Accessibility Links
- Skip to content
- Skip to search IOPscience
- Skip to Journals list
- Accessibility help
- Accessibility Help
Click here to close this panel.
Purpose-led Publishing is a coalition of three not-for-profit publishers in the field of physical sciences: AIP Publishing, the American Physical Society and IOP Publishing.
Together, as publishers that will always put purpose above profit, we have defined a set of industry standards that underpin high-quality, ethical scholarly communications.
We are proudly declaring that science is our only shareholder.
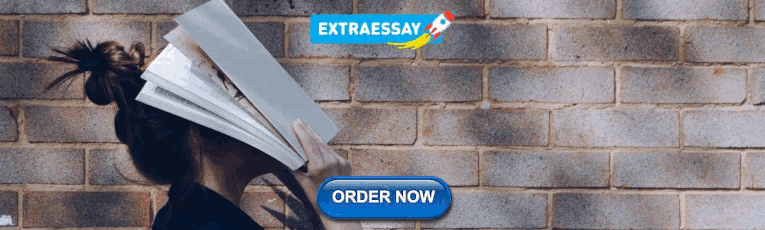
ABC Analysis: A Qualitative Case Study on Inventory Management in Giant Superstore Taman Connaught, An Outlet Of GCH Retail (Malaysia) SDN. BHD.
Shamani Jayakumaran 1 , Wong Zi Shan 1 and Dazmin Daud 1
Published under licence by IOP Publishing Ltd IOP Conference Series: Materials Science and Engineering , Volume 780 , 2019 2nd International Symposium on Traffic Transportation and Civil Architecture (ISTTCA 2019) 13-15 December 2019, Chengdu, China Citation Shamani Jayakumaran et al 2020 IOP Conf. Ser.: Mater. Sci. Eng. 780 072016 DOI 10.1088/1757-899X/780/7/072016
Article metrics
2708 Total downloads
Share this article
Author e-mails.
Author affiliations
1 Faculty of Business and Information Science, UCSI University, Jalan Menara Gading, 56000 Cheras, Kuala Lumpur, Malaysia
Buy this article in print
This study aimed to understand the inventory management of Giant Superstore Taman Connaught. The study was qualitative study that explored the ABC analysis efficiency of inventory management in Giant Superstore, Taman Connaught an outlet of GCH Retail (Malaysia) SDN. BHD. ABC analysis was one method to manage the inventory management who good position was arranged according to the category such as A category product was high in value but low in quantity and C category product was low in value and high in quantity. The elements that were selected as significance included the product consumption rate, carrying cost and replenishment product lead time which contributed toward ABC analysis of inventory management efficiency in Giant Superstore. The study indicated dissimilarities in controlling the inventory in Giant superstore. The study found Giant had used POM.net in their inventory management since this software from ABC tool. HR manager needs to recruit quality skilled workers that have proper qualifications and acceptable for particular job duties.
Export citation and abstract BibTeX RIS
Content from this work may be used under the terms of the Creative Commons Attribution 3.0 licence . Any further distribution of this work must maintain attribution to the author(s) and the title of the work, journal citation and DOI.
Academia.edu no longer supports Internet Explorer.
To browse Academia.edu and the wider internet faster and more securely, please take a few seconds to upgrade your browser .
Enter the email address you signed up with and we'll email you a reset link.
- We're Hiring!
- Help Center
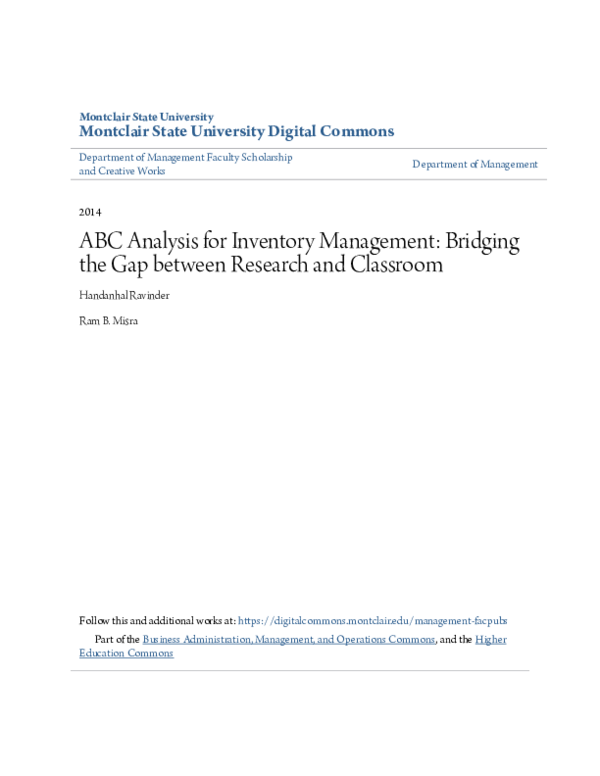
ABC Analysis For Inventory Management: Bridging The Gap Between Research And Classroom

Related Papers
rahmat nurcahyo
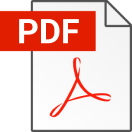
IOSR Journal of Business and Management
Dr. Dinesh Dhoka
Industrial Engineering and Management
Ephrem Gidey
Ankit Sappal
Inherent uncertainties in demands and supply make it difficult for supply chains to achieve optimum inventory replenishment, resulting in loss of sales or keeping excessive inventories. An unkempt inventory can take up to one-third of an organization's annual investment. Therefore, in order to compete with invariably erratic demands, it is not only challenging to develop an intelligent system to maintain and control an optimum level of inventory but has also become mandatory. Here we have tried to study the supply chain logistic management in manufacturing industry using XYZ analysis method. Supply chain: Supply chain management (SCM) is the oversight of materials, information, and finances as they move in a process from supplier to manufacturer to wholesaler to retailer to consumer. Supply chain management involves coordinating and integrating these flows both within and among companies Logistic: Logistics management is that part of the supply chain that plans, implements, and ...
International Journal of Research in Engineering, Science and Management
Maheshwar jaybhaye
This article is a contextual analysis of ABC and HML examination of inventory control methods for an effective Inventory administration framework in the assembling business of India. The organization needs to control its inventory expense, so it utilized various kinds of inventory methods to control this. There are various strategies, for example, ABC, HML, VED, XYZ and S-O-S. In the ABC analysis, things are classifications in classifications A, B, C as per the utilization of the all-out expense. In the HML analysis, things are classifications in H, M, L classification as per the unit cost. Information assortment is chiefly a year through the head supervisor of the store and other work force associated with the Inventory control activity of the assembling business.
Journal of Advanced Management Science
makram ben jeddou
As a result to today's uncertain economy, companies are searching for alternative ways to stay competitive. This study goes through the process of analyzing the company's current forecasting model and recommending an inventory control model to help them solve their current issue. As a result, an Economic Order Quantity (EOQ) and a Reorder Point was recommended to help them reduce their product stock outs. The shortage of raw material for production always makes the process discontinuous and reduces the productivity. The ABC analysis technique for the inventory control system is first used to identify the most important multiple products and then the economic order quantity (EOQ) of each product is developed to find their inventory model equation individually.
shashank soni
Abbas Toloie Eshlaghy
Today, the effective inventory management plays an im-portant role in the success of the organizations in the new business environment. It is not clearly possible for the organizations that store hundreds of inventory items to economically design an inventory management policy for each inventory item separately. Moreover, various inven-tory items may play quite different roles in the business of the organization. Hence, the managers need to classify these items in order to control each inventory category properly based on its importance rating. This research is composed of the following sections: In the first section, the criteria affecting the evaluation of the inventory control system of the studied factory and the priority of each one of them will be identified, in the second section, the prior-ity of each criterion in each inventory category (A, B, C) is calculated using the analytical hierarchy process, in the third section, a mathematical model will be presented to evaluate th...
Antonella Petrillo , Fabio De Felice
The success parameters for any company are on time completion, within specific budget and with requisite performance. In particular an efficient and effective inventory management helps a firm maintaining competitive advantage, especially in a time of accelerating globalization. From this point of view several organizations employ the ABC analysis to have an efficient control on a large number of inventory items. With the increasing levels of integration in manufacturing and service systems conventional ABC analysis is limited because it accounts for only one criterion, mostly "annual dollar usage", for classifying inventory items. To alleviate this shortcoming, this paper proposes a modified version of ABC analysis and Cross Analysis based on Analytic Network Process, a multicriteria approach, that allows to consider several criteria all at once for the optimal choice of materials management.
RELATED TOPICS
- We're Hiring!
- Help Center
- Find new research papers in:
- Health Sciences
- Earth Sciences
- Cognitive Science
- Mathematics
- Computer Science
- Academia ©2024
Inventory Control Through ABC/XYZ Analysis
- Conference paper
- First Online: 24 January 2019
- Cite this conference paper
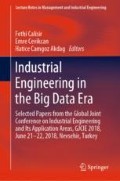
- Esra Agca Aktunc 5 ,
- Meltem Basaran 5 ,
- Gozde Ari 5 ,
- Mumin Irican 5 &
- Sahna Gungor 5
Part of the book series: Lecture Notes in Management and Industrial Engineering ((LNMIE))
2620 Accesses
4 Citations
In this study, inventory and production control of a group of products from a major manufacturer of domestic and industrial gas meters is examined. ABC and XYZ analyses are carried out for the inventory items to determine the production strategy of each item class and the Economic Order Quantity (EOQ). After this examination, one of the end products of the company is chosen to develop the Materials Requirement Plan (MRP) for. The Bill of Materials (BOM) for the chosen product is created and MRP is developed according to the BOM levels. The monthly demand data for the final product is obtained based on the annual demand and the required quantities for all sub materials of the final product are calculated with MRP. Finally, after the ABC/XYZ analysis, BOM structuring, and MRP calculations, a user interface is developed in Excel using Visual Basic for Applications (VBA) to access, edit, and add the desired information easily.
This is a preview of subscription content, log in via an institution to check access.
Access this chapter
- Available as PDF
- Read on any device
- Instant download
- Own it forever
- Available as EPUB and PDF
- Durable hardcover edition
- Dispatched in 3 to 5 business days
- Free shipping worldwide - see info
Tax calculation will be finalised at checkout
Purchases are for personal use only
Institutional subscriptions
Flores, B. E., & Whybark, D. C. (1986). Multiple criteria ABC analysis. International Journal of Operations & Production Management, 6 (3), 38–46.
Article Google Scholar
Hopp, W. J., & Spearman, M. L. (2008). Factory physics (3rd ed.). New York, NY: McGraw-Hill/Irwin.
Google Scholar
Johnston, F. R., & Boylan, J. E. (1996). Forecasting for items with intermittent demand. Journal of the Operational Research Society, 47 (1), 113–121.
Liu, J., Liao, X., Zhao, W., & Yang, N. (2016). A classification approach based on the outranking model for multiple criteria ABC analysis. Omega, 61, 19–34.
McKay, K. N. (2011). The historical foundations of manufacturing planning and control systems. In Kempf, K., Keskinocak, P., & Uzsoy, R. (Eds.), International Series in Operations Research & Management Science, Vol. 151. Planning production and inventories in the extended enterprise . Boston, MA: Springer.
Millstein, M. A., Yang, L., & Li, H. (2014). Optimizing ABC inventory grouping decisions. International Journal of Production Economics, 148, 71–80.
Min, H., & Zhou, G. (2002). Supply chain modeling: Past, present and future. Computers & Industrial Engineering, 43 (1–2), 231–249.
Nahmias, S. (2005). Production and operations analysis (5th ed.). New York, NY: McGraw-Hill/Irwin.
Orlicky, J. (1975). Material requirements planning . New York, NY: McGraw-Hill.
Partovi, F. Y., & Burton, J. (1993). Using the analytic hierarchy process for ABC analysis. International Journal of Operations & Production Management, 13 (9), 29–44.
Reiner, G., & Trcka, M. (2004). Customized supply chain design: Problems and alternatives for a production company in the food industry—A simulation based analysis. International Journal of Production Economics, 89 , 217–229.
Scholz-Reiter, B., Heger, J., Meinecke, C., & Bergmann, J. (2012). Integration of demand forecasts in ABC-XYZ analysis: Practical investigation at an industrial company. International Journal of Productivity and Performance Management, 61 (4), 445–451.
Silver, E. A., Pyke, D. F., & Peterson, R. (1998). Inventory management and production planning and Scheduling (3rd ed.). New York, NY: Wiley.
Teunter, R. H., Babai, M. Z., & Syntetos, A. A. (2010). ABC classification: Service levels and inventory costs. Production and Operations Management, 19 (3), 343–352.
van Kampen, T. J., Akkerman, R., & van Donk, D. P. (2012). SKU classification: A literature review and conceptual framework. International Journal of Operations & Production Management, 32 (7), 850–876.
Vollmann, T. E., Berry, W. L., Whybark, D. C., & Jacobs, F. R. (2005). Manufacturing planning and control for supply chain management . New York, NY: McGraw-Hill/Irwin.
Yu, M. C. (2011). Multi-criteria ABC analysis using artificial-intelligence-based classification techniques. Expert Systems with Applications, 38 (4), 3416–3421.
Article MathSciNet Google Scholar
Download references
Author information
Authors and affiliations.
Department of Industrial Engineering, Faculty of Engineering and Natural Sciences, Kadir Has University, Istanbul, Turkey
Esra Agca Aktunc, Meltem Basaran, Gozde Ari, Mumin Irican & Sahna Gungor
You can also search for this author in PubMed Google Scholar
Corresponding author
Correspondence to Esra Agca Aktunc .
Editor information
Editors and affiliations.
Department of Industrial Engineering, Istanbul Technical University, Macka, Istanbul, Turkey
Fethi Calisir
Emre Cevikcan
Department of Management Engineering, Istanbul Technical University, Macka, Istanbul, Turkey
Hatice Camgoz Akdag
Rights and permissions
Reprints and permissions
Copyright information
© 2019 Springer Nature Switzerland AG
About this paper
Cite this paper.
Aktunc, E.A., Basaran, M., Ari, G., Irican, M., Gungor, S. (2019). Inventory Control Through ABC/XYZ Analysis. In: Calisir, F., Cevikcan, E., Camgoz Akdag, H. (eds) Industrial Engineering in the Big Data Era. Lecture Notes in Management and Industrial Engineering. Springer, Cham. https://doi.org/10.1007/978-3-030-03317-0_15
Download citation
DOI : https://doi.org/10.1007/978-3-030-03317-0_15
Published : 24 January 2019
Publisher Name : Springer, Cham
Print ISBN : 978-3-030-03316-3
Online ISBN : 978-3-030-03317-0
eBook Packages : Engineering Engineering (R0)
Share this paper
Anyone you share the following link with will be able to read this content:
Sorry, a shareable link is not currently available for this article.
Provided by the Springer Nature SharedIt content-sharing initiative
- Publish with us
Policies and ethics
- Find a journal
- Track your research
Click through the PLOS taxonomy to find articles in your field.
For more information about PLOS Subject Areas, click here .
Loading metrics
Open Access
Peer-reviewed
Research Article
Computed ABC Analysis for Rational Selection of Most Informative Variables in Multivariate Data
Affiliation DataBionics Research Group, University of Marburg, Hans–Meerwein—Straße, 35032, Marburg, Germany
* E-mail: [email protected]
Affiliations Institute of Clinical Pharmacology, Goethe—University, Theodor—Stern—Kai 7, 60590, Frankfurt am Main, Germany, Fraunhofer Institute for Molecular Biology and Applied Ecology IME, Project Group Translational Medicine and Pharmacology TMP, Theodor–Stern—Kai 7, 60596, Frankfurt am Main, Germany
- Alfred Ultsch,
- Jörn Lötsch
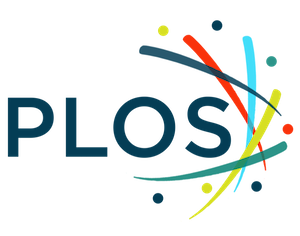
- Published: June 10, 2015
- https://doi.org/10.1371/journal.pone.0129767
- Reader Comments
Multivariate data sets often differ in several factors or derived statistical parameters, which have to be selected for a valid interpretation. Basing this selection on traditional statistical limits leads occasionally to the perception of losing information from a data set. This paper proposes a novel method for calculating precise limits for the selection of parameter sets.
The algorithm is based on an ABC analysis and calculates these limits on the basis of the mathematical properties of the distribution of the analyzed items. The limits im-plement the aim of any ABC analysis, i.e., comparing the increase in yield to the required additional effort. In particular, the limit for set A, the “important few”, is optimized in a way that both, the effort and the yield for the other sets (B and C), are minimized and the additional gain is optimized.
