phys Act. 2015;12:11.
Article Google Scholar
Tudor-Locke C, Camhi SM, Troiano RP. A catalog of rules, variables, and definitions applied to accelerometer data in the National Health and Nutrition Examination Survey, 2003–2006. Prev Chronic Dis. 2012;9:E113.
PubMed PubMed Central Google Scholar
Rogers LQ. Objective monitoring of physical activity after a cancer diagnosis: challenges and opportunities for enhancing cancer control. Phys Ther Rev. 2010;15(3):224–37.
Article PubMed PubMed Central Google Scholar
Trost SG, McIver KL, Pate RR. Conducting accelerometer-based activity assessments in field-based research. Med Sci Sports Exerc. 2005;37(11 Suppl):S531–43.
Article PubMed Google Scholar
Crouter SE, Flynn JI, Bassett DR. Estimating physical activity in youth using a wrist accelerometer. Med Sci Sports Exerc. 2015;47(5):944–51.
Hart TL, McClain JJ, Tudor-Locke C. Controlled and free-living evaluation of objective measures of sedentary and active behaviors. J Phys Act Health. 2011;8(6):848–57.
PubMed Google Scholar
Gorelick ML, Bizzini M, Maffiuletti NA, et al. Test–retest reliability of the IDEEA system in the quantification of step parameters during walking and stair climbing. Clin Physiol Funct Imaging. 2009;29(4):271–6.
Kozey-Keadle S, Libertine A, Staudenmayer J, et al. The feasibility of reducing and measuring sedentary time among overweight, non-exercising office workers. J Obes. 2012;2012:282303.
Stanton R, Guertler D, Duncan MJ, et al. Validation of a pouch-mounted activPAL3 accelerometer. Gait Posture. 2014;40(4):688–93.
Harrington DM, Dowd KP, Tudor-Locke C, et al. A steps/minute value for moderate intensity physical activity in adolescent females. Pediatr Exerc Sci. 2012;24:399–408.
Audrey S, Bell S, Hughes R, et al. Adolescent perspectives on wearing accelerometers to measure physical activity in population-based trials. Eur J Public Health. 2013;23(3):475–80.
Meltzer LJ, Montgomery-Downs HE, Insana SP, et al. Use of actigraphy for assessment in pediatric sleep research. Sleep Med Rev. 2012;16(5):463–75.
Rosenberger ME, Haskell WL, Albinali F, et al. Estimating activity and sedentary behavior from an accelerometer on the hip or wrist. Med Sci Sports Exerc. 2013;45(5):964–75.
Masse LC, Fuemmeler BF, Anderson CB, et al. Accelerometer data reduction: a comparison of four reduction algorithms on select outcome variables. Med Sci Sports Exerc. 2005;37(11 Suppl):S544–54.
Tudor-Locke C, Johnson WD, Katzmarzyk PT. U.S. population profile of time-stamped accelerometer outputs: impact of wear time. J Phys Act Health. 2011;8:693–8.
Choi L, Ward SC, Schnelle JF, et al. Assessment of wear/nonwear time classification algorithms for triaxial accelerometer. Med Sci Sports Exerc. 2012;44(10):2009–16.
Troiano RP, McClain JJ, Brychta RJ, et al. Evolution of accelerometer methods for physical activity research. Br J Sports Med. 2014;48(13):1019–23.
Aoyagi Y, Shephard RJ. Sex differences in relationship between habitual physical activity and health in the elderly: practical implications for epidemiologists based on pedometer/accelerometer data from the Nakanojo Study. Arch Gerontol Geriatr. 2013;56:327–38.
Kinder JR, Lee KA, Thompson H, et al. Validation of a hip-worn accelerometer in measuring sleep time in children. J Pediatr Nurs. 2012;27(2):127–33.
Swartz AM, Strath SJ, Bassett DR, et al. Estimation of energy expenditure using CSA accelerometers at hip and wrist sites. Med Sci Sports Exerc. 2000;32(9 Suppl):S450–6.
Article CAS PubMed Google Scholar
Tudor-Locke C, Barreira TV, Schuna Jr JM. Comparison of step outputs for waist and wrist accelerometer attachment sites. Med Sci Sports Exerc. 2015;47(4):839–42.
Nilsson A, Ekelund U, Yngve A, et al. Assessing physical activity among children with accelerometers using different time sampling intervals and placements. Pediatr Exerc Sci. 2002;14(1):87–96.
Google Scholar
McClain JJ, Abraham TL, Brusseau TA, et al. Epoch length and accelerometer outputs in children: comparison to direct observation. Med Sci Sports Exerc. 2008;40(12):2080–7.
Evenson KR, Catellier DJ, Gill K, et al. Calibration of two objective measures of physical activity for children. J Sports Sci. 2008;26(14):1557–65.
Lee IM, Shiroma EJ. Using accelerometers to measure physical activity in large-scale epidemiological studies: issues and challenges. Br J Sports Med. 2014;48(3):197–201.
Inoue S, Ohya Y, Tudor-Locke C, et al. Time trends for step-determined physical activity among Japanese adults. Med Sci Sports Exerc. 2011;43(10):1913–9.
Matthews CE, Ainsworth BE, Thompson RW, et al. Sources of variance in daily physical activity levels as measured by an accelerometer. Med Sci Sports Exerc. 2002;34(8):1376–81.
Yoshiuchi K, Nakahara R, Kumano H, et al. Yearlong physical activity and depressive symptoms in older Japanese adults: cross-sectional data from the Nakanojo study. Am J Geriatr Psychiatry. 2006;14(7):621–4.
Togo F, Watanabe E, Park H, et al. Meteorology and the physical activity of the elderly: the Nakanojo Study. Int J Biometeorol. 2005;50(2):83–9.
Felton GM, Tudor-Locke C, Burkett L. Reliability of pedometer-determined free-living physical activity data in college women. Res Q Exerc Sport. 2006;77(3):304–8.
Vincent SD, Pangrazi RP. An examination of the activity patterns of elementary school children. Pediatr Exerc Sci. 2002;14:432–41.
Nunnally JC, Bernstein IH. Psychometric theory. New York: McGraw-Hill; 1994.
Rowe DA, Kemble CD, Robinson TS, et al. Daily walking in older adults: day-to-day variability and criterion-referenced validity of total daily step counts. J Phys Act Health. 2007;4(4):434–46.
Trost SG, Pate RR, Freedson PS, et al. Using objective physical activity measures with youth: how many days of monitoring are needed? Med Sci Sports Exerc. 2000;32(2):426–31.
Brooke HL, Corder K, Atkin AJ, et al. A systematic literature review with meta-analyses of within- and between-day differences in objectively measured physical activity in school-aged children. Sports Med. 2014;44(10):1427–38.
Tudor-Locke C, McClain JJ, Hart TL, et al. Expected values for pedometer-determined physical activity in youth. Res Q Exerc Sport. 2009;80(2):164–74.
Dasgupta K, Joseph L, Pilote L, et al. Daily steps are low year-round and dip lower in fall/winter: findings from a longitudinal diabetes cohort. Cardiovasc Diabetol. 2010;9:81.
Silva P, Santos R, Welk G, et al. Seasonal differences in physical activity and sedentary patterns: the relevance of the PA context. J Sports Sci Med. 2011;10(1):66–72.
Oliver M, Schluter PJ, Schofield GM, et al. Factors related to accelerometer-derived physical activity in Pacific children aged 6 years. Asia Pac J Public Health. 2011;23(1):44–56.
Chan CB, Ryan DA, Tudor-Locke C. Relationship between objective measures of physical activity and weather: a longitudinal study. Int J Behav Nutr Phys Act. 2006;3:21.
Tudor-Locke C, Bassett DR, Swartz AM, et al. A preliminary study of one year of pedometer self-monitoring. Behav Med. 2004;28(3):158–62.
Togo F, Watanabe E, Park H, et al. How many days of pedometer use predict the annual activity of the elderly reliably? Med Sci Sports Exerc. 2008;40(6):1058–64.
Troiano RP, Berrigan D, Dodd KW, et al. Physical activity in the United States measured by accelerometer. Med Sci Sports Exerc. 2008;40(1):181–8.
Rowlands AV, Gomersall SR, Tudor-Locke C, et al. Introducing novel approaches for examining the variability of individuals’ physical activity. J Sports Sci. 2014;21:1–10.
Clemes SA, Deans NK. Presence and duration of reactivity to pedometers in adults. Med Sci Sports Exerc. 2012;44(6):1097–101.
Matevey C, Rogers LQ, Dawson B, et al. Lack of reactivity during pedometer self-monitoring in adults. Meas Phys Educ Exerc Sci. 2006;10(1):1–11.
Craig CL, Tudor-Locke C, Cragg S, et al. Process and treatment of pedometer data collection for youth: the CANPLAY study. Med Sci Sports Exerc. 2010;42(3):430–5.
Raustorp A, Ekroth Y. Eight-year secular trends of pedometer-determined physical activity in young Swedish adolescents. J Phys Act Health. 2010;7(3):369–74.
Dossegger A, Ruch N, Jimmy G, et al. Reactivity to accelerometer measurement of children and adolescents. Med Sci Sports Exerc. 2014;46(6):1140–6.
Yasunaga A, Togo F, Watanabe E, et al. Yearlong physical activity and health-related quality of life in older Japanese adults: the Nakanojo Study. J Phys Act Health. 2006;14(3):288–301.
Ward DS, Evenson KR, Vaughn A, et al. Accelerometer use in physical activity: best practices and research recommendations. Med Sci Sports Exerc. 2005;37(11 Suppl):S582–8.
Craig CL, Cameron C, Tudor-Locke C. CANPLAY pedometer normative reference data for 21,271 children and 12,956 adolescents. Med Sci Sports Exerc. 2013;45(1):123–9.
Heil DP, Brage S, Rothney MP. Modeling physical activity outcomes from wearable monitors. Med Sci Sports Exerc. 2012;44(1 Suppl 1):S50–60.
Kang M, Rowe DA, Barreira TV, et al. Individual information-centered approach for handling physical activity missing data. Res Q Exerc Sport. 2009;80(2):131–7.
Catellier DJ, Hannan PJ, Murray DM, et al. Imputation of missing data when measuring physical activity by accelerometry. Med Sci Sports Exerc. 2005;37(11 Suppl):S555–62.
Rowe DA, Mahar MT, Raedeke TD, et al. Measuring physical activity in children with pedometers: reliability, reactivity, and replacement of missing data. Pediatr Exerc Sci. 2004;16:343–54.
Schuna Jr JM, Johnson WD, Tudor-Locke C. Adult self-reported and objectively monitored physical activity and sedentary behavior: NHANES 2005–2006. Int J Behav Nutr Phys Act. 2013;10(1):126.
Herrmann SD, Barreira TV, Kang M, et al. How many hours are enough? Accelerometer wear time may provide bias in daily activity estimates. J Phys Act Health. 2012;10(5):742–9.
Schmidt MD, Blizzard CL, Venn AJ, et al. Practical considerations when using pedometers to assess physical activity in population studies: lessons from the Burnie Take Heart Study. Res Q Exerc Sport. 2007;78(3):162–70.
Tudor-Locke C, Barreira TV, Schuna JM, et al. Fully automated waist-worn accelerometer algorithm for detecting children’s sleep period time separate from 24-hour physical activity or sedentary behaviors. Appl Physiol Nutr Metab. 2014;39(1):53–7.
Barreira TV, Schuna Jr JM, Mire EF, et al. Identifying children’s nocturnal sleep using 24-hour waist accelerometry. Med Sci Sports Exerc. 2015;47(5):937–43.
Freedson PS, Melanson E, Sirard J. Calibration of the Computer Science and Applications, Inc. accelerometer. Med Sci Sports Exerc. 1998;30(5):777–81.
Crouter SE, Clowers KG, Bassett DR. A novel method for using accelerometer data to predict energy expenditure. J Appl Physiol. 2006;100(4):1324–31.
Tudor-Locke C, Sisson SB, Collova T, et al. Pedometer-determined step count guidelines for classifying walking intensity in a young ostensibly healthy population. Can J Appl Physiol. 2005;30(6):666–76.
Staudenmayer J, Pober D, Crouter S, et al. An artificial neural network to estimate physical activity energy expenditure and identify physical activity type from an accelerometer. J Appl Physiol. 2009;107(4):1300–7.
Pober DM, Staudenmayer J, Raphael C, et al. Development of novel techniques to classify physical activity mode using accelerometers. Med Sci Sports Exerc. 2006;38(9):1626–34.
Download references
Author information
Authors and affiliations.
Department of Kinesiology, University of Massachusetts Amherst, Amherst, MA, USA
Catrine Tudor-Locke
You can also search for this author in PubMed Google Scholar
Corresponding author
Correspondence to Catrine Tudor-Locke .
Editor information
Editors and affiliations.
University of Toronto, Brackendale, British Columbia, Canada
Roy J. Shephard
Pennington Biomedical Research Cent, Louisiana State University, Baton Rouge, Louisiana, USA
Rights and permissions
Reprints and permissions
Copyright information
© 2016 Springer International Publishing Switzerland
About this chapter
Tudor-Locke, C. (2016). Protocols for Data Collection, Management and Treatment. In: Shephard, R., Tudor-Locke, C. (eds) The Objective Monitoring of Physical Activity: Contributions of Accelerometry to Epidemiology, Exercise Science and Rehabilitation. Springer Series on Epidemiology and Public Health. Springer, Cham. https://doi.org/10.1007/978-3-319-29577-0_4
Download citation
DOI : https://doi.org/10.1007/978-3-319-29577-0_4
Published : 03 August 2016
Publisher Name : Springer, Cham
Print ISBN : 978-3-319-29575-6
Online ISBN : 978-3-319-29577-0
eBook Packages : Medicine Medicine (R0)
Share this chapter
Anyone you share the following link with will be able to read this content:
Sorry, a shareable link is not currently available for this article.
Provided by the Springer Nature SharedIt content-sharing initiative
- Publish with us
Policies and ethics
- Find a journal
- Track your research
Have a language expert improve your writing
Run a free plagiarism check in 10 minutes, automatically generate references for free.
- Knowledge Base
- Methodology
- Data Collection Methods | Step-by-Step Guide & Examples
Data Collection Methods | Step-by-Step Guide & Examples
Published on 4 May 2022 by Pritha Bhandari .
Data collection is a systematic process of gathering observations or measurements. Whether you are performing research for business, governmental, or academic purposes, data collection allows you to gain first-hand knowledge and original insights into your research problem .
While methods and aims may differ between fields, the overall process of data collection remains largely the same. Before you begin collecting data, you need to consider:
- The aim of the research
- The type of data that you will collect
- The methods and procedures you will use to collect, store, and process the data
To collect high-quality data that is relevant to your purposes, follow these four steps.
Table of contents
Step 1: define the aim of your research, step 2: choose your data collection method, step 3: plan your data collection procedures, step 4: collect the data, frequently asked questions about data collection.
Before you start the process of data collection, you need to identify exactly what you want to achieve. You can start by writing a problem statement : what is the practical or scientific issue that you want to address, and why does it matter?
Next, formulate one or more research questions that precisely define what you want to find out. Depending on your research questions, you might need to collect quantitative or qualitative data :
- Quantitative data is expressed in numbers and graphs and is analysed through statistical methods .
- Qualitative data is expressed in words and analysed through interpretations and categorisations.
If your aim is to test a hypothesis , measure something precisely, or gain large-scale statistical insights, collect quantitative data. If your aim is to explore ideas, understand experiences, or gain detailed insights into a specific context, collect qualitative data.
If you have several aims, you can use a mixed methods approach that collects both types of data.
- Your first aim is to assess whether there are significant differences in perceptions of managers across different departments and office locations.
- Your second aim is to gather meaningful feedback from employees to explore new ideas for how managers can improve.
Prevent plagiarism, run a free check.
Based on the data you want to collect, decide which method is best suited for your research.
- Experimental research is primarily a quantitative method.
- Interviews , focus groups , and ethnographies are qualitative methods.
- Surveys , observations, archival research, and secondary data collection can be quantitative or qualitative methods.
Carefully consider what method you will use to gather data that helps you directly answer your research questions.
When you know which method(s) you are using, you need to plan exactly how you will implement them. What procedures will you follow to make accurate observations or measurements of the variables you are interested in?
For instance, if you’re conducting surveys or interviews, decide what form the questions will take; if you’re conducting an experiment, make decisions about your experimental design .
Operationalisation
Sometimes your variables can be measured directly: for example, you can collect data on the average age of employees simply by asking for dates of birth. However, often you’ll be interested in collecting data on more abstract concepts or variables that can’t be directly observed.
Operationalisation means turning abstract conceptual ideas into measurable observations. When planning how you will collect data, you need to translate the conceptual definition of what you want to study into the operational definition of what you will actually measure.
- You ask managers to rate their own leadership skills on 5-point scales assessing the ability to delegate, decisiveness, and dependability.
- You ask their direct employees to provide anonymous feedback on the managers regarding the same topics.
You may need to develop a sampling plan to obtain data systematically. This involves defining a population , the group you want to draw conclusions about, and a sample, the group you will actually collect data from.
Your sampling method will determine how you recruit participants or obtain measurements for your study. To decide on a sampling method you will need to consider factors like the required sample size, accessibility of the sample, and time frame of the data collection.
Standardising procedures
If multiple researchers are involved, write a detailed manual to standardise data collection procedures in your study.
This means laying out specific step-by-step instructions so that everyone in your research team collects data in a consistent way – for example, by conducting experiments under the same conditions and using objective criteria to record and categorise observations.
This helps ensure the reliability of your data, and you can also use it to replicate the study in the future.
Creating a data management plan
Before beginning data collection, you should also decide how you will organise and store your data.
- If you are collecting data from people, you will likely need to anonymise and safeguard the data to prevent leaks of sensitive information (e.g. names or identity numbers).
- If you are collecting data via interviews or pencil-and-paper formats, you will need to perform transcriptions or data entry in systematic ways to minimise distortion.
- You can prevent loss of data by having an organisation system that is routinely backed up.
Finally, you can implement your chosen methods to measure or observe the variables you are interested in.
The closed-ended questions ask participants to rate their manager’s leadership skills on scales from 1 to 5. The data produced is numerical and can be statistically analysed for averages and patterns.
To ensure that high-quality data is recorded in a systematic way, here are some best practices:
- Record all relevant information as and when you obtain data. For example, note down whether or how lab equipment is recalibrated during an experimental study.
- Double-check manual data entry for errors.
- If you collect quantitative data, you can assess the reliability and validity to get an indication of your data quality.
Data collection is the systematic process by which observations or measurements are gathered in research. It is used in many different contexts by academics, governments, businesses, and other organisations.
When conducting research, collecting original data has significant advantages:
- You can tailor data collection to your specific research aims (e.g., understanding the needs of your consumers or user testing your website).
- You can control and standardise the process for high reliability and validity (e.g., choosing appropriate measurements and sampling methods ).
However, there are also some drawbacks: data collection can be time-consuming, labour-intensive, and expensive. In some cases, it’s more efficient to use secondary data that has already been collected by someone else, but the data might be less reliable.
Quantitative research deals with numbers and statistics, while qualitative research deals with words and meanings.
Quantitative methods allow you to test a hypothesis by systematically collecting and analysing data, while qualitative methods allow you to explore ideas and experiences in depth.
Reliability and validity are both about how well a method measures something:
- Reliability refers to the consistency of a measure (whether the results can be reproduced under the same conditions).
- Validity refers to the accuracy of a measure (whether the results really do represent what they are supposed to measure).
If you are doing experimental research , you also have to consider the internal and external validity of your experiment.
In mixed methods research , you use both qualitative and quantitative data collection and analysis methods to answer your research question .
Operationalisation means turning abstract conceptual ideas into measurable observations.
For example, the concept of social anxiety isn’t directly observable, but it can be operationally defined in terms of self-rating scores, behavioural avoidance of crowded places, or physical anxiety symptoms in social situations.
Before collecting data , it’s important to consider how you will operationalise the variables that you want to measure.
Cite this Scribbr article
If you want to cite this source, you can copy and paste the citation or click the ‘Cite this Scribbr article’ button to automatically add the citation to our free Reference Generator.
