- Bipolar Disorder
- Therapy Center
- When To See a Therapist
- Types of Therapy
- Best Online Therapy
- Best Couples Therapy
- Best Family Therapy
- Managing Stress
- Sleep and Dreaming
- Understanding Emotions
- Self-Improvement
- Healthy Relationships
- Student Resources
- Personality Types
- Verywell Mind Insights
- 2023 Verywell Mind 25
- Mental Health in the Classroom
- Editorial Process
- Meet Our Review Board
- Crisis Support
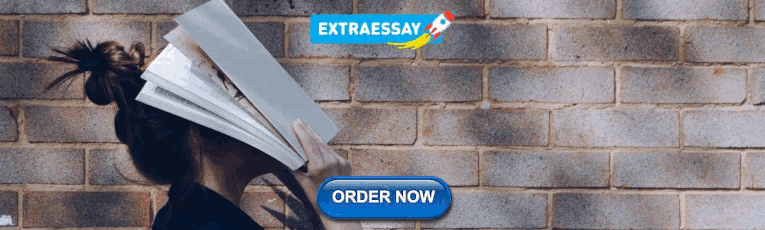
How to Write a Great Hypothesis
Hypothesis Format, Examples, and Tips
Kendra Cherry, MS, is a psychosocial rehabilitation specialist, psychology educator, and author of the "Everything Psychology Book."
:max_bytes(150000):strip_icc():format(webp)/IMG_9791-89504ab694d54b66bbd72cb84ffb860e.jpg)
Amy Morin, LCSW, is a psychotherapist and international bestselling author. Her books, including "13 Things Mentally Strong People Don't Do," have been translated into more than 40 languages. Her TEDx talk, "The Secret of Becoming Mentally Strong," is one of the most viewed talks of all time.
:max_bytes(150000):strip_icc():format(webp)/VW-MIND-Amy-2b338105f1ee493f94d7e333e410fa76.jpg)
Verywell / Alex Dos Diaz
- The Scientific Method
Hypothesis Format
Falsifiability of a hypothesis, operational definitions, types of hypotheses, hypotheses examples.
- Collecting Data
Frequently Asked Questions
A hypothesis is a tentative statement about the relationship between two or more variables. It is a specific, testable prediction about what you expect to happen in a study.
One hypothesis example would be a study designed to look at the relationship between sleep deprivation and test performance might have a hypothesis that states: "This study is designed to assess the hypothesis that sleep-deprived people will perform worse on a test than individuals who are not sleep-deprived."
This article explores how a hypothesis is used in psychology research, how to write a good hypothesis, and the different types of hypotheses you might use.
The Hypothesis in the Scientific Method
In the scientific method , whether it involves research in psychology, biology, or some other area, a hypothesis represents what the researchers think will happen in an experiment. The scientific method involves the following steps:
- Forming a question
- Performing background research
- Creating a hypothesis
- Designing an experiment
- Collecting data
- Analyzing the results
- Drawing conclusions
- Communicating the results
The hypothesis is a prediction, but it involves more than a guess. Most of the time, the hypothesis begins with a question which is then explored through background research. It is only at this point that researchers begin to develop a testable hypothesis. Unless you are creating an exploratory study, your hypothesis should always explain what you expect to happen.
In a study exploring the effects of a particular drug, the hypothesis might be that researchers expect the drug to have some type of effect on the symptoms of a specific illness. In psychology, the hypothesis might focus on how a certain aspect of the environment might influence a particular behavior.
Remember, a hypothesis does not have to be correct. While the hypothesis predicts what the researchers expect to see, the goal of the research is to determine whether this guess is right or wrong. When conducting an experiment, researchers might explore a number of factors to determine which ones might contribute to the ultimate outcome.
In many cases, researchers may find that the results of an experiment do not support the original hypothesis. When writing up these results, the researchers might suggest other options that should be explored in future studies.
In many cases, researchers might draw a hypothesis from a specific theory or build on previous research. For example, prior research has shown that stress can impact the immune system. So a researcher might hypothesize: "People with high-stress levels will be more likely to contract a common cold after being exposed to the virus than people who have low-stress levels."
In other instances, researchers might look at commonly held beliefs or folk wisdom. "Birds of a feather flock together" is one example of folk wisdom that a psychologist might try to investigate. The researcher might pose a specific hypothesis that "People tend to select romantic partners who are similar to them in interests and educational level."
Elements of a Good Hypothesis
So how do you write a good hypothesis? When trying to come up with a hypothesis for your research or experiments, ask yourself the following questions:
- Is your hypothesis based on your research on a topic?
- Can your hypothesis be tested?
- Does your hypothesis include independent and dependent variables?
Before you come up with a specific hypothesis, spend some time doing background research. Once you have completed a literature review, start thinking about potential questions you still have. Pay attention to the discussion section in the journal articles you read . Many authors will suggest questions that still need to be explored.
To form a hypothesis, you should take these steps:
- Collect as many observations about a topic or problem as you can.
- Evaluate these observations and look for possible causes of the problem.
- Create a list of possible explanations that you might want to explore.
- After you have developed some possible hypotheses, think of ways that you could confirm or disprove each hypothesis through experimentation. This is known as falsifiability.
In the scientific method , falsifiability is an important part of any valid hypothesis. In order to test a claim scientifically, it must be possible that the claim could be proven false.
Students sometimes confuse the idea of falsifiability with the idea that it means that something is false, which is not the case. What falsifiability means is that if something was false, then it is possible to demonstrate that it is false.
One of the hallmarks of pseudoscience is that it makes claims that cannot be refuted or proven false.
A variable is a factor or element that can be changed and manipulated in ways that are observable and measurable. However, the researcher must also define how the variable will be manipulated and measured in the study.
For example, a researcher might operationally define the variable " test anxiety " as the results of a self-report measure of anxiety experienced during an exam. A "study habits" variable might be defined by the amount of studying that actually occurs as measured by time.
These precise descriptions are important because many things can be measured in a number of different ways. One of the basic principles of any type of scientific research is that the results must be replicable. By clearly detailing the specifics of how the variables were measured and manipulated, other researchers can better understand the results and repeat the study if needed.
Some variables are more difficult than others to define. How would you operationally define a variable such as aggression ? For obvious ethical reasons, researchers cannot create a situation in which a person behaves aggressively toward others.
In order to measure this variable, the researcher must devise a measurement that assesses aggressive behavior without harming other people. In this situation, the researcher might utilize a simulated task to measure aggressiveness.
Hypothesis Checklist
- Does your hypothesis focus on something that you can actually test?
- Does your hypothesis include both an independent and dependent variable?
- Can you manipulate the variables?
- Can your hypothesis be tested without violating ethical standards?
The hypothesis you use will depend on what you are investigating and hoping to find. Some of the main types of hypotheses that you might use include:
- Simple hypothesis : This type of hypothesis suggests that there is a relationship between one independent variable and one dependent variable.
- Complex hypothesis : This type of hypothesis suggests a relationship between three or more variables, such as two independent variables and a dependent variable.
- Null hypothesis : This hypothesis suggests no relationship exists between two or more variables.
- Alternative hypothesis : This hypothesis states the opposite of the null hypothesis.
- Statistical hypothesis : This hypothesis uses statistical analysis to evaluate a representative sample of the population and then generalizes the findings to the larger group.
- Logical hypothesis : This hypothesis assumes a relationship between variables without collecting data or evidence.
A hypothesis often follows a basic format of "If {this happens} then {this will happen}." One way to structure your hypothesis is to describe what will happen to the dependent variable if you change the independent variable .
The basic format might be: "If {these changes are made to a certain independent variable}, then we will observe {a change in a specific dependent variable}."
A few examples of simple hypotheses:
- "Students who eat breakfast will perform better on a math exam than students who do not eat breakfast."
- Complex hypothesis: "Students who experience test anxiety before an English exam will get lower scores than students who do not experience test anxiety."
- "Motorists who talk on the phone while driving will be more likely to make errors on a driving course than those who do not talk on the phone."
Examples of a complex hypothesis include:
- "People with high-sugar diets and sedentary activity levels are more likely to develop depression."
- "Younger people who are regularly exposed to green, outdoor areas have better subjective well-being than older adults who have limited exposure to green spaces."
Examples of a null hypothesis include:
- "Children who receive a new reading intervention will have scores different than students who do not receive the intervention."
- "There will be no difference in scores on a memory recall task between children and adults."
Examples of an alternative hypothesis:
- "Children who receive a new reading intervention will perform better than students who did not receive the intervention."
- "Adults will perform better on a memory task than children."
Collecting Data on Your Hypothesis
Once a researcher has formed a testable hypothesis, the next step is to select a research design and start collecting data. The research method depends largely on exactly what they are studying. There are two basic types of research methods: descriptive research and experimental research.
Descriptive Research Methods
Descriptive research such as case studies , naturalistic observations , and surveys are often used when it would be impossible or difficult to conduct an experiment . These methods are best used to describe different aspects of a behavior or psychological phenomenon.
Once a researcher has collected data using descriptive methods, a correlational study can then be used to look at how the variables are related. This type of research method might be used to investigate a hypothesis that is difficult to test experimentally.
Experimental Research Methods
Experimental methods are used to demonstrate causal relationships between variables. In an experiment, the researcher systematically manipulates a variable of interest (known as the independent variable) and measures the effect on another variable (known as the dependent variable).
Unlike correlational studies, which can only be used to determine if there is a relationship between two variables, experimental methods can be used to determine the actual nature of the relationship—whether changes in one variable actually cause another to change.
A Word From Verywell
The hypothesis is a critical part of any scientific exploration. It represents what researchers expect to find in a study or experiment. In situations where the hypothesis is unsupported by the research, the research still has value. Such research helps us better understand how different aspects of the natural world relate to one another. It also helps us develop new hypotheses that can then be tested in the future.
Some examples of how to write a hypothesis include:
- "Staying up late will lead to worse test performance the next day."
- "People who consume one apple each day will visit the doctor fewer times each year."
- "Breaking study sessions up into three 20-minute sessions will lead to better test results than a single 60-minute study session."
The four parts of a hypothesis are:
- The research question
- The independent variable (IV)
- The dependent variable (DV)
- The proposed relationship between the IV and DV
Castillo M. The scientific method: a need for something better? . AJNR Am J Neuroradiol. 2013;34(9):1669-71. doi:10.3174/ajnr.A3401
Nevid J. Psychology: Concepts and Applications. Wadworth, 2013.
By Kendra Cherry, MSEd Kendra Cherry, MS, is a psychosocial rehabilitation specialist, psychology educator, and author of the "Everything Psychology Book."
- Affiliate Program

- UNITED STATES
- 台灣 (TAIWAN)
- TÜRKIYE (TURKEY)
- Academic Editing Services
- - Research Paper
- - Journal Manuscript
- - Dissertation
- - College & University Assignments
- Admissions Editing Services
- - Application Essay
- - Personal Statement
- - Recommendation Letter
- - Cover Letter
- - CV/Resume
- Business Editing Services
- - Business Documents
- - Report & Brochure
- - Website & Blog
- Writer Editing Services
- - Script & Screenplay
- Our Editors
- Client Reviews
- Editing & Proofreading Prices
- Wordvice Points
- Partner Discount
- Plagiarism Checker
- APA Citation Generator
- MLA Citation Generator
- Chicago Citation Generator
- Vancouver Citation Generator
- - APA Style
- - MLA Style
- - Chicago Style
- - Vancouver Style
- Writing & Editing Guide
- Academic Resources
- Admissions Resources
How to Write a Research Hypothesis: Good & Bad Examples
What is a research hypothesis?
A research hypothesis is an attempt at explaining a phenomenon or the relationships between phenomena/variables in the real world. Hypotheses are sometimes called “educated guesses”, but they are in fact (or let’s say they should be) based on previous observations, existing theories, scientific evidence, and logic. A research hypothesis is also not a prediction—rather, predictions are ( should be) based on clearly formulated hypotheses. For example, “We tested the hypothesis that KLF2 knockout mice would show deficiencies in heart development” is an assumption or prediction, not a hypothesis.
The research hypothesis at the basis of this prediction is “the product of the KLF2 gene is involved in the development of the cardiovascular system in mice”—and this hypothesis is probably (hopefully) based on a clear observation, such as that mice with low levels of Kruppel-like factor 2 (which KLF2 codes for) seem to have heart problems. From this hypothesis, you can derive the idea that a mouse in which this particular gene does not function cannot develop a normal cardiovascular system, and then make the prediction that we started with.
What is the difference between a hypothesis and a prediction?
You might think that these are very subtle differences, and you will certainly come across many publications that do not contain an actual hypothesis or do not make these distinctions correctly. But considering that the formulation and testing of hypotheses is an integral part of the scientific method, it is good to be aware of the concepts underlying this approach. The two hallmarks of a scientific hypothesis are falsifiability (an evaluation standard that was introduced by the philosopher of science Karl Popper in 1934) and testability —if you cannot use experiments or data to decide whether an idea is true or false, then it is not a hypothesis (or at least a very bad one).
So, in a nutshell, you (1) look at existing evidence/theories, (2) come up with a hypothesis, (3) make a prediction that allows you to (4) design an experiment or data analysis to test it, and (5) come to a conclusion. Of course, not all studies have hypotheses (there is also exploratory or hypothesis-generating research), and you do not necessarily have to state your hypothesis as such in your paper.
But for the sake of understanding the principles of the scientific method, let’s first take a closer look at the different types of hypotheses that research articles refer to and then give you a step-by-step guide for how to formulate a strong hypothesis for your own paper.
Types of Research Hypotheses
Hypotheses can be simple , which means they describe the relationship between one single independent variable (the one you observe variations in or plan to manipulate) and one single dependent variable (the one you expect to be affected by the variations/manipulation). If there are more variables on either side, you are dealing with a complex hypothesis. You can also distinguish hypotheses according to the kind of relationship between the variables you are interested in (e.g., causal or associative ). But apart from these variations, we are usually interested in what is called the “alternative hypothesis” and, in contrast to that, the “null hypothesis”. If you think these two should be listed the other way round, then you are right, logically speaking—the alternative should surely come second. However, since this is the hypothesis we (as researchers) are usually interested in, let’s start from there.
Alternative Hypothesis
If you predict a relationship between two variables in your study, then the research hypothesis that you formulate to describe that relationship is your alternative hypothesis (usually H1 in statistical terms). The goal of your hypothesis testing is thus to demonstrate that there is sufficient evidence that supports the alternative hypothesis, rather than evidence for the possibility that there is no such relationship. The alternative hypothesis is usually the research hypothesis of a study and is based on the literature, previous observations, and widely known theories.
Null Hypothesis
The hypothesis that describes the other possible outcome, that is, that your variables are not related, is the null hypothesis ( H0 ). Based on your findings, you choose between the two hypotheses—usually that means that if your prediction was correct, you reject the null hypothesis and accept the alternative. Make sure, however, that you are not getting lost at this step of the thinking process: If your prediction is that there will be no difference or change, then you are trying to find support for the null hypothesis and reject H1.
Directional Hypothesis
While the null hypothesis is obviously “static”, the alternative hypothesis can specify a direction for the observed relationship between variables—for example, that mice with higher expression levels of a certain protein are more active than those with lower levels. This is then called a one-tailed hypothesis.
Another example for a directional one-tailed alternative hypothesis would be that
H1: Attending private classes before important exams has a positive effect on performance.
Your null hypothesis would then be that
H0: Attending private classes before important exams has no/a negative effect on performance.
Nondirectional Hypothesis
A nondirectional hypothesis does not specify the direction of the potentially observed effect, only that there is a relationship between the studied variables—this is called a two-tailed hypothesis. For instance, if you are studying a new drug that has shown some effects on pathways involved in a certain condition (e.g., anxiety) in vitro in the lab, but you can’t say for sure whether it will have the same effects in an animal model or maybe induce other/side effects that you can’t predict and potentially increase anxiety levels instead, you could state the two hypotheses like this:
H1: The only lab-tested drug (somehow) affects anxiety levels in an anxiety mouse model.
You then test this nondirectional alternative hypothesis against the null hypothesis:
H0: The only lab-tested drug has no effect on anxiety levels in an anxiety mouse model.
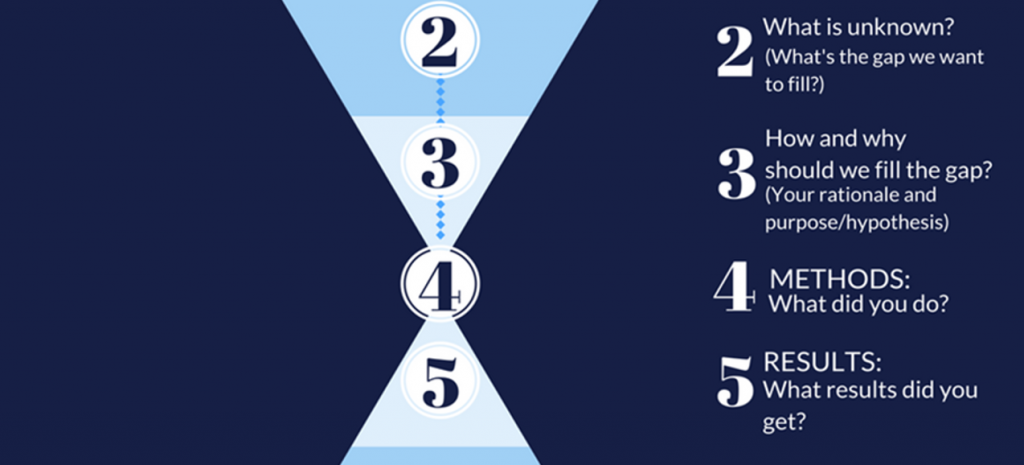
How to Write a Hypothesis for a Research Paper
Now that we understand the important distinctions between different kinds of research hypotheses, let’s look at a simple process of how to write a hypothesis.
Writing a Hypothesis Step:1
Ask a question, based on earlier research. Research always starts with a question, but one that takes into account what is already known about a topic or phenomenon. For example, if you are interested in whether people who have pets are happier than those who don’t, do a literature search and find out what has already been demonstrated. You will probably realize that yes, there is quite a bit of research that shows a relationship between happiness and owning a pet—and even studies that show that owning a dog is more beneficial than owning a cat ! Let’s say you are so intrigued by this finding that you wonder:
What is it that makes dog owners even happier than cat owners?
Let’s move on to Step 2 and find an answer to that question.
Writing a Hypothesis Step 2:
Formulate a strong hypothesis by answering your own question. Again, you don’t want to make things up, take unicorns into account, or repeat/ignore what has already been done. Looking at the dog-vs-cat papers your literature search returned, you see that most studies are based on self-report questionnaires on personality traits, mental health, and life satisfaction. What you don’t find is any data on actual (mental or physical) health measures, and no experiments. You therefore decide to make a bold claim come up with the carefully thought-through hypothesis that it’s maybe the lifestyle of the dog owners, which includes walking their dog several times per day, engaging in fun and healthy activities such as agility competitions, and taking them on trips, that gives them that extra boost in happiness. You could therefore answer your question in the following way:
Dog owners are happier than cat owners because of the dog-related activities they engage in.
Now you have to verify that your hypothesis fulfills the two requirements we introduced at the beginning of this resource article: falsifiability and testability . If it can’t be wrong and can’t be tested, it’s not a hypothesis. We are lucky, however, because yes, we can test whether owning a dog but not engaging in any of those activities leads to lower levels of happiness or well-being than owning a dog and playing and running around with them or taking them on trips.
Writing a Hypothesis Step 3:
Make your predictions and define your variables. We have verified that we can test our hypothesis, but now we have to define all the relevant variables, design our experiment or data analysis, and make precise predictions. You could, for example, decide to study dog owners (not surprising at this point), let them fill in questionnaires about their lifestyle as well as their life satisfaction (as other studies did), and then compare two groups of active and inactive dog owners. Alternatively, if you want to go beyond the data that earlier studies produced and analyzed and directly manipulate the activity level of your dog owners to study the effect of that manipulation, you could invite them to your lab, select groups of participants with similar lifestyles, make them change their lifestyle (e.g., couch potato dog owners start agility classes, very active ones have to refrain from any fun activities for a certain period of time) and assess their happiness levels before and after the intervention. In both cases, your independent variable would be “ level of engagement in fun activities with dog” and your dependent variable would be happiness or well-being .
Examples of a Good and Bad Hypothesis
Let’s look at a few examples of good and bad hypotheses to get you started.
Good Hypothesis Examples
Bad hypothesis examples, tips for writing a research hypothesis.
If you understood the distinction between a hypothesis and a prediction we made at the beginning of this article, then you will have no problem formulating your hypotheses and predictions correctly. To refresh your memory: We have to (1) look at existing evidence, (2) come up with a hypothesis, (3) make a prediction, and (4) design an experiment. For example, you could summarize your dog/happiness study like this:
(1) While research suggests that dog owners are happier than cat owners, there are no reports on what factors drive this difference. (2) We hypothesized that it is the fun activities that many dog owners (but very few cat owners) engage in with their pets that increases their happiness levels. (3) We thus predicted that preventing very active dog owners from engaging in such activities for some time and making very inactive dog owners take up such activities would lead to an increase and decrease in their overall self-ratings of happiness, respectively. (4) To test this, we invited dog owners into our lab, assessed their mental and emotional well-being through questionnaires, and then assigned them to an “active” and an “inactive” group, depending on…
Note that you use “we hypothesize” only for your hypothesis, not for your experimental prediction, and “would” or “if – then” only for your prediction, not your hypothesis. A hypothesis that states that something “would” affect something else sounds as if you don’t have enough confidence to make a clear statement—in which case you can’t expect your readers to believe in your research either. Write in the present tense, don’t use modal verbs that express varying degrees of certainty (such as may, might, or could ), and remember that you are not drawing a conclusion while trying not to exaggerate but making a clear statement that you then, in a way, try to disprove . And if that happens, that is not something to fear but an important part of the scientific process.
Similarly, don’t use “we hypothesize” when you explain the implications of your research or make predictions in the conclusion section of your manuscript, since these are clearly not hypotheses in the true sense of the word. As we said earlier, you will find that many authors of academic articles do not seem to care too much about these rather subtle distinctions, but thinking very clearly about your own research will not only help you write better but also ensure that even that infamous Reviewer 2 will find fewer reasons to nitpick about your manuscript.
Perfect Your Manuscript With Professional Editing
Now that you know how to write a strong research hypothesis for your research paper, you might be interested in our free AI proofreader , Wordvice AI, which finds and fixes errors in grammar, punctuation, and word choice in academic texts. Or if you are interested in human proofreading , check out our English editing services , including research paper editing and manuscript editing .
On the Wordvice academic resources website , you can also find many more articles and other resources that can help you with writing the other parts of your research paper , with making a research paper outline before you put everything together, or with writing an effective cover letter once you are ready to submit.
- Resources Home 🏠
- Try SciSpace Copilot
- Search research papers
- Add Copilot Extension
- Try AI Detector
- Try Paraphraser
- Try Citation Generator
- April Papers
- June Papers
- July Papers

