- En español – ExME
- Em português – EME
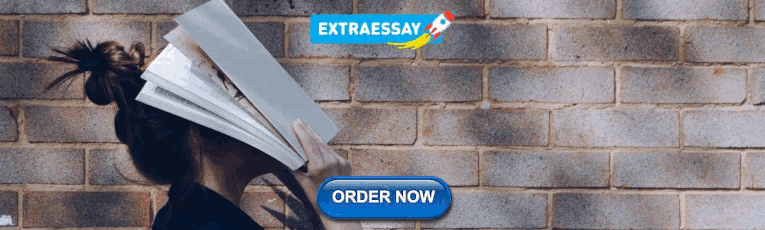
Multivariate analysis: an overview
Posted on 9th September 2021 by Vighnesh D
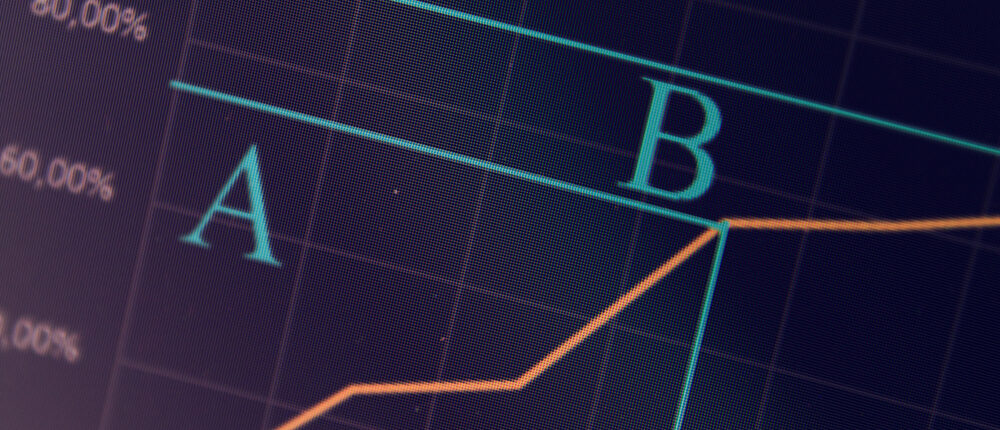
Data analysis is one of the most useful tools when one tries to understand the vast amount of information presented to them and synthesise evidence from it. There are usually multiple factors influencing a phenomenon.
Of these, some can be observed, documented and interpreted thoroughly while others cannot. For example, in order to estimate the burden of a disease in society there may be a lot of factors which can be readily recorded, and a whole lot of others which are unreliable and, therefore, require proper scrutiny. Factors like incidence, age distribution, sex distribution and financial loss owing to the disease can be accounted for more easily when compared to contact tracing, prevalence and institutional support for the same. Therefore, it is of paramount importance that the data which is collected and interpreted must be done thoroughly in order to avoid common pitfalls.
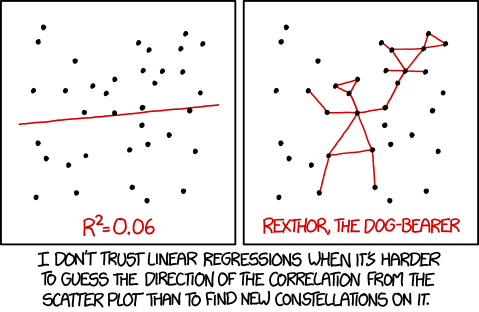
Image from: https://imgs.xkcd.com/comics/useful_geometry_formulas.png under Creative Commons License 2.5 Randall Munroe. xkcd.com.
Why does it sound so important?
Data collection and analysis is emphasised upon in academia because the very same findings determine the policy of a governing body and, therefore, the implications that follow it are the direct product of the information that is fed into the system.
Introduction
In this blog, we will discuss types of data analysis in general and multivariate analysis in particular. It aims to introduce the concept to investigators inclined towards this discipline by attempting to reduce the complexity around the subject.
Analysis of data based on the types of variables in consideration is broadly divided into three categories:
- Univariate analysis: The simplest of all data analysis models, univariate analysis considers only one variable in calculation. Thus, although it is quite simple in application, it has limited use in analysing big data. E.g. incidence of a disease.
- Bivariate analysis: As the name suggests, bivariate analysis takes two variables into consideration. It has a slightly expanded area of application but is nevertheless limited when it comes to large sets of data. E.g. incidence of a disease and the season of the year.
- Multivariate analysis: Multivariate analysis takes a whole host of variables into consideration. This makes it a complicated as well as essential tool. The greatest virtue of such a model is that it considers as many factors into consideration as possible. This results in tremendous reduction of bias and gives a result closest to reality. For example, kindly refer to the factors discussed in the “overview” section of this article.
Multivariate analysis is defined as:
The statistical study of data where multiple measurements are made on each experimental unit and where the relationships among multivariate measurements and their structure are important
Multivariate statistical methods incorporate several techniques depending on the situation and the question in focus. Some of these methods are listed below:
- Regression analysis: Used to determine the relationship between a dependent variable and one or more independent variable.
- Analysis of Variance (ANOVA) : Used to determine the relationship between collections of data by analyzing the difference in the means.
- Interdependent analysis: Used to determine the relationship between a set of variables among themselves.
- Discriminant analysis: Used to classify observations in two or more distinct set of categories.
- Classification and cluster analysis: Used to find similarity in a group of observations.
- Principal component analysis: Used to interpret data in its simplest form by introducing new uncorrelated variables.
- Factor analysis: Similar to principal component analysis, this too is used to crunch big data into small, interpretable forms.
- Canonical correlation analysis: Perhaps one of the most complex models among all of the above, canonical correlation attempts to interpret data by analysing relationships between cross-covariance matrices.
ANOVA remains one of the most widely used statistical models in academia. Of the several types of ANOVA models, there is one subtype that is frequently used because of the factors involved in the studies. Traditionally, it has found its application in behavioural research, i.e. Psychology, Psychiatry and allied disciplines. This model is called the Multivariate Analysis of Variance (MANOVA). It is widely described as the multivariate analogue of ANOVA, used in interpreting univariate data.
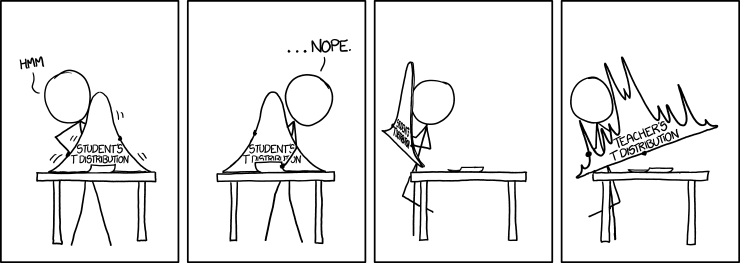
Image from: https://imgs.xkcd.com/comics/t_distribution.png under Creative Commons License 2.5 Randall Munroe. xkcd.com.
Interpretation of results
Interpretation of results is probably the most difficult part in the technique. The relevant results are generally summarized in a table with an associated text. Appropriate information must be highlighted regarding:
- Multivariate test statistics used
- Degrees of freedom
- Appropriate test statistics used
- Calculated p-value (p < x)
Reliability and validity of the test are the most important determining factors in such techniques.
Applications
Multivariate analysis is used in several disciplines. One of its most distinguishing features is that it can be used in parametric as well as non-parametric tests.
Quick question: What are parametric and non-parametric tests?
- Parametric tests: Tests which make certain assumptions regarding the distribution of data, i.e. within a fixed parameter.
- Non-parametric tests: Tests which do not make assumptions with respect to distribution. On the contrary, the distribution of data is assumed to be free of distribution.
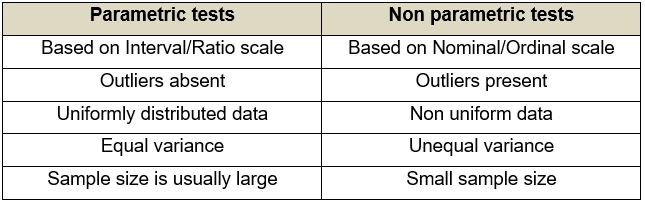
Uses of Multivariate analysis: Multivariate analyses are used principally for four reasons, i.e. to see patterns of data, to make clear comparisons, to discard unwanted information and to study multiple factors at once. Applications of multivariate analysis are found in almost all the disciplines which make up the bulk of policy-making, e.g. economics, healthcare, pharmaceutical industries, applied sciences, sociology, and so on. Multivariate analysis has particularly enjoyed a traditional stronghold in the field of behavioural sciences like psychology, psychiatry and allied fields because of the complex nature of the discipline.
Multivariate analysis is one of the most useful methods to determine relationships and analyse patterns among large sets of data. It is particularly effective in minimizing bias if a structured study design is employed. However, the complexity of the technique makes it a less sought-out model for novice research enthusiasts. Therefore, although the process of designing the study and interpretation of results is a tedious one, the techniques stand out in finding the relationships in complex situations.
References (pdf)
Leave a Reply Cancel reply
Your email address will not be published. Required fields are marked *
Save my name, email, and website in this browser for the next time I comment.
No Comments on Multivariate analysis: an overview
I got good information on multivariate data analysis and using mult variat analysis advantages and patterns.
Great summary. I found this very useful for starters
Thank you so much for the dscussion on multivariate design in research. However, i want to know more about multiple regression analysis. Hope for more learnings to gain from you.
Thank you for letting the author know this was useful, and I will see if there are any students wanting to blog about multiple regression analysis next!
When you want to know what contributed to an outcome what study is done?
Dear Philip, Thank you for bringing this to our notice. Your input regarding the discussion is highly appreciated. However, since this particular blog was meant to be an overview, I consciously avoided the nuances to prevent complicated explanations at an early stage. I am planning to expand on the matter in subsequent blogs and will keep your suggestion in mind while drafting for the same. Many thanks, Vighnesh.
Sorry, I don’t want to be pedantic, but shouldn’t we differentiate between ‘multivariate’ and ‘multivariable’ regression? https://stats.stackexchange.com/questions/447455/multivariable-vs-multivariate-regression https://www.ajgponline.org/article/S1064-7481(18)30579-7/fulltext
Subscribe to our newsletter
You will receive our monthly newsletter and free access to Trip Premium.
Related Articles
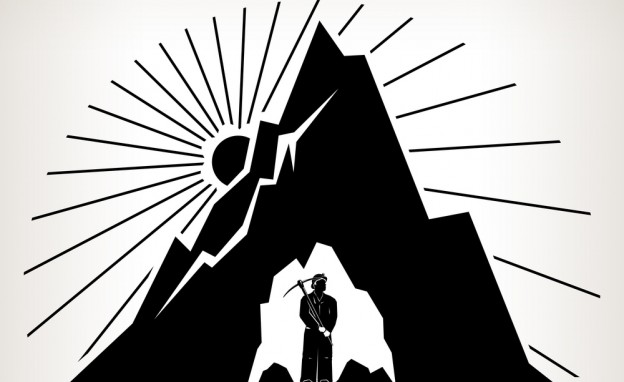
Data mining or data dredging?
Advances in technology now allow huge amounts of data to be handled simultaneously. Katherine takes a look at how this can be used in healthcare and how it can be exploited.
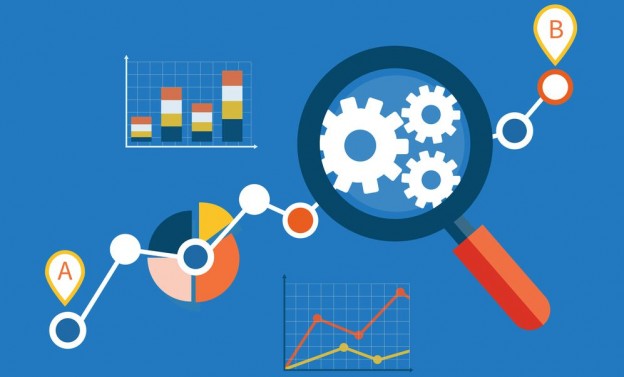
Nominal, ordinal, or numerical variables?
How can you tell if a variable is nominal, ordinal, or numerical? Why does it even matter? Determining the appropriate variable type used in a study is essential to determining the correct statistical method to use when obtaining your results. It is important not to take the variables out of context because more often than not, the same variable that can be ordinal can also be numerical, depending on how the data was recorded and analyzed. This post will give you a specific example that may help you better grasp this concept.
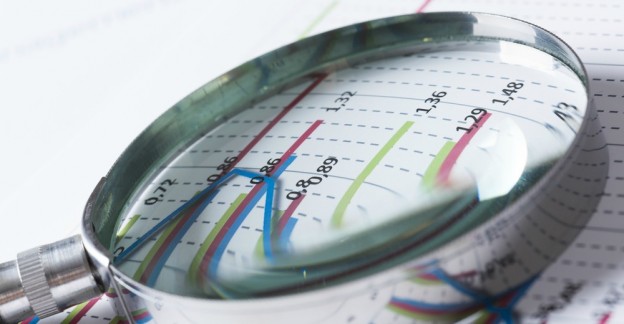
Data analysis methods
A description of the two types of data analysis – “As Treated” and “Intention to Treat” – using a hypothetical trial as an example
A Guide on Data Analysis
22 multivariate methods.
\(y_1,...,y_p\) are possibly correlated random variables with means \(\mu_1,...,\mu_p\)
\[ \mathbf{y} = \left( \begin{array} {c} y_1 \\ . \\ y_p \\ \end{array} \right) \]
\[ E(\mathbf{y}) = \left( \begin{array} {c} \mu_1 \\ . \\ \mu_p \\ \end{array} \right) \]
Let \(\sigma_{ij} = cov(y_i, y_j)\) for \(i,j = 1,…,p\)
\[ \mathbf{\Sigma} = (\sigma_{ij}) = \left( \begin{array} {cccc} \sigma_{11} & \sigma_{22} & ... & \sigma_{1p} \\ \sigma_{21} & \sigma_{22} & ... & \sigma_{2p} \\ . & . & . & . \\ \sigma_{p1} & \sigma_{p2} & ... & \sigma_{pp} \end{array} \right) \]
where \(\mathbf{\Sigma}\) (symmetric) is the variance-covariance or dispersion matrix
Let \(\mathbf{u}_{p \times 1}\) and \(\mathbf{v}_{q \times 1}\) be random vectors with means \(\mu_u\) and \(\mu_v\) . Then
\[ \mathbf{\Sigma}_{uv} = cov(\mathbf{u,v}) = E[(\mathbf{u} - \mu_u)(\mathbf{v} - \mu_v)'] \]
in which \(\mathbf{\Sigma}_{uv} \neq \mathbf{\Sigma}_{vu}\) and \(\mathbf{\Sigma}_{uv} = \mathbf{\Sigma}_{vu}'\)
Properties of Covariance Matrices
- Symmetric \(\mathbf{\Sigma}' = \mathbf{\Sigma}\)
- Non-negative definite \(\mathbf{a'\Sigma a} \ge 0\) for any \(\mathbf{a} \in R^p\) , which is equivalent to eigenvalues of \(\mathbf{\Sigma}\) , \(\lambda_1 \ge \lambda_2 \ge ... \ge \lambda_p \ge 0\)
- \(|\mathbf{\Sigma}| = \lambda_1 \lambda_2 ... \lambda_p \ge 0\) ( generalized variance ) (the bigger this number is, the more variation there is
- \(trace(\mathbf{\Sigma}) = tr(\mathbf{\Sigma}) = \lambda_1 + ... + \lambda_p = \sigma_{11} + ... + \sigma_{pp} =\) sum of variance ( total variance )
- \(\mathbf{\Sigma}\) is typically required to be positive definite, which means all eigenvalues are positive, and \(\mathbf{\Sigma}\) has an inverse \(\mathbf{\Sigma}^{-1}\) such that \(\mathbf{\Sigma}^{-1}\mathbf{\Sigma} = \mathbf{I}_{p \times p} = \mathbf{\Sigma \Sigma}^{-1}\)
Correlation Matrices
\[ \rho_{ij} = \frac{\sigma_{ij}}{\sqrt{\sigma_{ii} \sigma_{jj}}} \]
\[ \mathbf{R} = \left( \begin{array} {cccc} \rho_{11} & \rho_{12} & ... & \rho_{1p} \\ \rho_{21} & \rho_{22} & ... & \rho_{2p} \\ . & . & . &. \\ \rho_{p1} & \rho_{p2} & ... & \rho_{pp} \\ \end{array} \right) \]
where \(\rho_{ij}\) is the correlation, and \(\rho_{ii} = 1\) for all i
Alternatively,
\[ \mathbf{R} = [diag(\mathbf{\Sigma})]^{-1/2}\mathbf{\Sigma}[diag(\mathbf{\Sigma})]^{-1/2} \]
where \(diag(\mathbf{\Sigma})\) is the matrix which has the \(\sigma_{ii}\) ’s on the diagonal and 0’s elsewhere
and \(\mathbf{A}^{1/2}\) (the square root of a symmetric matrix) is a symmetric matrix such as \(\mathbf{A} = \mathbf{A}^{1/2}\mathbf{A}^{1/2}\)
\(\mathbf{x}\) and \(\mathbf{y}\) be random vectors with means \(\mu_x\) and \(\mu_y\) and variance -variance matrices \(\mathbf{\Sigma}_x\) and \(\mathbf{\Sigma}_y\) .
\(\mathbf{A}\) and \(\mathbf{B}\) be matrices of constants and \(\mathbf{c}\) and \(\mathbf{d}\) be vectors of constants
\(E(\mathbf{Ay + c} ) = \mathbf{A} \mu_y + c\)
\(var(\mathbf{Ay + c}) = \mathbf{A} var(\mathbf{y})\mathbf{A}' = \mathbf{A \Sigma_y A}'\)
\(cov(\mathbf{Ay + c, By+ d}) = \mathbf{A\Sigma_y B}'\)
\(E(\mathbf{Ay + Bx + c}) = \mathbf{A \mu_y + B \mu_x + c}\)
\(var(\mathbf{Ay + Bx + c}) = \mathbf{A \Sigma_y A' + B \Sigma_x B' + A \Sigma_{yx}B' + B\Sigma'_{yx}A'}\)
Multivariate Normal Distribution
Let \(\mathbf{y}\) be a multivariate normal (MVN) random variable with mean \(\mu\) and variance \(\mathbf{\Sigma}\) . Then the density of \(\mathbf{y}\) is
\[ f(\mathbf{y}) = \frac{1}{(2\pi)^{p/2}|\mathbf{\Sigma}|^{1/2}} \exp(-\frac{1}{2} \mathbf{(y-\mu)'\Sigma^{-1}(y-\mu)} ) \]
\(\mathbf{y} \sim N_p(\mu, \mathbf{\Sigma})\)
22.0.1 Properties of MVN
Let \(\mathbf{A}_{r \times p}\) be a fixed matrix. Then \(\mathbf{Ay} \sim N_r (\mathbf{A \mu, A \Sigma A'})\) . \(r \le p\) and all rows of \(\mathbf{A}\) must be linearly independent to guarantee that \(\mathbf{A \Sigma A}'\) is non-singular.
Let \(\mathbf{G}\) be a matrix such that \(\mathbf{\Sigma}^{-1} = \mathbf{GG}'\) . Then \(\mathbf{G'y} \sim N_p(\mathbf{G' \mu, I})\) and \(\mathbf{G'(y-\mu)} \sim N_p (0,\mathbf{I})\)
Any fixed linear combination of \(y_1,...,y_p\) (say \(\mathbf{c'y}\) ) follows \(\mathbf{c'y} \sim N_1 (\mathbf{c' \mu, c' \Sigma c})\)
Define a partition, \([\mathbf{y}'_1,\mathbf{y}_2']'\) where
\(\mathbf{y}_1\) is \(p_1 \times 1\)
\(\mathbf{y}_2\) is \(p_2 \times 1\) ,
\(p_1 + p_2 = p\)
\(p_1,p_2 \ge 1\) Then
\[ \left( \begin{array} {c} \mathbf{y}_1 \\ \mathbf{y}_2 \\ \end{array} \right) \sim N \left( \left( \begin{array} {c} \mu_1 \\ \mu_2 \\ \end{array} \right), \left( \begin{array} {cc} \mathbf{\Sigma}_{11} & \mathbf{\Sigma}_{12} \\ \mathbf{\Sigma}_{21} & \mathbf{\Sigma}_{22}\\ \end{array} \right) \right) \]
The marginal distributions of \(\mathbf{y}_1\) and \(\mathbf{y}_2\) are \(\mathbf{y}_1 \sim N_{p1}(\mathbf{\mu_1, \Sigma_{11}})\) and \(\mathbf{y}_2 \sim N_{p2}(\mathbf{\mu_2, \Sigma_{22}})\)
Individual components \(y_1,...,y_p\) are all normally distributed \(y_i \sim N_1(\mu_i, \sigma_{ii})\)
The conditional distribution of \(\mathbf{y}_1\) and \(\mathbf{y}_2\) is normal
\(\mathbf{y}_1 | \mathbf{y}_2 \sim N_{p1}(\mathbf{\mu_1 + \Sigma_{12} \Sigma_{22}^{-1}(y_2 - \mu_2),\Sigma_{11} - \Sigma_{12} \Sigma_{22}^{-1} \sigma_{21}})\)
- In this formula, we see if we know (have info about) \(\mathbf{y}_2\) , we can re-weight \(\mathbf{y}_1\) ’s mean, and the variance is reduced because we know more about \(\mathbf{y}_1\) because we know \(\mathbf{y}_2\)
which is analogous to \(\mathbf{y}_2 | \mathbf{y}_1\) . And \(\mathbf{y}_1\) and \(\mathbf{y}_2\) are independently distrusted only if \(\mathbf{\Sigma}_{12} = 0\)
If \(\mathbf{y} \sim N(\mathbf{\mu, \Sigma})\) and \(\mathbf{\Sigma}\) is positive definite, then \(\mathbf{(y-\mu)' \Sigma^{-1} (y - \mu)} \sim \chi^2_{(p)}\)
If \(\mathbf{y}_i\) are independent \(N_p (\mathbf{\mu}_i , \mathbf{\Sigma}_i)\) random variables, then for fixed matrices \(\mathbf{A}_{i(m \times p)}\) , \(\sum_{i=1}^k \mathbf{A}_i \mathbf{y}_i \sim N_m (\sum_{i=1}^{k} \mathbf{A}_i \mathbf{\mu}_i, \sum_{i=1}^k \mathbf{A}_i \mathbf{\Sigma}_i \mathbf{A}_i)\)
Multiple Regression
\[ \left( \begin{array} {c} Y \\ \mathbf{x} \end{array} \right) \sim N_{p+1} \left( \left[ \begin{array} {c} \mu_y \\ \mathbf{\mu}_x \end{array} \right] , \left[ \begin{array} {cc} \sigma^2_Y & \mathbf{\Sigma}_{yx} \\ \mathbf{\Sigma}_{yx} & \mathbf{\Sigma}_{xx} \end{array} \right] \right) \]
The conditional distribution of Y given x follows a univariate normal distribution with
\[ \begin{aligned} E(Y| \mathbf{x}) &= \mu_y + \mathbf{\Sigma}_{yx} \Sigma_{xx}^{-1} (\mathbf{x}- \mu_x) \\ &= \mu_y - \Sigma_{yx} \Sigma_{xx}^{-1}\mu_x + \Sigma_{yx} \Sigma_{xx}^{-1}\mathbf{x} \\ &= \beta_0 + \mathbf{\beta'x} \end{aligned} \]
where \(\beta = (\beta_1,...,\beta_p)' = \mathbf{\Sigma}_{xx}^{-1} \mathbf{\Sigma}_{yx}'\) (e.g., analogous to \(\mathbf{(x'x)^{-1}x'y}\) but not the same if we consider \(Y_i\) and \(\mathbf{x}_i\) , \(i = 1,..,n\) and use the empirical covariance formula: \(var(Y|\mathbf{x}) = \sigma^2_Y - \mathbf{\Sigma_{yx}\Sigma^{-1}_{xx} \Sigma'_{yx}}\) )
Samples from Multivariate Normal Populations
A random sample of size n, \(\mathbf{y}_1,.., \mathbf{y}_n\) from \(N_p (\mathbf{\mu}, \mathbf{\Sigma})\) . Then
Since \(\mathbf{y}_1,..., \mathbf{y}_n\) are iid, their sample mean, \(\bar{\mathbf{y}} = \sum_{i=1}^n \mathbf{y}_i/n \sim N_p (\mathbf{\mu}, \mathbf{\Sigma}/n)\) . that is, \(\bar{\mathbf{y}}\) is an unbiased estimator of \(\mathbf{\mu}\)
The \(p \times p\) sample variance-covariance matrix, \(\mathbf{S}\) is \(\mathbf{S} = \frac{1}{n-1}\sum_{i=1}^n (\mathbf{y}_i - \bar{\mathbf{y}})(\mathbf{y}_i - \bar{\mathbf{y}})' = \frac{1}{n-1} (\sum_{i=1}^n \mathbf{y}_i \mathbf{y}_i' - n \bar{\mathbf{y}}\bar{\mathbf{y}}')\)
- where \(\mathbf{S}\) is symmetric, unbiased estimator of \(\mathbf{\Sigma}\) and has \(p(p+1)/2\) random variables.
\((n-1)\mathbf{S} \sim W_p (n-1, \mathbf{\Sigma})\) is a Wishart distribution with n-1 degrees of freedom and expectation \((n-1) \mathbf{\Sigma}\) . The Wishart distribution is a multivariate extension of the Chi-squared distribution.
\(\bar{\mathbf{y}}\) and \(\mathbf{S}\) are independent
\(\bar{\mathbf{y}}\) and \(\mathbf{S}\) are sufficient statistics. (All of the info in the data about \(\mathbf{\mu}\) and \(\mathbf{\Sigma}\) is contained in \(\bar{\mathbf{y}}\) and \(\mathbf{S}\) , regardless of sample size).
Large Sample Properties
\(\mathbf{y}_1,..., \mathbf{y}_n\) are a random sample from some population with mean \(\mathbf{\mu}\) and variance-covariance matrix \(\mathbf{\Sigma}\)
\(\bar{\mathbf{y}}\) is a consistent estimator for \(\mu\)
\(\mathbf{S}\) is a consistent estimator for \(\mathbf{\Sigma}\)
Multivariate Central Limit Theorem : Similar to the univariate case, \(\sqrt{n}(\bar{\mathbf{y}} - \mu) \dot{\sim} N_p (\mathbf{0,\Sigma})\) where n is large relative to p ( \(n \ge 25p\) ), which is equivalent to \(\bar{\mathbf{y}} \dot{\sim} N_p (\mu, \mathbf{\Sigma}/n)\)
Wald’s Theorem : \(n(\bar{\mathbf{y}} - \mu)' \mathbf{S}^{-1} (\bar{\mathbf{y}} - \mu)\) when n is large relative to p.
Maximum Likelihood Estimation for MVN
Suppose iid \(\mathbf{y}_1 ,... \mathbf{y}_n \sim N_p (\mu, \mathbf{\Sigma})\) , the likelihood function for the data is
\[ \begin{aligned} L(\mu, \mathbf{\Sigma}) &= \prod_{j=1}^n (\frac{1}{(2\pi)^{p/2}|\mathbf{\Sigma}|^{1/2}} \exp(-\frac{1}{2}(\mathbf{y}_j -\mu)'\mathbf{\Sigma}^{-1})(\mathbf{y}_j -\mu)) \\ &= \frac{1}{(2\pi)^{np/2}|\mathbf{\Sigma}|^{n/2}} \exp(-\frac{1}{2} \sum_{j=1}^n(\mathbf{y}_j -\mu)'\mathbf{\Sigma}^{-1})(\mathbf{y}_j -\mu) \end{aligned} \]
Then, the MLEs are
\[ \hat{\mu} = \bar{\mathbf{y}} \]
\[ \hat{\mathbf{\Sigma}} = \frac{n-1}{n} \mathbf{S} \]
using derivatives of the log of the likelihood function with respect to \(\mu\) and \(\mathbf{\Sigma}\)
Properties of MLEs
Invariance: If \(\hat{\theta}\) is the MLE of \(\theta\) , then the MLE of \(h(\theta)\) is \(h(\hat{\theta})\) for any function h(.)
Consistency: MLEs are consistent estimators, but they are usually biased
Efficiency: MLEs are efficient estimators (no other estimator has a smaller variance for large samples)
Asymptotic normality: Suppose that \(\hat{\theta}_n\) is the MLE for \(\theta\) based upon n independent observations. Then \(\hat{\theta}_n \dot{\sim} N(\theta, \mathbf{H}^{-1})\)
\(\mathbf{H}\) is the Fisher Information Matrix, which contains the expected values of the second partial derivatives fo the log-likelihood function. the (i,j)th element of \(\mathbf{H}\) is \(-E(\frac{\partial^2 l(\mathbf{\theta})}{\partial \theta_i \partial \theta_j})\)
we can estimate \(\mathbf{H}\) by finding the form determined above, and evaluate it at \(\theta = \hat{\theta}_n\)
Likelihood ratio testing: for some null hypothesis, \(H_0\) we can form a likelihood ratio test
The statistic is: \(\Lambda = \frac{\max_{H_0}l(\mathbf{\mu}, \mathbf{\Sigma|Y})}{\max l(\mu, \mathbf{\Sigma | Y})}\)
For large n, \(-2 \log \Lambda \sim \chi^2_{(v)}\) where v is the number of parameters in the unrestricted space minus the number of parameters under \(H_0\)
Test of Multivariate Normality
Check univariate normality for each trait (X) separately
Can check \[Normality Assessment\]
The good thing is that if any of the univariate trait is not normal, then the joint distribution is not normal (see again [m]). If a joint multivariate distribution is normal, then the marginal distribution has to be normal.
However, marginal normality of all traits does not imply joint MVN
Easily rule out multivariate normality, but not easy to prove it
Mardia’s tests for multivariate normality
Multivariate skewness is \[ \beta_{1,p} = E[(\mathbf{y}- \mathbf{\mu})' \mathbf{\Sigma}^{-1} (\mathbf{x} - \mathbf{\mu})]^3 \]
where \(\mathbf{x}\) and \(\mathbf{y}\) are independent, but have the same distribution (note: \(\beta\) here is not regression coefficient)
Multivariate kurtosis is defined as
\[ \beta_{2,p} - E[(\mathbf{y}- \mathbf{\mu})' \mathbf{\Sigma}^{-1} (\mathbf{x} - \mathbf{\mu})]^2 \]
For the MVN distribution, we have \(\beta_{1,p} = 0\) and \(\beta_{2,p} = p(p+2)\)
For a sample of size n, we can estimate
\[ \hat{\beta}_{1,p} = \frac{1}{n^2}\sum_{i=1}^n \sum_{j=1}^n g^2_{ij} \]
\[ \hat{\beta}_{2,p} = \frac{1}{n} \sum_{i=1}^n g^2_{ii} \]
- where \(g_{ij} = (\mathbf{y}_i - \bar{\mathbf{y}})' \mathbf{S}^{-1} (\mathbf{y}_j - \bar{\mathbf{y}})\) . Note: \(g_{ii} = d^2_i\) where \(d^2_i\) is the Mahalanobis distance
( Mardia 1970 ) shows for large n
\[ \kappa_1 = \frac{n \hat{\beta}_{1,p}}{6} \dot{\sim} \chi^2_{p(p+1)(p+2)/6} \]
\[ \kappa_2 = \frac{\hat{\beta}_{2,p} - p(p+2)}{\sqrt{8p(p+2)/n}} \sim N(0,1) \]
Hence, we can use \(\kappa_1\) and \(\kappa_2\) to test the null hypothesis of MVN.
When the data are non-normal, normal theory tests on the mean are sensitive to \(\beta_{1,p}\) , while tests on the covariance are sensitive to \(\beta_{2,p}\)
Alternatively, Doornik-Hansen test for multivariate normality ( Doornik and Hansen 2008 )
Chi-square Q-Q plot
Let \(\mathbf{y}_i, i = 1,...,n\) be a random sample sample from \(N_p(\mathbf{\mu}, \mathbf{\Sigma})\)
Then \(\mathbf{z}_i = \mathbf{\Sigma}^{-1/2}(\mathbf{y}_i - \mathbf{\mu}), i = 1,...,n\) are iid \(N_p (\mathbf{0}, \mathbf{I})\) . Thus, \(d_i^2 = \mathbf{z}_i' \mathbf{z}_i \sim \chi^2_p , i = 1,...,n\)
plot the ordered \(d_i^2\) values against the qualities of the \(\chi^2_p\) distribution. When normality holds, the plot should approximately resemble a straight lien passing through the origin at a 45 degree
it requires large sample size (i.e., sensitive to sample size). Even if we generate data from a MVN, the tail of the Chi-square Q-Q plot can still be out of line.
If the data are not normal, we can
use nonparametric methods
use models based upon an approximate distribution (e.g., GLMM)
try performing a transformation

