
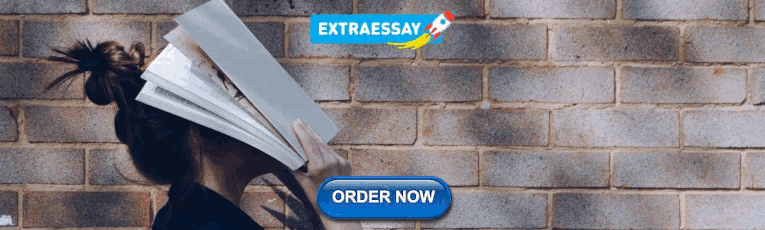
Community Blog
Keep up-to-date on postgraduate related issues with our quick reads written by students, postdocs, professors and industry leaders.
Types of Research – Explained with Examples

- By DiscoverPhDs
- October 2, 2020
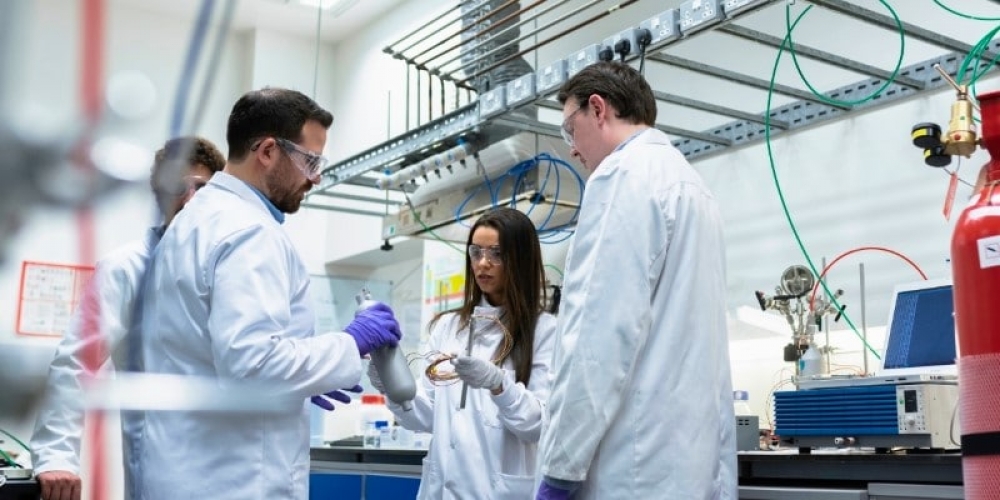
Types of Research
Research is about using established methods to investigate a problem or question in detail with the aim of generating new knowledge about it.
It is a vital tool for scientific advancement because it allows researchers to prove or refute hypotheses based on clearly defined parameters, environments and assumptions. Due to this, it enables us to confidently contribute to knowledge as it allows research to be verified and replicated.
Knowing the types of research and what each of them focuses on will allow you to better plan your project, utilises the most appropriate methodologies and techniques and better communicate your findings to other researchers and supervisors.
Classification of Types of Research
There are various types of research that are classified according to their objective, depth of study, analysed data, time required to study the phenomenon and other factors. It’s important to note that a research project will not be limited to one type of research, but will likely use several.
According to its Purpose
Theoretical research.
Theoretical research, also referred to as pure or basic research, focuses on generating knowledge , regardless of its practical application. Here, data collection is used to generate new general concepts for a better understanding of a particular field or to answer a theoretical research question.
Results of this kind are usually oriented towards the formulation of theories and are usually based on documentary analysis, the development of mathematical formulas and the reflection of high-level researchers.
Applied Research
Here, the goal is to find strategies that can be used to address a specific research problem. Applied research draws on theory to generate practical scientific knowledge, and its use is very common in STEM fields such as engineering, computer science and medicine.
This type of research is subdivided into two types:
- Technological applied research : looks towards improving efficiency in a particular productive sector through the improvement of processes or machinery related to said productive processes.
- Scientific applied research : has predictive purposes. Through this type of research design, we can measure certain variables to predict behaviours useful to the goods and services sector, such as consumption patterns and viability of commercial projects.
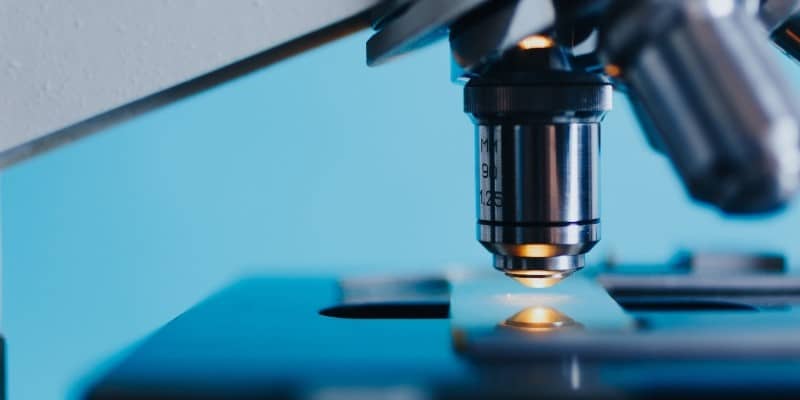
According to your Depth of Scope
Exploratory research.
Exploratory research is used for the preliminary investigation of a subject that is not yet well understood or sufficiently researched. It serves to establish a frame of reference and a hypothesis from which an in-depth study can be developed that will enable conclusive results to be generated.
Because exploratory research is based on the study of little-studied phenomena, it relies less on theory and more on the collection of data to identify patterns that explain these phenomena.
Descriptive Research
The primary objective of descriptive research is to define the characteristics of a particular phenomenon without necessarily investigating the causes that produce it.
In this type of research, the researcher must take particular care not to intervene in the observed object or phenomenon, as its behaviour may change if an external factor is involved.
Explanatory Research
Explanatory research is the most common type of research method and is responsible for establishing cause-and-effect relationships that allow generalisations to be extended to similar realities. It is closely related to descriptive research, although it provides additional information about the observed object and its interactions with the environment.
Correlational Research
The purpose of this type of scientific research is to identify the relationship between two or more variables. A correlational study aims to determine whether a variable changes, how much the other elements of the observed system change.
According to the Type of Data Used
Qualitative research.
Qualitative methods are often used in the social sciences to collect, compare and interpret information, has a linguistic-semiotic basis and is used in techniques such as discourse analysis, interviews, surveys, records and participant observations.
In order to use statistical methods to validate their results, the observations collected must be evaluated numerically. Qualitative research, however, tends to be subjective, since not all data can be fully controlled. Therefore, this type of research design is better suited to extracting meaning from an event or phenomenon (the ‘why’) than its cause (the ‘how’).
Quantitative Research
Quantitative research study delves into a phenomena through quantitative data collection and using mathematical, statistical and computer-aided tools to measure them . This allows generalised conclusions to be projected over time.

According to the Degree of Manipulation of Variables
Experimental research.
It is about designing or replicating a phenomenon whose variables are manipulated under strictly controlled conditions in order to identify or discover its effect on another independent variable or object. The phenomenon to be studied is measured through study and control groups, and according to the guidelines of the scientific method.
Non-Experimental Research
Also known as an observational study, it focuses on the analysis of a phenomenon in its natural context. As such, the researcher does not intervene directly, but limits their involvement to measuring the variables required for the study. Due to its observational nature, it is often used in descriptive research.
Quasi-Experimental Research
It controls only some variables of the phenomenon under investigation and is therefore not entirely experimental. In this case, the study and the focus group cannot be randomly selected, but are chosen from existing groups or populations . This is to ensure the collected data is relevant and that the knowledge, perspectives and opinions of the population can be incorporated into the study.
According to the Type of Inference
Deductive investigation.
In this type of research, reality is explained by general laws that point to certain conclusions; conclusions are expected to be part of the premise of the research problem and considered correct if the premise is valid and the inductive method is applied correctly.
Inductive Research
In this type of research, knowledge is generated from an observation to achieve a generalisation. It is based on the collection of specific data to develop new theories.
Hypothetical-Deductive Investigation
It is based on observing reality to make a hypothesis, then use deduction to obtain a conclusion and finally verify or reject it through experience.

According to the Time in Which it is Carried Out
Longitudinal study (also referred to as diachronic research).
It is the monitoring of the same event, individual or group over a defined period of time. It aims to track changes in a number of variables and see how they evolve over time. It is often used in medical, psychological and social areas .
Cross-Sectional Study (also referred to as Synchronous Research)
Cross-sectional research design is used to observe phenomena, an individual or a group of research subjects at a given time.
According to The Sources of Information
Primary research.
This fundamental research type is defined by the fact that the data is collected directly from the source, that is, it consists of primary, first-hand information.
Secondary research
Unlike primary research, secondary research is developed with information from secondary sources, which are generally based on scientific literature and other documents compiled by another researcher.
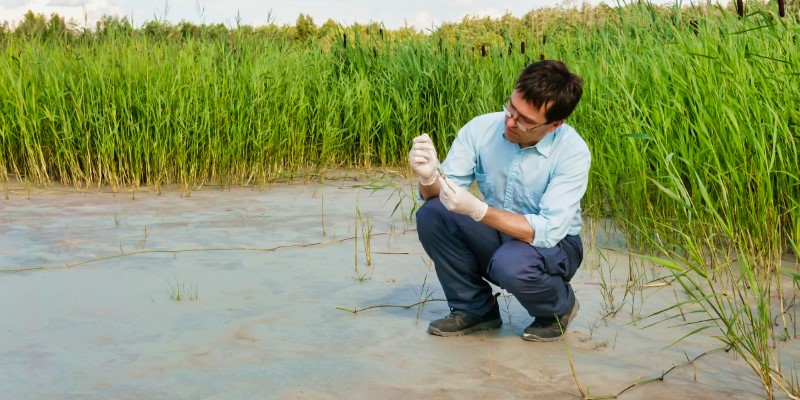
According to How the Data is Obtained
Documentary (cabinet).
Documentary research, or secondary sources, is based on a systematic review of existing sources of information on a particular subject. This type of scientific research is commonly used when undertaking literature reviews or producing a case study.
Field research study involves the direct collection of information at the location where the observed phenomenon occurs.
From Laboratory
Laboratory research is carried out in a controlled environment in order to isolate a dependent variable and establish its relationship with other variables through scientific methods.
Mixed-Method: Documentary, Field and/or Laboratory
Mixed research methodologies combine results from both secondary (documentary) sources and primary sources through field or laboratory research.
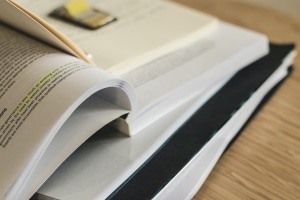
Find out the differences between a Literature Review and an Annotated Bibliography, whey they should be used and how to write them.
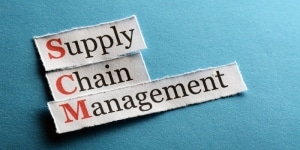
Need to write a list of abbreviations for a thesis or dissertation? Read our post to find out where they go, what to include and how to format them.
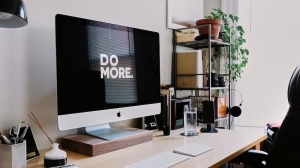
Learn more about using cloud storage effectively, video conferencing calling, good note-taking solutions and online calendar and task management options.
Join thousands of other students and stay up to date with the latest PhD programmes, funding opportunities and advice.
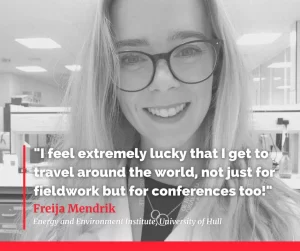
Browse PhDs Now
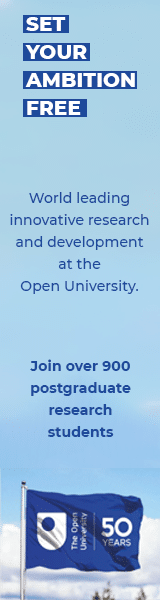
A concept paper is a short document written by a researcher before starting their research project, explaining what the study is about, why it is needed and the methods that will be used.

Stay up to date with current information being provided by the UK Government and Universities about the impact of the global pandemic on PhD research studies.

Emmanuel is a year and half into his PhD at The Open University School of Ecosystems, Earth and Environmental Studies. His research is on understand the influence of environmental and social factors on smallholder macadamia production.

Dr Thirlaway gained his PhD in immunology from the University of Nottingham in 2018. He is now a Science Communicator at the Natural History Museum, London.
Join Thousands of Students
- Skip to main content
- Skip to primary sidebar
- Skip to footer
- QuestionPro

- Solutions Industries Gaming Automotive Sports and events Education Government Travel & Hospitality Financial Services Healthcare Cannabis Technology Use Case NPS+ Communities Audience Contactless surveys Mobile LivePolls Member Experience GDPR Positive People Science 360 Feedback Surveys
- Resources Blog eBooks Survey Templates Case Studies Training Help center

Home Market Research
What is Research: Definition, Methods, Types & Examples
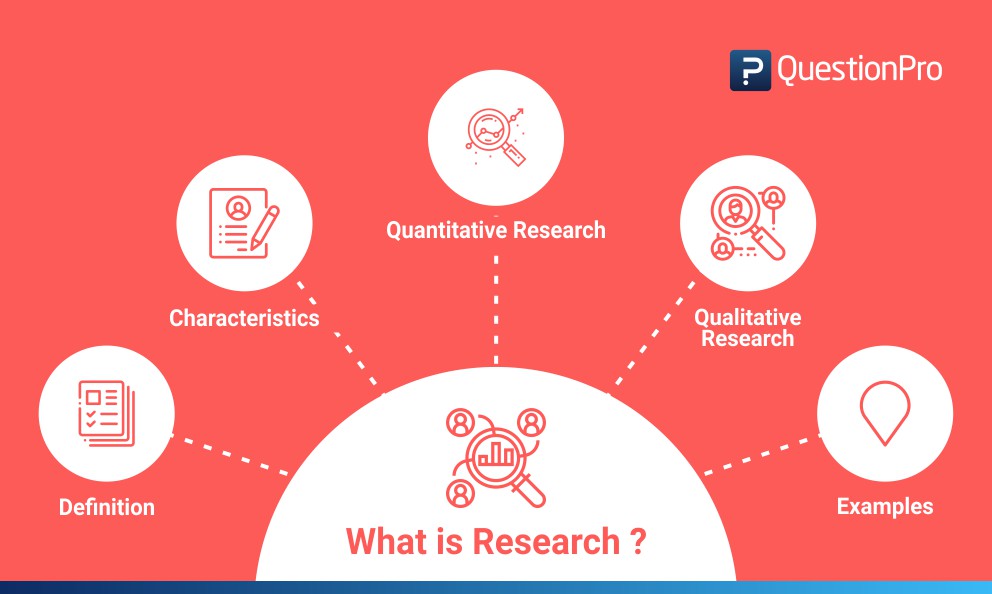
The search for knowledge is closely linked to the object of study; that is, to the reconstruction of the facts that will provide an explanation to an observed event and that at first sight can be considered as a problem. It is very human to seek answers and satisfy our curiosity. Let’s talk about research.
Content Index
What is Research?
What are the characteristics of research.
- Comparative analysis chart
Qualitative methods
Quantitative methods, 8 tips for conducting accurate research.
Research is the careful consideration of study regarding a particular concern or research problem using scientific methods. According to the American sociologist Earl Robert Babbie, “research is a systematic inquiry to describe, explain, predict, and control the observed phenomenon. It involves inductive and deductive methods.”
Inductive methods analyze an observed event, while deductive methods verify the observed event. Inductive approaches are associated with qualitative research , and deductive methods are more commonly associated with quantitative analysis .
Research is conducted with a purpose to:
- Identify potential and new customers
- Understand existing customers
- Set pragmatic goals
- Develop productive market strategies
- Address business challenges
- Put together a business expansion plan
- Identify new business opportunities
- Good research follows a systematic approach to capture accurate data. Researchers need to practice ethics and a code of conduct while making observations or drawing conclusions.
- The analysis is based on logical reasoning and involves both inductive and deductive methods.
- Real-time data and knowledge is derived from actual observations in natural settings.
- There is an in-depth analysis of all data collected so that there are no anomalies associated with it.
- It creates a path for generating new questions. Existing data helps create more research opportunities.
- It is analytical and uses all the available data so that there is no ambiguity in inference.
- Accuracy is one of the most critical aspects of research. The information must be accurate and correct. For example, laboratories provide a controlled environment to collect data. Accuracy is measured in the instruments used, the calibrations of instruments or tools, and the experiment’s final result.
What is the purpose of research?
There are three main purposes:
- Exploratory: As the name suggests, researchers conduct exploratory studies to explore a group of questions. The answers and analytics may not offer a conclusion to the perceived problem. It is undertaken to handle new problem areas that haven’t been explored before. This exploratory data analysis process lays the foundation for more conclusive data collection and analysis.
LEARN ABOUT: Descriptive Analysis
- Descriptive: It focuses on expanding knowledge on current issues through a process of data collection. Descriptive research describe the behavior of a sample population. Only one variable is required to conduct the study. The three primary purposes of descriptive studies are describing, explaining, and validating the findings. For example, a study conducted to know if top-level management leaders in the 21st century possess the moral right to receive a considerable sum of money from the company profit.
LEARN ABOUT: Best Data Collection Tools
- Explanatory: Causal research or explanatory research is conducted to understand the impact of specific changes in existing standard procedures. Running experiments is the most popular form. For example, a study that is conducted to understand the effect of rebranding on customer loyalty.
Here is a comparative analysis chart for a better understanding:
It begins by asking the right questions and choosing an appropriate method to investigate the problem. After collecting answers to your questions, you can analyze the findings or observations to draw reasonable conclusions.
When it comes to customers and market studies, the more thorough your questions, the better the analysis. You get essential insights into brand perception and product needs by thoroughly collecting customer data through surveys and questionnaires . You can use this data to make smart decisions about your marketing strategies to position your business effectively.
To make sense of your study and get insights faster, it helps to use a research repository as a single source of truth in your organization and manage your research data in one centralized data repository .
Types of research methods and Examples
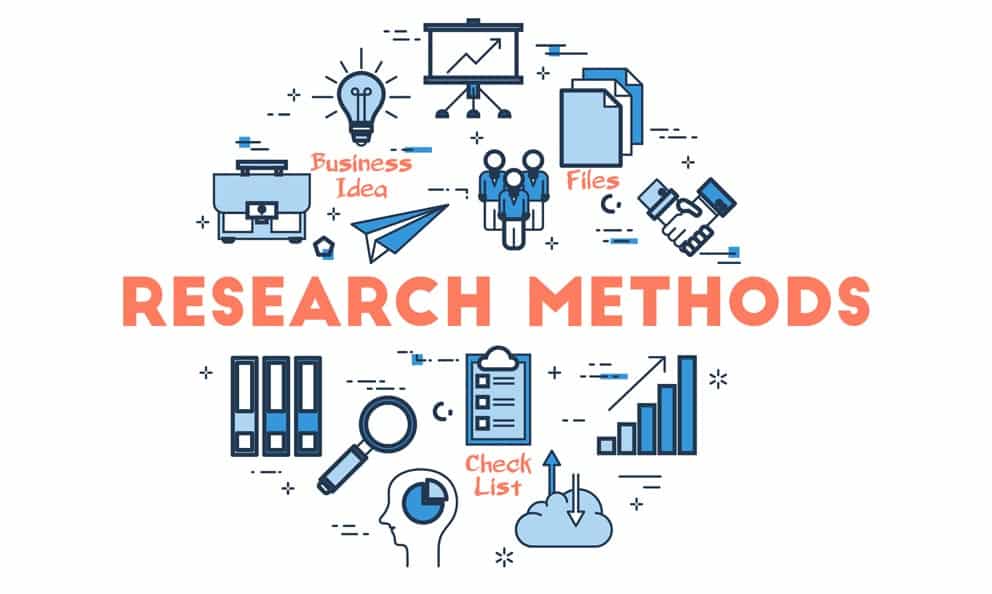
Research methods are broadly classified as Qualitative and Quantitative .
Both methods have distinctive properties and data collection methods.
Qualitative research is a method that collects data using conversational methods, usually open-ended questions . The responses collected are essentially non-numerical. This method helps a researcher understand what participants think and why they think in a particular way.
Types of qualitative methods include:
- One-to-one Interview
- Focus Groups
- Ethnographic studies
- Text Analysis
Quantitative methods deal with numbers and measurable forms . It uses a systematic way of investigating events or data. It answers questions to justify relationships with measurable variables to either explain, predict, or control a phenomenon.
Types of quantitative methods include:
- Survey research
- Descriptive research
- Correlational research
LEARN MORE: Descriptive Research vs Correlational Research
Remember, it is only valuable and useful when it is valid, accurate, and reliable. Incorrect results can lead to customer churn and a decrease in sales.
It is essential to ensure that your data is:
- Valid – founded, logical, rigorous, and impartial.
- Accurate – free of errors and including required details.
- Reliable – other people who investigate in the same way can produce similar results.
- Timely – current and collected within an appropriate time frame.
- Complete – includes all the data you need to support your business decisions.
Gather insights
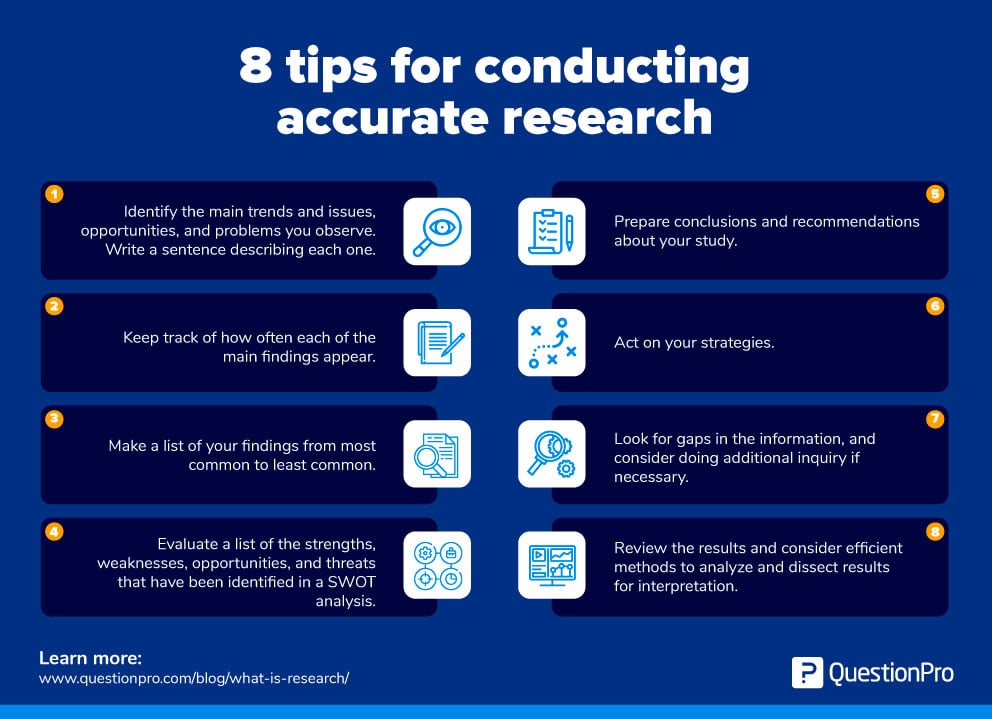
- Identify the main trends and issues, opportunities, and problems you observe. Write a sentence describing each one.
- Keep track of the frequency with which each of the main findings appears.
- Make a list of your findings from the most common to the least common.
- Evaluate a list of the strengths, weaknesses, opportunities, and threats identified in a SWOT analysis .
- Prepare conclusions and recommendations about your study.
- Act on your strategies
- Look for gaps in the information, and consider doing additional inquiry if necessary
- Plan to review the results and consider efficient methods to analyze and interpret results.
Review your goals before making any conclusions about your study. Remember how the process you have completed and the data you have gathered help answer your questions. Ask yourself if what your analysis revealed facilitates the identification of your conclusions and recommendations.
LEARN MORE ABOUT OUR SOFTWARE FREE TRIAL
MORE LIKE THIS
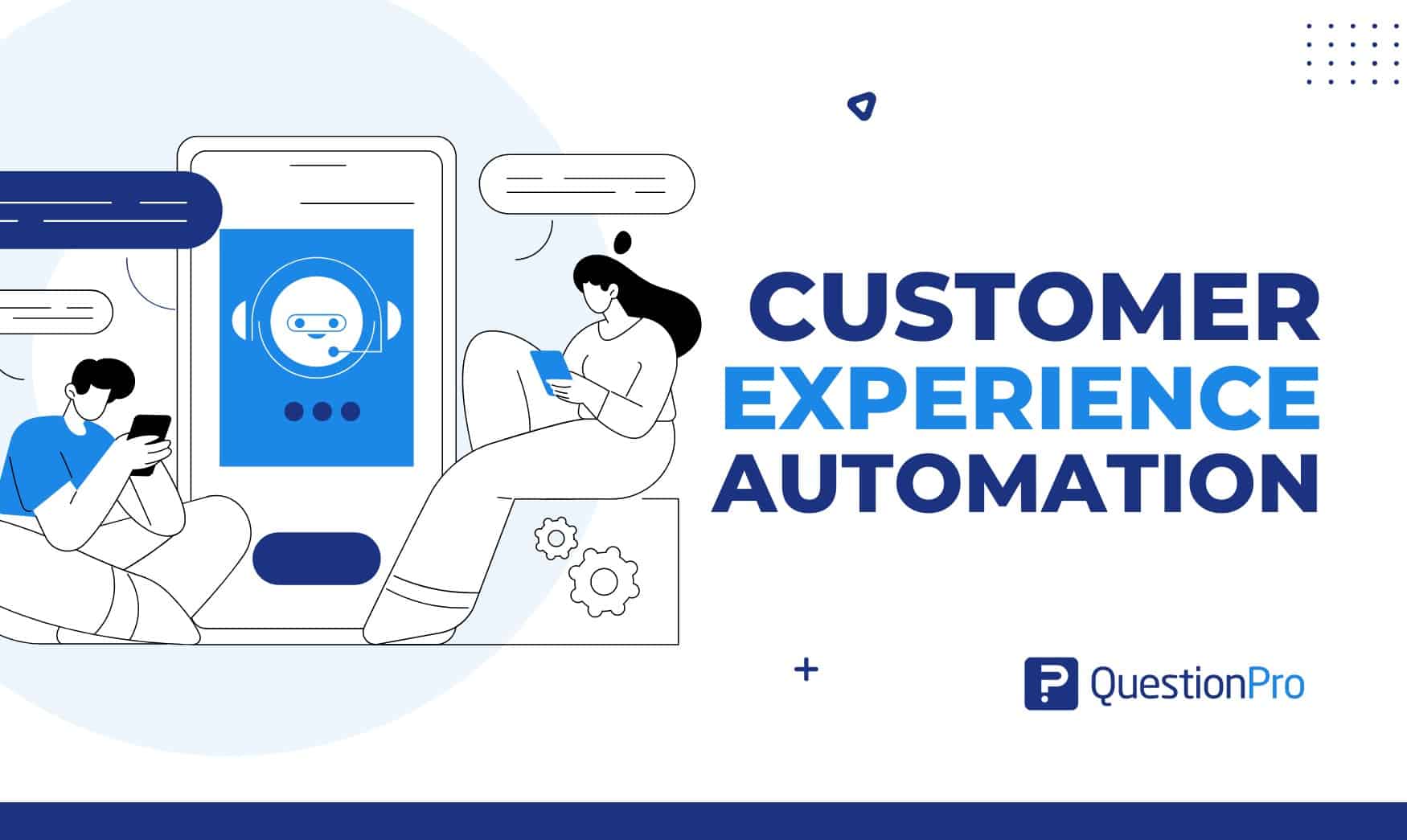
Customer Experience Automation: Benefits and Best Tools
Apr 1, 2024
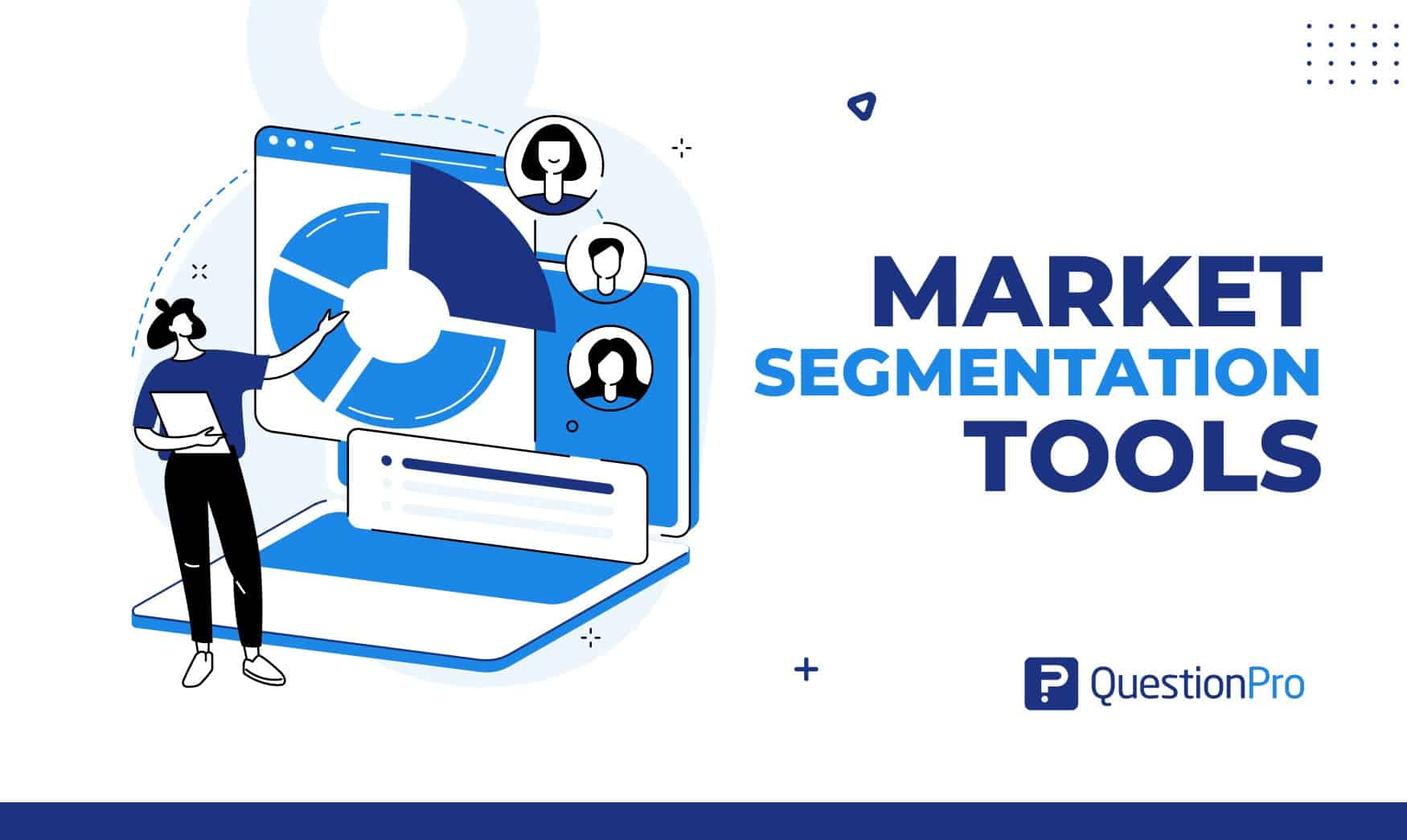
7 Best Market Segmentation Tools in 2024
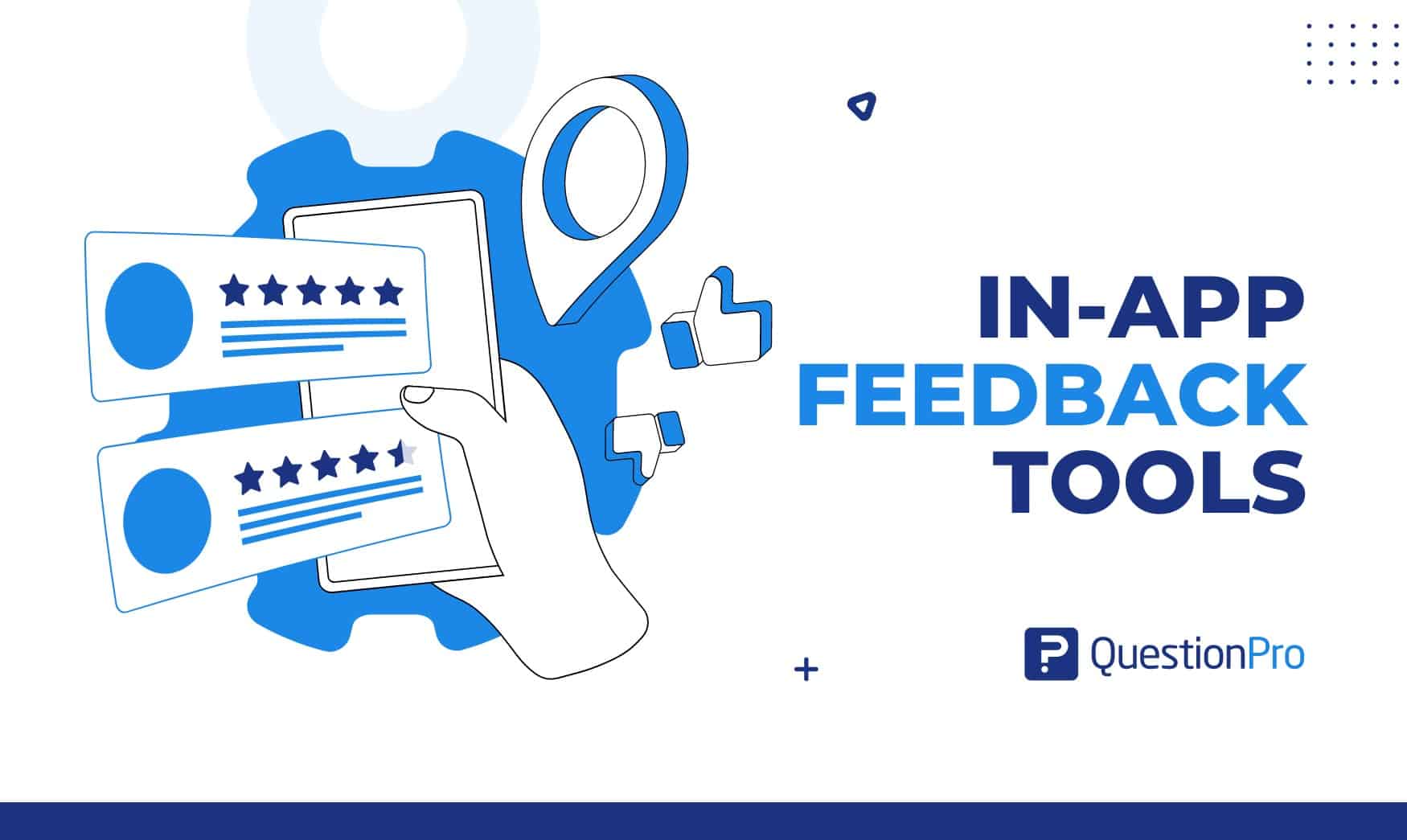
In-App Feedback Tools: How to Collect, Uses & 14 Best Tools
Mar 29, 2024
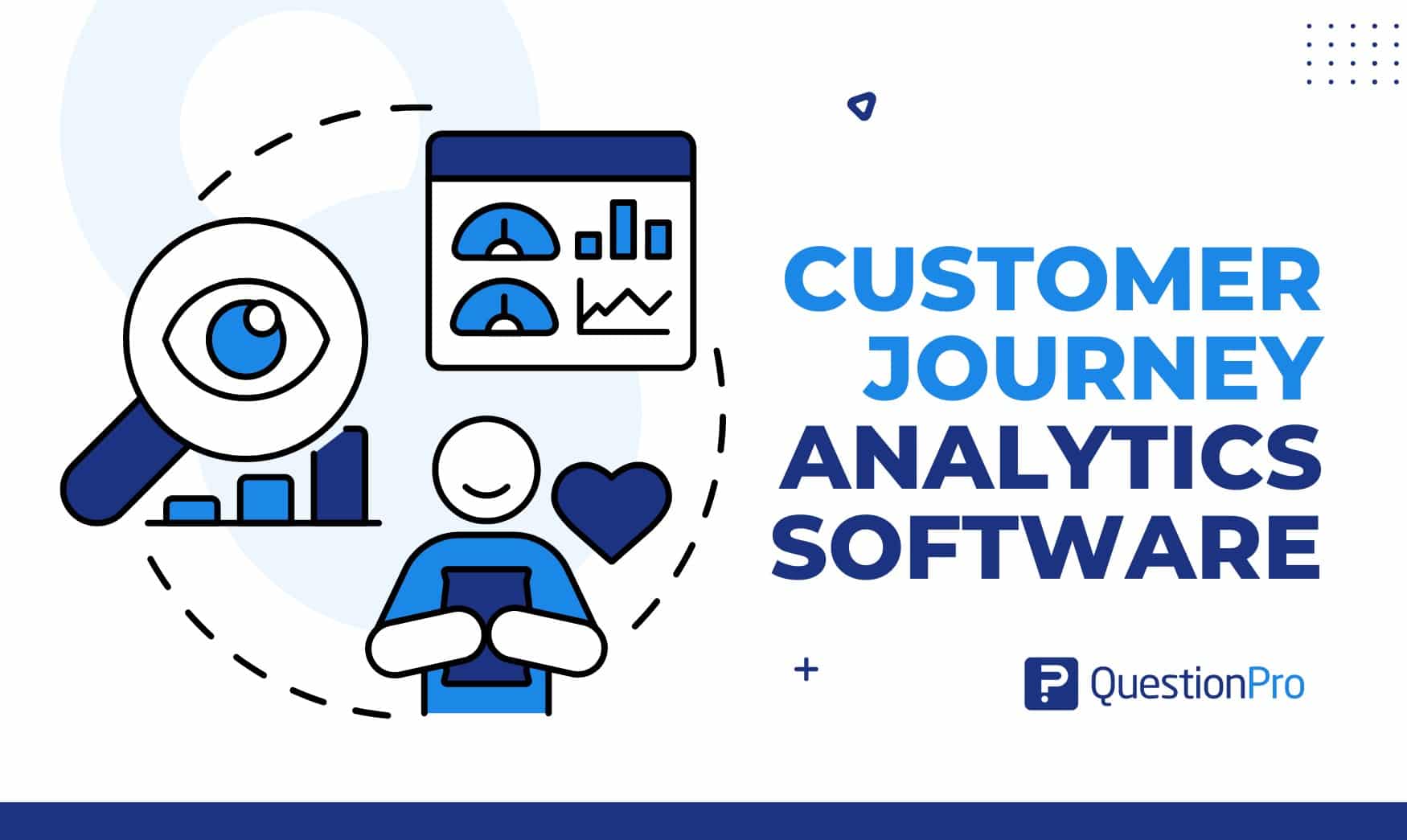
11 Best Customer Journey Analytics Software in 2024
Other categories.
- Academic Research
- Artificial Intelligence
- Assessments
- Brand Awareness
- Case Studies
- Communities
- Consumer Insights
- Customer effort score
- Customer Engagement
- Customer Experience
- Customer Loyalty
- Customer Research
- Customer Satisfaction
- Employee Benefits
- Employee Engagement
- Employee Retention
- Friday Five
- General Data Protection Regulation
- Insights Hub
- Life@QuestionPro
- Market Research
- Mobile diaries
- Mobile Surveys
- New Features
- Online Communities
- Question Types
- Questionnaire
- QuestionPro Products
- Release Notes
- Research Tools and Apps
- Revenue at Risk
- Survey Templates
- Training Tips
- Uncategorized
- Video Learning Series
- What’s Coming Up
- Workforce Intelligence
News alert: UC Berkeley has announced its next university librarian
Secondary menu
- Log in to your Library account
- Hours and Maps
- Connect from Off Campus
- UC Berkeley Home
Search form
Research methods--quantitative, qualitative, and more: overview.
- Quantitative Research
- Qualitative Research
- Data Science Methods (Machine Learning, AI, Big Data)
- Text Mining and Computational Text Analysis
- Evidence Synthesis/Systematic Reviews
- Get Data, Get Help!
About Research Methods
This guide provides an overview of research methods, how to choose and use them, and supports and resources at UC Berkeley.
As Patten and Newhart note in the book Understanding Research Methods , "Research methods are the building blocks of the scientific enterprise. They are the "how" for building systematic knowledge. The accumulation of knowledge through research is by its nature a collective endeavor. Each well-designed study provides evidence that may support, amend, refute, or deepen the understanding of existing knowledge...Decisions are important throughout the practice of research and are designed to help researchers collect evidence that includes the full spectrum of the phenomenon under study, to maintain logical rules, and to mitigate or account for possible sources of bias. In many ways, learning research methods is learning how to see and make these decisions."
The choice of methods varies by discipline, by the kind of phenomenon being studied and the data being used to study it, by the technology available, and more. This guide is an introduction, but if you don't see what you need here, always contact your subject librarian, and/or take a look to see if there's a library research guide that will answer your question.
Suggestions for changes and additions to this guide are welcome!
START HERE: SAGE Research Methods
Without question, the most comprehensive resource available from the library is SAGE Research Methods. HERE IS THE ONLINE GUIDE to this one-stop shopping collection, and some helpful links are below:
- SAGE Research Methods
- Little Green Books (Quantitative Methods)
- Little Blue Books (Qualitative Methods)
- Dictionaries and Encyclopedias
- Case studies of real research projects
- Sample datasets for hands-on practice
- Streaming video--see methods come to life
- Methodspace- -a community for researchers
- SAGE Research Methods Course Mapping
Library Data Services at UC Berkeley
Library Data Services Program and Digital Scholarship Services
The LDSP offers a variety of services and tools ! From this link, check out pages for each of the following topics: discovering data, managing data, collecting data, GIS data, text data mining, publishing data, digital scholarship, open science, and the Research Data Management Program.
Be sure also to check out the visual guide to where to seek assistance on campus with any research question you may have!
Library GIS Services
Other Data Services at Berkeley
D-Lab Supports Berkeley faculty, staff, and graduate students with research in data intensive social science, including a wide range of training and workshop offerings Dryad Dryad is a simple self-service tool for researchers to use in publishing their datasets. It provides tools for the effective publication of and access to research data. Geospatial Innovation Facility (GIF) Provides leadership and training across a broad array of integrated mapping technologies on campu Research Data Management A UC Berkeley guide and consulting service for research data management issues
General Research Methods Resources
Here are some general resources for assistance:
- Assistance from ICPSR (must create an account to access): Getting Help with Data , and Resources for Students
- Wiley Stats Ref for background information on statistics topics
- Survey Documentation and Analysis (SDA) . Program for easy web-based analysis of survey data.
Consultants
- D-Lab/Data Science Discovery Consultants Request help with your research project from peer consultants.
- Research data (RDM) consulting Meet with RDM consultants before designing the data security, storage, and sharing aspects of your qualitative project.
- Statistics Department Consulting Services A service in which advanced graduate students, under faculty supervision, are available to consult during specified hours in the Fall and Spring semesters.
Related Resourcex
- IRB / CPHS Qualitative research projects with human subjects often require that you go through an ethics review.
- OURS (Office of Undergraduate Research and Scholarships) OURS supports undergraduates who want to embark on research projects and assistantships. In particular, check out their "Getting Started in Research" workshops
- Sponsored Projects Sponsored projects works with researchers applying for major external grants.
- Next: Quantitative Research >>
- Last Updated: Apr 3, 2023 3:14 PM
- URL: https://guides.lib.berkeley.edu/researchmethods
Have a language expert improve your writing
Run a free plagiarism check in 10 minutes, automatically generate references for free.
- Knowledge Base
- Methodology
Research Methods | Definition, Types, Examples
Research methods are specific procedures for collecting and analysing data. Developing your research methods is an integral part of your research design . When planning your methods, there are two key decisions you will make.
First, decide how you will collect data . Your methods depend on what type of data you need to answer your research question :
- Qualitative vs quantitative : Will your data take the form of words or numbers?
- Primary vs secondary : Will you collect original data yourself, or will you use data that have already been collected by someone else?
- Descriptive vs experimental : Will you take measurements of something as it is, or will you perform an experiment?
Second, decide how you will analyse the data .
- For quantitative data, you can use statistical analysis methods to test relationships between variables.
- For qualitative data, you can use methods such as thematic analysis to interpret patterns and meanings in the data.
Table of contents
Methods for collecting data, examples of data collection methods, methods for analysing data, examples of data analysis methods, frequently asked questions about methodology.
Data are the information that you collect for the purposes of answering your research question . The type of data you need depends on the aims of your research.
Qualitative vs quantitative data
Your choice of qualitative or quantitative data collection depends on the type of knowledge you want to develop.
For questions about ideas, experiences and meanings, or to study something that can’t be described numerically, collect qualitative data .
If you want to develop a more mechanistic understanding of a topic, or your research involves hypothesis testing , collect quantitative data .
You can also take a mixed methods approach, where you use both qualitative and quantitative research methods.
Primary vs secondary data
Primary data are any original information that you collect for the purposes of answering your research question (e.g. through surveys , observations and experiments ). Secondary data are information that has already been collected by other researchers (e.g. in a government census or previous scientific studies).
If you are exploring a novel research question, you’ll probably need to collect primary data. But if you want to synthesise existing knowledge, analyse historical trends, or identify patterns on a large scale, secondary data might be a better choice.
Descriptive vs experimental data
In descriptive research , you collect data about your study subject without intervening. The validity of your research will depend on your sampling method .
In experimental research , you systematically intervene in a process and measure the outcome. The validity of your research will depend on your experimental design .
To conduct an experiment, you need to be able to vary your independent variable , precisely measure your dependent variable, and control for confounding variables . If it’s practically and ethically possible, this method is the best choice for answering questions about cause and effect.
Prevent plagiarism, run a free check.
Your data analysis methods will depend on the type of data you collect and how you prepare them for analysis.
Data can often be analysed both quantitatively and qualitatively. For example, survey responses could be analysed qualitatively by studying the meanings of responses or quantitatively by studying the frequencies of responses.
Qualitative analysis methods
Qualitative analysis is used to understand words, ideas, and experiences. You can use it to interpret data that were collected:
- From open-ended survey and interview questions, literature reviews, case studies, and other sources that use text rather than numbers.
- Using non-probability sampling methods .
Qualitative analysis tends to be quite flexible and relies on the researcher’s judgement, so you have to reflect carefully on your choices and assumptions.
Quantitative analysis methods
Quantitative analysis uses numbers and statistics to understand frequencies, averages and correlations (in descriptive studies) or cause-and-effect relationships (in experiments).
You can use quantitative analysis to interpret data that were collected either:
- During an experiment.
- Using probability sampling methods .
Because the data are collected and analysed in a statistically valid way, the results of quantitative analysis can be easily standardised and shared among researchers.
Quantitative research deals with numbers and statistics, while qualitative research deals with words and meanings.
Quantitative methods allow you to test a hypothesis by systematically collecting and analysing data, while qualitative methods allow you to explore ideas and experiences in depth.
In mixed methods research , you use both qualitative and quantitative data collection and analysis methods to answer your research question .
A sample is a subset of individuals from a larger population. Sampling means selecting the group that you will actually collect data from in your research.
For example, if you are researching the opinions of students in your university, you could survey a sample of 100 students.
Statistical sampling allows you to test a hypothesis about the characteristics of a population. There are various sampling methods you can use to ensure that your sample is representative of the population as a whole.
The research methods you use depend on the type of data you need to answer your research question .
- If you want to measure something or test a hypothesis , use quantitative methods . If you want to explore ideas, thoughts, and meanings, use qualitative methods .
- If you want to analyse a large amount of readily available data, use secondary data. If you want data specific to your purposes with control over how they are generated, collect primary data.
- If you want to establish cause-and-effect relationships between variables , use experimental methods. If you want to understand the characteristics of a research subject, use descriptive methods.
Methodology refers to the overarching strategy and rationale of your research project . It involves studying the methods used in your field and the theories or principles behind them, in order to develop an approach that matches your objectives.
Methods are the specific tools and procedures you use to collect and analyse data (e.g. experiments, surveys , and statistical tests ).
In shorter scientific papers, where the aim is to report the findings of a specific study, you might simply describe what you did in a methods section .
In a longer or more complex research project, such as a thesis or dissertation , you will probably include a methodology section , where you explain your approach to answering the research questions and cite relevant sources to support your choice of methods.
Is this article helpful?
More interesting articles.
- A Quick Guide to Experimental Design | 5 Steps & Examples
- Between-Subjects Design | Examples, Pros & Cons
- Case Study | Definition, Examples & Methods
- Cluster Sampling | A Simple Step-by-Step Guide with Examples
- Confounding Variables | Definition, Examples & Controls
- Construct Validity | Definition, Types, & Examples
- Content Analysis | A Step-by-Step Guide with Examples
- Control Groups and Treatment Groups | Uses & Examples
- Controlled Experiments | Methods & Examples of Control
- Correlation vs Causation | Differences, Designs & Examples
- Correlational Research | Guide, Design & Examples
- Critical Discourse Analysis | Definition, Guide & Examples
- Cross-Sectional Study | Definitions, Uses & Examples
- Data Cleaning | A Guide with Examples & Steps
- Data Collection Methods | Step-by-Step Guide & Examples
- Descriptive Research Design | Definition, Methods & Examples
- Doing Survey Research | A Step-by-Step Guide & Examples
- Ethical Considerations in Research | Types & Examples
- Explanatory Research | Definition, Guide, & Examples
- Explanatory vs Response Variables | Definitions & Examples
- Exploratory Research | Definition, Guide, & Examples
- External Validity | Types, Threats & Examples
- Extraneous Variables | Examples, Types, Controls
- Face Validity | Guide with Definition & Examples
- How to Do Thematic Analysis | Guide & Examples
- How to Write a Strong Hypothesis | Guide & Examples
- Inclusion and Exclusion Criteria | Examples & Definition
- Independent vs Dependent Variables | Definition & Examples
- Inductive Reasoning | Types, Examples, Explanation
- Inductive vs Deductive Research Approach (with Examples)
- Internal Validity | Definition, Threats & Examples
- Internal vs External Validity | Understanding Differences & Examples
- Longitudinal Study | Definition, Approaches & Examples
- Mediator vs Moderator Variables | Differences & Examples
- Mixed Methods Research | Definition, Guide, & Examples
- Multistage Sampling | An Introductory Guide with Examples
- Naturalistic Observation | Definition, Guide & Examples
- Operationalisation | A Guide with Examples, Pros & Cons
- Population vs Sample | Definitions, Differences & Examples
- Primary Research | Definition, Types, & Examples
- Qualitative vs Quantitative Research | Examples & Methods
- Quasi-Experimental Design | Definition, Types & Examples
- Questionnaire Design | Methods, Question Types & Examples
- Random Assignment in Experiments | Introduction & Examples
- Reliability vs Validity in Research | Differences, Types & Examples
- Reproducibility vs Replicability | Difference & Examples
- Research Design | Step-by-Step Guide with Examples
- Sampling Methods | Types, Techniques, & Examples
- Semi-Structured Interview | Definition, Guide & Examples
- Simple Random Sampling | Definition, Steps & Examples
- Stratified Sampling | A Step-by-Step Guide with Examples
- Structured Interview | Definition, Guide & Examples
- Systematic Review | Definition, Examples & Guide
- Systematic Sampling | A Step-by-Step Guide with Examples
- Textual Analysis | Guide, 3 Approaches & Examples
- The 4 Types of Reliability in Research | Definitions & Examples
- The 4 Types of Validity | Types, Definitions & Examples
- Transcribing an Interview | 5 Steps & Transcription Software
- Triangulation in Research | Guide, Types, Examples
- Types of Interviews in Research | Guide & Examples
- Types of Research Designs Compared | Examples
- Types of Variables in Research | Definitions & Examples
- Unstructured Interview | Definition, Guide & Examples
- What Are Control Variables | Definition & Examples
- What Is a Case-Control Study? | Definition & Examples
- What Is a Cohort Study? | Definition & Examples
- What Is a Conceptual Framework? | Tips & Examples
- What Is a Double-Barrelled Question?
- What Is a Double-Blind Study? | Introduction & Examples
- What Is a Focus Group? | Step-by-Step Guide & Examples
- What Is a Likert Scale? | Guide & Examples
- What is a Literature Review? | Guide, Template, & Examples
- What Is a Prospective Cohort Study? | Definition & Examples
- What Is a Retrospective Cohort Study? | Definition & Examples
- What Is Action Research? | Definition & Examples
- What Is an Observational Study? | Guide & Examples
- What Is Concurrent Validity? | Definition & Examples
- What Is Content Validity? | Definition & Examples
- What Is Convenience Sampling? | Definition & Examples
- What Is Convergent Validity? | Definition & Examples
- What Is Criterion Validity? | Definition & Examples
- What Is Deductive Reasoning? | Explanation & Examples
- What Is Discriminant Validity? | Definition & Example
- What Is Ecological Validity? | Definition & Examples
- What Is Ethnography? | Meaning, Guide & Examples
- What Is Non-Probability Sampling? | Types & Examples
- What Is Participant Observation? | Definition & Examples
- What Is Peer Review? | Types & Examples
- What Is Predictive Validity? | Examples & Definition
- What Is Probability Sampling? | Types & Examples
- What Is Purposive Sampling? | Definition & Examples
- What Is Qualitative Observation? | Definition & Examples
- What Is Qualitative Research? | Methods & Examples
- What Is Quantitative Observation? | Definition & Examples
- What Is Quantitative Research? | Definition & Methods
- What Is Quota Sampling? | Definition & Examples
- What is Secondary Research? | Definition, Types, & Examples
- What Is Snowball Sampling? | Definition & Examples
- Within-Subjects Design | Explanation, Approaches, Examples
Our websites may use cookies to personalize and enhance your experience. By continuing without changing your cookie settings, you agree to this collection. For more information, please see our University Websites Privacy Notice .
Neag School of Education
Educational Research Basics by Del Siegle
Types of Research
How do we know something exists? There are a numbers of ways of knowing…
- -Sensory Experience
- -Agreement with others
- -Expert Opinion
- -Scientific Method (we’re using this one)
The Scientific Process (replicable)
- Identify a problem
- Clarify the problem
- Determine what data would help solve the problem
- Organize the data
- Interpret the results
General Types of Educational Research
- Descriptive — survey, historical, content analysis, qualitative (ethnographic, narrative, phenomenological, grounded theory, and case study)
- Associational — correlational, causal-comparative
- Intervention — experimental, quasi-experimental, action research (sort of)
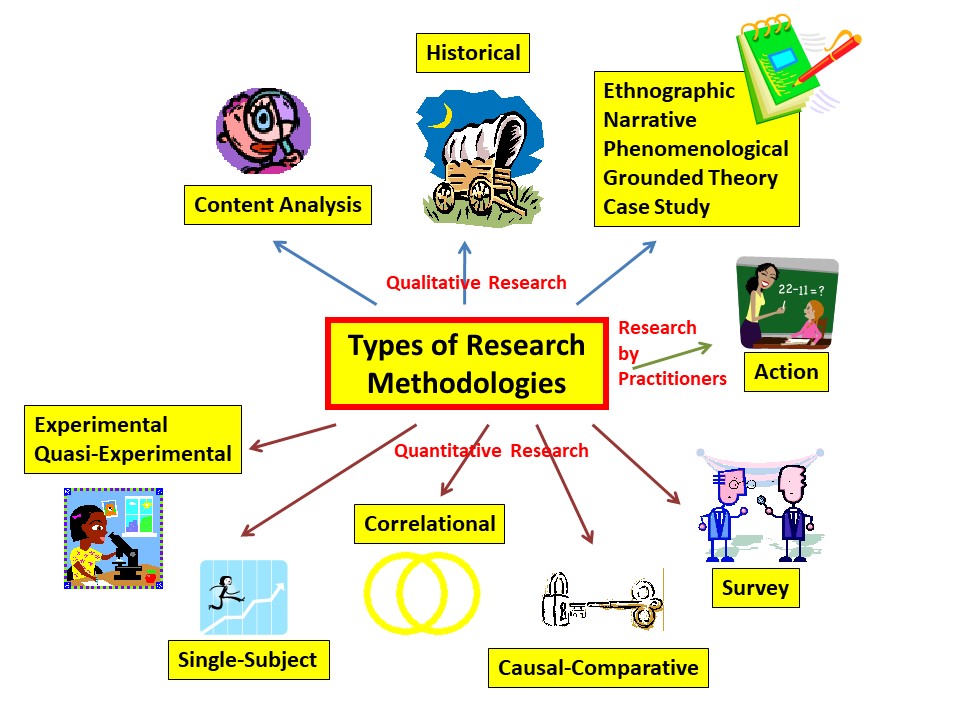
Researchers Sometimes Have a Category Called Group Comparison
- Ex Post Facto (Causal-Comparative): GROUPS ARE ALREADY FORMED
- Experimental: RANDOM ASSIGNMENT OF INDIVIDUALS
- Quasi-Experimental: RANDOM ASSIGNMENT OF GROUPS (oversimplified, but fine for now)
General Format of a Research Publication
- Background of the Problem (ending with a problem statement) — Why is this important to study? What is the problem being investigated?
- Review of Literature — What do we already know about this problem or situation?
- Methodology (participants, instruments, procedures) — How was the study conducted? Who were the participants? What data were collected and how?
- Analysis — What are the results? What did the data indicate?
- Results — What are the implications of these results? How do they agree or disagree with previous research? What do we still need to learn? What are the limitations of this study?
Del Siegle, PhD [email protected]
Last modified 6/18/2019
Introduction to Research Methods
3 types of research.
In the last chapter we talked about the ways that research is all around us. You do it yourself almost every day in small and big ways, but we’re not really here to help you become more rigorous in your search for the best tacos in town. Looking at yelp is research, it’s just not really the type of research we’re going to talk about today. In the first section of this chapter we’ll talk about different types of research. Then we’ll describe different fields of research within social sciences, and finally we’ll discuss the steps of doing research.
I’m going to break types of research into three categories, which probably don’t match the way they’re described in other textbooks.
A lot of the research you do in your daily life could probably be called secondary research . You have a question (“where are the best tacos?”, “when did the Civil War start?”, “is coffee bad for my heart?”) and so you seek an answer. That’s still research, it just doesn’t involve the collection of new data or a lot of detailed steps. Google and other search engines are incredible tools that will direct you towards an answer to your questions. What you’re doing there is secondary research, using the research of others to answer your question. Your collecting, reviewing, or synthesizing existing research, not creating new data to answer a question.
You can be better at secondary research by identifying reputable sources, accessing multiple opinions, and understanding how they produced their findings. That’s part of the research we’re talking about in this class, but only a small part. We’ll return to secondary research in a later chapter, because in order to be really good at it you have to understand how to do the original research yourself. Secondary research thus involves reviewing the research of others and is motivated by getting an answer to a question.
You can only do secondary research if someone has already researched the question you have. Another type of research people do is what could be called applied research, or research that is intended for immediate public dissemination. The idea of applied research is that there is a very clear connection between the research question and the importance of the research. Imagine you’re in a sorority and you’re planning dinner for the new pledges, so you poll everyone to ask which of three options they’d pick. You gather the data and you get an answer – the most common answer is tacos. Why do you care about the answer? Because you needed to know where to go for dinner.
Would people be open to changing the colors on the United States flag? I don’t know, and based on a quick Google search no one has answered the question. No one has polled Americans to find out whether they think red white and blue is a little dated (or maybe just too similar to France). I don’t know why we’d want to change the colors, maybe so everyone has to go buy a new flag. I can’t get an answer to that question based on secondary research though. I have to collect original data if I want an answer.
Polls are a great example of applied research. Who is currently winning the race for president? How do people feel about policies designed to slow climate change? How much trust do citizens have in their government? Those are all questions you can find written about in wonky news sources like the [NY Times], Vox, 538, or others. Why do we care? Because we want to know who is winning the race, or peoples views towards certain policies.
Take another example. A radio station wants to know the demographics of its listeners so that it can make sure the commercials they run are matched to who listens. There isn’t an esoteric question to answer, but they need to collect data to improve their business operations.
The research question and the importance are very directly linked. Thus, applied research involves original research, not just reviewing what others have done, but like secondary research it is motivated to get an answer.
The third type is the least common, but is also generally the focus of a textbook like this. Academic research is the type of research that your professors do most of the time. What differentiates public research from academic research? Public research is concerned with providing new facts, academic research is concerned with testing theories and seeking explanations.
I could spend thousands of dollars to run a new poll with a very rigorous research design to understand exactly what percentage of Americans would support new taxes. If I did that research I might be able to get it published in popular sources like the New York Times, but I could never get it published in an academic journal – and those are the papers that get professors tenure.
Why? Good polls tell us something about the world at this moment, but sciences goal, both the social and hard sciences, is to tell us something about the world beyond this moment. More accurately, it’s concerned with explaining the causes of the phenomena we see. Scientists weren’t just concerned with tracking that rocks fell from buildings, but wanted to identify the force that explained why that occurred (gravity). Similarly, social scientists aren’t just concerned with knowing what percentage of people are in poverty (although that is important) they want to identify the cause of poverty so that those conditions can be changed.
My poll might find that 46% of Americans plan to vote. What academic research is concerned with is the ‘why?’ Why did 46% say that, why did one person say yes and the other no, what does that help us to understand about the society? What we want to understand is the causes of the phenomena we see every day so that we can better understand the world of tomorrow.
Let’s say we did a study and found that 32% of elementary age children are significantly overweight. That’s good to know, it gives everyone an idea of the status of the health of children at this moment. What would be more important to know is why. If we know why 32% of children are significantly overweight and the other 68% aren’t, we can make changes that affect the future. Is it a lack of recess in schools, do children not have enough access to fruits and vegetable, are the foods they’re eating changing – understanding why is just as important as knowing the what, so that we know where to make changes.
Change is the only constant. I do most of my research on urban policy, which sort of means I study cities and the changes they undergo. If I looked at data on all of the neighborhoods in a city a decade ago and look again a decade later some would have gotten richer and others would have gotten poorer.