Bhandari, P. (2022, May 04). Data Collection Methods | Step-by-Step Guide & Examples. Scribbr. Retrieved 15 April 2024, from https://www.scribbr.co.uk/research-methods/data-collection-guide/
Is this article helpful?
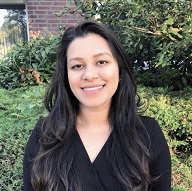
Pritha Bhandari
Other students also liked, qualitative vs quantitative research | examples & methods, triangulation in research | guide, types, examples, what is a conceptual framework | tips & examples.
Collect Data
Data will be collected and subsequently analyzed during the Manage stage, using the protocol for collecting data developed in the Plan/Propose stage and the processes and technical resources established during the Setup stage. Strict adherence to the data collection protocol design is critical to assuring that the data collected as well as the results of their analysis can be validated.
- The Principal Investigator (PI) is responsible for the overall conduct (including administration and compliance) and results of the research, including the collection of data.
- Members of the study team who will be involved in any aspect of data collection are each responsible for observing best research, administrative and compliance practices, appropriate to their role in the project.
- A statistician or other data analyst on the study team may be involved in monitoring data collection.
- Clinical studies may engage a Data Safety and Monitoring Board (DSMB) responsible for periodically monitoring the data collection protocol.
- Information technology professionals may be involved in providing support for hardware and software used for data collection, according to best technical practices.
Forms, Tools, and Resources
Policy, regulation, and guidance.
- Office of the Chief Information Security Officer: Laws
Announcements
Or support offices.
- Human Subjects Division (HSD)
- Office of Animal Welfare (OAW)
- Office of Research (OR)
- Office of Research Information Services (ORIS)
- Office of Sponsored Programs (OSP)
OR Research Units
- Applied Physics Laboratory (APL-UW)
- WA National Primate Research Center (WaNPRC)
Research Partner Offices
- Corporate and Foundation Relations (CFR)
- Enivronmental Health and Safety (EH&S)
- Grant and Contract Accounting (GCA)
- Institute of Translational Health Sciences (ITHS)
- Management Accounting and Analysis (MAA)
- Post Award Fiscal Compliance (PAFC)
Collaboration
- Centers and Institutes
- Collaborative Proposal Development Resources
- Research Fact Sheet
- Research Annual Report
- Stats and Rankings
- Honors and Awards
- Office of Research
© 2024 University of Washington | Seattle, WA
Thank you for visiting nature.com. You are using a browser version with limited support for CSS. To obtain the best experience, we recommend you use a more up to date browser (or turn off compatibility mode in Internet Explorer). In the meantime, to ensure continued support, we are displaying the site without styles and JavaScript.
- View all journals
- My Account Login
- Explore content
- About the journal
- Publish with us
- Sign up for alerts
- Review Article
- Open access
- Published: 16 February 2024
Approaches to protocol standardization and data harmonization in the ECHO-wide cohort study
- Lisa P. Jacobson ORCID: orcid.org/0000-0003-1722-6826 1 ,
- Corette B. Parker 2 ,
- David Cella 3 ,
- Daniel K. Mroczek 3 , 4 &
- Barry M. Lester 5 , 6
on behalf of program collaborators for Environmental influences on Child Health Outcomes
Pediatric Research ( 2024 ) Cite this article
299 Accesses
Metrics details
The United States (U.S.) National Institutes of Health–funded Environmental influences on Child Health Outcomes (ECHO)-wide Cohort was established to conduct high impact, transdisciplinary science to improve child health and development. The cohort is a collaborative research design in which both extant and new data are contributed by over 57,000 children across 69 cohorts. In this review article, we focus on two key challenging issues in the ECHO-wide Cohort: data collection standardization and data harmonization. Data standardization using a Common Data Model and derived analytical variables based on a team science approach should facilitate timely analyses and reduce errors due to data misuse. However, given the complexity of collaborative research designs, such as the ECHO-wide Cohort, dedicated time is needed for harmonization and derivation of analytic variables. These activities need to be done methodically and with transparency to enhance research reproducibility.
Many collaborative research studies require data harmonization either prior to analyses or in the analyses of compiled data.
The Environmental influences on Child Health Outcomes (ECHO) Cohort pools extant data with new data collection from over 57,000 children in 69 cohorts to conduct high-impact, transdisciplinary science to improve child health and development, and to provide a national database and biorepository for use by the scientific community at-large.
We describe the tools, systems, and approaches we employed to facilitate harmonized data for impactful analyses of child health outcomes.
Similar content being viewed by others
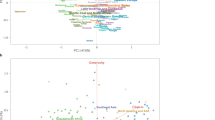
Principal component analysis
Michael Greenacre, Patrick J. F. Groenen, … Elena Tuzhilina
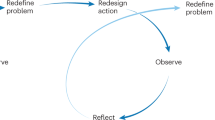
Participatory action research
Flora Cornish, Nancy Breton, … Darrin Hodgetts
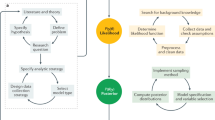
Bayesian statistics and modelling
Rens van de Schoot, Sarah Depaoli, … Christopher Yau
Introduction
The landmark Environmental influences on Child Health Outcomes (ECHO; https://www.nih.gov/echo ) research program was launched in 2016 by the National Institutes of Health (NIH). 1 , 2 , 3 The ECHO Program includes the ECHO-wide Cohort Study (EWC), an observational cohort created by pooling existing studies, and the Institutional Development Award (IDeA) States Pediatric Clinical Trials Network (ISPCTN) that centers on intervention research among children from 17 states generally underrepresented in clinical trials. The EWC was established to conduct high impact, transdisciplinary science to improve child health and development and to provide a national database and biorepository for use by the scientific community at-large. In this review article, we focus on two key challenging issues in the EWC: data collection standardization and data harmonization.
The EWC was established to address issues that may not be addressed by individual studies, which typically focus on a single outcome area or exposure, have limited statistical power to study rare clinical outcomes, and have limited generalizability. Pooling cohorts with common outcomes of interest and harmonizing data enable the EWC to conduct in-depth analyses of critical issues and account for confounding and modification unencumbered by traditional limitations, including sample size, diversity, and individual cohort characteristics.
The EWC began with existing pregnancy, birth, and early childhood cohorts that were collecting longitudinal data and expanded recruitment and continued follow-up. A total of 69 cohorts funded by 31 awards were involved in contributing data from >57,000 children from diverse backgrounds across the United States (U.S.). Five outcome areas were targeted: (1) pre-, peri-, and postnatal outcomes; (2) upper and lower airway conditions; (3) obesity; (4) neurodevelopment; and (5) positive health.
In ECHO, environmental exposures include the totality of early life conditions, not just traditional exposures, such as air pollution and chemical toxicants, but also home, neighborhood, socioeconomic, behavioral, and psychosocial factors. ECHO chose these factors because they pinpoint modifiable aspects of the environment. ECHO’s goal is to inform programs, policies, and practices by illuminating the risk factors of poor child outcomes and protective factors that buffer the child and facilitate resilience.
The 69 cohorts represent a demographically diverse cross-section of U.S. geographic regions, including children from metropolitan and rural populations and with socioeconomic heterogeneity. The EWC includes cohorts from Native American populations and cohorts with over-representation of Black/African American and Hispanic/Latino children. ECHO, comprising the EWC and ISPCTN, promotes translating observational research into intervention trials and accelerating the development of solution-oriented treatments. As of February 14, 2022, ECHO had 909 published articles ( https://echochildren.org/echo-program-publications/ ).
The EWC database comprises extant data collected by the cohorts prior to ECHO and new data collected by the cohorts using a common protocol. The combined data provide a powerful resource for the pediatric research community. Leveraging the existing infrastructure and extant data, EWC investigators developed and implemented the ECHO-wide Cohort Protocol (EWCP) to launch this large-scale collaborative research study. 2 , 4 , 5
Successful EWC science requires both standardization of new data collection and harmonization of the extant data containing different measures used by the cohorts to assess data elements or constructs of interest. The EWCP defines the data elements for new data collection and extant data transfer; data elements are deemed either essential (must collect) or recommended (collect if possible) for new data collection. These designated data elements also are the required set to be submitted by cohort investigators from their existing data. In addition, cohorts have other related extant data to be submitted for potential harmonization. The EWCP further specifies preferred and acceptable measures that cohorts may use for new data collection. The use of multiple measures for the same element requires harmonization to capitalize on the breadth of data offered by the EWC. Here, we describe harmonization practices that advance collaborative research studies to answer compelling questions about pediatric health.
The ECHO-wide Cohort approach
As described by LeWinn and colleagues, 2 the EWC Protocol Working Group developed the EWCP to standardize new data collection. They describe the Protocol Working Group life stage subcommittees, interactions with Outcome Working Groups and the Alternative Measures Task Force, and the processes for managing communication across these constituents.
In this review article, we demonstrate how the EWC collaborative structure was further mobilized to achieve large-scale, high impact science, including novel approaches for data harmonization. We provide details on the Data Analysis Center (DAC) Data Systems used by the cohorts to map and upload data, efforts by the Person-Reported Outcomes (PRO) Core to link and otherwise harmonize similar measures of latent constructs, and the processes overseen by the Data Harmonization Working Group (DHWG) that support data harmonization as prioritized by Steering Committee-approved analysis proposals.
The cohorts that contribute to the EWC are heterogeneous in participant demographics, enrollment criteria, follow-up period, data elements, data collection modes, and study designs. Whereas combining data from these cohorts improves the generalizability and transportability of research findings, creating a Common Data Model (CDM) with such content diversity is a great challenge. By limiting the data collection measures, a common protocol standardizes new data collection. However, incorporating existing data into the CDM requires extensive harmonization. The DHWG was therefore established to coordinate harmonization efforts and to develop best practice guidelines. Harmonization is the responsibility of all components, including the DAC, the PRO Core, and substantive experts from various cohorts. Here, we describe harmonization challenges and our approaches to facilitate analyses using a CDM.
Standardizing new data collection
As previously described, 2 the EWCP defines the elements that constitute the EWC platform for analysis and the measures that cohorts should use for new data collection. These elements are listed according to participant life stage: prenatal, perinatal, infancy, early childhood, middle childhood, and adolescence.
An initial decision surrounded whether the study would require cohorts to use the same measures to collect data during the life stage or whether cohort-specific measures would be allowed. Using the same measure increases standardization and facilitates a quicker and less error-prone path to data analysis. Using cohort-specific measures allows implementation with less new training and facilitates longitudinal analyses of legacy measures within a cohort but ultimately requires harmonization prior to analysis of data across cohorts. For each essential data element, the protocol allows cohorts to use preferred or acceptable measures for its collection, with the understanding that the data may be harmonized. In some instances, cohorts were allowed to continue to collect data with measures that they previously used; these legacy measures were defined as “alternative” measures. An Alternative Measures Task Force developed the process for investigators to use when requesting the inclusion of an alternative measure in subsequent versions of the protocol. This was the first step toward ECHO-wide standardization and data harmonization.
In addition to the essential core elements that all cohorts are required to collect from participants during a specific life stage, the protocol also contains recommended elements. These elements provide data for a deeper investigation into an area. Not all cohorts need to collect data for a recommended element, but if so desired, the measure listed on the protocol should be used for new data collection.
Measures delineated in the protocol include proprietary instruments, other standardized and validated instruments, data collection forms modified from the cohorts, and new instruments.
As part of the protocol development process, the DAC developed the Cohort Measurement Identification Tool (CMIT). For every element in each life stage, each cohort was asked to identify the measure(s) they most recently used and which proposed protocol measure they planned to use for new data collection; Fig. 1a shows the CMIT survey instrument with pages for identifying the relevant life stage (left Panel), the collection of information on the Sleep Health outcome in the relevant life stage (middle Panel) and Stressful Life Events, one of the many potential exposures, in its relevant life stage (right Panel). Figure 1b shows an excerpt from a report template summarizing the Stressful Life Events across cohorts.
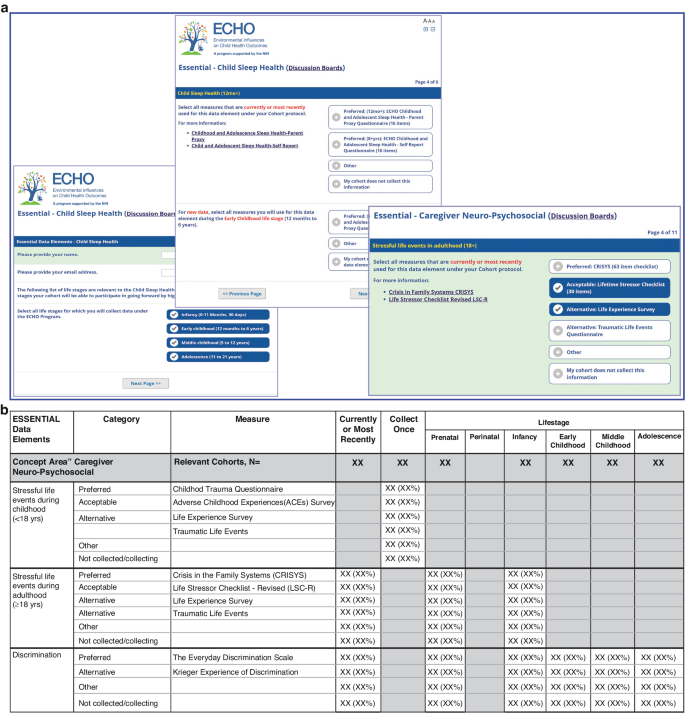
a shows, in select screenshots, how the cohort used the tool to identify the relevant life stage, select the measures that will be used to collect data on Child Sleep Health (an outcome), and on Caregiver Stressful Life Events (an exposures). b is an excerpt from the Cohort Measurement Identification Tool summary report template that demonstrates how the Caregiver Stressful Life Events measures selected by the cohorts were summarized by frequencies across life stages.
Using this information, the Protocol Working Group revised the protocol draft to delete measures that were rarely selected (i.e., measures the cohorts did not plan to use). The responses also identified legacy measures used by multiple cohorts for the Protocol Working Group to consider for inclusion as preferred, acceptable, or alternative measures. Lastly, the responses helped ECHO components to understand the complexities across the cohorts and to prepare for implementation. Figure 2 highlights varied uses of the CMIT tool to evaluate the draft protocol and to begin the development of necessary materials and systems for implementation.
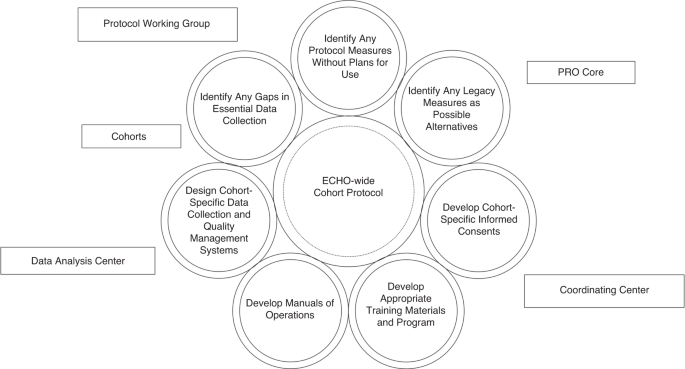
Collaborative uses of the Cohort Measurement Identification Tool (CMIT).
Data systems
The DAC developed highly customized, web-based systems and tools to register cohort participants and classify them according to the extent of their participation in the EWC (e.g., contributing new and extant data versus contributing only extant data); transform data collected in local systems to a format consistent with the CDM; track biospecimen collection, processing, and storage; and capture new data (Fig. 3 ). A tool branded Data Transform allows cohorts to provide all the necessary details (the “roadmap”) to the DAC for converting existing and new data from cohort data systems to the EWC structured-query-language (SQL) server database. The data capture system, based on Research Electronic Data Capture (REDCap) 6 , 7 and named REDCap Central, allows cohorts to directly administer and enter data collected from participants in a secured web-based system. Cohorts can use REDCap Central, a local data capture system, or a hybrid of the two for new data collection (Fig. 3 ). Cohorts using a local data capture system map and transfer new data similarly to extant data.
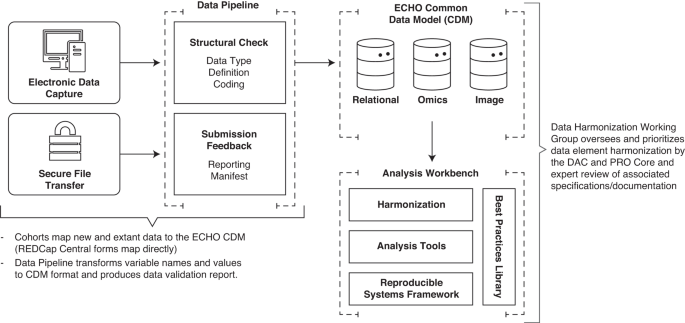
Data systems managed by the Data Analysis Center (DAC).
For new data, each cohort selects its planned structure of visits and protocol measures within appropriate life stages from Data Transform menus. If using REDCap Central, the selected visits and measures within life stages then become visible in the REDCap Central dashboard with the participants loaded from the registration system. In addition to cohorts utilizing REDCap Central for interactive data entry, the DAC developed a survey manager for cohorts to send surveys directly to participants via e-mail. The survey allows cohorts to send a single e-mail for multiple surveys and guides the participant via a custom menu indicating surveys that are completed and surveys remaining to be completed. This advanced remote administration of the measures has become critically important during the period of social isolation due to the COVID-19 pandemic. Another feature that enhances accessibility and utilization of the REDCap Central data capture system is a multilingual support module within REDCap Central that allows cohorts and ECHO participants to toggle between English and Spanish versions of the data collection forms.
Extant data
Since cohorts collected data prior to ECHO, the DAC initially focused on the development of systems to transform and load disparate data to the CDM. These systems and related processes supported early harmonization efforts. Since cohorts would have the best knowledge about their extant data and how they relate to the CDM, the cohorts used Data Transform to: (1) confirm or modify the visit structures that were initially identified using the CMIT tool; (2) select the forms and measures for which they collected data elements in the protocol, either exactly as stated in the CDM or related data for the elements of interest; (3) map their data formats to the formats specified in the CDM data dictionaries or to customized data dictionaries, which the cohorts created based on standardized formatting that would be expected in pipeline processing; and (4) upload their data accordingly.
Prior to the development of the protocol, the DAC administered surveys to the cohorts to gather information about their individual cohort studies and populations, and subsequently placed this information in a metadata catalog. These surveys, administered in modules, ascertained information about the type of data that existed in each cohort (i.e., the domains) according to life stage. The metadata catalog permits faceted browsing and contains advanced search features that facilitate interactive searching and summarization of the metadata by investigators.
On the CMIT survey, the cohorts reported instruments in current use, including any protocol-named measures. The DAC used this information and information that the PRO Core gathered in interviews with some of the cohorts to develop a tool that listed all the forms on the protocol and more than 400 related forms. Cohorts were asked to indicate the forms for which they had existing data. For those forms reported by more than two cohorts, the DAC and PRO Core developed data dictionaries if the form was a standardized instrument. Otherwise, the cohorts submitted customized data dictionaries, which the DAC then reviewed to confirm that their formats adhered to that required by a data pipeline developed by the DAC for standard processing. As of April 2022, a total of 605 customized data dictionaries were in use by cohorts for submission of data. Each of these forms requires data harmonization, reflecting the magnitude of the harmonization effort.
Data harmonization approaches
Many approaches exist for analyzing individual-level data collected with multiple measures. These include: (1) joint latent variable modeling of item-level data using item response theory (IRT), (2) harmonization through identification of commonalities and linkages prior to statistical analysis, (3) central review of cohort-specific data to identify common threads and instrument linking when possible (see indirect harmonization description). Fig. 4 shows how DAC and PRO Core review and harmonize data following their receipt. Other approaches take place during data analysis. Harmonization usually refers to measurement harmonization, which includes linking two or more measures of the same construct, such that a score obtained on one can be expressed as a score on the other(s). However, harmonization can also refer to alignment across studies of types of statistical estimators, functional form of models (e.g., linear vs. polynomial), or sets of predictors and covariates. 8 It is important to keep in mind that there are many ways in which heterogeneity of study features and measures may introduce unwanted variation when attempting cross-study data synthesis. As such, most EWC data analyses require some degree of harmonization.
