A Review on Social Network Analysis Methods and Algorithms
Ieee account.
- Change Username/Password
- Update Address
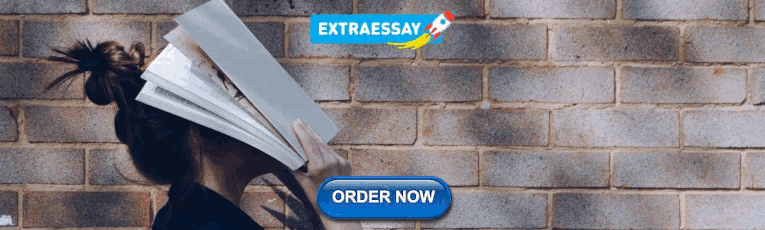
Purchase Details
- Payment Options
- Order History
- View Purchased Documents
Profile Information
- Communications Preferences
- Profession and Education
- Technical Interests
- US & Canada: +1 800 678 4333
- Worldwide: +1 732 981 0060
- Contact & Support
- About IEEE Xplore
- Accessibility
- Terms of Use
- Nondiscrimination Policy
- Privacy & Opting Out of Cookies
A not-for-profit organization, IEEE is the world's largest technical professional organization dedicated to advancing technology for the benefit of humanity. © Copyright 2024 IEEE - All rights reserved. Use of this web site signifies your agreement to the terms and conditions.
- Open access
- Published: 10 January 2022
The use of social network analysis in social support and care: a systematic scoping review protocol
- Rosario Fernández-Peña ORCID: orcid.org/0000-0002-6830-6001 1 , 2 , 3 ,
- María-Antonia Ovalle-Perandones 3 , 4 ,
- Pilar Marqués-Sánchez 3 ,
- Carmen Ortego-Maté 1 , 2 &
- Nestor Serrano-Fuentes 3 , 5
Systematic Reviews volume 11 , Article number: 9 ( 2022 ) Cite this article
5434 Accesses
2 Citations
11 Altmetric
Metrics details
In recent decades, the literature on Social Network Analysis and health has experienced a significant increase. Disease transmission, health behavior, organizational networks, social capital, and social support are among the different health areas where Social Network Analysis has been applied. The current epidemiological trend is characterized by a progressive increase in the population’s ageing and the incidence of long-term conditions. Thus, it seems relevant to highlight the importance of social support and care systems to guarantee the coverage of health and social needs within the context of acute illness, chronic disease, and disability for patients and their carers. Thus, the main aim is to identify, categorize, summarize, synthesize, and map existing knowledge, literature, and evidence about the use of Social Network Analysis to study social support and care in the context of illness and disability.
This scoping review will be conducted following Arksey and O'Malley's framework with adaptations from Levac et al. and Joanna Briggs Institute’s methodological guidance for conducting scoping reviews. We will search the following databases (from January 2000 onwards): PubMed, MEDLINE, Web of Science Core Collection, SCOPUS, CINAHL, PsycINFO, Cochrane Database of Systematic Reviews, PROSPERO, and DARE. Complementary searches will be conducted in selected relevant journals. Only articles related to social support or care in patients or caregivers in the context of acute illnesses, disabilities or long-term conditions will be considered eligible for inclusion. Two reviewers will screen all the citations, full-text articles, and abstract the data independently. A narrative synthesis will be provided with information presented in the main text and tables.
The knowledge about the scientific evidence available in the literature, the methodological characteristics of the studies identified based on Social Network Analysis, and its main contributions will highlight the importance of health-related research's social and relational dimensions. These results will shed light on the importance of the structure and composition of social networks to provide social support and care and their impact on other health outcomes. It is anticipated that results may guide future research on network-based interventions that might be considered drivers to provide further knowledge in social support and care from a relational approach at the individual and community levels.
Trial registration
Open Science Framework https://osf.io/dqkb5 .
Peer Review reports
Social Network Analysis (SNA) is a research approach within the social and behavioral sciences which focuses on ways of interaction and interconnection between individuals and social groups to explain social patterns of feelings, thoughts, and behaviors [ 1 , 2 ]. In recent decades, research based on SNA has been increasingly used in health, including areas such as disease transmission, health behavior, organizational networks, social capital, and social support [ 3 , 4 , 5 ].
The literature on social networks and health begins by referring to the idea that people are embedded in a network of relationships. The first empirical studies were published in the Annual Review of Public Health in the mid-1990s [ 6 ]. They showed the usefulness of specific SNA techniques to evaluate prevention programs among the involved organizations [ 7 ], or the relationship between HIV status, drug use and sexual relations [ 8 ]. Since then, there has been an exponential increase in scientific publications based on this methodology, especially in the last decade.
Different SNA studies have focused on showing the relationship between the characteristics of the social network and different health-related outcomes such as health behaviors [ 9 , 10 ], satisfaction with social support in chronic illness [ 11 ], quality of care and patient safety [ 12 ], the influence of social networks on HIV prevention and treatment outcomes [ 13 ], behavior change and risk of disease transmission [ 14 ], or performance in health care organizations and health care providers [ 15 , 16 , 17 ]. Also, SNA has been applied in health interventions based on social networks [ 18 , 19 , 20 , 21 ].
As mentioned above, one of the application areas of SNA is social support. The current epidemiological trend is characterized by a progressive increase in the population’s ageing and the incidence of long-term conditions. Thus, it seems relevant to highlight the importance of both social support and care systems to guarantee the coverage of health and social needs within the context of acute illness, chronic disease, and disability for patients and their careers. In its conceptual differentiation, caring and social support are dynamic processes that allude to interpersonal relationships [ 5 , 22 , 23 , 24 ]. However, they exist predominantly in separate domains. Care belongs to the professional context, while social support refers mainly to non-professional providers [ 25 ]. Unlike other approaches, research that uses SNA to study social support and care considers the network’s structural properties as the object of study [ 26 , 27 ] to know their relationship with other variables of interest. In this review, social networks are considered a structural framework to understand social support and care as relational concepts or as resources transferred through relationships [ 28 , 29 ]. Since there is no previous research that synthesizes the current knowledge on this research topic, we aim to identify, categorize, summarize, synthesize, and map existing knowledge, literature, and evidence about social network analysis to study social support and care in patients or caregivers in the context of illness, disability, or long-term conditions.
A scoping review is selected as an exploratory form of knowledge synthesis due to the extensive and growing literature that uses SNA in social support and care. This type of review is commonly undertaken to examine the extent, range, and nature of research activity in a topic area [ 30 ]: (a) to identify the types of available evidence in a given field, (b) to clarify key concepts/definitions in the literature, (c) to examine how research is conducted on a certain topic or area, (d) to identify key characteristics or factors related to a concept, (e) as a precursor to a systematic review, and (f) to identify and analyze knowledge gaps [ 31 ].
Arksey and O’Malley’s methodology framework [ 32 ], its advance by Levac and colleagues [ 33 ], and Joanna Briggs Institute’s methodological guidance [ 34 ] will be followed to conduct this scoping review through five stages: (a) identifying and stating research questions, (b) identifying relevant studies, (c) study selection, (d) charting data, and (e) collating and summarizing results [ 32 ].
This protocol is registered within the Open Science Framework platform (registration ID: https://osf.io/dqkb5 ). This scoping review has been reported using PRISMA-P [ 35 ] (Additional file 1 ). The final output will adhere to the Preferred Reporting for Systematic Reviews (PRISMA-ScR) checklist [ 36 ].
Stage 1: identification of the research question
The following research questions will guide the review:
What scientific evidence or studies are available in the literature on social support and care using the SNA methods?
What methodological characteristics constitute this body of literature?
What are the main contributions of these studies?
What knowledge and research gaps can be identified in the literature?
Stage 2: identifying relevant studies
The PCC framework (Population-Concept-Context) (Table 1 ) will be used to clearly define the concepts in the main review question, determine the eligibility of studies and guide the selection process [ 34 ]. We use a glossary of Terms for Community Heath Care from the World Health Organization to clarify the concepts used in our review [ 37 ].
The limits to be used in online databases searches will be: articles published in Spanish and English and the year of publication (from January 2000 onwards). The inclusion criteria will be (a) empirical studies with SNA methodology (quantitative or mixed methods design) and (b) studies whose participants are patients or caregivers as receivers of care or social support in the context of illness or disability provided by both, health professionals or personal/informal contacts with no age limits. The exclusion criteria will be (a) theoretical papers, (b) grey literature, and (c) qualitative studies.
The PRISMA flow chart [ 38 ] (Additional file 2 ) will capture and present our planned screening and selection process. The search strategy developed by MAOP will follow a comprehensive and sequential three steps and be checked by RMM. The Peer Review of Electronic Search Strategies Evidence-Based Checklist (PRESS EBC) will be followed to assess the search strategy's quality [ 39 ].
In the first step, the authors will work with an initial limited search in the PubMed database. The keywords and index terms will be identified in the titles and abstracts of the retrieved papers. In the second step, these keywords and index terms will be used to search across different databases. A structured search strategy will include Boolean operators (and, or, not), and truncations, either individually or in combination to ensure the search process. We will search the following databases: PubMed, MEDLINE, Web of Science Core Collection, SCOPUS, CINAHL, PsycINFO, Cochrane Database of Systematic Reviews, PROSPERO, and DARE (see Additional file 3 for search strategy). In a third step, a primary source search will be driven in the following journals: Social Networks, Connections, Journal of Social Structure, Redes, and Portularia. The retrieved references will be managed, and duplicates will also be removed using Mendeley and excel spreadsheet as a data extraction tool for the study selection.
Stage 3: study selection
Titles and abstracts of identified records will be assessed by two authors (RFP and NSF), independently. Disagreements will be resolved by consensus or with the assistance of a third author (PMS). The selected studies’ full text will be retrieved and checked independently by two authors (RFP and NSF). Sources of information that do not meet the eligibility criteria will be disregarded. A record of those sources and the reasons for their exclusion will be kept in a separate file.
Quality assessment
Scoping reviews are designed to provide an overview of the existing literature, regardless of quality. Therefore, a formal assessment of the quality of the included studies will not be conducted [ 32 ].
Stage 4: charting the data
The data charting aims to provide a descriptive summary of the results that align with this scoping review’s research questions. Thus, a data extraction tool designed for this study has been adapted from the template data extraction instrument for scoping reviews provided for JBI Manual for evidence synthesis [ 34 ] and will be used to capture the research purpose's most relevant information (see Table 2 ).
Charting the results will be an iterative process. Table 2 will be updated continuously until the end of the analysis. We will trial the extraction form on two or three sources to ensure all relevant results are extracted by at least two members of the review team [ 34 ].
Stage 5: collating and summarizing our results
According to the data extraction template, the obtained information will be part of built evidence tables with an overall description of the papers. We will follow the Arksey and O’Malley’s methods [ 32 ] to provide a descriptive numerical analysis of the topic, including the extent, characteristics, and their distribution in the included studies. We will present specific features and outcome measures of all included studies in a diagrammatic or tabular form. A descriptive summary will accompany the tabulated and/or charted results and will describe how the results relate to the review objectives and questions. This procedure will allow identifying specific gaps in the literature that might require further research.
The results of this scoping review will be added to the existing review articles on the use of the SNA in the health research area as complex health care interventions [ 40 ], the behavior change [ 41 ], nursing [ 42 ], inter-organizational networks [ 43 ], or healthcare providers [ 44 ]. Specifically, this protocol describes a systematic method synthesizing the existing literature on the use of SNA to study social support and care within the context of illness and disability.
This type of review is a convenient tool to determine the coverage of the body of literature on this specific area and will give a precise indication of the number of studies available and an overview of its focus. It might be useful for uncovering emerging evidence when it is still unclear what other more explicit questions can be addressed by a more precise systematic review [ 45 ]. Thus, the broader scope and nature justify the election of a scoping review versus a traditional systematic review that would answer specific questions and require more expansive inclusion criteria [ 31 ].
The authors anticipate that this review’s results will shed light on the importance of the structure and composition of social networks to provide social support and care and their impact on other health outcomes. This differs from many studies in this topic which use non-network approaches. The knowledge about the scientific evidence available in the literature, the methodological characteristics of the studies identified based on SNA, and its main contributions will highlight the importance of health-related research’s social and relational dimensions. Furthermore, it will identify areas for future research where social networks might be considered drivers to provide further knowledge in social support and care from a relational approach at the individual and community levels. The findings of this study will be disseminated through peer-review publications and national and international conferences.
Availability of data and materials
Further information related to this review can be provided upon reasonable request. Interested readers should contact the corresponding author.
Abbreviations
Social network analysis
Wasserman S, Faust K. Social network analysis. Methods and applications. Cambridge: Cambridge University Press; 1994.
Book Google Scholar
Scott J. What is social network analysis? Crow G, editor. New York: Bloomsbury Academic; 2012. p. 127.
Luke DA, Harris JK. Network analysis in public health: history, methods, and applications. Annu Rev Public Health. 2007;28(1):69–93. Available from. https://doi.org/10.1146/annurev.publhealth.28.021406.144132 .
Article PubMed Google Scholar
Moore S, Kawachi I. Twenty years of social capital and health research: a glossary. J Epidemiol Community Health. 2017;71(5):513–7. Available from:. https://doi.org/10.1136/jech-2016-208313 .
Article CAS PubMed Google Scholar
Smith K, Christakis N. Social networks and health. Annu Rev Sociol. 2008;34:405–18. Available from. https://doi.org/10.1146/annurev.soc.34.040507.134601 .
Article Google Scholar
Valente TW. Networks and public health : a review of network epidemiology : a handbook for survey. Soc Networks. 2007;29:154–9. Available from. https://doi.org/10.1016/j.socnet.2006.07.002 .
Wickizer TM, Von Korff M, Cheadle A, Maeser J, Wagner EH, Pearson D, et al. Activating communities for health promotion: a process evaluation method. Am J Public Health. 1993;83(4):561–7. Available from. https://doi.org/10.1016/j.socnet.2006.07.002 .
Article CAS PubMed PubMed Central Google Scholar
Friedman SR, Neaigus A, Jose B, Curtis R, Goldstein M, Ildefonso G, et al. Sociometric risk networks and risk for HIV infection. Am J Public Health. 1997;87(8):1289–97. Available from. https://doi.org/10.2105/AJPH.87.8.1289 .
Latkin C, Knowlton A. Social network assessments and interventions for health behavior change: a critical review. Behav Med. 2015;41(3):90–7. Available from. https://doi.org/10.1080/08964289.2015.1034645 .
Lin S, Faust L, Robles-Granda P, Kajdanowicz T, Chawla V. Social network structure is predictive of health and wellness. PLoS One. 2019;14(6):1–17. Available from. https://doi.org/10.1371/journal.pone.0217264 .
Article CAS Google Scholar
Fernández-Peña R, Molina J, Valero O. Satisfaction with social support received from social relationships in cases of chronic pain: The influence of personal network characteristics in terms of structure, composition and functional content. Int J Environ Res Public Health. 2020, 17(8):1–19. Available from. https://doi.org/10.3390/ijerph17082706 .
Cunningham FC, Ranmuthugala G, Plumb J, Georgiou A, Westbrook JI, Braithwaite J. Health professional networks as a vector for improving healthcare quality and safety: A systematic review. BMJ Qual Saf. 2012;21(3):239–49. Available from:. https://doi.org/10.1136/bmjqs-2011-000187 .
Ghosh D, Krishnan A, Gibson B, Brown S-E, Latkin CA, Altice FL. Social network strategies to adress HIV prevention and treatment continuum of care amont at-risk and HIV-infected substance users: a systematic scoping review. AIDS Behav. 2017;21(4):1183–207. Available from. https://doi.org/10.1016/j.jaad.2017.03.040 .
Article PubMed PubMed Central Google Scholar
Perkins JM, Subramanian SV, Christakis NA. Social networks and health: a systematic review of sociocentric network studies in low- and middle-income countries. Soc Sci Med. 2015;125:60–78. Available from:. https://doi.org/10.1016/j.socscimed.2014.08.019 .
Tasselli S. Social networks of professionals in health care organizations: a review. Med Care Res Rev. 2014;71(6):619–60. Available from. https://doi.org/10.1177/1077558714557079 .
Chambers D, Wilson P, Thompson C, Harden M. Social network analysis in healthcare settings: a systematic scoping review. PLoS One. 2012;7(8):1–10. Available from. https://doi.org/10.1371/journal.pone.0041911 .
Sabot K, Wickremasinghe D, Blanchet K, Avan B, Schellenberg J. Use of social network analysis methods to study professional advice and performance among healthcare providers : a systematic review. Syst Rev. 2017;6:1–23. Available from. https://doi.org/10.1186/s13643-017-0597-1 .
Pinto R. Using social network interventions to improve mentally ill clients’well-being. Clin Soc Work J. 2006;34(1):83–100. Available from. https://doi.org/10.1007/s10615-005-0005-5 .
Valente T. Network interventions. Science. 2012;337(6090):49–53. Available from. https://doi.org/10.1126/science.1217330 .
Gesell S, Barkin S, Valente T. Social network diagnostics: a tool for monitoring group interventions. Implement Sci. 2013;8(1):1–12. Available from. https://doi.org/10.1186/1748-5908-8-116 .
Valente TW. Putting the network in network interventions. PNAS. 2017;114(36):1500–1. Available from. https://doi.org/10.1073/pnas.1712473114 .
Campbell SM, Roland MO, Buetow SA. Defining quality of care. Soc Sci Med. 2000;51:1611–25. Available from. https://doi.org/10.1016/S0277-9536(00)00057-5 .
House JS, Umberson D, Landis KR. Structures and processes of social support. Annu Rev or Sociol. 1988;1988(14):293–318.
Finfgeld-Connett D. Clarification of social support. J Nurs Scholarsh. 2005;37(1):4–9. Available from. https://doi.org/10.1111/j.1547-5069.2005.00004.x .
Finfgeld-Connett D. Concept comparison of caring and social support. Int J Nurs Terminol Classif. 2007;18(2):58–68. Available from. https://doi.org/10.1111/j.1744-618X.2007.00051.x .
O’Malley JA, Marsden PV. The analysis of social networks. Heal Serv Outcomes Res Methodol. 2008;8:222–69. Available from. https://doi.org/10.1007/s10742-008-0041-z .
Hawe P, Webster C, Shiell A. A glossary of terms for navigating the field of social network analysis. J Epidemiol Community Health. 2004;58(12):971–5. Available from:. https://doi.org/10.1136/jech.2003.014530 .