As a typical example from biomedical research, the feasibility of the ABC analysis as an objective replacement for classical subjective limits to select highly relevant variance components of pain thresholds is presented. The proposed method improved the biological inter-pretation of the results and increased the fraction of valid information that was obtained from the experimental data.
Conclusions
The method is applicable to many further biomedical problems in-cluding the creation of diagnostic complex biomarkers or short screening tests from comprehensive test batteries. Thus, the ABC analysis can be proposed as a mathematically valid replacement for traditional limits to maximize the information obtained from multivariate research data.
Citation: Ultsch A, Lötsch J (2015) Computed ABC Analysis for Rational Selection of Most Informative Variables in Multivariate Data. PLoS ONE 10(6): e0129767. https://doi.org/10.1371/journal.pone.0129767
Academic Editor: Yongtang Shi, Nankai University, CHINA
Received: February 19, 2015; Accepted: May 13, 2015; Published: June 10, 2015
Copyright: © 2015 Ultsch, Lötsch. This is an open access article distributed under the terms of the Creative Commons Attribution License , which permits unrestricted use, distribution, and reproduction in any medium, provided the original author and source are credited
Data Availability: This is a method paper. The example used for demonstration purposes is taken from the authors' previous work. All data is available in Table 2 of the paper, originally from the published reference [ 3 ] (Neddermeyer TJ, Flühr K, Lötsch J (2008) Principal components analysis of pain thresholds to thermal, electrical, and mechanical stimuli suggests a predominant common source of variance. Pain 138: 286-291) In addition, the related R library is freely available at http://cran.r-project.org/web/packages/ABCanalysis/index.html .
Funding: The research received funding, in particular the necessary computation equipment, from the European Union Seventh Framework Programme (FP7/2007 – 2013) under grant agreement no. 602919 (JL). The funders had no role in method design, data selection and analysis, decision to publish, or preparation of the manuscript.
Competing interests: The authors have declared that no competing interests exist.
Introduction
A recurring problem in biomedical research is the high dimensionality of data sets and the complexity of derived results. Multivariate data sets often differ in several factors or derived statistical parameters, which have to be selected for a valid interpretation. This selection is usually based on contextual and mainly traditional statistical limits. This leads occasionally to the perception of losing information from a data set; however, crossing the accepted statistical limits will be rejected almost certainly by a scientific audience. Dealing with the problem of statistical limits is an active research topic; however, the correct statistical approach at a rational selection of the most informative set of variables derived from multivariate analyses is not obvious. Scientists are therefore often inclined to use conservative statistical selection criteria to avoid α error. This is widely accepted but has a tendency toward occasionally disregard of valid information from experimental data.
Therefore, a theoretical basis for the selection of parameter sets that are interpretable in multivariate data is highly desirable to identify the optimum information that can be validly retrieved from biomedical data. The present report proposes a novel method that uses concepts developed in economical sciences. In particular concepts are used in the search for a minimum possible effort that gives the maximum yield. In many circumstances it has been observed that this converges toward the effect that with 20% of the effort 80% of all yield can be obtained, which is commonly called the “Pareto 80/20 rule” [ 1 , 2 ]. A more general approach is the so-called “ABC analysis”, which divides the data set into the three disjoint sets A, B and C, in such way that set “A” should contain the “important few”while set “C” contains the “trivial many” [ 2 ].
The determination of the set limits for an ABC analysis has so far been left to subjective considerations. In this paper, a calculation method is presented that allows calculating these limits on the basis of the mathematical properties of the distribution of the analyzed items. The utility of the proposed method will be illustrated by an example from own previous research [ 3 ] where this method improves the biological interpretation of the results and increased the fraction of valid information that can be obtained from experimental data. Further biomedical applications, such as deriving screening tests from complex test batteries, will be discussed.
Properties of ABC curves
- PPT PowerPoint slide
- PNG larger image
- TIFF original image
In addition, APC plots of the identity distribution, x i = constant (magenta line), and of the uniform distribution in the data range U[min(xi) , max(xi)] (green line) are shown. The second main diagonal (dashed line) delivers the points where the yield Y equals the remaining effort described as Y = 1—E . The BreakEven point, i.e., the point on the ABC curve where the profit gain dABC equals 1 which means that the slope of the ABC curve at this point equals a value of 1, is marked a as green star. The limits of sets A, B and C resulting from the present ABC analysis are drawn as red lines.
https://doi.org/10.1371/journal.pone.0129767.g001
ABC curves are always non-decreasing concave functions in the unit square space. They are scale-invariant in the values of x . That is, a multiplicative modification of the x-values does not modify the ABC curve, i.e., ABC(x) = ABC(x∙c) for any c ≠ 0. However they are not invariant to the location of x . That is, an additive modification of the values of x does modify the ABC curve, i.e., ABC(p) ≠ ABC(p+c) for any c ≠ 0. For x i ’ = x i + c with c > 0, the ABC curve ABC(x’) will flatten and approach the identity distribution (green line in Fig 1 ). A special case is observed when x i approaches a value of 0. Then, the curve approaches a “winner-takes-it all” distribution, i.e., tends to take a path through the points (0,0)—(0,1)—(1,1). Thus, the localization of ABC curves of a given data set relatively to (i) the identity distribution, i.e., all x i have the same value x i = c , and (ii) the uniform distribution, i.e., all values that x i can take are equally likely in the interval from min(x i ) to max(x i ) , can be used to investigate the inequality of a distribution ( Fig 1 ). Specifically, for all more right-skewed data distributions than the uniform distribution, the ABC curves will be located more toward the upper left corner of the plot.
ABC curves are related to Lorenz curves as follows: Let L(p) be a Lorenz curve for a probability distribution. The corresponding ABC curve ABC(p) can be derived as ABC(p) = 1—L(1—p) and vice versa L(p) = 1—ABC(1—p) . Following this interrelations, theoretical properties of ABC curves can be derived from the corresponding Lorenz curves. Moreover, analytical derivations of well-known distributions available for Lorenz curves can also be used for ABC curves ( Table 1 ).
https://doi.org/10.1371/journal.pone.0129767.t001
Calculation of precise limits for ABC analysis
An ABC analysis aims at identifying the minimum possible effort that gives the maximum yield. It divides the values x 1 , …, x n into three disjoined sets A, B, and C [ 8 ]. Set A should contain the “critical few”, i.e., those elements that allow obtaining a maximum of yield with a minimal effort [ 1 , 2 ]. Set B comprises those elements where an increase in effort is proportional to the increase in yield. In contrast, set C contains the “trivial many”, i.e., those elements with which the yield can only be achieved with an over-proportionally large additional effort. The determination of these sets has been so far left to subjective judgments [ 8 , 9 ].
The derivation of statistically justified set limits regards the increase in “yield”( Y ) versus the increase in “effort” ( E ). Formally, this is the first derivative (slope) of the ABC curve ( dY/dE = dABC ), in the following called “profit gain”. Set A should contain profit gains > 1 ( COND1 ), set B should contain profit gains around a value of 1 ( COND2 ), while the profit gain in set C should be substantially less than 1 ( COND3 ). During ABC analysis the yield Y should be maximized while the necessary effort E minimized. Thus, to obtain the limit between sets A and B two variables need to be optimized. Moreover, as maximizing Y can be achieved via maximizing the unrealized yield UY = 1 –E , the optimization problem can be reduced to concomitantly minimizing both, E and UY .
Derivation of the limit between sets A and B.
The fraction of the first i elements to n (i/n) represents costs or efforts ( E i = i/n ), the fraction of the cumulative sum of the first xi values with regard to the total sum of the x i is called the yield, Y i , of the set x 1 , …x i . The ABC curve (blue line) is a plot of Y i versus E i . Intermediate points are interpolated by means of quadratic splines [ 7 ]. The ABC curve is independent of m. The red star marks the so called Pareto point A(A x , A y ) , i.e., the point at the smallest distance (left oblique black line) to the ideal point at, xy, E = 0 and Y = 1 . The green star marks the point on the ABC curve where its slope, dY/dE , equals 1. At this point, the profit gain dABC equals 1, therefore it is called the BreakEven point B(Bx , By) . The blue star marks the point C(C x , C y ) that has the smallest distance to the ideal situation where all gain has been achieved, i.e., E = B x and Y = 1 (right oblique black line).
https://doi.org/10.1371/journal.pone.0129767.g002
The point on the ABC curve which has the smallest (Euclidean) distance to ABCideal is called the Pareto point A(A x , A y ) . Its x-value, A x , provides a precise limit for data points in the set A. The point on the ABC curve where the profit gain dABC equals a value of 1 is called the “ BreakEven” point B(B x , B y ) . Usually A x ≤ B x holds in practice. However, this cannot be guaranteed for all possible distributions. In the case of A x > B x the points A and B exchange their role in ABC analysis. This procedure assures COND1 for all distributions, i.e., for x i ≤ A x the “profit gain” is ≥ 1.
From this derivations the set limit between sets A and B, t AB , is given as min(A x , B x ) . Set A is defined as A = {x i | x i ≤ icdf(X , t AB *100)} where icdf(X , p) for X = { x 1 , …, x n . } is the x i corresponding to the p th percentile. Set A contains the largest values of x i down to a point where the ABC curve is closest to the ideal situation of zero effort and complete yield, as long as the ProfitGain is larger than 1. For the Uniform distribution set A contains the largest 41% of all values ( Fig 2 , red star). A geometric interpretation of the set limit between A and B is the point on the ABC curve that has the smallest distance from the ideal point (0,1; black line to the red star in Fig 2 ).
Derivation of the limit between sets B and C.
According to the characteristics of an ABC analysis, the profit gain in set C should be substantially less than 1 ( COND3 ). At an ideal point B y = 1 , called the BreakEven point, all yield would be gained. The point on the ABC curve at a minimum distance from this ideal point ( B x , 1 ) is called SubMarginal point ( C x ,C y ). For all points to the right of C x , i.e., x i ≥ C x , the profit gain is substantially less than 1 as required by COND3 . From this derivations the set limit between sets B and C is given by t BC = C x and set C is C = {x i | x i > icdf(X , t BC *100))} . For the Uniform distribution, set C contains the smallest 38% of the values ( Fig 2 blue star). The remaining values of x i , neither associated to set A nor to set C, have to belong to set B. This set contains the values “around” a profit gain of 1 ( COND2 ). For the uniform distribution these profit gains are in the range of 0.78 to 1.18. A geometric interpretation of the set limit between B and C is determined by the point at the smallest distance to the ideal point ( B x , 1 ; black line to the blue star in Fig 2 ).
The programs used to calculate the following ABC curves, which also perform the described precise ABC analysis and draw ABC plots, are part of the R package “ABCanalysis” (M. Thrun, Marburg, Germany) published on CRAN at http://cran.r-project.org/web/packages/ABCanalysis/index.html .
ABC analyses of known distributions
This probability distribution is a typical example of a right skewed inequality distribution. The magenta lines indicate the location of the median (0.47) of the distribution (see also Fig 1 for comparison with less skewed distributions).
https://doi.org/10.1371/journal.pone.0129767.g003
https://doi.org/10.1371/journal.pone.0129767.g004
ABC analysis of biomedical sample data
The following example from biomedical research shows the utility of the present analysis for providing a statistically valid rationale selection of components for principal components analysis. Specifically, empirical data often consist of a high dimensional set of observed variables. For example, we have previously analyzed the sources of variance of pain thresholds to six different nociceptive stimuli, i.e., thermal (heat or cold), electrical or mechanical (blunt or punctate pressure) pain stimuli [ 3 ]. Some of these variables showed a (linear) correlation with others. For dimensionality reduction without losing too much information and a conversion of the possibly correlated variables into a set of values of linearly uncorrelated variables, a principal component analysis (PCA) was used. This resulted in eight variance components ( Table 2 ). Setting the limit of the number of principal components, PCs, ( Fig 5 ) at the traditionally advised Kaiser-Guttman criterion of an eigenvalue > 1 of the covariance matrix [ 11 , 12 ] resulted in three major sources of variance that could be used to interpret the most important sources of variance of human pain thresholds. A similar selection of PCs also results when applying the “elbow criterion” in a so-called scree plot of the absolute values of the eigenvalues sorted for decreasing size ( Fig 5 top left, red curve). The elbow criterion is estimated as the point where the steep slope to the left of the scree plot levels to a flat slope [ 13 ]. The PCs thus identified by these classical criteria as results of this analysis carried high loadings from all pain stimuli (PC #1), from electrical, blunt pressure and thermal pain stimuli (PC #2) or from punctate pressure pain stimuli (PC #3). However, these PCs failed to translate the distinction between thresholds despite the involvement of different receptors in their perception [ 14 ].
Left panel: sample data showing the original results of a principal component analysis (PCA) of the covariance matrix among six different measurements of pain thresholds [ 3 ]. For classical selection of the set of relevant (largest) eigenvalues, either the Kaiser-Guttman criterion (eigenvalue > 1, left top panel , magenta line) [ 11 , 12 ] or the “elbow criterion” [ 13 ] in a so-called scree plot of the absolute values of eigenvalues sorted by descending size (left top panel, red curve) can be used. According to the PCA results, the sources of variance of pain thresholds to different nociceptive stimuli comprise eight variance components (see Table 2 in [ 3 ]). The left bottom panel shows the cumulative fraction of the variance explained by the principal components, with indication of the variance explained by the components that could be selected based on the classical criteria. It can also be seen that the inclusion of a further principal component would have provided a better coverage of the total variance. Right panel: ABC plot of the same data. The ABC curve (blue line) shows from bottom to top the increasing faction of the total sum of all eigenvalues and from left to right the increasing fraction of the total number of variance components which contribute these eigenvalues. The goal of the analysis is to identify the number of variance components (abscissa) that is associated with a satisfactorily high coverage (ordinate) of the total sum of available eigenvalues. Set A contains the largest data points, corresponding to the largest proportion of yield. For this particular distribution the set limit for A is obtained by the point with slope of the ABC curve of 1 (green star) resulting in the two largest eigenvalues. The set B consist of the next two eigenvalues including the Pareto point, i.e., the point closest to (0,1) (red star). In the present example the cumulative variance of component #1, 2and 3 contributed 75% to the total variance. The inclusion of component #4 results in a cumulative explained variance of 84%. On the other end, set C contains 50% of the eigenvalues, which share only 16% of the variance and are obviously of minor importance.
https://doi.org/10.1371/journal.pone.0129767.g005
https://doi.org/10.1371/journal.pone.0129767.t002
The present ABC analysis can provide a better alternative to the rather subjective Kaiser-Guttman or Elbow criteria. Specifically, following calculation of precise limits for the obtained eigenvalues, set A contained the largest eigenvalue while set B contained three further eigenvalues ( Fig 5 ). Thus, when disregarding set C with the four smallest eigenvalues, the present analysis provides support to take four eigenvalues into account, instead of three eigenvalues when applying classical limits. The attritional PC, with an eigenvalue of 0.74 that had to be dropped from the results in the classical analyses, carried loadings from Heat + capsaicin pain threshold (see Table 2 in [ 3 ]). This better reflects the different molecular biology involved in the perception of heat pain, mediated via ion channels such as TRPV1 and TRPV4 [ 15 ], from the perception of pain evoked by other stimuli. Thus, the present ABC analysis substantially improved the identification of the important few among the variance components of pain thresholds. The curvatures of the ABC curve for the eigenvalues correspond to a multimodal probability density function of the data [ 16 ]. Moreover, the modified results were not only biologically more meaningful; they also included a larger part of the information contained in the pain thresholds data set. That is, while the Kaiser-Guttman criterion [ 11 , 12 ], requesting an eigenvalue > 1 for a PC to be considered, explained only 75% of the total variance in the pain thresholds, the ABC analysis resulting in n = 4 PCs provided 84% of the total variance explained, to which set A of n = 1 PCs contributed 48% ( Fig 5 bottom left).
In the analysis of multivariate biomedical data the usually peremptory application of traditional statistical limits is sometimes perceived as leading to a loss of information that could have been validly drawn from a data set. Without a theoretical basis, however, crossing classical limits cannot be advised. We therefore suggest a method to identify the “important few” from sets of items that show a clear inequality in their distribution and provide a calculation of precise set limits based on mathematical properties of the distribution of the analyze items. The present method is based on a calculated ABC analysis, replacing the traditional subjective estimations of ABC set limits by algorithmically determined optimal limits. The innovation of the present method consists of using minimization of the effort and of the unrealized yield, together with optimization of the slope of the ABC curve to precisely calculate these limits as a basis for a valid selection criterion for items from a set of data or parameters.
