Thank you for visiting nature.com. You are using a browser version with limited support for CSS. To obtain the best experience, we recommend you use a more up to date browser (or turn off compatibility mode in Internet Explorer). In the meantime, to ensure continued support, we are displaying the site without styles and JavaScript.
- View all journals
- My Account Login
- Explore content
- About the journal
- Publish with us
- Sign up for alerts
- Open access
- Published: 21 February 2024
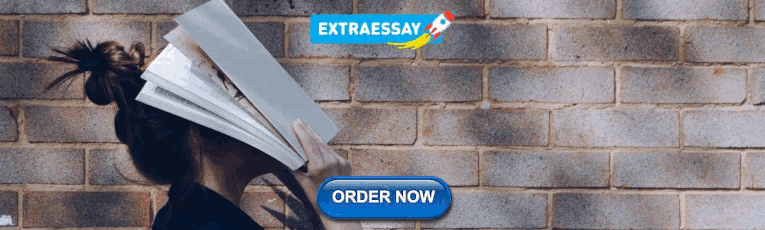
A precise model for skin cancer diagnosis using hybrid U-Net and improved MobileNet-V3 with hyperparameters optimization
- Umesh Kumar Lilhore 1 ,
- Sarita Simaiya 1 ,
- Yogesh Kumar Sharma 2 ,
- Kuldeep Singh Kaswan 3 ,
- K. B. V. Brahma Rao 2 ,
- V. V. R. Maheswara Rao 4 ,
- Anupam Baliyan 1 ,
- Anchit Bijalwan 5 &
- Roobaea Alroobaea 6
Scientific Reports volume 14 , Article number: 4299 ( 2024 ) Cite this article
874 Accesses
1 Altmetric
Metrics details
- Cancer imaging
- Skin cancer
Skin cancer is a frequently occurring and possibly deadly disease that necessitates prompt and precise diagnosis in order to ensure efficacious treatment. This paper introduces an innovative approach for accurately identifying skin cancer by utilizing Convolution Neural Network architecture and optimizing hyperparameters. The proposed approach aims to increase the precision and efficacy of skin cancer recognition and consequently enhance patients' experiences. This investigation aims to tackle various significant challenges in skin cancer recognition, encompassing feature extraction, model architecture design, and optimizing hyperparameters. The proposed model utilizes advanced deep-learning methodologies to extract complex features and patterns from skin cancer images. We enhance the learning procedure of deep learning by integrating Standard U-Net and Improved MobileNet-V3 with optimization techniques, allowing the model to differentiate malignant and benign skin cancers. Also substituted the crossed-entropy loss function of the Mobilenet-v3 mathematical framework with a bias loss function to enhance the accuracy. The model's squeeze and excitation component was replaced with the practical channel attention component to achieve parameter reduction. Integrating cross-layer connections among Mobile modules has been proposed to leverage synthetic features effectively. The dilated convolutions were incorporated into the model to enhance the receptive field. The optimization of hyperparameters is of utmost importance in improving the efficiency of deep learning models. To fine-tune the model's hyperparameter, we employ sophisticated optimization methods such as the Bayesian optimization method using pre-trained CNN architecture MobileNet-V3. The proposed model is compared with existing models, i.e., MobileNet, VGG-16, MobileNet-V2, Resnet-152v2 and VGG-19 on the “HAM-10000 Melanoma Skin Cancer dataset". The empirical findings illustrate that the proposed optimized hybrid MobileNet-V3 model outperforms existing skin cancer detection and segmentation techniques based on high precision of 97.84%, sensitivity of 96.35%, accuracy of 98.86% and specificity of 97.32%. The enhanced performance of this research resulted in timelier and more precise diagnoses, potentially contributing to life-saving outcomes and mitigating healthcare expenditures.
Similar content being viewed by others
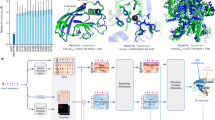
Highly accurate protein structure prediction with AlphaFold
John Jumper, Richard Evans, … Demis Hassabis
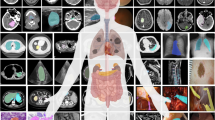
Segment anything in medical images
Jun Ma, Yuting He, … Bo Wang
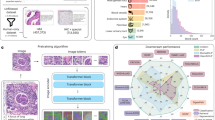
A visual-language foundation model for computational pathology
Ming Y. Lu, Bowen Chen, … Faisal Mahmood
Introduction
Skin cancer is considered one of the most widespread and significant forms of cancer globally, with its occurrence consistently rising in recent years. The prompt and precise identification of a medical condition is crucial for successfully implementing treatment strategies and enhancing patient results. Historically, dermatologists have traditionally depended on their professional knowledge and visual examination of skin lesions in order to detect possible malignancies. Nevertheless, this procedure is inherently subjective and prone to diagnostic inaccuracies 1 . In response to all these barriers, Computer-Aided Diagnosis (CAD) frameworks significantly improved medical dermatology. Deep learning models that fall into the category of "convolutional neural networks," also known as "CNNs," have shown remarkable promise for improving the accuracy of skin cancer recognition 2 . Figure 1 presents an image of a skin cancer sample.
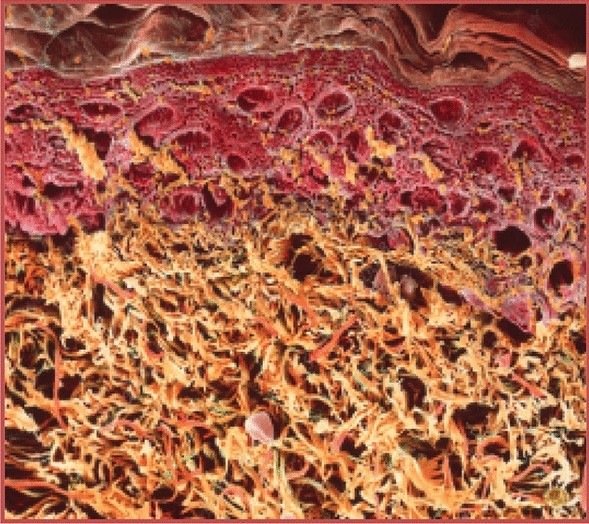
Skin cancer sample 23 .
Melanoma and non-melanoma are the two primary subtypes of skin cancer, distinguished based on the cell that is transformed into a cancerous form. Various machine learning and deep learning frameworks and methodologies have been suggested to detect, classify, and segment skin cancer. Examples of these include Support Vector Machines (SVM), Decision Trees (DT), Fuzzy C-means, Recurrent Neural Networks (RNN), Convolutional Neural Networks (CNN) and Deep Neural Networks (DNN) 3 . CNN is a widely employed algorithm for automated feature learning and extraction, known for its exceptional performance in detection tasks 4 . Moreover, the training stage for deep learning networks often necessitates a substantial volume of data. Therefore, using transfer learning to refine a pre-existing network on comparable tasks can reduce training complexity, expedite convergence, and decrease training duration 5 .
Additionally, it is possible to utilize pre-trained deep learning models as feature extractors without additional training, provided they have already been trained on tasks or domains that are similar or related 6 . The deep learning model may acquire features that contain noise, thereby impacting the accuracy of the final classification. This can be attributed to the inclusion of non-relevant features and the naturally high dimensionality of the characteristics learned. Therefore, utilizing optimization techniques, specifically metaheuristic methods, can provide a viable approach for selecting elements. This approach involves identifying and choosing the most pertinent features, thereby enhancing the precision of recognition 7 .
This research paper introduces a comprehensive methodology to handle the complex issues related to skin cancer detection. This research presents a novel skin cancer recognition model based on optimized CNN architecture. This research utilizes deep learning and advanced optimization techniques to improve skin cancer detection's precision and effectiveness. The motivation behind this research originates from the critical requirement to deal with the primary healthcare problem related to dermatological cancer. Millions of people worldwide have skin cancer, and the disease's incidence is still rising. The prompt and precise identification of a condition or disease is crucial to administer the correct therapy and, finally, to preserve human life. Conventional diagnostic techniques, dependent on individual observation, are subjective and may change in precision based on the training and experience of the medical professional 8 .
The presence of subjectivity and the possibility of error underscore the utmost significance of advancing the development of more accurate and automated mechanisms for identifying skin cancer. The primary motivation behind our research stems from the pressing demand for enhanced techniques in detecting skin cancer. We harness their capabilities by harnessing deep learning and optimization methodologies to address this objective. Furthermore, the emergence of deep learning methods, i.e., CNNs, has shown the capacity for transformation in analyzing healthcare images. The capability of CNNs to identify complicated characteristics from visuals and to make decisions based on information presents an exciting chance for improvement in the discipline of skin diseases 9 . The convergence of urgent medical necessity and state-of-the-art technology constitutes the primary impetus for our investigation, compelling us to construct a sophisticated model for identifying skin cancer that harnesses the capabilities of deep learning and optimization methodologies.
The primary contributions of our research encompass innovative model architecture, meticulous hyperparameter optimization, persuasive experimental findings, and significant clinical implications. These collective achievements propel the current state of advancement in dermatological examinations and contribute to the enhancement of the treatment of patients. The proposed research presents several significant contributions to the domain of skin cancer detection and healthcare:
We enhance the learning procedure of deep learning by integrating Standard U-Net and Improved MobileNet-V3 with optimization techniques, which allows the model to accomplish better differentiation among malignant and benign skin cancers.
Our research focuses on the meticulous examination of hyperparameter optimization, a crucial factor that substantially influences the efficacy of deep learning models. Advanced optimization techniques, i.e., Bayesian optimization and grid search, are utilized to optimize the hyperparameter of the model effectively.
The optimization of hyperparameters is of utmost importance in enhancing the efficiency of deep learning models. To fine-tune the model's hyperparameter, we employ sophisticated optimization methods such as the Bayesian optimization method using pre-trained CNN architectures, i.e., MobileNet-V3, on the “HAM-10000 Melanoma Skin Cancer” dataset.
The proposed hybrid MobileNet-V3 model outperforms existing techniques based on high precision of 97.84%, sensitivity of 96.35%, accuracy of 98.86%, and specificity of 97.32%.
The article is organized in the following way: after an introductory part that provides an overview of the motivation and aims, a comprehensive examination of existing literature on skin cancer detection is presented in section two. The methodology section three offers a thorough account of the procedures employed for data collection, preliminary processing, and the development of a hybrid architecture specifically designed to detect skin cancer. Additionally, it includes a detailed explanation of the process of hyperparameter optimization. Section four provides a detailed description of the experimental setup, presents the results and performance indicators obtained, and compares them to existing methods. An extensive examination of the consequences, advantages, and drawbacks of the proposed method follows. The conclusion section five serves the purpose of combining the main findings of the research and highlighting their potential clinical significance.
Literature review
Previous research was investigated concerning the categorization of Skin Cancer using deep learning techniques. In the context of the categorization of Skin Cancer utilizing deep learning techniques, scholars generated skin lesion images sourced from publicly available websites.
Data augmentation methods were employed alongside five-fold cross-validation methods to enhance the dataset. The investigators conducted experiments to evaluate the performance of pre-trained VGG-16, ResNet50, and InceptionNet-V3 models in classifying Skin Cancer diseases. The ResNet50 model was selected due to its superior accuracy rate compared to the other models. The ResNet50 model achieves an F1 Score of 0.82, as reported in reference 10 . The researchers used the Xception training model with the Grad-Cam and the LIME algorithm techniques to predict skin cancer 11 . The Xception and the DenseNet models have implemented an integrated strategy utilizing unity. The research involved the analysis of the efficiency scores retrieved from experiments conducted on a publicly accessible dataset. These experiments were carried out using this suggested ensemble strategy. The results indicated an average recall, precision, F1 score, and accuracy rate of 86.74%, 85.8%, 86.24%, and 88.93%, respectively.
The article 12 presents research on the utilization of YOLOv4-DarkNet along with active contour techniques to localize and segment melanoma. The method was evaluated on the International Skin Imaging Collaboration (ISIC) datasets for 2016 and 2018. The values of one and the reported Jaccard coefficient were 1 and 0.989, respectively. The paper 13 discusses the topology of FC-DPN segmentation. The construction of the network involved the implementation of a dual-path and fully convolutional architecture. In the revised ISIC 2017 test dataset, the suggested approach achieved a Jaccard index of 81.32% and an average dice coefficient of 87.2%.
Similarly, for the PH2 dataset, a Jaccard index of 84.3% and an average dice coefficient of 91.76% were obtained. The paper discusses a CNN model for the classification of skin cancer 14 . The dataset was initially gathered and subsequently categorized into four distinct groups of skin cancer images. Later, augmentation techniques were employed to expand the dataset's size. During the testing phase, the algorithm developed by the researchers achieved an accuracy of 96.28%, surpassing the accuracy of the GoogleNet and MobileNet models by 1.76% and 1.12%, respectively.
The application of deep learning techniques for skin cancer detection has been the subject of considerable investigation in recent academic literature. Several deep learning techniques, including CNNs, have been utilized to categorize skin lesions as either cancerous or non-cancerous precisely. The authors of 15 present a unique deep learning network called the Dual Optimisation Utilising Deep Learning Network. This network was designed for skin cancer identification using dermoscopic images and achieved an impressive precision of 98.76%. This paper presents a thorough examination of conventional and deep-learning methodologies employed in the prompt identification of skin cancer. It critically evaluates the performance of these techniques and examines the datasets utilized for training and testing purposes 16 . The authors have developed a deep learning system using a CNN to detect melanoma lesions. This system has demonstrated superior performance regarding diagnostic accuracy compared to existing methods, as reported in reference 17 . The research proposes using convolutional-based neural networks with deep reinforcement learning to identify cancer-affected skin. It aims to overcome the challenges related to generalizability by implying the adoption of ensemble models to achieve optimal output, as indicated by reference 18 .
Recent studies have utilized various types of classification and preliminary processing methods to conduct morphological change examines on grey-level skin cancer images. These images were obtained from the PH2 repository and were subjected to classification and clustering procedures using a pre-trained Levenberg-Mean neural network 6 . Transfer learning has been utilized to forecast skin cancer visuals from the HAM10000 database precisely using the MobileNet CNN 19 . The recent research on the application of CNN in predicting skin cancer has identified significant challenges in incorporating the entire spectrum of the patient population and various melanoma subtypes 20 . In addition, ongoing efforts are being made to develop optimization algorithms that enhance accuracy by adjusting hyperparameters 21 . Moreover, recent studies have put forth methods to identify instances of shortcut development during the training of models based on convolutional neural networks on both the ISIC Storage collection. Recent research has also emphasized using comprehensible deep-learning techniques for the multi-class separation and categorization of skin lesion images 22 .
Predicting skin diseases using AI holds enormous promise for better early detection and treatment results 23 . This study offered a new way to improve skin disease prediction models by addressing class imbalance utilizing data balancing using class weighting and transfer learning approaches. They have also investigated the usefulness of data balancing using class weighting to boost TL performance for skin disease prediction. Experiments and performance evaluations have yielded important insights and outcomes, proving that the suggested method works. Optimized kernel-enhanced Resnet-dropped extended short-term memory technique for varicose vein disease detection was addressed in 24 . After processing 11,350 photos of leg veins, the experiment was evaluated using metrics such as accuracy, precision, kappa, mean square error (MSE), and time complexity. With a time complexity of 25.36 ms, a kappa of 95.77%, a precision of 98.69%, and an MSE of 1.73, the suggested model attained an accuracy of 98.5%.
Although skin cancer is dangerous, it is successfully treatable if caught early. A procedure is involved in the suggested approach 25 . Improving the quality and extracting useful information from the raw photos is the first step in the pre-processing phase. The next step is to feed the pre-processed pictures into a GRU Network, a deep-learning model that excels at capturing sequential information. The GRU Network was fine-tuned by the author using an improved version of the Orca Predation Algorithm (OPA). In comparison to previous approaches, the GRU/IOPA system achieved better results in terms of accuracy (0.96), sensitivity (0.95), specificity (0.97), PPV (0.95), and NPV (0.96). When compared to more conventional methods, these findings show that the suggested technique is superior in detecting skin cancer.
In order to better identify skin lesions or cancer, researchers have built an upgraded machine-learning framework 26 . This study segments and categorizes skin diseases and cancers using a machine-learning framework and an optimization method derived from fruit flies. The proposed method provides enhanced classification efficiency with a 98% accuracy rate, 99% specificity, 96% sensitivity, 95% JSI, and 99% DSC.
In 27 , we looked at a framework for multi-class skin disease classification that uses an LSTM, a sophisticated CNN, and MobileNet-V2 to improve the reliability and accuracy of cancer diagnoses. The proposed method leverages CNNs' self-learning discriminative features from unprocessed skin photos and long short-term memory (LSTM) capability to manage multi-class classification problems. The hybrid model showed promise in the experiments for improving the accuracy and efficiency of skin disease classification across a variety of categories.
The authors of 28 laid up a two-pronged approach to skin lesion categorization. First, during the image pre-processing phase, two distinct techniques were suggested for picture segmentation and feature extraction. Finally, S-MobileNet, a model for a deep convolutional neural network, is being developed with the intention of classifying seven distinct skin lesion types. When it comes to skin lesion picture classification in the HAM10000 dataset, the suggested deep learning-based S-MobileNet model is the way to go, according to experimental results.
In 29 , the authors provide a deep-learning architecture that can identify Melanoma and multi-class skin cancers. Four main phases make up the suggested architecture: pre-processing images, extracting and fusing features, selecting features, and finally, classification. Using the picture luminance data, a new method for enhancing contrast was suggested. The next step is to train two pre-trained deep models, DensNet-201 and DarkNet-53, using transfer learning to modify them with respect to a residual block at the end. The last step in the classification process was to use machine learning classifiers on the features that were chosen. For this experiment, we used two datasets: ISIC2018 and ISIC2019 29 , 30 . This dataset achieved the highest precision of 85.4%, whereas the other achieved 98.80%. Table 1 presents a comparative analysis of existing skin cancer research using a deep learning model.
Materials and methods
Skin cancer dataset.
This research utilizes the online ‘HAM-10000’ skin cancer dataset 33 . The dataset HAM-10000, referred to as 'Human against the machine', with 10,000 images used for training, is a collection of images of skin lesions utilized in skincare research. The dataset comprises visual representations of diverse dermatological disorders, encompassing Melanoma, nevus, and several related afflictions. The provided dataset is used to classify and diagnose skin diseases. As mentioned earlier, deep learning models trained on the dataset can discern different types of skin cancer, including malignant tumours that may exhibit malignant characteristics.
This dataset contains 10,015 dermatoscopic images with size (450*600) pixels. The dataset comprises seven diagnostic Skin cancer categories. The dataset classes include classes from zero to six, names as ‘melanoma’, ‘melanocytic nevus’, ‘basal cell carcinoma’, ‘actinic keratosis’, ‘benign keratosis’, ‘dermatofibroma’ and ‘vascular lesion’. Figure 2 presents the details of skin cancer classes and counts, and Fig. 3 illustrates the gender-wise distribution of skin cancer infection, including a 54% male, 45.5% Female and 0.5% unknown patient distribution in the dataset.
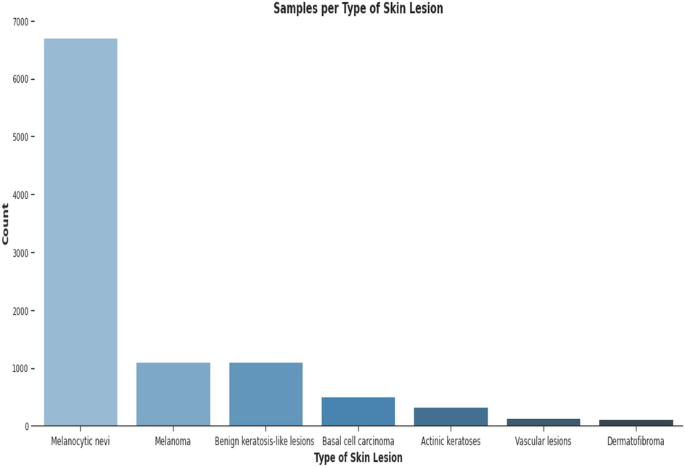
Skin cancer classes and counts.
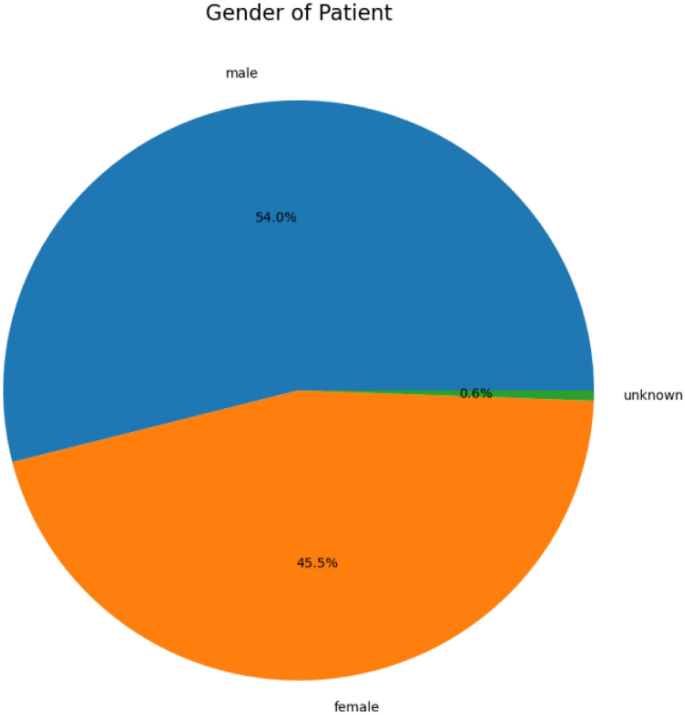
Gender-based skin cancer distribution.
Data pre-preprocessing
A critical first step in getting a skin cancer dataset ready for training a skin cancer identification framework is data preliminary processing. In addition to promising that the data is in a format appropriate for training, proper data pre-processing can enhance model performance 31 . The skin cancer dataset is pre-processed using the following crucial steps.
Data augmentation
Since there are unequal numbers of images in each category, data balancing is used to balance all types before the training procedure. Data augmentation techniques are used for data balancing. In this process, the following ranges are used: (a) rotation 25 degrees; (b) width and height shifting 15%; (c) shearing 15%; (d) employing flipping in both the vertical and horizontal directions; and (5) adjusting brightness within the [0.9: 1.5] range. Data augmentation is also applied to the images during the learning and optimization stages to prevent over-fitting and boost diversity. Table 2 presents the parameters used for the skin cancer dataset. After pre-processing, the images of (450*600) pixels were converted into 192*256 pixels. Figure 4 presents data augmentation results. Table 3 shows the data count after the data pre-processing steps for class 0 to class 6.
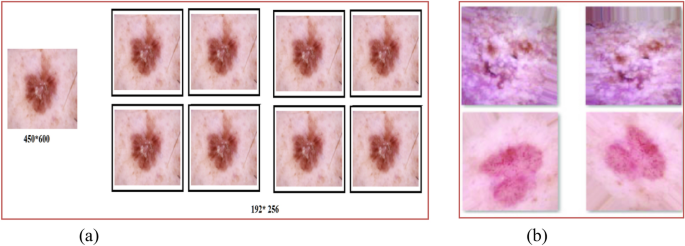
Data augmentation ( a ) image resizing, ( b ) zooming.
We use Eq. ( 1 ) for horizontal flipping operation, i.e., x-axis, 2 for rotation and 3 for shifting, 4 for shearing, and 5 for zooming. In these equations, h represents the rotating angle, t x represents the amount of shifting in tandem with the x-axis, t y represents the shifting in tandem with the y-axis, sh x represents the shear factor in tandem with the x-axis, sh y represents the shear factor together the y-axis, and C x represents the zoom factor together the x-axis, and C y represents the zoom factor in tandem the y-axis 32 . Here, FM is the flipping Matrix, RM is the rotation Matrix, SM is the shifting Matrix, SheM is the shearing Matrix, and ZM is the zooming Matrix.