Pescosolido BA, Levy JA. The role of social networks in health, illness, disease and healing: the accepting present, the forgotten past, and the dangerous potential for a complacent future. In: Levy JA, Pescosolido BA, editors. Social networks and health: advances in medical sociology. Oxford: Elsevier Science; 2002. p. 3–25.
Chapter Google Scholar
Faber A, Wasserman S. Social support and social networks: synthesis and review. In: Levy J, Pescosolido B, editors. Social Networks and Health: Advances in Medical Sociology. Oxford: Elservier Science; 2002. p. 29–72.
Pham MTM, Rajić A, Greig JDJ, Sargeant JMJ, Papadopoulos A, Mcewen SAS. A scoping review of scoping reviews: advancing the approach and enhancing the consistency. Res Synth Methods. 2014;5(4):371–85. Available from. https://doi.org/10.1002/jrsm.1123 .
Munn Z, Peters M, Stern C, Tufanaru C, McArthur A, Aromataris E. Systematic review or scoping review? Guidance for authors when choosing between a systematic or scoping review approach. BMC Med Res Methodol. 2018;18(143):1–7. Available from. https://doi.org/10.1186/s12874-018-0611-x .
Arksey H, O’Malley L. Scoping studies: towards a methodological framework. Int J Soc Res Methodol. 2005;8(1):19–32. Available from. https://doi.org/10.1080/1364557032000119616 .
Levac D, Colquhoun H, Obrien K. Scoping studies:advancing the methodology. Implement Sci. 2010;5(69):1–9 Available from: http://www.implementationscience.com/content/5/1/69 .
Google Scholar
Peters MDJ, Godfrey C, McInerney P, Munn Z, Tricco AC, Khalil H. Scoping reviews. In: Z AE and M, editor. JBI Reviewer ’ s Manual: In; 2020. Available from: https://reviewersmanual.joannabriggs.org/ .
Shamseer L, Moher D, Clarke M, Ghersi D, Liberati A, Petticrew M, et al. Preferred reporting items for systematic review and meta-analysis protocols (Prisma-P) 2015: Elaboration and explanation. BMJ. 2015;349:1–25. Available from. https://doi.org/10.1136/bmj.g7647 .
Tricco A, Lillie E, Zarin W, O’Brien K, Al E. PRISMA extension for scoping reviews (PRISMA-ScR) : checklist and explanation. Ann Intern Med. 2018;169(7):467–73. Available from. https://doi.org/10.7326/M18-0850 .
Gary A, Faulkner D. A glossary of terms for community health care and services for older persons. Kobe, Japan: WHO Centre for Health Development. 2004;5. https://apps.who.int/iris/handle/10665/68896 .
Moher D, Liberati A, Tetzlaff J, Altman DG, Group TP. Preferred reporting items for systematic reviews and meta-analyses : The PRISMA Statement. PLoS Med. 2009;6(7):e1000097.
McGowan J, Sampson M, Salzwedel DM, Cogo E, Foerster V, Lefebvre C. PRESS peer review of electronic search strategies: 2015 Guideline Statement. J Clin Epidemiol. 2016;75:40–6. Available from:. https://doi.org/10.1016/j.jclinepi.2016.01.021 .
Smit L, Dikken J, Schuurmans M, De Wit N, Bleijenberg N. Value of social network analysis for developing and evaluating complex healthcare interventions: A scoping review. BMJ Open. 2020;10:1–15.
Chambers D, Wilson P, Thompson C, Harden M. Social network analysis in healthcare settings: a systematic scoping review. PLoS One. 2012;7(8):e41911. https://doi.org/10.1371/journal.pone.0041911 .
Benton DC, Pérez-Raya F, Fernández-Fernández MP, González-Jurado MA. A systematic review of nurse-related social network analysis studies. Int Nurs Rev. 2015;62(3):321–39.
Timm I, Rapp S, Jeuter C, Bachert P, Reichert M, Woll A, et al. Interorganizational networks in physical activity promotion: a systematic review. Int J Environ Res Public Health. 2021;18:1–17.
Bae S, Nikolaev A, Seo JY, Castner J. Health care provider social network analysis: a systematic review. Nurs Outlook. 2015;63(5):566–84.
Armstrong R, Hall BBJ, Doyle J, Waters E. ‘Scoping the scope’ of a cochrane review. J Public Health. 2011;33(1):147–50. Available from. https://doi.org/10.1093/pubmed/fdr015 .
Download references
Acknowledgements
To Mr. Roberto Martín Melón, Librarian of the Medicine Area of the University of Cantabria, for his collaboration in the literature search process. To Professor Jose Luis Molina of the Autonomous University of Barcelona, and Professor Isidro Maya-Jariego of the University of Seville for their support in consultations during the review work.
Not applicable
Author information
Authors and affiliations.
Department of Nursing, University of Cantabria, Santander, Spain
Rosario Fernández-Peña & Carmen Ortego-Maté
IDIVAL Nursing Research Group, Santander, Spain
SALBIS Research Group, Leon, University of Leon, Leon, Spain
Rosario Fernández-Peña, María-Antonia Ovalle-Perandones, Pilar Marqués-Sánchez & Nestor Serrano-Fuentes
Library and Information Science Department, Universidad Complutense de Madrid, Madrid, Spain
María-Antonia Ovalle-Perandones
NIHR ARC Wessex, School of Health Sciences, University of Southampton, Southampton, UK
Nestor Serrano-Fuentes
You can also search for this author in PubMed Google Scholar
Contributions
RFP conceived the study. NSF, PMS, and COM contributed to the study conceptualization. MAOP will carry out the search strategy. RFP and NSF will perform the initial screening of the articles and PMS, COM, and MAOP will be involved in the study methodology, screening, and data extraction. All authors have read and approved the final version of this protocol.
Corresponding author
Correspondence to Rosario Fernández-Peña .
Ethics declarations
Ethics approval and consent to participate.
Not applicable. This review does not involve human participants.
Consent for publication
Not applicable.
Competing interests
The authors declare that they have no competing interests.
Additional information
Publisher’s note.
Springer Nature remains neutral with regard to jurisdictional claims in published maps and institutional affiliations.
Supplementary Information
Additional file 1..
PRISMA-P 2015 Checklist.
Additional file 2.
PRISMA-Flow Diagram.
Additional file 3.
Literature search strategy.
Rights and permissions
Open Access This article is licensed under a Creative Commons Attribution 4.0 International License, which permits use, sharing, adaptation, distribution and reproduction in any medium or format, as long as you give appropriate credit to the original author(s) and the source, provide a link to the Creative Commons licence, and indicate if changes were made. The images or other third party material in this article are included in the article's Creative Commons licence, unless indicated otherwise in a credit line to the material. If material is not included in the article's Creative Commons licence and your intended use is not permitted by statutory regulation or exceeds the permitted use, you will need to obtain permission directly from the copyright holder. To view a copy of this licence, visit http://creativecommons.org/licenses/by/4.0/ . The Creative Commons Public Domain Dedication waiver ( http://creativecommons.org/publicdomain/zero/1.0/ ) applies to the data made available in this article, unless otherwise stated in a credit line to the data.
Reprints and permissions
About this article
Cite this article.
Fernández-Peña, R., Ovalle-Perandones, MA., Marqués-Sánchez, P. et al. The use of social network analysis in social support and care: a systematic scoping review protocol. Syst Rev 11 , 9 (2022). https://doi.org/10.1186/s13643-021-01876-2
Download citation
Received : 04 February 2021
Accepted : 20 December 2021
Published : 10 January 2022
DOI : https://doi.org/10.1186/s13643-021-01876-2
Share this article
Anyone you share the following link with will be able to read this content:
Sorry, a shareable link is not currently available for this article.
Provided by the Springer Nature SharedIt content-sharing initiative
- Health care
- Scoping review protocol
- Social care
- Social networks
- Social Network Analysis
- Social support
Systematic Reviews
ISSN: 2046-4053
- Submission enquiries: Access here and click Contact Us
- General enquiries: [email protected]
- Open access
- Published: 22 July 2019
Thematic series on Social Network Analysis and Mining
- Rodrigo Pereira dos Santos 1 &
- Giseli Rabello Lopes 2
Journal of Internet Services and Applications volume 10 , Article number: 14 ( 2019 ) Cite this article
5951 Accesses
2 Citations
4 Altmetric
Metrics details
Social networks were first investigated in social, educational and business areas. Academic interest in this field though has been growing since the mid twentieth century, given the increasing interaction among people, data dissemination and exchange of information. As such, the development and evaluation of new techniques for social network analysis and mining (SNAM) is a current key research area for Internet services and applications. Key topics include contextualized analysis of social and information networks, crowdsourcing and crowdfunding, economics in networks, extraction and treatment of social data, mining techniques, modeling of user behavior and social networks, and software ecosystems. These topics have important areas of application in a wide range of fields, such as academia, politics, security, business, marketing, and science.
1 Introduction
This Thematic Series of the Journal of Internet Services and Applications (JISA) presents a collection of articles around the topic of Social Network Analysis and Mining (SNAM). From advances in Computer Science research and practice, the field of SNAM has become an important subject due to (i) the large amount and diversity of data that could be analyzed, (ii) the capacity of processing and solving complex analysis with efficiency, (iii) the development of new solutions for visualization of complex networks, and (iv) the application of SNAM concepts in different domains.
The study of social networks was leveraged by the social, educational and business communities. Academic interest in this field has been growing since the mid twentieth century [ 1 ], given the increasing interaction among people, data dissemination and exchange of information. In this scenario, big data sets require more accurate analyses. As such, the development and evaluation of new techniques for social network analysis and mining (SNAM) is a current key research area for Internet services and applications. These topics have important areas of application in a wide range of fields.
A social network is composed of actors who have relationships with each other. Networks can have a few to many actors (nodes) and one or many types of relationships (arrows) between pairs of actors [ 2 ]. In our daily life, we have several practical examples of social networks: our family, friends, and colleagues from the university, gym, work, or casual meetings. Individuals and organizations – seen as nodes in social networks – can be connected due to several reasons, such as friendship and genealogy, but also values, visions, ideas, finances, disagreements, conflicts, services, computer networks, air routes etc. The structure created from such a large amount of relationships is complex. Therefore, researchers study the network as a whole from a sociocentric view (all the links referring to specific relations in a given population), or as a social structure in an egocentric view (with links selected from specific people) [ 3 ].
In addition, people join and create groups in any society [ 4 ], but the web platform fostered critical changes in the way people can interact and think about the reality. Interactions (i) become easier, (ii) allow a frequent exchange of information, and (iii) transform communications tools and social media (e.g., microblogs, blogs, wikis, Facebook) to mass communication means that are more agile and far-reaching. As such, the use of social media contributes to the sharing of different types of information, especially in real time. Some examples are personal data, location, opinions and preferences. In this context, SNAM can support the understanding of preferences and associations, the identification of interactions, the recognition of influences, and the comprehension of information flow (context and concepts) among network actors.
Finally, the understanding of interactions in a specific scenario can produce concrete results. In an organization, employees should work to avoid problems regarding knowledge sharing [ 5 ]. In natural science, social networks can aid in the study of endemies and epidemies propagation [ 6 ]. In marketing, SNAM can be used as a tool for brand spread, or for the study of a market segment towards the understanding of how information propagates [ 7 ]. The last (but not least) example is the use of SNAM for the identification of criminal networks [ 8 ].
This JISA Thematic Series originates from the 6th Brazilian Workshop on Social Network Analysis and Mining (BraSNAM 2017) that was held in São Paulo, Brazil, on July 04–05, 2017. BraSNAM 2017 was affiliated with the 37th Brazilian Computer Society Congress (CSBC 2017) which is the official event of the Brazilian Computer Society (SBC). BraSNAM is focused on bringing together researchers and professionals interested in social networks and related fields. The workshop aims at providing innovative contributions to the research, development and evaluation of novel techniques for SNAM and applications. Finally, the main goal is to provide a valuable opportunity for multidisciplinary groups to meet and engage in discussions on SNAM.
Continuing in this direction, this JISA Thematic Series targets new techniques for the field of SNAM, mainly fostered by the context of Internet services and applications. We received contributions at various levels: from theoretical foundations to experiments and case studies based on real cases and applications; from modeling to mining and analysis of big data sets; and from different subjects and domains, such as entertainment, public transportation, elections, and personal social circles.
This Thematic Series presents high-quality research and technical contributions. We received six submissions as extended versions of the best papers of BraSNAM 2017. Topics included: analysis of online discussion and comments, complex networks, graph mining, government open data, power metrics, community detection, link assessment, homophily, and sentiment analysis. The five out of six submissions that were selected for publication and appear in this issue are summarized in the following section.
2 The papers
Loures et al. [ 9 ] investigate the potential that online comments have to describe television series. The authors implement and evaluated several different summarization methods. Their results reveal that a small set of comments can help to describe the corresponding episodes and, when taken together, the series as a whole.
Caminha et al. [ 10 ] use graph mining techniques for the detection of overcrowding and waste of resources in public transport. The authors propose a new data processing methodology for the evaluation of collective transportation systems. The results show that their approach is capable of identifying global imbalances in the system based on an evaluation of the weight distributions of the edges of the supply and demand networks.
Verona et al. [ 11 ] propose metrics for power analysis on political and economic networks based on a sociology theory and network topology. The authors present a case study using a network built on data from Brazilian Elections about electoral donations explaining how the metrics can help in the analysis of power and influence of the different actors (corporations and persons) in this network.
Leão et al. [ 12 ] propose a method to handle social network data that exploits temporal features to improve the detection of communities by existing algorithms. By removing random relationships, the authors observe that social networks converge to a topology with more pure social relationships and better quality community structures.
Finally, Caetano et al. [ 13 ] propose an analysis of political homophily among Twitter users during the 2016 US presidential election. Their results showed that the homophily level increases when there are reciprocal connections, similar speeches or multiplex connections.
3 Paper selection process
The paper selection process was run during 2018 and the papers were published as soon as they were accepted and online-first versions became ready. Each submission went through two to four revisions before the final decision. We invited leading experts who are international researchers in the field of SNAM and related topics to form this Thematic Series’ editorial committee. All manuscripts were reviewed by at least three members of this editorial committee. Guest editors checked the new version produced after each review cycle in order to decide whether the authors carefully addressed the reviewers’ comments. Otherwise, a further review cycle was requested by the guest editors. The papers were reviewed by a total of 19 reviewers. The names of the editorial committee members are listed on the acknowledgements of this editorial.
4 Conclusion
The future of research and practice in the field of SNAM is challenging. Opportunities are many: theoretical and applied research has been published in specific conferences and journals, but also in traditional venues since it requires a multidisciplinary arrangement. Based on the papers accepted to this Thematic Series, we can highlight some research gaps. For example, Loures et al. [ 9 ] point out the challenge of abstractive summarization for online comments : it is usually a much more complex task than the extractive one, since it requires a natural language generation module and a domain dependent component to process and rank the extracted knowledge. In turn, Caminha et al. [ 10 ] point out the need for a simulator for reproducing the dynamics of human mobility through the bus system in the case of a large metropolis. In this context, the use of data mining to estimate probability can represent the current demand for a bus system.
Regarding applications of SNAM in the context of presidential elections, Verona et al. [ 11 ] point out the challenge of redesigning the power metric to show relative values inside the network, instead of big absolute values. Moreover, information about company owners should be integrated in order to reveal hidden connections behind donations and politicians. In turn, Caetano et al. [ 13 ] point out the need for further investigation on the temporal political homophily analysis correlating it with external events that may have influenced the users’ sentiments. This effort can allow user classification through data mining techniques to identify candidates’ advocates, political bots, and other actors. Finally, regarding community detection, Leão et al. [ 12 ] point out the challenge of adopting different approaches for community detection , consider additional algorithms to explore temporal aspects or identify overlapping communities, and evaluate filtered networks. Moreover, different alternatives to measure the strength of ties should be investigated.
In the end, this Thematic Series comes out with some meaningful over-arching results:
SNAM researchers and practitioners recognize the importance of sentiment analysis for in the identification of conflicts and agreements, as well as social trends and movements, in different domains. As such, new methods and techniques should be developed based on the large set of existing empirical studies on this topic;
The dynamic nature of social networks makes community detection somehow a hard work. Different algorithms exist and are many. However, the treatment of randomness and noise in social relations requires further investigation. In addition, the assessment of those relations over time is also a topic of interested in SNAM;
Another challenge in the area is the understanding of social power and the way it manifests in social networks. In this context, power is tightly related to the notion of influence and authority. Research can vary from the development and use of SNAM algorithms and tools to the theorization based on qualitative studies (e.g., case studies, ethnography, sociotechnical approaches);
SNAM opens opportunities to investigate different types of systems, such as (i) systems-of-systems: a set of constituent software-intensive systems that are managerially and operationally independent, and present some emergent behavior and evolutionary development (e.g., smart cities, transportation, air space, flood monitoring), and (ii) software ecosystems: a set of actors and artifacts as well as their relations over a common technological platform (e.g., iOS, Android, Eclipse, SAP);
Finally, SNAM can support research on new trends of collaborative systems, such as crowdsourcing, free and open source software development, accountability, transparency and community engagement. A common interest lies on how to improve information visualization and recommendation based on actors’ characteristics and behaviors as well as the changes in their relations over time.
Berkowitz SD. An introduction to structural analysis: the network approach to social research. Toronto: Butterworth; 1982.
Google Scholar
Wasserman S, Faust K. Social network analysis: methods and applications. Cambridge: Cambridge University Press; 1994. p. 1994.
Book Google Scholar
Hanneman RA, Riddle M. Introduction to social network methods. Riverside, CA: University of California, Riverside; 2005.
Castells, M., 2000, The Rise of the Network Society (The Information Age: Economy, Society and Culture, Volume 1, 2nd ed Wiley-Blackwell.
Studart RM, Oliveira J, Faria FF, Ventura LVF, Souza JM, Campos MLM. Using social networks analysis for collaboration and team formation identification. In: Proceedings of the 15th international conference on computer supported cooperative work in design, Lausanne; 2011. p. 562–9.
Mikolajczyk RT, Kretzschmar M. Collecting social contact data in the context of disease transmission: prospective and retrospective study designs. Soc Networks. 2008;30(2):127–35.