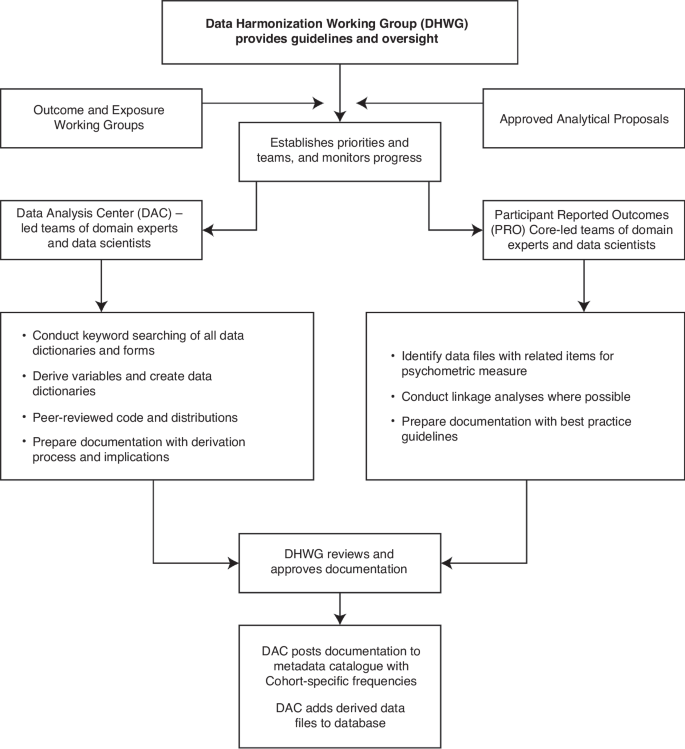
Central data harmonization processes following receipt of data files from the cohorts.
Harmonization at the level of measures can be classified as Direct methods of alignment. An example of a direct method is to transform or standardize scores under an invariant component by first ensuring factorial invariance for each unidimensional construct and using the invariant factor component to perform a transformation that allows some degree of comparability across different scores. Indirect methods make use of the finer distinctions in the data, namely item-level information. Methods for harmonizing item-level data include moderated nonlinear factor analysis 9 and IRT alignment. 10 Test-equating techniques, such as those used in PROsetta Stone ( https://www.prosettastone.org/ ), 11 , 12 , 13 , 14 may be used when the measures share common or overlapping items. Imputation of a missing variable based on prior information is another common approach to harmonization. However, the use of existing correlational data to impute values of missing information assumes that the high correlation observed in other sample(s) is present in the target sample. This assumption may not be the case and should ideally be checked in subsequent confirmatory work. Data harmonization may also require evaluation of heterogeneity across samples or cohorts (e.g., Cochran’s Q). When total scores or T-scores for different PROs can be harmonized to a common metric, analysts can convert scores to the common metric using crosswalk tables and perform individual-level analyses that include all participants with data on the harmonized measures (pooled analysis or mega-analysis 15 , 16 ). While not as precise as item-level analyses, this approach is much simpler to implement and allows all harmonizable data to be used in a single individual-level analysis model.
Indirect method example—harmonizing depression
Depression is a commonly assessed construct in ECHO. We first evaluated measures from multiple cohorts for common item overlap (i.e., the same question on different measures). With the advent of item banks beginning in the 1990s, increasing numbers of shared items can be found across different measures for the same construct and others. For example, stress and anxiety may share items with some depression measures. Linking functions, test-equating algorithms, and co-calibration all require sets of common items. Depression-related items from all the various measures can then be combined into a common dataset and an IRT model that includes a test-equating or linking function uses the overlapping items to first define a common depression metric and secondarily, estimate item location and discrimination parameters based on that underlying metric. Individual person-level scores can then be derived from whatever set of items a given person was administered. For example, using EWC data, a recent publication linked PROMIS® Depression with the Edinburgh Postnatal Depression Scale. 11 Using a dataset in which both questionnaires were administered, the full set of items were calibrated onto a single, unidimensional construct (i.e., ‘depression’). This now allows one to “crosswalk” scores from one to the other, thereby harmonizing analyses where one or another of the questionnaires was used.
Hierarchical example—harmonizing gestational age
Harmonizing some types of variables is more straightforward than harmonizing others. Gestational age can often be directly harmonized without use of complex linking approaches. Some bias, misclassification, and loss of precision will occur when combining estimates based on dating ultrasound scans, last menstrual period, or self-reported information. For gestational age, we include a hierarchy of parameters (Supplementary Table 1 ) used for the estimation and the various sources that were available for an individual. Therefore, analysts may conduct sensitivity analyses by data source to assess the impact on the results, such as when assessing the performance of a placental analyte in maternal serum for predicting an adverse pregnancy outcome.
Simple comparable variable linkage example—harmonizing units or time
Differences in variable units, such as days to weeks, may be easily converted. For example, the number of cigarettes smoked per day can be converted with relative ease to cigarettes smoked per week, although some questions may elicit more precise and accurate counts than other questions. When similarly asked, pooling of data across studies can occur without much concern about measurement differences creating artifacts in results. Similarly with laboratory or analyte results, there are different mathematical algorithms used for standardization by urinary dilution 17 and treating undetectable values for data which have been generated. When distributed approaches are used to generate new data, use of standards and reliability samples, such as blinded duplicates or common sample, are two commonly used approaches for quality assurance and adjustment.
ECHO use of meta-analysis for harmonization
When pooled or mega-analysis 15 , 16 is not possible due to a lack of harmonized measures, meta-analytic techniques may be used (i.e., conduct analyses in clusters of cohorts that used the same measure and then synthesize the results across the clusters). Prior to central availability of EWC data, the DAC provided statistical code to cohorts for implementation and meta-analyzed the results. 18 , 19 This distributed collective analysis 5 is also known as “coordinated analysis,” 20 “parallel analysis,” or “coordinated meta-analysis.” 21 , 22 This type of analysis differs from traditional meta-analysis based on published results since we controlled the statistical methods used by each cohort. With centrally available individual-level data, we may still stratify analyses based on clusters of cohorts and pool the resulting estimates using meta-analysis (e.g., weighted summaries of effect sizes) to synthesize findings while still preserving cohort and measurement heterogeneity. We and others also use additional approaches to address cohort heterogeneity. 23 , 24
Data harmonization working group (DHWG)
The enormity of the data elements in the EWCP, the large number of cohorts, and the related instruments that have been used over time necessitate parallel data harmonization processes in ECHO. The DHWG was responsible for developing EWC data harmonization guidelines to ensure a consistent approach across the program, and best practices for data harmonization, adhering to the principles of fairness, inclusiveness, and accuracy. Investigators from all ECHO components self-selected into this cross-cutting group.
The DHWG prioritized harmonization for: (1) common exposures, (2) primary child health outcomes, and (3) psychological and other latent variables constructed from instruments on the protocol. The DHWG initially asked Outcome and Exposure Working Groups to prioritize five constructs that they envisioned imminently needing and to name individuals from their groups to contribute to these harmonization efforts. The DHWG then established teams and provided templates for data harmonization processes and documentation.
Concurrently, the DAC initiated harmonization of variables needed for approved analyses, and the PRO Core started conducting linking analyses of psychometric measures. The DHWG integrated these lists with the variables prioritized for DHWG teams.
When cohorts could not directly map their data to the CDM, they submitted cohort-specific data files using custom data dictionaries. The DAC developed an R script that uses keywords to systematically search and report on form questions and responses found across all data dictionaries and SQL tables that potentially relate to the data construct. For example, to harmonize data on income, keywords included income, wage, and salary. We searched data tables and the dictionaries since related data may be found in text fields in the data tables and in variable descriptions in the data dictionaries.
Harmonization teams review the reports (variable descriptions, response categories, and data content/text) to determine relevance. For example, to study prenatal opioid use, the word “pain” identified pain medications but also picked up the environmental exposure ‘paint.’ Harmonization requires consideration of all potentially related data. The team reviews the source that contained the identified information since there may be related variables beyond those detected by the keyword search. After determining commonalities, the team derives analytical variables. We incorporate external data, such as reference tables required for normalization and standardization and note their sources in documentation files. Creating derived variables facilitates standardization across analyses. Transparent documentation that describes the process and deliberations facilitates decision-making by subsequent users of the data and reproducibility by other researchers.
Checking the harmonization
Quality assurance of data harmonization assesses accuracy (see example below) and applicability (usefulness for end users). The teams creating the harmonization plans include experts in the subject matter who are familiar with the related body of literature so that the derived variables are of use to the greater scientific community. The DHWG reviews harmonization documents and places them in central locations for review by the ECHO community. The derivations, challenges, and resolutions are peered-reviewed by the DAC statistical team, and distributional properties of derived variables are provided in the documentation. A metadata catalog of the EWC database that is managed by the DAC contains the final harmonization documents, including frequencies of derived variables by cohort so that each cohort may review and confirm for accuracy.
When ECHO teams harmonize instruments that represent nesting or item reduction (e.g., a short form created from a long form), the correlation and scoring are checked by mimicking the reduction within the longer form data file. Graphically, the correlation is examined with scatterplots and the score agreement using Bland–Altman plots. This approach is demonstrated using the Wechsler Intelligence Scale 25 , 26 in the Supplementary material.
Collaborative study designs require data standardization and harmonization. In the EWC, standardization is evident in the creation of the data collection protocol with its manual of procedures, data collection forms, and policies for study practices, including data sharing, data harmonization, and publication. Data standardization using the CDM and derived variables should facilitate timely analyses and reduce errors due to data misuse. However, given the complexity of the EWC, dedicated time is needed for harmonization and derivation of analytic variables. These activities need to be conducted methodically and with transparency to enhance research reproducibility. Establishing a DHWG with membership from across the ECHO Program and having the group define, monitor, and document the data harmonization and standardization process, helps accomplish these goals.
Data availability
A restricted version of the EWC data may be requested from the NICHD Data and Specimen Hub ( https://dash.nichd.nih.gov/study/417122 ; https://doi.org/10.57982/ng1v-pz07 ).
Blaisdell, C. J. et al. The NIH ECHO Program: investigating how early environmental influences affect child health. Pediatr. Res . 92 , 1215–1216 (2021).
LeWinn, K. Z. et al. SPR perspectives: Environmental influences on Child Health Outcomes (ECHO) Program: overcoming challenges to generate engaged, multidisciplinary science. Pediatr. Res . 92 , 1262–1269 (2021).
Romano, M. E. et al. SPR perspectives: scientific opportunities in the Environmental influences on Child Health Outcomes Program. Pediatr. Res . 92 , 1255–1261 (2021).
Lesko, C. R. et al. Collaborative, pooled and harmonized study designs for epidemiologic research: challenges and opportunities. Int J. Epidemiol. 47 , 654–668 (2018).
Article PubMed PubMed Central Google Scholar
Jacobson, L. P., Lau, B., Catellier, D. & Parker, C. B. An Environmental influences on Child Health Outcomes viewpoint of data analysis centers for collaborative study designs. Curr. Opin. Pediatr. 30 , 269–275 (2018).
Harris, P. A. et al. Research electronic data capture (REDCap)—A metadata-driven methodology and workflow process for providing translational research informatics support. J. Biomed. Inf. 42 , 377–381 (2009).
Article Google Scholar
Harris, P. A. et al. The REDCap consortium: building an international community of software partners. J. Biomed. Inf. 95 , 103208 (2019).
Graham, E. K. et al. Coordinated data analysis: Knowledge accumulation in lifespan developmental psychology. Psychol. Aging 37 , 125–135 (2022).
Article PubMed Google Scholar
Gottfredson, N. C. et al. Simplifying the implementation of modern scale scoring methods with an automated R package: automated moderated nonlinear factor analysis (aMNLFA). Addictive Behav. 94 , 65–73 (2019).
Mansolf, M. et al. Extensions of multiple-group item response theory alignment: application to psychiatric phenotypes in an international genomics consortium. Educ. Psychol. Meas. 80 , 870–909 (2020).
Blackwell, C. et al. Developing a common metric for depression across adulthood: linking PROMIS depression with the Edinburgh Postnatal Depression Scale. Psychol. Assess. 33 , 610–618 (2021).
Cella, D. et al. PROsetta Stone®: a method and common metric to link pro measures for comparative effectiveness research (CER). Qual. Life Res. 22 , 32 (2013).
Google Scholar
Choi, S. W., Lim, S., Schalet, B. D., Kaat, A. J. & Cella, D. PROsetta: an R package for linking patient-reported outcome measures. Appl. Psychol. Meas. 45 , 386–388 (2021).
Article CAS PubMed PubMed Central Google Scholar
Choi, S., Schalet, B., Cook, K. & Cella, D. Establishing a common metric for depressive symptoms: linking BDI-II CES-D and PHQ-9 to PROMIS depression. Psychol. Assess. 26 , 513–527 (2014).
McArdle, J. J., Grimm, K. J., Hamagami, F., Bowles, R. P. & Meredith, W. Modeling life-span growth curves of cognition using longitudinal data with multiple samples and changing scales of measurement. Psychol. Methods 14 , 126–149 (2009).
Dodge, H. H. et al. Cohort effects in verbal memory function and practice effects: a population-based study. Int. Psychogeriatr. 29 , 137–148 (2017).
Kuiper, J. et al. Combining urinary biomarker data from studies with different measures of urinary dilution. Epidemiology 33 , 533–540 (2022).
Tylavsky, F. A. et al. Understanding childhood obesity in the US: the NIH environmental influences on child health outcomes (ECHO) program. Int J. Obes. 44 , 617–627 (2020).
Dunlop, A. L. et al. Racial and geographic variation in effects of maternal education and neighborhood-level measures of socioeconomic status on gestational age at birth: findings from the ECHO cohorts. PLoS One 16 , e0245064 (2021).
Hofer, S. M. & Piccinin, A. M. Integrative data analysis through coordination of measurement and analysis protocol across independent longitudinal studies. Psychol. Methods 14 , 150 (2009).
Matsushita, K. et al. Estimated glomerular filtration rate and albuminuria for prediction of cardiovascular outcomes: a collaborative meta-analysis of individual participant data. Lancet Diabetes Endocrinol. 3 , 514–525 (2015).
Siddique, J. et al. Multiple imputation for harmonizing longitudinal non-commensurate measures in individual participant data meta-analysis. Stat. Med. 34 , 3399–3414 (2015).
Article MathSciNet PubMed PubMed Central Google Scholar
Curran, P. J. & Hussong, A. M. Integrative data analysis: the simultaneous analysis of multiple data sets. Psychol. Methods 14 , 81 (2009).
Baker, W. et al. Understanding heterogeneity in meta‐analysis: the role of meta‐regression. Int. J. Clin. Pract. 63 , 1426–1434 (2009).
Article CAS PubMed Google Scholar
Wechsler, D. Manual for the Wechsler Intelligence Scale for Children —3rd edn, (Psychological Corporation, 1991).
Wechsler, D. Wechsler Intelligence Scale for Children –5th Edn Technical And Interpretive Manual. (NCS Pearson, 2014).
Download references
Acknowledgements
The authors wish to thank our ECHO colleagues; the medical, nursing, and program staff; and the children and families participating in the ECHO cohorts.
ECHO research is supported by the Environmental influences on Child Health Outcomes (ECHO) Program, Office of The Director, NIH, under Award Numbers U2COD023375 (Coordinating Center), U24OD023382 (DAC), U24 OD03319 (PRO Core); Cohort awards: UH3 OD023244; UH3 OD023248; UH3 OD023249; UH3 OD023251; UH3 OD023253; UH3 OD023268; UH3 OD023271; UH3 OD023272; UH3 OD023275; UH3 OD023279; UH3 OD023282; UH3 OD023285; UH3 OD023286; UH3 OD023287; UH3 OD023288; UH3 OD023289; UH3 OD023290; UH3 OD023305; UH3 OD023313; UH3 OD023318; UH3 OD023320; UH3 OD023328; UH3 OD023332; UH3 OD023337; UH3 OD023342; UH3 OD023344; UH3 OD023347; UH3 OD023348; UH3 OD023349; UH3 OD023365; UH3 OD023389; Laboratories: U24 ES026539. U2C ES026533; U2C ES026542; U2C ES030859; U2C ES030857; U2C ES026555; U2C ES026561; U2C ES030851; U2C OD023375; IDeA States Pediatric Clinical Trial Network: U24 OD024957-02; UG1 OD024943-02; UG1 OD024945-02; UG1 OD024947-02; UG1 OD024954-02; UG1 OD024958-02; UG1 OD024952-02; UG1 OD024956-02; UG1 OD024946-02; UG1 OD024951-02; UG1 OD024959-02; UG1 OD024948-02; UG1 OD024950-02; UG1 OD024953-02; UG1 OD024955-02; UG1 OD024942-02; UG1 OD024944-02; and UG1 OD024949-02. L.P.J. and C.B.P. were supported by U24 OD023382, D.C. was supported by U24 OD023319, B.M.L. was supported by UH3 OD023347, and D.K.M. was supported by U24 OD023319, R01-AG067622, R01-AG064006 and P30AG059988. The content is solely the responsibility of the authors and does not necessarily represent the official views of the NIH.
Author information
Authors and affiliations.
Department of Epidemiology, Johns Hopkins University Bloomberg School of Public Health, Baltimore, MD, USA
Lisa P. Jacobson
Research Triangle Institute, Research Triangle Park, NC, USA
Corette B. Parker
Department of Medical Social Sciences, Northwestern University, Chicago, IL, USA
David Cella & Daniel K. Mroczek
Department of Psychology, Northwestern University, Evanston, IL, USA
Daniel K. Mroczek
Department of Psychiatry & Human Behavior, Alpert Medical School of Brown University, Providence, RI, USA
Barry M. Lester
Department of Pediatrics, Alpert Medical School of Brown University, Providence, RI, USA
Coordinating Center: Duke Clinical Research Institute, Durham, NC, USA
P. B. Smith & K. L. Newby
Research Triangle Institute, Durham, NC, USA
D. J. Catellier
Person-Reported Outcomes Core: Northwestern University, Evanston, IL, USA
R. Gershon & D. Cella
You can also search for this author in PubMed Google Scholar
- P. B. Smith
- , K. L. Newby
- , Lisa P. Jacobson
- , D. J. Catellier
- , R. Gershon
- & D. Cella
Contributions
All authors contributed to the conception and design of this manuscript and participated in its drafting. All authors have provided final approval of the version submitted for publication.
Corresponding author
Correspondence to Lisa P. Jacobson .
Ethics declarations
Competing interests.
The authors declare no competing interests.
Additional information
Publisher’s note Springer Nature remains neutral with regard to jurisdictional claims in published maps and institutional affiliations.
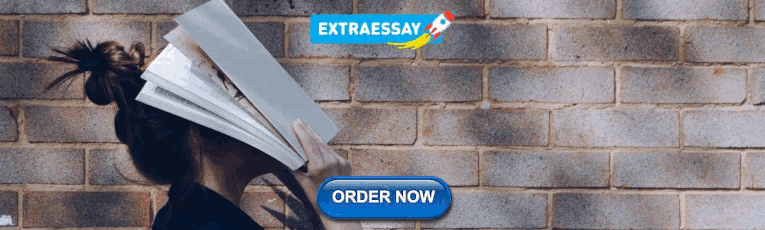
Supplementary information
Supplementary figure, supplementary text, supplementary table 1, rights and permissions.
Open Access This article is licensed under a Creative Commons Attribution 4.0 International License, which permits use, sharing, adaptation, distribution and reproduction in any medium or format, as long as you give appropriate credit to the original author(s) and the source, provide a link to the Creative Commons licence, and indicate if changes were made. The images or other third party material in this article are included in the article’s Creative Commons licence, unless indicated otherwise in a credit line to the material. If material is not included in the article’s Creative Commons licence and your intended use is not permitted by statutory regulation or exceeds the permitted use, you will need to obtain permission directly from the copyright holder. To view a copy of this licence, visit http://creativecommons.org/licenses/by/4.0/ .
Reprints and permissions
About this article
Cite this article.
Jacobson, L.P., Parker, C.B., Cella, D. et al. Approaches to protocol standardization and data harmonization in the ECHO-wide cohort study. Pediatr Res (2024). https://doi.org/10.1038/s41390-024-03039-0
Download citation
Received : 30 January 2023
Revised : 07 December 2023
Accepted : 13 December 2023
Published : 16 February 2024
DOI : https://doi.org/10.1038/s41390-024-03039-0
Share this article
Anyone you share the following link with will be able to read this content:
Sorry, a shareable link is not currently available for this article.
Provided by the Springer Nature SharedIt content-sharing initiative
Quick links
- Explore articles by subject
- Guide to authors
- Editorial policies