Change in Income in Nashville 2000 to 2018
That map might look interesting, and it might be important for people living in those communities to know. But unless we can provide an explanation for the change, we haven’t really learned anything. Neighborhoods change. People change. Demographics change. Everything changes. Are the neighborhoods shaded darker going to keep getting richer, or will there be a return to the mean and they’ll get poorer in the next decade? Did something happen that changed the fate of those neighborhoods, or was it just random decisions by a lot of different actors that lead to a new geography of the city? Those questions might seem unnecessary. Some neighborhoods are getting poorer, they need more support! Who cares about the why!? But if we’re going to try and figure out what neighborhoods will get poorer in the future, or want to change the future, we have to understand the underlying causes of those changes. That’s what academic research is trying to untangle. Not just what’s going on, but why, so that we can try to get more control of the future. Thus, academic research involves original research, like applied research, but is focused on developing theories as much as it is getting an answer to a question.
3.2 What’s theory got to do with it?
Let’s first define theory , because the way it’s used in science and the way it’s used in everyday conversation are slightly different. In everyday conversation you might hear the word theory used as the equivalent of “hunch” or “idea”: “oh, that’s just your theory”. In the sciences it means a bit more. A theory in the sciences is a well-substantiated explanation for a set of observations. A law is accepted as true by scientists, it is confirmed fact. A theory is on its way to becoming a law, it just needs more observations to be fully accepted.
The social sciences have plenty of theories, and fewer explanations that can be accepted as laws because as we discussed in the previous chapter humans just make it hard to get consistent findings.
For instance, researchers in in political science and public administration often use:
- elite theory which posits that a small minority of elites be they the wealthy or those that drive the creation of policy, holds most of the power in society even within democratic systems.
- democratic peace theory argues that democracies generally do not go to war or have armed conflicts with other democracies
- representative bureaucracy argues that governing bodies throughout society should be representative of the community they serve or govern.
Those theories and others get applied in different studies to continue testing them and refining them. For instance, I might want to study whether counties with growing Latinx/Latino/Latina populations see changes in who is elected for county wide offices. If I just do that research and report the results it could just be applied research. The public has an interest in knowing who their elected representatives are. If I use the research to test representative bureaucracy as a way of explaining my results, my research is now venturing into academic territory. The theoretical argument is all about explaining whatever I find, whether representation changes in those counties or not.
3.3 Inputs to research
The most difficult thing to accept and internalize in developing a research project is that it is iterative, not linear. We like linear processes like following a recipe. You can follow those steps and you get the end and then you have cookies. Following the research recipe isn’t that clean. It will be a lot of one step forward two steps back, which is progress, but can be frustrating.
Research values novelty. One should not spend a lot of time gathering original data in order to answer a question that has already been answered. So in order to develop a research question worth researching, it is really important to understand what has already been studied on that topic. I’ve learned this from experience, both in my own research and teaching, but you really can’t develop a good research question without doing a lot of reading.
If you’re starting to develop a research project, start with the things you care about. You’re going to spend a lot of time studying it and reading about the subject – it should be something you enjoy. Think about the things you observe in the world, the odd processes or changes you see around you. And think about the things you know a lot about. Whatever your interests are, whether they’re video games or hiking or reading, try to embed that interest in the research.
But again, you have to do a lot of reading. If I was walking down the street and someone stopped me and forced me to come up with a research question in sociology I would surely stumble and I might eventually stammer something out like “why do people leave online communities?” I don’t know much about that, it sounds kind of interesting. And online communities are a somewhat recent development (in comparison to say churches) so maybe the research will be interesting. Almost certainly not. I can guarantee a lot of research has been done on that question. That doesn’t mean I have to abandon the idea, it just means I need to start by reading all the research that has been done, and continue to refine my question. As I read thorough the literature on online group membership I’ll probably find answers to questions that never occurred to me, and as I read I might find questions that haven’t been answered yet.
A similar pattern occurred as I began my PhD. I wanted to study big important questions, and when I got to my program I was given a lot of freedom to decide what I would do research on. I decided I’d start by answering a question I constantly heard debated by policymakers: do sports stadiums create economic activity. I was going to be the researcher that answered the question. But pretty immediately I discovered that, actually, about 100 other researchers had already answered that question (it’s a definitive no, stadiums don’t create any economic activity, they’re a really bad deal for cities). The fact that the public didn’t regard it as a settled question doesn’t mean that researchers hadn’t already answered it. Did I give up? No, I kept reading the literature and I started to uncover related questions that hadn’t been answered yet. I ended up doing my dissertation on minor league baseball stadiums and their impact on the neighborhoods where they are located, which wasn’t the most important question ever but it hadn’t been answered before.
One recommendation I would make as you start reading the literature in a given area is to keep an annotated bibliography . As you read new articles to down a few sentences summarizing them - those few sentences can often be gleaned just from the abstract of the article. That way you’ll have a record of what you read, and as the project changes you can go back and wont have to search through the literature over and over. As your project begins to gain focus, you can pull the relevant articles from your annotated bibliography and begin to build out your paper. I would also recommend using a computer program like Zotero where you can save the details of articles and generate the bibliography of papers later. I don’t know the difference between MLA or APA or any system, because I make the computer do it for me. In the video below I walk through these things with a brief demonstration for anyone starting out a new research project.
One problem you will face in reading about your topic is accessing the articles that are relevant to your topic. If you go to a journals webpage, you’ll see that you can buy the article for probably $30. $30! That’s as much as a book, and you probably won’t even be sure if the article is good before buying it. There have been a lot of arguments made against these paywalls particularly given that taxpayers fund most of the research that is then sent to these journals. Researchers aren’t paid directly for the researcher they publish, which we give the copyright over to journals because it helps us to get tenure; journals then charge for people to read the research, and universities pay subscription fees so their researchers have access. It’s a circular economy, with me working for free, and journals making out like bandits. When you find an article you want to read see if you can access it through your library, but you can also check a few different websites where people post articles in order to “free” them. You can also just reach out to the author of the article and request a copy. Authors generally have the right to share the article even though they’ve given the journal the copyright over its contents, and most researchers are just happy to see someone engage with their work.
Once you’ve got a research question that’s worth studying and hasn’t been answered before, it’s time to answer it yourself. That’ll mean collecting data though, to answer that question. I should probably start by trying to figure out if data already exists that was collected by someone else first. You can do a lot of research in political science based on surveys that are posted online by Gallup or Pew. It’ll be easier to do the research if I can find the data from the start. If I can’t find the data, I’ll be faced with the choice of changing my research question to match the data that’s available or collecting my own data. Collecting your own data can be expensive and difficult, but if you’re interested in breaking new ground in your research it might be necessary.
The two steps are thus iterative. Knowing the literature on a topic will help you to develop new questions and lead you towards data necessary to answer them. But looking at data may help you to generate new questions and lead you back to the literature to understand how it can be used.

As you settle on a research question, and begin to look around for data to answer it, it is good to be explicit about your unit of analysis . The unit of analysis is whatever entity of body you wish to be able to answer your question about at the end of your study. Related, there is also the unit of observation , which is whatever unit you are measuring phenomena at. The unit of analysis and observation can be the same (they often are), but they can also be different.
Your unit of analysis (and observation) can be nations, cities, neighborhoods, individuals, or any other such grouping. Let’s use a few examples. If our research question is ‘why are some nations rich?’ we can answer that by collecting data for different nations, or we could use survey data about the individuals within different countries to make a comparison. Our unit of analysis is the same (countries), but our unit of observation (countries in the first, individuals in the second) can change.
Often we can study similar subjects using different units of observation or analysis. If I want to broadly study volunteering, I could collect data to understand volunteering rates for individuals, cities, states, or countries. The unit of observation will all depend upon what data I either collect or is available. And the unit of analysis can change as well, because I might want to study predictors of why some individuals volunteer and others don’t, or I may want to understand why different countries have different rates of volunteering. Which is all to say that it’ll depend on what you’re actually studying, but you should be explicit from the start about who you are studying.
3.5 Writing research
This is sort of where I get frustrated with myself as a teacher, or more specifically frustrated at myself for you. I just laid out three types of research. One you definitely do, which is secondary research. The second, applied research, is something you’ll see in the world all around you, and there’s some chance you might end up doing in your professional life. And the third, academic research, is generally inaccessible, uncommon, and probably not something you’ll ever do in your life. And yet, here we are, in a class on research, about to start talking about how to write a highly structure research paper using a format you’ll never use again.
Why? Why am I going to do that? In part it’s so that you can better understand the field you’re studying. It’s important to understand what it means not just to be curious about politics or sociology, but to understand what it means to study that field.
Beyond that, the best way to learn something new is to break it apart. This will be a bit like learning to drive by first building a car from parts. You could just move straight to secondary research or driving the car, but for you to really understand why things are working or where a breakdown might be, you have to understand the underlying parts. Each part of your car is important for getting you from point A to B, and each step that goes into research is important to getting you from your question to the right answer.
So when you see a headline in the future like

You’ll be able to better understand how that headline was made. The people that wrote that headline were using some academic research that is being translated to the public to use as secondary research. Where did it come from though, what did the researchers do to know that was the right answer? If it’s good research, they probably followed a process like what we’re about to lay out.
3.5.1 Introduction
If you look at published research in an academic journal it will typically follow a basic structure with 5 sections. The introduction explains the subject of your research and clearly identifies your research question. It provides a bit of background about the subject the current relevancy of it or maybe recent events that heightened its importance.
A good introduction thus has two purposes. First, it should explain to the reader what the paper is about. The thing you learn as you continue to write is the value of being clear in the introduction. Tell the reader what you do in the paper, the order of information you’re going to present, and what they takeaway is going to be. There shouldn’t be any surprise endings or twists. Just give them everything up front.
You can see that is done in the article excerpted and annotated below. This is from an article I published in 2020 Evaluating the impact of short-term rental regulations on Airbnb in New Orleans . The title should generally give you an idea of what the paper is about. It’s not the best paper I’ve ever written, but it’s short and so it’s easy to identify the structure of what I’m describing there. Read the complete introduction below to see how I describe the purpose of the paper in a direct manner with some background to prepare the reader.

3.5.2 Literature Review
There are two themes that should be described in any literature review . The two aren’t separate sections, they’re both intertwined.
- What has been done in the area of your research before
- What I need to know as a reader to understand what you’re going to do.
You want to prove to the reader that you’re aware of what’s been done in the area of your research before so they’ll believe your research is informed and new. The worst feedback you can get from a reviewer is that someone has already done the same study you’re attempting to publish. With any research question you identify you’ll find that a lot has been done before, and that’s fine. But describe what has been done so that I can better understand what makes yours different or unique.
And you’re also trying to make sure I can understand the background of your topic. What are the key words you’re going to be using? How have other people studied the issue? It’s all the background I need to understand your new contribution. Imagine you’re explaining a movie to someone so that they can see the sequel with you. Who are the main characters, what was the story, where did it leave off? Get them excited to see the sequel because you’re going to finally answer the question that was left lingering by all those past researchers!
You’ll want to use the literature you review to build hypotheses for your article. A hypothesis is a statement of what you expect to find. Hypothesis: toddlers that drink milk will be taller as adults.
That statement about toddlers and milk might be right or wrong, and that’s okay! That’s what the paper is building towards, proving whether the hypothesis is correct or not. Because right or wrong, if that hypothesis hasn’t been tested before we’re learning something new. But the hypothesis will be a lot better and more reasonable if it’s based on existing literature. Why would I think milk would help toddlers grow? I’d want to base that prediction on studies about milks effect on the bodies and what non-milk drinkers might consume and anything else that would be relevant.
The literature review for the paper below is 10 paragraphs in all, but I want to just pull out two. What the literature review is trying to do is just get the reader ready. Again, it’s the “previously on…” intro to your favorite show.

3.5.3 Methodology
The introduction and literature review are all used to set up your new study. Now you can explain how you’ll do whatever new and impressive thing you’re about to do in the methodology section. Describe the data you collected and how you’ll analyze it. Essentially you want to draw the reader a road map so they can understand exactly how to redo your study. It’s similar to the chain of custody in evidence for criminal cases. How did you find this information, where did it come from, why should we trust that this data is good? You don’t just wake up and find data on the side of the road, it had be collected somehow and the way it’s collected could impact whether we trust it or not.
One of the big concerns in science is replicability. We’ll talk about that later, but the study design section is a nod towards it. If I wanted to redesign your study, recreate the experiment with similar subjects in a similar setting, how would I do that? In science, we don’t just trust your word for how you generated your results. Tell us how you generated them, so that we can consider whether there were any potential problems present.
You can start writing the methodology section as you begin the research. As you start you should have a design in mind at the beginning of any project, including what data you are going to collect, how will you collect it, and how you will analyze it. Answers to those questions might evolve as you conduct the research, but you can begin by setting it out as a research design , describing your reserach plan, and then revise it as you write the paper. Regardless, your collection and analysis should be guided by a research design, whether formally written or just a mental plan.
Where did you get the data, and what are you gonna do with it now that you have it? What’s written below may or may not make sense at this stage, but I’m including it just to illustrate the way that researchers attempt to write clearly and directly in describing their studies.

3.6 Results
Once you’ve explained how you conducted your study, you can go ahead and tell the reader what you found in the results section. Exactly what you’ll say here will differ based on what you studied, but there isn’t a lot more to say at this stage.

3.6.1 Discussion
The paper then concludes with a discussion of the significance of the results and their implications. You found something, why do we care? How does that change the field? Should policymakers react? Should scientists react? You’ll often start with an overview of what the paper found, before launching into some of the more specific takeaways you want readers to get.

