- Research article
- Open access
- Published: 28 March 2022
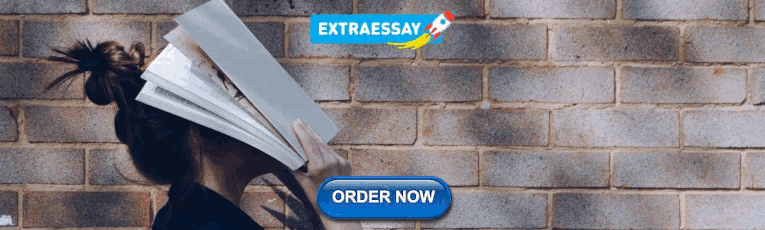
Social networking sites use and college students’ academic performance: testing for an inverted U-shaped relationship using automated mobile app usage data
- Wondwesen Tafesse ORCID: orcid.org/0000-0002-1284-7167 1
International Journal of Educational Technology in Higher Education volume 19 , Article number: 16 ( 2022 ) Cite this article
32k Accesses
7 Citations
4 Altmetric
Metrics details
With the widespread adoption of social networking sites among college students, discerning the relationship between social networking sites use and college students’ academic performance has become a major research endeavor. However, much of the available research in this area rely on student self-reports and findings are notably inconsistent. Further, available studies typically cast the relationship between social networking sites use and college students’ academic performance in linear terms, ignoring the potential moderating role of the intensity of social networking sites use. In this study, we draw on contrasting arguments in the literature predicting positive and negative effects of social networking sites use on college students’ academic performance to propose an inverted U-shaped relationship. We collected data on social networking sites use by having college students install a tracking app on their smartphones for 1 week and data on academic performance from internal college records. Our findings indicate that social networking sites use indeed exhibits an inverted U-shaped relationship with college students’ academic performance. Specifically, we find that spending up to 88.87 min daily on social networking sites is positively associated with academic performance, but beyond that, social networking sites use is negatively associated with academic performance. We discuss the implications of our findings.
Introduction
With the widespread adoption of social networking sites among college students, discerning the relationship between social networking sites use and college students’ academic performance has become a major research endeavor (Doleck & Lajoie, 2018 ; Koranteng et al., 2019 ; Liu et al., 2017 ; Tafesse, 2020 ). Numerous studies have been published on this topic to date and the relevant literature is accumulating rapidly (Doleck & Lajoie, 2018 ; Masrom et al., 2021 ). However, findings have been highly inconsistent (Astatke et al., 2021 ), with some studies documenting a negative relationship between social networking sites use and academic performance (e.g., Junco, 2015 ; Karpinski et al., 2013 ; Tafesse, 2020 ) and others documenting a positive relationship (e.g., Park et al., 2018 ; Samad et al., 2019 ; Sarwar et al., 2019 ).
Notably, much of the available research relies on student self-reports to measure social networking sites use (Astatke et al., 2021 ; Doleck & Lajoie, 2018 ). Students are asked to self-report the frequency or duration of their social networking sites use. Because students have been shown to substantially underestimate their social networking sites use, however, self-report data is prone to measurement error, thereby potentially biasing the magnitude and direction of reported findings (Felisoni & Godoi, 2018 ; Giunchiglia et al., 2018 ; Wang et al., 2015 ). To overcome these limitations, researchers have begun to employ software programs and mobile applications that can automatically track the frequency and duration of social networking sites use, which enables precise measurement (e.g., Felisoni & Godoi, 2018 ; Giunchiglia et al., 2018 ; Wang et al., 2015 ). Coupled with the use of institutional records to measure students’ academic performance, these latter studies have managed to overcome the measurement difficulties afflicting self-reported data. However, even these more recent efforts typically cast the relationship between social networking sites use and academic performance in linear terms. That is, social networking sites use is proposed to linearly co-vary with academic performance.
In the current study, we maintain that the linear relationship typically tested in the literature may not fully capture the complex interplay between social networking sites use and academic performance. We contend that the relationship between social networking sites use and academic performance can be characterized as an inverted U-shape. The fact that both positive and negative effects have been reported in the literature (Astatke et al., 2021 ; Masrom et al., 2021 ; Raza et al., 2020 ) points to the possibility that social networking sites use might produce both positive and negative academic outcomes depending on the intensity of their use. For instance, heavy use of social networking sites can be detrimental to academic performance by having college students reallocate time away from academic work or requiring them to multi-task (Alt, 2015 ; Junco, 2015 ; Kapriniski et al., 2013 ; Marker et al., 2018 ). Modest use of social networking sites, on the other hand, might contribute positively to academic performance by facilitating collaborative learning and offering informational and entertainment values (Al-Qaysi et al., 2021 ; Hoi, 2021 ; Lampe et al., 2015 ; Lemay et al., 2020 ; Raza et al., 2020 ). Prior studies have suggested that not all social networking sites use is maladaptive (Lemay et al., 2020 ).
We combine the positive and negative effects of social networking sites use reported in the literature into an inverted U-shaped relationship by positing the intensity of social networking sites use as a moderating variable. The inverted U-shaped model fits the data better than the linear model, highlighting the crucial role that the intensity of social networking sites use plays in shaping the relationship between social networking sites use and college students’ academic performance. By demonstrating that social networking sites can be associated with both negative and positive academic outcomes depending on their intensity of use, our approach serves to reconcile empirical inconsistencies observed in the literature (Astatke et al., 2021 ). Further, the findings serve to synthesize the contrasting theoretical perspectives offered in the literature––some arguing for a positive effect of social networking sites use, others arguing for a negative effect––into a coherent curvilinear relationship. Overall, our findings contribute to a more nuanced understanding of the relationship between social networking sites use and college students’ academic performance.
Literature review
Social networking sites: an overview.
Ellison and Boyd ( 2013 ) defined social networking sites as “a networked communication platform in which participants (1) have uniquely identifiable profiles that consist of user-supplied content, content provided by other users, and/or system-level data; (2) can publicly articulate connections that can be viewed and traversed by others; and (3) can consume, produce, and/or interact with streams of user-generated content provided by their connections on the site” (p. 180). This definition emphasizes three defining features of social networking sites.
First, social networking sites allow users to create uniquely identifiable profiles animated by both user- and system-supplied information. Examples of these user- and system-supplied information that define a user’s profile on social networking sites include biographic details, self-descriptions, photos, interests and activities (Ellison & Boyd, 2013 ). These pieces of information facilitate online peer-to-peer networking by revealing users’ identities (Kane et al., 2014 ; Zhang & Leung, 2015 ). Second, social networking sites allow users to articulate connections that can be viewed and traversed by others. These connections are typically manifested in the form of friends lists, followers lists, group memberships, liked pages and so on. These publicly stated connections enable users to discern other users’ social connections, further facilitating peer-to-peer networking activities on the platforms (Ellison & Boyd, 2013 ). Zhang and Leung ( 2015 ) maintained that the ability to traverse and view other users’ connections and activities is an innovative feature of social networking sites that is virtually unknown in traditional forms of communication. Finally, social networking sites allow users to consume, produce and interact with the streams of user-generated content provided by their connections (Kane et al., 2014 ). Users create their content by combining text, images, videos, emoticons, animations and so forth—all languages of social networking sites (Dumpit & Fernandez, 2017 ). As well as sharing their own content, users can consume and interact with other users’ content, by liking, sharing and commenting on them, thereby creating a dynamic and continuous cycle of online interaction and engagement, which is essential to the vitality of social networking sites (Masrom et al., 2021 ; Smith, 2017 ).
College students rely heavily on social networking sites for their daily communication, entertainment and information needs (Ansari & Khan, 2020 ; Doleck et al., 2018 ; Ifinedo, 2016 ; Lemay et al., 2020 ). Studies tracking college students’ social media habits have indicated that students spend a significant amount of time daily, switching between multiple social networking sites such as Facebook, Twitter, Instagram, YouTube and Snapchat (Alhabash & Ma, 2017 ; Dumpit & Fernandez, 2017 ; Felisoni & Godoi, 2018 ; Smith, 2017 ; Wang et al., 2015 ). College students use social networking sites for various purposes including opinion sharing, information acquisition, entertainment, self-documentation, self-expression and social interactions, among others (Alhabash & Ma, 2017 ; Chawinga, 2017 ; Lemay et al., 2020 ). Educational use of social networking sites, such as accessing course information, organizing group work, receiving feedback and interacting with instructors, have also been noted in the literature (Al-Qaysi et al., 2021 ; Al-Rahmi et al., 2020 ; Ansari & Khan, 2020 ; Hoi, 2021 ; Raza et al., 2020 ; Smith, 2017 ).
Review of the empirical literature
The pervasive adoption and use of social networking sites among college students have spurred a flurry of research into how social networking sites use influences academic performance (Masrom et al., 2021 ). Several studies have been published and the relevant literature has accumulated over the past years. In response, several systematic literature reviews (e.g., Astatke et al., 2021 ; Doleck & Lajoie, 2018 ; Masrom et al., 2021 ) and meta-analyses (e.g., Huang, 2018 ; Liu et al., 2017 ) have been carried out. Yet, these reviews and meta-analyses document major inconsistencies in the literature. Despite the expanding literature and efforts to consolidate it, results remain inconsistent. Below, we present a summary of representative works.
In an early study, Karpinski et al. ( 2013 ) looked at the relationship between social networking sites use and academic performance among college students in the USA and Europe. They find that social networking sites use is negatively associated with college students’ academic performance both in the US and European samples, but the association is stronger for the US sample. In another widely cited study, Junco ( 2015 ) investigated the relationship between social networking sites use and college students’ academic performance by considering class standing as a moderating variable. The researcher finds that freshmen suffered the highest decline in academic performance from increased social networking sites use, while seniors were less severely affected. Recently, Tafesse ( 2020 ) finds that increased use of social networking sites is negatively associated with academic performance both directly, and indirectly, via decreased student engagement.
In a study that examined the relationship between social networking sites use and student engagement among Korean college students, Park et al. ( 2018 ) reported a positive relationship. But when used for purposes such as image management and social pressure, social networking sites use tends to reduce student engagement. Similarly, Sarwar et al. ( 2019 ) find that social networking sites use contributes positively to college students’ academic performance both directly, and indirectly, by enabling collaborative learning. Finally, Al-Rahmi et al. ( 2020 ) find that college students’ increased perceptions of social presence, interest, perceived enjoyment and perceived usefulness of social networking sites are positively associated with collaborative learning.
Despite their contributions to a deeper understanding of how social networking sites use influence academic performance, the reviewed studies relied on student self-reports to measure both social networking sites use and academic performance, which might introduce measurement errors by, for instance, eliciting socially desirable answers or artificially inflating the correlation among measured variables due to common method bias (Podsakoff et al., 2003 ). To overcome these measurement issues, researchers have begun to deploy software programs and mobile applications that are installed on students’ PCs or smartphones to automatically track the frequency and duration of social networking sites use (Felisoni & Godoi, 2018 ; Giunchiglia et al., 2018 ; Wang et al., 2015 ). Increasingly also, researchers are obtaining data about students’ academic performance from institutional records instead of student self-reports. Collecting data from multiple sources is one of the most effective procedural remedies against common method bias (Podsakoff et al., 2003 ).
Pertinent among this latter group of studies is a pioneering investigation by Wang et al. ( 2015 ), which tracked the social media behavior of college students in the USA for one week by having them install a software program on their PCs and smartphones. The researchers subsequently divided their sample into heavy versus light users and compared their perceptions of how social networking sites use affect academic performance. Their findings suggest that heavy users felt more distracted and fell behind on schoolwork relative to light users. Although the researchers did not formally test the moderating effect of the intensity of social networking sites use, their findings reveal sharp differences in perceptions between heavy and light users.
In a more recent study, Giunchiglia et al. ( 2018 ) measured social networking sites use by having college students install a mobile usage tracking app on their devices and run it for a week. In addition, they employed time diaries to measure social networking sites use during lecture hours and study time. Their findings indicate that increased social networking sites use during lecture hours and study time is negatively predictive of semester GPA. Conversely, social networking sites inactivity during lecture hours and study time is positively predictive of semester GPA. In another study, Felisoni and Godoi ( 2018 ) tracked college students’ overall smartphone use for one week using a tracking app. They find a negative relationship between increased smartphone use and semester GPA.
Following the latter group of studies, we measured social networking sites use by having college students install a mobile usage tracking app on their smartphones and run it for one week and students’ academic performance using semester and cumulative GPAs obtained from internal college records. However, we departed from previous studies by testing for an inverted U-shaped relationship. Extant studies typically model the relationship between social networking sites use and academic performance linearly, which ignores the potential moderating role of the intensity of social networking sites use. By testing for an inverted U-shaped relationship, we demonstrate the moderating role of the intensity of social networking sites use in the relationship between social networking sites use and college students’ academic performance.
Theoretical perspectives
Two main theoretical perspectives are put forth in the literature to explain the relationship between social networking sites use and college students' academic performance: the time-displacement/multitasking argument; and the collaborative learning argument.
The first perspective holds that social networking sites distract students from attaining deeper engagement with their academic study (Alt, 2015 ; Astatke et al., 2021 ; Cao et al., 2018 ; Doleck et al., 2018 ; Junco, 2012 ; Karpinski et al., 2013 ). Two important theoretical mechanisms are proposed to explain this negative relationship: time displacement and multitasking. The time displacement explanation is based on the notion that time is inelastic and daily human activities are scheduled around a fixed, 24-h cycle. The introduction of a new activity, therefore, comes at the expense of other established activities as less time would be available for them (Nie, 2001 ; Tokunaga, 2016 ). According to the time displacement argument, time spent on social networking sites is time reallocated from important academic activities such as studying, attending classes or doing assignments (Doleck et al., 2018 ; Tafesse, 2020 ). By forcing the reallocation of time from academically productive to academically nonproductive tasks, social networking sites use is argued to adversely affect students’ academic performance (Alt, 2015 ; Cao et al., 2018 ; Tafesse, 2020 ).
The multitasking explanation, on the other hand, suggests that attending to two or more tasks at the same time can result in cognitive overload, which reduces students’ ability to correctly and completely execute the tasks at hand (Junco, 2012 ; Junco & Cotton, 2012 ; Karpinski et al., 2013 ; Lau, 2017 ). The multitasking argument implies that trying to accomplish academic tasks while staying on social networking sites reduces students’ attention span and their cognitive ability to effectively engage in academic work, thereby adversely affecting their academic performance (Junco, 2012 ; Karpinski et al., 2013 ; Lau, 2017 ; Lepp et al., 2015 ).
The second perspective holds that social networking sites can be harnessed to facilitate collaborative learning and motivate students into a more constructive learning engagement (Eid & Al-Jabri, 2016 ; Hoi, 2021 ; Lampe et al., 2015 ; Liu et al., 2017 ; Raza et al., 2020 ). Researchers subscribing to this perspective point to the fact that the interactive and social features of social networking sites can be utilized to exchange information, arrange group work, receive feedback and facilitate interaction with instructors (Al-Rahmi et al., 2020 ; Ansari & Khan, 2020 ; Chawinga, 2017 ; Lampe et al., 2015 ; Smith, 2017 ). Social networking sites emphasize collaboration and group engagement as opposed to individual learning, thereby allowing students to become active partners and socially engaged in the process of exchanging information, discovering knowledge and solving problems, which should increase their overall learning and academic performance (Ansari & Khan, 2020 ; Astatke et al., 2021 ; Lampe et al., 2015 ; Sarwar et al., 2019 ; Smith, 2017 ).
With the growing role of social networking sites as a platform for opinion sharing and information exchange at a societal level (Ellison & Boyd, 2013 ), exposure to social networking sites can further widen students’ perspectives and introduce them to diverse worldviews (Alloway et al., 2013 ; Chawinga, 2017 ; Park et al., 2018 ). Social networking sites could also offer students relief from demanding academic tasks by availing entertaining content, such as funny videos, jokes and memes, which can increase their motivation for subsequent tasks (Ansari & Khan, 2020 ; Eid & Al-Jabri, 2016 ; Phua et al., 2017 ; Raza et al., 2020 ).
We draw on the two contrasting perspectives presented above to propose an inverted U-shaped relationship between social networking sites use and college students’ academic performance. The proposed model anticipates a positive relationship between social networking sites use and academic performance when the intensity of social networking sites use is low and a negative relationship when the intensity of social networking sites use is high.
Methodology
Sampling and data collection.
The current study was carried out at a large public university in an Eastern African country in 2019. The study targeted undergraduate students studying business and economics subjects. Business and economics students were chosen for the simple reason that the researchers involved in the study were affiliated with the Business and Economics College. The necessary ethical clearance was obtained from the Office of the Vice-Dean to conduct the study.
Data on students’ social networking sites use was collected by asking voluntary students to install “App Usage”—a freely available mobile usage tracking app—on their smartphones in the Spring 2019 semester. Although we evaluated several candidate mobile usage tracking apps for the purpose of our study, we settled on App Usage for two reasons. First, App Usage offers an accurate measurement of users’ smartphone activities. We installed App Usage on our smartphones, personally checked its accuracy and we were satisfied with the result. Second, App Usage has an intuitive and convenient feature for downloading and sharing one’s app usage history either via email or messaging apps. Because usage history is rendered in a CSV file format, it facilitates faster data capture and processing. An example of custom reports produced by App Usage is presented in the Appendix.
Due to the sensitivity of the data we were after, we resorted to a snowball approach to recruit participants. We start by recruiting an initial batch of students based on personal rapport and solicited their voluntary participation. We then asked this initial batch to recruit additional participants. Through this process, we recruited about 51 voluntary participants. To minimize the effect of social desirability bias, we excluded students attending any one of our classes. Subsequently, we familiarized the participants with the basic functionality of App Usage and asked them to install it on their smartphones. To increase the number of valid responses from the participants, we took several confidence-building steps. First, we limited the applicable usage history to only one week. Second, we excluded weekends since social media use during weekends can be particularly personal relative to weekdays. Likewise, to minimize the potential impact of installing App Usage on students’ smartphone habits, we let the participants run App Usage for three weeks before asking them to submit their usage history in the fourth week. Further, we let students install App Usage after three weeks into the Spring semester. This allowed us to avoid tracking students’ smartphone activities during exam periods, which might underreport their smartphone behavior.
Eventually, 40 students submitted valid app usage data. The remaining 11 students failed to send in their usage data despite our best efforts. Although relatively small, the final sample (N = 40) was representative of the student population in terms of departmental affiliation (accounting = 47%; management = 28%; marketing = 25%), gender-mix (male = 60%; female = 40%) and academic year (second year = 62%; third year = 38%). Notably, first-year students were underrepresented in our data. This is because the initial batch of participants we approached were all second-and third-year students. However, the departmental affiliation and gender proportion in the data map well to the departmental affiliation and gender proportion of the student population. Table 1 summarizes the sample characteristics.
Measurement of variables
The usage history submitted by the students contained details including the names of the mobile apps they used, the amount of time they spent on each mobile app and the start and end dates of the usage history. We constructed two relevant variables from this data. The first was daily average minutes spent on social networking sites, which was used to measure the intensity of students’ social networking sites use (Felisoni & Godoi, 2018 ; Giunchiglia et al., 2018 ). The second variable was daily average minutes spent on smartphone, which was used to measure the amount of time students spent on their smartphones overall. This second variable was used as a control variable.
In order to construct daily average minutes spent on social networking sites, we first had to identify those applications that would qualify as social networking sites. For this purpose, we turned to the definition by Ellison and Boyd ( 2013 ) discussed in “Social networking sites: An overview”. We analyzed the usage history of each student and identified those mobile apps that offer social networking affordances as explicated in Ellison and Boyd’s definition. This process resulted in the identification of about 24 mobile apps, many of them household names around the world and their official variants, such as Facebook (Facebook Lite), Twitter, Instagram, YouTube (YouTube Go), Messenger (Messenger Lite, + Messenger), Telegram (Telegram+ , Telegram X), IMO, WhatsApp, Viber and Google+ . Some of the less-known names we came across include VidMate, Mobogram and Russogram. Our coding scheme is also consistent with previous categorization of social networking sites. For instance, Smith ( 2017 ) identified several social networking sites used in undergraduate learning, many of these sites are included in our coding.
Data on students’ academic performance were collected from the Office of the Vice-Dean, which is responsible for storing students’ academic records at the college level. We gathered semester and cumulative GPAs. Both GPAs were measured on a four-point scale (0.0 to 4.0). We also gathered information about participants’ departmental affiliation, academic year and gender from the same official source. Table 2 reports the descriptive statistics and pairwise correlation of the measured variables.
Analysis strategy
Traditionally, an inverted U-shaped relationship is empirically established by adding a squared term to the predictor variable of interest—in our case, social networking sites use—to a standard linear regression equation, as shown below:
where \({y}_{i}\) is the semester GPA for student i ; \({X}_{i}\) is the daily average minutes spent on social networking sites by student i ; \({x}_{i}^{2}\) is the squared term of daily average minutes spent on social networking sites by student i ; \({Z}_{ij}\) is the \(j^{\prime}s\) control variable for student i including daily average minutes spent on smartphone, gender, academic year and departmental affiliation; \({\beta }_{0}\) , \({\beta }_{1},\) …, \({\beta }_{j}\) are parameters to be estimated; and \({\varepsilon }_{i}\) is a normally distributed error term.
