Thank you for visiting nature.com. You are using a browser version with limited support for CSS. To obtain the best experience, we recommend you use a more up to date browser (or turn off compatibility mode in Internet Explorer). In the meantime, to ensure continued support, we are displaying the site without styles and JavaScript.
- View all journals
- Explore content
- About the journal
- Publish with us
- Sign up for alerts
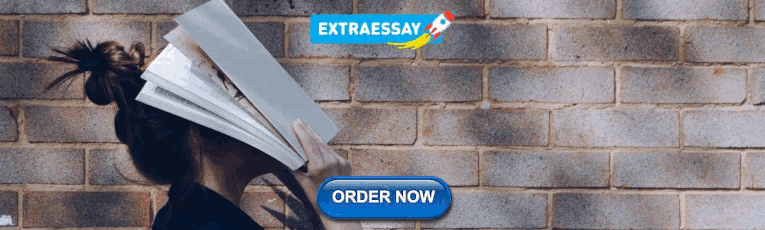
Fibre optics and optical communications articles within Nature Communications
Article 05 April 2024 | Open Access
A metasurface-based full-color circular auto-focusing Airy beam transmitter for stable high-speed underwater wireless optical communications
Authors present an adaptive underwater optical communication (UWOC) technology based on multi-wavelength lasers and a full-color metasurface for converting visible-band Gaussian to circular autofocusing Airy beams. The potential of Airy beams to mitigate optical power degradation is demonstrated, enabling stable data rate transmission via 4 K video transmission for these systems.
- , Zeyuan Guo
- & Chao Shen
Article 19 February 2024 | Open Access
Self-supervised dynamic learning for long-term high-fidelity image transmission through unstabilized diffusive media
This work introduces a cutting-edge technique to overcome dynamic scattering challenges in long-distance multimode fiber transmission, achieving >99.9% accuracy for 1024 modes over 1 km, hence promises applications in diverse scattering scenarios.
- & Qionghai Dai
Article 07 February 2024 | Open Access
Statistics of modal condensation in nonlinear multimode fibers
The authors investigate light beam propagation in multimode optical fibers, considering linear random mode coupling and Kerr nonlinearity. They utilize a 3D mode decomposition technique, enabling them to accurately characterize modal distributions over extended lengths of graded-index fiber.
- Mario Zitelli
- , Fabio Mangini
- & Stefan Wabnitz
Article 24 November 2023 | Open Access
Mode attraction, rejection and control in nonlinear multimode optics
The authors introduce and demonstrate experimentally a novel fundamental property of nonlinear multimode optical systems, named mode rejection. This paves the way towards a more general idea of all-optical mode control and its related applications.
- , Ian Davidson
- & Massimiliano Guasoni
Article 21 November 2023 | Open Access
Woven organic crystals
The entanglement of fibrous elements produces flexible structures with enhanced strength and resilience to abrasion. Here, the authors report the weaving of organic crystals into flexible and robust patches with plain, twill, and satin topologies of arbitrary porosity, expanding one-dimensional crystals into flexible, two-dimensional planar structures with potential for future applications in flexible electronics.
- Linfeng Lan
- & Hongyu Zhang
Article 16 November 2023 | Open Access
On-chip silicon electro-optical modulator with ultra-high extinction ratio for fiber-optic distributed acoustic sensing
On-chip optoelectronic devices are promising to build compact and efficient distributed acoustic sensing (DAS) systems. Here, the authors demonstrate an ultra-high extinction ratio electro-optical modulator on silicon and its application for DAS.
- , Xiaoqian Shu
- & Yunjiang Rao
Article 13 November 2023 | Open Access
Mitigating stimulated Brillouin scattering in multimode fibers with focused output via wavefront shaping
The authors demonstrate a high-power delivery through a highly multimode optical fiber by shaping the incident wavefront of a laser beam to strongly suppress the stimulated Brillouin scattering in the fiber and simultaneously control the output beam profile.