Article Google Scholar
Kempe D, Kleinberg J, Tardos É. Maximizing the spread of influence through a social network. In: Proceedings of the 9th ACM SIGKDD international conference on knowledge discovery and data mining. Washington, D.C., USA; 2003.
Svenson P, Svensson P, Tullberg H. Social network analysis and information fusion for anti-terrorism. In: Proceedings of the conference on civil and military readiness. Sweden: Enköping. p. 2006.
Loures TC, de Melo PO, Veloso AA. Is it possible to describe television series from online comments? J Internet Serv Appl. 2018;9:25.
Caminha C, Furtado V, Pinheiro V, Ponte C. Graph mining for the detection of overcrowding and waste of resources in public transport. J Internet Serv Appl. 2018;9:22.
Verona L, Oliveira J, Hisse JVC, Campos MLM. Metrics for network power based on Castells’ network theory of power: a case study on Brazilian elections. J Internet Serv Appl. 2018;9:23.
Leão, J. C., Brandão, M. A., VAZ DE Melo, P. O. S., Laender, A. H. F. “Who is really in my social circle?”, Journal of Internet Services and Applications (2018) 9:23.
Caetano JA, Lima HS, Santos MF, Marques-Neto HT. Using sentiment analysis to define twitter political users’ classes and their homophily during the 2016 American presidential election. J Internet Serv Appl. 2018;9:18.
Download references
Acknowledgments
We thank all the authors, reviewers, editors-in-chief, and staff for the great work, which supported this Thematic Series on a very important topic both for the research community and for the industry. In particular, we thank all the editorial committee members: Alessandro Rozza ( lastminute.com Group, ITALY), Altigran Soares da Silva (Federal University of Minas Gerais, BRAZIL), Antonio Loureiro (Federal University of Minas Gerais, BRAZIL), Ari-Veikko Anttiroiko (Tampere University), Artur Ziviani (National Laboratory for Scientific Computing, BRAZIL), Bernardo Pereira Nunes (Pontifical Catholic University of Rio de Janeiro, BRAZIL), Claudio Miceli de Farias (Federal University of Rio de Janeiro, BRAZIL), Daniel Batista (University of São Paulo, BRAZIL), Flavia Bernardini (Federal Fluminense University, BRAZIL), Giacomo Livan (University College London, UK), Isabela Gasparini (Santa Catarina State University, BRAZIL), Jesús Mena-Chalco (Federal University of ABC, BRAZIL), Jonice Oliveira (Federal University of Rio de Janeiro, BRAZIL), Leandro Augusto Silva (Mackenzie Presbyterian University, BRAZIL), Luciano Antonio Digiampietri (University of São Paulo, BRAZIL), Luiz André Portes Paes Leme (Federal Fluminense University, BRAZIL), Mirella Moro (Federal University of Minas Gerais, BRAZIL), Raimundo Moura (Federal University of Piauí, BRAZIL), Yosh Halberstam (University of Toronto, CANADA). The voluntary work of these researchers was crucial for this Thematic Series.
Author information
Authors and affiliations.
Department of Applied Informatics, Federal University of the State of Rio de Janeiro – UNIRIO, Rio de Janeiro, Brazil
Rodrigo Pereira dos Santos
Department of Computer Science, Federal University of Rio de Janeiro – UFRJ, Rio de Janeiro, Brazil
Giseli Rabello Lopes
You can also search for this author in PubMed Google Scholar
Contributions
Both authors read and approved the final manuscript.
Corresponding author
Correspondence to Rodrigo Pereira dos Santos .
Ethics declarations
Competing interests.
The authors declare that they have no competing interests.
Publisher’s Note
Springer Nature remains neutral with regard to jurisdictional claims in published maps and institutional affiliations.
Rights and permissions
Open Access This article is distributed under the terms of the Creative Commons Attribution 4.0 International License ( http://creativecommons.org/licenses/by/4.0/ ), which permits unrestricted use, distribution, and reproduction in any medium, provided you give appropriate credit to the original author(s) and the source, provide a link to the Creative Commons license, and indicate if changes were made.
Reprints and permissions
About this article
Cite this article.
dos Santos, R.P., Lopes, G.R. Thematic series on Social Network Analysis and Mining. J Internet Serv Appl 10 , 14 (2019). https://doi.org/10.1186/s13174-019-0113-z
Download citation
Published : 22 July 2019
DOI : https://doi.org/10.1186/s13174-019-0113-z
Share this article
Anyone you share the following link with will be able to read this content:
Sorry, a shareable link is not currently available for this article.
Provided by the Springer Nature SharedIt content-sharing initiative
- Original Article
- Open access
- Published: 20 October 2013
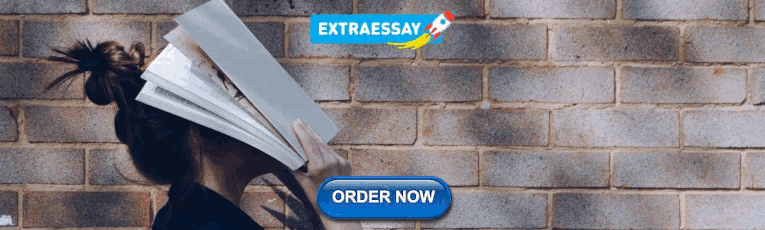
Social network analysis in innovation research: using a mixed methods approach to analyze social innovations
- Nina Kolleck 1
European Journal of Futures Research volume 1 , Article number: 25 ( 2013 ) Cite this article
18k Accesses
24 Citations
5 Altmetric
Metrics details
The importance of social networks for innovation diffusion and processes of social change is widely recognized in many areas of practice and scientific disciplines. Social networks have the potential to influence learning processes, provide opportunities for problem-solving, and establish new ideas. Thus, they can foster synergy effects, bring together key resources such as know-how of participating actors, and promote innovation diffusion. There is wide agreement regarding the usefulness of empirical methods of Social Network Analysis (SNA) for innovation and futures research. Even so, studies that show the chances of implementing SNA in these fields are still missing. This contribution addresses the research gap by exploring the opportunities of a mixed methods SNA approach for innovation research. It introduces empirical results of the author’s own quantitative and qualitative investigations that concentrate on five different innovation networks in the field of Education for Sustainable Development.
Introduction
Scholars interested in innovation processes and futures research have often stressed the importance of social networks. Social networks are seen as an important factor in how ideas, norms, and innovations are realized. Social network research understands individuals within their social context, acknowledging the influence of relationships with others on one’s behavior. Hence, social networks can promote innovation processes and expand opportunities for learning. Despite the consensus regarding the value of social network approaches, there is a lack of empirical investigations in innovation and futures studies that use Social Network Analysis (SNA). In most cases, the scientific literature uses the concept of social networks metaphorically, ignoring the chances presented by SNA methods. At the same time, conventional empirical research in innovation and futures studies often disregards relational information. Hence, analyses of statistical data on structural and individual levels are treated as separately. Activities that are expected to have impacts on future developments are usually modeled as isolated individual or group behavior, on the one hand, or as the characteristics of structural issues, on the other hand. SNA provides us with empirical tools that capture the social context and help to better understand how innovations are implemented and diffused and why social change takes place. Network approaches explicitly challenge the difference between deduction and induction and highlight the relevance of relationships. Individuals both shape and are shaped by the social context in which they interact. By applying techniques of SNA, actor-centered and structuralist reductions are avoided. Instead, SNA emphasizes the mutual influence of structure and social connections. In order to better understand and model developments in innovation and futures research, relational data inherent to the social network perspective is needed.
This contribution addresses the opportunities of SNA for innovation research. It is divided into six sections. After this introduction , the second section briefly defines crucial concepts of SNA and provides theoretical background. The third section discusses the value of a social network perspective for innovation research. The methodological approach, along with the empirical case studies used, is outlined in the fourth section. The fifth section shows how a combination of both insights from structure based on quantitative SNA and subjective perceptions revealed with qualitative SNA is helpful for understanding innovation processes. Here, the integration of qualitative SNA such as egocentric network maps in quantitative techniques of SNA is illustrated. The contribution concludes with a summary of main arguments.
Theoretical and methodological background
While in the scientific literature there are diverse understandings on what a social network is, this contribution draws on the definition used by Stanley Wassermann and Katherine Faust:
“A social network consists of a finite set or sets of actors and the relation or relations defined on them. The presence of relational information is a critical and defining feature of a social network” [ 1 ].
This conception of social network permits both a governance approach and empirical techniques of SNA. Scholars of governance research understand social networks as a certain type of governance that can be differentiated from other ideal types of governance: markets and hierarchies. Social networks combine market-based and hierarchic dimensions and serve as a form of hybrid governance [ 2 ]. Both weak and strong modes of coordination are integrated into the network concept of governance research, where strong coordination is defined as “the spectrum of activity in which one party alters its own … strategies to accommodate the activity of others in pursuit of a similar goal” [ 3 ]. Weak coordination, on the other hand, takes place when actors observe each other’s behavior, “and then alter their actions to make their … strategies complementary with respect to a common goal” [ 3 ].
Because they promote constant exchange and deliberation, social networks have strong potential to promote ideological or structural changes and to generate new knowledge. Hence, network governance is not reduced to governmental action, but refers to the search for collective and participative problem-solving strategies and the promotion of innovations. Footnote 1 This article uses the concept of network governance to highlight the relevance of relationships for innovation research. Hence, it confronts the assumption that individual behavior is independent of any others, but instead conceives “problem-solving as a collaborative effort in which a network of actors, including both state and non-state organizations, play a part” [ 4 ]. Footnote 2
In order to better understand the opportunities of SNA for innovation research, this contribution introduces innovation networks in five different regions as case studies. Innovation networks are understood as social networks that aim at establishing a social innovation. Here, the social innovation of Education for Sustainable Development (ESD) is used. At the same time, the term social innovation refers to processes of implementing and diffusing new social concepts across different sectors of society. While “innovation” implies a kind of renewal, “social” connotes interaction of actors. Social innovations have a direct connection with the search for solutions to social problems and challenges [ 6 , 7 ]. Likewise, Education for Sustainable Development can be defined as education that empowers people to foresee, try to understand, and solve the problems that threaten life on our planet. With the goal of promoting behavioral changes that will shape a more sustainable future, ESD integrates principles of sustainable development into all aspects of education and learning.
Change and innovation through social relations
How can social networks evoke changes and what are the opportunities for SNA to promote innovation processes? SNA has the potential to overcome uncertainties related to innovation processes. The chance of an innovation gaining acceptance increase significantly if it is supported by interconnected actors rather than singular individuals. Social networks foster change processes and promote innovation diffusion. SNA techniques thus help to understand existing networks and to identify innovation potentials in order to generate new information and reveal options for structural developments. SNA has the capacity to promote innovation processes by dealing with the following issues:
Identification of innovation networks (existing, missing, possible, and realistic cooperation) and investigation of actors, structures, and network boundaries:
By using SNA methods, network structures were determined in previously defined fields. Thus, techniques of egocentric SNA provide us with necessary information with respect to network membership and structural interconnections between actors. Structural properties detected in the context of this project are, for example, centrality, prestige, or weak and strong ties.
Innovation potentials through network development strategies:
Looking at network structures not only fosters the development and diffusion of new ideas. It can also reveal where and how structural conditions enable innovations and development processes. Furthermore, Social Network Analyses disclose where and how cooperation can be optimized and where and how alterations are possible and reasonable. Presenting stakeholders the results of SNA can foster structural changes.
Identification and promotion of coordination, information, and motivation:
Analysis of social networks provides us with useful insights into knowledge transfer processes, showing where they exist and how “well” they function. Also, problems of coordination, information, and motivation become evident, providing us with knowledge related to development potentials.
Development of strategies to reduce uncertainties related to innovation processes:
The costs of information exchange are not only material (money, time), but also social. Uncertainties, lack of confidence, and the fear of a loss of reputation can prevent actors from sharing information and knowledge. Results of SNA help us to identify weaknesses in the knowledge transfer process.
Social network analysis in innovation research
In order to illustrate the key opportunities of SNA in innovation research, this section draws on the author’s own empirical investigations that used a mixed methods approach based on quantitative and qualitative SNA. Data on network members was drawn from five different German municipalities and included initiatives, institutions, thematic groups, and individuals engaged in the field of ESD. The municipalities studied are Alheim, Erfurt, Frankfurt am Main, Gelsenkirchen, and Minden. These municipalities have been awarded by the United Nations Decade of Education for Sustainable Development (UNDESD), 2005–2014, and are characterized by active networks in the field of the social innovation of ESD. Organizations, initiatives, and actors from different sectors of non-formal, informal, and formal education seek to further establish and diffuse the concept of ESD worldwide. Thus, networks within these municipalities can be regarded as best practices concerning their performance in the area of ESD. It should be taken into account, however, that the social networks analyzed here are neither institutionalized nor formally established organizations. Instead, every person engaged in the field of the social innovation is regarded as part of the network to be analyzed. Hence, defining the network boundaries was an important part of the empirical investigation.
The research design included three main steps. First, qualitative data was collected in order to gain a better understanding of the object of research and generate research hypotheses. Second, quantitative SNA was conducted, using both egocentric SNA and complete SNA techniques. Network membership and network boundaries were defined by mixed-mode egocentric SNA. In a first step, a 12-page questionnaire was sent to all persons in each of the five municipalities listed in the data base of the UNDESD. In a second step, all persons from different sectors named more than once were also approached with the questionnaire [ 8 – 10 ]. Referring to Fischer [ 11 ] and Burt [ 12 , 13 ], a name generator was used which allowed to name all relevant persons in the field of ESD. In this way, nodes were only included if they were mentioned more than once by an interviewee in the field of ESD.
The questionnaire first asked respondents to mark people in their ESD network, defined by efforts to contact, cooperation, collaboration, problem-solving, and idea exchange. Respondents were also asked to assess the quality and contact frequency for each relation mentioned and to name those persons with whom the interviewee cooperated especially closely or had established high levels of trust. They were then requested to score their named connections’ impact and the relevance with respect to the diffusion of information and the implementation of ESD. Finally, the questionnaire included questions on future prospects, desires, and developmental possibilities.
Egocentric network data was aggregated in order to enable applications of complete SNA. The (strictly adjusted) dataset of the whole network of all five municipalities consists of 1,306 persons and 2,195 edges. Subsequent to the quantitative studies, qualitative network maps were created in order to gain deeper insights into the qualitative characteristics of the networks’ structural properties. Footnote 3 This article focuses mainly on results from the second and the third part of the data analysis.
Insights from structures and individuals: engaging top-down and bottom-up approaches
Empirical results were visualized drawing back on UCINET, Netdraw, and Pajek in order to provide a comprehensive foundation for stakeholders [ 14 , 15 ]. Top-down visualizations of network data were used to generate courses of action, guidance, and network management strategies with the persons involved in the process. Thus, network visualizations and empirical insights enabled stakeholders to detect weaknesses related to structural issues, information flows, and communication problems.
In order to visualize the networks, directional relations between network members were entered into UCINET and mapped with Netdraw. The iterative method of “spring embedding” was chosen for the graph-theoretic layout, because it supports neat illustrations of data sets. Thus, the lengths of the ties do not have information content. The nodes in network visualizations represent persons engaged in implementing ESD in their municipalities. Against the backdrop of the definition of network boundaries, persons that are represented by nodes with only one ingoing link and no outgoing link were not interviewed.
To give an example, one surprising result was the low level of cooperation beyond municipal borders, as measured by network connections, as seen in Fig. 1 .
Trans-regional ESD network, generated with the graph theoretical layout spring embedding, source: Author’s data
In contrast to Manuel Castells [ 16 ], who observed a diminishing relevance of space due to the information age, the present study finds that space remains a constraint for diffusion of ESD. It seems much easier to establish the social innovation ESD in the local context with dense network structures and to subsequently foster its diffusion through weak ties [ 17 , 18 ].
Furthermore, municipal stakeholders were confronted with the unexpected existence of many structural holes and brokerage positions. The concepts “brokerage” and “structural hole” refer to actors’ structural embeddedness. A person who maintains connections with people, who do themselves not become interconnected, has the ability to mediate between these contacts and to obtain benefits from his brokerage position [ 19 – 21 ]. At the same time, structural holes impede innovation processes and information flows.
Figures 2 and 3 take Erfurt and Gelsenkirchen as examples and show relations regarding to the question of who is contacted to develop new ideas related to ESD. Only those relationships with a contact frequency of at least once a month are represented in this figure. The ESD network of Erfurt is chosen as an extreme example, because the structure of its social network exhibits the highest number of structural holes.
Cooperation in the development of new ideas in Erfurt, source: Author’s data
Cooperation in the development of new ideas in Gelsenkirchen, source: Author’s data
There are only a few network members engaged in developing new ideas with respect to ESD in Erfurt; many structural holes shape the ESD network.
In contrast, cooperation in the development of new ideas related to ESD works very well in Gelsenkirchen, as seen in Fig. 3 .
Figure 3 presents productive relationships in Gelsenkirchen. Gelsenkirchen was chosen as an example here because it demonstrates a nearly perfect cooperation basis, which is very supportive for successful innovation processes. Such results can be used by involved actors in order to disclose strengths and weaknesses and reveal where and how structural conditions enable innovations and development processes.
The network visualizations shown so far are mainly reduced to structural information. Network visualizations can also integrate further actor-related information. Not least, structural characteristics of social networks, processes of innovation, idea exchange, and trust also depend on the areas of activity to which network members belong. Thus, Fig. 4 integrates some actor-specific information. Nodes represent those people who are actively engaged in the field of ESD in Alheim. The color of the nodes indicates the sector in which the relevant person deals with ESD. The size of the nodes correlates with the individual centrality index. Centrality is measured by the frequency of the responses—the indegree [ 1 , 22 ]. The more often a person was identified by others, the more central she appears in the picture. The thickness of the connections varies depending on its individual clustering value. While there are two different measures (global and local) for clustering, the local version was used to give an indication of the embeddedness of single nodes [ 23 ]. Thus, clustering is defined as the number of common acquaintances; the thickness of the arrow connecting two nodes points to the number of triangular connections.