22.0.2 Mean Vector Inference
In the univariate normal distribution, we test \(H_0: \mu =\mu_0\) by using
\[ T = \frac{\bar{y}- \mu_0}{s/\sqrt{n}} \sim t_{n-1} \]
under the null hypothesis. And reject the null if \(|T|\) is large relative to \(t_{(1-\alpha/2,n-1)}\) because it means that seeing a value as large as what we observed is rare if the null is true
Equivalently,
\[ T^2 = \frac{(\bar{y}- \mu_0)^2}{s^2/n} = n(\bar{y}- \mu_0)(s^2)^{-1}(\bar{y}- \mu_0) \sim f_{(1,n-1)} \]
22.0.2.1 Natural Multivariate Generalization
\[ \begin{aligned} &H_0: \mathbf{\mu} = \mathbf{\mu}_0 \\ &H_a: \mathbf{\mu} \neq \mathbf{\mu}_0 \end{aligned} \]
Define Hotelling’s \(T^2\) by
\[ T^2 = n(\bar{\mathbf{y}} - \mathbf{\mu}_0)'\mathbf{S}^{-1}(\bar{\mathbf{y}} - \mathbf{\mu}_0) \]
which can be viewed as a generalized distance between \(\bar{\mathbf{y}}\) and \(\mathbf{\mu}_0\)
Under the assumption of normality,
\[ F = \frac{n-p}{(n-1)p} T^2 \sim f_{(p,n-p)} \]
and reject the null hypothesis when \(F > f_{(1-\alpha, p, n-p)}\)
The \(T^2\) test is invariant to changes in measurement units.
- If \(\mathbf{z = Cy + d}\) where \(\mathbf{C}\) and \(\mathbf{d}\) do not depend on \(\mathbf{y}\) , then \(T^2(\mathbf{z}) - T^2(\mathbf{y})\)
The \(T^2\) test can be derived as a likelihood ratio test of \(H_0: \mu = \mu_0\)
22.0.2.2 Confidence Intervals
22.0.2.2.1 confidence region.
An “exact” \(100(1-\alpha)\%\) confidence region for \(\mathbf{\mu}\) is the set of all vectors, \(\mathbf{v}\) , which are “close enough” to the observed mean vector, \(\bar{\mathbf{y}}\) to satisfy
\[ n(\bar{\mathbf{y}} - \mathbf{\mu}_0)'\mathbf{S}^{-1}(\bar{\mathbf{y}} - \mathbf{\mu}_0) \le \frac{(n-1)p}{n-p} f_{(1-\alpha, p, n-p)} \]
- \(\mathbf{v}\) are just the mean vectors that are not rejected by the \(T^2\) test when \(\mathbf{\bar{y}}\) is observed.
In case that you have 2 parameters, the confidence region is a “hyper-ellipsoid”.
In this region, it consists of all \(\mathbf{\mu}_0\) vectors for which the \(T^2\) test would not reject \(H_0\) at significance level \(\alpha\)
Even though the confidence region better assesses the joint knowledge concerning plausible values of \(\mathbf{\mu}\) , people typically include confidence statement about the individual component means. We’d like all of the separate confidence statements to hold simultaneously with a specified high probability. Simultaneous confidence intervals: intervals against any statement being incorrect
22.0.2.2.1.1 Simultaneous Confidence Statements
- Intervals based on a rectangular confidence region by projecting the previous region onto the coordinate axes:
\[ \bar{y}_{i} \pm \sqrt{\frac{(n-1)p}{n-p}f_{(1-\alpha, p,n-p)}\frac{s_{ii}}{n}} \]
for all \(i = 1,..,p\)
which implied confidence region is conservative; it has at least \(100(1- \alpha)\%\)
Generally, simultaneous \(100(1-\alpha) \%\) confidence intervals for all linear combinations , \(\mathbf{a}\) of the elements of the mean vector are given by
\[ \mathbf{a'\bar{y}} \pm \sqrt{\frac{(n-1)p}{n-p}f_{(1-\alpha, p,n-p)}\frac{\mathbf{a'Sa}}{n}} \]
works for any arbitrary linear combination \(\mathbf{a'\mu} = a_1 \mu_1 + ... + a_p \mu_p\) , which is a projection onto the axis in the direction of \(\mathbf{a}\)
These intervals have the property that the probability that at least one such interval does not contain the appropriate \(\mathbf{a' \mu}\) is no more than \(\alpha\)
These types of intervals can be used for “data snooping” (like \[Scheffe\] )
22.0.2.2.1.2 One \(\mu\) at a time
- One at a time confidence intervals:
\[ \bar{y}_i \pm t_{(1 - \alpha/2, n-1} \sqrt{\frac{s_{ii}}{n}} \]
Each of these intervals has a probability of \(1-\alpha\) of covering the appropriate \(\mu_i\)
But they ignore the covariance structure of the \(p\) variables
If we only care about \(k\) simultaneous intervals, we can use “one at a time” method with the \[Bonferroni\] correction.
This method gets more conservative as the number of intervals \(k\) increases.
22.0.3 General Hypothesis Testing
22.0.3.1 one-sample tests.
\[ H_0: \mathbf{C \mu= 0} \]
- \(\mathbf{C}\) is a \(c \times p\) matrix of rank c where \(c \le p\)
We can test this hypothesis using the following statistic
\[ F = \frac{n - c}{(n-1)c} T^2 \]
where \(T^2 = n(\mathbf{C\bar{y}})' (\mathbf{CSC'})^{-1} (\mathbf{C\bar{y}})\)
\[ H_0: \mu_1 = \mu_2 = ... = \mu_p \]
\[ \begin{aligned} \mu_1 - \mu_2 &= 0 \\ &\vdots \\ \mu_{p-1} - \mu_p &= 0 \end{aligned} \]
a total of \(p-1\) tests. Hence, we have \(\mathbf{C}\) as the \(p - 1 \times p\) matrix
\[ \mathbf{C} = \left( \begin{array} {ccccc} 1 & -1 & 0 & \ldots & 0 \\ 0 & 1 & -1 & \ldots & 0 \\ \vdots & \vdots & \vdots & \ddots & \vdots \\ 0 & 0 & \ldots & 1 & -1 \end{array} \right) \]
number of rows = \(c = p -1\)
Equivalently, we can also compare all of the other means to the first mean. Then, we test \(\mu_1 - \mu_2 = 0, \mu_1 - \mu_3 = 0,..., \mu_1 - \mu_p = 0\) , the \((p-1) \times p\) matrix \(\mathbf{C}\) is
\[ \mathbf{C} = \left( \begin{array} {ccccc} -1 & 1 & 0 & \ldots & 0 \\ -1 & 0 & 1 & \ldots & 0 \\ \vdots & \vdots & \vdots & \ddots & \vdots \\ -1 & 0 & \ldots & 0 & 1 \end{array} \right) \]
The value of \(T^2\) is invariant to these equivalent choices of \(\mathbf{C}\)
This is often used for repeated measures designs , where each subject receives each treatment once over successive periods of time (all treatments are administered to each unit).
Let \(y_{ij}\) be the response from subject i at time j for \(i = 1,..,n, j = 1,...,T\) . In this case, \(\mathbf{y}_i = (y_{i1}, ..., y_{iT})', i = 1,...,n\) are a random sample from \(N_T (\mathbf{\mu}, \mathbf{\Sigma})\)
Let \(n=8\) subjects, \(T = 6\) . We are interested in \(\mu_1, .., \mu_6\)
\[ H_0: \mu_1 = \mu_2 = ... = \mu_6 \]
\[ \begin{aligned} \mu_1 - \mu_2 &= 0 \\ \mu_2 - \mu_3 &= 0 \\ &... \\ \mu_5 - \mu_6 &= 0 \end{aligned} \]
We can test orthogonal polynomials for 4 equally spaced time points. To test for example the null hypothesis that quadratic and cubic effects are jointly equal to 0, we would define \(\mathbf{C}\)
\[ \mathbf{C} = \left( \begin{array} {cccc} 1 & -1 & -1 & 1 \\ -1 & 3 & -3 & 1 \end{array} \right) \]
22.0.3.2 Two-Sample Tests
Consider the analogous two sample multivariate tests.
Example: we have data on two independent random samples, one sample from each of two populations
\[ \begin{aligned} \mathbf{y}_{1i} &\sim N_p (\mathbf{\mu_1, \Sigma}) \\ \mathbf{y}_{2j} &\sim N_p (\mathbf{\mu_2, \Sigma}) \end{aligned} \]
equal variance-covariance matrices
independent random samples
We can summarize our data using the sufficient statistics \(\mathbf{\bar{y}}_1, \mathbf{S}_1, \mathbf{\bar{y}}_2, \mathbf{S}_2\) with respective sample sizes, \(n_1,n_2\)
Since we assume that \(\mathbf{\Sigma}_1 = \mathbf{\Sigma}_2 = \mathbf{\Sigma}\) , compute a pooled estimate of the variance-covariance matrix on \(n_1 + n_2 - 2\) df
\[ \mathbf{S} = \frac{(n_1 - 1)\mathbf{S}_1 + (n_2-1) \mathbf{S}_2}{(n_1 -1) + (n_2 - 1)} \]
\[ \begin{aligned} &H_0: \mathbf{\mu}_1 = \mathbf{\mu}_2 \\ &H_a: \mathbf{\mu}_1 \neq \mathbf{\mu}_2 \end{aligned} \]
At least one element of the mean vectors is different
\(\mathbf{\bar{y}}_1 - \mathbf{\bar{y}}_2\) to estimate \(\mu_1 - \mu_2\)
\(\mathbf{S}\) to estimate \(\mathbf{\Sigma}\)
Note: because we assume the two populations are independent, there is no covariance
\(cov(\mathbf{\bar{y}}_1 - \mathbf{\bar{y}}_2) = var(\mathbf{\bar{y}}_1) + var(\mathbf{\bar{y}}_2) = \frac{\mathbf{\Sigma_1}}{n_1} + \frac{\mathbf{\Sigma_2}}{n_2} = \mathbf{\Sigma}(\frac{1}{n_1} + \frac{1}{n_2})\)
Reject \(H_0\) if
\[ \begin{aligned} T^2 &= (\mathbf{\bar{y}}_1 - \mathbf{\bar{y}}_2)'\{ \mathbf{S} (\frac{1}{n_1} + \frac{1}{n_2})\}^{-1} (\mathbf{\bar{y}}_1 - \mathbf{\bar{y}}_2)\\ &= \frac{n_1 n_2}{n_1 +n_2} (\mathbf{\bar{y}}_1 - \mathbf{\bar{y}}_2)'\{ \mathbf{S} \}^{-1} (\mathbf{\bar{y}}_1 - \mathbf{\bar{y}}_2)\\ & \ge \frac{(n_1 + n_2 -2)p}{n_1 + n_2 - p - 1} f_{(1- \alpha,n_1 + n_2 - p -1)} \end{aligned} \]
or equivalently, if
\[ F = \frac{n_1 + n_2 - p -1}{(n_1 + n_2 -2)p} T^2 \ge f_{(1- \alpha, p , n_1 + n_2 -p -1)} \]
A \(100(1-\alpha) \%\) confidence region for \(\mu_1 - \mu_2\) consists of all vector \(\delta\) which satisfy
\[ \frac{n_1 n_2}{n_1 + n_2} (\mathbf{\bar{y}}_1 - \mathbf{\bar{y}}_2 - \mathbf{\delta})' \mathbf{S}^{-1}(\mathbf{\bar{y}}_1 - \mathbf{\bar{y}}_2 - \mathbf{\delta}) \le \frac{(n_1 + n_2 - 2)p}{n_1 + n_2 -p - 1}f_{(1-\alpha, p , n_1 + n_2 - p -1)} \]
The simultaneous confidence intervals for all linear combinations of \(\mu_1 - \mu_2\) have the form
\[ \mathbf{a'}(\mathbf{\bar{y}}_1 - \mathbf{\bar{y}}_2) \pm \sqrt{\frac{(n_1 + n_2 -2)p}{n_1 + n_2 - p -1}}f_{(1-\alpha, p, n_1 + n_2 -p -1)} \times \sqrt{\mathbf{a'Sa}(\frac{1}{n_1} + \frac{1}{n_2})} \]
Bonferroni intervals, for k combinations
\[ (\bar{y}_{1i} - \bar{y}_{2i}) \pm t_{(1-\alpha/2k, n_1 + n_2 - 2)}\sqrt{(\frac{1}{n_1} + \frac{1}{n_2})s_{ii}} \]
22.0.3.3 Model Assumptions
If model assumption are not met
Unequal Covariance Matrices
If \(n_1 = n_2\) (large samples) there is little effect on the Type I error rate and power fo the two sample test
If \(n_1 > n_2\) and the eigenvalues of \(\mathbf{\Sigma}_1 \mathbf{\Sigma}^{-1}_2\) are less than 1, the Type I error level is inflated
If \(n_1 > n_2\) and some eigenvalues of \(\mathbf{\Sigma}_1 \mathbf{\Sigma}_2^{-1}\) are greater than 1, the Type I error rate is too small, leading to a reduction in power
Sample Not Normal
Type I error level of the two sample \(T^2\) test isn’t much affect by moderate departures from normality if the two populations being sampled have similar distributions
One sample \(T^2\) test is much more sensitive to lack of normality, especially when the distribution is skewed.
Intuitively, you can think that in one sample your distribution will be sensitive, but the distribution of the difference between two similar distributions will not be as sensitive.
Transform to make the data more normal
Large large samples, use the \(\chi^2\) (Wald) test, in which populations don’t need to be normal, or equal sample sizes, or equal variance-covariance matrices
- \(H_0: \mu_1 - \mu_2 =0\) use \((\mathbf{\bar{y}}_1 - \mathbf{\bar{y}}_2)'( \frac{1}{n_1} \mathbf{S}_1 + \frac{1}{n_2}\mathbf{S}_2)^{-1}(\mathbf{\bar{y}}_1 - \mathbf{\bar{y}}_2) \dot{\sim} \chi^2_{(p)}\)
22.0.3.3.1 Equal Covariance Matrices Tests
With independent random samples from k populations of \(p\) -dimensional vectors. We compute the sample covariance matrix for each, \(\mathbf{S}_i\) , where \(i = 1,...,k\)
\[ \begin{aligned} &H_0: \mathbf{\Sigma}_1 = \mathbf{\Sigma}_2 = \ldots = \mathbf{\Sigma}_k = \mathbf{\Sigma} \\ &H_a: \text{at least 2 are different} \end{aligned} \]
Assume \(H_0\) is true, we would use a pooled estimate of the common covariance matrix, \(\mathbf{\Sigma}\)
\[ \mathbf{S} = \frac{\sum_{i=1}^k (n_i -1)\mathbf{S}_i}{\sum_{i=1}^k (n_i - 1)} \]
with \(\sum_{i=1}^k (n_i -1)\)
22.0.3.3.1.1 Bartlett’s Test
(a modification of the likelihood ratio test). Define
\[ N = \sum_{i=1}^k n_i \]
and (note: \(| |\) are determinants here, not absolute value)
\[ M = (N - k) \log|\mathbf{S}| - \sum_{i=1}^k (n_i - 1) \log|\mathbf{S}_i| \]
\[ C^{-1} = 1 - \frac{2p^2 + 3p - 1}{6(p+1)(k-1)} \{\sum_{i=1}^k (\frac{1}{n_i - 1}) - \frac{1}{N-k} \} \]
Reject \(H_0\) when \(MC^{-1} > \chi^2_{1- \alpha, (k-1)p(p+1)/2}\)
If not all samples are from normal populations, \(MC^{-1}\) has a distribution which is often shifted to the right of the nominal \(\chi^2\) distribution, which means \(H_0\) is often rejected even when it is true (the Type I error level is inflated). Hence, it is better to test individual normality first, or then multivariate normality before you do Bartlett’s test.
22.0.3.4 Two-Sample Repeated Measurements
Define \(\mathbf{y}_{hi} = (y_{hi1}, ..., y_{hit})'\) to be the observations from the i-th subject in the h-th group for times 1 through T
Assume that \(\mathbf{y}_{11}, ..., \mathbf{y}_{1n_1}\) are iid \(N_t(\mathbf{\mu}_1, \mathbf{\Sigma})\) and that \(\mathbf{y}_{21},...,\mathbf{y}_{2n_2}\) are iid \(N_t(\mathbf{\mu}_2, \mathbf{\Sigma})\)
\(H_0: \mathbf{C}(\mathbf{\mu}_1 - \mathbf{\mu}_2) = \mathbf{0}_c\) where \(\mathbf{C}\) is a \(c \times t\) matrix of rank \(c\) where \(c \le t\)
The test statistic has the form
\[ T^2 = \frac{n_1 n_2}{n_1 + n_2} (\mathbf{\bar{y}}_1 - \mathbf{\bar{y}}_2)' \mathbf{C}'(\mathbf{CSC}')^{-1}\mathbf{C} (\mathbf{\bar{y}}_1 - \mathbf{\bar{y}}_2) \]
where \(\mathbf{S}\) is the pooled covariance estimate. Then,
\[ F = \frac{n_1 + n_2 - c -1}{(n_1 + n_2-2)c} T^2 \sim f_{(c, n_1 + n_2 - c-1)} \]
when \(H_0\) is true
If the null hypothesis \(H_0: \mu_1 = \mu_2\) is rejected. A weaker hypothesis is that the profiles for the two groups are parallel.
\[ \begin{aligned} \mu_{11} - \mu_{21} &= \mu_{12} - \mu_{22} \\ &\vdots \\ \mu_{1t-1} - \mu_{2t-1} &= \mu_{1t} - \mu_{2t} \end{aligned} \]
The null hypothesis matrix term is then
\(H_0: \mathbf{C}(\mu_1 - \mu_2) = \mathbf{0}_c\) , where \(c = t - 1\) and
\[ \mathbf{C} = \left( \begin{array} {ccccc} 1 & -1 & 0 & \ldots & 0 \\ 0 & 1 & -1 & \ldots & 0 \\ \vdots & \vdots & \vdots & \ddots & \vdots \\ 0 & 0 & 0 & \ldots & -1 \end{array} \right)_{(t-1) \times t} \]
can’t reject the null of hypothesized vector of means
reject the null that the two labs’ measurements are equal

reject null. Hence, there is a difference in the means of the bivariate normal distributions
22.1 MANOVA
Multivariate Analysis of Variance
One-way MANOVA
Compare treatment means for h different populations
Population 1: \(\mathbf{y}_{11}, \mathbf{y}_{12}, \dots, \mathbf{y}_{1n_1} \sim idd N_p (\mathbf{\mu}_1, \mathbf{\Sigma})\)
Population h: \(\mathbf{y}_{h1}, \mathbf{y}_{h2}, \dots, \mathbf{y}_{hn_h} \sim idd N_p (\mathbf{\mu}_h, \mathbf{\Sigma})\)
Assumptions
- Independent random samples from \(h\) different populations
- Common covariance matrices
- Each population is multivariate normal
Calculate the summary statistics \(\mathbf{\bar{y}}_i, \mathbf{S}\) and the pooled estimate of the covariance matrix \(\mathbf{S}\)
Similar to the univariate one-way ANVOA, we can use the effects model formulation \(\mathbf{\mu}_i = \mathbf{\mu} + \mathbf{\tau}_i\) , where
\(\mathbf{\mu}_i\) is the population mean for population i
\(\mathbf{\mu}\) is the overall mean effect
\(\mathbf{\tau}_i\) is the treatment effect of the i-th treatment.
For the one-way model: \(\mathbf{y}_{ij} = \mu + \tau_i + \epsilon_{ij}\) for \(i = 1,..,h; j = 1,..., n_i\) and \(\epsilon_{ij} \sim N_p(\mathbf{0, \Sigma})\)
However, the above model is over-parameterized (i.e., infinite number of ways to define \(\mathbf{\mu}\) and the \(\mathbf{\tau}_i\) ’s such that they add up to \(\mu_i\) . Thus we can constrain by having
\[ \sum_{i=1}^h n_i \tau_i = 0 \]
\[ \mathbf{\tau}_h = 0 \]
The observational equivalent of the effects model is
\[ \begin{aligned} \mathbf{y}_{ij} &= \mathbf{\bar{y}} + (\mathbf{\bar{y}}_i - \mathbf{\bar{y}}) + (\mathbf{y}_{ij} - \mathbf{\bar{y}}_i) \\ &= \text{overall sample mean} + \text{treatement effect} + \text{residual} \text{ (under univariate ANOVA)} \end{aligned} \]
After manipulation
\[ \sum_{i = 1}^h \sum_{j = 1}^{n_i} (\mathbf{\bar{y}}_{ij} - \mathbf{\bar{y}})(\mathbf{\bar{y}}_{ij} - \mathbf{\bar{y}})' = \sum_{i = 1}^h n_i (\mathbf{\bar{y}}_i - \mathbf{\bar{y}})(\mathbf{\bar{y}}_i - \mathbf{\bar{y}})' + \sum_{i=1}^h \sum_{j = 1}^{n_i} (\mathbf{\bar{y}}_{ij} - \mathbf{\bar{y}})(\mathbf{\bar{y}}_{ij} - \mathbf{\bar{y}}_i)' \]
LHS = Total corrected sums of squares and cross products (SSCP) matrix
1st term = Treatment (or between subjects) sum of squares and cross product matrix (denoted H;B)
2nd term = residual (or within subject) SSCP matrix denoted (E;W)
\[ \mathbf{E} = (n_1 - 1)\mathbf{S}_1 + ... + (n_h -1) \mathbf{S}_h = (n-h) \mathbf{S} \]
MANOVA table
\[ H_0: \tau_1 = \tau_2 = \dots = \tau_h = \mathbf{0} \]
We consider the relative “sizes” of \(\mathbf{E}\) and \(\mathbf{H+E}\)
Wilk’s Lambda
Define Wilk’s Lambda
\[ \Lambda^* = \frac{|\mathbf{E}|}{|\mathbf{H+E}|} \]
Properties:
Wilk’s Lambda is equivalent to the F-statistic in the univariate case
The exact distribution of \(\Lambda^*\) can be determined for especial cases.
For large sample sizes, reject \(H_0\) if
\[ -(\sum_{i=1}^h n_i - 1 - \frac{p+h}{2}) \log(\Lambda^*) > \chi^2_{(1-\alpha, p(h-1))} \]
22.1.1 Testing General Hypotheses
\(h\) different treatments
with the i-th treatment
applied to \(n_i\) subjects that
are observed for \(p\) repeated measures.
Consider this a \(p\) dimensional obs on a random sample from each of \(h\) different treatment populations.
\[ \mathbf{y}_{ij} = \mathbf{\mu} + \mathbf{\tau}_i + \mathbf{\epsilon}_{ij} \]
for \(i = 1,..,h\) and \(j = 1,..,n_i\)
\[ \mathbf{Y} = \mathbf{XB} + \mathbf{\epsilon} \]
where \(n = \sum_{i = 1}^h n_i\) and with restriction \(\mathbf{\tau}_h = 0\)
\[ \mathbf{Y}_{(n \times p)} = \left[ \begin{array} {c} \mathbf{y}_{11}' \\ \vdots \\ \mathbf{y}_{1n_1}' \\ \vdots \\ \mathbf{y}_{hn_h}' \end{array} \right], \mathbf{B}_{(h \times p)} = \left[ \begin{array} {c} \mathbf{\mu}' \\ \mathbf{\tau}_1' \\ \vdots \\ \mathbf{\tau}_{h-1}' \end{array} \right], \mathbf{\epsilon}_{(n \times p)} = \left[ \begin{array} {c} \epsilon_{11}' \\ \vdots \\ \epsilon_{1n_1}' \\ \vdots \\ \epsilon_{hn_h}' \end{array} \right] \]
\[ \mathbf{X}_{(n \times h)} = \left[ \begin{array} {ccccc} 1 & 1 & 0 & \ldots & 0 \\ \vdots & \vdots & \vdots & & \vdots \\ 1 & 1 & 0 & \ldots & 0 \\ \vdots & \vdots & \vdots & \ldots & \vdots \\ 1 & 0 & 0 & \ldots & 0 \\ \vdots & \vdots & \vdots & & \vdots \\ 1 & 0 & 0 & \ldots & 0 \end{array} \right] \]
\[ \mathbf{\hat{B}} = (\mathbf{X'X})^{-1} \mathbf{X'Y} \]
Rows of \(\mathbf{Y}\) are independent (i.e., \(var(\mathbf{Y}) = \mathbf{I}_n \otimes \mathbf{\Sigma}\) , an \(np \times np\) matrix, where \(\otimes\) is the Kronecker product).
\[ \begin{aligned} &H_0: \mathbf{LBM} = 0 \\ &H_a: \mathbf{LBM} \neq 0 \end{aligned} \]
\(\mathbf{L}\) is a \(g \times h\) matrix of full row rank ( \(g \le h\) ) = comparisons across groups
\(\mathbf{M}\) is a \(p \times u\) matrix of full column rank ( \(u \le p\) ) = comparisons across traits
The general treatment corrected sums of squares and cross product is
\[ \mathbf{H} = \mathbf{M'Y'X(X'X)^{-1}L'[L(X'X)^{-1}L']^{-1}L(X'X)^{-1}X'YM} \]
or for the null hypothesis \(H_0: \mathbf{LBM} = \mathbf{D}\)
\[ \mathbf{H} = (\mathbf{\hat{LBM}} - \mathbf{D})'[\mathbf{X(X'X)^{-1}L}]^{-1}(\mathbf{\hat{LBM}} - \mathbf{D}) \]
The general matrix of residual sums of squares and cross product
\[ \mathbf{E} = \mathbf{M'Y'[I-X(X'X)^{-1}X']YM} = \mathbf{M'[Y'Y - \hat{B}'(X'X)^{-1}\hat{B}]M} \]
We can compute the following statistic eigenvalues of \(\mathbf{HE}^{-1}\)
Wilk’s Criterion: \(\Lambda^* = \frac{|\mathbf{E}|}{|\mathbf{H} + \mathbf{E}|}\) . The df depend on the rank of \(\mathbf{L}, \mathbf{M}, \mathbf{X}\)
Lawley-Hotelling Trace: \(U = tr(\mathbf{HE}^{-1})\)
Pillai Trace: \(V = tr(\mathbf{H}(\mathbf{H}+ \mathbf{E}^{-1})\)
Roy’s Maximum Root: largest eigenvalue of \(\mathbf{HE}^{-1}\)
If \(H_0\) is true and n is large, \(-(n-1- \frac{p+h}{2})\ln \Lambda^* \sim \chi^2_{p(h-1)}\) . Some special values of p and h can give exact F-dist under \(H_0\)
reject the null of equal multivariate mean vectors between the three admmission groups
- If independent = time with 3 levels -> univariate ANOVA (require sphericity assumption (i.e., the variances for all differences are equal))
- If each level of independent time as a separate variable -> MANOVA (does not require sphericity assumption)
can’t reject the null hypothesis of sphericity, hence univariate ANOVA is also appropriate.We also see linear significant time effect, but no quadratic time effect

reject the null hypothesis of no difference in means between treatments
there is no significant difference in means between the control and bww9 drug
there is a significant difference in means between ax23 drug treatment and the rest of the treatments
22.1.2 Profile Analysis
Examine similarities between the treatment effects (between subjects), which is useful for longitudinal analysis. Null is that all treatments have the same average effect.
\[ H_0: \mu_1 = \mu_2 = \dots = \mu_h \]
\[ H_0: \tau_1 = \tau_2 = \dots = \tau_h \]
The exact nature of the similarities and differences between the treatments can be examined under this analysis.
Sequential steps in profile analysis:
- Are the profiles parallel ? (i.e., is there no interaction between treatment and time)
- Are the profiles coincidental ? (i.e., are the profiles identical?)
- Are the profiles horizontal ? (i.e., are there no differences between any time points?)
If we reject the null hypothesis that the profiles are parallel, we can test
Are there differences among groups within some subset of the total time points?
Are there differences among time points in a particular group (or groups)?
Are there differences within some subset of the total time points in a particular group (or groups)?
4 times (p = 4)
3 treatments (h=3)
22.1.2.1 Parallel Profile
Are the profiles for each population identical expect for a mean shift?
\[ \begin{aligned} H_0: \mu_{11} - \mu_{21} - \mu_{12} - \mu_{22} = &\dots = \mu_{1t} - \mu_{2t} \\ \mu_{11} - \mu_{31} - \mu_{12} - \mu_{32} = &\dots = \mu_{1t} - \mu_{3t} \\ &\dots \end{aligned} \]
for \(h-1\) equations
\[ H_0: \mathbf{LBM = 0} \]
\[ \mathbf{LBM} = \left[ \begin{array} {ccc} 1 & -1 & 0 \\ 1 & 0 & -1 \end{array} \right] \left[ \begin{array} {ccc} \mu_{11} & \dots & \mu_{14} \\ \mu_{21} & \dots & \mu_{24} \\ \mu_{31} & \dots & \mu_{34} \end{array} \right] \left[ \begin{array} {ccc} 1 & 1 & 1 \\ -1 & 0 & 0 \\ 0 & -1 & 0 \\ 0 & 0 & -1 \end{array} \right] = \mathbf{0} \]
where this is the cell means parameterization of \(\mathbf{B}\)
The multiplication of the first 2 matrices \(\mathbf{LB}\) is
\[ \left[ \begin{array} {cccc} \mu_{11} - \mu_{21} & \mu_{12} - \mu_{22} & \mu_{13} - \mu_{23} & \mu_{14} - \mu_{24}\\ \mu_{11} - \mu_{31} & \mu_{12} - \mu_{32} & \mu_{13} - \mu_{33} & \mu_{14} - \mu_{34} \end{array} \right] \]
which is the differences in treatment means at the same time
Multiplying by \(\mathbf{M}\) , we get the comparison across time
\[ \left[ \begin{array} {ccc} (\mu_{11} - \mu_{21}) - (\mu_{12} - \mu_{22}) & (\mu_{11} - \mu_{21}) -(\mu_{13} - \mu_{23}) & (\mu_{11} - \mu_{21}) - (\mu_{14} - \mu_{24}) \\ (\mu_{11} - \mu_{31}) - (\mu_{12} - \mu_{32}) & (\mu_{11} - \mu_{31}) - (\mu_{13} - \mu_{33}) & (\mu_{11} - \mu_{31}) -(\mu_{14} - \mu_{34}) \end{array} \right] \]
Alternatively, we can also use the effects parameterization
\[ \mathbf{LBM} = \left[ \begin{array} {cccc} 0 & 1 & -1 & 0 \\ 0 & 1 & 0 & -1 \end{array} \right] \left[ \begin{array} {c} \mu' \\ \tau'_1 \\ \tau_2' \\ \tau_3' \end{array} \right] \left[ \begin{array} {ccc} 1 & 1 & 1 \\ -1 & 0 & 0 \\ 0 & -1 & 0 \\ 0 & 0 & -1 \end{array} \right] = \mathbf{0} \]
In both parameterizations, \(rank(\mathbf{L}) = h-1\) and \(rank(\mathbf{M}) = p-1\)
We could also choose \(\mathbf{L}\) and \(\mathbf{M}\) in other forms
\[ \mathbf{L} = \left[ \begin{array} {cccc} 0 & 1 & 0 & -1 \\ 0 & 0 & 1 & -1 \end{array} \right] \]
\[ \mathbf{M} = \left[ \begin{array} {ccc} 1 & 0 & 0 \\ -1 & 1 & 0 \\ 0 & -1 & 1 \\ 0 & 0 & -1 \end{array} \right] \]
and still obtain the same result.
22.1.2.2 Coincidental Profiles
After we have evidence that the profiles are parallel (i.e., fail to reject the parallel profile test), we can ask whether they are identical?
Given profiles are parallel , then if the sums of the components of \(\mu_i\) are identical for all the treatments, then the profiles are identical .
\[ H_0: \mathbf{1'}_p \mu_1 = \mathbf{1'}_p \mu_2 = \dots = \mathbf{1'}_p \mu_h \]
\[ H_0: \mathbf{LBM} = \mathbf{0} \]
where for the cell means parameterization
\[ \mathbf{L} = \left[ \begin{array} {ccc} 1 & 0 & -1 \\ 0 & 1 & -1 \end{array} \right] \]
\[ \mathbf{M} = \left[ \begin{array} {cccc} 1 & 1 & 1 & 1 \end{array} \right]' \]
multiplication yields
\[ \left[ \begin{array} {c} (\mu_{11} + \mu_{12} + \mu_{13} + \mu_{14}) - (\mu_{31} + \mu_{32} + \mu_{33} + \mu_{34}) \\ (\mu_{21} + \mu_{22} + \mu_{23} + \mu_{24}) - (\mu_{31} + \mu_{32} + \mu_{33} + \mu_{34}) \end{array} \right] = \left[ \begin{array} {c} 0 \\ 0 \end{array} \right] \]
Different choices of \(\mathbf{L}\) and \(\mathbf{M}\) can yield the same result
22.1.2.3 Horizontal Profiles
Given that we can’t reject the null hypothesis that all \(h\) profiles are the same, we can ask whether all of the elements of the common profile equal? (i.e., horizontal)
\[ \mathbf{L} = \left[ \begin{array} {ccc} 1 & 0 & 0 \end{array} \right] \]
\[ \left[ \begin{array} {ccc} (\mu_{11} - \mu_{12}) & (\mu_{12} - \mu_{13}) & (\mu_{13} + \mu_{14}) \end{array} \right] = \left[ \begin{array} {ccc} 0 & 0 & 0 \end{array} \right] \]
- If we fail to reject all 3 hypotheses, then we fail to reject the null hypotheses of both no difference between treatments and no differences between traits.
22.1.3 Summary
22.2 Principal Components
- Unsupervised learning
- find important features
- reduce the dimensions of the data set
- “decorrelate” multivariate vectors that have dependence.
- uses eigenvector/eigvenvalue decomposition of covariance (correlation) matrices.
According to the “spectral decomposition theorem”, if \(\mathbf{\Sigma}_{p \times p}\) i s a positive semi-definite, symmetric, real matrix, then there exists an orthogonal matrix \(\mathbf{A}\) such that \(\mathbf{A'\Sigma A} = \Lambda\) where \(\Lambda\) is a diagonal matrix containing the eigenvalues \(\mathbf{\Sigma}\)
\[ \mathbf{\Lambda} = \left( \begin{array} {cccc} \lambda_1 & 0 & \ldots & 0 \\ 0 & \lambda_2 & \ldots & 0 \\ \vdots & \vdots & \ddots & \vdots \\ 0 & 0 & \ldots & \lambda_p \end{array} \right) \]
\[ \mathbf{A} = \left( \begin{array} {cccc} \mathbf{a}_1 & \mathbf{a}_2 & \ldots & \mathbf{a}_p \end{array} \right) \]
the i-th column of \(\mathbf{A}\) , \(\mathbf{a}_i\) , is the i-th \(p \times 1\) eigenvector of \(\mathbf{\Sigma}\) that corresponds to the eigenvalue, \(\lambda_i\) , where \(\lambda_1 \ge \lambda_2 \ge \ldots \ge \lambda_p\) . Alternatively, express in matrix decomposition:
\[ \mathbf{\Sigma} = \mathbf{A \Lambda A}' \]
\[ \mathbf{\Sigma} = \mathbf{A} \left( \begin{array} {cccc} \lambda_1 & 0 & \ldots & 0 \\ 0 & \lambda_2 & \ldots & 0 \\ \vdots & \vdots& \ddots & \vdots \\ 0 & 0 & \ldots & \lambda_p \end{array} \right) \mathbf{A}' = \sum_{i=1}^p \lambda_i \mathbf{a}_i \mathbf{a}_i' \]
where the outer product \(\mathbf{a}_i \mathbf{a}_i'\) is a \(p \times p\) matrix of rank 1.
For example,
\(\mathbf{x} \sim N_2(\mathbf{\mu}, \mathbf{\Sigma})\)
\[ \mathbf{\mu} = \left( \begin{array} {c} 5 \\ 12 \end{array} \right); \mathbf{\Sigma} = \left( \begin{array} {cc} 4 & 1 \\ 1 & 2 \end{array} \right) \]

\[ \mathbf{A} = \left( \begin{array} {cc} 0.9239 & -0.3827 \\ 0.3827 & 0.9239 \\ \end{array} \right) \]
Columns of \(\mathbf{A}\) are the eigenvectors for the decomposition
Under matrix multiplication ( \(\mathbf{A'\Sigma A}\) or \(\mathbf{A'A}\) ), the off-diagonal elements equal to 0
Multiplying data by this matrix (i.e., projecting the data onto the orthogonal axes); the distribution of the resulting data (i.e., “scores”) is
\[ N_2 (\mathbf{A'\mu,A'\Sigma A}) = N_2 (\mathbf{A'\mu, \Lambda}) \]
\[ \mathbf{y} = \mathbf{A'x} \sim N \left[ \left( \begin{array} {c} 9.2119 \\ 9.1733 \end{array} \right), \left( \begin{array} {cc} 4.4144 & 0 \\ 0 & 1.5859 \end{array} \right) \right] \]