- Chun-Wei Chen
- , Linh V. Nguyen
- & Hui Cao
Article 09 November 2023 | Open Access
Metafiber transforming arbitrarily structured light
Structured light has proven useful for numerous photonic applications. However, its current use in optical fibers is severely limited. The authors report a highly integrated metafiber platform based on 3D laser nanoprinting, capable of creating arbitrarily structured light.
- , Torsten Wieduwilt
- & Haoran Ren
Article 20 October 2023 | Open Access
A random optical parametric oscillator
In this work the authors develop a Random optical parametric oscillator - the parametric analogous of random lasers. This system shows improved key metrics like tuneable repetition rates, tuneable pulse duration, inter-pulse coherence as well as simpler configuration compared with standard systems.
- Pedro Tovar
- , Jean Pierre von der Weid
- & Xiaoyi Bao
Article 17 October 2023 | Open Access
4D Optical fibers based on shape-memory polymers
The authors demonstrate here a method for the production of arbitrarily long, light-guiding microstructured fibers with shape-memory properties. The showcased design of adaptative fibers here holds potential for the development of functional actuators and sensors.
- Clément Strutynski
- , Marianne Evrard
- & Frédéric Smektala
Article 28 September 2023 | Open Access
Differential phase-diversity electrooptic modulator for cancellation of fiber dispersion and laser noise
In this work, the authors showcase four-phase electrooptic modulators (FEOMs) implemented on thin-film lithium niobate. This innovation effectively addresses challenges related to dispersion and semiconductor laser noise limitations, offering a promising solution for integrated photonic applications.
- Ehsan Ordouie
- , Tianwei Jiang
- & Bahram Jalali
Article 13 July 2023 | Open Access
Earthquake focal mechanisms with distributed acoustic sensing
Determining earthquake faulting orientation is essential for understanding earthquake-stress interactions. Here, the authors present a technique that leverages telecom fiber optic cables to improve the estimation of this fundamental parameter.
- , Weiqiang Zhu
- & Zhongwen Zhan
Article 05 June 2023 | Open Access
Characterization of diffusing sub-10 nm nano-objects using single anti-resonant element optical fibers
Characterizing diffusing species is increasingly important for revealing nanoscale processes. Here, the authors uncover the potential of fiber-assisted nanoparticle tracking analysis by characterizing nanoparticles as small as 9 nm at record precision levels, reaching fundamental limits.
- Torsten Wieduwilt
- , Ronny Förster
- & Markus A. Schmidt
Article 31 March 2023 | Open Access
All-fibre phase filters with 1-GHz resolution for high-speed passive optical logic processing
Authors present a fibre Bragg grating-based all-pass spectral phase filter with an unprecedented frequency resolution of 1 GHz, at least 10× improvement compared to a standard optical waveshaper. Using the all-fibre phase filter, fully passive NOT and XNOR logic operations are experimentally demonstrated at ultrafast speeds with few-fJ/bit energy consumption.
- Saket Kaushal
- & José Azaña
Article 18 February 2023 | Open Access
Twin-field quantum key distribution without optical frequency dissemination
Twin-field QKD should allow secure quantum communication with favourable rate-loss scaling, but requires interferometric implementations which are often impractical for long distances. Here, the authors show how to realise it without the need for closed interferometers.
- , Jinping Lin
- & Zhiliang Yuan
Perspective 15 February 2023 | Open Access
Wireless communications sensing and security above 100 GHz
The field of terahertz wireless communication is growing rapidly. Here the authors discuss the challenges for the architectures of wireless platforms above 100 GHz and their potential applications.
- Josep M. Jornet
- , Edward W. Knightly
- & Daniel M. Mittleman
Article 10 December 2022 | Open Access
High-capacity free-space optical communications using wavelength- and mode-division-multiplexing in the mid-infrared region
A 300-Gbit/s free-space optical communication system is demonstrated in the mid-IR wavelength region by using both wavelength- and mode-division multiplexing.
