
An official website of the United States government
The .gov means it’s official. Federal government websites often end in .gov or .mil. Before sharing sensitive information, make sure you’re on a federal government site.
The site is secure. The https:// ensures that you are connecting to the official website and that any information you provide is encrypted and transmitted securely.
- Publications
- Account settings
Preview improvements coming to the PMC website in October 2024. Learn More or Try it out now .
- Advanced Search
- Journal List
- HHS Author Manuscripts

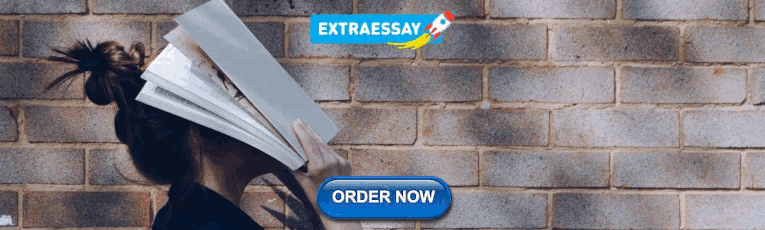
Single-Case Design, Analysis, and Quality Assessment for Intervention Research
Michele a. lobo.
1 Biomechanics & Movement Science Program, Department of Physical Therapy, University of Delaware, Newark, DE, USA
Mariola Moeyaert
2 Division of Educational Psychology & Methodology, State University of New York at Albany, Albany, NY, USA
Andrea Baraldi Cunha
Iryna babik, background and purpose.
The purpose of this article is to describe single-case studies, and contrast them with case studies and randomized clinical trials. We will highlight current research designs, analysis techniques, and quality appraisal tools relevant for single-case rehabilitation research.
Summary of Key Points
Single-case studies can provide a viable alternative to large group studies such as randomized clinical trials. Single case studies involve repeated measures, and manipulation of and independent variable. They can be designed to have strong internal validity for assessing causal relationships between interventions and outcomes, and external validity for generalizability of results, particularly when the study designs incorporate replication, randomization, and multiple participants. Single case studies should not be confused with case studies/series (ie, case reports), which are reports of clinical management of one patient or a small series of patients.
Recommendations for Clinical Practice
When rigorously designed, single-case studies can be particularly useful experimental designs in a variety of situations, even when researcher resources are limited, studied conditions have low incidences, or when examining effects of novel or expensive interventions. Readers will be directed to examples from the published literature in which these techniques have been discussed, evaluated for quality, and implemented.
Introduction
The purpose of this article is to present current tools and techniques relevant for single-case rehabilitation research. Single-case (SC) studies have been identified by a variety of names, including “n of 1 studies” and “single-subject” studies. The term “single-case study” is preferred over the previously mentioned terms because previous terms suggest these studies include only one participant. In fact, as will be discussed below, for purposes of replication and improved generalizability, the strongest SC studies commonly include more than one participant.
A SC study should not be confused with a “case study/series “ (also called “case report”. In a typical case study/series, a single patient or small series of patients is involved, but there is not a purposeful manipulation of an independent variable, nor are there necessarily repeated measures. Most case studies/series are reported in a narrative way while results of SC studies are presented numerically or graphically. 1 , 2 This article defines SC studies, contrasts them with randomized clinical trials, discusses how they can be used to scientifically test hypotheses, and highlights current research designs, analysis techniques, and quality appraisal tools that may be useful for rehabilitation researchers.
In SC studies, measurements of outcome (dependent variables) are recorded repeatedly for individual participants across time and varying levels of an intervention (independent variables). 1 – 5 These varying levels of intervention are referred to as “phases” with one phase serving as a baseline or comparison, so each participant serves as his/her own control. 2 In contrast to case studies and case series in which participants are observed across time without experimental manipulation of the independent variable, SC studies employ systematic manipulation of the independent variable to allow for hypothesis testing. 1 , 6 As a result, SC studies allow for rigorous experimental evaluation of intervention effects and provide a strong basis for establishing causal inferences. Advances in design and analysis techniques for SC studies observed in recent decades have made SC studies increasingly popular in educational and psychological research. Yet, the authors believe SC studies have been undervalued in rehabilitation research, where randomized clinical trials (RCTs) are typically recommended as the optimal research design to answer questions related to interventions. 7 In reality, there are advantages and disadvantages to both SC studies and RCTs that should be carefully considered in order to select the best design to answer individual research questions. While there are a variety of other research designs that could be utilized in rehabilitation research, only SC studies and RCTs are discussed here because SC studies are the focus of this article and RCTs are the most highly recommended design for intervention studies. 7
When designed and conducted properly, RCTs offer strong evidence that changes in outcomes may be related to provision of an intervention. However, RCTs require monetary, time, and personnel resources that many researchers, especially those in clinical settings, may not have available. 8 RCTs also require access to large numbers of consenting participants that meet strict inclusion and exclusion criteria that can limit variability of the sample and generalizability of results. 9 The requirement for large participant numbers may make RCTs difficult to perform in many settings, such as rural and suburban settings, and for many populations, such as those with diagnoses marked by lower prevalence. 8 To rely exclusively on RCTs has the potential to result in bodies of research that are skewed to address the needs of some individuals while neglecting the needs of others. RCTs aim to include a large number of participants and to use random group assignment to create study groups that are similar to one another in terms of all potential confounding variables, but it is challenging to identify all confounding variables. Finally, the results of RCTs are typically presented in terms of group means and standard deviations that may not represent true performance of any one participant. 10 This can present as a challenge for clinicians aiming to translate and implement these group findings at the level of the individual.
SC studies can provide a scientifically rigorous alternative to RCTs for experimentally determining the effectiveness of interventions. 1 , 2 SC studies can assess a variety of research questions, settings, cases, independent variables, and outcomes. 11 There are many benefits to SC studies that make them appealing for intervention research. SC studies may require fewer resources than RCTs and can be performed in settings and with populations that do not allow for large numbers of participants. 1 , 2 In SC studies, each participant serves as his/her own comparison, thus controlling for many confounding variables that can impact outcome in rehabilitation research, such as gender, age, socioeconomic level, cognition, home environment, and concurrent interventions. 2 , 11 Results can be analyzed and presented to determine whether interventions resulted in changes at the level of the individual, the level at which rehabilitation professionals intervene. 2 , 12 When properly designed and executed, SC studies can demonstrate strong internal validity to determine the likelihood of a causal relationship between the intervention and outcomes and external validity to generalize the findings to broader settings and populations. 2 , 12 , 13
Single Case Research Designs for Intervention Research
There are a variety of SC designs that can be used to study the effectiveness of interventions. Here we discuss: 1) AB designs, 2) reversal designs, 3) multiple baseline designs, and 4) alternating treatment designs, as well as ways replication and randomization techniques can be used to improve internal validity of all of these designs. 1 – 3 , 12 – 14
The simplest of these designs is the AB Design 15 ( Figure 1 ). This design involves repeated measurement of outcome variables throughout a baseline control/comparison phase (A ) and then throughout an intervention phase (B). When possible, it is recommended that a stable level and/or rate of change in performance be observed within the baseline phase before transitioning into the intervention phase. 2 As with all SC designs, it is also recommended that there be a minimum of five data points in each phase. 1 , 2 There is no randomization or replication of the baseline or intervention phases in the basic AB design. 2 Therefore, AB designs have problems with internal validity and generalizability of results. 12 They are weak in establishing causality because changes in outcome variables could be related to a variety of other factors, including maturation, experience, learning, and practice effects. 2 , 12 Sample data from a single case AB study performed to assess the impact of Floor Play intervention on social interaction and communication skills for a child with autism 15 are shown in Figure 1 .

An example of results from a single-case AB study conducted on one participant with autism; two weeks of observation (baseline phase A) were followed by seven weeks of Floor Time Play (intervention phase B). The outcome measure Circles of Communications (reciprocal communication with two participants responding to each other verbally or nonverbally) served as a behavioral indicator of the child’s social interaction and communication skills (higher scores indicating better performance). A statistically significant improvement in Circles of Communication was found during the intervention phase as compared to the baseline. Note that although a stable baseline is recommended for SC studies, it is not always possible to satisfy this requirement, as you will see in Figures 1 – 4 . Data were extracted from Dionne and Martini (2011) 15 utilizing Rohatgi’s WebPlotDigitizer software. 78
If an intervention does not have carry-over effects, it is recommended to use a Reversal Design . 2 For example, a reversal A 1 BA 2 design 16 ( Figure 2 ) includes alternation of the baseline and intervention phases, whereas a reversal A 1 B 1 A 2 B 2 design 17 ( Figure 3 ) consists of alternation of two baseline (A 1 , A 2 ) and two intervention (B 1 , B 2 ) phases. Incorporating at least four phases in the reversal design (i.e., A 1 B 1 A 2 B 2 or A 1 B 1 A 2 B 2 A 3 B 3 …) allows for a stronger determination of a causal relationship between the intervention and outcome variables, because the relationship can be demonstrated across at least three different points in time – change in outcome from A 1 to B 1 , from B 1 to A 2 , and from A 2 to B 2 . 18 Before using this design, however, researchers must determine that it is safe and ethical to withdraw the intervention, especially in cases where the intervention is effective and necessary. 12

An example of results from a single-case A 1 BA 2 study conducted on eight participants with stable multiple sclerosis (data on three participants were used for this example). Four weeks of observation (baseline phase A 1 ) were followed by eight weeks of core stability training (intervention phase B), then another four weeks of observation (baseline phase A 2 ). Forward functional reach test (the maximal distance the participant can reach forward or lateral beyond arm’s length, maintaining a fixed base of support in the standing position; higher scores indicating better performance) significantly improved during intervention for Participants 1 and 3 without further improvement observed following withdrawal of the intervention (during baseline phase A 2 ). Data were extracted from Freeman et al. (2010) 16 utilizing Rohatgi’s WebPlotDigitizer software. 78

An example of results from a single-case A 1 B 1 A 2 B 2 study conducted on two participants with severe unilateral neglect after a right-hemisphere stroke. Two weeks of conventional treatment (baseline phases A 1, A 2 ) alternated with two weeks of visuo-spatio-motor cueing (intervention phases B 1 , B 2 ). Performance was assessed in two tests of lateral neglect, the Bells Cancellation Test (Figure A; lower scores indicating better performance) and the Line Bisection Test (Figure B; higher scores indicating better performance). There was a statistically significant intervention-related improvement in participants’ performance on the Line Bisection Test, but not on the Bells Test. Data were extracted from Samuel at al. (2000) 17 utilizing Rohatgi’s WebPlotDigitizer software. 78
A recent study used an ABA reversal SC study to determine the effectiveness of core stability training in 8 participants with multiple sclerosis. 16 During the first four weekly data collections, the researchers ensured a stable baseline, which was followed by eight weekly intervention data points, and concluded with four weekly withdrawal data points. Intervention significantly improved participants’ walking and reaching performance ( Figure 2 ). 16 This A 1 BA 2 design could have been strengthened by the addition of a second intervention phase for replication (A 1 B 1 A 2 B 2 ). For instance, a single-case A 1 B 1 A 2 B 2 withdrawal design aimed to assess the efficacy of rehabilitation using visuo-spatio-motor cueing for two participants with severe unilateral neglect after a severe right-hemisphere stroke. 17 Each phase included 8 data points. Statistically significant intervention-related improvement was observed, suggesting that visuo-spatio-motor cueing might be promising for treating individuals with very severe neglect ( Figure 3 ). 17
The reversal design can also incorporate a cross over design where each participant experiences more than one type of intervention. For instance, a B 1 C 1 B 2 C 2 design could be used to study the effects of two different interventions (B and C) on outcome measures. Challenges with including more than one intervention involve potential carry-over effects from earlier interventions and order effects that may impact the measured effectiveness of the interventions. 2 , 12 Including multiple participants and randomizing the order of intervention phase presentations are tools to help control for these types of effects. 19
When an intervention permanently changes an individual’s ability, a return to baseline performance is not feasible and reversal designs are not appropriate. Multiple Baseline Designs (MBDs) are useful in these situations ( Figure 4 ). 20 MBDs feature staggered introduction of the intervention across time: each participant is randomly assigned to one of at least 3 experimental conditions characterized by the length of the baseline phase. 21 These studies involve more than one participant, thus functioning as SC studies with replication across participants. Staggered introduction of the intervention allows for separation of intervention effects from those of maturation, experience, learning, and practice. For example, a multiple baseline SC study was used to investigate the effect of an anti-spasticity baclofen medication on stiffness in five adult males with spinal cord injury. 20 The subjects were randomly assigned to receive 5–9 baseline data points with a placebo treatment prior to the initiation of the intervention phase with the medication. Both participants and assessors were blind to the experimental condition. The results suggested that baclofen might not be a universal treatment choice for all individuals with spasticity resulting from a traumatic spinal cord injury ( Figure 4 ). 20

An example of results from a single-case multiple baseline study conducted on five participants with spasticity due to traumatic spinal cord injury. Total duration of data collection was nine weeks. The first participant was switched from placebo treatment (baseline) to baclofen treatment (intervention) after five data collection sessions, whereas each consecutive participant was switched to baclofen intervention at the subsequent sessions through the ninth session. There was no statistically significant effect of baclofen on viscous stiffness at the ankle joint. Data were extracted from Hinderer at al. (1990) 20 utilizing Rohatgi’s WebPlotDigitizer software. 78
The impact of two or more interventions can also be assessed via Alternating Treatment Designs (ATDs) . In ATDs, after establishing the baseline, the experimenter exposes subjects to different intervention conditions administered in close proximity for equal intervals ( Figure 5 ). 22 ATDs are prone to “carry-over effects” when the effects of one intervention influence the observed outcomes of another intervention. 1 As a result, such designs introduce unique challenges when attempting to determine the effects of any one intervention and have been less commonly utilized in rehabilitation. An ATD was used to monitor disruptive behaviors in the school setting throughout a baseline followed by an alternating treatment phase with randomized presentation of a control condition or an exercise condition. 23 Results showed that 30 minutes of moderate to intense physical activity decreased behavioral disruptions through 90 minutes after the intervention. 23 An ATD was also used to compare the effects of commercially available and custom-made video prompts on the performance of multi-step cooking tasks in four participants with autism. 22 Results showed that participants independently performed more steps with the custom-made video prompts ( Figure 5 ). 22