If β 2 from Eq. 1 is negative and statistically significant, an inverted U-shaped relationship can be claimed. However, this traditional approach has come under growing criticism for being simplistic and lacking in rigor (Haans et al., 2016 ; Simonsohn, 2018 ). Lind and Mehlum ( 2010 ) proposed a stricter approach that requires three necessary and sufficient conditions for establishing an inverted U-shaped relationship. The first condition is β 2 from Eq. 1 should be negative and statistically significant. The second condition is the turning point in Eq. 1 should fall within the data range (i.e., between the minimum and maximum values of the dependent variable). The turning point is arrived at by taking the first derivative of Eq. 1 and setting it to zero, which yields − β 1 /2β 2 . The third and final condition is the slope at the lower half of the data should be positive and statistically significant and the slope at the upper half of the data should be negative and statistically significant. This condition can be tested by dividing the dataset into two parts, typically by using the turning point as a cutoff point, and estimating two separate linear regression equations for each part of the dataset (Simonsohn, 2018 ).
In addition to Lind and Mehlum’s ( 2010 ) three conditions, one also needs to establish that the quadratic regression model fits the data better than the linear model. If adding the squared term to the linear model leads to a significant improvement in model fit, as measured by a statistically significant R 2 change, for instance, the quadratic regression model should be retained (Weisberg, 2005 ). Otherwise, it has to be rejected in favor of the more parsimonious linear regression model. We analyzed the data according to the three conditions outlined above.
We started off our analysis by estimating the linear regression model. To correct for heteroscedasticity, we reported White’s heteroscedastic consistent standard errors (White, 1980 ). The linear regression model was statistically significant ( F = 5.844; p < 0.01), attaining R 2 = 0.401 and adjusted R 2 = 0.293. Likewise, the regression coefficient for daily average minutes spent on social networking sites was negative and statistically significant ( β 1 = − 0.004; p < 0.01). Table 3 reports the estimation results of the linear regression model.
Second, we estimated the quadratic regression model (Eq. 1 ). As in the linear model, we reported White’s heteroscedastic consistent standard errors. The quadratic regression model was also statistically significant ( F = 12.75; p < 0.01). It attained R 2 = 0.609 and adjusted R 2 = 0.524. The F-change from the linear model ( F lin = 5.844; p < 0.01) to the quadratic model ( F qdr = 12.75; p < 0.01) was statistically significant at p < 0.01. We, therefore, retained the quadratic regression model as it offered a better fit to the data than the linear model (Weisberg, 2005 ). Table 4 reports the estimation results of the quadratic regression model.
Importantly, the squared term for the daily average minutes spent on social networking sites in the quadratic regression model was negative and statistically significant ( β 2 = − 0.0000467; p < 0.01). This result satisfied the first condition of Lind and Mehlum’s ( 2010 ) test, thereby offering initial evidence for an inverted U-shaped relationship between social networking sites use and academic performance.
The turning point (i.e., − β 1 / − 2 β 2 = − 0.0083 / − 2 × 0.0000934) occurred at 88.87 min, which is approximately one and half hours of daily average social networking sites use. This turning point lies well within the data range for daily average minutes spent on social networking sites (minimum daily average minutes spent on social networking sites = 5.62 min, maximum daily average minutes spent on social networking sites = 280.5 min), hence satisfying the second condition of Lind and Mehlum’s ( 2010 ) test.
To test the third condition, we grouped the students into two: low users (n = 25) and high users (n = 15). The turning point was used to create the two groups (i.e., students who spent a daily average of 88.87 min or less were categorized into the low user group; students who spent a daily average of 88.87 min or more were categorized into the high user group). Subsequently, we estimated two linear regression equations for each group. The estimation results are summarized in Tables 5 and 6 . The slope for the low user group was positive and statistically significant ( β 1 = 0.005; p < 0.1), whereas the slope for the high user group was negative and statistically significant ( β 1 = − 0.0097; p < 0.01). Because of the limited observation in both the low and high user groups, we find it reasonable to reject the null hypothesis at p < 0.1. The statistically significant and positive slope for the low user group and the statistically significant and negative slope for the high user group satisfied the third and final condition of Lind and Mehlum’s ( 2010 ) test.
To summarize, we find strong evidence for an inverted U-shaped relationship between college students’ social networking sites use and academic performance. We should further note that we conducted regression diagnostics (e.g., QQ plots, residual plots) for all estimated models and found that the models were well-behaved. Figure 1 visualizes the regression plots for the linear and quadratic regression models.
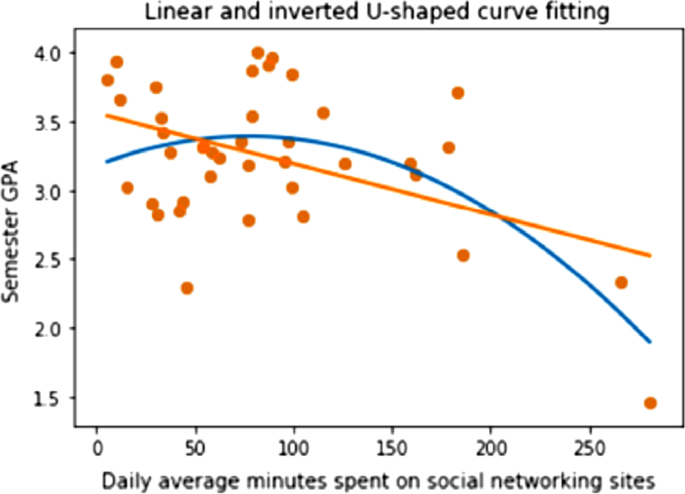
Regression plots
Robustness check
We implemented a robustness check to examine whether the inverted U-shaped relationship holds under different specifications of the dependent variable. Specifically, we replaced semester GPA with cumulative GPA as the dependent variable. While semester GPA captures academic performance in a single semester, cumulative GPA captures academic performance for several semesters. Therefore, cumulative GPA offers a more stable measure of academic performance. The results from the main model were fully replicated when cumulative GPA was used as the dependent variable. Specifically, the quadratic regression model fit the data better than the linear model ( R 2 lin = 0.3 vs. R 2 qdr = 0.39; F lin = 3.57, p < 0.01 vs. F qdr = 5.34, p < 0.01). The F-change was significant at p < 0.05. As in the case of semester GPA, the squared term for daily average social networking sites use was negative and statistically significant ( β 2 = − 1.21; p < 0.05). Finally, the linear regression coefficient for the low user group was positive and statistically significant ( β 1 = 0.29; p < 0.1), while it was negative and statistically significant for the high user group ( β 1 = − 0.62; p < 0.01). Overall, the results from the semester GPA model were fully replicated when cumulative GPA was employed as the dependent variable, suggesting that the inverted U-shaped relationship remained robust to a different measure of academic performance.
The pervasive adoption of social networking sites among college students has spurred a stream of research into the implications of social networking sites use for college students’ academic performance (Doleck & Lajoie, 2018 ; Koranteng et al., 2019 ; Masrom et al., 2021 ). Reported findings have been highly inconsistent, however, with some studies reporting negative relationships and others reporting positive relationships (Astatke et al., 2021 ; Masrom et al., 2021 ). Against this backdrop, we proposed and found support for an inverted U-shaped relationship. Following recent advances in the literature (Felisoni & Godoi, 2018 ; Giunchiglia et al., 2018 ), we measured social networking sites use with the help of a tracking app installed on students’ smartphones. Further, we measured students’ academic performance using semester and cumulative GPAs obtained from internal college records. By employing a combination of automatically tracked and institutional data, we avoided the measurement error common in self-reported data (Podsakoff et al., 2003 ).
Our main finding reveals that the inverted U-shaped relationship fits the data better than the linear relationship. The turning point on the inverted U-shaped regression curve occurred at 88.87 min, suggesting that spending up to 88.87 min daily on social networking sites (about an hour and a half) is positively associated with students’ academic performance, while spending more than 88.87 min daily on social networking sites is negatively associated with students’ academic performance. This finding was robust to an alternative specification of academic performance.
It thus appears that, when used modestly, social networking sites are positively associated with students’ academic performance. Modest use is less likely to interfere with students’ academic performance as they will be forced neither to reallocate time away for academic tasks nor to multi-task (Chawinga, 2017 ; Wang et al., 2015 ). In fact, modest use of social networking sites might boost students’ academic engagement (Al-Rahmi et al., 2020 ; Masrom et al., 2021 ). For instance, social networking sites have been shown to facilitate collaborative learning, where students engage in socially interactive learning by completing group work, receiving feedback, sharing course material and interacting with each other and their instructors (Al-Qaysi et al., 2020 ; Eid & Al-Jabri, 2016 ; Hoi et al., 2021 ; Lampe et al., 2015 ). Similarly, social networking sites offer students access to information and entertaining content that might contribute to improved academic performance (Alloway et al., 2013 ; Ansari & Kahn, 2020 ; Lepp et al., 2015 ; Masrom et al., 2021 ; Raza et al., 2020 ). This last point is particularly poignant in the national context of our study, where the media infrastructure is neither well developed nor widely accessible to satisfy college students’ demand for information and entertainment (Tafesse, 2020 ). Social networking sites thus double as a source of information and pastime for the college students in our sample (Alhabash & Ma, 2017 ; Chawinga, 2017 ).
In contrast, heavy use of social networking sites can interfere with students’ academic activities (Koranteng et al., 2019 ; Tafesse, 2020 ). With heavy use, students will be forced either to divert time away from crucial academic tasks or to multi-task, which will eventually hamper their academic performance (Kapriniski et al., 2013 ; Lepp et al., 2015 ). In fact, heavy social networking sites use can degenerate into compulsive behavior, such as excessive use and addiction, which can detriment not only students’ academic performance but also their overall well-being (Alt, 2015 ; Cao et al., 2018 ; Hsiao et al., 2017 ; Masrom et al., 2021 ).
Overall, our work contributes to a more nuanced understanding of the relationship between social networking sites use and academic performance among college students. The inverted U-shaped relationship that we proposed and validated serves to reconcile the empirical inconsistencies observed in the literature in terms of positive and negative effects of social networking sites use (Astatke et al., 2021 ; Masrom et al., 2021 ). As our findings demonstrate, social networking sites can produce both positive and negative academic outcomes depending on the intensity of their use. What is crucial to the relationship is the intensity of use, which can easily be captured by an inverted U-shaped model.
Finally, our study comes with a set of limitations that must be considered while interpreting the findings. First, the study relied on a small set of observations sampled using a snowball approach. As such, the sample may not offer an accurate representation of the total student population. The personal and highly sensitive nature of the data we gathered meant that we had to settle with the small number of participants we were able to recruit. However, the sample size we used is not unusual for studies of this nature. For instance, Felisoni and Godoi’s ( 2018 ) study, which tracked college students’ cellphone use in Brazil, was based on 42 observations. Second, we exclusively studied business and economics students. Since students from other disciplines were not included in our study, the findings may not extend to these other disciplines. Third, our sample is taken from a setting that has its own peculiarities. For instance, students at public universities in our setting live on campus for the entire academic year, have access to free WIFI connection and are connected to the internet almost exclusively by way of their smartphones. The students thus connect to social networking sites—and the internet more generally—at no personal cost to them, which might incentivize heavier use. Moreover, the mainstream media infrastructure in the country is rather underdeveloped, which amplifies the informational and entertainment value of social networking sites for college students. These combinations of factors must be considered when efforts are made to extend the findings to new settings.
With the pervasive adoption and use of social networking sites among college students, probing their relationship with college students’ academic performance has become an important research priority. Building on the extant literature, we proposed and validated an inverted U-shaped relationship between social networking sites use and college students’ academic performance. In so doing, we departed from the more traditional approach that casts the relationship between the two in linear terms.
As our findings suggest, moderate use of social networking sites is positively associated with academic performance, while heavy use is negatively associated with academic performance. These findings highlight the crucial role that the intensity of social networking sites use play in shaping the influence of social networking sites on college students’ academic performance.
To our knowledge, our study is the first to test and find support for an inverted U-shaped relationship between social networking sites use and college students’ academic performance. As such, the proposed model should be validated using fresh data, preferably from new contexts, to develop further confidence in the findings. With further validation, the findings can help in the continued effort to harness social networking sites for productive academic purposes in higher education settings (Masrom et al., 2021 ; Smith, 2017 ).
Availability of data and materials
No data will be publicly available for this study since the data was collected after assuring students that their data will not be publicly shared.
Alhabash, S., & Ma, M. (2017). A tale of four platforms: Motivations and uses of Facebook, twitter, Instagram, and Snapchat among college students? Social Media + Society, 3 (1), 1–13.
Google Scholar
Alloway, T. P., Horton, J., Alloway, R. G., & Dawson, C. (2013). Social networking sites and cognitive abilities: Do they make you smarter? Computers & Education, 63 , 10–16.
Al-Qaysi, N., Mohamad-Nordin, N., & Al-Emran, M. (2020). What leads to social learning? Students’ attitudes towards using social media applications in Omani higher education. Education and Information Technologies, 25 (3), 2157–2174.
Al-Qaysi, N., Mohamad-Nordin, N., & Al-Emran, M. (2021). Developing a comprehensive theoretical model for adopting social media in higher education. Interactive Learning Environments . https://doi.org/10.1080/10494820.2021.1961809
Article Google Scholar
Al-Rahmi, W. M., Yahaya, N., Alturki, U., Alrobai, A., Aldraiweesh, A. A., Omar Alsayed, A., & Kamin, Y. B. (2020). Social media-based collaborative learning: the effect on learning success with the moderating role of cyberstalking and cyberbullying. Interactive Learning Environments . https://doi.org/10.1080/10494820.2020.1728342
Alt, D. (2015). College students’ academic motivation, media engagement and fear of missing out. Computers in Human Behavior, 49 , 111–119.
Ansari, J. A. N., & Khan, N. A. (2020). Exploring the role of social media in collaborative learning the new domain of learning. Smart Learning Environments, 7 (1), 1–16.
Astatke, M., Weng, C., & Chen, S. (2021). A literature review of the effects of social networking sites on secondary school students’ academic achievement. Interactive Learning Environments . https://doi.org/10.1080/10494820.2021.1875002
Cao, X., Masood, A., Luqman, A., & Ali, A. (2018). Excessive use of mobile social networking sites and poor academic performance: Antecedents and consequences from stressor-strain outcome perspective. Computers in Human Behavior, 85 , 163–174.
Chawinga, W. D. (2017). Taking social media to a university classroom: Teaching and learning using Twitter and blogs. International Journal of Educational Technology in Higher Education., 14 (3), 2–19.
Doleck, T., Bazelais, P., & Lemay, D. J. (2018). Social networking and academic performance: A generalized structured component approach. Journal of Educational Computing Research, 56 , 1129–1148.
Doleck, T., & Lajoie, S. (2018). Social networking and academic performance: A review. Education and Information Technologies, 23 , 435–465.
Dumpit, D. Z., & Fernandez, C. J. (2017). Analysis of the use of social media in Higher Education Institutions (HEIs) using the Technology Acceptance Model. International Journal of Educational Technology in Higher Education, 14 (1), 1–16.
Eid, M. I. M., & Al-Jabri, I. M. (2016). Social networking, knowledge sharing and student learning: The case of university students. Computers & Education, 99 , 14–27.
Ellison, N., & Boyd, D. (2013). Sociality through social network sites. In W. Dutton (Ed.), The Oxford handbook of internet studies (pp. 151–172). Oxford University Press.
Felisoni, D. D., & Godoi, A. S. (2018). Cell phone usage and academic performance: An experiment. Computers & Education, 117 , 175–187.
Giunchiglia, F., Zeni, M., Gobbi, E., Bignotti, E., & Bison, I. (2018). Mobile social media usage and academic performance. Computers in Human Behavior, 82 , 177–185.
Haans, R. F. J., Pieters, C., & He, Z. (2016). Thinking about U: Theorizing and testing U-and inverted U-shaped relationships in strategy research. Strategic Management Journal, 37 , 1177–1195.
Hoi, N. V. (2021). Augmenting student engagement through the use of social media: The role of knowledge sharing behaviour and knowledge sharing self-efficacy. Interactive Learning Environments . https://doi.org/10.1080/10494820.2021.1948871
Hsiao, K., Shu, Y., & Huang, T. (2017). Exploring the effect of compulsive social app usage on technostress and academic performance: Perspectives from personality traits. Telematics and Informatics, 34 , 679–690.
Huang, C. (2018). Social network site use and academic achievement: A meta-analysis. Computers & Education. , 119 , 76–83.
Ifinedo, P. (2016). Applying uses and gratifications theory and social influence processes to understand students’ pervasive adoption of social networking sites: Perspectives from the Americas. International Journal of Information Management , 36 (2), 192–206.
Junco, R. (2012). The relationship between frequency of Facebook use, participation in Facebook activities, and student engagement. Computers & Education, 58 (1), 162–171.
Junco, R. (2015). Student class standing, Facebook use, and academic performance. Journal of Applied Developmental Psychology, 36 , 18–29.
Junco, R., & Cotten, S. R. (2012). No A 4 U: The relationship between multitasking and academic performance. Computers & Education, 59 , 505–514.
Kane, G. C., Alavi, M., Labianca, G., & Borgatti, S. P. (2014). What’s different about social media networks? A framework and research agenda. MIS Quarterly, 38 (1), 274–304.
Karpinski, A. C., Kirschner, P. A., Ozer, I., Mellott, J. A., & Ochwo, P. (2013). An exploration of social networking site use, multitasking, and academic performance among United States and European university students. Computers in Human Behavior, 29 , 1182–1192.
Koranteng, F. N., Wiafe, I., & Kuada, E. (2019). An empirical study of the relationship between social networking sites and students’ engagement in higher education. Journal of Educational Computing Research, 57 (5), 1131–1159.
Lampe, C., Wohn, D. Y., Vitak, J., Ellison, N. B., & Wash, R. (2015). Student use of Facebook for organizing collaborative classroom activities. Computer Supported Collaborative Learning, 6 , 329–347.
Lau, W. W. F. (2017). Effects of social media usage and social media multitasking on the academic performance of university students. Computers in Human Behavior , 68 , 286–291.
Lemay, D. J., Bazelais, P., & Doleck, T. (2020). Patterns of social networking use and academic performance: Examining the link between quality and frequency of social networking use and academic performance among college-level students. Education and Information Technologies . https://doi.org/10.1007/s10639-019-10065-7
Lepp, A., Barkley, J. E., & Karpinski, A. C. (2015). The relationship between cell phone use and academic performance in a sample of US college students. SAGE Open . https://doi.org/10.1177/2158244015573169
Lind, J. T., & Mehlum, H. (2010). With or without U? The appropriate test for a U-shaped relationship. Oxford Bulletin of Economics and Statistics, 72 , 109–118.
Liu, D., Kirschner, P. A., & Karpinski, A. C. (2017). A meta-analysis of the relationship of academic performance and social network site use among adolescents and young adults. Computers in Human Behavior, 77 , 148–157.
Manca, S., & Ranieri, M. (2016). Facebook and the others. Potentials and obstacles of social media for teaching in higher education. Computers & Education, 95 , 216–230.
Marker, C., Gnambs, T., & Appel, M. (2018). Active on Facebook and failing at school? Meta-analytic findings on the relationship between online social networking activities and academic achievement. Educational Psychology Review. https://doi.org/10.1007/s10648-017-9430-6
Masrom, M. B., Busalim, A. H., Abuhassna, H., & Mahmood, N. H. N. (2021). Understanding students’ behavior in online social networks: A systematic literature review. International Journal of Educational Technology in Higher Education, 18 (1), 1–27.
Nie, N. H. (2001). Sociability, interpersonal relations, and the Internet: Reconciling conflicting findings. American Behavioral Scientist, 45 , 420–435.
Park, E., Song, H., & Hong, A. J. (2018). The use of social networking services for classroom engagement? The effects of Facebook usage and the moderating role of user motivation. Active Learning in Higher Education. https://doi.org/10.1177/1469787418809227
Phua, J., Jin, S. V., & Kim, J. J. (2017). Uses and gratifications of social networking sites for bridging and bonding social capital: A comparison of Facebook, Twitter, Instagram, and Snapchat. Computers in Human Behavior, 72 , 115–122.
Podsakoff, P. M., MacKenzie, S. B., Lee, J. Y., & Podsakoff, N. P. (2003). Common method biases in behavioral research: A critical review of the literature and recommended remedies. Journal of Applied Psychology, 88 (5), 879–903.
Raza, S. A., Qazi, W., Umer, B., & Khan, K. A. (2020). Influence of social networking sites on life satisfaction among university students: A mediating role of social benefit and social overload. Health Education, 120 (2), 141–164.
Samad, S., Nilashi, M., & Ibrahim, O. (2019). The impact of social networking sites on students’ social wellbeing and academic performance. Education and Information Technologies, 24 (3), 2081–2094.
Sarwar, B., Zulfiqar, S., Aziz, S., & Ejaz, C. K. (2019). Usage of social media tools for collaborative learning: The effect on learning success with the moderating role of cyberbullying. Journal of Educational Computing Research, 57 , 246–279.
Simonsohn, U. (2018). Two lines: A valid alternative to the invalid testing of U-shaped relationships with quadratic regressions. Advances in Methods and Practices in Psychology Science, 1 , 538–555.
Smith, E. E. (2017). Social media in undergraduate learning: Categories and characteristics. International Journal of Educational Technology in Higher Education, 14 (1), 1–24.
Tafesse, W. (2020). The effect of social networking site use on college students’ academic performance: The mediating role of student engagement. Education and Information Technologies, 25 , 4747–4763.