The Craft of Writing a Strong Hypothesis

Table of Contents
Writing a hypothesis is one of the essential elements of a scientific research paper. It needs to be to the point, clearly communicating what your research is trying to accomplish. A blurry, drawn-out, or complexly-structured hypothesis can confuse your readers. Or worse, the editor and peer reviewers.
A captivating hypothesis is not too intricate. This blog will take you through the process so that, by the end of it, you have a better idea of how to convey your research paper's intent in just one sentence.
What is a Hypothesis?
The first step in your scientific endeavor, a hypothesis, is a strong, concise statement that forms the basis of your research. It is not the same as a thesis statement , which is a brief summary of your research paper .
The sole purpose of a hypothesis is to predict your paper's findings, data, and conclusion. It comes from a place of curiosity and intuition . When you write a hypothesis, you're essentially making an educated guess based on scientific prejudices and evidence, which is further proven or disproven through the scientific method.
The reason for undertaking research is to observe a specific phenomenon. A hypothesis, therefore, lays out what the said phenomenon is. And it does so through two variables, an independent and dependent variable.
The independent variable is the cause behind the observation, while the dependent variable is the effect of the cause. A good example of this is “mixing red and blue forms purple.” In this hypothesis, mixing red and blue is the independent variable as you're combining the two colors at your own will. The formation of purple is the dependent variable as, in this case, it is conditional to the independent variable.
Different Types of Hypotheses

Types of hypotheses
Some would stand by the notion that there are only two types of hypotheses: a Null hypothesis and an Alternative hypothesis. While that may have some truth to it, it would be better to fully distinguish the most common forms as these terms come up so often, which might leave you out of context.
Apart from Null and Alternative, there are Complex, Simple, Directional, Non-Directional, Statistical, and Associative and casual hypotheses. They don't necessarily have to be exclusive, as one hypothesis can tick many boxes, but knowing the distinctions between them will make it easier for you to construct your own.
1. Null hypothesis
A null hypothesis proposes no relationship between two variables. Denoted by H 0 , it is a negative statement like “Attending physiotherapy sessions does not affect athletes' on-field performance.” Here, the author claims physiotherapy sessions have no effect on on-field performances. Even if there is, it's only a coincidence.
2. Alternative hypothesis
Considered to be the opposite of a null hypothesis, an alternative hypothesis is donated as H1 or Ha. It explicitly states that the dependent variable affects the independent variable. A good alternative hypothesis example is “Attending physiotherapy sessions improves athletes' on-field performance.” or “Water evaporates at 100 °C. ” The alternative hypothesis further branches into directional and non-directional.
- Directional hypothesis: A hypothesis that states the result would be either positive or negative is called directional hypothesis. It accompanies H1 with either the ‘<' or ‘>' sign.
- Non-directional hypothesis: A non-directional hypothesis only claims an effect on the dependent variable. It does not clarify whether the result would be positive or negative. The sign for a non-directional hypothesis is ‘≠.'
3. Simple hypothesis
A simple hypothesis is a statement made to reflect the relation between exactly two variables. One independent and one dependent. Consider the example, “Smoking is a prominent cause of lung cancer." The dependent variable, lung cancer, is dependent on the independent variable, smoking.
4. Complex hypothesis
In contrast to a simple hypothesis, a complex hypothesis implies the relationship between multiple independent and dependent variables. For instance, “Individuals who eat more fruits tend to have higher immunity, lesser cholesterol, and high metabolism.” The independent variable is eating more fruits, while the dependent variables are higher immunity, lesser cholesterol, and high metabolism.
5. Associative and casual hypothesis
Associative and casual hypotheses don't exhibit how many variables there will be. They define the relationship between the variables. In an associative hypothesis, changing any one variable, dependent or independent, affects others. In a casual hypothesis, the independent variable directly affects the dependent.
6. Empirical hypothesis
Also referred to as the working hypothesis, an empirical hypothesis claims a theory's validation via experiments and observation. This way, the statement appears justifiable and different from a wild guess.
Say, the hypothesis is “Women who take iron tablets face a lesser risk of anemia than those who take vitamin B12.” This is an example of an empirical hypothesis where the researcher the statement after assessing a group of women who take iron tablets and charting the findings.
7. Statistical hypothesis
The point of a statistical hypothesis is to test an already existing hypothesis by studying a population sample. Hypothesis like “44% of the Indian population belong in the age group of 22-27.” leverage evidence to prove or disprove a particular statement.
Characteristics of a Good Hypothesis
Writing a hypothesis is essential as it can make or break your research for you. That includes your chances of getting published in a journal. So when you're designing one, keep an eye out for these pointers:
- A research hypothesis has to be simple yet clear to look justifiable enough.
- It has to be testable — your research would be rendered pointless if too far-fetched into reality or limited by technology.
- It has to be precise about the results —what you are trying to do and achieve through it should come out in your hypothesis.
- A research hypothesis should be self-explanatory, leaving no doubt in the reader's mind.
- If you are developing a relational hypothesis, you need to include the variables and establish an appropriate relationship among them.
- A hypothesis must keep and reflect the scope for further investigations and experiments.
Separating a Hypothesis from a Prediction
Outside of academia, hypothesis and prediction are often used interchangeably. In research writing, this is not only confusing but also incorrect. And although a hypothesis and prediction are guesses at their core, there are many differences between them.
A hypothesis is an educated guess or even a testable prediction validated through research. It aims to analyze the gathered evidence and facts to define a relationship between variables and put forth a logical explanation behind the nature of events.
Predictions are assumptions or expected outcomes made without any backing evidence. They are more fictionally inclined regardless of where they originate from.
For this reason, a hypothesis holds much more weight than a prediction. It sticks to the scientific method rather than pure guesswork. "Planets revolve around the Sun." is an example of a hypothesis as it is previous knowledge and observed trends. Additionally, we can test it through the scientific method.
Whereas "COVID-19 will be eradicated by 2030." is a prediction. Even though it results from past trends, we can't prove or disprove it. So, the only way this gets validated is to wait and watch if COVID-19 cases end by 2030.
Finally, How to Write a Hypothesis
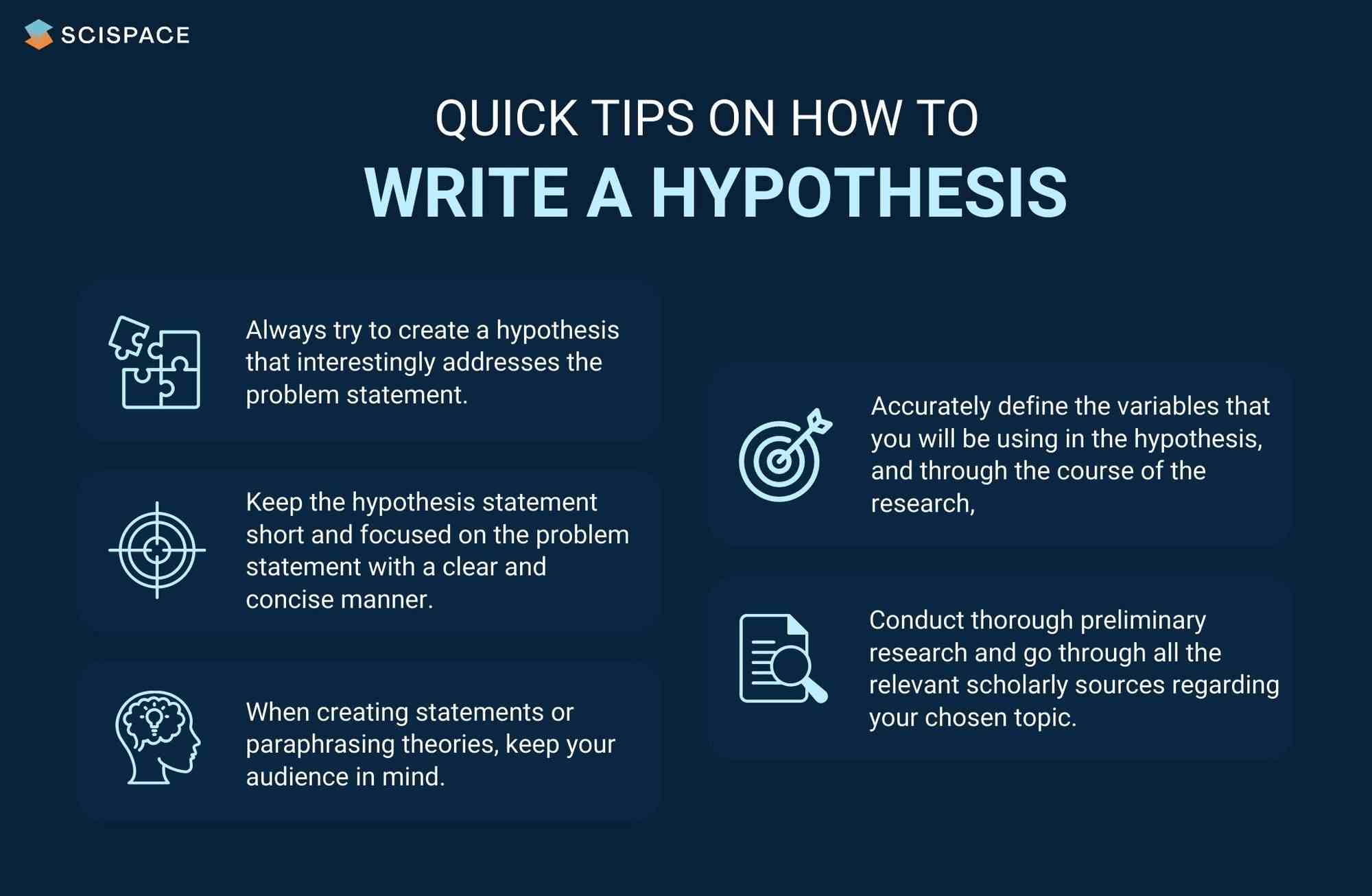
Quick tips on writing a hypothesis
1. Be clear about your research question
A hypothesis should instantly address the research question or the problem statement. To do so, you need to ask a question. Understand the constraints of your undertaken research topic and then formulate a simple and topic-centric problem. Only after that can you develop a hypothesis and further test for evidence.
2. Carry out a recce
Once you have your research's foundation laid out, it would be best to conduct preliminary research. Go through previous theories, academic papers, data, and experiments before you start curating your research hypothesis. It will give you an idea of your hypothesis's viability or originality.
Making use of references from relevant research papers helps draft a good research hypothesis. SciSpace Discover offers a repository of over 270 million research papers to browse through and gain a deeper understanding of related studies on a particular topic. Additionally, you can use SciSpace Copilot , your AI research assistant, for reading any lengthy research paper and getting a more summarized context of it. A hypothesis can be formed after evaluating many such summarized research papers. Copilot also offers explanations for theories and equations, explains paper in simplified version, allows you to highlight any text in the paper or clip math equations and tables and provides a deeper, clear understanding of what is being said. This can improve the hypothesis by helping you identify potential research gaps.
3. Create a 3-dimensional hypothesis
Variables are an essential part of any reasonable hypothesis. So, identify your independent and dependent variable(s) and form a correlation between them. The ideal way to do this is to write the hypothetical assumption in the ‘if-then' form. If you use this form, make sure that you state the predefined relationship between the variables.
In another way, you can choose to present your hypothesis as a comparison between two variables. Here, you must specify the difference you expect to observe in the results.
4. Write the first draft
Now that everything is in place, it's time to write your hypothesis. For starters, create the first draft. In this version, write what you expect to find from your research.
Clearly separate your independent and dependent variables and the link between them. Don't fixate on syntax at this stage. The goal is to ensure your hypothesis addresses the issue.
5. Proof your hypothesis
After preparing the first draft of your hypothesis, you need to inspect it thoroughly. It should tick all the boxes, like being concise, straightforward, relevant, and accurate. Your final hypothesis has to be well-structured as well.
Research projects are an exciting and crucial part of being a scholar. And once you have your research question, you need a great hypothesis to begin conducting research. Thus, knowing how to write a hypothesis is very important.
Now that you have a firmer grasp on what a good hypothesis constitutes, the different kinds there are, and what process to follow, you will find it much easier to write your hypothesis, which ultimately helps your research.
Now it's easier than ever to streamline your research workflow with SciSpace Discover . Its integrated, comprehensive end-to-end platform for research allows scholars to easily discover, write and publish their research and fosters collaboration.
It includes everything you need, including a repository of over 270 million research papers across disciplines, SEO-optimized summaries and public profiles to show your expertise and experience.
If you found these tips on writing a research hypothesis useful, head over to our blog on Statistical Hypothesis Testing to learn about the top researchers, papers, and institutions in this domain.
Frequently Asked Questions (FAQs)
1. what is the definition of hypothesis.
According to the Oxford dictionary, a hypothesis is defined as “An idea or explanation of something that is based on a few known facts, but that has not yet been proved to be true or correct”.
2. What is an example of hypothesis?
The hypothesis is a statement that proposes a relationship between two or more variables. An example: "If we increase the number of new users who join our platform by 25%, then we will see an increase in revenue."
3. What is an example of null hypothesis?
A null hypothesis is a statement that there is no relationship between two variables. The null hypothesis is written as H0. The null hypothesis states that there is no effect. For example, if you're studying whether or not a particular type of exercise increases strength, your null hypothesis will be "there is no difference in strength between people who exercise and people who don't."
4. What are the types of research?
• Fundamental research
• Applied research
• Qualitative research
• Quantitative research
• Mixed research
• Exploratory research
• Longitudinal research
• Cross-sectional research
• Field research
• Laboratory research
• Fixed research
• Flexible research
• Action research
• Policy research
• Classification research
• Comparative research
• Causal research
• Inductive research
• Deductive research
5. How to write a hypothesis?
• Your hypothesis should be able to predict the relationship and outcome.
• Avoid wordiness by keeping it simple and brief.
• Your hypothesis should contain observable and testable outcomes.
• Your hypothesis should be relevant to the research question.
6. What are the 2 types of hypothesis?
• Null hypotheses are used to test the claim that "there is no difference between two groups of data".
• Alternative hypotheses test the claim that "there is a difference between two data groups".
7. Difference between research question and research hypothesis?
A research question is a broad, open-ended question you will try to answer through your research. A hypothesis is a statement based on prior research or theory that you expect to be true due to your study. Example - Research question: What are the factors that influence the adoption of the new technology? Research hypothesis: There is a positive relationship between age, education and income level with the adoption of the new technology.
8. What is plural for hypothesis?
The plural of hypothesis is hypotheses. Here's an example of how it would be used in a statement, "Numerous well-considered hypotheses are presented in this part, and they are supported by tables and figures that are well-illustrated."
9. What is the red queen hypothesis?
The red queen hypothesis in evolutionary biology states that species must constantly evolve to avoid extinction because if they don't, they will be outcompeted by other species that are evolving. Leigh Van Valen first proposed it in 1973; since then, it has been tested and substantiated many times.
10. Who is known as the father of null hypothesis?
The father of the null hypothesis is Sir Ronald Fisher. He published a paper in 1925 that introduced the concept of null hypothesis testing, and he was also the first to use the term itself.
11. When to reject null hypothesis?
You need to find a significant difference between your two populations to reject the null hypothesis. You can determine that by running statistical tests such as an independent sample t-test or a dependent sample t-test. You should reject the null hypothesis if the p-value is less than 0.05.