ESD network in Alheim; color of the nodes according to the area of activity ( blue black : non-formal education, red : administration/policy, yellow : NGOs, green : economy, light blue : formal education, orange : church, grey : other areas), numbers indicate the IDs of individuals, illustration in cooperation with fas.research, source: Author’s data
Figure 4 indicates the central role that people in the field of non-formal education play in Alheim, as measured by how often they were named by other people. Another central position is held by someone in government. The big red node has many incoming and outgoing links, but few triangle relations and thus a low clustering value. Further comparative quantitative studies reveal that despite its high density value, there is little clustering in Alheim. Certainly, the clustering value always depends on the data collection process, but as the study for this article has used the same methodological approach for all five municipal networks, it is possible to compare the municipal clustering values. However, the low clustering value in Alheim is because cooperation beyond institutional borders works very well in this municipality and persons are not always connected to the same partners. The fear expressed by other municipalities, that ESD in Alheim would be dominated by powerful politicians, cannot be confirmed from these results.
In general, quantitative SNA is able to highlight network boundaries and structural characteristics of social networks that are important to understand innovation potential and impediments. It is difficult or even impossible, however, to reveal the causes, motivations, ideas, or perceptions that lie behind such network structures by solely drawing back on quantitative SNA. How, for example, can we explain the central role of one politician in Alheim, while there are many other central persons from non-formal education? What role does this central politician play for the clustering value in Alheim? In order to answer these questions, the study had to draw on further qualitative social network research methods. The researchers thus used a combination of egocentric network maps and semi-structured interviews.
Egocentric network maps are more individual-oriented than quantitative SNA methods. One benefit of network map visualizations lies in their potential for mental or cognitive support. Such visualizations are able to promote subjective validations of interview narratives as well as to highlight subjective perceptions, reasons, motivations, and network dynamics. The technique of structured and standardized network maps, which has often been described as the “method of concentric circles” [ 24 ], was chosen for this study [ 25 ]. Here, network maps are not only aids, but a main purpose of the survey. A sheet with four concentric circles is given to the interviewee. The inner circle represents the ego, that is to say the interviewee. Interviewees are then asked to draw the initials of people important to them personally, differentiated by the degree of emotional proximity or contact frequency. The three circles around the ego represent the emotional closeness or formal distance with respect to her or his alters (or connections). The closer to the ego, the tighter a contact person is perceived by the interviewee. In addition, the circles are divided in parts through lines; each part represents a different area of activity. In this way, interviewees can dedicate their contacts to specific areas of activity, such as civil society, formal education, non-formal education, business or government. The space around ego is structured by both concentric circles that illustrate the closeness of the alters to ego and the area of activity in which alters are engaged for ESD. An essential advantage of the structured and standardized instruments in relation to unstructured techniques lies in the comparability between different network cards (both intrapersonal and interpersonal).
At the same time, the high degree of structuring and standardization constrains the significance of the data obtained. Indications beyond the pre-fixed circles are only possible if interviewers get the opportunity to pose further questions or if interviewees are encouraged to further discuss issues that are not explicitly part of the visualization process. In order to combine both standardization and openness, this study enabled the interviewers to pose further important questions and explore relevant information related to the research aims. The application of egocentric network maps also served as a medium through which interviewees talked about their relationships. In this sense, network maps were integrated into semi-structured interviews in order to generate narratives and disclose relevant relationships and action orientation. In addition, interviewees had the opportunity to choose the categories representing different areas of activity as well as the colors for the visualizations. Thus, the technique implemented in the study supported the comparability of the cases, but it was also open for new variables and dimensions related to the specific context.
Altogether 25 network maps and interviews, five in every municipality, were generated. Interviewees were chosen according to their area of activity (to obtain a variance of the cases), their position within the social network, and their centrality indexes. To give an example, Fig. 5 presents the network map of a central politician in Alheim. This network map of Alheim is also chosen to further illustrate the case of Alheim, which was also depicted in Fig. 4 . Furthermore, this ESD actor in Alheim possesses a high centrality value according to quantitative SNA.
Network Map of a central politician in Alheim, anonymized, source: Author’s data
As Fig. 5 shows, the interviewee mainly distinguishes five areas of activity: civil society, educational institutions, government/administration, business, and persons from trans-regional contexts. In some cases, the politician just wrote down an organization. During the interview, he referred to concrete persons from these organizations. Surprisingly, the sector of government/administration, to which the interviewee himself belongs, is empty: no persons or organizations are indicated. This is also reflected in the visualization based on quantitative network data (Fig. 4 ), where only one individual from government plays a central role. In a sense, qualitative studies validate quantitative results by showing that the social innovation ESD in Alheim is mainly implemented by actors from non-formal education. At the same time, qualitative results stress that the topic is supported and disseminated by one central politician who bridges structural holes between different sectors. Furthermore, school actors are not represented in the network map, whereas the closest contact persons are from civil society, educational institutions, and business. The great variety of close contact persons from different sectors can be regarded as one reason for the success of the social innovation in Alheim. The central politician in Alheim himself mentions this as playing a significant role. Further actors within the community stress that the ideological foundation and the adoption of ESD would not be possible without this politician. Hence, the establishment of ESD in Alheim can also be traced back to its structural and discursive power and the general trust of ESD actors in this well-connected politician.
The central role of the interviewee in Alheim can be ascribed to the fact that he bridges institutional clusters, supports cooperation beyond government/administration, and combines close cooperation with weak ties in the field of ESD. Furthermore, centrality is not reduced to one person or one sector. Instead, actors from different sectors play a central role in the field of ESD and cooperation between state and non-state actors is very high. In this way, it was possible to develop and realize aims in the area of political accountability in a short space of time. The dense network structure, supported by strong relations between one central politician and actors from other sectors, resulted in the elaboration of an innovative educational plan, composed according to the principles of ESD. At the same time, future strategies should focus on integrating actors from other important areas such as schools. In addition, strategies that foster trans-regional cooperation would be helpful with the diffusion of ESD.
With respect to some of the municipalities, a future strategy that fosters greater participation of stakeholders from other areas of activity, as required by the UN’s International Implementation Scheme (IIS) and the National Action Plan of the UN Decade may be helpful in promoting the implementation and diffusion of the social innovation ESD. Business actors and teachers, in particular, complain about not being sufficiently integrated into ESD networks and that the same people always take control and create turf wars. Furthermore, a lack of transparency and information exchange on existing ESD projects was seen. Business actors in these municipalities faced biases from other actors concerned that they ignored ecological and social dimensions of sustainable development. In some municipalities, ESD is mainly concentrated on environmental topics and many ESD actors express reservations about business aims. However, if different sectors are not integrated, it’s difficult to achieve a balance between ecological, economic, and social dimensions, as it has been proclaimed by the concept of sustainable development as such.
This article has explored the role of Social Network Analysis in analyzing and supporting innovation processes. In order to better understand the opportunities of SNA in innovation research, the author presented empirical results of her own quantitative and qualitative research on innovation networks in five German municipalities actively engaged in the field of ESD. The article showed the value of using a combination of both quantitative and qualitative SNA in order to better understand how and why social innovations are implemented and the opportunities to further develop the network.
Quantitative SNA was implemented to analyze the impact of structural characteristics of social networks on the implementation and the diffusion of the social innovation of ESD. It was discovered, for example, that cooperation in the field of ESD mainly takes place within municipalities and that cooperation beyond municipal borders is low and marked by structural holes. Furthermore, it was shown that social networks in the area of ESD are mostly composed of small and dense groups each representing different sets of actors (e.g., local administration, educational institutions, and business) and pursuing different interests and ideas under the umbrella of ESD. Weak ties, on the contrary, are very important in the field of ESD as they are responsible for the diffusion of innovations.
However, structural holes also exist within the municipalities with respect to the quality of the relations. The extreme example of Erfurt illustrated how the development of new ideas can be hampered by structural weaknesses. In contrast, cooperation and innovation development in the field of ESD are regarded to work well in Gelsenkirchen. In Alheim, actors from different sectors are integrated. Most central roles are played by non-formal education actors, whereas one central role is wielded by a politician. In terms of innovation diffusion, Alheim can be regarded as a best practice. Not least, cooperation beyond institutional borders works well and individual clustering values are low: persons are not always connected to the same clusters. Finally, the implementation of ESD in Alheim benefits from strong relations between one well-connected political and actors from other sectors. The central politician connects different areas of activity and promotes the integration of ecological, economic, and social dimensions in terms of sustainable development.
Quantitative techniques of SNA enabled to identify innovation networks, to determine network boundaries, to define actors within the innovation network, and to investigate the network position of actors. Problems of coordination, information, and qualitative relations were discussed. At the same time, quantitative SNA was shown unable to analyze reasons, motivations, and perceptions behind network structure. These issues were then analyzed by using qualitative SNA methods, such as network maps. A combination of qualitative and quantitative SNA techniques may thus prove the most fruitful for innovation research. In order to better understand the role of social networks in the diffusion of social innovations and to generate knowledge related to innovation potential and courses of action, qualitative techniques were used to supplement the quantitative analysis. It was assumed that the costs of information exchange are not only material (money, time), but also social. Conflicts and lack of confidence between actors, for example, may prevent successful innovation diffusion. Qualitative egocentric network maps could validate quantitative results as well as disclose subjective perceptions and orientations. The central position of one politician in Alheim could thus be traced back to its discursive and structural power. Actors in Alheim have great trust in the ideological competences of the well-connected person who supports the establishment of ESD in many sectors. Visualizations with qualitative network maps support the completion of the interview situation with visual representations. Visualized networks can also serve as mental or cognitive assistance. In combination with quantitative results, however, qualitative network maps enable us to detect where and how innovations and development processes may be possible due to structural and subjective conditions. Finally, compared to conventional statistical analysis that treat structural and individual levels as separately, analyses and visualizations of network data give us more information about the influence of social relations. SNA enables us to capture the interaction between actors and social context, to better understand how innovations are implemented and diffused, to analyze how and why social or educational change takes place or does not take place, and to disclose opportunities for future strategies.
This contribution has shown that SNA can begin to answer questions related to innovation processes. I hope it will open new avenues for further uses of SNA in innovation and futures research.
At the same time, there is little research on the democratic implications of network governance [ 5 ] as well as on the strengths and limits of the concept related to issues of educational innovations such as Education for Sustainable Development (ESD).
When examined through the framework of Social Network Analysis (SNA), the deficits of the concept of ‘Educational Governance’ become evident. In the scientific literature and in educational and political praxis, the concept of Educational Governance is often exclusively related to institutions of formal learning such as schools or educational training. In this manner, it is not possible to capture the real boundaries of social networks and to conceptualize social networks as can be done with SNA techniques. Furthermore, many actors, initiatives, and activities that play an important role in learning processes are analytically excluded in current applications of Educational Governance. For that reason, this article does not use an Educational Governance approach. Instead, it uses a governance approach that draws on theoretical concepts developed in social science.
Qualitative network maps were gathered in cooperation with a research project coordinated by Inka Bormann.
Wasserman S, Faust K (2009) Social network analysis: Methods and applications. Cambridge University Press, Cambridge
Google Scholar
Wald A, Jansen D (2007) Netzwerke. In: Benz A, Lütz S, Schimank U, Simonis G (eds) Handbuch Governance. Theoretische Grundlagen und empirische Anwendungsbereich. VS Verlag für Sozialwissenschaften, Wiesbaden, pp 93–106
Zafonte M, Sabatier P (1998) Shared beliefs and imposed interdependencies as determinants of ally networks in overlapping subsystems. J Theo Pol 10(4):473–505
Article Google Scholar
Hajer M (2009) Authoritative Governance: Policy-making in the Age of Mediatization. Oxford University Press, Oxford
Book Google Scholar
Sørensen E, Torfing J (2005) Network governance and post-liberal democracy. Admin Theory Praxis J 27(2):197–237
Rogers EM (2003) Diffusion of innovations, 5th edn. Free Press, New York
Zapf W (1989) Über soziale innovationen. Soziale Welt 40(1/2):170–183
Straus F (2002) Netzwerkanalysen: gemeindepsychologische Perspektiven füür Forschung und Praxis. DUV, Wiesbaden
Wolf C (2010) Egozentrierte Netzwerke: Datenerhebung und Datenanalyse. In: Stegbauer C, Häußling R (eds) Handbuch Netzwerkforschung. VS Verlag für Sozialwissenschaften, Wiesbaden, pp 471–483
Chapter Google Scholar
Kolleck N (forthcoming) Qualität, Netzwerke und Vertrauen - Der Einsatz von Netzwerkanalysen in Qualitätsentwicklungsprozessen. Zeitschrift für Erziehungswissenschaft
Fischer C (1982) To Dwell among friends. Personal networks in Town and City. The University Press of Chicago, Chicago
Burt R (1982) Toward a structural theory of action: Network models of a social structure, perceptions and action. Academic, New York
Hennig M (2006) Individuen und ihre sozialen Beziehungen. VS Verlag für Sozialwissenschaften, Wiesbaden
Freeman LC (2000) Visualizing social networks. J Soc Struct 1 1. http://www.cmu.edu/joss/content/articles/volume1/Freeman.html . Accessed 09 July 2013
Krempel L (2005) Visualisierung komplexer Strukturen: Grundlagen der Darstellung mehrdimensionaler Netzwerke. Campus Verlag, Frankfurt
Castells M (2009) The rise of the network society: The information age: Economy, society, and culture, 2nd edn. Oxford, Wiley-Blackwell
Granovetter MS (1973) The strength of weak ties. Am J Soc 78(6):1360–1380
Granovetter MS (1985) Economic action and social structure: the problem of Embeddedness. Am J Soc 91(3):481–510
Burt R (1992) Structural holes. Harvard University Press, Cambridge
Burt R (2004) Structural holes and good ideas. Am J Soc 110(2):349–399
Podolny JM, Baron JN (1997) Resources and relationships: social networks and mobility in the workplace. Am Soc Rev 62(5):673–693
Freeman LC (1979) Centrality in social networks: conceptual clarification. Soc Net 1(3):215–239
Watts DJ, Strogatz SH (1998) Collective dynamics of ‘small-world’ networks. Nature 393(6684):440–442
Hollstein B, Pfeffer J (2010) Netzwerkkarten als instrument zur Erhebung egozentierter Netzwerke. http://www.wiso.uni-hamburg.de/fileadmin/sozialoekonomie/hollstein/Team/Hollstein_Betina/Literatur_Betina/Netzwerkkarten_Hollstein_Pfeffer_2010.pdf . Accessed 09 July 2013
Kahn RL, Antonucci TC (1980) Convoys over the life course. Attachment, roles, and social support. In: Baltes PB, Brim OG (eds) Life pan development and behaviour. Academic, New York, pp 253–286
Download references
Author information
Authors and affiliations.
Universität Heidelberg & Hertie School of Governance, Friedrichstraße 180, 10117, Berlin, Germany
Nina Kolleck
You can also search for this author in PubMed Google Scholar
Corresponding author
Correspondence to Nina Kolleck .
Additional information
I thank the editors and two anonymous reviewers for their constructive comments, which helped me to improve the article. The article is based on results of a study I conducted at the Freie Universität Berlin. I would also like to thank Gerhard de Haan for useful information and for supporting my research.
Rights and permissions
This article is published under license to BioMed Central Ltd. Open Access This article is distributed under the terms of the Creative Commons Attribution License which permits any use, distribution, and reproduction in any medium, provided the original author(s) and the source are credited.
Reprints and permissions
About this article
Cite this article.
Kolleck, N. Social network analysis in innovation research: using a mixed methods approach to analyze social innovations. Eur J Futures Res 1 , 25 (2013). https://doi.org/10.1007/s40309-013-0025-2
Download citation
Received : 29 July 2013
Accepted : 24 September 2013
Published : 20 October 2013
DOI : https://doi.org/10.1007/s40309-013-0025-2
Share this article
Anyone you share the following link with will be able to read this content:
Sorry, a shareable link is not currently available for this article.
Provided by the Springer Nature SharedIt content-sharing initiative
- Social network analysis
- Social innovations
- Education for sustainable development
- Egocentric network maps
This paper is in the following e-collection/theme issue:
Published on 16.4.2024 in Vol 26 (2024)
This is a member publication of University of Cambridge (Jisc)
Factors Influencing Recovery From Pediatric Stroke Based on Discussions From a UK-Based Online Stroke Community: Qualitative Thematic Study
Authors of this article:

There are no citations yet available for this article according to Crossref .
Advertisement
A review on sentiment analysis and emotion detection from text
- Review Paper
- Published: 28 August 2021
- Volume 11 , article number 81 , ( 2021 )
Cite this article
- Pansy Nandwani ORCID: orcid.org/0000-0001-7544-5395 1 &
- Rupali Verma 1
57k Accesses
199 Citations
19 Altmetric
Explore all metrics
Social networking platforms have become an essential means for communicating feelings to the entire world due to rapid expansion in the Internet era. Several people use textual content, pictures, audio, and video to express their feelings or viewpoints. Text communication via Web-based networking media, on the other hand, is somewhat overwhelming. Every second, a massive amount of unstructured data is generated on the Internet due to social media platforms. The data must be processed as rapidly as generated to comprehend human psychology, and it can be accomplished using sentiment analysis, which recognizes polarity in texts. It assesses whether the author has a negative, positive, or neutral attitude toward an item, administration, individual, or location. In some applications, sentiment analysis is insufficient and hence requires emotion detection, which determines an individual’s emotional/mental state precisely. This review paper provides understanding into levels of sentiment analysis, various emotion models, and the process of sentiment analysis and emotion detection from text. Finally, this paper discusses the challenges faced during sentiment and emotion analysis.
Similar content being viewed by others
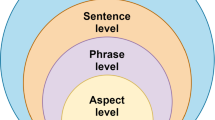
A survey on sentiment analysis methods, applications, and challenges
Mayur Wankhade, Annavarapu Chandra Sekhara Rao & Chaitanya Kulkarni
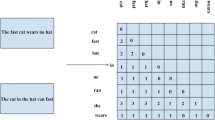
A supervised deep learning-based sentiment analysis by the implementation of Word2Vec and GloVe Embedding techniques
Pranati Rakshit & Avik Sarkar
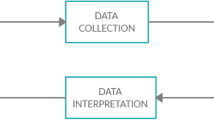
Sentiment Analysis in Social Media Data for Depression Detection Using Artificial Intelligence: A Review
Nirmal Varghese Babu & E. Grace Mary Kanaga
Avoid common mistakes on your manuscript.