Tokunaga, R. S. (2016). An examination of functional difficulties from Internet use: Media habit and displacement theory explanations. Human Communication Research, 42 , 339–370.
Wang, Y., Niiya, M., Mark, G., Reich, S.M., & Warschauer, M. (2015). Coming of age (digitally): An ecological view of social media use among college students. Paper presented at the proceedings of the 18th ACM conference on computer supported cooperative work & social computing. 571–582.
Weisberg, S. (2005). Applied linear regression . Wiley.
MATH Google Scholar
White, H. (1980). A heteroskedasticity-consistent covariance matrix estimator and a direct test for heteroskedasticity. Econometrica, 48 , 817–838.
MathSciNet MATH Google Scholar
Zhang, Y., & Leung, L. (2015). A review of social networking service (SNS) research in communication journals from 2006 to 2011. New Media and Society, 17 (7), 1007–1024.
Download references
Acknowledgements
I would like to thank Zeleke Siraye and Elias Shitemam for their support in collecting the data for this study.
Funding Acknowledgement: This research is supported by United Arab Emirate University research grant. (Grant code: G00003359; Funding Number: 31B125).
Author information
Authors and affiliations.
United Arab Emirates University, Al Ain, United Arab Emirates
Wondwesen Tafesse
You can also search for this author in PubMed Google Scholar
Corresponding author
Correspondence to Wondwesen Tafesse .
Ethics declarations
Competing interests.
The authors declare that they do not have any competing interest.
Additional information
Publisher's note.
Springer Nature remains neutral with regard to jurisdictional claims in published maps and institutional affiliations.
Appendix: Illustration of custom reports produced by App Usage
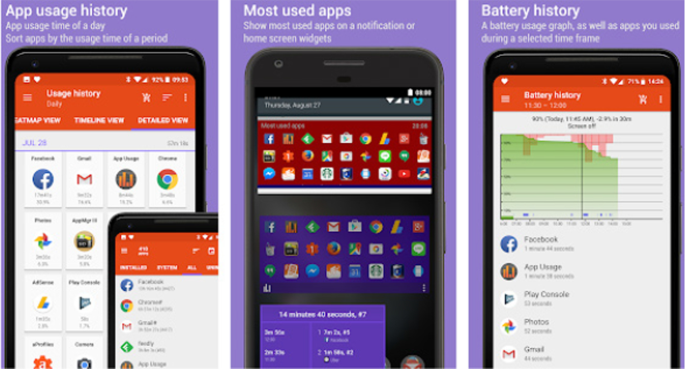
Rights and permissions
Open Access This article is licensed under a Creative Commons Attribution 4.0 International License, which permits use, sharing, adaptation, distribution and reproduction in any medium or format, as long as you give appropriate credit to the original author(s) and the source, provide a link to the Creative Commons licence, and indicate if changes were made. The images or other third party material in this article are included in the article's Creative Commons licence, unless indicated otherwise in a credit line to the material. If material is not included in the article's Creative Commons licence and your intended use is not permitted by statutory regulation or exceeds the permitted use, you will need to obtain permission directly from the copyright holder. To view a copy of this licence, visit http://creativecommons.org/licenses/by/4.0/ .
Reprints and permissions
About this article
Cite this article.
Tafesse, W. Social networking sites use and college students’ academic performance: testing for an inverted U-shaped relationship using automated mobile app usage data. Int J Educ Technol High Educ 19 , 16 (2022). https://doi.org/10.1186/s41239-022-00322-0
Download citation
Received : 11 November 2021
Accepted : 12 January 2022
Published : 28 March 2022
DOI : https://doi.org/10.1186/s41239-022-00322-0
Share this article
Anyone you share the following link with will be able to read this content:
Sorry, a shareable link is not currently available for this article.
Provided by the Springer Nature SharedIt content-sharing initiative
- Social media
- Mobile applications
- Academic performance
- Inverted U-shape
- Curvilinear relationship

An official website of the United States government
The .gov means it’s official. Federal government websites often end in .gov or .mil. Before sharing sensitive information, make sure you’re on a federal government site.
The site is secure. The https:// ensures that you are connecting to the official website and that any information you provide is encrypted and transmitted securely.
- Publications
- Account settings
Preview improvements coming to the PMC website in October 2024. Learn More or Try it out now .
- Advanced Search
- Journal List

Media use, attention, mental health and academic performance among 8 to 12 year old children
Pedro cardoso-leite.
1 University of Luxembourg, Department of Behavioral and Cognitive Science, Esch-sur-Alzette, Luxembourg
Albert Buchard
2 Université de Genève, Faculté de Psychologie et Sciences de l’Education (FPSE), Geneva, Switzerland
3 Campus Biotech, Geneva, Switzerland
Isabel Tissieres
Dominic mussack, daphne bavelier, associated data.
All relevant data and code are available on https://osf.io/aj2bc/ .
The rise in digital media consumption, especially among children, raises the societal question of its impact on cognition, mental health and academic achievement. Here, we investigate three different ways of measuring technology use-—total hours of media consumed, hours of video game play and number of media used concurrently—-in 118 eight-to-twelve year-old children. At stake is the question of whether different technology uses have different effects, which could explain some of the past mixed findings. We collected data about children’s media uses as well as (i) attentional and behavioral control abilities, (ii) psychological distress, psychosocial functioning, and sleep, and (iii) academic achievement and motivation. While attentional control abilities were assessed using both cognitive tests and questionnaires, mental health and sleep were all questionnaire-based. Finally, academic performance was based on self-reported grades, with motivational variables being measured through the grit and the growth-mindset questionnaires. We present partial correlation analyses and construct a psychological network to assess the structural associations between different forms of media consumption and the three categories of measures. We observe that children consume large amounts of media and media multitask substantially. Partial correlation analyses show that media multitasking specifically was mostly correlated with negative mental health, while playing video games was associated with faster responding and better mental health. No significant partial correlations were observed for total hours on media. Psychological network analysis complement these first results by indicating that all three ways of consuming technology are only indirectly related to self-reported grades. Thus, technology uses appear to only indirectly relate to academic performance, while more directly affecting mental health. This work emphasizes the need to differentiate among technology uses if one is to understand how every day digital consumption impacts human behavior.
Introduction
Digital media consumption (e.g., watching videos, listening to music, playing video games) has increased drastically over the past decades. In the US, 8–12 year old children spend an average of almost 6 hours on digital media every single day [ 1 ] with a substantial fraction of that time spent on multiple media at the same time [29% for 7th to 12th graders; [ 2 ]]. In the European Union, 10–14 year olds spend an average of 2.8 hours a day on digital screens; for 15–19 year olds that number increases to about 3 hours per day [ 3 ]. These numbers reflect the ubiquitous role that digital media has come to play in our lives, a role that is very likely to keep growing both in terms of the magnitude and the diversity of digital media consumption. This state of affairs raises increasing concerns about the impact of digital media consumption, in particular among children.
The emerging literature on the impact of media use on cognition paints a rather complex picture whereby different media have distinct, and possibly opposite effects. For example video games have been shown to have different effects on various cognitive dimensions depending on the specific game genre played [ 4 , 5 ]. Children’s media consumption is frequently assessed through total time on media as if all forms of media represented a unitary experience. Yet, this is clearly not the case [ 6 ]. Research increasingly suggests that the impact of digital media use on cognition, academic performance or health is complex [e.g., 7 , 8 ], as it depends on the type of media (e.g., video games, social networks), its content (e.g., fantasy, documentary), the context (e.g., alone, in groups) and the traits of the person consuming media [e.g., age, gender]. To understand how digital media impacts children’s attentional and behavioral control, mental health and academic achievement requires a finer grained approach [ 9 – 11 ].
The present work builds on this recognized need for a finer grained approach. First it presents new experimental data which takes a more granular approach to media consumption by investigating three media consumption indices—-total time on media, media multitasking and video game play. Second, this work assesses the relationships of these forms of media consumption with children’s cognition as measured not only through surveys but also task-based measures of attentional and behavioral control; the latter providing finer measures of cognition than surveys. In addition, mental health, and school related variables were also collected on the same children, allowing us to evaluate the relative association strength of different media consumption types on different aspects of children’s lives. The third contribution of this work is methodological. Studying the impact of media on humans poses a number of challenges: many of the variables considered in this research field correlate with each other (e.g., total hours on media correlates with the amount of media multitasking) and there are yet no clear, established causal models in this emerging field (e.g., does multitasking increase impulsivity or do impulsive people multitask more?). We apply psychological networks—a relatively new modeling technique—and discuss how it provides valuable insights that complement the more traditional pairwise correlations, linear regressions or mediation analyses.
Previous studies
The current cognitive literature suggests that different forms of media consumption have different relationships and possibly casual effects on human cognition. In particular, while playing action video games has been linked to enhanced cognition, and in particular attentional control, media multitasking has been linked to worse attentional control, greater distractibility or attention lapses. Such contrasting relationships also call into question the rationale of considering all screen times in an undifferentiated way as is common in the literature. This work therefore focuses on three measures of media usage: total time on media, media multitasking and video gaming. We review in turn their respective relationships with cognition, mental health and a few school related variables, among which academic performance.
Total time on media
Many studies report that total time on media (all types of media or all screen based media) is associated with adverse attentional and behavioral outcomes [e.g., 12 – 15 ] and in particular Attention-Deficit/Hyperactivity Disorder (ADHD) symptoms. A two year longitudinal study on more than three thousand 15–16 year olds reported that a higher frequency of media use at baseline was associated with a subsequent increase in ADHD symptoms [ 16 ]. Similarly, a meta-analysis on the relation between total time on media and ADHD-related behaviors reported a small but significant association [ 17 ], as measured by either cognitive tasks, surveys, or observations.
Total time on media has also been linked to various mental health problems. Limtrakul et al. [ 18 ], for example, explored the relationships between self-reported media use variables and psychosocial health—as measured by the Strengths and Difficulties Questionnaire [ 19 ] which has been linked to Problematic Media Use [ 20 ] among 10–15 year olds. Their results suggest that larger amounts of total time spent on media is associated with lower prosocial behaviour scores (measured with items like: the child is “kind to younger children”). More time on digital technology has also been linked to decreased well-being in adolescents. Indeed, Orben & Przybylski (2019) documented such a negative tendency using several large-scale datasets [ 21 ]. Yet, these authors also stressed that this relationship is so small as to be negligible.
The relationship between total time on media and school related variables is less clear, with large-scale studies reporting somewhat contradictory results [e.g., 22 , 23 ]. A recent meta-analysis on the relationship between overall “screen” media use (i.e., computer, internet, mobile phone, television, video game) and academic performance among children and adolescents (4–18 year olds) reported no relationship between total time on digital media and academic performance [ 7 ]. This same study however, reported that both larger amounts of time spent watching television or playing video games were associated with lower academic performance.
Media multitasking
Media multitasking, the simultaneous use of multiple digital media (e.g., listening to music while surfing the web), is both an expanding, recent societal phenomenon [ 1 , 24 , 25 ] and an active research topic since the seminal study by Ophir et al. [ 26 ] reported that young adults who media multitask heavily exhibited impairments in suppressing distractions across multiple cognitive tests.
Media multitasking has been associated with a broad range of cognitive impairments [ 27 – 30 ], most notably in attentional and behavioral control—in particular top-down control of attention, such as inhibiting distractions or avoiding attention lapses, and behavioral control such as avoiding impulsive behavior. Indeed, media multitasking has been associated with higher scores on ADHD surveys [ 31 – 33 ], and higher levels of impulsivity [ 32 , 34 – 36 ] and mind-wandering [ 37 ], but see [ 38 ]—-in line with these results, Kobayashi et al. [ 39 ] reported differences in functional connectivity of the dorsal attentional network when comparing heavy and light media multitaskers. Furthermore, Ophir et al. [ 26 ] reported that heavy media multitaskers performed worse than light media multitaskers on a range of cognitive tasks, including working memory, task switching and selective attention tasks. A recent neuroimaging study further points to altered memory retrieval in high media multitaskers, owing to more frequent attentional lapses during the processing of memory retrieval cues [ 40 ]. Other studies did not always replicate these results [ 34 , 41 – 43 ] or suggested that the relationships between levels of media multitasking and cognitive performance may be non-linear [ 44 , 45 ]. The results seem clearer when using surveys and self-reports rather than computerized tests [ 31 ]: media multitasking has been associated with deficits in self-reported everyday executive and attentional functions [ 31 , 37 , 46 , 47 ] and could be particularly detrimental at younger ages where executive functions still develop [ 48 ]; see also [ 49 ].
Media multitasking has also been associated with mental health problems. Becker et al. [ 50 ] for instance, reported that media multitasking was positively correlated with depression and social anxiety scores, even after controlling for total time on media and personality traits. High levels of media multitasking have been linked to less sleep, difficulties to fall asleep at night and to keep awake during the day, at school [ 51 , 52 ]. However, a longitudinal study found no temporal association between media multitasking and sleep [ 53 ], suggesting that the relationship between media multitasking and sleep might not be a direct causal one.
Finally, media multitasking has been linked to negative academic performance and other school related variables . Some studies for instance report that heavy media multitaskers are less efficient academic learners [ 54 ] and may have less grit [ 50 ]—-the ability to maintain perseverance in otherwise aversive tasks, which seems important for academic success [ 55 ]. Cain et al. [ 56 ] studied 12–16 year olds and reported that heavy media multitasking was associated with lower academic performance on standardized tests (Math and English) but also with lower performance on computerized executive functions tests and higher impulsivity, along with lesser growth mindset (but neither grit nor conscientiousness, in contrast to other studies mentioned), suggesting that media multitasking is a critical variable to consider when investigating the effects of media [see also 57 , 58 ].
Video game play
Several meta-analyses document a positive impact of specifically action video games (AVG)–as compared to other types of video games–on cognition [e.g., 59 – 63 , but see 64 ]. Playing AVG has been frequently related to improved attentional control and in particular improved top-down (but not bottom-up) attention [ 63 ]. AVG, defined in this literature as those in the first or third-person shooter genres, appear to have a greater positive impact on cognition than other types of video games [ 63 ]. In cross-sectional studies of attentional control on 7–22 year old participants who were classified as either being AVG players or non-video game players, [ 65 ] observed systematic attentional advantages in the AVG players group. These results are further supported by a few intervention studies on children. For example, Franceschini et al. [ 66 , 67 ] trained 7–13 years old dyslexic children using various mini-games for 12 hours, distributed over multiple days. The experimental group played mini-games that used action video game mechanics while the control group played mini-games that did not share those features [ 4 , for a discussion of action game features, see 68 ]. The results showed an improvement in attention (and in reading) only for the experimental group that trained with action-like mini-games. The positive relationship between action video game and cognition is unlikely to hold for video games at large. Indeed, the bulk of intervention studies using action video games makes it clear that not all video games have the same impact on cognition.
The relationship between video game play and mental health are somewhat mixed. A large-scale study (N = 2442), on 7–11 year old children for instance, reported that large amounts of gaming (more than 9 hours per week)—but not smaller amounts—were associated with increased conduct problems and reduced prosocial behavior [ 69 ]. Similar conclusions seem to hold in older children [10-15 year-olds; [ 70 ]]: compared to children who do not play video games at all, children who play daily for more than 3 hours presented less prosocial behaviors, more conduct problems and decreased life-satisfaction. Children who played between 1 and 3 hours per day were equivalent in those measures as children who did not play at all. Surprisingly however, playing less than 1 hour per day was linked to the opposite pattern of results, suggesting that small amounts of video gaming might in fact have positive effects [ 70 ]. This hypothesis is corroborated by a European study on more than three thousand 6–11 year-olds [ 71 ] which reported no sign of increased mental health problems as a function of video game play and instead, suggested that gaming might have a protective effect against difficult social relationships.
Finally, there have also been mixed results on the relationship between playing video games and school related variables . After correcting for multiple demographic and trait-level variables, larger amounts of video gaming was linked with greater intellectual functioning and school achievement (as rated by the child’s teacher) relative to other children in the class [ 71 ]. Similarly, Pujol et al. [ 69 ] found a positive association between game play and the teacher’s rating of school achievement, but no trend with the number of hours played [see also 72 ]. The relationship between video gaming and academic performance remains, however, unclear [ 73 ]: it can be positive, negative or absent depending on various factors (e.g., playing during weekdays versus weekend days [ 74 ]; playing before versus after school [ 75 ]). The relationship between gaming and academic outcomes might be U-shaped rather than monotonic, and might for instance depend on the type of games played [ 76 ]. There are some reasons to believe that action video games in particular might benefit educational outcomes [ 66 , e.g., 77 , 78 ].
The present study
The reviewed literature shows that different forms of media consumption may affect attentional/behavioral control, mental health and school related variables in different ways. Given the ubiquity of digital media in our lives and the concern that their potential adverse effects may be amplified in younger children, it is imperative to further our understanding of these effects and how they relate to each other.
The reviewed literature is filled with hypotheses about the potential causal relationships between any two constructs, but oftentimes the data to support specific claims is simply missing [ 79 ]. It is unclear, for example, why exactly total time on media should correlate with attentional/behavioral control, mental health or school related variables. Total time on media is a rough measure of media consumption and there are potentially many confounding variables (e.g., media multitasking habits) which might be responsible for the observed associations. Furthermore, total time on media might have only an indirect effect. For instance, total time on media may affect attention, mental health and school related variables via its negative effects on sleep [ 80 ], which might be the real cause of decreased cognitive functioning, mental health and academic performance [e.g., 81 , 82 ]. It appears then, that in order to gain insights into the underlying relationships it is necessary to collect for each participant a larger set of measures covering both different aspects of their media use habits, but also aspects of their cognitive functioning, their mental health and, in the case of children, school related variables. This insight motivated the design of the present study. Note that cross-sectional data (as reported here and in most of the cited literature) is fundamentally limited in its ability to support causal claims; specific causal relationships are best established within intervention studies.
The second important insight is methodological and concerns how to best analyse such multivariate data. Previous cross-sectional research mostly used correlation and linear regression analyses to highlight the presence of a (positive or negative) relationship between some form of media use and a variable of interest. Correlations between pairs of variables may be misleading because those correlations might be explained by other variables. Linear regression on the other hand, implicitly assigns causal roles to the variables. Indeed, regressing, for example, academic performance on total hours of media is different from regressing total hours of media on academic performance and seems to suggest that total hours of media causes changes in academic performance. There are of course more advanced multivariate models which adequately treat measures as such (rather than implicitly assuming that some variables are measurement-free predictors, as is the case in linear regression) and explicitly define the directionality of the influence of the variables (most notably, structural equation models which include mediation and moderation models). However, there is currently no clear understanding of the relationships that might exist between the various constructs to justify a particular causal structure for such models.
This research field is currently characterized by both an ubiquity of correlations among variables and a paucity of evidence for specific causal relations among them. In this context, the method known as psychological network analysis [ 83 , 84 ] seems particularly useful. This method is analogous to partial correlation analyses in that it attempts to evaluate the specific association between pairs of variables, albeit within the context of a network of variables. Using this method one may evaluate, for instance, whether there is a direct link between total media time and self-reported grades or whether the data is compatible with the hypothesis that total media time has an indirect effect on grades by reducing the amount or quality of sleep. Under certain assumptions, the presence of a direct association between two variables in a psychological network may be indicative of a causal relationship between them; however the directionality of the relationship remains unspecified. When there are many possible variables under investigation and no clear theoretical model, the associations highlighted in psychological networks might provide relevant starting points for future studies to investigate causality experimentally. While at this stage, this type of analysis is mostly exploratory, it offers a new perspective on previously reported effects and may constitute a promising avenue going forward.
In this study we collected data on three different self-reported measures of media use (total time on media, media multitasking and video game play) as well as a collection of measures that have been highlighted in past research; these measures cover attentional/behavioral control, mental health and school related variables. These questions were addressed in the 8–12 years age range, a particularly vulnerable time period of development for identity formation, socio-emotional development and, importantly for this work, further maturation of cognitive skills such as executive functions [ 52 , 85 ]. In addition, it is during this age range that children transition to a more independent use of digital media, making it a specially interesting age range for researchers [ 52 , 65 ]. This dataset includes a large set of variables for the same subjects, that is 156 eight-to-twelve year-old children. Although a larger sample size would always be welcomed, such multivariate dataset to qualify media usage in children remains rare in the literature.
We first probe the specificity of previously reported pairwise relationships between variables using rather traditional methods, such as partial correlation analysis, before using psychological network analysis. This two-step approach allows us to relate our results to past research while also providing new insights.
Ethics review and consent
The study was approved by the Ethics Review Commission of the University of Geneva. Parents provided written consent for their children to participate in this study.
Participants
This study was conducted in a public primary school in the suburbs of Geneva, Switzerland. The school had expressed interest in participating in scientific studies. Children were recruited through teachers volunteering. These children were between 8 and 12 years old and were either in grade levels 5P to 8P (corresponding roughly to grades 3 to 6 in the United States [ 86 ]) or were part of a special needs class (we did not record or have access to any data further details characterizing children in this class). No inclusion/exclusion criteria were applied at data collection time. For the psychological network analyses reported below, we did not know what effect sizes to expect–instead we thrived to collect data from as many participants as we could, knowing that we would easily exceed the few tens of participants that are common in this type of study.