You might also like

Consensus GPT vs. SciSpace GPT: Choose the Best GPT for Research
Literature Review and Theoretical Framework: Understanding the Differences

Types of Essays in Academic Writing
- Privacy Policy
Buy Me a Coffee

Home » What is a Hypothesis – Types, Examples and Writing Guide
What is a Hypothesis – Types, Examples and Writing Guide
Table of Contents

Definition:
Hypothesis is an educated guess or proposed explanation for a phenomenon, based on some initial observations or data. It is a tentative statement that can be tested and potentially proven or disproven through further investigation and experimentation.
Hypothesis is often used in scientific research to guide the design of experiments and the collection and analysis of data. It is an essential element of the scientific method, as it allows researchers to make predictions about the outcome of their experiments and to test those predictions to determine their accuracy.
Types of Hypothesis
Types of Hypothesis are as follows:
Research Hypothesis
A research hypothesis is a statement that predicts a relationship between variables. It is usually formulated as a specific statement that can be tested through research, and it is often used in scientific research to guide the design of experiments.
Null Hypothesis
The null hypothesis is a statement that assumes there is no significant difference or relationship between variables. It is often used as a starting point for testing the research hypothesis, and if the results of the study reject the null hypothesis, it suggests that there is a significant difference or relationship between variables.
Alternative Hypothesis
An alternative hypothesis is a statement that assumes there is a significant difference or relationship between variables. It is often used as an alternative to the null hypothesis and is tested against the null hypothesis to determine which statement is more accurate.
Directional Hypothesis
A directional hypothesis is a statement that predicts the direction of the relationship between variables. For example, a researcher might predict that increasing the amount of exercise will result in a decrease in body weight.
Non-directional Hypothesis
A non-directional hypothesis is a statement that predicts the relationship between variables but does not specify the direction. For example, a researcher might predict that there is a relationship between the amount of exercise and body weight, but they do not specify whether increasing or decreasing exercise will affect body weight.
Statistical Hypothesis
A statistical hypothesis is a statement that assumes a particular statistical model or distribution for the data. It is often used in statistical analysis to test the significance of a particular result.
Composite Hypothesis
A composite hypothesis is a statement that assumes more than one condition or outcome. It can be divided into several sub-hypotheses, each of which represents a different possible outcome.
Empirical Hypothesis
An empirical hypothesis is a statement that is based on observed phenomena or data. It is often used in scientific research to develop theories or models that explain the observed phenomena.
Simple Hypothesis
A simple hypothesis is a statement that assumes only one outcome or condition. It is often used in scientific research to test a single variable or factor.
Complex Hypothesis
A complex hypothesis is a statement that assumes multiple outcomes or conditions. It is often used in scientific research to test the effects of multiple variables or factors on a particular outcome.
Applications of Hypothesis
Hypotheses are used in various fields to guide research and make predictions about the outcomes of experiments or observations. Here are some examples of how hypotheses are applied in different fields:
- Science : In scientific research, hypotheses are used to test the validity of theories and models that explain natural phenomena. For example, a hypothesis might be formulated to test the effects of a particular variable on a natural system, such as the effects of climate change on an ecosystem.
- Medicine : In medical research, hypotheses are used to test the effectiveness of treatments and therapies for specific conditions. For example, a hypothesis might be formulated to test the effects of a new drug on a particular disease.
- Psychology : In psychology, hypotheses are used to test theories and models of human behavior and cognition. For example, a hypothesis might be formulated to test the effects of a particular stimulus on the brain or behavior.
- Sociology : In sociology, hypotheses are used to test theories and models of social phenomena, such as the effects of social structures or institutions on human behavior. For example, a hypothesis might be formulated to test the effects of income inequality on crime rates.
- Business : In business research, hypotheses are used to test the validity of theories and models that explain business phenomena, such as consumer behavior or market trends. For example, a hypothesis might be formulated to test the effects of a new marketing campaign on consumer buying behavior.
- Engineering : In engineering, hypotheses are used to test the effectiveness of new technologies or designs. For example, a hypothesis might be formulated to test the efficiency of a new solar panel design.
How to write a Hypothesis
Here are the steps to follow when writing a hypothesis:
Identify the Research Question
The first step is to identify the research question that you want to answer through your study. This question should be clear, specific, and focused. It should be something that can be investigated empirically and that has some relevance or significance in the field.
Conduct a Literature Review
Before writing your hypothesis, it’s essential to conduct a thorough literature review to understand what is already known about the topic. This will help you to identify the research gap and formulate a hypothesis that builds on existing knowledge.
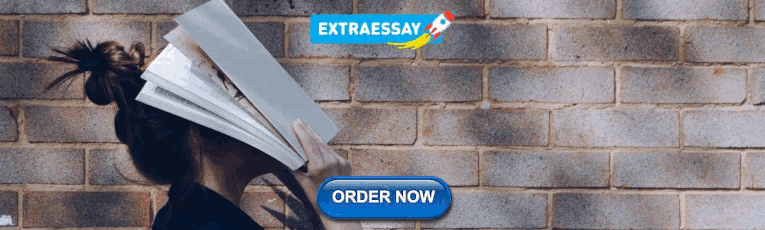
Determine the Variables
The next step is to identify the variables involved in the research question. A variable is any characteristic or factor that can vary or change. There are two types of variables: independent and dependent. The independent variable is the one that is manipulated or changed by the researcher, while the dependent variable is the one that is measured or observed as a result of the independent variable.
Formulate the Hypothesis
Based on the research question and the variables involved, you can now formulate your hypothesis. A hypothesis should be a clear and concise statement that predicts the relationship between the variables. It should be testable through empirical research and based on existing theory or evidence.
Write the Null Hypothesis
The null hypothesis is the opposite of the alternative hypothesis, which is the hypothesis that you are testing. The null hypothesis states that there is no significant difference or relationship between the variables. It is important to write the null hypothesis because it allows you to compare your results with what would be expected by chance.
Refine the Hypothesis
After formulating the hypothesis, it’s important to refine it and make it more precise. This may involve clarifying the variables, specifying the direction of the relationship, or making the hypothesis more testable.
Examples of Hypothesis
Here are a few examples of hypotheses in different fields:
- Psychology : “Increased exposure to violent video games leads to increased aggressive behavior in adolescents.”
- Biology : “Higher levels of carbon dioxide in the atmosphere will lead to increased plant growth.”
- Sociology : “Individuals who grow up in households with higher socioeconomic status will have higher levels of education and income as adults.”
- Education : “Implementing a new teaching method will result in higher student achievement scores.”
- Marketing : “Customers who receive a personalized email will be more likely to make a purchase than those who receive a generic email.”
- Physics : “An increase in temperature will cause an increase in the volume of a gas, assuming all other variables remain constant.”
- Medicine : “Consuming a diet high in saturated fats will increase the risk of developing heart disease.”
Purpose of Hypothesis
The purpose of a hypothesis is to provide a testable explanation for an observed phenomenon or a prediction of a future outcome based on existing knowledge or theories. A hypothesis is an essential part of the scientific method and helps to guide the research process by providing a clear focus for investigation. It enables scientists to design experiments or studies to gather evidence and data that can support or refute the proposed explanation or prediction.
The formulation of a hypothesis is based on existing knowledge, observations, and theories, and it should be specific, testable, and falsifiable. A specific hypothesis helps to define the research question, which is important in the research process as it guides the selection of an appropriate research design and methodology. Testability of the hypothesis means that it can be proven or disproven through empirical data collection and analysis. Falsifiability means that the hypothesis should be formulated in such a way that it can be proven wrong if it is incorrect.
In addition to guiding the research process, the testing of hypotheses can lead to new discoveries and advancements in scientific knowledge. When a hypothesis is supported by the data, it can be used to develop new theories or models to explain the observed phenomenon. When a hypothesis is not supported by the data, it can help to refine existing theories or prompt the development of new hypotheses to explain the phenomenon.
When to use Hypothesis
Here are some common situations in which hypotheses are used:
- In scientific research , hypotheses are used to guide the design of experiments and to help researchers make predictions about the outcomes of those experiments.
- In social science research , hypotheses are used to test theories about human behavior, social relationships, and other phenomena.
- I n business , hypotheses can be used to guide decisions about marketing, product development, and other areas. For example, a hypothesis might be that a new product will sell well in a particular market, and this hypothesis can be tested through market research.
Characteristics of Hypothesis
Here are some common characteristics of a hypothesis:
- Testable : A hypothesis must be able to be tested through observation or experimentation. This means that it must be possible to collect data that will either support or refute the hypothesis.
- Falsifiable : A hypothesis must be able to be proven false if it is not supported by the data. If a hypothesis cannot be falsified, then it is not a scientific hypothesis.
- Clear and concise : A hypothesis should be stated in a clear and concise manner so that it can be easily understood and tested.
- Based on existing knowledge : A hypothesis should be based on existing knowledge and research in the field. It should not be based on personal beliefs or opinions.
- Specific : A hypothesis should be specific in terms of the variables being tested and the predicted outcome. This will help to ensure that the research is focused and well-designed.
- Tentative: A hypothesis is a tentative statement or assumption that requires further testing and evidence to be confirmed or refuted. It is not a final conclusion or assertion.
- Relevant : A hypothesis should be relevant to the research question or problem being studied. It should address a gap in knowledge or provide a new perspective on the issue.
Advantages of Hypothesis
Hypotheses have several advantages in scientific research and experimentation:
- Guides research: A hypothesis provides a clear and specific direction for research. It helps to focus the research question, select appropriate methods and variables, and interpret the results.
- Predictive powe r: A hypothesis makes predictions about the outcome of research, which can be tested through experimentation. This allows researchers to evaluate the validity of the hypothesis and make new discoveries.
- Facilitates communication: A hypothesis provides a common language and framework for scientists to communicate with one another about their research. This helps to facilitate the exchange of ideas and promotes collaboration.
- Efficient use of resources: A hypothesis helps researchers to use their time, resources, and funding efficiently by directing them towards specific research questions and methods that are most likely to yield results.
- Provides a basis for further research: A hypothesis that is supported by data provides a basis for further research and exploration. It can lead to new hypotheses, theories, and discoveries.
- Increases objectivity: A hypothesis can help to increase objectivity in research by providing a clear and specific framework for testing and interpreting results. This can reduce bias and increase the reliability of research findings.
Limitations of Hypothesis
Some Limitations of the Hypothesis are as follows:
- Limited to observable phenomena: Hypotheses are limited to observable phenomena and cannot account for unobservable or intangible factors. This means that some research questions may not be amenable to hypothesis testing.
- May be inaccurate or incomplete: Hypotheses are based on existing knowledge and research, which may be incomplete or inaccurate. This can lead to flawed hypotheses and erroneous conclusions.
- May be biased: Hypotheses may be biased by the researcher’s own beliefs, values, or assumptions. This can lead to selective interpretation of data and a lack of objectivity in research.
- Cannot prove causation: A hypothesis can only show a correlation between variables, but it cannot prove causation. This requires further experimentation and analysis.
- Limited to specific contexts: Hypotheses are limited to specific contexts and may not be generalizable to other situations or populations. This means that results may not be applicable in other contexts or may require further testing.
- May be affected by chance : Hypotheses may be affected by chance or random variation, which can obscure or distort the true relationship between variables.
About the author
Muhammad Hassan
Researcher, Academic Writer, Web developer
You may also like

Data Collection – Methods Types and Examples

Delimitations in Research – Types, Examples and...

Research Process – Steps, Examples and Tips

Research Design – Types, Methods and Examples

Institutional Review Board – Application Sample...

Evaluating Research – Process, Examples and...

What Is A Research (Scientific) Hypothesis? A plain-language explainer + examples
By: Derek Jansen (MBA) | Reviewed By: Dr Eunice Rautenbach | June 2020
If you’re new to the world of research, or it’s your first time writing a dissertation or thesis, you’re probably noticing that the words “research hypothesis” and “scientific hypothesis” are used quite a bit, and you’re wondering what they mean in a research context .
“Hypothesis” is one of those words that people use loosely, thinking they understand what it means. However, it has a very specific meaning within academic research. So, it’s important to understand the exact meaning before you start hypothesizing.
Research Hypothesis 101
- What is a hypothesis ?
- What is a research hypothesis (scientific hypothesis)?
- Requirements for a research hypothesis
- Definition of a research hypothesis
- The null hypothesis
What is a hypothesis?
Let’s start with the general definition of a hypothesis (not a research hypothesis or scientific hypothesis), according to the Cambridge Dictionary:
Hypothesis: an idea or explanation for something that is based on known facts but has not yet been proved.
In other words, it’s a statement that provides an explanation for why or how something works, based on facts (or some reasonable assumptions), but that has not yet been specifically tested . For example, a hypothesis might look something like this:
Hypothesis: sleep impacts academic performance.
This statement predicts that academic performance will be influenced by the amount and/or quality of sleep a student engages in – sounds reasonable, right? It’s based on reasonable assumptions , underpinned by what we currently know about sleep and health (from the existing literature). So, loosely speaking, we could call it a hypothesis, at least by the dictionary definition.
But that’s not good enough…
Unfortunately, that’s not quite sophisticated enough to describe a research hypothesis (also sometimes called a scientific hypothesis), and it wouldn’t be acceptable in a dissertation, thesis or research paper . In the world of academic research, a statement needs a few more criteria to constitute a true research hypothesis .
What is a research hypothesis?
A research hypothesis (also called a scientific hypothesis) is a statement about the expected outcome of a study (for example, a dissertation or thesis). To constitute a quality hypothesis, the statement needs to have three attributes – specificity , clarity and testability .
Let’s take a look at these more closely.
Need a helping hand?
Hypothesis Essential #1: Specificity & Clarity
A good research hypothesis needs to be extremely clear and articulate about both what’ s being assessed (who or what variables are involved ) and the expected outcome (for example, a difference between groups, a relationship between variables, etc.).
Let’s stick with our sleepy students example and look at how this statement could be more specific and clear.
Hypothesis: Students who sleep at least 8 hours per night will, on average, achieve higher grades in standardised tests than students who sleep less than 8 hours a night.
As you can see, the statement is very specific as it identifies the variables involved (sleep hours and test grades), the parties involved (two groups of students), as well as the predicted relationship type (a positive relationship). There’s no ambiguity or uncertainty about who or what is involved in the statement, and the expected outcome is clear.
Contrast that to the original hypothesis we looked at – “Sleep impacts academic performance” – and you can see the difference. “Sleep” and “academic performance” are both comparatively vague , and there’s no indication of what the expected relationship direction is (more sleep or less sleep). As you can see, specificity and clarity are key.
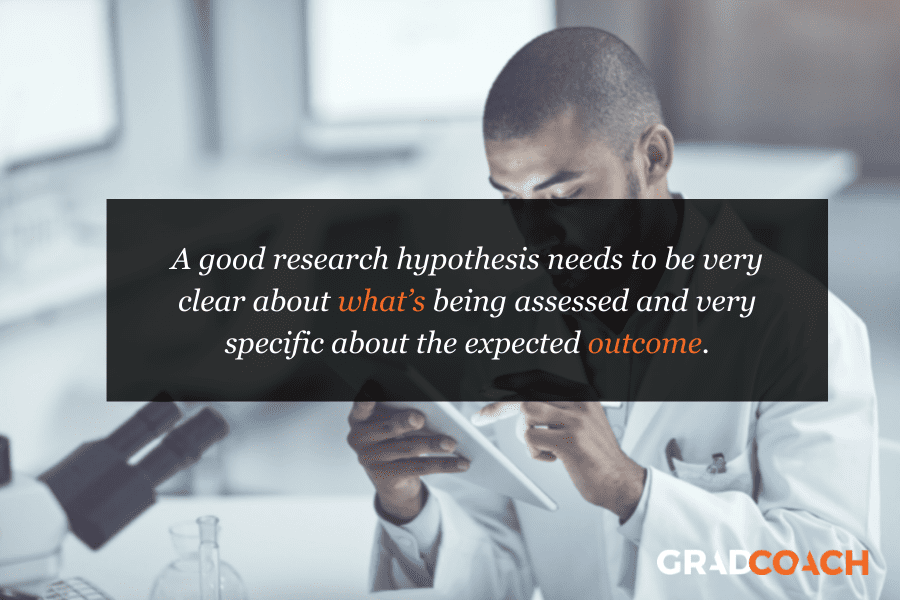
Hypothesis Essential #2: Testability (Provability)
A statement must be testable to qualify as a research hypothesis. In other words, there needs to be a way to prove (or disprove) the statement. If it’s not testable, it’s not a hypothesis – simple as that.
For example, consider the hypothesis we mentioned earlier:
Hypothesis: Students who sleep at least 8 hours per night will, on average, achieve higher grades in standardised tests than students who sleep less than 8 hours a night.
We could test this statement by undertaking a quantitative study involving two groups of students, one that gets 8 or more hours of sleep per night for a fixed period, and one that gets less. We could then compare the standardised test results for both groups to see if there’s a statistically significant difference.
Again, if you compare this to the original hypothesis we looked at – “Sleep impacts academic performance” – you can see that it would be quite difficult to test that statement, primarily because it isn’t specific enough. How much sleep? By who? What type of academic performance?
So, remember the mantra – if you can’t test it, it’s not a hypothesis 🙂
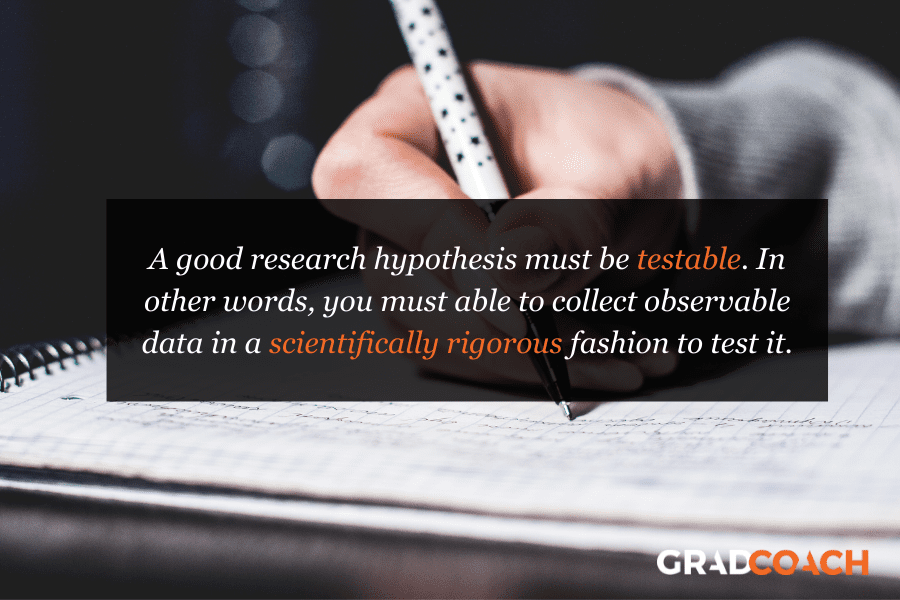
Defining A Research Hypothesis
You’re still with us? Great! Let’s recap and pin down a clear definition of a hypothesis.
A research hypothesis (or scientific hypothesis) is a statement about an expected relationship between variables, or explanation of an occurrence, that is clear, specific and testable.
So, when you write up hypotheses for your dissertation or thesis, make sure that they meet all these criteria. If you do, you’ll not only have rock-solid hypotheses but you’ll also ensure a clear focus for your entire research project.
What about the null hypothesis?
You may have also heard the terms null hypothesis , alternative hypothesis, or H-zero thrown around. At a simple level, the null hypothesis is the counter-proposal to the original hypothesis.
For example, if the hypothesis predicts that there is a relationship between two variables (for example, sleep and academic performance), the null hypothesis would predict that there is no relationship between those variables.
At a more technical level, the null hypothesis proposes that no statistical significance exists in a set of given observations and that any differences are due to chance alone.
And there you have it – hypotheses in a nutshell.
If you have any questions, be sure to leave a comment below and we’ll do our best to help you. If you need hands-on help developing and testing your hypotheses, consider our private coaching service , where we hold your hand through the research journey.
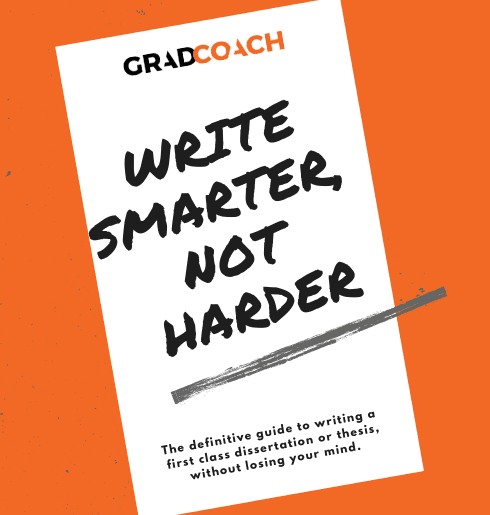
Psst… there’s more (for free)
This post is part of our dissertation mini-course, which covers everything you need to get started with your dissertation, thesis or research project.
You Might Also Like:
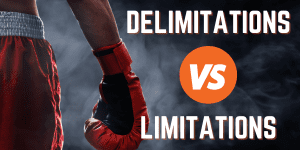
15 Comments
Very useful information. I benefit more from getting more information in this regard.
Very great insight,educative and informative. Please give meet deep critics on many research data of public international Law like human rights, environment, natural resources, law of the sea etc
In a book I read a distinction is made between null, research, and alternative hypothesis. As far as I understand, alternative and research hypotheses are the same. Can you please elaborate? Best Afshin
This is a self explanatory, easy going site. I will recommend this to my friends and colleagues.
Very good definition. How can I cite your definition in my thesis? Thank you. Is nul hypothesis compulsory in a research?
It’s a counter-proposal to be proven as a rejection
Please what is the difference between alternate hypothesis and research hypothesis?
It is a very good explanation. However, it limits hypotheses to statistically tasteable ideas. What about for qualitative researches or other researches that involve quantitative data that don’t need statistical tests?
In qualitative research, one typically uses propositions, not hypotheses.
could you please elaborate it more
I’ve benefited greatly from these notes, thank you.
This is very helpful
well articulated ideas are presented here, thank you for being reliable sources of information
Excellent. Thanks for being clear and sound about the research methodology and hypothesis (quantitative research)
I have only a simple question regarding the null hypothesis. – Is the null hypothesis (Ho) known as the reversible hypothesis of the alternative hypothesis (H1? – How to test it in academic research?
Trackbacks/Pingbacks
- What Is Research Methodology? Simple Definition (With Examples) - Grad Coach - […] Contrasted to this, a quantitative methodology is typically used when the research aims and objectives are confirmatory in nature. For example,…
Submit a Comment Cancel reply
Your email address will not be published. Required fields are marked *
Save my name, email, and website in this browser for the next time I comment.
- Print Friendly