3.7 Summary
This chapter has covered some of the different ways we do research, and one way (a formal paper) that we report our research. It might seem a little overwhelming to think about how to write up your research results before you even know how to do research. And that’s fine, this is something of getting a fly over of the forest before we start to look more closely at the trees. It’s good to have an idea of what your final paper might look like, before we get started. Now we can begin to get a little more detailed about how we fill in all those words between the title and the final period on a research paper.
- USC Libraries
- Research Guides
Organizing Your Social Sciences Research Paper
- Types of Research Designs
- Purpose of Guide
- Design Flaws to Avoid
- Independent and Dependent Variables
- Glossary of Research Terms
- Reading Research Effectively
- Narrowing a Topic Idea
- Broadening a Topic Idea
- Extending the Timeliness of a Topic Idea
- Academic Writing Style
- Choosing a Title
- Making an Outline
- Paragraph Development
- Research Process Video Series
- Executive Summary
- The C.A.R.S. Model
- Background Information
- The Research Problem/Question
- Theoretical Framework
- Citation Tracking
- Content Alert Services
- Evaluating Sources
- Primary Sources
- Secondary Sources
- Tiertiary Sources
- Scholarly vs. Popular Publications
- Qualitative Methods
- Quantitative Methods
- Insiderness
- Using Non-Textual Elements
- Limitations of the Study
- Common Grammar Mistakes
- Writing Concisely
- Avoiding Plagiarism
- Footnotes or Endnotes?
- Further Readings
- Generative AI and Writing
- USC Libraries Tutorials and Other Guides
- Bibliography
Introduction
Before beginning your paper, you need to decide how you plan to design the study .
The research design refers to the overall strategy and analytical approach that you have chosen in order to integrate, in a coherent and logical way, the different components of the study, thus ensuring that the research problem will be thoroughly investigated. It constitutes the blueprint for the collection, measurement, and interpretation of information and data. Note that the research problem determines the type of design you choose, not the other way around!
De Vaus, D. A. Research Design in Social Research . London: SAGE, 2001; Trochim, William M.K. Research Methods Knowledge Base. 2006.
General Structure and Writing Style
The function of a research design is to ensure that the evidence obtained enables you to effectively address the research problem logically and as unambiguously as possible . In social sciences research, obtaining information relevant to the research problem generally entails specifying the type of evidence needed to test the underlying assumptions of a theory, to evaluate a program, or to accurately describe and assess meaning related to an observable phenomenon.
With this in mind, a common mistake made by researchers is that they begin their investigations before they have thought critically about what information is required to address the research problem. Without attending to these design issues beforehand, the overall research problem will not be adequately addressed and any conclusions drawn will run the risk of being weak and unconvincing. As a consequence, the overall validity of the study will be undermined.
The length and complexity of describing the research design in your paper can vary considerably, but any well-developed description will achieve the following :
- Identify the research problem clearly and justify its selection, particularly in relation to any valid alternative designs that could have been used,
- Review and synthesize previously published literature associated with the research problem,
- Clearly and explicitly specify hypotheses [i.e., research questions] central to the problem,
- Effectively describe the information and/or data which will be necessary for an adequate testing of the hypotheses and explain how such information and/or data will be obtained, and
- Describe the methods of analysis to be applied to the data in determining whether or not the hypotheses are true or false.
The research design is usually incorporated into the introduction of your paper . You can obtain an overall sense of what to do by reviewing studies that have utilized the same research design [e.g., using a case study approach]. This can help you develop an outline to follow for your own paper.
NOTE : Use the SAGE Research Methods Online and Cases and the SAGE Research Methods Videos databases to search for scholarly resources on how to apply specific research designs and methods . The Research Methods Online database contains links to more than 175,000 pages of SAGE publisher's book, journal, and reference content on quantitative, qualitative, and mixed research methodologies. Also included is a collection of case studies of social research projects that can be used to help you better understand abstract or complex methodological concepts. The Research Methods Videos database contains hours of tutorials, interviews, video case studies, and mini-documentaries covering the entire research process.
Creswell, John W. and J. David Creswell. Research Design: Qualitative, Quantitative, and Mixed Methods Approaches . 5th edition. Thousand Oaks, CA: Sage, 2018; De Vaus, D. A. Research Design in Social Research . London: SAGE, 2001; Gorard, Stephen. Research Design: Creating Robust Approaches for the Social Sciences . Thousand Oaks, CA: Sage, 2013; Leedy, Paul D. and Jeanne Ellis Ormrod. Practical Research: Planning and Design . Tenth edition. Boston, MA: Pearson, 2013; Vogt, W. Paul, Dianna C. Gardner, and Lynne M. Haeffele. When to Use What Research Design . New York: Guilford, 2012.
Action Research Design
Definition and Purpose
The essentials of action research design follow a characteristic cycle whereby initially an exploratory stance is adopted, where an understanding of a problem is developed and plans are made for some form of interventionary strategy. Then the intervention is carried out [the "action" in action research] during which time, pertinent observations are collected in various forms. The new interventional strategies are carried out, and this cyclic process repeats, continuing until a sufficient understanding of [or a valid implementation solution for] the problem is achieved. The protocol is iterative or cyclical in nature and is intended to foster deeper understanding of a given situation, starting with conceptualizing and particularizing the problem and moving through several interventions and evaluations.
What do these studies tell you ?
- This is a collaborative and adaptive research design that lends itself to use in work or community situations.
- Design focuses on pragmatic and solution-driven research outcomes rather than testing theories.
- When practitioners use action research, it has the potential to increase the amount they learn consciously from their experience; the action research cycle can be regarded as a learning cycle.
- Action research studies often have direct and obvious relevance to improving practice and advocating for change.
- There are no hidden controls or preemption of direction by the researcher.
What these studies don't tell you ?
- It is harder to do than conducting conventional research because the researcher takes on responsibilities of advocating for change as well as for researching the topic.
- Action research is much harder to write up because it is less likely that you can use a standard format to report your findings effectively [i.e., data is often in the form of stories or observation].
- Personal over-involvement of the researcher may bias research results.
- The cyclic nature of action research to achieve its twin outcomes of action [e.g. change] and research [e.g. understanding] is time-consuming and complex to conduct.
- Advocating for change usually requires buy-in from study participants.
Coghlan, David and Mary Brydon-Miller. The Sage Encyclopedia of Action Research . Thousand Oaks, CA: Sage, 2014; Efron, Sara Efrat and Ruth Ravid. Action Research in Education: A Practical Guide . New York: Guilford, 2013; Gall, Meredith. Educational Research: An Introduction . Chapter 18, Action Research. 8th ed. Boston, MA: Pearson/Allyn and Bacon, 2007; Gorard, Stephen. Research Design: Creating Robust Approaches for the Social Sciences . Thousand Oaks, CA: Sage, 2013; Kemmis, Stephen and Robin McTaggart. “Participatory Action Research.” In Handbook of Qualitative Research . Norman Denzin and Yvonna S. Lincoln, eds. 2nd ed. (Thousand Oaks, CA: SAGE, 2000), pp. 567-605; McNiff, Jean. Writing and Doing Action Research . London: Sage, 2014; Reason, Peter and Hilary Bradbury. Handbook of Action Research: Participative Inquiry and Practice . Thousand Oaks, CA: SAGE, 2001.
Case Study Design
A case study is an in-depth study of a particular research problem rather than a sweeping statistical survey or comprehensive comparative inquiry. It is often used to narrow down a very broad field of research into one or a few easily researchable examples. The case study research design is also useful for testing whether a specific theory and model actually applies to phenomena in the real world. It is a useful design when not much is known about an issue or phenomenon.
- Approach excels at bringing us to an understanding of a complex issue through detailed contextual analysis of a limited number of events or conditions and their relationships.
- A researcher using a case study design can apply a variety of methodologies and rely on a variety of sources to investigate a research problem.
- Design can extend experience or add strength to what is already known through previous research.
- Social scientists, in particular, make wide use of this research design to examine contemporary real-life situations and provide the basis for the application of concepts and theories and the extension of methodologies.
- The design can provide detailed descriptions of specific and rare cases.
- A single or small number of cases offers little basis for establishing reliability or to generalize the findings to a wider population of people, places, or things.
- Intense exposure to the study of a case may bias a researcher's interpretation of the findings.
- Design does not facilitate assessment of cause and effect relationships.
- Vital information may be missing, making the case hard to interpret.
- The case may not be representative or typical of the larger problem being investigated.
- If the criteria for selecting a case is because it represents a very unusual or unique phenomenon or problem for study, then your interpretation of the findings can only apply to that particular case.
Case Studies. Writing@CSU. Colorado State University; Anastas, Jeane W. Research Design for Social Work and the Human Services . Chapter 4, Flexible Methods: Case Study Design. 2nd ed. New York: Columbia University Press, 1999; Gerring, John. “What Is a Case Study and What Is It Good for?” American Political Science Review 98 (May 2004): 341-354; Greenhalgh, Trisha, editor. Case Study Evaluation: Past, Present and Future Challenges . Bingley, UK: Emerald Group Publishing, 2015; Mills, Albert J. , Gabrielle Durepos, and Eiden Wiebe, editors. Encyclopedia of Case Study Research . Thousand Oaks, CA: SAGE Publications, 2010; Stake, Robert E. The Art of Case Study Research . Thousand Oaks, CA: SAGE, 1995; Yin, Robert K. Case Study Research: Design and Theory . Applied Social Research Methods Series, no. 5. 3rd ed. Thousand Oaks, CA: SAGE, 2003.
Causal Design
Causality studies may be thought of as understanding a phenomenon in terms of conditional statements in the form, “If X, then Y.” This type of research is used to measure what impact a specific change will have on existing norms and assumptions. Most social scientists seek causal explanations that reflect tests of hypotheses. Causal effect (nomothetic perspective) occurs when variation in one phenomenon, an independent variable, leads to or results, on average, in variation in another phenomenon, the dependent variable.
Conditions necessary for determining causality:
- Empirical association -- a valid conclusion is based on finding an association between the independent variable and the dependent variable.
- Appropriate time order -- to conclude that causation was involved, one must see that cases were exposed to variation in the independent variable before variation in the dependent variable.
- Nonspuriousness -- a relationship between two variables that is not due to variation in a third variable.
- Causality research designs assist researchers in understanding why the world works the way it does through the process of proving a causal link between variables and by the process of eliminating other possibilities.
- Replication is possible.
- There is greater confidence the study has internal validity due to the systematic subject selection and equity of groups being compared.
- Not all relationships are causal! The possibility always exists that, by sheer coincidence, two unrelated events appear to be related [e.g., Punxatawney Phil could accurately predict the duration of Winter for five consecutive years but, the fact remains, he's just a big, furry rodent].
- Conclusions about causal relationships are difficult to determine due to a variety of extraneous and confounding variables that exist in a social environment. This means causality can only be inferred, never proven.
- If two variables are correlated, the cause must come before the effect. However, even though two variables might be causally related, it can sometimes be difficult to determine which variable comes first and, therefore, to establish which variable is the actual cause and which is the actual effect.
Beach, Derek and Rasmus Brun Pedersen. Causal Case Study Methods: Foundations and Guidelines for Comparing, Matching, and Tracing . Ann Arbor, MI: University of Michigan Press, 2016; Bachman, Ronet. The Practice of Research in Criminology and Criminal Justice . Chapter 5, Causation and Research Designs. 3rd ed. Thousand Oaks, CA: Pine Forge Press, 2007; Brewer, Ernest W. and Jennifer Kubn. “Causal-Comparative Design.” In Encyclopedia of Research Design . Neil J. Salkind, editor. (Thousand Oaks, CA: Sage, 2010), pp. 125-132; Causal Research Design: Experimentation. Anonymous SlideShare Presentation; Gall, Meredith. Educational Research: An Introduction . Chapter 11, Nonexperimental Research: Correlational Designs. 8th ed. Boston, MA: Pearson/Allyn and Bacon, 2007; Trochim, William M.K. Research Methods Knowledge Base. 2006.
Cohort Design
Often used in the medical sciences, but also found in the applied social sciences, a cohort study generally refers to a study conducted over a period of time involving members of a population which the subject or representative member comes from, and who are united by some commonality or similarity. Using a quantitative framework, a cohort study makes note of statistical occurrence within a specialized subgroup, united by same or similar characteristics that are relevant to the research problem being investigated, rather than studying statistical occurrence within the general population. Using a qualitative framework, cohort studies generally gather data using methods of observation. Cohorts can be either "open" or "closed."
- Open Cohort Studies [dynamic populations, such as the population of Los Angeles] involve a population that is defined just by the state of being a part of the study in question (and being monitored for the outcome). Date of entry and exit from the study is individually defined, therefore, the size of the study population is not constant. In open cohort studies, researchers can only calculate rate based data, such as, incidence rates and variants thereof.
- Closed Cohort Studies [static populations, such as patients entered into a clinical trial] involve participants who enter into the study at one defining point in time and where it is presumed that no new participants can enter the cohort. Given this, the number of study participants remains constant (or can only decrease).
- The use of cohorts is often mandatory because a randomized control study may be unethical. For example, you cannot deliberately expose people to asbestos, you can only study its effects on those who have already been exposed. Research that measures risk factors often relies upon cohort designs.
- Because cohort studies measure potential causes before the outcome has occurred, they can demonstrate that these “causes” preceded the outcome, thereby avoiding the debate as to which is the cause and which is the effect.
- Cohort analysis is highly flexible and can provide insight into effects over time and related to a variety of different types of changes [e.g., social, cultural, political, economic, etc.].
- Either original data or secondary data can be used in this design.
- In cases where a comparative analysis of two cohorts is made [e.g., studying the effects of one group exposed to asbestos and one that has not], a researcher cannot control for all other factors that might differ between the two groups. These factors are known as confounding variables.
- Cohort studies can end up taking a long time to complete if the researcher must wait for the conditions of interest to develop within the group. This also increases the chance that key variables change during the course of the study, potentially impacting the validity of the findings.
- Due to the lack of randominization in the cohort design, its external validity is lower than that of study designs where the researcher randomly assigns participants.
Healy P, Devane D. “Methodological Considerations in Cohort Study Designs.” Nurse Researcher 18 (2011): 32-36; Glenn, Norval D, editor. Cohort Analysis . 2nd edition. Thousand Oaks, CA: Sage, 2005; Levin, Kate Ann. Study Design IV: Cohort Studies. Evidence-Based Dentistry 7 (2003): 51–52; Payne, Geoff. “Cohort Study.” In The SAGE Dictionary of Social Research Methods . Victor Jupp, editor. (Thousand Oaks, CA: Sage, 2006), pp. 31-33; Study Design 101. Himmelfarb Health Sciences Library. George Washington University, November 2011; Cohort Study. Wikipedia.
Cross-Sectional Design
Cross-sectional research designs have three distinctive features: no time dimension; a reliance on existing differences rather than change following intervention; and, groups are selected based on existing differences rather than random allocation. The cross-sectional design can only measure differences between or from among a variety of people, subjects, or phenomena rather than a process of change. As such, researchers using this design can only employ a relatively passive approach to making causal inferences based on findings.
- Cross-sectional studies provide a clear 'snapshot' of the outcome and the characteristics associated with it, at a specific point in time.
- Unlike an experimental design, where there is an active intervention by the researcher to produce and measure change or to create differences, cross-sectional designs focus on studying and drawing inferences from existing differences between people, subjects, or phenomena.
- Entails collecting data at and concerning one point in time. While longitudinal studies involve taking multiple measures over an extended period of time, cross-sectional research is focused on finding relationships between variables at one moment in time.
- Groups identified for study are purposely selected based upon existing differences in the sample rather than seeking random sampling.
- Cross-section studies are capable of using data from a large number of subjects and, unlike observational studies, is not geographically bound.
- Can estimate prevalence of an outcome of interest because the sample is usually taken from the whole population.
- Because cross-sectional designs generally use survey techniques to gather data, they are relatively inexpensive and take up little time to conduct.
- Finding people, subjects, or phenomena to study that are very similar except in one specific variable can be difficult.
- Results are static and time bound and, therefore, give no indication of a sequence of events or reveal historical or temporal contexts.
- Studies cannot be utilized to establish cause and effect relationships.
- This design only provides a snapshot of analysis so there is always the possibility that a study could have differing results if another time-frame had been chosen.
- There is no follow up to the findings.
Bethlehem, Jelke. "7: Cross-sectional Research." In Research Methodology in the Social, Behavioural and Life Sciences . Herman J Adèr and Gideon J Mellenbergh, editors. (London, England: Sage, 1999), pp. 110-43; Bourque, Linda B. “Cross-Sectional Design.” In The SAGE Encyclopedia of Social Science Research Methods . Michael S. Lewis-Beck, Alan Bryman, and Tim Futing Liao. (Thousand Oaks, CA: 2004), pp. 230-231; Hall, John. “Cross-Sectional Survey Design.” In Encyclopedia of Survey Research Methods . Paul J. Lavrakas, ed. (Thousand Oaks, CA: Sage, 2008), pp. 173-174; Helen Barratt, Maria Kirwan. Cross-Sectional Studies: Design Application, Strengths and Weaknesses of Cross-Sectional Studies. Healthknowledge, 2009. Cross-Sectional Study. Wikipedia.
Descriptive Design
Descriptive research designs help provide answers to the questions of who, what, when, where, and how associated with a particular research problem; a descriptive study cannot conclusively ascertain answers to why. Descriptive research is used to obtain information concerning the current status of the phenomena and to describe "what exists" with respect to variables or conditions in a situation.
- The subject is being observed in a completely natural and unchanged natural environment. True experiments, whilst giving analyzable data, often adversely influence the normal behavior of the subject [a.k.a., the Heisenberg effect whereby measurements of certain systems cannot be made without affecting the systems].
- Descriptive research is often used as a pre-cursor to more quantitative research designs with the general overview giving some valuable pointers as to what variables are worth testing quantitatively.
- If the limitations are understood, they can be a useful tool in developing a more focused study.
- Descriptive studies can yield rich data that lead to important recommendations in practice.
- Appoach collects a large amount of data for detailed analysis.
- The results from a descriptive research cannot be used to discover a definitive answer or to disprove a hypothesis.
- Because descriptive designs often utilize observational methods [as opposed to quantitative methods], the results cannot be replicated.
- The descriptive function of research is heavily dependent on instrumentation for measurement and observation.
Anastas, Jeane W. Research Design for Social Work and the Human Services . Chapter 5, Flexible Methods: Descriptive Research. 2nd ed. New York: Columbia University Press, 1999; Given, Lisa M. "Descriptive Research." In Encyclopedia of Measurement and Statistics . Neil J. Salkind and Kristin Rasmussen, editors. (Thousand Oaks, CA: Sage, 2007), pp. 251-254; McNabb, Connie. Descriptive Research Methodologies. Powerpoint Presentation; Shuttleworth, Martyn. Descriptive Research Design, September 26, 2008; Erickson, G. Scott. "Descriptive Research Design." In New Methods of Market Research and Analysis . (Northampton, MA: Edward Elgar Publishing, 2017), pp. 51-77; Sahin, Sagufta, and Jayanta Mete. "A Brief Study on Descriptive Research: Its Nature and Application in Social Science." International Journal of Research and Analysis in Humanities 1 (2021): 11; K. Swatzell and P. Jennings. “Descriptive Research: The Nuts and Bolts.” Journal of the American Academy of Physician Assistants 20 (2007), pp. 55-56; Kane, E. Doing Your Own Research: Basic Descriptive Research in the Social Sciences and Humanities . London: Marion Boyars, 1985.
Experimental Design
A blueprint of the procedure that enables the researcher to maintain control over all factors that may affect the result of an experiment. In doing this, the researcher attempts to determine or predict what may occur. Experimental research is often used where there is time priority in a causal relationship (cause precedes effect), there is consistency in a causal relationship (a cause will always lead to the same effect), and the magnitude of the correlation is great. The classic experimental design specifies an experimental group and a control group. The independent variable is administered to the experimental group and not to the control group, and both groups are measured on the same dependent variable. Subsequent experimental designs have used more groups and more measurements over longer periods. True experiments must have control, randomization, and manipulation.
- Experimental research allows the researcher to control the situation. In so doing, it allows researchers to answer the question, “What causes something to occur?”
- Permits the researcher to identify cause and effect relationships between variables and to distinguish placebo effects from treatment effects.
- Experimental research designs support the ability to limit alternative explanations and to infer direct causal relationships in the study.
- Approach provides the highest level of evidence for single studies.
- The design is artificial, and results may not generalize well to the real world.
- The artificial settings of experiments may alter the behaviors or responses of participants.
- Experimental designs can be costly if special equipment or facilities are needed.
- Some research problems cannot be studied using an experiment because of ethical or technical reasons.
- Difficult to apply ethnographic and other qualitative methods to experimentally designed studies.
Anastas, Jeane W. Research Design for Social Work and the Human Services . Chapter 7, Flexible Methods: Experimental Research. 2nd ed. New York: Columbia University Press, 1999; Chapter 2: Research Design, Experimental Designs. School of Psychology, University of New England, 2000; Chow, Siu L. "Experimental Design." In Encyclopedia of Research Design . Neil J. Salkind, editor. (Thousand Oaks, CA: Sage, 2010), pp. 448-453; "Experimental Design." In Social Research Methods . Nicholas Walliman, editor. (London, England: Sage, 2006), pp, 101-110; Experimental Research. Research Methods by Dummies. Department of Psychology. California State University, Fresno, 2006; Kirk, Roger E. Experimental Design: Procedures for the Behavioral Sciences . 4th edition. Thousand Oaks, CA: Sage, 2013; Trochim, William M.K. Experimental Design. Research Methods Knowledge Base. 2006; Rasool, Shafqat. Experimental Research. Slideshare presentation.
Exploratory Design
An exploratory design is conducted about a research problem when there are few or no earlier studies to refer to or rely upon to predict an outcome . The focus is on gaining insights and familiarity for later investigation or undertaken when research problems are in a preliminary stage of investigation. Exploratory designs are often used to establish an understanding of how best to proceed in studying an issue or what methodology would effectively apply to gathering information about the issue.
The goals of exploratory research are intended to produce the following possible insights:
- Familiarity with basic details, settings, and concerns.
- Well grounded picture of the situation being developed.
- Generation of new ideas and assumptions.
- Development of tentative theories or hypotheses.
- Determination about whether a study is feasible in the future.
- Issues get refined for more systematic investigation and formulation of new research questions.
- Direction for future research and techniques get developed.
- Design is a useful approach for gaining background information on a particular topic.
- Exploratory research is flexible and can address research questions of all types (what, why, how).
- Provides an opportunity to define new terms and clarify existing concepts.
- Exploratory research is often used to generate formal hypotheses and develop more precise research problems.
- In the policy arena or applied to practice, exploratory studies help establish research priorities and where resources should be allocated.
- Exploratory research generally utilizes small sample sizes and, thus, findings are typically not generalizable to the population at large.
- The exploratory nature of the research inhibits an ability to make definitive conclusions about the findings. They provide insight but not definitive conclusions.
- The research process underpinning exploratory studies is flexible but often unstructured, leading to only tentative results that have limited value to decision-makers.
- Design lacks rigorous standards applied to methods of data gathering and analysis because one of the areas for exploration could be to determine what method or methodologies could best fit the research problem.
Cuthill, Michael. “Exploratory Research: Citizen Participation, Local Government, and Sustainable Development in Australia.” Sustainable Development 10 (2002): 79-89; Streb, Christoph K. "Exploratory Case Study." In Encyclopedia of Case Study Research . Albert J. Mills, Gabrielle Durepos and Eiden Wiebe, editors. (Thousand Oaks, CA: Sage, 2010), pp. 372-374; Taylor, P. J., G. Catalano, and D.R.F. Walker. “Exploratory Analysis of the World City Network.” Urban Studies 39 (December 2002): 2377-2394; Exploratory Research. Wikipedia.
Field Research Design
Sometimes referred to as ethnography or participant observation, designs around field research encompass a variety of interpretative procedures [e.g., observation and interviews] rooted in qualitative approaches to studying people individually or in groups while inhabiting their natural environment as opposed to using survey instruments or other forms of impersonal methods of data gathering. Information acquired from observational research takes the form of “ field notes ” that involves documenting what the researcher actually sees and hears while in the field. Findings do not consist of conclusive statements derived from numbers and statistics because field research involves analysis of words and observations of behavior. Conclusions, therefore, are developed from an interpretation of findings that reveal overriding themes, concepts, and ideas. More information can be found HERE .
- Field research is often necessary to fill gaps in understanding the research problem applied to local conditions or to specific groups of people that cannot be ascertained from existing data.
- The research helps contextualize already known information about a research problem, thereby facilitating ways to assess the origins, scope, and scale of a problem and to gage the causes, consequences, and means to resolve an issue based on deliberate interaction with people in their natural inhabited spaces.
- Enables the researcher to corroborate or confirm data by gathering additional information that supports or refutes findings reported in prior studies of the topic.
- Because the researcher in embedded in the field, they are better able to make observations or ask questions that reflect the specific cultural context of the setting being investigated.
- Observing the local reality offers the opportunity to gain new perspectives or obtain unique data that challenges existing theoretical propositions or long-standing assumptions found in the literature.
What these studies don't tell you
- A field research study requires extensive time and resources to carry out the multiple steps involved with preparing for the gathering of information, including for example, examining background information about the study site, obtaining permission to access the study site, and building trust and rapport with subjects.
- Requires a commitment to staying engaged in the field to ensure that you can adequately document events and behaviors as they unfold.
- The unpredictable nature of fieldwork means that researchers can never fully control the process of data gathering. They must maintain a flexible approach to studying the setting because events and circumstances can change quickly or unexpectedly.
- Findings can be difficult to interpret and verify without access to documents and other source materials that help to enhance the credibility of information obtained from the field [i.e., the act of triangulating the data].
- Linking the research problem to the selection of study participants inhabiting their natural environment is critical. However, this specificity limits the ability to generalize findings to different situations or in other contexts or to infer courses of action applied to other settings or groups of people.
- The reporting of findings must take into account how the researcher themselves may have inadvertently affected respondents and their behaviors.
Historical Design
The purpose of a historical research design is to collect, verify, and synthesize evidence from the past to establish facts that defend or refute a hypothesis. It uses secondary sources and a variety of primary documentary evidence, such as, diaries, official records, reports, archives, and non-textual information [maps, pictures, audio and visual recordings]. The limitation is that the sources must be both authentic and valid.
- The historical research design is unobtrusive; the act of research does not affect the results of the study.
- The historical approach is well suited for trend analysis.
- Historical records can add important contextual background required to more fully understand and interpret a research problem.
- There is often no possibility of researcher-subject interaction that could affect the findings.
- Historical sources can be used over and over to study different research problems or to replicate a previous study.
- The ability to fulfill the aims of your research are directly related to the amount and quality of documentation available to understand the research problem.
- Since historical research relies on data from the past, there is no way to manipulate it to control for contemporary contexts.
- Interpreting historical sources can be very time consuming.
- The sources of historical materials must be archived consistently to ensure access. This may especially challenging for digital or online-only sources.
- Original authors bring their own perspectives and biases to the interpretation of past events and these biases are more difficult to ascertain in historical resources.
- Due to the lack of control over external variables, historical research is very weak with regard to the demands of internal validity.
- It is rare that the entirety of historical documentation needed to fully address a research problem is available for interpretation, therefore, gaps need to be acknowledged.
Howell, Martha C. and Walter Prevenier. From Reliable Sources: An Introduction to Historical Methods . Ithaca, NY: Cornell University Press, 2001; Lundy, Karen Saucier. "Historical Research." In The Sage Encyclopedia of Qualitative Research Methods . Lisa M. Given, editor. (Thousand Oaks, CA: Sage, 2008), pp. 396-400; Marius, Richard. and Melvin E. Page. A Short Guide to Writing about History . 9th edition. Boston, MA: Pearson, 2015; Savitt, Ronald. “Historical Research in Marketing.” Journal of Marketing 44 (Autumn, 1980): 52-58; Gall, Meredith. Educational Research: An Introduction . Chapter 16, Historical Research. 8th ed. Boston, MA: Pearson/Allyn and Bacon, 2007.
Longitudinal Design
A longitudinal study follows the same sample over time and makes repeated observations. For example, with longitudinal surveys, the same group of people is interviewed at regular intervals, enabling researchers to track changes over time and to relate them to variables that might explain why the changes occur. Longitudinal research designs describe patterns of change and help establish the direction and magnitude of causal relationships. Measurements are taken on each variable over two or more distinct time periods. This allows the researcher to measure change in variables over time. It is a type of observational study sometimes referred to as a panel study.
- Longitudinal data facilitate the analysis of the duration of a particular phenomenon.
- Enables survey researchers to get close to the kinds of causal explanations usually attainable only with experiments.
- The design permits the measurement of differences or change in a variable from one period to another [i.e., the description of patterns of change over time].
- Longitudinal studies facilitate the prediction of future outcomes based upon earlier factors.
- The data collection method may change over time.
- Maintaining the integrity of the original sample can be difficult over an extended period of time.
- It can be difficult to show more than one variable at a time.
- This design often needs qualitative research data to explain fluctuations in the results.
- A longitudinal research design assumes present trends will continue unchanged.
- It can take a long period of time to gather results.
- There is a need to have a large sample size and accurate sampling to reach representativness.
Anastas, Jeane W. Research Design for Social Work and the Human Services . Chapter 6, Flexible Methods: Relational and Longitudinal Research. 2nd ed. New York: Columbia University Press, 1999; Forgues, Bernard, and Isabelle Vandangeon-Derumez. "Longitudinal Analyses." In Doing Management Research . Raymond-Alain Thiétart and Samantha Wauchope, editors. (London, England: Sage, 2001), pp. 332-351; Kalaian, Sema A. and Rafa M. Kasim. "Longitudinal Studies." In Encyclopedia of Survey Research Methods . Paul J. Lavrakas, ed. (Thousand Oaks, CA: Sage, 2008), pp. 440-441; Menard, Scott, editor. Longitudinal Research . Thousand Oaks, CA: Sage, 2002; Ployhart, Robert E. and Robert J. Vandenberg. "Longitudinal Research: The Theory, Design, and Analysis of Change.” Journal of Management 36 (January 2010): 94-120; Longitudinal Study. Wikipedia.
Meta-Analysis Design
Meta-analysis is an analytical methodology designed to systematically evaluate and summarize the results from a number of individual studies, thereby, increasing the overall sample size and the ability of the researcher to study effects of interest. The purpose is to not simply summarize existing knowledge, but to develop a new understanding of a research problem using synoptic reasoning. The main objectives of meta-analysis include analyzing differences in the results among studies and increasing the precision by which effects are estimated. A well-designed meta-analysis depends upon strict adherence to the criteria used for selecting studies and the availability of information in each study to properly analyze their findings. Lack of information can severely limit the type of analyzes and conclusions that can be reached. In addition, the more dissimilarity there is in the results among individual studies [heterogeneity], the more difficult it is to justify interpretations that govern a valid synopsis of results. A meta-analysis needs to fulfill the following requirements to ensure the validity of your findings:
- Clearly defined description of objectives, including precise definitions of the variables and outcomes that are being evaluated;
- A well-reasoned and well-documented justification for identification and selection of the studies;
- Assessment and explicit acknowledgment of any researcher bias in the identification and selection of those studies;
- Description and evaluation of the degree of heterogeneity among the sample size of studies reviewed; and,
- Justification of the techniques used to evaluate the studies.
- Can be an effective strategy for determining gaps in the literature.
- Provides a means of reviewing research published about a particular topic over an extended period of time and from a variety of sources.
- Is useful in clarifying what policy or programmatic actions can be justified on the basis of analyzing research results from multiple studies.
- Provides a method for overcoming small sample sizes in individual studies that previously may have had little relationship to each other.
- Can be used to generate new hypotheses or highlight research problems for future studies.
- Small violations in defining the criteria used for content analysis can lead to difficult to interpret and/or meaningless findings.
- A large sample size can yield reliable, but not necessarily valid, results.
- A lack of uniformity regarding, for example, the type of literature reviewed, how methods are applied, and how findings are measured within the sample of studies you are analyzing, can make the process of synthesis difficult to perform.
- Depending on the sample size, the process of reviewing and synthesizing multiple studies can be very time consuming.
Beck, Lewis W. "The Synoptic Method." The Journal of Philosophy 36 (1939): 337-345; Cooper, Harris, Larry V. Hedges, and Jeffrey C. Valentine, eds. The Handbook of Research Synthesis and Meta-Analysis . 2nd edition. New York: Russell Sage Foundation, 2009; Guzzo, Richard A., Susan E. Jackson and Raymond A. Katzell. “Meta-Analysis Analysis.” In Research in Organizational Behavior , Volume 9. (Greenwich, CT: JAI Press, 1987), pp 407-442; Lipsey, Mark W. and David B. Wilson. Practical Meta-Analysis . Thousand Oaks, CA: Sage Publications, 2001; Study Design 101. Meta-Analysis. The Himmelfarb Health Sciences Library, George Washington University; Timulak, Ladislav. “Qualitative Meta-Analysis.” In The SAGE Handbook of Qualitative Data Analysis . Uwe Flick, editor. (Los Angeles, CA: Sage, 2013), pp. 481-495; Walker, Esteban, Adrian V. Hernandez, and Micheal W. Kattan. "Meta-Analysis: It's Strengths and Limitations." Cleveland Clinic Journal of Medicine 75 (June 2008): 431-439.
Mixed-Method Design
- Narrative and non-textual information can add meaning to numeric data, while numeric data can add precision to narrative and non-textual information.
- Can utilize existing data while at the same time generating and testing a grounded theory approach to describe and explain the phenomenon under study.
- A broader, more complex research problem can be investigated because the researcher is not constrained by using only one method.
- The strengths of one method can be used to overcome the inherent weaknesses of another method.
- Can provide stronger, more robust evidence to support a conclusion or set of recommendations.
- May generate new knowledge new insights or uncover hidden insights, patterns, or relationships that a single methodological approach might not reveal.
- Produces more complete knowledge and understanding of the research problem that can be used to increase the generalizability of findings applied to theory or practice.
- A researcher must be proficient in understanding how to apply multiple methods to investigating a research problem as well as be proficient in optimizing how to design a study that coherently melds them together.
- Can increase the likelihood of conflicting results or ambiguous findings that inhibit drawing a valid conclusion or setting forth a recommended course of action [e.g., sample interview responses do not support existing statistical data].
- Because the research design can be very complex, reporting the findings requires a well-organized narrative, clear writing style, and precise word choice.
- Design invites collaboration among experts. However, merging different investigative approaches and writing styles requires more attention to the overall research process than studies conducted using only one methodological paradigm.
- Concurrent merging of quantitative and qualitative research requires greater attention to having adequate sample sizes, using comparable samples, and applying a consistent unit of analysis. For sequential designs where one phase of qualitative research builds on the quantitative phase or vice versa, decisions about what results from the first phase to use in the next phase, the choice of samples and estimating reasonable sample sizes for both phases, and the interpretation of results from both phases can be difficult.
- Due to multiple forms of data being collected and analyzed, this design requires extensive time and resources to carry out the multiple steps involved in data gathering and interpretation.
Burch, Patricia and Carolyn J. Heinrich. Mixed Methods for Policy Research and Program Evaluation . Thousand Oaks, CA: Sage, 2016; Creswell, John w. et al. Best Practices for Mixed Methods Research in the Health Sciences . Bethesda, MD: Office of Behavioral and Social Sciences Research, National Institutes of Health, 2010Creswell, John W. Research Design: Qualitative, Quantitative, and Mixed Methods Approaches . 4th edition. Thousand Oaks, CA: Sage Publications, 2014; Domínguez, Silvia, editor. Mixed Methods Social Networks Research . Cambridge, UK: Cambridge University Press, 2014; Hesse-Biber, Sharlene Nagy. Mixed Methods Research: Merging Theory with Practice . New York: Guilford Press, 2010; Niglas, Katrin. “How the Novice Researcher Can Make Sense of Mixed Methods Designs.” International Journal of Multiple Research Approaches 3 (2009): 34-46; Onwuegbuzie, Anthony J. and Nancy L. Leech. “Linking Research Questions to Mixed Methods Data Analysis Procedures.” The Qualitative Report 11 (September 2006): 474-498; Tashakorri, Abbas and John W. Creswell. “The New Era of Mixed Methods.” Journal of Mixed Methods Research 1 (January 2007): 3-7; Zhanga, Wanqing. “Mixed Methods Application in Health Intervention Research: A Multiple Case Study.” International Journal of Multiple Research Approaches 8 (2014): 24-35 .
Observational Design
This type of research design draws a conclusion by comparing subjects against a control group, in cases where the researcher has no control over the experiment. There are two general types of observational designs. In direct observations, people know that you are watching them. Unobtrusive measures involve any method for studying behavior where individuals do not know they are being observed. An observational study allows a useful insight into a phenomenon and avoids the ethical and practical difficulties of setting up a large and cumbersome research project.
- Observational studies are usually flexible and do not necessarily need to be structured around a hypothesis about what you expect to observe [data is emergent rather than pre-existing].
- The researcher is able to collect in-depth information about a particular behavior.
- Can reveal interrelationships among multifaceted dimensions of group interactions.
- You can generalize your results to real life situations.
- Observational research is useful for discovering what variables may be important before applying other methods like experiments.
- Observation research designs account for the complexity of group behaviors.
- Reliability of data is low because seeing behaviors occur over and over again may be a time consuming task and are difficult to replicate.
- In observational research, findings may only reflect a unique sample population and, thus, cannot be generalized to other groups.
- There can be problems with bias as the researcher may only "see what they want to see."
- There is no possibility to determine "cause and effect" relationships since nothing is manipulated.
- Sources or subjects may not all be equally credible.
- Any group that is knowingly studied is altered to some degree by the presence of the researcher, therefore, potentially skewing any data collected.
Atkinson, Paul and Martyn Hammersley. “Ethnography and Participant Observation.” In Handbook of Qualitative Research . Norman K. Denzin and Yvonna S. Lincoln, eds. (Thousand Oaks, CA: Sage, 1994), pp. 248-261; Observational Research. Research Methods by Dummies. Department of Psychology. California State University, Fresno, 2006; Patton Michael Quinn. Qualitiative Research and Evaluation Methods . Chapter 6, Fieldwork Strategies and Observational Methods. 3rd ed. Thousand Oaks, CA: Sage, 2002; Payne, Geoff and Judy Payne. "Observation." In Key Concepts in Social Research . The SAGE Key Concepts series. (London, England: Sage, 2004), pp. 158-162; Rosenbaum, Paul R. Design of Observational Studies . New York: Springer, 2010;Williams, J. Patrick. "Nonparticipant Observation." In The Sage Encyclopedia of Qualitative Research Methods . Lisa M. Given, editor.(Thousand Oaks, CA: Sage, 2008), pp. 562-563.
Philosophical Design
Understood more as an broad approach to examining a research problem than a methodological design, philosophical analysis and argumentation is intended to challenge deeply embedded, often intractable, assumptions underpinning an area of study. This approach uses the tools of argumentation derived from philosophical traditions, concepts, models, and theories to critically explore and challenge, for example, the relevance of logic and evidence in academic debates, to analyze arguments about fundamental issues, or to discuss the root of existing discourse about a research problem. These overarching tools of analysis can be framed in three ways:
- Ontology -- the study that describes the nature of reality; for example, what is real and what is not, what is fundamental and what is derivative?
- Epistemology -- the study that explores the nature of knowledge; for example, by what means does knowledge and understanding depend upon and how can we be certain of what we know?
- Axiology -- the study of values; for example, what values does an individual or group hold and why? How are values related to interest, desire, will, experience, and means-to-end? And, what is the difference between a matter of fact and a matter of value?
- Can provide a basis for applying ethical decision-making to practice.
- Functions as a means of gaining greater self-understanding and self-knowledge about the purposes of research.
- Brings clarity to general guiding practices and principles of an individual or group.
- Philosophy informs methodology.
- Refine concepts and theories that are invoked in relatively unreflective modes of thought and discourse.
- Beyond methodology, philosophy also informs critical thinking about epistemology and the structure of reality (metaphysics).
- Offers clarity and definition to the practical and theoretical uses of terms, concepts, and ideas.
- Limited application to specific research problems [answering the "So What?" question in social science research].
- Analysis can be abstract, argumentative, and limited in its practical application to real-life issues.
- While a philosophical analysis may render problematic that which was once simple or taken-for-granted, the writing can be dense and subject to unnecessary jargon, overstatement, and/or excessive quotation and documentation.
- There are limitations in the use of metaphor as a vehicle of philosophical analysis.
- There can be analytical difficulties in moving from philosophy to advocacy and between abstract thought and application to the phenomenal world.
Burton, Dawn. "Part I, Philosophy of the Social Sciences." In Research Training for Social Scientists . (London, England: Sage, 2000), pp. 1-5; Chapter 4, Research Methodology and Design. Unisa Institutional Repository (UnisaIR), University of South Africa; Jarvie, Ian C., and Jesús Zamora-Bonilla, editors. The SAGE Handbook of the Philosophy of Social Sciences . London: Sage, 2011; Labaree, Robert V. and Ross Scimeca. “The Philosophical Problem of Truth in Librarianship.” The Library Quarterly 78 (January 2008): 43-70; Maykut, Pamela S. Beginning Qualitative Research: A Philosophic and Practical Guide . Washington, DC: Falmer Press, 1994; McLaughlin, Hugh. "The Philosophy of Social Research." In Understanding Social Work Research . 2nd edition. (London: SAGE Publications Ltd., 2012), pp. 24-47; Stanford Encyclopedia of Philosophy . Metaphysics Research Lab, CSLI, Stanford University, 2013.
Sequential Design
- The researcher has a limitless option when it comes to sample size and the sampling schedule.
- Due to the repetitive nature of this research design, minor changes and adjustments can be done during the initial parts of the study to correct and hone the research method.
- This is a useful design for exploratory studies.
- There is very little effort on the part of the researcher when performing this technique. It is generally not expensive, time consuming, or workforce intensive.
- Because the study is conducted serially, the results of one sample are known before the next sample is taken and analyzed. This provides opportunities for continuous improvement of sampling and methods of analysis.
- The sampling method is not representative of the entire population. The only possibility of approaching representativeness is when the researcher chooses to use a very large sample size significant enough to represent a significant portion of the entire population. In this case, moving on to study a second or more specific sample can be difficult.
- The design cannot be used to create conclusions and interpretations that pertain to an entire population because the sampling technique is not randomized. Generalizability from findings is, therefore, limited.
- Difficult to account for and interpret variation from one sample to another over time, particularly when using qualitative methods of data collection.
Betensky, Rebecca. Harvard University, Course Lecture Note slides; Bovaird, James A. and Kevin A. Kupzyk. "Sequential Design." In Encyclopedia of Research Design . Neil J. Salkind, editor. (Thousand Oaks, CA: Sage, 2010), pp. 1347-1352; Cresswell, John W. Et al. “Advanced Mixed-Methods Research Designs.” In Handbook of Mixed Methods in Social and Behavioral Research . Abbas Tashakkori and Charles Teddle, eds. (Thousand Oaks, CA: Sage, 2003), pp. 209-240; Henry, Gary T. "Sequential Sampling." In The SAGE Encyclopedia of Social Science Research Methods . Michael S. Lewis-Beck, Alan Bryman and Tim Futing Liao, editors. (Thousand Oaks, CA: Sage, 2004), pp. 1027-1028; Nataliya V. Ivankova. “Using Mixed-Methods Sequential Explanatory Design: From Theory to Practice.” Field Methods 18 (February 2006): 3-20; Bovaird, James A. and Kevin A. Kupzyk. “Sequential Design.” In Encyclopedia of Research Design . Neil J. Salkind, ed. Thousand Oaks, CA: Sage, 2010; Sequential Analysis. Wikipedia.
Systematic Review
- A systematic review synthesizes the findings of multiple studies related to each other by incorporating strategies of analysis and interpretation intended to reduce biases and random errors.
- The application of critical exploration, evaluation, and synthesis methods separates insignificant, unsound, or redundant research from the most salient and relevant studies worthy of reflection.
- They can be use to identify, justify, and refine hypotheses, recognize and avoid hidden problems in prior studies, and explain data inconsistencies and conflicts in data.
- Systematic reviews can be used to help policy makers formulate evidence-based guidelines and regulations.
- The use of strict, explicit, and pre-determined methods of synthesis, when applied appropriately, provide reliable estimates about the effects of interventions, evaluations, and effects related to the overarching research problem investigated by each study under review.
- Systematic reviews illuminate where knowledge or thorough understanding of a research problem is lacking and, therefore, can then be used to guide future research.
- The accepted inclusion of unpublished studies [i.e., grey literature] ensures the broadest possible way to analyze and interpret research on a topic.
- Results of the synthesis can be generalized and the findings extrapolated into the general population with more validity than most other types of studies .
- Systematic reviews do not create new knowledge per se; they are a method for synthesizing existing studies about a research problem in order to gain new insights and determine gaps in the literature.
- The way researchers have carried out their investigations [e.g., the period of time covered, number of participants, sources of data analyzed, etc.] can make it difficult to effectively synthesize studies.
- The inclusion of unpublished studies can introduce bias into the review because they may not have undergone a rigorous peer-review process prior to publication. Examples may include conference presentations or proceedings, publications from government agencies, white papers, working papers, and internal documents from organizations, and doctoral dissertations and Master's theses.
Denyer, David and David Tranfield. "Producing a Systematic Review." In The Sage Handbook of Organizational Research Methods . David A. Buchanan and Alan Bryman, editors. ( Thousand Oaks, CA: Sage Publications, 2009), pp. 671-689; Foster, Margaret J. and Sarah T. Jewell, editors. Assembling the Pieces of a Systematic Review: A Guide for Librarians . Lanham, MD: Rowman and Littlefield, 2017; Gough, David, Sandy Oliver, James Thomas, editors. Introduction to Systematic Reviews . 2nd edition. Los Angeles, CA: Sage Publications, 2017; Gopalakrishnan, S. and P. Ganeshkumar. “Systematic Reviews and Meta-analysis: Understanding the Best Evidence in Primary Healthcare.” Journal of Family Medicine and Primary Care 2 (2013): 9-14; Gough, David, James Thomas, and Sandy Oliver. "Clarifying Differences between Review Designs and Methods." Systematic Reviews 1 (2012): 1-9; Khan, Khalid S., Regina Kunz, Jos Kleijnen, and Gerd Antes. “Five Steps to Conducting a Systematic Review.” Journal of the Royal Society of Medicine 96 (2003): 118-121; Mulrow, C. D. “Systematic Reviews: Rationale for Systematic Reviews.” BMJ 309:597 (September 1994); O'Dwyer, Linda C., and Q. Eileen Wafford. "Addressing Challenges with Systematic Review Teams through Effective Communication: A Case Report." Journal of the Medical Library Association 109 (October 2021): 643-647; Okoli, Chitu, and Kira Schabram. "A Guide to Conducting a Systematic Literature Review of Information Systems Research." Sprouts: Working Papers on Information Systems 10 (2010); Siddaway, Andy P., Alex M. Wood, and Larry V. Hedges. "How to Do a Systematic Review: A Best Practice Guide for Conducting and Reporting Narrative Reviews, Meta-analyses, and Meta-syntheses." Annual Review of Psychology 70 (2019): 747-770; Torgerson, Carole J. “Publication Bias: The Achilles’ Heel of Systematic Reviews?” British Journal of Educational Studies 54 (March 2006): 89-102; Torgerson, Carole. Systematic Reviews . New York: Continuum, 2003.
- << Previous: Purpose of Guide
- Next: Design Flaws to Avoid >>
- Last Updated: Apr 1, 2024 9:56 AM
- URL: https://libguides.usc.edu/writingguide