ABC analyses have their roots in economic thinking. That is, the success of a business depends on efficiency in the sense that returns are always regarded with respect to the efforts or costs required to obtain them. Therefore a large application domain of ABC analysis is business administration or material management. However, its application into the biomedical domain relates to effect sizes, which are ubiquitously addressed in this field. For example, the modest predictive value of common genetic variants in human traits can be attributed, despite statistically significant effects, to the mostly small effect sizes conferred by these variants [ 17 ]. The present ABC approach directly addresses this issue by selecting the “important few”, i.e., those items that confer the relatively largest effect sizes. In this respect, it completely fits with contemporary statistical data analysis approaches and is meant to be used there as the example of pain threshold variance components emphasizes.
Indeed, when exemplary joining genetics and pain thresholds, common functional variants exert small effect sizes [ 18 ] but when combined, they are able to predict particular pain phenotypes at an accuracy of 80% [ 19 ]. When applying ABC analysis to that data, the variants that have previously been included in the predictive combined genotype were identified as those lying in ABC set A (details not shown). A further example of the utility of the present method in biomedicine is the applicability of the effort versus gain problem to common medical screening test problems. A common desire of physicians in practice is the availability of short and easily applicable tests. This has led to various efforts to create abbreviated tests from comprehensive test batteries, such as a three-item test for olfactory diagnosis derived from a comprehensive 48-item test [ 20 ]. The development of this test is, retrospectively, a candidate for an ABC analysis, which could provide the important few olfactory tests items on a statistically valid level rather than the intuitive selection that had been applied when developing the test.
An advantage of the present method is its applicability to small data sets such as the present example of pain threshold data containing only eight data points (the eight eigenvalues obtained by means of PCA). For small numbers of points the ABC analysis relied on the quadratic spline interpolation of the ABC curve. This interpolation has been established as optimal for generating valid Lorenz curves [ 7 ], therefore, via the above-explained relation of ABC curves to Lorenz cures this is also valid for ABC curves. Importantly, data preprocessing, typically consisting of adjustments of the data range and or variance, must take into account that ABC curves are invariant to scaling, i.e., multiplication by some constant but not to location, i.e., the addition/subtraction of a constant to the data. In particular ABC curves are only defined for non-negative data points x i ≥ 0. So a standardization of the data should be restricted to a mapping of the data to unit variance.
However, the method is neither restricted to biomedical data nor to small data sets. Another example where it can be applied is taken from demographic analyses. The “SwissInhabitants” data set was obtained from an official statistics source [ 21 ] and consists of the number of inhabitants in the 2896 villages and cities in Switzerland in the year 1900. Such data can be explored by the present method to describe the population structure of a country. Applying the present ABC analysis to this data set showed that 69% of the Swiss population lived in only 639 places (22%) in 1900( Fig 6 ). Nearly 80% of the villages were populated the remaining approximately 30% of the population. A reanalysis of the distribution for the year 1970 shows a concentration effect such that set A now contains only 19% of the cities in which 76% of the Swiss population lives. This somehow resembles the 80/20 rule. The “SwissInhabitants” data set was analyzed in detail in elsewhere [ 22 ] and is this freely available data set is also included as an example data set within the above-mentioned “ABCanalysis” R package published on CRAN at http://cran.r-project.org/web/packages/ABCanalysis/index.html .
The data consists of the number of inhabitants in the 2896 villages and cities in Switzerland in the year 1900. The analysis shows that 69% of the population lives in 22% of the locations. The ABC curve (blue line) is a plot of Y i , here the cumulative fraction of the Swiss population in 1900, versus E i , here the number of locations (villages or towns in Switzerland). The distribution shows higher inequality than the uniform (green line) and the identity (magenta line) distributions. The red star marks the so called Pareto point A(A x , A y ) , i.e., the point at the smallest distance (left oblique black line) to the ideal point at, xy, E = 0 and Y = 1 . The green star marks the point on the ABC curve where its slope, dY/dE , equals 1. At this point, the profit gain dABC equals 1, therefore it is called the BreakEven point B(Bx , By) . The freely available data set on which this analysis was done is also included as an example data set within the above-mentioned “ABCanalysis” R package published on CRAN at http://cran.r-project.org/web/packages/ABCanalysis/index.html .
https://doi.org/10.1371/journal.pone.0129767.g006
Finally, the relation of ABC curves to the so-called “Pareto 80/20 Rule”, mentioned above because of its broad recognition, needs clarification. There is no such thing as a “Pareto 80/20-Rule”. Juran has clarified [ 2 ] that he mistakenly attributed the 80/20 rule of “roughly 80% of the yield comes from 20% of the effects” to Vilfredo Pareto (1848–1923), who, however, has never published an “80/20-rule”. It should rather be called “Juran 80/20 Rule”. A family of probability distributions, which depend on a parameter α, are called Pareto distributions [ 26 ]. For a special value of α = 1.16 the ABC curve of this particular Pareto distribution passes through the point P = (0.2, 0.8). The, so called “80/20 rule” is just the observation that the ABC curve passes in many empirical situations close the point P. If there is a physical law, that systems tend to show ABC curves with the 80/20 rule, it is still unknown [ 27 ].
In this work mathematically defined unique and precise limits for an ABC analysis have been derived. The limits implement the aim of any ABC analysis, i.e., comparing the increase in yield to the required additional effort. In particular, the limit for set A, the “important few”, is optimized in a way that both, the effort and the yield for the other sets (B and C), are minimized. As a typical example from biomedical research, the feasibility of the ABC analysis as an objective replacement for classical subjective limits to select highly relevant variance components of pain thresholds is presented. The method is applicable to many further biomedical problems including the creation of diagnostic complex biomarkers or short screening tests from comprehensive test batteries. Thus, the ABC analysis can be proposed as a mathematically valid replacement for traditional limits to maximize the information obtained from multivariate research data.
Acknowledgments
We thank Michael Thrun for the implementation of the R package and his critical discussions.
Author Contributions
Conceived and designed the experiments: AU. Analyzed the data: AU JL. Wrote the paper: JL AU.
- 1. Pareto V (1909) Manuale di economia politica, Milan: Società editrice libraria, revised and translated into French as Manuel d’économie politique. Paris: Giard et Briére.
- View Article
- Google Scholar
- PubMed/NCBI
- 9. Wild T (2002) Best practice in inventory management. Oxford: Butterworth-Heinemann (imprint of Elsevier).
- 10. Krause EF (1988) Taxicab geometry: Adventure in non-euclidean geometry. Mineoly, NY: Dover Publications.
- 13. Krzanowski WJ (2000) Principles of Multivariate Analysis. A User’s Perspective. New York: Oxford University Press.
- 20. Hummel T, Pfetzing U, Lötsch J (2010) A short olfactory test based on the identification of three odors. J Neurol.
- 21. Schuler M, Ullmann D, Haug W (2002) Eidgenössische Volkszählung 2000—Bevölkerungsentwicklung der Gemeinden 1850–2000. In: Statistik Bf, editor. Neuchâtel: Bundesamt für Statistik. pmid:11186393
- 22. Behnisch M, Ultsch A (2010) Clustering of Population Patterns in Switzerland 1850–2000; Gaul W, Geyer-Schulz A, Schmidt-Thieme L, Kunze J, editors. Heidelberg: Springer.
- 23. Knoblauch J, Kurz J (2009) Die besten Mitarbeiter finden und halten. Die ABC-Strategie nutzen. Frankfurt, New Yoek: Campus Verlag.
- 24. Farrington B, Lysons K (2012) Purchasing and supply chain management. Harlow: Peason.
- 25. Färber I, Günnemann S, Kriegel H-P, Kröger P, Müller E, Schubert E, et al. On using class-labels in evaluation of clusterings. In: Fern XZ, Davidson I, Dy J, editors; 2010; Washington, DC.
- 26. Arnold BC (1983) Pareto distributions. Fairland, Maryland: International Cooperative Publishing House

An official website of the United States government
The .gov means it’s official. Federal government websites often end in .gov or .mil. Before sharing sensitive information, make sure you’re on a federal government site.
The site is secure. The https:// ensures that you are connecting to the official website and that any information you provide is encrypted and transmitted securely.
- Publications
- Account settings
Preview improvements coming to the PMC website in October 2024. Learn More or Try it out now .
- Advanced Search
- Journal List
- J Pharm Policy Pract
- PMC10129016

Analysis of pharmaceutical inventory management based on ABC-VEN analysis in Rwanda: a case study of Nyamagabe district
Ephrem mfizi.
1 East African Community Regional Centre of Excellence for Vaccines, Immunization, and Health Supply Chain Management, College of Medicine and Health Sciences, University of Rwanda, Kigali, Rwanda
François Niragire
2 Department of Applied Statistics, College of Business and Economics, University of Rwanda, Kigali, Rwanda
Thomas Bizimana
Marie françoise mukanyangezi.
3 College of Medicine and Health Sciences, University of Rwanda, Kigali, Rwanda
Associated Data
The data sets generated and analysed during the current study are included in the manuscript and are available from the corresponding author on reasonable request.
Pharmaceuticals account for a large portion of healthcare spending in healthcare organizations. Their effective inventory management is required to match the cost of stocks with the customer demand and avoid shortage of supplies at any health facility level. This study aimed to analyze pharmaceuticals' inventory management using ABC-VEN analysis.
The study was conducted at Rwanda Medical Supply (RMS) Ltd, Nyamagabe Branch for products distributed to health facilities in Nyamagabe District catchment area from the financial years 2017–2018 to 2019–2020. It consisted of a descriptive retrospective study of 457 items. The latter are generic essential medicines distributed to public health facilities during the study period. Products were arranged according to a descending order of importance, and we performed a breakdown of products according to the Pareto Principle. Following an ABC analysis of distribution data for such drugs billed to healthcare facilities, a VEN analysis was performed to identify high-value vital products that require more attention.
During the ABC analysis, 76 products were classified in group A. These accounted for 19.84% and had a value of 74.91% of the total cost of all products. Group B included 116 products, representing 30.29% with a value of 20% of the total cost, while Group C had 191 products, representing 49.87% with a value of only 5.09% of the total cost. During the VEN analysis, 202 products (44.20%) were classified as vital, 231 (50.54%) as essential, and 24 products (75.26%) as non-vital. The analysis with ABC-VEN resulted in Class I representing 55.80% of all medicines that cost 87.88% of all total cost, Class II representing 40.70% with a total cost of 11.82%, and Class III representing 3.50% with a cost of 0.3%.
Conclusions
This study results show that inventory management of vital and expensive products, such as antibiotics, antihypertensive pharmaceuticals, consumables, and massive solutions would be carefully monitored to prevent a shortage of such products at health facility levels. The ABC-VEN analysis is one of the practical and affordable method to achieve their optimized supply chain.
Supplementary Information
The online version contains supplementary material available at 10.1186/s40545-023-00540-5.
The third edition of the WHO Drug Situation Report 2011 recommended that countries increase their efforts to measure and monitor the prices and availability of medicines to respond to observed changes in the cost of medicines [ 1 ]. These efforts would improve procurement, reduce medicines prices by eliminating tariffs and taxes on pharmaceuticals, and promote high-quality generic medicines. In addition, profit margins should be regulated to avoid high costs [ 2 ]. Health commodities account for a large portion of healthcare spending in healthcare organizations [ 3 , 4 ]. However, with a frequent shortage of medicines, it would not be easy to ensure the best healthcare service delivery [ 5 – 8 ]. Thus, effective inventory management is needed to match the cost of stocks with the demand for medicines [ 3 , 9 , 10 ]. Optimal medicines management helps ensure that essential drugs are available at affordable prices [ 1 ]. Reliable data would, therefore, contribute to an uninterrupted healthcare supply chain by planning and estimating the medicines needed and the total charges in a given period [ 3 , 11 , 12 ].
Different inventory management techniques are used for pharmaceutical expenditure analysis [ 13 ]. Among inventory control techniques used, we may enumerate ABC, VEN, FSN, HML, SOS, and ABC-VEN [ 14 ]. Among those techniques, ABC-VEN analysis is often preferred since it contributes to analyzing the medicines expenditures based on public health value and cost [ 12 , 15 ]. ABC analysis enables efficient management of pharmaceuticals, especially in hospitals and healthcare supply chain facilities, helps compare the cost of managed items and may optimize services by predicting inventory level, ordering, and purchasing cost. Combined with VEN analysis, ABC gives better results for products with high priority considering the price and public health importance [ 4 , 7 , 13 ]. This analysis provides information on the budget spent on medicines in general, and on a particular group of medicines. It provides useful information to direct policymakers and managers to plan the required funding for medicines and how it would be allocated [ 7 ]. ABC-VEN analysis classifies health commodities into three categories, Category I with vital and expensive products and fast-moving products. Category II represents essential and quite expensive products, while category III represents non-essential and less expensive products [ 2 , 15 ]. ABC-VEN analysis has been conducted in different countries, giving relevant results supporting health supply chain systems. The studies conducted in India, Soudan, Ethiopia, and Kenya showed that medicines in the A categories consume an enormous amount of products, between 70% and 80% of the total [ 12 , 15 – 18 ].
In Africa, studies were conducted in South Sudan, Ethiopia, and Kenya on the inventory control and expenditure of medicines in health facilities and the supply chain, which provided information on the classification of drugs according to ABC-VEN and helped to improve medicine supply and resource management [ 16 – 18 ]. Studies done in Rwanda showed the shortage in essential medicine in terms of availability and affordability [ 5 , 6 , 19 , 20 ]. Currently, there is limited literature relating the accessibility of medicines to inventory control techniques in the Rwanda health sector. In particular, the status of pharmaceutical inventory management concerning the budget spent on vital, essential, and non-essential pharmaceuticals in Rwanda is currently unknown. This study aimed to assess inventory management of pharmaceutics using ABC-VEN analysis in Rwanda Medical Supply Ltd, Nyamagabe Branch.
This study was conducted at Rwanda Medical Supply (RMS) Ltd, Nyamagabe branch, Nyamagabe district, Rwanda. Rwanda Medical Supply Ltd is a company owned by the government of Rwanda and is tasked with ensuring the availability of medicines in the country. It was established in 2020 as a merger of the Central Medical Store, the then Medical Procurement and Production Division (MPPD), and thirty District Pharmacies [ 21 ]. The study covered the data from the RMS Ltd, Nyamagabe Branch in Nyamagabe district. The latter is one of the thirty administrative districts of Rwanda, located in the Southern province, with a surface area of 1090 km 2 and more than 341,491 inhabitants [ 22 ]. It is located 188 km from Kigali, the Rwanda’s capital.
Study design and data
We used a retrospective cross-sectional design to analyze the annual medicine expenditures and explore pharmaceutical inventory control management. The analyses concerned data covering the period from 2017 to 2020.
Study population and sample
This quantitative research targeted all health products distributed to the Nyamagabe administrative district's health facilities. A list of 457 essential medicines was used for this study. It included all articles distributed to health facilities in essential generic medicines.
The management of essential medicines is different from managing products of vertical programs. All medicines managed at Rwanda Medical Supply Ltd, Nyamagabe Branch, in the category of essential generics medicines, were part of the study. However, the vertical programs medicines such as anti-retroviral, anti-malarial, anti-tuberculosis, and maternal and child health (MCH) commodities were not part of the study. The products of the vertical programs were excluded, since there is a line for good management. Different stakeholders involved in managing vertical program medicines contribute to daily follow-up, quantification, and fund mobilization to mitigate any problem.
Data collection
A data collection tool was designed to capture information about medicines distributed, quantity, unit price, and total prices of items that are part of the study. The distribution data were collected on essential generic medicines, sales, unit cost, and total cost for a 3-year period spanning from July 2017 to June 2020. The secondary data were collected in the RMS Nyamagabe Branch database and other management support tools: stock cards and reports.
Data processing and data analysis
Microsoft Excel 2013 was used for data entry and analysis. We used descriptive statistics, including tabular, and numerical methods, to describe the main characteristics. In performing ABC analysis, the total expenditures for each item were calculated and arranged in descending order. Then, we calculated the percentage of cost and the cumulative cost. After that, we classified products into ABC categories. VEN analysis was done using judgmental methods by organizing products using existing references [ 3 , 4 , 18 ].
We conducted an ABC analysis to classify medicines based on their budget expenditure and a VEN analysis to classify pharmaceuticals based on their public health value (Additional file 1 : Table S1). After combining ABC and VEN analysis by cross-tabulation, we obtain the ABC-VEN matrix, which is used to form various categories [ 3 , 4 , 23 ].
According to the ABC-VEN analysis (Additional file 2 : Table S2), there are nine groups from which three classes of medicines were obtained as follows [ 23 ]. Category I contains all expensive or vital products: AV, BV, CV, AE, AN. Category II contains other essential or B group medicines: BE, BN, and CE. Category III includes the cheapest and non-essential medicines: CN.