15 Hypothesis Examples
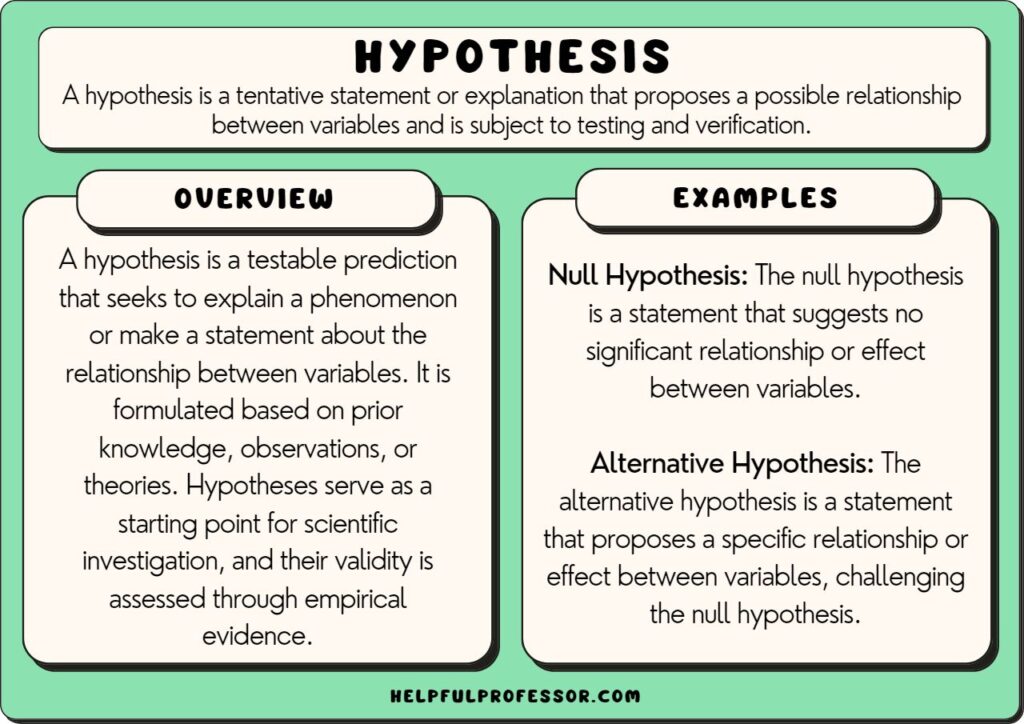
A hypothesis is defined as a testable prediction , and is used primarily in scientific experiments as a potential or predicted outcome that scientists attempt to prove or disprove (Atkinson et al., 2021; Tan, 2022).
In my types of hypothesis article, I outlined 13 different hypotheses, including the directional hypothesis (which makes a prediction about an effect of a treatment will be positive or negative) and the associative hypothesis (which makes a prediction about the association between two variables).
This article will dive into some interesting examples of hypotheses and examine potential ways you might test each one.
Hypothesis Examples
1. “inadequate sleep decreases memory retention”.
Field: Psychology
Type: Causal Hypothesis A causal hypothesis explores the effect of one variable on another. This example posits that a lack of adequate sleep causes decreased memory retention. In other words, if you are not getting enough sleep, your ability to remember and recall information may suffer.
How to Test:
To test this hypothesis, you might devise an experiment whereby your participants are divided into two groups: one receives an average of 8 hours of sleep per night for a week, while the other gets less than the recommended sleep amount.
During this time, all participants would daily study and recall new, specific information. You’d then measure memory retention of this information for both groups using standard memory tests and compare the results.
Should the group with less sleep have statistically significant poorer memory scores, the hypothesis would be supported.
Ensuring the integrity of the experiment requires taking into account factors such as individual health differences, stress levels, and daily nutrition.
Relevant Study: Sleep loss, learning capacity and academic performance (Curcio, Ferrara & De Gennaro, 2006)
2. “Increase in Temperature Leads to Increase in Kinetic Energy”
Field: Physics
Type: Deductive Hypothesis The deductive hypothesis applies the logic of deductive reasoning – it moves from a general premise to a more specific conclusion. This specific hypothesis assumes that as temperature increases, the kinetic energy of particles also increases – that is, when you heat something up, its particles move around more rapidly.
This hypothesis could be examined by heating a gas in a controlled environment and capturing the movement of its particles as a function of temperature.
You’d gradually increase the temperature and measure the kinetic energy of the gas particles with each increment. If the kinetic energy consistently rises with the temperature, your hypothesis gets supporting evidence.
Variables such as pressure and volume of the gas would need to be held constant to ensure validity of results.
3. “Children Raised in Bilingual Homes Develop Better Cognitive Skills”
Field: Psychology/Linguistics
Type: Comparative Hypothesis The comparative hypothesis posits a difference between two or more groups based on certain variables. In this context, you might propose that children raised in bilingual homes have superior cognitive skills compared to those raised in monolingual homes.
Testing this hypothesis could involve identifying two groups of children: those raised in bilingual homes, and those raised in monolingual homes.
Cognitive skills in both groups would be evaluated using a standard cognitive ability test at different stages of development. The examination would be repeated over a significant time period for consistency.
If the group raised in bilingual homes persistently scores higher than the other, the hypothesis would thereby be supported.
The challenge for the researcher would be controlling for other variables that could impact cognitive development, such as socio-economic status, education level of parents, and parenting styles.
Relevant Study: The cognitive benefits of being bilingual (Marian & Shook, 2012)
4. “High-Fiber Diet Leads to Lower Incidences of Cardiovascular Diseases”
Field: Medicine/Nutrition
Type: Alternative Hypothesis The alternative hypothesis suggests an alternative to a null hypothesis. In this context, the implied null hypothesis could be that diet has no effect on cardiovascular health, which the alternative hypothesis contradicts by suggesting that a high-fiber diet leads to fewer instances of cardiovascular diseases.
To test this hypothesis, a longitudinal study could be conducted on two groups of participants; one adheres to a high-fiber diet, while the other follows a diet low in fiber.
After a fixed period, the cardiovascular health of participants in both groups could be analyzed and compared. If the group following a high-fiber diet has a lower number of recorded cases of cardiovascular diseases, it would provide evidence supporting the hypothesis.
Control measures should be implemented to exclude the influence of other lifestyle and genetic factors that contribute to cardiovascular health.
Relevant Study: Dietary fiber, inflammation, and cardiovascular disease (King, 2005)
5. “Gravity Influences the Directional Growth of Plants”
Field: Agronomy / Botany
Type: Explanatory Hypothesis An explanatory hypothesis attempts to explain a phenomenon. In this case, the hypothesis proposes that gravity affects how plants direct their growth – both above-ground (toward sunlight) and below-ground (towards water and other resources).
The testing could be conducted by growing plants in a rotating cylinder to create artificial gravity.
Observations on the direction of growth, over a specified period, can provide insights into the influencing factors. If plants consistently direct their growth in a manner that indicates the influence of gravitational pull, the hypothesis is substantiated.
It is crucial to ensure that other growth-influencing factors, such as light and water, are uniformly distributed so that only gravity influences the directional growth.
6. “The Implementation of Gamified Learning Improves Students’ Motivation”
Field: Education
Type: Relational Hypothesis The relational hypothesis describes the relation between two variables. Here, the hypothesis is that the implementation of gamified learning has a positive effect on the motivation of students.
To validate this proposition, two sets of classes could be compared: one that implements a learning approach with game-based elements, and another that follows a traditional learning approach.
The students’ motivation levels could be gauged by monitoring their engagement, performance, and feedback over a considerable timeframe.
If the students engaged in the gamified learning context present higher levels of motivation and achievement, the hypothesis would be supported.
Control measures ought to be put into place to account for individual differences, including prior knowledge and attitudes towards learning.
Relevant Study: Does educational gamification improve students’ motivation? (Chapman & Rich, 2018)
7. “Mathematics Anxiety Negatively Affects Performance”
Field: Educational Psychology
Type: Research Hypothesis The research hypothesis involves making a prediction that will be tested. In this case, the hypothesis proposes that a student’s anxiety about math can negatively influence their performance in math-related tasks.
To assess this hypothesis, researchers must first measure the mathematics anxiety levels of a sample of students using a validated instrument, such as the Mathematics Anxiety Rating Scale.
Then, the students’ performance in mathematics would be evaluated through standard testing. If there’s a negative correlation between the levels of math anxiety and math performance (meaning as anxiety increases, performance decreases), the hypothesis would be supported.
It would be crucial to control for relevant factors such as overall academic performance and previous mathematical achievement.
8. “Disruption of Natural Sleep Cycle Impairs Worker Productivity”
Field: Organizational Psychology
Type: Operational Hypothesis The operational hypothesis involves defining the variables in measurable terms. In this example, the hypothesis posits that disrupting the natural sleep cycle, for instance through shift work or irregular working hours, can lessen productivity among workers.
To test this hypothesis, you could collect data from workers who maintain regular working hours and those with irregular schedules.
Measuring productivity could involve examining the worker’s ability to complete tasks, the quality of their work, and their efficiency.
If workers with interrupted sleep cycles demonstrate lower productivity compared to those with regular sleep patterns, it would lend support to the hypothesis.
Consideration should be given to potential confounding variables such as job type, worker age, and overall health.
9. “Regular Physical Activity Reduces the Risk of Depression”
Field: Health Psychology
Type: Predictive Hypothesis A predictive hypothesis involves making a prediction about the outcome of a study based on the observed relationship between variables. In this case, it is hypothesized that individuals who engage in regular physical activity are less likely to suffer from depression.
Longitudinal studies would suit to test this hypothesis, tracking participants’ levels of physical activity and their mental health status over time.
The level of physical activity could be self-reported or monitored, while mental health status could be assessed using standard diagnostic tools or surveys.
If data analysis shows that participants maintaining regular physical activity have a lower incidence of depression, this would endorse the hypothesis.
However, care should be taken to control other lifestyle and behavioral factors that could intervene with the results.
Relevant Study: Regular physical exercise and its association with depression (Kim, 2022)
10. “Regular Meditation Enhances Emotional Stability”
Type: Empirical Hypothesis In the empirical hypothesis, predictions are based on amassed empirical evidence . This particular hypothesis theorizes that frequent meditation leads to improved emotional stability, resonating with numerous studies linking meditation to a variety of psychological benefits.
Earlier studies reported some correlations, but to test this hypothesis directly, you’d organize an experiment where one group meditates regularly over a set period while a control group doesn’t.
Both groups’ emotional stability levels would be measured at the start and end of the experiment using a validated emotional stability assessment.
If regular meditators display noticeable improvements in emotional stability compared to the control group, the hypothesis gains credit.
You’d have to ensure a similar emotional baseline for all participants at the start to avoid skewed results.
11. “Children Exposed to Reading at an Early Age Show Superior Academic Progress”
Type: Directional Hypothesis The directional hypothesis predicts the direction of an expected relationship between variables. Here, the hypothesis anticipates that early exposure to reading positively affects a child’s academic advancement.
A longitudinal study tracking children’s reading habits from an early age and their consequent academic performance could validate this hypothesis.
Parents could report their children’s exposure to reading at home, while standardized school exam results would provide a measure of academic achievement.
If the children exposed to early reading consistently perform better acadically, it gives weight to the hypothesis.
However, it would be important to control for variables that might impact academic performance, such as socioeconomic background, parental education level, and school quality.
12. “Adopting Energy-efficient Technologies Reduces Carbon Footprint of Industries”
Field: Environmental Science
Type: Descriptive Hypothesis A descriptive hypothesis predicts the existence of an association or pattern related to variables. In this scenario, the hypothesis suggests that industries adopting energy-efficient technologies will resultantly show a reduced carbon footprint.
Global industries making use of energy-efficient technologies could track their carbon emissions over time. At the same time, others not implementing such technologies continue their regular tracking.
After a defined time, the carbon emission data of both groups could be compared. If industries that adopted energy-efficient technologies demonstrate a notable reduction in their carbon footprints, the hypothesis would hold strong.
In the experiment, you would exclude variations brought by factors such as industry type, size, and location.
13. “Reduced Screen Time Improves Sleep Quality”
Type: Simple Hypothesis The simple hypothesis is a prediction about the relationship between two variables, excluding any other variables from consideration. This example posits that by reducing time spent on devices like smartphones and computers, an individual should experience improved sleep quality.
A sample group would need to reduce their daily screen time for a pre-determined period. Sleep quality before and after the reduction could be measured using self-report sleep diaries and objective measures like actigraphy, monitoring movement and wakefulness during sleep.
If the data shows that sleep quality improved post the screen time reduction, the hypothesis would be validated.
Other aspects affecting sleep quality, like caffeine intake, should be controlled during the experiment.
Relevant Study: Screen time use impacts low‐income preschool children’s sleep quality, tiredness, and ability to fall asleep (Waller et al., 2021)
14. Engaging in Brain-Training Games Improves Cognitive Functioning in Elderly
Field: Gerontology
Type: Inductive Hypothesis Inductive hypotheses are based on observations leading to broader generalizations and theories. In this context, the hypothesis deduces from observed instances that engaging in brain-training games can help improve cognitive functioning in the elderly.
A longitudinal study could be conducted where an experimental group of elderly people partakes in regular brain-training games.
Their cognitive functioning could be assessed at the start of the study and at regular intervals using standard neuropsychological tests.
If the group engaging in brain-training games shows better cognitive functioning scores over time compared to a control group not playing these games, the hypothesis would be supported.
15. Farming Practices Influence Soil Erosion Rates
Type: Null Hypothesis A null hypothesis is a negative statement assuming no relationship or difference between variables. The hypothesis in this context asserts there’s no effect of different farming practices on the rates of soil erosion.
Comparing soil erosion rates in areas with different farming practices over a considerable timeframe could help test this hypothesis.
If, statistically, the farming practices do not lead to differences in soil erosion rates, the null hypothesis is accepted.
However, if marked variation appears, the null hypothesis is rejected, meaning farming practices do influence soil erosion rates. It would be crucial to control for external factors like weather, soil type, and natural vegetation.
The variety of hypotheses mentioned above underscores the diversity of research constructs inherent in different fields, each with its unique purpose and way of testing.
While researchers may develop hypotheses primarily as tools to define and narrow the focus of the study, these hypotheses also serve as valuable guiding forces for the data collection and analysis procedures, making the research process more efficient and direction-focused.
Hypotheses serve as a compass for any form of academic research. The diverse examples provided, from Psychology to Educational Studies, Environmental Science to Gerontology, clearly demonstrate how certain hypotheses suit specific fields more aptly than others.
It is important to underline that although these varied hypotheses differ in their structure and methods of testing, each endorses the fundamental value of empiricism in research. Evidence-based decision making remains at the heart of scholarly inquiry, regardless of the research field, thus aligning all hypotheses to the core purpose of scientific investigation.
Testing hypotheses is an essential part of the scientific method . By doing so, researchers can either confirm their predictions, giving further validity to an existing theory, or they might uncover new insights that could potentially shift the field’s understanding of a particular phenomenon. In either case, hypotheses serve as the stepping stones for scientific exploration and discovery.
Atkinson, P., Delamont, S., Cernat, A., Sakshaug, J. W., & Williams, R. A. (2021). SAGE research methods foundations . SAGE Publications Ltd.
Curcio, G., Ferrara, M., & De Gennaro, L. (2006). Sleep loss, learning capacity and academic performance. Sleep medicine reviews , 10 (5), 323-337.
Kim, J. H. (2022). Regular physical exercise and its association with depression: A population-based study short title: Exercise and depression. Psychiatry Research , 309 , 114406.
King, D. E. (2005). Dietary fiber, inflammation, and cardiovascular disease. Molecular nutrition & food research , 49 (6), 594-600.
Marian, V., & Shook, A. (2012, September). The cognitive benefits of being bilingual. In Cerebrum: the Dana forum on brain science (Vol. 2012). Dana Foundation.
Tan, W. C. K. (2022). Research Methods: A Practical Guide For Students And Researchers (Second Edition) . World Scientific Publishing Company.
Waller, N. A., Zhang, N., Cocci, A. H., D’Agostino, C., Wesolek‐Greenson, S., Wheelock, K., … & Resnicow, K. (2021). Screen time use impacts low‐income preschool children’s sleep quality, tiredness, and ability to fall asleep. Child: care, health and development, 47 (5), 618-626.
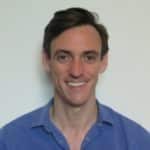
Chris Drew (PhD)
Dr. Chris Drew is the founder of the Helpful Professor. He holds a PhD in education and has published over 20 articles in scholarly journals. He is the former editor of the Journal of Learning Development in Higher Education. [Image Descriptor: Photo of Chris]
- Chris Drew (PhD) https://helpfulprofessor.com/author/chris-drew-phd/ 5 Top Tips for Succeeding at University
- Chris Drew (PhD) https://helpfulprofessor.com/author/chris-drew-phd/ 50 Durable Goods Examples
- Chris Drew (PhD) https://helpfulprofessor.com/author/chris-drew-phd/ 100 Consumer Goods Examples
- Chris Drew (PhD) https://helpfulprofessor.com/author/chris-drew-phd/ 30 Globalization Pros and Cons
Leave a Comment Cancel Reply
Your email address will not be published. Required fields are marked *