What is Research? Definition, Types, Methods and Process
By Nick Jain
Published on: July 25, 2023
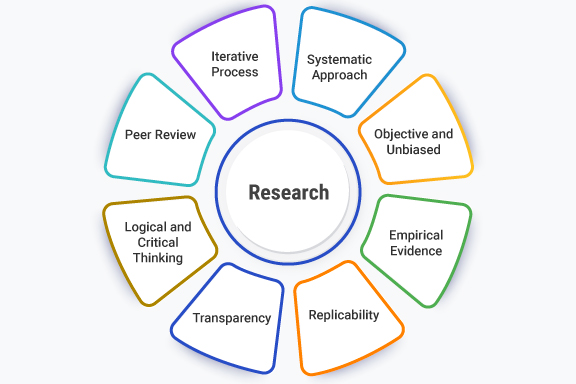
Table of Contents
What is Research?
Types of research methods, research process: how to conduct research, top 10 best practices for conducting research in 2023.
Research is defined as a meticulous and systematic inquiry process designed to explore and unravel specific subjects or issues with precision. This methodical approach encompasses the thorough collection, rigorous analysis, and insightful interpretation of information, aiming to delve deep into the nuances of a chosen field of study. By adhering to established research methodologies, investigators can draw meaningful conclusions, fostering a profound understanding that contributes significantly to the existing knowledge base. This dedication to systematic inquiry serves as the bedrock of progress, steering advancements across sciences, technology, social sciences, and diverse disciplines. Through the dissemination of meticulously gathered insights, scholars not only inspire collaboration and innovation but also catalyze positive societal change.
In the pursuit of knowledge, researchers embark on a journey of discovery, seeking to unravel the complexities of the world around us. By formulating clear research questions, researchers set the course for their investigations, carefully crafting methodologies to gather relevant data. Whether employing quantitative surveys or qualitative interviews, data collection lies at the heart of every research endeavor. Once the data is collected, researchers meticulously analyze it, employing statistical tools or thematic analysis to identify patterns and draw meaningful insights. These insights, often supported by empirical evidence, contribute to the collective pool of knowledge, enriching our understanding of various phenomena and guiding decision-making processes across diverse fields. Through research, we continually refine our understanding of the universe, laying the foundation for innovation and progress that shape the future.
Research embodies the spirit of curiosity and the pursuit of truth. Here are the key characteristics of research:
- Systematic Approach: Research follows a well-structured and organized approach, with clearly defined steps and methodologies. It is conducted in a systematic manner to ensure that data is collected, analyzed, and interpreted in a logical and coherent way.
- Objective and Unbiased: Research is objective and strives to be free from bias or personal opinions. Researchers aim to gather data and draw conclusions based on evidence rather than preconceived notions or beliefs.
- Empirical Evidence: Research relies on empirical evidence obtained through observations, experiments, surveys, or other data collection methods. This evidence serves as the foundation for drawing conclusions and making informed decisions.
- Clear Research Question or Problem: Every research study begins with a specific research question or problem that the researcher aims to address. This question provides focus and direction to the entire research process.
- Replicability: Good research should be replicable, meaning that other researchers should be able to conduct a similar study and obtain similar results when following the same methods.
- Transparency and Ethics: Research should be conducted with transparency, and researchers should adhere to ethical guidelines and principles. This includes obtaining informed consent from participants, ensuring confidentiality, and avoiding any harm to participants or the environment.
- Generalizability: Researchers often aim for their findings to be generalizable to a broader population or context. This means that the results of the study can be applied beyond the specific sample or situation studied.
- Logical and Critical Thinking: Research involves critical thinking to analyze and interpret data, identify patterns, and draw meaningful conclusions. Logical reasoning is essential in formulating hypotheses and designing the study.
- Contribution to Knowledge: The primary purpose of research is to contribute to the existing body of knowledge in a particular field. Researchers aim to expand understanding, challenge existing theories, or propose new ideas.
- Peer Review and Publication: Research findings are typically subject to peer review by experts in the field before being published in academic journals or presented at conferences. This process ensures the quality and validity of the research.
- Iterative Process: Research is often an iterative process, with findings from one study leading to new questions and further research. It is a continuous cycle of discovery and refinement.
- Practical Application: While some research is theoretical in nature, much of it aims to have practical applications and real-world implications. It can inform policy decisions, improve practices, or address societal challenges.
These key characteristics collectively define research as a rigorous and valuable endeavor that drives progress, knowledge, and innovation in various disciplines.
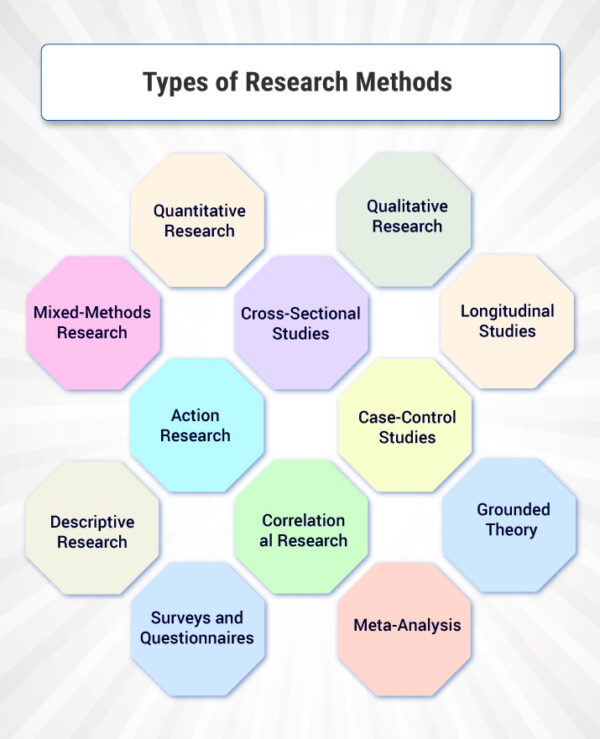
Research methods refer to the specific approaches and techniques used to collect and analyze data in a research study. There are various types of research methods, and researchers often choose the most appropriate method based on their research question, the nature of the data they want to collect, and the resources available to them. Some common types of research methods include:
1. Quantitative Research: Quantitative research methods focus on collecting and analyzing quantifiable data to draw conclusions. The key methods for conducting quantitative research are:
Surveys- Conducting structured questionnaires or interviews with a large number of participants to gather numerical data.
Experiments-Manipulating variables in a controlled environment to establish cause-and-effect relationships.
Observational Studies- Systematically observing and recording behaviors or phenomena without intervention.
Secondary Data Analysis- Analyzing existing datasets and records to draw new insights or conclusions.
2. Qualitative Research: Qualitative research employs a range of information-gathering methods that are non-numerical, and are instead intellectual in order to provide in-depth insights into the research topic. The key methods are:
Interviews- Conducting in-depth, semi-structured, or unstructured interviews to gain a deeper understanding of participants’ perspectives.
Focus Groups- Group discussions with selected participants to explore their attitudes, beliefs, and experiences on a specific topic.
Ethnography- Immersing in a particular culture or community to observe and understand their behaviors, customs, and beliefs.
Case Studies- In-depth examination of a single individual, group, organization, or event to gain comprehensive insights.
3. Mixed-Methods Research: Combining both quantitative and qualitative research methods in a single study to provide a more comprehensive understanding of the research question.
4. Cross-Sectional Studies: Gathering data from a sample of a population at a specific point in time to understand relationships or differences between variables.
5. Longitudinal Studies: Following a group of participants over an extended period to examine changes and developments over time.
6. Action Research: Collaboratively working with stakeholders to identify and implement solutions to practical problems in real-world settings.
7. Case-Control Studies: Comparing individuals with a particular outcome (cases) to those without the outcome (controls) to identify potential causes or risk factors.
8. Descriptive Research: Describing and summarizing characteristics, behaviors, or patterns without manipulating variables.
9. Correlational Research: Examining the relationship between two or more variables without inferring causation.
10. Grounded Theory: An approach to developing theory based on systematically gathering and analyzing data, allowing the theory to emerge from the data.
11. Surveys and Questionnaires: Administering structured sets of questions to a sample population to gather specific information.
12. Meta-Analysis: A statistical technique that combines the results of multiple studies on the same topic to draw more robust conclusions.
Researchers often choose a research method or a combination of methods that best aligns with their research objectives, resources, and the nature of the data they aim to collect. Each research method has its strengths and limitations, and the choice of method can significantly impact the findings and conclusions of a study.
Learn more: What is Research Design?
Conducting research involves a systematic and organized process that follows specific steps to ensure the collection of reliable and meaningful data. The research process typically consists of the following steps:
Step 1. Identify the Research Topic
Choose a research topic that interests you and aligns with your expertise and resources. Develop clear and focused research questions that you want to answer through your study.
Step 2. Review Existing Research
Conduct a thorough literature review to identify what research has already been done on your chosen topic. This will help you understand the current state of knowledge, identify gaps in the literature, and refine your research questions.
Step 3. Design the Research Methodology
Determine the appropriate research methodology that suits your research questions. Decide whether your study will be qualitative , quantitative , or a mix of both (mixed methods). Also, choose the data collection methods, such as surveys, interviews, experiments, observations, etc.
Step 4. Select the Sample and Participants
If your study involves human participants, decide on the sample size and selection criteria. Obtain ethical approval, if required, and ensure that participants’ rights and privacy are protected throughout the research process.
Step 5. Information Collection
Collect information and data based on your chosen research methodology. Qualitative research has more intellectual information, while quantitative research results are more data-oriented. Ensure that your data collection process is standardized and consistent to maintain the validity of the results.
Step 6. Data Analysis
Analyze the data you have collected using appropriate statistical or qualitative research methods . The type of analysis will depend on the nature of your data and research questions.
Step 7. Interpretation of Results
Interpret the findings of your data analysis. Relate the results to your research questions and consider how they contribute to the existing knowledge in the field.
Step 8. Draw Conclusions
Based on your interpretation of the results, draw meaningful conclusions that answer your research questions. Discuss the implications of your findings and how they align with the existing literature.
Step 9. Discuss Limitations
Acknowledge and discuss any limitations of your study. Addressing limitations demonstrates the validity and reliability of your research.
Step 10. Make Recommendations
If applicable, provide recommendations based on your research findings. These recommendations can be for future research, policy changes, or practical applications.
Step 11. Write the Research Report
Prepare a comprehensive research report detailing all aspects of your study, including the introduction, methodology, results, discussion, conclusion, and references.
Step 12. Peer Review and Revision
If you intend to publish your research, submit your report to peer-reviewed journals. Revise your research report based on the feedback received from reviewers.
Make sure to share your research findings with the broader community through conferences, seminars, or other appropriate channels, this will help contribute to the collective knowledge in your field of study.
Remember that conducting research is a dynamic process, and you may need to revisit and refine various steps as you progress. Good research requires attention to detail, critical thinking, and adherence to ethical principles to ensure the quality and validity of the study.
Learn more: What is Primary Market Research?
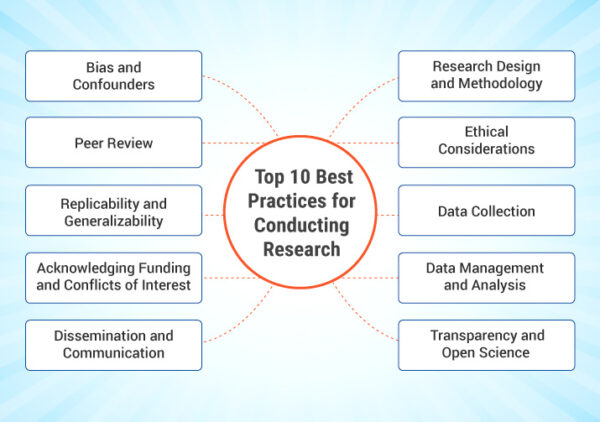
Best practices for conducting research remain rooted in the principles of rigor, transparency, and ethical considerations. Here are the essential best practices to follow when conducting research in 2023:
1. Research Design and Methodology
- Carefully select and justify the research design and methodology that aligns with your research questions and objectives.
- Ensure that the chosen methods are appropriate for the data you intend to collect and the type of analysis you plan to perform.
- Clearly document the research design and methodology to enhance the reproducibility and transparency of your study.
2. Ethical Considerations
- Obtain approval from relevant research ethics committees or institutional review boards, especially when involving human participants or sensitive data.
- Prioritize the protection of participants’ rights, privacy, and confidentiality throughout the research process.
- Provide informed consent to participants, ensuring they understand the study’s purpose, risks, and benefits.
3. Data Collection
- Ensure the reliability and validity of data collection instruments, such as surveys or interview protocols.
- Conduct pilot studies or pretests to identify and address any potential issues with data collection procedures.
4. Data Management and Analysis
- Implement robust data management practices to maintain the integrity and security of research data.
- Transparently document data analysis procedures, including software and statistical methods used.
- Use appropriate statistical techniques to analyze the data and avoid data manipulation or cherry-picking results.
5. Transparency and Open Science
- Embrace open science practices, such as pre-registration of research protocols and sharing data and code openly whenever possible.
- Clearly report all aspects of your research, including methods, results, and limitations, to enhance the reproducibility of your study.
6. Bias and Confounders
- Be aware of potential biases in the research process and take steps to minimize them.
- Consider and address potential confounding variables that could affect the validity of your results.
7. Peer Review
- Seek peer review from experts in your field before publishing or presenting your research findings.
- Be receptive to feedback and address any concerns raised by reviewers to improve the quality of your study.
8. Replicability and Generalizability
- Strive to make your research findings replicable, allowing other researchers to validate your results independently.
- Clearly state the limitations of your study and the extent to which the findings can be generalized to other populations or contexts.
9. Acknowledging Funding and Conflicts of Interest
- Disclose any funding sources and potential conflicts of interest that may influence your research or its outcomes.
10. Dissemination and Communication
- Effectively communicate your research findings to both academic and non-academic audiences using clear and accessible language.
- Share your research through reputable and open-access platforms to maximize its impact and reach.
By adhering to these best practices, researchers can ensure the integrity and value of their work, contributing to the advancement of knowledge and promoting trust in the research community.
Learn more: What is Consumer Research?
Enhance Your Research
Collect feedback and conduct research with IdeaScale’s award-winning software
Elevate Research And Feedback With Your IdeaScale Community!
IdeaScale is an innovation management solution that inspires people to take action on their ideas. Your community’s ideas can change lives, your business and the world. Connect to the ideas that matter and start co-creating the future.
Copyright © 2024 IdeaScale
Privacy Overview

Research Basics
- What Is Research?
- Types of Research
- Secondary Research | Literature Review
- Developing Your Topic
- Primary vs. Secondary Sources
- Evaluating Sources
- Responsible Conduct of Research
- Additional Help
When most people think of research, they usually think of what’s known as primary research or bench research—research that is conducted in a laboratory to discover new things. While this is an important part of research, it is only a small part. The majority of research consists of what's called secondary research. Research also breaks down along other lines besides just primary or secondary.
How Many Types of Research Are There?
In addition to primary/secondary, research is usually categorized as quantitative/qualitative, descriptive/analytical, or basic/applied. Because there are many subtle differences between how different disciplines conduct research, the following table provides only a brief summary of these concepts.
There are, however, many other types of research, often used only in certain narrow fields of research. Further complicating things, many of the types overlap, go by different names depending on the subject area, or are differentiated only by very subtle differences. For more detailed explanations of the types of research commonly used in your field, please consult references related to research in your specific subject area.
Because secondary research is so widely used, even by non-researchers, and because its practice is relatively consistent between disciplines, we will cover it in more detail on other pages of this guide.

- << Previous: What Is Research?
- Next: Secondary Research | Literature Review >>
- Last Updated: Dec 21, 2023 3:49 PM
- URL: https://guides.library.iit.edu/research_basics

An official website of the United States government
The .gov means it’s official. Federal government websites often end in .gov or .mil. Before sharing sensitive information, make sure you’re on a federal government site.
The site is secure. The https:// ensures that you are connecting to the official website and that any information you provide is encrypted and transmitted securely.
- Publications
- Account settings
Preview improvements coming to the PMC website in October 2024. Learn More or Try it out now .
- Advanced Search
- Journal List
- Indian J Anaesth
- v.60(9); 2016 Sep
Types of studies and research design
Mukul chandra kapoor.
Department of Anesthesiology, Max Smart Super Specialty Hospital, New Delhi, India
Medical research has evolved, from individual expert described opinions and techniques, to scientifically designed methodology-based studies. Evidence-based medicine (EBM) was established to re-evaluate medical facts and remove various myths in clinical practice. Research methodology is now protocol based with predefined steps. Studies were classified based on the method of collection and evaluation of data. Clinical study methodology now needs to comply to strict ethical, moral, truth, and transparency standards, ensuring that no conflict of interest is involved. A medical research pyramid has been designed to grade the quality of evidence and help physicians determine the value of the research. Randomised controlled trials (RCTs) have become gold standards for quality research. EBM now scales systemic reviews and meta-analyses at a level higher than RCTs to overcome deficiencies in the randomised trials due to errors in methodology and analyses.
INTRODUCTION
Expert opinion, experience, and authoritarian judgement were the norm in clinical medical practice. At scientific meetings, one often heard senior professionals emphatically expressing ‘In my experience,…… what I have said is correct!’ In 1981, articles published by Sackett et al . introduced ‘critical appraisal’ as they felt a need to teach methods of understanding scientific literature and its application at the bedside.[ 1 ] To improve clinical outcomes, clinical expertise must be complemented by the best external evidence.[ 2 ] Conversely, without clinical expertise, good external evidence may be used inappropriately [ Figure 1 ]. Practice gets outdated, if not updated with current evidence, depriving the clientele of the best available therapy.

Triad of evidence-based medicine
EVIDENCE-BASED MEDICINE
In 1971, in his book ‘Effectiveness and Efficiency’, Archibald Cochrane highlighted the lack of reliable evidence behind many accepted health-care interventions.[ 3 ] This triggered re-evaluation of many established ‘supposed’ scientific facts and awakened physicians to the need for evidence in medicine. Evidence-based medicine (EBM) thus evolved, which was defined as ‘the conscientious, explicit and judicious use of the current best evidence in making decisions about the care of individual patients.’[ 2 ]
The goal of EBM was scientific endowment to achieve consistency, efficiency, effectiveness, quality, safety, reduction in dilemma and limitation of idiosyncrasies in clinical practice.[ 4 ] EBM required the physician to diligently assess the therapy, make clinical adjustments using the best available external evidence, ensure awareness of current research and discover clinical pathways to ensure best patient outcomes.[ 5 ]
With widespread internet use, phenomenally large number of publications, training and media resources are available but determining the quality of this literature is difficult for a busy physician. Abstracts are available freely on the internet, but full-text articles require a subscription. To complicate issues, contradictory studies are published making decision-making difficult.[ 6 ] Publication bias, especially against negative studies, makes matters worse.
In 1993, the Cochrane Collaboration was founded by Ian Chalmers and others to create and disseminate up-to-date review of randomised controlled trials (RCTs) to help health-care professionals make informed decisions.[ 7 ] In 1995, the American College of Physicians and the British Medical Journal Publishing Group collaborated to publish the journal ‘Evidence-based medicine’, leading to the evolution of EBM in all spheres of medicine.
MEDICAL RESEARCH
Medical research needs to be conducted to increase knowledge about the human species, its social/natural environment and to combat disease/infirmity in humans. Research should be conducted in a manner conducive to and consistent with dignity and well-being of the participant; in a professional and transparent manner; and ensuring minimal risk.[ 8 ] Research thus must be subjected to careful evaluation at all stages, i.e., research design/experimentation; results and their implications; the objective of the research sought; anticipated benefits/dangers; potential uses/abuses of the experiment and its results; and on ensuring the safety of human life. Table 1 lists the principles any research should follow.[ 8 ]
General principles of medical research

Types of study design
Medical research is classified into primary and secondary research. Clinical/experimental studies are performed in primary research, whereas secondary research consolidates available studies as reviews, systematic reviews and meta-analyses. Three main areas in primary research are basic medical research, clinical research and epidemiological research [ Figure 2 ]. Basic research includes fundamental research in fields shown in Figure 2 . In almost all studies, at least one independent variable is varied, whereas the effects on the dependent variables are investigated. Clinical studies include observational studies and interventional studies and are subclassified as in Figure 2 .

Classification of types of medical research
Interventional clinical study is performed with the purpose of studying or demonstrating clinical or pharmacological properties of drugs/devices, their side effects and to establish their efficacy or safety. They also include studies in which surgical, physical or psychotherapeutic procedures are examined.[ 9 ] Studies on drugs/devices are subject to legal and ethical requirements including the Drug Controller General India (DCGI) directives. They require the approval of DCGI recognized Ethics Committee and must be performed in accordance with the rules of ‘Good Clinical Practice’.[ 10 ] Further details are available under ‘Methodology for research II’ section in this issue of IJA. In 2004, the World Health Organization advised registration of all clinical trials in a public registry. In India, the Clinical Trials Registry of India was launched in 2007 ( www.ctri.nic.in ). The International Committee of Medical Journal Editors (ICMJE) mandates its member journals to publish only registered trials.[ 11 ]
Observational clinical study is a study in which knowledge from treatment of persons with drugs is analysed using epidemiological methods. In these studies, the diagnosis, treatment and monitoring are performed exclusively according to medical practice and not according to a specified study protocol.[ 9 ] They are subclassified as per Figure 2 .
Epidemiological studies have two basic approaches, the interventional and observational. Clinicians are more familiar with interventional research, whereas epidemiologists usually perform observational research.
Interventional studies are experimental in character and are subdivided into field and group studies, for example, iodine supplementation of cooking salt to prevent hypothyroidism. Many interventions are unsuitable for RCTs, as the exposure may be harmful to the subjects.
Observational studies can be subdivided into cohort, case–control, cross-sectional and ecological studies.
- Cohort studies are suited to detect connections between exposure and development of disease. They are normally prospective studies of two healthy groups of subjects observed over time, in which one group is exposed to a specific substance, whereas the other is not. The occurrence of the disease can be determined in the two groups. Cohort studies can also be retrospective
- Case–control studies are retrospective analyses performed to establish the prevalence of a disease in two groups exposed to a factor or disease. The incidence rate cannot be calculated, and there is also a risk of selection bias and faulty recall.
Secondary research
Narrative review.
An expert senior author writes about a particular field, condition or treatment, including an overview, and this information is fortified by his experience. The article is in a narrative format. Its limitation is that one cannot tell whether recommendations are based on author's clinical experience, available literature and why some studies were given more emphasis. It can be biased, with selective citation of reports that reinforce the authors' views of a topic.[ 12 ]
Systematic review
Systematic reviews methodically and comprehensively identify studies focused on a specified topic, appraise their methodology, summate the results, identify key findings and reasons for differences across studies, and cite limitations of current knowledge.[ 13 ] They adhere to reproducible methods and recommended guidelines.[ 14 ] The methods used to compile data are explicit and transparent, allowing the reader to gauge the quality of the review and the potential for bias.[ 15 ]
A systematic review can be presented in text or graphic form. In graphic form, data of different trials can be plotted with the point estimate and 95% confidence interval for each study, presented on an individual line. A properly conducted systematic review presents the best available research evidence for a focused clinical question. The review team may obtain information, not available in the original reports, from the primary authors. This ensures that findings are consistent and generalisable across populations, environment, therapies and groups.[ 12 ] A systematic review attempts to reduce bias identification and studies selection for review, using a comprehensive search strategy and specifying inclusion criteria. The strength of a systematic review lies in the transparency of each phase and highlighting the merits of each decision made, while compiling information.
Meta-analysis
A review team compiles aggregate-level data in each primary study, and in some cases, data are solicited from each of the primary studies.[ 16 , 17 ] Although difficult to perform, individual patient meta-analyses offer advantages over aggregate-level analyses.[ 18 ] These mathematically pooled results are referred to as meta-analysis. Combining data from well-conducted primary studies provide a precise estimate of the “true effect.”[ 19 ] Pooling the samples of individual studies increases overall sample size, enhances statistical analysis power, reduces confidence interval and thereby improves statistical value.
The structured process of Cochrane Collaboration systematic reviews has contributed to the improvement of their quality. For the meta-analysis to be definitive, the primary RCTs should have been conducted methodically. When the existing studies have important scientific and methodological limitations, such as smaller sized samples, the systematic review may identify where gaps exist in the available literature.[ 20 ] RCTs and systematic review of several randomised trials are less likely to mislead us, and thereby help judge whether an intervention is better.[ 2 ] Practice guidelines supported by large RCTs and meta-analyses are considered as ‘gold standard’ in EBM. This issue of IJA is accompanied by an editorial on Importance of EBM on research and practice (Guyat and Sriganesh 471_16).[ 21 ] The EBM pyramid grading the value of different types of research studies is shown in Figure 3 .

The evidence-based medicine pyramid
In the last decade, a number of studies and guidelines brought about path-breaking changes in anaesthesiology and critical care. Some guidelines such as the ‘Surviving Sepsis Guidelines-2004’[ 22 ] were later found to be flawed and biased. A number of large RCTs were rejected as their findings were erroneous. Another classic example is that of ENIGMA-I (Evaluation of Nitrous oxide In the Gas Mixture for Anaesthesia)[ 23 ] which implicated nitrous oxide for poor outcomes, but ENIGMA-II[ 24 , 25 ] conducted later, by the same investigators, declared it as safe. The rise and fall of the ‘tight glucose control’ regimen was similar.[ 26 ]
Although RCTs are considered ‘gold standard’ in research, their status is at crossroads today. RCTs have conflicting interests and thus must be evaluated with careful scrutiny. EBM can promote evidence reflected in RCTs and meta-analyses. However, it cannot promulgate evidence not reflected in RCTs. Flawed RCTs and meta-analyses may bring forth erroneous recommendations. EBM thus should not be restricted to RCTs and meta-analyses but must involve tracking down the best external evidence to answer our clinical questions.
Financial support and sponsorship
Conflicts of interest.
There are no conflicts of interest.
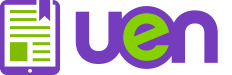
The Two Main Research Types – A Brief Overview
In this module, we will have a short overview of the two main types of research before we dive further into learning more about each in detail.
Content includes:
- Qualitative methods brief introductory overview
- Quantitative methods brief introductory overview
Objectives:
- Identify the three main types of qualitative research.
- Describe the processes of inductive versus deductive reasoning, and the types of research for both.
- Distinguish quantitative experimental and nonexperimental research.
Here we go! We are now going to start learning a bit about research. Remember, research is the underpinning for EBP. Research provides the evidence, and EBP takes that evidence and embeds it into practice to improve clinical outcomes.
The two major classes of research are:
- Qualitative Research – subjective, seeks a human’s experience as a narrative
- Quantitative Research – objective, seeks to statistically make inferences about a sample to generalize to the larger population
We need to have a solid understanding of the difference between the two main types of research before we study the nuances of each.
Three Main Types of Qualitative Research
Qualitative Research : Qualitative research is rooted in research that originated in anthropology, sociology, and psychology. Qualitative research is not experimental, it seeks to understand the lived experiences in humans and seeks to understanding meaning, and it is subjective in nature. The overarching goal of qualitative research is theory-generating. It is an inductive process (inductive reasoning). Most often, qualitative research features an interview style. This allows the researcher to ask open-ended questions and the participants share their experiences and/or explanation of particular meanings in life.
Qualitative research differs from quantitative research in that:
- It is completely subjective .
- It utilizes an inductive (versus deductive) approach.
- It does not utilize a hypothesis.
- It generates a theory from the data to explain the social phenomenon that the researchers were interested in.
- The researcher is involved with the participants for data collection.
- The data is analyzed with a thematic nature. That is, themes from the collected narratives are analyzed to see trends or themes in what the participants shared.
- The results are not generalizable to the population.
There are three types of qualitative research designs:
Inductive versus Deductive Reasoning
The main difference between inductive and deductive reasoning is that inductive reasoning aims at developing a theory while deductive reasoning aims at testing an existing theory.
Think of inductive (theory producing) as to qualitative research and deductive (theory testing) as to quantitative research.
Inductive reasoning moves from specific observations to broad generalizations, and deductive reasoning the other way around.
Both approaches are used in various types of research, and it’s not uncommon to combine them in one large study.
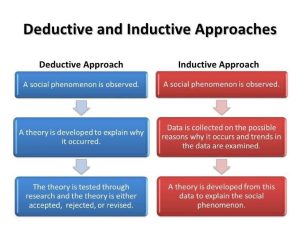
Here is a qualitative study in which the researchers conducted interviews in order to obtain the subjective perspectives of the participants.
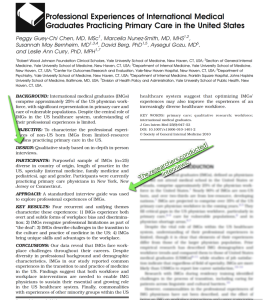
Quantitative Experimental and Nonexperimental Research.
Quantitative Research: In quantitative research, the goal is to utilize the statistical data to generalize results to the population studied. Some key features include utilizing the statistics to help answer the clinical question and determine whether the hypothesis is indeed statistically supported.
There are two main types of quantitative research:
- Experimental : In experimental research, the researcher introduces an intervention or treatment.
- Non-Experimental : In non-experimental research, the researcher does not introduce an intervention or treatment, but instead acts as a bystander. Meaning, they collect data without introducing a treatment.
We will explore those two types in much detail in the next module.
Quantitative research differs from qualitative research in that:
- It is completely objective .
- It utilizes a deductive (versus inductive) approach.
- It utilizes a hypothesis(es).
- It tests a theory.
- The researcher is usually not directly involved with the participants for data collection in order to minimize bias.
- The data is analyzed statistically in order to generalize results to the larger population.
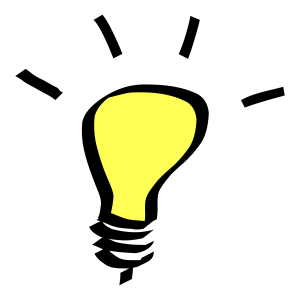
Experimental Research : In the following article, the researchers introduced an intervention, which was a “Program for Enhancing the Positive Aspects of Caregiving” (a particular education program).
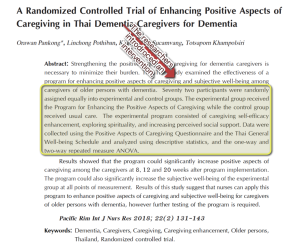
Non-experimental Research : In the following article, the researchers did not introduce an intervention or treatment. They handed out surveys for the participants to complete about their activity and depression levels.
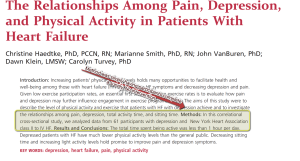
Video: Qualitative Types and Experimental/Nonexperimental Research
In summary, there are two main approaches to research designs: Quantitative and qualitative. They each seek to answer questions, but quantitative research is meant to generalize its findings to the population whereas qualitative research seeks to understand phenomenon and develop theories about the human lived experiences.
References & Attribution
“ Light bulb doodle ” by rawpixel licensed CC0
“ Orange flame ” by rawpixel licensed CC0 .
Chen, P., Nunez-Smith, M., Bernheim, S… (2010). Professional experiences of international medical graduates practicing primary care in the United States. Journal of General Internal Medicine, 25 (9), 947-53.
Haedtke, C., Smith, M., VanBuren, J., Kein, D., Turvey, C. (2017). The relationships among pain, depression, and physical activity in patients with heart failure. Journal of Cardiovascular Nursing, 32 (5), E21-E25.
Pankong, O., Pothiban, L., Sucamvang, K., Khampolsiri, T. (2018). A randomized controlled trial of enhancing positive aspects of caregiving in Thai dementia caregivers for dementia. Pacific Rim Internal Journal of Nursing Res, 22 (2), 131-143.
Evidence-Based Practice & Research Methodologies Copyright © by Tracy Fawns is licensed under a Creative Commons Attribution-NonCommercial-ShareAlike 4.0 International License , except where otherwise noted.
Share This Book
- Privacy Policy
Buy Me a Coffee

Home » Research Report – Example, Writing Guide and Types
Research Report – Example, Writing Guide and Types
Table of Contents

Research Report
Definition:
Research Report is a written document that presents the results of a research project or study, including the research question, methodology, results, and conclusions, in a clear and objective manner.
The purpose of a research report is to communicate the findings of the research to the intended audience, which could be other researchers, stakeholders, or the general public.
Components of Research Report
Components of Research Report are as follows:
Introduction
The introduction sets the stage for the research report and provides a brief overview of the research question or problem being investigated. It should include a clear statement of the purpose of the study and its significance or relevance to the field of research. It may also provide background information or a literature review to help contextualize the research.
Literature Review
The literature review provides a critical analysis and synthesis of the existing research and scholarship relevant to the research question or problem. It should identify the gaps, inconsistencies, and contradictions in the literature and show how the current study addresses these issues. The literature review also establishes the theoretical framework or conceptual model that guides the research.
Methodology
The methodology section describes the research design, methods, and procedures used to collect and analyze data. It should include information on the sample or participants, data collection instruments, data collection procedures, and data analysis techniques. The methodology should be clear and detailed enough to allow other researchers to replicate the study.
The results section presents the findings of the study in a clear and objective manner. It should provide a detailed description of the data and statistics used to answer the research question or test the hypothesis. Tables, graphs, and figures may be included to help visualize the data and illustrate the key findings.
The discussion section interprets the results of the study and explains their significance or relevance to the research question or problem. It should also compare the current findings with those of previous studies and identify the implications for future research or practice. The discussion should be based on the results presented in the previous section and should avoid speculation or unfounded conclusions.
The conclusion summarizes the key findings of the study and restates the main argument or thesis presented in the introduction. It should also provide a brief overview of the contributions of the study to the field of research and the implications for practice or policy.
The references section lists all the sources cited in the research report, following a specific citation style, such as APA or MLA.
The appendices section includes any additional material, such as data tables, figures, or instruments used in the study, that could not be included in the main text due to space limitations.
Types of Research Report
Types of Research Report are as follows:
Thesis is a type of research report. A thesis is a long-form research document that presents the findings and conclusions of an original research study conducted by a student as part of a graduate or postgraduate program. It is typically written by a student pursuing a higher degree, such as a Master’s or Doctoral degree, although it can also be written by researchers or scholars in other fields.
Research Paper
Research paper is a type of research report. A research paper is a document that presents the results of a research study or investigation. Research papers can be written in a variety of fields, including science, social science, humanities, and business. They typically follow a standard format that includes an introduction, literature review, methodology, results, discussion, and conclusion sections.
Technical Report
A technical report is a detailed report that provides information about a specific technical or scientific problem or project. Technical reports are often used in engineering, science, and other technical fields to document research and development work.
Progress Report
A progress report provides an update on the progress of a research project or program over a specific period of time. Progress reports are typically used to communicate the status of a project to stakeholders, funders, or project managers.
Feasibility Report
A feasibility report assesses the feasibility of a proposed project or plan, providing an analysis of the potential risks, benefits, and costs associated with the project. Feasibility reports are often used in business, engineering, and other fields to determine the viability of a project before it is undertaken.
Field Report
A field report documents observations and findings from fieldwork, which is research conducted in the natural environment or setting. Field reports are often used in anthropology, ecology, and other social and natural sciences.
Experimental Report
An experimental report documents the results of a scientific experiment, including the hypothesis, methods, results, and conclusions. Experimental reports are often used in biology, chemistry, and other sciences to communicate the results of laboratory experiments.
Case Study Report
A case study report provides an in-depth analysis of a specific case or situation, often used in psychology, social work, and other fields to document and understand complex cases or phenomena.
Literature Review Report
A literature review report synthesizes and summarizes existing research on a specific topic, providing an overview of the current state of knowledge on the subject. Literature review reports are often used in social sciences, education, and other fields to identify gaps in the literature and guide future research.
Research Report Example
Following is a Research Report Example sample for Students:
Title: The Impact of Social Media on Academic Performance among High School Students
This study aims to investigate the relationship between social media use and academic performance among high school students. The study utilized a quantitative research design, which involved a survey questionnaire administered to a sample of 200 high school students. The findings indicate that there is a negative correlation between social media use and academic performance, suggesting that excessive social media use can lead to poor academic performance among high school students. The results of this study have important implications for educators, parents, and policymakers, as they highlight the need for strategies that can help students balance their social media use and academic responsibilities.
Introduction:
Social media has become an integral part of the lives of high school students. With the widespread use of social media platforms such as Facebook, Twitter, Instagram, and Snapchat, students can connect with friends, share photos and videos, and engage in discussions on a range of topics. While social media offers many benefits, concerns have been raised about its impact on academic performance. Many studies have found a negative correlation between social media use and academic performance among high school students (Kirschner & Karpinski, 2010; Paul, Baker, & Cochran, 2012).
Given the growing importance of social media in the lives of high school students, it is important to investigate its impact on academic performance. This study aims to address this gap by examining the relationship between social media use and academic performance among high school students.
Methodology:
The study utilized a quantitative research design, which involved a survey questionnaire administered to a sample of 200 high school students. The questionnaire was developed based on previous studies and was designed to measure the frequency and duration of social media use, as well as academic performance.
The participants were selected using a convenience sampling technique, and the survey questionnaire was distributed in the classroom during regular school hours. The data collected were analyzed using descriptive statistics and correlation analysis.
The findings indicate that the majority of high school students use social media platforms on a daily basis, with Facebook being the most popular platform. The results also show a negative correlation between social media use and academic performance, suggesting that excessive social media use can lead to poor academic performance among high school students.
Discussion:
The results of this study have important implications for educators, parents, and policymakers. The negative correlation between social media use and academic performance suggests that strategies should be put in place to help students balance their social media use and academic responsibilities. For example, educators could incorporate social media into their teaching strategies to engage students and enhance learning. Parents could limit their children’s social media use and encourage them to prioritize their academic responsibilities. Policymakers could develop guidelines and policies to regulate social media use among high school students.
Conclusion:
In conclusion, this study provides evidence of the negative impact of social media on academic performance among high school students. The findings highlight the need for strategies that can help students balance their social media use and academic responsibilities. Further research is needed to explore the specific mechanisms by which social media use affects academic performance and to develop effective strategies for addressing this issue.
Limitations:
One limitation of this study is the use of convenience sampling, which limits the generalizability of the findings to other populations. Future studies should use random sampling techniques to increase the representativeness of the sample. Another limitation is the use of self-reported measures, which may be subject to social desirability bias. Future studies could use objective measures of social media use and academic performance, such as tracking software and school records.
Implications:
The findings of this study have important implications for educators, parents, and policymakers. Educators could incorporate social media into their teaching strategies to engage students and enhance learning. For example, teachers could use social media platforms to share relevant educational resources and facilitate online discussions. Parents could limit their children’s social media use and encourage them to prioritize their academic responsibilities. They could also engage in open communication with their children to understand their social media use and its impact on their academic performance. Policymakers could develop guidelines and policies to regulate social media use among high school students. For example, schools could implement social media policies that restrict access during class time and encourage responsible use.
References:
- Kirschner, P. A., & Karpinski, A. C. (2010). Facebook® and academic performance. Computers in Human Behavior, 26(6), 1237-1245.
- Paul, J. A., Baker, H. M., & Cochran, J. D. (2012). Effect of online social networking on student academic performance. Journal of the Research Center for Educational Technology, 8(1), 1-19.
- Pantic, I. (2014). Online social networking and mental health. Cyberpsychology, Behavior, and Social Networking, 17(10), 652-657.
- Rosen, L. D., Carrier, L. M., & Cheever, N. A. (2013). Facebook and texting made me do it: Media-induced task-switching while studying. Computers in Human Behavior, 29(3), 948-958.
Note*: Above mention, Example is just a sample for the students’ guide. Do not directly copy and paste as your College or University assignment. Kindly do some research and Write your own.
Applications of Research Report
Research reports have many applications, including:
- Communicating research findings: The primary application of a research report is to communicate the results of a study to other researchers, stakeholders, or the general public. The report serves as a way to share new knowledge, insights, and discoveries with others in the field.
- Informing policy and practice : Research reports can inform policy and practice by providing evidence-based recommendations for decision-makers. For example, a research report on the effectiveness of a new drug could inform regulatory agencies in their decision-making process.
- Supporting further research: Research reports can provide a foundation for further research in a particular area. Other researchers may use the findings and methodology of a report to develop new research questions or to build on existing research.
- Evaluating programs and interventions : Research reports can be used to evaluate the effectiveness of programs and interventions in achieving their intended outcomes. For example, a research report on a new educational program could provide evidence of its impact on student performance.
- Demonstrating impact : Research reports can be used to demonstrate the impact of research funding or to evaluate the success of research projects. By presenting the findings and outcomes of a study, research reports can show the value of research to funders and stakeholders.
- Enhancing professional development : Research reports can be used to enhance professional development by providing a source of information and learning for researchers and practitioners in a particular field. For example, a research report on a new teaching methodology could provide insights and ideas for educators to incorporate into their own practice.
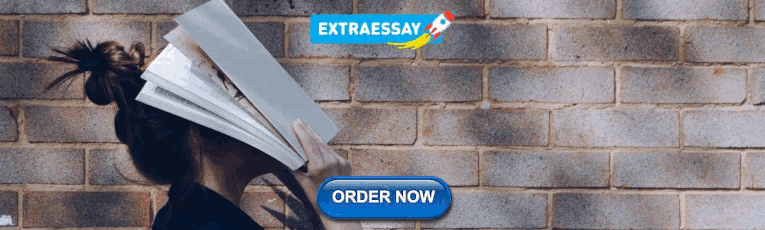
How to write Research Report
Here are some steps you can follow to write a research report:
- Identify the research question: The first step in writing a research report is to identify your research question. This will help you focus your research and organize your findings.
- Conduct research : Once you have identified your research question, you will need to conduct research to gather relevant data and information. This can involve conducting experiments, reviewing literature, or analyzing data.
- Organize your findings: Once you have gathered all of your data, you will need to organize your findings in a way that is clear and understandable. This can involve creating tables, graphs, or charts to illustrate your results.
- Write the report: Once you have organized your findings, you can begin writing the report. Start with an introduction that provides background information and explains the purpose of your research. Next, provide a detailed description of your research methods and findings. Finally, summarize your results and draw conclusions based on your findings.
- Proofread and edit: After you have written your report, be sure to proofread and edit it carefully. Check for grammar and spelling errors, and make sure that your report is well-organized and easy to read.
- Include a reference list: Be sure to include a list of references that you used in your research. This will give credit to your sources and allow readers to further explore the topic if they choose.
- Format your report: Finally, format your report according to the guidelines provided by your instructor or organization. This may include formatting requirements for headings, margins, fonts, and spacing.
Purpose of Research Report
The purpose of a research report is to communicate the results of a research study to a specific audience, such as peers in the same field, stakeholders, or the general public. The report provides a detailed description of the research methods, findings, and conclusions.
Some common purposes of a research report include:
- Sharing knowledge: A research report allows researchers to share their findings and knowledge with others in their field. This helps to advance the field and improve the understanding of a particular topic.
- Identifying trends: A research report can identify trends and patterns in data, which can help guide future research and inform decision-making.
- Addressing problems: A research report can provide insights into problems or issues and suggest solutions or recommendations for addressing them.
- Evaluating programs or interventions : A research report can evaluate the effectiveness of programs or interventions, which can inform decision-making about whether to continue, modify, or discontinue them.
- Meeting regulatory requirements: In some fields, research reports are required to meet regulatory requirements, such as in the case of drug trials or environmental impact studies.
When to Write Research Report
A research report should be written after completing the research study. This includes collecting data, analyzing the results, and drawing conclusions based on the findings. Once the research is complete, the report should be written in a timely manner while the information is still fresh in the researcher’s mind.
In academic settings, research reports are often required as part of coursework or as part of a thesis or dissertation. In this case, the report should be written according to the guidelines provided by the instructor or institution.
In other settings, such as in industry or government, research reports may be required to inform decision-making or to comply with regulatory requirements. In these cases, the report should be written as soon as possible after the research is completed in order to inform decision-making in a timely manner.
Overall, the timing of when to write a research report depends on the purpose of the research, the expectations of the audience, and any regulatory requirements that need to be met. However, it is important to complete the report in a timely manner while the information is still fresh in the researcher’s mind.
Characteristics of Research Report
There are several characteristics of a research report that distinguish it from other types of writing. These characteristics include:
- Objective: A research report should be written in an objective and unbiased manner. It should present the facts and findings of the research study without any personal opinions or biases.
- Systematic: A research report should be written in a systematic manner. It should follow a clear and logical structure, and the information should be presented in a way that is easy to understand and follow.
- Detailed: A research report should be detailed and comprehensive. It should provide a thorough description of the research methods, results, and conclusions.
- Accurate : A research report should be accurate and based on sound research methods. The findings and conclusions should be supported by data and evidence.
- Organized: A research report should be well-organized. It should include headings and subheadings to help the reader navigate the report and understand the main points.
- Clear and concise: A research report should be written in clear and concise language. The information should be presented in a way that is easy to understand, and unnecessary jargon should be avoided.
- Citations and references: A research report should include citations and references to support the findings and conclusions. This helps to give credit to other researchers and to provide readers with the opportunity to further explore the topic.
Advantages of Research Report
Research reports have several advantages, including:
- Communicating research findings: Research reports allow researchers to communicate their findings to a wider audience, including other researchers, stakeholders, and the general public. This helps to disseminate knowledge and advance the understanding of a particular topic.
- Providing evidence for decision-making : Research reports can provide evidence to inform decision-making, such as in the case of policy-making, program planning, or product development. The findings and conclusions can help guide decisions and improve outcomes.
- Supporting further research: Research reports can provide a foundation for further research on a particular topic. Other researchers can build on the findings and conclusions of the report, which can lead to further discoveries and advancements in the field.
- Demonstrating expertise: Research reports can demonstrate the expertise of the researchers and their ability to conduct rigorous and high-quality research. This can be important for securing funding, promotions, and other professional opportunities.
- Meeting regulatory requirements: In some fields, research reports are required to meet regulatory requirements, such as in the case of drug trials or environmental impact studies. Producing a high-quality research report can help ensure compliance with these requirements.
Limitations of Research Report
Despite their advantages, research reports also have some limitations, including:
- Time-consuming: Conducting research and writing a report can be a time-consuming process, particularly for large-scale studies. This can limit the frequency and speed of producing research reports.
- Expensive: Conducting research and producing a report can be expensive, particularly for studies that require specialized equipment, personnel, or data. This can limit the scope and feasibility of some research studies.
- Limited generalizability: Research studies often focus on a specific population or context, which can limit the generalizability of the findings to other populations or contexts.
- Potential bias : Researchers may have biases or conflicts of interest that can influence the findings and conclusions of the research study. Additionally, participants may also have biases or may not be representative of the larger population, which can limit the validity and reliability of the findings.
- Accessibility: Research reports may be written in technical or academic language, which can limit their accessibility to a wider audience. Additionally, some research may be behind paywalls or require specialized access, which can limit the ability of others to read and use the findings.
About the author
Muhammad Hassan
Researcher, Academic Writer, Web developer
You may also like

Data Collection – Methods Types and Examples

Delimitations in Research – Types, Examples and...

Research Process – Steps, Examples and Tips

Research Design – Types, Methods and Examples

Institutional Review Board – Application Sample...