An example of results from a single case alternating treatment study conducted on four participants with autism (data on two participants were used for this example). After the observation phase (baseline), effects of commercially available and custom-made video prompts on the performance of multi-step cooking tasks were identified (treatment phase), after which only the best treatment was used (best treatment phase). Custom-made video prompts were most effective for improving participants’ performance of multi-step cooking tasks. Data were extracted from Mechling at al. (2013) 22 utilizing Rohatgi’s WebPlotDigitizer software. 78
Regardless of the SC study design, replication and randomization should be incorporated when possible to improve internal and external validity. 11 The reversal design is an example of replication across study phases. The minimum number of phase replications needed to meet quality standards is three (A 1 B 1 A 2 B 2 ), but having four or more replications is highly recommended (A 1 B 1 A 2 B 2 A 3 …). 11 , 14 In cases when interventions aim to produce lasting changes in participants’ abilities, replication of findings may be demonstrated by replicating intervention effects across multiple participants (as in multiple-participant AB designs), or across multiple settings, tasks, or service providers. When the results of an intervention are replicated across multiple reversals, participants, and/or contexts, there is an increased likelihood a causal relationship exists between the intervention and the outcome. 2 , 12
Randomization should be incorporated in SC studies to improve internal validity and the ability to assess for causal relationships among interventions and outcomes. 11 In contrast to traditional group designs, SC studies often do not have multiple participants or units that can be randomly assigned to different intervention conditions. Instead, in randomized phase-order designs , the sequence of phases is randomized. Simple or block randomization is possible. For example, with simple randomization for an A 1 B 1 A 2 B 2 design, the A and B conditions are treated as separate units and are randomly assigned to be administered for each of the pre-defined data collection points. As a result, any combination of A-B sequences is possible without restrictions on the number of times each condition is administered or regard for repetitions of conditions (e.g., A 1 B 1 B 2 A 2 B 3 B 4 B 5 A 3 B 6 A 4 A 5 A 6 ). With block randomization for an A 1 B 1 A 2 B 2 design, two conditions (e.g., A and B) would be blocked into a single unit (AB or BA), randomization of which to different time periods would ensure that each condition appears in the resulting sequence more than two times (e.g., A 1 B 1 B 2 A 2 A 3 B 3 A 4 B 4 ). Note that AB and reversal designs require that the baseline (A) always precedes the first intervention (B), which should be accounted for in the randomization scheme. 2 , 11
In randomized phase start-point designs , the lengths of the A and B phases can be randomized. 2 , 11 , 24 – 26 For example, for an AB design, researchers could specify the number of time points at which outcome data will be collected, (e.g., 20), define the minimum number of data points desired in each phase (e.g., 4 for A, 3 for B), and then randomize the initiation of the intervention so that it occurs anywhere between the remaining time points (points 5 and 17 in the current example). 27 , 28 For multiple-baseline designs, a dual-randomization, or “regulated randomization” procedure has been recommended. 29 If multiple-baseline randomization depends solely on chance, it could be the case that all units are assigned to begin intervention at points not really separated in time. 30 Such randomly selected initiation of the intervention would result in the drastic reduction of the discriminant and internal validity of the study. 29 To eliminate this issue, investigators should first specify appropriate intervals between the start points for different units, then randomly select from those intervals, and finally randomly assign each unit to a start point. 29
Single Case Analysis Techniques for Intervention Research
The What Works Clearinghouse (WWC) single-case design technical documentation provides an excellent overview of appropriate SC study analysis techniques to evaluate the effectiveness of intervention effects. 1 , 18 First, visual analyses are recommended to determine whether there is a functional relation between the intervention and the outcome. Second, if evidence for a functional effect is present, the visual analysis is supplemented with quantitative analysis methods evaluating the magnitude of the intervention effect. Third, effect sizes are combined across cases to estimate overall average intervention effects which contributes to evidence-based practice, theory, and future applications. 2 , 18
Visual Analysis
Traditionally, SC study data are presented graphically. When more than one participant engages in a study, a spaghetti plot showing all of their data in the same figure can be helpful for visualization. Visual analysis of graphed data has been the traditional method for evaluating treatment effects in SC research. 1 , 12 , 31 , 32 The visual analysis involves evaluating level, trend, and stability of the data within each phase (i.e., within-phase data examination) followed by examination of the immediacy of effect, consistency of data patterns, and overlap of data between baseline and intervention phases (i.e., between-phase comparisons). When the changes (and/or variability) in level are in the desired direction, are immediate, readily discernible, and maintained over time, it is concluded that the changes in behavior across phases result from the implemented treatment and are indicative of improvement. 33 Three demonstrations of an intervention effect are necessary for establishing a functional relation. 1
Within-phase examination
Level, trend, and stability of the data within each phase are evaluated. Mean and/or median can be used to report the level, and trend can be evaluated by determining whether the data points are monotonically increasing or decreasing. Within-phase stability can be evaluated by calculating the percentage of data points within 15% of the phase median (or mean). The stability criterion is satisfied if about 85% (80% – 90%) of the data in a phase fall within a 15% range of the median (or average) of all data points for that phase. 34
Between-phase examination
Immediacy of effect, consistency of data patterns, and overlap of data between baseline and intervention phases are evaluated next. For this, several nonoverlap indices have been proposed that all quantify the proportion of measurements in the intervention phase not overlapping with the baseline measurements. 35 Nonoverlap statistics are typically scaled as percent from 0 to 100, or as a proportion from 0 to 1. Here, we briefly discuss the Nonoverlap of All Pairs ( NAP ), 36 the Extended Celeration Line ( ECL ), the Improvement Rate Difference ( IRD) , 37 and the TauU and the TauU-adjusted, TauU adj , 35 as these are the most recent and complete techniques. We also examine the Percentage of Nonoverlapping Data ( PND ) 38 and the Two Standard Deviations Band Method, as these are frequently used techniques. In addition, we include the Percentage of Nonoverlapping Corrected Data ( PNCD ) – an index applying to the PND after controlling for baseline trend. 39
Nonoverlap of all pairs (NAP)
Each baseline observation can be paired with each intervention phase observation to make n pairs (i.e., N = n A * n B ). Count the number of overlapping pairs, n o , counting all ties as 0.5. Then define the percent of the pairs that show no overlap. Alternatively, one can count the number of positive (P), negative (N), and tied (T) pairs 2 , 36 :
Extended Celeration Line (ECL)
ECL or split middle line allows control for a positive Phase A trend. Nonoverlap is defined as the proportion of Phase B ( n b ) data that are above the median trend plotted from Phase A data ( n B< sub > Above Median trend A </ sub > ), but then extended into Phase B: ECL = n B Above Median trend A n b ∗ 100
As a consequence, this method depends on a straight line and makes an assumption of linearity in the baseline. 2 , 12
Improvement rate difference (IRD)
This analysis is conceptualized as the difference in improvement rates (IR) between baseline ( IR B ) and intervention phases ( IR T ). 38 The IR for each phase is defined as the number of “improved data points” divided by the total data points in that phase. IRD, commonly employed in medical group research under the name of “risk reduction” or “risk difference” attempts to provide an intuitive interpretation for nonoverlap and to make use of an established, respected effect size, IR B - IR B , or the difference between two proportions. 37
TauU and TauU adj
Each baseline observation can be paired with each intervention phase observation to make n pairs (i.e., n = n A * n B ). Count the number of positive (P), negative (N), and tied (T) pairs, and use the following formula: TauU = P - N P + N + τ
The TauU adj is an adjustment of TauU for monotonic trend in baseline. Each baseline observation can be paired with each intervention phase observation to make n pairs (i.e., n = n A * n B ). Each baseline observation can be paired with all later baseline observations (n A *(n A -1)/2). 2 , 35 Then the baseline trend can be computed: TauU adf = P - N - S trend P + N + τ ; S trend = P A – NA
Online calculators might assist researchers in obtaining the TauU and TauU adjusted coefficients ( http://www.singlecaseresearch.org/calculators/tau-u ).
Percentage of nonoverlapping data (PND)
If anticipating an increase in the outcome, locate the highest data point in the baseline phase and then calculate the percent of the intervention phase data points that exceed it. If anticipating a decrease in the outcome, find the lowest data point in the baseline phase and then calculate the percent of the treatment phase data points that are below it: PND = n B Overlap A n b ∗ 100 . A PND < 50 would mark no observed effect, PND = 50–70 signifies a questionable effect, and PND > 70 suggests the intervention was effective. 40 The percentage of nonoverlapping (PNDC) corrected was proposed in 2009 as an extension of the PND. 39 Prior to applying the PND, a data correction procedure is applied eliminating pre-existing baseline trend. 38
Two Standard Deviation Band Method
When the stability criterion described above is met within phases, it is possible to apply the two standard deviation band method. 12 , 41 First, the mean of the data for a specific condition is calculated and represented with a solid line. In the next step, the standard deviation of the same data is computed and two dashed lines are represented: one located two standard deviations above the mean and the other – two standard deviations below. For normally distributed data, few points (less than 5%) are expected to be outside the two standard deviation bands if there is no change in the outcome score due to the intervention. However, this method is not considered a formal statistical procedure, as the data cannot typically be assumed to be normal, continuous, or independent. 41
Statistical Analysis
If the visual analysis indicates a functional relationship (i.e., three demonstrations of the effectiveness of the intervention effect), it is recommended to proceed with the quantitative analyses, reflecting the magnitude of the intervention effect. First, effect sizes are calculated for each participant (individual-level analysis). Moreover, if the research interest lies in the generalizability of the effect size across participants, effect sizes can be combined across cases to achieve an overall average effect size estimate (across-case effect size).
Note that quantitative analysis methods are still being developed in the domain of SC research 1 and statistical challenges of producing an acceptable measure of treatment effect remain. 14 , 42 , 43 Therefore, the WWC standards strongly recommend conducting sensitivity analysis and reporting multiple effect size estimators. If consistency across different effect size estimators is identified, there is stronger evidence for the effectiveness of the treatment. 1 , 18
Individual-level effect size analysis
The most common effect sizes recommended for SC analysis are: 1) standardized mean difference Cohen’s d ; 2) standardized mean difference with correction for small sample sizes Hedges’ g ; and 3) the regression-based approach which has the most potential and is strongly recommended by the WWC standards. 1 , 44 , 45 Cohen’s d can be calculated using following formula: d = X A ¯ - X B ¯ s p , with X A ¯ being the baseline mean, X B ¯ being the treatment mean, and s p indicating the pooled within-case standard deviation. Hedges’ g is an extension of Cohen’s d , recommended in the context of SC studies as it corrects for small sample sizes. The piecewise regression-based approach does not only reflect the immediate intervention effect, but also the intervention effect across time:
i stands for the measurement occasion ( i = 0, 1,… I ). The dependent variable is regressed on a time indicator, T , which is centered around the first observation of the intervention phase, D , a dummy variable for the intervention phase, and an interaction term of these variables. The equation shows that the expected score, Ŷ i , equals β 0 + β 1 T i in the baseline phase, and ( β 0 + β 2 ) + ( β 1 + β 3 ) T i in the intervention phase. β 0 , therefore, indicates the expected baseline level at the start of the intervention phase (when T = 0), whereas β 1 marks the linear time trend in the baseline scores. The coefficient β 2 can then be interpreted as an immediate effect of the intervention on the outcome, whereas β 3 signifies the effect of the intervention across time. The e i ’s are residuals assumed to be normally distributed around a mean of zero with a variance of σ e 2 . The assumption of independence of errors is usually not met in the context of SC studies because repeated measures are obtained within a person. As a consequence, it can be the case that the residuals are autocorrelated, meaning that errors closer in time are more related to each other compared to errors further away in time. 46 – 48 As a consequence, a lag-1 autocorrelation is appropriate (taking into account the correlation between two consecutive errors: e i and e i –1 ; for more details see Verbeke & Molenberghs, (2000). 49 In Equation 1 , ρ indicates the autocorrelation parameter. If ρ is positive, the errors closer in time are more similar; if ρ is negative, the errors closer in time are more different, and if ρ equals zero, there is no correlation between the errors.
Across-case effect sizes
Two-level modeling to estimate the intervention effects across cases can be used to evaluate across-case effect sizes. 44 , 45 , 50 Multilevel modeling is recommended by the WWC standards because it takes the hierarchical nature of SC studies into account: measurements are nested within cases and cases, in turn, are nested within studies. By conducting a multilevel analysis, important research questions can be addressed (which cannot be answered by single-level analysis of SC study data), such as: 1) What is the magnitude of the average treatment effect across cases? 2) What is the magnitude and direction of the case-specific intervention effect? 3) How much does the treatment effect vary within cases and across cases? 4) Does a case and/or study level predictor influence the treatment’s effect? The two-level model has been validated in previous research using extensive simulation studies. 45 , 46 , 51 The two-level model appears to have sufficient power (> .80) to detect large treatment effects in at least six participants with six measurements. 21
Furthermore, to estimate the across-case effect sizes, the HPS (Hedges, Pustejovsky, and Shadish) , or single-case educational design ( SCEdD)-specific mean difference, index can be calculated. 52 This is a standardized mean difference index specifically designed for SCEdD data, with the aim of making it comparable to Cohen’s d of group-comparison designs. The standard deviation takes into account both within-participant and between-participant variability, and is typically used to get an across-case estimator for a standardized change in level. The advantage of using the HPS across-case effect size estimator is that it is directly comparable with Cohen’s d for group comparison research, thus enabling the use of Cohen’s (1988) benchmarks. 53
Valuable recommendations on SC data analyses have recently been provided. 54 , 55 They suggest that a specific SC study data analytic technique can be chosen based on: (1) the study aims and the desired quantification (e.g., overall quantification, between-phase quantifications, randomization, etc.), (2) the data characteristics as assessed by visual inspection and the assumptions one is willing to make about the data, and (3) the knowledge and computational resources. 54 , 55 Table 1 lists recommended readings and some commonly used resources related to the design and analysis of single-case studies.
Recommend readings and resources related to the design and analysis of single-case studies.
Quality Appraisal Tools for Single-Case Design Research
Quality appraisal tools are important to guide researchers in designing strong experiments and conducting high-quality systematic reviews of the literature. Unfortunately, quality assessment tools for SC studies are relatively novel, ratings across tools demonstrate variability, and there is currently no “gold standard” tool. 56 Table 2 lists important SC study quality appraisal criteria compiled from the most common scales; when planning studies or reviewing the literature, we recommend readers consider these criteria. Table 3 lists some commonly used SC quality assessment and reporting tools and references to resources where the tools can be located.
Summary of important single-case study quality appraisal criteria.
Quality assessment and reporting tools related to single-case studies.