An official website of the United States government
The .gov means it’s official. Federal government websites often end in .gov or .mil. Before sharing sensitive information, make sure you’re on a federal government site.
The site is secure. The https:// ensures that you are connecting to the official website and that any information you provide is encrypted and transmitted securely.
- Publications
- Account settings
Preview improvements coming to the PMC website in October 2024. Learn More or Try it out now .
- Advanced Search
- Journal List
- HHS Author Manuscripts

Stress, coping, and depression: testing new hypotheses in a prospectively studied general population sample of U.S. born Whites and Blacks
1 Department of Epidemiology, Columbia University, New York, NY
The scarcity of empirically supported explanations for the Black/White prevalence difference in depression in the U.S. is a conspicuous gap in the literature. Recent evidence suggests that the paradoxical observation of decreased risk of depression but elevated rates of physical illness among Blacks in the U.S. compared with Whites may be accounted for by the use of coping behaviors (e.g., alcohol and nicotine consumption, overeating) among Blacks exposed to high stress levels. Such coping behaviors may mitigate deleterious effects of stressful exposures on mental health while increasing the risk of physical ailments. The racial patterning in mental and physical health outcomes could therefore be explained by this mechanism if a) these behaviors were more prevalent among Blacks than Whites and/or b) the effect of these behavioral responses to stress was differential by race. The present study challenges this hypothesis using longitudinal, nationally-representative data with comprehensive DSM-IV diagnoses. Data are drawn from 34,653 individuals sampled in Waves 1 (2001-2002) and 2 (2004-2005) as part of the US National Epidemiologic Survey on Alcohol and Related Conditions. Results showed that a) Blacks were less likely to engage in alcohol or nicotine consumption at low, moderate, and high levels of stress compared to Whites, and b) there was a significant three-way interaction between race, stress, and coping behavior for BMI only (F=2.11, df=12, p=0.03), but, contrary to the hypothesis, elevated BMI was protective against depression in Blacks at low, not high, levels of stress. Further, engagement in unhealthy behaviors, especially at pathological levels, did not protect against depression in Blacks or in Whites. In sum, the impact of stress and coping processes on depression do not appear to operate differently in Blacks versus Whites. Further research testing innovative hypotheses that would explain the difference in Black/White depression prevalence is warranted.
Introduction
Epidemiologic studies have consistently documented that Blacks living in the United States have higher rates of physical illness such as hypertension and diabetes, and higher rates of mortality, compared with non-Hispanic Whites controlling for indicators of socio-economic position (SEP) ( Heckler 1985 ; McCord and Freeman 1990 ; Williams and Jackson 2005 ). Conversely, major psychiatric epidemiologic household surveys have reported that Blacks have equal or lower rates of most psychiatric disorders, including major depression ( Kessler, McGonagle et al. 1994 ; Hasin, Goodwin et al. 2005 ; Breslau, Aguilar-Gaxiola et al. 2006 ; Williams, Gonzalez et al. 2007 ). These divergent patterns for mental and physical health outcomes have been termed a ‘paradox’ ( Williams 2001 ). Blacks in the U.S. face historic and contemporary institutionalized discrimination which exposes them to disadvantaged SEP, worse living conditions, and greater stress and adversity due to marginalized social status ( Kessler, Mickelson et al. 1999 ; Kreiger 2000 ; Williams and Williams-Morris 2000 ), all of which seemingly place Blacks at greater risk for depression compared with Whites ( Dohrenwend 2000 ). Indeed, among Blacks in the U.S., perception of discrimination and adversity due to race is associated with greater psychological distress and depressive symptoms ( Kessler, Mickelson et al. 1999 ; Williams and Williams-Morris 2000 ). However, absolute rates of depression remain lower among Blacks compared with Whites.
Many pathways have been posited to explain the elevated rates of physical health problems among Blacks in the U.S. compared with Whites. One well-studied mechanism is stress associated with disadvantaged social status. The physiologic responses to stress via allostatic load have been hypothesized to influence health by a process of ‘wear and tear’ whereby the body can no longer effectively regulate itself ( McEwen 2000 ; McEwen 2004 ). “Weathering” ( Geronimus 1994 ; Geronimus 1996 ), which describes a process of accelerated aging as an effect of the cumulative experience of stress and adversity, has been hypothesized to explain why Blacks have lower birthweights as well as higher mortality at younger ages than Whites after controlling for SEP. Further, interpersonal discrimination appraised by the individual as negative can result in fear, anger, and denial, thereby inducing injurious physiologic responses in cardiovascular, endocrine, neurologic and immune systems ( Krieger 1990 ; Krieger and Sidney 1996 ; Kreiger 2000 ). Adverse neighborhood conditions, to which Blacks have greater exposure than Whites, can influence health through inadequate access to social and health services, exposures to health hazards, and reduction in social cohesion and connectedness ( Massey 1985 ; Massey 2004 ). Greater stress, worse bodily wear and tear, reduced access to medical services, and greater exposure to deleterious neighborhood conditions are all risk factors for depression ( Leonard 2000 ; McEwen 2003 ; Stansfeld 2005 ), and yet Blacks consistently generate estimates of depression below those of Whites; this poses a perplexing, unresolved issue for social and psychiatric epidemiology.
Two methodological hypotheses advanced to explain this mental/physical health paradox posit that rates of depression among Blacks are underestimated in major psychiatric epidemiologic studies due to selection bias and measurement error. The selection bias hypothesis reflects the fact that all major psychiatric epidemiologic surveys conducted in the U.S. exclude institutionalized populations. Young Black men in the U.S. are overrepresented in prison and jail populations ( Petit and Western 2004 ), where depression is more prevalent compared with household populations ( Teplin 1990 ; Teplin, Abram et al. 1996 ). Thus, the underestimation of depression prevalence in household samples could affect Blacks to a greater extent compared with Whites, though the effect of this bias would primarily be age- and gender-specific. The measurement error hypothesis suggests potential diagnostic bias in the major survey instruments used to capture depression. Given the same symptom presentation, Blacks interviewed by clinicians in unstructured or semi-structured formats are more likely to be diagnosed as having a disorder in the psychotic spectrum and Whites as having a disorder in the mood spectrum ( Neighbors, Trierweiler et al. 1999 ; Neighbors, Trierweiler et al. 2003 ; Strakowski, Keck et al. 2003 ). Additionally, some argue that depression may manifest differently in Blacks compared with Whites, and current diagnostic nosology more appropriately captures depression in Whites compared with Blacks ( Rogler 1999 ; Baker 2001 ; Brown 2003 ; Kleinman 2004 ). Available data suggest that while these hypotheses may explain some of the Black/White difference in depression, methodological issues cannot account for the all of the difference ( Williams, Gonzalez et al. 2007 ; Breslau, Javaras et al. 2008 ). Thus, hypotheses exploring alternative mechanisms through which Blacks may have a lower prevalence of depression compared with Whites remain necessary.
In contrast to methodological hypotheses explaining the mental/physical health ‘paradox’, a recently advanced alternative hypothesis is that the patterning in physical and mental health outcomes in Blacks versus Whites arises from mechanisms for coping with stressors that on average operate differently for Black and White Americans ( Jackson and Knight 2006 ; Jackson, Knight et al. 2009 ). Jackson and colleagues have argued that Blacks in the U.S. face greater, and unique, stressors compared with Whites, and that strategies deployed to cope emotionally with this increased stress may protect mental health while having deleterious consequences for physical health. Recently, Jackson and colleagues reported that at high levels of stress, Blacks with elevated body mass index (BMI) and/or who smoke cigarettes and/or drink alcohol (collectively termed ‘unhealthy behaviors’ or ‘UHBs’ ( Jackson, Knight et al. 2009 )) were less likely than Blacks not engaging in these behaviors to develop depression, whereas the pattern trended in the opposite direction for Whites ( Jackson, Knight et al. 2009 ). Further empirical support for this hypothesis was recently reported using data from the Baltimore Epidemiologic Catchment Area Study ( Mezuk, Rafferty et al. 2010 ). Evidence indicates that UHBs can ameliorate immediate anxiety and depressive symptoms in response to stressful experiences by regulating corticotropin-releasing factor in the hypothalamic-pituitary-adrenalcortical (HPA) axis ( Benowitz 1988 ; Koob, Roberts et al. 1998 ; Dallman, Pecoraro et al. 2003 ). However, long-term heavy alcohol consumption, smoking, and high BMI can lead to a cascade of physical health consequences. This hypothesis suggests that, in the context of chronic stress, Blacks’ engagement in UHBs may serve to buffer the deleterious consequences of stress on depression through the HPA pathway, leading to a lower prevalence of depression but a greater prevalence of physical health problems than would have otherwise occurred. This hypothesis also suggests that the same processes operate differently or with different consequences in Whites. In the interest of brevity, we refer to these potentially differential patterns in the relationships between stress, coping, and depression between Blacks and Whites as “group-specific,” meaning that they arise from the unequal distribution of exposures and coping resources engendered by a racialized environment, rather than differences embedded in the individual.
Differences in stress and coping processes between Blacks and Whites could account for the mental/physical health ‘paradox’ under two scenarios. (1) UHBs are indeed protective against depression, among both Blacks and Whites, but Blacks are much more likely to engage in them compared with Whites at a given level of stress. This is unlikely in light of previous epidemiologic evidence suggesting that a) substance disorders and obesity are comorbid with depression ( Reiger, Farmer et al. 1990 ; Kessler, Crum et al. 1997 ; Hasin, Goodwin et al. 2005 ) and b) Blacks are less likely than Whites to engage in alcohol and nicotine consumption ( Grant, Hasin et al. 2004 ; Hasin, Stinson et al. 2007 ). However, patterns of comorbidity and Black/White differences in depression at all levels of stress have not been investigated systematically. (2) UHBs operate differentially by race, whereby they protect against depression to a greater extent among Blacks compared with Whites (either overall or variably by level of stress). This hypothesis is supported by data from the Americans’ Changing Lives Survey ( Jackson, Knight et al. 2009 ) and the Baltimore Epidemiologic Catchment Area Study ( Mezuk, Rafferty et al. 2010 ), as described above.
We propose to comprehensively investigate each of the above scenarios in a large nationally representative prospective study of U.S. adults. The present study is intended to both replicate and extend the analyses presented in Jackson et al. (2009) to provide a comprehensive test of the underlying theory. Using the National Epidemiologic Survey on Alcohol and Related Conditions (NESARC) we accomplish five main aims. First, we construct as exact a replication as possible of Jackson et al. (2009) in order to provide a baseline for comparison and from which to broaden the analyses. The remaining four aims systematically test the theory underlying the two scenarios outlined above. We examine whether alcohol consumption, nicotine consumption, and body mass index (as a proxy for overeating, consistent with Jackson et al., (2009) ) are prospectively protective against depression; we examine whether Blacks engage in more of these behaviors than Whites at low, moderate, and/or high levels of stress; and we test the hypothesis that Blacks exposed to high levels of stress are protected against depression if engaged in UHBs at the time of the stressors and, simultaneously, that Whites are not similarly conferred such protection from these behaviors. Finally, the hypothesis outlined by Jackson et al. (2009) suggests that the stress exposure of Blacks is qualitatively different compared to that of Whites. The NESARC data allows us to examine a measure of perceived racial discrimination in order to test whether Blacks who report high levels of discrimination and engage in UHBs have less depression than Blacks who report high levels of discrimination and do not engage in UHBs.
The data used in the present study have distinct advantages over those in ( Jackson, Knight et al. 2009 ): namely a larger sample size, DSM-IV diagnoses of major depression at two time points, DSM-IV diagnoses of nicotine dependence and alcohol use disorders, and comprehensive measures of nicotine, alcohol consumption, and stressful life events. Extending the analyses of Jackson et al, (2009) to include pathological alcohol and nicotine consumption is important in testing the hypothesis, as high and chronic levels of nicotine and alcohol use are behaviors most associated with poor physical health outcomes; if the Black/White “paradox” can be attributed to discrepant mental and physical health consequences of unhealthy behaviors, the nature and degree of engagement in those behaviors most implicated in poor somatic health should be considered.
Data are drawn from the National Epidemiologic Survey on Alcohol and Related Conditions (NESARC), a two-wave longitudinal survey of adults in the United States residing in households and group quarters. Wave 1 was conducted in 2001-2002 (N=43,093); young adults, Hispanics and Blacks were oversampled, with an overall response rate of 81%. Respondents were re-interviewed for Wave 2 approximately three years after Wave 1, with 34,653 (80.4%) successfully re-interviewed. More information on the study methods is found elsewhere ( Grant, Goldstein et al. 2009 ). The research protocol, including written informed consent procedures, received full ethical review and approval from the U.S. Census Bureau and the U.S. Office of Management and Budget.
All measures were assessed using the Alcohol Use Disorder and Associated Disabilities Interview Schedule-DSM-IV Version (AUDADIS-IV). We used measures of race/ethnicity, UHBs, and stressful life events ascertained at Wave 1 and measures of the outcomes, major depression and physical illness, from Wave 2. This design established the temporality of the exposures as occurring prior to the outcome; we controlled for major depression at Wave 1 in all analyses for which depression at Wave 2 was an outcome to further establish temporal sequence.
Race/ethnicity
We included self-identified non-Hispanic Whites (N=19,216) and non-Hispanic Blacks (N=6,065) who reported being born in the U.S. Foreign-born Blacks (N=664) and Whites (N=1,264) were excluded from the present analysis because patterns of substance use, depression and stressful life events differ between foreign- and non-foreign born individuals.
‘Unhealthy behaviors’ (UHBs)
We considered three types of ‘UHBs’: alcohol consumption, nicotine consumption, and body mass index (BMI). While a compelling argument can be made regarding the validity of terming alcohol consumption, nicotine consumption, and BMI collectively as ‘UHBs’ (e.g., moderate consumption of alcohol may be cardioprotective ( Klatsky 2009 )), we have used this terminology to remain consistent with the prior empirical support for the hypothesis we are testing ( Jackson, Knight et al. 2009 ). For our replication analysis, we use definitions exactly consistent with Jackson, Knight et al. (2009) , namely a UHB count measure (0-3) indicating if the individual ever consumed at least one alcoholic beverage in their lifetime, ever smoked at least 100+ cigarettes in their lifetime, and whether they currently have a BMI≥30. For our extended test of the overall theory, we used the following definitions:
- Alcohol consumption in the past 12 -months at Wave 1 was operationalized as a three-level variable: no consumption (N=2,293); non-pathological consumption (i.e., any level of consumption but no alcohol abuse/dependence diagnosis) at levels less than weekly binge (>4 drinks for men or >3 drinks per women on at least one occasion in the past year) (N=13,765); and DSM-IV alcohol abuse/dependence or at least weekly binge drinking (N=8,593). Measurement of alcohol consumption and diagnoses is a particular strength of the AUDADIS-IV instrument; diagnoses are made based on assessment of over 40 symptom items, and the excellent reliability and validity of alcohol diagnosis in the AUDADIS-IV have been extensively documented both the United States and internationally (see Hasin et al., 2007 ). Limited differential item functioning by race/ethnicity has been noted for alcohol disorder criteria in Item Response Theory analysis ( Saha, Chou et al. 2006 ).
- Nicotine consumption in the past 12 -months at Wave 1 was operationalized as a three-level variable: no nicotine use (N=18,601); non-pathological use (any level of use but no DSM-IV-defined nicotine dependence diagnosis) (N=3,358); and DSM-IV nicotine dependence (N=3,313). The good reliability and validity of nicotine dependence in the AUDADIS-IV have been well-documented ( Grant, Hasin et al. 2004 ). Limited differential item functioning by race/ethnicity has been noted for nicotine dependence criteria in Item Response Theory analysis ( Saha, Compton et al. 2010 ).
- Unhealthy eating in the past 12 -months at Wave 1 was operationalized using current BMI based on respondent’s self-reported height and weight. BMI an imperfect proxy for unhealthy eating; BMI is known to be determined by more than simply caloric intake, including exercise patterns and genetic vulnerability ( Hetherington and Cecil 2010 ). However, empirical studies have documented a robust correlation between unhealthy eating and BMI (e.g., ( Haimoto, Iwata et al. 2008 ; Kent and Worsley 2009 )). Three categories were created: BMI<25 (N=10,252), BMI greater than or equal to 25 but less than 35 (N=12,615), and BMI≥35 (N=2,414). While conventional cut-points define overweight as BMI between 25 and <30 and obese as ≥30 ( Centers for Disease Control and Prevention 2010 ), we chose more conservative cut points due to known error in the measurement BMI ( Rothman 2008 ), often overestimating an individual’s true body size.
- UHB count . The three measures described above were combined to create an overall measure of UHBs, comparable to that in Jackson et al. (2009) . Respondents were given a score of 0 for the least severe level of each behavior (i.e., no alcohol consumption, no nicotine consumption, or BMI<25), one point for the moderate level, and two points for the most severe level (i.e., DSM-IV alcohol abuse/dependence or at-least weekly binge drinking, DSM-IV nicotine dependence, or BMI≥35) within each unhealthy behavior category. Based on this summary score, we created a three level variable indicating no unhealthy behaviors (count was equal to zero) (N=2,539), low levels of unhealthy behavior (count was 1, 2, or 3) (N=20,077), and high levels of unhealthy behaviors (count of 4 or more) (N=2,656).
Stressful life events in the past 12-months
Twelve stressful life events were assessed at Wave 1 using a checklist with dichotomous response options: family member or close friend had a serious illness (38.4%), family member or close friend died (32.1%), respondent changed jobs/job responsibilities/work hours (23.8%), moved or someone new came to live with respondent (15.8%), major financial crisis/unable to pay bills/bankruptcy (10.6%), trouble with a boss or co-worker (8.7%), unemployed and looking for work >1 month (7.7%), respondent or family member was the victim of a crime (6.7%), fired or laid off (5.9%), problems with neighbor/friend/relative (5.8%), separated/divorced/broke up (5.4%), and respondent or a family member had trouble with police/got arrested/sent to jail (5.1%).
Figure 1 shows the relationship between number of past year stressful life events, race, and depression. As shown, the number of stressful life events at Wave 1 was not linearly related to depression at Wave 2 among Blacks, though the difference in prevalence between Blacks and Whites was not statistically significantly different at any level of stressful life events save for among those with one stressful life event (p=0.04). Also shown in Figure 1 , the confidence intervals for the proportions substantially overlap, indicative of the small sample sizes among those with high levels of stress. Therefore, we created a categorical measure of the number of stressful life events reported by the respondent. We extensively evaluated the appropriate threshold for ‘high stress’, and found that the direction and magnitude of the results were not dependent on the upper cutpoint used. We also evaluated whether the data would fit a quadratic term for past year stressful life events; the results did not change when a quadratic term was used. Therefore, to maximize statistical power and to provide the best fit to these data, we used the following cut points: no stressful life events in the past-year (N=7,274), one or two stressful life events (N=11,832), and three or more (N=6,175). Finer categorizations could not be utilized due to the minimum cell sizes required to conduct large-sample statistics.