- Kaiheng Zou
- & Alan E. Willner
High-order dynamic localization and tunable temporal cloaking in ac-electric-field driven synthetic lattices
Dynamic localization is a method of confining light. Here the authors demonstrate higher-order dynamic localization of photons in a synthetic temporal mesh lattice and discuss the idea of tunable temporal cloaking by combining different-order localizations.
- Shulin Wang
- , Chengzhi Qin
- & Peixiang Lu
Article 05 November 2022 | Open Access
Quantum capacities of transducers
A unified metric to assess the performances of quantum transducers, i.e., converters of quantum information between different physical systems - is still lacking. Here the authors propose quantum capacity as such metric, and use it to investigate the optimal designs of generic quantum transduction schemes.
- Chiao-Hsuan Wang
- , Fangxin Li
- & Liang Jiang
Article 18 October 2022 | Open Access
Controlled filamentation instability as a scalable fabrication approach to flexible metamaterials
The simple and scalable fabrication of high aspect ratio encapsulated flexible nanowire arrays with controlled size and periodicity is demonstrated and modelled, enabling nanophotonics applications requiring long-range order over several meters.
- William Esposito
- , Louis Martin-Monier
- & Fabien Sorin
Article 17 October 2022 | Open Access
Continuous entanglement distribution over a transnational 248 km fiber link
Fibre-based entanglement distribution represents a key primitive for quantum applications such as QKD. Here, the authors demonstrate it across 248 km of deployed fiber, observing stable detected pair rates of 9 Hz for 110 h.
- Sebastian Philipp Neumann
- , Alexander Buchner
- & Rupert Ursin
Article 13 October 2022 | Open Access
Real-time holographic lensless micro-endoscopy through flexible fibers via fiber bundle distal holography
Fiber-based micro-endoscopes are minimally invasive, but 3D imaging is limited by the need for bulky optical elements or rigid fibers. Here, the authors demonstrate holographic imaging through flexible and dynamically bent multi-core fibers by adding partially reflecting mirror to the distal fiber-tip.
- & Ori Katz
Article 12 August 2022 | Open Access
Practical continuous-variable quantum key distribution with composable security
Continuous-variable QKD protocols are usually easier to implement than discrete-variables ones, but their security analyses are less developed. Here, the authors propose and demonstrate in the lab a CVQKD protocol that can generate composable keys secure against collective attacks.
- , Hou-Man Chin
- & Ulrik L. Andersen
Article 25 July 2022 | Open Access
Spatial tomography of light resolved in time, spectrum, and polarisation
The work harnesses principles of spatial state tomography to fully characterise an optical beam in space, time, spectrum, and polarisation. Analysis of the output of a vertical-cavity surface-emitting laser illustrates the technique’s capabilities.
- Martin Plöschner
- , Marcos Maestre Morote
- & Joel Carpenter
Article 16 July 2022 | Open Access
Super-broadband on-chip continuous spectral translation unlocking coherent optical communications beyond conventional telecom bands
Continuous spectral translation could allow expansion of the bandwidth available for communication without having to develop transceivers for the new bands. Here, the authors demonstrate this using AlGaAsOI nanowaveguides as spectral translators between the mature telecom C band and the 2-μm wavelength band.
- Deming Kong
- & Hao Hu
Article 14 July 2022 | Open Access
Add drop multiplexers for terahertz communications using two-wire waveguide-based plasmonic circuits
Using 3D-printed two-wire plasmonic circuits, the authors demonstrate add-drop THz multiplexers featuring a grating-loaded side coupler design. They confirm the channel Drop, Add, and Through actions using ~6 Gbps data streams at ~140 GHz carrier frequency.