Proposed model architecture
The proposed model is based on Standard U-Net and Improved MobileNet-V3 with optimization techniques, i.e., the Bayesian optimization method. Standard U-Net performs semantic segmentation activities in the proposed model, and MobileNet-V3 is used as a Feature Extractor. We also utilize a Bayesian optimization method for hyperparameter optimization. This method can identify the most promising hyperparameters by prioritizing those that have shown favourable outcomes in previous results. Figure 5 shows the architecture of the proposed hybrid model.
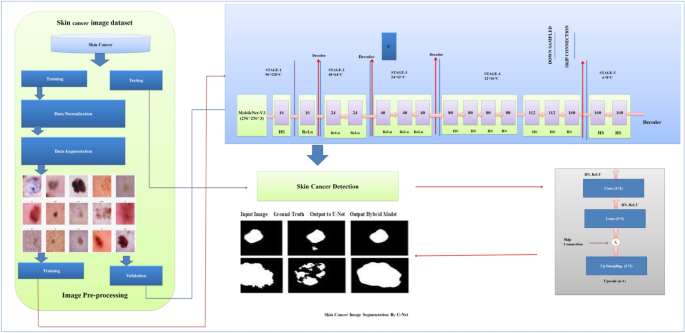
Architecture of proposed hybrid model.
Improved MobileNet-V3
In the proposed hybrid model, the existing MobileNet-V3 model is improved. The Improved MobileNet-V3 worked as an encoder in the proposed skin cancer prediction and segmentation model. The steps below enhanced the MobileNet-V3 structure for skin cancer recognition and segmentation. Figure 6 presents the architecture of the proposed MobileNet-V3 31 .
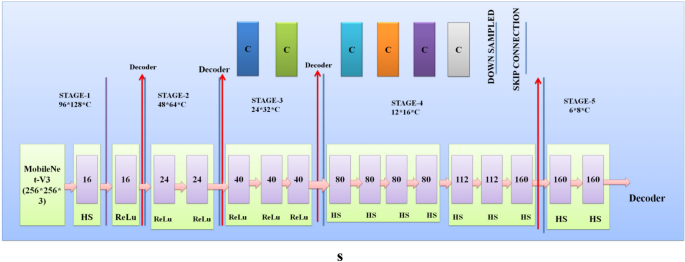
Architecture of proposed MobileNet-V3.
Architectural level Improvements: The following fundamental changes were performed at the existing MobileNet-V3 architecture 32 .
Deeper Model A deeper MobileNet-V3 model's depth (layer counts) is determined by the model's structural preferences. MobileNet-V3 is available in two predetermined sizes: "large" or "small," with "large" featuring more layers and greater capacity than "small." The "large" variant of MobileNet-V3 generally includes 157 layers. Depthwise separable convolution layers and flipped residuals using growing and squeeze-and-excitation (SE) segments are formed.
Wider Model MobileNet-V3 predominantly employs the flipped residual block and SE block. A depthwise convolution component is subsequently followed by a point-wise convolution layer to form the inverted residual block. The point-wise convolution changes the width (number of channels). Adjust the point-wise convolution layer output channels to widen a layer. This is typically accomplished by multiplying the total amount of channels through a scaling factor. Modulating channel-wise dependencies is done with the SE block. Although it cannot naturally regulate layer width, it can be utilized on layers to enhance model performance and adjust to particular tasks. Fully connected layers (FCL), Global average pooling (GAP), and element-wise scaling (EMS) comprise the SE block. The number of channels present in the map of input features is used as a criterion to establish the size of the SE block. MobileNet-V3 allows you to modify the range of channels within the starting convolutional layer with the last classification layer to adjust the total width of the resulting model for skin cancer analysis.
Attention Method We substituted the crossed-entropy loss function of the Mobilenet-v3 mathematical framework with a bias loss function that enhanced accuracy. The model's SE component was replaced with the adequate channel attention (ECA) component to achieve parameter reduction. Integrating cross-layer connections among Mobile modules has been proposed to leverage synthetic features effectively. The dilated convolutions were incorporated into the model to enhance the receptive field.
Regularization method
The following Regulization techniques were applied to improve the MobileNet-V3 model 32 .
Dropout Implement dropout layers inside the MobileNet-V3 structure to prevent overfitting throughout training. Dropout arbitrarily eliminates a portion of the neurons throughout each forward pass to improve the model's generalization ability.
L2 regularization's weight decay Applying a weight decay component to the model's loss function for the L2 regularization's weight decay step. Weight decay promotes the reduction of model weights, preventing unnecessary complexity and overfitting.
Normalization method
The following Normalization techniques were applied for improvements in the MobileNet-V3 model.
Batch Normalization To stabilize training, employ batch normalization layers to normalize the activations throughout each mini-batch. Batch normalization can boost the model convergence process and boost training.
Layer Normalization We have applied a layer normalization method that helps
Standard U-Net architecture
The Standard U-Net architecture is a convolutional neural network specifically developed for semantic segmentation tasks. The algorithm demonstrates exceptional proficiency in partitioning regions of interest within medical images, rendering it highly suitable for the analysis of skin cancer. The Standard U-Net architecture is distinguished by incorporating skip connections and embedded skip procedures, which efficiently capture multi-scale context-relevant data 33 .
Four Standard U-Net patterns, identical to U-Net, are used to complete the segmentation assignment. Batch normalization and GeLU for the hidden activation mechanism are utilized in the additional configuration, while the architecture described in 10 is used in the initial setup. Batch normalization (BN) mainly creates batches of a specific size. Along with batch normalization and the GeLU hidden activation function, the third and fourth patterns use VGG-19 and DenseNet-201 as their backbone. In the case of configuration four, deep supervision is not enabled. Figure 7 presents the standard U-Net architecture.
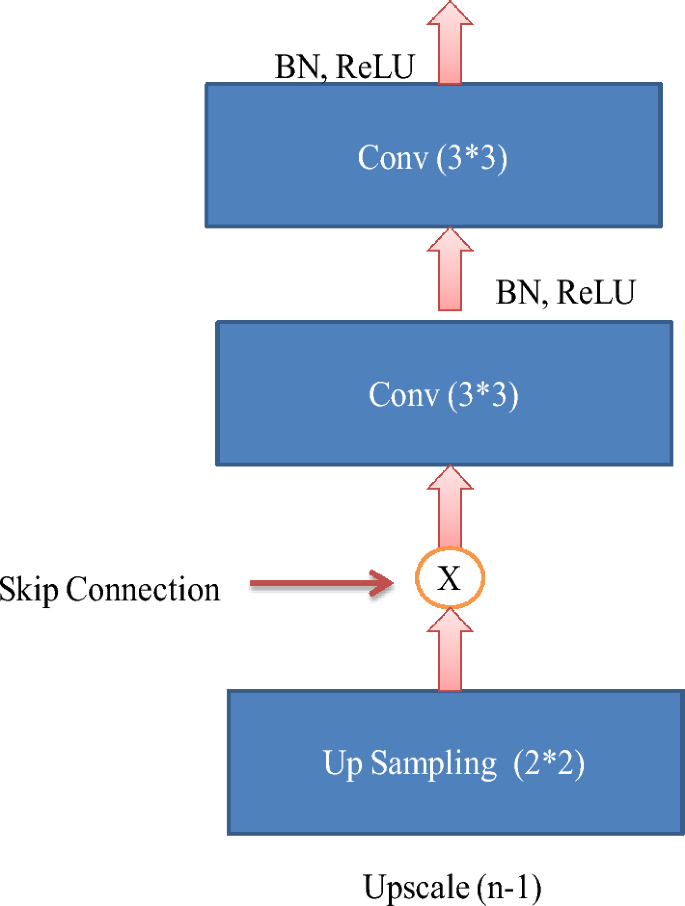
Standard U-Net architecture.
Bayesian optimization method
Bayesian optimization is an approach that employs probabilistic algorithms to search accurately for the most practical combination of hyperparameters to support a model built using deep learning. It functions by analyzing an ambiguous objective function within this instance, which evaluates the model's effectiveness as a Gaussian procedure, enabling it to make well-informed choices about where to start sampling the target function subsequently. The following describes the operation of Bayesian optimization in the hyperparameter optimization for skin cancer 6 .
Step 1: Initial Random Sampling To create an initial substitute framework for the objective function, Bayesian optimization usually begins with a few random specimens taken through the hyperparameter space. The function's behaviour can be partially understood because of these arbitrary specimens.
Step 2: Surrogate Model The algorithm generates a probabilistic substitute model using the initial specimens, generally a Gaussian procedure (GP). The GP prototypes the objective function's allocation and predicts the function's behaviour throughout the hyperparameter area. This substitute model encompasses average forecasting and an uncertainty determination (variance) at every point within the hyperparameter space.
Step 3: Acquisition Function Bayesian optimization utilizes an acquisition function for identifying the subsequent objective function specimen location. Typical acquisition functions consist of Probability of Improvement (PI), Expected Improvement (EI), and Upper Confidence Bound (UCB). These features balance examinations (sample collection in spaces of uncertainty) and exploitation (sampling in spots that are likely to produce more effective results).
Step 4: Next Sample Selection The acquisition function directs the selection of the subsequent collection of hyperparameters to be assessed. The method chooses hyperparameter values that maximize the acquisition function, indicating promising regions within the hyperparameter space for enhancing the objective function.
Step 5: Evaluate Objective Function The chosen hyperparameters are utilized for training and evaluating a deep learning framework via the skin cancer dataset. The objective function (including model precision and loss) gets calculated according to the model's efficiency.
Step 6: Update Surrogate Model: After assessing the objective function based on the chosen hyperparameter, the procedure upgrades the substitute model (GP) along with the newly acquired data point, including the evaluation results, to minimize uncertainty within the substitute model.
Step 7: Iteration Steps 3 through 6 are carried out for an established amount of iterations or until convergence is achieved. The method improves the substitute model iteratively and chooses a hyperparameter to enhance the model's accuracy.
Step 8: Final Result The Bayesian optimization method produces the hyperparameters, which generate the best objective function importance according to the model's behaviour.
- Transfer learning
ImageNet is used as a pre-training dataset for the MobileNet-V3 model, and then the Ham-10000 skin cancer dataset is used to fine-tune the model's parameters. Transfer learning enables the predictive algorithm to utilize features acquired within a broader context before becoming proficient in the skin cancer recognition assignment 34 .
Pseudo code for the proposed model
Algorithm 1 presents the steps for skin cancer analysis with the hybrid U-Net and MobileNet-V3 models 35 .
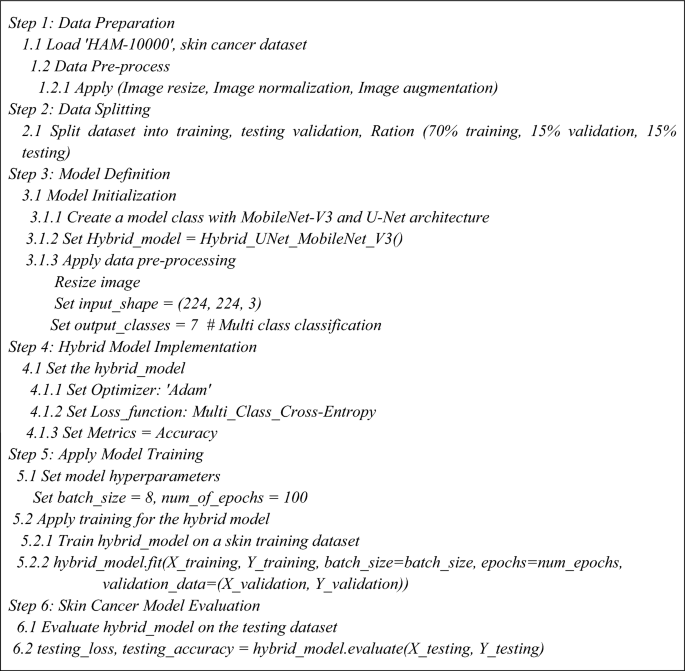
Skin Cancer analysis with Hybrid U-Net and MobileNet-V3.
Performance measuring parameters
We use parameters like accuracy, precision, F-measure, the area under the curve (AUC), and confusion metrics 6 , 31 , 32 , 33 to determine how to determine the present model and the one we have suggested works. The components of the confusion matrix, including TP (true positive), TN (true negative), FP (false positive), and FN (false negative), have been utilized in its construction. The subsequent passage comprehensively explains the previously referenced statistics 34 .
Precision (Prc) This illustrates the proportion of individuals who test positive correctly (true positives) and incorrectly (false positives) concerning the total number of positive individuals as described in Eq. ( 6 ).
Recall (RC) or Sensitivity The calculation of recall, also known as true positive rate 35 , involves dividing the number of true positives by the total number of instances that ought to be considered anticipated as positive, as described in Eq. ( 7 ).
F-Measure (FMs) The F-score, often referred to as the 'F1 score' or 'F-measure' 36 , serves as an empirical metric for assessing the effectiveness of a deep learning model. The integration of recall and precision results in an integrated score, as described in Eq. ( 8 ).
Accuracy (ACR) The evaluation of classification models often involves the consideration of various metrics, with accuracy being one of them 37 . In a more formal context, accuracy can be defined as the proportion of correct predictions our model makes, as described in Eq. ( 9 ).
Area Under the Curve (AUC) AUC refers to measuring the extent or size of two areas under a curve, which refers to the region bounded by the curve and the coordinate axes 33 , 38 . To determine this area, one can divide it into infinitesimally small rectangles and sum their areas. By incorporating the limit of this summation, as the rectangles become infinitely small, the total area under the curve can be calculated.
Ethical approval and consent to participate
No ethical approval is required, and the authors consent to participate in the paper.
Experimental results and evaluation
This section covers the simulation results of the existing and proposed model.
Experimental setup
The proposed and existing techniques, i.e., MobileNet, Resnet-152v2, VGG-16, MobileNet-V2, and VGG-19, were implemented using software and hardware components. Table 4 presents the parameters used in experimental analysis for proposed and existing models. Table 5 shows the Configuration parameters of Standard U-Net architecture used in a proposed hybrid model for image segmentation 39 , 40 , 41 , 42 , 43 .
Simulation results and discussion
The HAM-10000 Skin cancer dataset serves as the training ground for the proposed model and several existing deep learning models, i.e., MobileNet, Resnet-152v2, VGG-16, MobileNet-V2, and VGG-19. The dataset was divided into training, testing and validation with a ratio of 70:15:15. The experimental results and discussion are as follows.
Table 6 presents the experimental results of the testing dataset for 100 epochs for existing and proposed models. The combined utilization of the U-Net and MobileNet-V3 models yielded exceptional results in classifying skin cancer diseases.
Table 7 presents the experimental results of the validation dataset for 100 epochs for existing and proposed models. The proposed model demonstrated validation results with an accuracy of 98.03%, wherein the precision, recall, and F1-score all surpassed 94%.
Visualization of simulation results
Figures 8 , 9 , 10 present a visualization of simulation results for the proposed model on the “HAM-10000” skin cancer dataset. Figure 8 presents the Confusion Matrix of the Proposed Model, Fig. 9 illustrates the Model Accuracy and Loss of the Proposed Model, and Fig. 10 shows an ROC Curve of the Proposed Model. Figures 11 , 12 13 present a visualization of simulation results for the MobileNet Model on the “HAM-10000” skin cancer dataset. Figure 11 presents the Confusion Matrix of the MobileNet Model, Fig. 12 illustrates the Model Accuracy and Loss of the MobileNet Model, and Fig. 13 shows an ROC Curve of the MobileNet Model.
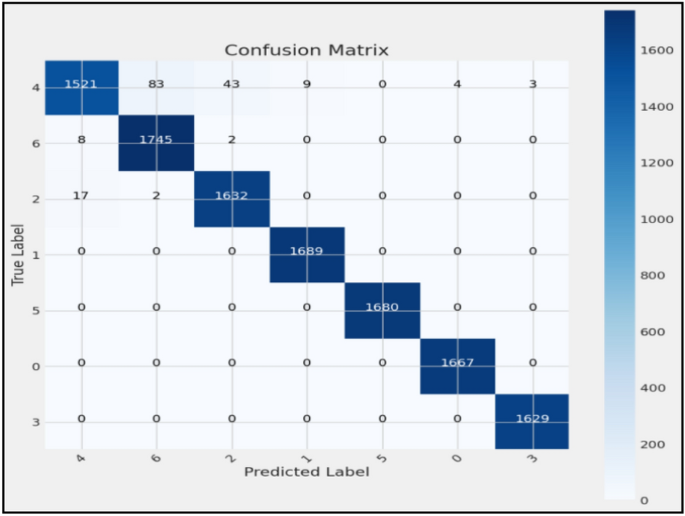
Confusion matrix of proposed model.
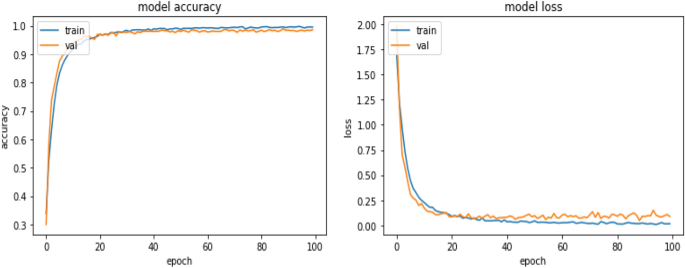
Model accuracy and loss of proposed model.
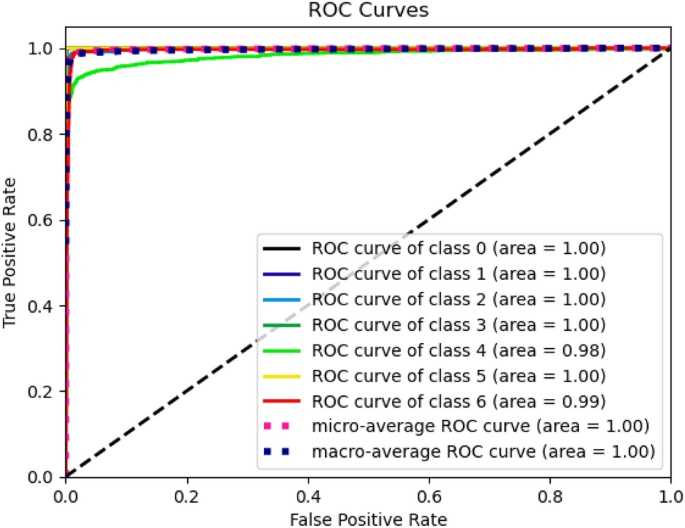
ROC curve of proposed model.
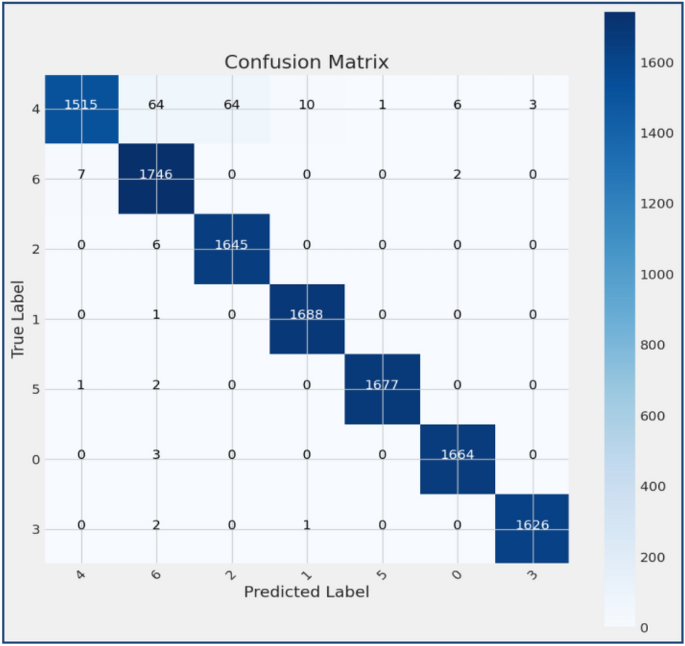
Confusion matrix of existing MobileNet model.
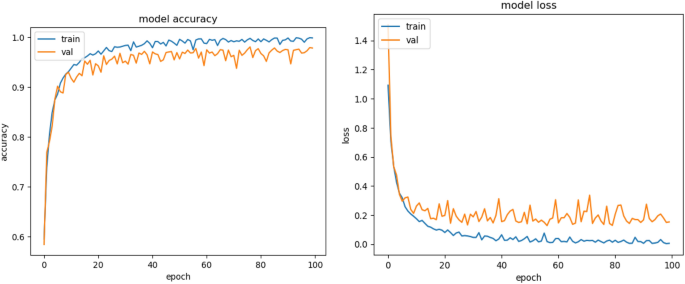
Model accuracy and loss of Existing MobileNet model.
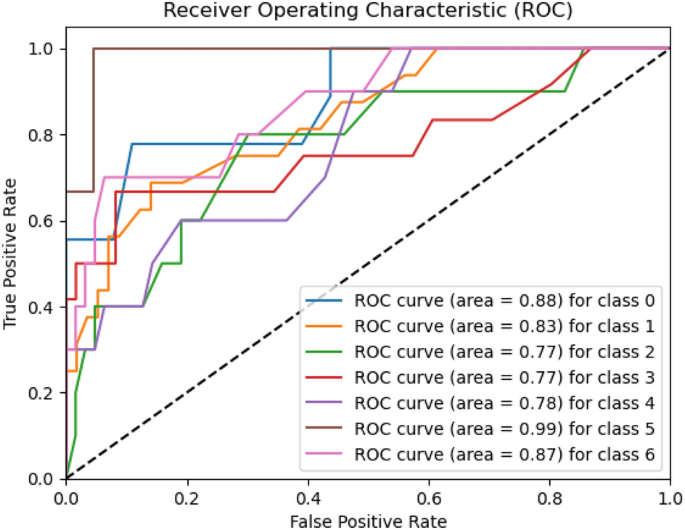
ROC curve of existing MobileNet model.
Figures 14 , 15 , 16 present a visualization of simulation results for the MobileNet-V2 Model on the “HAM-10000” skin cancer dataset. Figure 14 presents the Confusion Matrix of the MobileNet-V2 Model, Fig. 15 shows the Model Accuracy and Loss of the MobileNet-V2 Model, and Fig. 16 illustrates an ROC Curve of the MobileNet-V2 Model.
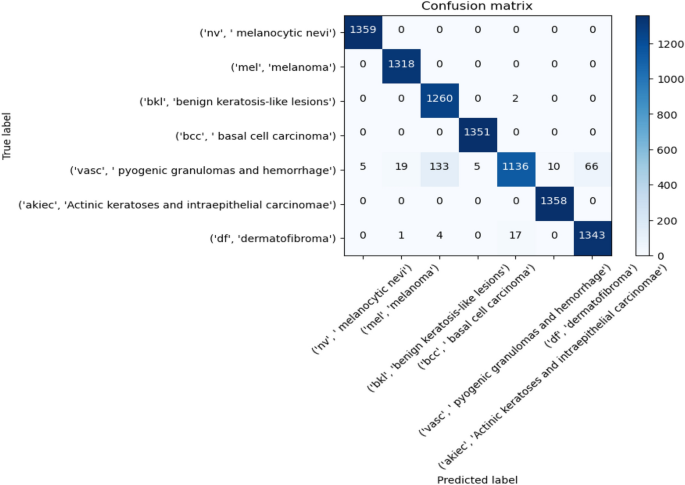
Confusion matrix existing MobileNet-V2 model.
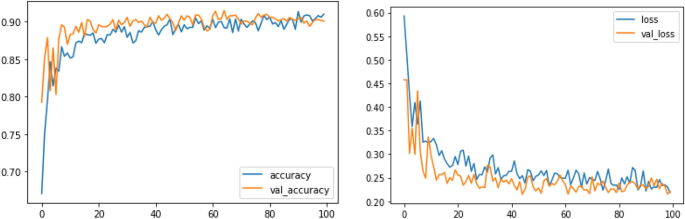
Model training, validation accuracy and loss existing MobileNet-V2 model.