No more dependence in the data structure, plot
The i-th eigenvalue is the variance of a linear combination of the elements of \(\mathbf{x}\) ; \(var(y_i) = var(\mathbf{a'_i x}) = \lambda_i\)
The values on the transformed set of axes (i.e., the \(y_i\) ’s) are called the scores. These are the orthogonal projections of the data onto the “new principal component axes
Variances of \(y_1\) are greater than those for any other possible projection
Covariance matrix decomposition and projection onto orthogonal axes = PCA
22.2.1 Population Principal Components
\(p \times 1\) vectors \(\mathbf{x}_1, \dots , \mathbf{x}_n\) which are iid with \(var(\mathbf{x}_i) = \mathbf{\Sigma}\)
The first PC is the linear combination \(y_1 = \mathbf{a}_1' \mathbf{x} = a_{11}x_1 + \dots + a_{1p}x_p\) with \(\mathbf{a}_1' \mathbf{a}_1 = 1\) such that \(var(y_1)\) is the maximum of all linear combinations of \(\mathbf{x}\) which have unit length
The second PC is the linear combination \(y_1 = \mathbf{a}_2' \mathbf{x} = a_{21}x_1 + \dots + a_{2p}x_p\) with \(\mathbf{a}_2' \mathbf{a}_2 = 1\) such that \(var(y_1)\) is the maximum of all linear combinations of \(\mathbf{x}\) which have unit length and uncorrelated with \(y_1\) (i.e., \(cov(\mathbf{a}_1' \mathbf{x}, \mathbf{a}'_2 \mathbf{x}) =0\)
continues for all \(y_i\) to \(y_p\)
\(\mathbf{a}_i\) ’s are those that make up the matrix \(\mathbf{A}\) in the symmetric decomposition \(\mathbf{A'\Sigma A} = \mathbf{\Lambda}\) , where \(var(y_1) = \lambda_1, \dots , var(y_p) = \lambda_p\) And the total variance of \(\mathbf{x}\) is
\[ \begin{aligned} var(x_1) + \dots + var(x_p) &= tr(\Sigma) = \lambda_1 + \dots + \lambda_p \\ &= var(y_1) + \dots + var(y_p) \end{aligned} \]
Data Reduction
To reduce the dimension of data from p (original) to k dimensions without much “loss of information”, we can use properties of the population principal components
Suppose \(\mathbf{\Sigma} \approx \sum_{i=1}^k \lambda_i \mathbf{a}_i \mathbf{a}_i'\) . Even thought the true variance-covariance matrix has rank \(p\) , it can be be well approximate by a matrix of rank k (k <p)
New “traits” are linear combinations of the measured traits. We can attempt to make meaningful interpretation fo the combinations (with orthogonality constraints).
The proportion of the total variance accounted for by the j-th principal component is
\[ \frac{var(y_j)}{\sum_{i=1}^p var(y_i)} = \frac{\lambda_j}{\sum_{i=1}^p \lambda_i} \]
The proportion of the total variation accounted for by the first k principal components is \(\frac{\sum_{i=1}^k \lambda_i}{\sum_{i=1}^p \lambda_i}\)
Above example , we have \(4.4144/(4+2) = .735\) of the total variability can be explained by the first principal component
22.2.2 Sample Principal Components
Since \(\mathbf{\Sigma}\) is unknown, we use
\[ \mathbf{S} = \frac{1}{n-1}\sum_{i=1}^n (\mathbf{x}_i - \bar{\mathbf{x}})(\mathbf{x}_i - \bar{\mathbf{x}})' \]
Let \(\hat{\lambda}_1 \ge \hat{\lambda}_2 \ge \dots \ge \hat{\lambda}_p \ge 0\) be the eigenvalues of \(\mathbf{S}\) and \(\hat{\mathbf{a}}_1, \hat{\mathbf{a}}_2, \dots, \hat{\mathbf{a}}_p\) denote the eigenvectors of \(\mathbf{S}\)
Then, the i-th sample principal component score (or principal component or score) is
\[ \hat{y}_{ij} = \sum_{k=1}^p \hat{a}_{ik}x_{kj} = \hat{\mathbf{a}}_i'\mathbf{x}_j \]
Properties of Sample Principal Components
The estimated variance of \(y_i = \hat{\mathbf{a}}_i'\mathbf{x}_j\) is \(\hat{\lambda}_i\)
The sample covariance between \(\hat{y}_i\) and \(\hat{y}_{i'}\) is 0 when \(i \neq i'\)
The proportion of the total sample variance accounted for by the i-th sample principal component is \(\frac{\hat{\lambda}_i}{\sum_{k=1}^p \hat{\lambda}_k}\)
The estimated correlation between the \(i\) -th principal component score and the \(l\) -th attribute of \(\mathbf{x}\) is
\[ r_{x_l , \hat{y}_i} = \frac{\hat{a}_{il}\sqrt{\lambda_i}}{\sqrt{s_{ll}}} \]
The correlation coefficient is typically used to interpret the components (i.e., if this correlation is high then it suggests that the l-th original trait is important in the i-th principle component). According to R. A. Johnson, Wichern, et al. ( 2002 ) , pp.433-434, \(r_{x_l, \hat{y}_i}\) only measures the univariate contribution of an individual X to a component Y without taking into account the presence of the other X’s. Hence, some prefer \(\hat{a}_{il}\) coefficient to interpret the principal component.
\(r_{x_l, \hat{y}_i} ; \hat{a}_{il}\) are referred to as “loadings”
To use k principal components, we must calculate the scores for each data vector in the sample
\[ \mathbf{y}_j = \left( \begin{array} {c} y_{1j} \\ y_{2j} \\ \vdots \\ y_{kj} \end{array} \right) = \left( \begin{array} {c} \hat{\mathbf{a}}_1' \mathbf{x}_j \\ \hat{\mathbf{a}}_2' \mathbf{x}_j \\ \vdots \\ \hat{\mathbf{a}}_k' \mathbf{x}_j \end{array} \right) = \left( \begin{array} {c} \hat{\mathbf{a}}_1' \\ \hat{\mathbf{a}}_2' \\ \vdots \\ \hat{\mathbf{a}}_k' \end{array} \right) \mathbf{x}_j \]
Large sample theory exists for eigenvalues and eigenvectors of sample covariance matrices if inference is necessary. But we do not do inference with PCA, we only use it as exploratory or descriptive analysis.
PC is not invariant to changes in scale (Exception: if all trait are rescaled by multiplying by the same constant, such as feet to inches).
PCA based on the correlation matrix \(\mathbf{R}\) is different than that based on the covariance matrix \(\mathbf{\Sigma}\)
PCA for the correlation matrix is just rescaling each trait to have unit variance
Transform \(\mathbf{x}\) to \(\mathbf{z}\) where \(z_{ij} = (x_{ij} - \bar{x}_i)/\sqrt{s_{ii}}\) where the denominator affects the PCA
After transformation, \(cov(\mathbf{z}) = \mathbf{R}\)
PCA on \(\mathbf{R}\) is calculated in the same way as that on \(\mathbf{S}\) (where \(\hat{\lambda}{}_1 + \dots + \hat{\lambda}{}_p = p\) )
The use of \(\mathbf{R}, \mathbf{S}\) depends on the purpose of PCA.
- If the scale of the observations if different, covariance matrix is more preferable. but if they are dramatically different, analysis can still be dominated by the large variance traits.
How many PCs to use can be guided by
Scree Graphs: plot the eigenvalues against their indices. Look for the “elbow” where the steep decline in the graph suddenly flattens out; or big gaps.
minimum Percent of total variation (e.g., choose enough components to have 50% or 90%). can be used for interpretations.
Kaiser’s rule: use only those PC with eigenvalues larger than 1 (applied to PCA on the correlation matrix) - ad hoc
Compare to the eigenvalue scree plot of data to the scree plot when the data are randomized.
22.2.3 Application
PCA on the covariance matrix is usually not preferred due to the fact that PCA is not invariant to changes in scale. Hence, PCA on the correlation matrix is more preferred
This also addresses the problem of multicollinearity
The eigvenvectors may differ by a multiplication of -1 for different implementation, but same interpretation.
Covid Example
To reduce collinearity problem in this dataset, we can use principal components as regressors.

MSE for the PC-based model is larger than regular regression, because models with a large degree of collinearity can still perform well.
pcr function in pls can be used for fitting PC regression (it will select the optimal number of components in the model).
22.3 Factor Analysis
Using a few linear combinations of underlying unobservable (latent) traits, we try to describe the covariance relationship among a large number of measured traits
Similar to PCA , but factor analysis is model based
More details can be found on PSU stat or UMN stat
Let \(\mathbf{y}\) be the set of \(p\) measured variables
\(E(\mathbf{y}) = \mathbf{\mu}\)
\(var(\mathbf{y}) = \mathbf{\Sigma}\)
\[ \begin{aligned} \mathbf{y} - \mathbf{\mu} &= \mathbf{Lf} + \epsilon \\ &= \left( \begin{array} {c} l_{11}f_1 + l_{12}f_2 + \dots + l_{tm}f_m \\ \vdots \\ l_{p1}f_1 + l_{p2}f_2 + \dots + l_{pm} f_m \end{array} \right) + \left( \begin{array} {c} \epsilon_1 \\ \vdots \\ \epsilon_p \end{array} \right) \end{aligned} \]
\(\mathbf{y} - \mathbf{\mu}\) = the p centered measurements
\(\mathbf{L}\) = \(p \times m\) matrix of factor loadings
\(\mathbf{f}\) = unobserved common factors for the population
\(\mathbf{\epsilon}\) = random errors (i.e., variation that is not accounted for by the common factors).
We want \(m\) (the number of factors) to be much smaller than \(p\) (the number of measured attributes)
Restrictions on the model
\(E(\epsilon) = \mathbf{0}\)
\(var(\epsilon) = \Psi_{p \times p} = diag( \psi_1, \dots, \psi_p)\)
\(\mathbf{\epsilon}, \mathbf{f}\) are independent
Additional assumption could be \(E(\mathbf{f}) = \mathbf{0}, var(\mathbf{f}) = \mathbf{I}_{m \times m}\) (known as the orthogonal factor model) , which imposes the following covariance structure on \(\mathbf{y}\)
\[ \begin{aligned} var(\mathbf{y}) = \mathbf{\Sigma} &= var(\mathbf{Lf} + \mathbf{\epsilon}) \\ &= var(\mathbf{Lf}) + var(\epsilon) \\ &= \mathbf{L} var(\mathbf{f}) \mathbf{L}' + \mathbf{\Psi} \\ &= \mathbf{LIL}' + \mathbf{\Psi} \\ &= \mathbf{LL}' + \mathbf{\Psi} \end{aligned} \]
Since \(\mathbf{\Psi}\) is diagonal, the off-diagonal elements of \(\mathbf{LL}'\) are \(\sigma_{ij}\) , the co variances in \(\mathbf{\Sigma}\) , which means \(cov(y_i, y_j) = \sum_{k=1}^m l_{ik}l_{jk}\) and the covariance of \(\mathbf{y}\) is completely determined by the m factors ( \(m <<p\) )
\(var(y_i) = \sum_{k=1}^m l_{ik}^2 + \psi_i\) where \(\psi_i\) is the specific variance and the summation term is the i-th communality (i.e., portion of the variance of the i-th variable contributed by the \(m\) common factors ( \(h_i^2 = \sum_{k=1}^m l_{ik}^2\) )
The factor model is only uniquely determined up to an orthogonal transformation of the factors.
Let \(\mathbf{T}_{m \times m}\) be an orthogonal matrix \(\mathbf{TT}' = \mathbf{T'T} = \mathbf{I}\) then
\[ \begin{aligned} \mathbf{y} - \mathbf{\mu} &= \mathbf{Lf} + \epsilon \\ &= \mathbf{LTT'f} + \epsilon \\ &= \mathbf{L}^*(\mathbf{T'f}) + \epsilon & \text{where } \mathbf{L}^* = \mathbf{LT} \end{aligned} \]
\[ \begin{aligned} \mathbf{\Sigma} &= \mathbf{LL}' + \mathbf{\Psi} \\ &= \mathbf{LTT'L} + \mathbf{\Psi} \\ &= (\mathbf{L}^*)(\mathbf{L}^*)' + \mathbf{\Psi} \end{aligned} \]
Hence, any orthogonal transformation of the factors is an equally good description of the correlations among the observed traits.
Let \(\mathbf{y} = \mathbf{Cx}\) , where \(\mathbf{C}\) is any diagonal matrix, then \(\mathbf{L}_y = \mathbf{CL}_x\) and \(\mathbf{\Psi}_y = \mathbf{C\Psi}_x\mathbf{C}\)
Hence, we can see that factor analysis is also invariant to changes in scale
22.3.1 Methods of Estimation
To estimate \(\mathbf{L}\)
- Principal Component Method
- Principal Factor Method
22.3.1.1 Principal Component Method
Spectral decomposition
\[ \begin{aligned} \mathbf{\Sigma} &= \lambda_1 \mathbf{a}_1 \mathbf{a}_1' + \dots + \lambda_p \mathbf{a}_p \mathbf{a}_p' \\ &= \mathbf{A\Lambda A}' \\ &= \sum_{k=1}^m \lambda+k \mathbf{a}_k \mathbf{a}_k' + \sum_{k= m+1}^p \lambda_k \mathbf{a}_k \mathbf{a}_k' \\ &= \sum_{k=1}^m l_k l_k' + \sum_{k=m+1}^p \lambda_k \mathbf{a}_k \mathbf{a}_k' \end{aligned} \]
where \(l_k = \mathbf{a}_k \sqrt{\lambda_k}\) and the second term is not diagonal in general.
\[ \psi_i = \sigma_{ii} - \sum_{k=1}^m l_{ik}^2 = \sigma_{ii} - \sum_{k=1}^m \lambda_i a_{ik}^2 \]
\[ \mathbf{\Sigma} \approx \mathbf{LL}' + \mathbf{\Psi} \]
To estimate \(\mathbf{L}\) and \(\Psi\) , we use the expected eigenvalues and eigenvectors from \(\mathbf{S}\) or \(\mathbf{R}\)
The estimated factor loadings don’t change as the number of actors increases
The diagonal elements of \(\hat{\mathbf{L}}\hat{\mathbf{L}}' + \hat{\mathbf{\Psi}}\) are equal to the diagonal elements of \(\mathbf{S}\) and \(\mathbf{R}\) , but the covariances may not be exactly reproduced
We select \(m\) so that the off-diagonal elements close to the values in \(\mathbf{S}\) (or to make the off-diagonal elements of \(\mathbf{S} - \hat{\mathbf{L}} \hat{\mathbf{L}}' + \hat{\mathbf{\Psi}}\) small)
22.3.1.2 Principal Factor Method
Consider modeling the correlation matrix, \(\mathbf{R} = \mathbf{L} \mathbf{L}' + \mathbf{\Psi}\) . Then
\[ \mathbf{L} \mathbf{L}' = \mathbf{R} - \mathbf{\Psi} = \left( \begin{array} {cccc} h_1^2 & r_{12} & \dots & r_{1p} \\ r_{21} & h_2^2 & \dots & r_{2p} \\ \vdots & \vdots & \ddots & \vdots \\ r_{p1} & r_{p2} & \dots & h_p^2 \end{array} \right) \]
where \(h_i^2 = 1- \psi_i\) (the communality)
Suppose that initial estimates are available for the communalities, \((h_1^*)^2,(h_2^*)^2, \dots , (h_p^*)^2\) , then we can regress each trait on all the others, and then use the \(r^2\) as \(h^2\)
The estimate of \(\mathbf{R} - \mathbf{\Psi}\) at step k is
\[ (\mathbf{R} - \mathbf{\Psi})_k = \left( \begin{array} {cccc} (h_1^*)^2 & r_{12} & \dots & r_{1p} \\ r_{21} & (h_2^*)^2 & \dots & r_{2p} \\ \vdots & \vdots & \ddots & \vdots \\ r_{p1} & r_{p2} & \dots & (h_p^*)^2 \end{array} \right) = \mathbf{L}_k^*(\mathbf{L}_k^*)' \]
\[ \mathbf{L}_k^* = (\sqrt{\hat{\lambda}_1^*\hat{\mathbf{a}}_1^* , \dots \hat{\lambda}_m^*\hat{\mathbf{a}}_m^*}) \]
\[ \hat{\psi}_{i,k}^* = 1 - \sum_{j=1}^m \hat{\lambda}_i^* (\hat{a}_{ij}^*)^2 \]
we used the spectral decomposition on the estimated matrix \((\mathbf{R}- \mathbf{\Psi})\) to calculate the \(\hat{\lambda}_i^* s\) and the \(\mathbf{\hat{a}}_i^* s\)
After updating the values of \((\hat{h}_i^*)^2 = 1 - \hat{\psi}_{i,k}^*\) we will use them to form a new \(\mathbf{L}_{k+1}^*\) via another spectral decomposition. Repeat the process
The matrix \((\mathbf{R} - \mathbf{\Psi})_k\) is not necessarily positive definite
The principal component method is similar to principal factor if one considers the initial communalities are \(h^2 = 1\)
if \(m\) is too large, some communalities may become larger than 1, causing the iterations to terminate. To combat, we can
fix any communality that is greater than 1 at 1 and then continues.
continue iterations regardless of the size of the communalities. However, results can be outside fo the parameter space.
22.3.1.3 Maximum Likelihood Method
Since we need the likelihood function, we make the additional (critical) assumption that
\(\mathbf{y}_j \sim N(\mathbf{\mu},\mathbf{\Sigma})\) for \(j = 1,..,n\)
\(\mathbf{f} \sim N(\mathbf{0}, \mathbf{I})\)
\(\epsilon_j \sim N(\mathbf{0}, \mathbf{\Psi})\)
and restriction
- \(\mathbf{L}' \mathbf{\Psi}^{-1}\mathbf{L} = \mathbf{\Delta}\) where \(\mathbf{\Delta}\) is a diagonal matrix. (since the factor loading matrix is not unique, we need this restriction).
Finding MLE can be computationally expensive
we typically use other methods for exploratory data analysis
Likelihood ratio tests could be used for testing hypotheses in this framework (i.e., Confirmatory Factor Analysis)
22.3.2 Factor Rotation
\(\mathbf{T}_{m \times m}\) is an orthogonal matrix that has the property that
\[ \hat{\mathbf{L}} \hat{\mathbf{L}}' + \hat{\mathbf{\Psi}} = \hat{\mathbf{L}}^*(\hat{\mathbf{L}}^*)' + \hat{\mathbf{\Psi}} \]
where \(\mathbf{L}^* = \mathbf{LT}\)
This means that estimated specific variances and communalities are not altered by the orthogonal transformation.
Since there are an infinite number of choices for \(\mathbf{T}\) , some selection criterion is necessary
For example, we can find the orthogonal transformation that maximizes the objective function
\[ \sum_{j = 1}^m [\frac{1}{p}\sum_{i=1}^p (\frac{l_{ij}^{*2}}{h_i})^2 - \{\frac{\gamma}{p} \sum_{i=1}^p (\frac{l_{ij}^{*2}}{h_i})^2 \}^2] \]
where \(\frac{l_{ij}^{*2}}{h_i}\) are “scaled loadings”, which gives variables with small communalities more influence.
Different choices of \(\gamma\) in the objective function correspond to different orthogonal rotation found in the literature;
Varimax \(\gamma = 1\) (rotate the factors so that each of the \(p\) variables should have a high loading on only one factor, but this is not always possible).
Quartimax \(\gamma = 0\)
Equimax \(\gamma = m/2\)
Parsimax \(\gamma = \frac{p(m-1)}{p+m-2}\)
Promax: non-orthogonal or olique transformations
Harris-Kaiser (HK): non-orthogonal or oblique transformations
22.3.3 Estimation of Factor Scores
\[ (\mathbf{y}_j - \mathbf{\mu}) = \mathbf{L}_{p \times m}\mathbf{f}_j + \epsilon_j \]
If the factor model is correct then
\[ var(\epsilon_j) = \mathbf{\Psi} = diag (\psi_1, \dots , \psi_p) \]
Thus we could consider using weighted least squares to estimate \(\mathbf{f}_j\) , the vector of factor scores for the j-th sampled unit by
\[ \begin{aligned} \hat{\mathbf{f}} &= (\mathbf{L}'\mathbf{\Psi}^{-1} \mathbf{L})^{-1} \mathbf{L}' \mathbf{\Psi}^{-1}(\mathbf{y}_j - \mathbf{\mu}) \\ & \approx (\mathbf{L}'\mathbf{\Psi}^{-1} \mathbf{L})^{-1} \mathbf{L}' \mathbf{\Psi}^{-1}(\mathbf{y}_j - \mathbf{\bar{y}}) \end{aligned} \]
22.3.3.1 The Regression Method
Alternatively, we can use the regression method to estimate the factor scores
Consider the joint distribution of \((\mathbf{y}_j - \mathbf{\mu})\) and \(\mathbf{f}_j\) assuming multivariate normality, as in the maximum likelihood approach. then,
\[ \left( \begin{array} {c} \mathbf{y}_j - \mathbf{\mu} \\ \mathbf{f}_j \end{array} \right) \sim N_{p + m} \left( \left[ \begin{array} {cc} \mathbf{LL}' + \mathbf{\Psi} & \mathbf{L} \\ \mathbf{L}' & \mathbf{I}_{m\times m} \end{array} \right] \right) \]
when the \(m\) factor model is correct
\[ E(\mathbf{f}_j | \mathbf{y}_j - \mathbf{\mu}) = \mathbf{L}' (\mathbf{LL}' + \mathbf{\Psi})^{-1}(\mathbf{y}_j - \mathbf{\mu}) \]
notice that \(\mathbf{L}' (\mathbf{LL}' + \mathbf{\Psi})^{-1}\) is an \(m \times p\) matrix of regression coefficients
Then, we use the estimated conditional mean vector to estimate the factor scores
\[ \mathbf{\hat{f}}_j = \mathbf{\hat{L}}'(\mathbf{\hat{L}}\mathbf{\hat{L}}' + \mathbf{\hat{\Psi}})^{-1}(\mathbf{y}_j - \mathbf{\bar{y}}) \]
Alternatively, we could reduce the effect of possible incorrect determination fo the number of factors \(m\) by using \(\mathbf{S}\) as a substitute for \(\mathbf{\hat{L}}\mathbf{\hat{L}}' + \mathbf{\hat{\Psi}}\) then
\[ \mathbf{\hat{f}}_j = \mathbf{\hat{L}}'\mathbf{S}^{-1}(\mathbf{y}_j - \mathbf{\bar{y}}) \]
where \(j = 1,\dots,n\)
22.3.4 Model Diagnostic
Check for outliers (recall that \(\mathbf{f}_j \sim iid N(\mathbf{0}, \mathbf{I}_{m \times m})\) )
Check for multivariate normality assumption
Use univariate tests for normality to check the factor scores
Confirmatory Factor Analysis : formal testing of hypotheses about loadings, use MLE and full/reduced model testing paradigm and measures of model fit
22.3.5 Application
In the psych package,
h2 = the communalities
u2 = the uniqueness
com = the complexity