- , Kathirvel Nallappan
- & Maksim Skorobogatiy
Article 11 July 2022 | Open Access
Enhancing fibre-optic distributed acoustic sensing capabilities with blind near-field array signal processing
Here, the authors demonstrate a blind and sparse near-field array signal processing approach to enhance the measurement quality of fibre-optic distributed acoustic sensors. It further enables the accurate estimation of the spatial coordinates of acoustic sources.
- Felipe Muñoz
- & Marcelo A. Soto
Article 09 July 2022 | Open Access
All-fibre heterogeneously-integrated frequency comb generation using silicon core fibre
Silicon core fibre is a highly nonlinear waveguide that combines the benefits of both fibre and planar waveguide systems. Here, the authors demonstrate frequency comb generation using a fully-fibre integrated silicon core fibre as a nonlinear mixer.
- Ronit Sohanpal
- , Haonan Ren
- & Zhixin Liu
Article 06 June 2022 | Open Access
The piston Riemann problem in a photon superfluid
The flow of light in nonlinear media can be considered as superfluid flow and described with quantum hydrodynamics. Here the authors demonstrate superfluid flow, optical rarefaction (retracting piston) and shock (pushing piston) wave pairs and characterize associated phase transitions by using fiber-based platform.
- Abdelkrim Bendahmane
- & Stefano Trillo
Article 13 May 2022 | Open Access
Localised structuring of metal-semiconductor cores in silica clad fibres using laser-driven thermal gradients
The authors show that micron size fibers comprised of semiconductor and metallic materials can be purified or doped, recrystallized or have the constituents rearranged into a variety of structures with device prospects.
- Seunghan Song
- , Fredrik Laurell
- & Ursula J. Gibson
Article 26 April 2022 | Open Access
Nanosecond optical switching and control system for data center networks
Several challenges still impede the deployment of optical switches in data centers. The authors report an optical switching and control system to synergistically overcome these challenges and provide enhanced performance for data center applications.
- & Nicola Calabretta
Article 19 April 2022 | Open Access
Two octave supercontinuum generation in a non-silica graded-index multimode fiber
Two-octave supercontinuum generation in the femtosecond regime from the visible to mid-infrared and self-cleaning dynamics are reported in a non-silica graded-index multimode fiber with nanostructured design and enhanced nonlinearity.
- Zahra Eslami
- , Lauri Salmela
- & Goëry Genty
Article 28 March 2022 | Open Access
Hollow-core optical fibre sensors for operando Raman spectroscopy investigation of Li-ion battery liquid electrolytes
New analytical tools are needed to identify chemical degradation and failure mechanisms in Li-ion batteries. Here, the authors report an operando Raman spectroscopy method, based on hollow-core optical fibres, that enables monitoring the chemistry of liquid electrolytes during battery cycling.
- Ermanno Miele
- , Wesley M. Dose
- & Tijmen G. Euser
Article 17 March 2022 | Open Access
All-fiber high-speed image detection enabled by deep learning
Here, the authors demonstrate high-speed imaging through multimode optical fibers by using the high intermodal dispersion to transform 2D spatial information into 1D temporal pulsed signal streams. Deep learning is used to reconstruct the images of micron-scale objects at high frame rates.
- Zhoutian Liu
- , Lele Wang
- & Qirong Xiao
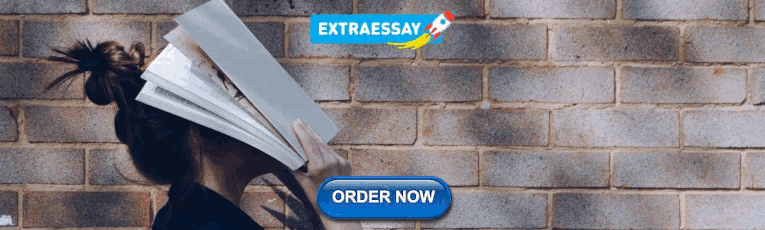
Large evanescently-induced Brillouin scattering at the surrounding of a nanofibre
The authors observe efficient Brillouin scattering generated by an evanescent field nearby a single pass sub-wavelength waveguide. This work creates an important bridge between Brillouin scattering in waveguides, Brillouin spectroscopy and microscopy.