Evaluating Research – Process, Examples and...
Have a language expert improve your writing
Run a free plagiarism check in 10 minutes, generate accurate citations for free.
- Knowledge Base
Methodology
- What Is Qualitative Research? | Methods & Examples
What Is Qualitative Research? | Methods & Examples
Published on June 19, 2020 by Pritha Bhandari . Revised on June 22, 2023.
Qualitative research involves collecting and analyzing non-numerical data (e.g., text, video, or audio) to understand concepts, opinions, or experiences. It can be used to gather in-depth insights into a problem or generate new ideas for research.
Qualitative research is the opposite of quantitative research , which involves collecting and analyzing numerical data for statistical analysis.
Qualitative research is commonly used in the humanities and social sciences, in subjects such as anthropology, sociology, education, health sciences, history, etc.
- How does social media shape body image in teenagers?
- How do children and adults interpret healthy eating in the UK?
- What factors influence employee retention in a large organization?
- How is anxiety experienced around the world?
- How can teachers integrate social issues into science curriculums?
Table of contents
Approaches to qualitative research, qualitative research methods, qualitative data analysis, advantages of qualitative research, disadvantages of qualitative research, other interesting articles, frequently asked questions about qualitative research.
Qualitative research is used to understand how people experience the world. While there are many approaches to qualitative research, they tend to be flexible and focus on retaining rich meaning when interpreting data.
Common approaches include grounded theory, ethnography , action research , phenomenological research, and narrative research. They share some similarities, but emphasize different aims and perspectives.
Note that qualitative research is at risk for certain research biases including the Hawthorne effect , observer bias , recall bias , and social desirability bias . While not always totally avoidable, awareness of potential biases as you collect and analyze your data can prevent them from impacting your work too much.
Receive feedback on language, structure, and formatting
Professional editors proofread and edit your paper by focusing on:
- Academic style
- Vague sentences
- Style consistency
See an example
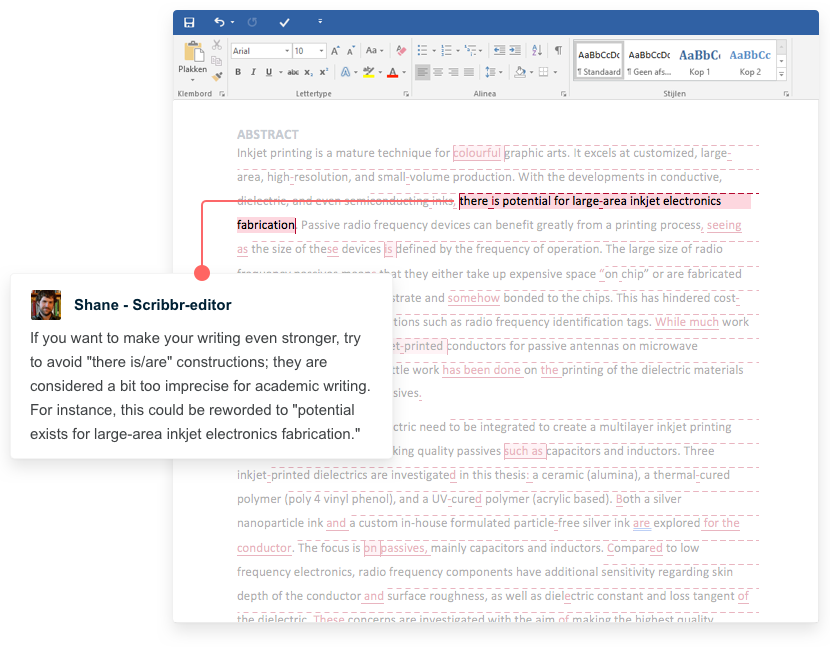
Each of the research approaches involve using one or more data collection methods . These are some of the most common qualitative methods:
- Observations: recording what you have seen, heard, or encountered in detailed field notes.
- Interviews: personally asking people questions in one-on-one conversations.
- Focus groups: asking questions and generating discussion among a group of people.
- Surveys : distributing questionnaires with open-ended questions.
- Secondary research: collecting existing data in the form of texts, images, audio or video recordings, etc.
- You take field notes with observations and reflect on your own experiences of the company culture.
- You distribute open-ended surveys to employees across all the company’s offices by email to find out if the culture varies across locations.
- You conduct in-depth interviews with employees in your office to learn about their experiences and perspectives in greater detail.
Qualitative researchers often consider themselves “instruments” in research because all observations, interpretations and analyses are filtered through their own personal lens.
For this reason, when writing up your methodology for qualitative research, it’s important to reflect on your approach and to thoroughly explain the choices you made in collecting and analyzing the data.
Qualitative data can take the form of texts, photos, videos and audio. For example, you might be working with interview transcripts, survey responses, fieldnotes, or recordings from natural settings.
Most types of qualitative data analysis share the same five steps:
- Prepare and organize your data. This may mean transcribing interviews or typing up fieldnotes.
- Review and explore your data. Examine the data for patterns or repeated ideas that emerge.
- Develop a data coding system. Based on your initial ideas, establish a set of codes that you can apply to categorize your data.
- Assign codes to the data. For example, in qualitative survey analysis, this may mean going through each participant’s responses and tagging them with codes in a spreadsheet. As you go through your data, you can create new codes to add to your system if necessary.
- Identify recurring themes. Link codes together into cohesive, overarching themes.
There are several specific approaches to analyzing qualitative data. Although these methods share similar processes, they emphasize different concepts.
Qualitative research often tries to preserve the voice and perspective of participants and can be adjusted as new research questions arise. Qualitative research is good for:
- Flexibility
The data collection and analysis process can be adapted as new ideas or patterns emerge. They are not rigidly decided beforehand.
- Natural settings
Data collection occurs in real-world contexts or in naturalistic ways.
- Meaningful insights
Detailed descriptions of people’s experiences, feelings and perceptions can be used in designing, testing or improving systems or products.
- Generation of new ideas
Open-ended responses mean that researchers can uncover novel problems or opportunities that they wouldn’t have thought of otherwise.
Researchers must consider practical and theoretical limitations in analyzing and interpreting their data. Qualitative research suffers from:
- Unreliability
The real-world setting often makes qualitative research unreliable because of uncontrolled factors that affect the data.
- Subjectivity
Due to the researcher’s primary role in analyzing and interpreting data, qualitative research cannot be replicated . The researcher decides what is important and what is irrelevant in data analysis, so interpretations of the same data can vary greatly.
- Limited generalizability
Small samples are often used to gather detailed data about specific contexts. Despite rigorous analysis procedures, it is difficult to draw generalizable conclusions because the data may be biased and unrepresentative of the wider population .
- Labor-intensive
Although software can be used to manage and record large amounts of text, data analysis often has to be checked or performed manually.
If you want to know more about statistics , methodology , or research bias , make sure to check out some of our other articles with explanations and examples.
- Chi square goodness of fit test
- Degrees of freedom
- Null hypothesis
- Discourse analysis
- Control groups
- Mixed methods research
- Non-probability sampling
- Quantitative research
- Inclusion and exclusion criteria
Research bias
- Rosenthal effect
- Implicit bias
- Cognitive bias
- Selection bias
- Negativity bias
- Status quo bias
Quantitative research deals with numbers and statistics, while qualitative research deals with words and meanings.
Quantitative methods allow you to systematically measure variables and test hypotheses . Qualitative methods allow you to explore concepts and experiences in more detail.
There are five common approaches to qualitative research :
- Grounded theory involves collecting data in order to develop new theories.
- Ethnography involves immersing yourself in a group or organization to understand its culture.
- Narrative research involves interpreting stories to understand how people make sense of their experiences and perceptions.
- Phenomenological research involves investigating phenomena through people’s lived experiences.
- Action research links theory and practice in several cycles to drive innovative changes.
Data collection is the systematic process by which observations or measurements are gathered in research. It is used in many different contexts by academics, governments, businesses, and other organizations.
There are various approaches to qualitative data analysis , but they all share five steps in common:
- Prepare and organize your data.
- Review and explore your data.
- Develop a data coding system.
- Assign codes to the data.
- Identify recurring themes.
The specifics of each step depend on the focus of the analysis. Some common approaches include textual analysis , thematic analysis , and discourse analysis .
Cite this Scribbr article
If you want to cite this source, you can copy and paste the citation or click the “Cite this Scribbr article” button to automatically add the citation to our free Citation Generator.
Bhandari, P. (2023, June 22). What Is Qualitative Research? | Methods & Examples. Scribbr. Retrieved March 31, 2024, from https://www.scribbr.com/methodology/qualitative-research/
Is this article helpful?
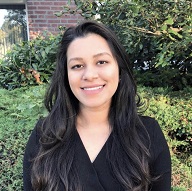
Pritha Bhandari
Other students also liked, qualitative vs. quantitative research | differences, examples & methods, how to do thematic analysis | step-by-step guide & examples, what is your plagiarism score.
- Open access
- Published: 28 March 2024
Using the consolidated Framework for Implementation Research to integrate innovation recipients’ perspectives into the implementation of a digital version of the spinal cord injury health maintenance tool: a qualitative analysis
- John A Bourke 1 , 2 , 3 ,
- K. Anne Sinnott Jerram 1 , 2 ,
- Mohit Arora 1 , 2 ,
- Ashley Craig 1 , 2 &
- James W Middleton 1 , 2 , 4 , 5
BMC Health Services Research volume 24 , Article number: 390 ( 2024 ) Cite this article
69 Accesses
Metrics details
Despite advances in managing secondary health complications after spinal cord injury (SCI), challenges remain in developing targeted community health strategies. In response, the SCI Health Maintenance Tool (SCI-HMT) was developed between 2018 and 2023 in NSW, Australia to support people with SCI and their general practitioners (GPs) to promote better community self-management. Successful implementation of innovations such as the SCI-HMT are determined by a range of contextual factors, including the perspectives of the innovation recipients for whom the innovation is intended to benefit, who are rarely included in the implementation process. During the digitizing of the booklet version of the SCI-HMT into a website and App, we used the Consolidated Framework for Implementation Research (CFIR) as a tool to guide collection and analysis of qualitative data from a range of innovation recipients to promote equity and to inform actionable findings designed to improve the implementation of the SCI-HMT.
Data from twenty-three innovation recipients in the development phase of the SCI-HMT were coded to the five CFIR domains to inform a semi-structured interview guide. This interview guide was used to prospectively explore the barriers and facilitators to planned implementation of the digital SCI-HMT with six health professionals and four people with SCI. A team including researchers and innovation recipients then interpreted these data to produce a reflective statement matched to each domain. Each reflective statement prefaced an actionable finding, defined as alterations that can be made to a program to improve its adoption into practice.
Five reflective statements synthesizing all participant data and linked to an actionable finding to improve the implementation plan were created. Using the CFIR to guide our research emphasized how partnership is the key theme connecting all implementation facilitators, for example ensuring that the tone, scope, content and presentation of the SCI-HMT balanced the needs of innovation recipients alongside the provision of evidence-based clinical information.
Conclusions
Understanding recipient perspectives is an essential contextual factor to consider when developing implementation strategies for healthcare innovations. The revised CFIR provided an effective, systematic method to understand, integrate and value recipient perspectives in the development of an implementation strategy for the SCI-HMT.
Trial registration
Peer Review reports
Injury to the spinal cord can occur through traumatic causes (e.g., falls or motor vehicle accidents) or from non-traumatic disease or disorder (e.g., tumours or infections) [ 1 ]. The onset of a spinal cord injury (SCI) is often sudden, yet the consequences are lifelong. The impact of a SCI is devastating, with effects on sensory and motor function, bladder and bowel function, sexual function, level of independence, community participation and quality of life [ 2 ]. In order to maintain good health, wellbeing and productivity in society, people with SCI must develop self-management skills and behaviours to manage their newly acquired chronic health condition [ 3 ]. Given the increasing emphasis on primary health care and community management of chronic health conditions, like SCI, there is a growing responsibility on all parties to promote good health practices and minimize the risks of common health complications in their communities.
To address this need, the Spinal Cord Injury Health Maintenance Tool (SCI-HMT) was co-designed between 2018 and 2023 with people living with SCI and their General Practitioners (GPs) in NSW, Australia [ 4 ] The aim of the SCI-HMT is to support self-management of the most common and arguably avoidable potentially life-threatening complications associated with SCI, such as mental health crises, autonomic dysreflexia, kidney infections and pressure injuries. The SCI-HMT provides comprehensible information with resources about the six highest priority health areas related to SCI (as indicated by people with SCI and GPs) and was developed over two phases. Phase 1 focused on developing a booklet version and Phase 2 focused on digitizing this content into a website and smartphone app [ 4 , 5 ].
Enabling the successful implementation of evidence-based innovations such as the SCI-HMT is inevitably influenced by contextual factors: those dynamic and diverse array of forces within real-world settings working for or against implementation efforts [ 6 ]. Contextual factors often include background environmental elements in which an intervention is situated, for example (but not limited to) demographics, clinical environments, organisational culture, legislation, and cultural norms [ 7 ]. Understanding the wider context is necessary to identify and potentially mitigate various challenges to the successful implementation of those innovations. Such work is the focus of determinant frameworks, which focus on categorising or classing groups of contextual determinants that are thought to predict or demonstrate an effect on implementation effectiveness to better understand factors that might influence implementation outcomes [ 8 ].
One of the most highly cited determinant frameworks is the Consolidated Framework for Implementation Research (CFIR) [ 9 ], which is often posited as an ideal framework for pre-implementation preparation. Originally published in 2009, the CFIR has recently been subject to an update by its original authors, which included a literature review, survey of users, and the creation of an outcome addendum [ 10 , 11 ]. A key contribution from this revision was the need for a greater focus on the place of innovation recipients, defined as the constituency for whom the innovation is being designed to benefit; for example, patients receiving treatment, students receiving a learning activity. Traditionally, innovation recipients are rarely positioned as key decision-makers or innovation implementers [ 8 ], and as a consequence, have not often been included in the application of research using frameworks, such as the CFIR [ 11 ].
Such power imbalances within the intersection of healthcare and research, particularly between those receiving and delivering such services and those designing such services, have been widely reported [ 12 , 13 ]. There are concerted efforts within health service development, health research and health research funding, to rectify this power imbalance [ 14 , 15 ]. Importantly, such efforts to promote increased equitable population impact are now being explicitly discussed within the implementation science literature. For example, Damschroder et al. [ 11 ] has recently argued for researchers to use the CFIR to collect data from innovation recipients, and that, ultimately, “equitable population impact is only possible when recipients are integrally involved in implementation and all key constituencies share power and make decisions together” (p. 7). Indeed, increased equity between key constituencies and partnering with innovation recipients promotes the likelihood of sustainable adoption of an innovation [ 4 , 12 , 14 ].
There is a paucity of work using the updated CFIR to include and understand innovation recipients’ perspectives. To address this gap, this paper reports on a process of using the CFIR to guide the collection of qualitative data from a range of innovation recipients within a wider co-design mixed methods study examining the development and implementation of SCI-HMT. The innovation recipients in our research are people living with SCI and GPs. Guided by the CFIR domains (shown in the supplementary material), we used reflexive thematic analysis [ 16 ]to summarize data into reflective summaries, which served to inform actionable findings designed to improve implementation of the SCI-HMT.
The procedure for this research is multi-stepped and is summarized in Fig. 1 . First, we mapped retrospective qualitative data collected during the development of the SCI-HMT [ 4 ] against the five domains of the CFIR in order to create a semi-structured interview guide (Step 1). Then, we used this interview guide to collect prospective data from health professionals and people with SCI during the development of the digital version of the SCI-HMT (Step 2) to identify implementation barriers and facilitators. This enabled us to interpret a reflective summary statement for each CFIR domain. Lastly, we developed an actionable finding for each domain summary. The first (RESP/18/212) and second phase (2019/ETH13961) of the project received ethical approval from The Northern Sydney Local Health District Human Research Ethics Committee. The reporting of this study was conducted in line with the consolidated Criteria for Reporting Qualitative Research (COREQ) guidelines [ 17 ]. All methods were performed in accordance with the relevant guidelines and regulations.
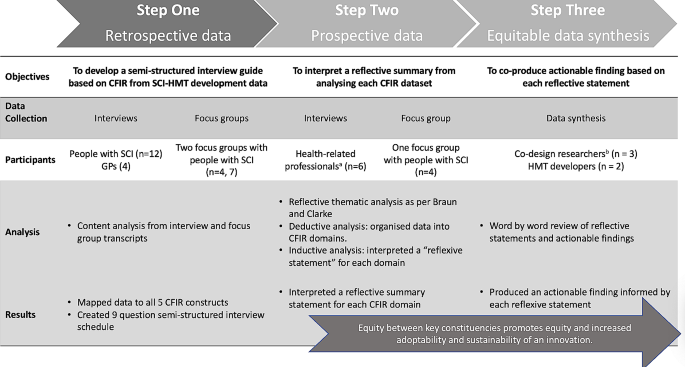
Procedure of synthesising datasets to inform reflective statements and actionable findings. a Two health professionals had a SCI (one being JAB); b Two co-design researchers had a SCI (one being JAB)
Step one: retrospective data collection and analysis
We began by retrospectively analyzing the data set (interview and focus group transcripts) from the previously reported qualitative study from the development phase of the SCI-HMT [ 4 ]. This analysis was undertaken by two team members (KASJ and MA). KASJ has a background in co-design research. Transcript data were uploaded into NVivo software (Version 12: QSR International Pty Ltd) and a directed content analysis approach [ 18 ] was applied to analyze categorized data a priori according to the original 2009 CFIR domains (intervention characteristics, outer setting, inner setting, characteristics of individuals, and process of implementation) described by Damschroder et al. [ 9 ]. This categorized data were summarized and informed the specific questions of a semi-structured interview guide. The final output of step one was an interview guide with context-specific questions arranged according to the CFIR domains (see supplementary file 1). The interview was tested with two people with SCI and one health professional.
Step two: prospective data collection and analysis
In the second step, semi-structured interviews were conducted by KASJ (with MA as observer) with consenting healthcare professionals who had previously contributed to the development of the SCI-HMT. Healthcare professionals included GPs, Nurse Consultants, Specialist Physiotherapists, along with Health Researchers (one being JAB). In addition, a focus group was conducted with consenting individuals with SCI who had contributed to the SCI-HMT design and development phase. The interview schedule designed in step one above guided data collection in all interviews and the focus group.
The focus group and interviews were conducted online, audio recorded, transcribed verbatim and uploaded to NVivo software (Version 12: QSR International Pty Ltd). All data were subject to reflexive, inductive and deductive thematic analysis [ 16 , 19 ] to better understand participants’ perspectives regarding the potential implementation of the SCI-HMT. First, one team member (KASJ) read transcripts and began a deductive analysis whereby data were organized into CFIR domains-specific dataset. Second, KASJ and JAB analyzed this domain-specific dataset to inductively interpret a reflective statement which served to summarise all participant responses to each domain. The final output of step two was a reflective summary statement for each CFIR domain.
Step three: data synthesis
In the third step we aimed to co-create an actionable finding (defined as tangible alteration that can be made to a program, in this case the SCI-HMT [ 20 ]) based on each domain-specific reflective statement. To achieve this, three codesign researchers (KAS and JAB with one person with SCI from Step 2 (deidentified)) focused on operationalising each reflective statement into a recommended modification for the digital version of the SCI-HMT. This was an iterative process guided by the specific CFIR domain and construct definitions, which we deemed salient and relevant to each reflective statement (see Table 2 for example). Data synthesis involved line by line analysis, group discussion, and repeated refinement of actionable findings. A draft synthesis was shared with SCI-HMT developers (JWM and MA) and refinement continued until consensus was agreed on. The final outputs of step three were an actionable finding related to each reflective statement for each CFIR domain.
The characteristics of both the retrospective and prospective study participants are shown in Table 1 . The retrospective data included data from a total of 23 people: 19 people with SCI and four GPs. Of the 19 people with SCI, 12 participated in semi-structured interviews, seven participated in the first focus group, and four returned to the second focus group. In step 2, four people with SCI participated in a focus group and six healthcare professionals participated in one-on-one semi-structured interviews. Two of the healthcare professionals (a GP and a registrar) had lived experience of SCI, as did one researcher (JAB). All interviews and focus groups were conducted either online or in-person and ranged in length between 60 and 120 min.
In our overall synthesis, we actively interpreted five reflective statements based on the updated CFIR domain and construct definitions by Damschroder et al. [ 11 ]. Table 2 provides a summary of how we linked the updated CFIR domain and construct definitions to the reflective statements. We demonstrate this process of co-creation below, including illustrative quotes from participants. Importantly, we guide readers to the actionable findings related to each reflective statement in Table 2 . Each actionable statement represents an alteration that can be made to a program to improve its adoption into practice.
Participants acknowledged that self-management is a major undertaking and very demanding, as one person with SCI said, “ we need to be informed without being terrified and overwhelmed”. Participants felt the HMT could indeed be adapted, tailored, refined, or reinvented to meet local needs. For example, another person with SCI remarked:
“Education needs to be from the get-go but in bite sized pieces from all quarters when readiness is most apparent… at all time points , [not just as a] a newbie tool or for people with [long-term impairment] ” (person with SCI_02).
Therefore, the SCI-HMT had to balance complexity of content while still being accessible and engaging, and required input from both experts in the field and those with lived experience of SCI, for example, a clinical nurse specialist suggested:
“it’s essential [the SCI-HMT] is written by experts in the field as well as with collaboration with people who have had a, you know, the lived experience of SCI” (healthcare professional_03).
Furthermore, the points of contact with healthcare for a person with SCI can be challenging to navigate and the SCI-HMT has the potential to facilitate a smoother engagement process and improve communication between people with SCI and healthcare services. As a GP suggested:
“we need a tool like this to link to that pathway model in primary health care , [the SCI-HMT] it’s a great tool, something that everyone can read and everyone’s reading the same thing” (healthcare professional_05).
Participants highlighted that the ability of the SCI-HMT to facilitate effective communication was very much dependent on the delivery format. The idea of digitizing the SCI-HMT garnered equal support from people with SCI and health care professionals, with one participant with SCI deeming it to be “ essential” ( person with SCI_01) and a health professional suggesting a “digitalized version will be an advantage for most people” (healthcare professional_02).
Outer setting
There was strong interest expressed by both people with SCI and healthcare professionals in using the SCI-HMT. The fundamental premise was that knowledge is power and the SCI-HMT would have strong utility in post-acute rehabilitation services, as well as primary care. As a person with SCI said,
“ we need to leave the [spinal unit] to return to the community with sufficient knowledge, and to know the value of that knowledge and then need to ensure primary healthcare provider [s] are best informed” (person with SCI_04).
The value of the SCI-HMT in facilitating clear and effective communication and shared decision-making between healthcare professionals and people with SCI was also highlighted, as shown by the remarks of an acute nurse specialist:
“I think this tool is really helpful for the consumer and the GP to work together to prioritize particular tests that a patient might need and what the regularity of that is” (healthcare professional_03).
Engaging with SCI peer support networks to promote the SCI-HMT was considered crucial, as one person with SCI emphasized when asked how the SCI-HMT might be best executed in the community, “…peers, peers and peers” (person with SCI_01). Furthermore, the layering of content made possible in the digitalized version will allow for the issue of approachability in terms of readiness for change, as another person with SCI said:
“[putting content into a digital format] is essential and required and there is a need to put summarized content in an App with links to further web-based information… it’s not likely to be accessed otherwise” (person with SCI_02).
Inner setting
Participants acknowledged that self-management of health and well-being is substantial and demanding. It was suggested that the scope, tone, and complexity of the SCI-HMT, while necessary, could potentially be resisted by people with SCI if they felt overwhelmed, as one person with SCI described:
“a manual that is really long and wordy, like, it’s [a] health metric… they maybe lack the health literacy to, to consume the content then yes, it would impede their readiness for [self-management]” (person with SCI_02).
Having support from their GPs was considered essential, and the HMT could enable GP’s, who are under time pressure, to provide more effective health and advice to their patients, as one GP said:
“We GP’s are time poor, if you realize then when you’re time poor you look quickly to say oh this is a patient tool - how can I best use this?” (healthcare professional_05).
Furthermore, health professional skills may be best used with the synthesis of self-reported symptoms, behaviors, or observations. A particular strength of a digitized version would be its ability to facilitate more streamlined communication between a person with SCI and their primary healthcare providers developing healthcare plans, as an acute nurse specialist reflected, “ I think that a digitalized version is essential with links to primary healthcare plans” (healthcare professional_03).
Efficient communication with thorough assessment is essential to ensure serious health issues are not missed, as findings reinforce that the SCI-HMT is an educational tool, not a replacement for healthcare services, as a clinical nurse specialist commented, “ remember, things will go wrong– people end up very sick and in acute care “ (healthcare professional_02).
The SCI-HMT has the potential to provide a pathway to a ‘hope for better than now’ , a hope to ‘remain well’ and a hope to ‘be happy’ , as the informant with SCI (04) declared, “self-management is a long game, if you’re keeping well, you’ve got that possibility of a good life… of happiness”. Participants with SCI felt the tool needed to be genuine and
“acknowledge the huge amount of adjustment required, recognizing that dealing with SCI issues is required to survive and live a good life” (person with SCI_04).
However, there is a risk that an individual is completely overwhelmed by the scale of the SCI-HMT content and the requirement for lifelong vigilance. Careful attention and planning were paid to layering the information accordingly to support self-management as a ‘long game’, which one person with SCI reflected in following:
“the first 2–3 year [period] is probably the toughest to get your head around the learning stuff, because you’ve got to a stage where you’re levelling out, and you’ve kind of made these promises to yourself and then you realize that there’s no quick fix” (person with SCI_01).
It was decided that this could be achieved by providing concrete examples and anecdotes from people with SCI illustrating that a meaningful, healthy life is possible, and that good health is the bedrock of a good life with SCI.
There was universal agreement that the SCI-HMT is aspirational and that it has the potential to improve knowledge and understanding for people with SCI, their families, community workers/carers and primary healthcare professionals, as a GP remarked:
“[different groups] could just read it and realize, ‘Ahh, OK that’s what that means… when you’re doing catheters. That’s what you mean when you’re talking about bladder and bowel function or skin care” (healthcare professional_04).
Despite the SCI-HMT providing an abundance of information and resources to support self-management, participants identified four gaps: (i) the priority issue of sexuality, including pleasure and identity, as one person with SCI remarked:
“ sexuality is one of the biggest issues that people with SCI often might not speak about that often cause you know it’s awkward for them. So yeah, I think that’s a that’s a serious issue” (person with SCI_03).
(ii) consideration of the taboo nature of bladder and bowel topics for indigenous people, (iii) urgent need to ensure links for SCI-HMT care plans are compatible with patient management systems, and (iv) exercise and leisure as a standalone topic taking account of effects of physical activity, including impact on mental health and wellbeing but more especially for fun.
To ensure longevity of the SCI-HMT, maintaining a partnership between people with SCI, SCI community groups and both primary and tertiary health services is required for liaison with the relevant professional bodies, care agencies, funders, policy makers and tertiary care settings to ensure ongoing education and promotion of SCI-HMT is maintained. For example, delivery of ongoing training of healthcare professionals to both increase the knowledge base of primary healthcare providers in relation to SCI, and to promote use of the tools and resources through health communities. As a community nurse specialist suggested:
“ improving knowledge in the health community… would require digital links to clinical/health management platforms” (healthcare professional_02).
In a similar vein, a GP suggested:
“ our common GP body would have continuing education requirements… especially if it’s online, in particular for the rural, rural doctors who you know, might find it hard to get into the city” (healthcare professional_04).
The successful implementation of evidence-based innovations into practice is dependent on a wide array of dynamic and active contextual factors, including the perspectives of the recipients who are destined to use such innovations. Indeed, the recently updated CFIR has called for innovation recipient perspectives to be a priority when considering contextual factors [ 10 , 11 ]. Understanding and including the perspectives of those the innovation is being designed to benefit can promote increased equity and validation of recipient populations, and potentially increase the adoption and sustainability of innovations.
In this paper, we have presented research using the recently updated CFIR to guide the collection of innovation recipients’ perspectives (including people with SCI and GPs working in the community) regarding the potential implementation barriers and facilitators of the digital version of the SCI-HMT. Collected data were synthesized to inform actionable findings– tangible ways in which the SCI-HMT could be modified according of the domains of the CFIR (e.g., see Keith et al. [ 20 ]). It is important to note that we conducted this research using the original domains of the CFIR [ 9 ] prior to Damschroder et al. publishing the updated CFIR [ 11 ]. However, in our analysis we were able to align our findings to the revised CFIR domains and constructs, as Damschroder [ 11 ] suggests, constructs can “be mapped back to the original CFIR to ensure longitudinal consistency” (p. 13).
One of the most poignant findings from our analyses was the need to ensure the content of the SCI-HMT balanced scientific evidence and clinical expertise with lived experience knowledge. This balance of clinical and experiential knowledge demonstrated genuine regard for lived experience knowledge, and created a more accessible, engaging, useable platform. For example, in the innovation and individual domains, the need to include lived experience quotes was immediately apparent once the perspective of people with SCI was included. It was highlighted that while the SCI-HMT will prove useful to many parties at various stages along the continuum of care following onset of SCI, there will be those individuals that are overwhelmed by the scale of the content. That said, the layering of information facilitated by the digitalized version is intended to provide an ease of navigation through the SCI-HMT and enable a far greater sense of control over personal health and wellbeing. Further, despite concerns regarding e-literacy the digitalized version of the SCI-HMT is seen as imperative for accessibility given the wide geographic diversity and recent COVID pandemic [ 21 ]. While there will be people who are challenged by the technology, the universally acceptable use of the internet is seen as less of a barrier than printed material.
The concept of partnership was also apparent within the data analysis focusing on the outer and inner setting domains. In the outer setting domain, our findings emphasized the importance of engaging with SCI community groups, as well as primary and tertiary care providers to maximize uptake at all points in time from the phase of subacute rehabilitation onwards. While the SCI-HMT is intended for use across the continuum of care from post-acute rehabilitation onwards, it may be that certain modules are more relevant at different times, and could serve as key resources during the hand over between acute care, inpatient rehabilitation and community reintegration.
Likewise, findings regarding the inner setting highlighted the necessity of a productive partnership between GPs and individuals with SCI to address the substantial demands of long-term self-management of health and well-being following SCI. Indeed, support is crucial, especially when self-management is the focus. This is particularly so in individuals living with complex disability following survival after illness or injury [ 22 ], where health literacy has been found to be a primary determinant of successful health and wellbeing outcomes [ 23 ]. For people with SCI, this tool potentially holds the most appeal when an individual is ready and has strong partnerships and supportive communication. This can enable potential red flags to be recognized earlier allowing timely intervention to avert health crises, promoting individual well-being, and reducing unnecessary demands on health services.
While the SCI-HMT is an educational tool and not meant to replace health services, findings suggest the current structure would lead nicely to having the conversation with a range of likely support people, including SCI peers, friends and family, GP, community nurses, carers or via on-line support services. The findings within the process domain underscored the importance of ongoing partnership between innovation implementers and a broad array of innovation recipients (e.g., individuals with SCI, healthcare professionals, family, funding agencies and policy-makers). This emphasis on partnership also addresses recent discussions regarding equity and the CFIR. For example, Damschroder et al. [ 11 ] suggests that innovation recipients are too often not included in the CFIR process, as the CFIR is primarily seen as a tool intended “to collect data from individuals who have power and/or influence over implementation outcomes” (p. 5).
Finally, we feel that our inclusion of innovation recipients’ perspectives presented in this article begins to address the notion of equity in implementation, whereby the inclusion of recipient perspectives in research using the CFIR both validates, and increases, the likelihood of sustainable adoption of evidence-based innovations, such as the SCI-HMT. We have used the CFIR in a pragmatic way with an emphasis on meaningful engagement between the innovation recipients and the research team, heeding the call from Damschroder et al. [ 11 ], who recently argued for researchers to use the CFIR to collect data from innovation recipients. Adopting this approach enabled us to give voice to innovation recipient perspectives and subsequently ensure that the tone, scope, content and presentation of the SCI-HMT balanced the needs of innovation recipients alongside the provision of evidence-based clinical information.
Our research is not without limitations. While our study was successful in identifying a number of potential barriers and facilitators to the implementation of the SCI-HMT, we did not test any implementation strategies to impact determinants, mechanisms, or outcomes. This will be the focus of future research on this project, which will investigate the impact of implementation strategies on outcomes. Focus will be given to the context-mechanism configurations which give rise to particular outcomes for different groups in certain circumstances [ 7 , 24 ]. A second potential concern is the relatively small sample size of participants that may not allow for saturation and generalizability of the findings. However, both the significant impact of secondary health complications for people with SCI and the desire for a health maintenance tool have been established in Australia [ 2 , 4 ]. The aim our study reported in this article was to achieve context-specific knowledge of a small sample that shares a particular mutual experience and represents a perspective, rather than a population [ 25 , 26 ]. We feel our findings can stimulate discussion and debate regarding participant-informed approaches to implementation of the SCI-HMT, which can then be subject to larger-sample studies to determine their generalisability, that is, their external validity. Notably, future research could examine the interaction between certain demographic differences (e.g., gender) of people with SCI and potential barriers and facilitators to the implementation of the SCI-HMT. Future research could also include the perspectives of other allied health professionals working in the community, such as occupational therapists. Lastly, while our research gave significant priority to recipient viewpoints, research in this space would benefit for ensuring innovation recipients are engaged as genuine partners throughout the entire research process from conceptualization to implementation.
Employing the CFIR provided an effective, systematic method for identifying recipient perspectives regarding the implementation of a digital health maintenance tool for people living with SCI. Findings emphasized the need to balance clinical and lived experience perspectives when designing an implementation strategy and facilitating strong partnerships with necessary stakeholders to maximise the uptake of SCI-HMT into practice. Ongoing testing will monitor the uptake and implementation of this innovation, specifically focusing on how the SCI-HMT works for different users, in different contexts, at different stages and times of the rehabilitation journey.
Data availability
The datasets supporting the conclusions of this article are available available upon request and with permission gained from the project Steering Committee.
Abbreviations
spinal cord injury
HMT-Spinal Cord Injury Health Maintenance Tool
Consolidated Framework for Implementation Research
Kirshblum S, Vernon WL. Spinal Cord Medicine, Third Edition. New York: Springer Publishing Company; 2018.
Middleton JW, Arora M, Kifley A, Clark J, Borg SJ, Tran Y, et al. Australian arm of the International spinal cord Injury (Aus-InSCI) Community Survey: 2. Understanding the lived experience in people with spinal cord injury. Spinal Cord. 2022;60(12):1069–79.
Article PubMed PubMed Central Google Scholar
Craig A, Nicholson Perry K, Guest R, Tran Y, Middleton J. Adjustment following chronic spinal cord injury: determining factors that contribute to social participation. Br J Health Psychol. 2015;20(4):807–23.
Article PubMed Google Scholar
Middleton JW, Arora M, Jerram KAS, Bourke J, McCormick M, O’Leary D, et al. Co-design of the Spinal Cord Injury Health Maintenance Tool to support Self-Management: a mixed-methods Approach. Top Spinal Cord Injury Rehabilitation. 2024;30(1):59–73.
Article Google Scholar
Middleton JW, Arora M, McCormick M, O’Leary D. Health maintenance Tool: how to stay healthy and well with a spinal cord injury. A tool for consumers by consumers. 1st ed. Sydney, NSW Australia: Royal Rehab and The University of Sydney; 2020.
Google Scholar
Nilsen P, Bernhardsson S. Context matters in implementation science: a scoping review of determinant frameworks that describe contextual determinants for implementation outcomes. BMC Health Serv Res. 2019;19(1):189.
Jagosh J. Realist synthesis for Public Health: building an Ontologically Deep understanding of how Programs Work, for whom, and in which contexts. Annu Rev Public Health. 2019;40(1):361–72.
Nilsen P. Making sense of implementation theories, models and frameworks. Implement Sci. 2015;10(1):53.
Damschroder LJ, Aron DC, Keith RE, Kirsh SR, Alexander JA, Lowery JC. Fostering implementation of health services research findings into practice: a consolidated framework for advancing implementation science. Implement Sci. 2009;4(1):50.
Damschroder LJ, Reardon CM, Opra Widerquist MA, Lowery JC. Conceptualizing outcomes for use with the Consolidated Framework for Implementation Research (CFIR): the CFIR outcomes Addendum. Implement Sci. 2022;17(1):7.
Damschroder LJ, Reardon CM, Widerquist MAO, Lowery JC. The updated Consolidated Framework for Implementation Research based on user feedback. Implement Sci. 2022;17(1):75.
Plamondon K, Ndumbe-Eyoh S, Shahram S. 2.2 Equity, Power, and Transformative Research Coproduction. Research Co-Production in Healthcare2022. p. 34–53.
Verville L, Cancelliere C, Connell G, Lee J, Munce S, Mior S, et al. Exploring clinicians’ experiences and perceptions of end-user roles in knowledge development: a qualitative study. BMC Health Serv Res. 2021;21(1):926.
Gainforth HL, Hoekstra F, McKay R, McBride CB, Sweet SN, Martin Ginis KA, et al. Integrated Knowledge Translation Guiding principles for conducting and Disseminating Spinal Cord Injury Research in Partnership. Arch Phys Med Rehabil. 2021;102(4):656–63.
Langley J, Knowles SE, Ward V. Conducting a Research Coproduction Project. Research Co-Production in Healthcare2022. p. 112– 28.
Braun V, Clarke V. One size fits all? What counts as quality practice in (reflexive) thematic analysis? Qualitative Research in Psychology. 2020:1–25.
Tong A, Sainsbury p, Craig J. Consolidated criteria for reporting qulaitative research (COREQ): a 32-item checklist for interviews and focus groups. Int J Qual Health Care. 2007;19(6):349–57.
Bengtsson M. How to plan and perform a qualitative study using content analysis. NursingPlus Open. 2016;2:8–14.
Braun V, Clarke V. Using thematic analysis in psychology. Qualitative Res Psychol. 2006;3(2):77–101.
Keith RE, Crosson JC, O’Malley AS, Cromp D, Taylor EF. Using the Consolidated Framework for Implementation Research (CFIR) to produce actionable findings: a rapid-cycle evaluation approach to improving implementation. Implement Science: IS. 2017;12(1):15.
Choukou M-A, Sanchez-Ramirez DC, Pol M, Uddin M, Monnin C, Syed-Abdul S. COVID-19 infodemic and digital health literacy in vulnerable populations: a scoping review. Digit HEALTH. 2022;8:20552076221076927.
PubMed PubMed Central Google Scholar
Daniels N. Just Health: Meeting Health needs fairly. Cambridge University Press; 2007. p. 397.
Parker SM, Stocks N, Nutbeam D, Thomas L, Denney-Wilson E, Zwar N, et al. Preventing chronic disease in patients with low health literacy using eHealth and teamwork in primary healthcare: protocol for a cluster randomised controlled trial. BMJ Open. 2018;8(6):e023239–e.
Salter KL, Kothari A. Using realist evaluation to open the black box of knowledge translation: a state-of-the-art review. Implement Sci. 2014;9(1):115.
Sebele-Mpofu FY. The Sampling Conundrum in qualitative research: can Saturation help alleviate the controversy and alleged subjectivity in Sampling? Int’l J Soc Sci Stud. 2021;9:11.
Malterud K, Siersma VD, Guassora AD. Sample size in qualitative interview studies: guided by Information Power. Qual Health Res. 2015;26(13):1753–60.
Download references
Acknowledgements
Authors of this study would like to thank all the consumers with SCI and healthcare professionals for their invaluable contribution to this project. Their participation and insights have been instrumental in shaping the development of the SCI-HMT. The team also acknowledges the support and guidance provided by the members of the Project Steering Committee, as well as the partner organisations, including NSW Agency for Clinical Innovation, and icare NSW. Author would also like to acknowledge the informant group with lived experience, whose perspectives have enriched our understanding and informed the development of SCI-HMT.
The SCI Wellness project was a collaborative project between John Walsh Centre for Rehabilitation Research at The University of Sydney and Royal Rehab. Both organizations provided in-kind support to the project. Additionally, the University of Sydney and Royal Rehab received research funding from Insurance and Care NSW (icare NSW) to undertake the SCI Wellness Project. icare NSW do not take direct responsibility for any of the following: study design, data collection, drafting of the manuscript, or decision to publish.
Author information
Authors and affiliations.
John Walsh Centre for Rehabilitation Research, Northern Sydney Local Health District, St Leonards, NSW, Australia
John A Bourke, K. Anne Sinnott Jerram, Mohit Arora, Ashley Craig & James W Middleton
The Kolling Institute, Faculty of Medicine and Health, The University of Sydney, Sydney, NSW, Australia
Burwood Academy Trust, Burwood Hospital, Christchurch, New Zealand
John A Bourke
Royal Rehab, Ryde, NSW, Australia
James W Middleton
State Spinal Cord Injury Service, NSW Agency for Clinical Innovation, St Leonards, NSW, Australia
You can also search for this author in PubMed Google Scholar
Contributions
Project conceptualization: KASJ, MA, JWM; project methodology: JWM, MA, KASJ, JAB; data collection: KASJ and MA; data analysis: KASJ, JAB, MA, JWM; writing—original draft preparation: JAB; writing—review and editing: JAB, KASJ, JWM, MA, AC; funding acquisition: JWM, MA. All authors contributed to the revision of the paper and approved the final submitted version.
Corresponding author
Correspondence to John A Bourke .
Ethics declarations
Ethics approval and consent to participate.
The first (RESP/18/212) and second phase (2019/ETH13961) of the project received ethical approval from The Northern Sydney Local Health District Human Research Ethics Committee. All participants provided informed, written consent. All data were to be retained for 7 years (23rd May 2030).
Consent for publication
Not applicable.
Competing interests
MA part salary (from Dec 2018 to Dec 2023), KASJ part salary (July 2021 to Dec 2023) and JAB part salary (Jan 2022 to Aug 2022) was paid from the grant monies. Other authors declare no conflicts of interest.
Additional information
Publisher’s note.
Springer Nature remains neutral with regard to jurisdictional claims in published maps and institutional affiliations.
Electronic supplementary material
Below is the link to the electronic supplementary material.
Supplementary Material 1
Rights and permissions.
Open Access This article is licensed under a Creative Commons Attribution 4.0 International License, which permits use, sharing, adaptation, distribution and reproduction in any medium or format, as long as you give appropriate credit to the original author(s) and the source, provide a link to the Creative Commons licence, and indicate if changes were made. The images or other third party material in this article are included in the article’s Creative Commons licence, unless indicated otherwise in a credit line to the material. If material is not included in the article’s Creative Commons licence and your intended use is not permitted by statutory regulation or exceeds the permitted use, you will need to obtain permission directly from the copyright holder. To view a copy of this licence, visit http://creativecommons.org/licenses/by/4.0/ . The Creative Commons Public Domain Dedication waiver ( http://creativecommons.org/publicdomain/zero/1.0/ ) applies to the data made available in this article, unless otherwise stated in a credit line to the data.
Reprints and permissions
About this article
Cite this article.
Bourke, J.A., Jerram, K.A.S., Arora, M. et al. Using the consolidated Framework for Implementation Research to integrate innovation recipients’ perspectives into the implementation of a digital version of the spinal cord injury health maintenance tool: a qualitative analysis. BMC Health Serv Res 24 , 390 (2024). https://doi.org/10.1186/s12913-024-10847-x
Download citation
Received : 14 August 2023
Accepted : 11 March 2024
Published : 28 March 2024
DOI : https://doi.org/10.1186/s12913-024-10847-x
Share this article
Anyone you share the following link with will be able to read this content:
Sorry, a shareable link is not currently available for this article.
Provided by the Springer Nature SharedIt content-sharing initiative
- Spinal Cord injury
- Self-management
- Innovation recipients
- Secondary health conditions
- Primary health care
- Evidence-based innovations
- Actionable findings
- Consolidated Framework for implementation research
BMC Health Services Research
ISSN: 1472-6963
- General enquiries: [email protected]
What is artificial general intelligence (AGI)?
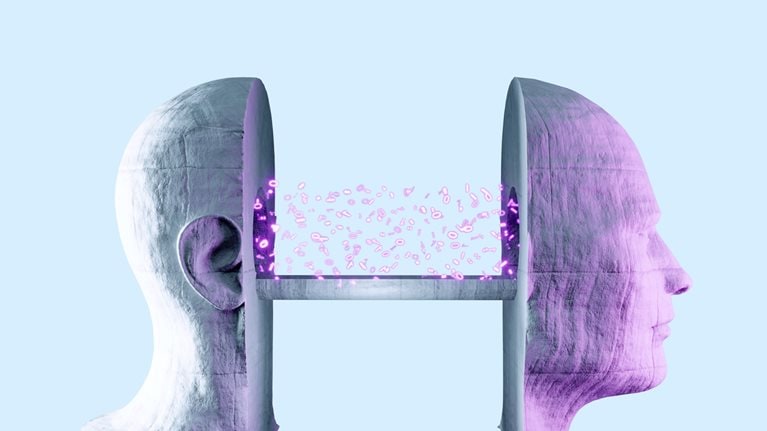
You’ve read the think pieces. AI—in particular, the generative AI (gen AI) breakthroughs achieved in the past year or so—is poised to revolutionize not just the way we create content but the very makeup of our economies and societies as a whole. But although gen AI tools such as ChatGPT may seem like a great leap forward, in reality they are just a step in the direction of an even greater breakthrough: artificial general intelligence, or AGI.
Get to know and directly engage with senior McKinsey experts on AGI
Aamer Baig is a senior partner in McKinsey’s Chicago office; Federico Berruti is a partner in the Toronto office; Ben Ellencweig is a senior partner in the Stamford, Connecticut, office; Damian Lewandowski is a consultant in the Miami office; Roger Roberts is a partner in the Bay Area office, where Lareina Yee is a senior partner; Alex Singla is a senior partner in the Chicago office and the global leader of QuantumBlack, AI by McKinsey; Kate Smaje and Alex Sukharevsky are senior partners in the London office; Jonathan Tilley is a partner in the Southern California office; and Rodney Zemmel is a senior partner in the New York office.
AGI is AI with capabilities that rival those of a human . While purely theoretical at this stage, someday AGI may replicate human-like cognitive abilities including reasoning, problem solving, perception, learning, and language comprehension. When AI’s abilities are indistinguishable from those of a human, it will have passed what is known as the Turing test , first proposed by 20th-century computer scientist Alan Turing.
But let’s not get ahead of ourselves. AI has made significant strides in recent years, but no AI tool to date has passed the Turing test. We’re still far from reaching a point where AI tools can understand, communicate, and act with the same nuance and sensitivity of a human—and, critically, understand the meaning behind it. Most researchers and academics believe we are decades away from realizing AGI; a few even predict we won’t see AGI this century (or ever). Rodney Brooks, a roboticist at the Massachusetts Institute of Technology and cofounder of iRobot, believes AGI won’t arrive until the year 2300 .
If you’re thinking that AI already seems pretty smart, that’s understandable. We’ve seen gen AI do remarkable things in recent years, from writing code to composing sonnets in seconds. But there’s a critical difference between AI and AGI. Although the latest gen AI technologies, including ChatGPT, DALL-E, and others, have been hogging headlines, they are essentially prediction machines—albeit very good ones. In other words, they can predict, with a high degree of accuracy, the answer to a specific prompt because they’ve been trained on huge amounts of data. This is impressive, but it’s not at a human level of performance in terms of creativity, logical reasoning, sensory perception, and other capabilities . By contrast, AGI tools could feature cognitive and emotional abilities (like empathy) indistinguishable from those of a human. Depending on your definition of AGI, they might even be capable of consciously grasping the meaning behind what they’re doing.
The timing of AGI’s emergence is uncertain. But when it does arrive—and it likely will at some point—it’s going to be a very big deal for every aspect of our lives, businesses, and societies. Executives can begin working now to better understand the path to machines achieving human-level intelligence and making the transition to a more automated world.
Learn more about QuantumBlack, AI by McKinsey .
What is needed for AI to become AGI?
Here are eight capabilities AI needs to master before achieving AGI. Click each card to learn more.
How will people access AGI tools?
Today, most people engage with AI in the same ways they’ve accessed digital power for years: via 2D screens such as laptops, smartphones, and TVs. The future will probably look a lot different. Some of the brightest minds (and biggest budgets) in tech are devoting themselves to figuring out how we’ll access AI (and possibly AGI) in the future. One example you’re likely familiar with is augmented reality and virtual reality headsets , through which users experience an immersive virtual world . Another example would be humans accessing the AI world through implanted neurons in the brain. This might sound like something out of a sci-fi novel, but it’s not. In January 2024, Neuralink implanted a chip in a human brain, with the goal of allowing the human to control a phone or computer purely by thought.
A final mode of interaction with AI seems ripped from sci-fi as well: robots. These can take the form of mechanized limbs connected to humans or machine bases or even programmed humanoid robots.
What is a robot and what types of robots are there?
The simplest definition of a robot is a machine that can perform tasks on its own or with minimal assistance from humans. The most sophisticated robots can also interact with their surroundings.
Programmable robots have been operational since the 1950s. McKinsey estimates that 3.5 million robots are currently in use, with 550,000 more deployed every year. But while programmable robots are more commonplace than ever in the workforce, they have a long way to go before they outnumber their human counterparts. The Republic of Korea, home to the world’s highest density of robots, still employs 100 times as many humans as robots.
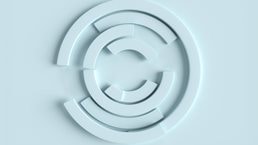
Introducing McKinsey Explainers : Direct answers to complex questions
But as hardware and software limitations become increasingly surmountable, companies that manufacture robots are beginning to program units with new AI tools and techniques. These dramatically improve robots’ ability to perform tasks typically handled by humans, including walking, sensing, communicating, and manipulating objects. In May 2023, Sanctuary AI, for example, launched Phoenix, a bipedal humanoid robot that stands 5’ 7” tall, lifts objects weighing as much as 55 pounds, and travels three miles per hour—not to mention it also folds clothes, stocks shelves, and works a register.
As we edge closer to AGI, we can expect increasingly sophisticated AI tools and techniques to be programmed into robots of all kinds. Here are a few categories of robots that are currently operational:
- Stand-alone autonomous industrial robots : Equipped with sensors and computer systems to navigate their surroundings and interact with other machines, these robots are critical components of the modern automated manufacturing industry.
- Collaborative robots : Also known as cobots, these robots are specifically engineered to operate in collaboration with humans in a shared environment. Their primary purpose is to alleviate repetitive or hazardous tasks. These types of robots are already being used in environments such as restaurant kitchens and more.
- Mobile robots : Utilizing wheels as their primary means of movement, mobile robots are commonly used for materials handling in warehouses and factories. The military also uses these machines for various purposes, such as reconnaissance and bomb disposal.
- Human–hybrid robots : These robots have both human and robotic features. This could include a robot with an appearance, movement capabilities, or cognition that resemble those of a human, or a human with a robotic limb or even a brain implant.
- Humanoids or androids : These robots are designed to emulate the appearance, movement, communicative abilities, and emotions of humans while continuously enhancing their cognitive capabilities via deep learning models. In other words, humanoid robots will think like a human, move like a human, and look like a human.
What advances could speed up the development of AGI?
Advances in algorithms, computing, and data have brought about the recent acceleration of AI. We can get a sense of what the future may hold by looking at these three capabilities:
Algorithmic advances and new robotics approaches . We may need entirely new approaches to algorithms and robots to achieve AGI. One way researchers are thinking about this is by exploring the concept of embodied cognition. The idea is that robots will need to learn very quickly from their environments through a multitude of senses, just like humans do when they’re very young. Similarly, to develop cognition in the same way humans do, robots will need to experience the physical world like we do (because we’ve designed our spaces based on how our bodies and minds work).
The latest AI-based robot systems are using gen AI technologies including large language models (LLMs) and large behavior models (LBMs). LLMs give robots advanced natural-language-processing capabilities like what we’ve seen with generative AI models and other LLM-enabled tools. LBMs allow robots to emulate human actions and movements. These models are created by training AI on large data sets of observed human actions and movements. Ultimately, these models could allow robots to perform a wide range of activities with limited task-specific training.
A real advance would be to develop new AI systems that start out with a certain level of built-in knowledge, just like a baby fawn knows how to stand and feed without being taught. It’s possible that the recent success of deep-learning-based AI systems may have drawn research attention away from the more fundamental cognitive work required to make progress toward AGI.
- Computing advancements. Graphics processing units (GPUs) have made the major AI advances of the past few years possible . Here’s why. For one, GPUs are designed to handle multiple tasks related to visual data simultaneously, including rendering images, videos, and graphics-related computations. Their efficiency at handling massive amounts of visual data makes them useful in training complex neural networks. They also have a high memory bandwidth, meaning faster data transfer. Before AGI can be achieved, similar significant advancements will need to be made in computing infrastructure. Quantum computing is touted as one way of achieving this. However, today’s quantum computers, while powerful, aren’t yet ready for everyday applications. But once they are, they could play a role in the achievement of AGI.
Growth in data volume and new sources of data . Some experts believe 5G mobile infrastructure could bring about a significant increase in data. That’s because the technology could power a surge in connected devices, or the Internet of Things . But, for a variety of reasons, we think most of the benefits of 5G have already appeared . For AGI to be achieved, there will need to be another catalyst for a huge increase in data volume.
New robotics approaches could yield new sources of training data. Placing human-like robots among us could allow companies to mine large sets of data that mimic our own senses to help the robots train themselves. Advanced self-driving cars are one example: data is being collected from cars that are already on the roads, so these vehicles are acting as a training set for future self-driving cars.
What can executives do about AGI?
AGI is still decades away, at the very least. But AI is here to stay—and it is advancing extremely quickly. Smart leaders can think about how to respond to the real progress that’s happening, as well as how to prepare for the automated future. Here are a few things to consider:
- Stay informed about developments in AI and AGI . Connect with start-ups and develop a framework for tracking progress in AGI that is relevant to your business. Also, start to think about the right governance, conditions, and boundaries for success within your business and communities.
- Invest in AI now . “The cost of doing nothing,” says McKinsey senior partner Nicolai Müller , “is just too high because everybody has this at the top of their agenda. I think it’s the one topic that every management board has looked into, that every CEO has explored across all regions and industries.” The organizations that get it right now will be poised to win in the coming era.
- Continue to place humans at the center . Invest in human–machine interfaces, or “human in the loop” technologies that augment human intelligence. People at all levels of an organization need training and support to thrive in an increasingly automated world. AI is just the latest tool to help individuals and companies alike boost their efficiency.
- Consider the ethical and security implications . This should include addressing cybersecurity , data privacy, and algorithm bias.
- Build a strong foundation of data, talent, and capabilities . AI runs on data; having a strong foundation of high-quality data is critical to its success.
- Organize your workers for new economies of scale and skill . Yesterday’s rigid organizational structures and operating models aren’t suited to the reality of rapidly advancing AI. One way to address this is by instituting flow-to-the-work models, where people can move seamlessly between initiatives and groups.
- Place small bets to preserve strategic options in areas of your business that are exposed to AI developments . For example, consider investing in technology firms that are pursuing ambitious AI research and development projects in your industry. Not all these bets will necessarily pay off, but they could help hedge some of the existential risk your business may face in the future.
Learn more about QuantumBlack, AI by McKinsey . And check out AI-related job opportunities if you’re interested in working at McKinsey.
Articles referenced:
- “ Generative AI in operations: Capturing the value ,” January 3, 2024, Marie El Hoyek and Nicolai Müller
- “ The economic potential of generative AI: The next productivity frontier ,” June 14, 2023, Michael Chui , Eric Hazan , Roger Roberts , Alex Singla , Kate Smaje , Alex Sukharevsky , Lareina Yee , and Rodney Zemmel
- “ What every CEO should know about generative AI ,” May 12, 2023, Michael Chui , Roger Roberts , Tanya Rodchenko, Alex Singla , Alex Sukharevsky , Lareina Yee , and Delphine Zurkiya
- “ An executive primer on artificial general intelligence ,” April 29, 2020, Federico Berruti , Pieter Nel, and Rob Whiteman
- “ Notes from the AI frontier: Applications and value of deep learning ,” April 17, 2018, Michael Chui , James Manyika , Mehdi Miremadi, Nicolaus Henke, Rita Chung, Pieter Nel, and Sankalp Malhotra
- “ Augmented and virtual reality: The promise and peril of immersive technologies ,” October 3, 2017, Stefan Hall and Ryo Takahashi
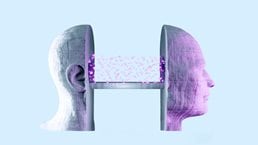
Want to know more about artificial general intelligence (AGI)?
Related articles.
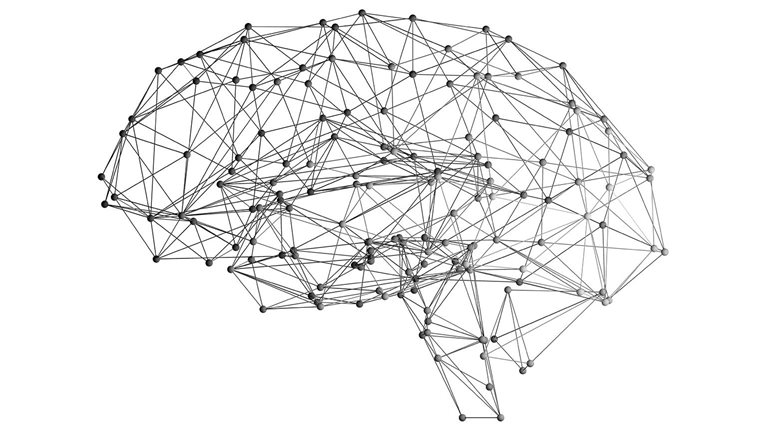
An executive primer on artificial general intelligence