From the 226 children in the targeted classrooms, we obtained parental consent for 156 children (84 boys and 72 girls). Data from these 156 children are available on https://osf.io/aj2bc/ . For the purposes of this study, we excluded from further analyses children from the special needs class (n = 16), children who did not complete the media questionnaire (n = 21). One additional child was excluded from further analyses because their reported number of daily hours on media (almost 35 hours) was much larger than for the remaining children (the second largest number was 17.5 hours)—note that it is possible to exceed 24 hours of media per day by consuming multiple media at the same time). This procedure ultimately led to an effective sample size of 118 children (57 girls, 61 boys, with a mean age of 10.38 years (SD = 1.16)). Note however that because of the multi-session nature of the study, some data are missing (e.g., children or their parents failed to complete one or more of the questionnaires or tests)—in the analyses below we report sample sizes for specific variables when relevant.
We collected data via paper-and-pencil questionnaires completed by the parents and teachers of the children enrolled in this study as well as via cognitive tasks that were completed by the children in their classroom during school time. Below we list the surveys and cognitive tasks that were used.
We included a large amount of paper-and-pencil surveys to span a wide range of dimensions that might be relevant within the scope of this study. This selection included standard surveys from the literature but also custom-made questions that are more exploratory. The surveys were all administered in French translations of their original versions (our translations are available to readers by request). Children were asked to complete these questionnaires with their parents unless otherwise noted.
Questionnaires
In addition to a general demographic questionnaire—which asked children about their birthdate, gender, handedness, number of siblings, self-reported health state, the languages spoken at home, as well as yes/no questions about difficulties in vision, audition, learning and verbal comprehension and expression—the questionnaires included in this study cover broady speaking four categories: digital technology use; attentional problems; mental health and sleep; grades, motivation and beliefs. Below we present these questionnaires briefly; for more details, see the S1 File .
Digital technology usage . The media multitasking inventory is an adapted version of the media multitasking questionnaire [ 26 ]. We used three main measures from this questionnaire: the total number of hours of media content consumed per day, the media multitasking index (as defined in our study) and the total number of hours of video gaming per day.
The video gameplay questionnaire asks about which video games children play, on what device and how frequently (“often,” “sometimes,” “rarely”). Combined with the reported number of hours of video gaming from the media questionnaire, this survey provides an estimate of how much time is spent on each game category.
Attentional problems . The Conners Teacher’s Rating Scale [ 87 ] requires teachers to evaluate their children’s school behavior and leads to a score, where a higher value is interpreted as having overall more ADHD-like behavior (e.g., difficulty paying attention or impulsive behaviors).
The Conners Parent’s Rating Scale is similar but is filled out by the child’s parents.
We assessed mind-wandering or the frequency of task unrelated thoughts using the 4-item short Mind-Wandering Questionnaire (MWQ) [ 88 ].
Mental health and sleep The K-6 distress scale [ 89 ] evaluates non-specific psychological distress with items relating to anxiety and depression; a higher score reflects higher levels of emotional distress.
This Strength and Difficulties questionnaire [ 19 ] covers 5 dimensions of children’s behaviors, emotions, and relationships and provides a total score which reflects general difficulties, encompassing both emotional and behavioral problems.
We also included a custom-made sleep questionnaire from which we compute a score, with higher values indicating better sleep and less fatigue.
Grades, grit and mindset . The custom-made grades questionnaire asked children to self-report their grades (the question translates to “what do you think is your average general grade at school?”), and their grade satisfaction (“I have good grades at school” with responses on a four-point Likert scale going from yes to no).
The grit questionnaire measures perseverance and passion for long-term goals [ 55 ], with a higher score corresponding to greater perseverance.
Finally, the Theory of Intelligence (or mindset) questionnaire measures childrens’ beliefs about the potential of intelligence to improve [ 90 ]; a higher score indicates a “growth mindset” or a stronger belief that intelligence can be improved.
Cognitive tests
We report here 3 of the 5 cognitive tasks completed by the children in this study (one task was excluded because technical problems compromised the integrity of the data, and the other because it was part of an exploratory study that is unrelated to the present study). Each of these 3 tasks taps mostly attentional processes and gives rise to three main measures (for a total of 9 measures across the three tasks): a response speed index (how fast children perform), an inattention index (how often they fail to respond when they should have) and an impulsivity index (how often they respond when they shouldn’t have). These indices were then z-scored within tasks and averaged across tasks to provide an overall score on speed, inattention and impulsivity. Below we present these tests briefly; for more details, see the S1 File .
D2 cancellation task . The D2 task is a paper and pencil task designed to measure selective attention [ 91 ]. Participants were given a sheet of paper filled with symbols composed of the letters “d” or “p” with zero, one or two bars above and/or below the letter. Children were orally instructed to circle every symbol that comprises the letter “d” that is surrounded by exactly two bars (e.g., one above and one below; two above and none below).
Sustained attention to response task (SART) . In the SART task [ 92 ] a digit (1–9) appears for 250 ms on the screen center every 1.150ms and children are instructed to tap on the screen in response to any digit except the digit “3.”
Bron lyon attention stability task (BLAST) . In the BLAST task [ 93 ], children are first shown a single target letter (e.g., “A”) for 250ms, followed 500ms later by a 2x2 array of letters that did (e.g., “A, K, B, R”) or did not (e.g., “X, K, B, R”) contain the target letter. They were asked to report on each trial whether or not the target letter was present in the array.
The teachers distributed and collected the consent forms from the parents at the beginning of the school year. Parents could fill out the questionnaires at home with their child and bring them back to the school once they were completed. Teachers also filled out a questionnaire about each of their pupils whose parents consented to the study.
Given the large number of questionnaires and cognitive tests involved in this study, data collection was split into three sessions (January, March and May). In each session, different questionnaires and cognitive tests were completed.
The cognitive tests reported in this study were all conducted in a classroom setting where groups of 14 to 16 children were tested in a classroom setting under the supervision of at least two experimenters and a teacher. Each child was given their own tablet, pencil and paper on which the tests were implemented. They were seated at a table to complete the pencil and paper tests as well as computerized cognitive tests and were allowed to set the screen distance or position as they wished. This data collection procedure was motivated both by practical considerations and theoretical ones. At the practical level, it allowed for more efficient data collection. At the theoretical level, it allowed us to measure cognition in a real-life situation rather than an artificial lab setting. In doing so, our data collection is better aligned with our interest in understanding how media use affects everyday cognitive functioning. Each classroom test session lasted about 40 minutes. ## Code
Data processing, analyses and visualization were run in R version 3.6.0 (2019–04-26) [ 94 ], using the packages tidyverse [ 95 ], lme4 [ 96 ], lmerTest [ 97 ] and bootnet [ 84 ]. For further details, see the S1 File . The code and data for this study are available on https://osf.io/aj2bc/ .
Reliability and descriptive statistics are reported in the S1 File . Here we first briefly describe some key results about media usage among 8 to 12 year old children before evaluating specific relationships between different forms of media usages, attention, mental health and school related measures.
Media consumption by age and gender
Total hours of media consumed per day increases with age (Spearman correlation r = 0.35, p < 0.001). At age 8, children consume on average 4 hours and 28 minutes of media per day; at age 12, that number increases to 8 hours and 14 minutes per day. For each additional year of age, total hours of media consumed increases by almost a full hour.
The total amount of media consumed does not differ among boys and girls (Wilcoxon rank sum test with continuity correction, W = 1669, p = 0.71). This result is confirmed by a linear mixed effects analysis on the hours spent per media by gender (F(1, 116) = 0.003, p = 0.957). Yet, this same analysis also shows that some media are consumed more than others (F(7, 812) = 16.1, p < 0.001) and differently by boys and girls (interaction effect, F(7, 812) = 5.6, p < 0.001). More specifically, boys spend more time on video games than girls (1.1±0.12 h/day versus 0.47±0.08 h/day; Wilcoxon rank sum test with continuity correction, W = 846, p < 0.001; gender differences for other media are smaller and would not resist multiple comparison correction).
Media multitasking also increases with age (Spearman correlation: r = 0.34, p < 0.001). At age 8, the media multitasking score—-which refers to the average number of additional media used while using a primary medium (a score of 0 meaning each medium is always consumed in isolation)—-is 0.66; at age 12, it increases to 1.61. For each additional year of age, the average number of additional media used simultaneously while using media increases by about 0.24.
Finally, there is no difference in media multitasking scores between boys and girls (Wilcoxon rank sum test with continuity correction, W = 1597, p = 0.613).
Within the age range studied, the number of hours spent on video games each day does not increase with age (Spearman correlation, r = 0.04, p = 0.695). Overall, boys spend more time on video games than girls do (mean±SEM: 1.1±0.12 versus 0.47±0.08 hours per day; Wilcoxon rank sum test with continuity correction, W = 846, p < 0.001). A linear mixed effects model on the hours of daily video gaming yields a significant interaction effect between gender and whether the games played were action-like or not (F(1, 186) = 12.6, p < 0.001): boys play more action-like video games than girls (W = 347, p < 0.001; 0.68±0.1 versus 0.13±0.05 hours per day) but there is no difference between them when considering time spent on other games (W = 1173.5, p = 0.535; 0.41±0.08 versus 0.5±0.1 hours per day).
Attentional performance by age and gender
As children get older, response speed increases (Spearman correlations: r = 0.52, p < 0.001), impulsivity decreases (i.e., tendency to make false alarms; r = -0.22, p = 0.029), and inattention decreases numerically although not in a statistically significant way according to the Spearman correlation test (i.e., miss rates; r = -0.15, p = 0.133; see Fig 1 ). To evaluate the effect of gender, in addition to age, we also ran linear regressions on the three cognitive indices (regressing separately the three cognitive indices on age, gender and their interaction). These analyses show a significant effect of age on speed (F(1,95) = 38.8, p < 0.001), on impulsivity (F(1,95) = 3.65, p = 0.059) and on inattention (F(1,95) = 5.14, p = 0.026). The effect of gender was significant on impulsivity (F(1,95) = 6.29, p = 0.014; with boys being more impulsive than girls) and on inattention (F(1,95) = 5.14, p = 0.026; with girls performing better) but not on response speed (F(1,95) = 3.22, p = 0.076). Finally, in none of the measures did we observe an interaction between age and gender (all p >= 0.208).

Partial pairwise Spearman correlations
Total media time and media multitasking.
As many of the variables are correlated—for example, response speed correlates with total hours of media consumed (r = 0.26, p = 0.008) but both response speed and total hours of media also correlate with age (r = 0.52, p < 0.001 and r = 0.35, p < 0.001)—it is not straightforward to interpret correlations between any pair of variables. We thus use partial correlations in an attempt to evaluate the specific relationship between two variables when controlling for age and other types of media use.
We computed the correlation between total hours of consumed media and each of our variables of interest while controlling for media multitasking and age and gender; we also did the reverse, i.e., compute the partial correlation between media multitasking and the variables of interest while controlling for total hours of media, age and gender. This procedure is justified by the fact that media multitasking and total hours of media are strongly correlated (r = 0.48, p < 0.001).
The partial correlation profiles corresponding to these two cases are shown in Fig 2 . Clearly, these results show no correlation between total hours of media and any of the measures of interest when controlling for age, gender and media multitasking. However, when controlling for age, gender and total hours of media, we observe relationships between media multitasking scores and most self-reported measures. High levels of media multitasking are linked to higher levels of distress (K6), lower socioemotional functioning (SDQ), more behavioral and attentional problems as measured by both Conner’s Parents and Conner’s Teachers, as well as a reduced quality of sleep and lesser grit. No significant partial correlation is observed between media multitasking and mind-wandering, mindset, grades, or any of the cognitive performance measures.

Video gaming
Recall that the reported daily hours of video game play was taken from the media questionnaire and that there was a separate set of questions asking participants to report which games they played and at what frequency. This second questionnaire was used to determine if the games played by the children contained action-like mechanics or not (as well as other video game related data that are not reported here). From our data, we could compute the fraction of time spent on action-like video games versus other games. We then estimated the time spent on those two types of media by multiplying those fractions with the total daily hours of video game play. We excluded from this analysis participants who did not play at all (n = 21) or failed to report which specific games they played (n = 10).
We evaluated how playing video games relates to cognitive measures and found, in agreement with the literature, that video gaming correlates positively with response speed (r = 0.3, p = 0.006) but neither with impulsivity (r = -0.04, p = 0.746) nor inattention (r = 0.03, p = 0.793). The response speed effect appears mostly driven by time on action-like games (r = 0.22, p = 0.046) rather than other types of games (r = 0.13, p = 0.23; all other correlations are not statistically significant).
Next, we looked at the relationships between playing video games (overall and separating action and non-action video games) and our variables of interest, while controlling for age, gender, total hours of media and media multitasking score.
Overall, more time on video gaming is associated with faster response speed in the attentional control tasks (r = 0.26, p = 0.024, n = 77; see Fig 3 ) without, however, any concomitant increase in error rates that could have been indicative of an increased impulsivity or inattention (p > 0.756). The largest effect was observed on the K6 distress scale with more time on video game being associated with lower levels of distress (r = -0.38, p = 0.006, n = 55). These effects were only observed when collapsing all games together; with no clearly dissociable effects between action-like video games and other video games genres. No other reliable effects were observed.

The three panels depict time spent on any kind of video games (left panel) or when considering separately time on action-like (middle panel) and non-action-like video games (right panel).
Psychological network
A generalization of the data analysis approach presented above consists in evaluating the partial correlations between any pair of variables while controlling for all the remaining variables; such a method is sometimes known as psychological network analysis. The resulting patterns of partial correlations may be represented as a network where nodes represent variables and edges the presence of a partial correlation between them [ 84 ]. Nodes connected by an edge therefore indicate a direct relationship between those variables, while indirect relationships are simply any non-direct pathway in the network. The advantage of this approach, compared to Structural Equation Modeling for example, is that it permits to simultaneously take into account a range of variables of interest without having to commit to a particular causal structure, which at this stage of the research remains largely unknown. It provides a picture of the complex relationships between various cognitive, demographic and life-style factors that might be a more accurate depiction of reality and less biased by a particular research agenda.
Description of the technique
Psychological Networks were estimated using the R package bootnet [ 84 ] and R [ 98 ]. The rationale is akin to estimating the partial correlation between each pair of variables while controlling for all remaining ones. However, as the number of possible partial correlations between each pair of variables increases rapidly with the number of variables, there is an increased chance of false positives (when not correcting for multiple testing) or a reduced probability to detect any effect at all (when controlling for multiple testing). An alternative approach, that circumvents these issues, estimates all the partial correlations at once and uses LASSO regularization and the Extended Bayesian Information Criterion to determine which model (with some of the partial correlations set to 0) best accounts for the observed data. There are no p-values associated with specific edges; rather, the estimated network as a whole, highlights the combination of edges that are reliable.
Variables entered into the analysis
Given our limited sample size and in order to limit spurious relationships, we included in this analysis only our primary variables for which we had the largest sample sizes. The eleven variables included in this analysis are the media multitasking score (N = 110), the total number of hours of media consumed each day (“Media Hours,” N = 118), the number of daily hours of video game play (“Gaming,” N = 118), child’s age and gender (as “Female,” N = 118), self-reported grades (“Grades,” N = 117), “Conner’s Teachers” (N = 92), “Sleep” score (N = 116) and the three composite scores from the attentional control task—i.e., “Speed,” “Inattention” and “Impulsivity” (N = 99).
Network analysis
The concentration plot in Fig 4 highlights several noteworthy relationships. First, and as expected, age is strongly associated with the attentional control variables, being linked positively to speed and negatively to false-alarms (i.e., “Impulsivity”) and misses (i.e., “Inattention”). Thus, as expected, older children respond faster, and suffer less from impulsivity and inattention. Second, this analysis also confirms the well-known speed accuracy trade-off. Taking all variables into account, “Speed” is positively associated with “Impulsivity” and “Inattention” as faster participants tend to make more errors. Third, and also as expected, the three measures of technology use are positively related to each other, with time on media (i.e., “Media Hours”) being associated with both higher levels of media multitasking (“MMI”) and more time spent playing video games (“Gaming”). Interestingly, no direct relationship between “Gaming” and “MMI” is observed. Furthermore, there is a positive association with age for both “Media Hours” and “MMI”—indicating greater technology consumption as children get older.

Nodes represent variables; edges represent the relationship between variables that cannot be explained by the remaining variables. The width and saturation of the edges reflect the strength of the relationship; positive associations are highlighted in orange and negative associations in blue (e.g., higher levels of media multitasking (MMI) are associated with being older, higher number of hours of media and worse sleep quality).
Of greater practical interest are the predictors of “Grades.” Both lower “Inattention” and lower “Impulsivity” levels are associated with better grades—“Speed” however is not directly related to grades. Furthermore, grades relate directly to “Teacher Ratings” with, as expected, higher ratings (i.e., higher levels of behavioral issues and attentional deficits) being associated with lower grades. “Teacher Ratings” are associated with “Impulsivity” as measured in the attentional control tasks, which attest to the consistency and validity of these measures. “Teacher Ratings” also relate to the child’s gender—-with girls receiving better ratings than boys-—and to sleep habits-—with poor self-reported sleep satisfaction being associated with worse teacher rated attentional/behavioral problems (i.e., higher “Teacher Ratings” scores).
Technology use is found to have little to no direct relationship with grades, except for MMI—higher levels of MMI are weakly associated with lower grades. Most relationships between media uses and grades appear instead to be indirect: higher levels of MMI are associated with poor sleep and with more hours of media consumed; worse sleep and more hours of media consumed are associated with worse teacher rated attentional/behavioral problems, which in turn are associated with lower grades. Total hours of media is only indirectly related to grades via worse teacher ratings. Finally, there are no clear links between gaming and either grades, teacher ratings, or sleep. Gaming is only associated with increased response speed, albeit weakly.
There are growing concerns about the potential of digital media to negatively impact everyday life functioning, in particular during childhood. These concerns call for empirical data on media use in children that is both granular (i.e., considering separate forms of media use), comprehensive (i.e., considering simultaneously a wide range of possible outcome variables) and which adequately handles the fact that many of these variables correlate with each other. Here we investigated three main aspects of media consumption behavior—total media hours, media multitasking and video gaming—among a population of 8 to 12-year old children. The present work addresses the relationships between these three distinct forms of media consumption and attentional and behavioral control (measured through both cognitive tests and questionnaires), mental health and sleep, grades, grit and mindset.
Our results confirm the well-established observation that as children age, they consume more media. In this representative sample of Swiss children, the amount of media content consumed each day increased steadily by one additional daily hour per year of age. From ages 8 to 12, the daily hours of media consumed increased from about 5 to 9 hours. This is in line with many reports in the literature [ 1 ], including Pea et al. [ 52 ] who used a similar (albeit online) survey on a sample of 3,461 8 to 12 year old girls and reported an average of 6.9 hours of daily total media use. This was concomitant with an increase in the number of media used at the same time by 1.4 additional media. In our work, the average number of additional media that children use when using more than one medium at the same time increased from a value of 0.66 at age 8 to a value of 1.61 at age 12. Notably, girls and boys did not differ in terms of total media time or amount of media multitasking—-unlike other studies reporting that girls media multitask more than boys [ 48 , 99 ]. Girls and boys did however differ in the types of media consumed, with boys reporting larger amounts of video game play, especially those containing action-like mechanics. The extent to which these differences in media consumption foster gender differences in cognitive skills remains an interesting open question [ 100 , 101 ].
Total media time and its limitations as a metric
We argue, as have many before us [ 6 , e.g., 9 – 11 ], that total time on media is not a sufficient metric. Total time on media correlates strongly with measures of more specific forms of media use, each of which having their unique, positive or negative impact. This is apparent in the psychological network analysis where total time on media is among the most connected nodes. Yet, this analysis also highlights the distinctness of media multitasking and video gaming, each of them in separate clusters. The shared variance captured by total time on media appears determinant to account for poor attentional behavior. Children who spend more time on media are more frequently reported by their teachers to manifest ADHD-like behavior. Conceptually, this relationship is in line with past research [ 16 , 17 , 82 ]. Yet, partial correlation analyses reveal that media multitasking might be driving this effect. We observe no significant relationships between total time on media and any of our outcome variables when controlling for media multitasking, age, and gender. Although these results could appear to contradict those published in the past [ 15 , 82 , 102 ], these past studies used total media time without controlling for other types of media consumption. In contrast to total media time, media multitasking is associated with more frequent ADHD-like behavior as rated by their teachers, when controlling for total media time, gender, and age.
Media multitasking versus video game play
The partial pairwise correlations highlight large and significant partial correlations linking media multitasking with numerous adverse, self-reported measures: higher levels of media multitasking were associated with higher levels of psychological distress (K6), lower levels of socioemotional functioning (SDQ), worse behavior and attention ratings by both teachers and parents, worse sleep and lower levels of grit. These results are in line with past research reporting an association between media multitasking and increased depression and anxiety among young adults after controlling for total time on media and various personality traits [ 50 ], or media multitasking and worse socioemotional outcomes and worse sleep among 8- to 12- year old girls [ 52 ]. The psychological network analysis further supports the negative link between media multitasking and worse sleep. Sleep is important because it is known to affect many aspects of our lives, including attentional/behavioral control, mental health and school related variables [for reviews see, [ 103 , 104 ]. In agreement with that literature, in our study, children who report worse sleep both have worse grades and receive less favorable attention and behavior ratings from their teachers. A relationship between higher levels of media multitasking and worse sleep has already been reported several times, both in children [ 52 ] and adolescents [ 51 , 53 ], with some researchers suggesting that sleep is more strongly associated with media multitasking than with total time on media [ 53 ]—this is also the case here, as we observe no relationship between total time on media and sleep.