Medicines expenditure according to ABC analysis
The ABC analysis considered the total number of 457 essential generic drugs (Additional file 1 : Table S1). It was found that 90 products, which accounted for 19.7% of all products sold, had a value of 77.70% of the total cost of all products, and these products were classified into group A. In Group B, 119 products, which represented 26.03% of all products, were ranked with 17.46% of the total cost, while in Group C, 248 products, which represented 54.27% of all products, were classified with a total cost of only 4.84%.
Top twenty pharmaceutical products ranked according to the cost during 3 years
Products that take up a large part of the budget include antibiotics, medical consumables such as examination gloves, massive solutions, and medicines to treat non-communicable diseases. All antibiotics in the top twenty commodities represent 15.06% of the total cost of all items. In all forms combined, Amoxycillin represented 9.68% of the total cost of all items distributed at the study site (Table (Table1 1 ).
List of top twenty products ranked by cost in 3 years
VEN analysis
In the VEN analysis, 202 products (44.20%) were classified as essential products, 231 products (50.54%) as essential products, and 24 products (5.26%) as non-essential products (Additional file 1 : Table S1). Vital products accounted for 38.2% of the total cost, essential products 59.17%, and non-essential products 2.63%.
ABC-VEN analysis
There were three classes in the ABC-VEN analysis classification: Class I, Class II, and Class III.
According to the ABC-VEN classification in Table Table2, 2 , Class I accounted for 55.80% of all medicines, corresponding to 87.88% of the total costs. Class II accounts for 40.70% of all medicines, which is 11.82% of the total cost, and Class III accounts for 3.50% of all medicines, which is 0.30% of the total cost.
Results of ABC-VEN analysis in three main classes
The objective of this study was to analyze inventory management of health commodities based on ABC-VEN analysis. In fact, conducting ABC or VEN analysis alone has its limitations. ABC analysis ignores the criticality of medicines, and VEN analysis alone also ignores the cost value of pharmaceuticals [ 14 ]. Therefore, to take advantage of the complementary benefits of the two methods, ABC-VEN matrix analysis is required to identify products that need strict control [ 4 , 14 ].
The ABC analysis in this study is comparable to other studies, such as Nang Nwe in Bangkok, Mohamed in Soudan, Deressa, and Nguyen [ 4 , 13 , 18 , 24 ]. Nang found that Category A represented 12% of the ABC analysis, with 78% of the total budget. Category B accounted for 22%, with 16% of expenditure, and Category C accounted for 66% and 6% of the total expenditure. Only a few commodities consume significant funds in this study, and only one item accounts for 23% [ 24 ]. A survey conducted by Vijaya in India in 2017 found that 23.7% of 414 items belonged to Class A and accounted for 70.5% of the total annual expenditure in selected hospitals in Tamil Nadu, India [ 13 , 25 ]. Compared to a study by Mohamed in the Republic of Sudan in 2016, the ABC analysis showed that a small number of items representing 16.98% of Class A accounted for 70.19%, while 61.15% of Class C accounted for only 9.92% of the total fund [ 26 ]. The similarity of these studies to the present study may be related to the fact that the classification of the ABC analysis follows the category and intervals recommended by Pareto. According to Jobira, in the study done in Ethiopia in 2021, the ABC analysis showed that 12.1% of medicines were assigned to Class A, 10.8% to Class B, and 77.7% to Class C. These classes accounted for 80.1%, 10.8%, and 9.1% of total annual medicine expenditures, respectively. Only 10 (1.8%) accounted for 39.8% of the yearly consumption value in class A products.
Pharmaceuticals that account for a significant portion of the budget include antibiotics, massive solutions, medical consumables, and antihypertensives. The results of this study are related to other studies conducted in different areas. The study conducted in the Republic of Soudan to analyze the medicines procured by the National Medicines Supply Fund (NMSF) for 2015–2017, which included 584 imported items, reached very similar results to our study. In that study, 14% of medicines in category A consumed 75% of the budget, 17% of items in the B category cost 15%, and 69% in category C accounted for 10% of total medicine spending. Among Class A products, sodium chloride solution 0.9% w/v 500 ml solution for intravenous infusion ranks first with a 5% share of total expenditure [ 18 ]. This product was among the top twenty products with high cost, ranked fifth with 2.03% of the total cost of items distributed. According to Bochkarev, antibiotics and antihypertensive are among the products that consume a large portion of the pharmaceutical budget [ 27 ]. A study conducted in Greek also revealed that antibacterial products consume a large part of the total pharmaceutical expenditure within Hospitals [ 28 ]. Our study shows that antibiotics are among the products that consume a large budget, and these results are similar to the survey conducted in South Africa. The study in South Africa by Sharma showed that a large portion of the pharmaceutical expenditure was spent on antibiotics. Approximately 7% of the total medicine price was spent on antibiotics [ 29 ]. The first item that consumed a considerable budget in our study, amoxicillin, was among the most commonly procured antibiotics in South Africa [ 29 ]. The three medicines accounted for 23.6% of annual medicines expenditures among the most frequently used products. The concerned products are Amoxicillin 500 mg capsules, cloxacillin 500 mg capsules, and ceftriaxone 1gm injections [ 17 ].
In VEN analysis, category N represents a small number of products and justifies using standards treatment documents and selection following a national essential medicines list. A study conducted in Sudan showed that 45% of all items were in Class N. The VEN analysis also showed that a small number of items (2.34%) in class V accounted for 5.46%, while class N comprised 45.01% of articles and 26.43% of total funds. The medicine class with the highest expenditure was that of general anti-infectives for systemic use (40.37%), which also contributed the most to the increase in total medicine expenditure (48.59%) [ 26 ]. According to Jobira, the VEN classification revealed that 16.9% classified as V and consumed 35.1%, 67.9% grouped as class E, which used 61.3%, 15.2% grouped as N class consumed 3.6% of the total cost [ 17 ]. The finding is in line with the Tumaini HL study conducted in Tanzania, which stated that 17% of items were vital, 68.5% items were essential, and 14.5% were non-essential medicines of total procured drugs.
In a study conducted by Jobira in Ethiopia in 2021, the ABC-VEN analysis showed that 26.6% of all items fell into category I, with the most significant proportion in categories A and V, accounting for 84.7% of annual income medicine expenditure. 49.2% and 24.2% of medicines fell into categories II and III, which accounted for only 13.2% and 2.1% of total medicine spending, respectively [ 17 ]. The ABC-VEN matrix analysis of the medicines inventory conducted by Woldeyohanins 2020 in Ethiopia revealed that 147 items (66.5%) identified as Category I consume 88.99% of the annual medicine expenditure. The remaining 51 (23.07%) and 23 (10.47%) items belong to categories II and III and consume 9.3% and 1.81%, respectively [ 30 ]. The results of the study are similar to those of other studies. According to Sabah, Category I accounted for 16.67% of the medicine population studied, and its expenditure accounted for 71.47% of total expenditure. Category II accounted for 58.69%, and their annual consumption accounted for 27.67% of the total expenditure. The third category, 24.64%, accounted for 0.86% of total expenditure [ 11 ]. Products in the first category include antibiotics, anti-inflammatories, antipyretics, antihypertensives, massive solutions, gastroenteritis remedies, medical consumables, medicines for mental health problems, and antacids. One product, camphor ointment, in the first category is non-essential. Omeprazole was ranked among the top medicines with high expenditure, possibly resulting in prescription habits. A study conducted in Tanzania at Mwananyamala regional hospital found the same results while investigating whether proton pump inhibitors, including omeprazole, are among products with high expenditure [ 10 ].
It is important to indicate that vertically managed program items were not included in the analysis as the related information is normally not available at a decentralized level.
Pharmaceuticals are lifesaving, costly, and may be harmful when they are not adequately managed and used. Considering the role of medicines in healthcare delivery, it is crucial to maintain an adequate stock level to ensure an uninterrupted supply chain. The stock level should be determined based on priority and available resources.
An ABC-VEN analysis revealed that the most used medicines, which occupy a considerable budget at the RMS Ltd., Nyamagabe Branch include antibiotics, massive solutions, and antihypertensive commodities. The findings suggest that high importance should be attached to these commodities, laboratory reagents, and vital products. Furthermore, a regular ABC-VEN analysis of medical supplies use is important to update the local and national essential medicines list and categories of health commodities.
Acknowledgements
The authors gratefully acknowledge the support from the management of the Masters of Health Supply Chain Management program that is offered by the East African Community Regional Center of Excellence for Vaccines, Immunization, and Health Supply Chain Management, College of Medicine and Health Sciences, University of Rwanda. The Center is funded by the German Federal Ministry for Economic Cooperation and Development (BMZ) through KfW Development Bank.
Abbreviations
Author contributions.
All the authors EM, MFM, TB and FN conceptualized the study, and contributed to the study design, data collection, data analysis and manuscript writing. EM collected data, analyzed them, and drafted the manuscript under the guidance of MFM and TB. MFM, TB and FN critically revised the manuscript. All authors read and approved the final manuscript.
No funding was received for this specific study.
Availability of data and materials
Declarations.
The ethical approval with approval notice number CMHS/IRB/321/2021 from the University of Rwanda was issued. The researchers requested the necessary information on VEN classification from the health professionals, and the recipient voluntarily agreed to provide the requested information by signing a consent form.
Not applicable.
The authors declare that they have no competing interests.
Publisher's Note
Springer Nature remains neutral with regard to jurisdictional claims in published maps and institutional affiliations.
This paper is in the following e-collection/theme issue:
Published on 12.4.2024 in Vol 26 (2024)
Application of AI in in Multilevel Pain Assessment Using Facial Images: Systematic Review and Meta-Analysis
Authors of this article:

- Jian Huo 1 * , MSc ;
- Yan Yu 2 * , MMS ;
- Wei Lin 3 , MMS ;
- Anmin Hu 2, 3, 4 , MMS ;
- Chaoran Wu 2 , MD, PhD
1 Boston Intelligent Medical Research Center, Shenzhen United Scheme Technology Company Limited, Boston, MA, United States
2 Department of Anesthesia, Shenzhen People's Hospital, The First Affiliated Hospital of Southern University of Science and Technology, Shenzhen Key Medical Discipline, Shenzhen, China
3 Shenzhen United Scheme Technology Company Limited, Shenzhen, China
4 The Second Clinical Medical College, Jinan University, Shenzhen, China
*these authors contributed equally
Corresponding Author:
Chaoran Wu, MD, PhD
Department of Anesthesia
Shenzhen People's Hospital, The First Affiliated Hospital of Southern University of Science and Technology
Shenzhen Key Medical Discipline
No 1017, Dongmen North Road
Shenzhen, 518020
Phone: 86 18100282848
Email: [email protected]
Background: The continuous monitoring and recording of patients’ pain status is a major problem in current research on postoperative pain management. In the large number of original or review articles focusing on different approaches for pain assessment, many researchers have investigated how computer vision (CV) can help by capturing facial expressions. However, there is a lack of proper comparison of results between studies to identify current research gaps.
Objective: The purpose of this systematic review and meta-analysis was to investigate the diagnostic performance of artificial intelligence models for multilevel pain assessment from facial images.
Methods: The PubMed, Embase, IEEE, Web of Science, and Cochrane Library databases were searched for related publications before September 30, 2023. Studies that used facial images alone to estimate multiple pain values were included in the systematic review. A study quality assessment was conducted using the Quality Assessment of Diagnostic Accuracy Studies, 2nd edition tool. The performance of these studies was assessed by metrics including sensitivity, specificity, log diagnostic odds ratio (LDOR), and area under the curve (AUC). The intermodal variability was assessed and presented by forest plots.
Results: A total of 45 reports were included in the systematic review. The reported test accuracies ranged from 0.27-0.99, and the other metrics, including the mean standard error (MSE), mean absolute error (MAE), intraclass correlation coefficient (ICC), and Pearson correlation coefficient (PCC), ranged from 0.31-4.61, 0.24-2.8, 0.19-0.83, and 0.48-0.92, respectively. In total, 6 studies were included in the meta-analysis. Their combined sensitivity was 98% (95% CI 96%-99%), specificity was 98% (95% CI 97%-99%), LDOR was 7.99 (95% CI 6.73-9.31), and AUC was 0.99 (95% CI 0.99-1). The subgroup analysis showed that the diagnostic performance was acceptable, although imbalanced data were still emphasized as a major problem. All studies had at least one domain with a high risk of bias, and for 20% (9/45) of studies, there were no applicability concerns.
Conclusions: This review summarizes recent evidence in automatic multilevel pain estimation from facial expressions and compared the test accuracy of results in a meta-analysis. Promising performance for pain estimation from facial images was established by current CV algorithms. Weaknesses in current studies were also identified, suggesting that larger databases and metrics evaluating multiclass classification performance could improve future studies.
Trial Registration: PROSPERO CRD42023418181; https://www.crd.york.ac.uk/prospero/display_record.php?RecordID=418181
Introduction
The definition of pain was revised to “an unpleasant sensory and emotional experience associated with, or resembling that associated with, actual or potential tissue damage” in 2020 [ 1 ]. Acute postoperative pain management is important, as pain intensity and duration are critical influencing factors for the transition of acute pain to chronic postsurgical pain [ 2 ]. To avoid the development of chronic pain, guidelines were promoted and discussed to ensure safe and adequate pain relief for patients, and clinicians were recommended to use a validated pain assessment tool to track patients’ responses [ 3 ]. However, these tools, to some extent, depend on communication between physicians and patients, and continuous data cannot be provided [ 4 ]. The continuous assessment and recording of patient pain intensity will not only reduce caregiver burden but also provide data for chronic pain research. Therefore, automatic and accurate pain measurements are necessary.
Researchers have proposed different approaches to measuring pain intensity. Physiological signals, for example, electroencephalography and electromyography, have been used to estimate pain [ 5 - 7 ]. However, it was reported that current pain assessment from physiological signals has difficulties isolating stress and pain with machine learning techniques, as they share conceptual and physiological similarities [ 8 ]. Recent studies have also investigated pain assessment tools for certain patient subgroups. For example, people with deafness or an intellectual disability may not be able to communicate well with nurses, and an objective pain evaluation would be a better option [ 9 , 10 ]. Measuring pain intensity from patient behaviors, such as facial expressions, is also promising for most patients [ 4 ]. As the most comfortable and convenient method, computer vision techniques require no attachments to patients and can monitor multiple participants using 1 device [ 4 ]. However, pain intensity, which is important for pain research, is often not reported.
With the growing trend of assessing pain intensity using artificial intelligence (AI), it is necessary to summarize current publications to determine the strengths and gaps of current studies. Existing research has reviewed machine learning applications for acute postoperative pain prediction, continuous pain detection, and pain intensity estimation [ 10 - 14 ]. Input modalities, including facial recordings and physiological signals such as electroencephalography and electromyography, were also reviewed [ 5 , 8 ]. There have also been studies focusing on deep learning approaches [ 11 ]. AI was applied in children and infant pain evaluation as well [ 15 , 16 ]. However, no study has focused on pain intensity measurement, and no comparison of test accuracy results has been made.
Current AI applications in pain research can be categorized into 3 types: pain assessment, pain prediction and decision support, and pain self-management [ 14 ]. We consider accurate and automatic pain assessment to be the most important area and the foundation of future pain research. In this study, we performed a systematic review and meta-analysis to assess the diagnostic performance of current publications for multilevel pain evaluation.
This study was registered with PROSPERO (International Prospective Register of Systematic Reviews; CRD42023418181) and carried out strictly following the PRISMA (Preferred Reporting Items for Systematic Reviews and Meta-Analyses) guidelines [ 17 ] .
Study Eligibility
Studies that reported AI techniques for multiclass pain intensity classification were eligible. Records including nonhuman or infant participants or 2-class pain detection were excluded. Only studies using facial images of the test participants were accepted. Clinically used pain assessment tools, such as the visual analog scale (VAS) and numerical rating scale (NRS), and other pain intensity indicators, were rejected in the meta-analysis. Textbox 1 presents the eligibility criteria.