What every CEO should know about generative AI
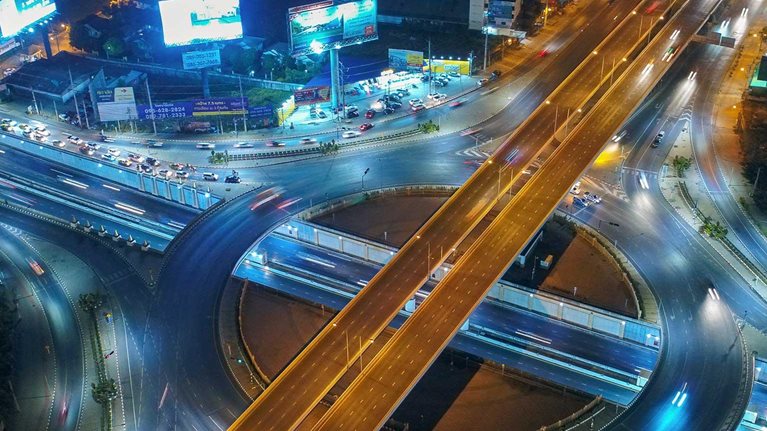
Notes from the AI frontier: Applications and value of deep learning
- Course Description
- Course Info
- Calendar/Links
Theme by Anders Norén
Project: Final Report
Project report.
Due: Wednesday, 4/24
Example report in box folder, linked here
General Directions
The final report is intended to provide a comprehensive account of your collaborative course project in data science. The report should demonstrate your ability to apply the data science skills you have learned to a real-world project holistically, from posing research questions and gathering data to analysis, visualization, interpretation, and communication. The report should stand on its own so that it makes sense to someone who has not read your proposal or prototype.
The report should contain at least the parts defined below. In terms of length, it should be 5-7 pages using standard margins (1 in.), font (11-12 pt), and line spacing (1-1.5). A typical submission is around 3-4 pages of text and 5-7 pages overall with tables and figures. It is important to stay within the page limit, as practicing being succinct is an important skill. Your final report should also have a descriptive title, not “CS216 Project Report”. You should convert your written report to a pdf and upload it to Gradescope under the assignment “Project Final Report” by the due date, and assign the appropriate pages to questions in the grading rubric. Be sure to include your names and NetIDs in your final document and use the group submission feature on Gradescope. You do not need to upload your accompanying data, code, or other supplemental resources demonstrating your work to Gradescope; instead, your report should contain instructions on how to access these resources (see the Results and Methods section below for more details).
In general, your approach to this report should be to write as if you had “planned this as your project all along.” A report is not a chronological story of your project, it is a summary of what you did where the “story” serves the reader’s comprehension.
- E (Exemplary, 20pts) – Work that meets all requirements in terms of formatting and sections.
- S (Satisfactory, 19 pts) – Work that meets all requirements but is over 7 pages.
- N (Not yet, 12pts) – Does not meet all requirements.
- U (Unassessable, 4pts) – Missing at least one section.
Part 1: Introduction and Research Questions (15 points)
Your final report should begin by introducing your topic and restating your research question(s) as in your proposal. As before, your research question(s) should be (1) substantial, (2) feasible, and (3) relevant. In contrast to the prior reports, the final report does not need to explicitly justify that the research questions are substantial and feasible in the text ; your results should demonstrate both of these points. Therefore, you should remove that text to save space.
You should still explicitly justify how your research questions are relevant. In other words, be sure to explain the motivation of your research questions. Remember that relevant research questions address a subject of importance and interest within the scientific community or broader society. Additionally, we are looking for why your group believes this research project is worthwhile to your time in this course.
You can start with the text from your prototype, but you should update your introduction and research questions to reflect changes in or refinements of the project vision. You should not state specific updates, rather, write the report as the final product and the prior milestones do not exist. Pretend the readers are unaware of the prior milestones. If you feel like an explanation of changes since the prototype is warranted, place that in the appendix. Your introduction should be sufficient to provide context for the rest of your report.
- E (Exemplary, 15pts) – Comprehensive introduction with clearly labeled, up-to-date research questions and a justification for how the research questions are relevant. Report introduction can stand alone without references to prior versions of the project; no text for explicit justifications for “substantial” and “feasible” are made for the research question(s).
- S (Satisfactory, 14pts) – Comprehensive introduction with clearly labeled, updated research questions and a justification for how the research questions are relevant. The introduction and research questions may not have been refined from the prototype (they have still kept reasoning for why their research questions are substantial and feasible).
- N (Not yet, 9pts) – Incomplete introduction where the research questions or justification are missing pieces, but at least some of it is present. Or the justification is clearly not reasonable.
- U (Unassessable, 3pts) – Incomplete introduction where it is entirely missing the research questions or justification or does not demonstrate meaningful effort.
Part 2: Data Sources (15 points)
Discuss the data you have collected and are using to answer your research questions. Be specific: name the datasets you are using, the information they contain, and where they were collected from / how they were prepared. You can begin with the text from your prototype, but be sure to update it to fit the vision for your final project.
- E (Exemplary, 15pts) – Origins of data are properly specified, cited, and relevant to answering the research question(s). If any significant data wrangling, cleaning, or other data preparation was done, these processes are explained.
- S (Satisfactory, 14pts) – Origins of data are properly specified and cited. However, the justification is not clear why the data is relevant to the proposed research question(s). If any significant data wrangling, cleaning, or other data preparation was done, these processes are explained.
- N (Not yet, 9pts) – Poorly specified data sources or the justification for using that data set or the methods to acquire the data is lacking. No discussion of preparing the dataset.
- U (Unassessable, 3pts) – Data sources or methods to acquire data are missing or do not demonstrate meaningful effort.
Part 3: What Modules Are You Using? (15 points)
Your project should utilize concepts from modules we have covered in this course to answer your research question(s). We will assume you will use modules 1 (Python), 2 (Numpy/Pandas), and 5 (Probability). Your final report should state at least 3 more modules that you have utilized for your project. Each module should have a short description of how you used the knowledge in this module and a justification for that use. In addition, include what specific concepts from the module you used and at what stage of your project you mostly used this module. Potential stages include, but are not limited to: data gathering, data cleaning, data investigation, data analysis, and final report.
- Module 3: Visualization
- Module 4: Data Wrangling
- Module 6: Combining Data
- Module 7: Statistical Inference
- Module 8: Prediction & Supervised Machine Learning
- Module 9: Databases and SQL
- Module 10: Deep Learning
Your overall report should clearly show that you used the modules discussed in this section. You should add any additional modules used and update the existing modules to be more specific to the different tasks and stages of your projects that changed since your prototype.
- E (Exemplary, 15pts) – States at least 3 modules. For each module, they provide an updated (1) short description of how they used the module, (2) justification for using this module, (3) specific concepts they used, (4) what stage they used it, and (5) clearly implemented it in the final report.
- S (Satisfactory, 14pts) – States at least 3 modules. For each module, they provide an updated (1) short description of how they used the module, (2) justification for using this module, (3) what concepts they used and (4) what stage they used it. Less than 3 modules are clearly implemented in the final report.
- N (Not yet, 9pts) – States at least 3 modules. For each module, they provide an updated (1) short description of how they used the module, (2) justification for using this module, (3) what concepts they used and (4) what stage they used it. Only one module is clearly implemented in the final report.
- U (Unassessable, 3pts) – Does not meet the Not Yet criteria.
Part 4: Results and Methods (15 points)
This is likely to be the longest section of your paper at multiple pages. The results and methods section of your report should explain your detailed results and the methods used to obtain them. Where possible, results should be summarized using clearly labeled tables or figures and supplemented with written explanations of the significance of the results with respect to the research questions outlined previously. Please note that a screenshot of your dataset does not count as a table or figure and should not be included in your final report.
Your description of your methods should be specific. For example, if you scraped multiple web databases, merged them, and created a visualization, then you should explain how each step was conducted in enough detail that an informed reader could reasonably be expected to reproduce your results with time and effort. Just saying, “we cleaned the data and dealt with missing values” or “we built a predictive model” is insufficient detail.
Your report should also contain instructions on how to access your full implementation (that is, your code, data, and any other supplemental resources like additional charts or tables). The simplest way to do so is to include a link to the box folder, GitLab repo, or whatever other platforms your group is using to house your data and code.
- E (Exemplary, 15pts) – Results are thoroughly discussed using clearly labeled tables or figures followed by written descriptions. Specific explanation of how the results were generated and from what data. Link to code/data to create charts or visualizations is provided.
- S (Satisfactory, 14pts) – Results are thoroughly discussed using clearly labeled tables or figures followed by written descriptions. Explanation of how the results were generated may lack some specification or it is somewhat unclear as to what data the results are from. Link provided.
- N (Not yet, 9pts) – Results are discussed using tables with missing labels or lacking written descriptions. It is unclear how the results were generated and from what data.
- U (Unassessable, 3pts) – Results are missing or do not demonstrate meaningful effort.
Part 5: Limitations and Future Work (10 points)
In this part, you should discuss any important limitations or caveats to your results with respect to answering your research questions. For example, if you don’t have as much data as you would like or are unable to fairly evaluate the performance of a predictive model, explain and contextualize those limitations. You may want to consider any ethical implications or potential biases of your results as well.
Finally, provide a brief discussion of future work. This could explain how future research might address the limitations you outline, or it could pose additional follow-up research questions based on your results so far. In short, explain how an informed reader (such as a peer in the class) could improve on and extend your results.
- E (Exemplary, 10pts) – Comprehensive and explicit discussion of important limitations and caveats to results. Brief discussion of future work and how results could be extended and improved upon.
- S (Satisfactory, 9pts) – Discussion of important limitations and caveats to results could be improved or the discussion of future work and how results could be extended and improved upon lacks some specification.
- N (Not yet, 6pts) – Incomplete discussion of important limitations and caveats to results. Discussion of future work and how results could be extended and improved upon may lack some specification.
- U (Unassessable, 2pts) – Limitations and future work are missing or do not demonstrate meaningful effort.
Part 6: Conclusion (5 points)
Provide a brief (one or two paragraphs) summary of your results. This summary of results should address all of your research questions.
If one of your research questions was “Did COVID-19 result in bankruptcy in North Carolina during 2020?” then a possible (and purely hypothetical) summary of results might be:
We aggregate the public records disclosures of small businesses in North Carolina from January 2019 to December 2020 and find substantial evidence that COVID-19 did result in a moderate increase in bankruptcy during 2020. This increase is not geographically uniform and is concentrated during summer and fall 2020. We also examined the impact of federal stimulus but cannot provide an evaluation of its impact from the available data.
- E (Exemplary, 10pts) – Research questions are clearly and completely addressed through a summary of results.
- S (Satisfactory, 9pts) – Research questions are clearly addressed through a summary of results. The results may be lacking in completely answering the research questions.
- N (Not yet, 6pts) – Research questions are somewhat addressed through a summary of results. The results are lacking in completely answering the research questions. Or the results of one of the research questions is missing.
- U (Unassessable, 2pts) – Conclusion is missing or does not demonstrate meaningful effort.
(Optional) Part 7: Appendix of additional figures, tables, and updates summary.
If you are struggling to keep your report within the 5-7 page limit, you may move some (not all) of your figures and tables to an optional appendix that will not count against your page limit. However, your report should stand on its own without the appendix . The appendix is for adding more nuance to your results, not to give you more space to talk about your results. Succinctness is an important skill to practice when doing data science. Your grader is not expected to look at the appendix when grading.
If you strongly feel like a summary of project updates since the proposal is required, you may put them in this appendix as well and mention they are in the appendix in the introduction.
Checklist Before You Submit:
- 5-7 pages in length
- Standard margins (1 in.)
- Font size is 11-12 pt
- Line spacing is 1-1.5
- Final document is a pdf
- Descriptive project title
- Do you feel as if this part meets the requirements of E (Exemplary) or S (Satisfactory) ?
Author Joey Scarpa
Posted March 30, 2024 — 4:30 pm
Categories Project
Leave a Reply Cancel reply
Your email address will not be published. Required fields are marked *
Save my name, email, and website in this browser for the next time I comment.
Thank you for visiting nature.com. You are using a browser version with limited support for CSS. To obtain the best experience, we recommend you use a more up to date browser (or turn off compatibility mode in Internet Explorer). In the meantime, to ensure continued support, we are displaying the site without styles and JavaScript.
- View all journals
- My Account Login
- Explore content
- About the journal
- Publish with us
- Sign up for alerts
- Open access
- Published: 13 March 2024
Global supply chains amplify economic costs of future extreme heat risk
- Yida Sun ORCID: orcid.org/0000-0001-8160-1358 1 na1 ,
- Shupeng Zhu ORCID: orcid.org/0000-0002-8446-9329 2 , 3 na1 ,
- Daoping Wang ORCID: orcid.org/0000-0001-5221-4965 4 , 5 na1 ,
- Jianping Duan 6 ,
- Hui Lu ORCID: orcid.org/0000-0003-1640-239X 1 , 7 ,
- Hao Yin 8 ,
- Chang Tan 1 ,
- Lingrui Zhang 9 ,
- Mengzhen Zhao 10 ,
- Wenjia Cai ORCID: orcid.org/0000-0002-4436-512X 1 ,
- Yong Wang ORCID: orcid.org/0000-0002-5831-9466 1 ,
- Yixin Hu ORCID: orcid.org/0000-0002-3986-9059 11 ,
- Shu Tao ORCID: orcid.org/0000-0002-7374-7063 12 &
- Dabo Guan ORCID: orcid.org/0000-0003-3773-3403 1 , 13
Nature volume 627 , pages 797–804 ( 2024 ) Cite this article
14k Accesses
249 Altmetric
Metrics details
- Interdisciplinary studies
Evidence shows a continuing increase in the frequency and severity of global heatwaves 1 , 2 , raising concerns about the future impacts of climate change and the associated socioeconomic costs 3 , 4 . Here we develop a disaster footprint analytical framework by integrating climate, epidemiological and hybrid input–output and computable general equilibrium global trade models to estimate the midcentury socioeconomic impacts of heat stress. We consider health costs related to heat exposure, the value of heat-induced labour productivity loss and indirect losses due to economic disruptions cascading through supply chains. Here we show that the global annual incremental gross domestic product loss increases exponentially from 0.03 ± 0.01 (SSP 245)–0.05 ± 0.03 (SSP 585) percentage points during 2030–2040 to 0.05 ± 0.01–0.15 ± 0.04 percentage points during 2050–2060. By 2060, the expected global economic losses reach a total of 0.6–4.6% with losses attributed to health loss (37–45%), labour productivity loss (18–37%) and indirect loss (12–43%) under different shared socioeconomic pathways. Small- and medium-sized developing countries suffer disproportionately from higher health loss in South-Central Africa (2.1 to 4.0 times above global average) and labour productivity loss in West Africa and Southeast Asia (2.0–3.3 times above global average). The supply-chain disruption effects are much more widespread with strong hit to those manufacturing-heavy countries such as China and the USA, leading to soaring economic losses of 2.7 ± 0.7% and 1.8 ± 0.5%, respectively.
Similar content being viewed by others
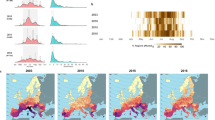
Current and projected regional economic impacts of heatwaves in Europe
David García-León, Ana Casanueva, … Lars Nybo
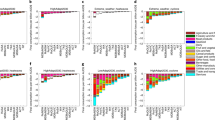
Impacts of climate change and extreme weather on food supply chains cascade across sectors and regions in Australia
Arunima Malik, Mengyu Li, … Mikhail Prokopenko
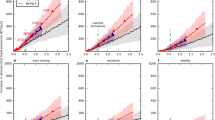
Exposure to excessive heat and impacts on labour productivity linked to cumulative CO2 emissions
Yann Chavaillaz, Philippe Roy, … H. Damon Matthews
Research has been showing a trend in rising temperature and increasing occurrence of extreme heatwaves since the 1950s 1 , 2 . This continuous pattern raises concerns about the potential impacts of climate change and its associated socioeconomic costs. Notable effects of heat stress are on human health and labour productivity. On the one hand, global heat stress makes it difficult for the body to maintain its core temperature, thereby increasing morbidity and mortality from heat stroke 5 , 6 , 7 . Countries across all latitudes, including Russia 8 , the USA 9 , China 5 , Australia 10 and North Africa 11 have suffered from increased heat stress since the deadly European heatwave in 2003 12 , which caused considerable mortality and morbidity. On the other hand, biometeorological studies suggest that heat stress can seriously decrease labour productivity 13 , 14 , 15 , 16 , measured in terms of lost worktime from recommended work/rest ratios during heat stress, reduced work efficiency as estimated from exposure–response functions and self-reported reduced work efficiency 13 , 17 , 18 .
In the context of increasingly integrated global supply chains, the impacts of heat stress are not just confined to specific populations and industrial sectors in low latitudes but extend to wider regions and sectors 19 , 20 , 21 , 22 . For example, a Western European country such as the UK is rarely directly and severely affected by heat stress. However, consumption of beer or coffee in the UK can drop as a result of the severe impact of heat stress on wheat and coffee bean suppliers in Africa and South America 23 . This kind of spillover effect can have important consequences in terms of global food security 24 , 25 , 26 , energy supply 27 and the supply of various mineral products 28 .
The direct mortality and productivity loss resulting from heat stress have been extensively studied. However, the indirect losses due to supply-chain disruptions have not been fully analysed 29 , 30 , as previous literature has either devoted insufficient discussion to the indirect effects by only reporting the total/aggregated effects 31 , 32 , 33 or ignored the amplifying effect of the global trade system on direct losses. As climate change will make the impacts of heat stress worse over time, developing methodologies that allow comprehensive quantifications of both the direct and indirect impacts of heat stress on human systems can help policy-makers to develop more effective climate change mitigation and adaptation policies. In this study, a disaster footprint analytical framework, by integrating climate, epidemiological and hybrid input–output and computable general equilibrium global trade modules, was constructed to provide a comprehensive assessment of the impact of heat stress on socioeconomic systems to 2060, including health loss (excess mortality due to extreme heatwaves), labour productivity loss (decreased daily labour productivity due to higher temperature and humidity) and indirect loss (production stagnation due to lack of supply or demand) across 141 regions and 65 sectors worldwide. Details of our analytical approach are provided in the Methods . In summary, we use the sixth phase of the coupled model intercomparison project phase 6 (CMIP6) 34 , 35 , where 14 widely applied global climate models (GCMs) are averaged to assess future daily temperature and humidity parameters. Grid-scale daily excess mortality (health loss) and labour loss rates (labour productivity loss) are calculated on the basis of empirical functions and statistics from previous studies 36 . On the basis of the above labour constraints in different regions and industries, a hybrid input–output and computable general equilibrium global trade module was developed in the disaster footprint analytical framework to assess the pattern of heat-related economic losses transmitted through the global supply chain. By quantifying indirect effects that were hardly analysed before, this model provides insight into the far-reaching impacts of heat stress across global supply chains and how such impacts evolve spatially and over long time scales. The estimated results are based on static production and trade relationships which may not accurately address the dynamic nexus among industries and countries in the long-term.
This study examines three scenarios combining various representative concentration pathways (RCPs) and shared socioeconomic pathways (SSPs). RCPs represent greenhouse gas concentration trajectories as adopted by the Intergovernmental Panel on Climate Change (IPCC). Each RCP scenario implies different magnitudes of future heat stress. SSPs represent socioeconomic development pathways. Different SSP scenarios imply different amounts of risks of heat stress exposure and societal adaptive capacity. Three SSP–RCP scenarios were considered: SSP 585, SSP 245 and SSP 119. Scenario SSP 585 represents a world of rapid and unconstrained growth in economic output and energy use. Scenario SSP 245 represents the middle of the range of plausible future pathways 37 , reflecting the continuation of historical mitigation efforts 38 . In scenario SSP 119, the world shifts pervasively toward a more sustainable path, emphasizing more inclusive development that respects perceived environmental boundaries. These three scenarios, from high carbon to sustainable trajectories, allow the quantification of the potential economic benefits of ambitious emissions reduction policies that have previously received little attention.
Nonlinear growth trend of global heat-related losses
Figure 1a–d depicts the total global economic loss and the specific components. Under the SSP 119 scenario, the total global gross domestic product (GDP) loss is 0.9% (0.6–1.1%) in 2040 and each component is estimated as follows: health loss (0.5%), labour productivity loss (0.3%) and indirect loss (0.1%). In 2060, global GDP loss slightly decreases to 0.8% (0.4% health loss, 0.3% labour productivity loss and 0.1% indirect loss), amounting to about US $3.75 trillion (values are constant 2020 price). The number of global average heatwave days (definition and calculation detailed in the Methods ) would increase by 24% compared to 2022 and the average annual number of heatwave deaths would be around 0.59 million (0.44–0.74 million). In the case of a high-emissions, high-growth development path, SSP 585, economic losses in 2060 increase by 500% compared to the SSP 119 scenario, up to 3.9% (2.9–4.5%) (1.6% health loss, 0.8% labour loss and 1.5% indirect loss), with a value of about US $24.70 (18.36–28.80) trillion. The global annual heatwave days would be 104% higher compared to 2022 and the global average annual number of heat-induced deaths would increase to around 1.12 million (0.85–1.39 million). The labour and health loss on regional and global scales are close to the results of previous studies 39 , 40 , 41 .
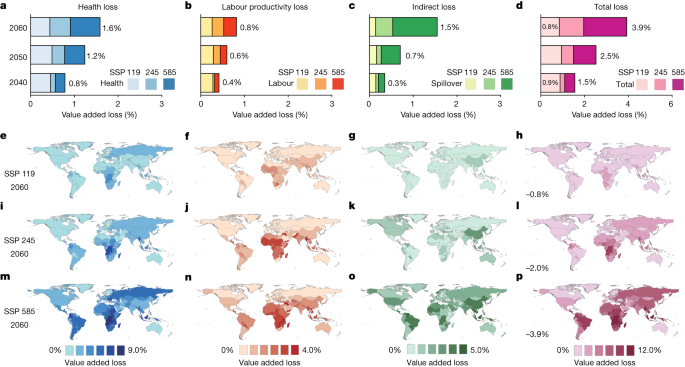
a – d , Evolutionary trends of the four types of losses from 2040 to 2060 under different scenarios (health loss ( a ); labour productivity loss ( b ); indirect loss (supply-chain disruptions) ( c ); and the total losses ( d )). The colours from light to dark, represent the economic losses from the three scenarios SSP 119, SSP 245, SSP 585, respectively. e – p , The spatial distribution of global losses as a percentage of each country’s GDP at midcentury under the SSP 119 ( e – h ), SSP 245 ( i – j , l ) and SSP 585 ( m – p ) scenarios. The values shown are 10-year averages (for example, loss reported in 2060 represents the average loss calculated over the period between 2055 and 2065).
Global economic losses show a nonlinear growth trend with respect to time and degree of heat stress, driven by increased indirect losses. Over time, total losses grow from 1.5% of GDP in 2040 to 2.5% of GDP in 2050 and to 3.9% of GDP in 2060 (Fig. 1d ) under the SSP 585 scenario. However, the proportion of global GDP loss due to supply-chain disruptions is 0.1%, 0.3%, 0.7% and 1.5% per decade from 2030 onwards (Fig. 1c ), showing an exponential-like growth pattern (Extended Data Fig. 5 and Supplementary Figs. 7 and 8 ). Growing indirect losses gradually become the dominant contributor to total losses. Looking at the scenario scale, total GDP losses in 2060 are 0.8% under SSP 119, 2.0% under SSP 245 and 3.9% under SSP 585, of which the losses due to indirect effects are 0.1% of global GDP (13% of total) under SSP 119, 0.5% (25% of total) under SSP 245 and 1.5% (38% of total) under SSP 585. As the degree of heat stress increases progressively, the indirect effects gain more weight in the total losses.
Figure 1e–p explains the mechanism behind the growing weight of the indirect effect in the total losses as the degree of heat stress increases: in terms of spatial patterns, when direct losses are of low to medium magnitude, their impact on the supply network is limited to the regional area; however, when direct losses are severe, they have wider ramifications impacting the supply chain globally and giving rise to further, indirect, losses. Under the SSP 119 scenario, health losses are most significant in South-Central Africa and Eastern Europe (Fig. 1e ); labour productivity losses are concentrated in lower latitudes, including West Africa and South Asia ( Fig. 1f ); indirect losses are concentrated in Central America and East Asia ( Fig. 1g ); in general, Central and Southern Africa, Southeast Asia and Latin America have the most severe total losses ( Fig. 1h ). The spatial patterns of direct economic loss of labour and health under the three scenarios are similar. However, it is noteworthy that persistent and severe heat stress expected under the rapid growth SSP 585 scenario leads to substantial disruptions beyond the regional scale through to global value chains (GVCs). Countries such as Brazil, China and Norway all suffer substantial economic ripple losses. China’s indirect economic losses due to supply-chain disruptions soar from 0.4% under SSP 119 to 2.7% of GDP under SSP 585, Brazil from 0.2% to 2.5% and Norway from less than 0.1% to 2.1%. Although developed countries at high latitudes can mitigate most potential losses through adaptation strategies such as air conditioning under SSP 119 scenario, they remain exposed to risk of declining supply or demand in the GVCs under SSP 585 scenario (Fig. 1o and Supplementary Fig. 10 ). European Union (EU) countries will face considerable indirect losses due to their trading partners’ reduced production capacity of minerals and food products, especially developing countries. Although severely affected countries in South Asia or Africa are not core trading partners of the EU and trade volumes between such countries and the EU are relatively small, indirect economic losses in the EU will be amplified when many of those developing countries are affected by heat stress.
Different sensitivities to heat stress across countries
Different economies face different risk of losses from heat stress, depending on their geographical locations and the position they occupy in the global supply chain. First, countries whose densely inhabited districts are expected to suffer from severe future warming and temperature anomalies, are the most vulnerable to health losses in terms of excess mortality. Under the SSP 119 scenario, South-Central Africa’s GDP loss due to heatwave deaths is 1.8% (1.2–2.5%) in 2060, the highest in the world. It is followed by Trinidad and Tobago (1.7%), Sri Lanka (1.5%) and Indonesia (1.5%; Fig. 2a ). Vulnerability to health impacts depends on the frequency of extreme weather events and the amount of adaptive capacity. For example, Hungary and Croatia suffer considerable health losses, even though in these countries the climate is cooler than in the Middle East and North Africa. Unlike labour losses, which occur in regions with very high average temperature and humidity, health losses depend largely on the variance and abrupt changes in summer temperatures. As climate change will lead to more frequent and intense heatwaves, populations in cooler climatic zones will experience considerable loss of life if the adaptive capacity does not keep pace with the abrupt and sudden changes.
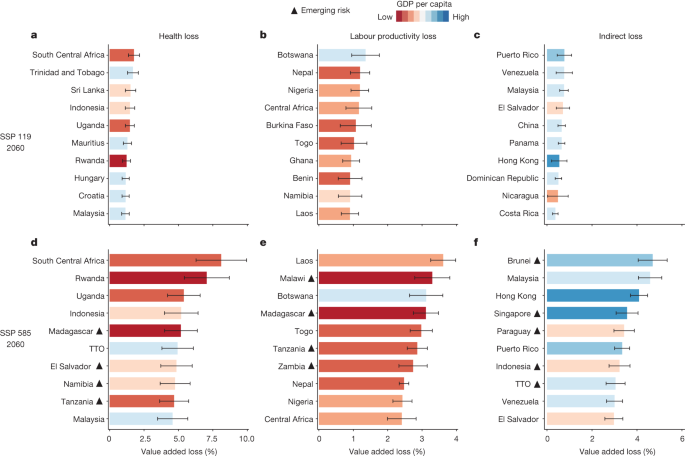
a – c , Top ten climate change-sensitive regions with the most severe health losses ( a ), labour productivity losses ( b ) and indirect losses ( c ) in 2060, under SSP 119 scenario. d – f , Top ten climate change-sensitive countries with the most severe health losses ( d ), labour productivity losses ( e ) and indirect losses ( f ) in 2060, under SSP 585 scenario. The countries marked with black triangles are newly ranked among the most vulnerable countries in 2060 under the SSP 585 scenario compared with SSP 119. The values shown are 10-year averages. Error bars represent 1 s.d. from the mean of decadal data. Upper and lower limits indicate mean + s.d. and mean − s.d., respectively. TTO, Trinidad and Tobago.
Second, low-income emerging economies in the warmest climatic zones are more likely to suffer labour productivity losses. Under the SSP 119 scenario for 2060, countries such as Botswana, Nepal and Nigeria suffer substantial labour productivity losses, up to 1.3%, 1.2% and 1.2% of GDP, respectively (Fig. 2b ). These emerging economies are predominantly located in southern and western Africa (except Nepal), where scorching climates combined with substantial warming over time result in labour-intensive activities during summer months being conducted under increasingly high temperatures. To add insult to injury, most of these countries depend on primary industries such as agriculture, forestry, mining and construction, where workers are mostly outdoors and will be severely affected by extreme heat. For example, agriculture accounts for 21.3% of Nepal’s GDP and 23.4% of Nigeria’s, whereas mining contributes to nearly 28% of Botswana’s GDP 42 . The widespread suspension and reduction of production in the agroforestry and extractive industries due to heat stress will have serious repercussions on national economies and international trade balances. Consequently, these countries are among the most affected by the loss of labour productivity.
Third, small to medium-sized economies with strong and diverse connections to the most affected regions in the GVC, are highly vulnerable to indirect effects. In the context of the SSP 119 scenario, value chains in Latin America and Southeast Asia are the most severely affected (Fig. 2c ). Puerto Rico suffered the highest losses, estimated at 0.8% (0.5–1.1%) of GDP, whereas Venezuela, Malaysia and other Latin American countries, including El Salvador, Panama and Dominican Republic, lost approximately 0.4–0.8% of GDP. Under the SSP 585 scenario, Southeast Asian economies such as Brunei, Malaysia, Singapore and Indonesia suffer the most. These losses stem from strong trade connections with highly vulnerable countries. For example, Brunei and Singapore are exposed to indirect effects as they import nearly 60% of their annual mineral and metal products from China, Malaysia and Indonesia. Caribbean countries like Puerto Rico and Panama generally have less economic diversity and depend heavily on the service sector and international trade. The complex mechanism of transmitting losses along the value chain necessitates thorough consideration by countries for managing future risk of instability across critical industries.
A comparison between the SSP 119 (Fig. 2a–c ) and SSP 585 (Fig. 2d–f ) scenarios shows that losses do not increase uniformly across developing and developed countries when faced with severe climate change impacts, indicating uneven exposures to climate risk. Under the high-emission SSP 585 pathway, a substantial portion of the rapidly escalating economic losses is shouldered by developing countries. Despite Africa contributing less than 5% of global greenhouse gas emissions, 12 countries in the continent, including Rwanda, Botswana, Uganda and Malawi, are projected to suffer some of the most substantial economic losses globally by the midcentury. Several East African countries such as Malawi, Madagascar and Tanzania are highly expected to suffer labour productivity losses of approximately 2.5–4.0% of GDP. Regarding health losses, South-Central Africa and Rwanda experience GDP losses of 8.6% and 7.2%, respectively, almost five times more compared to the SSP 119 scenario. In the SSP 585 scenario, indirect losses become more widespread, affecting both developed and developing economies. Brunei incurs the highest indirect losses at 4.7% (4.0–5.3%) of its GDP, whereas other emerging economies like Paraguay and Indonesia lose around 3.3% of their GDP. These findings demonstrated that the rapid growth of income and air-conditioning penetration in emerging economies under the SSP 585 scenario falls short of counteracting the immense impact of climate change on their economies.
Asymmetric effects of heat stress on global supply chains
Figure 3 highlights the three types of losses for sectors experiencing the highest losses across representative countries. The crop farming, construction and mining sectors are the most affected in most countries, especially in several African and Asian countries that rely on primary industries. For instance, an average summer wet bulb globe temperature (WBGT) above 30 in Tanzania challenges the ability of most outdoor workers to adapt in the midcentury. Sectors requiring workers to be directly exposed to sunlight, such as construction and farming, will suffer a loss of value-added (VA) of 1.9% in 2040 under the SSP 119 scenario. In 2060, rising incomes and a stable climate will result in a slightly reduced VA loss of 0.3% explained by lower labour productivity and health losses. However, under the high warming SSP 585 scenario, the same VA loss increases to 3.9% in 2040 and soars to 8.1% in 2060. In addition, most indoor manufacturing industries suffer a VA loss of 6.0–7.4% in 2060 under the SSP 585 scenario. As demonstrated in Extended Data Fig. 9 , countries like Tanzania, Zimbabwe and other African countries exhibit similar patterns of loss. Countries with comparable loss patterns tend to be situated at low latitudes, particularly in the Middle East, South Asia and Africa—regions most threatened by climate change. Most indoor manufacturing and service industries in developing countries have limited access to air conditioning and, as a result, labour capacity and economic development will be severely undermined by climate change.
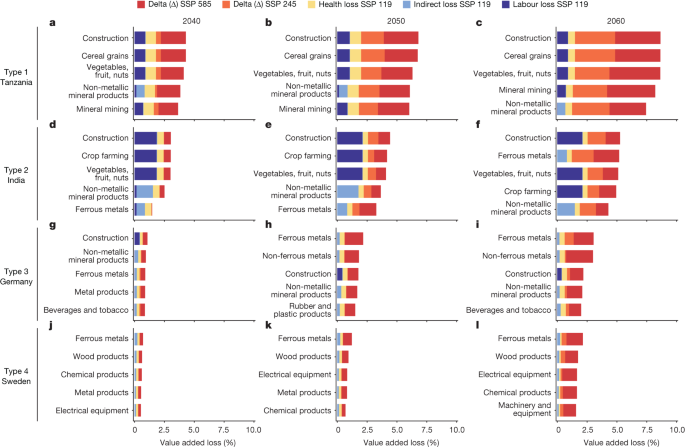
a – l , Showing the top five most vulnerable sectors in Tanzania ( a – c ), India ( d – f ), Germany ( g – i ) and Sweden ( j – l ) in 2040 ( a , d , g , j ), 2050 ( b , e , h , k ) and 2060 ( c , f , i , l ). Sectors with absolute VA losses exceeding the median were ranked by percentage of VA losses, from highest to lowest. The length of the bar represents the 10-year average percentage VA loss of a sector. Sectors with the same percentage loss (for example, wheat, rice and cereals) were combined. Colours indicate the three categories of losses under SSP 119: health losses (yellow bars), labour productivity losses (purple bars) and indirect losses (azure bars). The orange and red bars represent the increment of the total loss under SSP 245 and SSP 585 (without differentiating by type of loss), respectively. The four types of countries were derived by machine-learning clustering based on sectoral patterns of economic loss (Supplementary Figs. 11 and 12 and Supplementary Table 3 ).
Non-metallic products and ferrous metals are vulnerable to climate change because of simultaneous supply-chain shocks from both upstream (supply) and downstream (demand). For example, a country such as India is affected directly by high temperatures and indirectly by the close links with countries severely impacted by heat stress. In 2040, losses in non-metallic manufacturing are second only to construction and agriculture sectors at 2.2% of sectoral VA, whereas ferrous metals industry loses 1.4% of VA. These losses can be attributed to both insufficient demand in the domestic construction sector and shortage of minerals and coal supplies from countries in Southeast Asia and Africa (for example, Indonesia and South Africa). In 2060, under SSP 585 scenario, with the increasingly frequent shutdowns in mining and construction industries under extreme summer heat stress, the ferrous metals industry in India suffers the most substantial VA loss at 5.0%, of which more than 70% is due to indirect losses, followed by the loss from non-metallic manufacturing industry at 3.9%. The sectoral patterns of loss in India are characterized by a combination of health, labour and indirect losses. The decline in labour productivity in the domestic construction and plantation industries leads directly to high economic losses in the country’s related value chains. As shown in Extended Data Fig. 10 , countries located at low and middle latitudes, such as China and Vietnam, exhibit similar patterns of loss.
Light manufacturing, including metal products, rubber and plastic products, food processing and beverages and tobacco, are vulnerable to indirect effects because of a lack of raw materials supply, such as minerals, metals, crops, oil seeds and vegetables. For example, under the SSP 119 scenario, metal products and tobacco and beverage manufacturing in Germany lose around 0.3% of VA in 2040. Under the SSP 585 scenario, the economic loss of beverages and tobacco would increase by more than six times in 2060, reaching 2.0% of VA as imports of plantation products (palm oil, soybeans, coffee, spices and so on) from South America, Southeast Asia and Africa decline by around 5% to 8% (Extended Data Fig. 8 ). Losses of metal products rise even faster, reaching 2.4% of VA in 2060. This is because the main producers of raw materials, such as coal and metals, which are essential for the metal products industry, are primarily located in regions that are vulnerable to climate change. This leads to higher losses in the metal product-related chain in most countries with developed manufacturing industries, including Germany, France and Australia (Extended Data Fig. 11 ). These countries have a relatively low share of agricultural GDP (less than 3%), with slight losses. Labour productivity losses are high only in the construction or mining sector, whereas indirect losses are higher in the metal-related manufacturing sector because of insufficient supply from foreign trading partners.
Similarly, high-end machinery, equipment and chemical products industries suffer indirect losses as a result of multilevel cascading effects, even in very cool climates. Losses in these industries, especially in developed countries such as European countries, emerge slowly and are not substantial under the SSP 119 scenario but increase sharply under SSP 245 and SSP 585 scenarios. For example, Sweden’s industry-wide production suffers mainly from indirect losses through supply-chain disruptions and excess mortality due to heatwaves. From 2040 to 2060 under the SSP 119 scenario, impacts on production activities are moderate given the cool climate and dependence on the stable EU supply chain. Sectors like electrical equipment and chemical products experience less than 1% of VA loss, mostly health loss due to sudden extreme heatwaves. However, sector VA losses soar under the SSP 585 scenario. Losses in the mechanical equipment sector increase rapidly, growing by approximately five times compared to the SSP 119 scenario. Ferrous metals (2.2%), electrical equipment (1.9%) and machinery and equipment (1.6%) experience the highest VA losses. Indirect losses become a main constraint in many sectors because national adaptive strategies or close regional trade flows (as in the EU) can no longer support production when heat stress becomes increasingly more severe globally. As shown in Extended Data Fig. 12 , developed economies located at high latitudes, such as Norway and the UK, are characterized by similar loss patterns.
We also analyse the mechanism through which indirect losses from disruptions in international trade flows propagate through national supply chains of specific sectors. Figure 4 illustrates how climate risk propagates through two supply chains, the Indian food production and the Dominican Republic tourism sectors, respectively (see Extended Data Fig. 8 for other typical supply chains). Each of these sectors is important to the respective economies of India (13% of GDP) and the Dominican Republic (18% of GDP) and each is largely dependent on international supply chains. In the case of India’s food sector, we see a pattern of ‘upstream constraint’ through which insufficient upstream supply of intermediates (such as palm oil from Indonesia) impacts the downstream sector and the entire value chain, whereas in the case of tourism in the Dominican Republic, we see a pattern of ‘downstream constraint’—the impact of insufficient downstream demand affects the upstream sector and the entire value chain.
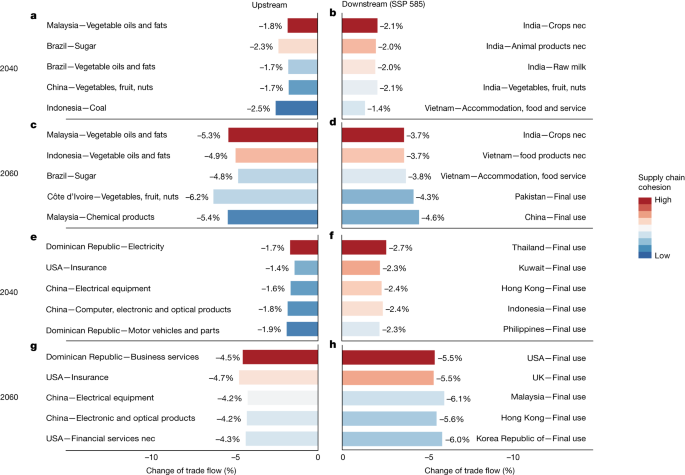
a – d , Trade flows between India food production sector and upstream ( a , c ) and downstream ( b , d ) sectors in 2040 ( a , b ) and 2060 ( c , d ). e – h , Trade flows between Dominican Republic tourism sector and upstream ( e , g ) and downstream ( f , h ) sectors in 2040 ( e , f ) and 2060 ( g , h ). Each bar represents a key trading partner (sector with trade volume above the 50% quartile of trade volumes of the selected sector with all partner sectors) and the length represents the percentage decrease in product flow compared to the base period of 2014. The colours of the bars represent the cohesion level of the particular sector to the Indian food production sector from blue (weak) to red (strong), which is measured by the trade volume between the particular sector and the Indian food production sector. nec, not elsewhere classified.
The supply chain of the Indian food production industry relies heavily on its upstream suppliers, the oil and fat sectors of Indonesia and Malaysia, and as a result it is vulnerable to higher temperatures. The unmitigated warming under the SSP 585 scenario exacerbates the shortage of raw materials. By 2060, palm oil supplies from Malaysia and Indonesia fall by 5.3% and 4.9%. Additionally, Brazilian sugar, Southeast Asian and African vegetables, fruits and nuts are also less available, with a supply decreased by around 4–6%. Consequently, downstream countries, including India, Vietnam, Pakistan and other important trading partners, experience a contraction of imports between 3.7% and 5.1% (Extended Data Fig. 6 ). These impacts can negatively affect food prices and security in both developing and developed countries.
In contrast to the Indian food production industry, the Dominican Republic’s tourism industry is more constrained by downstream demands. Under the SSP 585 scenario, the wealth generated by tourism in the Dominican Republic could drop substantially, as the largest source of foreign visitors, the USA, is likely to reduce annual demand for tourism in the Dominican Republic on average by around 5.5%. Demand from Malaysia and Indonesia is likely to fall by 6.1% and 8.1%. A drop in tourism output, the backbone of the Dominican Republic’s economy, is likely to reduce demand for upstream business services and manufacturing industries by approximately 4.5–4.7%, causing an extra impact on the Dominican national economy. The decline in the tourism sector is also likely to lead to a 4.7% and 4.3% drop in the Dominican Republic’s demand for insurance and financial services from the USA, as well as 4.2% and 4.5% drop in the demand for electronic equipment and chemical products from China (Extended Data Fig. 7 ). Furthermore, a smaller tourism sector leads to the slowdown in the construction of tourism infrastructure and the supply of tourism supporting products, posing considerable risks for tourism investment.
Implication for targeted risk governance and regional cooperation
By coupling climate, epidemiological and economic models, this study investigates the direct impact of heat stress on human activities and the indirect losses across the broader global supply chain. Focusing on the indirect effects of heat stress addresses a substantial gap in the literature. Comprehending the indirect effects of heat stress is crucial for devising effective and targeted adaptation strategies in the context of increasingly complex global supply-chain networks.
Our findings show that supply chains amplify the risk of future heat stress by causing nonlinear economic losses worldwide. In other words, the considerable adverse indirect effects of heat stress across interconnected markets cannot be overlooked. The indirect losses of heat stress highlight the need for countries to strengthen collaboration across global relevant supply-chain stakeholders to achieve successful heat stress adaptation. For instance, our results demonstrate that the impact of a heatwave on the agriculture and food manufacturing industry in India can further lead to a 0.9–2.3% loss of VA in the US food manufacturing industry. If the USA were to support India’s adaptation efforts through technology transfer, they would indirectly be reducing their own losses. These considerations could guide policy-makers working towards global cooperation for future climate change mitigation and heat stress adaptation efforts.
We also illustrate the sensitivity of different countries and sectors to the three types of losses caused by heat stress. For example, Caribbean and Central African countries are more likely to suffer health losses, whereas for low-income countries in Africa and Southeast Asia labour losses are more likely. By contrast, small to medium-sized economies dependent on international trade, such as Brunei, are more exposed to indirect losses. The way heat stress-related costs emerge demonstrates how extensive and diverse impacts from heat stress are propagated through global supply chains, resulting in economic losses to a country or sector that may not be immediately apparent. Our quantitative results provide valuable information for designing more targeted and effective heat stress adaptation strategies.
Our developed model and estimations are subject to uncertainties and limitation (detailed description in Supplementary Information sections 1.1 – 1.3 ). For example, although the disaster footprint module is widely used and performs well for single-country/single-region analyses, the substitutability of products in a multicountry scenario requires further discussion to ensure robustness. To quantify some of the uncertainties, we conducted a comprehensive sensitivity analysis, with details available in the Methods and Supplementary Information section 1 . Specifically, we used different years and versions of the input–output database for comparison to analyse the uncertainty in production and trade structures (Extended Data Fig. 4 ).
Globally, the estimate of the total amount of indirect losses is robust to changes in the data used (GTAP 2011 and GTAP 2014) for the base period. The results of the loss assessment at global scale differ by less than 5% in 2060. Most countries are distributed around the y = x line, which suggests a consistent assessment across different trade structures. Regionally, for a few countries, indirect loss assessments can show larger differences. By comparison, we find that when using GTAP 2014 data for the base period, indirect economic losses in East and Southeast Asian countries, such as Singapore, Korea and Japan, are amplified (Extended Data Fig. 4 and Supplementary Fig. 6 ). This can be explained by the fact that, in GTAP 2014, those countries have closer economic ties with climate-sensitive markets, including Malaysia, China, India and Vietnam. For instance, trade between Singapore and emerging economies such as China and Vietnam had increased substantially from 2010 to 2014. According to the Singapore Department of Statistics ( https://www.singstat.gov.sg/ ) and the United Nations Commodity Trade Statistics Database ( https://comtrade.un.org ), China became the largest trading partner of Singapore in 2014, up from fourth place in 2011, whereas Vietnam rose to the 13th largest partner in 2014, from the 20th place in 2011. Conversely, Singapore’s total trade share with the EU and the USA decreased slightly over the same period. Similarly, Japan, Korea and Myanmar developed closer trade relationships with emerging markets such as China, India and Vietnam.
The assessment of indirect losses under different trade relationships offers important insights into the likely supply-chain risks posed by climate change. As Africa, South America and Southeast Asia become increasingly involved in GVCs, the resilience of GVCs to the impacts of climate change must be properly assessed, rather than merely considering scale effects and comparative advantage in terms of economic efficiency.
For parameters such as the maximum stock ratio and excess production capacity, we conducted the experiment several times in the range of possible values from previous studies. For trade substitutability, upper and lower bounds of perfect substitution and non-substitution (traditional static input–output model) were used. We elaborate in more detail about the uncertainty intervals of the parameter for the three main modules and perform a Monte Carlo analysis, including simulation of economic loss dynamics for 10,000 periods (Supplementary Table 2 ). We have also conducted an historical validation using several authentic data sources (robustness tests and validation in the Supplementary Information ), encompassing government statistics, empirical studies and institution reports 3 , 43 , 44 , 45 , 46 , 47 (Supplementary Tables 1 , 6 and 7 , and Supplementary Fig. 1 ), in addition to a comparative analysis of previous studies 33 , 39 , 48 concerning future periods based on CMIP5 data and similar RCP scenarios (Extended Data Fig. 5 ).
Despite the uncertainties, our conclusion that projected climate change will continue to increase heat-related risks globally in the coming decades and that global supply chains will amplify economic losses by spreading indirect losses to wider regions, remain robust. Therefore, in the future, the organization of global supply chains should gradually shift from an exclusive focus on efficiency to one that places equal emphasis on efficiency and resilience. A concerted global strategy to reduce emissions will not only directly protect many people in developing economies from direct economic losses of heat stress but will also maintain resilient and efficient global supply chains and contribute to the long-term, sound development of the global economy.
Our methodology, in essence, combines three modules of climate, health and economy with full validation (Extended Data Fig. 1 ). The integrated model links climate module (estimating future climate parameters including surface air temperature and relative humidity and so on), demographic and health module (simulating future world population dynamics and exposure–response functions to warming) and economic module (dynamic footprint of heat-induced labour loss on global economy and supply chain).
Climate module
Fourteen GCMs involved in the framework of CMIP6 (Extended Data Table 1 ) with ten bias-corrected models from ISIMIP3b 49 , 50 are used to estimate the modelled heat stress projection for the end of the twenty-first century. Five models were randomly averaged several times from the climate model ensemble as a Monte Carlo uncertainty analysis. ERA5 re-analysis data 51 from 1985 to 2022 are used for bias-correction and validation. Climatic parameters such as maximum and average temperature and relative humidity on a daily scale are integrated, which are closely related to future working environment (Supplementary Fig. 9 ).
Many institutes, including International Standards Organization (ISO) and US National Institute for Occupational Safety and Health (NIOSH), use WBGT to quantify different amounts of heat stress and define the percentage of a typical working hour that a person can work while maintaining core body temperature. To facilitate the long-term calculation, we use 18 simplified WBGT, which approximates WBGT well using temperature ( T a ) and relative humidity (RH) 52 , 53 as parameters such as solar radiation and wind speed have higher uncertainty and weaker effects at the global scale. To take into account indoor heat exposures for industrial and service sector workers, we used the approximation that indoor WGBT indoor = WBGT outdoor − 4, based on a deduction of the radiation exposure factor from the formula below 18 :