1 Introduction
Human language understanding and human language generation are the two aspects of natural language processing (NLP). The former, however, is more difficult due to ambiguities in natural language. However, the former is more challenging due to ambiguities present in natural language. Speech recognition, document summarization, question answering, speech synthesis, machine translation, and other applications all employ NLP (Itani et al. 2017 ). The two critical areas of natural language processing are sentiment analysis and emotion recognition. Even though these two names are sometimes used interchangeably, they differ in a few respects. Sentiment analysis is a means of assessing if data is positive, negative, or neutral.
In contrast, Emotion detection is a means of identifying distinct human emotion types such as furious, cheerful, or depressed. “Emotion detection,” “affective computing,” “emotion analysis,” and “emotion identification” are all phrases that are sometimes used interchangeably (Munezero et al. 2014 ). People are using social media to communicate their feelings since Internet services have improved. On social media, people freely express their feelings, arguments, opinions on wide range of topics. In addition, many users give feedbacks and reviews various products and services on various e-commerce sites. User's ratings and reviews on multiple platforms encourage vendors and service providers to enhance their current systems, goods, or services. Today almost every industry or company is undergoing some digital transition, resulting in vast amounts of structured and unstructured increase data. The enormous task for companies is to transform unstructured data into meaningful insights that can help them in decision-making (Ahmad et al. 2020 )
For instance, in the business world, vendors use social media platforms such as Instagram, YouTube, Twitter, and Facebook to broadcast information about their product and efficiently collect client feedback (Agbehadji and Ijabadeniyi 2021 ). People’s active feedback is valuable not only for business marketers to measure customer satisfaction and keep track of the competition but also for consumers who want to learn more about a product or service before buying it. Sentiment analysis assists marketers in understanding their customer's perspectives better so that they may make necessary changes to their products or services (Jang et al. 2013 ; Al Ajrawi et al. 2021 ). In both advanced and emerging nations, the impact of business and client sentiment on stock market performance may be witnessed. In addition, the rise of social media has made it easier and faster for investors to interact in the stock market. As a result, investor's sentiments impact their investment decisions which can swiftly spread and magnify over the network, and the stock market can be altered to some extent (Ahmed 2020 ). As a result, sentiment and emotion analysis has changed the way we conduct business (Bhardwaj et al. 2015 ).
In the healthcare sector, online social media like Twitter have become essential sources of health-related information provided by healthcare professionals and citizens. For example, people have been sharing their thoughts, opinions, and feelings on the Covid-19 pandemic (Garcia and Berton 2021 ). Patients were directed to stay isolated from their loved ones, which harmed their mental health. To save patients from mental health issues like depression, health practitioners must use automated sentiment and emotion analysis (Singh et al. 2021 ). People commonly share their feelings or beliefs on sites through their posts, and if someone seemed to be depressed, people could reach out to them to help, thus averting deteriorated mental health conditions.
Sentiment and emotion analysis plays a critical role in the education sector, both for teachers and students. The efficacy of a teacher is decided not only by his academic credentials but also by his enthusiasm, talent, and dedication. Taking timely feedback from students is the most effective technique for a teacher to improve teaching approaches (Sangeetha and Prabha 2020 ). Open-ended textual feedback is difficult to observe, and it is also challenging to derive conclusions manually. The findings of a sentiment analysis and emotion analysis assist teachers and organizations in taking corrective action. Since social site's inception, educational institutes are increasingly relying on social media like Facebook and Twitter for marketing and advertising purposes. Students and guardians conduct considerable online research and learn more about the potential institution, courses and professors. They use blogs and other discussion forums to interact with students who share similar interests and to assess the quality of possible colleges and universities. Thus, applying sentiment and emotion analysis can help the student to select the best institute or teacher in his registration process (Archana Rao and Baglodi 2017 ).
Sentiment and emotion analysis has a wide range of applications and can be done using various methodologies. There are three types of sentiment and emotion analysis techniques: lexicon based, machine learning based, and deep learning based. Each has its own set of benefits and drawbacks. Despite different sentiment and emotion recognition techniques, researchers face significant challenges, including dealing with context, ridicule, statements conveying several emotions, spreading Web slang, and lexical and syntactical ambiguity. Furthermore, because there are no standard rules for communicating feelings across multiple platforms, some express them with incredible effect, some stifle their feelings, and some structure their message logically. Therefore, it is a great challenge for researchers to develop a technique that can efficiently work in all domains.
In this review paper, Sect. 2 , introduces sentiment analysis and its various levels, emotion detection, and psychological models. Section 3 discusses multiple steps involved in sentiment and emotion analysis, including datasets, pre-processing of text, feature extraction techniques, and various sentiment and emotion analysis approaches. Section 4 addresses multiple challenges faced by researchers during sentiment and emotion analysis. Finally, Sect. 5 concludes the work.
2 Background
2.1 sentiment analysis.
Many people worldwide are now using blogs, forums, and social media sites such as Twitter and Facebook to share their opinions with the rest of the globe. Social media has become one of the most effective communication media available. As a result, an ample amount of data is generated, called big data, and sentiment analysis was introduced to analyze this big data effectively and efficiently (Nagamanjula and Pethalakshmi 2020 ). It has become exceptionally crucial for industry or organization to comprehend the sentiments of the user. Sentiment analysis, often known as opinion mining, is a method for detecting whether an author’s or user’s viewpoint on a subject is positive or negative. Sentiment analysis is defined as the process of obtaining meaningful information and semantics from text using natural processing techniques and determining the writer’s attitude, which might be positive, negative, or neutral (Onyenwe et al. 2020 ). Since the purpose of sentiment analysis is to determine polarity and categorize opinionated texts as positive or negative, dataset’s class range involved in sentiment analysis is not restricted to just positive or negative; it can be agreed or disagreed, good or bad. It can also be quantified on a 5-point scale: strongly disagree, disagree, neutral, agree, or strongly agree (Prabowo and Thelwall 2009 ). For instance, Ye et al. ( 2009 ) applied sentiment analysis on reviews on European and US destinations labeled on the scale of 1 to 5. They associated 1-star or 2-star reviews with the negative polarity and more than 2-star reviews with positive polarity. Gräbner et al. ( 2012 ) built a domain-specific lexicon that consists of tokens with their sentiment value. These tokens were gathered from customer reviews in the tourism domain to classify sentiment into 5-star ratings from terrible to excellent in the tourism domain. Moreover, Sentiment analysis from the text can be performed at three levels discussed in the following section. Salinca ( 2015 ) applied machine learning algorithms on the Yelp dataset, which contains reviews on service providers scaled from 1 to 5. Sentiment analysis can be categorized at three levels, mentioned in the following section.
2.1.1 Levels of sentiment analysis
Sentiment analysis is possible at three levels: sentence level, document level, and aspect level. At the sentence-level or phrase-level sentiment analysis, documents or paragraphs are broken down into sentences, and each sentence’s polarity is identified (Meena and Prabhakar 2007 ; Arulmurugan et al. 2019 ; Shirsat et al. 2019 ). At the document level, the sentiment is detected from the entire document or record (Pu et al. 2019 ). The necessity of document-level sentiment analysis is to extract global sentiment from long texts that contain redundant local patterns and lots of noise. The most challenging aspect of document-level sentiment classification is taking into account the link between words and phrases and the full context of semantic information to reflect document composition (Rao et al. 2018 ; Liu et al. 2020a ). It necessitates a deeper understanding of the intricate internal structure of sentiments and dependent words (Liu et al. 2020b ). At the aspect level, sentiment analysis, opinion about a specific aspect or feature is determined. For instance, the speed of the processor is high, but this product is overpriced. Here, speed and cost are two aspects or viewpoints. Speed is mentioned in the sentence hence called explicit aspect, whereas cost is an implicit aspect. Aspect-level sentiment analysis is a bit harder than the other two as implicit features are hard to identify. Devi Sri Nandhini and Pradeep ( 2020 ) proposed an algorithm to extract implicit aspects from documents based on the frequency of co-occurrence of aspect with feature indicator and by exploiting the relation between opinionated words and explicit aspects. Ma et al. ( 2019 ) took care of the two issues concerning aspect-level analysis: various aspects in a single sentence having different polarities and explicit position of context in an opinionated sentence. The authors built up a two-stage model based on LSTM with an attention mechanism to solve these issues. They proposed this model based on the assumption that context words near to aspect are more relevant and need greater attention than farther context words. At stage one, the model exploits multiple aspects in a sentence one by one with a position attention mechanism. Then, at the second state, it identifies (aspect, sentence) pairs according to the position of aspect and context around it and calculates the polarity of each team simultaneously.
As stated earlier, sentiment analysis and emotion analysis are often used interchangeably by researchers. However, they differ in a few ways. In sentiment analysis, polarity is the primary concern, whereas, in emotion detection, the emotional or psychological state or mood is detected. Sentiment analysis is exceptionally subjective, whereas emotion detection is more objective and precise. Section 2.2 describes all about emotion detection in detail.
2.2 Emotion detection
Emotions are an inseparable component of human life. These emotions influence human decision-making and help us communicate to the world in a better way. Emotion detection, also known as emotion recognition, is the process of identifying a person’s various feelings or emotions (for example, joy, sadness, or fury). Researchers have been working hard to automate emotion recognition for the past few years. However, some physical activities such as heart rate, shivering of hands, sweating, and voice pitch also convey a person’s emotional state (Kratzwald et al. 2018 ), but emotion detection from text is quite hard. In addition, various ambiguities and new slang or terminologies being introduced with each passing day make emotion detection from text more challenging. Furthermore, emotion detection is not just restricted to identifying the primary psychological conditions (happy, sad, anger); instead, it tends to reach up to 6-scale or 8-scale depending on the emotion model.
2.2.1 Emotion models/emotion theories
In English, the word 'emotion' came into existence in the seventeenth century, derived from the French word 'emotion, meaning a physical disturbance. Before the nineteenth century, passion, appetite, and affections were categorized as mental states. In the nineteenth century, the word 'emotion' was considered a psychological term (Dixon 2012 ). In psychology, complex states of feeling lead to a change in thoughts, actions, behavior, and personality referred to as emotions. Broadly, psychological or emotion models are classified into two categories: dimensional and categorical.
Dimensional Emotion model This model represents emotions based on three parameters: valence, arousal, and power (Bakker et al. 2014 . Valence means polarity, and arousal means how exciting a feeling is. For example, delighted is more exciting than happy. Power or dominance signifies restriction over emotion. These parameters decide the position of psychological states in 2-dimensional space, as illustrated in Fig. 1 .
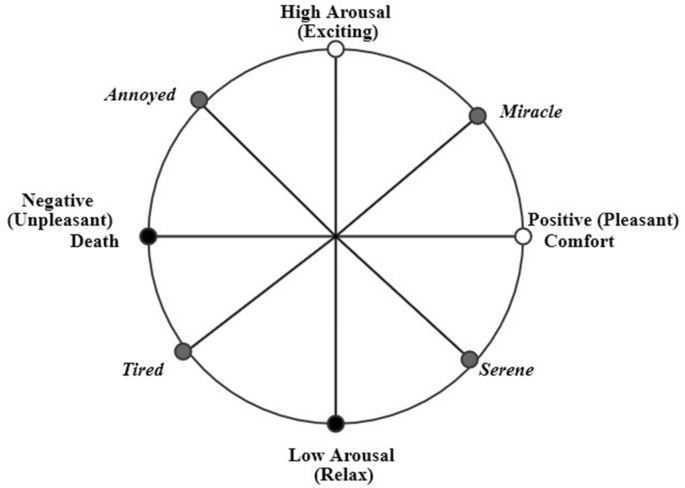
Dimensional model of emotions
Categorical Emotion model
In the categorical model, emotions are defined discretely, such as anger, happiness, sadness, and fear. Depending upon the particular categorical model, emotions are categorized into four, six, or eight categories.
Table 1 demonstrates numerous emotion models that are dimensional and categorical. In the realm of emotion detection, most researchers adopted Ekman and Plutchik’s emotion model. The emotional states defined by the models make up the set of labels used to annotate the sentences or documents. Batbaatar et al. ( 2019 ), Becker et al. ( 2017 ), Jain et al. ( 2017 ) adopted Ekman’s six basic emotions. Sailunaz and Alhajj ( 2019 ) used Ekman models for annotating tweets. Some researchers used customized emotion models by extending the model with one or two additional states. Roberts et al. ( 2012 ) used the Ekman model to annotate the tweets with the 'love' state. Ahmad et al. ( 2020 ) adopted the wheel of emotion modeled by Plutchik for labeling Hindi sentences with nine different Plutchik model states, decreasing semantic confusion, among other words. Plutchik and Ekman’s model's states are also utilized in various handcrafted lexicons like WordNet-Affect (Strapparava et al. 2004 ) and NRC (Mohammad and Turney 2013 ) word–emotion lexicons. Laubert and Parlamis ( 2019 ) referred to the Shaver model because of its three-level hierarchy structure of emotions. Valence or polarity is presented at the first level, followed by the second level consisting of five emotions, and the third level shows discrete 24 emotion states. Some researchers did not refer to any model and classified the dataset into three basic feelings: happy, sad, or angry.
Figure 2 depicts the numerous emotional states that can be found in various models. These states are plotted on a four-axis by taking the Plutchik model as a base model. The most commonly used emotion states in different models include anger, fear, joy, surprise, and disgust, as depicted in the figure above. It can be seen from the figure that emotions on two sides of the axis will not always be opposite of each other. For example, sadness and joy are opposites, but anger is not the opposite of fear.
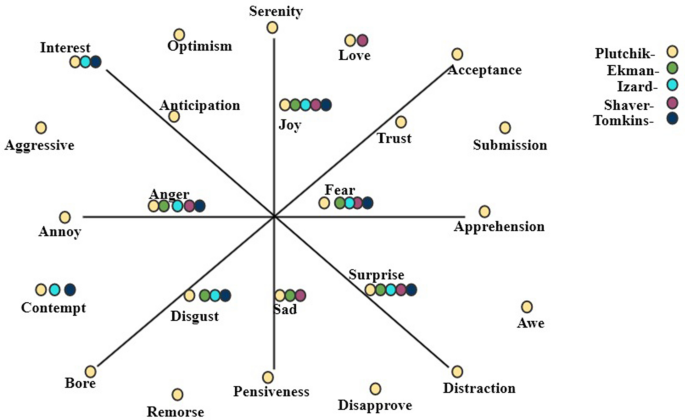
Illustration of various emotional models with some psychological states
3 Process of sentiment analysis and emotion detection
Process of sentiment analysis and emotion detection comes across various stages like collecting dataset, pre-processing, feature extraction, model development, and evaluation, as shown in Fig. 3 .
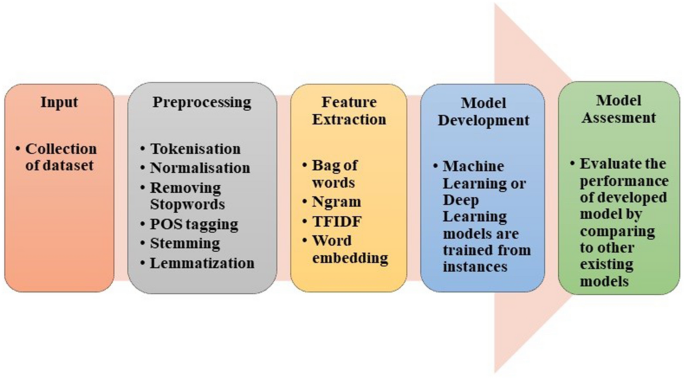
Basic steps to perform sentiment analysis and emotion detection
3.1 Datasets for sentiment analysis and emotion detection
Table 2 lists numerous sentiment and emotion analysis datasets that researchers have used to assess the effectiveness of their models. The most common datasets are SemEval, Stanford sentiment treebank (SST), international survey of emotional antecedents and reactions (ISEAR) in the field of sentiment and emotion analysis. SemEval and SST datasets have various variants which differ in terms of domain, size, etc. ISEAR was collected from multiple respondents who felt one of the seven emotions (mentioned in the table) in some situations. The table shows that datasets include mainly the tweets, reviews, feedbacks, stories, etc. A dimensional model named valence, arousal dominance model (VAD) is used in the EmoBank dataset collected from news, blogs, letters, etc. Many studies have acquired data from social media sites such as Twitter, YouTube, and Facebook and had it labeled by language and psychology experts in the literature. Data crawled from various social media platform's posts, blogs, e-commerce sites are usually unstructured and thus need to be processed to make it structured to reduce some additional computations outlined in the following section.
3.2 Pre-processing of text
On social media, people usually communicate their feelings and emotions in effortless ways. As a result, the data obtained from these social media platform's posts, audits, comments, remarks, and criticisms are highly unstructured, making sentiment and emotion analysis difficult for machines. As a result, pre-processing is a critical stage in data cleaning since the data quality significantly impacts many approaches that follow pre-processing. The organization of a dataset necessitates pre-processing, including tokenization, stop word removal, POS tagging, etc. (Abdi et al. 2019 ; Bhaskar et al. 2015 ). Some of these pre-processing techniques can result in the loss of crucial information for sentiment and emotion analysis, which must be addressed.
Tokenization is the process of breaking down either the whole document or paragraph or just one sentence into chunks of words called tokens (Nagarajan and Gandhi 2019 ). For instance, consider the sentence “this place is so beautiful” and post-tokenization, it will become 'this,' "place," is, "so," beautiful.’ It is essential to normalize the text for achieving uniformity in data by converting the text into standard form, correcting the spelling of words, etc. (Ahuja et al. 2019 ).
Unnecessary words like articles and some prepositions that do not contribute toward emotion recognition and sentiment analysis must be removed. For instance, stop words like "is," "at," "an," "the" have nothing to do with sentiments, so these need to be removed to avoid unnecessary computations (Bhaskar et al. 2015 ; Abdi et al. 2019 ). POS tagging is the way to identify different parts of speech in a sentence. This step is beneficial in finding various aspects from a sentence that are generally described by nouns or noun phrases while sentiments and emotions are conveyed by adjectives (Sun et al. 2017 ).
Stemming and lemmatization are two crucial steps of pre-processing. In stemming, words are converted to their root form by truncating suffixes. For example, the terms "argued" and "argue" become "argue." This process reduces the unwanted computation of sentences (Kratzwald et al. 2018 ; Akilandeswari and Jothi 2018 ). Lemmatization involves morphological analysis to remove inflectional endings from a token to turn it into the base word lemma (Ghanbari-Adivi and Mosleh 2019 ). For instance, the term "caught" is converted into "catch" (Ahuja et al. 2019 ). Symeonidis et al. ( 2018 ) examined the performance of four machine learning models with a combination and ablation study of various pre-processing techniques on two datasets, namely SS-Tweet and SemEval. The authors concluded that removing numbers and lemmatization enhanced accuracy, whereas removing punctuation did not affect accuracy.