Prevalence of depression at Wave 2 by number of past year stressor among non-Hispanic U.S.-born Whites (N=19,216) and non-Hispanic U.S.-born Blacks (N=6,065) in the general population
Perceived discrimination
Respondents self-reporting Black race were asked at the Wave 2 interview, “How often have you experienced discrimination, been prevented from doing something, or been hassled or made to feel inferior in any of the following situations because of your race?” The frequencies of seven discrimination experiences in the past 12 months were assessed (e.g., obtaining health care or health insurance coverage, obtaining a job or while on the job, or being called a racist name) ( Krieger, Smith et al. 2005 ). The scale showed good internal consistency reliability (α=0.76) ( Ruan, Goldstein et al. 2008 ). Responses were summed and a three-level variable was created indicating: no discriminatory experiences reported (N=3,708), a low level of discriminatory experiences (more than zero but less than the 75 th percentile on the scale, N=1,753), and a high level of discriminatory experiences (75 th percentile or greater, N=604).
Major Depressive Episode (MDE)
The good reliability and validity of DSM-IV major depression diagnosis in the AUDADIS-IV have been well-documented ( Hasin, Goodwin et al. 2005 ). At Wave 1, major depression in the past 12-months or prior to the past 12-months was assessed; we combined these timeframes to create a W1 lifetime depression diagnosis, and used this variable as a control in all analyses predicting major depression at Wave 2. At Wave 2, major depression was assessed in the past 12-months, and since the last interview but prior to the past 12-months. We combined these times frames to create a W2 depression diagnosis.
Physical illness
We examined Black/White differences in fourteen physical illnesses (e.g., arteriosclerosis, hypertension, diabetes, heart attack, high cholesterol, ulcer) assessed at Wave 2. Physical illness status was based on respondent self-report of a physician diagnosis.
Control variables
In all analyses we also controlled for age, sex, past-year personal income, education, and region of residence as assessed at Wave 1. We also controlled for major depression at prior to the past-year or the past-year Wave 1; Blacks had a lower prevalence of past year (OR=0.80, 95% C.I. 0.66-0.96) and lifetime depression (OR=0.56, 95% C.I. 0.49-0.64) at Wave 1.
Statistical analysis
Prevalence estimates by race, stressful life events, and UHBs were generated using cross-tabulations. Odds ratios and 95% confidence intervals were generated using logistic regression. All interaction tests are on the multiplicative scale. All analyses were conducted using SUDAAN software to adjust standard errors for the non-random probability of selection into the sample. All prevalence estimates and odds ratios are sample weighted to be representative of the U.S. population based on the year 2000 census.
Overall Black/White differences in the NESARC data
Consistent with prior literature, Blacks were less likely to have Wave 2 major depression (OR=0.80, 95% C.I. 0.70-0.91) and more likely to have a Wave 2 physical illness (OR=1.20, 95% C.I. 1.08-1.35) compared with Whites (data not shown).
Results of the Jackson et al. (2009) replication attempt
Our findings did not replicate those of Jackson et al. (2009) . Figures 2a and 2b show the predicted probability of depression based on the results of a logistic regression model categorizing UHB consumption (any lifetime smoking, any lifetime drinking, and/or current obesity) and past-year stressful life events, and controlling for age, sex, past-year personal income, education, region of residence, and major depression at Wave 1. Among Whites, the figures indicate a higher predicted probability of depression with each increasing level of UHB consumption and each increasing level of stressful life events. Among Blacks, the pattern is less consistent, but no evidence emerges suggesting that those who engage in more UHB consumption have less depression. There were no significant interactions between stressful life events and UHBs predicting depression among Blacks (F=1.20, df=6, p=0.32) or Whites (F=0.43, df=6, p=0.86), and no significant three-way interaction between stressful life events, UHBs, and race (F=1.05, df=17, p=0.42).

Predicted probability of depression at Wave 2 based on unhealthy behaviors as defined by Jackson et al. (2009) * and past-year stressful life events at Wave 1, by race, among non-Hispanic U.S.-born Whites (Figure 2a, N=19,216) and non-Hispanic U.S.-born Blacks Figure 2b, N=6,065) in the general population
* UHBs defined to be consistent with Jackson et al. (2009) : any consumption of at least one alcoholic beverage in lifetime, any consumption of 100+ cigarettes in lifetime, and/or current BMI≥30.
As part of our replication attempt, we also conducted an analysis with a mean centered, continuous stress variable in order to more closely approximate Jackson et al. (2009) and Mezuk et al. (2010) , despite the evident violation of the linearity assumption among Blacks (shown in Figure 1 ). Among Blacks at high levels of mean-centered stress, those with 0 UHBs have a higher predicted probability of depression compared to those with 1 UHB, and those with 1 UHB have a higher predicted probability of depression compared to those with 2 or 3 UHBs (consistent with Jackson et al. (2009) and Mezuk et al. (2010) ). The same pattern is not evident among Whites (results not shown). However, this result arises entirely from the misspecification of the regression model by entering stress as a continuous variable among Blacks. Further, none of the interactions were significant when using the mean centered, continuous stress variable (interaction of stress and UHBs among Blacks: F=0.89, df=3, p=0.45; interaction of stress and UHBs among Whites: F=1.46, df=3, p=0.23; three way interaction of stress, UHBs, and race: F=1.37, df=7, p=0.23).
Results of the extended test of the Jackson et al hypothesis
Are uhbs protective against depression and is stress associated with more depression.
We found that Wave 1 UHBs, at any level, are not protective against Wave 2 depression. Stress, however, is prospectively predictive of Wave 2 depression. Table 1 shows the odds of Wave 2 depression given Wave 1 UHBs among the whole sample, and for Blacks and Whites separately. Among Whites, greater Wave 1 alcohol, nicotine, BMI severity, and overall UHB score predicted higher odds of depression at Wave 2. Among Blacks, no significant odds ratios were observed for the relation between Wave 1 UHBs and Wave 2 depression. However, all four odds ratios for the highest UHB category were in the direction consistent with high levels of UHB predicting greater odds of depression. Among both Whites and Blacks, more stressful life events at Wave 1 predicted greater odds of depression at Wave 2 (see Table 1 ).
Alcohol consumption, nicotine consumption, BMI, and stressful life events prospectively predicting major depression three years later among non-Hispanic U.S.-Born Whites (N=19,216) and non-Hispanic U.S-born Blacks (N=6,065)
Bold text indicates statistical significance at p<0.05
AOR = odds ratio adjusted for age, sex, past-year personal income, education, region of residence, and major depression at Wave 1
Do Blacks report higher levels of UHBs?
Blacks had lower odds of Wave 1 alcohol consumption, nicotine consumption, and any UHBs compared with Whites, but a higher proportion of Blacks were in high Wave 1 BMI categories compared with Whites. This finding held in every level of Wave 1 stressful life events (see Table 2 ) save the lowest level. Among those with no stressful life events, there was no significant relationship between UHBs and race. The magnitude and strength of the relationship between UHB and race increased with each level of stressful life event category.
Odds of alcohol consumption, nicotine consumption, high BMI, and ‘unhealthy behaviors’ in non-Hispanic U.S.-born Blacks (N=6, 065) compared to non-Hispanic U.S.-born Whites (N=19,216) at low, moderate, and high levels of stress
AOR = odds ratio adjusted for age, sex, past-year personal income, education, and region of residence
Do UHBs have a differential effect on depression among Blacks and Whites at certain stress levels?
Little support was found for the hypothesis that UHBs have a differential effect on Blacks and Whites at high levels of stress. We examined whether the effect of Wave 1 UHBs on Wave 2 depression differed by race and Wave 1 stressful life event status ( Table 3 ). Results indicated that a low level of unhealthy behaviors is protective against depression in Blacks (OR=0.06, 95% C.I. 0.01-0.24) but not Whites (OR=2.14, 95% C.I. 0.71-6.48) among those at low levels of stress. However, the three-way interaction test was not significant, limiting the conclusions that can be drawn from this association. We did find a significant three-way interaction between race, Wave 1 stress, and Wave 1 BMI (F=2.11, df=12, p=0.03). Based on the patterns of odds ratios shown in Table 3 , we conclude that there is evidence to suggest a protective effect of Wave 1 BMI 25-34 on Wave 2 depression in Blacks but not Whites, but only at very low levels of stress.
Odds of depression at Wave 2 based on unhealthy behaviors and past-year stressful life events at Wave 1, by race and sex, among non-Hispanic U.S.-born Blacks (N=6,065) and non-Hispanic U.S.-born Whites (N=19,216) in the general population
We also examined these patterns by sex (results not shown). UHBs significantly interacted with race among men only (F=6.07, df=2, p=0.004), whereby Black men reporting a low level of unhealthy behaviors had significantly lower odds of depression compared to White men (OR=0.06, 95% C.I. 0.01-0.24). Similar to the aggregated analysis, a three way interaction between Wave 1 BMI, race, and Wave 1 stress was statistically significant in men (F=2.28, df=12, p=0.02). This interaction was significant at the trend level among women (F=1.7, df=12, p=0.09).
Are UHBs protective against depression among Blacks reporting more race-specific stress?
Wave 1 UHBs did not moderate the effect of perceived discrimination reported at Wave 2 among Blacks. The observed prevalence of Wave 2 depression by UHB consumption and discrimination experiences are shown in Figure 3 . In unadjusted analyses, Wave 1 non-pathological nicotine use was associated with a lower odds of depression at Wave 2 compared to no nicotine use (OR=0.48, 95% C.I. 0.24-0.96) (data not shown). However, the effect was no longer significant when lifetime depression at Wave 1 was controlled (OR=0.52, 95% C.I. 0.26, 1.05) (data not shown). No other odds ratios were significant in unadjusted or adjusted analyses. We also replicated these analyses using UHBs defined at Wave 2 (concurrent to measurement of discrimination): results were unchanged.