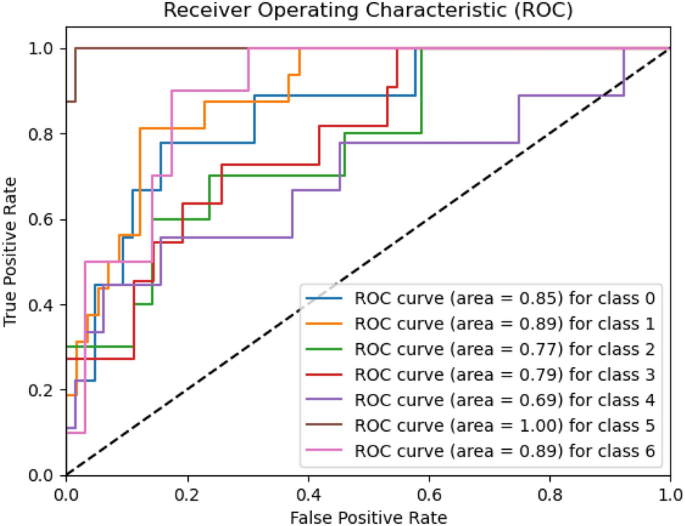
ROC curve of existing MobileNet-V2 model.
Figures 17 , 18 , 19 present a visualization of simulation results for the VGG-16 Model on the “HAM-10000” skin cancer dataset. Figure 17 presents the Confusion Matrix of the VGG-16 Model; Fig. 18 illustrates the Model Accuracy and Loss of the VGG-16; Fig. 19 shows an ROC Curve of the VGG-16 Model.
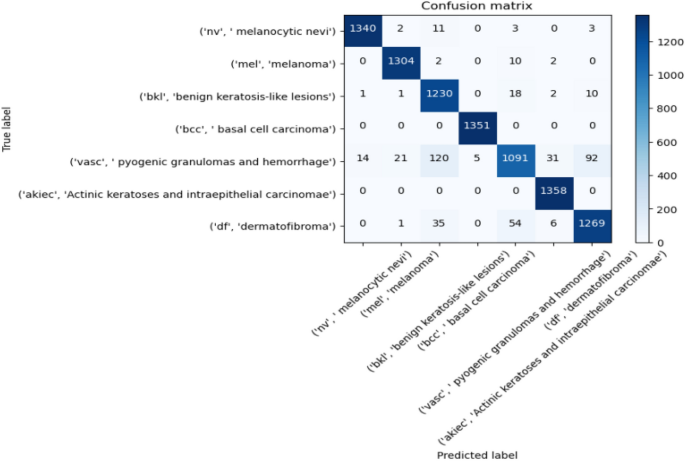
Confusion matrix of existing VGG-16 model.
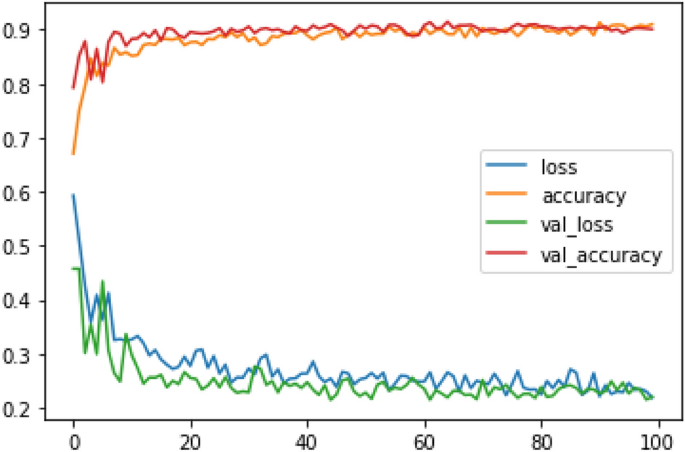
Model training, validation accuracy and loss existing VGG-16 model.
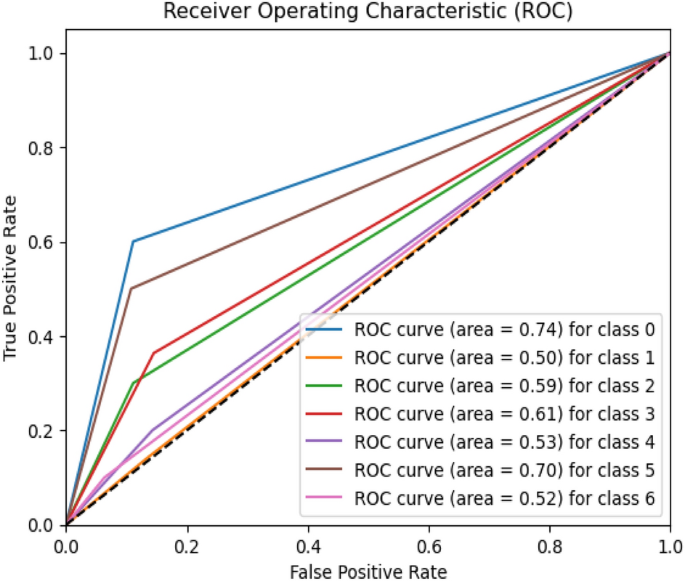
ROC curve of existing VGG-16 model.
Figures 20 , 21 , 22 present a visualization of simulation results for the VGG-19 Model on the “HAM-10000” skin cancer dataset. Figure 20 presents the Confusion Matrix of the VGG-19 Model; Fig. 21 illustrates the Model Accuracy and Loss of the VGG-19; Fig. 22 shows an ROC Curve of the VGG-19 Model. Figures 23 , 24 , 25 present a visualization of simulation results for the Resnet-152v2Model on the “HAM-10000” skin cancer dataset. Figure 23 presents the Confusion Matrix of the Resnet-152v2 Model; Fig. 24 illustrates the Model accuracy and loss of the Resnet-152v2; Fig. 25 shows an ROC Curve of the Resnet-152v2 Model. Based on the analysis of the results, it’s clearly proven that the proposed model has better simulation outcomes than existing models.
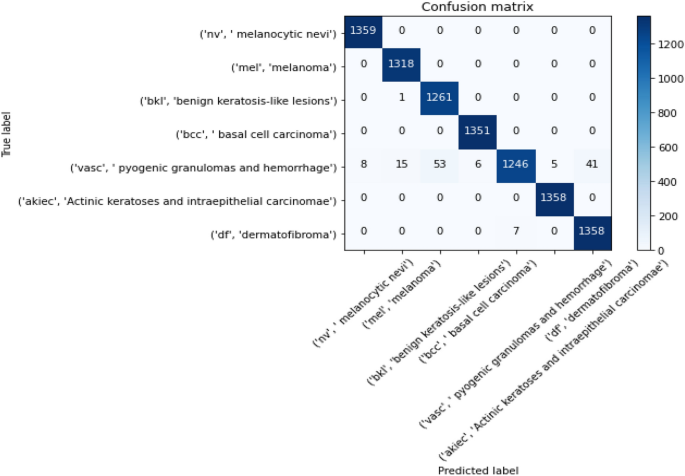
Confusion matrix of existing VGG-19 model.
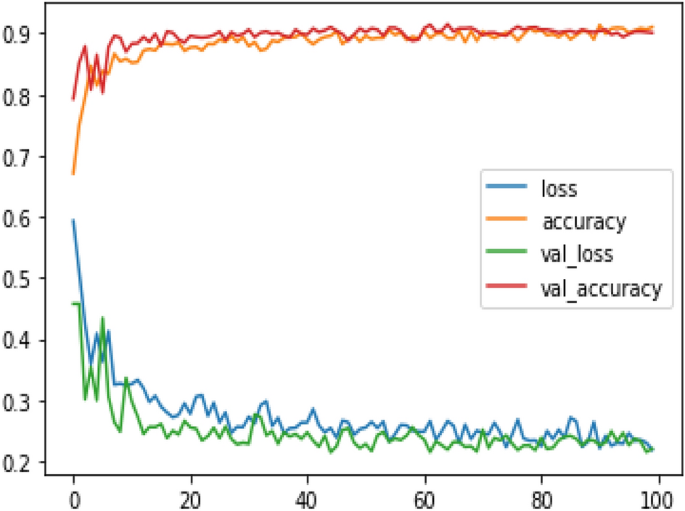
Model training accuracy and validation of existing VGG-19 model.
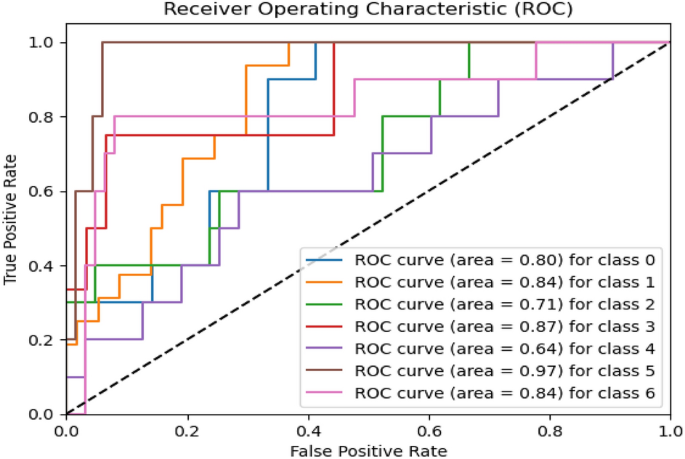
ROC curve of existing VGG-19 model.
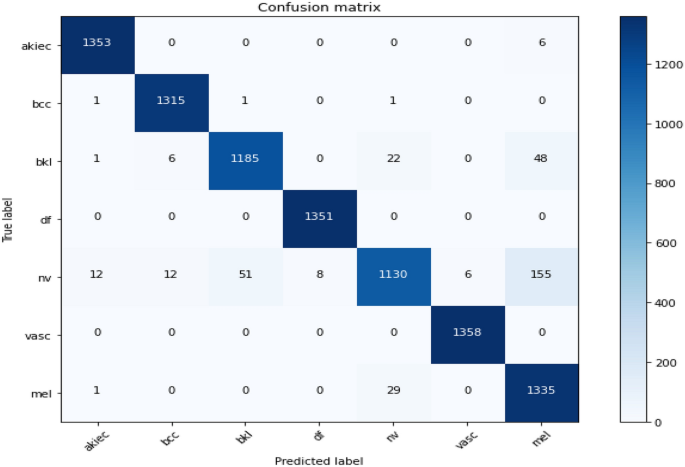
Confusion matrix of resnet-152v2.
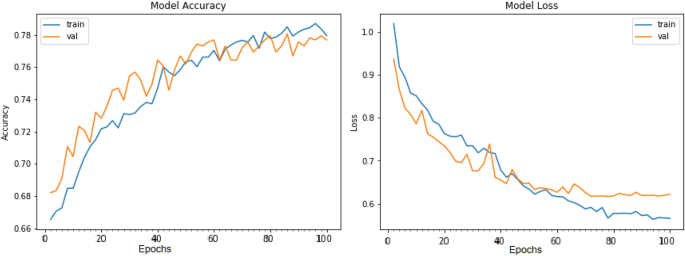
Training and validation accuracy, loss results of resnet-152v2.
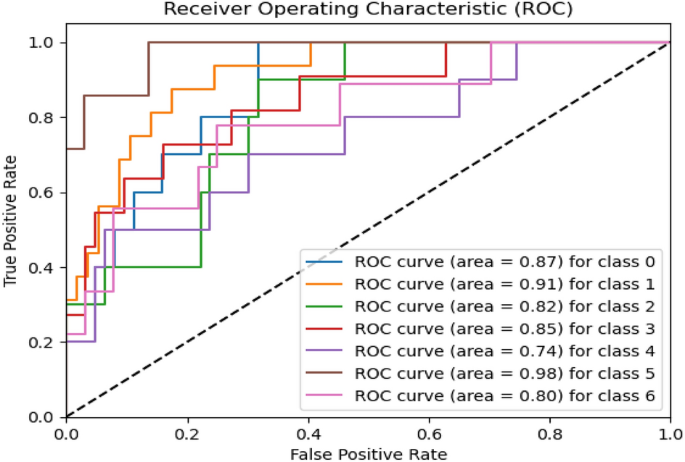
ROC-curve of resnet-152v2.
Results and discussion
The HAM-10000 Skin cancer dataset is utilized for the proposed model and several existing deep learning models, i.e., MobileNet, Resnet-152v2, VGG-16, MobileNet-V2, and VGG-19. Table 6 presents the experimental results of the testing dataset for 100 epochs for existing and proposed models. The combined utilization of the U-Net and MobileNet-V3 models yielded exceptional results in classifying skin cancer diseases. The model demonstrated a set of validation results with an accuracy of 98.86%, wherein the precision, recall, and F1-score all surpassed 95%. The model's capability to distinguish between cancerous and non-cancerous tumours is demonstrated by the ROC-AUC score of 98.45%, indicating excellent performance. The model consistently demonstrated a strong performance on the test set, achieving an accuracy rate of 98.45%.
The evaluation metrics, precision, accuracy, recall, ROC-AUC and F1-score, exhibited remarkable performance on the testing dataset, surpassing 95% for each metric. The ROC-AUC result of 98.45% reaffirms the model's robustness compared to the proposed model, existing MobileNet achieved, reaching 89% for each performance metric. Resnet-152V2 was achieved, surpassing 88% for each performance metric; VGG-16 was achieved, exceeding 89% for each performance metric. MobileNet-V2 achieved, exceeding 90% for each performance metric, and VGG-19 achieved, surpassing 90.50% for each performance metric. These results indicate that the proposed model achieved outstanding results over existing deep learning models.
Table 7 presents the experimental results of the validation dataset for 100 epochs for existing and proposed models. The proposed model demonstrated validation results with an accuracy of 98.03%, wherein the precision, recall, and F1-score all surpassed 94%. The model's capability to distinguish between cancerous and non-cancerous tumours is demonstrated by the ROC-AUC score of 97.09%, indicating excellent performance. The evaluation results show precision, accuracy, recall, ROC-AUC, and F1-score, exhibiting remarkable performance on the validation dataset, surpassing 94% for each metric. The ROC-AUC result of 97.09% reaffirms the model's robustness. As compared to the proposed model, the existing model, i.e., MobileNet achieved, surpassing 87% for each performance metric, Resnet-152V2 achieved, surpassing 87.02% for each performance metric VGG-16 achieved, reaching 88% for each performance metric, MobileNet-V2 achieved, exceeding 89% for each performance metric, and VGG-19 achieved, surpassing 88.50% for each performance metric. These results indicate that the proposed model achieved outstanding results over existing deep learning models.
Figures 8 , 9 , 10 present a visualization of simulation results for the proposed model, Figs. 11 , 12 , 13 present a visualization of simulation results for the MobileNet Model, Figs. 14 , 15 , 16 present a visualization of simulation results for the MobileNet-V2 Model, Figs. 17 , 18 , 19 shows a visualization of simulation results for the VGG-16 Model, Figs. 20 , 21 , 22 present a visualization of simulation results for the VGG-19 Model on the “HAM-10000” skin cancer dataset. Similar Figs. 23 , 24 , 25 present a visualization of simulation results for the Resnet-152v2Model on the “HAM-10000” skin cancer dataset. Based on the analysis of the results, it’s clearly proven that the proposed model has better simulation outcomes than existing models. Existing models such as MobileNet, VGG-16, MobileNet-V2, ResNet-152v2, and VGG-19 did not perform as well as the Hybrid U-Net, and Improved MobileNet-V3 model with hyperparameter optimization did on the "HAM-10000 Melanoma Skin Cancer dataset." There are several reasons for this.
Architecture of U-Net The U-Net architecture has gained recognition for its efficacy in the field of image segmentation, particularly in tasks related to skin cancer detection, where the objective is to delineate skin tumours from the tissue that surrounds them accurately. The U-Net's capacity to effectively capture intricate details and delineations contributes to enhanced feature extraction and superior classification outcomes.
Improved MobileNet-V3 MobileNet-V3 is a CNN architecture that has been specifically developed to prioritize both efficiency and accuracy. The enhanced iteration of the dataset takes into account alterations and refinements that render it better suited for the unique attributes of the skin malignancies dataset.
Feature extraction by Hybrid Model The hybrid models were created by integrating the advantageous features of both U-Net and MobileNet-V3. The provided system offers precise segmentation masks, whereas MobileNet-V3 efficiently extracts features from the segmented regions. The integration of these characteristics enhances the model's comprehension of images related to skin cancer.
Optimization of Hyperparameter The utilization of hyperparameter optimization techniques enables the refinement of the model's parameters and structural decisions, specifically tailored to the skin cancer dataset. In comparison with models with standard hyperparameters, this led to better performance and broad applicability.
Ablation analysis
We performed ablation research to determine the effect of critical parameters in the proposed skin cancer analysis model. To check the proposed model performance, we have conducted an ablation analysis with various conditions, which include (a) with and without Hybrid U-Net, (b) with and without MobileNet-V3, (c) with and without Augmentation, (d) with and without Batch Normalization, (e) with and without Hyperparameters. After methodically removing and testing with every single subsequent component eliminated, the following observations were found.
Various factors on the skin dataset were compared in the ablation analysis, as shown in Table 8 and Fig. 26 . For example, when it came to identifying complicated characteristics inside skin lesions, performance metrics dropped when the Hybrid U-Net was not used. There has been a significant gain in accuracy, suggesting that the Hybrid U-Net is vital in lowering the model's false positive rate and improving its capacity to detect cancerous tumours accurately. Improving MobileNet-V3 is crucial for efficient feature extraction since its absence significantly reduced total accuracy. There is strong evidence from the improved accuracy that Improved MobileNet-V3 helps cut down on false positives.
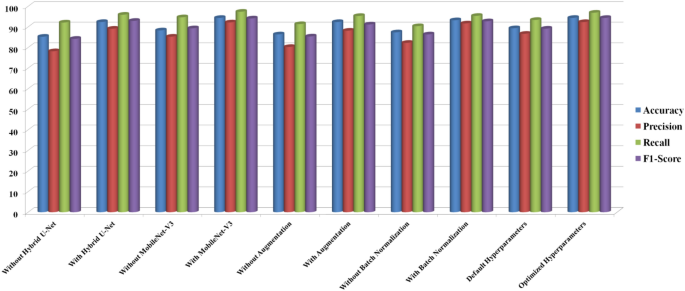
Comparative analysis of ablation analysis for different parameters on skin dataset.
Results showed that model resilience was reduced in the absence of data augmentation, highlighting the need for dataset augmentation for improved generalizability. Increases in accuracy and F1-score demonstrate how data augmentation enhances the model's capacity to categorize cancerous tumours accurately. A decrease in overall model performance and a slowdown in training convergence were the outcomes of removing batch normalization layers. Improving the model's capacity to distinguish between benign and malignant lesions and stabilizing the training process are both achieved using batch normalization, as seen by the significant gains in accuracy and F1-score. We found that the model's sensitivity to distinguishing between benign and malignant lesions is greatly affected by the ideal values of the hyperparameters. These enhanced precision and accuracy show how critical hyperparameter adjustment is for getting the most out of a model. All of the steps and parts of our skin cancer diagnostic methodology are vital, as these results show. In addition to shedding light on the model, the ablation research provides valuable information for making future upgrades and enhancements. Here are some practical ways to improve the model's diagnostic accuracy and reliability: observe the changes in performance measures.
Conclusion and future works
The proposed research integrates Hybrid U-Net and Improved MobileNet-V3 features to formulate a new hybrid model for skin cancer detection. Our model, showcasing superior diagnostic accuracy, emphasizes the pivotal roles of Hybrid U-Net and Improved MobileNet-V3 through a comprehensive assessment involving an ablation study and a comparison with state-of-the-art models. Beyond delivering a high-performing model, the study offers insightful directions for future research, contributing to the advancements in automated skin cancer detection. The observed enhancements, coupled with our competitive standing against current models, suggest a potentially significant therapeutic impact. According to the authors, this study introduces new possibilities for research and development in medical image analysis, promising faster and more accurate skin cancer diagnostics.
This research introduces an innovative skin cancer detection method incorporating U-Net and MobileNetV3 architecture, employing hyperparameter optimization strategies. By combining advanced deep learning techniques and meticulous parameter optimization, the result is a highly accurate and efficient system for diagnosing skin cancer. This work significantly improves skincare and medical services by providing a valuable tool for skin specialists and doctors to enhance the timely detection of skin malignancies and improve patient outcomes. The proposed model is compared with existing models, namely MobileNet, VGG-16, MobileNet-V2, Resnet-152v2, and VGG-19 on the "HAM-10000 Melanoma Skin Cancer dataset".
To fine-tune the model's hyperparameter, we employ sophisticated optimization methods such as the Bayesian optimization method using the pre-trained CNN architecture MobileNet-V3. Empirical findings demonstrate that the proposed optimized hybrid MobileNet-V3 model outperforms existing skin cancer detection and segmentation techniques, achieving high precision (97.84%), sensitivity (96.35%), accuracy (98.86%), and specificity (97.32%). The enhanced performance of this research can lead to timelier and more precise diagnoses, potentially contributing to life-saving outcomes and mitigating healthcare expenditures. The developed model holds significant potential in terms of clinical relevance, as suggested by its precision and recall scores, making it a valuable tool for dermatologists in the early detection of skin cancer. The elevated accuracy level presents a potential avenue for mitigating misdiagnoses and improving patient outcomes.
In the proposed model, computational demands during training and inference may arise as a consequence of integrating two complicated designs. This limitation should be taken into account, particularly in situations when resources are limited. Also, interpretability is a common issue for deep learning models like the proposed model. In clinical situations, the model's interpretability is limited because, despite its good performance, comprehending the precise elements underlying the classification judgments might be difficult.
In order to reduce computing complexity, future studies should concentrate on improving the model. To improve the practicality of deployment, it may be worthwhile to investigate model compression approaches or lightweight designs. Solving problems with interpretability might be crucial. Healthcare providers may gain confidence and knowledge of the model's decision-making process with the use of explainable AI approaches. Future research in the area of automated skin cancer detection will be guided by this balanced approach, which adds to the current conversation in the field. Future research should explore incorporating supplementary clinical data, developing interpretability methods tailored for medical professionals, and addressing the ethical implications associated with implementing artificial intelligence models for skin cancer diagnosis. Our commitment to innovation remains central as we aim to make a significant impact within dermatology. While the proposed model exhibits exemplary performance on the HAM-10000 dataset, it is crucial to recognize that real-world medical records may show unforeseen variations. Additional validation is necessary to extend the model's capabilities to datasets and situations in healthcare that have not been previously encountered.
Data availability
The data supporting the research results of the present research can be obtained by contacting the corresponding author.
Abbreviations
- Deep learning
International skin imaging collaboration
Adaptive moment optimization algorithm
Fully connected
Convolution neural network
Batch normalization
Support vector machine
Rectified linear unit
Ismail, W. N. & Alsalamah, H. A. Efficient Harris Hawk optimization (HHO)-based framework for accurate skin cancer prediction. Mathematics 11 (16), 3601 (2023).
Article Google Scholar
Mohakud, R. & Dash, R. A hybrid model for classification of skin cancer images after segmentation. Int. J. Image Graph. 31 , 2550022 (2023).
Ogundokun, R. O. et al. Enhancing skin cancer detection and classification in dermoscopic images through concatenated MobileNetV2 and xception models. Bioengineering 10 (8), 979 (2023).
Article PubMed PubMed Central Google Scholar
Nancy, V. A., Osvin, P. P., Arya, M. S. & Shamreen Ahamed, B. Comparative research and analysis on skin cancer detection using machine learning and deep learning algorithms. Multim. Tools Appl. 82 , 1–45 (2023).
Sharma, G., & Raman, C. An optimized predictive model based on deep neural network for detection of skin cancer and oral cancer. In 2023 2nd International Conference for Innovation in Technology (INOCON) , 1–6 (IEEE, 2023).
Behara, K., Bhero, E. & Agee, J. T. Skin lesion synthesis and classification using an improved DCGAN classifier. Diagnostics 13 , 2635 (2023).
Mampitiya, L. I., Rathnayake, N. & De Silva, S. Efficient and low-cost skin cancer detection system implementation with a comparative research between traditional and CNN-based models. J. Comput. Cogn. Eng. 2 (3), 226–235 (2023).
Google Scholar
Akilandasowmya, G., Nirmaladevi, G., Suganthi, S. U. & Aishwariya, A. Skin cancer diagnosis: Leveraging deep hidden features and ensemble classifiers for early detection and classification. Biomed. Signal Process. Control 88 , 105306 (2023).
Salih, O. & Duffy, K. J. Optimization convolutional neural network for automatic skin lesion diagnosis using a genetic algorithm. Appl. Sci. 13 (5), 3248 (2023).