The output info for the null hypothesis of no common factors is in the statement “The degrees of freedom for the null model ..”
The output info for the null hypothesis that number of factors is sufficient is in the statement “The total number of observations was …”
One factor is not enough, two is sufficient, and not enough data for 3 factors (df of -2 and NA for p-value). Hence, we should use 2-factor model.
22.4 Discriminant Analysis
Suppose we have two or more different populations from which observations could come from. Discriminant analysis seeks to determine which of the possible population an observation comes from while making as few mistakes as possible
This is an alternative to logistic approaches with the following advantages:
when there is clear separation between classes, the parameter estimates for the logic regression model can be surprisingly unstable, while discriminant approaches do not suffer
If X is normal in each of the classes and the sample size is small, then discriminant approaches can be more accurate
Similar to MANOVA, let \(\mathbf{y}_{j1},\mathbf{y}_{j2},\dots, \mathbf{y}_{in_j} \sim iid f_j (\mathbf{y})\) for \(j = 1,\dots, h\)
Let \(f_j(\mathbf{y})\) be the density function for population j . Note that each vector \(\mathbf{y}\) contain measurements on all \(p\) traits
- Assume that each observation is from one of \(h\) possible populations.
- We want to form a discriminant rule that will allocate an observation \(\mathbf{y}\) to population j when \(\mathbf{y}\) is in fact from this population
22.4.1 Known Populations
The maximum likelihood discriminant rule for assigning an observation \(\mathbf{y}\) to one of the \(h\) populations allocates \(\mathbf{y}\) to the population that gives the largest likelihood to \(\mathbf{y}\)
Consider the likelihood for a single observation \(\mathbf{y}\) , which has the form \(f_j (\mathbf{y})\) where j is the true population.
Since \(j\) is unknown, to make the likelihood as large as possible, we should choose the value j which causes \(f_j (\mathbf{y})\) to be as large as possible
Consider a simple univariate example. Suppose we have data from one of two binomial populations.
The first population has \(n= 10\) trials with success probability \(p = .5\)
The second population has \(n= 10\) trials with success probability \(p = .7\)
to which population would we assign an observation of \(y = 7\)
\(f(y = 7|n = 10, p = .5) = .117\)
\(f(y = 7|n = 10, p = .7) = .267\) where \(f(.)\) is the binomial likelihood.
Hence, we choose the second population
Another example
We have 2 populations, where
First population: \(N(\mu_1, \sigma^2_1)\)
Second population: \(N(\mu_2, \sigma^2_2)\)
The likelihood for a single observation is
\[ f_j (y) = (2\pi \sigma^2_j)^{-1/2} \exp\{ -\frac{1}{2}(\frac{y - \mu_j}{\sigma_j})^2\} \]
Consider a likelihood ratio rule
\[ \begin{aligned} \Lambda &= \frac{\text{likelihood of y from pop 1}}{\text{likelihood of y from pop 2}} \\ &= \frac{f_1(y)}{f_2(y)} \\ &= \frac{\sigma_2}{\sigma_1} \exp\{-\frac{1}{2}[(\frac{y - \mu_1}{\sigma_1})^2- (\frac{y - \mu_2}{\sigma_2})^2] \} \end{aligned} \]
Hence, we classify into
pop 1 if \(\Lambda >1\)
pop 2 if \(\Lambda <1\)
for ties, flip a coin
Another way to think:
we classify into population 1 if the “standardized distance” of y from \(\mu_1\) is less than the “standardized distance” of y from \(\mu_2\) which is referred to as a quadratic discriminant rule .
(Significant simplification occurs in th special case where \(\sigma_1 = \sigma_2 = \sigma^2\) )
Thus, we classify into population 1 if
\[ (y - \mu_2)^2 > (y - \mu_1)^2 \]
\[ |y- \mu_2| > |y - \mu_1| \]
\[ -2 \log (\Lambda) = -2y \frac{(\mu_1 - \mu_2)}{\sigma^2} + \frac{(\mu_1^2 - \mu_2^2)}{\sigma^2} = \beta y + \alpha \]
Thus, we classify into population 1 if this is less than 0.
Discriminant classification rule is linear in y in this case.
22.4.1.1 Multivariate Expansion
Suppose that there are 2 populations
\(N_p(\mathbf{\mu}_1, \mathbf{\Sigma}_1)\)
\(N_p(\mathbf{\mu}_2, \mathbf{\Sigma}_2)\)
\[ \begin{aligned} -2 \log(\frac{f_1 (\mathbf{x})}{f_2 (\mathbf{x})}) &= \log|\mathbf{\Sigma}_1| + (\mathbf{x} - \mathbf{\mu}_1)' \mathbf{\Sigma}^{-1}_1 (\mathbf{x} - \mathbf{\mu}_1) \\ &- [\log|\mathbf{\Sigma}_2|+ (\mathbf{x} - \mathbf{\mu}_2)' \mathbf{\Sigma}^{-1}_2 (\mathbf{x} - \mathbf{\mu}_2) ] \end{aligned} \]
Again, we classify into population 1 if this is less than 0, otherwise, population 2. And like the univariate case with non-equal variances, this is a quadratic discriminant rule.
And if the covariance matrices are equal: \(\mathbf{\Sigma}_1 = \mathbf{\Sigma}_2 = \mathbf{\Sigma}_1\) classify into population 1 if
\[ (\mathbf{\mu}_1 - \mathbf{\mu}_2)' \mathbf{\Sigma}^{-1}\mathbf{x} - \frac{1}{2} (\mathbf{\mu}_1 - \mathbf{\mu}_2)' \mathbf{\Sigma}^{-1} (\mathbf{\mu}_1 - \mathbf{\mu}_2) \ge 0 \]
This linear discriminant rule is also referred to as Fisher’s linear discriminant function
By assuming the covariance matrices are equal, we assume that the shape and orientation fo the two populations must be the same (which can be a strong restriction)
In other words, for each variable, it can have different mean but the same variance.
- Note: LDA Bayes decision boundary is linear. Hence, quadratic decision boundary might lead to better classification. Moreover, the assumption of same variance/covariance matrix across all classes for Gaussian densities imposes the linear rule, if we allow the predictors in each class to follow MVN distribution with class-specific mean vectors and variance/covariance matrices, then it is Quadratic Discriminant Analysis. But then, you will have more parameters to estimate (which gives more flexibility than LDA) at the cost of more variance (bias -variance tradeoff).
When \(\mathbf{\mu}_1, \mathbf{\mu}_2, \mathbf{\Sigma}\) are known, the probability of misclassification can be determined:
\[ \begin{aligned} P(2|1) &= P(\text{calssify into pop 2| x is from pop 1}) \\ &= P((\mathbf{\mu}_1 - \mathbf{\mu}_2)' \mathbf{\Sigma}^{-1} \mathbf{x} \le \frac{1}{2} (\mathbf{\mu}_1 - \mathbf{\mu}_2)' \mathbf{\Sigma}^{-1} (\mathbf{\mu}_1 - \mathbf{\mu}_2)|\mathbf{x} \sim N(\mu_1, \mathbf{\Sigma}) \\ &= \Phi(-\frac{1}{2} \delta) \end{aligned} \]
\(\delta^2 = (\mathbf{\mu}_1 - \mathbf{\mu}_2)' \mathbf{\Sigma}^{-1} (\mathbf{\mu}_1 - \mathbf{\mu}_2)\)
\(\Phi\) is the standard normal CDF
Suppose there are \(h\) possible populations, which are distributed as \(N_p (\mathbf{\mu}_p, \mathbf{\Sigma})\) . Then, the maximum likelihood (linear) discriminant rule allocates \(\mathbf{y}\) to population j where j minimizes the squared Mahalanobis distance
\[ (\mathbf{y} - \mathbf{\mu}_j)' \mathbf{\Sigma}^{-1} (\mathbf{y} - \mathbf{\mu}_j) \]
22.4.1.2 Bayes Discriminant Rules
If we know that population j has prior probabilities \(\pi_j\) (assume \(\pi_j >0\) ) we can form the Bayes discriminant rule.
This rule allocates an observation \(\mathbf{y}\) to the population for which \(\pi_j f_j (\mathbf{y})\) is maximized.
- Maximum likelihood discriminant rule is a special case of the Bayes discriminant rule , where it sets all the \(\pi_j = 1/h\)
Optimal Properties of Bayes Discriminant Rules
let \(p_{ii}\) be the probability of correctly assigning an observation from population i
then one rule (with probabilities \(p_{ii}\) ) is as good as another rule (with probabilities \(p_{ii}'\) ) if \(p_{ii} \ge p_{ii}'\) for all \(i = 1,\dots, h\)
The first rule is better than the alternative if \(p_{ii} > p_{ii}'\) for at least one i.
A rule for which there is no better alternative is called admissible
Bayes Discriminant Rules are admissible
If we utilized prior probabilities, then we can form the posterior probability of a correct allocation, \(\sum_{i=1}^h \pi_i p_{ii}\)
Bayes Discriminant Rules have the largest possible posterior probability of correct allocation with respect to the prior
These properties show that Bayes Discriminant rule is our best approach .
Unequal Cost
We want to consider the cost misallocation
- Define \(c_{ij}\) to be the cost associated with allocation a member of population j to population i.
Assume that
\(c_{ij} >0\) for all \(i \neq j\)
\(c_{ij} = 0\) if \(i = j\)
We could determine the expected amount of loss for an observation allocated to population i as \(\sum_j c_{ij} p_{ij}\) where the \(p_{ij}s\) are the probabilities of allocating an observation from population j into population i
We want to minimize the amount of loss expected for our rule. Using a Bayes Discrimination, allocate \(\mathbf{y}\) to the population j which minimizes \(\sum_{k \neq j} c_{ij} \pi_k f_k(\mathbf{y})\)
We could assign equal probabilities to each group and get a maximum likelihood type rule. here, we would allocate \(\mathbf{y}\) to population j which minimizes \(\sum_{k \neq j}c_{jk} f_k(\mathbf{y})\)
Two binomial populations, each of size 10, with probabilities \(p_1 = .5\) and \(p_2 = .7\)
And the probability of being in the first population is .9
However, suppose the cost of inappropriately allocating into the first population is 1 and the cost of incorrectly allocating into the second population is 5.
In this case, we pick population 1 over population 2
In general, we consider two regions, \(R_1\) and \(R_2\) associated with population 1 and 2:
\[ R_1: \frac{f_1 (\mathbf{x})}{f_2 (\mathbf{x})} \ge \frac{c_{12} \pi_2}{c_{21} \pi_1} \]
\[ R_2: \frac{f_1 (\mathbf{x})}{f_2 (\mathbf{x})} < \frac{c_{12} \pi_2}{c_{21} \pi_1} \]
where \(c_{12}\) is the cost of assigning a member of population 2 to population 1.
22.4.1.3 Discrimination Under Estimation
Suppose we know the form of the distributions for populations of interests, but we still have to estimate the parameters.
we know the distributions are multivariate normal, but we have to estimate the means and variances
The maximum likelihood discriminant rule allocates an observation \(\mathbf{y}\) to population j when j maximizes the function
\[ f_j (\mathbf{y} |\hat{\theta}) \]
where \(\hat{\theta}\) are the maximum likelihood estimates of the unknown parameters
For instance, we have 2 multivariate normal populations with distinct means, but common variance covariance matrix
MLEs for \(\mathbf{\mu}_1\) and \(\mathbf{\mu}_2\) are \(\mathbf{\bar{y}}_1\) and \(\mathbf{\bar{y}}_2\) and common \(\mathbf{\Sigma}\) is \(\mathbf{S}\) .
Thus, an estimated discriminant rule could be formed by substituting these sample values for the population values
22.4.1.4 Native Bayes
The challenge with classification using Bayes’ is that we don’t know the (true) densities, \(f_k, k = 1, \dots, K\) , while LDA and QDA make strong multivariate normality assumptions to deal with this.
Naive Bayes makes only one assumption: within the k-th class, the p predictors are independent (i.e,, for \(k = 1,\dots, K\)
\[ f_k(x) = f_{k1}(x_1) \times f_{k2}(x_2) \times \dots \times f_{kp}(x_p) \]
where \(f_{kj}\) is the density function of the j-th predictor among observation in the k-th class.
This assumption allows the use of joint distribution without the need to account for dependence between observations. However, this (native) assumption can be unrealistic, but still works well in cases where the number of sample (n) is not large relative to the number of features (p).
With this assumption, we have
\[ P(Y=k|X=x) = \frac{\pi_k \times f_{k1}(x_1) \times \dots \times f_{kp}(x_p)}{\sum_{l=1}^K \pi_l \times f_{l1}(x_1)\times \dots f_{lp}(x_p)} \]
we only need to estimate the one-dimensional density function \(f_{kj}\) with either of these approaches:
When \(X_j\) is quantitative, assume it has a univariate normal distribution (with independence): \(X_j | Y = k \sim N(\mu_{jk}, \sigma^2_{jk})\) which is more restrictive than QDA because it assumes predictors are independent (e.g., a diagonal covariance matrix)
When \(X_j\) is quantitative, use a kernel density estimator Kernel Methods ; which is a smoothed histogram
When \(X_j\) is qualitative, we count the promotion of training observations for the j-th predictor corresponding to each class.
22.4.1.5 Comparison of Classification Methods
Assuming we have K classes and K is the baseline from (James , Witten, Hastie, and Tibshirani book)
Comparing the log odds relative to the K class
22.4.1.5.1 Logistic Regression
\[ \log(\frac{P(Y=k|X = x)}{P(Y = K| X = x)}) = \beta_{k0} + \sum_{j=1}^p \beta_{kj}x_j \]
22.4.1.5.2 LDA
\[ \log(\frac{P(Y = k | X = x)}{P(Y = K | X = x)} = a_k + \sum_{j=1}^p b_{kj} x_j \]
where \(a_k\) and \(b_{kj}\) are functions of \(\pi_k, \pi_K, \mu_k , \mu_K, \mathbf{\Sigma}\)
Similar to logistic regression, LDA assumes the log odds is linear in \(x\)
Even though they look like having the same form, the parameters in logistic regression are estimated by MLE, where as LDA linear parameters are specified by the prior and normal distributions
We expect LDA to outperform logistic regression when the normality assumption (approximately) holds, and logistic regression to perform better when it does not
22.4.1.5.3 QDA
\[ \log(\frac{P(Y=k|X=x}{P(Y=K | X = x}) = a_k + \sum_{j=1}^{p}b_{kj}x_{j} + \sum_{j=1}^p \sum_{l=1}^p c_{kjl}x_j x_l \]
where \(a_k, b_{kj}, c_{kjl}\) are functions \(\pi_k , \pi_K, \mu_k, \mu_K ,\mathbf{\Sigma}_k, \mathbf{\Sigma}_K\)
22.4.1.5.4 Naive Bayes
\[ \log (\frac{P(Y = k | X = x)}{P(Y = K | X = x}) = a_k + \sum_{j=1}^p g_{kj} (x_j) \]
where \(a_k = \log (\pi_k / \pi_K)\) and \(g_{kj}(x_j) = \log(\frac{f_{kj}(x_j)}{f_{Kj}(x_j)})\) which is the form of generalized additive model
22.4.1.5.5 Summary
LDA is a special case of QDA
LDA is robust when it comes to high dimensions
Any classifier with a linear decision boundary is a special case of naive Bayes with \(g_{kj}(x_j) = b_{kj} x_j\) , which means LDA is a special case of naive Bayes. LDA assumes that the features are normally distributed with a common within-class covariance matrix, and naive Bayes assumes independence of the features.
Naive bayes is also a special case of LDA with \(\mathbf{\Sigma}\) restricted to a diagonal matrix with diagonals, \(\sigma^2\) (another notation \(diag (\mathbf{\Sigma})\) ) assuming \(f_{kj}(x_j) = N(\mu_{kj}, \sigma^2_j)\)
QDA and naive Bayes are not special case of each other. In principal,e naive Bayes can produce a more flexible fit by the choice of \(g_{kj}(x_j)\) , but it’s restricted to only purely additive fit, but QDA includes multiplicative terms of the form \(c_{kjl}x_j x_l\)
None of these methods uniformly dominates the others: the choice of method depends on the true distribution of the predictors in each of the K classes, n and p (i.e., related to the bias-variance tradeoff).
Compare to the non-parametric method (KNN)
KNN would outperform both LDA and logistic regression when the decision boundary is highly nonlinear, but can’t say which predictors are most important, and requires many observations
KNN is also limited in high-dimensions due to the curse of dimensionality
Since QDA is a special type of nonlinear decision boundary (quadratic), it can be considered as a compromise between the linear methods and KNN classification. QDA can have fewer training observations than KNN but not as flexible.
From simulation:
- like linear regression, we can also introduce flexibility by including transformed features \(\sqrt{X}, X^2, X^3\)
22.4.2 Probabilities of Misclassification
When the distribution are exactly known, we can determine the misclassification probabilities exactly. however, when we need to estimate the population parameters, we have to estimate the probability of misclassification
Naive method
Plugging the parameters estimates into the form for the misclassification probabilities results to derive at the estimates of the misclassification probability.
But this will tend to be optimistic when the number of samples in one or more populations is small.
Resubstitution method
Use the proportion of the samples from population i that would be allocated to another population as an estimate of the misclassification probability
But also optimistic when the number of samples is small
Jack-knife estimates:
The above two methods use observation to estimate both parameters and also misclassification probabilities based upon the discriminant rule
Alternatively, we determine the discriminant rule based upon all of the data except the k-th observation from the j-th population
then, determine if the k-th observation would be misclassified under this rule
perform this process for all \(n_j\) observation in population j . An estimate fo the misclassification probability would be the fraction of \(n_j\) observations which were misclassified
repeat the process for other \(i \neq j\) populations
This method is more reliable than the others, but also computationally intensive
Cross-Validation
Consider the group-specific densities \(f_j (\mathbf{x})\) for multivariate vector \(\mathbf{x}\) .
Assume equal misclassifications costs, the Bayes classification probability of \(\mathbf{x}\) belonging to the j-th population is
\[ p(j |\mathbf{x}) = \frac{\pi_j f_j (\mathbf{x})}{\sum_{k=1}^h \pi_k f_k (\mathbf{x})} \]
\(j = 1,\dots, h\)
where there are \(h\) possible groups.
We then classify into the group for which this probability of membership is largest
Alternatively, we can write this in terms of a generalized squared distance formation
\[ D_j^2 (\mathbf{x}) = d_j^2 (\mathbf{x})+ g_1(j) + g_2 (j) \]
\(d_j^2(\mathbf{x}) = (\mathbf{x} - \mathbf{\mu}_j)' \mathbf{V}_j^{-1} (\mathbf{x} - \mathbf{\mu}_j)\) is the squared Mahalanobis distance from \(\mathbf{x}\) to the centroid of group j, and
\(\mathbf{V}_j = \mathbf{S}_j\) if the within group covariance matrices are not equal
\(\mathbf{V}_j = \mathbf{S}_p\) if a pooled covariance estimate is appropriate
\[ g_1(j) = \begin{cases} \ln |\mathbf{S}_j| & \text{within group covariances are not equal} \\ 0 & \text{pooled covariance} \end{cases} \]
\[ g_2(j) = \begin{cases} -2 \ln \pi_j & \text{prior probabilities are not equal} \\ 0 & \text{prior probabilities are equal} \end{cases} \]
then, the posterior probability of belonging to group j is
\[ p(j| \mathbf{x}) = \frac{\exp(-.5 D_j^2(\mathbf{x}))}{\sum_{k=1}^h \exp(-.5 D^2_k (\mathbf{x}))} \]
where \(j = 1,\dots , h\)
and \(\mathbf{x}\) is classified into group j if \(p(j | \mathbf{x})\) is largest for \(j = 1,\dots,h\) (or, \(D_j^2(\mathbf{x})\) is smallest).
22.4.2.1 Assessing Classification Performance
For binary classification, confusion matrix
and table 4.6 from ( James et al. 2013 )
ROC curve (receiver Operating Characteristics) is a graphical comparison between sensitivity (true positive) and specificity ( = 1 - false positive)
y-axis = true positive rate
x-axis = false positive rate
as we change the threshold rate for classifying an observation as from 0 to 1
AUC (area under the ROC) ideally would equal to 1, a bad classifier would have AUC = 0.5 (pure chance)
22.4.3 Unknown Populations/ Nonparametric Discrimination
When your multivariate data are not Gaussian, or known distributional form at all, we can use the following methods
22.4.3.1 Kernel Methods
We approximate \(f_j (\mathbf{x})\) by a kernel density estimate
\[ \hat{f}_j(\mathbf{x}) = \frac{1}{n_j} \sum_{i = 1}^{n_j} K_j (\mathbf{x} - \mathbf{x}_i) \]
\(K_j (.)\) is a kernel function satisfying \(\int K_j(\mathbf{z})d\mathbf{z} =1\)
\(\mathbf{x}_i\) , \(i = 1,\dots , n_j\) is a random sample from the j-th population.
Thus, after finding \(\hat{f}_j (\mathbf{x})\) for each of the \(h\) populations, the posterior probability of group membership is
\[ p(j |\mathbf{x}) = \frac{\pi_j \hat{f}_j (\mathbf{x})}{\sum_{k-1}^h \pi_k \hat{f}_k (\mathbf{x})} \]
where \(j = 1,\dots, h\)
There are different choices for the kernel function:
Epanechnikov
We these kernels, we have to pick the “radius” (or variance, width, window width, bandwidth) of the kernel, which is a smoothing parameter (the larger the radius, the more smooth the kernel estimate of the density).
To select the smoothness parameter, we can use the following method
If we believe the populations were close to multivariate normal, then
\[ R = (\frac{4/(2p+1)}{n_j})^{1/(p+1} \]
But since we do not know for sure, we might choose several different values and select one that vies the best out of sample or cross-validation discrimination.
Moreover, you also have to decide whether to use different kernel smoothness for different populations, which is similar to the individual and pooled covariances in the classical methodology.
22.4.3.2 Nearest Neighbor Methods
The nearest neighbor (also known as k-nearest neighbor) method performs the classification of a new observation vector based on the group membership of its nearest neighbors. In practice, we find
\[ d_{ij}^2 (\mathbf{x}, \mathbf{x}_i) = (\mathbf{x}, \mathbf{x}_i) V_j^{-1}(\mathbf{x}, \mathbf{x}_i) \]
which is the distance between the vector \(\mathbf{x}\) and the \(i\) -th observation in group \(j\)
We consider different choices for \(\mathbf{V}_j\)
\[ \begin{aligned} \mathbf{V}_j &= \mathbf{S}_p \\ \mathbf{V}_j &= \mathbf{S}_j \\ \mathbf{V}_j &= \mathbf{I} \\ \mathbf{V}_j &= diag (\mathbf{S}_p) \end{aligned} \]
We find the \(k\) observations that are closest to \(\mathbf{x}\) (where users pick \(k\) ). Then we classify into the most common population, weighted by the prior.
22.4.3.3 Modern Discriminant Methods
Logistic regression (with or without random effects) is a flexible model-based procedure for classification between two populations.
The extension of logistic regression to the multi-group setting is polychotomous logistic regression (or, mulinomial regression).
The machine learning and pattern recognition are growing with strong focus on nonlinear discriminant analysis methods such as:
radial basis function networks
support vector machines
multiplayer perceptrons (neural networks)
The general framework
\[ g_j (\mathbf{x}) = \sum_{l = 1}^m w_{jl}\phi_l (\mathbf{x}; \mathbf{\theta}_l) + w_{j0} \]
\(m\) nonlinear basis functions \(\phi_l\) , each of which has \(n_m\) parameters given by \(\theta_l = \{ \theta_{lk}: k = 1, \dots , n_m \}\)
We assign \(\mathbf{x}\) to the \(j\) -th population if \(g_j(\mathbf{x})\) is the maximum for all \(j = 1,\dots, h\)
Development usually focuses on the choice and estimation of the basis functions, \(\phi_l\) and the estimation of the weights \(w_{jl}\)
More details can be found ( Webb, Copsey, and Cawley 2011 )
22.4.4 Application
22.4.4.1 lda.
Default prior is proportional to sample size and lda and qda do not fit a constant or intercept term
LDA didn’t do well on both within sample and out-of-sample data.
22.4.4.2 QDA
22.4.4.3 knn.
knn uses design matrices of the features.
22.4.4.4 Stepwise
Stepwise discriminant analysis using the stepclass in function in the klaR package.

22.4.4.5 PCA with Discriminant Analysis
we can use both PCA for dimension reduction in discriminant analysis

- Introduction To MultiVariate Testing: A Complete Guide (2023)

Multivariate testing, also known as multivariant testing, is a method of experimenting with multiple variables on a website or mobile app to determine which variation performs the best. The goal is to identify the combination of variations that drive a desired outcome, like more conversions or higher revenue.
Multivariate testing is extremely valuable for making data-driven decisions about changes to your site or app. Testing multiple variables at once allows you to understand how different elements interact and impact each other. This provides deeper insights than changing one variable at a time. By identifying the optimal combination of variables, you can significantly improve key metrics like conversion rates.
In this article, you will learn what multivariate testing is, why it is important, how to design and run a multivariate test, and how to analyze and interpret the results. With this knowledge, you will be equipped to leverage multivariate testing to make smart decisions that improve your website’s or app’s performance.
Table of Contents
What is multivariate testing.
Multivariate testing, sometimes called multivariant testing, is a method of experimenting with multiple variables on a website or mobile app simultaneously. The goal is to determine which combination of variations performs the best to drive a desired outcome.
In a multivariate test, you select multiple elements on a page to vary at the same time. For example, you may test different headlines, call-to-action button colours, and image placements, and form layouts together in a single experiment. You can create different variation combinations and serve them randomly to visitors to see which performs the best.
This differs from A/B testing, where you only test two variants against each other, changing just one element at a time. With multivariate testing, you can test the interactions between different page elements and how changing multiple variables together impacts success metrics.
The key advantage of multivariate testing is that it provides a deeper understanding of the variables that influence performance. By testing multiple changes simultaneously, you can identify the optimal combination that drives the highest conversion rates, revenue, or other goals.
Importance Of Multivariate Testing
1. helps marketers find the best combination of elements.
Multivariate testing is invaluable for marketers looking to optimize ad campaigns across multiple dimensions.
Key variables that can be tested together include ad messaging, imagery, calls-to-action, audience targeting, placement, timing, and more.
By creating different combinations of these campaign elements and exposing audiences to them in a controlled experiment, marketers gain data-driven insights about what works best.
For example, testing different ad images with various types of copy and calls-to-action can uncover what imagery and text resonates most with each target customer segment.
These insights allow marketers to fine-tune campaign elements and allocate a budget toward what drives the highest conversion rates and ROI.
2. Helps in improving user experience
Multivariate testing is critical for UX/UI professionals looking to elevate user engagement, satisfaction, and conversions.
By testing different page layouts, UI flows, microcopy, visuals, and other design elements together, UX researchers gain a nuanced understanding of what combination of variables optimizes the user experience.
Testing can uncover how page layout impacts user attention, how microcopy influences behaviour, how visual cues affect perceptions, and how flows drive conversions.
With these multivariate insights, designers can craft targeted solutions. For example, shortening forms, enhancing navigational cues, simplifying instructions, and highlighting calls-to-action can together transform bounce rates.
Multivariate testing empirically models the complex relationships between design variables, equipping UX teams to build interfaces with the optimal balance of elements to delight users.
3. Informs Data-Driven Decision Making
The overarching benefit of multivariate testing is enabling data-driven decision-making. Rather than rely on hypotheses and guesses, multivariate testing allows for different ideas and combinations to be tried out and objectively evaluated.
By gathering performance data on multiple variables under live conditions, teams get clear validation about what works. These evidence-based insights justify meaningful investments of time and budget into the ideas that drive metrics like conversion rates and revenue.
Stakeholders can align around decisions backed by sound multivariate testing data.
Additionally, the insights uncovered allow for continual optimization over time as new concepts are tested against the current best performers. With multivariate testing, website and mobile app owners reap the benefits of innovation grounded in empirical facts rather than opinions.
4. Reduces Risk
Implementing changes without properly testing them first carries substantial risk.
Rolling out untested website, app or marketing changes can lead to wasted development time and resources if they fail to improve metrics.
Additionally, unvalidated changes run the risk of accidentally alienating or frustrating users by altering experiences in unvetted ways. Without seeing how changes impact performance, teams run the risk of making choices based on assumptions instead of data.
Multivariate testing minimizes risks by validating proposed changes empirically first. Testing changes simultaneously provides insights into interactions between variables that may produce unexpected results.
This allows teams to catch issues and correct course prior to rolling changes out fully. By taking the guesswork out of decision-making, multivariate testing builds internal stakeholder alignment around choices grounded in performance data.
Resources can be invested more confidently into proven solutions. In essence, multivariate testing reduces the risks associated with unvalidated changes – ensuring efforts remain focused on what will best serve users and move the business metrics forward based on evidence.
Preparing For Multivariate Testing
1. clearly define the testing goal.
Defining a clear, measurable goal is crucial for focusing the multivariate test and evaluating results. Goals should directly tie to key business or user experience metrics that stakeholders want to improve.
For an e-commerce website, common goals are increasing conversion rate, average order value, or overall revenue per visitor. For user engagement, goals could be reducing bounce rate, increasing pages per session, or boosting return visits.
Avoid vague or fuzzy goals like “improve performance” and instead precisely define the specific metric you want to optimize. Having a concrete, well-defined goal metric allows you to properly assess the significance of results.
It also enables accurately calculating projected impacts to the business, like the expected revenue lift from a 2% conversion rate increase.
A focused, quantifiable goal aligns the test around outcomes that connect to broader business priorities, which aids in gaining stakeholder buy-in. Overall, dedicating time upfront to define a clear testing goal focused on moving the needle for a key metric sets the experiment up for delivering tangible business value.
2. Select the Most Impactful Variables to Test
The specific page elements and experiences selected as test variables should have a clear, logical relationship to the defined goal metric.
For an e-commerce website, key variables likely include product page content and design, as these are what visitors directly interact with when making a purchase decision.
Consider elements website visitors need to view or use in order to convert, like headlines, product descriptions, images, pricing, specifications, and calls-to-action.
Prioritize testing variables that exist within the conversion path itself, such as checkout flows. The overarching aim is to identify and test the elements on a page that are highly likely to influence visitor behaviour related to the goal metric if optimized.
Document specific hypotheses about exactly how changing each selected variable may affect the goal, whether it be enhanced trust in a product through more detailed descriptions or higher perceived value through more premium images. Limit testing variables to the highest-impact areas on a page or within a user flow to maintain focus on changes that can meaningfully move the goal metric.
3. Design High-Quality, Balanced Variations
For each variable selected for testing, design well-crafted variations that will generate statistically meaningful data. Avoid extremes such as comparing a highly polished, premium variation against a very low-quality, distracting variation.
The different variations should be realistic options for a business to consider implementing.
Test 3-5 variants for each element to achieve balance, such as testing different headlines of similar length, formatting, and clarity. When testing visuals, ensure styles and production quality are comparable across variants.
The aim is to isolate the impact of individual variations, rather than having one variation skewing results through extremely high or low quality. Testing balanced, high-quality variations enhances the statistical validity of results and provides insights that can inform impactful changes.
4. Map Logical Variable Combinations
With multiple variables, determine reasonable combinations to test together in order to assess interaction effects between elements. Seek to test combinations where variables are likely to relate and collectively influence user responses and behaviour.
For example, pair aligned content messaging with related images or videos. Limit the total number of combinations to maintain statistical power as combinations divide overall test traffic.
To maximize test efficiency, prioritize mapping combinations between variables with the highest potential individual impact. Additionally, coordinate variation details so that combinations make sense when paired together and maintain a logical user flow. Testing logical, coordinated variable combinations optimizes learning while maintaining a streamlined, engaging experience.
How To Conduct A MultiVariate Test
Step-by-step guide to designing test versions.
- Create a test variation matrix detailing every possible combination of variables and versions. Map these systematically to visualize the scope. A clear matrix prevents gaps in test design.
- Design wireframes or mockups for each variation combination. Use clear naming like “Var A – Headline 1, Image 2, CTA 3”. Visualizing combinations enables refinement. Create multiple mockups to compare versions.
- Manage variations in modular templates, like separate files for each headline or image. This makes it easy to mix and match variations within combinations. Maintain organized structures for efficiency.
- Nest variable templates within master designs. Enable quick swapping between options. Set up folders or libraries to access all variations on demand. Organization is key with multiple assets.
- Do holistic reviews of combinations against brand style guides. Check colors, fonts, tones for consistency. Refine combinations to adhere to branding. Present combinations professionally and logically.
- Create a usage matrix detailing which combinations will be shown to each visitor segment. Coordinate this allocation with dev teams. Methodically map combinations to visitors.
- Establish naming conventions for implementation like “button-version-A” and “image-option-B”. Ensure naming protocols are clear and consistent across platforms and teams.
- Centralize approved assets and materials for each combination in a single well-organized repository. Enable easy access for design, engineering, and analysis.
- Conduct comprehensive QA testing of fully assembled variations before launch. Check flows, formatting, functionality, and errors. Identify and resolve any issues.
- Verify analytics are tracking key metrics for each combination to support data analysis. Implement tagging like URLs with version identifiers.
Instructions On How To Deploy These Test Versions On The Platform
- Collaborate extensively with engineering teams throughout implementation. Align on naming conventions, tagging protocols, redirects, and requirements early on. Provide assets and documentation in an organized, clear manner. Have regular check-ins to track progress and address issues proactively.
- For websites, build out a flexible test page template that dynamically pulls in the appropriate variation assets for each visitor based on segmentation in the backend testing tool. Take advantage of features like Optimizely’s custom snippets. Use clear CSS naming conventions like “button-version-A” to cleanly switch variations.
- For mobile apps, create separate app versions for each variation combination in the code and submit these as distinct releases to app stores. Configure the testing tool to route various user segments to specific app releases. Document app release details and allocate enough testing budget.
- Set up seamless redirects to map visitors to the appropriately assigned test variation page or app without disruption. Insert identifiers like ?var=B into URLs and analytics to denote variations. Confirm redirects work across devices.
- Implement event tracking and analytics uniformly across all variations to capture interaction data. Pipe this data directly into the testing tool’s dashboards for centralized analysis. Tag events like “button-click-varC” to identify behaviours by variation.
- Perform extensive quality assurance testing on each implemented variation across all major browsers, devices, and use cases. Verify functionality, design, numbering, and flows. Identify and rapidly resolve defects pre-launch. Sign off QA verbally with teams.
- Start with an initial soft launch of lower traffic to confirm a smooth rollout before ramping up. Monitor metrics closely across variations and act fast if issues emerge. Have a rollback plan if needed.
- Maintain full documentation covering implementation details, naming conventions, redirects, tags, URLs, assets used per variation, app release info, QA outcomes, and more. Thorough documentation improves analysis.
How To Collect and Record Data
- Define core metrics upfront that align with test goals and business priorities. For example, focus on conversion rate, average order value, new signups, or engagement score. Limit metrics to critical ones to streamline analysis.
- Implement comprehensive event tracking across all variations to capture granular user behaviours. Track clicks, hovers, page scrolling, videos watched, content downloads, engagement time and more. This reveals detailed user journeys.
- Pipe all data directly into the centralized test platform dashboard for unified access and reporting. Eliminate manual compiling of metrics across tools for streamlined analysis.
- Follow strict tagging protocols attributing events and attributes to each variation like “button-click-varA”. This structures data to compare performance. Use naming conventions consistently across teams.
- Build scheduled reports on key metrics and create real-time dashboards of ongoing performance. Monitor dashboards daily to catch issues quickly. Establish alert thresholds linked to goals.
- Insert variation identifiers into page URLs like ?var=B to facilitate segmentation of metrics by version during analysis. Document conventions thoroughly.
- Conduct recurring data audits and statistical checks to validate completeness, accuracy, and consistency across sources. Identify any gaps or anomalies requiring correction.
- Meticulously document all data collection implementations, metrics definitions, calculation logic, dependencies, protocols, and locations. Detailed documentation prevents errors.
- Securely store raw data with redundancy, including dashboard exports, spreadsheets, and analytics exports. Process into analysis-ready formats using ETL tools or scripts.
Interpreting The Results Of Multivariate Testing
Key metrics.
- Focus analysis on the specific metrics pre-defined upfront that align directly with core goals, such as conversion rate, average order value, subscriber retention rate, etc. Compare the performance of each variation against control on these key indicators.
- Supplement top-line metrics with detailed user behaviour data like click-through rates on buttons/links, scroll depth, video engagement, and page views per session. This reveals how users flow through variations.
- Look at metrics segmented by meaningful user cohorts like new vs returning visitors or geography. Any variance in responses to variations?
- Consider metrics in the context of external factors like seasonality, releases, and outages. How did confounding events potentially influence the environment?
Analyzing Results
- Leverage the multivariate testing tool’s statistical significance testing to validate metric differences exceeding 95%+ confidence as the minimum bar for identifying winners.
- Calculate lift vs the control for each variation’s metrics. Is the percentage increase large enough to meaningfully impact goals? Set lift effect size minimums.
- Visualize performance trends over time, side-by-side comparisons, and variation interaction effects through charts, graphs and heatmaps. Spot patterns.
- Review detailed user flows and behaviour data. Did combinations drive traffic to key pages as expected? Were unexpected interaction effects uncovered?
- Factor in qualitative assessments of ease of use, engagement, and branding fit. This contextualizes data.
Making Decisions
- Recommend implementation of variations with statistically significant lifts on key metrics exceeding effect size minimums. Lifts should meaningfully impact goals.
- Be cautious of acting on small metric differences that fall within typical statistical noise levels. Seek directional validation through further testing.
- Document insights, projected impact on goals, implementation plan, optimization roadmap. Share results cross-functionally.
- Avoid over-optimizing for one metric without considering holistic experience. Evaluate strategic brand and UX alignment.
- Take an iterative approach optimizing through continuous incremental gains over time vs sporadic big swings.
Common Challenges and Mistakes In Multivariate Testing
- Running many variations spreads traffic too thinly across underpowered branches, preventing statistically significant clear winners from emerging above normal data fluctuations.
Solution: Carefully limit the number of variations and combinations tested based on available traffic volumes to maintain adequate statistical power in each branch.
- Without predetermining minimum statistical confidence and effect size thresholds upfront, normal data variance over time may be incorrectly interpreted as wins rather than inconclusive results.
Solution: Define minimum 95% statistical confidence and minimum effect size thresholds before testing that variants must exceed to be considered winners and move forward.
- Limiting data collection and analysis to just aggregate metrics like conversion rates provides no qualitative insights into changes in detailed user behaviours across variations.
Solution: Implement comprehensive event tracking and analysis across every variation to capture detailed user interaction data that reveals insights behind the metric differences.
- Constraining experimentation to just the homepage or landing page changes in isolation loses sight of critical cross-touchpoint effects across the entire user journey that shape outcomes.
Solution: Identify multiple significant experimentation points across the full user experience journey to account for cross-touchpoint interaction effects.
- Inadequate quality assurance testing of implementations often overlooks discrepancies between variations such as broken flows, error states, tracking issues or missing data that completely invalidate any attempted data analysis.
Solution: Perform extensive QA testing on each implemented variation to verify that expected behavior matches designs before launching live traffic to ensure data accuracy.
- Optimizing for isolated metrics in a vacuum can degrade the holistic user experience and brand alignment when evaluated from a broader strategic perspective.
Solution: Maintain a holistic perspective aligning proposed optimizations to overarching brand strategy and goals versus isolated metrics in isolation.
- Prematurely committing resources to implement initial data trends risks acting on normal data fluctuations before collecting sufficient representative data over extended time periods to validate results through repetition.
Solution: Collect adequate sample sizes over meaningful time frames and replicate results before committing resources.
Frequently Asked Questions About Multivariate Testing
1. What is multivariate testing?
Multivariate testing is a method of experimentation where multiple variables on a page are tested simultaneously to determine the optimal combination that achieves the desired outcome. It allows the testing of variable interactions.
2. How is it different from A/B testing?
A/B testing evaluates one variable at a time. Multivariate testing combines multiple variables into different variations for a single experiment. This reveals how variables impact each other.
3. What are some key benefits of multivariate testing?
- Test combinations of changes together vs. incremental one-variable tests
- Uncover interactions between variables
- Reduce the number of required tests and speed up optimization
- Gain greater insights from fewer versions and samples
- Identify positive and negative variable correlations
4. What metrics should I track?
Focus on key performance indicators tied to goals, like conversion rates, revenue, and lead gen form submissions. Supplement with detailed user behaviour data.
5. How many variations should I test?
Limit based on available traffic so each variation has a large enough sample for statistical significance. Too many underpowered branches produce unclear results.
6. How long should I run a test?
Run tests long enough to collect sufficient statistically significant samples. Test duration depends on variables like traffic levels. Let the data and tool indicate readiness.
7. What mistakes should I avoid?
Avoid too many variations, acting on insufficient data, over-optimizing for one metric, or failing to QA implementations before launch.
- A/B Testing
- Company News
- Conversion Rate Optimisation
- CRO Tools and Resources
- Experimentation Articles
- Google Analytics
- Usability Testing
- User Research
- How To Use Surveys and Feedback For CRO
- The ROI of User Testing: Measuring Success
- CRO for Local Businesses: Driving Foot Traffic and Sales
- How To Use Customer Feedback For Website Optimization
- User Research Best Practices for E-commerce Websites
- What Is A CRO Test? Definition, Types & Examples
- The surprising truth about Amazon and Booking.com's culture of experimentation
- Top 19 Best CRO Books (recommended by experts)
- Key Metrics In CRO: What To Measure and Why
- Open-Ended Questions - A Complete Guide
- Post-Launch User Testing: Continuous Improvement Strategies
- Qualitative vs Quantitative Research: When to Use Each
- Psychological Principles in CRO: Nudging Users to Convert
- 8 ChatGPT prompting Tips for CRO and Experimentation

Is your CRO programme delivering the impact you hoped for ?
Benchmark your CRO now for immediate, free report packed with ACTIONABLE insights you and your team can implement today to increase conversion.
Takes only two minutes
If your CRO programme is not delivering the highest ROI of all of your marketing spend, then we should talk.
Before you go...