Contrary to the analysis on media multitasking, both partial correlation analyses on hours of video gaming (controlling for age, gender, total time of media consumed and media multitasking index) and the psychological network analyses revealed no significant adverse associations. More specifically, we observed no significant partial correlation involving video gaming and socioemotional functioning, attention and behavioral issues as rated by teachers and parents, sleep, mind-wandering, grit, growth mindset, grades and either impulsivity and inattention in cognitive tests. Rather, we found positive relationships between time spent playing video games and both faster response speed in our attentional control tests, and reduced levels of psychological distress; indicating that playing video games might have a positive impact on specific measures of cognitive control and mental health. The psychological network analysis (which included only a subset of the variables listed above) depicted a similar pattern of results, showing that more time spent on video games is associated with increased response speed in attention tests, in addition to being a male and spending more time on media overall.
When considering video game play in general, our results are partially in agreement with the literature which so far has yielded mixed results. Pujol et al. [ 69 ] for instance, tested over two thousand 7- to 11- year old children using a somewhat similar protocol to ours: children completed cognitive tests (of attention and working memory) and their parents and teachers filled out questionnaires about children’s video gaming habits, their socioemotional functioning (using the Strength and Difficulties Questionnaire), their sleep and overall school achievement. In agreement with our results, their study shows that playing video games was linked with increased response speed without however affecting overall performance on the cognitive tests. Contrary to our results however, they observed a link between video gaming and sleep (more time on video games was linked to sleeping fewer hours). It remains unclear in the Pujol dataset, whether this relationship may be related to video gaming per se or to other associated variables like media multitasking.
In our study we found no direct relationship between time spent on video games and grades. Both the evidence and the opinions in the literature on how video gaming relates to academic performance are somewhat mixed. Some data on children and adolescents is compatible with video gaming being associated with greater school achievement [ 69 , 71 ], while other suggests either no relationship or a small/moderate negative relationship for those children who play video games before going to school [ 7 , 73 , 75 ]. These mixed results are paralleled by different opinions on what relationship to expect. On the one hand, researchers have argued that some kinds of video games improve cognitive abilities which are thought to be crucial for academic performance [ 105 ]; on the other, researchers expect video gaming to negatively impact academic performance by either taking away time from other activities, impairing sleep or the ability to concentrate in a slow paced school environment [e.g., 75 , 106 ]. The present data do not support the view that video gaming impairs academic achievement as we find no direct relationship between video gaming and grades as well as no direct relationship between video gaming and sleep (we do however see the well-known link between better sleep quality and better grades). Gaming might affect educational performance by improving cognition, albeit indirectly, given the link between video gaming and cognition (increased speed) and the link between cognition and grades (lower impulsivity and inattention are linked to better grades).
While our study does not resolve the many inconsistencies in the field of media and their impact on cognitive functioning, mental health, and school related variables, it clearly highlights a few major points. First, it is absolutely necessary to take into account not only total time on media, but also other, more specific measures of media consumption. Here we considered media multitasking and video game playing (in addition to total media time); it seems highly valuable for future studies to include social media, internet browsing or TV/video watching to cite a few. From this point of view, media multitasking questionnaires could be further exploited to document these different forms of media consumption. Second, in the case of video games, the specific types of games that are played (e.g., action-like versus non-action), how and when they are played (e.g., before or after school; in the morning versus evening) appear to be important factors to consider. As different video games have been shown to differently affect cognition, considering their impact separately may help explain some of the discrepancies in the literature [ 4 , 68 ]. Third, while most empirical research focused on pairwise relationships (e.g., between playing video games and grades), researchers do in fact have implicit or explicit hypotheses of how specifically these variables relate (e.g., gaming improves educational attainment by improving attentional control)—the psychological network analysis presented in this study emerges as a powerful complementary tool to evaluate the plausibility of these hypotheses along with pairwise correlation.
Psychological network analysis as a promising tool to study media effects on humans
The psychological network analysis applied on this data set confirmed several well-known results (unrelated to media use), further underlining the value of the approach. For instance, age was associated with increased attentional control abilities [increased response speed, reduced impulsivity and reduced inattention; [ 107 ]]. Factoring out age, increased response speed was associated with greater impulsivity and greater inattention (as measured by more frequent false alarms and misses, respectively); this pattern of results reflects the well-known speed-accuracy tradeoff phenomenon. We also observed the expected relationships between academic grades and children’s behavioral and attentional problems as rated by their teachers. Inattention, impulsivity, worse teacher rated attentional/behavioral problems and reduced sleep quality were unsurprisingly also directly associated with lower grades [ 108 ]. Worse teacher rated attentional/behavioral problems were in turn associated with inattention, lower sleep quality and being male [e.g., 109 ]. Psychological network analysis therefore appears to be a very powerful tool to shed light on the relationships between multiple variables. It seems particularly well-suited to study the relationships between media consumption, attentional/behavioral control, mental health and academic achievement because many of these variables correlate with each other and their causal relationships remain largely unresolved. Applying psychological network analyses on a larger set of variables might provide a means to untangle some of the past results.
Limitations of the present study
We recognize this study has several important limitations. First, all the data reported here are correlational and as such provide no unequivocal indication about the causal relationships between any of the variables of interest. In particular, our study highlights that different types of media use are far from independent, calling for care when separating out direct relations. For example, media multitasking has been repeatedly associated with a greater tendency to mind-wander [ 37 , but see 38 ] and we did observe such a relationship as well in simple pairwise correlations (pairwise Spearman correlations: r = 0.31, p = 0.012). Yet, we also observed a correlation between mind-wandering and total hours of consumed media (r = 0.31, p = 0.009) which itself correlates with media multitasking (for completeness, note that hours of video gaming does not correlate with mind-wandering; r = 0.16, p = 0.186). Such a pattern of results highlights the fact that it may be problematic to attribute the variations in mind-wandering to media multitasking rather than to total hours of consumed media. Causal studies would certainly be highly valuable but may not be ethical given some of the negative impacts reported here and in the broader literature.
Second, given the number of tests and surveys used in the present study, it would have been desirable to include data from a larger population. For instance, while we have a nested structure for analysis (individual children are grouped in classes), we do not have the necessary size to perform such an analysis robustly. Third, while research has repeatedly indicated that the effects of video games on cognition depend largely on the video game genre [ 59 – 61 , 63 , 64 ], we did not have sample sizes large enough to substantiate any claims regarding genre-specific effects. The modest or absent effects observed in the present study might turn out very differently if video gaming activity is considered with greater minutiae (e.g., action, social, puzzle). Fourth, although this study included numerous measures it is likely that important measures were missed and deserve to be investigated in future studies. For instance, social media use is not expected to have the same attentional/behavioral or mental health impact as playing video games [ 110 – 112 ]. Finally, many of the measures collected in this study are self-reports and as such are subject to biases. It is well known, for instance, that self-reported media use questionnaires do not fully reflect real usage [e.g., 113 – 115 ]. Objective measures of media consumption, such as event-sampling or usage monitoring, would be preferable [ 116 ].
Future perspectives
Understanding how digital technologies impact our lives is extremely challenging because of the richness and ever changing landscape of digital media. Indeed, there are many ways the same technology may be used, multitude of facets in our daily lives that technologies may impact (e.g., mental health, cognition, well-being to name a few) and numerous routes through which these variables may interact with each other. Getting a better grasp of the effects of technology use will require new approaches including the development of benchmark tools both in terms of use measurement and impact, and the collection of larger datasets most likely within multi-laboratories initiatives. An important step towards that goal will involve clarifying concepts and building frameworks to characterize both technology use as well as psychological constructs of interest–an exemplary case in this context is the work conducted by Meier and Reineck [ 9 ] who proposed a hierarchy of six levels of analysis to structure research in this field: device (e.g., tablet versus laptop), type of application (e.g., email versus video), branded application (e.g., Facebook versus Instagram), feature (e.g., messenger versus chat), interaction (e.g., synchronous versus asynchronous) and message (e.g., text versus voice). It is encouraging to see that coming from a rather different, cognitive perspective, [ 11 ] have converged on a rather similar and complementary set of analysis levels, highlighting in addition the importance of content (e.g., social simulation game versus war-based games), context of use (e.g., single media versus multitasking; alone or in a social context), and user characteristics (e.g., children versus adults; typically developing versus with learning disabilities). Such frameworks and taxonomies will be useful for building unifying theories, making sense of the puzzle pieces collected so far but also to guide research in a more systematic way.
This study shows that different aspects of media consumption have different relationships with attentional/behavioral outcomes, mental health and school relevant variables, and thus highlights the importance of using more granular assessments than just total media time.
It is not uncommon to read that time in front of screens should be limited. The present paper indicates that such aggregate measures of media consumption are not sufficient and documents that the type of media used as well as how they are consumed both matter. In this study, we are able to highlight such differences through multiple related, but distinct, media use measures, and by using partial correlations and psychological networks. These analyses reveal that media multitasking more than video gaming and total time on media was associated with adverse psychological outcomes and that media multitasking should therefore be considered more intensively in future studies.
Finally, the complexity of measuring media consumption calls for a paradigm shift that integrates real-life usage sampling, event sampling along with self-reports. Given the rapidly changing landscape of digital media and the complexity of the topic it would seem beneficial for the field to coordinate multi-lab studies and to systematically share data and methods on best practice to representatively and usefully sample media consumption.
Supporting information
This file contains additional information about the methods and dataset as well as supplementary analyses.
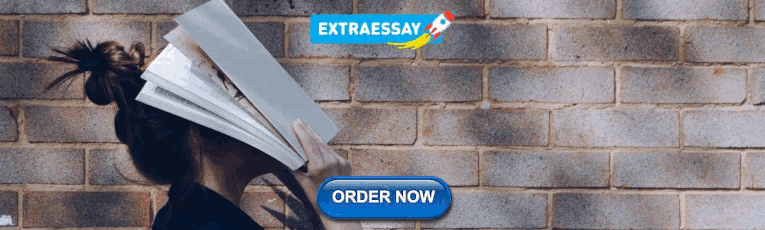
Acknowledgments
We thank the participating school, teachers, parents and students for their invaluable contribution to this research. We also thank Nuhamin Gebrewold Petros and Anna Flavia Di Natale for their help during the data collection process.
Funding Statement
This research was supported by the Luxembourg National Research Fund (ATTRACT/2016/ID/11242114/DIGILEARN) to PCL and the Swiss National Funds 100014_159506 and the Klaus J. Jacobs Foundation to DB. The funders had no role in study design, data collection and analysis, decision to publish, or preparation of the manuscript.
Data Availability
- Open access
- Published: 06 April 2024
Social media addiction and academic engagement as serial mediators between social anxiety and academic performance among college students
- Qiaoxing Mou 1 , 2 na1 ,
- Jie Zhuang 1 na1 ,
- Qunhong Wu 1 , 3 ,
- Yaqin Zhong 1 ,
- Qianqian Dai 3 ,
- Xin Cao 1 ,
- Yuexia Gao 1 ,
- Qingyun Lu 1 &
- Miaomiao Zhao 1
BMC Psychology volume 12 , Article number: 190 ( 2024 ) Cite this article
279 Accesses
Metrics details
Social anxiety has been shown to affect college students’ academic performance. However, the role of social media addiction and academic engagement in this association is unclear.
A total 2661 college students completed a self-report questionnaire including Liebowitz Social Anxiety Scale, the Bergen Social Media Addiction Scale, the Utrecht Student Work Engagement Scale for Students, and the grade point average. Hayes’ PROCESS macro for SPSS was employed to test the serial mediation effect.
Results indicated that social anxiety was negatively related to academic performance, only academic engagement played a single mediating role in the relationship between social anxiety and academic performance, meanwhile social media addiction and academic engagement acted as serial mediators between social anxiety on academic performance.
Conclusions
Social media addiction and academic engagement can explain the potential mechanisms of the association between social anxiety and academic performance, which have implications for devising intervention strategies to enhance the mental health and academic outcomes of college students.
Peer Review reports
Introduction
Social anxiety, a strong emotional reaction and avoidance behavior of apprehension, nervousness, or fear of one or more interpersonal situations, is the most common psychiatric disorder other than major depression and alcohol dependence owing to its high lifetime prevalence of 12% [ 1 ]. Social anxiety usually emerges in adolescence and its duration can last for approximately 25 years [ 2 ], which encompasses important stages in the educational, social, and professional development of college students. Entering college means adjusting to a new environment in which many college students face a series of challenges, such as meeting new people, establishing positive interpersonal relationships, and completing appropriate academic tasks [ 3 ]. However, in this context, college students may not like to express their emotions and opinions publicly and may become self-conscious and doubt their abilities [ 4 ]. According to studies conducted in Europe and the United States, the incidence rate of social anxiety ranges from 10 to 40% amongst college students [ 5 , 6 ]. In a study in China, 33.38% of the participants reported at least one symptom of social anxiety [ 7 ].
Individuals with social anxiety may isolate themselves and adopt avoidant behaviors to reduce feelings of anxiety [ 8 ]. Avoidant behavioral strategies, such as inability to engage in learning in class, avoidance of speaking, questioning, discussion, and other learning activities, can lead to problems including maladjustment and absenteeism that impair college students’ academic performance. Although previous studies have found that socially anxious college students exhibit low academic performance [ 9 , 10 ], the underlying causes have been less explored. Thus, it is important to further explore the underlying mechanisms of how social anxiety affects academic performance to provide a reference for the management of college students’ academic performance.
Tinto’s dropout theory model proposes that the key to college completion is the degree of integration of participation in the academic and social systems within the school [ 11 ]. Moreover, college students with social anxiety may have problems with academic failure due to blocked academic and social integration [ 8 , 12 ]. Through a survey of 300 college students and an analysis of grade point average (GPA) data, Parikh had found that academic engagement was positively correlated with GPA [ 13 ]. In a study of Canadian university students, social anxiety significantly and negatively predicted academic performance [ 14 ]. Russell and Topham also attributed college students’ low academic performance to the negative influence of social anxiety. Therefore, this study proposes the hypothesis.
Social anxiety is negatively associated with college students’ academic performance.
Social media addiction is a psychological state that involves a non-adaptive dependence on social media use that develops into behavioral addiction symptoms [ 15 , 16 ]. A meta-analysis showed that the overall prevalence of social media addiction was 24% in 32 countries and regions, with college students being the focus group for addiction to social media use [ 17 ]. Social media addiction manifests itself as obsession with social media, excessive use, emotional control, and so on. This is similar to and is considered a subtype of Internet addiction [ 18 ].. Difficulties in social interaction are also necessary for addiction. According to Social Compensation Hypothesis [ 19 ] and the Compensatory Internet Use Theory [ 20 ], individuals suffering from social anxiety may choose to communicate and interact via online social media as a compensation to reduce anxiety and isolation to satisfy the desire to escape, which makes them particularly susceptible to social media addiction. Previous research had proposed that individuals who experience social anxiety are more inclined to develop addictive tendencies towards the excessive use of social media that offers more control and comfort [ 21 , 22 ].Addiction to social media can lead students to spend excessive time on social media [ 23 ], with excessive access to specific websites for leisure reasons, leading to distractions from studying [ 24 ], causing a decrease in academic performance. It has also been proposed that student Facebook activity negatively predicts GPAs [ 25 ]. Hence, we propose the hypothesis.
Social media addiction mediates the relationship between social anxiety and academic performance.
Academic engagement is a continuous motivation to learn, characterized by a state of energy, dedication, and focus that is experienced as emotionally positive. Academic engagement is an important learning process factor that serves as an important factor in predicting academic performance [ 26 ]. Numerous studies have consistently highlighted the critical role of student engagement as a precursor to academic performance [ 27 , 28 , 29 ].. Notably, Salanova conducted a longitudinal study using cross-lagged correlation analyses, which revealed significant results supporting student engagement as a leading indicator of academic performance [ 26 ]. In fostering better academic engagement among adolescents, the significance of interpersonal relationships has been highlighted in previous research [ 30 ]. Conversely, social anxiety, which impairs individuals’ ability to effectively navigate social interactions [ 31 ], had been found to lead to reduced academic participation and engagement. It had previously been established that social anxiety affected classroom engagement due to limited communication with instructors [ 32 ]. Moreover, research had indicated a negative correlation between social anxiety and adolescents’ study engagement mediated through decreased intentional self-regulation and self-concept clarity [ 33 ]. Thus, social anxiety is associated with reduced academic performance through reduced academic engagement. Accordingly, we propose the hypothesis.
Academic engagement mediates the relationship between social anxiety and academic performance.
Academic engagement is characterized by energetic, purposeful, and sustained action [ 34 ], which may be regarded as visible and core manifestation of potential motivation [ 35 ]. One study found that Internet addiction was negatively correlated with motivation [ 36 ]. Furthermore, relevant empirical studies have shown that social media use can strongly interfere with learning [ 37 ]. Tong et al. suggested that prolonged use of social media may be detrimental to academic attention [ 38 ]. The above analysis suggests that when socially anxious individuals also have social media addiction, the interaction of these two factors will result in lower academic engagement, which in turn affects their academic performance. In other words, social media addiction and academic engagement may act as two distinct behavioral factors mediating the chain of social anxiety in relation to academic performance. In summary, this study proposes the hypothesis.
Social media addiction and academic engagement are negatively related, with social media addiction and academic engagement serially mediating the association between social anxiety and academic performance.
In summary, this study hypothesized that social anxiety and academic engagement are predictors of college students’ academic performance, which is a significant negative correlation between social anxiety and academic performance, as well as a moderating mediator role for social media addiction and academic engagement between the two. The proposed model is shown in Fig. 1 .
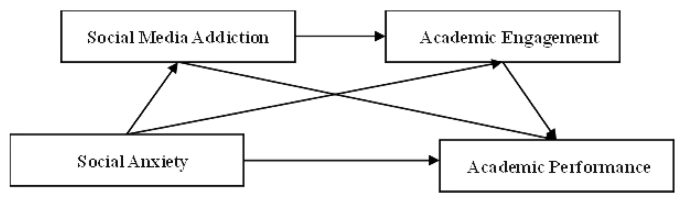
The proposed serial mediation model
Participants
The data used in this study was extracted from a larger investigation study about mental and behavior factors with health and academics, conducting from November 2020 to January 2021 at Nantong University in Jiangsu, China. Convenience sampling methods was performed. We firstly recruited 20 student investigators from all schools of main campus of the university. Then the student investigators were responsible for sending the online questionnaire by WeChat or QQ group to students of his/her school to invite them to participate on a voluntary basis. A total of 3451 students accessed the online survey and 2947 of them completed the questionnaire, with response rate of 85.4%. As the main campus of the university has about 30,000 students, our sample representative 10% of the target students. After data cleaning, 286 questionnaires with the omission of key study variables or logical errors were excluded, resulting in the inclusion of 2,661 participants in the present study (effective rate of 90.3%). Participants’ age was 19.97 ± 1.25 (ranging from 16 to 24 years). 1508 were female (56.7%), 1666 (62.6%) were non-medical students. The grades of participants are as followed: freshmen ( n = 682; 25.6%), sophomores ( n = 697; 26.2%), juniors ( n = 977; 36.7%), and seniors ( n = 305; 11.5%).
Measurements
- Social anxiety
Social anxiety was measured using the Liebowitz Social Anxiety Scale (LSAS) [ 39 ].The LSAS, including two subscales of fear and avoidance, is composed of 24 items including 11 items assessing social interaction and 13 items assessing performance/observation situations. Each item is rated on a 4-point Likert scale to measure the intensity of fear from 0 ( none ) to 3 ( severe fear ) and the frequency of avoidance from 0 ( never ) to 3 ( usually ). The total LSAS score is the sum of the subscale scores fear and avoidance (ranging from 0 to 144), with higher score indicating higher level of social anxiety. The Chinese version of the LSAS have been validated [ 40 ]. In the present study, Cronbach’s alpha of the LSAS was 0.973. Confirmatory factor analysis showed the four-dimensional scale had acceptable model fit (χ 2 /df = 3.26, p < 0.001, CFI = 0.884, TLI = 0.867, SRMR = 0.0615, RMSEA = 0.809), which were comparable with previous study [ 41 ].
- Social media addiction
Social media addiction was measured by the Bergen Social Media Addiction Scale (BSMAS) [ 42 ],which has been widely used in previous studies [ 43 , 44 ]. The BSMAS consists of 6 items, with each item rated on a 5-point Likert scale from 1 ( very rarely ) to 5( very often ). The total BSMAS score ranges from 6 to 30, with higher scores indicating higher addiction to social media. The Chinese version of the BSMAS has been used and validated [ 45 ]. In the present study, Cronbach’s alpha of the BSMAS was 0.877.
- Academic engagement
Academic engagement was evaluated by the Utrecht Work Engagement Scale for Students (UWES-9s) [ 46 ]. The UWES-9 S consists of 9 items assessing three dimensions of vigor, dedication, and absorption related to study. Each item of is UWES-9 S rated on a 7-point Likert scale from 0 (never) to 6 (always). The total UWES-9 S score ranges from 0 to 54, and the higher the total score, the higher the student’s academic engagement. The UWES-9 S shows good psychometric properties [ 47 , 48 ]. In the present study, Cronbach’s alpha for the UWES-9 S was 0.959. Confirmatory factor analysis showed the three-dimensional scale had good model fit (χ 2 /df = 2.90, p < 0.001, CFI = 0.975, TLI = 0.962, SRMR = 0.025, RMSEA = 0.083).