When an established tool is required for systematic review, we recommend use of the What Works Clearinghouse (WWC) Tool because it has well-defined criteria and is developed and supported by leading experts in the SC research field in association with the Institute of Education Sciences. 18 The WWC documentation provides clear standards and procedures to evaluate the quality of SC research; it assesses the internal validity of SC studies, classifying them as “Meeting Standards”, “Meeting Standards with Reservations”, or “Not Meeting Standards”. 1 , 18 Only studies classified in the first two categories are recommended for further visual analysis. Also, WWC evaluates the evidence of effect, classifying studies into “Strong Evidence of a Causal Relation”, “Moderate Evidence of a Causal Relation”, or “No Evidence of a Causal Relation”. Effect size should only be calculated for studies providing strong or moderate evidence of a causal relation.
The Single-Case Reporting Guideline In BEhavioural Interventions (SCRIBE) 2016 is another useful SC research tool developed recently to improve the quality of single-case designs. 57 SCRIBE consists of a 26-item checklist that researchers need to address while reporting the results of SC studies. This practical checklist allows for critical evaluation of SC studies during study planning, manuscript preparation, and review.
Single-case studies can be designed and analyzed in a rigorous manner that allows researchers strength in assessing causal relationships among interventions and outcomes, and in generalizing their results. 2 , 12 These studies can be strengthened via incorporating replication of findings across multiple study phases, participants, settings, or contexts, and by using randomization of conditions or phase lengths. 11 There are a variety of tools that can allow researchers to objectively analyze findings from SC studies. 56 While a variety of quality assessment tools exist for SC studies, they can be difficult to locate and utilize without experience, and different tools can provide variable results. The WWC quality assessment tool is recommended for those aiming to systematically review SC studies. 1 , 18
SC studies, like all types of study designs, have a variety of limitations. First, it can be challenging to collect at least five data points in a given study phase. This may be especially true when traveling for data collection is difficult for participants, or during the baseline phase when delaying intervention may not be safe or ethical. Power in SC studies is related to the number of data points gathered for each participant so it is important to avoid having a limited number of data points. 12 , 58 Second, SC studies are not always designed in a rigorous manner and, thus, may have poor internal validity. This limitation can be overcome by addressing key characteristics that strengthen SC designs ( Table 2 ). 1 , 14 , 18 Third, SC studies may have poor generalizability. This limitation can be overcome by including a greater number of participants, or units. Fourth, SC studies may require consultation from expert methodologists and statisticians to ensure proper study design and data analysis, especially to manage issues like autocorrelation and variability of data. 2 Fifth, while it is recommended to achieve a stable level and rate of performance throughout the baseline, human performance is quite variable and can make this requirement challenging. Finally, the most important validity threat to SC studies is maturation. This challenge must be considered during the design process in order to strengthen SC studies. 1 , 2 , 12 , 58
SC studies can be particularly useful for rehabilitation research. They allow researchers to closely track and report change at the level of the individual. They may require fewer resources and, thus, can allow for high-quality experimental research, even in clinical settings. Furthermore, they provide a tool for assessing causal relationships in populations and settings where large numbers of participants are not accessible. For all of these reasons, SC studies can serve as an effective method for assessing the impact of interventions.
Acknowledgments
This research was supported by the National Institute of Health, Eunice Kennedy Shriver National Institute of Child Health & Human Development (1R21HD076092-01A1, Lobo PI) and the Delaware Economic Development Office (Grant #109).
Some of the information in this manuscript was presented at the IV Step Meeting in Columbus, OH, June 2016.
Thank you for visiting nature.com. You are using a browser version with limited support for CSS. To obtain the best experience, we recommend you use a more up to date browser (or turn off compatibility mode in Internet Explorer). In the meantime, to ensure continued support, we are displaying the site without styles and JavaScript.
- View all journals
- Explore content
- About the journal
- Publish with us
- Sign up for alerts
- Published: 05 April 2024
Single-case experimental designs: the importance of randomization and replication
- René Tanious ORCID: orcid.org/0000-0002-5466-1002 1 ,
- Rumen Manolov ORCID: orcid.org/0000-0002-9387-1926 2 ,
- Patrick Onghena 3 &
- Johan W. S. Vlaeyen ORCID: orcid.org/0000-0003-0437-6665 1
Nature Reviews Methods Primers volume 4 , Article number: 27 ( 2024 ) Cite this article
23 Accesses
8 Altmetric
Metrics details
- Data acquisition
- Human behaviour
- Social sciences
Single-case experimental designs are rapidly growing in popularity. This popularity needs to be accompanied by transparent and well-justified methodological and statistical decisions. Appropriate experimental design including randomization, proper data handling and adequate reporting are needed to ensure reproducibility and internal validity. The degree of generalizability can be assessed through replication.
This is a preview of subscription content, access via your institution
Access options
Access Nature and 54 other Nature Portfolio journals
Get Nature+, our best-value online-access subscription
24,99 € / 30 days
cancel any time
Subscribe to this journal
Receive 1 digital issues and online access to articles
92,52 € per year
only 92,52 € per issue
Rent or buy this article
Prices vary by article type
Prices may be subject to local taxes which are calculated during checkout
Kazdin, A. E. Single-case experimental designs: characteristics, changes, and challenges. J. Exp. Anal. Behav. 115 , 56–85 (2021).
Article Google Scholar
Shadish, W. & Sullivan, K. J. Characteristics of single-case designs used to assess intervention effects in 2008. Behav. Res. 43 , 971–980 (2011).
Tanious, R. & Onghena, P. A systematic review of applied single-case research published between 2016 and 2018: study designs, randomization, data aspects, and data analysis. Behav. Res. 53 , 1371–1384 (2021).
Ferron, J., Foster-Johnson, L. & Kromrey, J. D. The functioning of single-case randomization tests with and without random assignment. J. Exp. Educ. 71 , 267–288 (2003).
Michiels, B., Heyvaert, M., Meulders, A. & Onghena, P. Confidence intervals for single-case effect size measures based on randomization test inversion. Behav. Res. 49 , 363–381 (2017).
Aydin, O. Characteristics of missing data in single-case experimental designs: an investigation of published data. Behav. Modif. https://doi.org/10.1177/01454455231212265 (2023).
De, T. K., Michiels, B., Tanious, R. & Onghena, P. Handling missing data in randomization tests for single-case experiments: a simulation study. Behav. Res. 52 , 1355–1370 (2020).
Baek, E., Luo, W. & Lam, K. H. Meta-analysis of single-case experimental design using multilevel modeling. Behav. Modif. 47 , 1546–1573 (2023).
Michiels, B., Tanious, R., De, T. K. & Onghena, P. A randomization test wrapper for synthesizing single-case experiments using multilevel models: a Monte Carlo simulation study. Behav. Res. 52 , 654–666 (2020).
Tate, R. L. et al. The single-case reporting guideline in behavioural interventions (SCRIBE) 2016: explanation and elaboration. Arch. Sci. Psychol. 4 , 10–31 (2016).
Google Scholar
Download references
Acknowledgements
R.T. and J.W.S.V. disclose support for the research of this work from the Dutch Research Council and the Dutch Ministry of Education, Culture and Science (NWO gravitation grant number 024.004.016) within the research project ‘New Science of Mental Disorders’ ( www.nsmd.eu ). R.M. discloses support from the Generalitat de Catalunya’s Agència de Gestió d’Ajusts Universitaris i de Recerca (grant number 2021SGR00366).
Author information
Authors and affiliations.
Experimental Health Psychology, Faculty of Psychology and Neuroscience, Maastricht University, Maastricht, the Netherlands
René Tanious & Johan W. S. Vlaeyen
Department of Social Psychology and Quantitative Psychology, Faculty of Psychology, University of Barcelona, Barcelona, Spain
Rumen Manolov
Methodology of Educational Sciences Research Group, Faculty of Psychology and Educational Science, KU Leuven, Leuven, Belgium
Patrick Onghena
You can also search for this author in PubMed Google Scholar
Corresponding author
Correspondence to René Tanious .
Ethics declarations
Competing interests.
The authors declare no competing interests.
Rights and permissions
Reprints and permissions
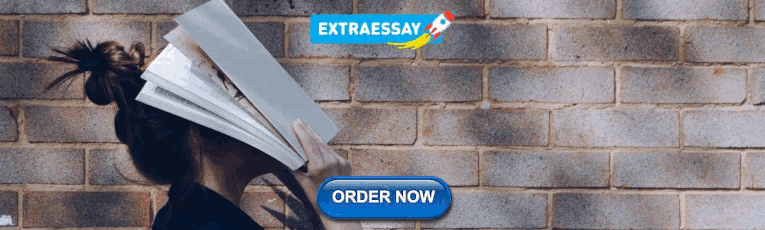
About this article
Cite this article.
Tanious, R., Manolov, R., Onghena, P. et al. Single-case experimental designs: the importance of randomization and replication. Nat Rev Methods Primers 4 , 27 (2024). https://doi.org/10.1038/s43586-024-00312-8
Download citation
Published : 05 April 2024
DOI : https://doi.org/10.1038/s43586-024-00312-8
Share this article
Anyone you share the following link with will be able to read this content:
Sorry, a shareable link is not currently available for this article.
Provided by the Springer Nature SharedIt content-sharing initiative
Quick links
- Explore articles by subject
- Guide to authors
- Editorial policies
Sign up for the Nature Briefing newsletter — what matters in science, free to your inbox daily.

Single-Case Design, Analysis, and Quality Assessment for Intervention Research
Affiliation.
- 1 Biomechanics & Movement Science Program, Department of Physical Therapy, University of Delaware, Newark, Delaware (M.A.L., A.B.C., I.B.); and Division of Educational Psychology & Methodology, State University of New York at Albany, Albany, New York (M.M.).
- PMID: 28628553
- PMCID: PMC5492992
- DOI: 10.1097/NPT.0000000000000187
Background and purpose: The purpose of this article is to describe single-case studies and contrast them with case studies and randomized clinical trials. We highlight current research designs, analysis techniques, and quality appraisal tools relevant for single-case rehabilitation research.
Summary of key points: Single-case studies can provide a viable alternative to large group studies such as randomized clinical trials. Single-case studies involve repeated measures and manipulation of an independent variable. They can be designed to have strong internal validity for assessing causal relationships between interventions and outcomes, as well as external validity for generalizability of results, particularly when the study designs incorporate replication, randomization, and multiple participants. Single-case studies should not be confused with case studies/series (ie, case reports), which are reports of clinical management of a patient or a small series of patients.
Recommendations for clinical practice: When rigorously designed, single-case studies can be particularly useful experimental designs in a variety of situations, such as when research resources are limited, studied conditions have low incidences, or when examining effects of novel or expensive interventions. Readers will be directed to examples from the published literature in which these techniques have been discussed, evaluated for quality, and implemented.
- Cohort Studies
- Medical Records*
- Quality Assurance, Health Care*
- Randomized Controlled Trials as Topic
- Research Design*
Grants and funding
- R21 HD076092/HD/NICHD NIH HHS/United States
The Advantages and Limitations of Single Case Study Analysis
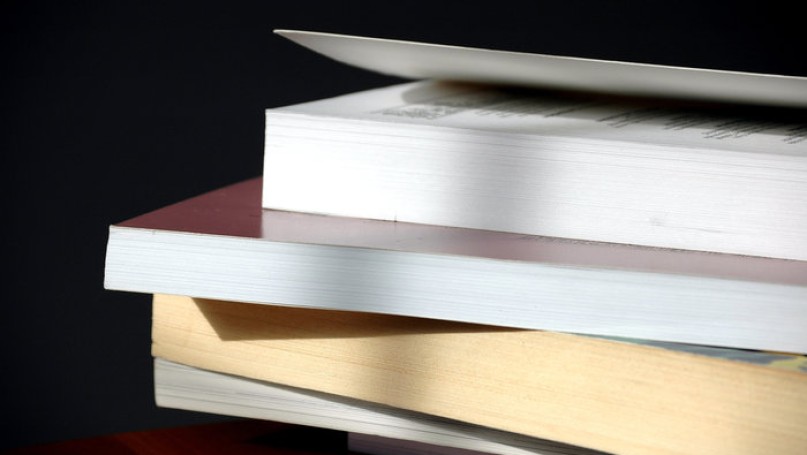
As Andrew Bennett and Colin Elman have recently noted, qualitative research methods presently enjoy “an almost unprecedented popularity and vitality… in the international relations sub-field”, such that they are now “indisputably prominent, if not pre-eminent” (2010: 499). This is, they suggest, due in no small part to the considerable advantages that case study methods in particular have to offer in studying the “complex and relatively unstructured and infrequent phenomena that lie at the heart of the subfield” (Bennett and Elman, 2007: 171). Using selected examples from within the International Relations literature[1], this paper aims to provide a brief overview of the main principles and distinctive advantages and limitations of single case study analysis. Divided into three inter-related sections, the paper therefore begins by first identifying the underlying principles that serve to constitute the case study as a particular research strategy, noting the somewhat contested nature of the approach in ontological, epistemological, and methodological terms. The second part then looks to the principal single case study types and their associated advantages, including those from within the recent ‘third generation’ of qualitative International Relations (IR) research. The final section of the paper then discusses the most commonly articulated limitations of single case studies; while accepting their susceptibility to criticism, it is however suggested that such weaknesses are somewhat exaggerated. The paper concludes that single case study analysis has a great deal to offer as a means of both understanding and explaining contemporary international relations.
The term ‘case study’, John Gerring has suggested, is “a definitional morass… Evidently, researchers have many different things in mind when they talk about case study research” (2006a: 17). It is possible, however, to distil some of the more commonly-agreed principles. One of the most prominent advocates of case study research, Robert Yin (2009: 14) defines it as “an empirical enquiry that investigates a contemporary phenomenon in depth and within its real-life context, especially when the boundaries between phenomenon and context are not clearly evident”. What this definition usefully captures is that case studies are intended – unlike more superficial and generalising methods – to provide a level of detail and understanding, similar to the ethnographer Clifford Geertz’s (1973) notion of ‘thick description’, that allows for the thorough analysis of the complex and particularistic nature of distinct phenomena. Another frequently cited proponent of the approach, Robert Stake, notes that as a form of research the case study “is defined by interest in an individual case, not by the methods of inquiry used”, and that “the object of study is a specific, unique, bounded system” (2008: 443, 445). As such, three key points can be derived from this – respectively concerning issues of ontology, epistemology, and methodology – that are central to the principles of single case study research.