“The world’s leading companies are using experimentation to generate better results for the whole business.
Download our latest ebook to discover how businesses have made the shift from CRO to experimentation and how you can too.
Join the next one!
Be the first to know about future events with top speakers from the CRO industry.
9 Multivariate methods for heterogeneous data

In Chapter 7 , we saw how to summarize rectangular matrices whose columns were continuous variables. The maps we made used unsupervised dimensionality reduction techniques such as principal component analysis aimed at isolating the most important signal component in a matrix \(X\) when all the columns have meaningful variances.
Here we extend these ideas to more complex heterogeneous data where continuous and categorical variables are combined. Indeed, sometimes our observations cannot be easily described by sets of individual variables or coordinates – but it is possible to determine distances or (dis)similarities between them, or to describe relationships between them using a graph or a tree. Examples include species in a species tree or biological sequences. Outside of biology, examples include text documents or movie files, where we may have a reasonable method to determine (dis)similarity between them, but no obvious variables or coordinates.
This chapter contains more advanced techniques, for which we often omit technical details. Having come this far, we hope that by giving you some hands-on experience with examples, and extensive references, to enable you to understand and use some of the more `cutting edge’ techniques in nonlinear multivariate analysis.
9.1 Goals for this chapter
In this chapter, we will:
Extend linear dimension reduction methods to cases when the distances between observations are available, known as m ulti d imensional s caling (MDS) or principal coordinates analysis.
Find modifications of MDS that are nonlinear and robust to outliers.
Encode combinations of categorical data and continuous data as well as so-called ‘supplementary’ information. We will see that this enables us to deal with batch effects .
Use chi-square distances and c orrespondence a nalysis (CA) to see where categorical data (contingency tables) contain notable dependencies.
Generalize clustering methods that can uncover latent variables that are not categorical. This will allow us to detect gradients, “pseudotime” and hidden nonlinear effects in our data.
Generalize the notion of variance and covariance to the study of tables of data from multiple different data domains.
9.2 Multidimensional scaling and ordination
Sometimes, data are not represented as points in a feature space. This can occur when we are provided with (dis)similarity matrices between objects such as drugs, images, trees or other complex objects, which have no obvious coordinates in \({\mathbb R}^n\) .
In Chapter 5 we saw how to produce clusters from distances. Here our goal is to visualize the data in maps in low dimensional spaces (e.g., planes) reminiscent of the ones we make from the first few principal axes in PCA.
We start with an intuitive example using geography data. In Figure 9.1 , a heatmap and clustering of the distances between cities and places in Ukraine 1 are shown.
1 The provenance of these data are described in the script ukraine-dists.R in the data folder.

Besides ukraine_dists , which contains the pairwise distances, the RData file that we loaded above also contains the dataframe ukraine_coords with the longitudes and latitudes; we will use this later as a ground truth. Given the distances, multidimensional scaling (MDS) provides a “map” of their relative locations. It will not be possible to arrange the cities such that their Euclidean distances on a 2D plane exactly reproduce the given distance matrix: the cities lie on the curved surface of the Earth rather than in a plane. Nevertheless, we can expect to find a two dimensional embedding that represents the data well. With biological data, our 2D embeddings are likely to be much less clearcut. We call the function with:
We make a function that we will reuse several times in this chapter to make a screeplot from the result of a call to the cmdscale function:

Question 9.1 Look at all the eigenvalues output by the cmdscale function: what do you notice?
If you execute:

you will note that unlike in PCA, there are some negative eigenvalues. These are due to the way cmdscale works.
The main output from the cmdscale function are the coordinates of the two-dimensional embedding, which we show in Figure 9.4 (we will discuss how the algorithm works in the next section).

Note that while relative positions are correct, the orientation of the map is unconventional: Crimea is at the top. This is a common phenomenon with methods that reconstruct planar embeddings from distances. Since the distances between the points are invariant under rotations and reflections (axis flips), any solution is as good as any other solution that relates to it via rotation or reflection. Functions like cmdscale will pick one of the equally optimal solutions, and the particular choice can depend on minute details of the data or the computing platform being used. Here, we can transform our result into a more conventional orientation by reversing the sign of the \(y\) -axis. We redraw the map in Figure 9.5 and compare this to the true longitudes and latitudes from the ukraine_coords dataframe ( Figure 9.6 ).

Question 9.2 We drew the longitudes and latitudes in the right panel of Figure 9.6 without attention to aspect ratio. What is the right aspect ratio for this plot?
There is no simple relationship between the distances that correspond to 1 degree change in longitude and to 1 degree change in latitude, so the choice is difficult to make. Even under the simplifying assumption that our Earth is spherical and has a radius of 6371 km, it’s complicated: one degree in latitude always corresponds to a distance of 111 km ( \(6371\times2\pi/360\) ), as does one degree of longitude on the equator. However, when you move away from the equator, a degree of longitude corresponds to shorter and shorter distances (and to no distance at all at the poles). Pragmatically, for displays such as in Figure 9.6 , we could choose a value for the aspect ratio that’s somewhere in the middle between the Northern and Southern most points, say, the cosine for 48 degrees.
Question 9.3 Add international borders and geographic features such as rivers to Figure 9.6 .
A start point is provided by the code below, which adds international borders ( Figure 9.7 ).

Note: MDS creates similar output as PCA, however there is only one ‘dimension’ to the data (the sample points). There is no ‘dual’ dimension, there are no biplots and no loading vectors. This is a drawback when coming to interpreting the maps. Interpretation can be facilitated by examining carefully the extreme points and their differences.
9.2.1 How does the method work?
Let’s take a look at what would happen if we really started with points whose coordinates were known 2 . We put these coordinates into the two columns of a matrix with as many rows as there are points. Now we compute the distances between points based on these coordinates. To go from the coordinates \(X\) to distances, we write \[d^2_{i,j} = (x_i^1 - x_j^1)^2 + \dots + (x_i^p - x_j^p)^2.\] We will call the matrix of squared distances DdotD in R and \(D\bullet D\) in the text . We want to find points such that the square of their distances is as close as possible to the \(D\bullet D\) observed. 3
2 Here we commit a slight ‘abuse’ by using the longitude and longitude of our cities as Cartesian coordinates and ignoring the curvature of the earth’s surface. Check out the internet for information on the Haversine formula.
3 Here we commit a slight ‘abuse’ by using the longitudes and latitudes of our cities as Cartesian coordinates and ignoring the fact that they are curvilinear coordinates on a sphere-like surface.
The relative distances do not depend on the point of origin of the data. We center the data by using the centering matrix \(H\) defined as \(H=I-\frac{1}{n}{\mathbf{11}}^t\) . Let’s check the centering property of \(H\) using:
Question 9.4 Call B0 the matrix obtained by applying the centering matrix both to the right and to the left of DdotD Consider the points centered at the origin given by the \(HX\) matrix and compute its cross product, we’ll call this B2 . What do you have to do to B0 to make it equal to B2 ?
Therefore, given the squared distances between rows ( \(D\bullet D\) ) and the cross product of the centered matrix \(B=(HX)(HX)^t\) , we have shown:
\[ -\frac{1}{2} H(D\bullet D) H=B \tag{9.1}\]
This is always true, and we use it to reverse-engineer an \(X\) which satisfies Equation 9.1 when we are given \(D\bullet D\) to start with.
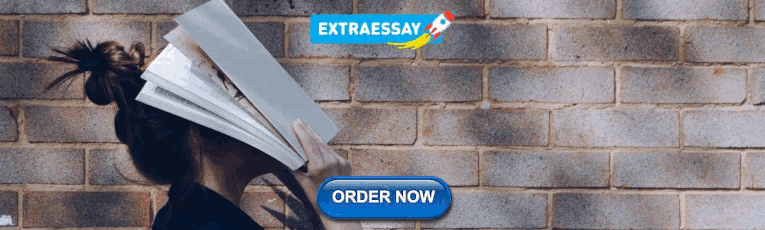
From \(D\bullet D\) to \(X\) using singular vectors.
We can go backwards from a matrix \(D\bullet D\) to \(X\) by taking the eigen-decomposition of \(B\) as defined in Equation 9.1 . This also enables us to choose how many coordinates, or columns, we want for the \(X\) matrix. This is very similar to how PCA provides the best rank \(r\) approximation. Note : As in PCA, we can write this using the singular value decomposition of \(HX\) (or the eigen decomposition of \(HX(HX)^t\) ):
\[ HX^{(r)} = US^{(r)}V^t \mbox{ with } S^{(r)} \mbox{ the diagonal matrix of the first } r \mbox{ singular values}, \]
This provides the best approximate representation in an Euclidean space of dimension \(r\) . The algorithm gives us the coordinates of points that have approximately the same distances as those provided by the \(D\) matrix.
Classical MDS Algorithm.
In summary, given an \(n \times n\) matrix of squared interpoint distances \(D\bullet D\) , we can find points and their coordinates \(\tilde{X}\) by the following operations:
Double center the interpoint distance squared and multiply it by \(-\frac{1}{2}\) : \(B = -\frac{1}{2}H D\bullet D H\) .
Diagonalize \(B\) : \(\quad B = U \Lambda U^t\) .
Extract \(\tilde{X}\) : \(\quad \tilde{X} = U \Lambda^{1/2}\) .
Finding the right underlying dimensionality.
As an example, let’s take objects for which we have similarities (surrogrates for distances) but for which there is no natural underlying Euclidean space.
In a psychology experiment from the 1950s, Ekman ( 1954 ) asked 31 subjects to rank the similarities of 14 different colors. His goal was to understand the underlying dimensionality of color perception. The similarity or confusion matrix was scaled to have values between 0 and 1. The colors that were often confused had similarities close to 1. We transform the data into a dissimilarity by subtracting the values from 1:
We compute the MDS coordinates and eigenvalues. We combine the eigenvalues in the screeplot shown in Figure 9.8 :

We plot the different colors using the first two principal coordinates as follows:

Figure 9.9 shows the Ekman data in the new coordinates. There is a striking pattern that calls for explanation. This horseshoe or arch structure in the points is often an indicator of a sequential latent ordering or gradient in the data ( Diaconis, Goel, and Holmes 2008 ) . We will revisit this in Section 9.5 .
9.2.2 Robust versions of MDS
Multidimensional scaling aims to minimize the difference between the squared distances as given by \(D\bullet D\) and the squared distances between the points with their new coordinates. Unfortunately, this objective tends to be sensitive to outliers: one single data point with large distances to everyone else can dominate, and thus skew, the whole analysis. Often, we like to use something that is more robust, and one way to achieve this is to disregard the actual values of the distances and only ask that the relative rankings of the original and the new distances are as similar as possible. Such a rank based approach is robust: its sensitivity to outliers is reduced.
We will use the Ekman data to show how useful robust methods are when we are not quite sure about the ‘scale’ of our measurements. Robust ordination, called non metric multidimensional scaling (NMDS for short) only attempts to embed the points in a new space such that the order of the reconstructed distances in the new map is the same as the ordering of the original distance matrix.
Non metric MDS looks for a transformation \(f\) of the given dissimilarities in the matrix \(d\) and a set of coordinates in a low dimensional space ( the map ) such that the distance in this new map is \(\tilde{d}\) and \(f(d)\thickapprox \tilde{d}\) . The quality of the approximation can be measured by the standardized residual sum of squares ( stress ) function:
\[ \text{stress}^2=\frac{\sum(f(d)-\tilde{d})^2}{\sum d^2}. \]
NMDS is not sequential in the sense that we have to specify the underlying dimensionality at the outset and the optimization is run to maximize the reconstruction of the distances according to that number. There is no notion of percentage of variation explained by individual axes as provided in PCA. However, we can make a simili-screeplot by running the program for all the successive values of \(k\) ( \(k=1, 2, 3, ...\) ) and looking at how well the stress drops. Here is an example of looking at these successive approximations and their goodness of fit. As in the case of diagnostics for clustering, we will take the number of axes after the stress has a steep drop.
Because each calculation of a NMDS result requires a new optimization that is both random and dependent on the \(k\) value, we use a similar procedure to what we did for clustering in Chapter 4 . We execute the metaMDS function, say, 100 times for each of the four possible values of \(k\) and record the stress values.
Let’s look at the boxplots of the results. This can be a useful diagnostic plot for choosing \(k\) ( Figure 9.10 ).

We can also compare the distances and their approximations using what is known as a Shepard plot for \(k=2\) for instance, computed with:

Both the Shepard’s plot in Figure 9.11 and the screeplot in Figure 9.10 point to a two-dimensional solution for Ekman’s color confusion study. Let us compare the output of the two different MDS programs, the classical metric least squares approximation and the nonmetric rank approximation method. The right panel of Figure 9.12 shows the result from the nonmetric rank approximation, the left panel is the same as Figure 9.9 . The projections are almost identical in both cases. For these data, it makes little difference whether we use a Euclidean or nonmetric multidimensional scaling method.

9.3 Contiguous or supplementary information

In Chapter 3 we introduced the R data.frame class that enables us to combine heterogeneous data types: categorical factors, text and continuous values. Each row of a dataframe corresponds to an object, or a record, and the columns are the different variables, or features.
Extra information about sample batches, dates of measurement, different protocols are often named metadata ; this can be misnomer if it is implied that metadata are somehow less important. Such information is real data that need to be integrated into the analyses. We typically store it in a data.frame or a similar R class and tightly link it to the primary assay data.
9.3.1 Known batches in data
Here we show an example of an analysis that was done by Holmes et al. ( 2011 ) on bacterial abundance data from Phylochip ( Brodie et al. 2006 ) microarrays. The experiment was designed to detect differences between a group of healthy rats and a group who had Irritable Bowel Disease ( Nelson et al. 2010 ) . This example shows a case where the nuisance batch effects become apparent in the analysis of experimental data. It is an illustration of the fact that best practices in data analyses are sequential and that it is better to analyse data as they are collected to adjust for severe problems in the experimental design as they occur , instead of having to deal with them post mortem 4 .
4 Fisher’s terminology, see Chapter 13 .
When data collection started on this project, days 1 and 2 were delivered and we made the plot that appears in Figure 9.14 . This showed a definite day effect. When investigating the source of this effect, we found that both the protocol and the array were different in days 1 and 2. This leads to uncertainty in the source of variation, we call this confounding of effects.
We load the data and the packages we use for this section:
Question 9.5 What class is the IBDchip ? Look at the last row of the matrix, what do you notice?
The data are normalized abundance measurements of 8634 taxa measured on 28 samples. We use a rank-threshold transformation, giving the top 3000 most abundant taxa scores from 3000 to 1, and letting the remaining (low abundant) ones all have a score of 1. We also separate out the proper assay data from the (awkwardly placed) day variable, which should be considered a factor 5 :
5 Below, we show how to arrange these data into a Bioconductor SummarizedExperiment , which is a much more sane way of storing such data.
Instead of using the continuous, somehow normalized data, we use a robust analysis replacing the values by their ranks. The lower values are considered ties encoded as a threshold chosen to reflect the number of expected taxa thought to be present:

Question 9.6 Why do we use a threshold for the ranks?
Low abundances, at noise level occur for species that are not really present, of which there are more than half. A large jump in rank for these observations could easily occur without any meaningful reason. Thus we create a large number of ties for low abundance.
Figure 9.14 shows that the sample arrange themselves naturally into two different groups according to the day of the samples. After discovering this effect, we delved into the differences that could explain these distinct clusters. There were two different protocols used (protocol 1 on day 1, protocol 2 on day 2) and unfortunately two different provenances for the arrays used on those two days (array 1 on day 1, array 2 on day 2).
A third set of data of four samples had to be collected to deconvolve the confounding effect. Array 2 was used with protocol 2 on Day 3, Figure 9.15 shows the new PCA plot with all the samples created by the following:

Question 9.7 In which situation would it be preferable to make confidence ellipses around the group means using the following code?

Through this visualization we were able to uncover a flaw in the original experimental design. The first two batches shown in green and brown were both balanced with regards to IBS and healthy rats. They do show very different levels of variability and overall multivariate coordinates. In fact, there are two confounded effects. Both the arrays and protocols were different on those two days. We had to run a third batch of experiments on day 3, represented in purple, this used protocol from day 1 and the arrays from day 2. The third group faithfully overlaps with batch 1, telling us that the change in protocol was responsible for the variability.
9.3.2 Removing batch effects
Through the combination of the continuous measurements from assayIBD and the supplementary batch number as a factor, the PCA map has provided an invaluable investigation tool. This is a good example of the use of supplementary points 6 . The mean-barycenter points are created by using the group-means of points in each of the three groups and serve as extra markers on the plot.
6 This is called a supplementary point because the new observation-point is not used in the matrix decomposition.
We can decide to re-align the three groups by subtracting the group means so that all the batches are centered on the origin. A slightly more effective way is to use the ComBat function available in the sva package. This function uses a similar, but slightly more sophisticated method (Empirical Bayes mixture approach ( Leek et al. 2010 ) ). We can see its effect on the data by redoing our robust PCA (see the result in Figure 9.17 ):

9.3.3 Hybrid data and Bioconductor containers
A more rational way of combining the batch and treatment information into compartments of a composite object is to use the SummarizedExperiment class. It includes special slots for the assay(s) where rows represent features of interest (e.g., genes, transcripts, exons, etc.) and columns represent samples. Supplementary information about the features can be stored in a DataFrame object, accessible using the function rowData . Each row of the DataFrame provides information on the feature in the corresponding row of the SummarizedExperiment object.
Here we insert the two covariates day and treatment in the colData object and combine it with assay data in a new SummarizedExperiment object.
This is the best way to keep all the relevant data together, it will also enable you to quickly filter the data while keeping all the information aligned properly.
Question 9.8 Make a new SummarizedExperiment object by choosing the subset of the samples that were created on day 2.
Columns of the DataFrame represent different attributes of the features of interest, e.g., gene or transcript IDs, etc. Here is an example of hybrid data container from single cell experiments (see Bioconductor workflow in Perraudeau et al. ( 2017 ) for more details).
After the pre-processing and normalization steps prescribed in the workflow, we retain the 1000 most variable genes measured on 747 cells.
Question 9.9 How many different batches do the cells belong to ?
We can look at a PCA of the normalized values and check graphically that the batch effect has been removed:

Since the screeplot in Figure 9.18 shows us that we must not dissociate axes 2 and 3, we will make a three dimensional plot with the rgl package. We use the following interactive code:

Note: Of course, the book medium is limiting here, as we are showing two static projections that do not do justice to the depth available when looking at the interactive dynamic plots as they appear using the plot3d function. We encourage the reader to experiment extensively with these and other interactive packages and they provide a much more intuitive experience of the data.
9.4 Correspondence analysis for contingency tables
9.4.1 cross-tabulation and contingency tables.
Categorical data abound in biological settings: sequence status (CpG/non-CpG), phenotypes, taxa are often coded as factors as we saw in Chapter 2. Cross-tabulation of two such variables gives us a contingency table ; the result of counting the co-occurrence of two phenotypes (sex and colorblindness was such an example). We saw that the first step is to look at the independence of the two categorical variables; the standard statistical measure of independence uses the chisquare distance . This quantity will replace the variance we used for continuous measurements.
The columns and rows of the table have the same `status’ and we are not in supervised/regression type setting. We won’t see a sample/variable divide; as a consequence the rows and columns will have the same status and we will ‘center’ both the rows and the columns. This symmetry will also translate in our use of biplots where both dimensions appear on the same plot.
Transforming the data to tabular form.
If the data are collected as long lists with each subject (or sample) associated to its levels of the categorical variables, we may want to transform them into a contingency table. Here is an example. In Table 9.1 HIV mutations are tabulated as indicator (0/1) binary variables. These data are then transformed into a mutation co-occurrence matrix shown in Table 9.2 .
Question 9.10 What information is lost in this cross-tabulation ? When will this matter?
Here are some co-occurrence data from the HIV database ( Rhee et al. 2003 ) . Some of these mutations have a tendency to co-occur.
Question 9.11 Test the hypothesis of independence of the mutations.
Before explaining the details of how correspondence analysis works, let’s look at the output of one of many correspondence analysis functions. We use dudi.coa from the ade4 package to plot the mutations in a lower dimensional projection; the procedure follows what we did for PCA.

After looking at a screeplot, we see that dimensionality of the underlying variation is definitely three dimensional, we plot these three dimensions. Ideally this would be done with an interactive three-dimensional plotting function such as that provided through the package rgl as shown in Figure 9.21 .
Question 9.12 Using the car and rgl packages make 3d scatterplot similar to Figure 9.21 . Compare to the plot obtained using aspect=FALSE with the plot3d function from rgl . What structure do you notice by rotating the cloud of points?

Question 9.13 Show the code for plotting the plane defined by axes 1 and 3 of the correspondence analysis respecting the scaling of the vertical axis as shown in the bottom figure of Figure 9.22 .
This first example showed how to map all the different levels of one categorical variable (the mutations) in a similar way to how PCA projects continuous variables. We will now explore how this can be extended to two or more categorical variables.
9.4.2 Hair color, eye color and phenotype co-occurrence
We will consider a small table, so we can follow the analysis in detail. The data are a contingency table of hair-color and eye-color phenotypic co-occurrence from students as shown in Table 9.3 . In Chapter 2 , we used a \(\chi^2\) test to detect possible dependencies:
However, stating non independence between hair and eye color is not enough. We need a more detailed explanation of where the dependencies occur: which hair color occurs more often with green eyes ? Are some of the variable levels independent? In fact we can study the departure from independence using a special weighted version of SVD. This method can be understood as a simple extension of PCA and MDS to contingency tables.
Independence: computationally and visually.
We start by computing the row and column sums; we use these to build the table that would be expected if the two phenotypes were independent. We call this expected table HCexp .
Now we compute the \(\chi^2\) (chi-squared) statistic, which is the sum of the scaled residuals for each of the cells of the table:
We can study these residuals from the expected table, first numerically then in Figure 9.23 .

Mathematical Formulation.
Here are the computations we just did in R in a more mathematical form. For a general contingency table \({\mathbf N}\) with \(I\) rows and \(J\) columns and a total sample size of \(n=\sum_{i=1}^I \sum_{j=1}^J n_{ij}= n_{\cdot \cdot}\) . If the two categorical variables were independent, each cell frequency would be approximately equal to
\[ n_{ij} = \frac{n_{i \cdot}}{n} \frac{n_{\cdot j}}{n} \times n \]
can also be written:
\[ {\mathbf N} = {\mathbf c r'} \times n, \qquad \mbox{ where } c= \frac{1}{n} {{\mathbf N}} {\mathbb 1}_m \;\mbox{ and }\; r'=\frac{1}{n} {\mathbf N}' {\mathbb 1}_p \]
The departure from independence is measured by the \(\chi^2\) statistic
\[ {\cal X}^2=\sum_{i,j} \frac{\left(n_{ij}-\frac{n_{i\cdot}}{n}\frac{n_{\cdot j}}{n}n\right)^2} {\frac{n_{i\cdot}n_{\cdot j}}{n^2}n} \]
Once we have ascertained that the two variables are not independent, we use a weighted multidimensional scaling using \(\chi^2\) distances to visualize the associations.
The method is called Correspondence Analysis (CA) or Dual Scaling and there are multiple R packages that implement it.
Here we make a simple biplot of the Hair and Eye colors.

Question 9.14 What percentage of the Chisquare statistic is explained by the first two axes of the Correspondence Analysis?
Question 9.15 Compare the results with those obtained by using CCA in the vegan package with the appropriate value for the scaling parameter.
Interpreting the biplots
CA has a special barycentric property: the biplot scaling is chosen so that the row points are placed at the center of gravity of the column levels with their respective weights. For instance, the Blue eyes column point is at the center gravity of the (Black, Brown, Red, Blond) with weights proportional to (9, 34, 7, 64). The Blond row point is very heavily weighted, this is why Figure 9.24 shows Blond and Blue quite close together.
9.5 Finding time…and other important gradients.
All the methods we have studied in the last sections are commonly known as ordination methods. In the same way clustering allowed us to detect and interpret a hidden factor/categorical variable, ordination enables us to detect and interpret a hidden ordering, gradient or latent variable in the data.

Ecologists have a long history of interpreting the arches formed by observations points in correspondence analysis and principal components as ecological gradients ( Prentice 1977 ) . Let’s illustrate this first with a very simple data set on which we perform a correspondence analysis.
We plot both the row-location points ( Figure 9.25 (a)) and the biplot of both location and plant species in the lower part of Figure 9.25 (b); this plot was made with:

Question 9.16 Looking back at the raw matrix lakes as it appears, do you see a pattern in its entries? What would happen if the plants had been ordered by actual taxa names for instance?
9.5.1 Dynamics of cell development
We will now analyse a more interesting data set that was published by Moignard et al. ( 2015 ) . This paper describes the dynamics of blood cell development. The data are single cell gene expression measurements of 3,934 cells with blood and endothelial potential from five populations from between embryonic days E7.0 and E8.25.

Remember from Chapter 4 that several different distances are available for comparing our cells. Here, we start by computing both an \(L_2\) distance and the \(\ell_1\) distance between the 3,934 cells.
The classical multidimensional scaling on these two distances matrices can be carried out using:
We look at the underlying dimension and see in Figure 9.27 that two dimensions can provide a substantial fraction of the variance.

The first 2 coordinates account for 78 % of the variability when the \(\ell_1\) distance is used between cells, and 57% when the \(L^2\) distance is used. We see in Figure 9.28 (a) the first plane for the MDS on the \(\ell_1\) distances between cells:

Figure 9.28 (b) is created in the same way and shows the two-dimensional projection created by using MDS on the L2 distances.
Figure 9.28 shows that both distances (L1 and L2) give the same first plane for the MDS with very similar representations of the underlying gradient followed by the cells.
We can see from Figure 9.28 that the cells are not distributed uniformly in the lower dimensions we have been looking at, we see a definite organization of the points. All the cells of type 4SG represented in red form an elongated cluster who are much less mixed with the other cell types.
9.5.2 Local, nonlinear methods
Multidimensional scaling and non metric multidimensional scaling aims to represent all distances as precisely as possible and the large distances between far away points skew the representations. It can be beneficial when looking for gradients or low dimensional manifolds to restrict ourselves to approximations of points that are close together. This calls for methods that try to represent local (small) distances well and do not try to approximate distances between faraway points with too much accuracy.
There has been substantial progress in such methods in recent years. The use of kernels computed using the calculated interpoint distances allows us to decrease the importance of points that are far apart. A radial basis kernel is of the form
\[ 1-\exp\left(-\frac{d(x,y)^2}{\sigma^2}\right), \quad\mbox{where } \sigma^2 \mbox{ is fixed.} \]
It has the effect of heavily discounting large distances. This can be very useful as the precision of interpoint distances is often better at smaller ranges; several examples of such methods are covered in Exercise 9.6 at the end of this chapter.
Question 9.17 Why do we take the difference between the 1 and the exponential? What happens when the distance between \(x\) and \(y\) is very big?
This widely used method adds flexibility to the kernel defined above and allows the \(\sigma^2\) parameter to vary locally (there is a normalization step so that it averages to one). The t-SNE method starts out from the positions of the points in the high dimensional space and derives a probability distribution on the set of pairs of points, such that the probabilities are proportional to the points’ proximities or similarities. It then uses this distribution to construct a representation of the dataset in low dimensions. The method is not robust and has the property of separating clusters of points artificially; however, this property can also help clarify a complex situation. One can think of it as a method akin to graph (or network) layout algorithms. They stretch the data to clarify relations between the very close (in the network: connected) points, but the distances between more distal (in the network: unconnected) points cannot be interpreted as being on the same scales in different regions of the plot. In particular, these distances will depend on the local point densities. Here is an example of the output of t-SNE on the cell data:

In this case in order to see the subtle differences between MDS and t-SNE, it is really necessary to use 3d plotting.
Use the rgl package to look at the three t-SNE dimensions and add the correct cell type colors to the display.
Two of these 3d snapshots are shown in Figure 9.30 , we see a much stronger grouping of the purple points than in the MDS plots.
Note: A site worth visiting in order to appreciate more about the sensitivity of the t-SNE method to the complexity and \(\sigma\) parameters can be found at http://distill.pub/2016/misread-tsne .

Question 9.18 Visualize a two-dimensional t-SNE embedding of the Ukraine distances from Section 9.2 .

There are several other nonlinear methods for estimating nonlinear trajectories followed by points in the relevant state spaces. Here are a few examples.
RDRToolbox Local linear embedding ( LLE ) and isomap
diffusionMap This package models connections between points as a Markovian kernel.
kernlab Kernel methods
LPCM-package Local principal curves
9.6 Multitable techniques
Current studies often attempt to quantify variation in the microbial, genomic, and metabolic measurements across different experimental conditions. As a result, it is common to perform multiple assays on the same biological samples and ask what features – microbes, genes, or metabolites, for example – are associated with different sample conditions. There are many ways to approach these questions. Which to apply depends on the study’s focus.
9.6.1 Co-variation, inertia, co-inertia and the RV coefficient
As in physics, we define inertia as a sum of distances with ‘weighted’ points. This enables us to compute the inertia of counts in a contingency table as the weighted sum of the squares of distances between observed and expected frequencies (as in the chisquare statistic).
If we want to study two standardized variables measured at the same 10 locations together, we use their covariance . If \(x\) represents the standardized PH, and and \(y\) the standardized humidity, we measure their covariation using the mean
\[ \text{cov}(x,y) = \text{mean}(x1*y1 + x2*y2 + x3*y3 + \cdots + x10*y10). \tag{9.2}\]
If \(x\) and \(y\) co-vary in the same direction, this will be big. We saw how useful the correlation coefficient we defined in Chapter 8 was to our multivariate analyses. Multitable generalizations will be just as useful.
9.6.2 Mantel coefficient and a test of distance correlation
The Mantel coefficient, one of the earliest version of matrix association, developed and used by Henry Daniels, FN David and co-authors ( Josse and Holmes 2016 ) is very popular, especially in ecology.
Given two dissimilarity matrices \({\mathbf D^X}\) and \({\mathbf D^Y}\) , make these matrices into vectors the way the R dist function does, and compute their correlation. This is defined mathematically as:
\[ \begin{aligned} \mbox{r}_{\rm m}(\bf X,\bf Y)= \frac{\sum_{i=1}^n \sum_{j=1,j \neq i}^n (d_{ij}^**X**- \bar{d}^**X**)(d_{ij}^**Y**-\bar d^**Y**)}{\sqrt{\sum_{i,j,j \neq i}(d_{ij}^**X**- \bar{d}^**X**)^2 \sum_{i,j,j \neq i}(d_{ij}^**Y**-\bar{d}^**Y**)^2}}, \end{aligned} \]
with \(\bar{d}^**X**\) (resp \(\bar{d}^**Y**\) ) the mean of the lower diagonal terms of the dissimilarity matrix associated to \(\bf X\) (resp. to \(\bf Y\) ). This formulation shows us that it is a measure of linear correlation between distances. It has been widely used for testing two sets of distances, for instance one distance \({\mathbf D^X}\) could be computed using the soil chemistry at 17 different locations. The other distance \({\mathbf D^Y}\) could record plant abundance dissimilarities using a Jaccard index between the 17 same locations.
The correlation’s significance is often assessed via a simple permutation test, see Josse and Holmes ( 2016 ) for a review with its historical background and modern incarnations. The coefficient and associated tests are implemented in several R packages such as ade4 ( Chessel, Dufour, and Thioulouse 2004 ) , vegan and ecodist ( Goslee, Urban, et al. 2007 ) .
RV coefficient The global measure of similarity of two data tables as opposed to two vectors can be done by a generalization of covariance provided by an inner product between tables that gives the RV coefficient, a number between 0 and 1, like a correlation coefficient, but for tables.
\[ RV(A,B)=\frac{Tr(A'BAB')}{\sqrt{Tr(A'A)}\sqrt{Tr(B'B)}} \]
There are several other measures of matrix correlation available in the package MatrixCorrelation .
If we do ascertain a link between two matrices, we then need to find a way to understand that link. One such method is explained in the next section.
9.6.3 Canonical correlation analysis (CCA)
CCA is a method similar to PCA as it was developed by Hotelling in the 1930s to search for associations between two sets of continuous variables \(X\) and \(Y\) . Its goal is to find a linear projection of the first set of variables that maximally correlates with a linear projection of the second set of variables.
Finding correlated functions (covariates) of the two views of the same phenomenon by discarding the representation-specific details (noise) is expected to reveal the underlying hidden yet influential factors responsible for the correlation.
Canonical Correlation Algorithm.
Let us consider two matrices \(X\) and \(Y\) of order \(n > p\) and \(n > q\) respectively. The columns of \(X\) and \(Y\) correspond to variables and the rows correspond to the same \(n\) experimental units. The jth column of the matrix \(X\) is denoted by \(X_j\) , likewise the kth column of \(Y\) is denoted by \(Y_k\) . Without loss of generality it will be assumed that the columns of \(X\) and \(Y\) are standardized (mean 0 and variance 1).
We denote by \(S_{XX}\) and \(S_{YY}\) the sample covariance matrices for variable sets \(X\) and \(Y\) respectively, and by \(S_{XY} = S_{YX}^t\) the sample cross-covariance matrix between \(X\) and \(Y\) .
Classical CCA assumes first \(p \leq n\) and \(q \leq n\) , then that matrices \(X\) and \(Y\) are of full column rank p and q respectively. In the following, the principle of CCA is presented as a problem solved through an iterative algorithm. The first stage of CCA consists in finding two vectors \(a =(a_1,...,a_p)^t\) and \(b =(b_1,...,b_q)^t\) that maximize the correlation between the linear combinations \(U\) and \(V\) defined as
\[ \begin{aligned} U=Xa&=&a_1 X_1 +a_2 X_2 +\cdots a_p X_p\\ V=Yb&=&b_1 Y_1 +b_2 Y_2 +\cdots a_q Y_q \end{aligned} \]
and assuming that vectors \(a\) and $ b$ are normalized so that \(var(U) = var(V) = 1\) In other words, the problem consists in finding \(a\) and \(b\) such that
\[ \rho_1 = \text{cor}(U, V) = \max_{a,b} \text{cor} (Xa, Yb)\quad \text{subject to}\quad \text{var}(Xa)=\text{var}(Yb) = 1. \tag{9.3}\]
The resulting variables \(U\) and \(V\) are called the first canonical variates and \(\rho_1\) is referred to as the first canonical correlation.
Note: Higher order canonical variates and canonical correlations can be found as a stepwise problem. For \(s = 1,...,p\) , we can successively find positive correlations \(\rho_1 \geq \rho_2 \geq ... \geq \rho_p\) with corresponding vectors \((a^1, b^1), ..., (a^p, b^p)\) , by maximizing
\[ \rho_s = \text{cor}(U^s,V^s) = \max_{a^s,b^s} \text{cor} (Xa^s,Yb^s)\quad \text{subject to}\quad \text{var}(Xa^s) = \text{var}(Yb^s)=1 \tag{9.4}\]
under the additional restrictions
\[ \text{cor}(U^s,U^t) = \text{cor}(V^s, V^t)=0 \quad\text{for}\quad 1 \leq t < s \leq p. \tag{9.5}\]
We can think of CCA as a generalization of PCA where the variance we maximize is the `covariance’ between the two matrices (see Holmes ( 2006 ) for more details).
9.6.4 Sparse canonical correlation analysis (sCCA)
When the number of variables in each table is very large finding two very correlated vectors can be too easy and unstable: we have too many degrees of freedom.
Then it is beneficial to add a penalty maintains the number of non-zero coefficients to a minimum. This approach is called sparse canonical correlation analysis (sparse CCA or sCCA), a method well-suited to both exploratory comparisons between samples and the identification of features with interesting co variation. We will use an implementation from the PMA package.
Here we study a dataset collected by Kashyap et al. ( 2013 ) with two tables. One is a contingency table of bacterial abundances and another an abundance table of metabolites. There are 12 samples, so \(n = 12\) . The metabolite table has measurements on \(p = 637\) feature and the bacterial abundances had a total of $ q = 20,609$ OTUs, which we will filter down to around 200. We start by loading the data.
We first filter down to bacteria and metabolites of interest, removing (“by hand”) those that are zero across many samples and giving an upper threshold of 50 to the large values. We transform the data to weaken the heavy tails.
A second step in our preliminary analysis is to look if there is any association between the two matrices using the RV.test from the ade4 package:
We can now apply sparse CCA. This method compares sets of features across high-dimensional data tables, where there may be more measured features than samples. In the process, it chooses a subset of available features that capture the most covariance – these are the features that reflect signals present across multiple tables. We then apply PCA to this selected subset of features. In this sense, we use sparse CCA as a screening procedure, rather than as an ordination method.
The implementation is below. The parameters penaltyx and penaltyz are sparsity penalties. Smaller values of penaltyx will result in fewer selected microbes, similarly penaltyz modulates the number of selected metabolites. We tune them manually to facilitate subsequent interpretation – we generally prefer more sparsity than the default parameters would provide.
With these parameters, 5 bacteria and 16 metabolites were selected based on their ability to explain covariation between tables. Further, these features result in a correlation of 0.99 between the two tables. We interpret this to mean that the microbial and metabolomic data reflect similar underlying signals, and that these signals can be approximated well by the selected features. Be wary of the correlation value, however, since the scores are far from the usual bivariate normal cloud. Further, note that it is possible that other subsets of features could explain the data just as well – sparse CCA has minimized redundancy across features, but makes no guarantee that these are the “true” features in any sense.
Nonetheless, we can still use these 21 features to compress information from the two tables without much loss. To relate the recovered metabolites and OTUs to characteristics of the samples on which they were measured, we use them as input to an ordinary PCA. We have omitted the code we used to generate Figure 9.32 , we refer the reader to the online material accompanying the book or the workflow published in Callahan et al. ( 2016 ) .
Figure 9.32 displays the PCA triplot , where we show different types of samples and the multidomain features (Metabolites and OTUs). This allows comparison across the measured samples – triangles for knockout and circles for wild type –and characterizes the influence the different features – diamonds with text labels. For example, we see that the main variation in the data is across PD and ST samples, which correspond to the different diets. Further, large values of 15 of the features are associated with ST status, while small values for 5 of them indicate PD status.