Prevalence of depression at Wave 2 based on unhealthy behaviors and lifetime perceived discrimination exposure among non-Hispanic U.S.-born Blacks (N=6,065) in the general population
The present study did not find support for the hypothesis that engaging in unhealthy behaviors ameliorates major depression among Blacks in the U.S. exposed to high levels of stress, or that a differential effect of UHBs on depression among Blacks compared with Whites accounts for the mental/physical health paradox. First, we showed that the results presented in Jackson et al. (2009) and Mezuk et al (2010) do not replicate in a nationally-representative sample using model specification to account for the non-linear association between stress and depression among Blacks that we observed in these data. Next, as part of a more in-depth investigation of the hypothesis, we demonstrated that the relationship between depression and alcohol consumption, nicotine consumption, and BMI among Blacks is not statistically significant and, in fact, the direction of association between UHBs and depression is positive, not negative. Third, we documented that Blacks are less likely to engage in alcohol and nicotine consumption but have higher BMI than Whites at all levels of stress. We note, however, that an overall count of UHBs was not associated with race among those with no stressful life events. This suggests a complex patterning of unhealthy behaviors and race across stress levels that should be more comprehensively examined in these and other data in future analyses.
Finally, we showed that while race, stress, and BMI did interact significantly to predict depression in this sample, being overweight was protective against depression among Blacks only at very low levels of stress . Similarly, among those with no stressful life events, we found that low levels of UHBs were associated with less depression in Blacks but not Whites, though this result was significant among men only and a three-way interaction test was not significant. Furthermore, examination of perceived discrimination as a measure of stress in Blacks revealed no significant effects of unhealthy behaviors on depression at any exposure level. Taken together, these results indicate that engagement in unhealthy behaviors, especially at pathological levels, does not protect against depression, and that stress pathways do not operate differently in Blacks compared with Whites in the U.S. Thus, our confidence in the group-specific stress and coping hypothesis proposed by Jackson and colleagues ( Jackson and Knight 2006 ; Jackson, Knight et al. 2009 ; Mezuk et al. 2010 ) is diminished. Although UHBs may have immediate positive psychological effects via the HPA axis, the evidence presented here suggests these effects are not robust enough to prevent the clinical manifestation of major depression.
We did find evidence that some measures of unhealthy behaviors were significant predictors of depression in Whites but not Blacks. For example, at high levels of stress, Whites with high BMI, nicotine dependence, and a high unhealthy behavior count were more likely to evidence depression compared with Whites without these unhealthy behaviors, but the same predictors were not significant among Blacks. However, we caution against over-interpretation of these findings; as noted by Gelman and Stern: “The Difference Between ‘Significant’ and ‘Not Significant’ is not Itself Statistically Significant” ( Gelman and Stern 2006 ). There were no significant interactions between race and unhealthy behaviors at high levels of stress, indicating that the odds ratios across race categories were not statistically distinguishable. Further, ignoring significance levels, thirty-two of forty-eight odds ratios calculated (67%) in the total sample were in the same direction for Whites and Blacks. Thus, the most appropriate conclusion from these data is the pathway through which unhealthy behaviors and stress impact depression does not differ in Blacks compared with Whites in the U.S., and that in general, UHBs increase the risk for depression at all levels of stress.
In the present analysis we predicted major depression during a three-year follow-up based on stressful events and UHBs assessed at baseline. While this strategy is most appropriate to establish temporality, it may overlook a key component of the hypothesis, namely, that UHBs in response to stress are active coping strategies for the suppression of immediate depressive symptoms ( Benowitz 1988 ; Koob, Roberts et al. 1998 ; Dallman, Pecoraro et al. 2003 ; Jackson and Knight 2006 ; Jackson, Knight et al. 2009 ). Thus, we may have failed to capture protective effects because we did not examine major depressive episodes concurrent with stressors and unhealthy behaviors. To explore this possibility, we re-analyzed our data using stress, UHBs and depression diagnoses all measured concurrently, at both baseline and follow-up. Neither analysis suggested different conclusions; results were generally consistent with those presented in our main analyses. Thus, we conclude that these data, analyzed multiple ways at multiple time points, do not provide sufficient evidence for either a protective effect of UHBs on depression, or a differential pathway through which unhealthy behaviors affect depression in Blacks compared with Whites, regardless of the exposure to stress.
These results decrease confidence in the validity of the hypothesis that group-specific stress processes explain the depression difference in Blacks and Whites, leaving open other theories to be tested. Measurement and selection bias hypotheses are unlikely to fully explain the “paradox” ( Breslau, Javaras et al. 2008 ), suggesting the need for both new theories and more direct tests of existing theories based on the premise that the lower prevalence of depression in Blacks is not artefactual. Studies suggest that Blacks develop different coping strategies when faced with life stress compared with Whites ( Smith 1985 ; Wilson 1989 ; Maton, Teti et al. 1996 ), which, given the extraordinary nature and degree of stress to which Blacks are exposed starting at a young age, are hypothesized to develop over the life course. Compared with Whites, Blacks are more likely to find emotional strength and support in religious communities ( Taylor, Chatters et al. 1996 ; Gibson and Hendricks 2006 ; Giger, Appel et al. 2008 ), and develop racial self-esteem and strong ethnic identity ( Nagel 1994 ). Further, extensive research has documented the ‘John Henryism’ effect among Blacks in the U.S., the personality trait characterized by active coping with stressful and negative experiences and associated with worse health outcomes for Blacks at high levels of socio-economic position ( James, Strogatz et al. 1987 ; James 1994 ). Alternatively, the present DSM nosology may not accurately tap Black psychological responses to their unique stress exposures, and therefore DSM-IV depression as currently defined may not be the appropriate outcome to fully understand racial differences in depressive mood states ( Brown 2003 ; Kendrick, Anderson et al. 2007 ). Support for this theory can be drawn from the multiple studies showing that Blacks report lower levels of well-being, higher levels of distress, and higher depressive scores when measured on non-DSM instruments ( Brown 2003 ; Mabry and Kiecolt 2005 ). Taken together, the results from the present study should serve as a catalyst to promote the advancement of innovative and alternative theories to explain the Black/White paradox in mental and physical health. Few studies are conducted with the primary aim of untangling this paradox, a situation that should be redressed. Such research should include rich measurement of the social and political context, and conduct in-depth examination of the role of ethnic identity, religion, and responses to group-specific stressor, such as racial discrimination.
Several limitations should be noted. First, our measure of stressful life events is a checklist of experiences susceptible to respondent subjectivity and appraisal processes, and without regard to salience, severity, or context of experience. Substantial evidence indicates that objective measures of stressful experiences as well as information on the context of the experience is necessary to fully analyze and interpret stress in mental health research ( Dohrenwend 1998 ; Dohrenwend 2006 ). Additionally, the stressful events experienced by Blacks in the U.S. may be more chronic and race-specific than what is captured in the scale of past-year stressors ( Jones 2000 ; Kreiger 2000 ). We attempted to mitigate this limitation by also using a measure of perceived racial/ethnic discrimination. Further, we evaluated whether results changed if higher cut-offs of past-year stressful experiences were used to define the ‘high stress’ exposure group; higher cut-offs did not change the results. Thus, the conclusions from these data are limited by the available stress measure.
In conclusion, the persistent differences in health outcomes between White and Black adults remain one of the most challenging public health issues in the U.S. As theories regarding the etiology of these differences continue to develop, the mental health ‘paradox’ will be increasingly important to explain as part of a robust etiologic pathway. Substantive etiologic hypotheses that simultaneously explain why Blacks in the U.S. have higher rates of physical illness and lower rates of mood disorders need to be tested directly in order to resolve the ‘paradox’ and progress toward intervention and prevention efforts.
Hypothesis that Black-White depression paradox is due to protective effects of unhealthy behaviors at high stress unsupported.
Blacks less likely to engage in alcohol consumption or cigarette smoking compared with Whites, but have higher average BMI.
Engaging in unhealthy behaviors is not protective against depression at any stress level in either Blacks or Whites.
Acknowledgments
This research was supported in part by a fellowship from the National Institute of Mental Health (T32MH013043-36, Barnes) and a fellowship from the National Institute of Drug Abuse (F31-DA026689, K. Keyes).
Publisher's Disclaimer: This is a PDF file of an unedited manuscript that has been accepted for publication. As a service to our customers we are providing this early version of the manuscript. The manuscript will undergo copyediting, typesetting, and review of the resulting proof before it is published in its final citable form. Please note that during the production process errors may be discovered which could affect the content, and all legal disclaimers that apply to the journal pertain.
- Baker FM. Diagnosing depression in African Americans. Community Mental Health Journal. 2001; 37 (1):31–38. [ PubMed ] [ Google Scholar ]
- Benowitz NL. Drug therapy. Pharmacologic aspects of cigarette smoking and nicotine addition. N Engl J Med. 1988; 319 (20):1318–30. [ PubMed ] [ Google Scholar ]
- Breslau J, Aguilar-Gaxiola S, et al. Specifying race-ethnic differences in risk for psychiatric disorder in a USA national sample. Psychol Med. 2006; 36 (1):57–68. [ PMC free article ] [ PubMed ] [ Google Scholar ]
- Breslau J, Javaras KN, et al. Differential item functioning between ethnic groups in the epidemiological assessment of depression. J Nerv Ment Dis. 2008; 196 (4):297–306. [ PMC free article ] [ PubMed ] [ Google Scholar ]
- Brown T. Critical race theory speaks to the sociology of mental health: mental health problems produced by racial stratification. Journal of Health and Social Behavior. 2003; 44 (3):292–301. [ PubMed ] [ Google Scholar ]
- Centers for Disease Control and Prevention. 2010. http://www.cdc.gov/healthyweight/assessing/bmi/adult_bmi/index.html .
- Dallman MF, Pecoraro N, et al. Chronic stress and obesity: a new view of “comfort food” Proc Natl Acad Sci U S A. 2003; 100 (20):11696–701. [ PMC free article ] [ PubMed ] [ Google Scholar ]
- Dohrenwend BP. Adversity, stress, and Psychopathology. New York: Oxford University Press, USA; 1998. [ Google Scholar ]
- Dohrenwend BP. The role of adversity and stress in psychopathology: some evidence and its implications for theory and research. J Health Soc Behav. 2000; 41 (1):1–19. [ PubMed ] [ Google Scholar ]
- Dohrenwend BP. Inventorying stressful life events as risk factors for psychopathology: Toward resolution of the problem of intracategory variability. Psychol Bull. 2006; 132 (3):477–95. [ PMC free article ] [ PubMed ] [ Google Scholar ]
- Gelman A, Stern H. The Difference Between “Significant” and “Not Significant” is not Itself Statistically Significant. American Statistician. 2006; 60 (4):328–331. [ Google Scholar ]
- Geronimus AT. Power and Decision: The Social Control of Reproduction. Cambridge, MA: Harvard University Press; 1994. The weathering hypothesis and the health of African American women and infants: Implicatins for reproductive strategies and policy analysis. [ Google Scholar ]
- Geronimus AT. Black/White differences in the relationship of maternal age to brithweight: A population basd test of the weathering hypothesis. Soc Sci Med. 1996; 42 (4):589–597. [ PubMed ] [ Google Scholar ]
- Gibson LM, Hendricks CS. Integrative review of spirituality in African American breast cancer survivors. ABNF J. 2006; 17 (2):67–72. [ PubMed ] [ Google Scholar ]
- Giger JN, Appel SJ, et al. Church and spirituality in the lives of the African American community. J Transcult Nurs. 2008; 19 (4):375–83. [ PubMed ] [ Google Scholar ]
- Grant BF, Goldstein RB, et al. Sociodemographic and psychopathologic predictors of first incidence of DSM-IV substance use, mood and anxiety disorders: results from the Wave 2 National Epidemiologic Survey on Alcohol and Related Conditions. Mol Psychiatry. 2009; 14 (11):1051–66. [ PMC free article ] [ PubMed ] [ Google Scholar ]
- Grant BF, Hasin DS, et al. Nicotine dependence and psychiatric disorders in the United States: results from the national epidemiologic survey on alcohol and related conditions. Arch Gen Psychiatry. 2004; 61 (11):1107–15. [ PubMed ] [ Google Scholar ]
- Haimoto H, Iwata M, et al. Long-term effects of a diet loosely restricting carbohydrates on HbA1c levels, BMI and tapering of sulfonylureas in type 2 diabetes: a 2-year follow-up study. Diabetes Res Clin Pract. 2008; 79 (2):350–6. [ PubMed ] [ Google Scholar ]
- Hasin DS, Goodwin RD, et al. Epidemiology of major depressive disorder: results from the National Epidemiologic Survey on Alcoholism and Related Conditions. Arch Gen Psychiatry. 2005; 62 (10):1097–106. [ PubMed ] [ Google Scholar ]
- Hasin DS, Stinson FS, et al. Prevalence, correlates, disability, and comorbidity of DSM-IV alcohol abuse and dependence in the United States: results from the National Epidemiologic Survey on Alcohol and Related Conditions. Arch Gen Psychiatry. 2007; 64 (7):830–42. [ PubMed ] [ Google Scholar ]
- Heckler MM. Report of the Secretary’s Task Force on Black and Minority Health. Wasington, DC: Natl. Academy Press; 1985. Task Force on Black and Minority Health. [ Google Scholar ]
- Hetherington MM, Cecil JE. Gene-environment interactions in obesity. Forum Nutr. 2010; 63 :195–203. [ PubMed ] [ Google Scholar ]
- Jackson JS, Knight KM. Race and self-regulatory behaviors: the role of the stress response and HPA axis in physical and mental health disparities. In: Carstensen LL, Schaie KW, editors. Social structure, Aging, and Self-Regulation in the Elderly. New York, NY: Springer; 2006. [ Google Scholar ]
- Jackson JS, Knight KM, et al. Race and Unhealthy Behaviors: Chronic Stress, the HPA Axis, and Physical and Mental Health Disparities Over the Life Course. Am J Public Health 2009 [ PMC free article ] [ PubMed ] [ Google Scholar ]
- James SA. John Henryism and the health of African Americans. Culture of Medicine and Psychiatry. 1994; 18 :163–182. [ PubMed ] [ Google Scholar ]
- James SA, Strogatz DS, et al. Socioeconomic status, John Henryism, and hypertension in blacks and whites. Am J Epidemiol. 1987; 126 (4):664–73. [ PubMed ] [ Google Scholar ]
- Jones CP. Levels of racism: a theoretic framework and a gardener’s tale. Am J Public Health. 2000; 90 (8):1212–5. [ PMC free article ] [ PubMed ] [ Google Scholar ]
- Kendrick L, Anderson NLR, et al. Perceptions of depression among young African American men. Family and Community Health. 2007; 30 (1):63–73. [ PubMed ] [ Google Scholar ]
- Kent LM, Worsley A. Trends in BMI, diet and lifestyle between 1976 and 2005 in North Sydney. Asia Pac J Clin Nutr. 2009; 18 (3):453–61. [ PubMed ] [ Google Scholar ]
- Kessler RC, Crum RM, et al. Lifetime co-occurrence of DSM-III-R Alcohol Abuse and Dependence WIth Other Psychiatric Disorders in the National COmorbidity Survey. Arch Gen Psychiatry. 1997; 54 :313–321. [ PubMed ] [ Google Scholar ]
- Kessler RC, McGonagle KA, et al. Lifetime and 12-month prevalence of DSM-III-R psychiatric disorders in the United States. Results from the National Comorbidity Survey. Arch Gen Psychiatry. 1994; 51 (1):8–19. [ PubMed ] [ Google Scholar ]
- Kessler RC, Mickelson KD, et al. The prevalence, distribution, and mental health correlates of perceived discrimination in the United States. J Health Soc Behav. 1999; 40 (3):208–30. [ PubMed ] [ Google Scholar ]
- Klatsky AL. Alcohol and cardiovascular diseases. Expert Rev Cardiovasc Ther. 2009; 7 (5):499–506. [ PubMed ] [ Google Scholar ]
- Kleinman A. Culture and depression. N Engl J Med. 2004; 351 (10):951–3. [ PubMed ] [ Google Scholar ]
- Koob GF, Roberts AJ, et al. Neurocircuitry targets in ethanol reward and dependence. Alcohol Clin Exp Res. 1998; 22 (1):3–9. [ PubMed ] [ Google Scholar ]
- Kreiger N. Social Epidemiology. New York: Oxford University Press; 2000. Discrimination and health; pp. 36–75. [ Google Scholar ]
- Krieger N. Racial and gender discrimination: risk factors for high blood pressure? Soc Sci Med. 1990; 30 (12):1273–81. [ PubMed ] [ Google Scholar ]
- Krieger N, Sidney S. Racial discrimination and blood pressure: the CARDIA Study of young black and white adults. Am J Public Health. 1996; 86 (10):1370–8. [ PMC free article ] [ PubMed ] [ Google Scholar ]
- Krieger N, Smith K, et al. Experiences of discrimination: validity and reliability of a self-report measure for population health research on racism and health. Soc Sci Med. 2005; 61 (7):1576–96. [ PubMed ] [ Google Scholar ]
- Leonard B. Stress, Depression, and the Activation of the Immune System. World Journal of Biological Psychiatry. 2000; 1 (1):17–25. [ PubMed ] [ Google Scholar ]
- Mabry JB, Kiecolt KJ. Anger in black and white: race, alienation, and anger. J Health Soc Behav. 2005; 46 (1):85–101. [ PubMed ] [ Google Scholar ]
- Massey DS. Residential segregation and neighborhood conditions in U.S. metropolitan areas. In: Smesler N, Wilson WJ, Mitchell F, editors. America Becoming Racial: Trends and Their Consequences. Wasington, D.C.: Natl Acad Press; 1985. pp. 391–434. [ Google Scholar ]
- Massey DS. Segregation and stratification: a biosocial perspective. DuBois Reviews of Social Science. 2004; 1 :7–25. [ Google Scholar ]
- Maton KI, Teti DM, et al. Cultural specificity of support sources, correlates and contexts: three studies of African-American and caucasian youth. Am J Community Psychol. 1996; 24 (4):551–87. [ PubMed ] [ Google Scholar ]
- McCord C, Freeman HP. Excess mortality in Harlem. N Engl J Med. 1990; 322 (3):173–7. [ PubMed ] [ Google Scholar ]
- McEwen BS. Allostasis and allostatic load: implications for neuropsychopharmacology. Neuropsychopharmacology. 2000; 22 (2):108–24. [ PubMed ] [ Google Scholar ]
- McEwen BS. Mood disordesr and allostatic load. Biological Psychiatry. 2003; 54 (3):200–207. [ PubMed ] [ Google Scholar ]
- McEwen BS. Protection and damage from acute and chronic stress: allostasis and allostatic overload and relevance to the pathophysiology of psychiatric disorders. Ann N Y Acad Sci. 2004; 1032 :1–7. [ PubMed ] [ Google Scholar ]
- Mezuk B, Rafferty JA, et al. Reconsidering the Role of Social Disadvantage in Physical and Mental Health: Stressful Life Events, Health Behaviors, Race, and Depression. Am J Epidemiology. 2010; 172 (11):1238–1249. [ PMC free article ] [ PubMed ] [ Google Scholar ]
- Nagel J. Constructing Ethnicity: Creating and Recreating Ethnic Identity and Culture. Social Problems. 1994; 41 (1) [ Google Scholar ]
- Neighbors HW, Trierweiler SJ, et al. Racial differences in DSM diagnosis using a semi-structured instrument: the importance of clinical judgment in the diagnosis of African Americans. J Health Soc Behav. 2003; 44 (3):237–56. [ PubMed ] [ Google Scholar ]
- Neighbors HW, Trierweiler SJ, et al. Psychiatric diagnosis of African Americans: diagnostic divergence in clinician-structured and semistructured interviewing conditions. J Natl Med Assoc. 1999; 91 (11):601–12. [ PMC free article ] [ PubMed ] [ Google Scholar ]
- Petit B, Western B. Mass imprisonment and the life course: race and class inequality in U.S. incarceration. American Sociological Review. 2004; 69 :601–612. [ Google Scholar ]
- Reiger DA, Farmer ME, et al. Comorbidity of mental disorders with alcohol and other drug abuse: results from the Epidemiologic Catchment Area (ECA) study. JAMA. 1990; 264 (19):2511–2518. [ PubMed ] [ Google Scholar ]
- Rogler LH. Methodological sources of cultural insensitivity in mental health research. Am Psychol. 1999; 54 (6):424–33. [ PubMed ] [ Google Scholar ]
- Rothman KJ. BMI-related errors in the measurement of obesity. Int J Obes (Lond) 2008; 32 (Suppl 3):S56–9. [ PubMed ] [ Google Scholar ]
- Ruan WJ, Goldstein RB, et al. The alcohol use disorder and associated disabilities interview schedule-IV (AUDADIS-IV): reliability of new psychiatric diagnostic modules and risk factors in a general population sample. Drug Alcohol Depend. 2008; 92 (1-3):27–36. [ PMC free article ] [ PubMed ] [ Google Scholar ]
- Saha TD, Chou SP, et al. Toward an alcohol use disorder continuum using item response theory: results from the National Epidemiologic Survey on Alcohol and Related Conditions. Psychol Med. 2006; 36 (7):931–41. [ PubMed ] [ Google Scholar ]
- Saha TD, Compton WM, et al. Dimensionality of DSM-IV nicotine dependence in a national sample: an item response theory application. Drug Alcohol Depend. 2010; 108 (1-2):21–8. [ PMC free article ] [ PubMed ] [ Google Scholar ]
- Smith E. Ethnic minorities: life stress, social support, and mental health issues. The Counseling Psychologist. 1985; 13 :537–579. [ Google Scholar ]
- Stansfeld SA. Social support and social cohesion. In: Marmot MG, Wilkinson RG, editors. Social determinants of health. New York: Oxford University Press; 2005. [ Google Scholar ]
- Strakowski SM, Keck PE, Jr, et al. Ethnicity and diagnosis in patients with affective disorders. J Clin Psychiatry. 2003; 64 (7):747–54. [ PubMed ] [ Google Scholar ]
- Taylor RJ, Chatters LM, et al. Black and white differences in religious participation: A multi-sample comparison. Journal for the Scientific Study of Religion. 1996; 35 :403–410. [ Google Scholar ]
- Teplin LA. The prevalence of severe mental disorder among male urban jail detainees: comparison with the Epidemiologic Catchment Area Program. Am J Public Health. 1990; 80 (6):663–9. [ PMC free article ] [ PubMed ] [ Google Scholar ]
- Teplin LA, Abram KM, et al. Prevalence of psychiatric disorders among incarcerated women. I. Pretrial jail detainees. Arch Gen Psychiatry. 1996; 53 (6):505–12. [ PubMed ] [ Google Scholar ]
- Williams DR. Racial variations in adult health status: patterns, paradoxes, and prospects. In: Smelser NJ, Wilson WJ, Mitchell F, editors. America Becoming: Racial Trends and Their Consequences. Washington, D.C.: National Academy Press; 2001. [ Google Scholar ]
- Williams DR, Gonzalez HM, et al. Prevalence and distribution of major depressive disorder in African Americans, Caribbean blacks, and non-Hispanic whites: results from the National Survey of American Life. Arch Gen Psychiatry. 2007; 64 (3):305–15. [ PubMed ] [ Google Scholar ]
- Williams DR, Jackson PB. Social sources of racial disparities in health. Health Aff (Millwood) 2005; 24 (2):325–34. [ PubMed ] [ Google Scholar ]
- Williams DR, Williams-Morris R. Racism and mental health: the African American experience. Ethn Health. 2000; 5 (3-4):243–68. [ PubMed ] [ Google Scholar ]
- Wilson MN. Child development in the context of the Black extended family. American Psychologist. 1989; 44 :380–383. [ Google Scholar ]
- Program Finder
- Admissions Services
- Course Directory
- Academic Calendar
- Hybrid Campus
- Lecture Series
- Convocation
- Strategy and Development
- Implementation and Impact
- Integrity and Oversight
- In the School
- In the Field
- In Baltimore
- Resources for Practitioners
- Articles & News Releases
- In The News
- Statements & Announcements
- At a Glance
- Student Life
- Strategic Priorities
- Inclusion, Diversity, Anti-Racism, and Equity (IDARE)
- What is Public Health?
Is the Hygiene Hypothesis True?
Did Covid shutdowns stunt kids' immune systems?
Caitlin Rivers
The hygiene hypothesis is the idea that kids need to be exposed to germs in order to develop healthy immune systems. We know that many common viruses did not circulate as widely during the pandemic, thanks to social distancing, masking, and other COVID mitigation measures. Are there downsides to those missed infections?
In this Q&A, Caitlin Rivers speaks with Marsha Wills-Karp, PhD, MHS , professor and chair of Environmental Health and Engineering , about the role of household microbiomes, birth, and vaccines in the development of kids’ immune systems—and whether early exposure really is the best medicine.
This Q&A is adapted from Rivers’ Substack blog, Force of Infection .
I think there’s some concern among parents who have heard about the hygiene hypothesis that there is a downside to all those stuffy noses that didn’t happen [during the COVID-19 pandemic]. Are there any upsides to viral infections? Do they help the immune system in some meaningful way?