First, the vital notion of ‘boundedness’ when it comes to the particular unit of analysis means that defining principles should incorporate both the synchronic (spatial) and diachronic (temporal) elements of any so-called ‘case’. As Gerring puts it, a case study should be “an intensive study of a single unit… a spatially bounded phenomenon – e.g. a nation-state, revolution, political party, election, or person – observed at a single point in time or over some delimited period of time” (2004: 342). It is important to note, however, that – whereas Gerring refers to a single unit of analysis – it may be that attention also necessarily be given to particular sub-units. This points to the important difference between what Yin refers to as an ‘holistic’ case design, with a single unit of analysis, and an ’embedded’ case design with multiple units of analysis (Yin, 2009: 50-52). The former, for example, would examine only the overall nature of an international organization, whereas the latter would also look to specific departments, programmes, or policies etc.
Secondly, as Tim May notes of the case study approach, “even the most fervent advocates acknowledge that the term has entered into understandings with little specification or discussion of purpose and process” (2011: 220). One of the principal reasons for this, he argues, is the relationship between the use of case studies in social research and the differing epistemological traditions – positivist, interpretivist, and others – within which it has been utilised. Philosophy of science concerns are obviously a complex issue, and beyond the scope of much of this paper. That said, the issue of how it is that we know what we know – of whether or not a single independent reality exists of which we as researchers can seek to provide explanation – does lead us to an important distinction to be made between so-called idiographic and nomothetic case studies (Gerring, 2006b). The former refers to those which purport to explain only a single case, are concerned with particularisation, and hence are typically (although not exclusively) associated with more interpretivist approaches. The latter are those focused studies that reflect upon a larger population and are more concerned with generalisation, as is often so with more positivist approaches[2]. The importance of this distinction, and its relation to the advantages and limitations of single case study analysis, is returned to below.
Thirdly, in methodological terms, given that the case study has often been seen as more of an interpretivist and idiographic tool, it has also been associated with a distinctly qualitative approach (Bryman, 2009: 67-68). However, as Yin notes, case studies can – like all forms of social science research – be exploratory, descriptive, and/or explanatory in nature. It is “a common misconception”, he notes, “that the various research methods should be arrayed hierarchically… many social scientists still deeply believe that case studies are only appropriate for the exploratory phase of an investigation” (Yin, 2009: 6). If case studies can reliably perform any or all three of these roles – and given that their in-depth approach may also require multiple sources of data and the within-case triangulation of methods – then it becomes readily apparent that they should not be limited to only one research paradigm. Exploratory and descriptive studies usually tend toward the qualitative and inductive, whereas explanatory studies are more often quantitative and deductive (David and Sutton, 2011: 165-166). As such, the association of case study analysis with a qualitative approach is a “methodological affinity, not a definitional requirement” (Gerring, 2006a: 36). It is perhaps better to think of case studies as transparadigmatic; it is mistaken to assume single case study analysis to adhere exclusively to a qualitative methodology (or an interpretivist epistemology) even if it – or rather, practitioners of it – may be so inclined. By extension, this also implies that single case study analysis therefore remains an option for a multitude of IR theories and issue areas; it is how this can be put to researchers’ advantage that is the subject of the next section.
Having elucidated the defining principles of the single case study approach, the paper now turns to an overview of its main benefits. As noted above, a lack of consensus still exists within the wider social science literature on the principles and purposes – and by extension the advantages and limitations – of case study research. Given that this paper is directed towards the particular sub-field of International Relations, it suggests Bennett and Elman’s (2010) more discipline-specific understanding of contemporary case study methods as an analytical framework. It begins however, by discussing Harry Eckstein’s seminal (1975) contribution to the potential advantages of the case study approach within the wider social sciences.
Eckstein proposed a taxonomy which usefully identified what he considered to be the five most relevant types of case study. Firstly were so-called configurative-idiographic studies, distinctly interpretivist in orientation and predicated on the assumption that “one cannot attain prediction and control in the natural science sense, but only understanding ( verstehen )… subjective values and modes of cognition are crucial” (1975: 132). Eckstein’s own sceptical view was that any interpreter ‘simply’ considers a body of observations that are not self-explanatory and “without hard rules of interpretation, may discern in them any number of patterns that are more or less equally plausible” (1975: 134). Those of a more post-modernist bent, of course – sharing an “incredulity towards meta-narratives”, in Lyotard’s (1994: xxiv) evocative phrase – would instead suggest that this more free-form approach actually be advantageous in delving into the subtleties and particularities of individual cases.
Eckstein’s four other types of case study, meanwhile, promote a more nomothetic (and positivist) usage. As described, disciplined-configurative studies were essentially about the use of pre-existing general theories, with a case acting “passively, in the main, as a receptacle for putting theories to work” (Eckstein, 1975: 136). As opposed to the opportunity this presented primarily for theory application, Eckstein identified heuristic case studies as explicit theoretical stimulants – thus having instead the intended advantage of theory-building. So-called p lausibility probes entailed preliminary attempts to determine whether initial hypotheses should be considered sound enough to warrant more rigorous and extensive testing. Finally, and perhaps most notably, Eckstein then outlined the idea of crucial case studies , within which he also included the idea of ‘most-likely’ and ‘least-likely’ cases; the essential characteristic of crucial cases being their specific theory-testing function.
Whilst Eckstein’s was an early contribution to refining the case study approach, Yin’s (2009: 47-52) more recent delineation of possible single case designs similarly assigns them roles in the applying, testing, or building of theory, as well as in the study of unique cases[3]. As a subset of the latter, however, Jack Levy (2008) notes that the advantages of idiographic cases are actually twofold. Firstly, as inductive/descriptive cases – akin to Eckstein’s configurative-idiographic cases – whereby they are highly descriptive, lacking in an explicit theoretical framework and therefore taking the form of “total history”. Secondly, they can operate as theory-guided case studies, but ones that seek only to explain or interpret a single historical episode rather than generalise beyond the case. Not only does this therefore incorporate ‘single-outcome’ studies concerned with establishing causal inference (Gerring, 2006b), it also provides room for the more postmodern approaches within IR theory, such as discourse analysis, that may have developed a distinct methodology but do not seek traditional social scientific forms of explanation.
Applying specifically to the state of the field in contemporary IR, Bennett and Elman identify a ‘third generation’ of mainstream qualitative scholars – rooted in a pragmatic scientific realist epistemology and advocating a pluralistic approach to methodology – that have, over the last fifteen years, “revised or added to essentially every aspect of traditional case study research methods” (2010: 502). They identify ‘process tracing’ as having emerged from this as a central method of within-case analysis. As Bennett and Checkel observe, this carries the advantage of offering a methodologically rigorous “analysis of evidence on processes, sequences, and conjunctures of events within a case, for the purposes of either developing or testing hypotheses about causal mechanisms that might causally explain the case” (2012: 10).
Harnessing various methods, process tracing may entail the inductive use of evidence from within a case to develop explanatory hypotheses, and deductive examination of the observable implications of hypothesised causal mechanisms to test their explanatory capability[4]. It involves providing not only a coherent explanation of the key sequential steps in a hypothesised process, but also sensitivity to alternative explanations as well as potential biases in the available evidence (Bennett and Elman 2010: 503-504). John Owen (1994), for example, demonstrates the advantages of process tracing in analysing whether the causal factors underpinning democratic peace theory are – as liberalism suggests – not epiphenomenal, but variously normative, institutional, or some given combination of the two or other unexplained mechanism inherent to liberal states. Within-case process tracing has also been identified as advantageous in addressing the complexity of path-dependent explanations and critical junctures – as for example with the development of political regime types – and their constituent elements of causal possibility, contingency, closure, and constraint (Bennett and Elman, 2006b).
Bennett and Elman (2010: 505-506) also identify the advantages of single case studies that are implicitly comparative: deviant, most-likely, least-likely, and crucial cases. Of these, so-called deviant cases are those whose outcome does not fit with prior theoretical expectations or wider empirical patterns – again, the use of inductive process tracing has the advantage of potentially generating new hypotheses from these, either particular to that individual case or potentially generalisable to a broader population. A classic example here is that of post-independence India as an outlier to the standard modernisation theory of democratisation, which holds that higher levels of socio-economic development are typically required for the transition to, and consolidation of, democratic rule (Lipset, 1959; Diamond, 1992). Absent these factors, MacMillan’s single case study analysis (2008) suggests the particularistic importance of the British colonial heritage, the ideology and leadership of the Indian National Congress, and the size and heterogeneity of the federal state.
Most-likely cases, as per Eckstein above, are those in which a theory is to be considered likely to provide a good explanation if it is to have any application at all, whereas least-likely cases are ‘tough test’ ones in which the posited theory is unlikely to provide good explanation (Bennett and Elman, 2010: 505). Levy (2008) neatly refers to the inferential logic of the least-likely case as the ‘Sinatra inference’ – if a theory can make it here, it can make it anywhere. Conversely, if a theory cannot pass a most-likely case, it is seriously impugned. Single case analysis can therefore be valuable for the testing of theoretical propositions, provided that predictions are relatively precise and measurement error is low (Levy, 2008: 12-13). As Gerring rightly observes of this potential for falsification:
“a positivist orientation toward the work of social science militates toward a greater appreciation of the case study format, not a denigration of that format, as is usually supposed” (Gerring, 2007: 247, emphasis added).
In summary, the various forms of single case study analysis can – through the application of multiple qualitative and/or quantitative research methods – provide a nuanced, empirically-rich, holistic account of specific phenomena. This may be particularly appropriate for those phenomena that are simply less amenable to more superficial measures and tests (or indeed any substantive form of quantification) as well as those for which our reasons for understanding and/or explaining them are irreducibly subjective – as, for example, with many of the normative and ethical issues associated with the practice of international relations. From various epistemological and analytical standpoints, single case study analysis can incorporate both idiographic sui generis cases and, where the potential for generalisation may exist, nomothetic case studies suitable for the testing and building of causal hypotheses. Finally, it should not be ignored that a signal advantage of the case study – with particular relevance to international relations – also exists at a more practical rather than theoretical level. This is, as Eckstein noted, “that it is economical for all resources: money, manpower, time, effort… especially important, of course, if studies are inherently costly, as they are if units are complex collective individuals ” (1975: 149-150, emphasis added).
Limitations
Single case study analysis has, however, been subject to a number of criticisms, the most common of which concern the inter-related issues of methodological rigour, researcher subjectivity, and external validity. With regard to the first point, the prototypical view here is that of Zeev Maoz (2002: 164-165), who suggests that “the use of the case study absolves the author from any kind of methodological considerations. Case studies have become in many cases a synonym for freeform research where anything goes”. The absence of systematic procedures for case study research is something that Yin (2009: 14-15) sees as traditionally the greatest concern due to a relative absence of methodological guidelines. As the previous section suggests, this critique seems somewhat unfair; many contemporary case study practitioners – and representing various strands of IR theory – have increasingly sought to clarify and develop their methodological techniques and epistemological grounding (Bennett and Elman, 2010: 499-500).
A second issue, again also incorporating issues of construct validity, concerns that of the reliability and replicability of various forms of single case study analysis. This is usually tied to a broader critique of qualitative research methods as a whole. However, whereas the latter obviously tend toward an explicitly-acknowledged interpretive basis for meanings, reasons, and understandings:
“quantitative measures appear objective, but only so long as we don’t ask questions about where and how the data were produced… pure objectivity is not a meaningful concept if the goal is to measure intangibles [as] these concepts only exist because we can interpret them” (Berg and Lune, 2010: 340).
The question of researcher subjectivity is a valid one, and it may be intended only as a methodological critique of what are obviously less formalised and researcher-independent methods (Verschuren, 2003). Owen (1994) and Layne’s (1994) contradictory process tracing results of interdemocratic war-avoidance during the Anglo-American crisis of 1861 to 1863 – from liberal and realist standpoints respectively – are a useful example. However, it does also rest on certain assumptions that can raise deeper and potentially irreconcilable ontological and epistemological issues. There are, regardless, plenty such as Bent Flyvbjerg (2006: 237) who suggest that the case study contains no greater bias toward verification than other methods of inquiry, and that “on the contrary, experience indicates that the case study contains a greater bias toward falsification of preconceived notions than toward verification”.
The third and arguably most prominent critique of single case study analysis is the issue of external validity or generalisability. How is it that one case can reliably offer anything beyond the particular? “We always do better (or, in the extreme, no worse) with more observation as the basis of our generalization”, as King et al write; “in all social science research and all prediction, it is important that we be as explicit as possible about the degree of uncertainty that accompanies out prediction” (1994: 212). This is an unavoidably valid criticism. It may be that theories which pass a single crucial case study test, for example, require rare antecedent conditions and therefore actually have little explanatory range. These conditions may emerge more clearly, as Van Evera (1997: 51-54) notes, from large-N studies in which cases that lack them present themselves as outliers exhibiting a theory’s cause but without its predicted outcome. As with the case of Indian democratisation above, it would logically be preferable to conduct large-N analysis beforehand to identify that state’s non-representative nature in relation to the broader population.
There are, however, three important qualifiers to the argument about generalisation that deserve particular mention here. The first is that with regard to an idiographic single-outcome case study, as Eckstein notes, the criticism is “mitigated by the fact that its capability to do so [is] never claimed by its exponents; in fact it is often explicitly repudiated” (1975: 134). Criticism of generalisability is of little relevance when the intention is one of particularisation. A second qualifier relates to the difference between statistical and analytical generalisation; single case studies are clearly less appropriate for the former but arguably retain significant utility for the latter – the difference also between explanatory and exploratory, or theory-testing and theory-building, as discussed above. As Gerring puts it, “theory confirmation/disconfirmation is not the case study’s strong suit” (2004: 350). A third qualification relates to the issue of case selection. As Seawright and Gerring (2008) note, the generalisability of case studies can be increased by the strategic selection of cases. Representative or random samples may not be the most appropriate, given that they may not provide the richest insight (or indeed, that a random and unknown deviant case may appear). Instead, and properly used , atypical or extreme cases “often reveal more information because they activate more actors… and more basic mechanisms in the situation studied” (Flyvbjerg, 2006). Of course, this also points to the very serious limitation, as hinted at with the case of India above, that poor case selection may alternatively lead to overgeneralisation and/or grievous misunderstandings of the relationship between variables or processes (Bennett and Elman, 2006a: 460-463).