- Academic performance
GPA was employed to reflect academic performance. GPA can evaluate students’ learning quality in a more scientific, comprehensive, dynamic and quantitative way, which is widely used in academic performance studies [ 49 , 50 ].
Data analyses
IBM SPSS Statistics 25.0 was used to conduct data analyses. Prior to the formal analysis, the variance inflation factor (VIF) [ 51 ] and Harman’s single-factor test [ 52 ] was used to test multi-collinearity and common method bias between the study variables. Descriptive statistics and Pearson correlation analyses were then performed. Model 6 in the SPSS PROCESS macro was used to test the serial mediation effect [ 53 ]. In regression-based analysis, effect sizes are interpreted according to Cohen’s conventions: β coefficients below 0.3 were considered small, between 0.3 and 0.5 were considered medium, and equal to or greater than 0.5 are considered large [ 54 , 55 ]. Finally, bootstrap confidence interval (CI) analysis based on 5000 random resamples was performed to test the significance of the mediation effect, with the effects being considered significant if the CIs did not include zero.
Preliminary analyses
Collinearity diagnostics showed that none of the VIF values of the study variables were > 3, and no tolerance values were < 0.3, indicating low concern for multi-collinearity issues [ 56 ]. Harman’s single-factor test showed that 11 factors had eigenvalues greater than 1, with 36.77% of the total variance explained by the largest component, which was below 50% [ 57 ]. Consequently, the common method bias was not an issue.
Descriptive and correlational analyses
As Table 1 showed that the study variables were significantly associated with each other, providing preliminary support for our hypotheses.
Test of the serial mediation model
The results of the serial mediation model were showed in Table 2 . Results revealed that social anxiety was significantly negatively associated with academic performance ( β = -0.097, p < 0.001), indicating that for per one standard deviation (SD) increase in social anxiety, academic performance would decrease by 0.097 SD. Consequently, hypothesis H1 was validated. Furthermore, social anxiety showed a positive correlation with social media addiction ( β = 0.364, p < 0.001), implying that social media addiction would increase 0.364 SD per one SD increase in social anxiety. However, the association between social media addiction and academic performance was not found to be significant, thus hypothesis H2 was not supported. As predicted by hypothesis H3, social anxiety was significantly negatively related to academic engagement ( β = -0.307, p < 0.001). This suggested that for per one SD increase in social anxiety, academic engagement would decrease by 0.307 SD. Then, academic engagement in turn significantly positively related to academic performance ( β = 0.219, p < 0.001), indicating that academic performance would increase 0.219 SD per one SD increase in academic engagement. Finally, social media addiction and academic engagement was significantly negative related ( β = -0.160, p < 0.001), meaning that academic engagement would decrease 0.160 SD per one SD increase in social media addiction. Thus, hypothesis H4 was validated. Moreover, these findings indicated the presence of small and medium correlations among the study variables.
The bootstrap results (Table 3 ) showed that all the three indirect paths were significant. The total indirect effect was − 0.094 (95% CI: -0.117 to -0.071), accounting for 49.2% of the total effect. Specifically, although the pathway of social anxiety →social media addiction → academic performance was not statistically significant (effect= -0.014, 95% CI: -0.031 to 0.003), the other specific pathway of social anxiety → academic engagement → academic performance was significant (effect= -0.067, 95% CI: -0.084 to -0.052), accounting for 35.1% of the total effect; the sequential pathway of social anxiety → social media addiction → academic engagement → academic performance was also significant (effect= -0.013, 95% CI: -0.018 to -0.008), accounting for 6.8% of the total effect. The detailed pathway model was shown in Fig. 2 .
Furthermore, social anxiety has a significant direct on academic performance (effect= -0.097, 95% CI: -0.138 to -0.056), suggesting that social media addiction and academic engagement played a partial mediating role in link between social anxiety and academic performance. The serial mediation model explained 8.9% of the variance in college students’ academic performance (F = 43.240, p < 0.001).
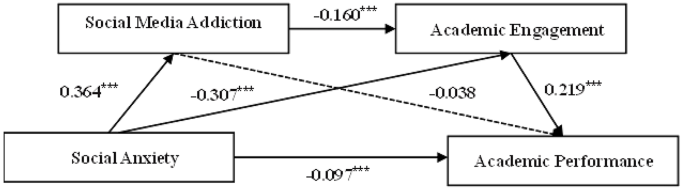
The serial mediation model of the relationship between social anxiety and academic performance. *** p < 0.001
This study provided a possible explanatory mechanism for the effects of social anxiety on academic performance among college students. By examining the serial mediation pathway of social media addiction and academic engagement, the study expands on existing knowledge regarding the negative impact of mental disorders on academic life. Consistent with previous research [ 58 , 59 , 60 ], the findings demonstrate a significant negative correlation between social anxiety and academic performance. This aligns with self-presentation theory [ 61 ], which suggests that the characteristics of social anxiety, such as reluctance, avoidance, and fear, hinder students’ learning activities, particularly in teacher-student interactions and academic integration. These hindrances can lead students to doubt their own abilities to achieve their goals and lower their expectations of impression-related outcomes. Consequently, their task performance may be weakened, ultimately affecting their academic performance in a negative manner. Social anxiety may lead to cognitive and attentional biases that interfere with information processing, problem-solving, and decision-making [ 62 ]. Students with social anxiety may have difficulty focusing on academic tasks due to excessive worry or fear of negative evaluation, leading to decreased academic performance [ 63 ].Furthermore, social anxiety can increase stress levels and reduce self-esteem, which can have a detrimental effect on academic performance [ 64 ].
Our research has revealed that social media addiction can develop as a result of social anxiety. This finding is consistent with previous studies that have highlighted the dominant role of social anxiety in online addiction [ 65 ]. Socially anxious individuals may become excessively fixated on social media to compensate for a lack of fulfilling face-to-face social interaction. The desire for connection could be one possible explanation for this behavior [ 66 ]. Socially anxious individuals who are overly concerned with the evaluations and approvals of others may spend excessive time and energy on social media to satisfy their connection desires, ultimately leading to social media addiction [ 67 ]. Surprisingly, we did not find a significant association between social media addiction and academic performance. Previous research is not without precedents. A study among Qatari university students showed no linear relationship between social media use and academic performance [ 68 ]. This could be attributed to the fact that different individuals perceive and use social media differently [ 69 ]. Our finding suggested that while college students with social anxiety may have a higher tendency towards social media addiction, this does not necessarily translate into lower academic performance unless it is accompanied by reduced academic engagement.
This study found a significant independent mediation effect of academic engagement on the association between social anxiety and academic performance, revealing that low academic engagement due to social anxiety was associated with poor academic performance. Individuals who experience social anxiety may fear not being valued equally in interpersonal or social interactions, further inhibit their willingness to participate or engagement in the classroom [ 8 ].In contrast, the vigor of engagement, dedication and absorption are all related to the intensive effort required to learning in higher education [ 70 ]. Students who are more vigorous and dedicated to their studies are more likely to excel [ 71 ]. Therefore, students will achieve better academic performance when they have a proactive engagement toward emotional and energy.
In addition, as hypothesized, we found a significant sequential mediation effect between social anxiety and academic performance, that is, social media addiction was an antecedent of academic engagement. In a majority of college students, excessive use of social media focuses on non-academic needs. These non-targeted tasks on social media can compete with learning, which may consistently disrupt the learning process [ 72 ]. In addition, social media addiction manifests in excessive attention to social media and wasting time and energy on social platforms [ 73 ]. Combined this with the difficulties in inhibitory control associated with addiction, social media addiction leads to more attention deficit and hyperactivity disorder [ 74 ], which in turn inhibits academic engagement. Thus, when social media addiction is combined with low academic engagement, individuals with social anxiety exhibit poor academic performance.
Given the non-significant results of the single indirect effect of social anxiety on academic performance through social media addiction, our finding demonstrated that although an increase in social anxiety could lead to a higher level of social media addiction, this did not necessarily lead to decreased academic performance unless it was followed by decreased academic engagement. In other words, the negative effect of social anxiety on college students’ academic performance was more strongly related to academic engagement than social media addiction. These findings are of great importance in revealing the significant role of academic engagement that is affected by social anxiety in the academic performance of college students, which is conducive to formulating psychological and behavioral intervention strategies aimed at improving their academic achievement.
Although this study successfully established a serial mediation pathway to understand the impact of social anxiety on academic performance in college students, it is important to acknowledge that academic performance is influenced by a multitude of factors including but not limited to cognitive abilities, academic skills, pedagogical approach, study habits, motivation, and external support systems. This is a possible exaptation of small R 2 in this study. Therefore, future research should aim to investigate the complex interplay between these factors and how they relate to academic performance in students with social anxiety to provide a more nuanced understanding of the complex factors that influence academic performance in college students with social anxiety and identify effective strategies for improving their academic outcomes.
This study had several limitations that should be considered. First, the current study was limited to students from one college in Nantong, China, and the cross-regional and cross-cultural applicability of the findings must be properly validated. Second, the present study relied only on self-reported data, and the effects of subjective bias and social desirability effects on the findings should be considered. Third, owing to the limitations of cross-sectional and non-experimental studies, no causal inferences can be made. In addiction we were unable to exclude the effects of all potential confounding variables.
Our findings contribute to a better understanding of the negative effect of mental disorders on academic performance, highlighting the importance of social media addiction and academic engagement as serial mediators between social anxiety and academic performance. In addition, the negative effect of social anxiety on academic performance is more strongly related to academic engagement than to social media addiction. Findings of this study suggest that a joint psychological, behavioral and academic support intervention targeting social anxiety and social media addiction to improve academic engagement and outcomes among college students. It is recommended to incorporated personalized academic support, mentoring programs to enhance academic engagement. Additionally, interventions aimed at reducing social anxiety and social media addiction such as cognitive-behavioral techniques, exposure-based interventions, and promoting a supportive campus environment can also play a role in improving academic engagement and outcomes.
Data availability
The datasets used and/or analyses during the current study are available from the corresponding author on reasonable request.
Abbreviations
grade point average
Liebowitz Social Anxiety Scale
Bergen Social Media Addiction Scale
Utrecht Work Engagement Scale for Students
variance inflation factor
confidence interval
standard deviation
Standard Error
Stein DJ, Lim CCW, Roest AM, de Jonge P, Aguilar-Gaxiola S, Al-Hamzawi A, Alonso J, Benjet C, Bromet EJ, Bruffaerts R et al. The cross-national epidemiology of social anxiety disorder: data from the World Mental Health Survey Initiative. BMC MED 2017, 15(1).
Steinert C, Hofmann M, Leichsenring F, Kruse J. What do we know today about the prospective long-term course of social anxiety disorder? A systematic literature review. J Anxiety Disord. 2013;27(7):692–702.
Article PubMed Google Scholar
Dyson R, Renk K. Freshmen adaptation to university life: depressive symptoms, stress, and coping. J Clin Psychol. 2006;4(410):1231–44.
Article Google Scholar
Elemo AS, Turkum AS. The Liebowitz Social Anxiety Scale (LSAS): Psychometric properties of the Amharic Version in Ethiopian University Student Sample. Int J Ment Health AD 2021.
Russell G, Shaw S. A study to investigate the prevalence of social anxiety in a sample of higher education students in the United Kingdom. J Ment Health. 2009;18(3):198–206.
Tillfors M, Furmark T. Social phobia in Swedish university students: prevalence, subgroups and avoidant behavior. Soc Psych Psych Epid. 2007;42(1):79–86.
Meng T, He Y, Zhang Q, Yu F, Zhao L, Zhang S, Chen Z, Wang S, Gong J, Liu J. Analysis of features of social anxiety and exploring the relationship between childhood major adverse experiences and social anxiety in early adulthood among Chinese college students. J Affect Disorders. 2021;292:614–22.
Russell G, Topham P. The impact of social anxiety on student learning and well-being in higher education. J Ment Health. 2012;21(4):375–85.
Baptista CA, Loureiro SR, de Lima Osório F, Zuardi AW, Magalhães PV, Kapczinski F, Filho AS, Freitas-Ferrari MC, Crippa JAS. Social phobia in Brazilian university students: prevalence, under-recognition and academic impairment in women. J Affect Disorders. 2012;136(3):857–61.
Djidonou A, Tchégnonsi FT, Adoukonou T, Ataïgba INE, Assa-Issa CN, Gandaho P. Associated factors and impacts of Social Phobia on Academic Performance among students from the University of Parakou (UP). Open J Psychiatry. 2016;06(02):151–7.
Tinto V. Dropout from higher education: a theoretical synthesis of recent research. Rev Educ Res. 1975;45(1):89–125.
Stein MB, Kean YM. Disability and quality of life in Social Phobia: epidemiologic findings. Am J Psychiatry. 2000;157(10):1606–13.
Parikh M. The relationship between student engagement and academic performance: An exploration of the paradox of international undergraduates.|.*69* 69 .: ProQuest Dissertations Publishing; 2008:1699.
Brook CA, Willoughby T. The Social ties that bind: social anxiety and academic achievement across the University years. J Youth Adolescence. 2015;44(5):1139–52.
Cao X, Gong M, Yu L, Dai B. Exploring the mechanism of social media addiction: an empirical study from WeChat users. Internet Res. 2020;30(4):1305–28.
Chen A. From attachment to addiction: the mediating role of need satisfaction on social networking sites. Comput Hum Behav. 2019;98:80–92.
Cheng C, Lau Y, Chan L, Luk JW. Prevalence of social media addiction across 32 nations: Meta-analysis with subgroup analysis of classification schemes and cultural values. Addict Behav. 2021;117:106845.
Ryan T, Reece J, Chester A, Xenos S. Who gets hooked on Facebook? An exploratory typology of problematic Facebook users. Cyberpsychology 2016, 10(3).
Valkenburg PM, Peter J. Social consequences of the internet for adolescents: a decade of Research. Curr Dir Psychol Science: J Am Psychol Soc. 2009;18(1):1–5.
Kardefelt-Winther. Daniel: a conceptual and methodological critique of internet addiction research: towards a model of compensatory internet use. Comput Hum Behav. 2014;31(31):351–4.
de Bérail P, Guillon M, Bungener C. The relations between YouTube addiction, social anxiety and parasocial relationships with YouTubers: a moderated-mediation model based on a cognitive-behavioral framework. Comput Hum Behav. 2019;99:190–204.
Ali F, Ali A, Iqbal A, Ullah Zafar A. How socially anxious people become compulsive social media users: the role of fear of negative evaluation and rejection. Telemat Inf. 2021;63:101658.
Malita L. Social media time management tools and tips. Procedia Comput Sci. 2011;3:747–53.
Kolhar M, Kazi RNA, Alameen A. Effect of social media use on learning, social interactions, and sleep duration among university students. Saudi J Biol Sci. 2021;28(4):2216–22.
Article PubMed PubMed Central Google Scholar
Michikyan M, Subrahmanyam K, Dennis J. Facebook use and academic performance among college students: a mixed-methods study with a multi-ethnic sample. Comput Hum Behav. 2015;45:265–72.
Salanova M, Schaufeli W, Martínez I, Bresó E. How obstacles and facilitators predict academic performance: the mediating role of study burnout and engagement. Anxiety Stress Coping. 2010;23(1):53–70.
Lee J. The relationship between Student Engagement and Academic Performance: is it a myth or reality? J Educational Res. 2013;107(3):177–85.
Lei H, Cui Y, Zhou W. Relationships between student engagement and academic achievement: a meta-analysis. Social Behav Personality: Int J. 2018;46(3):517–28.
van Beek I, Kranenburg IC, Taris TW, Schaufeli WB. BIS- and BAS-activation and study outcomes: a mediation study. Pers Indiv DiffeR. 2013;55(5):474–9.
Amerstorfer CM, Freiin Von Münster-Kistner C. Student perceptions of Academic Engagement and Student-Teacher relationships in Problem-based learning. Front Psychol. 2021;12:713057.
Klein AM, Salemink E, de Hullu E, Houtkamp E, Papa M, van der Molen M. Cognitive Bias Modification reduces social anxiety symptoms in socially anxious adolescents with mild intellectual disabilities: a Randomized Controlled Trial. J Autism Dev Disord. 2018;48(9):3116–26.
Archbell KA, Coplan RJ. Too anxious to talk: social anxiety, Academic Communication, and students’ experiences in Higher Education. J Emot Behav Disord. 2022;30(4):273–86.
Zhou A, Yanbing H, Guan X, Chen X, Lu X, Pan T. Social Anxiety and Study Engagement in Adolescents: The Role of Self Cognition Model. In: 2019-1-1 2019. IEEE; 2019:209–213.
Qahri-Saremi H, Turel O. School engagement, information technology use, and educational development: an empirical investigation of adolescents. Comput Educ. 2016;102:65–78.
Zhang Y, Qin X, Ren P. Adolescents’ academic engagement mediates the association between internet addiction and academic achievement: the moderating effect of classroom achievement norm. Comput Hum Behav. 2018;89:299–307.
Odaci H. Academic self-efficacy and academic procrastination as predictors of problematic internet use in university students. Comput Educ. 2011;57(1):1109–13.
Boer M, Stevens GWJM, Info EDN, Finkenauer C, Info EDN, van den Eijnden RJJM, Info EDNX, Finkenauer L, Youth ICCC. Attention deficit hyperactivity Disorder-Symptoms, Social Media Use Intensity, and social media use problems in adolescents: investigating directionality. Child Dev. 2020;91(4):e853–65.
Wen TT, Islam MA, Low WY, Choo WY, Abdullah A. Prevalence and Determinants of Pathological Internet Use among Undergraduate Students in a Public University in Malaysia. 2019.
Liebowitz MR. Social phobia. Mod Probl Pharmacopsychiatry. 1987;22:141–73.
He YL, Zhang MY. Psychometric investigation of Liebowitz Social Anxiety Scale. J Diagnostics Concepts Pract. 2004;3(2):5.
Google Scholar
Beard C, Rodriguez BF, Moitra E, Sibrava NJ, Bjornsson A, Weisberg RB, Keller MB. Psychometric properties of the Liebowitz Social Anxiety Scale (LSAS) in a longitudinal study of African americans with anxiety disorders. J Anxiety Disord. 2011;25(5):722–6.
Andreassen CS, Billieux J, Griffiths MD, Kuss DJ, Demetrovics Z, Mazzoni E, Pallesen S. The relationship between addictive use of social media and video games and symptoms of psychiatric disorders: a large-scale cross-sectional study. Psychol Addict Behav. 2016;30(2):252–62.
Lin C, Brostrom A, Nilsen P, Griffiths MD, Pakpour AH. Psychometric validation of the Persian Bergen Social Media Addiction Scale using classic test theory and Rasch models. J Behav Addict. 2017;6(4):620–9.
Monacis L, De Palo V, Griffiths MD, Sinatra M. Social networking addiction, attachment style, and validation of the Italian version of the Bergen Social Media Addiction Scale. J Behav Addict. 2017;6(2):178–86.
Huang L, Zhang J, Duan W, He L. Peer relationship increasing the risk of social media addiction among Chinese adolescents who have negative emotions. Curr Psychol 2021.
Schaufeli WB, Bakker AB, Salanova M. The measurement of work engagement with a short questionnaire - A cross-national study. Educ Psychol Meas. 2006;66(4):701–16.
Loscalzo Y, Giannini M. Study Engagement in Italian University students: a confirmatory factor analysis of the Utrecht Work Engagement ScaleStudent Version. Soc Indic Res. 2019;142(2):845–54.
Tang H, Huo Z, Liu C, Liu Z, Wong YJ. Psychometric properties of the academic encouragement scale in Chinese University students. J Psychoeduc Assess. 2021;39(2):258–63.
Lau WWF. Effects of social media usage and social media multitasking on the academic performance of university students. Comput Hum Behav. 2017;68:286–91.
Reuter PR, Forster BL. Student health behavior and academic performance. Peer J. 2021;9:e11107.
Hair JF, Black WC, Babin BJ, Anderson RE. Multivariate Data Analysis: A Global Perspective. 7th Edition. New York: Prentice Hall; 2009.
Podsakoff PM, MacKenzie SB, Lee JY, Podsakoff NP. Common method biases in behavioral research: a critical review of the literature and recommended remedies. J Appl Psychol. 2003;88(5):879–903.
Hayes AF. Introduction to mediation, moderation, and conditional process analysis: a regression-based approach. 2nd ed. New York: The Guilford; 2018.
Cohen J. Statistical Power Analysis for the behavioral sciences. 2nd ed. Routledge; 1988.
Nieminen P. Application of standardized regression coefficient in Meta-Analysis. BioMedInformatics. 2022;2(3):434–58.
Akinwande MO, Dikko HG, Samson A. Variance inflation factor: as a Condition for the inclusion of suppressor variable(s) in regression analysis. Open J Stat. 2015;05(07):754–67.
Shiau W, Luo MM. Factors affecting online group buying intention and satisfaction: a social exchange theory perspective. Comput Hum Behav. 2012;28(6):2431–44.
Vilaplana-Perez A, Perez-Vigil A, Sidorchuk A, Brander G, Isomura K, Hesselmark E, Kuja-Halkola R, Larsson H, Mataix-Cols D, Fernandez DLCL. Much more than just shyness: the impact of social anxiety disorder on educational performance across the lifespan. Psychol Med. 2021;51(5):861–9.