The advantage of the sparse CCA screening is now clear – we can display most of the variation across samples using a relatively simple plot, and can avoid plotting the hundreds of additional points that would be needed to display all of the features.
9.6.5 Canonical (or constrained) correspondence analysis (CCpnA)
The term constrained correspondence analysis translates the fact that this method is similar to a constrained regression. The method attempts to force the latent variables to be correlated with the environmental variables provided as `explanatory’.
CCpnA creates biplots where the positions of samples are determined by similarity in both species signatures and environmental characteristics. In contrast, principal components analysis or correspondence analysis only look at species signatures. More formally, it ensures that the resulting CCpnA directions lie in the span of the environmental variables. For thorough explanations see Braak ( 1985 ; Greenacre 2007 ) .
This method can be run using the function ordinate in phyloseq . In order to use the covariates from the sample data, we provide an extra argument, specifying which of the features to consider.
Here, we take the data we denoised using dada2 in Chapter 4 . We will see more details about creating the phyloseq object in Chapter 10 . For the time being, we use the otu_table component containing a contingency table of counts for different taxa. We would like to compute the constrained correspondence analyses that explain the taxa abundances by the age and family relationship (both variables are contained in the sample_data slot of the ps1 object).
We would like to make two dimensional plots showing only using the four most abundant taxa (making the biplot easier to read):
To access the positions for the biplot, we can use the scores function in the vegan . Further, to facilitate figure annotation, we also join the site scores with the environmental data in the sample_data slot. Of the 23 total taxonomic orders, we only explicitly annotate the four most abundant – this makes the biplot easier to read.

Question 9.19 Look up the extra code for creating the tax and species objects in the online resources accompanying the book. Then make the analogue of Figure 9.33 but using litter as the faceting variable.

Figures 9.33 and 9.34 show the plots of these annotated scores, splitting sites by their age bin and litter membership, respectively. Note that to keep the appropriate aspect ratio in the presence of faceting, we have taken the vertical axis as our first canonical component. We have labeled individual bacteria that are outliers along the second CCpnA direction.
Evidently, the first CCpnA direction distinguishes between mice in the two main age bins. Circles on the left and right of the biplot represent bacteria that are characteristic of younger and older mice, respectively. The second CCpnA direction splits off the few mice in the oldest age group; it also partially distinguishes between the two litters. These samples low in the second CCpnA direction have more of the outlier bacteria than the others.
This CCpnA analysis supports the conclusion that the main difference between the microbiome communities of the different mice lies along the age axis. However, in situations where the influence of environmental variables is not so strong, CCA can have more power in detecting such associations. In general, it can be applied whenever it is desirable to incorporate supplemental data, but in a way that (1) is less aggressive than supervised methods, and (2) can use several environmental variables at once.
9.7 Summary of this chapter
Heterogeneous data A mixture of many continuous and a few categorical variables can be handled by adding the categorical variables as supplementary information to the PCA. This is done by projecting the mean of all points in a group onto the map.
Using distances Relations between data objects can often be summarized as interpoint distances (whether distances between trees, images, graphs, or other complex objects).
Ordination A useful representation of these distances is available through a method similar to PCA called multidimensional scaling (MDS), otherwise known as PCoA (principal coordinate analysis). It can be helpful to think of the outcome of these analyses as uncovering latent variable. In the case of clustering the latent variables are categorical, in ordination they are latent variables like time or environmental gradients like distance to the water. This is why these methods are often called ordination.
Robust versions can be used when interpoint distances are wildly different. NMDS (nonmetric multidimensional scaling) aims to produce coordinates such that the order of the interpoint distances is respected as closely as possible.
Correspondence analysis : a method for computing low dimensional projections that explain dependencies in categorical data. It decomposes chisquare distance in much the same way that PCA decomposes variance. Correspondence analysis is usually the best way to follow up on a significant chisquare test. Once we have ascertained there are significant dependencies between different levels of categories, we can map them and interpret proximities on this map using plots and biplots.
Permutation test for distances Given two sets of distances between the same points, we can measure whether they are related using the Mantel permutation test.
Generalizations of variance and covariance When dealing with more than one matrix of measurements on the same data, we can generalize the notion of covariance and correlations to vectorial measurements of co-inertia.
Canonical correlation is a method for finding a few linear combinations of variables from each table that are as correlated as possible. When using this method on matrices with large numbers of variables, we use a regularized version with an L1 penalty that reduces the number of non-zero coefficients.
9.8 Further reading
Interpretation of PCoA maps and nonlinear embeddings can also be enhanced the way we did for PCA using generalizations of the supplementary point method, see Trosset and Priebe ( 2008 ) or Bengio et al. ( 2004 ) . We saw in Chapter 7 how we can project one categorical variable onto a PCA. The correspondence analysis framework actually allows us to mix several categorical variables in with any number of continuous variables. This is done through an extension called multiple correspondence analysis (MCA) whereby we can do the same analysis on a large number of binary categorical variables and obtain useful maps. The trick here will be to turn the continuous variables into categorical variables first. For extensive examples using R see for instance the book by Pagès ( 2016 ) .
A simple extension to PCA that allows for nonlinear principal curve estimates instead of principal directions defined by eigenvectors was proposed in Hastie and Stuetzle ( 1989 ) and is available in the package princurve .
Finding curved subspaces containing a high density data for dimensions higher than \(1\) is now called manifold embedding and can be done through Laplacian eigenmaps ( Belkin and Niyogi 2003 ) , local linear embedding as in Roweis and Saul ( 2000 ) or using the isomap method ( Tenenbaum, De Silva, and Langford 2000 ) . For textbooks covering nonlinear unsupervised learning methods see Hastie, Tibshirani, and Friedman ( 2008, chap. 14 ) or Izenman ( 2008 ) .
A review of many multitable correlation coefficients, and analysis of applications can be found in Josse and Holmes ( 2016 ) .
9.9 Exercises
Exercise 9.1
We are going to take another look at the Phylochip data, replacing the original expression values by presence/absence. We threshold the data to retain only those that have a value of at least 8.633 in at least 8 samples 7 .
7 These values were chosen to give about retain about 3,000 taxa, similar to our previous choice of threshold.
Perform a correspondence analysis on these binary data and compare the plot you obtain to what we saw in Figure 9.15 .
See Figure 9.37 .

Exercise 9.2
Correspondence Analysis on color association tables: Here is an example of data collected by looking at the number of Google hits resulting from queries of pairs of words. The numbers in Table 9.4 are to be multiplied by 1000. For instance, the combination of the words “quiet” and “blue” returned 2,150,000 hits.
Perform a correspondence analysis of these data. What do you notice when you look at the two-dimensional biplot?
See Figure 9.38 . The code is not rendered here, but is shown in the document’s source file.

Exercise 9.3
The dates Plato wrote his various books are not known. We take the sentence endings and use those pattern frequencies as the data.

From the biplot in Figure 9.39 can you guess at the chronological order of Plato’s works? Hint: the first (earliest) is known to be Republica . The last (latest) is known to be Laws .
Which sentence ending did Plato use more frequently early in his life?
What percentage of the inertia ( \(\chi^2\) -distance) is explained by the map in Figure 9.39 ?
To compute the percentage of inertia explained by the first two axes we take the cumulative sum of the eigenvalues at the value 2:
Exercise 9.4
We are going to look at two datasets, one is a perturbed version of the other and they both present gradients as often seen in ecological data. Read in the two species count matrices lakelike and lakelikeh , which are stored as the object lakes.RData . Compare the output of correspondence analysis and principal component analysis on each of the two data sets; restrict yourself two dimensions. In the plots and the eigenvalues, what do you notice?
Comparison (output not shown):
Exercise 9.5
We analyzed the normalized Moignard data in Section 9.5.1 . Now redo the analysis with the raw data (in file nbt.3154-S3-raw.csv ) and compare the output with that obtained using the normalized values.
Exercise 9.6
We are going to explore the use of kernel methods.
Compute kernelized distances using the kernlab for the Moignard data using various values for the sigma tuning parameter in the definition of the kernels. Then perform MDS on these kernelized distances. What difference is there in variability explained by the first four components of kernel multidimensional scaling?
Make interactive three dimensional representations of the components: is there a projection where you see a branch for the purple points?
- kernelized distances
Use kernelized distances to protect against outliers and allows discovery of non-linear components.
- using a 3d scatterplot interactively:

Exercise 9.7
Higher resolution study of cell data. Take the original expression data blom we generated in Section 9.5.1 . Map the intensity of expression of each of the top 10 most variable genes onto the 3d plot made with the diffusion mapping. Which dimension, or which one of the principal coordinates (1,2,3,4) can be seen as the one that clusters the 4SG (red) points the most?
An implementation in the package LPCM provides the function lpc , which estimates principal curves. Here we constrain ourselves to three dimensions chosen from the output of the diffusion map and create smoothed curves.
To get a feel for what the smoothed data are showing us, we take a look at the interactive graphics using the function plot3d from them rgl package.
One way of plotting both the smoothed line and the data points is to add the line using the plot3d function.

Exercise 9.8
Here we explore more refined distances and diffusion maps that can show cell development trajectories as in Figure 9.45 .

The diffusion map method restricts the estimation of distances to local points, thus further pursuing the idea that often only local distances should be represented precisely and as points become further apart they are not being measured with the same ‘reference’. This method also uses the distances as input but then creates local probabilistic transitions as indicators of similarity, these are combined into an affinity matrix for which the eigenvalues and eigenvectors are also computed much like in standard MDS.
Compare the output of the diffuse function from the diffusionMap package on both the l1 and l2 distances computed between the cells available in the dist2n.euclid and dist1n.l1 objects from Section 9.5.1 .
Notice that the vanilla plot for a dmap object does not allow the use of colors. As this essential to our understanding of cell development, we add the colors by hand. Of course, here we use static 3d plots but these should supplemented by the plot3d examples we give in the code.
We use a tailored wrapper function scp3d , so that we can easily insert relevant parameters:
The best way of visualizing the data is to make a rotatable interactive plot using the rgl package.
Page built on 2023-08-03 21:37:40.85015 using R version 4.3.0 (2023-04-21)

Multivariate analysis — definition, methods, and examples

Multivariate analysis allows you to find patterns between variables, helping you better understand the effects that different factors have on each other and the relationships between them. It represents a critical tool for marketers looking for ways to get deeper insight into the outcome of campaign decisions.
Business owners can also use multivariate analysis to better determine the impact of complex market and global factors on everything from sales to consumer behavior.
This article will demonstrate how multivariate analysis can help you determine how different variables interact with each other in complex scenarios, and the power that comes with identifying patterns and the relationships between those variables. It will also detail multiple methods, examples, and possible use cases to help you understand the strengths of each.
Specifically, it will explain:
- What multivariate analysis is
Multiple linear regression
Multiple logistic regressions, multivariate analysis of variance (manova), factor analysis, cluster analysis, discriminant analysis, conjoint analysis, correlation analysis, what is multivariate analysis.
Multivariate analysis is used to find patterns and correlations between multiple factors by analyzing two or more variables at once. Experts generally group the various approaches of multivariate analysis into two camps — dependent techniques for those instances when the outlined variables depend on one another, and independent techniques for when they do not.
There are many ways to conduct a multivariate analysis. Below we’ll explore some of the most common methods.
Multiple linear regression is an example of a dependent technique that looks at the relationship between one dependent variable and two or more independent variables. For instance, say a couple decides to sell their home. The price they can get for it depends as a variable on many independent factors, including location, time of year, interest rates, and the existence of similar listings in the area.
Let’s think about another example. You want to understand how social media spend across multiple platforms is likely to affect future traffic to your website. In this scenario, the amount and type of spend and platform type are all independent variables, and web traffic is the dependent variable.
Multiple logistic regressions, meanwhile, examine the probability of one of two events occurring. Will one’s partner accept an offer of marriage? Will a child graduate from college? In both scenarios, myriad factors may contribute to the final outcome — has the partner expressed interest in marriage previously? Does the child study hard? Ultimately, both come down to a simple yes or no.
For a business owner trying to determine how likely a certain kind of client is to contract with her demolition company for a job, multiple logistic regression is the way to go.
Multivariate analysis of variance (MANOVA) tests the difference in the effect of multiple independent variables on multiple dependent variables. Say, for example, a marketer wants to study the impact of pairing a price reduction with an increase in campaign budget — both independent variables — on the sales of a certain face cream.
Moving inventory isn’t her only concern, however. She also wants to know what the new price and spend will do to her total revenues on the cream for that period. Both sales and revenues represent dependent variables. In this case, a MANOVA would prove most helpful.
Sometimes too many variables find their way into a dataset. When that happens, it can be difficult to identify any clear patterns in all the information spread out in front of you. Trim back some of those variables using factor analysis, and you can begin to make sense of your data.
Do this by combining closely correlated variables, such as condensing a target audience’s income and education into “socioeconomic status” — or multiple behaviors, such as leaving a positive review and signing up for your newsletter into “customer loyalty.”
Like factor analysis, cluster analysis is a technique you can use to turn down the noise in your data to zero in on what’s most important. Only instead of grouping similar factors together, cluster analysis includes combining similar observations. A scientist performs cluster analysis when, upon discovering new types of deep-sea fish, she organizes them into a single species based on shared traits.
Applied to marketing, cluster analysis represents a powerful market segmentation tool that — when done regularly using powerful, automated software — can help teams deliver personalized experiences based on similar relevant behaviors.
In contrast to factor and cluster analysis, both of which represent independent techniques, discriminant analysis is a dependent technique. Put another way, cluster and factor analysis are exploratory, allowing you to determine the grouping pattern that maximizes similarities within groups — and, by extension, differences between groups.
Discriminant analysis is also concerned with simplifying datasets through classification. However, unlike the previous two types of analysis, it’s a dependent technique. This means it begins with a specific dependent variable, leaving you to focus on the impact your independent variable or variables have on distinguishing between groups. For example, let’s say you want to look at repeat buyers. The repeat buyers represent your dependent variable, a group that you can then examine more closely using any independent variables you have available to you — be it age, location, gender, or something else.
Conjoint analysis refers to the process of surveying consumers to identify which part of a product or service they value most. Used widely in market research, conjoint analysis provides real-world insight into which factors consumers consider most important when making a purchase. Such data is invaluable for minimizing guesswork when it comes to rolling out new products or services (or when tweaking old ones).
Not all feedback deserves equal weight, however. Only invest in a survey that is sure to reach consumers that match your target audience for the product. Just asking the right people isn’t enough. For a response of any real value, you’ll want to be sure to survey as many individuals as possible so as to root out outlying opinions and establish a statistically significant trend.
Equally important for actionable outputs is your survey’s format. Offer too many options, and the respondent becomes overwhelmed. Too few, however, and the survey may fail to pick up on key nuances in consumer preference. One solution researchers have developed to solve this problem is that of asking respondents to rank paired attributes. Do they value clothing that is “affordable and on trend” or “high quality and durable?” This way, the individual taking the survey only has to weigh two options at any given time.
Rarely does life ever offer up just two choices, however, which is why more and more marketers find themselves opting for choice-based conjoint analysis. In this approach, respondents must select one from a handful of full-profile concepts.
For example, say you want to test out an idea for a new winter jacket. Choice-based conjoint analysis would require you to design between three and five different jackets, each with distinct features for respondents to choose from. In the case of a clear winner, you are ready to move forward with confidence knowing that at least compared to the other possibilities, the jacket performs well with your target audience.
Oftentimes the responses are a bit more muddied, however, in which case iteration based on the results of a second or even third survey can help refine your data.
Just because two variables are correlated does not imply causation. Ice cream sales and pool drownings both increase in the summer, but one does not lead to the other. Correlation analysis is designed to help determine the relationship between two variables, including whether or not they are directly linked.
There are three main types of correlation analysis. Pearson’s correlation coefficient is used for linearly related variables — that is to say, as one variable goes up, the other follows (and vice versa). This relationship is measured using a number between 0 and 1, with 1 representing a perfectly linear relationship.
Spearman’s rank-order correlation is used to organize data defined by a set order, also known as ordinal association. For example, say the same runners face off in a series of matches and you want to determine the correlation between contests. You would use their results and Spearman’s rank-order correlation to calculate your answer.
Finally, Kendall’s tau correlation allows you to determine how dependent two variables are on one another, with 0 indicating complete independence and 1 the complete dependence. Note that this goes beyond occurrence. As in the ice cream and drowning example, just because two variables increase in frequency in tandem does not mean they are dependent on each other for happening. Similarly, just because your web traffic and web sales tend to rise and fall in tandem does not necessarily mean one causes another.
For marketers and business owners, correlation analysis can help take the guesswork out of where a company should invest in order to boost its bottom line. Suppose you notice that those consumers who test-drive the cars at your dealership for a minimum of 15 minutes are twice as likely to purchase a vehicle that same day. You want to know if it’s that extra time in the car that pushes them over the edge, or if customers are simply driving the cars longer because they already know they’re inclined to sign the dotted line.
Correlation analysis can help clarify the relationship between the two and possibly uncover a critical consumer behavior for driving sales.
Conducting your own multivariate analyses
Multivariate analysis helps you understand complex scenarios by uncovering patterns and relationships between multiple variables.
The term itself may sound like a highly technical and specialized skill. But in truth, most of us are engaged in some form of multivariate analysis all day long. New parents try to tease out how nap lengths, feeding intervals, and sleep environment combine to influence the number of times a child wakes up during the night. Homeowners must consider a dozen factors — some personal, some global — when deciding whether or not to refinance.
Likewise, marketers understand intuitively that sales and revenue are the result of many factors, ranging from price and campaign spending all the way up to inflation and global market trends.
Ready to get started on your own multivariate analysis? Cluster analysis is a great way to use your existing customer segmentation data to find correlations that might reveal a group that could benefit from a targeted campaign.
Adobe Target allows you to quickly perform multivariate testing, helping you to deliver more personalized experiences to your customers.
Watch an overview video to find out how Adobe Target can help you conduct your own multivariate analyses.
https://business.adobe.com/blog/basics/define-data-analytics
https://business.adobe.com/blog/basics/cluster-analysis-definition
https://business.adobe.com/blog/basics/market-segmentation-examples

R (BGU course)
Chapter 9 multivariate data analysis.
The term “multivariate data analysis” is so broad and so overloaded, that we start by clarifying what is discussed and what is not discussed in this chapter. Broadly speaking, we will discuss statistical inference , and leave more “exploratory flavored” matters like clustering, and visualization, to the Unsupervised Learning Chapter 11 .
We start with an example.
Formally, let \(y\) be single (random) measurement of a \(p\) -variate random vector. Denote \(\mu:=E[y]\) . Here is the set of problems we will discuss, in order of their statistical difficulty.
Signal Detection : a.k.a. multivariate test , or global test , or omnibus test . Where we test whether \(\mu\) differs than some \(\mu_0\) .
Signal Counting : a.k.a. prevalence estimation , or \(\pi_0\) estimation . Where we count the number of entries in \(\mu\) that differ from \(\mu_0\) .
Signal Identification : a.k.a. selection , or multiple testing . Where we infer which of the entries in \(\mu\) differ from \(\mu_0\) . In the ANOVA literature, this is known as a post-hoc analysis, which follows an omnibus test .
Estimation : Estimating the magnitudes of entries in \(\mu\) , and their departure from \(\mu_0\) . If estimation follows a signal detection or signal identification stage, this is known as selective estimation .
9.1 Signal Detection
Signal detection deals with the detection of the departure of \(\mu\) from some \(\mu_0\) , and especially, \(\mu_0=0\) . This problem can be thought of as the multivariate counterpart of the univariate hypothesis t-test.
9.1.1 Hotelling’s T2 Test
The most fundamental approach to signal detection is a mere generalization of the t-test, known as Hotelling’s \(T^2\) test .
Recall the univariate t-statistic of a data vector \(x\) of length \(n\) : \[\begin{align} t^2(x):= \frac{(\bar{x}-\mu_0)^2}{Var[\bar{x}]}= (\bar{x}-\mu_0)Var[\bar{x}]^{-1}(\bar{x}-\mu_0), \tag{9.1} \end{align}\] where \(Var[\bar{x}]=S^2(x)/n\) , and \(S^2(x)\) is the unbiased variance estimator \(S^2(x):=(n-1)^{-1}\sum (x_i-\bar x)^2\) .
Generalizing Eq (9.1) to the multivariate case: \(\mu_0\) is a \(p\) -vector, \(\bar x\) is a \(p\) -vector, and \(Var[\bar x]\) is a \(p \times p\) matrix of the covariance between the \(p\) coordinated of \(\bar x\) . When operating with vectors, the squaring becomes a quadratic form, and the division becomes a matrix inverse. We thus have \[\begin{align} T^2(x):= (\bar{x}-\mu_0)' Var[\bar{x}]^{-1} (\bar{x}-\mu_0), \tag{9.2} \end{align}\] which is the definition of Hotelling’s \(T^2\) one-sample test statistic. We typically denote the covariance between coordinates in \(x\) with \(\hat \Sigma(x)\) , so that \(\widehat \Sigma_{k,l}:=\widehat {Cov}[x_k,x_l]=(n-1)^{-1} \sum (x_{k,i}-\bar x_k)(x_{l,i}-\bar x_l)\) . Using the \(\Sigma\) notation, Eq. (9.2) becomes \[\begin{align} T^2(x):= n (\bar{x}-\mu_0)' \hat \Sigma(x)^{-1} (\bar{x}-\mu_0), \end{align}\] which is the standard notation of Hotelling’s test statistic.
For inference, we need the null distribution of Hotelling’s test statistic. For this we introduce some vocabulary 17 :
- Low Dimension : We call a problem low dimensional if \(n \gg p\) , i.e. \(p/n \approx 0\) . This means there are many observations per estimated parameter.
- High Dimension : We call a problem high dimensional if \(p/n \to c\) , where \(c\in (0,1)\) . This means there are more observations than parameters, but not many.
- Very High Dimension : We call a problem very high dimensional if \(p/n \to c\) , where \(1<c<\infty\) . This means there are less observations than parameters.
Hotelling’s \(T^2\) test can only be used in the low dimensional regime. For some intuition on this statement, think of taking \(n=20\) measurements of \(p=100\) physiological variables. We seemingly have \(20\) observations, but there are \(100\) unknown quantities in \(\mu\) . Say you decide that \(\mu\) differs from \(\mu_0\) based on the coordinate with maximal difference between your data and \(\mu_0\) . Do you know how much variability to expect of this maximum? Try comparing your intuition with a quick simulation. Did the variabilty of the maximum surprise you? Hotelling’s \(T^2\) is not the same as the maxiumum, but the same intuition applies. This criticism is formalized in Bai and Saranadasa ( 1996 ) . In modern applications, Hotelling’s \(T^2\) is rarely recommended. Luckily, many modern alternatives are available. See J. Rosenblatt, Gilron, and Mukamel ( 2016 ) for a review.
9.1.2 Various Types of Signal to Detect
In the previous, we assumed that the signal is a departure of \(\mu\) from some \(\mu_0\) . For vactor-valued data \(y\) , that is distributed \(\mathcal F\) , we may define “signal” as any departure from some \(\mathcal F_0\) . This is the multivaraite counterpart of goodness-of-fit (GOF) tests.
Even when restricting “signal” to departures of \(\mu\) from \(\mu_0\) , “signal” may come in various forms:
- Dense Signal : when the departure is in a large number of coordinates of \(\mu\) .
- Sparse Signal : when the departure is in a small number of coordinates of \(\mu\) .
Process control in a manufactoring plant, for instance, is consistent with a dense signal: if a manufacturing process has failed, we expect a change in many measurements (i.e. coordinates of \(\mu\) ). Detection of activation in brain imaging is consistent with a dense signal: if a region encodes cognitive function, we expect a change in many brain locations (i.e. coordinates of \(\mu\) .) Detection of disease encodig regions in the genome is consistent with a sparse signal: if susceptibility of disease is genetic, only a small subset of locations in the genome will encode it.
Hotelling’s \(T^2\) statistic is best for dense signal. The next test, is a simple (and forgotten) test best with sparse signal.
9.1.3 Simes’ Test
Hotelling’s \(T^2\) statistic has currently two limitations: It is designed for dense signals, and it requires estimating the covariance, which is a very difficult problem.
An algorithm, that is sensitive to sparse signal and allows statistically valid detection under a wide range of covariances (even if we don’t know the covariance) is known as Simes’ Test . The statistic is defined vie the following algorithm:
- Compute \(p\) variable-wise p-values: \(p_1,\dots,p_j\) .
- Denote \(p_{(1)},\dots,p_{(j)}\) the sorted p-values.
- Simes’ statistic is \(p_{Simes}:=min_j\{p_{(j)} \times p/j\}\) .
- Reject the “no signal” null hypothesis at significance \(\alpha\) if \(p_{Simes}<\alpha\) .
9.1.4 Signal Detection with R
We start with simulating some data with no signal. We will convince ourselves that Hotelling’s and Simes’ tests detect nothing, when nothing is present. We will then generate new data, after injecting some signal, i.e., making \(\mu\) depart from \(\mu_0=0\) . We then convince ourselves, that both Hotelling’s and Simes’ tests, are indeed capable of detecting signal, when present.
Generating null data:
Now making our own Hotelling one-sample \(T^2\) test using Eq.( (9.2) ).
Things to note:
- stopifnot(n > 5 * p) is a little verification to check that the problem is indeed low dimensional. Otherwise, the \(\chi^2\) approximation cannot be trusted.
- solve returns a matrix inverse.
- %*% is the matrix product operator (see also crossprod() ).
- A function may return only a single object, so we wrap the statistic and its p-value in a list object.
Just for verification, we compare our home made Hotelling’s test, to the implementation in the rrcov package. The statistic is clearly OK, but our \(\chi^2\) approximation of the distribution leaves room to desire. Personally, I would never trust a Hotelling test if \(n\) is not much greater than \(p\) , in which case I would use a high-dimensional adaptation (see Bibliography).
Let’s do the same with Simes’:
And now we verify that both tests can indeed detect signal when present. Are p-values small enough to reject the “no signal” null hypothesis?
… yes. All p-values are very small, so that all statistics can detect the non-null distribution.
9.2 Signal Counting
There are many ways to approach the signal counting problem. For the purposes of this book, however, we will not discuss them directly, and solve the signal counting problem as a signal identification problem: if we know where \(\mu\) departs from \(\mu_0\) , we only need to count coordinates to solve the signal counting problem.
9.3 Signal Identification
The problem of signal identification is also known as selective testing , or more commonly as multiple testing .
In the ANOVA literature, an identification stage will typically follow a detection stage. These are known as the omnibus F test , and post-hoc tests, respectively. In the multiple testing literature there will typically be no preliminary detection stage. It is typically assumed that signal is present, and the only question is “where?”
The first question when approaching a multiple testing problem is “what is an error”? Is an error declaring a coordinate in \(\mu\) to be different than \(\mu_0\) when it is actually not? Is an error an overly high proportion of falsely identified coordinates? The former is known as the family wise error rate (FWER), and the latter as the false discovery rate (FDR).
9.3.1 Signal Identification in R
One (of many) ways to do signal identification involves the stats::p.adjust function. The function takes as inputs a \(p\) -vector of the variable-wise p-values . Why do we start with variable-wise p-values, and not the full data set?
- Because we want to make inference variable-wise, so it is natural to start with variable-wise statistics.
- Because we want to avoid dealing with covariances if possible. Computing variable-wise p-values does not require estimating covariances.
- So that the identification problem is decoupled from the variable-wise inference problem, and may be applied much more generally than in the setup we presented.
We start be generating some high-dimensional multivariate data and computing the coordinate-wise (i.e. hypothesis-wise) p-value.
We now compute the pvalues of each coordinate. We use a coordinate-wise t-test. Why a t-test? Because for the purpose of demonstration we want a simple test. In reality, you may use any test that returns valid p-values.
- t.pval is a function that merely returns the p-value of a t.test.
- We used the apply function to apply the same function to each column of x .
- MARGIN=2 tells apply to compute over columns and not rows.
- The output, p.values , is a vector of 100 p-values.
We are now ready to do the identification, i.e., find which coordinate of \(\mu\) is different than \(\mu_0=0\) . The workflow for identification has the same structure, regardless of the desired error guarantees:
- Compute an adjusted p-value .
- Compare the adjusted p-value to the desired error level.
If we want \(FWER \leq 0.05\) , meaning that we allow a \(5\%\) probability of making any mistake, we will use the method="holm" argument of p.adjust .
If we want \(FDR \leq 0.05\) , meaning that we allow the proportion of false discoveries to be no larger than \(5\%\) , we use the method="BH" argument of p.adjust .
We now inject some strong signal in \(\mu\) just to see that the process works. We will artificially inject signal in the first 10 coordinates.
Indeed- we are now able to detect that the first coordinates carry signal, because their respective coordinate-wise null hypotheses have been rejected.
9.4 Signal Estimation (*)
The estimation of the elements of \(\mu\) is a seemingly straightforward task. This is not the case, however, if we estimate only the elements that were selected because they were significant (or any other data-dependent criterion). Clearly, estimating only significant entries will introduce a bias in the estimation. In the statistical literature, this is known as selection bias . Selection bias also occurs when you perform inference on regression coefficients after some model selection, say, with a lasso, or a forward search 18 .
Selective inference is a complicated and active research topic so we will not offer any off-the-shelf solution to the matter. The curious reader is invited to read Rosenblatt and Benjamini ( 2014 ) , Javanmard and Montanari ( 2014 ) , or Will Fithian’s PhD thesis (Fithian 2015 ) for more on the topic.
9.5 Bibliographic Notes
For a general introduction to multivariate data analysis see Anderson-Cook ( 2004 ) . For an R oriented introduction, see Everitt and Hothorn ( 2011 ) . For more on the difficulties with high dimensional problems, see Bai and Saranadasa ( 1996 ) . For some cutting edge solutions for testing in high-dimension, see J. Rosenblatt, Gilron, and Mukamel ( 2016 ) and references therein. Simes’ test is not very well known. It is introduced in Simes ( 1986 ) , and proven to control the type I error of detection under a PRDS type of dependence in Benjamini and Yekutieli ( 2001 ) . For more on multiple testing, and signal identification, see Efron ( 2012 ) . For more on the choice of your error rate see Rosenblatt ( 2013 ) . For an excellent review on graphical models see Kalisch and Bühlmann ( 2014 ) . Everything you need on graphical models, Bayesian belief networks, and structure learning in R, is collected in the Task View .
9.6 Practice Yourself
Generate multivariate data with:
- Use Hotelling’s test to determine if \(\mu\) equals \(\mu_0=0\) . Can you detect the signal?
- Perform t.test on each variable and extract the p-value. Try to identify visually the variables which depart from \(\mu_0\) .
- Use p.adjust to identify in which variables there are any departures from \(\mu_0=0\) . Allow 5% probability of making any false identification.
- Use p.adjust to identify in which variables there are any departures from \(\mu_0=0\) . Allow a 5% proportion of errors within identifications.
- Do we agree the groups differ?
- Implement the two-group Hotelling test described in Wikipedia: ( https://en.wikipedia.org/wiki/Hotelling%27s_T-squared_distribution#Two-sample_statistic ).
- Verify that you are able to detect that the groups differ.
- Perform a two-group t-test on each coordinate. On which coordinates can you detect signal while controlling the FWER? On which while controlling the FDR? Use p.adjust .
Return to the previous problem, but set n=9 . Verify that you cannot compute your Hotelling statistic.
Anderson-Cook, Christine M. 2004. “An Introduction to Multivariate Statistical Analysis.” Journal of the American Statistical Association 99 (467). American Statistical Association: 907–9.
Bai, Zhidong, and Hewa Saranadasa. 1996. “Effect of High Dimension: By an Example of a Two Sample Problem.” Statistica Sinica . JSTOR, 311–29.
Benjamini, Yoav, and Daniel Yekutieli. 2001. “The Control of the False Discovery Rate in Multiple Testing Under Dependency.” Annals of Statistics . JSTOR, 1165–88.
Efron, Bradley. 2012. Large-Scale Inference: Empirical Bayes Methods for Estimation, Testing, and Prediction . Vol. 1. Cambridge University Press.
Everitt, Brian, and Torsten Hothorn. 2011. An Introduction to Applied Multivariate Analysis with R . Springer Science & Business Media.
Fithian, William. 2015. “Topics in Adaptive Inference.” PhD thesis, STANFORD UNIVERSITY.
Javanmard, Adel, and Andrea Montanari. 2014. “Confidence Intervals and Hypothesis Testing for High-Dimensional Regression.” Journal of Machine Learning Research 15 (1): 2869–2909.
Kalisch, Markus, and Peter Bühlmann. 2014. “Causal Structure Learning and Inference: A Selective Review.” Quality Technology & Quantitative Management 11 (1). Taylor & Francis: 3–21.
Rosenblatt, Jonathan. 2013. “A Practitioner’s Guide to Multiple Testing Error Rates.” arXiv Preprint arXiv:1304.4920 .
Rosenblatt, Jonathan D, and Yoav Benjamini. 2014. “Selective Correlations; Not Voodoo.” NeuroImage 103. Elsevier: 401–10.
Rosenblatt, Jonathan, Roee Gilron, and Roy Mukamel. 2016. “Better-Than-Chance Classification for Signal Detection.” arXiv Preprint arXiv:1608.08873 .
Simes, R John. 1986. “An Improved Bonferroni Procedure for Multiple Tests of Significance.” Biometrika 73 (3). Oxford University Press: 751–54.
This vocabulary is not standard in the literature, so when you read a text, you will need to verify yourself what the author means. ↩
You might find this shocking, but it does mean that you cannot trust the summary table of a model that was selected from a multitude of models. ↩
Multivariate Analysis: A Complete And Easy Guide For 2021
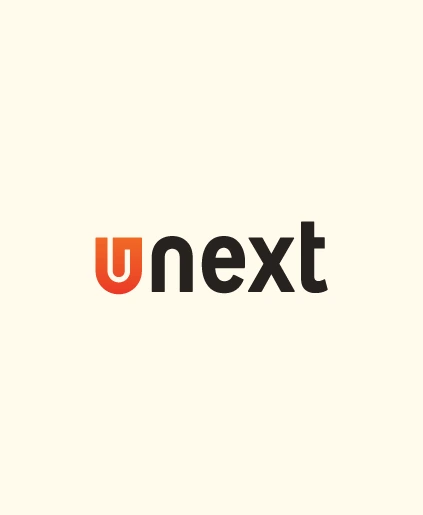
Introduction
Data is crucial today in short Data can also be described as ‘new oil’. Data is used in large organizations to learn the consumer behaviour of what makes them invest in a particular product. The huge amount of data is grouped to analyze and learn and understand the consumer. The multivariate analysis definition is analyzing the data is known as Multivariate analysis. Here is an introduction to multivariate statistical analysis.
- What is Multivariate Analysis?
- History of Multivariate Analysis
- The objective of Multivariate Analysis
- Types of Multivariate Analysis of Variance and Covariance
- Advantages and Disadvantages of Multivariate Analysis
1) What is Multivariate Analysis?
Multivariate means more than one variable behind the resultant outcome. Anything that happens in the world or business is not due to one reason but multiple reasons behind the outcome known as multivariate. With the introduction to multivariate analysis let’s take an example. Weather is dependent on multiple factors like pollution, precipitation, humidity to name a few. Now knowing the multivariate analysis meaning, let’s take a look at the multivariate analysis applications, the history behind the multivariate analysis, and applied multivariate analysis in various fields.
2) History of Multivariate Analysis
The Multivariate analysis (MVA) was started in 1928 by Wishart presenting the paper. The paper was about the distribution of the covariance matrix of a normal population with multiple variables. Later, in the 1930s Hotelling, R. A Fischer, and others published theoretical work on MVA. During those times, multivariate analysis was widely found in education, psychology, and biology fields.
With the advent of computers, multivariate analysis expanded its area to meteorological, geological, science, and medical sectors in the mid-1950s. New theories were proposed and tested at regular intervals by practice at the same time in different fields. Computers opened new venues to apply the MVA methods to verify the complex statistical dataset for multivariate analysis.
3) The objective of Multivariate Analysis
MVA or Multivariate Analysis considers multiple factors. The objectives of MVA are listed below.
- Reduction in data or simplification of the structure
MVA helps to simplify the data as much as possible without losing out on the critical information. This aids in drawing interpretation later.
- Grouping and Sorting the data
MVA has multiple variables. The variables are grouped based on their unique features.
- Data is verified based on the variables
Understanding the variables and collected data is verified. Concluding, the state of the variables is critical. The variables can be independent or dependent on the other variables.
- Establishing a connection between the variables
The relationship between the variables is vital to understand the behavior of the variables based on observations and other variables present.
- Testing and construction of hypothesis
Creating a statistical hypothesis based on the parameters of the multivariate data is tested. This testing is done to understand if the assumptions are correct or not.
4) Types of Multivariate Analysis of Variance and Covariance
MANOVA is a Multivariate analysis of variance a continuance of the ANOVA (common analysis of variance). The MANOVA includes more than one factor with two or more than two interdependent variables. The multivariate analysis is a continuance of the linear model approach as found in ANOVA. The various multivariate analysis techniques in research methodology are listed below .
- Canonical Correlation Analysis
The canonical correlation analysis is a study of the straight line relations between two types of variables. The CCA has two main purposes. They are
- Reduction of Data
- Interpretation of Data
Computation of all probability correlations is performed between the two types of variables. The interpretation can be challenging when the two types of correlations are large. CCA helps to outline the relationship between the two variables.
- Structural Equation Modelling
SEM is a multivariate statistical analysis technique applied to analyze the structural relationships. This is a flexible and broad network of data analysis. SEM assesses the variables that are dependent and independent. Further, metrics of latent variables and verification of the model measurement is taken. SEM is a combination of analysis of the metrics and structural model. This considers the errors in measurement and variables observed for multivariate data analysis. The multivariate analysis tools are used to evaluate the variables. This is a vital part of the SEM model.
- Interdependence technique
In this technique, the relationships of the variables are analyzed to understand. This helps in establishing the pattern of the data and assumptions behind the variables.
- Factor Analysis
In factor analysis data in many variables are reduced to few variables. It is also known as dimension reduction. This technique is used to reduce the data before going ahead with analysis. On completion of factor analysis, the patterns are clear and much easier to analyze.
- Cluster Analysis
Cluster analysis is a combination of techniques that are used to segregate the cases or objects into groups known as clusters. While conducting the analysis, the data is separated based on similarity and then labelled to the group. This is a data mining function and allows them to gain insight into the data distribution based on the unique feature of each group.
- Multidimensional Scaling
MDS or multidimensional scaling is a technique wherein a map is developed with positions of the variables along with the distances between them in a table. The map can have one or more dimensions. The program can provide a metric or non-metric solution. The tabular details of the distances are called the proximity matrix. This tabular column is updated from the results of the experiments or by a correlation matrix.
- Correspondence analysis
A correspondence analysis method has a table that has a two-way array of non-negative quantities. This array gives the relation between the row entry and the column entry of the table. A common multivariate analysis example is a table of contingency in which the column and row entries refer to the two variables and the quantities in the table cells refer to frequencies.
5) Advantages and Disadvantages of Multivariate Analysis
Multivariate Analysis aids in understanding the behaviour of the variables. It also gives a peek to know the dependence of the variables and how they can influence the outcomes.
- MVA considers multiple variables. These variables can be independent or dependent on each other. The analysis considers the factors and draws an accurate conclusion.
- The analysis is tested and conclusions are drawn. The drawn conclusions are close to real-life situations.
Disadvantages
- MVA is laborious and as it includes complex computations.
- The analysis requires a huge amount of observations for multiple variables that are collected and tabulated. This observation process is time-consuming.
The multivariate analysis techniques are being used at large by organizations. This applied multivariate statistical analysis is the outcome of the multivariate correlation analysis is the basis for the sales plan. These methods of multivariate analysis are used to set goals too in the organizations.
If you are interested in making a career in the Data Science domain, our 11-month in-person Postgraduate Certificate Diploma in Data Science course can help you immensely in becoming a successful Data Science professional.
- What is Data Visualization In 2020?