- , Flavien Gyger
- & Luc Thévenaz
Article 16 March 2022 | Open Access
Integrated dual-laser photonic chip for high-purity carrier generation enabling ultrafast terahertz wireless communications
A photonic Terahertz source based on injection-locking an integrated dual-laser chip generates and transmits a 131 Gbps THz signal over 10.7-m distance, showing great potential towards fully integrated and energy-efficient THz transmitters for 6G.
- , Mu-Chieh Lo
- & Leif K. Oxenløwe
Article 28 February 2022 | Open Access
Coherent optical communications using coherence-cloned Kerr soliton microcombs
Kerr frequency combs are a promising laser source for future optical networks. The authors demonstrate coherence-cloning between two remote Kerr combs and improve optical coherent communication in terms of performance, power consumption, and simplicity.
- , Heng Zhou
- & Kun Qiu
Article 10 February 2022 | Open Access
Shaping lightwaves in time and frequency for optical fiber communication
The authors report nonlinear interference changes with shaping block length, modulation symbol rate, and chromatic dispersion when sphere-shaped lightwaves propagate through optical fibre, and suggest a method to predict that change.
- & Robert Tkach
Article 11 January 2022 | Open Access
Comparing ultrastable lasers at 7 × 10 −17 fractional frequency instability through a 2220 km optical fibre network
Precision measurement plays an important role in frequency metrology and optical communications. Here the authors compare two geographically separate ultrastable lasers at 7 × 10 −17 fractional frequency instability over a 2220 km optical fibre link and these measurements can be useful for dissemination of ultrastable light to distant optical clocks.
- M. Schioppo
- , J. Kronjäger
- & G. Grosche
Article 10 January 2022 | Open Access
Coherent phase transfer for real-world twin-field quantum key distribution
Exploiting technologies derived from the optical clocks community, the authors demonstrate a setup for twin-field QKD which extends the coherence times by three orders of magnitude, overcoming the main challenge towards real-world implementation.
- Cecilia Clivati
- , Alice Meda
- & Davide Calonico
Article 03 November 2021 | Open Access
Laser nano-filament explosion for enabling open-grating sensing in optical fibre
Engineered stop bands to sense an ambient environment can enable many applications. Here, the authors demonstrate well-controlled processes to open high-aspect ratio nanoholes through optical fibre for Bragg gratings in the telecomm spectrum and to enable high-resolution refractive index sensing
- Keivan Mahmoud Aghdami
- , Abdullah Rahnama
- & Peter R. Herman
Article 20 October 2021 | Open Access
Experimental demonstration of peripherally-excited antenna arrays
Two-dimensional antennas that can create and steer narrow beams are of interest for modern communication systems. Here, the authors demonstrate a scalable antenna array design that performs these functions with fewer elements than standard approaches and excited only with peripheral sources.
- Ayman H. Dorrah
- & George V. Eleftheriades
Article 15 October 2021 | Open Access
Ultrafast optical circuit switching for data centers using integrated soliton microcombs
Optical technologies could enable fast and power-efficient networks for data centers. Here, the authors report Si 3 N 4 microcomb based ultrafast photonic switching to provide enhanced performance for data center applications.
- Arslan Sajid Raja
- , Sophie Lange
- & Hitesh Ballani
Article 20 August 2021 | Open Access
Ultrahigh-speed graphene-based optical coherent receiver
Graphene-based photodetectors have many advantages for applications. Here, the authors demonstrate a high-speed optical coherent receiver for optical communications based on graphene-on-plasmonic slot waveguide photodetectors.