- Privacy Policy
Buy Me a Coffee

Home » Data Collection – Methods Types and Examples
Data Collection – Methods Types and Examples
Table of Contents

Data Collection
Definition:
Data collection is the process of gathering and collecting information from various sources to analyze and make informed decisions based on the data collected. This can involve various methods, such as surveys, interviews, experiments, and observation.
In order for data collection to be effective, it is important to have a clear understanding of what data is needed and what the purpose of the data collection is. This can involve identifying the population or sample being studied, determining the variables to be measured, and selecting appropriate methods for collecting and recording data.
Types of Data Collection
Types of Data Collection are as follows:
Primary Data Collection
Primary data collection is the process of gathering original and firsthand information directly from the source or target population. This type of data collection involves collecting data that has not been previously gathered, recorded, or published. Primary data can be collected through various methods such as surveys, interviews, observations, experiments, and focus groups. The data collected is usually specific to the research question or objective and can provide valuable insights that cannot be obtained from secondary data sources. Primary data collection is often used in market research, social research, and scientific research.
Secondary Data Collection
Secondary data collection is the process of gathering information from existing sources that have already been collected and analyzed by someone else, rather than conducting new research to collect primary data. Secondary data can be collected from various sources, such as published reports, books, journals, newspapers, websites, government publications, and other documents.
Qualitative Data Collection
Qualitative data collection is used to gather non-numerical data such as opinions, experiences, perceptions, and feelings, through techniques such as interviews, focus groups, observations, and document analysis. It seeks to understand the deeper meaning and context of a phenomenon or situation and is often used in social sciences, psychology, and humanities. Qualitative data collection methods allow for a more in-depth and holistic exploration of research questions and can provide rich and nuanced insights into human behavior and experiences.
Quantitative Data Collection
Quantitative data collection is a used to gather numerical data that can be analyzed using statistical methods. This data is typically collected through surveys, experiments, and other structured data collection methods. Quantitative data collection seeks to quantify and measure variables, such as behaviors, attitudes, and opinions, in a systematic and objective way. This data is often used to test hypotheses, identify patterns, and establish correlations between variables. Quantitative data collection methods allow for precise measurement and generalization of findings to a larger population. It is commonly used in fields such as economics, psychology, and natural sciences.
Data Collection Methods
Data Collection Methods are as follows:
Surveys involve asking questions to a sample of individuals or organizations to collect data. Surveys can be conducted in person, over the phone, or online.
Interviews involve a one-on-one conversation between the interviewer and the respondent. Interviews can be structured or unstructured and can be conducted in person or over the phone.
Focus Groups
Focus groups are group discussions that are moderated by a facilitator. Focus groups are used to collect qualitative data on a specific topic.
Observation
Observation involves watching and recording the behavior of people, objects, or events in their natural setting. Observation can be done overtly or covertly, depending on the research question.
Experiments
Experiments involve manipulating one or more variables and observing the effect on another variable. Experiments are commonly used in scientific research.
Case Studies
Case studies involve in-depth analysis of a single individual, organization, or event. Case studies are used to gain detailed information about a specific phenomenon.
Secondary Data Analysis
Secondary data analysis involves using existing data that was collected for another purpose. Secondary data can come from various sources, such as government agencies, academic institutions, or private companies.
How to Collect Data
The following are some steps to consider when collecting data:
- Define the objective : Before you start collecting data, you need to define the objective of the study. This will help you determine what data you need to collect and how to collect it.
- Identify the data sources : Identify the sources of data that will help you achieve your objective. These sources can be primary sources, such as surveys, interviews, and observations, or secondary sources, such as books, articles, and databases.
- Determine the data collection method : Once you have identified the data sources, you need to determine the data collection method. This could be through online surveys, phone interviews, or face-to-face meetings.
- Develop a data collection plan : Develop a plan that outlines the steps you will take to collect the data. This plan should include the timeline, the tools and equipment needed, and the personnel involved.
- Test the data collection process: Before you start collecting data, test the data collection process to ensure that it is effective and efficient.
- Collect the data: Collect the data according to the plan you developed in step 4. Make sure you record the data accurately and consistently.
- Analyze the data: Once you have collected the data, analyze it to draw conclusions and make recommendations.
- Report the findings: Report the findings of your data analysis to the relevant stakeholders. This could be in the form of a report, a presentation, or a publication.
- Monitor and evaluate the data collection process: After the data collection process is complete, monitor and evaluate the process to identify areas for improvement in future data collection efforts.
- Ensure data quality: Ensure that the collected data is of high quality and free from errors. This can be achieved by validating the data for accuracy, completeness, and consistency.
- Maintain data security: Ensure that the collected data is secure and protected from unauthorized access or disclosure. This can be achieved by implementing data security protocols and using secure storage and transmission methods.
- Follow ethical considerations: Follow ethical considerations when collecting data, such as obtaining informed consent from participants, protecting their privacy and confidentiality, and ensuring that the research does not cause harm to participants.
- Use appropriate data analysis methods : Use appropriate data analysis methods based on the type of data collected and the research objectives. This could include statistical analysis, qualitative analysis, or a combination of both.
- Record and store data properly: Record and store the collected data properly, in a structured and organized format. This will make it easier to retrieve and use the data in future research or analysis.
- Collaborate with other stakeholders : Collaborate with other stakeholders, such as colleagues, experts, or community members, to ensure that the data collected is relevant and useful for the intended purpose.
Applications of Data Collection
Data collection methods are widely used in different fields, including social sciences, healthcare, business, education, and more. Here are some examples of how data collection methods are used in different fields:
- Social sciences : Social scientists often use surveys, questionnaires, and interviews to collect data from individuals or groups. They may also use observation to collect data on social behaviors and interactions. This data is often used to study topics such as human behavior, attitudes, and beliefs.
- Healthcare : Data collection methods are used in healthcare to monitor patient health and track treatment outcomes. Electronic health records and medical charts are commonly used to collect data on patients’ medical history, diagnoses, and treatments. Researchers may also use clinical trials and surveys to collect data on the effectiveness of different treatments.
- Business : Businesses use data collection methods to gather information on consumer behavior, market trends, and competitor activity. They may collect data through customer surveys, sales reports, and market research studies. This data is used to inform business decisions, develop marketing strategies, and improve products and services.
- Education : In education, data collection methods are used to assess student performance and measure the effectiveness of teaching methods. Standardized tests, quizzes, and exams are commonly used to collect data on student learning outcomes. Teachers may also use classroom observation and student feedback to gather data on teaching effectiveness.
- Agriculture : Farmers use data collection methods to monitor crop growth and health. Sensors and remote sensing technology can be used to collect data on soil moisture, temperature, and nutrient levels. This data is used to optimize crop yields and minimize waste.
- Environmental sciences : Environmental scientists use data collection methods to monitor air and water quality, track climate patterns, and measure the impact of human activity on the environment. They may use sensors, satellite imagery, and laboratory analysis to collect data on environmental factors.
- Transportation : Transportation companies use data collection methods to track vehicle performance, optimize routes, and improve safety. GPS systems, on-board sensors, and other tracking technologies are used to collect data on vehicle speed, fuel consumption, and driver behavior.
Examples of Data Collection
Examples of Data Collection are as follows:
- Traffic Monitoring: Cities collect real-time data on traffic patterns and congestion through sensors on roads and cameras at intersections. This information can be used to optimize traffic flow and improve safety.
- Social Media Monitoring : Companies can collect real-time data on social media platforms such as Twitter and Facebook to monitor their brand reputation, track customer sentiment, and respond to customer inquiries and complaints in real-time.
- Weather Monitoring: Weather agencies collect real-time data on temperature, humidity, air pressure, and precipitation through weather stations and satellites. This information is used to provide accurate weather forecasts and warnings.
- Stock Market Monitoring : Financial institutions collect real-time data on stock prices, trading volumes, and other market indicators to make informed investment decisions and respond to market fluctuations in real-time.
- Health Monitoring : Medical devices such as wearable fitness trackers and smartwatches can collect real-time data on a person’s heart rate, blood pressure, and other vital signs. This information can be used to monitor health conditions and detect early warning signs of health issues.
Purpose of Data Collection
The purpose of data collection can vary depending on the context and goals of the study, but generally, it serves to:
- Provide information: Data collection provides information about a particular phenomenon or behavior that can be used to better understand it.
- Measure progress : Data collection can be used to measure the effectiveness of interventions or programs designed to address a particular issue or problem.
- Support decision-making : Data collection provides decision-makers with evidence-based information that can be used to inform policies, strategies, and actions.
- Identify trends : Data collection can help identify trends and patterns over time that may indicate changes in behaviors or outcomes.
- Monitor and evaluate : Data collection can be used to monitor and evaluate the implementation and impact of policies, programs, and initiatives.
When to use Data Collection
Data collection is used when there is a need to gather information or data on a specific topic or phenomenon. It is typically used in research, evaluation, and monitoring and is important for making informed decisions and improving outcomes.
Data collection is particularly useful in the following scenarios:
- Research : When conducting research, data collection is used to gather information on variables of interest to answer research questions and test hypotheses.
- Evaluation : Data collection is used in program evaluation to assess the effectiveness of programs or interventions, and to identify areas for improvement.
- Monitoring : Data collection is used in monitoring to track progress towards achieving goals or targets, and to identify any areas that require attention.
- Decision-making: Data collection is used to provide decision-makers with information that can be used to inform policies, strategies, and actions.
- Quality improvement : Data collection is used in quality improvement efforts to identify areas where improvements can be made and to measure progress towards achieving goals.
Characteristics of Data Collection
Data collection can be characterized by several important characteristics that help to ensure the quality and accuracy of the data gathered. These characteristics include:
- Validity : Validity refers to the accuracy and relevance of the data collected in relation to the research question or objective.
- Reliability : Reliability refers to the consistency and stability of the data collection process, ensuring that the results obtained are consistent over time and across different contexts.
- Objectivity : Objectivity refers to the impartiality of the data collection process, ensuring that the data collected is not influenced by the biases or personal opinions of the data collector.
- Precision : Precision refers to the degree of accuracy and detail in the data collected, ensuring that the data is specific and accurate enough to answer the research question or objective.
- Timeliness : Timeliness refers to the efficiency and speed with which the data is collected, ensuring that the data is collected in a timely manner to meet the needs of the research or evaluation.
- Ethical considerations : Ethical considerations refer to the ethical principles that must be followed when collecting data, such as ensuring confidentiality and obtaining informed consent from participants.
Advantages of Data Collection
There are several advantages of data collection that make it an important process in research, evaluation, and monitoring. These advantages include:
- Better decision-making : Data collection provides decision-makers with evidence-based information that can be used to inform policies, strategies, and actions, leading to better decision-making.
- Improved understanding: Data collection helps to improve our understanding of a particular phenomenon or behavior by providing empirical evidence that can be analyzed and interpreted.
- Evaluation of interventions: Data collection is essential in evaluating the effectiveness of interventions or programs designed to address a particular issue or problem.
- Identifying trends and patterns: Data collection can help identify trends and patterns over time that may indicate changes in behaviors or outcomes.
- Increased accountability: Data collection increases accountability by providing evidence that can be used to monitor and evaluate the implementation and impact of policies, programs, and initiatives.
- Validation of theories: Data collection can be used to test hypotheses and validate theories, leading to a better understanding of the phenomenon being studied.
- Improved quality: Data collection is used in quality improvement efforts to identify areas where improvements can be made and to measure progress towards achieving goals.
Limitations of Data Collection
While data collection has several advantages, it also has some limitations that must be considered. These limitations include:
- Bias : Data collection can be influenced by the biases and personal opinions of the data collector, which can lead to inaccurate or misleading results.
- Sampling bias : Data collection may not be representative of the entire population, resulting in sampling bias and inaccurate results.
- Cost : Data collection can be expensive and time-consuming, particularly for large-scale studies.
- Limited scope: Data collection is limited to the variables being measured, which may not capture the entire picture or context of the phenomenon being studied.
- Ethical considerations : Data collection must follow ethical principles to protect the rights and confidentiality of the participants, which can limit the type of data that can be collected.
- Data quality issues: Data collection may result in data quality issues such as missing or incomplete data, measurement errors, and inconsistencies.
- Limited generalizability : Data collection may not be generalizable to other contexts or populations, limiting the generalizability of the findings.
About the author
Muhammad Hassan
Researcher, Academic Writer, Web developer
You may also like

Delimitations in Research – Types, Examples and...

Research Process – Steps, Examples and Tips

Research Design – Types, Methods and Examples

Institutional Review Board – Application Sample...

Evaluating Research – Process, Examples and...

Research Questions – Types, Examples and Writing...

An official website of the United States government
The .gov means it’s official. Federal government websites often end in .gov or .mil. Before sharing sensitive information, make sure you’re on a federal government site.
The site is secure. The https:// ensures that you are connecting to the official website and that any information you provide is encrypted and transmitted securely.
- Publications
- Account settings
Preview improvements coming to the PMC website in October 2024. Learn More or Try it out now .
- Advanced Search
- Journal List
- HHS Author Manuscripts