As Tim May (2011: 226) notes, “the goal for many proponents of case studies […] is to overcome dichotomies between generalizing and particularizing, quantitative and qualitative, deductive and inductive techniques”. Research aims should drive methodological choices, rather than narrow and dogmatic preconceived approaches. As demonstrated above, there are various advantages to both idiographic and nomothetic single case study analyses – notably the empirically-rich, context-specific, holistic accounts that they have to offer, and their contribution to theory-building and, to a lesser extent, that of theory-testing. Furthermore, while they do possess clear limitations, any research method involves necessary trade-offs; the inherent weaknesses of any one method, however, can potentially be offset by situating them within a broader, pluralistic mixed-method research strategy. Whether or not single case studies are used in this fashion, they clearly have a great deal to offer.
References
Bennett, A. and Checkel, J. T. (2012) ‘Process Tracing: From Philosophical Roots to Best Practice’, Simons Papers in Security and Development, No. 21/2012, School for International Studies, Simon Fraser University: Vancouver.
Bennett, A. and Elman, C. (2006a) ‘Qualitative Research: Recent Developments in Case Study Methods’, Annual Review of Political Science , 9, 455-476.
Bennett, A. and Elman, C. (2006b) ‘Complex Causal Relations and Case Study Methods: The Example of Path Dependence’, Political Analysis , 14, 3, 250-267.
Bennett, A. and Elman, C. (2007) ‘Case Study Methods in the International Relations Subfield’, Comparative Political Studies , 40, 2, 170-195.
Bennett, A. and Elman, C. (2010) Case Study Methods. In C. Reus-Smit and D. Snidal (eds) The Oxford Handbook of International Relations . Oxford University Press: Oxford. Ch. 29.
Berg, B. and Lune, H. (2012) Qualitative Research Methods for the Social Sciences . Pearson: London.
Bryman, A. (2012) Social Research Methods . Oxford University Press: Oxford.
David, M. and Sutton, C. D. (2011) Social Research: An Introduction . SAGE Publications Ltd: London.
Diamond, J. (1992) ‘Economic development and democracy reconsidered’, American Behavioral Scientist , 35, 4/5, 450-499.
Eckstein, H. (1975) Case Study and Theory in Political Science. In R. Gomm, M. Hammersley, and P. Foster (eds) Case Study Method . SAGE Publications Ltd: London.
Flyvbjerg, B. (2006) ‘Five Misunderstandings About Case-Study Research’, Qualitative Inquiry , 12, 2, 219-245.
Geertz, C. (1973) The Interpretation of Cultures: Selected Essays by Clifford Geertz . Basic Books Inc: New York.
Gerring, J. (2004) ‘What is a Case Study and What Is It Good for?’, American Political Science Review , 98, 2, 341-354.
Gerring, J. (2006a) Case Study Research: Principles and Practices . Cambridge University Press: Cambridge.
Gerring, J. (2006b) ‘Single-Outcome Studies: A Methodological Primer’, International Sociology , 21, 5, 707-734.
Gerring, J. (2007) ‘Is There a (Viable) Crucial-Case Method?’, Comparative Political Studies , 40, 3, 231-253.
King, G., Keohane, R. O. and Verba, S. (1994) Designing Social Inquiry: Scientific Inference in Qualitative Research . Princeton University Press: Chichester.
Layne, C. (1994) ‘Kant or Cant: The Myth of the Democratic Peace’, International Security , 19, 2, 5-49.
Levy, J. S. (2008) ‘Case Studies: Types, Designs, and Logics of Inference’, Conflict Management and Peace Science , 25, 1-18.
Lipset, S. M. (1959) ‘Some Social Requisites of Democracy: Economic Development and Political Legitimacy’, The American Political Science Review , 53, 1, 69-105.
Lyotard, J-F. (1984) The Postmodern Condition: A Report on Knowledge . University of Minnesota Press: Minneapolis.
MacMillan, A. (2008) ‘Deviant Democratization in India’, Democratization , 15, 4, 733-749.
Maoz, Z. (2002) Case study methodology in international studies: from storytelling to hypothesis testing. In F. P. Harvey and M. Brecher (eds) Evaluating Methodology in International Studies . University of Michigan Press: Ann Arbor.
May, T. (2011) Social Research: Issues, Methods and Process . Open University Press: Maidenhead.
Owen, J. M. (1994) ‘How Liberalism Produces Democratic Peace’, International Security , 19, 2, 87-125.
Seawright, J. and Gerring, J. (2008) ‘Case Selection Techniques in Case Study Research: A Menu of Qualitative and Quantitative Options’, Political Research Quarterly , 61, 2, 294-308.
Stake, R. E. (2008) Qualitative Case Studies. In N. K. Denzin and Y. S. Lincoln (eds) Strategies of Qualitative Inquiry . Sage Publications: Los Angeles. Ch. 17.
Van Evera, S. (1997) Guide to Methods for Students of Political Science . Cornell University Press: Ithaca.
Verschuren, P. J. M. (2003) ‘Case study as a research strategy: some ambiguities and opportunities’, International Journal of Social Research Methodology , 6, 2, 121-139.
Yin, R. K. (2009) Case Study Research: Design and Methods . SAGE Publications Ltd: London.
[1] The paper follows convention by differentiating between ‘International Relations’ as the academic discipline and ‘international relations’ as the subject of study.
[2] There is some similarity here with Stake’s (2008: 445-447) notion of intrinsic cases, those undertaken for a better understanding of the particular case, and instrumental ones that provide insight for the purposes of a wider external interest.
[3] These may be unique in the idiographic sense, or in nomothetic terms as an exception to the generalising suppositions of either probabilistic or deterministic theories (as per deviant cases, below).
[4] Although there are “philosophical hurdles to mount”, according to Bennett and Checkel, there exists no a priori reason as to why process tracing (as typically grounded in scientific realism) is fundamentally incompatible with various strands of positivism or interpretivism (2012: 18-19). By extension, it can therefore be incorporated by a range of contemporary mainstream IR theories.
— Written by: Ben Willis Written at: University of Plymouth Written for: David Brockington Date written: January 2013
Further Reading on E-International Relations
- Identity in International Conflicts: A Case Study of the Cuban Missile Crisis
- Imperialism’s Legacy in the Study of Contemporary Politics: The Case of Hegemonic Stability Theory
- Recreating a Nation’s Identity Through Symbolism: A Chinese Case Study
- Ontological Insecurity: A Case Study on Israeli-Palestinian Conflict in Jerusalem
- Terrorists or Freedom Fighters: A Case Study of ETA
- A Critical Assessment of Eco-Marxism: A Ghanaian Case Study
Please Consider Donating
Before you download your free e-book, please consider donating to support open access publishing.
E-IR is an independent non-profit publisher run by an all volunteer team. Your donations allow us to invest in new open access titles and pay our bandwidth bills to ensure we keep our existing titles free to view. Any amount, in any currency, is appreciated. Many thanks!
Donations are voluntary and not required to download the e-book - your link to download is below.
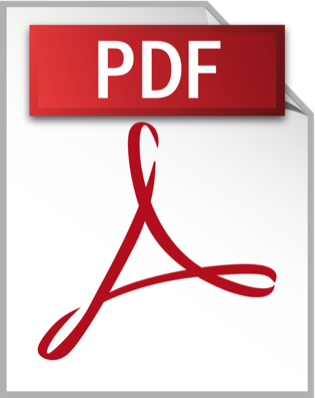
Power analysis for single-case designs: Computations for (AB) k designs
- Published: 12 October 2022
- Volume 55 , pages 3494–3503, ( 2023 )
Cite this article
- Larry V. Hedges 1 ,
- William R. Shadish 2 &
- Prathiba Natesan Batley 3
1644 Accesses
5 Citations
7 Altmetric
Explore all metrics
Currently, the design standards for single-case experimental designs (SCEDs) are based on validity considerations as prescribed by the What Works Clearinghouse. However, there is a need for design considerations such as power based on statistical analyses. We compute and derive power using computations for (AB) k designs with multiple cases which are common in SCEDs. Our computations show that effect size has the maximum impact on power followed by the number of subjects and then the number of phase reversals. An effect size of 0.75 or higher, at least one set of phase reversals (i.e., where k > 1), and at least three subjects showed high power. The latter two conditions agree with current standards about either having at least an ABAB design or a multiple baseline design with three subjects to meet design standards. An effect size of 0.75 or higher is not uncommon in SCEDs either. Autocorrelations, the number of time-points per phase, and intraclass correlations had a smaller but non-negligible impact on power. In sum, power analyses in the present study show that conditions to meet power requirements are not unreasonable in SCEDs. The software code to compute power is available on GitHub for the use of the reader.
Similar content being viewed by others
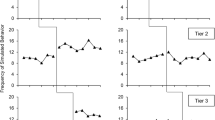
How Many Tiers Do We Need? Type I Errors and Power in Multiple Baseline Designs
Marc J. Lanovaz & Stéphanie Turgeon
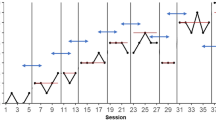
Type I error rates and power of two randomization test procedures for the changing criterion design
Rumen Manolov & René Tanious
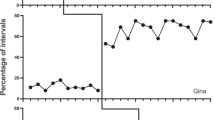
Revisiting an Analysis of Threats to Internal Validity in Multiple Baseline Designs
Timothy A. Slocum, P. Raymond Joslyn, … Sarah E. Pinkelman
Avoid common mistakes on your manuscript.
In all empirical studies, wise design mandates that the data collection plan should provide the basis for inferences about the phenomenon under study that are as unambiguous as possible. When studies are conducted for the purpose of evaluating the efficacy of an intervention (in this paper we will use the word “treatment”), design focuses on organizing the data collection to ensure that unambiguous inferences about the treatment effect are possible. In experimental studies that use statistical hypothesis testing as a primary means of analysis, statistical power analysis plays an important role in design. Power analysis is often used to help the investigator determine whether the study, as planned, is sufficiently sensitive to detect effects that are expected (that is, whether the design has a sufficiently large probability of detecting a treatment effect of the size that is expected). Alternatively, sometimes power analysis is used to ensure that a study is likely to detect the smallest treatment effect deemed to be practically meaningful.
Power analysis plays a major role in designing experimental studies where the probability of detecting an effect (the statistical power) depends on several design parameters in complex ways. For example, in cluster-randomized experiments (the most common design for randomized experiments used in education), statistical power depends on several factors that are under the control of the investigator: the number of clusters used, the sample size per cluster, and the significance level used. It also depends on several factors that are not under the control of the investigator: the treatment effect size, the ratio of between-cluster variation to total variation (also known as the intraclass correlation), and the effectiveness of any covariates that are used to control variation at various levels of the design. Power analysis informs decisions about how to choose values of the design parameters that are under the control of the investigator (e.g., number of clusters and sample size per cluster) given the assumed values of the design parameters that are not under the control of the investigator (e.g., the intraclass correlation and the covariate-outcome correlations). For obvious reasons, agencies that fund experimental work involving statistics, such as the US Institute of Education Sciences (IES) or the National Institutes of Health (NIH), require power analyses to support claims about the sensitivity of the designs in studies proposed for funding.
Research using single-case designs does not always use statistics as a primary mode of analysis. However, funding agencies typically expect that proposals for research should provide evidence that the designs chosen are sufficiently sensitive to detect the effects that treatments are expected to produce. As single-case designs are increasingly used in research that will be evaluated by funding agencies like IES and NIH, and as statistical analyses for those designs become increasingly accepted, some principled means of addressing the issue of design sensitivity is needed.
One approach to the issue of design sensitivity builds on the work on statistical analysis of data from single-case designs, namely statistical effect size measures (Hedges et al., 2012 ; Hedges et al., 2013 ). The focus of that work is not specifically the statistical analysis of single-case designs, but the representation of effects obtained via measures of effect size that are in the same metric as those employed in between-subjects designs, so-called design-comparable effect sizes (Shadish et al., 2013 ). However, because the null hypothesis corresponds to an effect size of zero, the statistical properties of the effect size estimate provide one method of statistical hypothesis testing and power analysis of the associated statistical test provides one means of assessing design sensitivity in single-case designs. We would argue that this principled method of evaluating design sensitivity is useful even if the ultimate analysis does not use the associated hypothesis testing apparatus.
Analysis of the results of single-subject designs has typically involved the visual search for functional relations between treatment assignment and outcome. That is, the study is designed so that each treatment (or baseline) phase is continued for enough measurements that the pattern of outcome values is clearly established. To establish functional relations, researchers often emphasize stability within treatment phases. Treatment effects are conceived as differences in these stable patterns between treatment and baseline phases.
Stability, however, can be conceptualized in several different ways. For example, the pattern could be one of fluctuation around a constant value with a common mean within a phase with a common residual variance within all phases. The pattern could also involve systematic increase or decrease across measurements in a phase, such as a linear or quadratic trend and a common residual variance within phases. Alternatively, the pattern could include a constant mean or a trend over measurements accompanied by systematic, increasing or decreasing residual variation around the trend. From this perspective, functional relations between treatment and outcome (what one would call treatment effects in between-subject designs) are understood to be differences between the stable states established within treatment phases. The simplest pattern of stability, and the one that a given set of data has most information about, is one that involves fluctuation around a constant value (a common mean with a common residual variance within phases). In this model of stability, treatment impacts correspond to shifts in the mean level of the outcome, although other models of stability are possible but not recommended for commonly seen SCED data conditions (see Natesan Batley & Hedges, 2021 ). We offered a statistical model in which the effect size parameter estimated corresponds to the standardized mean difference (Cohen’s d ), a well-known effect size parameter in between-subjects designs (Hedges et al., 2012 ).
In this article, we discuss power in the (AB) k design, the focus of Hedges et al. ( 2012 ). In that design, A is typically a baseline phase, B is typically a treatment phase, and k indicates the number of times that the AB pair is repeated. For instance, (AB) 2 indicates an ABAB design in which the initial baseline phase (A) is followed by a treatment phase (B), then treatment is removed in a return to baseline (A), and the treatment is reintroduced (B). Shadish and Sullivan ( 2008 ) found that the (AB) k design was the second most frequently used design in their systematic sample of single-case designs in 2008.