3.3 Feature extraction
The machine understands text in terms of numbers. The process of converting or mapping the text or words to real-valued vectors is called word vectorization or word embedding. It is a feature extraction technique wherein a document is broken down into sentences that are further broken into words; after that, the feature map or matrix is built. In the resulting matrix, each row represents a sentence or document while each feature column represents a word in the dictionary, and the values present in the cells of the feature map generally signify the count of the word in the sentence or document. To carry out feature extraction, one of the most straightforward methods used is 'Bag of Words' (BOW), in which a fixed-length vector of the count is defined where each entry corresponds to a word in a pre-defined dictionary of words. The word in a sentence is assigned a count of 0 if it is not present in the pre-defined dictionary, otherwise a count of greater than or equal to 1 depending on how many times it appears in the sentence. That is why the length of the vector is always equal to the words present in the dictionary. The advantage of this technique is its easy implementation but has significant drawbacks as it leads to a sparse matrix, loses the order of words in the sentence, and does not capture the meaning of a sentence (Bandhakavi et al. 2017 ; Abdi et al. 2019 ). For example, to represent the text “are you enjoying reading” from the pre-defined dictionary I, Hope, you, are, enjoying, reading would be (0,0,1,1,1,1). However, these representations can be improved by pre-processing of text and by utilizing n-gram, TF-IDF.
The N-gram method is an excellent option to resolve the order of words in sentence vector representation. In an n-gram vector representation, the text is represented as a collaboration of unique n-gram means groups of n adjacent terms or words. The value of n can be any natural number. For example, consider the sentence “to teach is to touch a life forever” and n = 3 called trigram will generate 'to teach is,' 'teach is to,' 'is to touch,' 'to touch a,' 'touch a life,' 'a life forever.' In this way, the order of the sentence can be maintained (Ahuja et al. 2019 ). N-grams features perform better than the BOW approach as they cover syntactic patterns, including critical information (Chaffar and Inkpen 2011 ). However, though n-gram maintains the order of words, it has high dimensionality and data sparsity (Le and Mikolov 2014 ).
Term frequency-inverse document frequency, usually abbreviated as TFIDF, is another method commonly used for feature extraction. This method represents text in matrix form, where each number quantifies how much information these terms carry in a given document. It is built on the premise that rare terms have much information in the text document (Liu et al. 2019 ). Term frequency is the number of times a word w appears in a document divided by the total number of words W in the document, and IDF is log (total number of documents (N) divided by the total number of documents in which word w appears (n)) (Songbo and Jin 2008 ). Ahuja et al. ( 2019 ) implemented six pre-processing techniques and compared two feature extraction techniques to identify the best approach. They applied six machine learning algorithms and used n-grams with n = 2 and TF-IDF for feature extraction over the SS-tweet dataset and concluded TF-IDF gives better performance over n-gram.
The availability of vast volumes of data allows a deep learning network to discover good vector representations. Feature extraction with word embedding based on neural networks is more informative. In neural network-based word embedding, the words with the same semantics or those related to each other are represented by similar vectors. This is more popular in word prediction as it retains the semantics of words. Google’s research team, headed by Tomas Mikolov, developed a model named Word2Vec for word embedding. With Word2Vec, it is possible to understand for a machine that “queen” + “female” + “male” vector representation would be the same as a vector representation of “king” (Souma et al. 2019 ).
Other examples of deep learning-based word embedding models include GloVe, developed by researchers at Stanford University, and FastText, introduced by Facebook. GloVe vectors are faster to train than Word2vec. FastText vectors have better accuracy as compared to Word2Vec vectors by several varying measures. Yang et al. ( 2018 ) proved that the choice of appropriate word embedding based on neural networks could lead to significant improvements even in the case of out of vocabulary (OOV) words. Authors compared various word embeddings, trained using Twitter and Wikipedia as corpora with TF-IDF word embedding.
3.4 Techniques for sentiment analysis and emotion detection
Figure 4 presents various techniques for sentiment analysis and emotion detection which are broadly classified into a lexicon-based approach, machine learning-based approach, deep learning-based approach. The hybrid approach is a combination of statistical and machine learning approaches to overcome the drawbacks of both approaches. Transfer learning is also a subset of machine learning which allows the use of the pre-trained model in other similar domain.
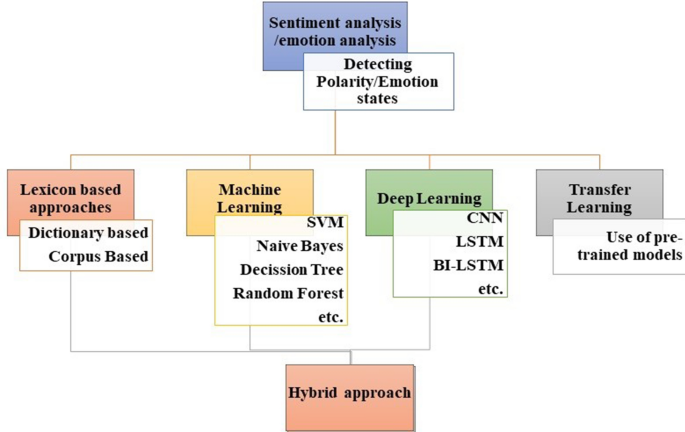
Techniques for sentiment analysis and emotion detection
3.4.1 Sentiment analysis techniques
Lexicon-based approach This method maintains a word dictionary in which each positive and negative word is assigned a sentiment value. Then, the sum or mean of sentiment values is used to calculate the sentiment of the entire sentence or document. However, Jurek et al. ( 2015 ) tried a different approach called the normalization function to calculate the sentiment value more accurately than this basic summation and mean function. Dictionary-based approach and corpus-based approach are two types of lexicon-based approaches based on sentiment lexicon. In general, a dictionary maintains words of some language systemically, whereas a corpus is a random sample of text in some language. The exact meaning applies here in the dictionary-based approach and corpus-based approach. In the dictionary-based approach, a dictionary of seed words is maintained (Schouten and Frasincar 2015 ). To create this dictionary, the first small set of sentiment words, possibly with very short contexts like negations, is collected along with its polarity labels (Bernabé-Moreno et al. 2020 ). The dictionary is then updated by looking for their synonymous (words with the same polarity) and antonymous (words with opposite polarity). The accuracy of sentiment analysis via this approach will depend on the algorithm. However, this technique does not contain domain specificity. The Corpus-based approach solves the limitations of the dictionary-based approach by including domain-specific sentiment words where the polarity label is assigned to the sentiment word according to its context or domain. It is a data-driven approach where sentiment words along with context can be accessed. This approach can certainly be a rule-based approach with some NLP parsing techniques. Thus corpus-based approach tends to have poor generalization but can attain excellent performance within a particular domain. Since the dictionary-based approach does not consider the context around the sentiment word, it leads to less efficiency. Thus, Cho et al. ( 2014 ) explicitly handled the contextual polarity to make dictionaries adaptable in multiple domains with a data-driven approach. They took a three-step strategy: merge various dictionaries, remove the words that do not contribute toward classification, and switch the polarity according to a particular domain.
SentiWordNet (Esuli and Sebastiani 2006 ) and Valence Aware Dictionary and Sentiment Reasoner (VADER) (Hutto and Gilbert 2014 ) are popular lexicons in sentiment. Jha et al. ( 2018 ) tried to extend the lexicon application in multiple domains by creating a sentiment dictionary named Hindi Multi-Domain Sentiment Aware Dictionary (HMDSAD) for document-level sentiment analysis. This dictionary can be used to annotate the reviews into positive and negative. The proposed method labeled 24% more words than the traditional general lexicon Hindi Sentiwordnet (HSWN), a domain-specific lexicon. The semantic relationships between words in traditional lexicons have not been examined, improving sentiment classification performance. Based on this premise, Viegas et al. ( 2020 ) updated the lexicon by including additional terms after utilizing word embeddings to discover sentiment values for these words automatically. These sentiment values were derived from “nearby” word embeddings of already existing words in the lexicon.
Machine Learning-based approach There is another approach for sentiment analysis called the machine learning approach. The entire dataset is divided into two parts for training and testing purposes: a training dataset and a testing dataset. The training dataset is the information used to train the model by supplying the characteristics of different instances of an item. The testing dataset is then used to see how successfully the model from the training dataset has been trained. Generally, the machine learning algorithms used for sentiment analysis fall under supervised classification. Different kinds of algorithms required for sentiment classification may include Naïve Bayes, support vector machine (SVM), decision trees, etc. each having its pros and cons. Gamon ( 2004 ) applied a support vector machine over 40,884 customer feedbacks collected from surveys. The authors implemented various feature set combinations and achieved accuracy up to 85.47%. Ye et al. ( 2009 ) worked with SVM, N-gram model, and Naïve Bayes on sentiment and review on seven popular destinations of Europe and the USA, which was collected from yahoo.com. The authors achieved an accuracy of up to 87.17% with the n-gram model. indent Bučar et al. ( 2018 ) created the lexicon called JOB 1.0 and labeled news corpora called SentiNews 1.0 for sentiment analysis in Slovene texts. JOB 1.0 consists of 25,524 headwords extended with sentiment scaling from – 5 to 5 based on the AFINN model. For the construction of corpora, data were scraped from various news Web media. Then, after cleaning and pre-processing of data, the annotators were asked to annotate 10,427 documents on the 1–5 scale where one means negative and 5 means very positive. Then these documents were labeled with positive, negative, and neutral labels as per the specific average scale rating. The authors observed that Naïve Bayes performed better as compared to the support vector machine (SVM). Naive Bayes achieved an F1 score above 90% in binary classification and an F1 score above 60% for the three-class classification of sentiments. Tiwari et al. ( 2020 ) implemented three machine learning algorithms called SVM, Naive Bayes, and maximum entropy with the n-gram feature extraction method on the rotten tomato dataset. The training and testing dataset constituted 1600 reviews in each. The authors observed a decrease in accuracy with higher values of n in n-grams such as n = four, five, and six. Soumya and Pramod ( 2020 ) classified 3184 Malayalam tweets into positive and negative opinions using different feature vectors like BOW, Unigram with Sentiwordnet, etc. The authors implemented machine learning algorithms like random forest and Naïve Bayes and observed that the random forest with an accuracy of 95.6% performs better with Unigram Sentiwordnet considering negation words.
Deep Learning-based Approach In recent years, deep learning algorithms are dominating other traditional approaches for sentiment analysis. These algorithms detect the sentiments or opinions from text without doing feature engineering. There are multiple deep learning algorithms, namely recurrent neural network and convolutional neural networks, that can be applied to sentiment analysis and gives results that are more accurate than those provided by machine learning models. This approach makes humans free from constructing the features from text manually as deep learning models extract those features or patterns themselves. Jian et al. ( 2010 ) used a model based upon neural networks technology for categorizing sentiments which consisted of sentimental features, feature weight vectors, and prior knowledge base. The authors applied the model to review the data of Cornell movie. The experimental results of this paper revealed that the accuracy level of the I-model is extraordinary compared to HMM and SVM. Pasupa and Ayutthaya ( 2019 ) executed five-fold cross-validation on the children’s tale (Thai) dataset and compared three deep learning models called CNN, LSTM, and Bi-LSTM. These models are applied with or without features: POS-tagging (pre-processing technique to identify different parts of speech); Thai2Vec (word embedding trained from Thai Wikipedia); sentic (to understand the sentiment of the word). The authors observed the best performance in the CNN model with all the three features mentioned earlier. As stated earlier, social media platforms act as a significant source of data in the field of sentiment analysis. Data collected from this social sites consist lot of noise due to its free writing syle of users. Therefore, Arora and Kansal ( 2019 ) proposed a model named Conv-char-Emb that can handle the problem of noisy data and use small memory space for embedding. For embedding, convolution neural network (CNN) has been used that uses less parameters in feature representation. Dashtipour et al. ( 2020 ) proposed a deep learning framework to carry out sentiment analysis in the Persian language. The researchers concluded that deep neural networks such as LSTM and CNN outperformed the existing machine learning algorithms on the hotel and product review dataset.
Transfer Learning Approach and Hybrid Approach Transfer learning is also a part of machine learning. A model trained on large datasets to resolve one problem can be applied to other related issues. Re-using a pre-trained model on related domains as a starting point can save time and produce more efficient results. Zhang et al. ( 2012 ) proposed a novel instance learning method by directly modeling the distribution between different domains. Authors classified the dataset: Amazon product reviews and Twitter dataset into positive and negative sentiments. Tao and Fang ( 2020 ) proposed extending recent classification methods in aspect-based sentiment analysis to multi-label classification. The authors also developed transfer learning models called XLNet and Bert and evaluated the proposed approach on different datasets Yelp, wine reviews rotten tomato dataset from other domains. Deep learning and machine learning approaches yield good results, but the hybrid approach can give better results since it overcomes the limitations of each traditional model. Mladenović et al. ( 2016 ) proposed a feature reduction technique, a hybrid framework made of sentiment lexicon and Serbian wordnet. The authors expanded both lexicons by addition some morphological sentiment words to avoid loss of critical information while stemming. Al Amrani et al. ( 2018 ) compared their hybrid model made of SVM and random forest model, i.e., RFSVM, on amazon’s product reviews. The authors concluded RFSVM, with an accuracy level of 83.4%, performs better than SVM with 82.4% accuracy and random forest with 81% accuracy individually over the dataset of 1000 reviews. Alqaryouti et al. ( 2020 ) proposed the hybrid of the rule-based approach and domain lexicons for aspect-level sentiment detection to understand people’s opinions regarding government smart applications. The authors concluded that the proposed technique outperforms other lexicon-based baseline models by 5%. Ray and Chakrabarti ( 2020 ) combined the rule-based approach to extract aspects with a 7-layer deep learning CNN model to tag each aspect. The hybrid model achieved 87% accuracy, whereas the individual models had 75% accuracy with rule-based and 80% accuracy with the CNN model.
Table 3 describes various machine learning and deep learning algorithms used for analyzing sentiments in multiple domains. Many researchers implemented the proposed models on their dataset collected from Twitter and other social networking sites. The authors then compared their proposed models with other existing baseline models and different datasets. It is observed from the table above that accuracy by various models ranges from 80 to 90%.
3.4.2 Emotion detection techniques
Lexicon-based Approach Lexicon-based approach is a keyword-based search approach that searches for emotion keywords assigned to some psychological states (Rabeya et al. 2017 ). The popular lexicons for emotion detection are WordNet-Affect (Strapparava et al. 2004 and NRC word–emotion lexicon (Mohammad and Turney 2013 ). WordNet-Affect is an extended form of WordNet which consists of affective words annotated with emotion labels. NRC lexicon consists of 14,182 words, each assigned to one particular emotion and two sentiments. These lexicons are categorical lexicons that tag each word with an emotional state for emotion classification. However, by ignoring the intensity of emotions, these traditional lexicons become less informative and less adaptable. Thus, Li et al. ( 2021 ) suggested an effective strategy to obtain word-level emotion distribution to assign emotions with intensities to the sentiment words by merging a dimensional dictionary named NRC-Valence arousal dominance. EmoSenticNet (Poria et al. 2014 ) also consists of a large number assigned to both qualitative and quantitative labels. Generally, researchers generate their lexicons and directly apply them for emotion analysis, but lexicons can also be used for feature extraction purposes. Abdaoui et al. ( 2017 ) took the benefit of using online translation tools to create a French lexicon called FEEL (French expanded emotion lexicon) consisting of more than 14,000 words with both polarity and emotion labels. This lexicon was created by increasing the number of words in the NRC emotion lexicon and semi-automatic translation using six online translators. Those entries obtained from at least three translators were considered pre-validated and then validated by the manual translator. Bandhakavi et al. ( 2017 ) applied a domain-specific lexicon for the process of feature extraction in emotion analysis. The authors concluded that features derived from their proposed lexicon outperformed the other baseline features. Braun et al. ( 2021 ) constructed a multilingual corpus called MEmoFC, which stands for Multilingual Emotional Football Corpus, consisting of football reports from English, Dutch and German Web sites and match statistics crawled from Goal.com. The corpus was created by creating two metadata tables: one explaining details of a match like a date, place, participation teams, etc., and the second table consisted of abbreviations of football clubs. Authors demonstrated the corpus with various approaches to know the influence of the reports on game outcomes.
Machine Learning-based Techniques Emotion detection or classification may require different types of machine learning models such as Naïve Bayes, support vector machine, decision trees, etc. Jain et al. ( 2017 ) extracted the emotions from multilingual texts collected from three different domains. The authors used a novel approach called rich site summary for data collection and applied SVM and Naïve Bayes machine learning algorithms for emotion classification of twitter text. Results revealed that an accuracy level of 71.4% was achieved with the Naïve Bayes algorithm. Hasan et al. ( 2019 ) evaluated the machine learning algorithms like Naïve Bayes, SVM, and decision trees to identify emotions in text messages. The task is divided into two subtasks: Task 1 includes a collection of the dataset from Twitter and automatic labeling of the dataset using hashtags and model training. Task 2 is developing a two-stage EmotexStream that separates emotionless tweets at the first stage and identifies emotions in the text by utilizing the models trained in the task1. The authors observed accuracy of 90% in classifying emotions. Asghar et al. ( 2019 ) aimed to apply multiple machine learning models on the ISEAR dataset to find the best classifier. They found that the logistic regression model performed better than other classifiers with a recall value of 83%.