We also calculated the spatial and temporal evolutionary trends in the occurrence of future heatwaves to calculate excess mortality. There is no consistent definition for heatwave worldwide because people may have acclimatized to their local climatic zones and different studies have applied various temperature metrics 54 , 55 . Heatwaves are usually defined by absolute or relative temperature threshold in consecutive days 56 . There are various ways to define a heatwave. For example, the IPCC defines heatwave as “a period of abnormally hot weather, often defined with reference to a relative temperature threshold, lasting from two days to months”, whereas the Chinese Meteorological Administration defined heatwave as “at least three consecutive days with maximum temperature exceeding 35 °C”. Others 31 identified heatwave using the TX90p criterion, that is, when the 90th percentile of the distribution of regional maximum temperatures spanned by data from the period 1981–2010 was exceeded for at least three consecutive days. In our study, two or more consecutive days above the 95% threshold of the 1985–2015 ERA5 daily mean temperature 51 , 57 were defined as a heatwave, which is considered to be a moderate estimation and is widely used in epidemiological studies 36 , 58 , 59 . Several definitions, such as four or more consecutive days above the 97.5% threshold, are used as sensitivity analysis. Considering certain amounts of climate adaptation of the local resident along the warming climate, dynamic heatwave thresholds 60 are defined as part of the uncertainty analysis in this study; that is two or more consecutive days above the 95% threshold of the daily mean temperature between 1985 and the year before the target year were defined as a heatwave (ERA5 data are used for 1985–2014; climate projection data are used after 2015). The use of a dynamic threshold based on both historical and climate projections data helps to incorporate the human adaptation of heat stress in a long-term warming scenario, as reported in recent studies 61 , 62 , 63 , 64 .
Health costs related to heat exposure
Some studies have shown that the health impact of heatwaves could vary substantially with location 65 , 66 . Few studies have investigated the heatwave-induced mortality risk at a global scale 41 , 67 . A primitive health risk function associating heatwave mortality risks with four different climate zones was established by ref. 36 on the basis of a comprehensive study using data from 400 communities in 18 countries/regions across several years (1972–2012). Here, we used the relative risk coefficients (Extended Data Table 2 ) from figure 4 of ref. 36 for four different climate zones (Extended Data Fig. 3 ) to estimate potential heatwave-related death due to climate change on a global scale. The simplified four-climate-zone-based estimation may neglect subregional characters and should be interpreted with caution, as further factors affecting heat-induced death (such as air condition accessibility 68 , age 69 , 70 , 71 , 72 and humidity 73 ) are not included in this study.
The number of excess deaths D hw during a heatwave period was calculated at each grid cell level (0.5°) with the following equation:
POP is the population at the given location consistent with the SSPs 74 . MR is the average daily mortality rate (2009–2019) at the country level obtained from the World Bank 75 . For 37 countries with large territory and more refined data (for example, European Union (including UK), Russia, Ukraine, China, the USA, Canada, Brazil, South Africa, India and Australia), we used state/provincial statistics based on data from national statistical offices (Source, World Bank; state/province level data for European Union, Eurostat 76 ; Russia, The Russian Fertility and Mortality database 77 ; China, China Statistical Yearbook 2019 78 ; the USA, National Institutes of Health 79 ; Brazil, Fundação Amazônia de Amparo a Estudos e Pesquisas 80 ; Canada, Statistics Canada 81 ; Australia, Australian Bureau of Statistics 82 ; India, Ministry of Finance Economic Survey 83 ). RR is the relative risk of mortality caused by heatwaves. HWN is the number of heatwave days for the given year and location (Extended Data Fig. 2 ).
The calculated excess deaths are translated to a social-economic loss on the basis of the value of statistical life (VSL). The concept of VSL is widely used throughout the world to monetize fatality risks in benefit–cost analyses. The VSL represents the individual’s local money–mortality risk tradeoff value, which is the value of small changes in risk, not the value attached to identified lives. The country-based VSL estimation used in this research is adopted from the global health risks pricing study by ref. 84 . The estimation is based on the estimated VSL in the USA (US$ 2019 11 million) and coupled with an income elasticity of 1.0 to adjust the VSL to other countries using the fixed-effects specification. A similar health valuation method has been adopted in past studies 85 , 86 and was recommended in the report of the World Bank 87 . Moreover, a sensitivity test is conducted under the assumption that all life would be valued equally across the world (Supplementary Figs. 2 and 3 ). For such a test, an averaged VSL is calculated by summing up each country’s income-based VSL times its population then dividing by the total population of the world.
Expose function of labour productivity
The increase in daily temperatures affects the efficiency of workers and reduces safe working time. A compromise in endurance capacity due to thermoregulatory stress was already evident at 21 °C. Different studies used similar methods to evaluate the labour loss function. The form of logistic function with ‘S’ shape has become the consensus of the academic community but the specific functional equation and parameters are various in different studies. The loss functions used in mainstream research include exponential function 88 as equation ( 4 ), cumulative normal distribution function 5 , 41 as equation ( 5 ) and so on. In this research, we adopt the cumulative normal distribution function (equation ( 5 )) as our benchmark function because it was extensively applied and case proven in 3-year reports of the Lancet Countdown on health and climate change 5 , 41 , 89 , 90 . Because the Hothaps function (equation ( 4 )) is subject to parameter uncertainty as a result of being based on a few empirical studies, we use it to test for the sensitivity of our estimates (Supplementary Figs. 4 and 5 ). Our methodology identifies three ISO standard work intensity amounts: 200 W (assumed to be office workers in the service industry, engaged in light work indoors), 300 W (assumed to be industrial workers, engaged in moderate work indoors) and 400 W (assumed to be construction or agricultural workers, engaged in heavy work outside). For example, to calculate workability loss fraction in India’s food production sector (300 W, indoor), we bring the corresponding parameters (Extended Data Table 3 ) and WBGT indoor into equation ( 5 ). Previous studies have tended to ignore indoor workforce loss, assuming that the indoor workforce was very low under current climate condition or protected by air conditioning 91 . However, a growing number of studies have proved that future indoor labour losses cannot be underestimated 31 . For example, only 7% of households in India possess an air conditioner, despite having extremely high cooling needs. Considering the severe adaptation cooling deficit in emerging economies 92 , indoor labour losses must be fully considered in global-scale studies. This study uses the climate–income–air conditioner usage function published by ref. 93 to assess the rate of air conditioning protection in conjunction with the per capita income of each country under each SSP scenario. Higher per capita income in each country leads to higher air-conditioning penetration, whereas the climate base determines the rate and trend of increase in air-conditioning penetration (elasticity of penetration to income). In our study, we improved the function by replacing cooling degree days (CDDs) with indoor WBGT, as CDDs only consider temperature neglecting humidity. Only the indoor workforce under air conditioning, will be protected from heat-induced loss.
Of which the parameters for a given activity level (Prod mean and Prod SD , defined as the amount of internal heat generated in performing the activity) are given in Extended Data Table 3 , and ERF is the error function defined as:
To calculate average daily impacts, we use an approximation for hourly data based on the 4 + 4 + 4 method implemented by ref. 14 . We assume that 4 h per day is close to WBGT max and 4 h per day is close to WBGT mean (early morning and early evening). The remaining 4 h of a 12 h daylight day is assumed to be halfway between WBGT mean and WBGT max (labelled WBGT half ). The analysis above gives the summer daily potential workability lost in each grid cell at each amount of work intensity and environment (200–400 W, indoor or outdoor). By combining this with the dynamic population grid under each SSP scenario (see Supplementary Fig. 13 for comparison with static population setting), we aggregate to obtain country-scale labour productivity losses. In the disaster footprint model, we adopt the approach presented by ref. 5 which defines the timeframe for computing labour productivity losses as the warm season (June to 30 September in the Northern Hemisphere and December to 30 March in the Southern Hemisphere) to adjust the overestimation of the risk of moderate hot temperature, as the model is more applicable to sudden and strong shocks rather than moderate changes throughout the year.
Global disaster footprint analysis module
The global economic loss will be calculated using the following hybrid input–output and computable general equilibrium (CGE) global trade module. Our global trade module is an extension of the adaptive regional input–output (ARIO) model 20 , 94 , 95 , which was widely used in the literature to simulate the propagation of negative shocks throughout the economy 96 , 97 , 98 , 99 . Our model improves the ARIO model in two ways. The first improvement is related to the substitutability of products from the same sector sourced from different regions. Second, in our model, clients will choose their suppliers across regions on the basis of their capacity. These two improvements contribute to a more realistic representation of bottlenecks along global supply chains 100 .
Our global trade module mainly includes four modules: production module, allocation module, demand module and simulation module. The production module is mainly designed for characterizing the firm’s production activities. The allocation module is mainly used to describe how firms allocate output to their clients, including downstream firms (intermediate demand) and households (final demand). The demand module is mainly used to describe how clients place orders to their suppliers. And the simulation module is mainly designed for executing the whole simulation procedure.
Production module
The production module is used to characterize production processes. Firms rent capital and use labour to process natural resources and intermediate inputs produced by other firms into a specific product. The production process for firm i can be expressed as follows,
where x i denotes the output of the firm i , in monetary value; p denotes type of intermediate products; \({z}_{i}^{{\rm{p}}}\) denotes intermediate products used in production processes; va i denotes the primary inputs to production, such as labour ( L ), capital ( K ) and natural resources (NR). The production function for firms is f ( · ). There is a wide range of functional forms, such as Leontief 101 , Cobb–Douglas and constant elasticity of substitution production function 102 . Different functional forms reflect the possibility for firms to substitute an input for another. Considering that heat stress tends to be concentrated in a specific short period of time, during which economic agents cannot easily replace inputs as suitable substitutes, might temporarily be unavailable, we use Leontief production function which does not allow substitution between inputs.
where \({a}_{i}^{{\rm{p}}}\) and \({b}_{i}\) are the input coefficients calculated as
where the horizontal bar indicates the value of that variable in the equilibrium state. In an equilibrium state, producers use intermediate products and primary inputs to produce goods and services to satisfy demand from their clients. After a disaster, output will decline. From a production perspective, there are mainly the following constraints.
Labour supply constraints
Labour constraints during heat stress or after a disaster may impose severe knock-on effects on the rest of the economy 21 , 103 . This makes labour constraints a key factor to consider in disaster impact analysis. For example, in the case of heat stress, these constraints can arise from employees’ inability to work as a result of illness or extreme environmental temperatures beyond health threshold. In this model, the proportion of surviving productive capacity from the constrained labour productive capacity ( \({x}_{i}^{{\rm{L}}}\) ) after a shock is defined as:
Where \({\gamma }_{i}^{{\rm{L}}}(t)\) is the proportion of labour that is unavailable at each time step t during heat stress; \((1-{\gamma }_{i}^{{\rm{L}}}(t))\) contains the available proportion of employment at time t .
The proportion of the available productive capacity of labour is thus a function of the losses from the sectoral labour forces and its predisaster employment level. Following the assumption of the fixed proportion of production functions, the productive capacity of labour in each region after a disaster ( \({x}_{i}^{{\rm{L}}}\) ) will represent a linear proportion of the available labour capacity at each time step. Take heatwaves as an example; during extreme heatwaves that last for days on end, governments and businesses often shut down work to reduce the risk of serious illnesses such as pyrexia. This imposes an exogenous negative shock on the economic network.
Constraints on productive capital
Similar to labour constraints, the productive capacity of industrial capital in each region during the aftermath of a disaster ( \({x}_{i}^{{\rm{K}}}\) ) will be constrained by the surviving capacity of the industrial capital 30 , 96 , 104 , 105 , 106 . The share of damage to each sector is directly considered as the proportion of the monetized damage to capital assets in relation to the total value of industrial capital for each sector, which is disclosed in the event account vector for each region \(({\gamma }_{i}^{{\rm{K}}})\) , following ref. 107 . This assumption is embodied in the essence of the input–output model, which is hard-coded through the Leontief-type production function and its restricted substitution. As capital and labour are considered perfectly complementary as well as the main production factors and the full employment of those factors in the economy is also assumed, we assume that damage in capital assets is directly related with production level and, therefore, VA level. Then, the remaining productive capacity of the industrial capital at each time step is defined as:
Where, \({\bar{K}}_{i}\) is the capital stock of firm \(i\) in the predisaster situation and K i ( t ) is the surviving capital stock of firm \(i\) at time \(t\) during the recovery process
Supply constraints
Firms will purchase intermediate products from their supplier in each period. Insufficient inventory of a firm’s intermediate products will create a bottleneck for production activities. The potential production level that the inventory of the p th intermediate product can support is
where \({S}_{i}^{{\rm{p}}}(t-1)\) refers to the amount of p th intermediate products held by firm i at the end of time step t − 1.
Considering all the limitation mentioned above, the maximum supply capacity of firm i can be expressed as
The actual production of firm i , \({x}_{i}^{{\rm{a}}}(t)\) , depends on both its maximum supply capacity and the total orders the firm received from its clients, \({{\rm{TD}}}_{i}(t-1)\) (see section on the ‘Demand module’),
The inventory held by firm i will be consumed during the production process,
Allocation module
The allocation module mainly describes how suppliers allocate products to their clients. When some firms in the economic system suffer a negative shock, their production will be constrained by a shortage to primary inputs such as a shortage of labour supply during extreme heat stress. In this case, a firm’s output will not be able to fill all orders of its clients. A rationing scheme that reflects a mechanism on the basis of which a firm allocates an insufficient amount of products to its clients is needed 108 . For this case study, we applied a proportional rationing scheme according to which a firm allocates its output in proportion to its orders. Under the proportional rationing scheme, the amounts of products of firm i allocated to firm j , \({{\rm{F}}{\rm{R}}{\rm{C}}}_{j}^{i}\) and household h , \({{\rm{H}}{\rm{R}}{\rm{C}}}_{h}^{i}\) are as follows,
where \({{\rm{F}}{\rm{O}}{\rm{D}}}_{i}^{j}(t-1)\) refers to the order issued by firm j to its supplier i in time step t − 1, and \({{\rm{H}}{\rm{O}}{\rm{D}}}_{i}^{h}(t-1)\) refers to the order issued by household h to its supplier j . Firm j received intermediates to restore its inventories,
Therefore, the amount of intermediate p held by firm i at the end of period t is
Demand module
The demand module represents a characterization of how firms and households issues orders to their suppliers at the end of each period. A firm orders its supplier because of the need to restore its intermediate product inventory. We assume that each firm has a specific target inventory level based on its maximum supply capacity in each time step,
Then the order issued by firm i to its supplier j is
Households issue orders to their suppliers on the basis of their demand and the supply capacity of their suppliers. In this study, the demand of household h to final products q , \({{\rm{HD}}}_{h}^{q}\left(t\right)\) , is given exogenously at each time step. Then, the order issued by household (HOD) h to its supplier j is
The total order received (TOD) by firm j is
Simulation module
At each time step, the actions of firms and households are as follows in Monte Carlo simulations.
Firms plan and execute their production on the basis of three factors: (1) inventories of intermediate products they have, (2) supply of primary inputs and (3) orders from their clients. Firms will maximize their output under these constraints.
Product allocation
Firms allocate outputs to clients on the basis of their orders. In equilibrium, the output of firms just meets all orders. When production is constrained by exogenous negative shocks, outputs may not cover all orders. In this case, we use a proportional rationing scheme proposed in the literature 20 , 108 (see section on ‘Allocation module’) to allocate products of firms.
Firms and households issue orders to their suppliers for the next time step. Firms place orders with their suppliers on the basis of the gaps in their inventories (target inventory level minus existing inventory level). Households place orders with their suppliers on the basis of their demand. When a product comes from several suppliers, the allocation of orders is adjusted according to the production capacity of each supplier.
This discrete-time dynamic procedure can reproduce the equilibrium of the economic system and can simulate the propagation of exogenous shocks, both from firm and household side or transportation disruptions, in the economic network. From the firm side, if the supply of a firm’s primary inputs is constrained, it will have two effects. On the one hand, the decline in output in this firm means that its clients’ orders cannot be fulfilled. This will result in a decrease in inventory of these clients, which will constrain their production. This is the so-called forward or downstream effect. On the other hand, less output in this firm also means less use of intermediate products from its suppliers. This will reduce the number of orders it places on its suppliers, which will further reduce the production level of its suppliers. This is the so-called backward or upstream effect. From the household side, the fluctuation of household demand caused by exogenous shocks will also trigger the aforementioned backward effect. Take tourism as an example, when the temperature is well beyond the comfort range of the visitor, the demand for tourism from households all over the world will decline significantly. This influence will further propagate to the accommodation and catering industry through supplier–client links.
Economic footprint
We define the VA decrease of all firms in a network caused by an exogenous negative shock as the disaster footprint of the shock. For the firm directly affected by exogenous negative shocks, its loss includes two parts: (1) the VA decrease caused by exogenous constraints and (2) the VA decrease caused by propagation. The former is the direct loss, whereas the latter is the indirect loss. A negative shock’s total economic footprint (TEF i,r ), direct economic footprint (DEF i,r ) and propagated economic footprint (PEF i,r ) for firm i in region r are,
Global supply-chain network
We build a global supply-chain network based on v.10 of the Global Trade Analysis Project (GTAP) database 109 and use GTAP 9 (ref. 110 ), EMERGING database 111 for robustness analysis. GTAP 10 provides a multiregional input–output (MRIO) table for the year 2014. Also, the database for the year 2011 was used for robustness testing. This MRIO table divides the world into 141 economies, each of which contains 65 production sectors (Supplementary Tables 4 and 5 ). If we treat each sector as a firm (producer) and assume that each region has a representative household, we can obtain the following information in the MRIO table: (1) suppliers and clients of each firm; (2) suppliers for each household and (3) the flow of each supplier–client connection under the equilibrium condition. This provides a benchmark for our model. We also used a dynamic CGE model consistent with the SSP scenarios for a parallel assessment and as part of the robustness check of the ARIO results. Specifically, the CGE model we used is a G-RDEM 112 with aggregated ten regions and ten sectors 113 , 114 , 115 (Supplementary Information section 1.3 ).
When applying such a realistic and aggregated network to the disaster footprint model, we need to consider the substitutability of intermediate products supplied by suppliers from the same sector in different regions 115 , 116 , 117 . The substitution between some intermediate products is straightforward. For example, for a firm that extracts spices from bananas it does not make much of a difference if the bananas are sourced from the Philippines or Thailand. However, for a car manufacturing firm in Japan, which uses screws from Chinese auto parts suppliers and engines from German auto parts suppliers to assemble cars, the products of the suppliers in these two regions are non-substitutable. If we assume that all goods are non-substitutable as in the traditional input–output model, then we will overestimate the loss of producers such as the case of the fragrance extraction firm. If we assume that products from suppliers in the same sector can be completely substitutable, then we will substantially underestimate the losses of producers such as the Japanese car manufacturing firm. To alleviate these shortcomings in the evaluation of losses under the two assumptions, we allow for the possibility of substitution for each sector depending on the region and sector of the supplier (Supplementary Information section 1.3 ).
Nonetheless, our estimates of economic damages from heat stress are subject to some important uncertainties 118 and our methods may not capture all types of economic damages. We only include economic losses caused by heat stress on human activities without considering the impacts on infrastructure, crop growth and other factors. Considering the challenges of predicting changes to socioeconomic systems globally, we have followed the approach from the literature 23 , 31 , 91 , 119 to simulate supply-chain indirect losses by considering the impact of future climate risks on current socioeconomic settings. We have not considered the potential substitution of labour with capital resulting from technological advances, such as mechanization. Our analysis ignores the different levels of trade openness and globalization among SSP narratives, as well as the role of dynamic factors such as technology and price. Again, although we have conducted robustness tests for different degrees of trade substitutability, the relevant parameter is set randomly in the Monte Carlo simulation rather than derived through a general equilibrium model. The results should therefore be interpreted with caution as indicating potential future climate change risks to the existing economy rather than as quantitative predictions, given that the static representation of the economic structure in our model inevitably skews the assessment in the long run.
Data availability
Data for the numerical results of this research are provided at https://zenodo.org/records/10032431 . The global trade dataset used to simulate the presented results are licensed by the Global Trade Analysis Project at the Centre for Global Trade Analysis, Department of Agricultural Economics, Purdue University. The GTAP v.10 can be obtained for a fee from its official website: https://www.gtap.agecon.purdue.edu/databases/v10/index.aspx . Owing to the restriction in the licensing agreement with GTAP, the authors have no right to disclose the original dataset publicly. Multimodal meteorological data are derived from World Climate Research Programme (WCRP CMIP6): https://esgf-node.llnl.gov/search/cmip6/ . Socioeconomic data for the different SSP scenarios are derived from IIASA: https://secure.iiasa.ac.at/web-apps/ene/SspDb/ . Global population projection grids are from Socioeconomic Data and Applications Center (SEDAC) ( https://sedac.ciesin.columbia.edu/data/set/popdynamics-1-8th-pop-base-year-projection-ssp-2000-2100-rev01/data-download ).
Code availability
The climate and epidemiological module processes daily surface temperature, dynamic population grid and baseline mortality data to determine heatwave days and the associated excess deaths. The economic module simulates changes of values and flows in global multiregional input–output table under shocks. All of the codes can be accessed at https://zenodo.org/records/10334260 . The minimal input for the code is multiregional input–output table. The sample code and test data for the minimal inputs are also provided.
Callendar, G. S. The artificial production of carbon dioxide and its influence on temperature. Q. J. R. Meteorolog. Soc. 64 , 223–240 (1938).
Article ADS Google Scholar
Seneviratne, S. I. et al. in Climate Change 2021: The Physical Science Basis (eds Masson-Delmotte, V. et al.) 1513–1766 (Cambridge Univ. Press, 2021).
Callahan, C. W. & Mankin, J. S. Globally unequal effect of extreme heat on economic growth. Sci. Adv. 8 , eadd3726 (2022).
Article CAS PubMed PubMed Central Google Scholar
Lenton, T. M. et al. Quantifying the human cost of global warming. Nat. Sustain. 6 , 1237–1247 (2023).
Article Google Scholar
Cai, W. et al. The 2020 China report of the Lancet Countdown on health and climate change. Lancet Public Health 6 , e64–e81 (2021).
Article PubMed Google Scholar
Gasparrini, A. et al. Mortality risk attributable to high and low ambient temperature: a multicountry observational study. Lancet 386 , 369–375 (2015).
Article PubMed PubMed Central Google Scholar
Flouris, A. D. et al. Workers’ health and productivity under occupational heat strain: a systematic review and meta-analysis. Lancet Planet. Health 2 , e521–e531 (2018).
Revich, B. & Shaposhnikov, D. Excess mortality during heat waves and cold spells in Moscow, Russia. Occup. Environ. Med. 65 , 691–696 (2008).
Article CAS PubMed Google Scholar
Kjellstrom, T. & Crowe, J. Climate change, workplace heat exposure and occupational health and productivity in Central America. Int. J. Occup. Environ. Health 17 , 270–281 (2011).
Cowan, T. et al. More frequent, longer and hotter heat waves for Australia in the twenty-first century. J. Clim. 27 , 5851–5871 (2014).
Ahmadalipour, A. & Moradkhani, H. Escalating heat-stress mortality risk due to global warming in the Middle East and North Africa (MENA). Environ. Int. 117 , 215–225 (2018).
Christidis, N., Jones, G. S. & Stott, P. A. Dramatically increasing chance of extremely hot summers since the 2003 European heatwave. Nat. Clim. Change 5 , 46–50 (2015).
Dunne, J. P., Stouffer, R. J. & John, J. G. Reductions in labour capacity from heat stress under climate warming. Nat. Clim. Change 3 , 563–566 (2013).
Article CAS ADS Google Scholar
Kjellstrom, T., Freyberg, C., Lemke, B., Otto, M. & Briggs, D. Estimating population heat exposure and impacts on working people in conjunction with climate change. Int. J. Biometeorol. 62 , 291–306 (2018).
Article PubMed ADS Google Scholar
Lee, S.-W., Lee, K. & Lim, B. Effects of climate change-related heat stress on labor productivity in South Korea. Int. J. Biometeorol. 62 , 2119–2129 (2018).
Nunfam, V. F., Adusei-Asante, K., Frimpong, K., Van Etten, E. J. & Oosthuizen, J. Barriers to occupational heat stress risk adaptation of mining workers in Ghana. Int. J. Biometeorol. 64 , 1085–1101 (2020).
Borg, M. A. et al. Occupational heat stress and economic burden: a review of global evidence. Environ. Res. 195 , 110781 (2021).
Kjellstrom, T., Kovats, R. S., Lloyd, S. J., Holt, T. & Tol, J. S. R. The direct impact of climate change on regional labor productivity. Arch. Environ. Occup. Health 64 , 217–227 (2009).
Hasegawa, T. et al. Climate change impact and adaptation assessment on food consumption utilizing a new scenario framework. Environ. Sci. Technol. 48 , 438–445 (2014).
Article CAS PubMed ADS Google Scholar
Wenz, L. & Levermann, A. Enhanced economic connectivity to foster heat stress-related losses. Sci. Adv. 2 , e1501026–e1501026 (2016).
Article PubMed PubMed Central ADS Google Scholar
Xia, Y. et al. Assessment of the economic impacts of heat waves: a case study of Nanjing, China. J. Clean. Prod. 171 , 811–819 (2018).
Zhao, M., Lee, J. K. W., Kjellstrom, T. & Cai, W. Assessment of the economic impact of heat-related labor productivity loss: a systematic review. Clim. Change 167 , 22 (2021).
Xie, W. et al. Decreases in global beer supply due to extreme drought and heat. Nat. Plants 4 , 964–973 (2018).
Lima, C. Zde et al. Heat stress on agricultural workers exacerbates crop impacts of climate change. Environ. Res. Lett. 16 , 044020 (2021).
Hertel, T. W. & Rosch, S. D. Climate change, agriculture and poverty. Appl. Econ. Perspect. Policy 32 , 355–385 (2010).
Xie, W., Cui, Q. & Ali, T. Role of market agents in mitigating the climate change effects on food economy. Nat. Hazards 99 , 1215–1231 (2019).
Pierce, R. J. Energy independence and global warming. Nat. Res. Environ. 21 , 68–71 (2007).
ADS Google Scholar
Bleischwitz, R. Mineral resources in the age of climate adaptation and resilience. J. Ind. Ecol. 24 , 291–299 (2020).
Wang, D. et al. Economic footprint of California wildfires in 2018. Nat. Sustain. 4 , 252–260 (2021).
Xia, Y. et al. Assessing the economic impacts of IT service shutdown during the York flood of 2015 in the UK. Proc. R. Soc. A 475 , 20180871 (2019).
García-León, D. et al. Current and projected regional economic impacts of heatwaves in Europe. Nat. Commun. 12 , 5807 (2021).
Knittel, N., Jury, M. W., Bednar-Friedl, B., Bachner, G. & Steiner, A. K. A global analysis of heat-related labour productivity losses under climate change—implications for Germany’s foreign trade. Clim. Change 160 , 251–269 (2020).
Takakura, J. et al. Limited role of working time shift in offsetting the increasing occupational-health cost of heat exposure. Earth’s Future 6 , 1588–1602 (2018).
Eyring, V. et al. Overview of the Coupled Model Intercomparison Project Phase 6 (CMIP6) experimental design and organization. Geosci. Model Dev. 9 , 1937–1958 (2016).
Fasullo, J. T. Evaluating simulated climate patterns from the CMIP archives using satellite and reanalysis datasets using the Climate Model Assessment Tool (CMATv1). Geosci. Model Dev. 13 , 3627–3642 (2020).
Guo, Y. et al. Heat wave and mortality: a multicountry, multicommunity study. Environ. Health Perspect. 125 , 087006 (2017).
Riahi, K. et al. The Shared Socioeconomic Pathways and their energy, land use and greenhouse gas emissions implications: an overview. Glob. Environ. Change 42 , 153–168 (2017).
Fricko, O. et al. The marker quantification of the Shared Socioeconomic Pathway 2: a middle-of-the-road scenario for the 21st century. Glob. Environ. Change 42 , 251–267 (2017).
Orlov, A., Sillmann, J., Aunan, K., Kjellstrom, T. & Aaheim, A. Economic costs of heat-induced reductions in worker productivity due to global warming. Glob. Environ. Change 63 , 102087 (2020).
Takakura, J. et al. Cost of preventing workplace heat-related illness through worker breaks and the benefit of climate-change mitigation. Environ. Res. Lett. 12 , 064010 (2017).
Watts, N. et al. The 2019 report of The Lancet Countdown on health and climate change: ensuring that the health of a child born today is not defined by a changing climate. Lancet 394 , 1836–1878 (2019).
World Development Indicators , https://databank.worldbank.org/source/world-development-indicators (World Bank, 2022).
Hsiang, S. M. Temperatures and cyclones strongly associated with economic production in the Caribbean and Central America. Proc. Natl Acad. Sci. USA 107 , 15367–15372 (2010).
Article CAS PubMed PubMed Central ADS Google Scholar
Colacito, R., Hoffmann, B. & Phan, T. Temperature and growth: a panel analysis of the United States. J. Money Credit Bank. 51 , 313–368 (2019).
EM-DAT , www.emdat.be/ (CRED, accessed 1 February 2023).
Gridded Population of the World, Version 4 (GPWv4): Population Count, Revision 11 , https://doi.org/10.7927/H4JW8BX5 (CIESIN, 2018).
The Human Cost of Disasters: An Overview of the Last 20 Years (2000–2019) , www.undrr.org/publication/human-cost-disasters-overview-last-20-years-2000-2019 (UNDRR, 2020).
Dellink, R., Lanzi, E. & Chateau, J. The sectoral and regional economic consequences of climate change to 2060. Environ. Res. Econ. 72 , 309–363 (2019).
Lange, S. & Büchner, M. Secondary ISIMIP3b Bias-Adjusted Atmospheric Climate Input Data (v1.1) , https://doi.org/10.48364/ISIMIP.581124.1 (ISIMIP Repository, 2022).
Warszawski, L. et al. The Inter-Sectoral Impact Model Intercomparison Project (ISI–MIP): project framework. Proc. Natl Acad. Sci. USA 111 , 3228–3232 (2014).
Hersbach, H. et al. The ERA5 global reanalysis. Q. J. R. Meteorolog. Soc. 146 , 1999–2049 (2020).
Casanueva, A. et al. Climate projections of a multivariate heat stress index: the role of downscaling and bias correction. Geosci. Model Dev. 12 , 3419–3438 (2019).
Lemke, B. & Kjellstrom, T. Calculating workplace WBGT from meteorological data: a tool for climate change assessment. Indust. Health 50 , 267–278 (2012).
Tong, S., Wang, X. Y. & Barnett, A. G. Assessment of heat-related health impacts in Brisbane, Australia: comparison of different heatwave definitions. PLoS ONE 5 , e12155 (2010).
Xu, Z., FitzGerald, G., Guo, Y., Jalaludin, B. & Tong, S. Impact of heatwave on mortality under different heatwave definitions: a systematic review and meta-analysis. Environ. Int. 89–90 , 193–203 (2016).
Sun, X. et al. Heat wave impact on mortality in Pudong New Area, China in 2013. Sci. Total Environ. 493 , 789–794 (2014).
Setchell, H. ECMWF Reanalysis v5 , www.ecmwf.int/en/forecasts/dataset/ecmwf-reanalysis-v5 (ECMWF, 2020).
Nairn, J., Fawcett, R. & Ray, D. Defining and Predicting Excessive Heat Events, a National System (CAWCR, 2009).
Tong, S., Wang, X. Y., Yu, W., Chen, D. & Wang, X. The impact of heatwaves on mortality in Australia: a multicity study. BMJ Open 4 , e003579 (2014).
Guo, X. et al. Threat by marine heatwaves to adaptive large marine ecosystems in an eddy-resolving model. Nat. Clim. Change 12 , 179–186 (2022).
Åström, D. O., Tornevi, A., Ebi, K. L., Rocklöv, J. & Forsberg, B. Evolution of minimum mortality temperature in Stockholm, Sweden, 1901–2009. Environ. Health Perspect. 124 , 740–744 (2016).
Yin, Q., Wang, J., Ren, Z., Li, J. & Guo, Y. Mapping the increased minimum mortality temperatures in the context of global climate change. Nat. Commun. 10 , 4640 (2019).
Todd, N. & Valleron, A.-J. Space–time covariation of mortality with temperature: a systematic study of deaths in France, 1968–2009. Environ. Health Perspect. 123 , 659–664 (2015).
Folkerts, M. A. et al. Long term adaptation to heat stress: shifts in the minimum mortality temperature in the Netherlands. Front. Physiol. 11 , 225 (2020).
Anderson, B. G. & Bell, M. L. Weather-related mortality. Epidemiology 20 , 205–213 (2009).
Guo, Y. et al. Global variation in the effects of ambient temperature on mortality: a systematic evaluation. Epidemiology 25 , 781–789 (2014).
Guo, Y. et al. Quantifying excess deaths related to heatwaves under climate change scenarios: a multicountry time series modelling study. PLoS Med. 15 , e1002629 (2018).
Sera, F. et al. Air conditioning and heat-related mortality: a multi-country longitudinal study. Epidemiology 31 , 779 (2020).
Benmarhnia, T. et al. A difference-in-differences approach to assess the effect of a heat action plan on heat-related mortality and differences in effectiveness according to sex, age and socioeconomic status (Montreal, Quebec). Environ. Health Perspect. 124 , 1694–1699 (2016).
Cheng, J. et al. Heatwave and elderly mortality: an evaluation of death burden and health costs considering short-term mortality displacement. Environ. Int. 115 , 334–342 (2018).
Wondmagegn, B. Y. et al. Impact of heatwave intensity using excess heat factor on emergency department presentations and related healthcare costs in Adelaide, South Australia. Sci. Total Environ. 781 , 146815 (2021).
Zhang, L. et al. Mortality effects of heat waves vary by age and area: a multi-area study in China. Environ. Health 17 , 54 (2018).
Benmarhnia, T., Deguen, S., Kaufman, J. S. & Smargiassi, A. Vulnerability to heat-related mortality: a systematic review, meta-analysis and meta-regression analysis. Epidemiology 26 , 781 (2015).
Jones, B. & O’Neill, B. C. Spatially explicit global population scenarios consistent with the Shared Socioeconomic Pathways. Environ. Res. Lett. 11 , 084003 (2016).
Death Rate, Crude (per 1,000 People) | Data , https://data.worldbank.org/indicator/SP.DYN.CDRT.IN (World Bank, 2021).
Population and Demography—Eurostat Database , https://ec.europa.eu/eurostat/web/population-demography/demography-population-stock-balance/database (European Union, accessed 8 September 2023).
The Russian Fertility and Mortality Database (RusFMD) (Center for Demographic Research, 2022).
China Statistical Yearbook 2019 (NBSC, 2019).
Mortality Table for US by State | HDPulse Data Portal , https://hdpulse.nimhd.nih.gov/data-portal/mortality (NIH, date accessed 8 September 2023).
Taxa de Mortalidade Geral , ftp.ibge.gov.br/Tabuas_Completas_de_Mortalidade/Tabuas_Completas_de_Mortalidade_2016/tabua_de_mortalidade_2016_analise.pdf (Instituto Brasileiro de Geografia e Estatística - IBGE, 2017).
Mortality Rates, by Age Group , www.statcan.gc.ca/en/subjects-start/health/life_expectancy_and_deaths (Government of Canada, 2021).
Deaths, Australia, 2019 , www.abs.gov.au/statistics/people/population/deaths-australia/2019 (Australian Bureau of Statistics, 2020).
Economic Survey , www.indiabudget.gov.in/economicsurvey/ (Government of India, accessed 8 September 2023).
Viscusi, W. K. Pricing the global health risks of the COVID-19 pandemic. J. Risk Uncertain. 61 , 101–128 (2020).
Alkire, B. C., Peters, A. W., Shrime, M. G. & Meara, J. G. The economic consequences of mortality amenable to high-quality health care in low- and middle-income countries. Health Affairs 37 , 988–996 (2018).
Hammitt, J. K. & Robinson, L. A. The income elasticity of the value per statistical life: transferring estimates between high and low income populations. J. Benefit–Cost Anal. 2 , 1–29 (2011).
Narain, U. & Sall, C. Methodology for Valuing the Health Impacts of Air Pollution: Discussion of Challenges and Proposed Solutions , https://openknowledge.worldbank.org/handle/10986/24440 (World Bank, 2016).
Bröde, P., Fiala, D., Lemke, B. & Kjellstrom, T. Estimated work ability in warm outdoor environments depends on the chosen heat stress assessment metric. Int. J. Biometeorol. 62 , 331–345 (2018).
Watts, N. et al. The 2020 report of The Lancet Countdown on health and climate change: responding to converging crises. Lancet 397 , 129–170 (2021).
Romanello, M. et al. The 2021 report of the Lancet Countdown on health and climate change: code red for a healthy future. Lancet 398 , 1619–1662 (2021).
Parsons, L. A., Shindell, D., Tigchelaar, M., Zhang, Y. & Spector, J. T. Increased labor losses and decreased adaptation potential in a warmer world. Nat. Commun. 12 , 7286 (2021).
Pavanello, F. et al. Air-conditioning and the adaptation cooling deficit in emerging economies. Nat. Commun. 12 , 6460 (2021).
The Future of Cooling—Analysis , https://www.iea.org/reports/the-future-of-cooling (IEA, 2018).
Hallegatte, S. An adaptive regional input-output model and its application to the assessment of the economic cost of Katrina. Risk Anal. 28 , 779–799 (2008).
Hallegatte, S. Modeling the role of inventories and heterogeneity in the assessment of the economic costs of natural disasters: modeling the role of inventories and heterogeneity. Risk Anal. 34 , 152–167 (2014).
Koks, E. E. & Thissen, M. A multiregional impact assessment model for disaster analysis. Econ. Syst. Res. 28 , 429–449 (2016).
Li, J., Crawford-Brown, D., Syddall, M. & Guan, D. Modeling imbalanced economic recovery following a natural disaster using input–output analysis: modeling imbalanced economic recovery. Risk Anal. 33 , 1908–1923 (2013).
Okuyama, Y. & Santos, J. R. Disaster impact and input–output analysis. Econ. Syst. Res. 26 , 1–12 (2014).
Inoue, H. & Todo, Y. Firm-level propagation of shocks through supply-chain networks. Nat. Sustain. 2 , 841–847 (2019).
Bardazzi, R. & Ghezzi, L. Large-scale multinational shocks and international trade: a non-zero-sum game. Econ. Syst. Res. 34 , 383–409 (2022).
Zhang, Z., Li, N., Xu, H. & Chen, X. Analysis of the economic ripple effect of the United States on the world due to future climate change. Earth’s Future 6 , 828–840 (2018).
Inoue, H. & Todo, Y. Propagation of negative shocks across nationwide firm networks. PLoS ONE 14 , e0213648 (2019).
Miller, R. E. & Blair, P. D. Input–Output Analysis: Foundations and Extensions (Cambridge Univ. Press, 2009).
Koks, E. E. et al. Regional disaster impact analysis: comparing input–output and computable general equilibrium models. Nat. Hazards Earth Syst. Sci. 16 , 1911–1924 (2016).
Liu, X. et al. Assessing the indirect economic losses of sea ice disasters: an adaptive regional input–output modeling approach. Int. J. Offshore Polar Eng. 29 , 415–420 (2019).
Matsuo, H. Implications of the Tohoku earthquake for Toyota’s coordination mechanism: supply chain disruption of automotive semiconductors. Int. J. Prod. Econ. 161 , 217–227 (2015).
Steenge, A. E. & Bočkarjova, M. Thinking about imbalances in post-catastrophe economies: an input–output based proposition. Econ. Syst. Res. 19 , 205–223 (2007).
Bénassy, J.-P. Nonclearing markets: microeconomic concepts and macroeconomic applications. J. Econ. Lit. 31 , 732–761 (1993).
Google Scholar
Aguiar, A., Chepeliev, M., Corong, E. & Mcdougall, R. The GTAP data base: version 10. J. Glob. Econ. Anal. 4 , 27 (2019).
Aguiar, A., Narayanan, B. & McDougall, R. An overview of the GTAP 9 data base. J. Glob. Econ. Anal. 1 , 181–208 (2016).
Huo, J. et al. Full-scale, near real-time multi-regional input–output table for the global emerging economies (EMERGING). J. Ind. Ecol. 26 , 1218–1232 (2022).
Britz, W. & Roson, R. G-RDEM: a GTAP-based recursive dynamic CGE model for long-term baseline generation and analysis. J. Glob. Econ. Anal. 4 , 50–96 (2019).
Lenzen, M. Aggregating input–output systems with minimum error. Econ. Syst. Res. 31 , 594–616 (2019).
Ara, K. The aggregation problem in input–output analysis. Econometrica 27 , 257–262 (1959).
Article MathSciNet Google Scholar
Fei, J. C.-H. A fundamental theorem for the aggregation problem of input–output analysis. Econometrica 24 , 400–412 (1956).
Lindner, S., Legault, J. & Guan, D. Disaggregating the electricity sector of China’s input–output table for improved environmental life-cycle assessment. Econ. Syst. Res. 25 , 300–320 (2013).
Lindner, S., Legault, J. & Guan, D. Disaggregating input–output models with incomplete information. Econ. Syst. Res. 24 , 329–347 (2012).
Han, Q., Sun, S., Liu, Z., Xu, W. & Shi, P. Accelerated exacerbation of global extreme heatwaves under warming scenarios. Int. J. Climatol. 42 , 5430–5441 (2022).
Hsiang, S. et al. Estimating economic damage from climate change in the United States. Science 356 , 1362–1369 (2017).
Download references
Acknowledgements
This study was supported by the National Natural Science Foundation of China (grant nos. 72242105, 72091514 and 72250710169) and the startup funding from Zhejiang University to S.Z.
Author information
These authors contributed equally: Yida Sun, Shupeng Zhu, Daoping Wang
Authors and Affiliations
Department of Earth System Science, Ministry of Education Key Laboratory for Earth System Modeling, Institute for Global Change Studies, Tsinghua University, Beijing, China
Yida Sun, Hui Lu, Chang Tan, Wenjia Cai, Yong Wang & Dabo Guan
Department of Atmospheric Sciences, School of Earth Sciences, Zhejiang University, Hangzhou, China
Shupeng Zhu
Advanced Power and Energy Program, University of California Irvine, Irvine, CA, USA
Department of Geography, King’s College London, London, UK
Daoping Wang
Centre for Climate Engagement, Department of Computer Science and Technology, University of Cambridge, Cambridge, UK
State Key Laboratory of Earth Surface and Ecological Resources, Faculty of Geographical Science, Beijing Normal University, Beijing, China
Jianping Duan
Tsinghua University (Department of Earth System Science)—Xi’an Institute of Surveying and Mapping Joint Research Center for Next-Generation Smart Mapping, Beijing, China
Department of Economics, University of Southern California, Los Angeles, CA, USA
Department of Economics, University of Waterloo, Waterloo, Ontario, Canada
Lingrui Zhang
School of Management and Economics, Beijing Institute of Technology, Beijing, China
Mengzhen Zhao
School of Economics and Management, Southeast University, Nanjing, China
College of Urban Environment, Peking University, Beijing, China
The Bartlett School of Sustainable Construction, University College London, London, UK
You can also search for this author in PubMed Google Scholar
Contributions
D.G. designed and supervised the study. Y.S., S.Z. and D.W. conducted the study, collected the data, analysed the results and drafted the paper. J.D. and Y.W. collected and processed the meteorological data. H.Y., M.Z. and W.C. provided guidance on the calculation of health and labour productivity losses. C.T., Y.H. and L.Z. participated in the writing of the manuscript. S.T. and H.L. guided the uncertainty analysis and validation.
Corresponding author
Correspondence to Dabo Guan .
Ethics declarations
Competing interests.
The authors declare no competing interests.
Peer review
Peer review information.
Nature thanks Birgit Bednar-Friedl, Andreas Flouris, G. Jason Jolley, Elco Koks and Vikki Thompson for their contribution to the peer review of this work. Peer reviewer reports are available.
Additional information
Publisher’s note Springer Nature remains neutral with regard to jurisdictional claims in published maps and institutional affiliations.
Extended data figures and tables
Extended data fig. 1 schematic diagram of the methodological framework..
Coupling mechanisms for climate, health and economic modules.
Extended Data Fig. 2 Heatwave days in the 2040 s and 2060 s under SSP585 Scenario.
The number of heatwave days in each cell was calculated from the ten-year average.
Extended Data Fig. 3 Climate zones classification of relative risk.
Cold area: mean temperature of hot season: ≤<= 20.7 °C; moderate cold areas: mean temperature of hot season: 20.7–24.1 °C; moderate hot areas: mean temperature of hot season: 24.1–27.6 °C; and hot areas: mean temperature of hot season: >27.6 °C, based on ERA5 1985–2010.
Extended Data Fig. 4 Estimates for the ten countries with the highest indirect losses under the SSP585 scenario using different base period trade data.
Estimates are displayed as 10-year averages for the year 2060, using the GTAP2011 (a), GTAP2014 (b) and EMERGING 2019 (c) databases separately. The colours of the bars represent GDP per capita from low to high. (d), indirect losses under different benchmark trade structures in each region. The horizontal axis measures the indirect losses as a percentage of GDP using the GTAP2014 trade structure and the vertical axis measures the indirect losses as a percentage of GDP using the GTAP2011 trade structure. Details of Extended Data Fig. 4d can be checked in Supplementary Fig. 6 .
Extended Data Fig. 5 Global economic losses for each scenario under Monte Carlo simulations.
The assessment results of existing studies are marked with symbols for comparison. None of the previous studies were based on CMIP6 SSP119 scenario, so we use RCP2.6 to compare with the SSP119 scenario in our study. The studies above did not simulate health loss and the mean values of the health loss simulations in this paper were added for consistency.
Extended Data Fig. 6 Impacts of heat stress on India food manufacturing supply chains.
( a ), ( c ) panels represent the upstream sectors of the India’s food production sector in 2040 and 2060, respectively. ( b ), ( d ) panels represent the downstream sectors. Each bar represents a key trading partner (i.e. sector with trade volume above the 50 percent quartile of trade volumes of the selected sector with all partner sectors) and the length represents the percentage decrease in product flow compared to the base period of 2014. The colours of the bars represent the cohesion level of the particular sector to the Indian food production sector from blue (weak) to red (strong), which is measured by the trade volume between the particular sector and the Indian food production sector.
Extended Data Fig. 7 Impacts of heat stress on Dominican Republic tourism supply chains.
( a ), ( c ) panels represent the upstream sectors of the Dominican Republic’s tourism sector in 2040 and 2060, respectively. ( b ), ( d ) panels represent the downstream sectors. Each bar represents a key trading partner (i.e. sector with trade volume above the 50 percent quartile of trade volumes of the selected sector with all partner sectors) and the length represents the percentage decrease in product flow compared to the base period of 2014. The colours of the bars represent the cohesion level of the particular sector to the Dominican Republic’s tourism sector from blue (weak) to red (strong), which is measured by the trade volume between the particular sector and the Dominican Republic’s tourism sector.
Extended Data Fig. 8 Impacts of heat stress on Germany beverages and tobacco products supply chains.
( a ), ( c ) panels represent the upstream sectors of the Germany’s beverages and tobacco products sector in 2040 and 2060, respectively. ( b ), ( d ), panels represent the downstream sectors.
Extended Data Fig. 9 Sectoral loss patterns of type 1 countries.
The top 5 most vulnerable sectors in Tanzania (a–c), Botswana(d–f) and Malawi (g–i). The column length represents each sector’s percentage loss of annual value-added. Sectors with the same loss percentage (e.g. wheat, rice, cereals, etc.) were combined. Colours indicate the three categories of losses in SSP119: health losses (Yellow bars), labour productivity losses (Blue bars) and supply-chain disruption losses (Green bars). The orange and red bars represent total loss increments for SSP245 and SSP585 (no distinction between types of loss in this part), respectively. The red dashed line indicates the mean value of losses for all sectors in the SSP585 scenario.
Extended Data Fig. 10 Sectoral loss patterns of type 2 countries.
The top 5 most vulnerable sectors in India (a–c), Vietnam (d–f) and China (g–i). The column length represents each sector’s percentage loss of annual value-added. Sectors with the same loss percentage (e.g. wheat, rice, cereals, etc.) were combined. Colours indicate the three categories of losses in SSP119: health losses (Yellow bars), labour productivity losses (Blue bars) and supply-chain disruption losses (Green bars). The orange and red bars represent total loss increments for SSP245 and SSP585 (no distinction between types of loss in this part), respectively. The red dashed line indicates the mean value of losses for all sectors in the SSP585 scenario.
Extended Data Fig. 11 Sectoral loss patterns of type 3 countries.
The top 5 most vulnerable sectors in Germany (a–c), France (d–f) and Australia (g–i). The column length represents each sector’s percentage loss of annual value-added. Sectors with the same loss percentage (e.g. wheat, rice, cereals, etc.) were combined. Colours indicate the three categories of losses in SSP119: health losses (Yellow bars), labour productivity losses (Blue bars) and supply-chain disruption losses (Green bars). The orange and red bars represent total loss increments for SSP245 and SSP585 (no distinction between types of loss in this part), respectively. The red dashed line indicates the mean value of losses for all sectors in the SSP585 scenario.
Extended Data Fig. 12 Sectoral loss patterns of type 4 countries.
The top 5 most vulnerable sectors in Sweden (a–c), Norway(d–f) and United Kingdom (g–i). The column length represents each sector’s percentage loss of annual value-added. Sectors with the same loss percentage (e.g. wheat, rice, cereals, etc.) were combined. Colours indicate the three categories of losses in SSP119: health losses (Yellow bars), labour productivity losses (Blue bars) and supply-chain disruption losses (Green bars). The orange and red bars represent total loss increments for SSP245 and SSP585 (no distinction between types of loss in this part), respectively. The red dashed line indicates the mean value of losses for all sectors in the SSP585 scenario.
Supplementary information
Supplementary information.
Supplementary sections 1–7, including Figs. 1–13, Tables 1–7 and references.
Peer Review File
Rights and permissions.
Open Access This article is licensed under a Creative Commons Attribution 4.0 International License, which permits use, sharing, adaptation, distribution and reproduction in any medium or format, as long as you give appropriate credit to the original author(s) and the source, provide a link to the Creative Commons licence, and indicate if changes were made. The images or other third party material in this article are included in the article’s Creative Commons licence, unless indicated otherwise in a credit line to the material. If material is not included in the article’s Creative Commons licence and your intended use is not permitted by statutory regulation or exceeds the permitted use, you will need to obtain permission directly from the copyright holder. To view a copy of this licence, visit http://creativecommons.org/licenses/by/4.0/ .
Reprints and permissions
About this article
Cite this article.
Sun, Y., Zhu, S., Wang, D. et al. Global supply chains amplify economic costs of future extreme heat risk. Nature 627 , 797–804 (2024). https://doi.org/10.1038/s41586-024-07147-z
Download citation
Received : 16 August 2022
Accepted : 01 February 2024
Published : 13 March 2024
Issue Date : 28 March 2024
DOI : https://doi.org/10.1038/s41586-024-07147-z
Share this article
Anyone you share the following link with will be able to read this content:
Sorry, a shareable link is not currently available for this article.
Provided by the Springer Nature SharedIt content-sharing initiative
By submitting a comment you agree to abide by our Terms and Community Guidelines . If you find something abusive or that does not comply with our terms or guidelines please flag it as inappropriate.
Quick links
- Explore articles by subject
- Guide to authors
- Editorial policies
Sign up for the Nature Briefing newsletter — what matters in science, free to your inbox daily.