Model for the (AB) k design with n observations per phase
Suppose that the Y ij are normally distributed and that the data series for each individual i is weakly stationary within each phase with first-order autocorrelation φ . Specifically, if there be n observations in each phase for each individual, the statistical model for the j th observation which occurs in the p th phase is
The expressions in square brackets just assure that, in odd numbered phases (baseline phases), the coefficient of μ C is one and the coefficient of μ T is zero and that in even numbered phases (treatment phases), the coefficient of μ T is one and the coefficient of μ C is zero. Thus, for example, the statistical model for the first (baseline) phase, where p = 1, is
and the statistical model for the second (treatment) phase, where p = 2, is
Here, μ T – μ C represents the shift between baseline and treatment periods. We assume that individuals are independent and that the individual effects η i are independently normally distributed with variance τ 2 . The assumption that the time series is weakly stationary implies that the covariance of Y ij with Y i ( j+t ) depends only on t . We assume further that the ε ij have variance σ 2 and first-order autocorrelation φ within individuals. This autocorrelation model implies that the 2 kn x 2 kn covariance matrix of the errors within individuals for 2 k phases is of the form given in notation N1
The effect size parameter
It is conventional to assume that observations from different individuals are independent. Let us define the variance of observations within individuals within phases to be σ 2 and the variance of observations between individuals to be τ 2 , so that the total variance of each observation is σ 2 + τ 2 . Define the mean of the observations in the treatment phase by μ T and the mean of the observations in the baseline (control) phase by μ C . Under this model, define the effect size parameter as
This definition of the effect size is precisely the standardized mean difference (Cohen’s d -index) that is widely used in between-subjects experiments. As we discuss below, this effect size parameter can be estimated from single-case experiments as long as there are replications across individuals (that is m > 1). Note that the effect size parameter is the same in either the single-subject design or a corresponding between-subjects design (see Hedges et al., 2012 ).
Estimation and testing hypotheses about δ
The numerator of the effect size is the unweighted difference between the means in the baseline and treatment phases, namely
where \(\underline{Y}_{i^{\kern.05em\cdot}}^{2p}\) is the mean of phase 2 p and \(\underline{Y}_{i^{\kern.05em\cdot}}^{2p-1}\) is the mean for phase 2 p – 1 for individual i . Equation 2 assumes that there are equal number of observations in each phase.
The denominator of the effect size S is the square root of the variance across individuals at each timepoint but pooled across timepoints (and across phases). Thus, S 2 is defined as
where \({\underline{Y}}{\cdot j}\) is the average across individuals of the j th observations within individuals, given by
The effect size estimate ES is therefore
where \(\overline{D}\) is given in (2) and S 2 is given in (3). The sampling distribution of this effect size is related to that of the noncentral t -distribution and was given in Hedges et al. ( 2012 ). In Eq. ( 4 ), the expected value of \(\overline{D}\) is the average of within-person contrasts. Let the constant a be the variance of \(\overline{D}\) , while b and c are the (standardized) expectation and variance of S 2 . When σ 2 ≠ 0 (so that ρ ≠ 1), the statistic
has the noncentral t -distribution with h degrees of freedom and noncentrality parameter λ that are given as Footnote 1
In Eqs. ( 5 ), ( 6 ), and ( 7 ), the expressions for the constants a, b , and c depend on k, n, m, ϕ , σ , and τ and are given in the appendix to this paper. It turns out that these constants and the sampling distribution of the statistic t depend on σ and τ only through the ratio
the proportion of the total variance that is between persons. Thus, ρ is a kind of intraclass correlation for single-case designs.
Generally, h is a decreasing function of ρ, taking a maximum (which depends on φ, k, n, and m ) when ρ = 0 and a minimum of h = m – 1 when ρ = 1, as expected. When ρ = 1, σ = 0, so the statistic t is just a one sample t test on the baseline-treatment mean differences, which has m – 1 degrees of freedom. One interpretation of this behavior is that, when ρ = 1 (which implies σ 2 = 0), pooling the standard deviation across timepoints provides no more information about σ 2 + τ 2 = τ 2 than can be obtained from a single timepoint (because σ 2 = 0, observations do not vary across timepoints within individuals). However, when σ 2 > 0, pooling the standard deviation across timepoints does increase the information about σ 2 + τ 2 , so that the effective degrees of freedom are typically larger than m – 1. When both ϕ = 0 and ρ = 0, h = 2 kn ( m – 1) as expected since the m observations at any one of the 2 kn timepoints (with m – 1 degrees of freedom at each timepoint) are independent of the observations at other timepoints.
When the null hypothesis is true λ = 0 so the statistic t has the central t -distribution with ν degrees of freedom. The relation between ν and the autocorrelation φ is more complex. Holding other parameters constant, ν takes a maximum for a negative value of φ and decreases for larger or smaller values. In general, for moderate values of φ (e.g., – 0.5 ≤ φ ≤ 0.5), the dependence of ν on φ is less pronounced than the dependence of ν on ρ. Thus, a formal test of the hypothesis
(conditional on ρ and φ ) involves computing the statistic t given in Eq. ( 5 ) and rejecting H 0 if | t | > c α/2 , where c α/2 is the two-tailed level α critical value of the t -distribution with ν degrees of freedom. Note that the degrees of freedom will typically be fractional, so that interpolation between tabled critical values (or computation of exact values based on non-integer degrees of freedom) will be necessary.
Power analysis
The statistical power of the level α two-tailed test is
and the power of the level α one-tailed test is
where f( x | λ, ν ) is the cumulative distribution function of the noncentral t with noncentrality parameter λ and ν degrees of freedom, and λ and ν are given in Eqs. ( 7 ) and ( 6 ). This distribution function is available in many statistical packages, including R, STATA, SAS, and SPSS.
Power values can also be computed using standard power tables (such as those in Cohen, 1977 ) for the one sample t test. To use such tables, one typically enters the table on a row corresponding to the sample size and the column corresponding to the effect size and the value corresponding to that row and column is the power. Because those tables were designed for a slightly different purpose, it is necessary to enter the table with a synthetic sample size and a synthetic effect size to use such tables to compute the statistical power of the test for treatment effects in single-case designs. The synthetic total sample size is
and the synthetic effect size is
where ν is given by Eq. ( 6 ) above and a and b are given in the appendix. Note that interpolation between tabled values will usually be necessary because N Synthetic will usually not be an integer.
Generally, the parameters δ,φ , and ρ are not entirely under the control of the investigator. However, the number of subjects m and the number of observations per treatment period n are under the control of the investigator and they can be varied to ensure that the design has adequate sensitivity, given the values of δ, φ , and ρ . The situation is similar to that in the design of cluster-randomized experiments, where power depends on the effect size, intraclass correlation, and covariate–outcome correlations, which are not under the control of the investigator, but are determined by the context of the experiment. The number of clusters randomized and the number of individuals per cluster are under the control of the investigator and can be varied to ensure that the design has adequate sensitivity to detect the effect size expected.
Expression ( 5 ), ( 6 ), ( 7 ), and ( 9 ) conceal the somewhat complicated relation between the design parameters m, n, δ, φ , and ρ and statistical power. The most obvious fact that follows from Eq. ( 7 ) is that power is an increasing function of the effect size δ and the number of cases m . Calculations using the results of this paper reveal other, less obvious, relations. First power is a decreasing function of ρ , so that the larger the between-individual variance (as a fraction of the total variation), the lower the power.
Table 1 gives the eta-squared values of an ANOVA where power is the dependent variable and the data conditions such as k , m , n , ρ , φ , and d . The italicized values show the total variance explained by planned contrasts. Effect size explains the most variation in power (36.69%) followed by the number of subjects (16.71%), and the number of phases (10.11%). Interestingly, the number of observations, the autocorrelations, and the intraclass correlation had a small effect on power with each having an effect size of 2.7% or lower. Having an effect size of 0.5 versus higher explained the most variation in power (27.09%) followed by 0.75 versus higher values (7.10%). However, effect sizes of 1 or larger had very little variability in power. Similarly, increasing the number of participants beyond 3 did not lead to much increase in power. Finally, the power for an (AB) k design where k = 1 was much smaller than the power for a design where k ≥ 2 (8.82%). Beyond that, adding more phases did not lead to more power.
Figure 1 gives the statistical power as a function of ρ for φ = 0.1, 0.3, and 0.5 when m = 4, n = 4, k = 2, and δ = 0.75. These values for m and n are reasonably representative of the average single-case (AB) k design in the literature (Shadish & Sullivan, 2008 ); and (AB) k designs rarely have more pairs of phases than k = 2. Preliminary research suggests that effect sizes in single-case designs are typically used to investigate treatment that have relatively large effects (by the standards of between-subjects designs) and that effects are often larger than δ = 0.75 on average. Thus, the calculations we report here may underestimate the typical power of single-case designs that expect larger effects.
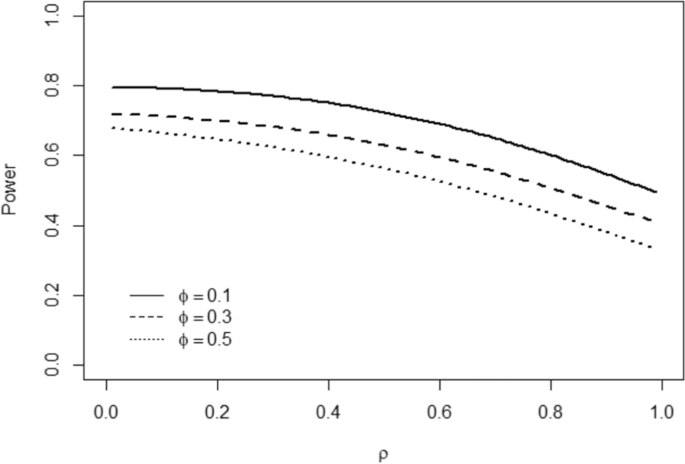
Power of the α = 0.05 two-tailed test as a function of ρ when φ = 0.1, 0.3, or 0.5 for effect size δ = 0.75, when k = 2, m = 4, and n = 4
The relation between statistical power and autocorrelation is not monotonic across the entire range of possible values. Holding k, n, m, and ρ equal, power decreases towards a minimum as ϕ increases from 0, but at some point, begins to increase again as ϕ approaches 1. Although there is a decrease in power followed by an increase in power, since the effect size of ϕ was only 2.1 we did not deem this to be practically significant enough to warrant an investigation. The location of the minimum depends primarily on ρ . Figure 2 gives the statistical power as a function of φ for ρ = 0.2, 0.5, and 0.8 when m = 4, n = 4, k = 2, and δ = 0.75. While the shape of the functions is somewhat different for different values of ρ , they all seem to have a minimum in the vicinity of φ = 0.6 to 0.8.
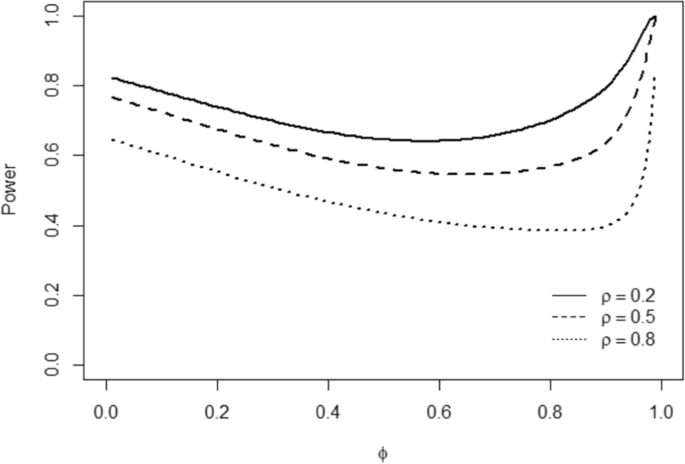
Power of the α = 0.05 two-tailed test as a function of φ when ρ = 0.2, 0.5, or 0.8 for effect size δ = 0.75, when k = 2, m = 4, and n = 4
Figures 1 and 2 provide some insight into the impact of design parameters that are not under the control of the investigator and will need to be imputed for the purposes of power analysis. Because the exact values of these parameters are unknown to the investigator planning a study, it is sensible (and likely considered essential by reviewers of research proposals) to estimate these parameters conservatively. That is, they should be imputed in a manner that is likely to err by underestimating, rather than overestimating, statistical power. The systematic behavior of power as a function of φ and empirical evidence from single-case design studies (Shadish et al., 2013 ; Shadish & Sullivan, 2008 ) suggests that using the value φ = 0.5 may be a sensible conservative default value for power analyses. Logical grounds dictate that the between-subject variation τ 2 is likely to be greater than within-subject-within-phase variation σ 2 , an argument for which there is also some empirical support (Shadish et al., 2013 ; Shadish & Sullivan, 2008 ). This would imply that ρ = 0.5 is a sensible conservative default value for power analyses. We urge the user to be skeptical of these default values and neither should be used if there is empirical or strong theoretical evidence about the values of these parameters when the study is being designed.
Turning to the variables under the control of the investigator, power is an increasing function of both m and n , but changes in m (the number of subjects) generally have a larger effect than corresponding changes in n (the number of observations per phase) when both are small. Figure 3 gives the statistical power as a function of m for n = 3, 6, and 9 where k = 2, ρ = 0.5, φ = 0.5, δ = 0.75. In contrast, Fig. 4 gives the statistical power as a function of n for m = 3, 6, and 9 where k = 2, ρ = 0.5, φ = 0.5, δ = 0.75. Comparing these two figures, the stronger dependence of power on m than on n is evident.
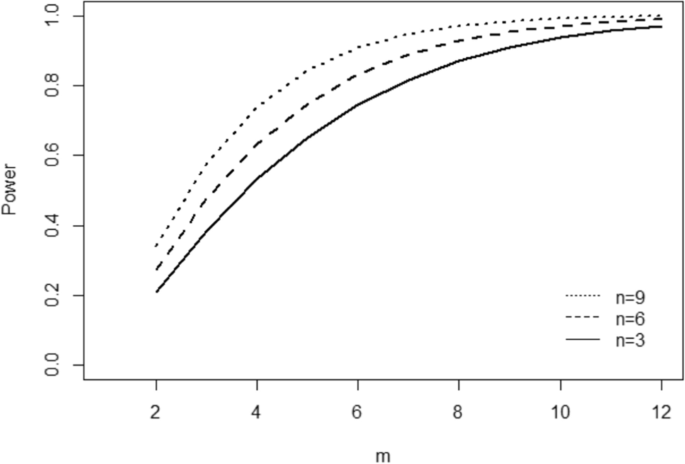
Power of the α = 0.05 two-tailed test as a function of m when n = 3, 6, or 9 for effect size δ = 0.75, when k = 2, φ = 0.5, and ρ = 0.5
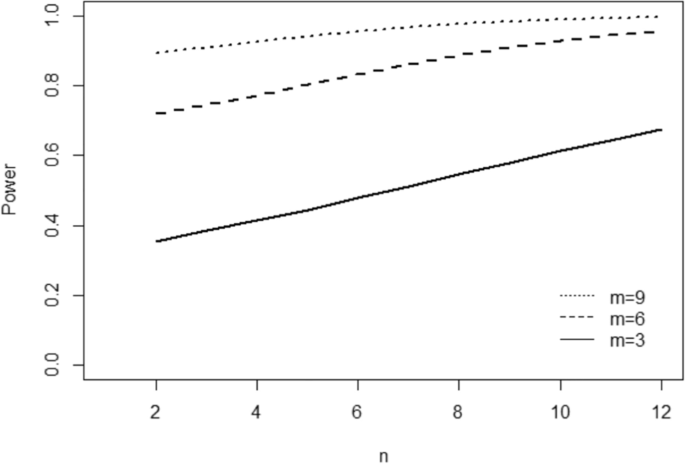
Power of the α = 0.05 two-tailed test as a function of n when m = 3, 6, or 9 for effect size δ = 0.75, when k = 2, φ = 0.5, and ρ = 0.5
Implications of design sensitivity for standards for single-subject designs
Power calculations can shed some light on general recommendations about single-case designs, like the standards that have been proposed by the US Institute of Education Sciences What Works Clearinghouse (WWC) (Kratochwill et al., 2010 ). Those recommendations were made based on many factors, and design sensitivity was only one of them. However, it is interesting to evaluate the consequences of those recommendations for design sensitivity. In the context of (AB) k designs, their recommendations imply at least k = 2 (to obtain three reversals) and n = 3 measurements per phase to meet standards with reservations or n = 5 measurements per phase to meet standards without reservations.