Al AN, Zaidi U, Awad SS, Alroqaiba N, Aldhahi MI, Alsaleh H, Akil S, Mortada EM. Moderating effects of Self-Esteem on the relationship between Communication Anxiety and Academic Performance among Female Health College Students during the COVID-19 pandemic. Int J Env Res Pub He 2022, 19(21).
Al-Hazmi BH, Sabur SS, Al-Hazmi RH. Social anxiety disorder in medical students at Taibah University, Saudi Arabia. J Fam Med Prim Care. 2020;9(8):4329–32.
Schlenker BR, Leary MR. Social anxiety and self-presentation: a conceptualization and model. Psychol Bull. 1982;92(3):641–69.
Peschard V, Philippot P. Social anxiety and information processing biases: an integrated theoretical perspective. Cognition Emot. 2016;30(4):762–77.
Exploring the Links Between Social Anxiety., Performance-Avoidance Goal Orientation, Fear of Negative Evaluation and Task Persistence.
Nordstrom AH, Goguen LMS, Hiester M. The effect of social anxiety and self-esteem on College Adjustment, Academics, and Retention. J Coll Couns. 2014;17(1):48–63.
Prizant-Passal S, Shechner T, Aderka IM. Social anxiety and internet use– A meta-analysis: what do we know? What are we missing? Comput Hum Behav. 2016;62:221–9.
Allen KA, Ryan T, Gray DL, McInerney DM, Waters L. Social Media Use and Social Connectedness in adolescents: the positives and the potential pitfalls. Australian Educational Dev Psychol. 2014;31(1):18–31.
Chen Y, Li R, Zhang P, Liu X. The moderating role of state attachment anxiety and avoidance between social anxiety and social networking sites Addiction. Psychol Rep. 2020;123(3):633–47.
Al-Yafi K, El-Masri M, Tsai R. The effects of using social network sites on academic performance: the case of Qatar. J Enterp Inf Manag. 2018;31(3):446–62.
Giunchiglia F, Zeni M, Gobbi E, Bignotti E, Bison I. Mobile social media usage and academic performance. Comput Hum Behav. 2018;82:177–85.
Vizoso C, Rodríguez C, Arias-Gundín O. Coping, academic engagement and performance in university students. High Educ Res Dev. 2018;37(7):1515–29.
Salmela-Aro K, Upadaya K. The Schoolwork Engagement Inventory. Eur J Psychol Assess. 2011;28(1):60–7.
Throuvala MA, Griffiths MD, Rennoldson M, Kuss DJ. A ‘Control Model’ of social media engagement in adolescence: a grounded theory analysis. Int J Environ Res Public Health 2019, 16(23).
Andreassen CS, Torsheim T, Brunborg GS, Pallesen S. Development of a Facebook Addiction Scale. Psychol Rep. 2012;110(2):501–17.
Montagni I, Guichard E, Kurth T. Association of screen time with self-perceived attention problems and hyperactivity levels in French students: a cross-sectional study. BMJ Open 2016, 6(2).
Download references
Acknowledgements
The authors would like to thank all participants and research assistants for their cooperation. We would also like to thank the reviewers for their valuable comments and suggestions.
This study is supported by the National Natural Science Foundation of China [grant number 72004104]; the Innovation Training Program for College Students in Jiangsu province [grant number 202010304104Y]; and the 14th Five Year Plan of Jiangsu Education Science [grant number B/2021/01/74]. The funders had no role in the design, data collection, analysis, interpretation of the data, and writing of this work.
Author information
Qiaoxing Mou and Jie Zhuang contributed equally to this work.
Authors and Affiliations
Department of Health Management, School of Public Health, Nantong University, Nantong, Jiangsu, China
Qiaoxing Mou, Jie Zhuang, Qunhong Wu, Yaqin Zhong, Xin Cao, Yuexia Gao, Qingyun Lu & Miaomiao Zhao
School of Public Health, Chengdu University of Traditional Chinese Medicine, Chengdu, China
Qiaoxing Mou
Department of Group Health Care, Affiliated Maternity and Child Health Care Hospital of Nantong University, Nantong, Jiangsu, China
Qunhong Wu & Qianqian Dai
You can also search for this author in PubMed Google Scholar
Contributions
QM: Formal analysis, Methodology, Writing - original draft. JZ: Investigation; Methodology. QW: Conceptualization, Resources, Methodology, Writing - review & editing. YZ: Funding acquisition, Formal analysis, Writing - original draft. QD: Conceptualization, Resources, Methodology. XC: Investigation; Methodology. YG: Project administration, Writing- review & editing. QL: Investigation, Methodology. MZ: Conceptualization; Project administration, Funding acquisition, Writing - review & editing, Supervision. All authors reviewed the manuscript.
Corresponding authors
Correspondence to Yuexia Gao , Qingyun Lu or Miaomiao Zhao .
Ethics declarations
Ethics approval and informed consent.
This study was approved by the Nantong University Research Ethics Committee. All procedures followed were in accordance with the ethical standards of the responsible committee on human experimentation (institutional and national) and with the Helsinki Declaration of 1975, as revised in 2000 (5). Informed consent was obtained from all patients for being included in the study.
Consent for publication
Not applicable.
Competing interests
The authors declare no competing interests.
Additional information
Publisher’s note.
Springer Nature remains neutral with regard to jurisdictional claims in published maps and institutional affiliations.
Rights and permissions
Open Access This article is licensed under a Creative Commons Attribution 4.0 International License, which permits use, sharing, adaptation, distribution and reproduction in any medium or format, as long as you give appropriate credit to the original author(s) and the source, provide a link to the Creative Commons licence, and indicate if changes were made. The images or other third party material in this article are included in the article’s Creative Commons licence, unless indicated otherwise in a credit line to the material. If material is not included in the article’s Creative Commons licence and your intended use is not permitted by statutory regulation or exceeds the permitted use, you will need to obtain permission directly from the copyright holder. To view a copy of this licence, visit http://creativecommons.org/licenses/by/4.0/ . The Creative Commons Public Domain Dedication waiver ( http://creativecommons.org/publicdomain/zero/1.0/ ) applies to the data made available in this article, unless otherwise stated in a credit line to the data.
Reprints and permissions
About this article
Cite this article.
Mou, Q., Zhuang, J., Wu, Q. et al. Social media addiction and academic engagement as serial mediators between social anxiety and academic performance among college students. BMC Psychol 12 , 190 (2024). https://doi.org/10.1186/s40359-024-01635-7
Download citation
Received : 07 June 2023
Accepted : 29 February 2024
Published : 06 April 2024
DOI : https://doi.org/10.1186/s40359-024-01635-7
Share this article
Anyone you share the following link with will be able to read this content:
Sorry, a shareable link is not currently available for this article.
Provided by the Springer Nature SharedIt content-sharing initiative
- Serial mediators
BMC Psychology
ISSN: 2050-7283
- General enquiries: [email protected]
Academia.edu no longer supports Internet Explorer.
To browse Academia.edu and the wider internet faster and more securely, please take a few seconds to upgrade your browser .
Enter the email address you signed up with and we'll email you a reset link.
- We're Hiring!
- Help Center
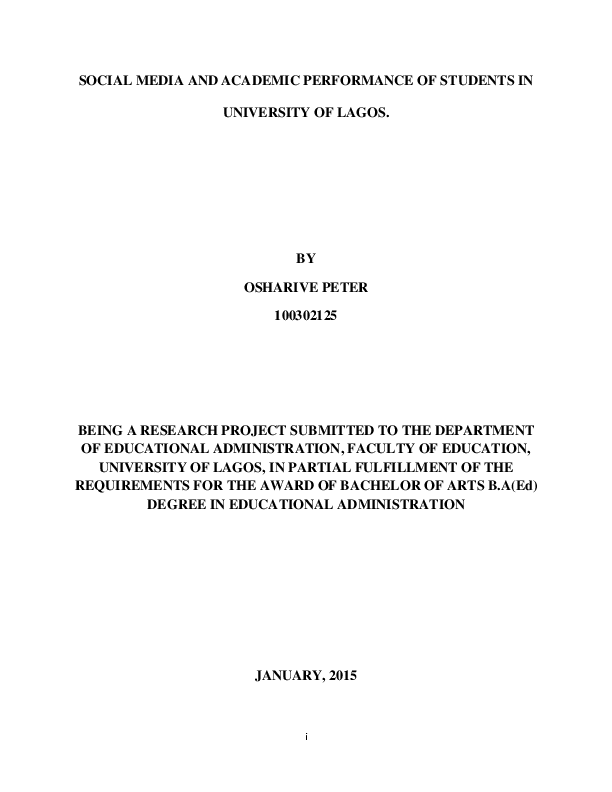
SOCIAL MEDIA AND ACADEMIC PERFORMANCE

The purpose of this research study is to examine the influence of Social Media and Academic Performance Of students in University of Lagos. Five Research questions and five Research hypotheses guided the study. To achieve this, the descriptive survey research design was adopted. The study focused on University of Lagos hence, population consists of all the 24,661 full-time undergraduate students. The simple random sampling technique was used to select a sample of 378 students. A four point Likert Type Rating Scale Questionnaire type, titled: Social Media and Academic Performance of Students Questionnaire (SMAAPOS) was used to collect data from the participants. The descriptive statistics of frequency counts and percentage, were used to analyze the demographic data while inferential statistics of Chi-square(x2) was used in testing the research hypotheses. Research findings showed that a great number of students in University of Lagos, are addicted to social media. To this end, the researcher recommended that social media should be used for educational purposes as well; Social Networking Sites should be expanded and new pages should be created to enhance academic activities and avoid setbacks in the students’ academic performance; and Students should be monitored by teachers and parents on how they use these sites This is to create a balance between social media and academic activities of students to avoid setbacks in the academic performance of the students.
Related Papers
Peter Osharive
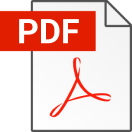
International Journal of Learning, Teaching and Educational Research
Adenrele AFOLORUNSO
The study examined the effect of social media (SM) on the academic performance (AP) of senior secondary school (SSS) students in Uyo, Akwa Ibom State, Nigeria. Four objectives served as the study’s guiding principles, and these were followed by research questions and hypotheses. A descriptive survey research design was used for the investigation. Using a simple random sampling technique, 200 students were selected as a sample from four SSSs in Uyo, Akwa Ibom State, Nigeria. A four-point Likert scale survey was used to poll the participants. The study questions were analysed using descriptive statistics such as frequency counts and percentages, whereas the null hypotheses were tested using chi-square, t-test, and Pearson product-moment correlation. Findings revealed that a large percentage of students are addicted to SM, with no significant differences in usage between boys and girls. It was also found that there is a link between SM addiction and a student's AP. In order to prev...
micah munang
Social media is a web-based service that gives individual the opportunity to create either a public or semi-public profile within a bounded system, furthermore it’s add a list of others with which they share a connection, view their list of connections and those made within the system. Therefore, this study is aim at examining the impact of use of social networking on students’ academic performance in Ahmadu Bello University Zaria. In other to measure social media platforms a questionnaire was developed based on past literatures. The independent variables includes: time appropriateness, time duration, Nature of Usage and type of social networking, while the dependent variable is student CGPA. The sample of 130 students from Department of computer science was selected using convenient sampling method. The data collected was analyzed using description means python programming. Thus considering the abnormal use of Social networking platforms by students, it is expedient that Ahmadu Bel...
Journal of Science, Technology, Mathematics and Education (JOSTMED)
Prof. Amosa I S I A K A GAMBARI
The study investigated the relationship between academic performance and use of social media among secondary school students in Minna metropolis, Niger State. Correlational survey design was employed. The study has two hypotheses. Simple random sampling was used to select 500 students out of 6,653 Senior Secondary School students from five coeducational secondary school in Minna Metropolis. Social Media Questionnaire (SMQ) was used for data collection with reliability coefficient of 0.76 using Pearson Product Moment Correlation (PPMC). Spearman rank correlation was used to test the hypothesis. Findings revealed that revealed that there was no significant relationship between students' rate of social media usage and their academic performance and gender has no significant influence on students' rate of usage, frequency of usage and time spent on social media in relation to their academic performance. Based on the above findings, it was recommended that the students should be encouraged to use social media for educational activities rather than for only entertainment activities as this will improve their academic achievement.
EduQuad (International and Peer Reviewed Journal of Education )
Manasseh T E R N E N G E Adi
This paper explored the effects of social media on the students' academic performance of Benue State Polytechnic Ugbokolo, a descriptive survey research design, simple random sampling technique and purposive sampling were implored, also four research questions guided the study. The research findings showed that a great number of students from Benue State Polytechnic had access to the internet. The researcher recommended that more sites should be created for academic purposes as this is will create a balance between social media and academic activities of students in order to avert setbacks in the academic performances of the students.
Dr. Lucky U . OGHENETEGA
The article works is on impact of social media on the Academic performance of University students in Nigeria. Causal-comparative research design was adopted. The Four tertiary institutions used for study- Nnamdi Azikiwe University-Awka, Delta State University, Abraka, Madonna University Okija campus and Anambra State University. Population of this study comprised of all the students in various departments in each schools. The total population selected through purposive sampling technique are twenty- five students in each school and the total therefore are hundred undergraduates and. Capture sampling is the method used to administer the instrument. Findings show that social media usage among students is not for academic purposes. Found out that the following are often used by students -Facebook 40(40.81%), WhatsApp 20(20.40%) 2go/Skype 14(14.28%) and while Myspace, Twitter, badoo, Blogs/web scholars, Google+/ Social bookmarking are not often used by undergraduates in the four universities for the study. Another finding showed that students in the four universities use social media for reaching out to close/distance friends and general information about life. The researchers proffer solutions to social media impact on education performance of students in Nigeria. Keywords: Social media (websites), educational performance, Students and Universities
Influence of Social Media on the Academic Performance of Students of Alex Ekwueme Federal University Ndufu-Alike Ikwo in Ebonyi State, Nigeria
benedict immaculate
This study was carried out to examine the influence of social media on the academic performance of students of Ale Ekwueme Federal University Ndufu Alike (AE-FUNAI) in Ikwo Local government area of Ebonyi state, Nigeria. The specific objective of the study was to find out whether the students of AE-FUNAI make use of social media or not. The theory of social constructivism was chosen as the theoretical frameworks. Survey research was used for the conduct of the research.. Thirteen thousand (13,000) was the population of the study, but the researcher used Taro Yamane’s formula to reduce the population into manageable size. Three hundred and sixty eight (368) questionnaires out of four hundred (400) shared to the students were retrieved. These data were analyzed through the utilization of Pearson’s chi-square statistics and percentages. The study found out that students who are exposed to social media are more likely to perform poorly in their academics than those who are not. Hence, there is a significant relationship between the number of social media accounts operated by students and their levels of academic performance. Also students who use social media are more likely to engage in online activities than those who do not use social media. These outcomes of data analysis constitute the findings of this study. Sequel to the findings, the study recommended that the university authority should maximize the immense academic values surrounding the use of social media by creating on-line educational and informational communities that will serve as platforms from which students can derive some academic values that will enhance their academic performance and also students should place emphasis on the use of social media for academic purpose while de-emphasizing its use for non-academic purpose. Keywords: Academic performance, Influence, Social Media, Students.
NNAJI JOHNSON C
johnson nnaji
The study investigated the impacts of social media on academic performance of student in Kaduna Polytechnic. The research work was guided by four objectives and four research questions which were answered. The population of the study was 100 students from Kaduna polytechnic. Purposive and cluster group sampling technique was used for the study. The instrument used for data collection was questionnaire which has 10 items. Simple percentage with the aid of tables were used to analyze the data. The findings of the study revealed that the usage of social media has influenced the students positively, followed by a minimum number of students which was affected negatively. The findings also revealed that the students use social media in their daily lives for; research, assignments and to see information. According to the questionnaire retrieved, the students prefer social media as means of communication as opposed to traditional method. The research findings showed that social media bring behavioral changes on student of Kaduna polytechnic. Base on the research findings, it was recommended that social media should be expanded and new pages of the internet sites should be created in order to help student use them more often. There should create a system between chatting and academic activities, so that majority of the student can be influenced positively. Social media should be made to have positive behavioral changes on students.
IJAR Indexing
The study examined perception of student on influence of use of social media on academic performance of students in Enugu State University of Science and Technology. One research question and one hypothesis guided the study. A survey research design was adopted for the study. Purposeful sampling technique was used to sample 160 students, 80 females and 80 males third year students of Faculty of Education. The instrument for data collection was a 10-iteme questionnaire developed by the researcher. The questionnaire was validated by three experts in the fields of education and a reliability of 0.79 was obtained using Cronbach alpha method of determining internal consistency of instrument. The research question was answered using mean and the hypothesis was tested at 0.05 level of significance using t-test statistic. The result indicated that students agreed that use of social media influence students academic performance and there is no significant difference in the mean response of female and male students on the influence of social media on academic performance. Encouraging students to spend more time on academic activities, provision of entertaining and amusement centres in the universities among others were some of the recommendation made.
International Journal of Educational Research
Joseph Okon
This study examined influence of social media on students ’ academic performance among secondary school students in Alimosho Local Government Area of Lagos State. The study sample comprised two hundred and fifty (n =250) senior secondary school two (ss2) students drawn from five secondary schools in the study areas, through stratified random sampling technique. The study was guided by three hypotheses while it adopted a descriptive survey design. A 25-item self-designed questionnaire titled Influence of Social Media on Students Academic Performance Questionnaire (ISMSAPQ) and Students Achievement Test (SAT) were used in the data collection. The instruments were validated and the reliability established through a pilot study with reliability coefficients of 0.76 and 0.74 (r=0.76;r= 0.74) respectively. The formulated hypotheses were tested using Chi-square, t-test and Analysis of Variance (ANOVA) statistical tools. Findings revealed that social media usage has a significant influence ...
RELATED PAPERS
Revista Geografica De America Central
Leonel Meneses
Proceedings of the 4th …
isabella pentimone
JURNAL ILMIAH EDUNOMIKA
sri hartono
Microbiology
Stefan Stumpe
Journal of Crohn's and Colitis
Serafino Vanella
Pflügers Archiv - European Journal of Physiology
Thomas Michel
James Putzel
Alfika Sendy Yonita
International Journal of Multimedia and Ubiquitous Engineering
Rahim Rahmani
kavita pareek
International Journal of …
Hildur Kalman
Licínio Ferreira
Micromachines
Noha Ali Gaber
Serena Newmark
Journal of the American Society of Nephrology
Markus Schlaich
Technium Social Sciences Journal
Takashi Tsuchiya
Bulletin of the Psychonomic Society
michael grubbs
Molecular Catalysis
mohamad Reza KHODADADI
Andreas Vött
INRAE Productions Animales
Jaime Gómez-morales
Microscopy and Microanalysis
Nestor Zaluzec
AGROMAX - Aries Agro Limited
Ayman Yousef
RELATED TOPICS
- We're Hiring!
- Help Center
- Find new research papers in:
- Health Sciences
- Earth Sciences
- Cognitive Science
- Mathematics
- Computer Science
- Academia ©2024
Advertisement
Social Media Addiction and Its Impact on College Students' Academic Performance: The Mediating Role of Stress
- Regular Article
- Published: 01 November 2021
- Volume 32 , pages 81–90, ( 2023 )
Cite this article
- Lei Zhao ORCID: orcid.org/0000-0002-7337-3065 1 , 2
6761 Accesses
12 Citations
1 Altmetric
Explore all metrics
Social media use can bring negative effects to college students, such as social media addiction (SMA) and decline in academic performance. SMA may increase the perceived stress level of college students, and stress has a negative impact on academic performance, but this potential mediating role of stress has not been verified in existing studies. In this paper, a research model was developed to investigate the antecedent variables of SMA, and the relationship between SMA, stress and academic performance. With the data of 372 Chinese college students (mean age 21.3, 42.5% males), Partial Least Squares, Structural Equation Model was adopted to evaluate measurement model and structural model. The results show that use intensity is an important predictor of SMA, and both SMA and stress have a negative impact on college students’ academic performance. In addition, we further confirmed that stress plays a mediating role in the relationship between SMA and college students’ academic performance.
This is a preview of subscription content, log in via an institution to check access.
Access this article
Price includes VAT (Russian Federation)
Instant access to the full article PDF.
Rent this article via DeepDyve
Institutional subscriptions
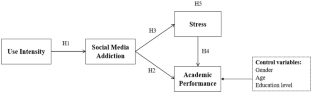
Similar content being viewed by others
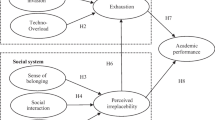
An investigation of the social media overload and academic performance
Xiongfei Cao, Yuntao Wu, … Ahsan Ali
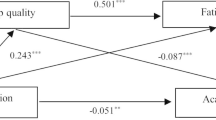
A serial mediation model of social media addiction and college students’ academic engagement: the role of sleep quality and fatigue
Jie Zhuang, Qiaoxing Mou, … Miaomiao Zhao
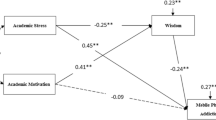
Associations Between Academic Motivation, Academic Stress, and Mobile Phone Addiction: Mediating Roles of Wisdom
Abolghasem Yaghoobi, Kambiz Karimi, … Sahar Mohammadi
Al-Yafi, K., El-Masri, M., & Tsai, R. (2018). The effects of using social network sites on academic performance: The case of Qatar. Journal of Enterprise Information Management , 31 (3), 446–462. https://doi.org/10.1108/JEIM-08-2017-0118 .