- Action Alerts
- Construction Advocacy Fund
- Election Center
- Infrastructure
- AGC Foundation Awards
- Research Initiatives
- Scholarship Program
- What You Can Do
- 2024 Construction Safety Week & OSHA Falls Stand-Down
- Mental Health & Suicide Prevention
- Awards & Recognition
- Education and Training
- Hard Hats to Helmets
- Crane & Derricks in Construction
- AGC-Autodesk Safety Harness Grant Program
- Workforce Development
- Culture of CARE
- Diversity & Inclusion Committee
- Diversity & Inclusion Assessment
- State Law Matrix
- Contract Risk Management
- Dispute Resolution and Mitigation
- HRTED Practices E-Forum Archives
- Labor and Employment Advocacy
- Labor & HR Publications
- AGC Career Center
- Labor & HR Topical Resources
- Annual Risk Conference
- Crisis Response & Communication
- Risk SmartBrief
- Insurance & Surety Bonding
- Meet the Steering Committee
- Procurement
- Climate Change
- Education Catalog
- Virtual Learning
- AGC Emerging Contractors Education Series
- Instructor Resources
- Lean Construction Forum
- CM-BIM Holders Directory
- Construction Supervision Fundamentals
- Advanced Management
- Safety & Health
- News Releases
- Construction Employment
- Producer Price Indexes
- Construction Spending
- Construction Trend Power Point
- State Fact Sheet
- AGC Construction Inflation Alert
- AGC Construction Impact Model
- Data DIGest
- Supplemental Economic Resources
- Construction Diversity Image Library
- Newsletters
- ConstructorCast
- Member Directory
- Events Calendar
- AGC Convention
- Sponsorship
- Building Division
- Federal & Heavy Division
- Highway & Transportation Division
- Utility Infrastructure Division
- Construction Leadership Council
- AGC e-Forums
- Student Chapters
- AGC Connection
- Get Involved
- Get Started
- Discount Programs
- Capstone Supporters
- Member Company Employees
- Membership FAQs
- Create new account
- Forgot Password?
Employer Guidance on Selecting Head Protection for Construction
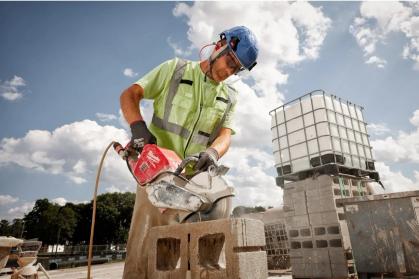
The Center for Construction Research and Training (CPWR) Expert Evaluation Panel recently released guidance for employers on selecting head protection equipment in construction . It covers the importance of head protection, types of hazards, standards and regulations, choosing the right helmet, and maintenance and replacement considerations. It emphasizes the need for proper fit, comfort, and suitability for the specific work environment.
For more information, visit AGC of America’s Hard Hats to Helmets webpage and/or contact Nazia Shah at [email protected] .
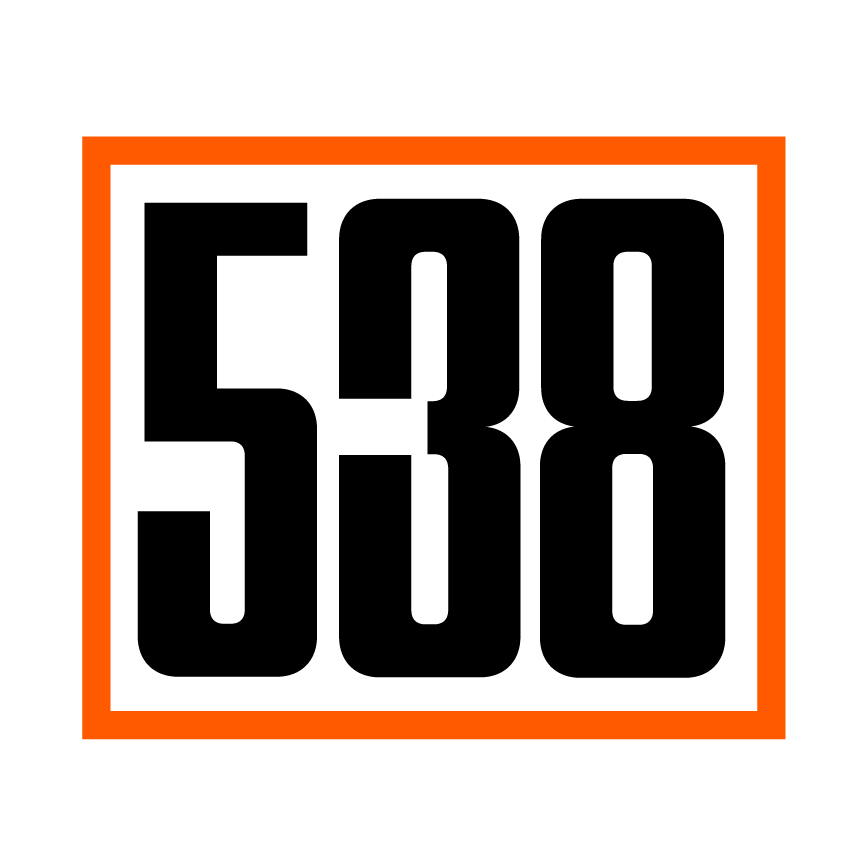
UPDATED Apr. 1, 2024, at 2:49 PM
Latest Polls
Check out our popular polling averages
Do Voters Want Republicans Or Democrats In Congress?
Generic ballot polling average
Who’s Ahead in West Virginia Senate Primary Polls?
State polling average
How Popular Is Joe Biden?
Approval polling average
Do Americans Have A Favorable Opinion Of Donald Trump?
Favorability polling average
Who’s ahead in the Maryland Senate general?
An updating average, accounting for each poll's recency, sample size, methodology and house effects..
95% OF POLLS PROJECTED TO FALL IN THIS RANGE
Candidates with insufficient polling data are not displayed in the averages. Read the full methodology here .
U.S. Senate, Maryland, 2024, General election
Indicates a polling average for this poll type
Candidate is an incumbent
Partisan types
Democratic-funded
Republican-funded
Other party-funded
Campaign poll
The position of the flag indicates whether the organization is partisan. Organizations are considered partisan if they operate on behalf of a candidate, party, campaign committee, PAC, super PAC, hybrid PAC , 501(c)(4), 501(c)(5) or 501(c)(6) organization that conducts a large majority of its political activity on behalf of one political party.
No matching polls
Read about how we use polls
Download the data.
When the dates of tracking polls from the same pollster overlap, only the most recent version is shown.
Data for FiveThirtyEight's previous favorability, presidential approval and national 2024 Republican primary averages is available for download here .
Notice any bugs or missing polls? Send us an email .
Design and development by Ryan Best , Aaron Bycoffe , Holly Fuong , Christopher Groskopf , Ritchie King , Ella Koeze , Dhrumil Mehta , Jasmine Mithani , Mary Radcliffe , Anna Wiederkehr and Julia Wolfe . Polling averages by G. Elliott Morris . Cooper Burton , Holly Fuong , Andrea Jones-Rooy , Dhrumil Mehta , Mary Radcliffe , Nathaniel Rakich , Derek Shan , Geoffrey Skelley and Julia Wolfe contributed research. Editing by Sarah Frostenson and Amelia Thomson-DeVeaux . Copy editing by Cooper Burton , Holly Fuong , Alex Kimball , Jennifer Mason , Andrew Mangan , Maya Sweedler and Curtis Yee .
Related Stories
Comments .
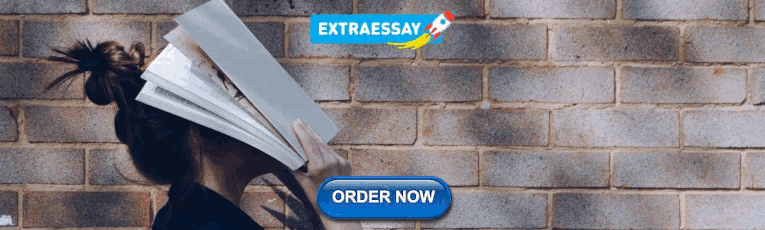
IMAGES
VIDEO
COMMENTS
There are various types of research that are classified by objective, depth of study, analysed data and the time required to study the phenomenon etc. Search for PhDs; ... In this type of research, reality is explained by general laws that point to certain conclusions; conclusions are expected to be part of the premise of the research problem ...
In general, though, the form your research design takes will be shaped by: The type of knowledge you aim to produce; The type of data you will collect and analyze; The sampling methods, timescale and location of the research; ... Choosing between all these different research types is part of the process of creating your research design, which ...
Research methods are specific procedures for collecting and analyzing data. Developing your research methods is an integral part of your research design. When planning your methods, there are two key decisions you will make. First, decide how you will collect data. Your methods depend on what type of data you need to answer your research question:
Types of research refer to the different methodologies used to conduct research. Different types may be better suited for certain studies based on your goals, timelines and purposes. The first task is to determine what you want to study and your goals. For example, you may want to learn more about a general topic or determine how a new policy ...
Research is the careful consideration of study regarding a particular concern or research problem using scientific methods. According to the American sociologist Earl Robert Babbie, "research is a systematic inquiry to describe, explain, predict, and control the observed phenomenon. It involves inductive and deductive methods.".
About Research Methods. This guide provides an overview of research methods, how to choose and use them, and supports and resources at UC Berkeley. As Patten and Newhart note in the book Understanding Research Methods, "Research methods are the building blocks of the scientific enterprise. They are the "how" for building systematic knowledge.
A research design is a strategy for answering your research question using empirical data. Creating a research design means making decisions about: Your overall research objectives and approach. Whether you'll rely on primary research or secondary research. Your sampling methods or criteria for selecting subjects. Your data collection methods.
You can also take a mixed methods approach, where you use both qualitative and quantitative research methods. Primary vs secondary data. Primary data are any original information that you collect for the purposes of answering your research question (e.g. through surveys, observations and experiments). Secondary data are information that has already been collected by other researchers (e.g. in ...
General Types of Educational Research. Descriptive — survey, historical, content analysis, qualitative (ethnographic, narrative, phenomenological, grounded theory, and case study) Associational — correlational, causal-comparative. Intervention — experimental, quasi-experimental, action research (sort of)
Their motivations will affect the design of the research and will, to a great extent, determine the way the results are interpreted and reported. Academic research. Academic research is primarily concerned with developing a theoretical explanation or understanding of an issue. For most people the motivation will be clear - the research will ...
Thus, applied research involves original research, not just reviewing what others have done, but like secondary research it is motivated to get an answer. The third type is the least common, but is also generally the focus of a textbook like this. Academic research is the type of research that your professors do most of the time.
The Research Methods Videos database contains hours of tutorials, interviews, video case studies, and mini-documentaries covering the entire research process. ... There are two general types of observational designs. In direct observations, people know that you are watching them. Unobtrusive measures involve any method for studying behavior ...
Section 2 provides general descriptions on each of the research types. Section 3 is provided to discuss the qual-itative approaches and the process. Section 4 includes the quantitative process and its different methods, and then the advantages and limitations of both methods are discussed in section 5. Finally, section 6 shows different
This will guide your research design and help you select appropriate methods. Select a research design: There are many different research designs to choose from, including experimental, survey, case study, and qualitative designs. Choose a design that best fits your research question and objectives.
Research methods refer to the specific approaches and techniques used to collect and analyze data in a research study. There are various types of research methods, and researchers often choose the most appropriate method based on their research question, the nature of the data they want to collect, and the resources available to them. Some ...
Expands human knowledge. Similar to analytical or basic research. Secondary. Consolidates existing knowledge. Establishes what's already known. Proves the need for primary research. Discovers methods and protocols for primary research. Similar to descriptive research. There are, however, many other types of research, often used only in ...
Types of study design. Medical research is classified into primary and secondary research. Clinical/experimental studies are performed in primary research, whereas secondary research consolidates available studies as reviews, systematic reviews and meta-analyses. Three main areas in primary research are basic medical research, clinical research ...
The two major classes of research are: Qualitative Research - subjective, seeks a human's experience as a narrative. Quantitative Research - objective, seeks to statistically make inferences about a sample to generalize to the larger population. We need to have a solid understanding of the difference between the two main types of research ...
Qualitative Research Methodology. This is a research methodology that involves the collection and analysis of non-numerical data such as words, images, and observations. This type of research is often used to explore complex phenomena, to gain an in-depth understanding of a particular topic, and to generate hypotheses.
Research methodology is classified based on different categories. They include a general category, nature of the study, purpose, research design, and data type. There are also interviews and case studies based on research methodology. In some research, the researcher combines more than two and very few methods.
Thesis is a type of research report. A thesis is a long-form research document that presents the findings and conclusions of an original research study conducted by a student as part of a graduate or postgraduate program. It is typically written by a student pursuing a higher degree, such as a Master's or Doctoral degree, although it can also ...
Qualitative research involves collecting and analyzing non-numerical data (e.g., text, video, or audio) to understand concepts, opinions, or experiences. It can be used to gather in-depth insights into a problem or generate new ideas for research. Qualitative research is the opposite of quantitative research, which involves collecting and ...
Procedure. The procedure for this research is multi-stepped and is summarized in Fig. 1.First, we mapped retrospective qualitative data collected during the development of the SCI-HMT [] against the five domains of the CFIR in order to create a semi-structured interview guide (Step 1).Then, we used this interview guide to collect prospective data from health professionals and people with SCI ...
Invest in human-machine interfaces, or "human in the loop" technologies that augment human intelligence. People at all levels of an organization need training and support to thrive in an increasingly automated world. AI is just the latest tool to help individuals and companies alike boost their efficiency.
General Directions. The final report is intended to provide a comprehensive account of your collaborative course project in data science. The report should demonstrate your ability to apply the data science skills you have learned to a real-world project holistically, from posing research questions and gathering data to analysis, visualization ...
a-d, Evolutionary trends of the four types of losses from 2040 to 2060 under different scenarios (health loss (a); labour productivity loss (b); indirect loss (supply-chain disruptions) (c); and ...
The Center for Construction Research and Training (CPWR) Expert Evaluation Panel recently released guidance for employers on selecting head protection equipment in construction. It covers the importance of head protection, types of hazards, standards and regulations, choosing the right helmet, and maintenance and replacement considerations. It emphasizes the need for proper fit, comfort, and ...
The position of the flag indicates whether the organization is partisan. Organizations are considered partisan if they operate on behalf of a candidate, party, campaign committee, PAC, super PAC, hybrid PAC, 501(c)(4), 501(c)(5) or 501(c)(6) organization that conducts a large majority of its political activity on behalf of one political party.
1 VACANCY NOTICE Open to Internal and External Candidates Position Title : Senior Programme Assistant - Data and Research VN No : IOMKCO/VN/022/2024 Duty Station : Nairobi, Kenya Classification : General Service Staff, Grade G7 Type of Appointment : One Year Fixed Term, with possibility of extension Estimated Start Date : As soon as possible