Fill in the details to know more

PEOPLE ALSO READ
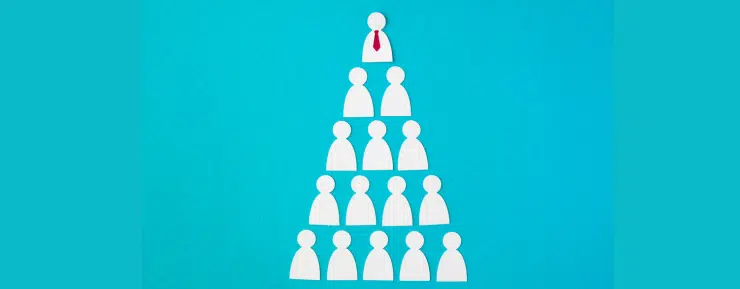
Related Articles

From The Eyes Of Emerging Technologies: IPL Through The Ages
April 29, 2023
Data Visualization Best Practices
March 23, 2023
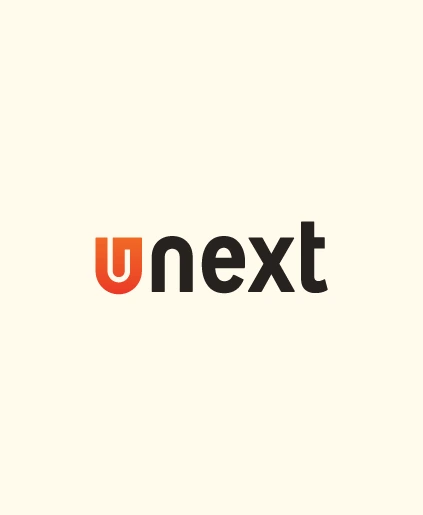
What Are Distribution Plots in Python?
March 20, 2023
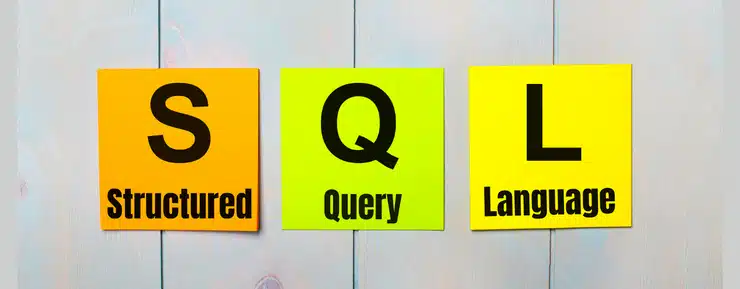
What Are DDL Commands in SQL?
March 10, 2023
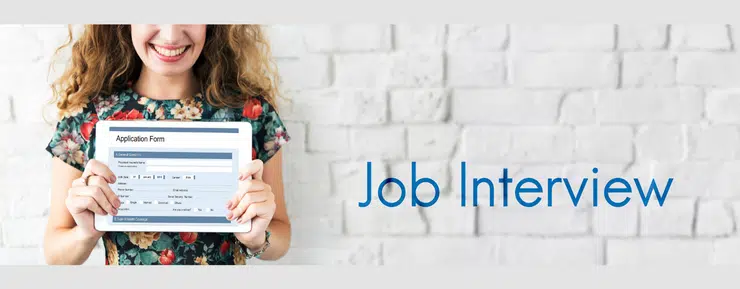
Best TCS Data Analyst Interview Questions and Answers for 2023
March 7, 2023
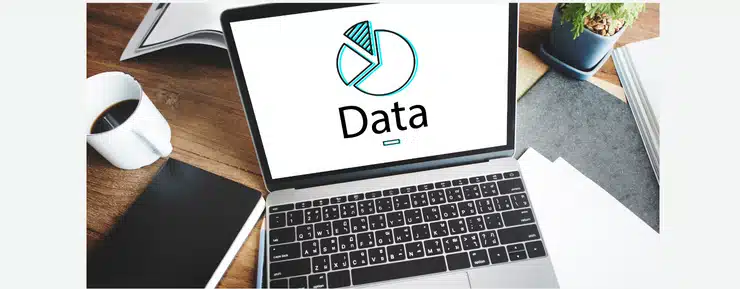
Best Data Science Companies for Data Scientists !
February 26, 2023

Are you ready to build your own career?

Query? Ask Us
Enter Your Details ×

Chapter 3 -- Introducing a Control Variable (Multivariate Analysis)
Human behavior is usually too complicated to be studied with only two variables. Often we will want to consider sets of three or more variables (called multivariate analysis ). We will want to consider three or more variables when we have discovered a relationship between two variables and want to find out 1) if this relationship might be due to some other factor, 2) how or why these variables are related, or 3) if the relationship is the same for different types of individuals. In each situation, we identify a third variable that we want to consider. This is called the control or the test variable . (Although it is possible to use several control variables simultaneously, we will limit ourselves to one control variable at a time.) To introduce a third variable, we identify the control variable and separate the cases in our sample by the categories of the control variable. For example, if the control variable is age divided into these two categories--younger and older, we would separate the cases into two groups. One group would consist of individuals who are younger and the other group would be those who are older. We would then obtain the crosstabulation of the independent and dependent variables for each of these age groups. Since there are two categories in this control variable, we obtain two partial tables , each containing part of the original sample. (If there were three categories in our control variable, for example, young, middle aged, and old, we would have three partial tables.) The process of using a control variable in the analysis is called elaboration and was developed at Columbia University by Paul Lazarsfeld and his associates. There are several different types of outcomes to the elaboration process. We will discuss each briefly. Table 2.3 showed that females were more likely than males to say they were willing to vote for a woman. Let's introduce a control variable and see what happens. In this example we are going to use age as the control variable. Table 3.1 is the three-variable table with voting preference as the dependent variable, sex as the independent variable, and age as the control variable. When we look at the older respondents (the left-hand partial table), we discover that this partial table is very similar to the original two-variable table (Table 2.3). The same is true for the younger respondents (the right-hand partial table). Each partial table is very similar to the original two-variable table. This is often referred to as replication because the partial tables repeat the original two-variable table (see Babbie 1997: 393-396). It is not necessary that they be identical; just that each partial table be basically the same as the original two-variable table. Our conclusion is that age is not affecting the relationship between sex and voting preference. In other words, the difference between males and females in voting preference is not due to age. Table 3.1 -- Voting Preference by Sex Controlling for Age Older Younger Male % Female % Total % Male % Female % Total % Voting Preference Willing to Vote for a Woman 43.8 56.1 49.0 44.2 55.8 52.9 Not Willing to Vote for a Woman 56.2 43.9 51.0 55.8 44.2 100.0 100.0 100.0 100.0 100.0 100.0 (240) (180) (420) (120) (360) (480) Since this is a hypothetical example, imagine a different outcome. Suppose we introduce age as a control variable and instead of getting Table 2.1, we get Table 3.2. How do these two tables differ? In Table 3.2, the percentage difference between males and females has disappeared in both of the partial tables. This is called explanation because the control variable, age, has explained away the original relationship between sex and voting preference. (We often say that the relationship between the two variables is spurious , not genuine.) When age is held constant, the difference between males and females disappears. The difference in the relationship does not have to disappear entirely, only be reduced substantially in each of the partial tables. This can only occur when there is a relationship between the control variable (age) and each of the other two variables (sex and voting preference). Next, we are interested in how or why the two variables are related. Suppose females are more likely than males to vote for a woman and that this difference cannot be explained away by age or by any other variable we have considered. We need to think about why there might be such a difference in the preferences of males and females. Perhaps females are more often liberal Table 3.2 -- Voting Preference by Sex Controlling for Age Older Younger Male % Female % Total % Male % Female % Total % Voting Preference Willing to Vote for a Woman 32.9 33.9 33.3 65.8 66.9 66.7 Not Willing to Vote for a Woman 67.1 66.1 66.7 34.2 33.1 33.3 100.0 100.0 100.0 100.0 100.0 100.0 (240) (180) (420) (120) (360) (480) than males, and liberals are more likely to say they would vote for a woman. So we introduce liberalism/conservatism as a control variable in our analysis. If females are more likely to support a woman because they are more liberal, then the difference between the preferences of men and women should disappear or be substantially reduced when liberalism/conservatism is held constant. This process is called interpretation because we are interpreting how one variable is related to another variable. Table 3.3 shows what we would expect to find if females supported the woman because they were more liberal. Notice that in both partial tables, the differences in the percentages between men and women has disappeared. (It is not necessary that it disappears entirely, but only that it is substantially reduced in each of the partial tables.) Table 3.3 -- Voting Preference by Sex Controlling for Liberalism/Conservatism Older Younger Male % Female % Total % Male % Female % Total % Voting Preference Willing to Vote for a Woman 32.9 33.9 33.3 65.8 66.9 66.7 Not Willing to Vote for a Woman 67.1 66.1 66.7 34.2 33.1 33.3 100.0 100.0 100.0 100.0 100.0 100.0 (240) (180) (420) (120) (360) (480) Finally, let's focus on the third of the situations outlined at the beginning of this section--whether the relationship is the same for different types of individuals. Perhaps the relationship between sex and voter preference varies with other characteristics of the individuals. Maybe among whites, females are more likely to prefer women candidates than the males are, but among blacks, there is little difference between males and females in terms of voter preference. This is the outcome shown in Table 3.4. This process is called specification because it specifies the conditions under which the relationship between sex and voter preference varies. In the earlier section on bivariate analysis, we discussed the use of chi square. Remember that chi square is a test of independence used to determine if there is a relationship between two variables. Chi square is used in multivariate analysis the same way it is in bivariate analysis. There will be a separate value of chi square for each partial table in the multivariate analysis. You should keep a number of warnings in mind. Chi square assumes that the expected frequencies for each cell are five or larger. As long as 80% of these expected frequencies are five or larger and no single expected frequency is very small, we don't have to worry. However, the expected frequencies often drop below five when the number of cases in a column or row gets too small. If this should occur, you will have to either recode (i.e., combine columns or rows) or eliminate a column or row from the table. Table 3.4 -- Voting Preference by Sex Controlling for Race White African American Male % Female % Total % Male % Female % Total % Voting Preference Willing to Vote for a Woman 42.9 56.5 51.2 50.0 50.0 50.0 Not Willing to vote for a Woman 57.1 43.5 48.8 50.0 50.0 50.0 100.00 100.00 100.00 100.00 100.00 100.00 (310) (490) (800) (50) (50) (100) Another point to keep in mind is that chi square is affected by the number of cases in the table. With a lot of cases it is easy to reject the null hypothesis of no relationship. With a few cases, it can be quite hard to reject the null hypothesis. Also, consider the percentages within the table. Look for patterns. Do not rely on any single piece of information. Look at the whole picture. We have concentrated on crosstabulation and chi square. There are other types of statistical analysis such as regression and log-linear analysis. When you have mastered these techniques, look at some other types of analysis. REFERENCES AND SUGGESTED READING Methods of Social Research Riley, Matilda White. 1963. Sociological Research I: A Case Approach . New York: Harcourt, Brace and World. Survey Research and Sampling Babbie, Earl R. 1990. Survey Research Methods (2 nd Ed.). Belmont, CA: Wadsworth. Babbie, Earl R. 1997. The Practice of Social Research (8 th Ed.). Belmont, CA: Wadsworth. Statistical Analysis K noke, David, and George W. Bohrnstedt. 1991. Basic Social Statistics . Itesche, IL: Peacock. Riley, Matilda White. 1963. Sociological Research II Exercises and Manual . New York: Harcourt, Brace & World. Norusis, Marija J. 1997. SPSS 7.5 Guide to Data Analysis . Upper Saddle River, New Jersey: Prentice Hall. Elaboration and Causal Analysis Hirschi, Travis and Hanan C. Selvin. 1967. Delinquency Research--An Appraisal of Analytic Methods . New York: Free Press. Rosenberg, Morris. 1968. The Logic of Survey Analysis . New York: Basic Books. Data Sources The Field Institute. 1985. California Field Poll Study, July, 1985 . Machine-readable codebook. The Field Institute. 1991. California Field Poll Study, September, 1991 . Machine-readable codebook. The Field Institute. 1995. California Field Poll Study, February, 1995. Machine-readable codebook.
Document Viewers
- Free PDF Viewer
- Free Word Viewer
- Free Excel Viewer
- Free PowerPoint Viewer


- Media Centre |
- Campus Tour |
- Vision and Mission
- Chairman’s Message
- Director’s Message
- Executive Board
- Academic Council
- Recognitions & Accreditations
- Courses in Full time PGDM
- Courses in Full time PGDM IB
- Courses in PGDM Financial Management
- Courses in PGDM (Big Data Analytics)
- Full-Time Fellow Program in Management (FPM)
- Full-Time Executive PGDM
- Merit-cum-Means Scholarships
- Duplicate Diploma Mark Sheet
- International Immersion Program
- Student Exchange Program
- Full Time PGDM
- Full Time PGDM IB
- Full Time PGDM FM
- Full Time PGDM(BDA)
- Executive PGDM
- Full Time Fellow Program in Management
- Placement Process
- Placement Team
- Placement Report 2020
- Placement Report 2019
- Placement Report 2018
- Placement Report 2017
- Online Registration Payment
- Open MDP Calendar
- Registration & Payment
- Online MDP Training Calendar
- 11 Months Online Professional Certificate in Big Data
- Deep Learning And Artificial Intelligence
- Certificate in Machine Learning & Deep Learning
- Big Data And Data Analytics Program
- Online Programme on Big Data and AI Techniques
- Online Hands-on Machine Learning and Artificial Intelligence Program
- Corporate Training Programs
- Participating Companies
- Testimonials
- Corporate Brochure
- FDP Calendar 2020-2021
- FDP Calendar 2019-2020
- All Faculty
- Economics And Business Policy
- Finance And Accounting
- Information Technology
- International Business
- Organisational Behaviour & Human Resource
- Quantitative Techniques and Operations Management
- Communication
- Faculty Speaks
- Visiting Faculty
- Labour Compliances
- Resources and Services
- Membership and Privileges
- Ask the Librarian
- Computer Centre
- Hostel Facilities
- Language Laboratory
- Faculty Publication 2020
- Faculty Publication 2019
- Faculty Publications 2018
- Faculty Publications 2017
- Faculty Publications 2016
- Faculty Publications 2015
- Book Publications
- Faculty Publications 2014
- Faculty Publications 2013
- Forthcoming Books
- Abhigyan 2020
- Abhigyan 2019
- Abhigyan 2018
- Abhigyan 2017
- Abhigyan 2016
- Abhigyan 2015
- Abhigyan 2014
- Abhigyan 2013
- Abhigyan 2012 and Archive
- Working Paper 2020
- Working Paper 2019
- Working Paper 2018
- Working Paper 2017
- Working Paper 2016
- Working Paper 2015
- Working Papers 2014
- Working Papers 2013
- Working Papers 2012 and Archive
- FOREprints Newsletter of FORE 2020
- FOREprints Newsletter of FORE 2019
- FOREprints Newsletter of FORE 2018
- FOREprints Newsletter of FORE 2017
- FOREprints Newsletter of FORE 2016
- FOREprints Newsletter of FORE 2015
- FOREprints Newsletter of FORE 2014
- FOREprints Newsletter of FORE 2013
- FOREprints Newsletter of FORE 2012
- Past Conferences
- Upcoming Conference
- Center for Customer Management and Analytics
- Center for Digital Innovation
- Center for Entrepreneurship Development
- Center for Operations and Supply Chain Management
- Center for Psychometric Testing and Research
- Center for Sustainable Development
- Center for Research and Innovation in Frugal Technology Management (CRIFT)
- eLearning and eWorking Platform
- TEDxFORESchool
- Centre for Entrepreneurship Development
Introduction to Multivariate Data Analysis
What is multivariate data analysis.
Data analysis is as old as data itself, however very early forms were limited due to the manual nature of analysis and later forms by computing power limitations. The explosion in data coincided with build-up of computer processing capacities and advanced data analysis techniques became accessible to governments, then organizations, and later, to anyone with a modern day personal computer.
Multivariate statistics involves observation and analysis of multiple statistical variables at a time. The objective is to detect patterns and relationships between several variables simultaneously, and draw conclusions from them. The conclusions are usually in the form of establishing relationships that allow us to predict the effect of a change in one variable on other variables. Researchers typically use multivariate procedures when they are interested in studying more than one outcome simultaneously (also known as the dependent or phenomenon of interest), more than one impacting variable (also known as an independent or predictor), or both. This gives multivariate analysis a decisive advantage over other forms of analysis. This type of analysis is also desirable because researchers often hypothesize that a given outcome of interest is influenced by more than one variable. There are many statistical techniques for conducting multivariate analysis, and the most appropriate technique for a given study varies with the type of study and the key research questions.
Objective of Multivariate Analysis
Multivariate analysis navigates complex datasets to uncover hidden relationships and patterns, offering a comprehensive view of data structures. By considering multiple variables simultaneously, it goes beyond simplistic views, enabling a deeper exploration of real-world phenomena. This approach facilitates predictive modelling for forecasting future outcomes, crucial across sectors for informed decision-making. Additionally, it streamlines datasets through dimensionality reduction, enhancing efficiency and clarity in interpretation, thus driving actionable insights and informed decision-making.
Analysis of Multivariate Data
The analysis of multivariate data involves using various statistical techniques to understand complex datasets with multiple variables. Analysts start by exploring the data visually and then use statistical tests to measure relationships between variables. For example, correlation analysis shows the strength of linear relationships, while principal component analysis (PCA) reveals underlying patterns and simplifies the data. Regression analysis is key for understanding how variables interact, while techniques like classification and discrimination help group observations based on their unique traits. By combining visualization and statistical methods, analysts can extract valuable insights for making informed decisions across different fields.
Applications and techniques of Multivariate Data Analysis
Applications of Multivariate Data Analysis include:
● Multivariate hypothesis testing ● Dimensionality reduction ● Latent structure discovery ● Cluster analysis ● Multivariate regression analysis ● Classification and discrimination analysis ● Variable selection ● Multidimensional Scaling ● Data mining
Examples of Multivariate Data Analysis
Example 1: Healthcare. A dietician collects patient data on cholesterol, blood pressure, sugar levels and weight. She also collects data on dietary habits. Using Multivariate Data Analysis, she can determine how much each element of diet influences health outcomes. Example 2: Academics. A researcher has collected data on three demographic variables, and four academic variables (let’s say standardized test scores) for 1,000 students along with the programmes they are enrolled for. The researcher wants to determine how demographics and academic variables are related and the choice of program.
FORE School of Management, New Delhi’s FDP on Multivariate Data Analysis
FORE School of Management, New Delhi has been designing, developing and conducting innovative Executive Education (EE), Management Development Programmes (MDPs), and Faculty Development Programmes (FDPs) for working executives and academicians in India for over three decades. These programmes meet the need for faculty, researchers, and business managers to continuously update themselves about changing business paradigms and innovative business practices to stay ahead of competition. The purpose of FORE School of Management, New Delhi’s Multivariate Data Analysis FDP is to provide faculty, research scholars, and business executives with the tools to gain a better understanding of the available techniques for data analysis with a focus on multivariate techniques. This program also aims to provide hands-on training on SPSS, AMOS software for analysing the data and interpreting outcomes of various analyses. The programme will be conducted by Subject Matter Experts and is designed with an appropriate blend of conceptual and experiential learning. For more details, visit: https://bit.ly/34yzHbj

An official website of the United States government
The .gov means it’s official. Federal government websites often end in .gov or .mil. Before sharing sensitive information, make sure you’re on a federal government site.
The site is secure. The https:// ensures that you are connecting to the official website and that any information you provide is encrypted and transmitted securely.
- Publications
- Account settings
Preview improvements coming to the PMC website in October 2024. Learn More or Try it out now .
- Advanced Search
- Journal List