Andreassen, C. S., & Pallesen, S. (2014). Social network site addiction—an overview. Current Pharmaceutical Design, 20 , 1–9.
Article Google Scholar
Andreassen, C. S., Pallesen, S., & Griffiths, M. D. (2017). The relationship between addictive use of social media, narcissism, and self-esteem: Findings from a large national survey. Addictive Behaviors, 64 , 287–293. https://doi.org/10.1016/j.addbeh.2016.03.006
Andreassen, C. S., Torsheim, T., Brunborg, G. S., & Pallesen, S. (2012). Development of a facebook addiction scale. Psychological Reports, 110 (2), 501–517.
Argyris, Y. E., & Xu, J. (2016). Enhancing self-efficacy for career development in Facebook. Computers in Human Behavior, 55 (2), 921–931. https://doi.org/10.1016/j.chb.2015.10.023
Arnett, J. J. (2000). Emerging adulthood: A theory of development from the late teens through the twenties. American Psychologist, 55 (5), 469–480. https://doi.org/10.1016/j.chb.2015.10.023
Bano, S., Cisheng, W., Khan, A., & Khan, N. A. (2019). WhatsApp use and student’s psychological well-being: Role of social capital and social integration. Children and Youth Services Review, 103 , 200–208. https://doi.org/10.1016/j.childyouth.2019.06.002
Barclay, D., Higgins, C., & Thompson, R. (1995). The partial least squares (PLS) approach to causal modeling: Personal computer adoption and use as an illustration. Technology Studies, 2 (2), 285–309.
Google Scholar
Bijari, B., Javadinia, S. A., Erfanian, M., Abedini, M. R., & Abassi, A. (2013). The impact of virtual social networks on students’ academic achievement in Birjand University of Medical Sciences in East Iran. Procedia Social and Behavioural Sciences, 83 , 103–106. https://doi.org/10.1016/j.sbspro.2013.06.020
Błachnio, A., Przepiorka, A., & Pantic, I. (2016). Association between facebook addiction, self-esteem and life satisfaction: A cross-sectional study. Computers in Human Behavior, 55 , 701–705. https://doi.org/10.1016/j.chb.2015.10.026
Brailovskaia, J., Rohmann, E., Bierhoff, H. W., Schillack, H., & Margraf, J. (2019). The relationship between daily stress, social support and Facebook addiction disorder. Psychiatry Research, 276 , 167–174. https://doi.org/10.1016/j.psychres.2019.05.014
Brailovskaia, J., Schillack, H., & Margraf, J. (2018). Facebook addiction disorder in Germany. Cyberpsychology, Behavior, and Social Networking, 21 (7), 450–456. https://doi.org/10.1089/cyber.2018.0140
Buil, I., Martínez, E., & Matute, J. (2016). From internal brand management to organizational citizenship behaviors: Evidence from frontline employees in the hotel industry. Tourism Management, 57 (12), 256–271. https://doi.org/10.1016/j.tourman.2016.06.009
Busalim, A. H., Masrom, M., & Zakaria, W. N. B. W. (2019). The impact of Facebook addiction and self-esteem on students’ academic performance: A multi-group analysis. Computers & Education, 142 , 103651. https://doi.org/10.1016/j.compedu.2019.103651
Carolus, A., Binder, J. F., Muench, R., Schmidt, C., Schneider, F., & Buglass, S. L. (2019). Smartphones as digital companions: Characterizing the relationship between users and their phones. New Media & Society, 21 , 914–938. https://doi.org/10.1177/1461444818817074
China Internet Network Information Center (CNNIC), 2020. The 44th Statistical Report on Internet Development in China (in Chinese). Retrieved August 28, 2020, from http://www.cac.gov.cn/2020-08/30/c_1124938750.htm
Cohen, S., Kamarck, T., & Mermelstein, R. (1983). A global measure of perceived stress. Journal of Health and Social Behavior, 24 (4), 385–396. https://doi.org/10.2307/2136404
Dendle, C., Baulch, J., Pellicano, R., Hay, M., Lichtwark, I., Ayoub, S., & Horne, K. (2018). Medical student psychological distress and academic performance. Medical Teacher . https://doi.org/10.4236/ce.2018.99099
Doleck, T., & Lajoie, S. (2018). Social networking and academic performance: A review. Education and Information Technologies, 23 (1), 435–465. https://doi.org/10.1007/s10639-017-9612-3
Griffths, M. D., Kuss, D. J., & Demetrovics, Z. (2014). Social networking addictions: An overview of preliminary findings. Behavioral Addictions Criteria, Evidence, and Treatment , 119–141. https://doi.org/10.1016/B978-0-12-407724-9.00006-9 .
Hair, J. F. J., Hult, G. T. M., Ringle, C., & Sarstedt, M. (2016). A primer on partial least squares structural equation modeling (PLS-SEM) . Sage Publication.
Hair, J. F., Risher, J. J., Sarstedt, M., & Ringle, C. M. (2019). When to use and how to report the results of pls-sem. European Business Review, 31 (1), 2–24. https://doi.org/10.1108/EBR-11-2018-0203
Hayes, A. F. (2009). Beyond Baron and Kenny: Statistical mediation analysis in the new millennium. Communication Monographs, 76 (4), 408–420. https://doi.org/10.1080/03637750903310360
Hormes, J. M., Kearns, B., & Timko, C. A. (2014). Craving Facebook? Behavioral addiction to online social networking and its association with emotion regulation deficits. Addiction, 109 (12), 2079–2088. https://doi.org/10.1111/add.12713
Hsiao, K. L., Shu, Y., & Huang, T. C. (2017). Exploring the effect of compulsive social app usage on technostress and academic performance: Perspectives from personality traits. Telematics and Informatics, 34 , 679–690. https://doi.org/10.1016/j.tele.2016.11.001
Jie, T., Yizhen, Y., Yukai, D., Ying, M., Dongying, Z., & Jiaji, W. (2014). Addictive behaviors prevalence of internet addiction and its association with stressful life events and psychological symptoms among adolescent internet users. Addictive Behaviors, 39 (3), 744–747. https://doi.org/10.1016/j.adolescence.2016.07.012
Juana, I., Serafín, B., Jesús, L., et al. (2020). Effectiveness of music therapy and progressive muscle relaxation in reducing stress before exams and improving academic performance in nursing students: A randomized trial. Nurse Education Today, 84 , 104217. https://doi.org/10.1016/j.nedt.2019.104217
Kimberly, Y. (2009). Facebook addiction disorder . The Center for Online Addiction.
Lau, W. W. F. (2017). Effects of social media usage and social media multitasking on the academic performance of university students. Computers in Human Behavior, 68 (3), 286–291. https://doi.org/10.1016/j.chb.2016.11.043
Lazarus, R. S., & Folkman, S. (1984). Stress, appraisal, and coping . Springer.
Lee, W. W. S. (2017). Relationships among grit, academic performance, perceived academic failure, and stress in associate degree students. Journal of Adolescence, 60 , 148–152. https://doi.org/10.1016/j.adolescence.2017.08.006
Leung, L. (2007). Stressful life events, motives for Internet use, and social support among digital kids. CyberPsychology & Behavior, 10 (2), 204–214. https://doi.org/10.1089/cpb.2006.9967
Liang, C., Gu, D., Tao, F., Jain, H. K., Zhao, Y., & Ding, B. (2017). Influence of mechanism of patient-accessible hospital information system implementation on doctor-patient relationships: A service fairness perspective. Information & Management, 54 (1), 57–72.
Lim, K., & Jeoung, Y. S. (2010). Understanding major factors in taking Internet based lectures for the national college entrance exam according to academic performance by case studies. The Journal of the Korea Contents Association, 10 (12), 477–491. https://doi.org/10.5392/JKCA.2010.10.12.477
Lim, Y., & Nam, S.-J. (2016). Time spent on the Internet by multicultural adolescents in Korea. Asia Pacific Journal of Education, 37 (1), 55–68. https://doi.org/10.1080/02188791.2016.1169994
Lin, C. L., Jin, Y. Q., Zhao, Q., Yu, S. W., & Su, Y. S. (2021). Factors influence students’ switching behavior to online learning under covid-19 pandemic: A push-pull-mooring model perspective. The Asia-Pacific Education Researcher, 30 (3), 229–245. https://doi.org/10.1007/s40299-021-00570-0
Lovibond, P. F., & Lovibond, S. H. (1995). The structure of negative emotional states: Comparison of the depression anxiety stress scales (DASS) with the Beck depression and anxiety inventories. Behaviour Research and Therapy, 33 (3), 335–343. https://doi.org/10.1016/j.jad.2015.12.004
Marino, C., Gini, G., Vieno, A., & Spada, M. M. (2018). A comprehensive meta-analysis on problematic Facebook use. Computers in Human Behavior, 83 , 262–277. https://doi.org/10.1016/j.chb.2018.02.009
Masood, A., Luqman, A., Feng, Y., & Ali, A. (2020). Adverse consequences of excessive social networking site use on academic performance: Explaining underlying mechanism from stress perspective. Computers in Human Behavior . https://doi.org/10.1016/j.chb.2020.106476
McDaniel, B. (2015). ‘Technoference’: Everyday intrusions and inter-ruptions of technology in couple and family relationships. In C. Bruess Içinde (Ed.), Family communication in the age of digital and social media. Peter Lang Publishing.
Nayak, J. K. (2018). Relationship among smartphone usage, addiction, academic performance and the moderating role of gender: A study of higher education students in India. Computers and Education, 123 (5), 164–173. https://doi.org/10.1016/j.compedu.2018.05.007
O'Brien, L. (2012). Six ways to use social media in education. Retrieved from https://cit.duke.edu/blog/2012/04/six-ways-to-use-social-media-in-education/
Orosz, G., Istvan, T., & Beata, B. (2016). Four facets of Facebook intensity—the development of the multidimensional facebook intensity scale. Personality and Individual Differences, 100 , 95–104. https://doi.org/10.1016/j.paid.2015.11.038
Oye, N. D., Adam, M. H., & Nor Zairah, A. R. (2012). Model of perceived influence of academic performance using social networking. International Journal of Computers and Technology, 2 (2), 24–29. https://doi.org/10.24297/ijct.v2i1.2612
Pang, H. (2018). How does time spent on WeChat bolster subjective well-being through social integration and social capital? Telematics and Informatics, 25 , 2147–2156. https://doi.org/10.1016/j.tele.2018.07.015
Paul, J. A., Baker, H. M., & Cochran, J. D. (2012). Effect of online social networking on student academic performance. Computers in Human Behavior, 28 (6), 2117–2127. https://doi.org/10.1016/j.chb.2012.06.016
Peng, Y., & Li, J. (2021). The effect of customer education on service innovation satisfaction: The mediating role of customer participation. Journal of Hospitality and Tourism Management, 47 (5), 326–334. https://doi.org/10.1016/j.jhtm.2020.12.014
Phu, B., & Gow, A. J. (2019). Facebook use and its association with subjective happiness and loneliness. Computers in Human Behavior, 92 , 151–159. https://doi.org/10.1016/j.chb.2018.11.020
Podsakoff, P. M., MacKenzie, S. B., Lee, J.-Y., & Podsakoff, N. P. (2003). Common method biases in behavioral research: A critical review of the literature and recommended remedies. Journal of Applied Psychology , 88 (5), 879–903. https://doi.org/10.1037/0021-9010.88.5.879 .
Prato, C. A., & Yucha, C. B. (2013). Biofeedback-assisted relaxation training to decrease test anxiety in nursing students. Nursing Education Perspectives, 34 (2), 76–81. https://doi.org/10.1097/00024776-201303000-00003
Rouis, S. (2012). Impact of cognitive absorption on facebook on students’ achievement. Cyberpsychology, Behavior, and Social Networking, 15 (6), 296–303. https://doi.org/10.1089/cyber.2011.0390
Ryan, T., Chester, A., Reece, J., & Xenos, S. (2014). The uses and abuses of facebook: A review of facebook addiction. Journal of Behavioral Addictions, 3 (3), 133–148. https://doi.org/10.1556/JBA.3.2014.016
Sandra, K., Dawans, B. V., Heinrichs, M., & Fuchs, R. (2013). Does the level of physical exercise affect physiological and psychological responses to psychosocial stress in women. Psychology of Sport and Exercise, 14 , 266–274. https://doi.org/10.1016/j.psychsport.2012.11.003
Shaw, M., & Black, D. W. (2008). Internet addiction . Springer.
Shi, C., Yu, L., Wang, N., Cheng, B., & Cao, X. (2020). Effects of social media overload on academic performance: A stressor–strain–outcome perspective. Asian Journal of Communication, 30 (2), 1–19. https://doi.org/10.1080/01292986.2020.1748073
Statista (2020). Number of social media users worldwide from 2010 to 2021 (in billions). Retrieved from https://www.statista.com/statistics/278414/number-of-worldwide-social-network-users/
Tang, J. H., Chen, M. C., Yang, C. Y., Chung, T. Y., & Lee, Y. A. (2016). Personality traits, interpersonal relationships, online social support, and Facebook addiction. Telematics and Informatics, 33 (1), 102–108. https://doi.org/10.1016/j.tele.2015.06.003
Wang, J. L., Wang, H. Z., Gaskin, J., & Wang, L. H. (2015). The role of stress and motivation in problematic smartphone use among college students. Computers in Human Behavior, 53 , 181–188. https://doi.org/10.1016/j.chb.2015.07.005
Wartberg, L., Kriston, L., & Thomasius, R. (2020). Internet gaming disorder and problematic social media use in a representative sample of German adolescents: Prevalence estimates, comorbid depressive symptoms and related psychosocial aspects. Computers in Human Behavior, 103 , 31–36. https://doi.org/10.1016/j.chb.2019.09.014
Xu, X. A., Xue, K. N., Wang, L. L., Gursoy, D., & Song, Z. B. (2021). Effects of customer-to-customer social interactions in virtual travel communities on brand attachment: the mediating role of social well-being. Tourism Management Perspectives, 38 , 100790. https://doi.org/10.1016/j.tmp.2021.100790
Zhao, L. (2021). The impact of social media use types and social media addiction on subjective well-being of college students: A comparative analysis of addicted and non-addicted students. Computers in Human Behavior Reports, 4 (2), 100122. https://doi.org/10.1016/j.chbr.2021.100122
Zhao, L., Liang, C., & Gu, D. (2021). Mobile social media use and trailing parents’ life satisfaction: Social capital and social integration perspective. International Journal of Aging and Human Development, 92 (3), 383–405. https://doi.org/10.1177/0091415020905549
Download references
Acknowledgements
This study is supported by the Planning Subject for the 14th Five-year Plan of National Education Sciences (Grant No. EIA210425).
Author information
Authors and affiliations.
School of Management, Hefei University of Technology, Hefei, 230009, Anhui, China
Wendian College, Anhui University, Hefei, 230601, Anhui, China
You can also search for this author in PubMed Google Scholar
Corresponding author
Correspondence to Lei Zhao .
Ethics declarations
Conflict of interest.
The authors declared no potential conflict of interest with respect to the research, authorship, and/or publication of this article.
Additional information
Publisher's note.
Springer Nature remains neutral with regard to jurisdictional claims in published maps and institutional affiliations.
Rights and permissions
Reprints and permissions
About this article
Zhao, L. Social Media Addiction and Its Impact on College Students' Academic Performance: The Mediating Role of Stress. Asia-Pacific Edu Res 32 , 81–90 (2023). https://doi.org/10.1007/s40299-021-00635-0
Download citation
Accepted : 21 October 2021
Published : 01 November 2021
Issue Date : February 2023
DOI : https://doi.org/10.1007/s40299-021-00635-0
Share this article
Anyone you share the following link with will be able to read this content:
Sorry, a shareable link is not currently available for this article.
Provided by the Springer Nature SharedIt content-sharing initiative
- Use intensity
- Social media addiction
- Academic performance
- Find a journal
- Publish with us
- Track your research
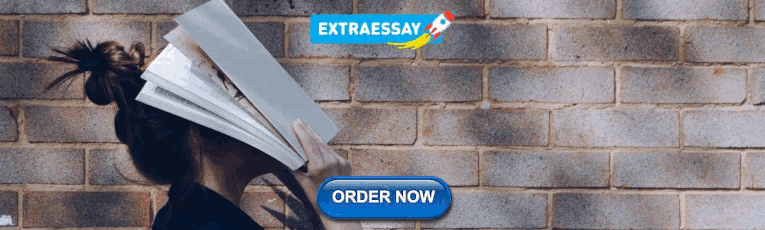
IMAGES
VIDEO
COMMENTS
social media has significantly in fluence on the academic performance of the students, 299. (23%) Agree, 376 (29%) Disagree, while 262 (20%) Strongly Disagree. Research Question 4: Is there gender ...
However, other research reported no correlation between social media usage and academic performance (Martin, n.d.; Stollak et al., 2011). Therefore, one purpose of this study was to clarify research results in determining whether there was a relationship between social media usage and academic success.
It has been evident that time spent using social media/social media addiction has a strong negative predictor of academic performance.[9,11,14,20,21,22] This might be due to the distractive nature of social media websites.[20,22] It is imperative to use social media to aid undergraduates' academic success and to make connections with peers ...
The advent of technology in education has seen a revolutionary change in the teaching-learning process. Social media is one such invention which has a major impact on students' academic performance. This research analyzed the impact of social media on the academic performance of extraversion and introversion personality students.
2.1 Social learning theory of Bandura. In a current research study, we have employed Bandura's social learning theory to form a theoretical framework that links social media usage and its association with academic performance (Abaalzamat et al., 2021; Aqeel et al., 2022; Li et al., 2022; Yu et al., 2022).The following theory helps in acknowledging two aspects of this study. i.e., competition ...
The first part of this literature review documents the various ways in which academics use social media and, as will become apparent, academics use social media for a wide range of activities spanning the typical academic duties of research and teaching, alongside other activities including professional development, career and image enhancement, and networking (Dermentzi and Papagiannidis 2018 ...
Humans are social beings and socializing is part of our lives. Digital 2021 Global Overview Report released by DataReportal places the global social media population at 4.3 billion, which is around 53% of the world's population (Simon, 2021a, p8).In Ghana the situation is not any different, 50% of the population uses internet and 26.1% are active on social media (Simon, 2021b, p17).
With the widespread adoption of social networking sites among college students, discerning the relationship between social networking sites use and college students' academic performance has become a major research endeavor. However, much of the available research in this area rely on student self-reports and findings are notably inconsistent. Further, available studies typically cast the ...
Accessing social media is common and although concerns have been raised regarding the impact of social media on academic success, research in this area is sparse and inconsistent. Survey responses were collected from 659 undergraduate and graduate students to determine the relationship between social media usage and overall academic performance, as well as explore if this relationship is ...
The rest of this research paper is ordered into 5 sections: • Section 2 - a review of literature based on the work related to the mobile payment system • Section 3 - analyzes the academic performance of students by social media sites usage • Section 4 - research methodology • Section 5 - research and discussions • Section 6 ...
Results. 41.5% of students used social media for upto 3 h per day. Whatsapp (98.25%) and Youtube (91.75%) were the most commonly used social media applications. 73.5% used social media to read health-related news, 71.5% used it to complete assignments and more than 50% used it for seminar preparation, test preparation and research-related ...
Research investigating social media use typically focus on late adolescents and young adults, despite a growing number of early adolescents, 93% to 97%—having at least one social media platform. Also, early adolescents are more likely to engage with newer sites, such as Snapchat and Instagram, than older platforms such as Facebook and Twitter.
Conclusions. A majority of the participants reported prolonged use of social networking sites for nonacademic purposes. These habitual behaviors can distract students from their academic work, adversely affect their academic performance, social interactions, and sleep duration, and lead to a sedentary lifestyle and physical inactivity, which in turn can render them vulnerable to non ...
This research article is identifying the impact of online and social media platforms on student's academic performance in Indian education system. While measuring the impact of online and social media platforms on academic performance, the personality trait of an individual learner and education levels have been considered as the moderating ...
of social media sites on their academic work. 1.3 Objectives of the Study The general objective of the study was to find out the impact of social media on academic performance among students. The specific objectives of the study were as follows: i. To examine the impact of social media on academic performance among the students. ii.
Research increasingly suggests that the impact of digital media use on cognition, academic performance or health is complex [e.g., 7, 8], as it depends on the type of media (e.g., video games, social networks), its content (e.g., fantasy, documentary), the context (e.g., alone, in groups) and the traits of the person consuming media [e.g., age ...
Social anxiety has been shown to affect college students' academic performance. However, the role of social media addiction and academic engagement in this association is unclear. A total 2661 college students completed a self-report questionnaire including Liebowitz Social Anxiety Scale, the Bergen Social Media Addiction Scale, the Utrecht Student Work Engagement Scale for Students, and the ...
Purpose of the Study The purpose of this study generally is to examine the influence of Social Media on the Academic Performance of students in University of Lagos. Specifically, the study seeks; 1. To examine the level of student addictiveness to social media and the influence on their academic performance. 2.
Social media use can bring negative effects to college students, such as social media addiction (SMA) and decline in academic performance. SMA may increase the perceived stress level of college students, and stress has a negative impact on academic performance, but this potential mediating role of stress has not been verified in existing studies. In this paper, a research model was developed ...
This paper provides a comprehensive review of existing literature on the impact of cooperative learning on students' academic performance and social interactions. Drawing upon empirical studies ...