Article CAS Google Scholar
Tabrizchi, H., Parvizpour, S. & Razmara, J. An improved VGG model for skin cancer detection. Neural Process. Lett. 55 (4), 3715–3732 (2023).
Balaha, H. M. & Hassan, A.E.-S. Skin cancer diagnosis based on deep transfer learning and sparrow search algorithm. Neural Comput. Appl. 35 (1), 815–853 (2023).
Anupama, C. S. S. et al. Sand cat swarm optimization with deep transfer learning for skin cancer classification. Comput. Syst. Sci. Eng. 47 , 2 (2023).
Shamsi, A. et al. A novel uncertainty-aware deep learning technique with an application on skin cancer diagnosis. Neural Comput. Appl. 35 (30), 22179–22188 (2023).
Adla, D. et al. A full-resolution convolutional network with a dynamic graph cut algorithm for skin cancer classification and detection. Healthcare Analytics 3 , 100154 (2023).
Rajeshwari, J. & Sughasiny, M. Skin cancer severity prediction model based on modified deep neural network with horse herd optimization. Optic. Memory Neural Netw. 31 (2), 206–222 (2022).
Shinde, R. K. et al. Squeeze-mnet: Precise skin cancer detection model for low computing IOT devices using transfer learning. Cancers 15 (1), 12 (2022).
Prasad, V., Emil Selvan, G. S. R. & Ramkumar, M. P. ADTBO: Aquila driving training-based optimization with deep learning for skin cancer detection. Imag. Sci. J. 4 , 1–19 (2023).
Mohakud, R. & Dash, R. Skin cancer image segmentation utilizing a novel EN-GWO based hyper-parameter optimized FCEDN. J. King Saud Univ. Comput. Inf. Sci. 34 (10), 9889–9904 (2022).
Shetty, B. et al. Skin lesion classification of dermoscopic images using machine learning and convolutional neural network. Sci. Rep. 12 (1), 18134 (2022).
Article ADS CAS PubMed PubMed Central Google Scholar
Barburiceanu, S., & R. Terebeș. Automatic detection of melanoma by deep learning models-based feature extraction and fine-tuning strategy. In IOP Conference Series: Materials Science and Engineering , Vol. 1254, 012035 (IOP Publishing, 2022).
Houssein, E. H., Emam, M. M. & Ali, A. A. An optimized deep learning architecture for breast cancer diagnosis based on improved marine predators algorithm. Neural Comput. Appl. 34 (20), 18015–18033 (2022).
Gouda, W., Sama, N. U., Al-Waakid, G., Humayun, M. & Jhanjhi, N. Z. Detection of skin cancer based on skin lesion images using deep learning. Healthcare 10 (7), 1183 (2022).
El Gannour, O. et al. Improving skin diseases prediction through data balancing via classes weighting and transfer learning. Bull. Electr. Eng. Inf. 13 (1), 628–637 (2024).
Arunkumar, M., Mohanarathinam, A. & Subramaniam, K. Detection of varicose vein disease using optimized kernel Boosted ResNet-Dropped long Short term Memory. Biomed. Signal Process. Control 87 , 105432 (2024).
Zhang, L. et al. A deep learning outline aimed at prompt skin cancer detection utilizing gated recurrent unit networks and improved orca predation algorithm. Biomed. Signal Process. Control 90 , 105858 (2024).
Sonia, R. et al. Segmenting and classifying skin lesions using a fruit fly optimization algorithm with a machine learning framework. Automatika 65 (1), 217–231 (2024).
Deshmukh, A. A. et al. Multi-class skin diseases classification using hybrid deep convolutional neural network. Int. J. Intell. Syst. Appl. Eng. 11 (10s), 11–22 (2023).
Sulthana, R., Chamola, V., Hussain, A., Hussain, Z. & Albalwy, F. A novel end-to-end deep convolutional neural network based skin lesion classification framework. Expert Syst. Appl. 246 , 123056 (2023).
Bibi, S. et al. MSRNet: Multi-class skin lesion recognition using additional residual block based fine-tuned deep models information fusion and best feature selection. Diagnostics 13 (19), 3063 (2023).
Awotunde, J. B. et al. An enhanced hyper-parameter optimization of a convolutional neural network model for leukemia cancer diagnosis in a smart healthcare system. Sensors 22 (24), 9689 (2022).
Article ADS PubMed PubMed Central Google Scholar
Abdar, M. et al. Uncertainty quantification in skin cancer classification using three-way decision-based Bayesian deep learning. Comput. Biol. Med. 135 , 104418 (2021).
Article PubMed Google Scholar
Kilicarslan, S., Celik, M. & Sahin, Ş. Hybrid models based on genetic algorithm and deep learning algorithms for nutritional Anemia disease classification. Biomed. Signal Process. Control 63 , 102231 (2021).
Sayed, G. I., Soliman, M. M. & Hassanien, A. E. A novel melanoma prediction model for imbalanced data using optimized SqueezeNet by bald eagle search optimization. Comput. Boil. Med. 136 , 104712 (2021).
Tan, T. Y., Zhang, L. & Lim, C. P. Intelligent skin cancer diagnosis using improved particle swarm optimization and deep learning models. Appl. Soft Comput. 84 , 105725 (2019).
ElGhany, S. A., Ibraheem, M. R., Alruwaili, M. & Elmogy, M. Diagnosis of various skin cancer lesions based on fine-tuned ResNet50 deep network. Comput. Mater. Cont. 68 , 1 (2021).
Pham, T. C., et al. Improving binary skin cancer classification based on best model selection method combined with optimizing full connected layers of Deep CNN. In 2020 International conference on multimedia analysis and pattern recognition (MAPR) , 1–6. (IEEE, 2020).
Gouda, W., Sama, N. U., Al-Waakid, G., Humayun, M. & Jhanjhi, N. Z. Detection of skin cancer based on skin lesion images using deep learning.". Healthcare 10 (7), 1183 (2022).
Nour, A., & Boubakeur, B. Convolutional neural network strategy for skin cancer lesions classifications and detections. In Proceedings of the 11th ACM International Conference on Bioinformatics, Computational Biology and Health Informatics , 1–9 (2020).
Tan, T. Y., Zhang, L., Neoh, S. C. & Lim, C. P. Intelligent skin cancer detection using enhanced particle swarm optimization. Knowl. Syst. 158 , 118–135 (2018).
Khan, M. A., Akram, T., Zhang, Y. D., Alhaisoni, M., Al Hejaili, A., Shaban, K. A., & Zayyan, M. H. SkinNet‐ENDO: Multi-class skin lesion recognition using deep neural network and Entropy‐Normal distribution optimization algorithm with ELM. Int. J. Imaging Syst. Technol. (2023).
Ajmal, M. et al. BF2SkNet: Best deep learning features fusion-assisted framework for multi-class skin lesion classification. Neural Comput. Appl. 35 (30), 22115–22131 (2023).
Dillshad, V., Khan, M. A., Nazir, M., Saidani, O., Alturki, N., & Kadry, S. D2LFS2Net: Multi‐class skin lesion diagnosis using deep learning and variance‐controlled Marine Predator optimization: An application for precision medicine. CAAI Trans. Intell. Technol. (2023).
Hussain, M. et al. SkinNet-INIO: Multi-class skin lesion localization and classification using fusion-assisted deep neural networks and improved nature-inspired optimization algorithm. Diagnostics 13 (18), 2869 (2023).
Download references
This research received no external funding.
Author information
Authors and affiliations.
Department of Computer Science and Engineering, Chandigarh University, Mohali, Punjab, 140413, India
Umesh Kumar Lilhore, Sarita Simaiya & Anupam Baliyan
Department of Computer Science and Engineering, Koneru Lakshmaiah Education Foundation, Greenfield, Vaddeswaram, Guntur, AP, India
Yogesh Kumar Sharma & K. B. V. Brahma Rao
School of Computing Science and Engineering, Galgotias University, Greater Noida, Uttar Pradesh, India
Kuldeep Singh Kaswan
Departmentt of Computer Science and Engineering, Shri Vishnu Engineering College for Women (A), Bhimavaram, India
V. V. R. Maheswara Rao
Arba Minch University, Arba Minch, Ethiopia
Anchit Bijalwan
Department of Computer Science, College of Computers and Information Technology, Taif University, P. O. Box 11099, 21944, Taif, Saudi Arabia
Roobaea Alroobaea
You can also search for this author in PubMed Google Scholar
Contributions
Conceptualization: Ideas and overarching research goals and aims by S.S. and A.B.; Methodology: Development and design of methodology by U.K.L. and V.V.R.M.R.; Software: Programming, software development by Y.K.S. and A.B.; Investigation and analysis: Conducting a research and investigation process and result analysis by; K.S.K.R. and K.B.V.B.R.; Supervision: Guidance and supervision for the research by U.K.L. Authors provide support for publication.
Corresponding author
Correspondence to Anchit Bijalwan .
Ethics declarations
Competing interests.
The authors declare no competing interests.
Additional information
Publisher's note.
Springer Nature remains neutral with regard to jurisdictional claims in published maps and institutional affiliations.
Rights and permissions
Open Access This article is licensed under a Creative Commons Attribution 4.0 International License, which permits use, sharing, adaptation, distribution and reproduction in any medium or format, as long as you give appropriate credit to the original author(s) and the source, provide a link to the Creative Commons licence, and indicate if changes were made. The images or other third party material in this article are included in the article's Creative Commons licence, unless indicated otherwise in a credit line to the material. If material is not included in the article's Creative Commons licence and your intended use is not permitted by statutory regulation or exceeds the permitted use, you will need to obtain permission directly from the copyright holder. To view a copy of this licence, visit http://creativecommons.org/licenses/by/4.0/ .
Reprints and permissions
About this article
Cite this article.
Kumar Lilhore, U., Simaiya, S., Sharma, Y.K. et al. A precise model for skin cancer diagnosis using hybrid U-Net and improved MobileNet-V3 with hyperparameters optimization. Sci Rep 14 , 4299 (2024). https://doi.org/10.1038/s41598-024-54212-8
Download citation
Received : 29 September 2023
Accepted : 09 February 2024
Published : 21 February 2024
DOI : https://doi.org/10.1038/s41598-024-54212-8
Share this article
Anyone you share the following link with will be able to read this content:
Sorry, a shareable link is not currently available for this article.
Provided by the Springer Nature SharedIt content-sharing initiative
- Health care
- Convolution Neural Network
By submitting a comment you agree to abide by our Terms and Community Guidelines . If you find something abusive or that does not comply with our terms or guidelines please flag it as inappropriate.
Quick links
- Explore articles by subject
- Guide to authors
- Editorial policies
Sign up for the Nature Briefing: Cancer newsletter — what matters in cancer research, free to your inbox weekly.

Skin Cancer Detection: A Review Using Deep Learning Techniques
Affiliations.
- 1 Government Associate College for Women Mari Sargodha, Sargodha 40100, Pakistan.
- 2 Department of Computer Science and Information Technology, University of Sargodha, Sargodha 40100, Pakistan.
- 3 Electrical Engineering Department, College of Engineering, Najran University Saudi Arabia, Najran 61441, Saudi Arabia.
- 4 Department of Computer Science, Wah Campus, Comsats University, Wah Cantt 47040, Pakistan.
- 5 Department of Computer Science, School of Systems and Technology, University of Management and Technology, Lahore 54782, Pakistan.
- 6 Department of Computer Science, COMSATS University Islamabad, Islamabad 440000, Pakistan.
- 7 Department of Internal Medicine, Faculty of Medicine, Najran University, Najran 61441, Saudi Arabia.
- 8 Department of Dermatology, Najran University Hospital, Najran 61441, Saudi Arabia.
- 9 Department of Internal Medicine, Faculty of Medicine, University of Tabuk, Tabuk 71491, Saudi Arabia.
- 10 Department of Medicinal Chemistry, Pharmacy School, Najran University, Najran 61441, Saudi Arabia.
- PMID: 34065430
- PMCID: PMC8160886
- DOI: 10.3390/ijerph18105479
Skin cancer is one of the most dangerous forms of cancer. Skin cancer is caused by un-repaired deoxyribonucleic acid (DNA) in skin cells, which generate genetic defects or mutations on the skin. Skin cancer tends to gradually spread over other body parts, so it is more curable in initial stages, which is why it is best detected at early stages. The increasing rate of skin cancer cases, high mortality rate, and expensive medical treatment require that its symptoms be diagnosed early. Considering the seriousness of these issues, researchers have developed various early detection techniques for skin cancer. Lesion parameters such as symmetry, color, size, shape, etc. are used to detect skin cancer and to distinguish benign skin cancer from melanoma. This paper presents a detailed systematic review of deep learning techniques for the early detection of skin cancer. Research papers published in well-reputed journals, relevant to the topic of skin cancer diagnosis, were analyzed. Research findings are presented in tools, graphs, tables, techniques, and frameworks for better understanding.
Keywords: deep learning; deep neural network (DNN); machine learning; melanoma; skin lesion; support vector machine (SVM).
Publication types
- Deep Learning
- Melanoma* / diagnosis
- Melanoma* / epidemiology
- Skin Neoplasms* / diagnosis
- Skin Neoplasms* / epidemiology
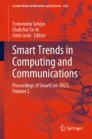
International Conference on Smart Trends for Information Technology and Computer Communications
SmartCom 2023: Smart Trends in Computing and Communications pp 521–531 Cite as
Skin Cancer Detection Using Deep Learning Approach
- Ankush 12 ,
- Samridhi Singh 12 ,
- Nagendra Pratap Singh 12 &
- Priyanka Rathee 12
- Conference paper
- First Online: 15 June 2023
264 Accesses
Part of the book series: Lecture Notes in Networks and Systems ((LNNS,volume 650))
Skin cancer is a severe health issue. Thus, the major concern of physicians is to investigate a precise clinical diagnosis. At present, some mechanisms are developed in the area of image processing with the help of algorithms and systems to detect and classify skin cancer. Computer-based technology provides a relaxed, inexpensive and quick diagnosis of skin cancer symptoms. Several techniques, non-invasive in nature, have been proposed to investigate the symptoms of skin cancer, whether they represent melanoma or non-melanoma. The general process applied in skin cancer detection is image acquisition, pre-processing, segmentation of the acquired pre-processed image, extraction of the required features and classification of disease skin cancer. After implementing the model we get an accuracy of 88.48% in the case of AlexNet and 90.41% in the case of VGG 16 respectively.
Download conference paper PDF
1 Introduction
Melanoma sometimes goes by the term of malignant melanoma. Melanocytes, or cells that create pigment, are the source of melanoma, a type of skin cancer. In individuals with low amounts of the skin pigment melanin, exposure to ultraviolet (UV) light is the main factor contributing to melanoma development. Cutaneous lesions are one of the most prevalent cancer types, and pandemics are expected to significantly increase their prevalence in the coming years. In addition to other factors like allergies or infections, exposure to the sun is the main cause of skin alterations, which lead to aberrant melanocyte proliferation and melanomas. Basal cell carcinoma, squamous cell carcinoma, Markel cell carcinoma, and melanoma are the four main kinds of skin cancer. This study allows us to recognize melanoma skin cancer. In this research paper, we’ve examined and reviewed many state of the art methods for the detection of skin cancer. In this study we will use a well known CNN model on our dataset. In this paper we take 5000 images dataset of benign & 4605 images dataset of malignant melanoma.
The main contributions of this paper are as follows:
We thoroughly reviewed the literature on the detection of cancer diseases.
A comparison of the performance between the VGG 16 and AlexNet deep learning models.
The methods produce results with a reduced error detection rate.
2 Related Work
Deep learning has emerged as the new standard because it is clear that it performs the majority of the work. The underlying reason behind this is that deep learning can automatically extract features, whereas handcrafted features must be extracted manually. As a result, in this section we provide an overview of the associations that the concept of deep learning encompasses.
Ahn et al. (2016) suggested that using failures in reconstruction caused by a sparse representation model was able to detect a new background as well as more accurately discriminate the lesion from the nearby areas [ 1 ] . This work also presented a Bayesian model to characterize the size and borders of the lesion in a superior way. The presented scheme was evaluated on two publicly available datasets containing 1100 dermoscopic images. It was also compared with other methodologies. The presented scheme showed more accuracy and robustness in segmenting lesions over other methods in results. This work also held a discussion on a universal expansion of new architecture as a saliency optimization approach for segmentation of lesions. After 2 years, Do et al. (2018) proposed an existent mobile healthcare strategy for melanoma detection by analyzing mobile images [ 2 ] . The salient features of the proposed model were: an effectual categorial segmentation approach apt for a platform of limited resources, a fresh feature set that proficiently captured color difference and bound from an image clicked with a smartphone, and a new instrument for choosing a close-knit group of most discriminatory set of attributes. The results depicted that the proposed solution was accurate in segmenting and classifying skin lesions in 184 camera images. In the same year, Singh et al. (2018) classified the retrieved characteristics of the lesions using a variety of approaches, including SVM, KNN, decision trees, and ensemble classifiers [ 3 ]. Here, they used an SVM classifier to get accuracy of 100.0%, however when using other classifiers, the accuracy was lower. Also, Díaz et al. (2019) presented a commodity embedded hardware-based device. This device used dynamic thermal imaging to apply an automated process for initial skin-cancer screening [ 4 ] . The process took advantage of segmenting images in the visible range and actual Using multimodal registration, the TRC (Temperature Recovery Curve) of dubious skin lesions is calculated. The tool applied two algorithms that estimated lesion lethality from the calculated TRCs. In this project, a Raspberry Pi 3 Model B+ board was used to build a model of the device and obtain a visible image of the wound. The statistical detection algorithmic approach applied on a dataset of 116 volunteer subjects obtained a specificity of 95% and sensitivity of 98%. Goel et al. (2019) designed fully automated ensemble deep learning schemes using two efficient segmentation approaches, namely DeepLab v3+, and Mask R-CNN (instance segmentation) to improve the accuracy of the results in various skin (Semantic Segmentation) lesion datasets consisting of noise expert observations [ 5 ] . This work used two datasets called ISIC-2017 test set and the PH2 to analyze the productivity of the combined network. The results validated that the formulated techniques provided the sensitivity up to 89.93% and specificity around 97.94% while segmenting the skin lesions. Mansutti et al. (2019) proposed an effective and affordable near-field investigation, intended for the diagnosis of skin cancer in early stages [ 6 ] . Furthermore, this work ensured anon-complex and low-cost fabrication operation by adopting substrate integrated waveguide (SIW) technology. This property was required to confirm the practicality and effectiveness of the presented framework as a mechanism for swift scanning numerous doubtful skin regions. The main objective of inspect designing was to perform its operation at approx. 40 GHz to identify tiny lumps of cancer in the skin, while stopping the fields from collaborating with the fundamental organisms' tissues. The probe had the potential to work on all skin types and areas of the body. The mechanical device that was demonstrated has a 0.55 mm detecting depth and a 0.20 mm lateral sensitivity. In the very next year, Song et al. (2020) suggested an E2E (End-to-End) multi-task DL (Deep Learning) model in order to analyze the skin lesion automatically [ 7 ]. This model was capable of detecting, classifying and segmenting the skin lesion. A three-fold joint training strategy was adopted for ensuring that the feature learning was effective, when the suggested model was trained. The findings on the ISBI 2016 challenge dataset for melanoma classification and the ISIC 2017 challenge dataset for melanoma segmentation demonstrated the superiority of the proposed model over the conventional approaches. Moreover, this model was utilized as an optimal computer-aided tool to diagnose melanoma. Also, Adegun et al. (2020) presented a new model for both segmenting and classifying skin lesions for the automatic diagnosis of skin cancer [ 8 ]. The primary phase exploited an encoder–decoder FCN (Fully Convolutional Network) with the encoder stage learning the coarse form to learn the characteristics of complex and non- uniform skin lesions and the decoder learning the details of the lesion boundaries. The next phase introduced a new Dense Net architecture based on FCN. This architecture included dense blocks, combined and coupled through the concatenation approach and a transition layer. More than 10000 datasets of freely accessible HAM10000 were used in this work to evaluate the presented framework providing accuracy, recall and AUC score of respectively 98, 98.5, and 99%. Again in the same year, Arab et al. (2020) presented a 77 GHz low-energy multitone continuous-wave radar system for skin cancer detection in biomedical imaging [ 9 ]. The implementation of sensor was dependent on an inexpensive MHMIC (Miniature Hybrid Microwave Integrated Circuit) architecture. A six-port homodyne quadrature down- converter acted as the receiver. This work designed and applied two cascade amplifier circuits to amplify the baseband voltage at the output of every power detector. In order to increase its adaptability for precisely detecting melanoma on the order of tens of microns, the proposed radar architecture has the capacity to identify the dielectric properties of tissues. Also, Ashraf et al. (2020) presented using a transfer learning strategy, a ROI (Region of Interest) inspired model that can identify and distinguish melanoma from nevus cancer [ 10 ]. This model extracted required images areas by applying an improved k-mean algorithm. These ROI centric strategies helped identifying discriminatory attributes as only images comprising melanoma cells were employed used for the model training. This work additionally used a transfer learning model based on ConvNet with data augmentation for the desired regions of images. The results demonstrated that the introduced system provided the accuracy up to 97.9% for DermIS and 97.4% for DermQuest for detecting skin cancer successfully. Wei et al. (2020) presented a fine-grained classification rule based on mild skin cancer detection architecture with feature difference [ 11 ]. The new framework consisted of two universal feature extraction units of the Lesion Classification Network. The recognition approach used in this work had the potential to take out more discriminatory lesion attributes. Additionally, a light semantic segmentation specimen of the lesion region of the dermos copy picture was constructed, in order to obtain edge-to-edge high-precision lesion region segmentation without complex image pre-processing exercises. The outcomes of analysis conducted on ISBI 2016 revealed the superiority of the investigated framework over others for detecting skin cancer. Kwasigroch et al. (2020) focused on implementing an architecture search mechanism so that the medical task of detecting the malignant melanoma was accomplished [ 12 ]. An appropriate network structure was discovered using the hill-climbing search strategy with network morphism operations for investigating the search space. These operations assisted in maximizing the network size through the earlier trained network. Such knowledge reutilization led to mitigating the computational cost. The experimental outcomes depicted the suggested mechanism capable of alleviating the computational cost. Albahali et al. (2020) proposed a strategy to detect and segment melanoma. This strategy yielded considerable enhancements from the accuracy perspective [ 13 ]. The first stage involved removing artifacts from dermoscopic images through the implementation of morphological processes, and provided sharpened image areas. Subsequently, to detect the affected area, this work employed properly tuned YOLOv4 object detector for melanoma diagnosis by discriminating between highly correlated diseased and unaffected areas. This work used two datasets called ISIC2018 and ISIC2016 for the evaluation of the presented scheme. YOLOv4 detector had the potential to detect many skin disorders of the same patient and manifold disorders of different patients. In the very next year, Pacheco et al. (2021) addressed the issue of merging pictures and metadata characteristics to classify skin cancer with deep learning [ 14 ]. The Metadata Processing Block (MetaBlock), a novel technique that employs metadata to help data classification by boosting the most crucial features that were retrieved from the images along the classification pipeline, was introduced in this work. The suggested strategy was contrasted with two other combination techniques, one of which used feature concatenation and the MetaNet. Results from two different datasets on skin lesions demonstrate that our method improves classification for every model evaluated and outperforms previous combination approaches in six out of ten instances. Also, Thurnhofer-Hemsi et al. (2021) proposed for the classification of skin lesions, an ensemble of improved convolutional neural networks paired with a test-time regularly spaced shifting technique [ 15 ]. The relocation vectors are shifted using a regular lattice in the potential shift zone, and the shifting method generates several repetitions of the test input image. Each ensemble member will then receive these modified versions of the training images. The results from each classifier are merged to produce the final product. In comparison to the well- known HAM10000 dataset, the experiment's results demonstrate a considerable improvement in accuracy and F-score. It is specifically shown that our approach, which combines clothes with test-time spaced evenly shifting, produces superior results than either of the two approaches used independently. Recently, Lan et al. (2022) proposed to classify dermoscopic images Because FixCaps uses a high-performance large-kernel at the bottom convolution layer rather than the more typical 99 kernel, it has a larger receptive field than CapsNets [ 16 ]. The loss of spatial information brought on by convolution and pooling was lessened by the use of the convolutional block attention module. To prevent model under fitting in the capsule layer, the group convolution technique was employed. When compared to many other methods, the network can significantly reduce the number of processes while increasing the accuracy of the detection. According to the experimental findings, FixCaps is more accurate at detecting skin cancer than IRv2-SA, which had a 96.49% accuracy rate on the HAM10000 dataset.