- & Xinliang Zhang
Matters Arising 12 August 2021 | Open Access
Evaluating measurement uncertainty in Brillouin distributed optical fibre sensors using image denoising
- Marcelo A. Soto
- , Zhisheng Yang
Article 20 July 2021 | Open Access
Full-duplex reflective beamsteering metasurface featuring magnetless nonreciprocal amplification
Metasurfaces promise complex functionalities in electromagnetic wave control. Here the authors present an architecture for full-duplex reflective beamsteering based on series-connected radiative patches and nonreciprocal phase shifters.
- Sajjad Taravati
Article 15 July 2021 | Open Access
Polarization-transparent silicon photonic add-drop multiplexer with wideband hitless tuneability
Reconfigurable wavelength-selective devices are essential components of flexible optical networks. Here the authors show a silicon-photonic add-drop multiplexer meeting the strict requirements of telecom systems in terms of broadband operation range, hitless tunability and polarization transparency.
- Francesco Morichetti
- , Maziyar Milanizadeh
- & Andrea Melloni
Article 09 July 2021 | Open Access
Peta-bit-per-second optical communications system using a standard cladding diameter 15-mode fiber
Space division multiplexing solutions are one way to increase future fiber information capacity. Here, the authors show peta-bit/s transmission in a standard-diameter, multimode fiber enabled by combining several practical multiplexing technologies.
- Georg Rademacher
- , Benjamin J. Puttnam
- & Hideaki Furukawa
Review Article 28 June 2021 | Open Access
Semiconductor core fibres: materials science in a bottle
The application space for optical fibers is growing, enabled by fibers built using special materials and processes. In this Review, the authors discuss the materials science behind producing crystalline core fibers for diverse applications and progress in the field.
- Ursula J. Gibson
- & John Ballato
Article 07 June 2021 | Open Access
Private communication with quantum cascade laser photonic chaos
Free-space communication in the mid-IR domain has many potential applications, but security is still challenging. Here, the authors use chaos synchronization in a QCL-based free-space link as a way to increase privacy of such transmissions.
- Olivier Spitz
- , Andreas Herdt
- & Frédéric Grillot
Browse broader subjects
- Applied optics
Quick links
- Explore articles by subject
- Guide to authors
- Editorial policies

Help | Advanced Search
Physics > Optics
Title: an ultra-broadband photonic-chip-based traveling-wave parametric amplifier.
Abstract: Optical amplification, crucial for modern communication, primarily relies on erbium-doped fiber amplifiers (EDFAs) to enhance signals without distortion. However, EDFAs only cover a portion of the low-loss spectrum of optical fibers. Optical parametric amplification, utilizing material nonlinearities, offers expanded capacity, but has faced limitations in gain and bandwidth. Addressing this, we present a high-gain continuous-traveling-wave parametric amplifier, surpassing the erbium gain window. Leveraging low-loss gallium phosphide-on-insulator photonic integrated waveguides, we achieve up to 35 dB of parametric gain, with a fiber-to-fiber net gain exceeding 10 dB across a wide wavelength range of approximately 140 nm centered at 1550 nm. We demonstrate the capability to amplify ultra-weak signals, extending over 6 orders of magnitude of input powers, optical frequency combs, and communication signals, highlighting the practical potential of our photonic-chip-based amplifier.
Submission history
Access paper:.
- HTML (experimental)
- Other Formats

References & Citations
- Google Scholar
- Semantic Scholar
BibTeX formatted citation

Bibliographic and Citation Tools
Code, data and media associated with this article, recommenders and search tools.
- Institution
arXivLabs: experimental projects with community collaborators
arXivLabs is a framework that allows collaborators to develop and share new arXiv features directly on our website.
Both individuals and organizations that work with arXivLabs have embraced and accepted our values of openness, community, excellence, and user data privacy. arXiv is committed to these values and only works with partners that adhere to them.
Have an idea for a project that will add value for arXiv's community? Learn more about arXivLabs .
Optical communications research and technology: Fiber optics
Ieee account.