Commonly Utilized Data Collection Approaches in Clinical Research
Jane s. saczynski.
1 Department of Medicine, University of Massachusetts Medical School, Worcester, MA
2 Department of Quantitative Health Sciences, University of Massachusetts Medical School, Worcester, MA
David D. McManus
Robert j. goldberg.
In this article we provide an overview of the different data collection approaches that are commonly utilized in carrying out clinical, public health, and translational research. We discuss several of the factors researchers need to consider in using data collected in questionnaire surveys, from proxy informants, through the review of medical records, and in the collection of biologic samples. We hope that the points raised in this overview will lead to the collection of rich and high quality data in observational studies and randomized controlled trials.
Collecting Meaningful Data in a Clinical Research Study
In a recent editorial, we described the different types of observational studies and randomized controlled trial designs that investigators often utilize in carrying out clinical and public health research 1 . Although two of the most important steps in successfully carrying out a research project are the clear formulation of key testable hypotheses and careful selection of a cost-efficient, rigorous study design, less information is available for researchers with respect to contemporary methods of high quality and reliable data collection. With increasing attention being paid to patient-reported outcomes in observational, comparative effectiveness, and clinical trials research, data collection approaches that combine medical record abstraction, patient interviews, and administrative data will be more commonly utilized in the future.
In the present editorial, we discuss a number of issues that pertain to the collection of high-quality data in the conduct of clinical, translational, and epidemiologic research projects and ways to enhance the collection of reliable and meaningful data. We also discuss issues related to the accuracy of these data, and factors to consider in the possible independent confirmation of information collected from different data sources. The data collection instruments reviewed include questionnaire surveys and patient self-reported data; use of proxy/informant information; hospital and ambulatory medical records; and analysis of biologic materials.
1. Questionnaire Surveys and Patient Reported Data
Much of the information collected in observational epidemiologic studies is collected in the form of patient/participant self-reports on standardized questionnaires which are either self or interviewer administered in person, by phone, or via mail or the internet. The factors on which information is routinely collected in these studies include socio-demographic characteristics, lifestyle practices, medical history, and use of prescribed and/or over the counter medications. Questions are also often asked about participant’s knowledge and attitudes toward various lifestyle and disease predisposing factors. With increasing attention being paid to patient reported outcomes by funding agencies such as the National Institutes of Health (NIH), Agency for Healthcare Research and Quality (AHRQ), and the newly formed Patient-Centered Outcomes Research Institute (PCORI), measures of patient-centered factors such as Quality of Life (QoL), depression, anxiety, cognitive, and functional status are increasingly included in these surveys. The CONSORT (consolidated standards of reporting trials) Statement was recently updated to include standards for reporting patient reported outcomes in randomized controlled trials, highlighting the increasing awareness of the inclusion of such measures as key outcomes of these rigorous investigations 2 .
Patient reported outcomes are ideally measured using standardized, validated instruments to promote the collection of high-quality data and allow for meaningful comparisons across observational studies or randomized trials. Use of standardized assessments also facilitates pooling of data across studies with the goal of establishing clinically relevant cut-points or clinically meaningful change in important patient related outcomes in response to a lifestyle intervention or medical treatment. Recent federally funded initiatives, such as the NIH Toolbox ( www.nihtoolbox.org ) and Patient Reported Outcomes Measurement Information System (PROMISE) ( www.nihpromis.org ), have highlighted the importance of harmonization of patient reported outcomes data collection instruments.
Surveyed individuals are typically asked to respond to these questions in either a yes/no manner, on a Likert type scale (e.g., very often - not at all often), or with open-ended responses. The choice of responses is dictated by the investigator, and, by of course, the standardized instrument (if one is used). The selection of the type of response desired is often made on the basis of the difficulty of the question asked and the depth of knowledge and level of precision the investigator would like to have about a particular factor.
Standardized instruments often have different forms that vary in length, so an investigator can decide whether a ‘long’ (e.g. SF-36) 3 , 4 or ‘short’ (SF-12) version is best suited for their study. Tests with multiple length versions typically have published psychometric properties (e.g., sensitivity and specificity of screening tests) which guide investigators in choosing a test version. For example, a consenting study participant might be asked a series of questions about their level of physical activity, either in the present or during a recent period of pertinent exposure. The number and depth of these questions would be determined, in part, by how this variable would be used in subsequent analyses and presented in peer reviewed publications. If the factor of physical activity was to be simply used as a controlling variable in either stratified or multivariable adjusted regression analyses, then a briefer assessment of physical activity might be more acceptable with the added benefit of reduced respondent burden. On the other hand, if an investigator is particularly interested in the role of type of aerobic activity, level of exercise intensity, or duration of physical activity, then a more extensive battery of questions might be asked about this factor with objective validation of self-reported activity carried out or a standardized instrument used.
Although the use of validated, standardized instruments is preferred, these data collection tools are not always available. If standardized instruments do not exist for a specific construct to be measured, investigators will often create ‘home-grown’ scales. It is extremely important to carefully design these home-grown instruments, ideally with the input of a psychometrician, and to pilot test all measures before using them in a formal research study. Ideally, these pilot efforts would involve validation of the instrument against a ‘gold-standard’ (e.g., clinical diagnosis) or important study outcome. One needs to carefully balance the need for independent validation of participant responses, and the attendant costs and logistical issues associated with such, versus simply discussing the lack of validation of certain variables as a study limitation. These decisions should be discussed with a senior, experienced mentor who has been involved in observational clinical research studies or randomized trials for many years. The advantages and disadvantages of questionnaire data are summarized in Table 1 .
Advantages and Disadvantages of Questionnaire Survey Data
2. Proxy/Informant Data
The collection of information about study participants through the use of proxy respondents can be one of the more challenging tasks for an investigator. Moreover, the accuracy/validity of the proxy’s responses, and their extent of knowledge about various health related aspects of the study participant needs to be thoughtfully considered in determining the type and quantity of information to be elicited from the proxy respondent. On the other hand, especially in observational studies where the cases or controls in a retrospective study may have died or may not be capable of/competent to provide their own responses, information from proxies may be the only source of data available. In some situations, informant perspectives are important data elements, even if different from that of the patient. For instance, family member reports of the type and amount of assistance a patient requires with activities of daily living may be qualitatively different, but equally important, as that reported by the patient.
Informal caregivers are increasingly being recognized as ‘stakeholders’ in many research studies, particularly those that focus on patient reported outcomes such as quality of life. In cases of questionable mental status, or non-communicative state of a patient, informants can be very helpful and important in providing information to help establish a ‘baseline’ for a patient. In these situations, informants can report on the patient’s level of cognitive and physical function as well as level of independence, important outcomes in many contemporary clinical research studies. For some domains, validated informant questionnaires exist. For instance, the Informant Questionnaire on Cognitive Decline in the Elderly is an informant measure of cognitive function and informant responses on the SF-36 and activities of daily living 5 and these scales have been used as assessments of health related quality of life and functional status with varying results 6 , 7 .
3. Review of Ambulatory or Hospital Medical Records
Due to its ubiquity, and the abundance of high-quality data embedded within it, a commonly used source of information in clinical research studies is the medical record. Information contained in hospital or ambulatory care records may be used either as the sole source of data, or complementary to other instruments used to elicit information. Decisions about the adequacy of using the medical record as the sole or main source of data for a given study hinges on the investigator’s hypotheses, study sample size, budget and timeline, as well as the extent and type of data available in a given record system. Medical records can be important sources of information that can reliably document participants’ medical history, clinical, laboratory, or physiologic profile at varying time points in a cost-efficient manner. On the other hand, the data contained in medical records can be frustrating to use and, in some cases, conflicting or of questionable accuracy, due to the non-standardized manner in which this information is collected, recorded, and/or abstracted by various health care professionals and members of research teams. The increasing use of electronic medical records and their merger with administrative data has eased data abstraction efforts and, with increasing use of standardized data entry sets, reduced data heterogeneity.
One major limitation of using the medical record as a primary data source is that potentially important patient reported information is often lacking, which is typically limited to the reporting of a “chief complaint” or symptoms directly related to the present complaint. If clinical information is stigmatized (e.g., sexual history, alcohol or drug use), or difficult to systematically assess in primary care settings (e.g., cognitive status, depression), it is often under-reported in the medical record. It is also important to note that factors (e.g., medication use) are defined by clinicians, not by trained study staff or study participants, and certain variables may not be accurately coded. Moreover, the extent of documentation about key medical history or clinical variables can vary widely between providers (including conflicting data) and health care systems. Heterogeneity can create considerable difficulties in either the construction of key study variables or in their use.
For example, in studying a purported association between macular degeneration and a number of different dietary components, it would be important to document the presence of various medical history conditions which may affect an individual’s dietary practices as well as the development of macular degeneration. In this example, we would be particularly interested in ascertaining the presence of a history of type 2 diabetes mellitus based on information contained in medical records. Inasmuch, one needs to consider how this condition and related chronic medical conditions would be classified based on information contained in medical records. For example, is diabetes considered present if there is a simple notation of this condition in the patient’s medical history by a sole provider? On the other hand, might there be a need for the documentation of various key elements of each condition to be noted in the medical records (e.g., multiple elevated serum glucose levels obtained under fasting conditions) before a diagnosis of diabetes can be accepted? For several relatively common conditions, such as heart failure and stroke, independently and extensively validated algorithms have been developed to ascertain the presence of these important chronic diseases 8 – 10 .
Depending on the major research questions under study, resources available, and amount of variability/precision willing to be accepted in documenting the presence (or equally importantly absence) of each of these comorbid conditions, rules of acceptance and rejection can be applied in the consideration of these factors. Similarly, the investigator might also decide to simply ask the survey participant whether or not diabetes had been ever diagnosed in their past. This should be a very simple thing to do but the investigator needs to have considered beforehand how they will analyze the data if personal responses are not consistent with their medical record findings. Table 2 summarizes the advantages and disadvantages of using medical records.
Advantages and Disadvantages of Hospital/Ambulatory Care Records
4. Collection of Biologic Material
An increasing number and array of contemporary clinical and translational research investigations involve the collection of biologic samples from study participants. These include personal factors such as hair, saliva, urine, and serum. Biologic samples are increasingly being used to profile participants metabolic, proteomic, or genomic status and, thereby, better understand their underlying pathophysiology or their response to a treatment or disease. Although it is beyond the scope of the present manuscript, the ethical implications of genetic research warrant special thought and consideration. Furthermore, various imaging modalities (e.g., computed tomographic or magnetic resonance imaging, nuclear scans, ultrasonography) are being used to obtain deeper insights into underlying anatomic, pathologic, and biologic mechanisms involved in the development of disease, its prognosis, or response to treatment and suggest areas of future research endeavor.
Despite the important information these biologic samples provide into disease, its various causes, and natural history, there are a number of factors to consider in the collection of biologic materials ( Table 3 ). One important factor to consider when obtaining biologic samples is the frequency of collection (often a balance between participant burden and pathophysiologic insights gained from the ability to assess change in a factor over time), timing of specimen collection (especially when this biologic variable has been shown to exhibit circadian variation), cost (both to the participant and investigator in terms of invasiveness and complexity, respectively), variability in test measurement (often presented as a coefficient of variation), and careful need for standardization of test methods and their interpretation (e.g., referencing vs. a “gold-standard”).
Advantages and Disadvantages of Biologic Data
For example, an investigator may be contemplating carrying out a prospective study of racial differences in serum biomarkers and echocardiographic determinants of atrial fibrillation. In addition to the collection of clinical and demographic historical information, a baseline echocardiogram and serum levels of various biomarkers, such as B-type natriuretic peptide, are to be assessed. Investigators need to balance the need for further information with regards to changes in each of these parameters leading to atrial fibrillation with participant burden.
Based on the current literature and existing clinical knowledge, the investigators in this study would need to know how much echocardiographic atrial size and B-type natriuretic peptides change over key periods of time in patients with, or at risk for, atrial fibrillation. These concerns need to be built into data collection efforts and need for systematic assessment of serial changes in these factors. Depending on the degree of change in these parameters, this might entail the collection of serial echocardiograms every 2 years, every 4 years, or more often, such as every 3 months, depending on the extent of change in left atrial size that might predispose an individual to the development of atrial fibrillation. On the other hand, since there may be more volatility and/or change in the serum biomarkers being examined, more frequent blood assays may be required and balanced with participant’s willingness to return to the clinic and associated discomfort/burden. Inasmuch, compromises in the intensity of data collection efforts need to be balanced with patient related concerns and the importance of keeping high rates of retention in a long-term longitudinal study.
Another major consideration with respect to the use of biologic data is when such samples are obtained relative to the definition of key study variables and outcomes (e.g., are they concurrent or separated by considerable time). The importance of timing of the collection of various descriptive or risk factors is illustrated by the following example. An investigator wants to perform metabolomic profiling to examine differences between hepatic and circulating levels of a certain factor. In order to obtain in vivo hepatic tissue samples, he/she performs the investigation using patients undergoing a hepatic biopsy. However, he/she obtains blood samples in the pre-surgical holding area at the time of IV placement in order to minimize participant inconvenience. This study could be undermined, however, should the metabolomic profile of the liver be influenced by medications administered for procedural sedation, thereby confounding any comparisons between hepatic and circulating levels of factors of primary interest.
Storage of biologic samples, as well as technical factors relating to their measurement, also warrant special consideration when interpreting or performing studies involving biologic specimens.
Summary and Overview
There are a number of factors to consider in deciding which data, and amount of data, are to be collected in any clinical research investigation. Investigators often believe that “more is better”, and that it is important to collect information on as many scientifically “interesting” factors as possible. This premise may be misguided and place an unnecessary burden on study participants as well as lead to the collection of considerable data that would never be utilized, analyzed, or presented in a scientific publication.
It is often very useful and time well spent to identify those data elements that are essential from those that are academically “interesting”, but may not be considered central to the key study hypothesis; this will greatly assist in narrowing down one’s study questions and collecting data in as timely and rigorous a manner as possible. Moreover, it helps to create a list of the 5–10 major papers that might result from one’s proposed research study and create an analysis plan for each manuscript. By doing so, you will be able to separate the “data wheat” from the “data chaff” and hone in on those questions of key relevance and the data elements that comprise these variables.
One needs to also carefully think about the independent validation of any self-reported responses and how intrusive, costly, and potentially burdensome this process may be. Validation of one’s data, while important, can be a tricky and cumbersome route to follow with its attendant logistical and staffing complexities.
Acknowledgments
Funding support for this research was provided by the National Institutes of Health (RO1 HL35434). Partial salary support for Drs. Saczynski, McManus, and Goldberg was provided for by the National Institutes of Health grant 1U01HL105268-01. Dr. Saczynski was supported in part by funding from the National Institute on Aging (K01 AG33643).
There are no conflicts of interests for any of the authors on this manuscript. All authors has access to the data and a role in writing the manuscript.
Publisher's Disclaimer: This is a PDF file of an unedited manuscript that has been accepted for publication. As a service to our customers we are providing this early version of the manuscript. The manuscript will undergo copyediting, typesetting, and review of the resulting proof before it is published in its final citable form. Please note that during the production process errors may be discovered which could affect the content, and all legal disclaimers that apply to the journal pertain.
Log in using your username and password
- Search More Search for this keyword Advanced search
- Latest content
- For authors
- Browse by collection
- BMJ Journals More You are viewing from: Google Indexer
You are here
- Volume 7, Issue 9
- Protocol for a qualitative study exploring perspectives on the INternational CLassification of Diseases (11th revision); Using lived experience to improve mental health Diagnosis in NHS England: INCLUDE study
- Article Text
- Article info
- Citation Tools
- Rapid Responses
- Article metrics