Study characteristics and inclusion criteria
- Participants: children and adults aged 12 months or older
- Setting: no restrictions
- Index test: artificial intelligence models that measure pain intensity from facial images
- Reference standard: no restrictions for systematic review; Prkachin and Solomon pain intensity score for meta-analysis
- Study design: no need to specify
Study characteristics and exclusion criteria
- Participants: infants aged 12 months or younger and animal subjects
- Setting: no need to specify
- Index test: studies that use other information such as physiological signals
- Reference standard: other pain evaluation tools, e.g., NRS, VAS, were excluded from meta-analysis
- Study design: reviews
Report characteristics and inclusion criteria
- Year: published between January 1, 2012, and September 30, 2023
- Language: English only
- Publication status: published
- Test accuracy metrics: no restrictions for systematic reviews; studies that reported contingency tables were included for meta-analysis
Report characteristics and exclusion criteria
- Year: no need to specify
- Language: no need to specify
- Publication status: preprints not accepted
- Test accuracy metrics: studies that reported insufficient metrics were excluded from meta-analysis
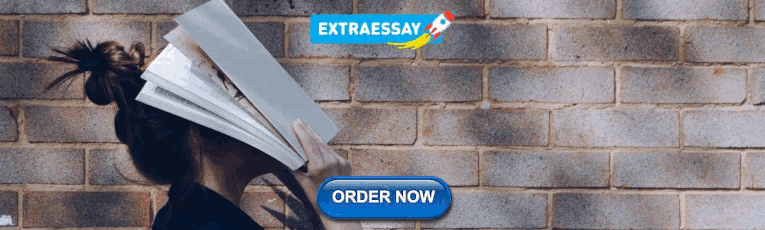
Search Strategy
In this systematic review, databases including PubMed, Embase, IEEE, Web of Science, and the Cochrane Library were searched until December 2022, and no restrictions were applied. Keywords were “artificial intelligence” AND “pain recognition.” Multimedia Appendix 1 shows the detailed search strategy.
Data Extraction
A total of 2 viewers screened titles and abstracts and selected eligible records independently to assess eligibility, and disagreements were solved by discussion with a third collaborator. A consentient data extraction sheet was prespecified and used to summarize study characteristics independently. Table S5 in Multimedia Appendix 1 shows the detailed items and explanations for data extraction. Diagnostic accuracy data were extracted into contingency tables, including true positives, false positives, false negatives, and true negatives. The data were used to calculate the pooled diagnostic performance of the different models. Some studies included multiple models, and these models were considered independent of each other.
Study Quality Assessment
All included studies were independently assessed by 2 viewers using the Quality Assessment of Diagnostic Accuracy Studies 2 (QUADAS-2) tool [ 18 ]. QUADAS-2 assesses bias risk across 4 domains, which are patient selection, index test, reference standard, and flow and timing. The first 3 domains are also assessed for applicability concerns. In the systematic review, a specific extension of QUADAS-2, namely, QUADAS-AI, was used to specify the signaling questions [ 19 ].
Meta-Analysis
Meta-analyses were conducted between different AI models. Models with different algorithms or training data were considered different. To evaluate the performance differences between models, the contingency tables during model validation were extracted. Studies that did not report enough diagnostic accuracy data were excluded from meta-analysis.
Hierarchical summary receiver operating characteristic (SROC) curves were fitted to evaluate the diagnostic performance of AI models. These curves were plotted with 95% CIs and prediction regions around averaged sensitivity, specificity, and area under the curve estimates. Heterogeneity was assessed visually by forest plots. A funnel plot was constructed to evaluate the risk of bias.
Subgroup meta-analyses were conducted to evaluate the performance differences at both the model level and task level, and subgroups were created based on different tasks and the proportion of positive and negative samples.
All statistical analyses and plots were produced using RStudio (version 4.2.2; R Core Team) and the R package meta4diag (version 2.1.1; Guo J and Riebler A) [ 20 ].
Study Selection and Included Study Characteristics
A flow diagram representing the study selection process is shown in ( Figure 1 ). After removing 1039 duplicates, the titles and abstracts of a total of 5653 papers were screened, and the percentage agreement of title or abstract screening was 97%. After screening, 51 full-text reports were assessed for eligibility, among which 45 reports were included in the systematic review [ 21 - 65 ]. The percentage agreement of the full-text review was 87%. In 40 of the included studies, contingency tables could not be made. Meta-analyses were conducted based on 8 AI models extracted from 6 studies. Individual study characteristics included in the systematic review are provided in Tables 1 and 2 . The facial feature extraction method can be categorized into 2 classes: geometrical features (GFs) and deep features (DFs). One typical method of extracting GFs is to calculate the distance between facial landmarks. DFs are usually extracted by convolution operations. A total of 20 studies included temporal information, but most of them (18) extracted temporal information through the 3D convolution of video sequences. Feature transformation was also commonly applied to reduce the time for training or fuse features extracted by different methods before inputting them into the classifier. For classifiers, support vector machines (SVMs) and convolutional neural networks (CNNs) were mostly used. Table 1 presents the model designs of the included studies.

a No temporal features are shown by – symbol, time information extracted from 2 images at different time by +, and deep temporal features extracted through the convolution of video sequences by ++.
b SVM: support vector machine.
c GF: geometric feature.
d GMM: gaussian mixture model.
e TPS: thin plate spline.
f DML: distance metric learning.
g MDML: multiview distance metric learning.
h AAM: active appearance model.
i RVR: relevance vector regressor.
j PSPI: Prkachin and Solomon pain intensity.
k I-FES: individual facial expressiveness score.
l LSTM: long short-term memory.
m HCRF: hidden conditional random field.
n GLMM: generalized linear mixed model.
o VLAD: vector of locally aggregated descriptor.
p SVR: support vector regression.
q MDS: multidimensional scaling.
r ELM: extreme learning machine.
s Labeled to distinguish different architectures of ensembled deep learning models.
t DCNN: deep convolutional neural network.
u GSM: gaussian scale mixture.
v DOML: distance ordering metric learning.
w LIAN: locality and identity aware network.
x BiLSTM: bidirectional long short-term memory.
a UNBC: University of Northern British Columbia-McMaster shoulder pain expression archive database.
b LOSO: leave one subject out cross-validation.
c ICC: intraclass correlation coefficient.
d CT: contingency table.
e AUC: area under the curve.
f MSE: mean standard error.
g PCC: Pearson correlation coefficient.
h RMSE: root mean standard error.
i MAE: mean absolute error.
j ICC: intraclass coefficient.
k CCC: concordance correlation coefficient.
l Reported both external and internal validation results and summarized as intervals.
Table 2 summarizes the characteristics of model training and validation. Most studies used publicly available databases, for example, the University of Northern British Columbia-McMaster shoulder pain expression archive database [ 57 ]. Table S4 in Multimedia Appendix 1 summarizes the public databases. A total of 7 studies used self-prepared databases. Frames from video sequences were the most used test objects, as 37 studies output frame-level pain intensity, while few measure pain intensity from video sequences or photos. It was common that a study redefined pain levels to have fewer classes than ground-truth labels. For model validation, cross-validation and leave-one-subject-out validation were commonly used. Only 3 studies performed external validation. For reporting test accuracies, different evaluation metrics were used, including sensitivity, specificity, mean absolute error (MAE), mean standard error (MSE), Pearson correlation coefficient (PCC), and intraclass coefficient (ICC).
Methodological Quality of Included Studies
Table S2 in Multimedia Appendix 1 presents the study quality summary, as assessed by QUADAS-2. There was a risk of bias in all studies, specifically in terms of patient selection, caused by 2 issues. First, the training data are highly imbalanced, and any method to adjust the data distribution may introduce bias. Next, the QUADAS-AI correspondence letter [ 19 ] specifies that preprocessing of images that changes the image size or resolution may introduce bias. However, the applicability concern is low, as the images properly represent the feeling of pain. Studies that used cross-fold validation or leave-one-out cross-validation were considered to have a low risk of bias. Although the Prkachin and Solomon pain intensity (PSPI) score was used by most of the studies, its ability to represent individual pain levels was not clinically validated; as such, the risk of bias and applicability concerns were considered high when the PSPI score was used as the index test. As an advantage of computer vision techniques, the time interval between the index tests was short and was assessed as having a low risk of bias. Risk proportions are shown in Figure 2 . For all 315 entries, 39% (124) were assessed as high-risk. In total, 5 studies had the lowest risk of bias, with 6 domains assessed as low risk [ 26 , 27 , 31 , 32 , 59 ].

Pooled Performance of Included Models
In 6 studies included in the meta-analysis, there were 8 different models. The characteristics of these models are summarized in Table S1 in Multimedia Appendix 2 [ 23 , 24 , 26 , 32 , 41 , 57 ]. Classification of PSPI scores greater than 0, 2, 3, 6, and 9 was selected and considered as different tasks to create contingency tables. The test performance is shown in Figure 3 as hierarchical SROC curves; 27 contingency tables were extracted from 8 models. The sensitivity, specificity, and LDOR were calculated, and the combined sensitivity was 98% (95% CI 96%-99%), the specificity was 98% (95% CI 97%-99%), the LDOR was 7.99 (95% CI 6.73-9.31) and the AUC was 0.99 (95% CI 0.99-1).

Subgroup Analysis
In this study, subgroup analysis was conducted to investigate the performance differences within models. A total of 8 models were separated and summarized as a forest plot in Multimedia Appendix 3 [ 23 , 24 , 26 , 32 , 41 , 57 ]. For model 1, the pooled sensitivity, specificity, and LDOR were 95% (95% CI 86%-99%), 99% (95% CI 98%-100%), and 8.38 (95% CI 6.09-11.19), respectively. For model 2, the pooled sensitivity, specificity, and LDOR were 94% (95% CI 84%-99%), 95% (95% CI 88%-99%), and 6.23 (95% CI 3.52-9.04), respectively. For model 3, the pooled sensitivity, specificity, and LDOR were 100% (95% CI 99%-100%), 100% (95% CI 99%-100%), and 11.55% (95% CI 8.82-14.43), respectively. For model 4, the pooled sensitivity, specificity, and LDOR were 83% (95% CI 43%-99%), 94% (95% CI 79%-99%), and 5.14 (95% CI 0.93-9.31), respectively. For model 5, the pooled sensitivity, specificity, and LDOR were 92% (95% CI 68%-99%), 94% (95% CI 78%-99%), and 6.12 (95% CI 1.82-10.16), respectively. For model 6, the pooled sensitivity, specificity, and LDOR were 94% (95% CI 74%-100%), 94% (95% CI 78%-99%), and 6.59 (95% CI 2.21-11.13), respectively. For model 7, the pooled sensitivity, specificity, and LDOR were 98% (95% CI 90%-100%), 97% (95% CI 87%-100%), and 8.31 (95% CI 4.3-12.29), respectively. For model 8, the pooled sensitivity, specificity, and LDOR were 98% (95% CI 93%-100%), 97% (95% CI 88%-100%), and 8.65 (95% CI 4.84-12.67), respectively.
Heterogeneity Analysis
The meta-analysis results indicated that AI models are applicable for estimating pain intensity from facial images. However, extreme heterogeneity existed within the models except for models 3 and 5, which were proposed by Rathee and Ganotra [ 24 ] and Semwal and Londhe [ 32 ]. A funnel plot is presented in Figure 4 . A high risk of bias was observed.

Pain management has long been a critical problem in clinical practice, and the use of AI may be a solution. For acute pain management, automatic measurement of pain can reduce the burden on caregivers and provide timely warnings. For chronic pain management, as specified by Glare et al [ 2 ], further research is needed, and measurements of pain presence, intensity, and quality are one of the issues to be solved for chronic pain studies. Computer vision could improve pain monitoring through real-time detection for clinical use and data recording for prospective pain studies. To our knowledge, this is the first meta-analysis dedicated to AI performance in multilevel pain level classification.
In this study, one model’s performance at specific pain levels was described by stacking multiple classes into one to make each task a binary classification problem. After careful selection in both the medical and engineering databases, we observed promising results of AI in evaluating multilevel pain intensity through facial images, with high sensitivity (98%), specificity (98%), LDOR (7.99), and AUC (0.99). It is reasonable to believe that AI can accurately evaluate pain intensity from facial images. Moreover, the study quality and risk of bias were evaluated using an adapted QUADAS-2 assessment tool, which is a strength of this study.
To investigate the source of heterogeneity, it was assumed that a well-designed model should have familiar size effects regarding different levels, and a subgroup meta-analysis was conducted. The funnel and forest plots exhibited extreme heterogeneity. The model’s performance at specific pain levels was described and summarized by a forest plot. Within-model heterogeneity was observed in Multimedia Appendix 3 [ 23 , 24 , 26 , 32 , 41 , 57 ] except for 2 models. Models 3 and 5 were different in many aspects, including their algorithms and validation methods, but were both trained with a relatively small data set, and the proportion of positive and negative classes was relatively close to 1. Because training with imbalanced data is a critical problem in computer vision studies [ 66 ], for example, in the University of Northern British Columbia-McMaster pain data set, fewer than 10 frames out of 48,398 had a PSPI score greater than 13. Here, we emphasized that imbalanced data sets are one major cause of heterogeneity, resulting in the poorer performance of AI algorithms.
We tentatively propose a method to minimize the effect of training with imbalanced data by stacking multiple classes into one class, which is already presented in studies included in the systematic review [ 26 , 32 , 42 , 57 ]. Common methods to minimize bias include resampling and data augmentation [ 66 ]. This proposed method is used in the meta-analysis to compare the test results of different studies as well. The stacking method is available when classes are only different in intensity. A disadvantage of combined classes is that the model would be insufficient in clinical practice when the number of classes is low. Commonly used pain evaluation tools, such as VAS, have 10 discrete levels. It is recommended that future studies set the number of pain levels to be at least 10 for model training.
This study is limited for several reasons. First, insufficient data were included because different performance metrics (mean standard error and mean average error) were used in most studies, which could not be summarized into a contingency table. To create a contingency table that can be included in a meta-analysis, the study should report the following: the number of objects used in each pain class for model validation, and the accuracy, sensitivity, specificity, and F 1 -score for each pain class. This table cannot be created if a study reports the MAE, PCC, and other commonly used metrics in AI development. Second, a small study effect was observed in the funnel plot, and the heterogeneity could not be minimized. Another limitation is that the PSPI score is not clinically validated and is not the only tool that assesses pain from facial expressions. There are other clinically validated pain intensity assessment methods, such as the Faces Pain Scale-revised, Wong-Baker Faces Pain Rating Scale, and Oucher Scale [ 3 ]. More databases could be created based on the above-mentioned tools. Finally, AI-assisted pain assessments were supposed to cover larger populations, including incommunicable patients, for example, patients with dementia or patients with masked faces. However, only 1 study considered patients with dementia, which was also caused by limited databases [ 50 ].
AI is a promising tool that can help in pain research in the future. In this systematic review and meta-analysis, one approach using computer vision was investigated to measure pain intensity from facial images. Despite some risk of bias and applicability concerns, CV models can achieve excellent test accuracy. Finally, more CV studies in pain estimation, reporting accuracy in contingency tables, and more pain databases are encouraged for future studies. Specifically, the creation of a balanced public database that contains not only healthy but also nonhealthy participants should be prioritized. The recording process would be better in a clinical environment. Then, it is recommended that researchers report the validation results in terms of accuracy, sensitivity, specificity, or contingency tables, as well as the number of objects for each pain class, for the inclusion of a meta-analysis.
Acknowledgments
WL, AH, and CW contributed to the literature search and data extraction. JH and YY wrote the first draft of the manuscript. All authors contributed to the conception and design of the study, the risk of bias evaluation, data analysis and interpretation, and contributed to and approved the final version of the manuscript.
Data Availability
The data sets generated during and analyzed during this study are available in the Figshare repository [ 67 ].
Conflicts of Interest
None declared.
PRISMA checklist, risk of bias summary, search strategy, database summary and reported items and explanations.
Study performance summary.
Forest plot presenting pooled performance of subgroups in meta-analysis.