Deep Learning and Hybrid Technique Deep learning area is part of machine learning that processes information or signals in the same way as the human brain does. Deep learning models contain multiple layers of neurons. Thousands of neurons are interconnected to each other, which speeds up the processing in a parallel fashion. Chatterjee et al. ( 2019 ) developed a model called sentiment and semantic emotion detection (SSBED) by feeding sentiment and semantic representations to two LSTM layers, respectively. These representations are then concatenated and then passed to a mesh network for classification. The novel approach is based on the probability of multiple emotions present in the sentence and utilized both semantic and sentiment representation for better emotion classification. Results are evaluated over their own constructed dataset with tweet conversation pairs, and their model is compared with other baseline models. Xu et al. ( 2020 ) extracted features emotions using two-hybrid models named 3D convolutional-long short-term memory (3DCLS) and CNN-RNN from video and text, respectively. At the same time, the authors implemented SVM for audio-based emotion classification. Authors concluded results by fusing audio and video features at feature level with MKL fusion technique and further combining its results with text-based emotion classification results. It provides better accuracy than every other multimodal fusion technique, intending to analyze the sentiments of drug reviews written by patients on social media platforms. Basiri et al. ( 2020 ) proposed two models using a three-way decision theory. The first model is a 3-way fusion of one deep learning model with the traditional learning method (3W1DT), while the other model is a 3-way fusion of three deep learning models with the conventional learning method (3W3DT). The results derived using the Drugs.com dataset revealed that both frameworks performed better than traditional deep learning techniques. Furthermore, the performance of the first fusion model was noted to be much better as compared to the second model in regards to accuracy and F1-metric. In recent days, social media platforms are flooded with posts related to covid-19. Singh et al. ( 2021 ) applied emotion detection analysis on covid-19 tweets collected from the whole world and India only with Bidirectional Encoder Representations from Transformers (BERT) model on the Twitter data sets and achieved accuracy 94% approximately.
Transfer Learning Approach In traditional approaches, the common presumption is that the dataset is from the same domain; however, there is a need for a new model when the domain changes. The transfer learning approach allows you to reuse the existing pre-trained models in the target domain. For example, Ahmad et al. ( 2020 ) used a transfer learning technique due to the lack of resources for emotion detection in the Hindi language. The researchers pre-trained a model on two different English datasets: SemEval-2018, sentiment analysis, and one Hindi dataset with positive, neutral, conflict, and negative labels. They achieved a score of 0.53 f1 using the transfer learning and 0.47 using only base models CNN and Bi-LSTM with cross-lingual word embedding. Hazarika et al. ( 2020 ) created a TL-ERC model where the model was pre-trained over source multi-turn conversations and then transferred over emotion classification task on exchanged messages. The authors emphasized the issues like lack of labeled data in multi-conversations with the framework based on inductive transfer learning.
Table 4 shows that most researchers implemented models by combining machine learning and deep learning techniques with various feature extraction techniques. Most of the datasets are available in the English language. However, some researchers constructed the dataset of their regional language. For example, Sasidhar et al. ( 2020 ) created the dataset of Hindi-English code mixed with three basic emotions: happy, sad, and angry, and observed CNN-BILSTM gave better performance compared to others.
3.5 Model assessment
Finally, the model is compared with baseline models based on various parameters. There is a requirement of model evaluation metrics to quantify model performance. A confusion matrix is acquired, which provides the count of correct and incorrect judgments or predictions based on known actual values. This matrix displays true positive (TP), false negative (FN), false positive (FP), true negative (TN) values for data fitting based on positive and negative classes. Based on these values, researchers evaluated their model with metrics like accuracy, precision, and recall, F1 score, etc., mentioned in Table 5 .
4 Challenges in sentiment analysis and emotion analysis
In the Internet era, people are generating a lot of data in the form of informal text. Social networking sites present various challenges, as shown in Fig. 5 , which includes spelling mistakes, new slang, and incorrect use of grammar. These challenges make it difficult for machines to perform sentiment and emotion analysis. Sometimes individuals do not express their emotions clearly. For instance, in the sentence “Y have u been soooo late?”, 'why' is misspelled as 'y,' 'you' is misspelled as 'u,' and 'soooo' is used to show more impact. Moreover, this sentence does not express whether the person is angry or worried. Therefore, sentiment and emotion detection from real-world data is full of challenges due to several reasons (Batbaatar et al. 2019 ).
One of the challenges faced during emotion recognition and sentiment analysis is the lack of resources. For example, some statistical algorithms require a large annotated dataset. However, gathering data is not difficult, but manual labeling of the large dataset is quite time-consuming and less reliable (Balahur and Turchi 2014 ). The other problem regarding resources is that most of the resources are available in the English language. Therefore, sentiment analysis and emotion detection from a language other than English, primarily regional languages, are a great challenge and an opportunity for researchers. Furthermore, some of the corpora and lexicons are domain specific, which limits their re-use in other domains.
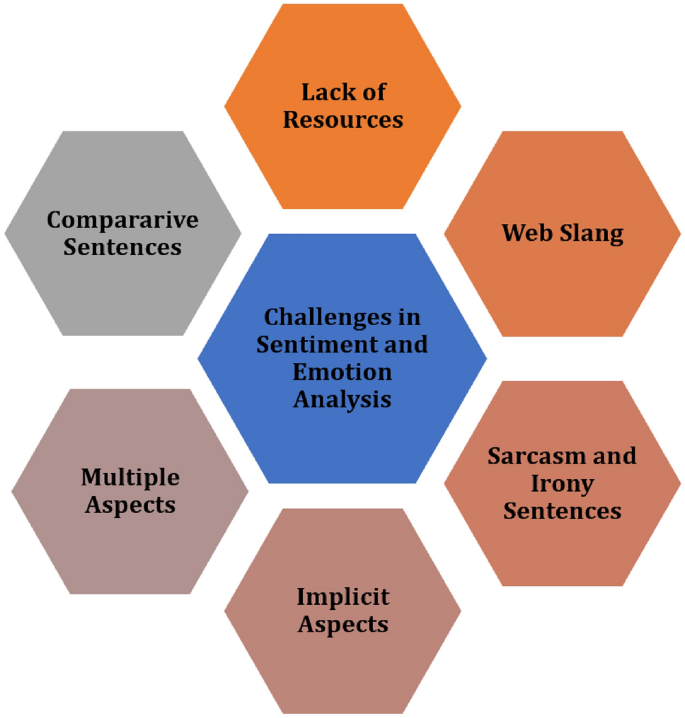
Challenges in sentiment analysis and emotion detection
Another common problem is usually seen on Twitter, Facebook, and Instagram posts and conversations is Web slang. For example, the Young generation uses words like 'LOL,' which means laughing out loud to express laughter, 'FOMO,' which means fear of missing out, which says anxiety. The growing dictionary of Web slang is a massive obstacle for existing lexicons and trained models.
People usually express their anger or disappointment in sarcastic and irony sentences, which is hard to detect (Ghanbari-Adivi and Mosleh 2019 ). For instance, in the sentence, “This story is excellent to put you in sleep,” the excellent word signifies positive sentiment, but in actual the reviewer felt it quite dull. Therefore, sarcasm detection has become a tedious task in the field of sentiment and emotion detection.
The other challenge is the expression of multiple emotions in a single sentence. It is difficult to determine various aspects and their corresponding sentiments or emotions from the multi-opinionated sentence. For instance, the sentence “view at this site is so serene and calm, but this place stinks” shows two emotions, 'disgust' and 'soothing' in various aspects. Another challenge is that it is hard to detect polarity from comparative sentences. For example, consider two sentences 'Phone A is worse than phone B' and 'Phone B is worse than Phone A.' The word ’worse’ in both sentences will signify negative polarity, but these two sentences oppose each other (Shelke 2014 ).
5 Conclusion
In this paper, a review of the existing techniques for both emotion and sentiment detection is presented. As per the paper’s review, it has been analyzed that the lexicon-based technique performs well in both sentiment and emotion analysis. However, the dictionary-based approach is quite adaptable and straightforward to apply, whereas the corpus-based method is built on rules that function effectively in a certain domain. As a result, corpus-based approaches are more accurate but lack generalization. The performance of machine learning algorithms and deep learning algorithms depends on the pre-processing and size of the dataset. Nonetheless, in some cases, machine learning models fail to extract some implicit features or aspects of the text. In situations where the dataset is vast, the deep learning approach performs better than machine learning. Recurrent neural networks, especially the LSTM model, are prevalent in sentiment and emotion analysis, as they can cover long-term dependencies and extract features very well. But RNN with attention networks performs very well. At the same time, it is important to keep in mind that the lexicon-based approach and machine learning approach (traditional approaches) are also evolving and have obtained better outcomes. Also, pre-processing and feature extraction techniques have a significant impact on the performance of various approaches of sentiment and emotion analysis.
Abdaoui A, Azé J, Bringay S, Poncelet P (2017) Feel: a French expanded emotion lexicon. Lang Resour Eval 51(3):833–855
Article Google Scholar
Abdi A, Shamsuddin SM, Hasan S, Piran J (2019) Deep learning-based sentiment classification of evaluative text based on multi-feature fusion. Inf Process Manag 56(4):1245–1259
Adoma AF, Henry N-M, Chen W (2020) Comparative analyses of bert, roberta, distilbert, and xlnet for text-based emotion recognition. In: 2020 17th International Computer Conference on Wavelet Active Media Technology and Information Processing (ICCWAMTIP), IEEE, pp 117–121. https://doi.org/10.1109/ICCWAMTIP51612.2020.9317379
Agbehadji IE, Ijabadeniyi A (2021) Approach to sentiment analysis and business communication on social media. In: Fong S, Millham R (eds) Bio-inspired algorithms for data streaming and visualization, big data management, and fog computing, Springer Tracts in Nature-Inspired Computing. Springer, Singapore. https://doi.org/10.1007/978-981-15-6695-0_9
Agrawal A, An A (2012) Unsupervised emotion detection from text using semantic and syntactic relations. In: 2012 IEEE/WIC/ACM international conferences on web intelligence and intelligent agent technology, pp 346–353. https://doi.org/10.1109/WI-IAT.2012.170.
Ahmad Z, Jindal R, Ekbal A, Bhattachharyya P (2020) Borrow from rich cousin: transfer learning for emotion detection using cross lingual embedding. Expert Syst Appl 139:112851
Ahmed WM (2020) Stock market reactions to domestic sentiment: panel CS-ARDL evidence. Res Int Bus Finance 54:101240
Ahuja R, Chug A, Kohli S, Gupta S, Ahuja P (2019) The impact of features extraction on the sentiment analysis. Procedia Comput Sci 152:341–348
Akilandeswari J, Jothi G (2018) Sentiment classification of tweets with non-language features. Procedia Comput Sci 143:426–433
Al Ajrawi S, Agrawal A, Mangal H, Putluri K, Reid B, Hanna G, Sarkar M (2021) Evaluating business yelp’s star ratings using sentiment analysis. Materials Today: Proceedings. https://doi.org/10.1016/j.matpr.2020.12.137
Al Amrani Y, Lazaar M, El Kadiri KE (2018) Random forest and support vector machine based hybrid approach to sentiment analysis. Procedia Comput Sci 127:511–520
Alqaryouti O, Siyam N, Monem AA, Shaalan K (2020) Aspect-based sentiment analysis using smart government review data. Appl Comput Inf. https://doi.org/10.1016/j.aci.2019.11.003
Alswaidan N, Menai MEB (2020) A survey of state-of-the-art approaches for emotion recognition in text. Knowl Inf Syst 62(8):1–51
Archana Rao PN, Baglodi K (2017) Role of sentiment analysis in education sector in the era of big data: a survey. Int J Latest Trends Eng Technol 22–24
Arora M, Kansal V (2019) Character level embedding with deep convolutional neural network for text normalization of unstructured data for twitter sentiment analysis. Soc Netw Anal Min 9(1):1–14
Arulmurugan R, Sabarmathi K, Anandakumar H (2019) Classification of sentence level sentiment analysis using cloud machine learning techniques. Cluster Comput 22(1):1199–1209
Asghar MZ, Subhan F, Imran M, Kundi FM, Shamshirband S, Mosavi A, Csiba P, Várkonyi-Kóczy AR (2019) Performance evaluation of supervised machine learning techniques for efficient detection of emotions from online content. arXiv preprint arXiv:190801587
Bakker I, Van Der Voordt T, Vink P, De Boon J (2014) Pleasure, arousal, dominance: Mehrabian and Russell revisited. Curr Psychol 33(3):405–421
Balahur A, Turchi M (2014) Comparative experiments using supervised learning and machine translation for multilingual sentiment analysis. Comput Speech Lang 28(1):56–75
Bandhakavi A, Wiratunga N, Padmanabhan D, Massie S (2017) Lexicon based feature extraction for emotion text classification. Pattern Recogn Lett 93:133–142
Basiri ME, Abdar M, Cifci MA, Nemati S, Acharya UR (2020) A novel method for sentiment classification of drug reviews using fusion of deep and machine learning techniques. Knowl Based Syst 198:105949
Batbaatar E, Li M, Ryu KH (2019) Semantic-emotion neural network for emotion recognition from text. IEEE Access 7:111866–111878
Becker K, Moreira VP, dos Santos AG (2017) Multilingual emotion classification using supervised learning: comparative experiments. Inf Process Manag 53(3):684–704
Bernabé-Moreno J, Tejeda-Lorente A, Herce-Zelaya J, Porcel C, Herrera-Viedma E (2020) A context-aware embeddings supported method to extract a fuzzy sentiment polarity dictionary. Knowl-Based Syst 190:105236
Bhardwaj A, Narayan Y, Dutta M et al (2015) Sentiment analysis for Indian stock market prediction using sensex and nifty. Procedia Comput Sci 70:85–91
Bhaskar J, Sruthi K, Nedungadi P (2015) Hybrid approach for emotion classification of audio conversation based on text and speech mining. Procedia Comput Sci 46:635–643
Braun N, van der Lee C, Gatti L, Goudbeek M, Krahmer E (2021) Memofc: introducing the multilingual emotional football corpus. Lang Resour Eval 55(2):389–430
Bučar J, Žnidaršič M, Povh J (2018) Annotated news corpora and a lexicon for sentiment analysis in Slovene. Lang Resour Eval 52(3):895–919
Buechel S, Hahn U (2017) Emobank: Studying the impact of annotation perspective and representation format on dimensional emotion analysis. In: Proceedings of the 15th conference of the european chapter of the association for computational linguistics: volume 2, Short Papers, pp 578–585
Chaffar S, Inkpen D (2011) Using a heterogeneous dataset for emotion analysis in text. In: Butz C, Lingras P (eds) Advances in artificial intelligence. Canadian AI 2011. Lecture notes in computer science, vol 6657. Springer, Berlin, Heidelberg. https://doi.org/10.1007/978-3-642-21043-3_8
Chatterjee A, Gupta U, Chinnakotla MK, Srikanth R, Galley M, Agrawal P (2019) Understanding emotions in text using deep learning and big data. Comput Hum Behav 93:309–317
Chen T, Xu R, He Y, Wang X (2017) Improving sentiment analysis via sentence type classification using BILSTM-CRF and CNN. Expert Syst Appl 72:221–230
Cho H, Kim S, Lee J, Lee JS (2014) Data-driven integration of multiple sentiment dictionaries for lexicon-based sentiment classification of product reviews. Knowl-Based Syst 71:61–71
Chowanda A, Sutoyo R, Tanachutiwat S et al (2021) Exploring text-based emotions recognition machine learning techniques on social media conversation. Procedia Comput Sci 179:821–828
Dahou A, Xiong S, Zhou J, Haddoud MH, Duan P (2016) Word embeddings and convolutional neural network for arabic sentiment classification. In: Proceedings of coling 2016, the 26th international conference on computational linguistics: Technical papers, pp 2418–2427
Dashtipour K, Gogate M, Li J, Jiang F, Kong B, Hussain A (2020) A hybrid Persian sentiment analysis framework: integrating dependency grammar based rules and deep neural networks. Neurocomputing 380:1–10
Devi Sri Nandhini M, Pradeep G (2020) A hybrid co-occurrence and ranking-based approach for detection of implicit aspects in aspect-based sentiment analysis. SN Comput Sci 1:1–9
Dheeraj K, Ramakrishnudu T (2021) Negative emotions detection on online mental-health related patients texts using the deep learning with MHA-BCNN model. Expert Syst Appl 182:115265
Dixon T (2012) “Emotion”: the history of a keyword in crisis. Emot Rev 4(4):338–344
Ekman P (1992) An argument for basic emotions. Cognit Emot 6(3–4):169–200
Esuli A, Sebastiani F (2006) Sentiwordnet: a publicly available lexical resource for opinion mining. LREC, Citeseer 6:417–422
Google Scholar
Gamon M (2004) Sentiment classification on customer feedback data: noisy data, large feature vectors, and the role of linguistic analysis. In: COLING 2004: Proceedings of the 20th international conference on computational linguistics, pp 841–847
Garcia K, Berton L (2021) Topic detection and sentiment analysis in twitter content related to covid-19 from brazil and the USA. Appl Soft Comput 101:107057
Ghanbari-Adivi F, Mosleh M (2019) Text emotion detection in social networks using a novel ensemble classifier based on Parzen tree estimator (tpe). Neural Comput Appl 31(12):8971–8983
Goularas D, Kamis S (2019) Evaluation of deep learning techniques in sentiment analysis from twitter data. In: 2019 International conference on deep learning and machine learning in emerging applications (Deep-ML), IEEE, pp 12–17
Gräbner D, Zanker M, Fliedl G, Fuchs M, et al. (2012) Classification of customer reviews based on sentiment analysis. In: ENTER, Citeseer, pp 460–470
Hasan M, Rundensteiner E, Agu E (2014) Emotex: detecting emotions in twitter messages. In: 2014 ASE BIGDATA/SOCIALCOM/CYBERSECURITY Conference. Stanford University, Academy of Science and Engineering (ASE), USA, ASE, pp 1–10
Hasan M, Rundensteiner E, Agu E (2019) Automatic emotion detection in text streams by analyzing twitter data. Int J Data Sci Anal 7(1):35–51
Hazarika D, Poria S, Zimmermann R, Mihalcea R (2020) Conversational transfer learning for emotion recognition. Inf Fusion 65:1–12
Hosseini AS (2017) Sentence-level emotion mining based on combination of adaptive meta-level features and sentence syntactic features. Eng Appl Artif Intell 65:361–374
Hutto C, Gilbert E (2014) Vader: a parsimonious rule-based model for sentiment analysis of social media text. In: Proceedings of the international AAAI conference on web and social media, vol 8
Itani M, Roast C, Al-Khayatt S (2017) Developing resources for sentiment analysis of informal Arabic text in social media. Procedia Comput Sci 117:129–136
Izard CE (1992) Basic emotions, relations among emotions, and emotion-cognition relations. Psychol Rev 99(3):561–565
Jain VK, Kumar S, Fernandes SL (2017) Extraction of emotions from multilingual text using intelligent text processing and computational linguistics. J Comput Sci 21:316–326
Jang HJ, Sim J, Lee Y, Kwon O (2013) Deep sentiment analysis: mining the causality between personality-value-attitude for analyzing business ads in social media. Expert Syst Appl 40(18):7492–7503
Jha V, Savitha R, Shenoy PD, Venugopal K, Sangaiah AK (2018) A novel sentiment aware dictionary for multi-domain sentiment classification. Comput Electr Eng 69:585–597
Jian Z, Chen X, Wang Hs (2010) Sentiment classification using the theory of ANNs. J China Univ Posts Telecommun 17:58–62