3 Methodology
The study will begin following a thorough examination of the existing skin cancer disease identification in human beings. We will train the CNN model (AlexNet/VGG16) on a dataset which are having cancerous and non-cancerous images acquired from Kaggle. Experiments will be conducted on the acquired data, and the performance will be evaluated and determined. The primary actions that must be taken are listed below.
Dataset distribution
Convolutional Neural Network (CNN)
The dataset obtained through Kaggle consists of 9,605 photos, which are further separated into 4,605 images of malignancy and 5,000 images of benignity. The Table 1 demonstrates how these subdivisions are again split into two datasets that are used to both train and test the models in the ratio 80:20 (Fig. 1 ).
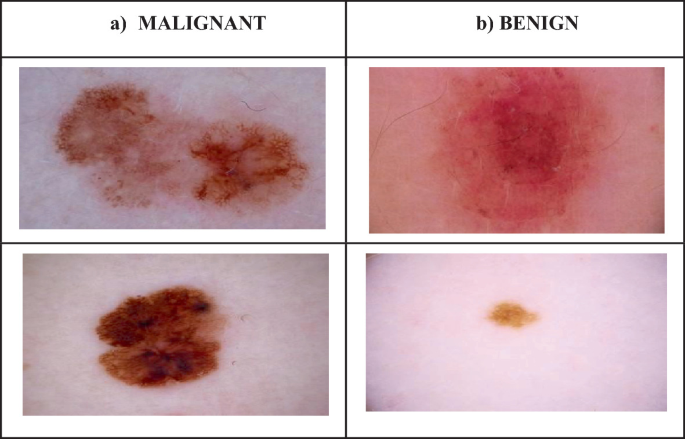
Images of a malignant cancer b benign cancer
One of the most often used deep learning approaches for image processing is convolutional neural networks (CNNs). Animal visual cortex served as the inspiration for CNNs. One of the first truly successful deep learning systems, they excel at both video and image processing. Decision-support systems for image categorization and object recognition have successfully applied CNNs with the help of contemporary GPU- accelerated computing techniques. Deep networks are efficient techniques for analyzing medical images, according to recent research. They therefore have a lot of potential for melanoma categorization. For this task, CNN ensemble approaches in particular have been highly effective (Fig. 2 ).
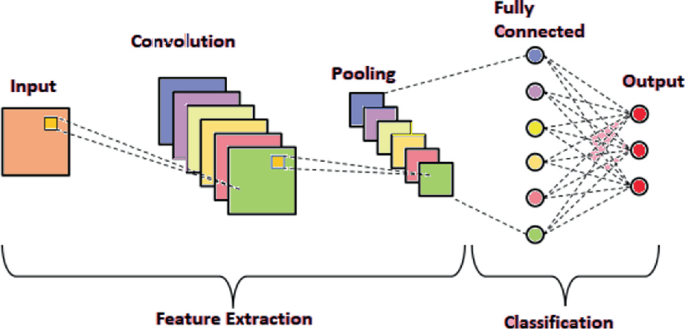
The architecture of deep CNN model
Convolution layer
The convolution operation is the core element of CNN. It handles the entirety of the network’s computation complexity. The kernel—a group of trainable parameters in the limited region of the perceptron are dual matrices that are combined in this phase to form a dot product. The kernel is bigger yet shorter in space than an image.
Pooling layer
By calculating overall statistics from the surrounding outputs, the pooling layer substitutes for the network's output at specific locations. This aids in shrinking the size of the representation's image, minimizing the amount of calculation and weights necessary. Every slice of the representation receives a separate application of the pooling technique.
Fully connected layer
Similar to a conventional CNN, every neuron in this layer is intimately connected to every other neuron in every layer before and after it. As a result, it can be calculated using the bias effect and conventional matrix multiplication. Between the input and output, the FC layer maps the expression.
One of the most popular deep learning architectures is VGG16, which Oxford University introduced. A convolutional neural network with 16 layers is called VGG-16. 13 are convolutional layers and three layers are fully connected (FC) layers. VGG16 has a total of 138 million parameters. All Convolutional Layers in VGG16 use a small (3x3) kernel (filter) with stride 1. Convolutional layer is always followed by maximum pooling layers. Three fixed-size channels of (224x224) pixels make the input for VGG16. The three FC layers in VGG16 have various depths. In a FC layer, the input is combined by a weight matrix and then given a bias vector (Fig. 3 ).
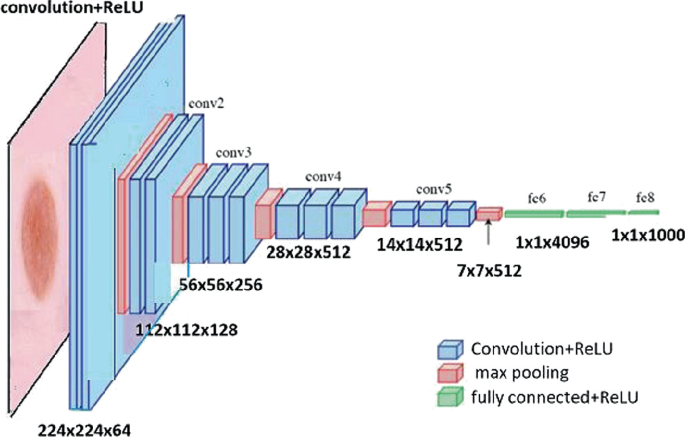
The architecture of VGG16
The soft-max layer, which is in charge of the probability specified for the input image, serves as the output layer. Pros of VGG16: It is easy to understand and also for explanation. Cons: Large model due to several weights parameters, each of which is close to 550 MB in size. High Time of Interpretation.
The Convolutional neural network (CNN) architecture known as AlexNet was established by Alex Krizhevsky, Ilya Sutskever, and Geoffrey Hinton, who worked as Krizhevsky’s Ph.D. advisor. One of the transfer learning models is AlexNet CNN. The proposed CNN achieves a high degree of accuracy, which is more effective than the accuracy attained by traditional neural network systems. There were eight layers in AlexNet. There were eight layers in AlexNet. The input to this model is the images of size (227 × 227 × 3). The first five layers were convolutional, some of them followed by layers that used maximum pooling, and the final three layers were fully connected. Pros of Alexnet: CNN views AlexNet as a turning point in image classification. The direct image input to the classification model is AlexNet’s special benefit. Cons of Alexnet: In comparison to newer models like VGGNet, GoogLENet, and ResNet, AlexNet is NOT deep enough. Shortly after that, the usage of big convolution filters (5 * 5) is discouraged (Fig. 4 ).
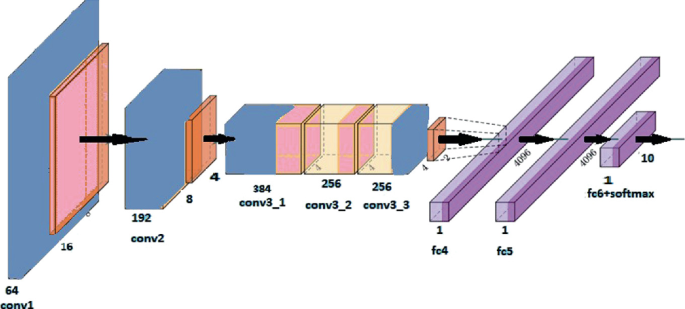
The architecture of Alexnet
4 Experiments
Different experiments are performed on different epochs. In the following sections, we list various results obtained in various environments.
During the experimentations we were able to get the highest accuracy at 20 epochs. The following are the graphs of the results obtained (Table 2 and Fig. 5 ).
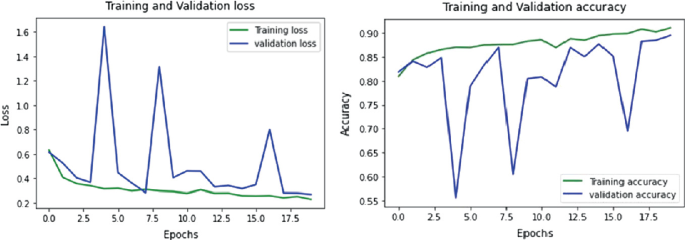
Graphs of AlexNet based on loss and accuracy respectively
See Table 3 and Fig. 6 .
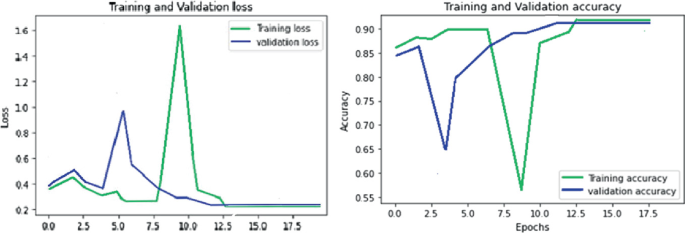
Graphs of VGG16 based on loss and accuracy respectively
5 Conclusion
Within the scope of this research work, we analyzed and compared two distinct types of deep learning models. We proceeded to train each model implementing on a number of different epochs. The results suggest which model has the best accuracy and the least amount of error at a certain epoch. For instance, during epoch 25 AlexNet had an accuracy of 88.48%, while VGG 16 had an accuracy of 90.41% at the same epoch.
Ahn E, Kim J, Bi L, Kumar A, Li C, Fulham M, Feng DD (2016) Saliency-based lesion segmentation via background detection in dermoscopic images. IEEE J Biomed Health Inf
Google Scholar
Do T-T, Hoang T, Pomponiu V, Zhou Y, Chen Z, Cheung N-M, Koh D, Tan A, Tan S-H (2018) Accessible melanoma detection using smartphones and mobile image analysis. IEEE Trans Multimedia
Singh NP, Shahi P, Yadav S, Singh N (2018) Skin cancer detection using various classifiers. In: 2018 5th IEEE Uttar Pradesh section international conference on electrical, electronics and computer engineering
Díaz S, Krohmer T, Moreira Á, Godoy SE, Figueroa M (2019) An instrument for accurate and non-invasive screening of skin cancer based on multimodal imaging. IEEE Access 7:176646–176657
Article Google Scholar
Goyal M, Oakley A, Bansal P, Dancey D, Yap MH (2019) Skin lesion segmentation in dermoscopic images with ensemble deep learning methods. IEEE Access
Mansutti G, Mobashsher AT, Bialkowski K, Mohammed B, Abbosh A (2019) Millimeter-wave substrate integrated waveguide probe for skin cancer detection. IEEE Trans Biomed Eng
Song L, Lin J, Jane Wang Z, Wang H (2020) An end-to-end multi-task deep learning framework for skin lesion analysis. IEEE J Biomed Health Inf
Adegun AA, Viriri S (2020) FCN-based DenseNet framework for automated detection and classification of skin lesions in dermoscopy images. IEEE Access 8:150377–150396
Arab H, Chioukh L, Ardakani MD, Dufour S, Tatu SO (2020) Early-stage detection of melanoma skin cancer using contactless millimeter-wave sensors. IEEE Sens J 20(13):7310–7317
Ashraf R et al (2020) Region-of-interest based transfer learning assisted framework for skin cancer detection. IEEE Access 8:147858–147871
Wei L, Ding K, Hu H (2020) Automatic skin cancer detection in dermoscopy images based on ensemble lightweight deep learning network. IEEE Access 8:99633–99647
Kwasigroch A, Grochowski M, Mikołajczyk A (2020) Neural architecture search for skin lesion classification. IEEE Access
Albahli S, Nida N, Irtaza A, Yousaf MH, Mahmood MT (2020) Melanoma lesion detection and segmentation using YOLOv4-DarkNet and active contour. IEEE Access
Pacheco AGC, Krohling RA (2021) An attention-based mechanism to combine images and metadata in deep learning models applied to skin cancer classification. IEEE J Biomed Health Inf 25(9):3554–3563
Thurnhofer-Hemsi K, López-Rubio E, Domínguez E, Elizondo DA (2021) Skin lesion classification by ensembles of deep convolutional networks and regularly spaced shifting. IEEE Access 9:112193–112205
Lan Z, Cai S, He X, Wen X (2022) FixCaps: an improved capsules network for diagnosis of skin cancer. IEEE Access 10:76261–76267
Download references
Author information
Authors and affiliations.
Department of Computer Science and Engineering, National Institute of Technology, Hamirpur, HP, India
Ankush, Samridhi Singh, Nagendra Pratap Singh & Priyanka Rathee
You can also search for this author in PubMed Google Scholar
Corresponding author
Correspondence to Ankush .
Editor information
Editors and affiliations.
University of the Ryukyus, Nishihara, Japan
Tomonobu Senjyu
Khon Kaen University, Khon Kaen, Thailand
Chakchai So-In
Global Knowledge Research Foundation, Ahmedabad, India
Rights and permissions
Reprints and permissions
Copyright information
© 2023 The Author(s), under exclusive license to Springer Nature Singapore Pte Ltd.
About this paper
Cite this paper.
Ankush, Singh, S., Singh, N.P., Rathee, P. (2023). Skin Cancer Detection Using Deep Learning Approach. In: Senjyu, T., So-In, C., Joshi, A. (eds) Smart Trends in Computing and Communications. SmartCom 2023. Lecture Notes in Networks and Systems, vol 650. Springer, Singapore. https://doi.org/10.1007/978-981-99-0838-7_45
Download citation
DOI : https://doi.org/10.1007/978-981-99-0838-7_45
Published : 15 June 2023
Publisher Name : Springer, Singapore
Print ISBN : 978-981-99-0837-0
Online ISBN : 978-981-99-0838-7
eBook Packages : Intelligent Technologies and Robotics Intelligent Technologies and Robotics (R0)
Share this paper
Anyone you share the following link with will be able to read this content:
Sorry, a shareable link is not currently available for this article.
Provided by the Springer Nature SharedIt content-sharing initiative
- Publish with us
Policies and ethics
- Find a journal
- Track your research
Skin Cancer Detection using Convolutional Neural Network
Ieee account.
- Change Username/Password
- Update Address
Purchase Details
- Payment Options
- Order History
- View Purchased Documents
Profile Information
- Communications Preferences
- Profession and Education
- Technical Interests
- US & Canada: +1 800 678 4333
- Worldwide: +1 732 981 0060
- Contact & Support
- About IEEE Xplore
- Accessibility
- Terms of Use
- Nondiscrimination Policy
- Privacy & Opting Out of Cookies
A not-for-profit organization, IEEE is the world's largest technical professional organization dedicated to advancing technology for the benefit of humanity. © Copyright 2024 IEEE - All rights reserved. Use of this web site signifies your agreement to the terms and conditions.
- See us on facebook
- See us on twitter
- See us on youtube
- See us on linkedin
- See us on instagram
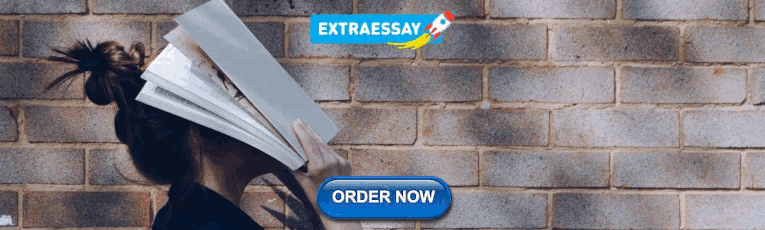
AI improves accuracy of skin cancer diagnoses in Stanford Medicine-led study
Artificial intelligence algorithms powered by deep learning improve skin cancer diagnostic accuracy for doctors, nurse practitioners and medical students in a study led by the Stanford Center for Digital Health.
April 11, 2024 - By Krista Conger
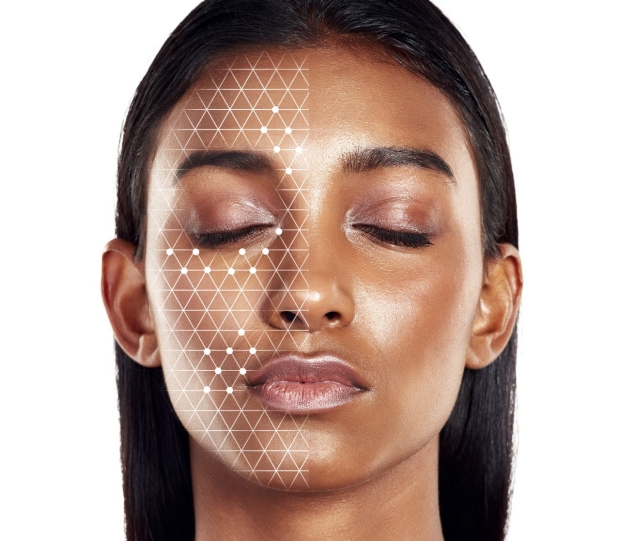
Artificial intelligence helped clinicians diagnose skin cancer more accurately, a Stanford Medicine-led study found. Chanelle Malambo/peopleimages.com - stock.adobe.com
A new study led by researchers at Stanford Medicine finds that computer algorithms powered by artificial intelligence based on deep learning can help health care practitioners to diagnose skin cancers more accurately. Even dermatologists benefit from AI guidance, although their improvement is less than that seen for non-dermatologists.
“This is a clear demonstration of how AI can be used in collaboration with a physician to improve patient care,” said professor of dermatology and of epidemiology Eleni Linos , MD. Linos leads the Stanford Center for Digital Health , which was launched to tackle some of the most pressing research questions at the intersection of technology and health by promoting collaboration between engineering, computer science, medicine and the humanities.
Linos, associate dean of research and the Ben Davenport and Lucy Zhang Professor in Medicine, is the senior author of the study , which was published on April 9 in npj Digital Medicine . Postdoctoral scholar Jiyeong Kim , PhD, and visiting researcher Isabelle Krakowski, MD, are the lead authors of the research.
“Previous studies have focused on how AI performs when compared with physicians,” Kim said. “Our study compared physicians working without AI assistance with physicians using AI when diagnosing skin cancers.”
AI algorithms are increasingly used in clinical settings, including dermatology. They are created by feeding a computer hundreds of thousands or even millions of images of skin conditions labeled with information such as diagnosis and patient outcome. Through a process called deep learning, the computer eventually learns to recognize telltale patterns in the images that correlate with specific skin diseases including cancers. Once trained, an algorithm written by the computer can be used to suggest possible diagnoses based on an image of a patient’s skin that it has not been exposed to.
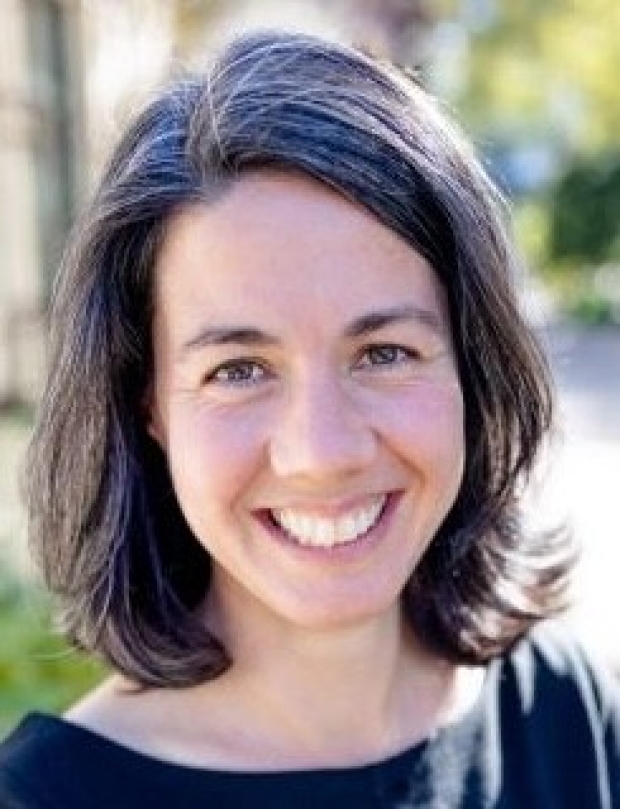
Eleni Linos
These diagnostic algorithms aren’t used alone, however. They are overseen by clinicians who also assess the patient, come to their own conclusions about a patient’s diagnosis and choose whether to accept the algorithm’s suggestion.
An accuracy boost
Kim and Linos’ team reviewed 12 studies detailing more than 67,000 evaluations of potential skin cancers by a variety of practitioners with and without AI assistance. They found that, overall, health care practitioners working without aid from artificial intelligence were able to accurately diagnose about 75% of people with skin cancer — a statistical measurement known as sensitivity. Conversely, the workers correctly diagnosed about 81.5% of people with cancer-like skin conditions but who did not have cancer — a companion measurement known as specificity.
Health care practitiones who used AI to guide their diagnoses did better. Their diagnoses were about 81.1% sensitive and 86.1% specific. The improvement may seem small, but the differences are critical for people told they don’t have cancer, but do, or for those who do have cancer but are told they are healthy.
When the researchers split the health care practitioners by specialty or level of training, they saw that medical students, nurse practitioners and primary care doctors benefited the most from AI guidance — improving on average about 13 points in sensitivity and 11 points in specificity. Dermatologists and dermatology residents performed better overall, but the sensitivity and specificity of their diagnoses also improved with AI.
“I was surprised to see everyone’s accuracy improve with AI assistance, regardless of their level of training,” Linos said. “This makes me very optimistic about the use of AI in clinical care. Soon our patients will not just be accepting, but expecting, that we use AI assistance to provide them with the best possible care.”
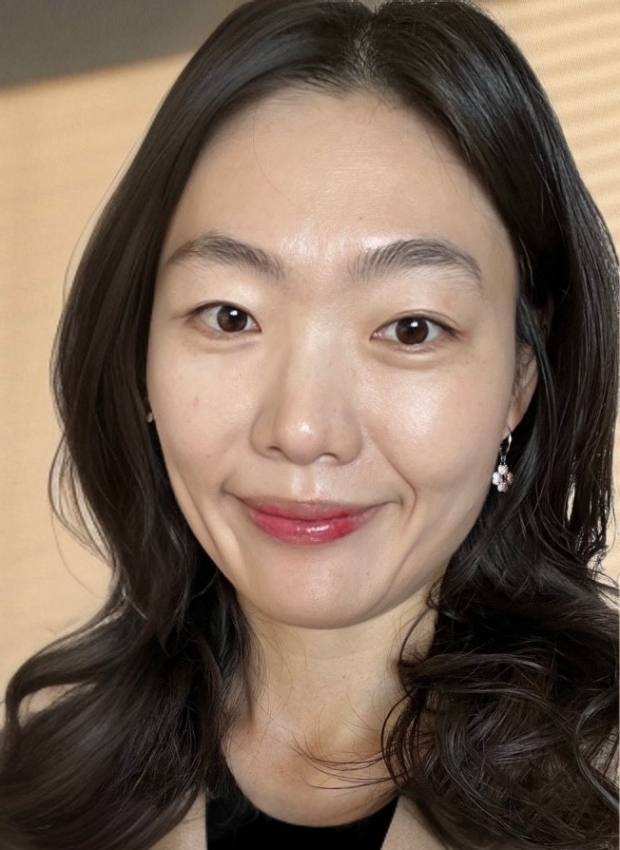
Jiyeong Kim
Researchers at the Stanford Center for Digital Health, including Kim, are interested in learning more about the promise of and barriers to integrating AI-based tools into health care. In particular, they are planning to investigate how the perceptions and attitudes of physicians and patients to AI will influence its implementation.
“We want to better understand how humans interact with and use AI to make clinical decisions,” Kim said.
Previous studies have indicated that a clinician’s degree of confidence in their own clinical decision, the degree of confidence of the AI, and whether the clinician and the AI agree on the diagnosis all influence whether the clinician incorporates the algorithm’s advice when making clinical decisions for a patient.
Medical specialties like dermatology and radiology, which rely heavily on images — visual inspection, pictures, X-rays, MRIs and CT scans, among others — for diagnoses are low-hanging fruit for computers that can pick out levels of detail beyond what a human eye (or brain) can reasonably process. But even other more symptom-based specialties, or prediction modeling, are likely to benefit from AI intervention, Linos and Kim feel. And it’s not just patients who stand to benefit.