The multivariate analysis of variance as a powerful approach for circular data
Lukas landler.
1 Institute of Zoology, University of Natural Resources and Life Sciences (BOKU), Gregor-Mendel-Straße 33, 1180 Vienna, Austria
Graeme D. Ruxton
2 School of Biology, University of St Andrews, St Andrews, KY16 9TH UK
E. Pascal Malkemper
3 Research Group Neurobiology of Magnetoreception, Max Planck Institute for Neurobiology of Behavior – caesar, Ludwig-Erhard-Allee 2, 53175 Bonn, Germany
4 Department of Game Management and Wildlife Biology, Faculty of Forestry and Wood Sciences, Czech University of Life Sciences, 16521 Prague 6, Czech Republic
Associated Data
Data and code are available in the Additional file 1 .
A broad range of scientific studies involve taking measurements on a circular, rather than linear, scale (often variables related to times or orientations). For linear measures there is a well-established statistical toolkit based on linear modelling to explore the associations between this focal variable and potentially several explanatory factors and covariates. In contrast, statistical testing of circular data is much simpler, often involving either testing whether variation in the focal measurements departs from circular uniformity, or whether a single explanatory factor with two levels is supported.
We use simulations and example data sets to investigate the usefulness of a MANOVA approach for circular data in comparison to commonly used statistical tests.
Here we demonstrate that a MANOVA approach based on the sines and cosines of the circular data is as powerful as the most-commonly used tests when testing deviation from a uniform distribution, while additionally offering extension to multi-factorial modelling that these conventional circular statistical tests do not.
Conclusions
The herein presented MANOVA approach offers a substantial broadening of the scientific questions that can be addressed statistically using circular data.
Supplementary Information
The online version contains supplementary material available at 10.1186/s40462-022-00323-8.
Introduction
Some scales of measurement in science are inherently periodic rather than linear. Data on compass orientations, times of day and times of year are obvious examples of this. Such data are often called circular, since it is easy to imagine the data being mapped onto the circumference of a circle. As soon as one considers that 355° is closer to 5° than it is to 340°, it is clear that such circular data needs different statistical treatment from linear variables (such as mass and age), and a body of statistical theory has developed to allow investigation of circular data (for example: Batschelet [ 1 ], Fisher [ 2 ], Pewsey et al. [ 3 ] and Landler et al. [ 4 ]).
Rayleigh’s test on the null hypothesis that the population mean vector length is zero is often cited as the first statistical testing procedure designed for circular data. Since Lord Rayleigh [ 5 ] formulated his seminal Rayleigh test in the late nineteenth century, circular statistics as an academic discipline has steadily developed and expanded the range of possible approaches, nowadays including a myriad of test alternatives and software [ 6 – 8 ], second order analyses [ 9 ], as well as Akaike and Bayesian Information criterion methods [ 10 – 12 ]. All have the goal of helping researchers understand and correctly interpret data distributed on a circle. However, recently we confirmed that, despite all the developments, the Rayleigh test is still one of the most powerful tests for using a single sample to test the null hypothesis that the underlying population is uniformly distributed with no preferred direction [ 4 ]. In contrast to some alternative tests, the Rayleigh test also controls type I error rates for aggregated data (e.g. if directions are only recorded to the nearest degree, or even ten degrees) and can be used to detect a variety of deviations from uniformity, with the exception of multimodal symmetrical distributions [ 13 , 14 ].
One feature all commonly-used tests for circular statistics based on null-hypothesis testing have in common is the inability to use multiple covariates and/or multiple explanatory factors if the circular variable is the response. That is, investigation is limited to a single independent variable (although this variable can be discrete, continuous and circular, or continuous and linear). This is in stark contrast to analyses of linear dependent variables, where multiple independent variables are analyzed routinely within one testing procedure.
In a recent analysis we compared a suite of available tests designed to compare two samples to test the null hypothesis that they come from the same underlying population and found that also in this testing situation a very common test, the Watson U 2 test [ 15 ], showed superior power to many other standard options [ 16 ]. Within this study, we also experimented with a new approach, which exploits a well-known procedure to linearize circular data by using sines and cosines of the angles (see for example Pewsey et al. [ 3 ]). In order to use two linear factors (the sines and cosines of the recorded angles) as dependent variables, we needed to switch from univariate linear models to a multivariate analysis of variance. This approach allows the use of multiple response variables [ 17 ]. In our study we used MANOVA to test for a difference of two circular distributions by using the sample id as a factor with two levels (see Pail et al. [ 18 ] for one earlier study that used a MANOVA to analyze effects of a linear variable on a directional response, and Sect. 7.4 of Mardia & Jupp [ 19 ] for methods to deal with more than two distributions). Perhaps surprisingly, this novel approach offered very similar performance to the best-established tests in this simple testing situation, for instance, the Watson U 2 test [ 16 ]. It is important to add that the sine and cosine of an angle (theta), while derived from the same number, are orthogonal to each other by definition. This fact might add to the power of the MANOVA approach.
Although our previous investigation was restricted to a single two-level explanatory factor, it is clear that the MANOVA approach allows for the use of more than two levels per factor, multiple grouping factors, as well as linear covariates [ 20 – 22 ]. However, it is less clear how this would relate to circular distributions, or in other words, how p values obtained in such more-complex analyses would relate to specific null hypotheses.
While we have already shown that differences between two populations can be reliably tested, we wanted to also use this approach to test for significantly clustered (i.e., non-random) unimodal distributions based on just a single sample of data. Testing the null-hypothesis that a single sample of data comes from an underlying uniform distribution is very likely the most commonly applied procedure in circular statistics. We hypothesized that the intercept of the null model without any explanatory variables could be used to derive p values related to significant clustering. As such approach would be novel to circular statistics, we tested this hypothesis and compared this MANOVA intercept approach with the two most powerful tests for unimodal deviations from uniformity in a single sample, the Rayleigh and Hermans–Rasson tests [ 4 , 23 ]. We then simulated some example situations with known underlying distributions to further explore the MANOVA approach’s general usefulness in situations including grouping and linear factors.
This paper explores the use of MANOVA applied to circular response variables, and provides advice for people interested in using this for their own research. We show that the intercept of the MANOVA models is a reliable proxy of unimodal clustering and that grouping factors as well as linear covariates can be accommodated in the very flexible approach, which considerably enhances model performance and interpretation of circular data.
Statistical tests used
All analyses were performed in the statistical software R [ 24 ], R code used for simulations and R output can be found in the Additional file 1 (the R code and example data can also be found on github: https://github.com/Malkemperlab/Circular-MANOVA ). For all analyses we performed the MANOVA approaches using the summary function for the “manova” function in base R. We exploited a common transformation of circular variables into two orthogonal linear variables for the response matrix. This was achieved by using sine and cosine of the angle (in radians). This approach has previously been used to perform linear models with angles as independent variables (see [ 3 ]). If the angles are viewed as vectors on a unit circle, the sine and cosine of the angle represent the x and y component of such a vector. However, this view might only be valid for the case of a MANOVA model without any independent variables (intercept only). In such a special case, a significant p value is expected to indicate that the (vector) distribution differs significantly from the center of such unit circle (0,0). Following this logic, independent factors can then change the weight (length) of the vectors (linear variables) or group the vectors and allow testing for differences between the groups (grouping factors).
In our power analysis the independent variables were either absent (intercept-only approach) or different for each of the analyses. The intercept-only approach used no response variables, therefore, only a single p value was reported. In the linear MANOVA approach, a linear variable was added, therefore reporting the p value of the intercept and the covariate. In the grouped MANOVA approach a grouping factor was added, therefore, generating p values for both the intercept and the grouping factor. In the last variant of MANOVA model explored, linear and grouping factors were combined in one MANOVA analysis, allowing the analysis of significant intercept, linear and grouping effects. Throughout, we compared two MANOVA approaches, using both conventional theory and numerical simulation to evaluate p values. For the simulation-based MANOVA approaches, the obtained p values were adjusted by comparing them to 9999 iterations of the same analysis using samples from a uniform random distribution. The Rayleigh test was calculated using the function rayleigh.test of the package circular [ 8 ]. The Hermans-Rasson (HR) test was performed using the R package CircMLE [ 10 ] and function HermansRasson2T [ 23 ].
Simulation tests
All tests described above were applied to the following samples, which were drawn from the given distribution (9999 iterations), power was defined as the proportion of significant test results (p< 0.05). For all simulations circular distributions were generated using the function rcircmix from the package NPcirc [ 25 ].
First, in order to test type I error rate, we simulated samples from circular uniform distributions (type “unif” in rcircmix, sample sizes: 5, 10, 15, 25, 50 and 100), from a continuous as well as binned distribution (with data aggregated into 10° bins around the circle, for all binned data the minimum sample size used was 10). The binning was done to test for sensitivity to such rounding of measured data, which can cause issues with some circular tests [ 14 ].
In a next step, we tested samples drawn from a unimodal von Mises (type “vm”, kappa = 1, mean direction: 0, sample sizes: 5, 10, 15, 25, 50 and 100) and wrapped skew normal (type “wsn”, dispersion parameter = 2, skewness: 30, location parameter: 0, sample sizes: 5, 10, 15, 25, 50 and 100) distribution. We then simulated samples from both a symmetrical (mean directions: 0° and 180°) and an asymmetrical (mean directions: 0° and 240°) bimodal von Mises distribution (type “vm”, kappa = 1, sample sizes: 10,20,30,50,100,200). To further extend this power comparison of basic distributions, we used samples from symmetrical (mean directions: 0°, 120° and 240°) and asymmetrical (mean directions: 0°, 120° and 270°) trimodal von Mises distributions (type “vm”, kappa = 1, sample sizes: 15, 30, 45, 75, 150, 300).
In order to gain insights into the usefulness of the MANOVA approach for comparisons we simulated data for a hypothetical scenario. In this scenario, we work on an animal population where we know the age and sex (called group 1 and group 2 herein) of the tested individuals. The assumption is that the homeward orientation after displacement becomes more clustered with age (which in this example ranges from 1 year to 5 years) and that group 1 is either less clustered or directionally differently oriented than group 2. To keep the presentation consistent, data were plotted according to the sample size of the potential treatment combination. Two groups and five ages resulted in 10 treatment combinations, hence the minimum sample size was 10.
Real data examples
In order to test the presented approach on real data, we used three publicly available data sets from studies on animal behavior. The first one is a data set from Gagliardo et al. [ 26 ] embedded in the R package circular . In this study a translocation experiment was performed on pigeons, with three groups: control, sectioned olfactory nerve and section of the ophthalmic branch of the trigeminal nerve. The expectations were a difference between groups, an overall orientation towards the home direction, and no directional clustering in the group with the sectioned olfactory nerve.
The second data set was from Lindecke et al. [ 27 ] involving migratory bats ( Pipistrella pygmaeus ). The expectation was a difference between two treatment groups (with a 180° switch in preferred direction) and an effect of age on the treatment effect (interaction between age and treatment). For our analysis we started with a full model including the factors age, sex, treatment, temperature and wind speed. Then we used our self-written AIC based model selection function (see Additional file 1 ). This function first orders the independent variables based on their Eta-squared values from the full model (using the function eta_squared from the package effectsize [ 28 ] on both underlying ANOVAs, i.e., for each response variable). The first n -variables are then used in an AIC based model comparison ( n can be specified in the function input - in the case of the bat examples no variable was discarded at this step). For the AIC based model comparison all possible variable combinations are used in a MANOVA model and ranked according to their AIC values (mean AIC from both underlying ANOVAs, i.e., for each of the two response variables).
The third data set is from Obleser et al. [ 29 ], where the flight direction of deer was investigated. This data set included numerous potential explanatory variables, i.e. hour of the day, temperature, light intensity, wind speed, wind direction, sun direction, hide direction, previous alignment, distance of the hide, group size, sex, age, observer direction and vegetation height, which were included in a full model. In a further step we used our AIC based model selection function to reduce the model (the first 10 independent variables were used in the first selection step to allow fast calculation, see above for explanation). The original study observed a north-south flight direction of roe deer; thus the dependent variable was hypothesized to be axial. We, therefore, doubled the angles and reduced to modulo 360° (= 2*pi in radians) for our analysis [ 1 ]. All independent variables were added as axial as well as non-transformed directions. Hence this data set represented a complex system of multiple variables. In the published paper this was overcome by performing several different graphical and statistical analyses, in our approach we tested all the underlying hypotheses in one single model.
Type I error
Type I error was slightly above 0.05 at very low sample sizes for the MANOVA approach but kept expected alpha values at sample sizes of at least 10–15 (Fig. 1 ). The simulation MANOVA approach controlled type I error throughout all sample sizes. The HR test showed slightly increasing type I error rates with increasing sample sizes for binned data (10° bins), as demonstrated earlier [ 14 ].

Type I error rates at different sample sizes of the four statistical tests used for continuous data ( a ) and binned data ( b )
Power analysis—basic distributions
For unimodal distributions the MANOVA approach and the Rayleigh test performed equally well, with the HR test showing just slightly lower power (Fig. 2 a, b). The standard MANOVA approach showed artificially increased power levels at a sample size of 5, due to increased type I error rate at such small sample sizes. This was controlled for by the simulation MANOVA approach. When evaluating symmetrical bimodal distributions (Fig. 2 c), the HR test was the only test with useful power to detect clustering, however, for asymmetrical bimodal distributions (Fig. 2 d), the results were similar to the unimodal distribution, with the HR test performing slightly worse than both the other tests. None of the tests were able to detect clustering in symmetrical trimodal distributions (Fig. 2 e) over the range of sample size used (15–300). All of the tests had very similar (low) power to detect clustering of asymmetrical trimodal distributions (Fig. 2 f). Taken all the analyses together, for the standard distributions, the power of the MANOVA approach was almost identical to the very powerful Rayleigh test, and only shared the same weakness of low power with symmetrical distributions, which traditionally can be overcome by simple data transformation [ 1 ].

Power of the four statistical tests in situations with different underlying distributions: unimodal von Mises ( a ), unimodal wrapped skew normal ( b ), symmetrical bimodal von Mises ( c ), asymmetrical bimodal von Mises ( d ), symmetrical trimodal von Mises (E), asymmetrical trimodal von Mises ( f )
Power analysis—hypothetical examples
All tests controlled type I error when all treatment combinations were sampled from the same distributions (Fig. 3 a). The overall power to detect orientation non-uniformity was similar between tests when the mean direction was the same for all distributions, however, the MANOVA approach in addition appropriately identified a linear and/or grouping effect (Fig. 3 b–d). In the case of differing mean directions between groups, the MANOVA approaches performed very well in detecting deviations from uniformity (Fig. 4 ). The exception was the case of two groups with mean orientations 180° apart, in this special case only the HR test showed moderate power to detect non-random orientation (Fig. 4 c). However, the MANOVA approach showed good power to detect differences between groups, and hence an effect on orientation. The MANOVA approach performed almost as well as the HR test when there was a linear effect (different between groups) added to the two groups with opposite orientations (Fig. 4 d).

Power of several hypothetical examples for the proposed approach. In a hypothetical 5-year study (linear variable) of a population composed of two groups (grouping variable) the orientation performance of subjects is measured. P values for the MANOVA intercepts as well as Rayleigh and HR test are shown in the left panel, p values for the linear variables in the center and grouping variables in the right panel. In the case of randomized data ( a ), all p values of all measured tests remained at nominal levels. In all following distributions the clustering of orientations continuously increased each year, however, mean orientation was identical between all groups. First, we only changed the yearly increase from 0–2 ( b ) to 2–4 ( c ). In a second step, we used different yearly clustering increases for group 1 and group 2 ( d )

Continuation of our hypothetical example, analyzing the usefulness of the MANOVA approach for biological data. In addition to the yearly increase of clustering, we now manipulated the orientation direction for each group, using either a 90° ( a , b ) or a 180° ( c , d ) difference. This was then either combined with a group difference in yearly clustering increase ( b , d ), or not ( a , c ). The left panel shows the power for the intercept of MANOVA approaches as well as Rayleigh and HR test, the center panel shows the power of detecting the linear effect, the right panel shows power for detecting group differences
The MANOVA performed on the pigeon data showed a highly significant treatment effect and an overall significant non-uniform orientation (intercept) (Table 1 ), which is in line with the conclusions made in the original study [ 26 ].
MANOVA table for the pigeon example, showing the significant overall orientation (intercept) and treatment effect
For the bat example, the MANOVA analysis elegantly combined all possible separate analyses in one model and showed that the treatment as well as age-by-treatment interaction were both significant (Table 2 ). Hence, the treatment effect changes with age of the bat. This corroborates (in a much more compact way) the results already presented in the original study by Lindecke et al. [ 27 ]. The overall orientation (intercept) was not significant, which is expected as the treatment groups showed opposite (overall axial) orientation.
MANOVA table for the bat example, showing the significant treatment effect, as well as interaction with age
For the deer example, our approach showed the potential of the MANOVA approach to handle multiple potential explanatory variables. While in the original study numerous separate analyses were made and effect strengths compared [ 28 ], we can show in our rudimentary model selection approach that the axis of the hide as well as the previous alignment of the deer axis influenced the flight direction the most, i.e. were retained in the final model and showed a highly significant p value (Table 3 ). This supports the idea brought forward in the paper by Obleser et al. [ 29 ], that animals tend to align along the magnetic north-south axis, and (even more so) use the same axis as their preferred flight direction.
MANOVA table of the selected model, after eliminating non-significant factors, for the deer example, showing significant effects of the previous axial alignment as well as the hide axis—expressed as cosine and sine of the axial directions in radians. In addition, sun axis, wind speed, wind direction and temperature showed marginal significant/trending effects
Our previous published work demonstrated that MANOVA is as powerful as the available conventional tests to investigate whether two samples come from the same underlying distribution. Here we have demonstrated that the effectiveness of this approach extends much more widely. Firstly, our analysis shows that the MANOVA approach for circular data is as powerful to determine significant departure from uniformity in a single sample of data as the popular and effective Rayleigh test. In addition, adding linear and grouping variables can vastly improve the power of the MANOVA, but also enhance the range of hypothesis testing. If one suspected that a certain linear variable or a grouping factor might be responsible for a change in orientation this can be tested explicitly without applying multiple tests (i.e., avoiding the issue of multiple hypothesis testing and inflating the type I error). There are no restrictions on the type of distributions to be used for this approach.
A potential weakness of the conventional MANOVA approach (where the p values are obtained by theoretical approaches) is the increased type I error rate for very low sample sizes (i.e., over all of n < 15). However, in such cases the p value can be calculated by Monte Carlo simulations, in this case it retains power levels and controls alpha inflation. We provide R code to perform this test in Additional file 1 . If symmetrical multimodal orientations can be expected in a diversity of real-world situations generating circular data, the data can be transformed prior to analysis by MANOVA (see our deer example for axial data). However, if cofactors (or groupings) are not needed, and the type of modality is not known, one might prefer to use the HR test, which is more powerful than most other available options in such a situation.
In contrast to other more sophisticated circular models, e.g. the Bayesian GLM [ 11 ], our approach provides insight into the circular response variable and its deviation from uniformity for single samples, in addition to testing for the significant contributions of groups and linear factors.
In principle, MANOVAs might be used to incorporate random effects as well, e.g., in a repeated-measures MANOVA. However, such approach will require validation for the intercept approach described here, this potentially opens the possibility to perform experiments and accompanying analyses without any of the limitations previously experienced in circular statistics. Thus, the MANOVA approach demonstrated here, in combination with recent developments in Bayesian approaches, offers a substantial broadening of the scientific questions that can be addressed statistically with circular data. The fact that it also performs well when testing very simple hypotheses that are currently evaluated by a collection of specialist tests, should make its wider adoption all the more attractive.
We show that the herein presented MANOVA approach has the potential to extend the range of scientific questions substantially that can be tested statistically using circular data. In addition, using such approach would lead to more powerful analyses than currently possible and paves the way to make the linear statistical toolbox available for circular data.
Acknowledgements
We thank two anonymous reviewers for their valuable comments on an earlier version of this manuscript.
Author contributions
LL, GDR and EPM conceptualized the problem and discussed the analytic approaches. LL prepared the code. LL, GDR and EPM interpreted the results. LL, GDR and EPM wrote the manuscript. All authors read and approved the final manuscript.
LL is supported by the Austrian Science Fund (FWF, Grant Number: P32586). EPM receives funding from the European Research Council (ERC) under the European Union’s Horizon 2020 research and innovation program (Grant Agreement No. 948728).
Availability of data and materials
Declarations.
Not applicable.
All authors gave their consent.
The authors declare that they have no competing interests.
Publisher’s note
Springer Nature remains neutral with regard to jurisdictional claims in published maps and institutional affiliations.
Testing the equality of multivariate means when \(p>n\) by combining the Hotelling and Simes tests
- Original Paper
- Published: 15 July 2021
- Volume 31 , pages 390–415, ( 2022 )
Cite this article
- Tzviel Frostig ORCID: orcid.org/0000-0003-2761-8380 1 &
- Yoav Benjamini ORCID: orcid.org/0000-0002-3253-7588 1
305 Accesses
Explore all metrics
We propose a method of testing a shift between mean vectors of two multivariate Gaussian random variables in a high-dimensional setting incorporating the possible dependency and allowing \(p > n\) . This method is a combination of two well-known tests: the Hotelling test and the Simes test. The tests are integrated by sampling several dimensions at each iteration, testing each using the Hotelling test, and combining their results using the Simes test. We prove that this procedure is valid asymptotically. This procedure can be extended to handle non-equal covariance matrices by plugging in the appropriate extension of the Hotelling test. Using a simulation study, we show that the proposed test is advantageous over state-of-the-art tests in many scenarios and robust to violation of the Gaussian assumption.
This is a preview of subscription content, log in via an institution to check access.
Access this article
Price includes VAT (Russian Federation)
Instant access to the full article PDF.
Rent this article via DeepDyve
Institutional subscriptions
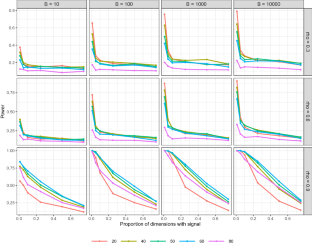
Similar content being viewed by others
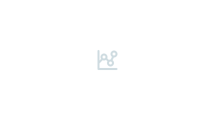
High-dimensional Tests for Mean Vector: Approaches without Estimating the Mean Vector Directly
Bo Chen & Hai-meng Wang
On testing the equality of high dimensional mean vectors with unequal covariance matrices
Jiang Hu, Zhidong Bai, … Wei Wang
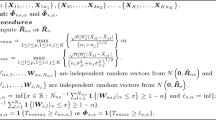
Multi-sample hypothesis testing of high-dimensional mean vectors under covariance heterogeneity
Lixiu Wu & Jiang Hu
Alon U, Barkai N, Notterman D, Gish K, Ybarra S, Mack D, Levine A (1999) Broad patterns of gene expression revealed by clustering analysis of tumor and normal colon tissues probed by oligonucleotide arrays. Proc Natl Acad Sci USA 96(12):6745–6750. https://doi.org/10.1073/pnas.96.12.6745
Article Google Scholar
Bai Z, Saranadasa H (1996) Effect of high dimension: by an example of a two sample problem. Statistica Sinica 6(2):311–329
MathSciNet MATH Google Scholar
Benjamini Y, Yekutieli D (2001) The control of the false discovery rate in multiple testing under dependency. Ann Stat 29(4):1165–1188
Article MathSciNet Google Scholar
Bibby J, Kent J, Mardia K (1979) Multivariate analysis
Cai TT, Liu W, Xia Y (2014) Two-sample test of high dimensional means under dependence. J Royal Stat Soc Ser B-Stat Methodol 76(2):349–372. https://doi.org/10.1111/rssb.12034
Chen SX, Qin YL (2010) A two-sample test for high-dimensional data with applications to gene-set testing. Ann Statist 38(2):808–835. https://doi.org/10.1214/09-AOS716
Article MathSciNet MATH Google Scholar
Derkach A, Lawless JF, Sun L (2014) Pooled association tests for rare genetic variants: a review and some new results. Stat Sci 29(2):302–321. https://doi.org/10.1214/13-STS456
Donoho D, Jin J (2004) Higher criticism for detecting sparse heterogeneous mixtures. Ann Stat 32(3):962–994. https://doi.org/10.1214/009053604000000265
Feng L, Zou C, Wang Z, Zhu L (2017) Composite t 2 test for high-dimensional data. Statistica Sinica, pp 1419–1436
Gregory K (2014) highD2pop: two-sample tests for equality of means in high dimension. https://CRAN.R-project.org/package=highD2pop , r package version 1.0
Gregory KB, Carroll RJ, Baladandayuthapani V, Lahiri SN (2015) A two-sample test for equality of means in high dimension. J Am Stat Assoc 110(510):837–849. https://doi.org/10.1080/01621459.2014.934826
Heller R, Heller Y, (2016) Multivariate tests of association based on univariate tests. In: Advances in Neural Information Processing Systems 29 (NIPS, (2016) vol 29, 30th Conference on Neural Information Processing Systems (NIPS). SPAIN, Barcelona, p 2016
Hemmelmann C, Horn M, Reiterer S, Schack B, Susse T, Weiss S (2004) Multivariate tests for the evaluation of high-dimensional EEG data. J Neurosci Methods 139(1):111–120. https://doi.org/10.1016/j.jneumeth.2004.04.013
Hotelling H (1931) The generalization of student’s ratios. Ann Math Stat 2:360–378
Hu Z, Tong T, Genton MG (2019) Diagonal likelihood ratio test for equality of mean vectors in high-dimensional data. Biometrics 75(1):256–267
Jacob L, Neuvial P, Dudoit S (2010) Gains in power from structured two-sample tests of means on graphs. arXiv preprint arXiv:1009.5173
Karlin S, Rinott Y (1981) Total positivity properties of absolute value multinormal variables with applications to confidence interval estimates and related probabilistic inequalities. Ann Stat 9(5):1035–1049. https://doi.org/10.1214/aos/1176345583
Keselman H, Cribbie R, Holland B (2002) Controlling the rate of Type I error over a large set of statistical tests. British J Math Stat Psychol 55(1):27–39. https://doi.org/10.1348/000711002159680
Krishnamoorthy K, Yu J (2004) Modified Nel and Van der Merwe test for the multivariate Behrens-Fisher problem. Stat Probab Lett 66(2):161–169. https://doi.org/10.1016/j.spl.2003.10.012
Läuter J (2013) Simes’ theorem is generally valid for dependent normally distributed variables. In: Invited talk at the international conference on simultaneous inference
Lin L, Pan W (2016) highmean: Two-Sample Tests for High-Dimensional Mean Vectors. https://CRAN.R-project.org/package=highmean , r package version 3.0
Lopes M, Jacob L, Wainwright MJ (2011) A more powerful two-sample test in high dimensions using random projection. In: Advances in Neural Information Processing Systems, pp 1206–1214
Reiner-Benaim A (2007) FDR control by the BH procedure for two-sided correlated tests with implications to gene expression data analysis. Biomet J 49(1):107–126. https://doi.org/10.1002/bimj.200510313 , 4th International Conference on Multiple Comparison Procedures (MCP2005), Shanghai, PEOPLES R CHINA, AUG 17-19, 2005
Ruiz-Meana M, Garcia-Dorado D, Pina P, Inserte J, Agullo L, Soler-Soler J (2003) Cariporide preserves mitochondrial proton gradient and delays ATP depletion in cardiomyocytes during ischemic conditions. Am J Physiol Heart Circulat Physiol 285(3):H999–H1006. https://doi.org/10.1152/ajpheart.00035.2003
Sarkar S (1998) Some probability inequalities for ordered MTP2 random variables: a proof of the Simes conjecture. Ann Stat 26(2):494–504
Simes RJ (1986) An improved Bonferroni procedure for multiple tests of significance. Biometrika 73(3):751–754. https://doi.org/10.2307/2336545
Srivastava MS, Du M (2008) A test for the mean vector with fewer observations than the dimension. J Multivariate Anal 99(3):386–402. https://doi.org/10.1016/j.jmva.2006.11.002
Stadje W (1990) The collector’s problem with group drawings. Adv Appl Probab, pp 866–882
Thulin M (2014) A high-dimensional two-sample test for the mean using random subspaces. Comput Stat Data Anal 74:26–38. https://doi.org/10.1016/j.csda.2013.12.003
Williams V, Jones L, Tukey J (1999) Controlling error in multiple comparisons, with examples from state-to-state differences in educational achievement. J Educat Behav Stat 24(1):42–69. https://doi.org/10.3102/10769986024001042
Xiong M, Zhao J, Boerwinkle E (2002) Generalized T-2 test for genome association studies. Am J Human Genet 70(5):1257–1268. https://doi.org/10.1086/340392
Yang Y, Yamada T, Hill KK, Hemberg M, Reddy NC, Cho HY, Guthrie AN, Oldenborg A, Heiney SA, Ohmae S, Medina JF, Holy TE, Bonni A (2016) Chromatin remodeling inactivates activity genes and regulates neural coding. Science 353(6296):300–305. https://doi.org/10.1126/science.aad4225
Yekutieli D (2008) False discovery rate control for non-positively regression dependent test statistics. J Stat Plan Inference 138(2):405–415. https://doi.org/10.1016/j.jspi.2007.06.006
Download references
Acknowledgements
We would like to thank the reviewers for their thoughtful comments and efforts toward improving our manuscript. The research leading to these results has received funding from the European Research Council: ERC grant agreement PSARPS{294519}.
Author information
Authors and affiliations.
Department of Statistics and Operations Research, Tel Aviv University, Tel Aviv University, Tel Aviv, 6997801, Israel
Tzviel Frostig & Yoav Benjamini
You can also search for this author in PubMed Google Scholar
Corresponding author
Correspondence to Tzviel Frostig .
Additional information
Publisher's note.
Springer Nature remains neutral with regard to jurisdictional claims in published maps and institutional affiliations.
The research leading to these results has received funding from the European Research Council: ERC grant agreement PSARPS{294519}.
Supplementary Information
Below is the link to the electronic supplementary material.
Supplementary material 1 (pdf 517 KB)
Rights and permissions.
Reprints and permissions
About this article
Frostig, T., Benjamini, Y. Testing the equality of multivariate means when \(p>n\) by combining the Hotelling and Simes tests. TEST 31 , 390–415 (2022). https://doi.org/10.1007/s11749-021-00781-z
Download citation
Received : 29 January 2020
Accepted : 13 June 2021
Published : 15 July 2021
Issue Date : June 2022
DOI : https://doi.org/10.1007/s11749-021-00781-z
Share this article
Anyone you share the following link with will be able to read this content:
Sorry, a shareable link is not currently available for this article.
Provided by the Springer Nature SharedIt content-sharing initiative
- Location alternative
- Global null
- Permutation testing
Mathematics Subject Classification
- Find a journal
- Publish with us
- Track your research
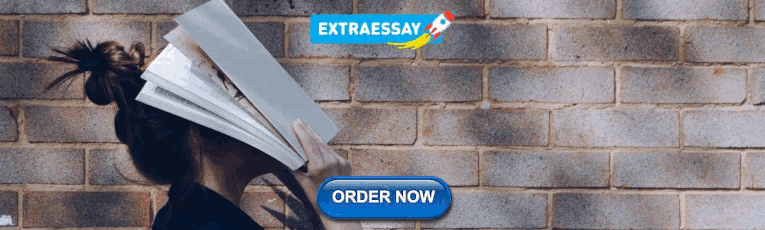
IMAGES
VIDEO
COMMENTS
STAT 542: Multivariate Analysis Spring 2021 Lecture 11: Multiple hypothesis test Instructor: Yen-Chi Chen 11.1 Introduction The multiple hypothesis testing is the scenario that we are conducting several hypothesis tests at the same time. Suppose we have ntests, each leads to a p-value. So we can view the 'data' as P 1; ;P n 2[0;1], where P
Multivariate statistics is a subdivision of statistics encompassing the simultaneous observation and analysis of more than one outcome variable, i.e., multivariate random variables.Multivariate statistics concerns understanding the different aims and background of each of the different forms of multivariate analysis, and how they relate to each other.
Multivariate Z -test (MZ; Wald test) For examinee i, the null hypothesis, H0:θi2 = θi1, is tested against the alternative hypothesis, Ha:θi2 ≠ θi1. This is an overall test; hence, the change can occur in any direction or pattern (i.e., a two-tailed test) and involve one or more dimensions.
Conclusion. Multivariate analysis is one of the most useful methods to determine relationships and analyse patterns among large sets of data. It is particularly effective in minimizing bias if a structured study design is employed. However, the complexity of the technique makes it a less sought-out model for novice research enthusiasts.
Test of Multivariate Normality. Check univariate normality for each trait (X) separately. Can check \[Normality Assessment\] The good thing is that if any of the univariate trait is not normal, then the joint distribution is not normal (see again [m]). If a joint multivariate distribution is normal, then the marginal distribution has to be normal.
Abstract. Multivariate analysis is appropriate whenever more than one variable is measured on each sample individual, and overall conclusions about the whole system are sought. Many different multivariate techniques now exist for addressing a variety of objectives. This brief review outlines, in broad terms, some of the more common objectives ...
Hypothesis: A tentative ... The 2-sample t-test: Also known as the independent sample t-test, it's a method used to examine whether the means of two or more unknown samples are equal or not. If your sample distribution is unequal, you can use a different estimate of the standard deviation to check results. ... Besides the usual A/B and Split ...
have no multivariate structure and we could just do univariate statistics on each variable (column) in turn. Multivariate statistics means we are interested in how the columns covary. We can compute covariances to evaluate the dependencies. If the data were multivariate normal with p variables,all the
Multivariate testing, also known as multivariant testing, is a method of experimenting with multiple variables on a website or mobile app to determine which variation performs the best. The goal is to identify the combination of variations that drive a desired outcome, like more conversions or higher revenue. ...
4.1 Multinomial Test Statistics. In this section we consider three well-known test statistics, namely the likelihood ratio test, the Wald test, and the Score test. We show that all three test statistics are asymptotically equivalent, and the score statistic is the same as Pearson's goodness of fit test statistic.
9 Multivariate methods for heterogeneous data. 9. Multivariate methods for heterogeneous data. Real situations often involve point clouds, gradients, graphs, attraction points, noise and different spatial milieux, a little like this picture where we have a rigid skeleton, waves, sun and starlings. In Chapter 7, we saw how to summarize ...
In effect a multivariate analysis will follow a three-step process: Regress each independent variable on the set of covariates and save in memory the residuals in that regression. Call these variables X1.C (the portion of X1 independent of the C variables), X2.C, etc. Similarly derive Y1.C, Y2.C, etc. by regressing Y1, Y2, etc. on the C variables.
It will also detail multiple methods, examples, and possible use cases to help you understand the strengths of each. Specifically, it will explain: What multivariate analysis is. Multiple linear regression. Multiple logistic regressions. Multivariate analysis of variance (MANOVA) Factor analysis. Cluster analysis. Discriminant analysis.
This problem can be thought of as the multivariate counterpart of the univariate hypothesis t-test. 9.1.1 Hotelling's T2 Test The most fundamental approach to signal detection is a mere generalization of the t-test, known as Hotelling's \(T^2\) test .
The table also gives the value of the constant, also known as the intercept. This is the value of the dependent variable when the independent variable has a value of zero. As will be shown, a formula that includes both the constant and the regression coefficient can be used to estimate, or predict, hypothetical values of the dependent variable.
The multivariate analysis definition is analyzing the data is known as Multivariate analysis. Here is an introduction to multivariate statistical analysis. ... Testing and construction of hypothesis; ... It is also known as dimension reduction. This technique is used to reduce the data before going ahead with analysis.
With a lot of cases it is easy to reject the null hypothesis of no relationship. With a few cases, it can be quite hard to reject the null hypothesis. Also, consider the percentages within the table. Look for patterns. Do not rely on any single piece of information. Look at the whole picture. We have concentrated on crosstabulation and chi square.
The analysis of multivariate data involves using various statistical techniques to understand complex datasets with multiple variables. Analysts start by exploring the data visually and then use statistical tests to measure relationships between variables. For example, correlation analysis shows the strength of linear relationships, while ...
namely the multivariate general linear model. Before we begin, it will be necessary to review some of the fundamentals of hypothesis testing, and then after, to introduce some mathematical details of use in this area. 8.2 Testing Multiple Hypotheses In Chapter 6, we covered two different approaches to testing hypotheses about the coefficients of
Within this study, we also experimented with a new approach, which exploits a well-known procedure to linearize circular data by using sines and cosines of the angles (see for example Pewsey et al. ). In order to use two linear factors (the sines and cosines of the recorded angles) as dependent variables, we needed to switch from univariate ...
We propose a method of testing a shift between mean vectors of two multivariate Gaussian random variables in a high-dimensional setting incorporating the p. ... One can also consider the hypothesis \(H_0: \varvec{\mu }_X = \varvec{\mu } ... also known as the Behrens-Fisher problem, has been of interest from the early age of Statistics. ...
Mathematically, a general k-sample problem, also known as one-way multivariate analy-sis of variance for functional data (FMANOVA) can be described as follows. Let SP p(η,Γ) denote a p-dimensional stochastic process with vector of mean functions η(t),t∈T, and
A KDE (kernel density estimation) plot, also known as a smooth version of a histogram, can be used to visualize the mpg distribution with breakdowns for each origin value. In terms of statistical testing, we can use one-way ANOVA. The hypothesis we want to test is whether there are significant mean differences in mpg between different car origins.
A/B testing (also known as bucket testing, split-run testing, or split testing) is a user experience research methodology. [1] A/B tests consist of a randomized experiment that usually involves two variants (A and B), [2] [3] [4] although the concept can be also extended to multiple variants of the same variable.