- Change Username/Password
- Update Address
Purchase Details
- Payment Options
- Order History
- View Purchased Documents
Profile Information
- Communications Preferences
- Profession and Education
- Technical Interests
- US & Canada: +1 800 678 4333
- Worldwide: +1 732 981 0060
- Contact & Support
- About IEEE Xplore
- Accessibility
- Terms of Use
- Nondiscrimination Policy
- Privacy & Opting Out of Cookies
A not-for-profit organization, IEEE is the world's largest technical professional organization dedicated to advancing technology for the benefit of humanity. © Copyright 2024 IEEE - All rights reserved. Use of this web site signifies your agreement to the terms and conditions.
NTRS - NASA Technical Reports Server
Available downloads, related records.
Volumes and issues
Volume 7 december 2010 dec 2010.
- Issue 1-4 December 2010
Volume 6 December 2009 Dec 2009
- Issue 1-6 December 2009
Volume 5 December 2008 Dec 2008
- Issue 1-6 December 2008
Volume 4 February - December 2007 Feb - Dec 2007
- Issue 6 December 2007
- Issue 4-5 September 2007
- Issue 3 June 2007
- Issue 2 April 2007
- Issue 1 February 2007
Volume 3 February - December 2006 Feb - Dec 2006
- Issue 6 December 2006
- Issue 5 October 2006
- Issue 4 August 2006
- Issue 3 June 2006
- Issue 2 April 2006
- Issue 1 February 2006
Volume 2 March - December 2005 Mar - Dec 2005
- Issue 6 December 2005
- Issue 5 November 2005
- Issue 4 October 2005
- Issue 3 September 2005
- Issue 2 June 2005
- Issue 1 March 2005
Volume 1 September - December 2004 Sept - Dec 2004
- Issue 4 December 2004
- Issue 3 November 2004
- Issue 2 October 2004
- Issue 1 September 2004
- Find a journal
- Publish with us
- Track your research
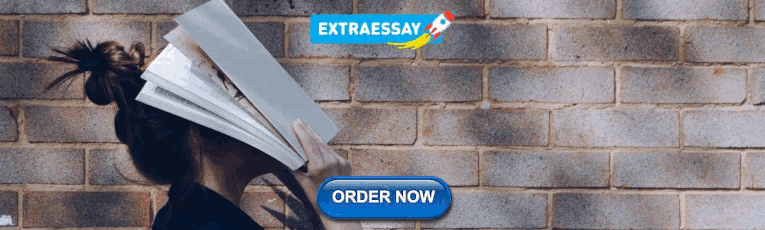
IMAGES
VIDEO
COMMENTS
The optic fiber communication system is an. essential infrastructure for the networks of. worldwide broadband [1]. The optical fibers. provide massive and unique transmission. bandwidth with ...
Fibre optics and optical communications is the use of thin strands of glass for sending information encoded into light over long distances. Total internal reflection prevents light inserted into ...
Fiber-based micro-endoscopes are minimally invasive, but 3D imaging is limited by the need for bulky optical elements or rigid fibers. Here, the authors demonstrate holographic imaging through ...
From gigabits to terabits of data transmission, Fiber optic communication is the most perfect as well as smartest choice. This sort of communication is used in the transmission of voice, video, images, and data over great distances, local area networks (LAN) or computer systems. The fiber optic communication system uses light-wave technology to transmit information over the fiber by converting ...
This paper represents a comprehensive exploration of mathematical modeling and analysis in the context of fiber communications, with a specific focus on optimizing performance and efficiency. Positioned at the vanguard of innovation within the field of nonlinear optics, this research stands as a pioneering effort, venturing into uncharted territory by comprehensively examining soliton ...
1. Introduction. The internet has become a fundamental human requirement in today's environment [1].Everyone wants a high-speed internet connection because they can't envision living without it [2].The optical fiber connection is a benefit to the internet because of the various advancements in internet services [3, 4].Light points are used to transmit information in optical fiber communication ...