“If this technology can simultaneously improve a doctor’s diagnostic accuracy and save them time, it’s really a win-win. In addition to helping patients, it could help reduce physician burnout and improve the human interpersonal relationships between doctors and their patients,” Linos said. “I have no doubt that AI assistance will eventually be used in all medical specialties. The key question is how we make sure it is used in a way that helps all patients regardless of their background and simultaneously supports physician well-being.”
Researchers from the Karolinska Institute, the Karolinska University Hospital and the University of Nicosia contributed to the research.
The study was funded by the National Institutes of Health (grants K24AR075060 and R01AR082109), Radiumhemmet Research, the Swedish Cancer Society and the Swedish Research Council.
For more news about responsible AI in health and medicine, sign up for the RAISE Health newsletter.
Register for the RAISE Health Symposium on May 14.
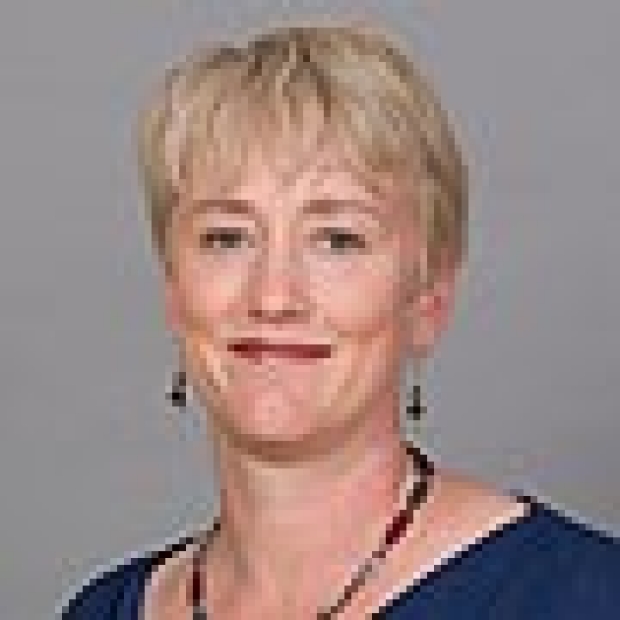
About Stanford Medicine
Stanford Medicine is an integrated academic health system comprising the Stanford School of Medicine and adult and pediatric health care delivery systems. Together, they harness the full potential of biomedicine through collaborative research, education and clinical care for patients. For more information, please visit med.stanford.edu .
Artificial intelligence
Exploring ways AI is applied to health care
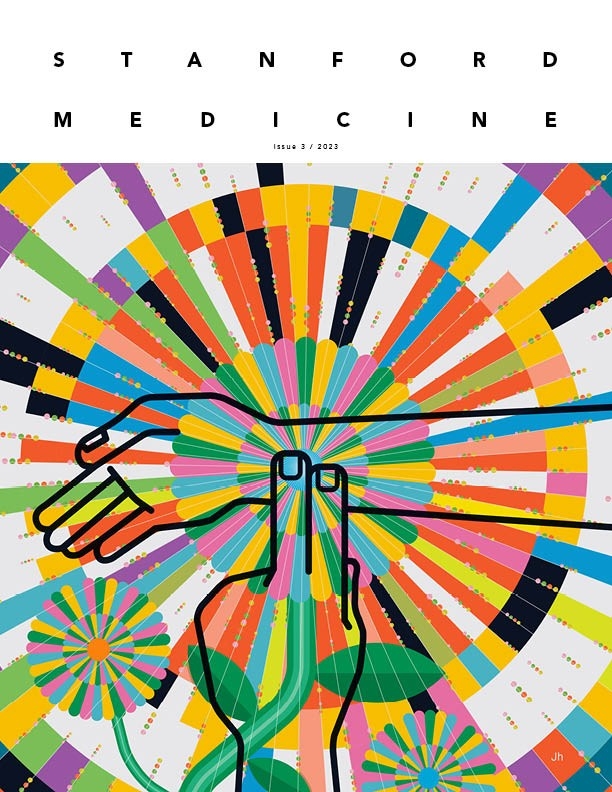

An official website of the United States government
The .gov means it’s official. Federal government websites often end in .gov or .mil. Before sharing sensitive information, make sure you’re on a federal government site.
The site is secure. The https:// ensures that you are connecting to the official website and that any information you provide is encrypted and transmitted securely.
- Publications
- Account settings
Preview improvements coming to the PMC website in October 2024. Learn More or Try it out now .
- Advanced Search
- Journal List
- Cancers (Basel)

AI-Powered Diagnosis of Skin Cancer: A Contemporary Review, Open Challenges and Future Research Directions
Navneet melarkode.
1 School of Computer Science and Engineering, Vellore Institute of Technology, Vellore 632014, India
Kathiravan Srinivasan
Saeed mian qaisar.
2 Electrical and Computer Engineering Department, Effat University, Jeddah 22332, Saudi Arabia
3 LINEACT CESI, 69100 Lyon, France
Pawel Plawiak
4 Department of Computer Science, Faculty of Computer Science and Telecommunications, Cracow University of Technology, Warszawska 24, 31-155 Krakow, Poland
5 Institute of Theoretical and Applied Informatics, Polish Academy of Sciences, Bałtycka 5, 44-100 Gliwice, Poland
Simple Summary
The proposed research aims to provide a deep insight into the deep learning and machine learning techniques used for diagnosing skin cancer. While maintaining a healthy balance between both Machine Learning as well as Deep Learning, the study also discusses open challenges and future directions in this field. The research includes a comparison on widely used datasets and prevalent review papers discussing skin cancer diagnosis using Artificial Intelligence. The authors of this study aim to set this review as a benchmark for further studies in the field of skin cancer diagnosis by also including limitations and benefits of historical approaches.
Skin cancer continues to remain one of the major healthcare issues across the globe. If diagnosed early, skin cancer can be treated successfully. While early diagnosis is paramount for an effective cure for cancer, the current process requires the involvement of skin cancer specialists, which makes it an expensive procedure and not easily available and affordable in developing countries. This dearth of skin cancer specialists has given rise to the need to develop automated diagnosis systems. In this context, Artificial Intelligence (AI)-based methods have been proposed. These systems can assist in the early detection of skin cancer and can consequently lower its morbidity, and, in turn, alleviate the mortality rate associated with it. Machine learning and deep learning are branches of AI that deal with statistical modeling and inference, which progressively learn from data fed into them to predict desired objectives and characteristics. This survey focuses on Machine Learning and Deep Learning techniques deployed in the field of skin cancer diagnosis, while maintaining a balance between both techniques. A comparison is made to widely used datasets and prevalent review papers, discussing automated skin cancer diagnosis. The study also discusses the insights and lessons yielded by the prior works. The survey culminates with future direction and scope, which will subsequently help in addressing the challenges faced within automated skin cancer diagnosis.
1. Introduction
Skin cancer is the abnormal growth of skin cells. The cancerous growth may affect both the layers—dermis and epidermis, but this review is concerned primarily with epidermal skin cancer; the two types of skin cancers that can arise from the epidermis are carcinomas and melanomas, depending on their cell type—keratinocytes or melanocytes, respectively [ 1 , 2 , 3 , 4 , 5 , 6 , 7 , 8 , 9 , 10 , 11 , 12 , 13 , 14 , 15 , 16 , 17 , 18 , 19 , 20 , 21 , 22 , 23 , 24 , 25 , 26 , 27 , 28 , 29 , 30 , 31 , 32 , 33 , 34 , 35 , 36 , 37 , 38 , 39 , 40 , 41 , 42 , 43 , 44 , 45 , 46 , 47 , 48 , 49 , 50 , 51 , 52 , 53 , 54 , 55 , 56 , 57 , 58 , 59 , 60 , 61 , 62 , 63 , 64 , 65 , 66 , 67 , 68 , 69 , 70 , 71 , 72 , 73 , 74 , 75 ]. It is a challenge to estimate the incidence of skin cancer due to various reasons, such as the multiple sub-types of skin cancer [ 76 , 77 , 78 , 79 , 80 , 81 , 82 , 83 , 84 , 85 , 86 , 87 , 88 , 89 , 90 , 91 , 92 , 93 , 94 , 95 , 96 , 97 , 98 , 99 ]. This poses as a problem while collating data, as non-melanoma is often not tracked by registries or are left incomplete because most cases are treated via surgery. As of 2020, the World Cancer Research Fund International reported a total of 300,000 cases of melanoma in skin, and a total of 1,198,073 cases of non-melanoma skin cancer [ 100 , 101 , 102 , 103 , 104 , 105 , 106 , 107 , 108 , 109 , 110 , 111 , 112 , 113 , 114 , 115 , 116 , 117 , 118 , 119 , 120 , 121 , 122 , 123 , 124 , 125 , 126 , 127 , 128 , 129 , 130 , 131 ]. The reasons for the occurrence of skin cancer cannot be singled out, but they include and are not limited to exposure to ultraviolet rays, family history, or a poor immune system [ 126 ]. The affected spot on the skin is called a lesion, which can be further segregated into multiple categories depending on its origin [ 1 ]. A comparison between different lesion types is usually accompanied by the presence or the absence of certain dermoscopic features.
There are three stages associated with an automated dermoscopy image analysis system, namely pre-processing, image segmentation, and feature extraction [ 2 , 4 ]. Segmentation plays a vital role, as the succeeding steps are dependent on this stage’s output. Segmentation can be carried out in a supervised manner by considering parameters such as shapes, sizes, and colors, coupled with skin texture and type. Melanoma development that takes place horizontally or radially along the epidermis is called “single cancer melanoma”, which carries critical importance in the early diagnosis of skin cancer [ 3 ]. Dermoscopy is a non-invasive diagnostic method which allows for a closer examination of the pigmented skin lesion. It is performed with the help of an instrument called a dermatoscope. The procedure of dermoscopy allows for a visualization of the skin structure in the epidermis that would not otherwise be possible to the naked eye. Studies [ 127 ] suggest that a growing number of practitioners are incorporating dermoscopy into their daily practices. Dermoscopy can be categorized into three modes—polarized contact, polarized non-contact, and nonpolarized contact (unpolarized dermoscopy). Polarized and nonpolarized dermoscopy are complementary, and utilizing both to acquire clinical images increases the diagnostic accuracy [ 128 ]. These images can then be processed with the help of AI methods to assist in the diagnosis of skin cancer [ 132 , 133 , 134 ].
Even though the mortality rate of skin cancer is significantly high, early detection helps to bolster the survival rate to over 95% [ 5 ]. Deep learning models are generalizations of multi-layer perceptron models and are widely used due to their high accuracy in visual imaging tasks. There are two major promising paths for skin cancer detection in this research. The first is employing machine learning techniques and strategies to assist in the detection of skin lesions, and classifying them accordingly. The second, as this article discusses, is deep learning frameworks and model-based approaches being implemented in the recent advancements concerning skin cancer diagnosis. Table A1 in Appendix A contains a list of abbreviations used in this review, as well as their definitions.
1.1. Contribution of this Survey
We provide a comprehensive study of the various machine learning and deep learning models used for skin cancer diagnosis. Brief explanations of several machine learning and deep learning methodologies are included.
- This survey comprehensively discusses the application of various machine learning and deep learning methods in the implementation of skin cancer diagnosis.
- There is a discussion of new techniques in skin lesion detection such as deep belief networks and extreme learning machines, along with the traditional Computational Intelligence techniques such as random forests, recurrent neural networks, and k-nearest neighbors, etc.
- There is a designated tabular summary of works on the deep learning and machine learning techniques used for skin cancer diagnosis and detection. The tabulated summary also includes key contributions and limitations for the same.
- There is a classification of various types of skin cancer based on tumor characteristics that have been elucidated for a deeper understanding of the problem statement.
- The study also describes various open challenges present and future research directions for further improvements in the field of skin cancer diagnosis.
Table 1 presents a comparison between the current review and the previous review articles of machine-learning-based and deep-learning-based techniques in skin cancer diagnosis. The depth of the discussion in Table 1 has been used as a criterion for comparing different review articles. A high or H depth of the discussion indicates that the article contains a dedicated session for the said topic. A moderate or M depth of the discussion denotes that the review article has a subsection or a paragraph corresponding to the topic. A low or L depth of the discussion implies that the article has mentioned the topic, but not explained it comprehensively. A not discussed or N depth of the discussion indicates that the topic has not been covered in the article.
Comparison of the current review with the previous reviews in AI-powered skin cancer diagnosis.
Depth of discussion: L—low, M—moderate, H—high, N—not discussed.
1.2. Survey Methodology
1.2.1. search strategy and literature sources.
Repositories and databases such as IEEE, ScienceDirect, and PubMed, etc., were used to find relevant research studies and articles. The relevancy was determined based on the paper’s context (the central theme of the paper being the diagnosis of skin cancer based on AI/ML/DL models), the research paper’s title, abstract screening, keyword matching, and the conclusion of the study. The keywords employed were cancer diagnosis, skin cancer, deep learning, machine learning, skin lesion, melanoma cancer, and cancer detection, etc. A total of 1057 non-duplicate articles were found initially. Table 2 includes the search terms and the corresponding set of keywords associated with these terms.
Search terms.
1.2.2. Inclusion Criteria
The articles included were primarily filtered based on their relevance. Apart from relevancy, only articles written in English were selected. Furthermore, only articles published after 2014 were considered for inclusion.
1.2.3. Elimination Criteria
The elimination of articles was based on abstract and introduction screening. Articles were then eliminated based on the quality of their research and the lack of references. The parameters used to judge the research quality were the reputation of the journal the article was published in, using metrics such as the h-index and impact factor, the date of publication (the older the date, the less relevant the article may be in present day), and the number of citations the research study had. In addition, any missing relevancy and the redundancy of the research were also considered in the elimination process.
1.2.4. Results
Out of the 1057 non-duplicate articles filtered out from the various research repositories, 826 articles were excluded during the abstract and title screening. From the remaining 231 articles, 62 articles were excluded during the redundancy check and 48 articles were excluded during the full text screening. Finally, 121 articles were obtained after applying the inclusion/exclusion criteria. Figure 1 shows the PRISMA method implementation for the same. Figure 2 indicates the number of reference papers published in each year. Figure 3 demonstrates the various methods that this study encapsulates, and the number of papers cited corresponding to each methodology.

Flow diagram for the selection process of research articles using PRISMA method.

Number of papers per year, used in the review.

ML and DL methods versus frequency of papers used in this work.
1.3. Structure of this Review
This paper is organized as follows. A comparison with previous reviews on skin cancer diagnosis and survey methodology for the same is covered in Section 1 . Section 2 provides an overview of skin cancer, as well as the datasets commonly used in various studies in the field of skin cancer diagnosis. Section 3 is divided into two major subsections. The subsections describe the techniques used to diagnose skin cancer using machine learning and deep learning frameworks and algorithms, respectively. Section 4 talks about the open challenges faced in the field of skin cancer diagnosis, while Section 5 gives an insight into future research directions. The conclusion is given in Section 6 , followed by the references used for this research. Figure 4 visualizes the structure of this study.

Structure of this review.
2. Skin Cancer
Skin cancer is associated with the abnormal growth of skin cells that are found either in the epidermis or the dermis. The skin cells are usually those that are exposed to sunlight, but skin cancer can also occur in those cells that are not ordinarily exposed [ 129 ]. This research focuses on the skin cancer that occurs in the epidermal cells, namely keratinocytes and melanocytes. Skin cancer can be largely divided into three subcategories.
- 1. Basal cell carcinoma: this type of cancer affects and originates from the basal cells. Basal cell carcinoma comes from keratinocytes, which are found in the epidermis. These may invade the entire epidermal thickness.
- 2. Squamous cell carcinoma: this subdivision deals with the uncontrollable growth of the abnormal squamous cells present in the root. Squamous cells are flat cells that are found in the tissue that constitutes the surface of the skin, and the lining of vital organs such as the respiratory organs, digestive tracts, and hollow organs of the body.
- 3. Melanoma: this form of cancer develops when melanocytes start to grow abnormally. Melanocytes are the cells that can become melanoma. Melanoma can develop anywhere in the skin, while it can also form in other parts of the body such as the eyes, mouth, and genitals, etc.
Figure 5 and Figure 6 include images from the International Skin Imaging Collaboration (ISIC) dataset to demonstrate the different types of skin cancer images that are available for training and testing. Figure 5 shows dermoscopic images, while Figure 6 displays clinical images from the skin cancer dataset.

Dermoscopic sample images of skin cancer: ( a ) squamous cell carcinoma, ( b ) basal cell carcinoma, ( c ) benign dermatofibroma, ( d ) benign seborrheic keratosis, ( e ) benign actinic keratosis, and ( f ) malignant melanoma.

Clinical sample images of skin cancer: ( a ) malignant melanoma, ( b ) squamous cell carcinoma, and ( c ) basal cell carcinoma.
Branching out of skin cancer are skin tumors, which are chiefly responsible for the mortality rate once diagnosed with the same [ 17 ]. Skin tumors can be categorized into two types, namely melanoma and non-melanoma. Irrespective of the technological advancements made in the field of curing cancer, to date, the early detection and diagnosis of any tumor combined with enough therapy leads the way to a successful treatment [ 18 ]. There are multiple ways to classify and categorize skin cancer. Most of them employ the use of deep learning techniques such as convolution neural networks in [ 19 ], while the others use specialized tools such as non-invasive imaging tools [ 20 ].
2.1. Skin Cancer Classification
When cells become cancerous, they start to grow uncontrollably due to various reasons, one such reason being a damaged cell DNA. This random behavior of the cell may lead to uneven accumulation and form a solid mass or lump, called a tumor. Tumors are often associated with uncontrollable growth in solid tissues such as muscles and bones. Tumors are further subdivided into two major categories, as described in the following section.
2.1.1. Benign Tumor
Benign tumors are a collection of these cells that grow abnormally but are non-cancerous. According to [ 21 ], these tumors are generally classified according to their level of origin within the skin. The skin has three levels of subclassification in this regard, the epidermal layer, the dermal layer, and the subcutaneous layer. Another common taxonomy followed for benign tumors is based on the cell of their origin. Certain well-known examples are the melanocyte or the keratinocyte [ 22 ].
2.1.2. Malignant Tumor
Malignant tumors are tumors that are cancerous. The affected cells metastasize through the bloodstream and the lymph nodes. In the context of skin cancer, malignant tumors emerging from the surface epithelium of the skin and the epidermis include cutaneous melanoma and non-melanoma cancers such as basal cell carcinoma [ 23 ]. Cutaneous melanomas constitute only 4% of all skin cancers, but they are by far the most significant ones, due to their lethality [ 24 ]. Ref. [ 25 ] conducted a study on developing deep learning techniques to help classify tumors as benign or malignant. False positives and negatives lead to a substandard prognosis of skin cancer. Article [ 26 ] discusses the challenges of detecting malignant tumors, which include, but are not limited to, noisy images, irregular tumor boundaries, and uneven image sizes. Hence, the need for deep learning and machine learning methods to detect malignant tumors is paramount.
2.1.3. Other Tumors
The last subclassification of tumors is loosely classified as pre-malignant tumors. These cells are not cancerous at that moment of time, but they have the potential to become malignant. The major problem faced by the authors of [ 27 ] while detecting pre-malignant tumors was the scarcity of images. This led to them use the same dataset for training and validation. This does not come a surprise, as the study conducted in [ 28 ] also faced difficulty in recording pre-malignant lesion data. Pre-malignant tumors are often clubbed with certain malignant subtypes such as actinic keratosis, which is a squamous cell carcinoma despite being premalignant as well [ 25 ]. This makes it difficult to distinguish between the different classes of tumors.
2.2. Skin Cancer Datasets
Table 3 describes the various datasets used in previous studies and analyzes the constituents of each dataset. Furthermore, the table also identifies the skin cancer image categories available in the respective datasets.
List of various skin cancer datasets employed by previous studies.
3. Machine Learning and Deep Learning Models for Skin Cancer Diagnosis
3.1. need for machine learning and deep learning models for skin cancer diagnosis.
Artificial Intelligence has laid the foundation for integrating computers into the medical field seamlessly [ 30 ]. It provides an added dimension to diagnosis, prognosis, and therapy [ 36 ]. Recent studies have indicated that machine learning and deep learning models for skin cancer screening have been on the rise. This is primarily because these models, as well as other variants of Artificial Intelligence, use a concoction of algorithms, and when provided with data, accomplish tasks. In the current scenario, the tasks include, but are not limited to, the diagnosis of the patient, the prognosis of the patient, or predicting the status governing the ongoing treatment [ 37 ]. Diagnosis is the process of understanding the prevailing state of the patient, while prognosis refers to the process of predicting the future condition of the patient by extrapolating all the current parameters and their corresponding outputs. AI has now progressed to the point where it can be successfully used to detect cancer earlier than the traditional methods [ 6 ]. As early detection is key for a fruitful treatment and better outcome of skin cancer, the need for machine learning and deep learning models in the field of skin cancer is paramount.
3.2. Machine Learning Techniques
3.2.1. artificial neural networks.
Artificial neural networks (ANNs) are systems that draw inspiration from the animal brain. ANNs have been used to predict non-melanoma skin cancer by inputting a certain set of tried and tested parameters fit for training, such as gender, vigorous exercise habits, hypertension, asthma, age, and heart disease etc. [ 38 ] The ANN takes the entire dataset as the input. To improve the accuracy of the model, the network inputs are normalized to values between 0 and 1. The outputs are treated as typical classification outputs, which return fractional values between 0 and 1. ANNs can also be used to detect skin cancer by taking an image input and subjecting it through hidden layers [ 39 ]. This process is carried out in four sequential steps, the first of which is to initialize random weights in the ANN system. Next, each of the activation values are calculated. Consequently, the magnitude of the error is also known as the loss change. The weights are updated proportionately, with respect to the loss. Until the loss reaches a certain lower bound or a floor value, the three steps are repeated. In this field that pertains to skin cancer detection, visual inspection is the introductory stage. This is due to the similarities shared between various subcategories of tumors, such as color, area, and distribution. Owing to this reason, the use of ANNs is encouraged to enhance multi-class skin lesion detection [ 40 ]. The trained network models are used with a logistic regression model to successfully detect skin lesions while reducing the false positives and negatives in the process. The choice of activation function for the ANN is completely dependent on the user, and it is to be noted that each function carries its own sets of advantages and disadvantages with respect to the convergence of the model and the computational load [ 40 ]. ANNs have been used to simultaneously predict various symptoms that generally occur in cancer-affected patients, as seen in [ 41 ]. The risk of symptoms predicted were that of pain, depression, and poor well-being. The input to the ANN was a list of 39 distinct covariates. The input features can be classified into five subcategories, such as demographic characteristics such as age and sex, clinical characteristics such as the cancer type and stage, treatment characteristics such as the radiation treatment and cancer surgery, baseline patient reported measures such as the performance status and symptom burden status, and finally, health care utilization measures such as whether the patient has been hospitalized or if they have a live-in caregiver. ANNs play an important role in predicting skin cancer and the presence of a tumor, due to their flexible structure and data-driven nature, owing to which they are considered as a potential modeling approach [ 42 ].
The model proposed by [ 38 ] reports a sensitivity of 88.5% and a specificity of 62.2% on the training set, while the validation set showed a comparable sensitivity of 86.2% and a specificity of 62.7%. Similarly, the ANN model in [ 39 ] was tested over multiple sets, each using an increasing number of training and testing image ratios. The accuracy returned by the model falls between 80% and 88.88%.
In [ 38 , 39 , 40 ], emphasis is put on the need for optimizing predictors, increased model parameters, and the conduction of more clinical testing to improve the sensitivity and specificity of the model. Despite being easy to implement and cost effective, ANN models require further development in future studies for skin cancer diagnosis.