Jurek A, Mulvenna MD, Bi Y (2015) Improved lexicon-based sentiment analysis for social media analytics. Secur Inform 4(1):1–13
Kratzwald B, Ilić S, Kraus M, Feuerriegel S, Prendinger H (2018) Deep learning for affective computing: text-based emotion recognition in decision support. Decis Support Syst 115:24–35
Laubert C, Parlamis J (2019) Are you angry (happy, sad) or aren’t you? Emotion detection difficulty in email negotiation. Group Decis Negot 28(2):377–413
Le Q, Mikolov T (2014) Distributed representations of sentences and documents. In: International conference on machine learning, pp 1188–1196
Li Z, Xie H, Cheng G, Li Q (2021) Word-level emotion distribution with two schemas for short text emotion classification. Knowledge-Based Syst 227:107163
Liu Y, Wan Y, Su X (2019) Identifying individual expectations in service recovery through natural language processing and machine learning. Expert Syst Appl 131:288–298
Liu F, Zheng J, Zheng L, Chen C (2020a) Combining attention-based bidirectional gated recurrent neural network and two-dimensional convolutional neural network for document-level sentiment classification. Neurocomputing 371:39–50
Liu S, Lee K, Lee I (2020b) Document-level multi-topic sentiment classification of email data with bilstm and data augmentation. Knowl-Based Syst 197:105918
Lövheim H (2012) A new three-dimensional model for emotions and monoamine neurotransmitters. Med Hypoth 78(2):341–348
Ma X, Zeng J, Peng L, Fortino G, Zhang Y (2019) Modeling multi-aspects within one opinionated sentence simultaneously for aspect-level sentiment analysis. Future Gener Comput Syst 93:304–311
Meena A, Prabhakar TV (2007) Sentence level sentiment analysis in the presence of conjuncts using linguistic analysis. In: Amati G, Carpineto C, Romano G (eds) Advances in information retrieval. ECIR 2007. Lecture notes in computer science, vol 4425. Springer, Berlin, Heidelberg. https://doi.org/10.1007/978-3-540-71496-5_53
Mladenović M, Mitrović J, Krstev C, Vitas D (2016) Hybrid sentiment analysis framework for a morphologically rich language. J Intell Inf Syst 46(3):599–620
Mohammad SM, Turney PD (2013) Crowdsourcing a word-emotion association lexicon. Comput Intell 29(3):436–465
Article MathSciNet Google Scholar
Moraes R, Valiati JF, Gavião Neto WP (2013) Document-level sentiment classification: an empirical comparison between SVM and ANN. Expert Syst Appl 40(2):621–633
Mukherjee P, Badr Y, Doppalapudi S, Srinivasan SM, Sangwan RS, Sharma R (2021) Effect of negation in sentences on sentiment analysis and polarity detection. Procedia Comput Sci 185:370–379
Munezero M, Montero CS, Sutinen E, Pajunen J (2014) Are they different? affect, feeling, emotion, sentiment, and opinion detection in text. IEEE Trans Affect Comput 5(2):101–111
Nagamanjula R, Pethalakshmi A (2020) A novel framework based on bi-objective optimization and lan 2 fis for twitter sentiment analysis. Soc Netw Anal Min 10:1–16
Nagarajan SM, Gandhi UD (2019) Classifying streaming of twitter data based on sentiment analysis using hybridization. Neural Comput Appl 31(5):1425–1433
Nandal N, Tanwar R, Pruthi J (2020) Machine learning based aspect level sentiment analysis for amazon products. Spat Inf Res 28(5):601–607
Onyenwe I, Nwagbo S, Mbeledogu N, Onyedinma E (2020) The impact of political party/candidate on the election results from a sentiment analysis perspective using# anambradecides2017 tweets. Soc Netw Anal Min 10(1):1–17
Pasupa K, Ayutthaya TSN (2019) Thai sentiment analysis with deep learning techniques: a comparative study based on word embedding, pos-tag, and sentic features. Sustain Cities Soc 50:101615
Plutchik R (1982) A psychoevolutionary theory of emotions. https://doi.org/10.1177/053901882021004003
Poria S, Gelbukh A, Cambria E, Hussain A, Huang GB (2014) Emosenticspace: a novel framework for affective common-sense reasoning. Knowl-Based Syst 69:108–123
Prabowo R, Thelwall M (2009) Sentiment analysis: a combined approach. J Inform 3(2):143–157
Pu X, Wu G, Yuan C (2019) Exploring overall opinions for document level sentiment classification with structural SVM. Multim Syst 25(1):21–33
Rabeya T, Ferdous S, Ali HS, Chakraborty NR (2017) A survey on emotion detection: a lexicon based backtracking approach for detecting emotion from Bengali text. In: 2017 20th international conference of computer and information technology (ICCIT), IEEE, pp 1–7
Rao G, Huang W, Feng Z, Cong Q (2018) LSTM with sentence representations for document-level sentiment classification. Neurocomputing 308:49–57
Ray P, Chakrabarti A (2020) A mixed approach of deep learning method and rule-based method to improve aspect level sentiment analysis. Appl Comput Inform
Roberts K, Roach MA, Johnson J, Guthrie J, Harabagiu SM (2012) Empatweet: annotating and detecting emotions on twitter. Lrec, Citeseer 12:3806–3813
Russell JA (1980) A circumplex model of affect. J Pers Soc Psychol 39(6):1161
Sailunaz K, Alhajj R (2019) Emotion and sentiment analysis from twitter text. J Comput Sci 36:101003
Salinca A (2015) Business reviews classification using sentiment analysis. In: Proceedings of the 2015 17th international symposium on symbolic and numeric algorithms for scientific computing (SYNASC), IEEE, pp 247–250
Sangeetha K, Prabha D (2020) Sentiment analysis of student feedback using multi-head attention fusion model of word and context embedding for LSTM. J Ambient Intell Hum Comput 12:4117–4126
Sasidhar TT, Premjith B, Soman K (2020) Emotion detection in hinglish (hindi+ english) code-mixed social media text. Procedia Comput Sci 171:1346–1352
Schouten K, Frasincar F (2015) Survey on aspect-level sentiment analysis. IEEE Trans Knowl Data Eng 28(3):813–830
Seal D, Roy UK, Basak R (2020) Sentence-level emotion detection from text based on semantic rules. In: Information and communication technology for sustainable development. Springer, pp 423–430
Shamantha RB, Shetty SM, Rai P (2019) Sentiment analysis using machine learning classifiers: evaluation of performance. In: Proceedings of the 2019 IEEE 4th international conference on computer and communication systems (ICCCS), IEEE, pp 21–25
Sharma P, Sharma A (2020) Experimental investigation of automated system for twitter sentiment analysis to predict the public emotions using machine learning algorithms. Mater Today Proc
Shaver P, Schwartz J, Kirson D, O’connor C (1987) Emotion knowledge: further exploration of a prototype approach. J Pers Soc Psychol 52(6):1061
Shelke NM (2014) Approaches of emotion detection from text. Int J Comput Sci Inf Technol 2(2):123–128
Shirsat VS, Jagdale RS, Deshmukh SN (2019) Sentence level sentiment identification and calculation from news articles using machine learning techniques. In: Computing, communication and signal processing. Springer, pp 371–376
Shrivastava K, Kumar S, Jain DK (2019) An effective approach for emotion detection in multimedia text data using sequence based convolutional neural network. Multim Tools Appl 78(20):29607–29639
Singh M, Jakhar AK, Pandey S (2021) Sentiment analysis on the impact of coronavirus in social life using the Bert model. Soc Netw Anal Min 11(1):1–11
Songbo T, Jin Z (2008) An empirical study of sentiment analysis for Chinese documents. Expert Syst Appl 34(4):2622–2629
Souma W, Vodenska I, Aoyama H (2019) Enhanced news sentiment analysis using deep learning methods. J Comput Soc Sci 2(1):33–46
Soumya S, Pramod KV (2020) Sentiment analysis of malayalam tweets using machine learning techniques. ICT Express 6(4):300–305
Strapparava C, Valitutti A, et al. (2004) Wordnet affect: an affective extension of wordnet. In: Lrec, Citeseer, vol 4, pp 1083–1086
Sun S, Luo C, Chen J (2017) A review of natural language processing techniques for opinion mining systems. Inf Fusion 36:10–25
Symeonidis S, Effrosynidis D, Arampatzis A (2018) A comparative evaluation of pre-processing techniques and their interactions for twitter sentiment analysis. Expert Syst Appl 110:298–310
Tang D, Qin B, Liu T (2015) Learning semantic representations of users and products for document level sentiment classification. In: Proceedings of the 53rd annual meeting of the association for computational linguistics and the 7th international joint conference on natural language processing (Volume 1: Long Papers), pp 1014–1023
Tao J, Fang X (2020) Toward multi-label sentiment analysis: a transfer learning based approach. J Big Data 7(1):1–26
Tiwari P, Mishra BK, Kumar S, Kumar V (2020) Implementation of n-gram methodology for rotten tomatoes review dataset sentiment analysis. In: Cognitive analytics: concepts, methodologies, tools, and applications, IGI Global, pp 689–701
Tomkins SS, McCarter R (1964) What and where are the primary affects? Some evidence for a theory. Percept Mot Skills 18(1):119–158
Untawale TM, Choudhari G (2019) Implementation of sentiment classification of movie reviews by supervised machine learning approaches. In: Proceedings of the 2019 3rd international conference on computing methodologies and communication (ICCMC), IEEE, pp 1197–1200
Viegas F, Alvim MS, Canuto S, Rosa T, Gonçalves MA, Rocha L (2020) Exploiting semantic relationships for unsupervised expansion of sentiment lexicons. Inf Syst 94:101606
Xu G, Li W, Liu J (2020) A social emotion classification approach using multi-model fusion. Future Gen Comput Syst 102:347–356
Yang X, Macdonald C, Ounis I (2018) Using word embeddings in twitter election classification. Inf Retriev J 21(2–3):183–207
Ye Q, Zhang Z, Law R (2009) Sentiment classification of online reviews to travel destinations by supervised machine learning approaches. Expert Syst Appl 36(3):6527–6535
Zhang D, Si L, Rego VJ (2012) Sentiment detection with auxiliary data. Inf Retriev 15(3–4):373–390
Download references
Author information
Authors and affiliations.
Computer Science and Engineering Department, Punjab Engineering College, Chandigarh, India
Pansy Nandwani & Rupali Verma
You can also search for this author in PubMed Google Scholar
Corresponding authors
Correspondence to Pansy Nandwani or Rupali Verma .
Additional information
Publisher's note.
Springer Nature remains neutral with regard to jurisdictional claims in published maps and institutional affiliations.
Rights and permissions
Reprints and permissions
About this article
Nandwani, P., Verma, R. A review on sentiment analysis and emotion detection from text. Soc. Netw. Anal. Min. 11 , 81 (2021). https://doi.org/10.1007/s13278-021-00776-6
Download citation
Received : 06 April 2021
Revised : 25 June 2021
Accepted : 10 July 2021
Published : 28 August 2021
DOI : https://doi.org/10.1007/s13278-021-00776-6
Share this article
Anyone you share the following link with will be able to read this content:
Sorry, a shareable link is not currently available for this article.
Provided by the Springer Nature SharedIt content-sharing initiative
- Affective computing
- Natural language processing
- Opinion mining
- Pre-processing
- Word embedding
- Find a journal
- Publish with us
- Track your research
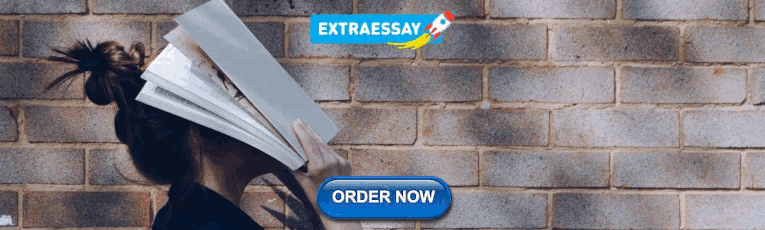
IMAGES
VIDEO
COMMENTS
Social network-based applications like Facebook, Twitter, and Instagram have been used by people of all age groups and backgrounds for the last few years. It is ... This review paper conducts a survey on community detection problem in social networks, its analysis, and a study of research done on related areas. Published in: ...
Social network analysis (SNA) is a research technique that is growing in popularity and in applicability. ... This paper examines the impact of mood and social presence of communication medium on ...
This paper is structured as follows: Section 2 contains the scientometric analysis performed to highlight the different research areas with high impact in SNA research field. Section 3 provides an analysis of the different concepts and research works that belongs to the SNA research field from the Computer Science point-of-view. The different application domains in SNA, as well as the emerging ...
Due to the explosive rise of online social networks, social network analysis (SNA) has emerged as a significant academic field in recent years. Understanding and examining social relationships in networks through network analysis opens up numerous research avenues in sociology, literature, media, biology, computer science, sports, and more.
Social network analysis is the process of investigating social structures through the use of networks and graph theory. It combines a variety of techniques for analyzing the structure of social networks as well as theories that aim at explaining the underlying dynamics and patterns observed in these structures. It is an inherently interdisciplinary field which originally emerged from the ...
Social network analysis (SNA) is a core pursuit of analyzing social networks today. In addition to the usual statistical techniques of data analysis, these networks are investigated using SNA ...
Social network analysis is a large and growing body of research on the measurement and analysis of relational structure. Here, we review the fundamental concepts of network analysis, as well as a range of methods currently used in the field.
1.4 Organization. The remaining part of the paper is organized as follows. Section 2 contains a brief description of deep learning techniques and online social networks. In Sect. 3, we describe an overview of the research related to opinion and sentiment analysis.Section 4 contains the research pertaining to text classification and recommender systems.
Search for more papers by this author. Fabiola S. F. Pereira, Fabiola S. F. Pereira. ... Social network analysis (SNA) is a core pursuit of analyzing social networks today. ... This research was carried out in the framework of the project "TEC4Growth—RL SMILES—Smart, mobile, Intelligent and Large Scale Sensing and analytics NORTE-01-0145 ...
Social network analysis is an applicable method for investigating relations and interactions. Social network analysis (SNA) is an approach rooted in anthropology, sociology and social psychology for assessing social structures [3, 16].The social network perspective illustrates social systems as networks of various relationships [2, 12].SNA centers upon the structure and design of relationships ...
This review of social network analysis focuses on identifying recent trends in interpersonal social networks research in organizations, and generating new research directions, with an emphasis on conceptual foundations. It is organized around two broad social network topics: structural holes and brokerage and the nature of ties. New research directions include adding affect, behavior, and ...
Social Network Analysis (SNA) is a research approach within the social and behavioral sciences which focuses on ways of interaction and interconnection between individuals and social groups to explain social patterns of feelings, thoughts, and behaviors [1, 2].In recent decades, research based on SNA has been increasingly used in health, including areas such as disease transmission, health ...
Social network analysis (SNA), in essence, is not a formal theory in social science, but rather an approach for investigating social structures, which is why SNA is often referred to as structural analysis [].The most important difference between social network analysis and the traditional or classic social research approach is that the contexts of the social actor, or the relationships ...
human societies, social networks have been there forging individual and collective behavior. In the academia, research on social networks can be traced to the rst decades of the twentieth century (Rice, 1927), while probably the most in uential early work on social network analysis was the seminal paper\Contacts and In uence"(de Sola Pool and ...
The representation and analysis of community network structure remains at the forefront of network research in the social sciences today, with growing interest in unraveling the structure of computer-supported virtual communities that have proliferated in recent years ( 12 ). By the 1960s, the network perspective was thriving in anthropology.
Social networks were first investigated in social, educational and business areas. Academic interest in this field though has been growing since the mid twentieth century, given the increasing interaction among people, data dissemination and exchange of information. As such, the development and evaluation of new techniques for social network analysis and mining (SNAM) is a current key research ...
The importance of social networks for innovation diffusion and processes of social change is widely recognized in many areas of practice and scientific disciplines. Social networks have the potential to influence learning processes, provide opportunities for problem-solving, and establish new ideas. Thus, they can foster synergy effects, bring together key resources such as know-how of ...
Social network analysis (SNA) consists of a set of theories and methods that considers the direct and indirect relationships in a clearly defined context. Although SNA research has witnessed rapid growth in the social sciences, school psychology has not kept pace. The lack of SNA studies in school psychology journals is interesting given that many topics of interest in the field both influence ...
This paper reviews the development of social network analysis and examines its major areas of application in sociology. Current developments, including those from outside the social sciences, are examined and their prospects for advances in substantive knowledge are considered. A concluding section looks at the implications of data mining techniques and highlights the need for ...
It's widely used in social science research. NetDraw: Often used in conjunction with UCINet, NetDraw is a free tool for visualizing networks. It supports the visualization of large networks and allows for various customization options. Pajek: Pajek is a program for the analysis and visualization of large networks.
This paper presents the analysis of journals publishing articles on social network analysis (SNA). The dataset consists of articles from the Web of Science database obtained by searching for "social network*", works intensively cited, written by the most prominent authors, and published in the main SNA journals up to July 2018. There were 8943 journals in 70,792 articles with complete ...
The analysis focused on users who talked about their experiences with pediatric stroke, as identified by a previous study. The posts were read by 3 authors, and factors influencing recovery from pediatric stroke were mapped into 4 areas: medical, physical, emotional, and social.
Overview. Social Network Analysis and Mining is a multidisciplinary journal focusing on theoretical and experimental work related to social network analysis and mining. Serves a wide range of researchers from computer science, network science, social sciences, mathematical sciences, medical, biological, financial, management, and political ...
Social networking platforms have become an essential means for communicating feelings to the entire world due to rapid expansion in the Internet era. Several people use textual content, pictures, audio, and video to express their feelings or viewpoints. Text communication via Web-based networking media, on the other hand, is somewhat overwhelming. Every second, a massive amount of unstructured ...