- Corinna Hackmann 1 ,
- Amanda Green 1 ,
- Caitlin Notley 2 ,
- Amorette Perkins 1 ,
- Geoffrey M Reed 3 ,
- Joseph Ridler 1 ,
- Jon Wilson 1 , 2 ,
- Tom Shakespeare 2
- 1 Department of Research and Development , Norfolk and Suffolk NHS Foundation Trust, Hellesdon Hospital , Norwich , UK
- 2 Department of Clinical Psychology , Norwich Medical School, University of East Anglia , Norwich , UK
- 3 Department of Psychiatry , Global Mental Health Program, Columbia University Medical Centre , New York , New York , USA
- Correspondence to Dr Corinna Hackmann; Corinna.hackmann{at}nsft.nhs.uk
Introduction Developed in dialogue with WHO, this research aims to incorporate lived experience and views in the refinement of the International Classification of Diseases Mental and Behavioural Disorders 11th Revision (ICD-11). The validity and clinical utility of psychiatric diagnostic systems has been questioned by both service users and clinicians, as not all aspects reflect their lived experience or are user friendly. This is critical as evidence suggests that diagnosis can impact service user experience, identity, service use and outcomes. Feedback and recommendations from service users and clinicians should help minimise the potential for unintended negative consequences and improve the accuracy, validity and clinical utility of the ICD-11.
Methods and analysis The name INCLUDE reflects the value of expertise by experience as all aspects of the proposed study are co-produced. Feedback on the planned criteria for the ICD-11 will be sought through focus groups with service users and clinicians. The data from these groups will be coded and inductively analysed using a thematic analysis approach. Findings from this will be used to form the basis of co-produced recommendations for the ICD-11. Two service user focus groups will be conducted for each of these diagnoses: Personality Disorder, Bipolar I Disorder, Schizophrenia, Depressive Disorder and Generalised Anxiety Disorder. There will be four focus groups with clinicians (psychiatrists, general practitioners and clinical psychologists).
Ethics and dissemination This study has received ethical approval from the Coventry and Warwickshire HRA Research Ethics Committee (16/WM/0479). The output for the project will be recommendations that reflect the views and experiences of experts by experience (service users and clinicians). The findings will be disseminated via conferences and peer-reviewed publications. As the ICD is an international tool, the aim is for the methodology to be internationally disseminated for replication by other groups.
Trial registration number ClinicalTrials.gov: NCT03131505 .
- International Classification of Diseases
- Personality Disorders
- Anxiety Disorders
This is an Open Access article distributed in accordance with the Creative Commons Attribution Non Commercial (CC BY-NC 4.0) license, which permits others to distribute, remix, adapt, build upon this work non-commercially, and license their derivative works on different terms, provided the original work is properly cited and the use is non-commercial. See: http://creativecommons.org/licenses/by-nc/4.0/
https://doi.org/10.1136/bmjopen-2017-018399
Statistics from Altmetric.com
Request permissions.
If you wish to reuse any or all of this article please use the link below which will take you to the Copyright Clearance Center’s RightsLink service. You will be able to get a quick price and instant permission to reuse the content in many different ways.
Strengths and limitations of this study
This study is the first to gather expert by experience views on the proposed criteria to be fed into the revision process of the International Classification of Diseases.
All aspects of the proposed study have been co-produced with experts by experience and agreed with a representative from WHO.
Qualitative focus group data will be thematically analysed to form the basis of co-produced recommendations to be fed back to WHO.
The themes and resulting recommendations will be limited to five diagnostic categories and will only reflect views from the UK.
Introduction
Diagnostic systems have a number of functions both from the perspective of the clinician and service user. 1–3 Diagnosis offers indications for treatment, may guide expectation regarding prognosis and can help people to make sense of their experiences of living with mental health (MH) difficulties. 1 2 In order for a diagnostic system to be useful, it is critical that it reflects the day-to-day experiences of people living with the symptoms. Service users have reported relief derived from diagnostic definitions that resonate with and explain their experiences. 1 4 On the other hand, some feel their diagnosis does not ‘fit’ with or describe their experiences, and thus has limited utility other than being a ‘tick box’ exercise of labelling and categorising. 5–7 To date, it appears that no revision of the major systems for psychiatric diagnosis (International Classification of Diseases (ICD) or Diagnostic and Statistical Manual of Mental Disorders) has sought feedback from service users prior to publication.
Diagnostic systems are designed for clinicians; despite this, service users can easily access the diagnostic criteria. Research shows that the labels, language and descriptions used in these systems can impact people’s self-perception, their interpretations of how other people view them and their understanding of the implications of having a diagnosis, including the prognosis and potential for recovery. 5 8 9 These interpretations can have a direct impact on factors such as self-worth and self-stigmatisation, social and occupational functioning, recovery and service use. 5 6 10 For example, service users have reported that terms like ‘disorder’ and ‘enduring’ suggest permanency, impeding their hope for recovery. 5 Similarly, others have reported that the descriptions and terms used in diagnostic systems (eg, language like ‘deviant’, ‘incompetent’, ‘disregard for social obligations’ and ‘limited capacity’) can be stigmatising and unhelpful, leading to feelings of rejection, anger and possible avoidance of services. 5 6 8 Clarity on the perceptions of individuals receiving a diagnosis, in terms of the language, meaning and implications of what is included in the system, may help to minimise possible negative consequences.
Evidence suggests that clinicians also have concerns regarding the validity and clinical utility of the current diagnostic systems. 3 11–13 For instance, health professionals have reported that some diagnostic definitions feel arbitrary, artificial or unreflective of the typical presentations they observe in practice. 11 12 Other evidence suggests that clinicians find the categories difficult to use, particularly for distinguishing between disorders. 9 12 14 Clinicians have also expressed reservations regarding the terminology and associated stigma, particularly for conditions such as Schizophrenia and Personality Disorder. 13 15 These findings are from studies that have been conducted after the criteria have been released. Prospective input from clinicians on the proposed criteria as part of the process of revision may therefore improve the validity and clinical utility of diagnostic systems.
The value of expertise by experience is increasingly recognised by policymakers, 16–18 service providers and researchers. 19 20 Many have argued that processes of diagnosis could be improved by including perspectives of those with lived experience. 10 21 It has been suggested that within the diagnostic categories, "the traditional language is useful for listing and sorting but not for living and experiencing. ‘Naming' a thing is not the same as 'knowing'a thing" (p90) 22 and therefore categories could be improved by viewing service users as ‘authors of knowledge from whom others have something to learn’ (p291). 21 Likewise, it has been argued that diagnostic systems could be improved by addressing problems identified by practising clinicians. 3
Input regarding the proposed content for the ICD-11 from service users and clinicians should be used to support the process of revision and improvement. Feedback and clarity from service users on (1) whether the content of the system is in line with their experience of symptoms and (2) their interpretations of the content and language should facilitate the development of a system that is more accurate and valid, with minimised unintended negative impact.
Aims and objectives
This research project will use a focus group methodology to ask service users and clinicians who use the ICD diagnostic tool (psychiatrists and general practitioners) their views on the proposed content for the ICD-11. Data collected through collaborative discussion in the groups will be inductively analysed, and resulting themes will be triangulated with an advisory group (involving additional service users and clinicians). The output will be recommendations for improvement to ICD-11 content that have been co-produced with a feedback group (of different service users and clinicians).
Research questions
What are the views and perspectives of service users and clinicians on the content of the ICD-11?
How could the system be improved for the benefit of service users and clinicians?
Methods and analysis
Study design.
This is a qualitative study. Data will be collected through focus groups. Focus groups are an appropriate method of data collection to answer the study research questions seeking to explore views and perspectives of service users and clinicians, where our analysis will aim to define key themes and points of consensus or divergence gathered through interaction, 23 24 drawing on participants own perspectives and choice of language. 25 Participants will be given a copy of the proposed diagnostic criteria relevant to their diagnosis to discuss in the group. This will include both the technical version (as it is proposed for the ICD-11) and a lay translation of the criteria. Thematic analysis 26–28 will be used to identify emergent recurring and/or salient themes in the focus group data. The themes will form the basis for co-produced recommendations to support the development of the ICD-11. Data collection for this study commenced in February 2017 and analyses are planned to be completed and fed back to WHO by the end of December 2017.
Co-production
The research team that developed this project includes a service user expert by experience (AG), two academics (TS, CN), two research clinicians (a consultant psychiatrist (JW) and a clinical psychologist (CH)) and two research assistant psychologists (AP, JR). A service user expert by experience research team member will be involved in all aspects of the research, including design, facilitating focus groups, analysis, write-up and dissemination.
In developing the project, team members consulted a local service user governor, service users and the service user involvement leads at the hosting National Health Service organisation. This input helped shape the design (changing and broadening the process of recruitment of service users and supporting the use of focus groups) and the initial selection of the diagnoses that were included.
Co-production with service users, clinicians and researchers will continue throughout the project. Data analysis will be co-produced through involvement of the service user expert by experience on the research team and the advisory and feedback groups.
Diagnoses under investigation
With agreement from WHO, five diagnoses have been selected for exploration: Personality Disorder, Bipolar I Disorder, Schizophrenia, Depressive Disorder and Generalised Anxiety Disorder. These diagnoses include a wide range of symptom phenomena. Personality Disorder, Bipolar I Disorder and Schizophrenia are found to be more stigmatised, rejected and negatively viewed than other diagnoses, meaning they may have a particularly negative impact and be more consistently associated with harm. 29 30 Depressive Disorder and Generalised Anxiety Disorder are highly prevalent, making the largest contribution to the burden of disease in middle-income and high-income countries, including the UK. 31
Lay translation
The lay translations of the criteria have been produced by members of the research team including psychiatrists and other clinicians, and approved by a representative from WHO to ensure they reflect the proposed ICD-11. Documents have been created presenting lay translations alongside the technical version as it is written in the ICD-11, so that participants are easily able to refer to either source. Copies of these are available in English for researchers wishing to replicate this study.
Recruitment
Sampling will be purposive and include a number of pathways to ensure maximum inclusivity. Recruitment of service users will be both via clinicians in a MH trust and self-referral via a number of routes. Promotion of the study will be via clinicians, service user involvement leads in a MH trust and non-governmental organisations (NGOs). Clinicians in the MH trust will be asked to identify potential participants and seek consent to be contacted by the research team. Service user involvement leads will disseminate information about the study to service users and the membership of a MH trust (which includes many previous service users), providing a telephone number and email address to self-refer if interested. NGOs will promote the study using the same materials. The study will be promoted through recruitment posters, service user involvement forums and on social media. Clinicians will be recruited via team leaders, word of mouth and email communications promoting the project.
Once self-referral or consent to contact has been established, a member of the research team will make contact, provide potential participants with a brief overview of the study, and answer any questions. If the individual wishes to be involved in the study, they will be sent a copy of the participant information sheet via post or email. This information sheet outlines the purpose and nature of the study, and the ethical safeguards regarding data protection and privacy. Potential participants will have at least 72 hours to consider whether they would like to be involved in the study. If the individual would like to take part in the study, researchers will arrange to meet them at least 1 week before the focus group to complete the consent process and give them the relevant proposed diagnostic criteria to read and consider.
Sample size
There will be two service user focus groups for each of the five diagnoses. Additionally, there will be four clinician focus groups. The ICD system is primarily used by medical doctors in the UK, although clinical psychologists have been included in this study as they also apply the system in their work. 32 In this study, the diagnostic criteria presented to participants are divided into distinct discussion points. During the focus groups, these discussion points will be addressed one by one and participants will be asked for their feedback through predefined questions and prompts. This includes asking people their views of the proposed features, the language used, the positives and negatives of what is included and how the classification might be improved for the benefit of service users. In light of this, the number of groups was agreed based on research stating that using more standardised interviews decreases variability and thus requires fewer focus groups. 33 In total, there will be 14 groups, containing three to six participants each. This will give a total sample of 42–84 participants (30–60 service users and 12–24 clinicians). The advisory group will comprise three to five additional service users and three clinicians. Lastly, the feedback group will comprise five service users and three clinicians. The focus group size was chosen to allow participants opportunity to discuss their views and experiences in detail, while increasing recruitment feasibility. 34 The sample size should be sufficient in providing data to meet the aims and to cover a range of views. Evidence suggests that the majority of themes are discovered in the first two to three focus groups. 35
Inclusion and exclusion criteria
Adult service users (18 years and older) may be included in the focus groups if they have formally received at least one of the five diagnoses under investigation and have accessed services within the last 5 years (including those currently in receipt of services). People with multiple diagnoses may only take part in one focus group, but will be given the option of which group. Clinicians will have had experience working in MH, including the use of the psychiatric diagnoses under investigation. Individuals may only participate in either one focus group, the advisory group or the feedback group.
Individuals will be excluded if they are under the age of 18 years, lack the capacity to consent, or have an inability to speak fluent English (as fluent English is required to participate in the focus groups). Individuals will also be excluded if their participation is deemed unsafe to themselves or others by their lead clinician or clinicians on the research team.
Data collection
Focus groups are the most applicable method for data collection to meet our research aims, as attitudes, opinions and beliefs are more likely to be revealed in the reflective process facilitated by the social interaction that a focus group entails than by other methods. 23–25 Additionally, focus groups have proved to be a useful way of exploring stigma issues in MH, 36 and service users are often familiar with group settings for discussing MH issues.
The summary of the new diagnostic guidelines and lay translation will enable participants to reflect on both the content and the language of the proposed criteria. During the groups, topic guides will encourage participants to discuss and share views of the relevant diagnostic category. This includes their overarching views, thoughts and feelings; as well as, specific reflections on areas such as the language used, aspects that may be helpful or unhelpful, and suggestions for improvement.
Each focus group will be led by an experienced and trained member of the research team and have an assistant facilitator. Service user focus groups will last 60–90 min, and clinician focus groups will last 2–2.5 hours to account for the discussion of multiple diagnoses.
The focus groups will be audio-recorded and transcribed verbatim. The transcripts will first be read and descriptively openly coded (using the same language as participants where possible) by the lead researcher. Approximately 25% of the transcripts will be independently open coded by another member of the research team, as a validity check. Codes will be compared and discussed until consensus is reached. The five diagnoses will initially be analysed separately to produce themes that are relevant to each diagnosis. Following this, these themes will be compared with identify common themes relevant to all the diagnostic categories. Analysis of data will mainly be descriptive. We will take a critical realist epistemological stance to analysis, recognising that there are multiple individual realities, but taking a pragmatic approach to analysing data at face value, drawing on the perspectives of individuals as they choose to represent themselves through discussion. 37 Thematic analysis will be used to inductively code themes that reoccur or appear important. 26–28 The concept of salience will be referred to here, to guide coding that is conceptually and inherently significant, not just frequently occurring. A qualitative data management software system (NVIVO-11) will be used to facilitate data analysis.
In addition to descriptive data for thematic coding, focus groups generate data that is conversational. Analysis of this requires an inductive approach that focuses on instances in the data where there is marked agreement (consensus), disagreement or divergence. These instances will be identified as ‘critical moments’. The sample size is small and purposive. Consequently, summary quantified coding matrices will not be produced. Instead there will be a focus on the 'critical moments' to direct the analysis and eventual findings, reporting on the issues that are of central importance to the participants.
Following analysis of each focus group, a second stage analysis will be conducted to compare and contrast findings across groups. The analysis will seek out consensus, disagreement and inconsistency within service user and clinician focus groups, and between diagnoses. This second stage analysis will involve discussions within the research team to refine the themes and to develop higher level themes, that is, grouping the open codes into meaningful conceptual categories. This will allow tentative conclusions to be drawn about aspects of the diagnostic criteria which may be particularly pertinent for some groups and less important for others. It will also enable conclusions to be drawn regarding generic language or overall responses to the diagnostic criteria, in comparison to more nuanced reactions to diagnostically specific categories.
The output from the analysis will be higher level themes and categories that form the basis of recommendations for the ICD-11. These themes will be triangulated with the advisory group. The resulting themes will be discussed with the feedback group in order to co-produce the recommendations. These recommendations will be contextualised with a description of the themes and identified areas of agreement and disagreement for feedback to WHO.
Data protection
All confidential data will be kept for 5 years on password-protected computers and/or locked filing cabinets only accessible to members of the research team. During transcription, audio-recordings will be anonymised, with all identifiable information removed prior to using the software analysis tool. All audio-recordings will be destroyed immediately after transcription.
Ethics and dissemination
Ethical considerations.
Written informed consent to participate and be audio-recorded will be obtained from all participants. Data management and storage will be subject to the UK Data Protection Act 1998. Ethical approval for the current study was obtained from the Coventry and Warwickshire Research Ethics Committee (Rec Ref: 16/WM/0479).
Declaration of Helsinki
This study complies with the Declaration of Helsinki, adopted by the 18th World Medical Association (WMA) General Assembly, Helsinki, Finland, June 1964 and last revised by the 64th WMA General Assembly, Fortaleza, Brazil, October (2013).
Output and dissemination
This research has been designed to obtain feedback with recommendations for the ICD-11, and to develop a methodology that can be replicated in other countries that use the ICD system. Additionally, the findings, and learning in terms of the process of co-producing and conducting research with experts by experience, will be disseminated via peer-reviewed publications, conferences, media and lay reports.
Service user involvement in MH is a priority. 19 Studies have found that both clinicians and service users have questioned the accuracy, validity and clinical utility of the ICD and other psychiatric diagnostic tools. 3 8 9 11 12 38 Despite this, to date, service user and clinician feedback has not been obtained prior to revision of the ICD manual. In light of this, is not clear whether the content resonates with the experiences of people giving and receiving the diagnoses, could lack clinical utility, or even, cause harm (eg, in terms of the language used).
Limitations
This study is designed to input feedback from service users and clinicians in the forthcoming revision of the ICD. The usefulness of the data and resulting recommendations is dependent on input, that is, reflective of the views of service users and clinicians that the new system will impact. The current study will include two focus groups for each disorder in an attempt to minimise bias 35 and to account for group-think processes that may occur within individual groups. Taking a critical realist epistemological stance is a pragmatic approach to work with discursive data created through the interactional context of a focus group. It is acknowledged that there are multiple competing realities and perspectives that may differ across time and context, and the analysis findings will be limited to the time and context of this study. Transferability of findings is nonetheless maximised by triangulation to ensure the inclusion of multiple stakeholder perspectives, enabled by the advisory and feedback groups of experts by experience that will co-produce the recommendations reported to WHO. Interpretation of the feedback will take into account potential limitations regarding the generalisability of the findings. The current project is exploring only five of the diagnoses that are included in the ICD-11. The ICD is internationally used, and the current project will reflect the experiences and views of service users and clinicians in the UK only. Future research may include both additional diagnostic categories and encapsulate expertise by experience and relevant clinicians in different countries.
The current study will use feedback from experts by experience to co-produce recommendations for the revised diagnostic system proposed for the ICD-11. This feedback aims to improve the accuracy, validity and clinical utility of the manual, and minimise the potential for unintended negative consequences. This qualitative approach has not been previously employed by any countries that use the ICD system. Our vision is that this process will become a routine feature in future revisions of all diagnostic systems.
Acknowledgments
We would like to thank the library services at Norfolk and Suffolk Foundation Trust for aiding the searching and retrieval of documents. We would like to thank Kevin James (service user governor), Lesley Drew and Sharon Picken (service user involvement leads) for their input during the development of the project. We would also really like to thank Dr Bonnie Teague who generously offered the benefit of her wisdom and proof reading skills.
- Kilbride M ,
- Welford M , et al
- Johnstone L ,
- Bonnington O ,
- Stalker K ,
- Ferguson I ,
- Castillo H ,
- van Rijswijk E ,
- van Hout H ,
- van de Lisdonk E , et al
- Shadbolt N ,
- Starcevic V , et al
- Milton AC ,
- Kelly B , et al
- 16. ↵ Department of Health . Putting People First: Planning together – peer support and self-directed support . London : Department of Health , 2010 .
- 17. ↵ Department of Health . No Health without Mental Health: A cross-government mental health outcomes strategy for people of all ages . London : Department of Health , 2011 .
- 18. ↵ Department of Health . Closing the Gap: Priorities for essential change in mental health . London : Social Care, Local Government and Care Partnership Directorate , 2014 .
- Simpson EL ,
- Beresford P
- Malone P , et al
- Wilkinson S
- MacQueen KM ,
- Stevens S ,
- Serfaty M , et al
- Carlyle D , et al
- 31. ↵ World Health Organization . The global burden of disease: 2004 update . Switzerland : World Health Organization , 2008 .
- 32. ↵ The British Psychological Society . Diagnosis – policy and guidance . http://www.bps.org.uk/system/files/documents/diagnosis-policyguidance.pdf ( accessed Jul 2017 ).
- Schulze B ,
- Angermeyer MC
- Denzin NK ,
Contributors CH is the chief investigator for this project and wrote the protocol. TS is supervising the project and helped to develop all aspects of the project. AG is the expert by experience on the research team, and led on developing the co-production, and the public and patient involvement. CN led the development of the methodology. AP had a specific contribution to the literature review. GMR is the WHO consultant for the project. GMR developed the original idea for the project and has had input into the development of the lay criteria. JR provided input to ethical considerations and the lay criteria. JW led on the development of the lay criteria. All authors supported the development and critical review of the protocol.
Competing interests None declared.
Ethics approval Coventry and Warwickshire HRA Research Ethics Committee (16/WM/0479).
Provenance and peer review Not commissioned; externally peer reviewed.
Read the full text or download the PDF:
This paper is in the following e-collection/theme issue:
Published on 19.4.2024 in Vol 13 (2024)
This is a member publication of University of Stirling (Jisc)
How a National Organization Works in Partnership With People Who Have Lived Experience in Mental Health Improvement Programs: Protocol for an Exploratory Case Study
Authors of this article:

- Ciara Robertson 1 , BSc, MEd ;
- Carina Hibberd 1 , BSc, PhD ;
- Ashley Shepherd 1 , BA, PhD ;
- Gordon Johnston, BSc
1 Faculty of Health Sciences and Sport, University of Stirling, Stirling, United Kingdom
Corresponding Author:
Carina Hibberd, BSc, PhD
Faculty of Health Sciences and Sport
University of Stirling
Pathfoot building
Stirling, FK9 4LA
United Kingdom
Phone: 44 1786 466334
Email: [email protected]
Background: This is a research proposal for a case study to explore how a national organization works in partnership with people with lived experience in national mental health improvement programs. Quality improvement is considered a key solution to addressing challenges within health care, and in Scotland, there are significant efforts to use quality improvement as a means of improving health and social care delivery. In 2016, Healthcare Improvement Scotland (HIS) established the improvement hub, whose purpose is to lead national improvement programs that use a range of approaches to support teams and services. Working in partnership with people with lived experience is recognized as a key component of such improvement work. There is, however, little understanding of how this is manifested in practice in national organizations. To address gaps in evidence and strengthen a consistent approach, a greater understanding is required to improve partnership working.
Objective: The aim of this study is to better understand how a national organization works in partnership with people who have lived experience with improvement programs in mental health services, exploring people’s experiences of partnership working in a national organization. An exploratory case study approach will be used to address the research questions in relation to the Personality Disorder (PD) Improvement Programme: (1) How is partnership working described in the PD Improvement Programme? (2) How is partnership working manifested in practice in the PD Improvement Programme? and (3) What factors influence partnership working in the PD Improvement Programme?
Methods: An exploratory case study approach will be used in relation to the PD Improvement Programme, led by HIS. This research will explore how partnership working with people with lived experience is described and manifested in practice, outlining factors influencing partnership working. Data will be gathered from various qualitative sources, and analysis will deepen an understanding of partnership working.
Results: This study is part of a clinical doctorate program at the University of Stirling and is unfunded. Data collection was completed in October 2023; analysis is expected to be completed and results will be published in January 2025.
Conclusions: This study will produce new knowledge on ways of working with people with lived experience and will have practical implications for all improvement-focused interventions. Although the main focus of the study is on national improvement programs, it is anticipated that this study will contribute to the understanding of how all national public service organizations work in partnership with people with lived experience of mental health care.
International Registered Report Identifier (IRRID): DERR1-10.2196/51779
Introduction
The need to improve quality in mental health (MH) care is widely recognized, in response to both long-standing problems and more contemporary pressures [ 1 , 2 ]. For several years, quality improvement (QI) has been considered a key solution to many health care challenges, supporting the design and delivery of services. Over the last decade, there has been a significant effort to use QI within health care settings, including the introduction of national organizations to lead improvement programs.
There are several national organizations in Scotland with an improvement focus, including the Centre for Sustainable Delivery, the Health and Social Care Alliance Scotland, the Improvement Service, and Healthcare Improvement Scotland (HIS). In 2016, HIS established the improvement hub (ihub), whose purpose is to enable health and care systems to apply improvement methodologies to the design and implementation of changes that deliver sustainable improvements in the health and well-being outcomes of people in Scotland [ 3 ]. The ihub within HIS is uniquely placed with a focus on improvement support for those delivering health and social care across Scotland, including MH services.
Work within the ihub is delivered through improvement programs that use a range of theories and techniques to support teams and services through an improvement journey. National improvement programs have an important role to play in health care. However, there are challenges within centrally led programs that require sensitive understanding and management [ 4 ]. The development of improvement programs recognizes growing evidence that the impact of QI in health care is mixed and of poor quality [ 5 ], and there is a need to reconceptualize improvement efforts in response to the evidence base [ 6 ]. In order to address some concerns within the literature, the ihub has outlined a broad approach to improvement that forms the basis of their improvement programs. The core components of improvement programs within the ihub are described in the framework for planned improvement ( Figure 1 [ 3 ]), which outlines the stages of improvement work. In the Framework for Planned Improvement, the initial focus is on understanding the system and designing, implementing, and evaluating changes, with people with lived experience at the center of this work. People with lived experience include people who have lived or living experience, their families, caregivers, and supporters. Improvement programs then aim to embed and sustain successful change within practice and spread the learning to other areas. Underpinning the framework is the recognition of the importance of the relational aspect of change and the use of technical QI approaches, including the model for improvement.