3.2.2. Naïve Bayes
Naïve Bayes classifiers are probabilistic classifiers that work by employing the use of Bayes’ theorem. Naïve Bayes classifiers have been used in the field of skin cancer to classify clinical and dermatological images with high precision [ 43 ]. The model has reached an accuracy of 70.15%, as it makes use of important pieces of data to develop a strong judgement and assists physicians in the diagnosis and precise detection of the disease. Naïve Bayes classifiers extend their applications by providing a means to detect and segment skin diseases [ 44 ]. For each output class of the classifier, a posterior probability distribution is obtained. This process is performed iteratively, which implies that the method requires lesser computational resources, as it avoids the need for multiple training sessions. The Bayesian approach has also been used to probabilistically predict the nature of a data point to a high degree of accuracy, as seen in [ 45 ]. The final classification made in this case combines the existing knowledge of data points to use in the Bayesian analysis. The Bayesian sequential framework has also been put into use to aid models that help to detect a melanoma invasion into human skin. A total of three model parameters were estimated with the help of the model, namely, the melanoma cell proliferation rate, the melanoma cell diffusivity, and ultimately, a constant that determines the degradation rate of melanoma cells in the skin tissue. The algorithm learns data through the following, in a sequential manner: a spatially uniform cell assay, a 2D circular barrier assay, and finally, a 3D invasion assay. This Bayesian framework can be extracted and used in other biological contexts due to its versatile nature. This is chiefly possible in situations where detailed quantitative biological measurements, such as skin lesion extraction from scientific images, is not easy; hence, the extraction method must incorporate simple measurements from the images provided, like the Bayesian framework does [ 46 ].
Naïve Bayes classifiers, as discussed in [ 43 ], achieve an accuracy of 70.15% and a specificity of 73.33%. At the same time, the classifiers do not breach the 70% mark in sensitivity and precision. The accuracy appears to follow a similar pattern in naïve Bayes classifiers from other studies such as [ 44 ], where the diagnostic accuracies reported are 72.7%.
The recurring scope of improvement in [ 43 , 44 , 45 ] revolves around experimenting with different color models, as well as using different types of dermal cancer datasets in the training. In [ 44 , 46 ], they elucidate the pressing need for further pre-processing before training naïve Bayes classifiers for skin cancer diagnosis.
3.2.3. Decision Tree
Decision trees are a supervised learning method which are primarily used for classification problems and are occasionally extended to fit regression problem statements as well. Decision trees have been used to provide an intuitive algorithm that helps quantify the long-term risk of non-melanoma skin cancer after a liver transplant. This is done by utilizing the variables closely associated with the peri-transplant period [ 47 ]. The classifier is used as a view for the patients which provides personalized solutions such as chemoprophylaxis. A slight variation of decision trees can also be employed, as seen in [ 48 ]. The article proposes a random decision tree algorithm to detect breast surgery infection. The risk factors that came along with the algorithm in this case were obesity, diabetes, and kidney failure, etc. While the study investigates breast cancer, skin cancer is most closely associated with breast cancer due to the presence of the dangerous melanoma type. Decision trees showcase its versatility in the way it is used. In [ 49 ], decision trees are used as a mode for the visual representation of problem by dividing each branch into the different outcomes possible during a clinical procedure. The decision tree model was used to gauge the cost effectiveness of the sentinel lymph node biopsy, a new standard technique used in the treatment of melanoma and breast cancer. The cost effectiveness was measured with respect to head and neck cutaneous squamous cell carcinoma, a subsection of skin cancer. The decision tree presented outputs to determine whether the treatment was cost effective for a particular set of tumors, or if it could be used generally. Decision trees can also be used as an intermediate layer instead of keeping them as a standalone classifier. In [ 50 ], they demonstrate the effectiveness of this architecture in extracting regions and classifying skin cancer, using deep convolution neural networks. Most of the features are classified using decision trees and other counterpart algorithms such as support vector machines and k-nearest neighbors. Decision trees are also used to attain clarity in the classification of breast cancer, as can be seen from [ 51 ]. The error analysis of the proposed model reveals that the foundational decision tree models provide users with easy-to-use outcomes and a very high degree of clinical detection and diagnostic performance, as compared to its predecessors.
The decision tree model from [ 47 ] reports a specificity of 42% and a sensitivity of 91%. Similarly, the models presented in [ 48 ] return a sensitivity, specificity, and accuracy greater than 90%. This trend follows suit in the model proposed by [ 50 ], where all the three parameters cross 94%. On the contrary, models like those in [ 49 ] return a slightly lower sensitivity of 77% but report a 100% specificity.
Decision trees’ model predictions are heavily dependent on the quality of the datasets. The common pitfalls encountered by [ 47 , 50 ] are that the model testing and training datasets had an identical distribution of variables; hence, this eliminates the prospect of training the model on entirely independent cohorts.
3.2.4. K-Nearest Neighbors
The k-nearest neighbors algorithm, also referred to as the KNN, is a parametric supervised classification algorithm that uses distance and proximity as metrics to classify the data points. KNNs were used as an evaluation algorithm to detect skin cancer and melanomas. The KNN model was then used to produce a confusion matrix which helps with visualizing the accuracy of the entire model [ 52 ]. Apart from this case of use, KNNs have also been used extensively by extending the model as per requirement. In [ 53 ], they extend KNN to use the Radius Nearest Neighbors classifier to classify breast cancer and calculate the evaluation metrics such as accuracy and specificity. The reason for augmenting the KNN solely lay in the limitations posed by an extreme value of k. For a small k, the KNN classifier is highly sensitive to outliers, and for a large value of k, the classifier underfits on the training data points. This problem is overcome by normalizing the radius value of each point to recognize outliers effectively. The applications of KNNs have been expanded by using them for detecting the anomalous growth of skin lesions [ 54 ]. KNNs are hybridized with Firefly to provide quantitative information about a skin lesion without having to perform any unnecessary skin biopsies. The hybrid classifier built upon KNN is used to predict and classify using two primary methods: threshold-based segmentation and ABCD feature extraction. The Firefly optimization coupled with KNN helps to recognize skin cancer much more effectively than its predecessors, while keeping computational and temporal complexity to a minimum. To classify and discriminate between melanoma and benign skin lesion in clinical images, ref. [ 55 ] made use of multiple classifiers, out of which the KNN classifier returned competent results. The article also makes use of different color spaces and tests the classifiers on each of them to demonstrate the feasibility of the algorithms to detect melanomas in various color spaces.
The KNN classifiers of [ 52 ], with the number of neighbors set to 15, returned an accuracy of 66.8%, with a precision and recall for positive predictions of 71% and 46%, respectively. The recall value increases almost twofold for negative predictions, while the precision score for the same lingers around 65%. The values in [ 53 ] provide a different perspective to the modified KNN classifiers, as they report an accuracy of over 96%. Fuzzy KNN classifiers, as shown in [ 54 ], have an accuracy of 93.33%, with a sensitivity of 88.89% and a specificity of 100%.
Despite being a viable approach to diagnosing skin cancer, KNN classifiers require the provision to be trained continually, as suggested by [ 52 ]. Furthermore, with the dearth of feasible datasets, the size of suitable training data proves to be a limitation for [ 52 , 53 ]. To mitigate the adverse effects of minimal training data, the KNN classifier can fit into an online learning method that builds over time and keeps learning as and when the classifier acquires more data.
3.2.5. K-Means Clustering
K-means clustering is a clustering method that is grouped under unsupervised learning. By employing a fuzzy logic with the existing k-means clustering algorithm, studies have been conducted on segmenting the skin melanoma at its earliest stage [ 56 ]. Fuzzy k-means clustering is applied to the pre-processed clinical images to delineate the affected regions. This aids the process to subsequently be used in melanoma disease recognition. K-means clustering has widespread cases of use and can be used to segment skin lesions, as seen in [ 57 ]. The algorithm groups objects, thereby ensuring that the variance within each group is at minimum. This enables the classifier to return high-feature segmented images. Each image pixel is assigned a randomly initialized class center. The centers are recalculated based on every data point added. The process is repeated until all the data points have been assigned clusters. Unlike a binary classifier like k-means, where each data point can belong to only one cluster, fuzzy c-means clustering enables the data points to be a part of any number of clusters, with a likelihood attached to hit. The fuzzy c-means algorithm outputs comparatively better results in comparison with the legacy k-means clustering algorithm. Fuzzy c-means provide a probability for data points that depends on the distance between the cluster center and the point itself. In [ 58 ], fuzzy c-means were used in place of the k-means algorithm to detect skin cancer, inspired by a differential evolution artificial neural network. The simulated results indicated that the proposed method outperformed traditional approaches in this regard. The k-means algorithm can also be used as an intermediate layer to produce outputs, as trained on by deep learning methods. In [ 59 ], they demonstrated an algorithm where k-means were used to segment the input images based on the variation of intensities. The clusters thus formed were then subjected to further processing to aid in the detection of melanoma cancer. The traditional k-means algorithm can also be used to detect skin lesions. To augment the quality of the results, it can be used with a gray level co-event matrix, a local binary pattern, and red, green, and blue color modes [ 60 ]. K-means clustering is heavily dependent on external factors being extracted successfully, such as color features, lesion orientation, and image contrast [ 56 , 58 , 59 ]. This engenders the need for coherency in the diagnosis pipeline that utilizes the k-means clustering. The pipeline must accurately extract external features before the clustering algorithms take them as input.
K-means clustering models tend to return a high detection accuracy. For instance, the model that extends fuzzy logic, like in [ 56 ], returns an accuracy of over 95%. Other k-means clustering models, like those in [ 58 ] and [ 59 ], also report a detection accuracy of 90%.
3.2.6. Random Forest
Random forests are an extension of decision trees. They are an ensemble learning method commonly used for classification problems. Random forests extend their applications to detect skin cancer and classify skin lesions, as done in [ 61 ]. Random forests permit the evaluation of sampling allocation. The steps followed in the proposed method are to initialize a training set. The training set is then bootstrapped to generate multiple sub-training sets. By calculating the Gini index for each of the sub-training sets, the model is then populated with decision values. The individual decision values are then combined to generate a model that classifies by voting on the test samples. Skin cancer can also be classified by characterizing the Mueller matrix elements using the random forest algorithm [ 62 ]. The random forest algorithm builds various sub-decision trees as the foundation for classification and categorization tasks. Every individual decision tree is provided with a unique logic that constitutes the binary question framework used in the entirety of the system. In comparison with the original decision tree, the random forest provides enhanced results while reducing the variance bias. This helps to prevent the overfitting of the data, which was otherwise seen in decision trees. Other studies in the classification of skin cancer involve classifying the dermoscopic images into seven sub-types. This has been implemented with the help of random forests [ 63 ]. The procedure to create a random forest is slightly unconventional in this study. After preparing a dataset to train on, the random forest is then amassed by arranging a relapse tree. The ballot casting is conducted after the forest architecture is built. The different types of sub-classifications that the random forest was trained on were basal cell carcinoma, benign keratosis lesion, dermatofibroma, melanocytic nevi, melanoma, and vascular types. Similarly, skin lesion classification has also been performed with the help of random forests and decision trees in [ 64 ]. Using random forests are key since predecessor algorithms lack the reliability aspect of skin image segmentation and classification. The random forest is generated by selecting a subset of random samples in the skin lesion dataset. For each feature in the subsets, a decision tree is created to get a prediction. A voting process is then established for each of the prior outputs, and a forecast result with the most votes is selected as the final step.
Random forest classifiers, as seen in [ 61 ], report an accuracy, sensitivity, and specificity of around 70%, regardless of the features incorporated to segment the required area, such as ABCD rule or GLCM features. Depending on the dataset used, random forest classifiers can also have a high accuracy while detecting skin cancer. The models in [ 62 ] achieved an average accuracy of 93%.
The features of a random forest classification algorithm are invariant to image translation and rotation [ 61 , 64 ]. This allows future research to be more liberal with its datasets and extend them to a variety of geographies to discern the consistency in results returned by skin cancer classification.
3.2.7. Support Vector Machine
Support vector machines (SVMs) are supervised learning models that help classify, predict, and extrapolate data by analyzing them. SVMs can be used to classify different types of skin lesions. In [ 65 ], ABCD features are used for extracting the characteristic features like shape, color, and size from the clinical images provided. After selecting the features, the skin lesion is classified with the help of SVMs into melanoma, seborrheic keratosis, and lupus erythematosus. This method of using ABCD along with SVM generates great results while delivering significant information. For a narrower classification, SVMs have also been used to classify skin lesions as melanoma or non-melanoma [ 66 ]. The process was divided into six phases: acquiring the image, pre-processing the image, segmentation, extracting the features, classifying the image, and viewing the result. From the experiment, the features extracted were texture, color, and shape. To extend the nature of the above model, SVMs have also been employed to identify and detect carcinoma or infection in the early stages before it aggravates [ 67 ]. The chief difference in the extension and itself lies in the feature extraction procedure. In [ 67 ], they pre-process the input image by employing grey scale conversion and then chaining the resultant image with noise removal and binarization subprocesses. The region of interest is removed in segmentation to help with accurate classification. Similarly, for the early detection and diagnosis of skin cancer, a bag-of-features method was used, which included spatial information. The SVM was developed with the help of a histogram of an oriented gradient optimized set. This resulted in encouraging results when compared to state-of-the-art algorithms [ 68 ]. By using Bendlet Transform (BT) as features of the SVM classifier, unwanted features such as hair and noise are discarded. These are removed using the preliminary step of median filtering. BT outperforms representation systems such as wavelets, curvelets, and contourlets, as it can classify singularities in images much more precisely [ 69 ].
The average accuracy of the SVM classifier models presented in [ 65 ] was about 98%, while the sensitivity and specificity averaged to 95%. The SVM model in [ 66 ] also had all three parameters greater than 90%.
3.2.8. Ensemble Learning
Ensemble learning is a machine learning model that combines the predictions of two or more models. The constituent models are also called ensemble members. These models can be trained on the same dataset or can be suited to something completely different. The ensemble members are grouped together to output a prediction for the problem statement. Ensemble classifiers have been used for diagnosing melanoma as malignant or benign [ 70 ]. The ensemble members for the same are trained individually on balanced subspaces, thereby reducing the redundant predictors. The remaining classifiers are grouped using a neural network fuser. The presented ensemble classifier model returns statistically better results than other individual dedicated classifier models. Furthermore, ensemble learning has also been used in the multi-class classification of skin lesions to assist clinicians in early detection [ 71 ]. The ensemble model made use of five deep neural network models: ResNeXt, SeResNeXt, ResNet, Xception, and DenseNet. Collectively, the ensemble model performed better than all of them individually.
3.2.9. Summary of Machine Learning Techniques
Analyzing the various implementations of machine learning models in the field of skin cancer diagnosis indicates that simple vector machines are undoubtedly the most precise and accurate models. The main caveat of using SVMs is the need for the meticulous pre-processing of input data. In terms of user flexibility, k-means clustering and k-nearest neighbors lead the way, without compromising much on accuracy and performance. KNNs, however, require to be trained continuously as more data points are added. This might prove to be quite tedious as the volume of input data is highly irregular and cannot be predicted. Naïve Bayes models have the lowest accuracy of all the machine learning techniques studied in this paper, and understandably so, as various techniques make use of the fundamentals of the naïve Bayes theorem and develop it further, such as decision trees and random forests. Decision trees perform decently but are highly dependent on the quality of the dataset, which is an uncontrollable variable in the system. Random forests do not have the provision to learn image rotation and translation on the fly, reflecting the same in their classification accuracy. Depending on the dataset used, random forests can either perform really well, or get only around 50% of classifications correct. ANNs, being the steppingstone for various techniques to develop, suggest that while the results may be good, they cannot be increased further. ANNs have reached a saturation point in terms of the modifications made, and other techniques must be employed if any improvement is expected. Ensemble models, although complicated and tough to implement, return accuracies higher than the models taken individually for the multi-class classification.
Table 4 provides an executive summary of the machine learning techniques used in the diagnosis of skin cancer. Figure 7 conceptualizes the machine learning models in skin cancer diagnosis discussed in this study.

Current machine learning models in skin cancer diagnosis: tree illustration.
Summary of works on machine learning techniques in skin cancer diagnosis.
3.3. Deep Learning Techniques
3.3.1. recurrent neural network.
A recurrent neural network (RNN) is categorized as a subdivision of artificial neural networks. RNNs have been used in the detection of melanoma skin cancer [ 72 ]. The classification phase of the proposed model employs deep learning techniques by combining the optimization notion into an RNN. The existing region growing algorithm and RNN have been improved by using them alongside the modified deer hunting optimization algorithm (DHOA). Apart from standalone models, RNNs have also been used in ensemble models alongside convolution neural networks, as seen in [ 73 ], to classify skin diseases. Predecessor models were unable to use the long-term dependence connection between key image features and image classes. This served as the motivation for the proposed model. Deep features are extracted from the clinical images, after which the features are fed into the dual bidirectional long short-term memory network to learn the features. Ultimately, a SoftMax activation function is used to classify the images. Similarly, ensemble models can also be used to automate the detection of mammogram breast cancer [ 74 ]. Just like in [ 73 ], the first step involves feature extraction through the grey level co-occurrence matrix and the grey level run-length matrix. These two are then given to the RNN layer as inputs, and the tumor segmented binary image is provided as input to the convolution neural network layer. The two independent classifiers’ results show an improved diagnostic accuracy. RNNs have also been used in the segmentation of various dermoscopic images [ 75 ]. The reason for incorporating a recurrent model is primarily due to its ability to train deeper and bigger models. Furthermore, recurrent models ensure better feature representation and ultimately, better performance for the same number of parameters.
Modified RNNs, as proposed in [ 72 ], have an average accuracy of slightly over 90%, with an F1-score of 0.865. By varying the variable value in the equation used, the accuracy follows a linear trend by increasing as the value increases. Like the previous result, the RNNs in [ 74 ] have an accuracy of 98% but an F1-score of 0.745. The model in [ 75 ] reports a testing accuracy of 87.09% and an average F1-score of 0.86.
3.3.2. Deep Autoencoder
Deep autoencoders are neural networks that are trained to emulate the input as the output. They consist of two symmetrical deep belief networks. In the field of skin cancer, deep autoencoders have been used for reconstructing the dataset, which is then used to detect melanocytes by employing spiked neural networks [ 76 ]. The structure of the autoencoder model consists of three main layers: the input layer, hidden layers, and the output layer. The model is run on the foundational principle that every feature is not independent of each other, otherwise it would compromise the efficiency of the model. Autoencoders have also been used to recognize and detect melanoma skin disease [ 77 ]. The various autoencoders used were Deeplabv3+, Inception-ResNet-v2-unet, mobilenetv2_unet, Resnet50_unet, and vgg19_unet. Quantitative evaluation metrics showed that the Deeplabv3+ was a significant upgrade from the other architectures used in the study to detect melanoma skin. Skin cancer detection has also been carried out with the help of custom algorithms involving autoencoders, such as the social bat optimization algorithm [ 78 ]. The detection process takes place in three steps. Firstly, the clinical images are pre-processed to remove the noise and artefacts present. The pre-processed images are then fed to the feature extraction stage through a convolution neural network and a local pixel pattern-based texture feature. Right after this stage, the classification is completed using a deep stacked autoencoder, much like the evaluation in [ 77 , 79 ] of different autoencoders for skin lesion detection. The five architectures evaluated in this study are u-net, resu-net, vgg16unet, desnenet121, and efficientnetb0. Among the evaluated architectures, the densenet121 architecture showed the highest accuracy.
The autoencoder-based dataset used in [ 76 ] returned an average accuracy of 87.32%, with the sensitivity and specificity within one point of accuracy as well. The study in [ 77 ] concluded that using autoencoders consistently increased the accuracy and F1-score in various datasets, as opposed to the models that did not employ deep autoencoders. The average accuracy of the models in [ 77 ] after using autoencoders is around 94%. In a similar fashion, the deep stacked autoencoder presented in [ 78 ] returned an average accuracy of 93%, sensitivity of 84%, and specificity of 96%.
3.3.3. Long Short-Term Memory
Long short-term memory, or LSTM, is an artificial neural network that uses feedback connections to enable the processing of not only single data points, but also sequential data. LSTM has helped in classifying skin diseases by efficiently maintaining stateful information for accurate predictions [ 80 ]. The robustness of the proposed algorithm helps to recognize target regions faster, while using almost half the number of computations compared to predecessor algorithms. The use of LSTM further bolsters the accuracy of prediction due to its previous timestamp retention properties. Other than plain recognition, LSTMs can also be used to predict cancer and tumors in irregular medical data [ 81 ]. This is made possible by the enhanced overall performance of LSTMs in screening time series data. The risk groups being dealt with in the proposed study correlated well to the temporal cancer data (time to cancer diagnosis). Skin disease classification models have been designed using deep learning approaches like LSTM with the assistance of hybrid optimization algorithms such as the Hybrid Squirrel Butterfly Search Optimization algorithm (HSBSO) [ 82 ]. The modified LSTM is developed by implementing the HSBSO and the optimized parameters of an LSTM model to maximize the classification accuracy. LSTMs help in improving the overall efficiency of the proposed skin disease classification model. Deep learning models are not only limited to the clinical images of tumors. Certain studies demonstrate the usage of convolutional LSTMs to detect aneurysms on angiography images [ 83 ]. The angiography images are obtained from the 2D digital subtraction angiography, thereby making it hard to distinguish cerebral aneurysms from the overlapping vessels. The convolutional LSTM (C-LSTM) is a variant of the LSTM. Each LSTM cell has a convolutional operation associated with it. C-LSTM inherits the advantages of LSTM while being very suitable for the analysis of spatiotemporal data due to its internal convolution architecture. In real-life diagnoses, physicians combine lateral and frontal sequences to aid the decision-making process. Employing a similar concept, the C-LSTM is fed with two inputs: frontal and lateral images to increase the spatial information, consequently improving the performance of the entire system.
The incorporation of LSTM components to pretrained models such as the MobileNet V2, as seen in [ 81 ], outperforms some state-of-the-art models, with a training accuracy of 93.89% and validation accuracy of 90.72%. The study conducted in [ 82 ] demonstrated that LSTM performs better than most machine learning models, with an average sensitivity of 53% and specificity of 80%.
3.3.4. Deep Neural Network
Deep neural networks are those neural networks that expand to a certain level of complexity and depth. Vaguely, the certain level is decided to be two or more layers. Deep nets have been used to estimate the uncertainty lying in skin cancer detection [ 84 ]. The motivation behind the model lies in the ineptness of publicly available skin cancer detection software for providing confident estimates of the predictions. The study proposes the Deep Uncertainty Estimation for Skin Cancer (DUNEScan) that provides an in-depth and intuitive analysis of the uncertainty involved in each prediction. Deep nets have also been used to classify skin cancer at a dermatological level [ 85 ]. The classification of skin lesions, with the help of images alone, is an arduous task due to the minute variations in the visual appearance of lesions. Deep nets show immense potential for varied tasks that constitute multiple fine subcategories. The performance of the model is evaluated using biopsy-proven clinical images that were classified into two binary classification problems: keratinocyte carcinomas and benign seborrheic keratoses, and malignant melanomas and benign nevi. The deep net model achieves a performance that matches and, in some cases, outperforms all the experts associated with the evaluation program. For instance, the confusion matrix comparison between deep nets and dermatologists (experts) exhibits similarities in the misclassification of tumors [ 85 ]. The distribution demonstrates the difficulty in classifying malignant dermal tumors for both experts as well as deep nets, but also shows that experts noticeably confuse benign and malignant melanocytic lesions with each other, while the deep net classifies it with a higher degree of accuracy. Deep nets are usually implemented as a single-stream network. Two-stream deep nets, on the other hand, combine two recognition streams to handle the separate features associated with the input data. Two-stream deep nets have been used to design intelligent systems that classify skin cancer [ 86 ]. The two streams in the proposed method are a fusion-based contrast enhancement technique coupled with a pretrained DenseNet201 architecture, and down sample the extracted features using the proposed selection framework. The evaluation parameters suggest that the proposed method returns an improved performance upgrade over the predecessor models. Deep net models have also been deployed in real world applications, empowering medical professionals by assisting the process of diagnosing skin cancer and employing a prediction model for over 100 skin disorders [ 87 ]. The deep learning algorithms have proven to be a successful method with which to diagnose malignant tumors, as well as suggest treatment if trained with a dataset consisting of substantial numbers of Asian and Caucasian populations. Using a convolution neural network as the ancillary tool, the performance is elevated and can be used to diagnose cutaneous skin diseases.