I don’t think so.
You mentioned the hygiene hypothesis, which was postulated back in the ‘80s. German scientists noticed that families with fewer children tended to have more allergic disease. This was interpreted [to mean] that allergic disease was linked to experiencing fewer infections. I have explored this idea in my research for a couple of decades now.
This phenomenon has helped us to understand the immune system, but our interpretation of it has grown and expanded—particularly with respect to viruses. Almost no virus is protective against allergic disease or other immune diseases. In fact, infections with viruses mostly either contribute to the development of those diseases or worsen them.
The opposite is true of bacteria. There are good bacteria and there are bad bacteria. The good bacteria we call commensals . Our bodies actually have more bacterial cells than human cells. What we’ve learned over the years is that the association with family life and the environment probably has more to do with the microbiome. So one thing I would say is sanitizing every surface in your home to an extreme is probably not a good thing. Our research team showed in animals that sterile environments don’t allow the immune system to develop at all. We don’t want that.
What does contribute to the development of the immune system, if not exposure to viruses?
There are a number of factors that we’ve associated with the hygiene hypothesis over the last 20 years, and these exposures start very early in life. Cesarean sections, which do not allow the baby to travel through the birth canal and get exposed to the mother’s really healthy bacterial content, is a risk factor for many different immune diseases. Getting that early seeding with good bacteria is critical for setting up the child going forward. Breastfeeding also contributes to the development of a healthy immune system.
There are other factors. Our diets have changed dramatically over the years. We eat a lot of processed food that doesn’t have the normal components of a healthy microbiome, like fiber. These healthy bacteria in our gut need that fiber to maintain themselves. They not only are important for our immune system but they’re absolutely critical to us deriving calories and nutrients from our food. All these things contribute to a healthy child.
We’ve also noticed that people who live on farms have fewer of these diseases because they’re exposed to—for lack of a better term—the fecal material of animals. And what we have found is that it’s due to these commensal bacteria. That is one of the components that help us keep a healthy immune system. Most of us will probably not adopt farm life. But we can have a pet, we can have a dog.
I think all the pet lovers out there will be pleased to hear that.
There’s a lot of evidence that owning a pet in early childhood is very protective.
What about the idea that you need to be exposed to viruses in early life because if you get them as an adult, you’ll get more severely ill? We know that’s true for chickenpox, for example. Do you have any concerns about that?
We should rely on vaccines for those exposures because we can never predict who is going to be susceptible to severe illness, even in early childhood. If we look back before vaccines, children under 4 often succumbed to infections. I don’t think we want to return to that time in history.
Let me just give you one example. There’s a virus called RSV, it’s a respiratory virus. Almost all infants are positive for it by the age of 2. But those who get severe disease are more likely to develop allergic disease and other problems. So this idea that we must become infected with a pathogenic virus to be healthy is not a good one.
Even rhinovirus, which is the common cold, most people recover fine. But there’s a lot of evidence that for somebody who is allergic, rhinovirus exposures make them much worse. In fact, most allergic or asthmatic kids suffer through the winter months when these viruses are more common.
And that’s particularly salient because there is a lot of rhinovirus and enterovirus circulating right now.
From my point of view, right now, avoiding flu and COVID-19 is a priority. Those are not going to help you develop a healthy immune response, and in fact, they can do a lot of damage to the lungs during that critical developmental time. Data [show] that children that have more infections in the first 6 months to a year of life go on to have more problems.
It’s always surprising to me when I look at the data of the fraction of time that young children spend with these common colds—and this is pre-pandemic—it’s not uncommon for kids to be sick 50% of the time. That feels right as a parent, but it’s startling.
The other thing people don’t know is that the GI tract is where you get tolerized to all of your foods, allergens and things. Without those healthy bacteria in your gut, you can’t tolerate common allergens.
How does that relate to the guidance that’s changed over the years—that you should withhold peanuts in early life and now you’re supposed to offer them in early life?
The guidance to delay exposure to peanuts didn’t consider the fact that oral exposure to peanuts was not the only exposure kids were getting. There were peanut oils in all kinds of skin creams and other things. So kids got exposed through their skin, but they had no gut protection—and the GI tract is important for a tolerant system. If you have a healthy immune response, you get tolerized in early life.
This concept is a little bit different for those families who may already have a predisposition to allergies. But for the general public, exposure is key to protecting them in early life.
I think some parents look at the guidance that you should now offer peanuts in early life and say, “Are we not doing that with rhinovirus by masking kids or improving ventilation?” How should people think about the development of the immune system for food allergies compared to infections?
The thing about rhinoviruses is that after recovering, you’re not protected from the next infection. There is no real immune protection there. Most of us suffer from colds throughout our whole life. Like I said, bacterial exposure is what’s key to priming the immune response.
Also, we forget that a lot of kids die from the flu. Unlike COVID-19, where younger kids are not quite as susceptible to severe illness, that’s not true for flu. RSV, too, can be quite severe in young children and older adults.
Caitlin Rivers, PhD, MPH , is a senior scholar at the Johns Hopkins Center for Health Security and an assistant professor in Environmental Health and Engineering at the Johns Hopkins Bloomberg School of Public Health.
- Study Finds That Children’s Antibody Responses to COVID-19 Are Stronger Than Adults’
- Back to School: COVID, CDC Guidance, Monkeypox, and More
- A New Shot Prevents Serious Illness from RSV
Related Content
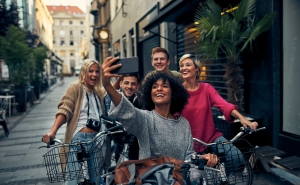
Research Identifies Characteristics of Cities That Would Support Young People’s Mental Health
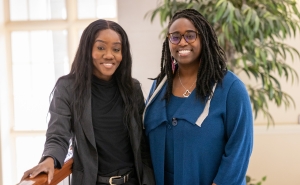
Student Spotlight: Glendedora Dolce
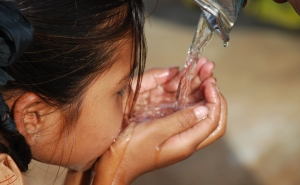
Study Estimates Nearly 70 Percent of Children Under Six in Chicago May Be Exposed to Lead-Contaminated Tap Water
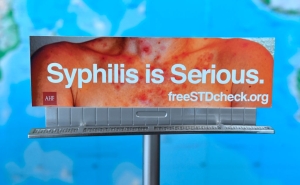
The U.S. Syphilis Spike Has Been Brewing for Decades
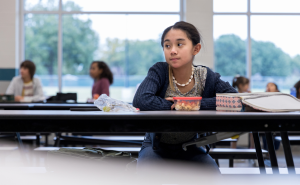
Hidden Food Insecurity: The Adolescents Who Aren’t Getting Enough to Eat
Mobile Menu Overlay
The White House 1600 Pennsylvania Ave NW Washington, DC 20500
A Proclamation on National Public Health Week, 2024
During National Public Health Week, we celebrate the life-saving care of public health professionals who work tirelessly to promote our country’s health, safety, and well-being. We recognize that the healthier Americans are as individuals, the stronger we are as a Nation.
In the last few years, we have made enormous progress in recovering from the pandemic, vaccinating 230 million Americans and getting kids back in school. None of that would have been possible without the courage and dedication of millions of first responders and social workers, doctors and nurses, and scientists and researchers. Public health professionals have always played an essential role in the life of our Nation — working to boost immunizations, improve safety standards for food and transportation, protect clean air and water, and more. We owe them for carrying us through tough times and making our country healthier and more prosperous long-term. We have to help make their jobs easier by investing in the health of the American people.
I have long said that health care should be a right in this country, not a privilege. That is why my Administration expanded coverage through the Affordable Care Act. Today, more Americans have health care coverage than under any other President, and millions of families are saving an average of $800 per year on insurance premiums. We have also invested $7.6 billion in community health centers so people in rural and underserved areas can get care close to home. After years of trying, we have succeeded in reducing prescription drug costs — for example, capping insulin at $35 per month for seniors on Medicare, down from as much as $400, and finally getting Medicare the authority to negotiate lower drug prices, as the Department of Defense and the Department of Veterans Affairs have long done. Starting next year, no senior on Medicare will have to pay more than $2,000 per year in total out-of-pocket drug costs — not even for expensive cancer medications that cost many times more.
At the same time, we are funding scientific research that will help us make quantum leaps forward in the prevention, detection, and treatment of deadly diseases. I established the Advanced Research Projects Agency for Health, securing $2.5 billion in bipartisan funding for scientists, innovators, and public health professionals making these advances. Further, the First Lady and I reignited the Cancer Moonshot, setting a bold goal to cut the cancer death rate by at least 50 percent over the next 25 years and boost support for people impacted by cancer. We also launched the first-ever White House Initiative on Women’s Health Research to close research gaps and maximize our ability to prevent, diagnose, and treat health conditions in women, like cardiovascular disease, Alzheimer’s disease, and endometriosis.
I am doing all I can to protect women’s fundamental freedom to make their own health care decisions. In the wake of the Supreme Court’s decision to overturn the constitutional right to choose, tens of millions of Americans are living under extreme State abortion bans that put women’s health and lives at risk and threaten doctors with jail time for providing the health care their patients need. In response, my Administration has taken steps to safeguard access to emergency medical care, support the ability to travel for reproductive health care, and strengthen privacy protections for patients and health care providers. I will keep pressing the Congress to restore the protections of Roe v. Wade into Federal law. It is the only way to ensure reproductive freedom for women in every State. At the same time, Vice President Harris is leading the effort to combat the maternal health crisis that is gripping our country, which has been especially devastating to Black and Native women and women in rural communities, where maternal mortality rates are unconscionably high.
We are also making historic investments in improving mental health by putting more counselors in schools and pushing insurers to cover mental health care at the same level as any other care.
We are working hard to ensure that substance use disorder is treated like any other disease by funding the expansion of prevention, harm reduction, treatment, and recovery support services. My Administration removed decades-long administrative barriers to treatment of opioid use disorder and expanded access to opioid overdose reversal medications like naloxone, and we are continuing to advance efforts to address the overdose epidemic and save lives. We are working to end the epidemic of gun violence that has shattered far too many American lives. Two summers ago, I signed the most significant gun safety law in nearly 30 years, funding States’ implementation of red flag laws and enhancing background checks for gun buyers under 21. I launched the first-ever White House Office of Gun Violence Prevention. I was also proud to sign the reauthorized Violence Against Women Act, building on the law that I first wrote years ago to expand protections and resources for today’s domestic violence survivors.
Meanwhile, my Administration is making the largest investment ever in fighting the public health crises caused by climate change. We are working to make communities more resilient to extreme weather and ensuring that 40 percent of our clean energy investments flow to the disadvantaged areas that have borne the brunt of toxic pollution for too long. Through the Bipartisan Infrastructure Law, we are also replacing every poisonous lead pipe in the country so anyone in America can turn on the faucet and drink clean water. We have released a national strategy to end hunger and reduce diet-related diseases, including expanding access to nutrition and obesity counseling, and we are providing millions of students with free, nutritious school meals.
Globally, we are making key investments to combat health challenges like HIV/AIDS, tuberculosis, malaria, cancer, and COVID. With the G20 and other partners, we created the Pandemic Fund to strengthen global pandemic preparedness, prevention, and response. At home, we invested over $7 billion to help State and local public health departments prepare for future crises, and we launched Public Health AmeriCorps to train a strong, diverse public health workforce for tomorrow.
These are vital steps needed to protect the American people. During National Public Health Week, we are reminded how interconnected everyone’s health and well-being are and that we are truly all in this together. By continuing to invest in public health, we can help ensure that the lessons of the last 4 years make our Nation stronger for the future.
NOW, THEREFORE, I, JOSEPH R. BIDEN JR., President of the United States of America, by virtue of the authority vested in me by the Constitution and the laws of the United States, do hereby proclaim April 1 through April 7, 2024, as National Public Health Week. I call on all citizens, government agencies, private businesses, nonprofit organizations, and other groups to take action to improve the health of our Nation.
IN WITNESS WHEREOF, I have hereunto set my hand this twenty-ninth day of March, in the year of our Lord two thousand twenty-four, and of the Independence of the United States of America the two hundred and forty-eighth.
JOSEPH R. BIDEN JR.
Stay Connected
We'll be in touch with the latest information on how President Biden and his administration are working for the American people, as well as ways you can get involved and help our country build back better.
Opt in to send and receive text messages from President Biden.
- UNC Chapel Hill
UNC Health Signs Long-Term Agreement with UnitedHealthcare
March 28, 2024
By Thomas Hughes
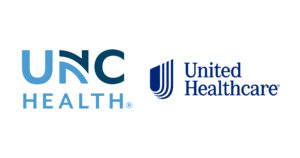
The organizations’ current contract was set to expire on April 1, raising the possibility of an “out of network” situation for thousands of patients. The new deal covers all United commercial, Individual Exchange and Medicare Advantage plans.
“This new agreement will benefit our patients across the state as well as our providers,” said Dr. Matt Ewend, Chief Clinical Officer at UNC Health. “Our patients will not face increased costs and stress of going out of network or seeking new providers for their care. We look forward to working with United in the coming years to improve access, reduce costs and eliminate obstacles to care.”
UNC Health will communicate with affected patients as quickly as possible to ensure they are aware of the new agreement with United and that care with their UNC Health providers will not change. UNC Health’s website about the negotiations, UNCHealthforNC.com , also will be updated for community members.
UNC Health began negotiations with United last year and agreed to a new deal this week. The new agreement with United follows UNC Health’s recent announcement of a long-term contract with Blue Cross and Blue Shield of North Carolina.
UNC Health’s mission is to improve the health and well-being of all North Carolinians. In order to continue to fulfill our mission, we will strive for fair and equitable partnerships with all insurance companies. UNC Health plays a critical role in serving North Carolinians across the state, particularly those in rural areas who would otherwise lack access to quality care close to home.
About UNC Health
UNC Health is a state entity and an affiliated enterprise of the University of North Carolina system, comprised of 15 hospitals , 19 hospital campuses and more than 900 clinics along with the clinical patient care programs of the UNC School of Medicine (SOM). It exists to improve the health and well-being of North Carolinians and others we serve and to further the teaching mission of the University of North Carolina SOM. UNC Health provided more than $800 million in Uncompensated Charity Care during the past five years. Our hospitals have received numerous awards and recognition for quality care, patient safety and the overall patient experience.
Filed Under:
More from Newsroom
- Four School of Medicine Students Named Schweitzer Fellows
- Newsworthy from the School of Medicine, Week of March 22 – March 28
- Temporary Age-Based Visitor Restrictions to be lifted on Monday, March 25 at UNC Hospitals, UNC Health Rex and UNC Health Chatham
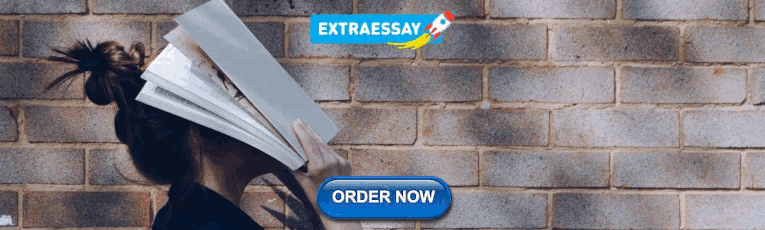
IMAGES
VIDEO
COMMENTS
What is a hypothesis and how can you write a great one for your research? A hypothesis is a tentative statement about the relationship between two or more variables that can be tested empirically. Find out how to formulate a clear, specific, and testable hypothesis with examples and tips from Verywell Mind, a trusted source of psychology and mental health information.
Developing a hypothesis (with example) Step 1. Ask a question. Writing a hypothesis begins with a research question that you want to answer. The question should be focused, specific, and researchable within the constraints of your project. Example: Research question.
Example: " There is a positive association between physical activity levels and overall health." 8. Causal hypothesis: A causal hypothesis proposes a cause-and-effect interaction between variables. Example: " Long-term alcohol use causes liver damage." Note that some of the types of research hypothesis mentioned above might overlap.
Step 5: Phrase your hypothesis in three ways. To identify the variables, you can write a simple prediction in if … then form. The first part of the sentence states the independent variable and the second part states the dependent variable. If a first-year student starts attending more lectures, then their exam scores will improve.
Example (continued) › But this is actually 2 hypotheses: › Hypothesis A: Intervention A will improve anxiety (as measured by the HADS anxiety subscale) in cancer patients with clinical levels of anxiety at 3 months compared to the control intervention. › Hypothesis B: Intervention A will improve depression (as measured by the HADS
Medical providers often rely on evidence-based medicine to guide decision-making in practice. Often a research hypothesis is tested with results provided, typically with p values, confidence intervals, or both. Additionally, statistical or research significance is estimated or determined by the investigators. Unfortunately, healthcare providers may have different comfort levels in interpreting ...
Another example for a directional one-tailed alternative hypothesis would be that. H1: Attending private classes before important exams has a positive effect on performance. Your null hypothesis would then be that. H0: Attending private classes before important exams has no/a negative effect on performance.
INTRODUCTION. Scientific research is usually initiated by posing evidenced-based research questions which are then explicitly restated as hypotheses.1,2 The hypotheses provide directions to guide the study, solutions, explanations, and expected results.3,4 Both research questions and hypotheses are essentially formulated based on conventional theories and real-world processes, which allow the ...
Simple hypothesis. A simple hypothesis is a statement made to reflect the relation between exactly two variables. One independent and one dependent. Consider the example, "Smoking is a prominent cause of lung cancer." The dependent variable, lung cancer, is dependent on the independent variable, smoking. 4.
Examples of Hypothesis. Here are a few examples of hypotheses in different fields: Psychology: "Increased exposure to violent video games leads to increased aggressive behavior in adolescents.". Biology: "Higher levels of carbon dioxide in the atmosphere will lead to increased plant growth.".
A research hypothesis (also called a scientific hypothesis) is a statement about the expected outcome of a study (for example, a dissertation or thesis). To constitute a quality hypothesis, the statement needs to have three attributes - specificity, clarity and testability. Let's take a look at these more closely.
15 Hypothesis Examples. A hypothesis is defined as a testable prediction, and is used primarily in scientific experiments as a potential or predicted outcome that scientists attempt to prove or disprove (Atkinson et al., 2021; Tan, 2022). In my types of hypothesis article, I outlined 13 different hypotheses, including the directional hypothesis ...
So, Nurse Jory's research purpose is "The purpose of this research study is to explore barriers to appointment attendance.". After the research problem and purpose statement comes the research hypothesis, by identifying the research variables. Research variables are the concepts that are measured, manipulated, or controlled in a study.
Present the findings in your results and discussion section. Though the specific details might vary, the procedure you will use when testing a hypothesis will always follow some version of these steps. Table of contents. Step 1: State your null and alternate hypothesis. Step 2: Collect data. Step 3: Perform a statistical test.
Examples. A research hypothesis, in its plural form "hypotheses," is a specific, testable prediction about the anticipated results of a study, established at its outset. It is a key component of the scientific method. Hypotheses connect theory to data and guide the research process towards expanding scientific understanding.
The alternative hypothesis (H a) is the other answer to your research question. It claims that there's an effect in the population. Often, your alternative hypothesis is the same as your research hypothesis. In other words, it's the claim that you expect or hope will be true. The alternative hypothesis is the complement to the null hypothesis.
In this second course of the Biostatistics in Public Health Specialization, you'll learn to evaluate sample variability and apply statistical hypothesis testing methods. Along the way, you'll perform calculations and interpret real-world data from the published scientific literature. Topics include sample statistics, the central limit theorem ...
The complexity of a hypothesis often varies, depending on its purpose. Here are seven example hypotheses you might use throughout your career: 1. Simple hypothesis. A simple hypothesis evaluates the basic relationship between an independent and a dependent variable.
To form a solid theory, the vital first step is creating a hypothesis. See the various types of hypotheses and how they can lead you on the path to discovery.
Hypothesis Testing Examples. We know it can be hard to write a good hypothesis unless you've seen some good hypothesis examples. We've included four hypothesis examples based on some made-up experiments. Use these as templates or launch pads for coming up with your own hypotheses. Experiment #1: Students Studying Outside (Writing a Hypothesis)
In contrast to methodological hypotheses explaining the mental/physical health 'paradox', a recently advanced alternative hypothesis is that the patterning in physical and mental health outcomes in Blacks versus Whites arises from mechanisms for coping with stressors that on average operate differently for Black and White Americans (Jackson ...
Child and Adolescent Health. The hygiene hypothesis is the idea that kids need to be exposed to germs in order to develop healthy immune systems. We know that many common viruses did not circulate as widely during the pandemic, thanks to social distancing, masking, and other COVID mitigation measures. Are there downsides to those missed infections?
2024. During National Public Health Week, we celebrate the life-saving care of public health professionals who work tirelessly to promote our country's health, safety, and well-being. We ...
hypothesis. noun. Definition of hypothesis. Synonyms for hypothesis. The results of the experiment did not support his hypothesis. Their hypothesis is that watching excessive amounts of television reduces a person's ability to concentrate. The coming days and weeks will put that hypothesis to the test. —.
CHAPEL HILL, N.C. - UNC Health has signed a new, long-term agreement with UnitedHealthcare. The multi-year deal will allow United members across North Carolina to continue receiving uninterrupted care from UNC Health providers, clinics and hospitals. The organizations' current contract was set to expire on April 1, raising the possibility ...