Consider first the implications for design sensitivity of the recommendation that k = 2 as opposed to k = 1 so that there are at least two reversals. Figure 5a gives the statistical power of the test for treatment effects as a function of δ for k =1 and k = 2 where n = 3, m = 3, ρ = 0.5, and φ = 0.5. This figure shows that the statistical power is quite low even for effect sizes as large as δ = 1.5, but that power is much higher for k = 2 than for k = 1. Figure 5b is analogous to Fig. 5a , except that the number m of individuals is increased to m = 5. Comparing Fig. 5a to Fig. 5b when k = 2, we see that power is generally larger with m = 5 than with m = 3, and power to detect effect sizes greater than or equal to δ =.8 is greater than 0.80 is achieved with when m = 5, but the power to detect effects of size δ = 0.75 is still only 0.39. With k = 2, n = 3, ρ = 0.5, and φ = 0.5, a total of m = 7 cases is required to obtain a power of 0.80 to detect an effect of δ = 0.75, but it would require m = 15 cases to do so when k = 1. Therefore, the requirement that studies have k ≥ 2 is quite sensible from the perspective of design sensitivity and power.
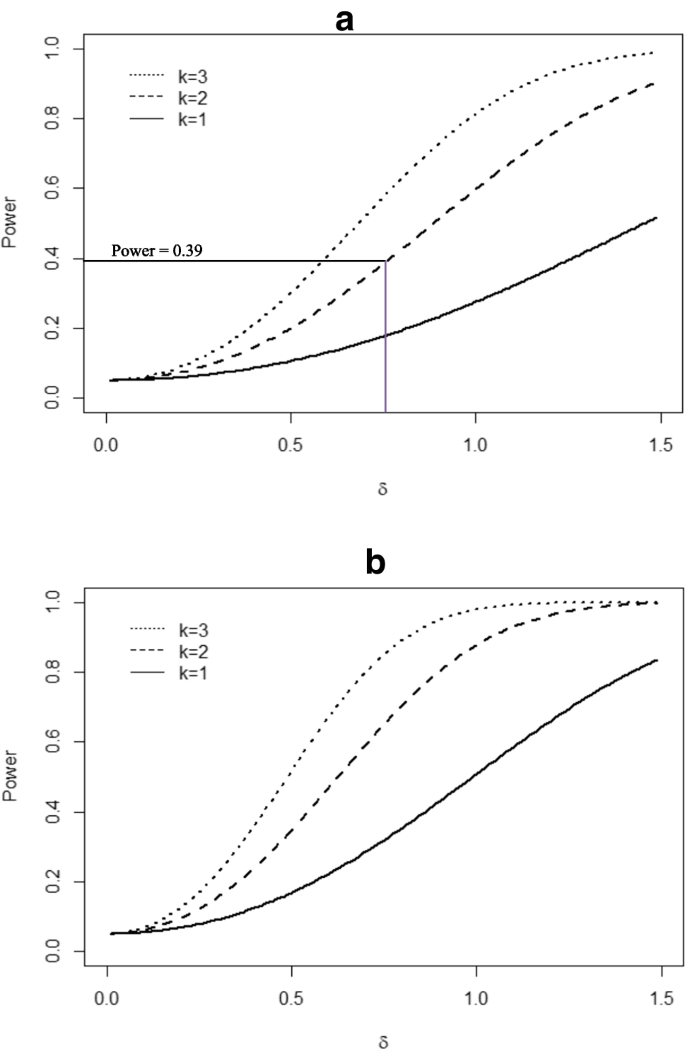
a Power of the α = 0.05 two-tailed test as a function of effect size δ for k = 1, k = 2, and k = 3, when n =3, m = 3, φ = 0.5, and ρ = 0.5
b Power of the α = 0.05 two-tailed test as a function of effect size δ for k = 1, k = 2, and k = 3, when n =3, m = 5, φ = 0.75, and ρ = 0.5
The WWC requires that there be at least three measurements per phase, that is n ≥ 3, to meet standards with reservations and at least five measurements per phase ( n ≥ 5) to meet standards without reservations. Figure 6a illustrates the statistical power of the test for treatment effects as a function of δ for n = 2, 3, and 5 where m = 2, ρ = 0.5, and φ = 0.5. This figure shows that the statistical power is quite low even for effect sizes as large as δ = 1, but that power is higher for n = 3 than for n = 2. Figure 6b is analogous to Fig. 6a , except that the number m of individuals is increased to m = 5. In Fig. 6b , when m = 5, the difference between the power with n = 2 and n = 3 is smaller than in Fig. 6a where m = 3. Comparing Fig. 6a with Fig. 6b when n = 3, we see that power is generally larger with m = 5 than with m = 3, and the difference between the power with n = 2 and n = 3 is smaller. Power to detect effect sizes of δ = 0.75 is greater than 0.80 when m = 5 and n = 5, but the power to detect effects of size δ = 0.75 is still only about 0.62 with n =2 and 0.652 with n = 3. With k = 2, n = 3, ρ = 0.5, and φ = 0.5, a total of m = 7 cases is required to obtain a power of at least 0.80 to detect an effect of δ = 0.75, but it would require eight cases to do so when n = 2. Therefore, the requirement that studies have n ≥ 3 is also sensible from the perspective of design sensitivity.
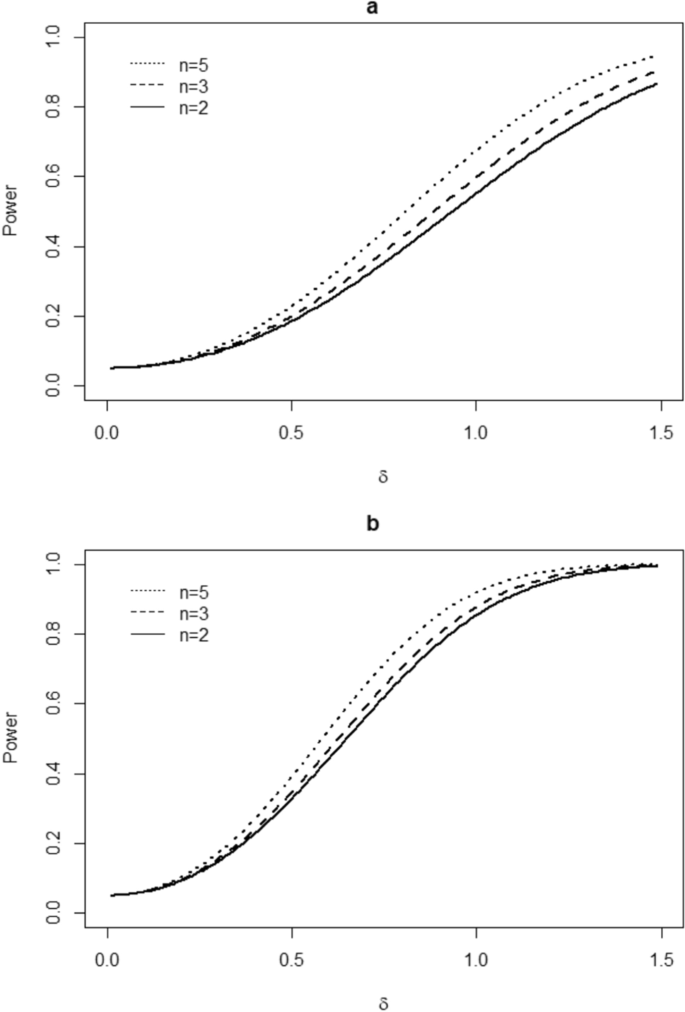
a Power of the α = 0.05 two-tailed test as a function of effect size δ for n = 2, 3, and 5 when m = 3, φ = 0.5, and ρ = 0.5
b Power of the α = 0.05 two-tailed test as a function of effect size δ for n = 2, 3, and 5, when m = 5, φ = 0.5, and ρ = 0.5
The number of replications across cases (the value of m ) has profound implications for design sensitivity. Figures 3 and 4 demonstrate that m has a greater effect on design sensitivity than does n . However, power is an increasing function of both m and n, and there are likely to be practical tradeoffs in the choice to increase one or the other. However, a case can be made, on design sensitivity grounds, that a sample size of m = 2 is too small to yield sensitive designs unless the effect size is exceptionally large. In the rest of this paragraph, consider a case where k = 2, ρ = 0.5, and φ = 0.5. To detect an effect of δ = 0.75 with at least 80% power, it would require n = 35 observations per phase (a total of 140 observations per case over the four phases of the design) if m = 2 and n = 18 observations per phase (a total of 72 observations per case) if m = 3, but only n = 12 observations per phase (a total of 48 observations per case) if m = 4, and n = 8 observations per phase (a total of 32 observations per case) if m = 5.
The findings mimic some of the earlier findings of simulation-based approaches in computing power for masked visual analysis (Ferron et al., 2017 ), multilevel models (Shadish & Zuur, 2014 ), or other complex models (Heyvaert et al., 2017 ; Natesan Batley & Hedges, 2021 ; Natesan Batley, Minka, & Hedges, 2020a ; Natesan & Hedges, 2017 ). All these studies, as expected show that more data means more information which means more power. However, not all types of data are the same. For instance, in this study we see that number of people can lead to more power than the number of observations.
The results given in this paper involve the assumption that the number of measurements in each phase for each subject is the same. This is analogous to the assumption of balance in experiments such as cluster randomized trials. This is typically a sensible assumption for assessing design sensitivity, but may be unrealistic in some studies, for example where the design is planned to give more observations during treatment phases than during baseline phases, or when k > 2 and its plan involves fewer observations in later phases of design. In such cases, the notation becomes considerably more complex as given in Appendix 2 .
Suppose that we are contemplating an (AB) 2 design to investigate a treatment that is expected to have an effect size of δ = 0.75 and wish to obtain a statistical power of at least 80% (0.80). We are unsure of the values of ρ and φ so we choose conservative values of ρ = 0.5 and φ = 0.5. We begin with the idea that we will observe n = 3 times in each phase and consider a sample size of m = 3 cases. Substituting k = 2, n = 3, and φ = 0.5 into expressions ( 8 ), ( 9 ), and ( 10 ), we obtain a = 0.1670, b = 1.6667, and c = 0.4571. Then substituting the values of b and c , along with φ = ρ = 0.5 into (6), we obtain h = 5.95. Substituting the values of a and b , along with m = 3, and ρ = 0.5 into expression ( 7 ), we obtain λ = 1.982. Substituting the value λ = 1.982 and h = 5.95 into expression ( 11 ), we obtain a two-tailed statistical power of p = 0.38, which is less than the target value of p = 0.80. At this point, we could consider increasing n , the number of measurements per phase, or m , the number of cases. Computing the statistical power with m = 3 but n = 7, 8, and 9 yields power of p = 0.47, 0.51, and 0.55, respectively, still less than the target value. Computing the statistical power with n = 3 but m = 5 yields power of p = 0.65, and increasing n to 6, 7, and 8 with m = 5 yields power values of 0.78, 0.81, and 0.84. If we both increase m to m = 6 and increase n to n = 5, the statistical power becomes p = 0.80. Design choices that yield power at or above 0.80 might depend on costs and feasibility of a larger number of measurements per phase versus a larger number of cases, a decision that would be best made in the context of a particular investigation.
Conclusions
The planning of research designs involves many considerations. Design sensitivity (statistical power) is only one of them. We would never advocate that power or design sensitivity should be the only consideration in planning a research design. However, sufficient design sensitivity is essential for statistical conclusion validity, and therefore should always be one consideration in planning research. We have provided one formal method of assessing design sensitivity of single-case research. These methods are consistent with recently developed methods for characterizing the effect size from single-case designs. Thus, they provide a natural complement to statistical analysis procedures involving effect sizes.
We argue that these methods may also be useful in planning research even if researchers do not intend to use statistical methods to analyze their findings or numerical effect sizes to characterize their magnitude. One reason is that visual methods do not offer an analogue to numerical methods for assessing design sensitivity. While rigorous visual analyses have many advantages, it is difficult to believe that they would be substantially more sensitive than statistical methods. Therefore, in the absence of visual analogues to power analysis, these numerical methods may be useful substitutes as input for planning single-case research studies.
One important caveat is that the effect sizes on which these methods are based address a specific kind of treatment effect: Shifts in the mean level of the outcome. The effect size measure, its associated significance test, and the power computations would not be relevant if a different kind of treatment effect were anticipated, such as a change in variation. However, a parallel method leading to numerical effect size measures, associated significance tests, and power analysis could be developed for treatment effects reflecting impacts on different stable patterns of outcome measurements. We are currently developing such methods. The power computations in the present study are only based on standardized mean difference effect sizes. However, there are several other effect sizes used in SCEDs for which power calculations would vary. This is an avenue for future research.