A key principle to improvement is working in partnership with others in the system, including other agencies, people with lived experience, and frontline staff. In Scotland, a seminal paper by Christie [ 7 ] recommended that there should be a stronger partnership working with people and communities in the design and delivery of services they use, including those involved in health care improvement. There is a growing evidence base supporting the need to work with people with lived experience in health care improvement. People with lived experience have a key role to play in understanding problems and identifying solutions to ensure change delivers outcomes that make a difference to patients [ 8 ]. Working with people with lived experience in improvement initiatives can strengthen and enrich the organizational agenda for improvement in health care [ 9 ] and should be seen as a core component of all improvement programs. Within MH services, people with lived experience should be able to participate in the development of policies to improve MH systems [ 10 ] and should therefore be involved in health care improvement initiatives. Working with people with lived experience should be based on authentic, interdependent partnership work [ 6 ], which will improve the quality and value of services.
Despite the recognition that working with people with lived experience is central to improvement-focused work, there are a number of challenges and a lack of critical examination of partnership working within the health care improvement literature [ 11 ]. There is a lack of understanding of the phenomenon of partnership working, including the mechanisms of partnership working, organizational features supporting partnership working (eg, leadership), and the impact and outcomes achieved from working with people with lived experience [ 11 , 12 ]. There is also little understanding in the literature of how working with people with lived experience is manifested in practice in national organizations [ 13 ].
This research will explore how a national organization works in partnership with people with lived experience in a MH improvement program. This research will focus on one improvement program—the Personality Disorder (PD) Improvement Programme within HIS’ ihub. The PD Improvement Programme is a commissioned piece of work funded by the Scottish Government to understand the current service provision in Scotland for people with a diagnosis of PD and identify the key opportunities for improvement. This research will use a case study approach to explore how partnership working is planned, conceptualized, and manifested in practice within the PD Improvement Programme.
The aim of this study is to better understand how a national organization works in partnership with people who have lived experience with improvement programs in MH services, exploring people’s experiences of partnership working in a national organization. An exploratory case study approach will be used to address the research questions in relation to the PD Improvement Programme:
- How is partnership working described in the PD Improvement Programme?
- How is partnership working manifested in practice in the PD Improvement Programme?
- What factors influence partnership working in the PD Improvement Programme?
This research will consist of 2 phases. The first phase will address the first 2 research questions through document analysis and observations of meetings within the early stages of the PD Improvement Programme. Semistructured interviews will be carried out in the second phase of this research to explore participants’ experiences of partnership working, addressing the third research question.
Benefits of This Research
It is anticipated that the findings of this research will contribute to an understanding of partnership working in national organizations and will be used to identify a framework for partnership working so that partnership working can be improved across the organization and other national organizations.
In order to address the research aim, it is appropriate to use case study methodology. A case study approach is appropriate when the focus of the study is on how and why questions; the behavior of participants will not be changed; the context is relevant to the phenomenon studied; and when there are unclear boundaries between phenomenon and context [ 14 ]. Partnership working sits within the wider context, and case study methodology is well placed to understand relationships between context and intervention [ 15 ], with partnership working conceptualized as the intervention in this research. A case study approach will enable a holistic exploration of the complex social processes and mechanisms underpinning partnership working within QI [ 16 ]. Data will be collected from a wide range of qualitative sources, including document data, participant observations, and semistructured interviews.
Case Study Design
The DESCARTE model [ 17 ] will be used in this research to inform the design, conduct, and reporting of the case study. There are 3 stages to this model: the situation of the research and the researcher, determining the components of the case study design, and data analysis.
Situation of the Research and the Researcher
In designing case study research, it has been recommended that the researcher state explicitly their informing philosophical approach, situation of “Self” within the research, and any ethical considerations to outline the position of research and the researcher [ 17 ].
The lead researcher is currently working as part of the improvement team within HIS and therefore will be considered an insider researcher. Although this position may support access to naturalistic data and respondents, there is a risk that there may be conflict between the researcher and participants who have professional relationships, and a risk that respondents may change their behavior or responses due to this relationship [ 18 ]. This will increase the risk of bias within the research, and strategies should be used throughout the different stages of the research process to reduce these risks [ 19 ]. For this study, strategies will include planning the interview process, using research diaries, reflection, and ongoing monitoring with the supervisory team. The lead researcher will also work closely with a public partner at key stages of this research. Public partners are volunteers who HIS trains and supports to provide a public perspective to their work, and a public partner with lived experience of mental illness will be involved at several stages of this research.
Components of the Case Study
Although case study research can have a level of creativity and flexibility—where the researcher may choose epistemologies and theories suited to their preferences and the nature of the inquiry, clear descriptions of paradigms, theories, and methods should be provided to demonstrate rigor [ 20 ]. These will be described to outline the main components of the case study.
Binding the Case
First, it is important to identify what the case will be and set clear parameters or boundaries to ensure the study has a clear and reasonable scope—a process referred to as binding [ 21 ]. The parameters of this study will be determined by definition and context; for this research, the case will consist of the PD Improvement Programme within HIS. Early involvement of people with lived experience in the conceptual stages of improvement work has been highlighted to ensure meaningful involvement with influence and impact [ 22 ]. The PD Improvement Programme is the first commissioned work for HIS to improve the understanding of the context of service provision for people with a diagnosis of PD across Scotland. The program will include working with people with lived experience and frontline staff working in clinical roles. The commission is from the Scottish Government and will run between June 2021 and March 2023. This case study will follow the PD Improvement Programme during the current stage of the program: creating the conditions and understanding the system. This stage will involve establishing the program and working practices for working in partnership during the PD Improvement Programme. The parameter for this case is to explore working in partnership with people with lived experience and will not include exploration of wider partnerships working in this program.
Type of Case Study
Exploratory case studies can be used to explore situations in which the intervention being researched does not have a clear, single set of outcomes [ 21 ]. Given the diversity within QI and the complexity of partnership work, an exploratory approach is considered appropriate.
In phase 1 of this case study, data will be collected from organizational documents, followed by nonparticipant observations of key program meetings. This data will help explore how partnership working is described, defined, and manifested in practice. This will be followed in phase 2 by semistructured interviews with key participants to explore their experiences of partnership working in the program.
Phase 1: Document Data
In the first phase of data collection, analysis of organizational documents will be used to provide an understanding of plans, infrastructure, and frameworks used to support partnerships working with people with lived experience. It is anticipated that documents may include commission agreements, planning papers, minutes of key meetings, presentations or diagrams describing the program infrastructure, and partnerships working in the program. Further documents relevant to the study may emerge and will be included as appropriate. Access to these documents will be through the program lead within HIS.
As there is no agreed definition of partnership working, documents will be analyzed for any description of partnership, which may include terms such as involvement, participation, engagement, and empowerment. The content of documents will be analyzed, including the document, author, date, description of partnership working, and any actions taken or recommendations. Meetings with the public partner will be agreed upon to discuss the data analysis and the identification of themes at each stage of the data analysis.
Themes developed from the document review will be included in the structure of observations and used to develop the interview proforma in the following phases of the research.
Phase 1: Nonparticipant Observations
Following document analysis, nonparticipant observations of PD Improvement Programme meetings will be used to gather data on how partnership working with people with lived experience in the program is manifested in practice. Meetings observed will be chosen based on a purposive sample, and there will be between 3 and 6 observations completed. The portfolio lead will be asked to provide a list of all meetings taking place in the early stages of the program, which is likely to be within the first 6-9 months of the program. A sample of meetings most likely to demonstrate partnership working in practice [ 23 ] will be selected to be observed, such as planning meetings and advisory group meetings. The meetings will be chosen by the researcher to address any potential bias and ensure the appropriate independence of the research.
A framework for partnership working will be used to guide observations ( Textbox 1 [ 24 ]). This model describes 4 key dimensions of partnership: process, actors (identity and position), decisions, and power relationships. Although the use of this framework provides some structure to the observations, a form of semistructured observation will be adopted to allow for some naturalistic observations [ 23 ] and include themes identified in the document analysis.
Nonparticipant observation will allow observation of the environment, language, nonverbal data, and interaction in partnership. General context will be noted for each observation, including location, time, duration, meeting roles, and purpose of the event or meeting.
There is a possibility that the presence of a researcher will increase the risk bias by changing the behavior of participants, and strategies will be used to reduce this risk. Strategies will include giving a clear explanation of the plan for observation and being aware of the position of the researcher to be as unobtrusive as possible [ 25 ]. Observations will be primarily descriptive and will provide the basis for the interpretation of data obtained by semistructured interviews in the final stage of data collection. Meetings will be held with the public partner to discuss themes developed at this stage of data collection and to agree on the format of semistructured interviews in phase 2.
Dimension of partnership working and observation guide
- How is partnership working planned for and what preparations are in place to support partnership working?
- How many events or meetings involve people with lived experience?
- Who is involved in setting the agenda and context for meetings?
- Who attends meetings?
- What are people’s positions within the organization or program?
- How are decisions in the program made?
- How are people with lived experience involved in decision-making in the program?
- Who contributes to the event or meeting?
- What is the response to people with lived experience’s contribution?
- What efforts are made to support contributions from people with lived experience?
Phase 2: Semistructured Interviews
The final stage of data gathering will be semistructured interviews with participants from the PD Improvement Programme, including people across disciplines and people with lived experience. Interviews will be used to gain an understanding of participants’ experiences and perceptions of partnership working with people with lived experience. A schedule for interviews will be prepared based on themes developed from the document review and observations. The interview proforma will be developed with people with lived experience working as a public partner in HIS to ensure questions are relevant and likely to receive meaningful responses [ 22 ]. All interviews will follow the schedule developed as an aide memoire; however, it is important to allow flexibility to adapt to each participant’s response to allow exploration of emerging and reported experiences [ 26 ]. Interviews will be held at a location agreed upon the researcher and participant and may be face-to-face, remote through Teams (Microsoft Corporation), or by telephone. All interviews will be recorded and transcribed.
The population within this case will include a purposive sample of staff and people with lived experience who are involved in and contribute to the work of the PD Improvement Programme. It is anticipated that this will be between 6 and 8 interviews. Participants will include clinical and improvement staff working directly on the PD Improvement Programme operating at different levels of the organization and people with lived experience working with the PD Improvement Programme. This should ensure diversity within the perspectives gained from the interviews.
Recruitment Strategy and Informed Consent
Participants will be recruited through the PD Improvement Programme and will include a purposive sample of people involved in the program based on their role. All people involved in the program will be offered the opportunity to participate in this study and will be asked to sign a consent form and return it to the researcher at the start of each stage of the research.
There will be a process of ongoing consent for each phase of this research. In phase 1, each participant in the meetings observed will be asked to consent to the observation and recording during selected meetings and consent to being contacted for an interview at the second phase of research if appropriate. This will ensure each participant has a full understanding of the research, their role within it, the benefits and risks, and their right to withdraw from the research. Each participant’s consent will be documented in a written form they will be invited to sign before the meeting. Consent will be reviewed at the start of the meeting as a process of ongoing informed consent. If there are participants in the meeting who do not consent, their contribution to the meeting will be omitted during transcription. For meetings held online, participants who do not consent will be offered the chance to turn their camera off during the meeting and use the chat box for contributions if required. This may affect the understanding of the wider context of discussions, and therefore, efforts will be made to observe meetings with full consent.
In phase 2, people will be asked to consent to participate in semistructured interviews. Consent will be documented for each participant; they will be asked to sign a written consent form, and consent will be confirmed verbally at the start of each interview. Once consent is documented, the researcher will select a purposive sample of people who will participate in interviews based on their role in the program. All people who have given consent will be contacted to discuss the next steps, and interviews will be arranged with participants to ensure they take place at a suitable time and setting.
Data Analysis
Data analysis will organize, find patterns, and elicit themes in the data to help deepen an understanding of partnership working within the national PD Improvement Programme. There are various mechanisms for quality assurance within this research, including the use of a reflexive field diary, discussions with supervisors, and member checking where participants can check transcriptions following observations and interviews. During analysis, there will also be regular meetings with a public partner working in HIS to review and discuss themes to check emerging findings and the researcher’s interpretation, as a form of participant validation to improve scientific rigor. A framework for data analysis is outlined in Table 1 [ 27 ].
In order to develop convergent evidence, the structure outlined in Figure 2 will be applied to data analysis.
Effective organization of data will be important to this case study to enable the tracking of data sources, notes, documents, narratives, and other data [ 14 ]. NVivo (version 12; QSR International) will be used to support the management of data and to assist within and across case study analysis, appropriate to case study research [ 27 ]. Data collection and analysis will occur concurrently, as is practiced in qualitative studies [ 14 ].

Patient Involvement
The objective of this research is to deepen an understanding of how national improvement programs work in partnership with people with lived experience. This focus was developed through a review of current literature and organizational objectives [ 28 ] and has been highlighted by people with lived experience who have worked with HIS in other national MH improvement programs [ 29 ].
Patient involvement has been central to the development and design of this research, and a public partner has been involved in the design and will be involved in the analysis of this research. In phase 1, this included involvement in the review and analysis of themes as a form of participant validation to improve scientific rigor [ 30 ]. The public partner advised on the burden of intervention for people with lived experience in this study and has been involved in the design of phase 2, including the design of interviews, the development of the distress response policy, and advising on participant recruitment. The public partner will continue to be involved during the data analysis of phase 2, reviewing and discussing themes developed at this phase, and will be invited to advise on plans for dissemination of the study results to participants and linked communities.
Ethical Considerations
Ethical approval has been granted from HIS’ research oversight group, the University of Stirling Research Ethics Committee, and the Integrated Research Application System through the Queen Square Research Ethics Committee (for phase 1; 318323) and the Black Country Research Ethics Committee (for phase 2; 309926). This study is part of a clinical doctorate program at the University of Stirling and is unfunded.
Data collection was completed in October 2023; analysis is expected to be completed and results published in January 2025.
This study will produce new knowledge on ways of working with people with lived experience and will have practical implications for all improvement focused interventions. Though the main focus of the study is on national improvement programs, it is anticipated that this study will contribute to the understanding of how all national public service organizations work in partnership with people with lived experience of MH care. The anticipated time for completion and write-up is 24 months. Information will be shared with key stakeholders on the progress of this research, including HIS and the University of Stirling, and opportunities for presentation of this research will be sought. These may include QI conferences and communities—including the Q Community (The Health Foundation), MH organization events, and NHS Scotland events. The findings will be completed with a thesis submitted to the University of Stirling and will be reported in an appropriate journal, such as BMJ Open Quality or the Journal for Healthcare Quality .
Acknowledgments
The authors thank Healthcare Improvement Scotland’s Mental Health Improvement Portfolio Team. This research has received no specific grant from any funding agency in the public, commercial, or not-for-profit sectors.
Data Availability
The data sets generated during and/or analyzed during this study are available from the corresponding author on reasonable request.
Authors' Contributions
CR is a student in the clinical doctorate program at the University of Stirling. CH is the lead supervisor and researcher. AS is the supervisor for this research. Both supervisors contributed to the design of the research protocol, advising on analysis, and developing the manuscript for this research. GJ is the public partner advising on the design, analysis, and dissemination of this research.
Conflicts of Interest
None declared.
- The state of health care and adult social care in England 2015/16. Care Quality Commission. Oct 12, 2016. URL: https://www.cqc.org.uk/sites/default/files/20161019_stateofcare1516_web.pdf [accessed 2023-01-28]
- Gilburt H. Mental health under pressure. The King's Fund. London.; 2015. URL: https://assets.kingsfund.org.uk/f/256914/x/78db101b90/mental_health_under_pressure_2015.pdf [accessed 2023-01-28]
- Our approach to supporting improvement. Healthcare Improvement Scotland. 2016. URL: https://ihub.scot/media/1870/improvement-hub-our-approach-to-supporting-improvement-v6-27092016.pdf [accessed 2023-01-28]
- Lining up: how do improvement programmes work? The Health Foundation. 2013. URL: https://www.health.org.uk/publications/lining-up-how-do-improvement-programmes-work [accessed 2024-03-28]
- Dixon-Woods M. How to improve healthcare improvement-an essay by Mary Dixon-Woods. BMJ. 2019;367:l5514. [ FREE Full text ] [ CrossRef ] [ Medline ]
- Batalden P, Foster T. From assurance to coproduction: a century of improving the quality of health-care service. Int J Qual Health Care. 2021;33(Supplement_2):ii10-ii14. [ FREE Full text ] [ CrossRef ] [ Medline ]
- Christie C. Christie Commission on the future delivery of public services. Scottish Government. Jun 29, 2011. URL: https://www.gov.scot/publications/commission-future-delivery-public-services/documents/ [accessed 2022-01-04]
- Alderwick H, Jones B, Charles A, Warburton W. Making the case for quality improvement: lessons for NHS boards and leaders. The King's Fund. 2017. URL: https://www.kingsfund.org.uk/publications/making-case-quality-improvement [accessed 2021-05-25]
- Fitzsimons B. Voices and stories are central to improving healthcare. BMJ. 2022;376:o114. [ FREE Full text ] [ CrossRef ] [ Medline ]
- Carbonell Á, Navarro-Pérez JJ, Mestre MV. Challenges and barriers in mental healthcare systems and their impact on the family: a systematic integrative review. Health Soc Care Community. 2020;28(5):1366-1379. [ FREE Full text ] [ CrossRef ] [ Medline ]
- Palmer VJ. The participatory zeitgeist in health care: it is time for a science of participation. J Particip Med. 2020;12(1):e15101-e15106. [ FREE Full text ] [ CrossRef ] [ Medline ]
- Kjellström S, Areskoug-Josefsson K, Gäre BA, Andersson AC, Ockander M, Käll J, et al. Exploring, measuring and enhancing the coproduction of health and well-being at the national, regional and local levels through comparative case studies in Sweden and England: the 'Samskapa' research programme protocol. BMJ Open. 2019;9(7):e029723. [ FREE Full text ] [ CrossRef ] [ Medline ]
- Connolly J, McGillivray S, Munro A, Mulherin T, Anderson J, Gray N, et al. How co-production and co-creation is understood, implemented and sustained as part of improvement programme delivery within the health and social care context in Scotland. University of the West of Scotland. 2020. URL: https://researchonline.gcu.ac.uk/en/publications/how-co-production-and-co-creation-is-understood-implemented-and-s [accessed 2024-03-28]
- Baxter P, Jack S. Qualitative case study methodology: study design and implementation for novice researchers. TQR. 2015;13(4):544-559. [ FREE Full text ] [ CrossRef ]
- Grant A, Bugge C, Wells M. Designing process evaluations using case study to explore the context of complex interventions evaluated in trials. Trials. 2020;21(1):982. [ FREE Full text ] [ CrossRef ] [ Medline ]
- Yin RK. Case Study Research: Design and Methods. London. Sage Publications, Inc; 2011;221-222.
- Carolan CM, Forbat L, Smith A. Developing the DESCARTE model: the design of case study research in health care. Qual Health Res. 2016;26(5):626-639. [ CrossRef ] [ Medline ]
- Caruana C. Ethical considerations when carrying out research in one's own academic institution. Symposia Melitensia. 2015;10(10):61-71. [ FREE Full text ]
- Fleming J. Recognizing and resolving the challenges of being an insider researcher in work-integrated learning. Int J Work-Integr Learn. 2018;19(3):311-320. [ FREE Full text ]
- Hyett N, Kenny A, Dickson-Swift V. Methodology or method? A critical review of qualitative case study reports. Int J Qual Stud Health Well-being. 2014;9:23606. [ FREE Full text ] [ CrossRef ] [ Medline ]
- Yin RK. Case Study Research: Design and Methods. Thousand Oaks. SAGE Publications; 2003.
- Byrne L, Wykes T. A role for lived experience mental health leadership in the age of Covid-19. J Ment Health. 2020;29(3):243-246. [ FREE Full text ] [ CrossRef ] [ Medline ]
- Simons H. Case Study Research in Practice. Los Angeles. SAGE Publications, Ltd; 2009.
- Carpentier N. Beyond the ladder of participation: an analytical toolkit for the critical analysis of participatory media processes. Javnost. 2016;23(1):70-88. [ FREE Full text ] [ CrossRef ]
- Creswell JW, Creswell JD. Research Design: Qualitative, Quantitative and Mixed Methods Approaches, Fifth Edition. Thousand Oaks, CA. Sage Publications, Inc; 2018.
- Smith JA, Flowers P, Larkin M. Interpretative Phenomenology Analysis: Theory, Method and Research. London. Sage Publications; 2009.
- Houghton C, Murphy K, Shaw D, Casey D. Qualitative case study data analysis: an example from practice. Nurse Res. 2015;22(5):8-12. [ CrossRef ] [ Medline ]
- Our strategy 2022—27 draft for consultation. Healthcare Improvement Scotland. 2022. URL: https://www.healthcareimprovementscotland.org/news_and_events/news/news_draft_strategy.aspx [accessed 2023-04-04]
- Lindsay A. Sharing recent lived experience to help improve services. Healthcare Improvement Scotland. 2021. URL: https://ihub.scot/improvement-programmes/mental-health-portfolio/early-intervention-in-psychosis/personal-reflections/sharing-recent-lived-experience-to-help-improve-services/ [accessed 2023-04-04]
- Crowe S, Cresswell K, Robertson A, Huby G, Avery A, Sheikh A. The case study approach. BMC Med Res Methodol. 2011;11:100. [ FREE Full text ] [ CrossRef ] [ Medline ]
Abbreviations
Edited by A Mavragani; submitted 12.08.23; peer-reviewed by J Abbas, D Bradford; comments to author 21.02.24; revised version received 13.03.24; accepted 14.03.24; published 19.04.24.
©Ciara Robertson, Carina Hibberd, Ashley Shepherd, Gordon Johnston. Originally published in JMIR Research Protocols (https://www.researchprotocols.org), 19.04.2024.
This is an open-access article distributed under the terms of the Creative Commons Attribution License (https://creativecommons.org/licenses/by/4.0/), which permits unrestricted use, distribution, and reproduction in any medium, provided the original work, first published in JMIR Research Protocols, is properly cited. The complete bibliographic information, a link to the original publication on https://www.researchprotocols.org, as well as this copyright and license information must be included.
This paper is in the following e-collection/theme issue:
Published on 19.4.2024 in Vol 8 (2024)
A Health Information Technology Protocol to Enhance Colorectal Cancer Screening
Authors of this article:

Research Letter
- Adam Baus 1 * , MA, MPH, PhD ;
- Dannell D Boatman 2 * , MS, EdD ;
- Andrea Calkins 1 * , MPH ;
- Cecil Pollard 1 * , MA ;
- Mary Ellen Conn 2 * , MS ;
- Sujha Subramanian 3 * , MA, PhD ;
- Stephenie Kennedy-Rea 2 * , MA, EdD
1 Department of Social and Behavioral Sciences, School of Public Health, West Virginia University, Morgantown, WV, United States
2 Cancer Prevention and Control, West Virginia University Cancer Institute, Morgantown, WV, United States
3 Implenomics, Dover, DE, United States
*all authors contributed equally
Corresponding Author:
Adam Baus, MA, MPH, PhD
Department of Social and Behavioral Sciences
School of Public Health
West Virginia University
64 Medical Center Drive
PO Box 9190
Morgantown, WV, 26506
United States
Phone: 1 304 293 1083
Fax:1 304 293 6685
Email: [email protected]
This study addresses barriers to electronic health records–based colorectal cancer screening and follow-up in primary care through the development and implementation of a health information technology protocol.
Introduction
Cancer is a pressing global public health problem and the second leading cause of death in the United States, accounting for an estimated 1670 deaths daily [ 1 ]. Colorectal cancer (CRC) is the third most commonly diagnosed cancer, the second leading cause of cancer death worldwide [ 2 ], and the third most common cause of cancer-related deaths in the United States [ 3 ]. More effective use of health information technology (HIT), including electronic health records (EHRs), can aid in improving CRC screening and care [ 4 ]. Studies from as early as the 1990s have shown that EHRs and associated clinical decision support tools have promise in helping with patient care and population health needs [ 5 ]. However, barriers like clinician readiness [ 6 ] and clinical workflow integration [ 7 ] hinder EHRs’ full benefits. This study aims to address barriers to EHR-based CRC screening and follow-up through the development and implementation of a universally applicable EHR protocol tailored to identify and overcome practice workflow and EHR challenges.
This study used a mixed methods approach, involving quantitative and qualitative data collection techniques, conducted across 3 diverse health systems in West Virginia to develop and implement an EHR protocol for CRC screening and follow-up. These health systems were purposefully chosen to encompass diverse sizes, organizational structures, geographic locations, patient demographics, and EHR preferences, thereby supporting the generalizability of the study’s findings. These included a free and charitable clinic, a larger, urban, federally qualified health center, and a smaller, rural, federally qualified health center. Key stakeholders, including health care administrators, clinicians, and information technology personnel, were identified as potential participants. This study was conducted from April 2021 through April 2022. Implementation mapping methodology guided the assessment of current CRC screening practices and the development, implementation, and evaluation of the EHR protocol. Data collection tools were pilot tested in Health System A to assess their reliability, validity, and feasibility, then refined prior to full implementation in Health Systems B and C to ensure quality and effectiveness in data collection. Evaluation of the protocol’s acceptability, appropriateness, and feasibility was conducted using the Acceptability of Intervention Measure (AIM), Intervention Appropriateness Measure (IAM), and Feasibility of Intervention Measure (FIM). Technical issues during the study were resolved collaboratively by the research team and technical staff through troubleshooting, protocol adjustments, and ongoing support.
Ethical Considerations
This study received ethics approval from the West Virginia University Institutional Review Board (protocol number 2107363377).
The development of the EHR protocol involved a collaborative process between the research team and key stakeholders from participating health systems. Initial assessments revealed common challenges in CRC screening and follow-up across the diverse settings, including issues related to data quality, workflow inefficiencies, and underutilization of EHR functionalities. Based on these findings, a draft protocol was formulated, emphasizing strategies to enhance EHR data quality and optimization specifically tailored to address the identified barriers. The protocol comprised three key components: (1) Quality Improvement Activities , guiding clinic staff through a Plan-Do-Study-Act cycle to identify and mitigate data entry errors; (2) EHR Optimization Factors , highlighting specific EHR features supporting CRC screening and follow-up when effectively used; and (3) Health Information Technology Assessment , facilitating structured discussions on EHR use roles, office workflows, knowledge, skills, abilities, challenges, and improvement opportunities.
The developed protocol was implemented in Health Systems B and C following its refinement based on feedback from the development site (Health System A). Implementation involved training sessions for clinic staff on protocol utilization and ongoing support from the research team. Eight staff members from the participating health systems completed the AIM, IAM, and FIM assessments, providing valuable insights into their perceptions of the protocol. The mean scores from AIM (mean 16.00, SD 4.24), IAM (mean 15.80, SD 4.54), and FIM (mean 16.80, SD 4.66) indicate favorable perceptions of protocol feasibility, acceptability, and appropriateness. Qualitative feedback from participants further supported the positive reception of the protocol, with respondents expressing satisfaction with its efficacy and intentions to integrate it into their clinical practices. All respondents indicated that they would use or would consider using the protocol within their clinics again. Open-ended responses included “very pleased with the protocol and leveraging EHR/staff/outreach” and “plan to now identify and track to completion of CRC testing.”
The results demonstrate the successful development and initial implementation of an EHR protocol aimed at enhancing CRC screening in primary care settings. The protocol’s favorable reception by clinic staff, as indicated by high scores on acceptability, appropriateness, and feasibility measures, suggests its potential effectiveness in addressing identified barriers. The diverse representation of health systems and EHR platforms involved in the study enhances the generalizability of findings. Limitations include the small sample size and the focus on a specific geographic region. Future research will assess the protocol’s performance across additional EHR systems and health care settings for enhanced scalability and further evaluate the protocol’s impact on CRC screening outcomes.
Acknowledgments
The authors acknowledge the funding and support from the Research Triangle Institute (grant 1-312-0216648-66244L).
Conflicts of Interest
None declared.
- Siegel RL, Miller KD, Fuchs HE, Jemal A. Cancer statistics, 2022. CA Cancer J Clin. Jan 2022;72(1):7-33. [ FREE Full text ] [ CrossRef ] [ Medline ]
- Morgan E, Arnold M, Gini A, Lorenzoni V, Cabasag CJ, Laversanne M, et al. Global burden of colorectal cancer in 2020 and 2040: incidence and mortality estimates from GLOBOCAN. Gut. Feb 2023;72(2):338-344. [ CrossRef ] [ Medline ]
- Division of Cancer Prevention and Control. Colorectal cancer statistics. Centers for Disease Control and Prevention. 2023. URL: https://www.cdc.gov/cancer/colorectal/statistics/index.htm [accessed 2023-12-04]
- Baus A, Wright L, Kennedy-Rea S, Conn ME, Eason S, Boatman D, et al. Leveraging electronic health records data for enhanced colorectal cancer screening efforts. J Appalach Health. 2020;2(4):53-63. [ FREE Full text ] [ CrossRef ] [ Medline ]
- Atasoy H, Greenwood BN, McCullough JS. The digitization of patient care: a review of the effects of electronic health records on health care quality and utilization. Annu Rev Public Health. Apr 01, 2019;40(1):487-500. [ FREE Full text ] [ CrossRef ] [ Medline ]
- Bates DW. Physicians and ambulatory electronic health records. Health Aff (Millwood). Sep 2005;24(5):1180-1189. [ CrossRef ] [ Medline ]
- Hersh W, Weiner M, Embi P, Logan JR, Payne PRO, Bernstam EV, et al. Caveats for the use of operational electronic health record data in comparative effectiveness research. Med Care. Aug 2013;51(8 Suppl 3):S30-S37. [ FREE Full text ] [ CrossRef ] [ Medline ]
Abbreviations
Edited by A Mavragani; submitted 08.12.23; peer-reviewed by Y Chu, A Banerjee; comments to author 05.02.24; revised version received 12.02.24; accepted 04.04.24; published 19.04.24.
©Adam Baus, Dannell D Boatman, Andrea Calkins, Cecil Pollard, Mary Ellen Conn, Sujha Subramanian, Stephenie Kennedy-Rea. Originally published in JMIR Formative Research (https://formative.jmir.org), 19.04.2024.
This is an open-access article distributed under the terms of the Creative Commons Attribution License (https://creativecommons.org/licenses/by/4.0/), which permits unrestricted use, distribution, and reproduction in any medium, provided the original work, first published in JMIR Formative Research, is properly cited. The complete bibliographic information, a link to the original publication on https://formative.jmir.org, as well as this copyright and license information must be included.

Clinical Research Coordinator I
Apply now Job no: 531175 Work type: Staff Full-Time Location: Main Campus (Gainesville, FL) Categories: Grant or Research Administration, Health Care Administration/Support Department: 29770100 - MD-Transplant Center - Admin
Advertised: 16 Apr 2024 Eastern Daylight Time Applications close: 23 Apr 2024 Eastern Daylight Time
Back to search results Apply now Refer a friend
Search results
Current opportunities.
Powered by PageUp
Refine search
- Staff Full-Time 1
- Grant or Research Administration 1
- Health Care Administration/Support 1
- Main Campus (Gainesville, FL) 1
- 29770100 - MD-Transplant Center - Admin 1
- Frequently Asked Questions
- Veteran Preference
- Applicant Tutorial
- UF Hiring Policies
- Disclosure of Campus Security Policy and Campus Crime Statistics
- Institute of Food and Agricultural Sciences Faculty Positions
- Labor Condition Application (ETA Form 9035): Notice of Filings
- Application for Permanent Employment Certification (ETA Form 9089): Notice of Job Availability
- Search Committee Public Meeting Notices
- Accessibility at UF
- Drug and Alcohol Abuse Prevention Program (DAAPP)
- Drug-Free Workplace
Equal Opportunity Employer
The University is committed to non-discrimination with respect to race, creed, color, religion, age, disability, sex, sexual orientation, gender identity and expression, marital status, national origin, political opinions or affiliations, genetic information and veteran status in all aspects of employment including recruitment, hiring, promotions, transfers, discipline, terminations, wage and salary administration, benefits, and training.
We will email you new jobs that match this search.
Ok, we will send you jobs like this.
The email address was invalid, please check for errors.
You must agree to the privacy statement
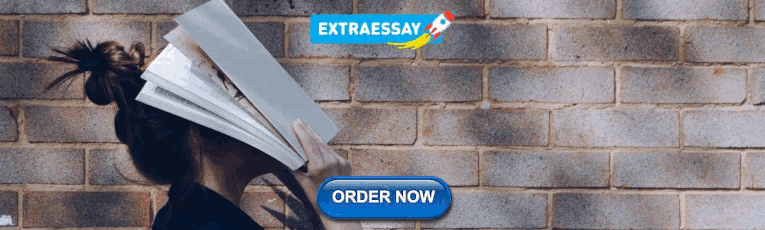
IMAGES
VIDEO
COMMENTS
Data Collection | Definition, Methods & Examples. Published on June 5, 2020 by Pritha Bhandari.Revised on June 21, 2023. Data collection is a systematic process of gathering observations or measurements. Whether you are performing research for business, governmental or academic purposes, data collection allows you to gain first-hand knowledge and original insights into your research problem.
This plan is called a protocol. Definition 10.1 (Protocol) A protocol is a procedure documenting the details of the design and implementation of studies, and for data collection. Unforeseen complications are not unusual, so often a pilot study (or a practice run) is conducted before the real data collection, to identify problems with the study ...
PDF | Learn how to choose the best data collection methods and tools for your research project, with examples and tips from ResearchGate experts. | Download and read the full-text PDF.
The methodology should be standardized and clearly defined if multiple sites are engaged in a specified protocol. 6. Safety Considerations. The safety of participants is a top-tier priority while conducting clinical research. Safety aspects of the research should be scrutinized and provided in the research protocol. 7.
research data. That is, they decide what methods of data collection (i.e., tests, questionnaires, interviews, focus groups, observations, constructed, secondary, and existing data) they will phys-ically use to obtain the research data. As you read this chapter, keep in mind the fundamental principle of mixed research originally defined in ...
We offer best-practice recommendations for journal reviewers, editors, and authors regarding data collection and preparation. Our recommendations are applicable to research adopting different epistemological and ontological perspectives—including both quantitative and qualitative approaches—as well as research addressing micro (i.e., individuals, teams) and macro (i.e., organizations ...
In this Rip Out we focus on data collection, but in qualitative research, the entire project must be considered. 1, 2 Careful design of the data collection phase requires the following: deciding who will do what, ... organization policies and protocols, letters, records, films, photographs, art, meeting notes, or checklists. The development of ...
Data collection is the process of gathering information from various sources via different research methods and consolidating it into a single database or repository so researchers can use it for further analysis. Data collection aims to provide information that individuals, businesses, and organizations can use to solve problems, track progress, and make decisions.
The most common methods of data collection are document study, (non-) participant observations, semi-structured interviews and focus groups. For data analysis, field-notes and audio-recordings are transcribed into protocols and transcripts, and coded using qualitative data management software. ... Protocols of qualitative research can be ...
Objective monitoring of physical activity and sedentary behaviour is a multi-stage process that includes planning and implementing protocols for data collection, management and treatment. Protocols represent the system of rules that epidemiologists and other researchers follow to ensure data quality and ultimately confidence in the study ...
Data Collection, Research Methodology, Data Collection Methods, Academic Research Paper, Data Collection Techniques. I. INTRODUCTION Different methods for gathering information regarding specific variables of the study aiming to employ them in the data analysis phase to achieve the results of the study, gain the answer of the research ...
Open in a separate window. First section: Description of the core center, contacts of the investigator/s, quantification of the involved centers. A research protocol must start from the definition of the coordinator of the whole study: all the details of the main investigator must be reported in the first paragraph.
Data Collection - Definition. The process of gathering and measuring information on variables of interest, in an established systematic fashion that enables one to answer stated research questions, test hypotheses, and evaluate outcomes. U.S. Department of Health & Human Services.
Step 2: Choose your data collection method. Based on the data you want to collect, decide which method is best suited for your research. Experimental research is primarily a quantitative method. Interviews, focus groups, and ethnographies are qualitative methods. Surveys, observations, archival research, and secondary data collection can be ...
Collect Data. Data will be collected and subsequently analyzed during the Manage stage, using the protocol for collecting data developed in the Plan/Propose stage and the processes and technical resources established during the Setup stage. Strict adherence to the data collection protocol design is critical to assuring that the data collected ...
In the EWC, standardization is evident in the creation of the data collection protocol with its manual of procedures, data collection forms, and policies for study practices, including data ...
Prior to data collection, we collaborated with the oncology social worker on the study team to co-create two distress protocols tailored to participant groups - patients/care partners and clinicians. For each protocol, we developed three sections: a triage pathway with scripted language, a tiered list of available referral resources for ...
Use this protocol template if your study will use ONLY: Data (retrospective or prospective data collection study with no patient contact). Samples from a biobank or leftover clinical samples that typically do not require. obtaining consent/authorization from subjects. If your study involves recruitment of subjects, please use the Prospective ...
Data collection is the process of gathering and collecting information from various sources to analyze and make informed decisions based on the data collected. This can involve various methods, such as surveys, interviews, experiments, and observation. In order for data collection to be effective, it is important to have a clear understanding ...
Abstract. In this article we provide an overview of the different data collection approaches that are commonly utilized in carrying out clinical, public health, and translational research. We discuss several of the factors researchers need to consider in using data collected in questionnaire surveys, from proxy informants, through the review of ...
back and forth between sampling, data collection, and data anal ysis, until data saturation is achieved, when there are no more information to be emerged (Bernard, 2002; Polit & B eck, 2006).
The data collection protocol. The data collection protocol is the procedure for executing the above steps of the Plan to collect and record the data. It deals with management and adminstrative issues such as who does what and when. It also includes a plan for monitoring the data as they are collected to ensure quality. Michelson gives us no ...
Focus groups are an appropriate method of data collection to answer the study research questions seeking to explore views and perspectives of service users and clinicians, where our analysis will aim to define key themes and points of consensus or divergence gathered through interaction,23 24 drawing on participants own perspectives and choice ...
Background: This is a research proposal for a case study to explore how a national organization works in partnership with people with lived experience in national mental health improvement programs. Quality improvement is considered a key solution to addressing challenges within health care, and in Scotland, there are significant efforts to use quality improvement as a means of improving ...
This study addresses barriers to electronic health records-based colorectal cancer screening and follow-up in primary care through the development and implementation of a health information technology protocol.
Coordination of Protocol Subjects & Data Collection - Performs subject screening and consent for clinical protocols under direction of Principal Investigator. - Serves as patient resource and educator for information regarding the study or clinical symptoms. - Prepares and ships central laboratory samples as per protocol requirements.
Data collection was standardized by using a data collection form to gather information from the medical records included in the study. All statistical analyses were performed using SPSS (version v27), and survival was assessed using a regression analysis of the number of days from the time of diagnosis to the most recent visit against the type ...