- Raja SN, Carr DB, Cohen M, Finnerup NB, Flor H, Gibson S, et al. The revised International Association for the Study of Pain definition of pain: concepts, challenges, and compromises. Pain. 2020;161(9):1976-1982. [ FREE Full text ] [ CrossRef ] [ Medline ]
- Glare P, Aubrey KR, Myles PS. Transition from acute to chronic pain after surgery. Lancet. 2019;393(10180):1537-1546. [ CrossRef ] [ Medline ]
- Chou R, Gordon DB, de Leon-Casasola OA, Rosenberg JM, Bickler S, Brennan T, et al. Management of postoperative pain: a clinical practice guideline from the American Pain Society, the American Society of Regional Anesthesia and Pain Medicine, and the American Society of Anesthesiologists' Committee on Regional Anesthesia, Executive Committee, and Administrative Council. J Pain. 2016;17(2):131-157. [ FREE Full text ] [ CrossRef ] [ Medline ]
- Hassan T, Seus D, Wollenberg J, Weitz K, Kunz M, Lautenbacher S, et al. Automatic detection of pain from facial expressions: a survey. IEEE Trans Pattern Anal Mach Intell. 2021;43(6):1815-1831. [ CrossRef ] [ Medline ]
- Mussigmann T, Bardel B, Lefaucheur JP. Resting-State Electroencephalography (EEG) biomarkers of chronic neuropathic pain. A systematic review. Neuroimage. 2022;258:119351. [ FREE Full text ] [ CrossRef ] [ Medline ]
- Moscato S, Cortelli P, Chiari L. Physiological responses to pain in cancer patients: a systematic review. Comput Methods Programs Biomed. 2022;217:106682. [ FREE Full text ] [ CrossRef ] [ Medline ]
- Thiam P, Hihn H, Braun DA, Kestler HA, Schwenker F. Multi-modal pain intensity assessment based on physiological signals: a deep learning perspective. Front Physiol. 2021;12:720464. [ FREE Full text ] [ CrossRef ] [ Medline ]
- Rojas RF, Brown N, Waddington G, Goecke R. A systematic review of neurophysiological sensing for the assessment of acute pain. NPJ Digit Med. 2023;6(1):76. [ FREE Full text ] [ CrossRef ] [ Medline ]
- Mansutti I, Tomé-Pires C, Chiappinotto S, Palese A. Facilitating pain assessment and communication in people with deafness: a systematic review. BMC Public Health. 2023;23(1):1594. [ FREE Full text ] [ CrossRef ] [ Medline ]
- El-Tallawy SN, Ahmed RS, Nagiub MS. Pain management in the most vulnerable intellectual disability: a review. Pain Ther. 2023;12(4):939-961. [ FREE Full text ] [ CrossRef ] [ Medline ]
- Gkikas S, Tsiknakis M. Automatic assessment of pain based on deep learning methods: a systematic review. Comput Methods Programs Biomed. 2023;231:107365. [ FREE Full text ] [ CrossRef ] [ Medline ]
- Borna S, Haider CR, Maita KC, Torres RA, Avila FR, Garcia JP, et al. A review of voice-based pain detection in adults using artificial intelligence. Bioengineering (Basel). 2023;10(4):500. [ FREE Full text ] [ CrossRef ] [ Medline ]
- De Sario GD, Haider CR, Maita KC, Torres-Guzman RA, Emam OS, Avila FR, et al. Using AI to detect pain through facial expressions: a review. Bioengineering (Basel). 2023;10(5):548. [ FREE Full text ] [ CrossRef ] [ Medline ]
- Zhang M, Zhu L, Lin SY, Herr K, Chi CL, Demir I, et al. Using artificial intelligence to improve pain assessment and pain management: a scoping review. J Am Med Inform Assoc. 2023;30(3):570-587. [ FREE Full text ] [ CrossRef ] [ Medline ]
- Hughes JD, Chivers P, Hoti K. The clinical suitability of an artificial intelligence-enabled pain assessment tool for use in infants: feasibility and usability evaluation study. J Med Internet Res. 2023;25:e41992. [ FREE Full text ] [ CrossRef ] [ Medline ]
- Fang J, Wu W, Liu J, Zhang S. Deep learning-guided postoperative pain assessment in children. Pain. 2023;164(9):2029-2035. [ FREE Full text ] [ CrossRef ] [ Medline ]
- Page MJ, McKenzie JE, Bossuyt PM, Boutron I, Hoffmann TC, Mulrow CD, et al. The PRISMA 2020 statement: an updated guideline for reporting systematic reviews. BMJ. 2021;372:n71. [ FREE Full text ] [ CrossRef ] [ Medline ]
- Whiting PF, Rutjes AWS, Westwood ME, Mallett S, Deeks JJ, Reitsma JB, et al. QUADAS-2: a revised tool for the quality assessment of diagnostic accuracy studies. Ann Intern Med. 2011;155(8):529-536. [ FREE Full text ] [ CrossRef ] [ Medline ]
- Sounderajah V, Ashrafian H, Rose S, Shah NH, Ghassemi M, Golub R, et al. A quality assessment tool for artificial intelligence-centered diagnostic test accuracy studies: QUADAS-AI. Nat Med. 2021;27(10):1663-1665. [ FREE Full text ] [ CrossRef ] [ Medline ]
- Guo J, Riebler A. meta4diag: Bayesian bivariate meta-analysis of diagnostic test studies for routine practice. J Stat Soft. 2018;83(1):1-31. [ CrossRef ]
- Hammal Z, Cohn JF. Automatic detection of pain intensity. Proc ACM Int Conf Multimodal Interact. 2012;2012:47-52. [ FREE Full text ] [ CrossRef ] [ Medline ]
- Adibuzzaman M, Ostberg C, Ahamed S, Povinelli R, Sindhu B, Love R, et al. Assessment of pain using facial pictures taken with a smartphone. 2015. Presented at: 2015 IEEE 39th Annual Computer Software and Applications Conference; July 01-05, 2015;726-731; Taichung, Taiwan. [ CrossRef ]
- Majumder A, Dutta S, Behera L, Subramanian VK. Shoulder pain intensity recognition using Gaussian mixture models. 2015. Presented at: 2015 IEEE International WIE Conference on Electrical and Computer Engineering (WIECON-ECE); December 19-20, 2015;130-134; Dhaka, Bangladesh. [ CrossRef ]
- Rathee N, Ganotra D. A novel approach for pain intensity detection based on facial feature deformations. J Vis Commun Image Represent. 2015;33:247-254. [ CrossRef ]
- Sikka K, Ahmed AA, Diaz D, Goodwin MS, Craig KD, Bartlett MS, et al. Automated assessment of children's postoperative pain using computer vision. Pediatrics. 2015;136(1):e124-e131. [ FREE Full text ] [ CrossRef ] [ Medline ]
- Rathee N, Ganotra D. Multiview distance metric learning on facial feature descriptors for automatic pain intensity detection. Comput Vis Image Und. 2016;147:77-86. [ CrossRef ]
- Zhou J, Hong X, Su F, Zhao G. Recurrent convolutional neural network regression for continuous pain intensity estimation in video. 2016. Presented at: 2016 IEEE Conference on Computer Vision and Pattern Recognition Workshops (CVPRW); June 26-July 01, 2016; Las Vegas, NV. [ CrossRef ]
- Egede J, Valstar M, Martinez B. Fusing deep learned and hand-crafted features of appearance, shape, and dynamics for automatic pain estimation. 2017. Presented at: 2017 12th IEEE International Conference on Automatic Face & Gesture Recognition (FG 2017); May 30-June 03, 2017;689-696; Washington, DC. [ CrossRef ]
- Martinez DL, Rudovic O, Picard R. Personalized automatic estimation of self-reported pain intensity from facial expressions. 2017. Presented at: 2017 IEEE Conference on Computer Vision and Pattern Recognition Workshops (CVPRW); July 21-26, 2017;2318-2327; Honolulu, HI. [ CrossRef ]
- Bourou D, Pampouchidou A, Tsiknakis M, Marias K, Simos P. Video-based pain level assessment: feature selection and inter-subject variability modeling. 2018. Presented at: 2018 41st International Conference on Telecommunications and Signal Processing (TSP); July 04-06, 2018;1-6; Athens, Greece. [ CrossRef ]
- Haque MA, Bautista RB, Noroozi F, Kulkarni K, Laursen C, Irani R. Deep multimodal pain recognition: a database and comparison of spatio-temporal visual modalities. 2018. Presented at: 2018 13th IEEE International Conference on Automatic Face & Gesture Recognition (FG 2018); May 15-19, 2018;250-257; Xi'an, China. [ CrossRef ]
- Semwal A, Londhe ND. Automated pain severity detection using convolutional neural network. 2018. Presented at: 2018 International Conference on Computational Techniques, Electronics and Mechanical Systems (CTEMS); December 21-22, 2018;66-70; Belgaum, India. [ CrossRef ]
- Tavakolian M, Hadid A. Deep binary representation of facial expressions: a novel framework for automatic pain intensity recognition. 2018. Presented at: 2018 25th IEEE International Conference on Image Processing (ICIP); October 07-10, 2018;1952-1956; Athens, Greece. [ CrossRef ]
- Tavakolian M, Hadid A. Deep spatiotemporal representation of the face for automatic pain intensity estimation. 2018. Presented at: 2018 24th International Conference on Pattern Recognition (ICPR); August 20-24, 2018;350-354; Beijing, China. [ CrossRef ]
- Wang J, Sun H. Pain intensity estimation using deep spatiotemporal and handcrafted features. IEICE Trans Inf & Syst. 2018;E101.D(6):1572-1580. [ CrossRef ]
- Bargshady G, Soar J, Zhou X, Deo RC, Whittaker F, Wang H. A joint deep neural network model for pain recognition from face. 2019. Presented at: 2019 IEEE 4th International Conference on Computer and Communication Systems (ICCCS); February 23-25, 2019;52-56; Singapore. [ CrossRef ]
- Casti P, Mencattini A, Comes MC, Callari G, Di Giuseppe D, Natoli S, et al. Calibration of vision-based measurement of pain intensity with multiple expert observers. IEEE Trans Instrum Meas. 2019;68(7):2442-2450. [ CrossRef ]
- Lee JS, Wang CW. Facial pain intensity estimation for ICU patient with partial occlusion coming from treatment. 2019. Presented at: BIBE 2019; The Third International Conference on Biological Information and Biomedical Engineering; June 20-22, 2019;1-4; Hangzhou, China.
- Saha AK, Ahsan GMT, Gani MO, Ahamed SI. Personalized pain study platform using evidence-based continuous learning tool. 2019. Presented at: 2019 IEEE 43rd Annual Computer Software and Applications Conference (COMPSAC); July 15-19, 2019;490-495; Milwaukee, WI. [ CrossRef ]
- Tavakolian M, Hadid A. A spatiotemporal convolutional neural network for automatic pain intensity estimation from facial dynamics. Int J Comput Vis. 2019;127(10):1413-1425. [ FREE Full text ] [ CrossRef ]
- Bargshady G, Zhou X, Deo RC, Soar J, Whittaker F, Wang H. Ensemble neural network approach detecting pain intensity from facial expressions. Artif Intell Med. 2020;109:101954. [ CrossRef ] [ Medline ]
- Bargshady G, Zhou X, Deo RC, Soar J, Whittaker F, Wang H. Enhanced deep learning algorithm development to detect pain intensity from facial expression images. Expert Syst Appl. 2020;149:113305. [ CrossRef ]
- Dragomir MC, Florea C, Pupezescu V. Automatic subject independent pain intensity estimation using a deep learning approach. 2020. Presented at: 2020 International Conference on e-Health and Bioengineering (EHB); October 29-30, 2020;1-4; Iasi, Romania. [ CrossRef ]
- Huang D, Xia Z, Mwesigye J, Feng X. Pain-attentive network: a deep spatio-temporal attention model for pain estimation. Multimed Tools Appl. 2020;79(37-38):28329-28354. [ CrossRef ]
- Mallol-Ragolta A, Liu S, Cummins N, Schuller B. A curriculum learning approach for pain intensity recognition from facial expressions. 2020. Presented at: 2020 15th IEEE International Conference on Automatic Face and Gesture Recognition (FG 2020); November 16-20, 2020;829-833; Buenos Aires, Argentina. [ CrossRef ]
- Peng X, Huang D, Zhang H. Pain intensity recognition via multi‐scale deep network. IET Image Process. 2020;14(8):1645-1652. [ FREE Full text ] [ CrossRef ]
- Tavakolian M, Lopez MB, Liu L. Self-supervised pain intensity estimation from facial videos via statistical spatiotemporal distillation. Pattern Recognit Lett. 2020;140:26-33. [ CrossRef ]
- Xu X, de Sa VR. Exploring multidimensional measurements for pain evaluation using facial action units. 2020. Presented at: 2020 15th IEEE International Conference on Automatic Face and Gesture Recognition (FG 2020); November 16-20, 2020;786-792; Buenos Aires, Argentina. [ CrossRef ]
- Pikulkaew K, Boonchieng W, Boonchieng E, Chouvatut V. 2D facial expression and movement of motion for pain identification with deep learning methods. IEEE Access. 2021;9:109903-109914. [ CrossRef ]
- Rezaei S, Moturu A, Zhao S, Prkachin KM, Hadjistavropoulos T, Taati B. Unobtrusive pain monitoring in older adults with dementia using pairwise and contrastive training. IEEE J Biomed Health Inform. 2021;25(5):1450-1462. [ CrossRef ] [ Medline ]
- Semwal A, Londhe ND. S-PANET: a shallow convolutional neural network for pain severity assessment in uncontrolled environment. 2021. Presented at: 2021 IEEE 11th Annual Computing and Communication Workshop and Conference (CCWC); January 27-30, 2021;0800-0806; Las Vegas, NV. [ CrossRef ]
- Semwal A, Londhe ND. ECCNet: an ensemble of compact convolution neural network for pain severity assessment from face images. 2021. Presented at: 2021 11th International Conference on Cloud Computing, Data Science & Engineering (Confluence); January 28-29, 2021;761-766; Noida, India. [ CrossRef ]
- Szczapa B, Daoudi M, Berretti S, Pala P, Del Bimbo A, Hammal Z. Automatic estimation of self-reported pain by interpretable representations of motion dynamics. 2021. Presented at: 2020 25th International Conference on Pattern Recognition (ICPR); January 10-15, 2021;2544-2550; Milan, Italy. [ CrossRef ]
- Ting J, Yang YC, Fu LC, Tsai CL, Huang CH. Distance ordering: a deep supervised metric learning for pain intensity estimation. 2021. Presented at: 2021 20th IEEE International Conference on Machine Learning and Applications (ICMLA); December 13-16, 2021;1083-1088; Pasadena, CA. [ CrossRef ]
- Xin X, Li X, Yang S, Lin X, Zheng X. Pain expression assessment based on a locality and identity aware network. IET Image Process. 2021;15(12):2948-2958. [ FREE Full text ] [ CrossRef ]
- Alghamdi T, Alaghband G. Facial expressions based automatic pain assessment system. Appl Sci. 2022;12(13):6423. [ FREE Full text ] [ CrossRef ]
- Barua PD, Baygin N, Dogan S, Baygin M, Arunkumar N, Fujita H, et al. Automated detection of pain levels using deep feature extraction from shutter blinds-based dynamic-sized horizontal patches with facial images. Sci Rep. 2022;12(1):17297. [ FREE Full text ] [ CrossRef ] [ Medline ]
- Fontaine D, Vielzeuf V, Genestier P, Limeux P, Santucci-Sivilotto S, Mory E, et al. Artificial intelligence to evaluate postoperative pain based on facial expression recognition. Eur J Pain. 2022;26(6):1282-1291. [ CrossRef ] [ Medline ]
- Hosseini E, Fang R, Zhang R, Chuah CN, Orooji M, Rafatirad S, et al. Convolution neural network for pain intensity assessment from facial expression. 2022. Presented at: 2022 44th Annual International Conference of the IEEE Engineering in Medicine & Biology Society (EMBC); July 11-15, 2022;2697-2702; Glasgow, Scotland. [ CrossRef ]
- Huang Y, Qing L, Xu S, Wang L, Peng Y. HybNet: a hybrid network structure for pain intensity estimation. Vis Comput. 2021;38(3):871-882. [ CrossRef ]
- Islamadina R, Saddami K, Oktiana M, Abidin TF, Muharar R, Arnia F. Performance of deep learning benchmark models on thermal imagery of pain through facial expressions. 2022. Presented at: 2022 IEEE International Conference on Communication, Networks and Satellite (COMNETSAT); November 03-05, 2022;374-379; Solo, Indonesia. [ CrossRef ]
- Swetha L, Praiscia A, Juliet S. Pain assessment model using facial recognition. 2022. Presented at: 2022 6th International Conference on Intelligent Computing and Control Systems (ICICCS); May 25-27, 2022;1-5; Madurai, India. [ CrossRef ]
- Wu CL, Liu SF, Yu TL, Shih SJ, Chang CH, Mao SFY, et al. Deep learning-based pain classifier based on the facial expression in critically ill patients. Front Med (Lausanne). 2022;9:851690. [ FREE Full text ] [ CrossRef ] [ Medline ]
- Ismail L, Waseem MD. Towards a deep learning pain-level detection deployment at UAE for patient-centric-pain management and diagnosis support: framework and performance evaluation. Procedia Comput Sci. 2023;220:339-347. [ FREE Full text ] [ CrossRef ] [ Medline ]
- Vu MT, Beurton-Aimar M. Learning to focus on region-of-interests for pain intensity estimation. 2023. Presented at: 2023 IEEE 17th International Conference on Automatic Face and Gesture Recognition (FG); January 05-08, 2023;1-6; Waikoloa Beach, HI. [ CrossRef ]
- Kaur H, Pannu HS, Malhi AK. A systematic review on imbalanced data challenges in machine learning: applications and solutions. ACM Comput Surv. 2019;52(4):1-36. [ CrossRef ]
- Data for meta-analysis of pain assessment from facial images. Figshare. 2023. URL: https://figshare.com/articles/dataset/Data_for_Meta-Analysis_of_Pain_Assessment_from_Facial_Images/24531466/1 [accessed 2024-03-22]
Abbreviations
Edited by A Mavragani; submitted 26.07.23; peer-reviewed by M Arab-Zozani, M Zhang; comments to author 18.09.23; revised version received 08.10.23; accepted 28.02.24; published 12.04.24.