Power, as prescribed by the current study, can be computed only in designs with more than one subject. A typical ABAB type design uses only one participant, but investigations of treatments show that almost always ABAB type studies involve more than one subject. The power computations in the present study are only applicable to balanced designs, that is, with equal number of observations in each phase. This is a limitation considering that researchers might want to use longer phases for implementing their treatments and shorter baseline phases just to obtain consistency in data. We recognize that computing power using the codes given on GitHub ( https://github.com/prathiba-stat/ABk-power/blob/main/Power ) might be challenging to applied researchers and having a graphical user interface (GUI) for this purpose would be helpful. We are also developing power computations for multiple baseline designs which are most used in SCEDs. These efforts are already underway. SCED data are often not intervally scaled and might be count or percentage data (Natesan Batley, Shukla Mehta, & Hitchcock, 2020b ), in which case the current power calculations might not hold to be very accurate. We are currently developing effect sizes for count data that are also computing power using Monte Carlo simulations (Natesan Batley & Hedges, 2022 ). It might be interesting to explore the extent to which other violations of assumptions would affect power in (AB) k designs.
In the R program, the non-central t -distribution is solved using the F distribution as a squared value of t.
Cohen, J. (1977). Statistical power analysis for the behavioral sciences (2nd ed.). Academic Press. https://doi.org/10.4324/9780203771587
Book Google Scholar
Ferron, J. M., Joo, S.-H., & Levin, J. R. (2017). A Monte Carlo evaluation of masked visual analysis in response-guided versus fixed-criteria multiple-baseline designs. Journal of Applied Behavior Analysis, 50 , 701–716. https://doi.org/10.1002/jaba.410
Article PubMed Google Scholar
Hedges, L. V. (2007). Effect sizes in cluster randomized designs. Journal of Educational and Behavioral Statistics, 32 , 341–370. https://doi.org/10.3102/1076998606298043
Article Google Scholar
Hedges, L. V., Pustejovsky, J. E., & Shadish, W. R. (2012). A standardized mean difference effect size for single case designs. Journal of Research Synthesis Methods, 3 , 224–239. https://doi.org/10.1002/jrsm.1052
Hedges, L. V., Pustejovsky, J. E., & Shadish, W. R. (2013). A standardized mean difference effect size for multiple baseline designs. Journal of Research Synthesis Methods, 4 , 324–341. https://doi.org/10.1002/jrsm.1086
Heyvaert, M., Moeyaert, M., Verkempynck, P., Van Den Noortgate, W., Vervloet, M., Ugille, M., & Onghena, P. (2017). Testing the intervention effect in single-case experiments: A Monte Carlo simulation study. The Journal of Experimental Education, 85 (2), 175–196. https://doi.org/10.1080/00220973.2015.1123667
Kratochwill, T. R., Hitchcock, J., Horner, R. H., Levin, J. R., Odom, S. L., Rindskopf, D. M. & Shadish, W. R. (2010). Single-case designs technical documentation. Retrieved from What Works Clearinghouse website: http://ies.ed.gov/ncee/wwc/pdf/wwc_scd.pdf . Accessed 2 Jan 2022.
Natesan Batley, P., & Hedges, L. V. (2021). Accurate models vs. accurate estimates: A simulation study of Bayesian single-case experimental designs. Behavior Research Methods, 53 , 1782–1798. https://doi.org/10.3758/s13428-020-01522-0
Article PubMed PubMed Central Google Scholar
Natesan Batley, P. & Hedges, L. V. (2022). Design comparable Bayesian rate ratios for single case experimental designs with count data for unequal phase lengths. San Diego: Presented at the annual meeting of the American Educational Research Association.
Natesan Batley, P., Minka, T., & Hedges, L. V. (2020a). Investigating immediacy in multiple phase-change single case experimental designs using a Bayesian unknown change-points model. Behavior Research Methods, 52 , 1714–1728. https://doi.org/10.3758/s13428-020-01345-z
Natesan Batley, P., Shukla Mehta, S., & Hitchcock, J. (2020b). A Bayesian rate ratio effect size to quantify intervention effects for count data in single case experimental research. Behavioral Disorders . https://doi.org/10.1177/0198742920930704
Natesan, P., & Hedges, L. V. (2017). Bayesian unknown change-point models to investigate immediacy in single case designs. Psychological Methods, 22 , 743–759. https://doi.org/10.1037/met0000134
Shadish, W. R., & Sullivan, K. J. (2008). Characteristics of single-case designs used to assess intervention effects in 2008. Behavioral Research Methods, 43 , 971–980. https://doi.org/10.3758/s13428-011-0111-y
Shadish, W. R., & Zuur, A. F. (2014). Power Analysis for Negative Binomial Glmms for Single-Case Designs . Society for Multivariate Experimental Psychology.
Google Scholar
Shadish, W. R., Rindskopf, D. M., Hedges, L. V., & Sullivan, K. J. (2013). Bayesian estimates of autocorrelations in single-case designs. Behavioral Research Methods, 45 , 813–821. https://doi.org/10.1177/0741932512452794
Download references
This work was funded by a grant from the Institute of Education Sciences (IES grant) R305D220052.
Author information
Authors and affiliations.
Department of Statistics, Northwestern University, Evanston, IL, USA
Larry V. Hedges
University of California, Merced, CA, USA
William R. Shadish
Department of Counseling and Human Development, University of Louisville, Louisville, KY, USA
Prathiba Natesan Batley
You can also search for this author in PubMed Google Scholar
Corresponding author
Correspondence to Prathiba Natesan Batley .
Additional information
Publisher’s note.
Springer Nature remains neutral with regard to jurisdictional claims in published maps and institutional affiliations.
Supplementary information
(DOCX 20.6 kb)
Technical Appendix
The theory leading to the distribution of ES is described in Hedges et al. ( 2012 ) using a theorem from the appendix of Hedges ( 2007 ). It is somewhat simpler to explicate for balanced designs in matrix notation. Order the vector of 2 kn observations for the i th individual as
and define the 2 kn x 1 contrast vector consisting of k repeats of the sequence ( 1 n ′, - 1 n ′) as
where 1 n is an n- dimensional column vector of 1’s. Then the covariance matrix of y i is
Therefore, the within-person contrast is y i w , which has variance w′V i w and the variance of \(\overline{D}\) is
The constants b and c are obtained from the expectation and variance of S 2 . Let y ij be the j th measurement ( j = 1, …, 2 kn ) on the i th person ( i = 1, …, m ). Order the 2 knm observations from all m individuals as
then partition the 2 knm x 2 knm covariance matrix of y as
τ 2 is the between-subject variation, and I m is an m x m identity matrix. We can write S 2 as a quadratic form in y as y ′ Ay/ 2 kn ( m - 1), where A can be partitioned as
where A ii = I m – 1 m 1 m / m . Then
where tr( X ) is the trace of a square matrix X .
Power Computations in Unbalanced (AB) k Designs
For unbalanced designs power computations can be carried out by using the results from (Hedges et al., 2012 ; Hedges et al., 2013 ) Hedges et al. ( 2012 ) in place of those given in this paper for balanced designs. Specifically, the noncentrality parameter λ given in expression ( 7 ) of this paper is replaced by
where V{ S 2 } is the variance of S 2 given in expression (26) and \(V\left\{\overline{D}\right\}\) is given in expression (25) of Hedges et al. ( 2012 ). Similarly, the degrees of freedom h in expression ( 6 ) of this paper is replaced by ν in expression (28) of Hedges et al. ( 2012 ). Once the substitutions are made, the power analysis in unbalanced designs proceeds in the same way as in the balanced case described in this paper.
Rights and permissions
Springer Nature or its licensor holds exclusive rights to this article under a publishing agreement with the author(s) or other rightsholder(s); author self-archiving of the accepted manuscript version of this article is solely governed by the terms of such publishing agreement and applicable law.
Reprints and permissions
About this article
Hedges, L.V., Shadish, W.R. & Natesan Batley, P. Power analysis for single-case designs: Computations for (AB) k designs. Behav Res 55 , 3494–3503 (2023). https://doi.org/10.3758/s13428-022-01971-9
Download citation
Accepted : 29 August 2022
Published : 12 October 2022
Issue Date : October 2023
DOI : https://doi.org/10.3758/s13428-022-01971-9
Share this article
Anyone you share the following link with will be able to read this content:
Sorry, a shareable link is not currently available for this article.
Provided by the Springer Nature SharedIt content-sharing initiative
- Single case experimental designs
- Type-II error
- Type-I error
- Single case designs
- ABAB designs
- Find a journal
- Publish with us
- Track your research
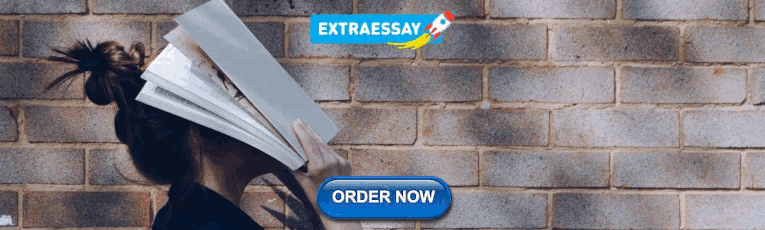
IMAGES
VIDEO
COMMENTS
The What Works Clearinghouse (WWC) single-case design technical documentation provides an excellent overview of appropriate SC study analysis techniques to evaluate the effectiveness of intervention effects. 1,18 First, visual analyses are recommended to determine whether there is a functional relation between the intervention and the outcome ...
A systematic review of applied single-case research published between 2016 and 2018: study designs, randomization, data aspects, and data analysis. Behav. Res. 53 , 1371-1384 (2021).
Single-case design research can be used to address a number of different types of research questions regarding treatment effectiveness that are likely to be of interest to researchers working in clinical settings. ... Single-case research design and analysis: new directions for psychology and education. Hillsdale: Lawrence Erlbaum Associates ...
This book is a compendium of tools and information for researchers considering single-case design (SCD) research, a newly viable and often essential methodology in applied psychology, education, and related fields. ... Visual Analysis of Single-Case Intervention Research: Conceptual and Methodological Issues Thomas R. Kratochwill, Joel R. Levin ...
Abstract. This chapter addresses the peculiarities, characteristics, and major fallacies of single case research designs. A single case study research design is a collective term for an in-depth analysis of a small non-random sample. The focus on this design is on in-depth.
When rigorously designed, single-case studies can be particularly useful experimental designs in a variety of situations, such as when research resources are limited, studied conditions have low incidences, or when examining effects of novel or expensive interventions. ... Single-Case Design, Analysis, and Quality Assessment for Intervention ...
David Rindskopf. We are pleased to introduce the first of two special issues dedicated to statistical and meta-analysis of single-case experimental designs (SCEDs). This first issue is focused on the analysis of data from SCEDs while the forthcoming second issue will document the state-of-the-art in SCED research synthesis.
The application of single-case research design (SCRD) offers counseling practitioners and researchers a practical and viable method for evaluating the effectiveness of interventions that target behavior, emotions, personal characteristics, and other counseling-related constructs of interest.
Increased use and dissemination of single-case intervention research has prompted efforts to clarify professional standards to guide the design, conduct, analysis, and reporting of research using single-case designs (SCDs; Ganz & Ayres, 2018; Kratochwill et al., 2013).The most recent proposal for SCD standards was released by the Institute of Education Sciences (IES) What Works Clearinghouse ...
Single-case research designs (SCRDs) are a vital tool for researchers and practitioners in counseling to evaluate intervention and treatment effectiveness. This content analysis reviews the application of SCRDs in counseling to highlight knowledge accrued and existing gaps in the literature.
Abstract. Single-case experimental designs (SCEDs) represent a family of research designs that use experimental methods to study the effects of treatments on outcomes. The fundamental unit of analysis is the single case—which can be an individual, clinic, or community—ideally with replications of effects within and/or between cases.
Abstract. Single-case-design researchers rarely used statistics in the past, but that is changing. In this article, I review the rapidly developing state of statistical analyses for single-case designs, including effect sizes, multilevel models, and Bayesian analyses. No analysis meets all the desiderata for an optimal single-case-design ...
This special issue of Perspective on Behavior Science is a productive contribution to current advances in the use and documentation of single-case research designs. We focus in this article on major themes emphasized by the articles in this issue and suggest directions for improving professional standards focused on the design, analysis, and dissemination of single-case research.
Studies that use a single-case design (SCD) measure outcomes for cases (such as a child or family) repeatedly during multiple phases of a study to determine the success of an intervention. The number of phases in the study will depend on the research questions, intervention, and outcome(s) of interest (see Types of SCDs on page 4 for examples).
General Readings on Single-Case Research Design and Analysis: Barlow DH, Nock MK, Hersen M. Single Case Experimental Designs: Strategies for Studying Behavior Change. 3rd ed. Needham Heights, MA: Allyn & Bacon; 2008. 3. Kazdin AE. Single-Case Research Designs: Methods for Clinical and Applied Settings. New York, NY: Oxford University Press; 2010. 4
This points to the important difference between what Yin refers to as an 'holistic' case design, with a single unit of analysis, and an 'embedded' case design with multiple units of analysis (Yin, 2009: 50-52). ... Absent these factors, MacMillan's single case study analysis (2008) suggests the particularistic importance of the ...
Multiple-case study design however has a distinct advantage over a single case study design. Multiple-case studies are generally considered more compelling and robust, and worthy of undertaking. This is because a multiple case study design has a greater chance of weeding out data collection errors and prejudices, and produces a more acceptable ...
Policies and ethics. This chapter addresses single-case research designs' peculiarities, characteristics, and significant fallacies. A single case research design is a collective term for an in-depth analysis of a small non-random sample. The focus of this design is in-depth.
Single-case experimental designs (SCEDs) have become a popular research methodology in educational science, psychology, and beyond. The growing popularity has been accompanied by the development of specific guidelines for the conduct and analysis of SCEDs. In this paper, we examine recent practices in the conduct and analysis of SCEDs by systematically reviewing applied SCEDs published over a ...
Single-case Experimental Designs in Clinical Settings. W.C. Follette, in International Encyclopedia of the Social & Behavioral Sciences, 2001 2 Characteristics of Single-case Design. Single-case designs study intensively the process of change by taking many measures on the same individual subject over a period of time. The degree of control in single-case design experiments can often lead to ...
In the realm of sustainable city development, evaluating the spatial vitality of urban green spaces (UGS) has become increasingly pivotal for assessing public space quality. This study delves into the spatial heterogeneity of park vitality across diverse urban landscapes at a city scale, addressing limitations inherent in conventional approaches to understanding the dynamics of park vitality ...
One approach to the issue of design sensitivity builds on the work on statistical analysis of data from single-case designs, namely statistical effect size measures (Hedges et al., 2012; Hedges et al., 2013).The focus of that work is not specifically the statistical analysis of single-case designs, but the representation of effects obtained via measures of effect size that are in the same ...