This Topic aims to collect the results of research in these and other relevant areas of fiber optic communication. The submission of papers within those areas with strong connection to beyond 5G/6G/THz over fiber applications is strongly encouraged. Prof. Dr. (John) Xiupu Zhang. Dr. Gaoming Xu. Topic Editors.
Feature papers represent the most advanced research with significant potential for high impact in the field. A Feature Paper should be a substantial original Article that involves several techniques or approaches, provides an outlook for future research directions and describes possible research applications. ... Optical fiber communication ...
In this paper we present the simulative analysis of a 200 Gbps per wavelength (λ) 8-level pulse amplitude modulation (PAM-8) downstream communication over up to 20 km single mode fiber (SMF) in C-band based on direct detection (DD) achieving at least a 29 dB link power budget in a PON environment. We use chromatic dispersion digital pre ...
Abstract. This chapter begins with a brief history of optical communication before describing the main components of a modern optical communication system. Specific attention is paid to the development of low-loss optical fibers as they played an essential role after 1975. The evolution of fiber-optic communication systems is described through ...
Optical-fiber transmission lines appear attractive for a variety of communication applications in which twisted copper pairs and coaxial cables are now used. These applications range from onpremises data links and equipment wiring to interoffice and intercity telecommunications trunks. Experiments to explore the technical feasibility of glass fibers in these areas are presently in progress ...
DOI: 10.29026/oea.2022.210127 Optical multiplexing techniques and their marriage for on-chip and optical fiber communication: a review Svetlana Nikolaevna Khonina1,2*, Nikolay Lvovich Kazanskiy1,2, Muhammad Ali Butt2,3 and Sergei Vladimirovich Karpeev1,2 Herein, an attention-grabbing and up-to-date review related to major multiplexing techniques is presented which in-
As one of the four most significant inventions since World War II, optical fiber communication has provided a foundation for the development of the information society, carrying more than 90% of the global data traffic. However, it is expected that it will encounter a "capacity crisis" in the next two decades. This paper focuses on the five development dimensions of optical fiber ...
Various optical fiber connectors have been developed during the 40 years since optical fiber communications systems were first put into practical use. This paper describes the key technologies for optical connectors and recent technical issues.
systems are primarily point-to-point, applications emphasis is now on optical networks. This paper presents a tutorial overview of the basic technology, systems and applications of optical fiber communication. It is an update and expansion of a more descriptive and less technical earlier paper on these topics [4]. 567 O. D. D. Soares (ed ...
Optical fiber integrated-light diffuser probes emerged in 2019 as a promising technology due to their compact design configuration and robust performance; however, it does require a light source of stable output. The market for fiber-optic sensors has been growing very slowly to compete with the conventional sensor technology since 1980.
Optical amplification, crucial for modern communication, primarily relies on erbium-doped fiber amplifiers (EDFAs) to enhance signals without distortion. However, EDFAs only cover a portion of the low-loss spectrum of optical fibers. Optical parametric amplification, utilizing material nonlinearities, offers expanded capacity, but has faced limitations in gain and bandwidth. Addressing this ...
The current state of the art of fiber optics components is reviewed and projected uses of fiber optical technology are detailed. A brief discussion is presented describing the fundamentals of light guiding and the factors which affect the performance of light guides. Current fiber Optics capabilities in terms of attenuation, information capacity, fiber strength and fabrication are discussed ...
This paper will give an overview of studies using photonic lanterns in photon counting optical communication receivers where the detectors are small in aperture and fiber coupled. This paper will also survey other potential uses of photonic lanterns in coherent optical communication receivers or as wavefront sensors in adaptive optical systems.
Volume 1 September - December 2004. Issue 4 December 2004. Issue 3 November 2004. Issue 2 October 2004. Issue 1 September 2004. Volumes and issues listings for Journal of Optical and Fiber Communications Research.