©Jian Huo, Yan Yu, Wei Lin, Anmin Hu, Chaoran Wu. Originally published in the Journal of Medical Internet Research (https://www.jmir.org), 12.04.2024.
This is an open-access article distributed under the terms of the Creative Commons Attribution License (https://creativecommons.org/licenses/by/4.0/), which permits unrestricted use, distribution, and reproduction in any medium, provided the original work, first published in the Journal of Medical Internet Research, is properly cited. The complete bibliographic information, a link to the original publication on https://www.jmir.org/, as well as this copyright and license information must be included.
AI Index Report
The AI Index Report tracks, collates, distills, and visualizes data related to artificial intelligence. Our mission is to provide unbiased, rigorously vetted, broadly sourced data in order for policymakers, researchers, executives, journalists, and the general public to develop a more thorough and nuanced understanding of the complex field of AI. The report aims to be the world’s most credible and authoritative source for data and insights about AI.
Subscribe to receive the 2024 report in your inbox!
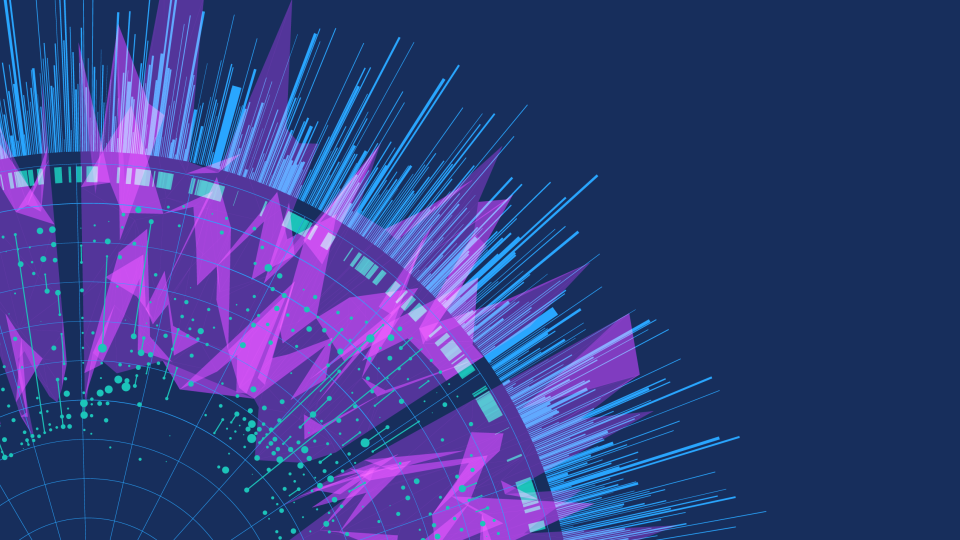
Coming Soon: 2024 AI Index Report!
The 2024 AI Index Report will be out April 15! Sign up for our mailing list to receive it in your inbox.
Steering Committee Co-Directors
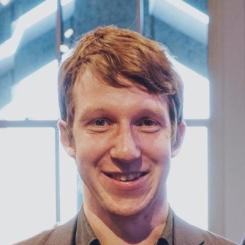
Ray Perrault
Steering committee members.
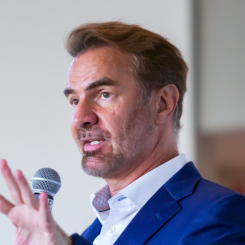
Erik Brynjolfsson
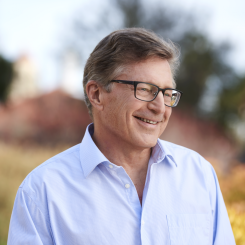
John Etchemendy
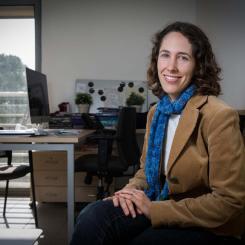
Katrina Ligett
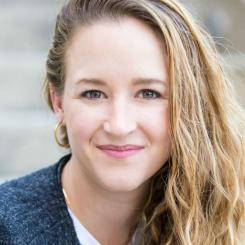
Terah Lyons
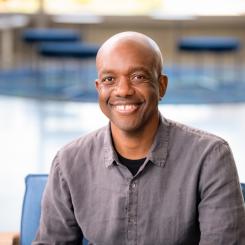
James Manyika
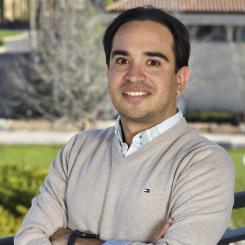
Juan Carlos Niebles
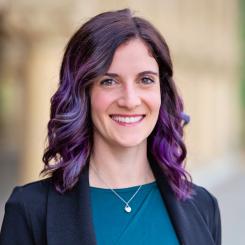
Vanessa Parli
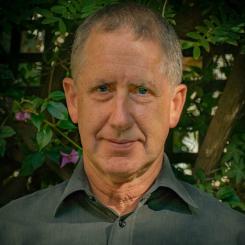
Yoav Shoham
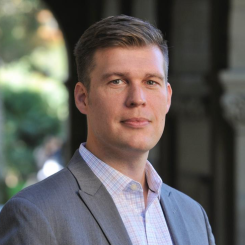
Russell Wald
Staff members.
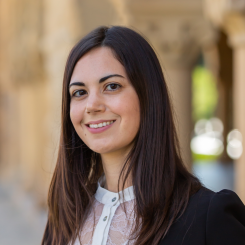
Loredana Fattorini
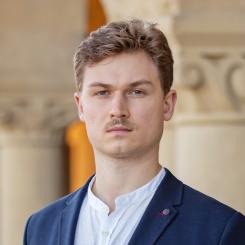
Nestor Maslej
Letter from the co-directors.
AI has moved into its era of deployment; throughout 2022 and the beginning of 2023, new large-scale AI models have been released every month. These models, such as ChatGPT, Stable Diffusion, Whisper, and DALL-E 2, are capable of an increasingly broad range of tasks, from text manipulation and analysis, to image generation, to unprecedentedly good speech recognition. These systems demonstrate capabilities in question answering, and the generation of text, image, and code unimagined a decade ago, and they outperform the state of the art on many benchmarks, old and new. However, they are prone to hallucination, routinely biased, and can be tricked into serving nefarious aims, highlighting the complicated ethical challenges associated with their deployment.
Although 2022 was the first year in a decade where private AI investment decreased, AI is still a topic of great interest to policymakers, industry leaders, researchers, and the public. Policymakers are talking about AI more than ever before. Industry leaders that have integrated AI into their businesses are seeing tangible cost and revenue benefits. The number of AI publications and collaborations continues to increase. And the public is forming sharper opinions about AI and which elements they like or dislike.
AI will continue to improve and, as such, become a greater part of all our lives. Given the increased presence of this technology and its potential for massive disruption, we should all begin thinking more critically about how exactly we want AI to be developed and deployed. We should also ask questions about who is deploying it—as our analysis shows, AI is increasingly defined by the actions of a small set of private sector actors, rather than a broader range of societal actors. This year’s AI Index paints a picture of where we are so far with AI, in order to highlight what might await us in the future.
- Jack Clark and Ray Perrault
Our Supporting Partners
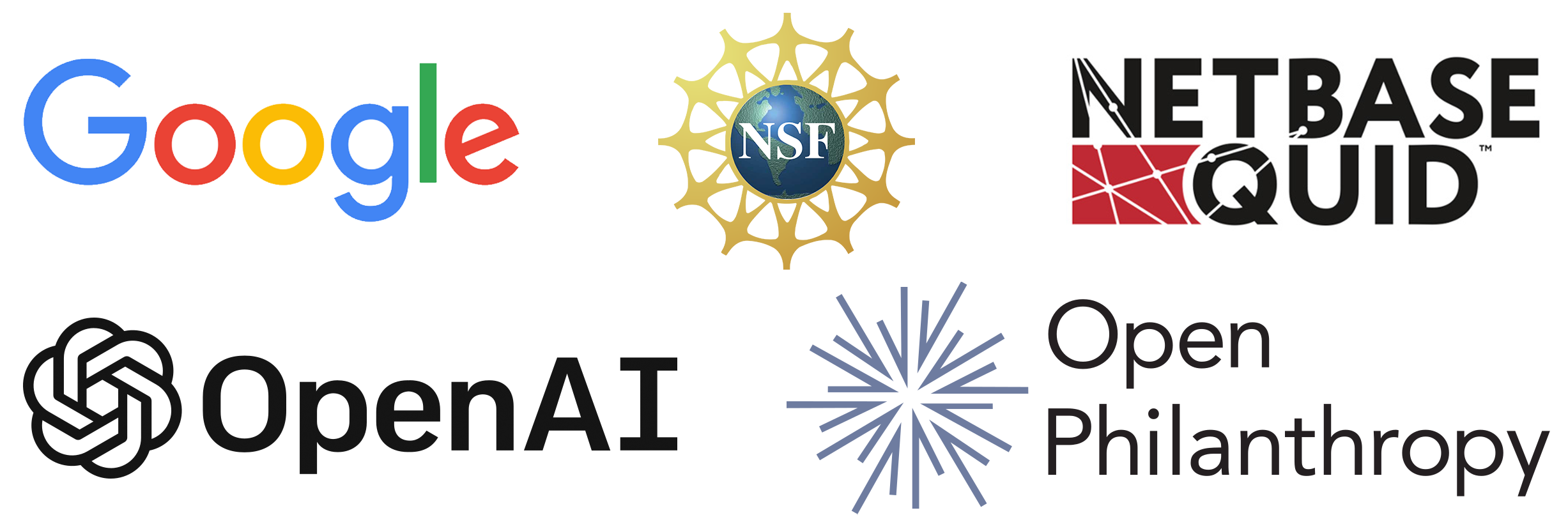
Analytics & Research Partners

Stay up to date on the AI Index by subscribing to the Stanford HAI newsletter.
Creative Australia's report into the music festival sector shows how many of the country's big events are struggling
More than one-third of Australian music festivals are losing money as they face skyrocketing operational costs and dwindling younger audiences, according to a new report from Creative Australia.
Billed as the first widespread report of its kind, Soundcheck: Insights into Australia's music festival sector delves into the cultural, social and economic impacts of Australian music festivals, and paints a clear picture of the landscape as it stood in the 2022-23 financial year.
Spanning the 535 music festivals held nationwide in that time — that's almost 1.5 festivals per day — the 116-page report reflects the scope, scale and diversity of the Australian music festival landscape.
Given the highly publicised recent struggles festivals have faced, it's timely research that looks to help Australian audiences and funding bodies understand the challenges these events face.
How much money do music festivals make?
Just 56 per cent of music festivals reported a profit in the 2022-23 financial year, with more than one third of festivals reporting a deficit and eight per cent breaking even.
The median average cost to stage a music festival is $3.3 million, and those events that do make a profit pull in a median average of $731,569 per event.
When looking at the mean average of the same data, though, that figure skyrockets to $2.6 million — confirming that some festivals are in a much better financial position and stand to gain far more than some of their contemporaries.
For instance, the highest profit for a festival surveyed for this data was $47.4 million, while the smallest profit was just $20,000.
What are the biggest challenges festivals face?
Rising operational costs had the most severe impact on almost half of festival organisers (47 per cent) — overheads like artist fees, production, suppliers, freight, transportation and insurance.
Other major barriers included a lack of funding and grants, as well as extreme weather events. Almost one third of festivals said skyrocketing insurance costs were a major challenge.
Australian live music venues' public liability insurance policies increased 10-fold in the past financial year, climbing from $20,000 per year to as much as $120,000.
One festival organiser noted that necessary event cancellation insurance costs had "pretty much doubled" since the COVID-19 pandemic.
"The excess used to be like a standard commercial policy, which is like $4,000 or $5,000. Our excess for this year is $250,000."
Another organiser said navigating insurance paperwork had become an "absolute minefield" after making the tough call to cancel their festival.
"We had to wait until the morning of the show to make the final determination to cancel, otherwise there's the possibility that the insurance company could have said we could have worked out other alternatives.
"You're left with this real balancing act of, do you let your patrons know … who may have been booking accommodation, may have been getting drivers, getting babysitters, outlaying some money to attend the festival?"
The rising costs of securing police and security was another sore point. More than a quarter of festivals noted the challenges of navigating police and security requirements, and the difficulties of dealing with different government and council regulations across different states and jurisdictions.
"There's not enough consistency," said one logistics/operations worker from New South Wales.
"Whether you do an event in the metro area, or you do an event in Newcastle, or you do an event down the South Coast, or whatever the case may be, all these authorities have different expectations in regards to what they want from security and from the event. That makes it hard because some of the implications are more costs for the event promoter."
By contrast, most festivals found health, medical and liquor licensing requirements were the least challenging regulatory challenge, with around seven per cent reporting these elements had an impact.
Ongoing festival cancellations have created a vicious cycle where the more events pull the plug or lose headliners last-minute, the more hesitation it creates in the wider market — from both the industry and from punters holding off on purchasing tickets.
Who's buying festival tickets?
While music festival revenue comes from various avenues — from corporate sponsorship to hospitality services to merchandise and more — it's ticket sales that determine the ultimate feasibility of a music festival.
There is some good news on that front, with average ticket sales in 2022-23 higher than pre-COVID levels.
The average festival sold 8,116 tickets in 2018-19, which ballooned to 9,506 for 2022-23, indicating that the industry is slowly recovering from the decimating impacts of the COVID-19 pandemic.
The research suggests that young people are no longer the main consumer of music festivals, nor are they attending as much as they have in the past.
The 18-24-year-old group is no longer the biggest ticket-buying demographic, with people in their mid-to-late twenties overtaking them. The younger crowd slumped from 41 per cent of all ticket buyers in 2018/19 to 27 per cent in 2022/23.
Genre specific events faring better
The report arrives amid a feast or famine crisis for the Australian music festival scene.
There's been a growing list of festival cancellations, from major events like Splendour In The Grass , Groovin The Moo and Mona Foma , to newer players like This That, Summerground , Vintage Vibes , Tent Pole , Valleyways, Costal Jam and more.
Amid those reports, however, genre-focused events — such as Good Things, Knotfest, Listen Out, CMC Rocks — are still proving popular, and summer staples — like Laneway Festival, Beyond The Valley and Field Day — are adapting to current challenges with great success.
The vast majority of Australian festivals predominantly feature homegrown line-ups, with four out of five acts being Australian. The most popular genre offering was electronic music, accounting for almost a quarter of Australian festivals. Other popular genres included rock (21 per cent) country (19 per cent) and indie (17 per cent).
Georgie McClean of Creative Australia says she hopes this research will serve as both a tool for those in the industry, as well as a way to exhibit the contributions music festivals make to Australia's creative sector.
"We hope this report will help us to better understand the role and contribution of festivals within the broader creative industries as they face multiple challenges.
"To inform the future work of Music Australia, we will be undertaking further research into how Australians discover, engage with and consume music, in order to better understand the broader ecosystem that underpins live music including festivals."
- X (formerly Twitter)
- Arts, Culture and Entertainment
- Carnivals and Festivals
- Music (Arts and Entertainment)
- Music Industry
- International edition
- Australia edition
- Europe edition
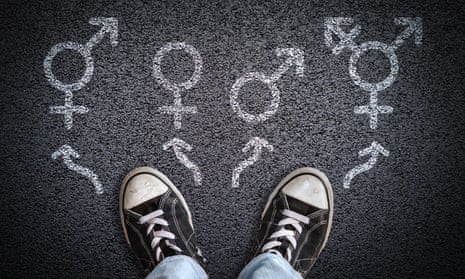
Gender medicine ‘built on shaky foundations’, Cass review finds
Analysis finds most research underpinning clinical guidelines, hormone treatments and puberty blockers to be low quality
Review of gender services has major implications for mental health services
The head of the world’s largest review into children’s care has said that gender medicine is “built on shaky foundations”.
Dr Hilary Cass, the paediatrician commissioned to conduct a review of the services provided by the NHS to children and young people questioning their gender identity, said that while doctors tended to be cautious in implementing new findings in emerging areas of medicine, “quite the reverse happened in the field of gender care for children”.
Cass commissioned the University of York to conduct a series of analyses as part of her review.
Two papers examined the quality and development of current guidelines and recommendations for managing gender dysphoria in children and young people. Most of the 23 clinical guidelines reviewed were not independent or evidence based, the researchers found.
A third paper on puberty blockers found that of 50 studies, only one was of high quality.
Similarly, of 53 studies included in a fourth paper on the use of hormone treatment, only one was of sufficiently high quality, with little or only inconsistent evidence on key outcomes.
Here are the main findings of the reviews:
Clinical guidelines
Increasing numbers of children and young people experiencing gender dysphoria are being referred to specialist gender services. There are various guidelines outlining approaches to the clinical care of these children and adolescents.
In the first two papers, the York researchers examined the quality and development of published guidelines or clinical guidance containing recommendations for managing gender dysphoria in children and young people up to the age of 18.
They studied a total of 23 guidelines published in different countries between 1998 and 2022. All but two were published after 2010.
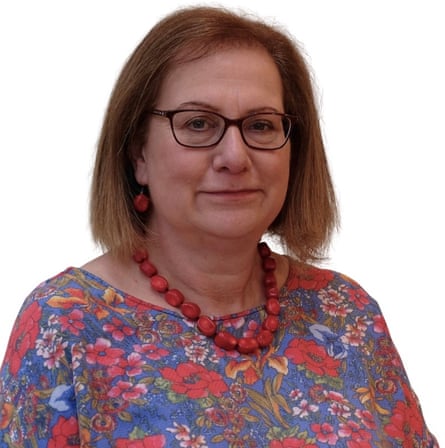
Most of them lacked “an independent and evidence-based approach and information about how recommendations were developed”, the researchers said.
Few guidelines were informed by a systematic review of empirical evidence and they lack transparency about how their recommendations were developed. Only two reported consulting directly with children and young people during their development, the York academics found.
“Healthcare services and professionals should take into account the poor quality and interrelated nature of published guidance to support the management of children and adolescents experiencing gender dysphoria/incongruence,” the researchers wrote.
Writing in the British Medical Journal (BMJ) , Cass said that while medicine was usually based on the pillars of integrating the best available research evidence with clinical expertise, and patient values and preferences, she “found that in gender medicine those pillars are built on shaky foundations”.
She said the World Professional Association of Transgender Healthcare (WPATH) had been “highly influential in directing international practice, although its guidelines were found by the University of York’s appraisal to lack developmental rigour and transparency”.
In the foreword to her report, Cass said while doctors tended to be cautious in implementing new findings “quite the reverse happened in the field of gender care for children”.
In one example, she said a single Dutch medical study, “suggesting puberty blockers may improve psychological wellbeing for a narrowly defined group of children with gender incongruence”, had formed the basis for their use to “spread at pace to other countries”. Subsequently, there was a “greater readiness to start masculinising/feminising hormones in mid-teens”.
She added: “Some practitioners abandoned normal clinical approaches to holistic assessment, which has meant that this group of young people have been exceptionalised compared to other young people with similarly complex presentations. They deserve very much better.”
Both papers repeatedly pointed to a key problem in this area of medicine: a dearth of good data.
She said: “Filling this knowledge gap would be of great help to the young people wanting to make informed choices about their treatment.”
Cass said the NHS should put in place a “full programme of research” looking at the characteristics, interventions and outcomes of every young person presenting to gender services, with consent routinely sought for enrolment in a research study that followed them into adulthood.
Gender medicine was “an area of remarkably weak evidence”, her review found, with study results also “exaggerated or misrepresented by people on all sides of the debate to support their viewpoint”.
Alongside a puberty blocker trial, which could be in place by December, there should be research into psychosocial interventions and the use of the masculinising and feminising hormones testosterone and oestrogen, the review found.
Hormone treatment
Many trans people who seek medical intervention in their transition opt to take hormones to masculinise or feminise their body, an approach that has been used in transgender adults for decades.
“It is a well-established practice that has transformed the lives of many transgender people,” the Cass review notes, adding that while these drugs are not without long-term problems and side-effects, for many they are dramatically outweighed by the benefits.
For birth-registered females, the approach means taking testosterone, which brings about changes including the growth of facial hair and a deepening of the voice, while for birth-registered males, it involves taking hormones including oestrogen to promote changes including the growth of breasts and an increase in body fat. Some of these changes may be irreversible.
However, in recent years a growing proportion of adolescents have begun taking these cross-sex, or gender-affirming, hormones, with the vast majority who are prescribed puberty blockers subsequently moving on to such medication.
This growing take-up among young people has led to questions over the impact of these hormones in areas ranging from mental health to sexual functioning and fertility.
Now researchers at the University of York have carried out a review of the evidence, comprising an analysis of 53 previously published studies, in an attempt to set out what is known – and what is not – about the risks, benefits and possible side-effects of such hormones on young people.
All but one study, which looked at side-effects, were rated of moderate or low quality, with the researchers finding limited evidence for the impact of such hormones on trans adolescents with respect to outcomes, including gender dysphoria and body satisfaction.
The researchers noted inconsistent findings around the impact of such hormones on growth, height, bone health and cardiometabolic effects, such as BMI and cholesterol markers. In addition, they found no study assessed fertility in birth-registered females, and only one looked at fertility in birth-registered males.
“These findings add to other systematic reviews in concluding there is insufficient and/or inconsistent evidence about the risks and benefits of hormone interventions in this population,” the authors write.
However, the review did find some evidence that masculinising or feminising hormones might help with psychological health in young trans people. An analysis of five studies in the area suggested hormone treatment may improve depression, anxiety and other aspects of mental health in adolescents after 12 months of treatment, with three of four studies reporting an improvement around suicidality and/or self-harm (one reported no change).
But unpicking the precise role of such hormones is difficult. “Most studies included adolescents who received puberty suppression, making it difficult to determine the effects of hormones alone,” the authors write, adding that robust research on psychological health with long-term follow-up was needed.
The Cass review has recommended NHS England should review the current policy on masculinising or feminising hormones, advising that while there should be the option to provide such drugs from age 16, extreme caution was recommended, and there should be a clear clinical rationale for not waiting until an individual reached 18.
Puberty blockers
Treatments to suppress puberty in adolescents became available through routine clinical practice in the UK a decade ago.
While the drugs have long been used to treat precocious puberty – when children start puberty at an extremely young age – they have only been used off-label in children with gender dysphoria or incongruence since the late 1990s. The rationale for giving puberty blockers, which originated in the Netherlands, was to buy thinking time for young people and improve their ability to smooth their transition in later life.
Data from gender clinics reported in the Cass review showed the vast majority of people who started puberty suppression went on to have masculinising or feminising hormones, suggesting that puberty blockers did not buy people time to think.
To understand the broader effects of puberty blockers, researchers at the University of York identified 50 papers that reported on the effects of the drugs in adolescents with gender dysphoria or incongruence. According to their systematic review, only one of these studies was high quality, with a further 25 papers regarded as moderate quality. The remaining 24 were deemed too weak to be included in the analysis.
Many of the reports looked at how well puberty was suppressed and the treatment’s side-effects, but fewer looked at whether the drugs had their intended benefits.
Of two studies that investigated gender dysphoria and body satisfaction, neither found a change after receiving puberty blockers. The York team found “very limited” evidence that puberty blockers improved mental health.
Overall, the researchers said “no conclusions” could be drawn about the impact on gender dysphoria, mental and psychosocial health or cognitive development, though there was some evidence bone health and height may be compromised during treatment.
Based on the York work, the Cass review finds that puberty blockers offer no obvious benefit in helping transgender males to help their transition in later life, particularly if the drugs do not lead to an increase in height in adult life. For transgender females, the benefits of stopping irreversible changes such as a deeper voice and facial hair have to be weighed up against the need for penile growth should the person opt for vaginoplasty, the creation of a vagina and vulva.
In March, NHS England announced that children with gender dysphoria would no longer receive puberty blockers as routine practice. Instead, their use will be confined to a trial that the Cass review says should form part of a broader research programme into the effects of masculinising and feminising hormones.
- Transgender
- Young people
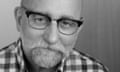
Veteran trans campaigner: ‘Cass review has potential for positive change’
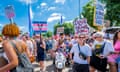
Cass review must be used as ‘watershed moment’ for NHS gender services, says Streeting
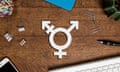
‘This isn’t how good scientific debate happens’: academics on culture of fear in gender medicine research
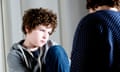
Five thousand children with gender-related distress awaiting NHS care in England
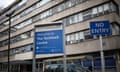
Ban on children’s puberty blockers to be enforced in private sector in England
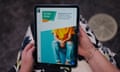
What Cass review says about surge in children seeking gender services
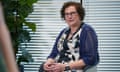
Adult transgender clinics in England face inquiry into patient care
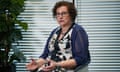
‘Children are being used as a football’: Hilary Cass on her review of gender identity services
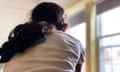
Thousands of children unsure of gender identity ‘let down by NHS’, report finds
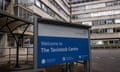
Most viewed
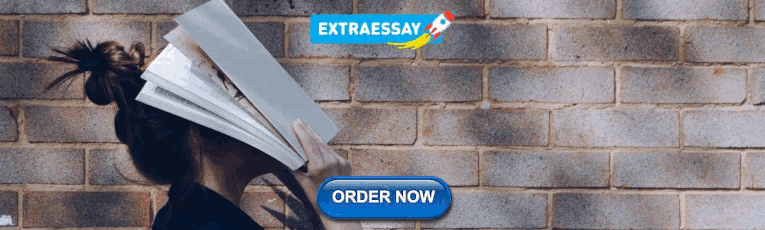
IMAGES
VIDEO
COMMENTS
ABC Analysis, an Inventory Management Technique at a. Manufacturing Company. Dr.S.Kavitha. A.Kandeepan. N.Narmadha. Abstract. The process of control and management of inventory is a very important ...
ABC Analysis For Inventory Management: Bridging The Gap Between Research And Classroom Handanhal Ravinder, Montclair State University, USA ... paper, it is argued that it is time to bring multi-criteria ABC analysis center-stage in the textbooks. Today's businesses and supply chains operate in a world where the ability to deliver the right ...
20% of total annual consumption value. The Class 'C' items normally constitute 70% to 75 % of all inventory items. and account for 5% to 10% of total annual consu mption value. Steps for ...
This study aimed to understand the inventory management of Giant Superstore Taman Connaught. The study was qualitative study that explored the ABC analysis efficiency of inventory management in Giant Superstore, Taman Connaught an outlet of GCH Retail (Malaysia) SDN. BHD. ABC analysis was one method to manage the inventory management who good position was arranged according to the category ...
The ABC-Analysis deals with a simple method of material classification that concerns value and quantity. It is a well-established categorization technique based on the Pareto Principle for ...
The large body of research was summarized based on multiple criteria ABC analysis that has accumulated since the 1980s and the authors recommend that textbooks incorporate their key findings and methods into their discussions of this topic. ABC analysis is a well-established categorization technique based on the Pareto Principle for determining which items should get priority in the management ...
Academia.edu is a platform for academics to share research papers. ABC Analysis For Inventory Management: Bridging The Gap Between Research And Classroom ... 3. STATUS OF RESEARCH ON MULTI-CRITERIA ABC ANALYSIS Since Flores and Whybark (1987) first proposed looking at more than one criterion, this has been an area of active research. ...
The main purpose of ABC analysis is to focus on tight control of class A items, less control of class B items, and very low control of class C items [ 4 ]. Conventional ABC analysis follows the 80/20 law of Pareto [ 5 ]. According to this law, class A is the mostly valued class by having 60-80% of the total value with 10-20% of inventory ...
The ABC analysis is perhaps the most widely used method in inventory management aiming to classify items into three ordered classes: class A contains a relatively small number (5-10%) of the most important items, class B includes a larger number (20-30%) of items with moderate importance while the remaining (50-70%) items with relatively little importance belong to class C. Footnote 1 ...
ABC and XYZ analyses are carried out for the inventory items to determine the production strategy of each item class and the Economic Order Quantity (EOQ). After this examination, one of the end products of the company is chosen to develop the Materials Requirement Plan (MRP) for. The Bill of Materials (BOM) for the chosen product is created ...
W. L. Ng, A simple classifier for multiple criteria ABC analysis, European Journal of Operational Research 177 (2007) 344-353. Crossref, ISI, Google Scholar; 8. A. H. Vencheh, An improvement to multiple criteria ABC inventory classification, European Journal of Operational Research 21 (2010) 962-965. Crossref, ISI, Google Scholar; 9. Y.
2.2.4. ABC analysis. The ABC stock control technique relies on that the decision a little bundle of the things may usually address the weight of money estimation of the total stock. It is used as a part of the era method, while a tremendous number of things may happen from a little part of the money estimation of stores.
ABC analysis is one of the most effective tools the material management, based on Pareto's Law, ... Research and Referral Healthcare Institute of India by Nigah et al 24 in which 13.78% (class A items), 21.85% (class B) and 64.37% (class C) consumed 69.97%, 19.95% and 10.08% of pharmaceutical expenditures respectively. Similarity of these ...
This paper proposes a novel method for calculating precise limits for the selection of parameter sets. ... Results As a typical example from biomedical research, the feasibility of the ABC analysis as an objective replacement for classical subjective limits to select highly relevant variance components of pain thresholds is presented. The ...
This quantitative research targeted all health products distributed to the Nyamagabe administrative district's health facilities. A list of 457 essential medicines was used for this study. ... The ABC analysis in this study is comparable to other studies, such as Nang Nwe in Bangkok, Mohamed in Soudan, Deressa, and Nguyen [4, 13, 18, 24]. Nang ...
ABC Analysis as a Inventory Control Technique. PCT No. PCT/US98/05628 Sec. 371 Date Mar. 20, 1998 Sec. 102 (e) Date Mar. 20, 1998 PCT Filed Mar. 20, 1998 PCT Pub. No. WO99/09547 PCT Pub. Date Feb. 25, 1999A storage device for storing information includes a disc storage medium having a disc surface. An armature is positioned over the disc ...
Most of the distribution centers of chain supermarkets in China adopt the excessively simple activity-based classification (ABC) as the management method of classifying warehousing work, which often leads to an increase in operation and storage costs of the enterprise, a decrease in efficiency of the commodity circulation operation, and, eventually, a loss in enterprise growth because the ...
Semantic Scholar extracted view of "Multi-criteria ABC analysis using artificial-intelligence-based classification techniques" by Min-Chun Yu. ... This research compares support vector machine (SVM), and K-nearest neighbours (KNN) with the MCDM method Technique for Order Performance by Similarity to Ideal Solution (TOPSIS) to determine the most ...
It has been over 30 years since the first conceptual papers on a new cost system, Activity-Based Costing (ABC), were published (Cooper, 1988; Cooper & Kaplan, 1991, 1992; Kaplan, 1989).This system was a better alternative to traditional costing systems (McGowan, 1998), which were based on the volume of production and had received considerable criticism (Cooper, 1987; Cooper & Kaplan, 1988) as ...
In this thesis we consider a model of college hostel mess stores items (grocery and vegetables) for inventory management through ABC analysis. This research is composed of the following sections ...
1. Introduction. The Activity-Based Costing (ABC) system was first demonstrated by Johnson and Kaplan (1987) as a new, advanced method for calculating production costs. The ABC system can be defined as a system to measure the cost and effectiveness of activities, products, and services on the basis of resources used to create the product or service.
This paper is a thematic research review on the ABC System, its development, applications, challenges, and benefits. Several researchers claim that ABC is efficient in product pricing, cost-cutting strategy, and customer and profitability analysis. Meanwhile, Time-Driven ABC was introduced with the advantages of firm-wide application and lower ...
views of the National Bureau of Economic Research. NBER working papers are circulated for discussion and comment purposes. They have not been ... The prior analysis shows that office work has large positive treatment effects compared to WFH, but initially-high-ability workers are less likely, not more, to sort into office jobs. ...
Background: The continuous monitoring and recording of patients' pain status is a major problem in current research on postoperative pain management. In the large number of original or review articles focusing on different approaches for pain assessment, many researchers have investigated how computer vision (CV) can help by capturing facial expressions.
AI Index Report. The AI Index Report tracks, collates, distills, and visualizes data related to artificial intelligence. Our mission is to provide unbiased, rigorously vetted, broadly sourced data in order for policymakers, researchers, executives, journalists, and the general public to develop a more thorough and nuanced understanding of the ...
In short: A new report from arts investment and advisory body Creative Australia has delved into the current state of play of Australia's music festivals. It found that only 56 per cent of music ...
Last modified on Wed 10 Apr 2024 01.00 EDT. The head of the world's largest review into children's care has said that gender medicine is "built on shaky foundations". Dr Hilary Cass, the ...