3.3.5. Deep Belief Network
Deep belief networks (DBN) are generative graphical models that are composed of multiple layers of latent variables. DBNs have been used for cancer prediction, as can be seen in [ 88 ]. They perform the model training in two steps. Firstly, each layer is separately trained in an unsupervised manner. This is done to retain the maximum feature information. Subsequently, the output features are taken and used to train the entity relationship classifier in a supervised manner. DBNs have been designed to automatically detect regions of breast mass and diagnose them as benign, malignant, or neither [ 89 ]. The proposed DBN performs comparatively better than its conventional counterparts. This is because the conventional approaches depend on the output of selection feature algorithms. On the contrary, all the features were directly used without any reduction in their dimensions for the DBN model. To improve the diagnosis of skin melanoma by using DBNs in place of the traditional approach, dermoscopy has been studied [ 90 ]. The deep belief learning network architecture disperses the weights and hyperparameters to every position in the clinical image. By doing so, this makes it possible to scale the algorithm to varying sizes. The images are first use a Gaussian filter to remove the high and low intensities from the images. Subsequently, the pre-processed images are segmented using the k-means algorithm. The resultant images are then classified as per the output format of the proposed DBN.
The DBN presented in [ 88 ] reports a diagnostic accuracy of 81.48%. According to the study, for the evaluation criteria tested, the DBN outperformed the RNNs and CNNs, which had an accuracy of 73% and 68%, respectively. DBNs that are used to complement computer-aided diagnosis, as seen in [ 89 ], report an average accuracy of around 91%. For unsegmented images, the DBN model in [ 90 ] achieves an accuracy of 73% while the same model, when subjected to segmented images, achieves an accuracy of 90%. This suggests that DBNs might accurately predict if the input is segmented and pre-processed correctly.
3.3.6. Deep Convolutional Neural Network
Convolutional neural networks (CNNs) are artificial neural networks that are primarily used in image processing and recognition. Deep convolutional neural networks have been implemented to classify skin cancer into four different categories: basal cell carcinoma, squamous cell carcinoma, actinic keratosis, and melanoma [ 91 ]. The methodology involves two methods, an error-correcting output codes simple vector machine (ECOC SVM) classifier, and a deep CNN. The authors use accuracy, sensitivity, and specificity as evaluation parameters. A slight variation from the previous method introduces a LeNet-5 architecture along with a deep CNN to classify the image data [ 92 ]. The model aids the diagnosis of melanoma cancer. The experiment results indicate that training data and number of epochs for training are integral to the process of the detection and diagnosis of melanoma cancer. Results suggest that training the model for over 100 epochs may lead to overfitting while training it for below 100 epochs leads to underfitting. In addition, there are several parameters which account for the accuracy of the results, such as the learning rate, number of layers, and dimensions of the input image. Since dermatologists use patient data along with deep CNNs for an increased diagnostic accuracy, recent studies have investigated the influence of integrating image feature data into the deep CNN model [ 93 ]. The commonly used patient data were sex, age, and lesion location. To accommodate the patient data, one-hot encoding was performed. The key differentiator between fusing the image features was the complexity associated with each classification, respectively. The studies indicate the potential benefits and advantages of amalgamating patient data into a deep CNN algorithm. Region-based CNNs have been employed to detect keratinocytic skin cancer on the face [ 94 ]. The algorithm aims to automatically locate the affected and suspected areas by returning a probabilistic value of a malignant lesion. The deep CNN was trained on over one million image crops to help locate and diagnose cancer. While the algorithm demonstrated great potential, certain pitfalls were highlighted: skin markings were mistaken as lesions by the deep CNN model. Secondly, the testing data usually made use of the physician’s evaluation data, rather than the clinical photographs alone, which ultimately led to the need for a multimodal approach. The developments of recent studies have enabled newly designed models to outperform expert dermatologists and contemporary deep learning methods in the field of multi-class skin cancer classification, using deep CNNs [ 95 ]. The model was fine-tuned over seven classes in the HAM10000 dataset. While ensemble models increase the accuracy for classification problems, they do not have a major role in refining the performance of the finely-tuned hyperparameter setup for deep CNNs.
The deep CNNs, as seen in [ 91 ], could classify skin cancer with an accuracy of 94.2%. Furthermore, the sensitivity and specificity of the model were also above 90%. Region-based CNN that is used to classify skin cancer on the face [ 94 ] returns an average accuracy of 91.5%. The study further emphasized the benefits of using a CNN-based model as a screening tool to improve public health, as the sensitivity of the general public was merely 50%. The model, on the other hand, averaged a sensitivity of 85%.
3.3.7. Deep Boltzmann Machine
Deep Boltzmann machines (DBM) are probabilistic, unsupervised, and generative models that possess undirected connections between multiple layers within the model. Multi-modal DBMs have been proposed to monitor and diagnose cancer before the mortality rate rises [ 96 ]. The multi-modal DBM learns the correlation between an instance’s genetic structure. The testing and evaluation phase use the same to predict the genes that are cancer-causing mutations specific to the specimen. By combining restricted Boltzmann machines (RBM) and a skin lesion classification model through optimal segmentation, the OS-RBM model helps to detect and classify the presence of skin lesions in clinical images [ 97 ]. The OS-RBM model carries out certain steps sequentially: image acquisition, pre-processing using Gaussian filters, segmenting the pre-processed images, extracting the features, and classifying the images. Segmenting images is executed through the Artificial Bee Colony algorithm.
3.3.8. Deep Reinforcement Learning
Reinforcement learning (RL) is a training method often associated with rewarding and punishing the desired and undesired behaviors, respectively. Reinforcement learning algorithms have been incorporated into the medical scene to automatically detect skin lesions [ 98 ]. This is done by initially proceeding from coarse segmentation to sharp and fine results. The model is trained on the popular ISIC 2017 dataset and HAM10000 dataset. The regions are initially delineated. By tuning the hyperparameters appropriately, the segmentation accuracy is also boosted. As deep RL methods have the capability to detect and segment small irregular shapes, the potential for deep RLs in the medical background is immense.
3.3.9. Extreme Learning Machine
Extreme learning machines (ELM) are essentially feedforward neural networks. While they provide a good generalization performance, the major difference arises in the learning speed. ELM models have been proposed to tackle the existing problem of skin cancer detection [ 99 ]. This detection takes place by differentiating between benign and malignant lesions. Upon pre-processing the clinical images, the regions are segmented using the Otsu method. The model optimizes and learns with the help of a deep belief network which introduces a Thermal Exchange Optimization algorithm. Using hybrid pretrained models along with ELMs for diagnosing skin cancer has also been researched [ 100 ]. The proposed diagnostic model makes use of the SqueezeNet model for the batch normalization layers. The layers towards the end of the model are replaced by ELMs. The ELMs are usually linked with a metaheuristic, for instance, the Bald Eagle Search Optimization metaheuristic, that enable the model to converge much faster than its contemporary counterparts. Instead of pretrained models, hybrid deep learning models have also been combined with extreme learning machines to classify skin lesions into multiple classes [ 101 ]. While majority of the steps remain the same, the major differences lie in the deep feature extraction that uses transfer learning and feature selection, which makes use of hybrid whale optimization and entropy-mutual information algorithms. Extreme learning machines can also be modified and used as an extreme gradient boosting method for the remote diagnosis of skin cancer [ 102 ]. Apart from diagnosis, the model also helps in the process of health triage. The major problem faced by the authors were the unbalanced categories in the dataset. To overcome this imbalance, data augmentation was incorporated. Integrating the skin lesions with the clinical data reinforced the accuracy and efficacy of the model.
ELM models that are used for multi-class skin lesion classification [ 101 ] produce high-quality predictions with an accuracy of over 94%. ELM models have been shown to consistently outperform respective benchmark studies, as seen in [ 102 ]. Even though the accuracy of the model in [ 102 ] hovers around 77%, it is significantly higher than the benchmark studies for the same set of data and conditions. When coupled with data augmentation, ELMs can avoid the risk of overfitting.
3.3.10. Summary of Deep Learning Models
Deep learning models provide robust solutions for skin cancer detection. Recurrent neural networks can accurately predict the incidence of skin cancer to a fairly high degree, but they come with the limitation of being efficient only when using large datasets. For smaller data points, RNNs will not have enough data to learn the features and predict as accurately. Autoencoders serve as a recourse for insufficient data. Deep autoencoder-based datasets, used with pretrained models, return highly accurate results. The major drawback involved in deep autoencoders is the parameter value initialization. Most of the studies employ a preliminary trial method to settle for the initial parameter values, which may prove to be infeasible for large models. Long short-term memory models outperform other deep learning techniques in terms of classification and tumor growth progress analysis, but the accuracy of the model sharply drops to below 80% when the quality of the images is substandard, such as poor illumination or conditions different from those in the testing dataset. Deep neural networks produce good results but cannot match the versatility of other deep learning techniques such as RNNs or LSTMs. DNN models find it tough to distinguish between blurry shadows or irregular borders unless they have been trained on such data. To be widely adopted, DNNs require training images with adequate quality, making it a cause of concern, as clinical data may not emulate the training dataset conditions. Deep belief network models return highly accurate results, but in similar conditions, are often outperformed by convolutional neural networks. CNNs provide users with the flexibility to extend the model with different learning techniques, as well as accurately predict different types of skin cancer. Most of the studies involving CNNs reported an average accuracy of over 90%. New techniques in the deep learning space make use of extreme learning machines. These models outperform state-of-the-art techniques, with reported accuracies of over 93%. While they return accurate results, they are susceptible to poorly augmented datasets, which can sharply decrease the accuracy of the model.
Table 5 summarizes the works discussed on deep learning models used for skin cancer diagnosis. Figure 8 shows the deep learning models in skin cancer diagnosis, as elucidated in this study.

Current deep learning models for skin cancer diagnosis: tree illustration.
Summary of works on deep learning models in skin cancer diagnosis.
4. Open Challenges in Skin Cancer Diagnosis
4.1. communication barrier between ai and dermatologists.
Giant strides in the field of Artificial Intelligence for skin cancer diagnosis mean it may have established its place in the scene for years to come, but it has still not been able to breach the communication barrier that exists between dermatologists and AI. In [ 103 ], it is suggested that dermatologists must embrace the potential shown by AI applications in various fields, such as clinical and research situations. The preconceived notion surrounding the use of AI in the cancer diagnosis domain that the introduction of technology may eventually downsize the workforce, has set the wrong precedent, and has brought about apprehensions about adopting AI for the same. It must be understood that, while AI has been ever improving and returns a higher accuracy with respect to diagnoses, clinicians are undoubtedly more skillful in identifying mimetics, as well as patterns that have not been made available to the models through the training datasets [ 103 ]. The authors of [ 103 ] reiterate that the role of dermatologists is not limited to detecting and identifying skin lesions, but also to extract valuable information and inferences from their observations. At present, the latter is not quite fine-tuned and is still in the rudimentary stages in machines. In a survey conducted on Chinese dermatologists [ 104 ], the majority of the participants believed that AI in the workplace assisted with daily activities involving diagnosis and treatment. In accordance with the claims made by the authors of [ 103 ], the survey results indicated that only 3% of the dermatologist participants believed that AI could and would replace their day-to-day work. Another survey in [ 105 ] indicated positive diagnosis results and a higher accuracy, as compared to traditional approaches, after dermatologists used the help of AI in making their decisions. The need to bridge the communication gap between AI and dermatologists is paramount, and efforts must be taken to involve the various functionalities offered by AIs in the workplace.
4.2. Dataset Availability and Features
Incorporating deep learning into cancer diagnosis in the real world comes with one major roadblock—the lack of availability of datasets. Machine learning and deep learning algorithms require huge amounts of datasets to be trained upon, without which the models will ultimately return subpar results. Some databases do not include benign lesions such as blisters and warts; these lesions are managed by dermatologists daily, making it a very common occurrence in day-to-day diagnoses. This poses the threat of missing skin cancer among benign lesions, as they are not included in the datasets. According to [ 7 ], most of the online publicly available datasets consist of only raw images. This essentially means that most of the headway must be generated by the researchers themselves. To tackle the prevalent issue of an imbalance in the datasets, researchers have started employing data augmentation techniques such as cropping, rotation, and filtering, which, in turn, increases the number of training images the models can use [ 8 ]. While the datasets provide a rich source of information for the researchers, the absence of clarity in the metadata for various characteristics, such as ethnicity and skin types, inhibits the utility of clinical images [ 33 ]. The future of datasets and the furthering of improvement in AI-based diagnostic methods have already been set in motion in the form of open science, such as providing clinical decision support for diagnoses and screening. To overcome the problems faced in obtaining datasets to a greater extent, the adoption of open science must gain traction.
4.3. Patient Perspectives on Artificial Intelligence
Artificial Intelligence is assured to change the way patients interact with healthcare-associated processes, but it has remained elusive in terms of patients’ outlook and perspectives on AI in healthcare. The survey conducted in [ 106 ] aims to decipher the reception of Artificial Intelligence in healthcare by the patients. The central theme of most responses revolved around the diagnosis of the illness. This establishes a symbiotic relation between patients and the use of AI. While around 75% of the patients were keen to recommend AI in healthcare to family members, the apprehension of the remaining 25% is concerning. One way to tackle this is by ensuring that the response to conflict can be resolved by seeking a biopsy to complement the Artificial Intelligence decision-making. Apart from incorrect diagnoses, patients also are wary about their medical and clinical history being made available if used for datasets. Without properly labeled images, AI will be unable to train properly, leading to incorrect diagnosis results. Data analytics involving AI models for skin cancer diagnoses heavily use these labels to infer observations for future research [ 35 ]. By concealing the data, or submitting falsified information, this destroys the purpose of training an AI. This can be mitigated by ensuring that data collection organizations maintain non-disclosure policies.
4.4. Variation in Lesion Images
Skin cancer diagnosis using machine learning and deep learning involves multiple steps, out of which the primary step is skin lesion segmentation. As straightforward as it may seem, this task is commonly associated with setbacks that inhibit its smooth completion, namely the variations in lesion sizes, imprecise and indistinct boundaries, and different skin colors. The variation in different images, such as illumination difference, leads to uneven shadows and bright areas, making it tougher to segment the skin lesions [ 107 ]. Conventional algorithms such as CNNs and CNN-based approaches may perform superiorly in terms of labeling, but they still return a poor contrast between lesion and regular skin images. This is due to the deviations in the dataset, such as skin tone and aberrations, etc. The immense variation in datasets leads to the lack of inference drawn from the results, as suggested in [ 108 ]. A varying methodological quality shows higher amounts of specificity and sensitivity when compared to an expert’s diagnosis, thus rendering the use of AI in healthcare less useful. The corresponding diagnostic score and criteria for qualifying as an expert have been covered in a review that studies optical coherence while diagnosing adult skin cancer [ 108 ]. To tackle the problem of the varying characteristics of skin lesions, AI models must look to maximize their diversification and intensification [ 109 ]. By employing such mechanisms, the models can overcome the stagnation faced due to the increasing variation. In addition, the diagnosis of skin cancer in people of color is put off until advanced stages, due to the variations in lesion images that engender from the difference in skin tones [ 122 ]. Furthermore, due to socio-economic factors such as care barriers, models are not trained on different skin tones [ 122 ]. They develop an inherent bias towards the dominant skin tone and lesion color that the model has been trained on, which ultimately compromises the quality of the skin cancer diagnosis.
4.5. Dermatological Image Acquisition
Image acquisition in dermatology generally deals with close-up images of lesions or dermatosis. In most cases, the anatomical context of such images is lost due to the exclusion of surrounding structures, while the primary focus of the image is the lesion. Furthermore, with the rapid adoption of digital skin imaging applications, the utilization of smartphone-acquired images in dermatology have also increased proportionally [ 123 ]. While many studies have proposed methods to detect melanomas from inconsistent dermoscopy images, most of them produce localized results that cannot be used universally due to the acquisitive conditions they are trained on, such as isolated datasets and specific illumination conditions, etc. [ 124 ]. In addition to these problems, the quality of the acquired images is significantly compromised due to the varying illumination conditions during the acquisition phase, such as specular reflection. In [ 125 ], a generative adversarial neural network is proposed to deal with the persisting present problem of color inconsistency. The wider adoption of such methods, and an increase in the novelty of ideas that overcome erratic dermoscopy images, are required for overcoming the challenges faced by image acquisition.
4.6. Ethical and Legal Perspectives
While the use of AI in the clinical practice and healthcare domains has lots of upsides, it raises many ethical challenges. The use of health AI, albeit for transforming the patient–clinician relationship, carries ambiguity around the use of informed consent. The clinicians are unaware about the circumstances under which they should or should not inform the patient about AI being a part of the relationship [ 110 ]. AI decisions carry lots of weight, and they come with the challenges of safety and transparency. While it is understood that no AI model can be correct all the time, incorrect decisions can prove to be fatal, as well as mold correct decisions as unsafe. This gives rise to the concern around model algorithms’ fairness and bias. Models are trained on a particular set of data, making them biased to the characters they inferred from the dataset. It is virtually impossible for any dataset to exactly sample the world’s population and thus raises a flag for a cause for concern. AI technology has been identified to have a tremendous capability to threaten patient preference [ 111 ]. Parallelly, the use of health-related AI inevitably intersects with the law in more than one way. The question of how liability should be attributed in the case of harmful treatment is still unanswered. Similarly, AI bias against historically disadvantaged groups can attract anti-discrimination and human rights laws [ 112 ]. It is yet unknown whether the existing privacy laws can protect patients undergoing AI-based healthcare. It is necessary to understand that, while the potential benefits of AI in healthcare are plenty, it cannot be adopted for commercial use unless the ethical and legal challenges are responded to, as they serve as the bedrock of the entire system.
Figure 9 visualizes the open problems in skin cancer diagnosis using machine-learning- and deep-learning-based techniques.

Open challenges in skin cancer diagnosis.
5. Future Research Directions
5.1. combining ai with next-generation sequencing for refining skin cancer diagnosis.
Next-generation sequencing (NGS) technologies have progressed to facilitate the increase in data output and the efficiencies associated with it. NGS is categorized based on its respective read lengths [ 113 ]. NGS is used to determine the order of nucleotides in entire genomes or targeted regions in RNA and DNA. High-throughput DNA sequencing technology and methods have paved the way for commercializing new techniques [ 114 ]. The goal of DNA sequencing methods is to support speed while staying accurate, coupled with lower input rates of DNA and RNA input data. Squamous cell carcinoma (SCC) carries one of the highest tumor mutation burdens amongst all cancers. By employing the targeted next-generation sequencing of localized and metastatic high-risk SCCs, gene mutations can be compared with the intention of identifying key differences and improving targeted treatment alternatives [ 115 ]. Since the introduction of molecular tools, the discovery of new viruses, such as the papillomavirus, has been accelerated. NGS can be combined with improved protocols to help detect known and unknown human papillomaviruses [ 116 ].
5.2. AI-powered Automated Decision Support Systems for Skin Cancer Diagnosis
Decision support systems are computerized programs used to assist with decision-making and choosing the right course of action; Artificial-Intelligence-powered decision support systems can be used in the diagnosis of skin cancer. They provide options of flexibility in designing deep learning classification models by hinting at the common procedures and looping patterns [ 117 ]. Support systems can be initialized with pretrained deep neural network models combined with transfer learning to classify skin lesion localization and classification [ 118 ]. Present day decision support systems are fused with automated deep learning methods. These methods are fine-tuned and trained with the help of transfer learning using imbalanced data [ 119 ]. The model extracts the features using an average pooling layer, although the extracted features are not sufficient. By employing a modified genetic algorithm based on metaheuristics, relevant and significant features are extracted which can further be sent to a classifier that acts as a decision support system. As mentioned in [ 120 ], using AI-powered decision support systems can help clinicians diagnose and potentially replace invasive diagnostic techniques.
5.3. Smart Robotics for Skin Cancer Diagnosis
Robots can be used to improve the detection of skin cancer. Existing robots like Vectra WB360 combine 3D images with the corresponding sequential digital dermoscopic images, owing to the non-invasive tracking of melanoma and non-melanoma skin cancer. The photographic analysis of the WB360 allows for a global view of the skin surface and generates a body map to record the evolution of the lesions. This feature is very useful in detecting any suspicious developments promptly.
5.4. Wearable Computing for Skin Cancer Diagnosis
Wearable computing is a paradigm that involves the computation of accessories that can be worn by humans. Any small device capable of computing and processing data that can be worn on the body is categorized as a wearable computer. Wearable computers have been used in the field of cancer detection [ 121 ]. A few challenges that have not allowed for widespread clinical adoption yet are the high fragility, bendability, non-cooperative form factor of the sensors used, inappropriate connectivity, clinical inertia, and ultimately the awareness, as well as the cost, associated with wearable devices. Future research and corporations should aim to reduce the cost policy while tackling the challenges of using wearable computing as a vital alternative for cancer detection.
These event-driven tools are beneficial when concerning computational effectiveness, higher efficiency, power consumption, flexibility, and improved real-time performance. The possibility of incorporating these tools into wearable devices could be beneficial in terms of performance enhancement.
5.5. Teledermatology
Teledermatology is described as technology that supports healthcare from a distance [ 130 ]. Teledermatology aims to provide clinical services to patients, monitor their health, and provide resources through remote locations. This technology can be used for diagnosing, screening, and even managing skin cancer effectively. In total, three prospective solutions for implementing these are the store and forward method, real-time video conferencing, and a hybrid solution that includes both. At present, the store and forward method is widely in use with patients taking pictures and videos, which are then forwarded to the dermatologist. The ease of the convenience and the inexpensiveness make it a very popular choice. Real-time video conferencing uses the interaction between patient and physician through a video calling software to provide immediate advice. This simulates an in-person clinic experience, where the physician can verify medical data and history before prescribing anything. This method requires a stable internet connection and a high-quality video camera if it must be used for skin cancer. A low-quality camera may not fully capture the border and may eventually lead to erroneous diagnoses. The hybrid method mixes the advantages of both the methods: real-time video conferencing coupled with high-quality images sent to the dermatologist. Together, these serve as a beneficial way to consult and diagnose skin cancer. Teledermatology can be used with many machine learning and deep learning techniques to make the entire process much smoother. For instance, there are various CNN architectures which can be employed using transfer learning [ 131 ]. Doing so can help the dermatologist make decisions with a higher degree of confidence. Ensemble models can be incorporated into the pipeline for the store and forward method, enabling the system to be more accurate after the patient sends adequate-quality photos or videos.
Figure 10 illustrates the future directions for machine-learning- and deep-learning-enabled skin cancer diagnosis.

Future research directions of skin cancer diagnosis.
The internet of medical things (IoMT) and cloud computing will be the essential elements in upcoming mobile AI-powered healthcare-related decision support systems. In this framework, the event-driven tools can be beneficial when concerning computational effectiveness, real-time compression, data transmission efficiency, power consumption, and flexibility [ 135 , 136 , 137 ]. The possibility of incorporating these tools into contemporary mobile healthcare solutions can be investigated in the future.
6. Conclusions
A comprehensive survey is presented on machine learning and deep learning techniques, deployed for an automated skin cancer diagnosis. A comparison is made to the widely used skin cancer datasets and dominant studies. An insight discussion is had while exploring the lessons from prior works. Its aim is to set this survey as a benchmark for further studies in the field of skin cancer diagnosis by also including the limitations and benefits of the previous works. It is concluded that the Artificial Intelligence (AI)-based healthcare solutions come with many pre-requisites, dependencies and issues that must first be resolved before they can scale up. The AI research carries ethical and legal ambiguities, along with a lack of clinical data on all skin types, thereby inducing unintended bias in the model’s prediction. Moreover, although AI is gaining traction in the dermatology discipline, it still has lots of room to grow and enhance further in terms of the sensitivity, specificity, and accuracy of detecting the skin lesions. Additionally, dermatologists must take the first step in accepting and embracing AI, not as a threat to their professions, but as an ancillary tool to complement their diagnoses. While considering the challenges for implanting end-to-end AI-based solutions in healthcare, there are lots of prospects, promises, and challenges. Wearable computing and robotics are evolving, and AI healthcare can be incorporated into these recent innovations to ease the apprehensive market. While the limited available data suggest a parity between those who are keen on adopting AI healthcare, and those aversive towards it, the room to improve automated skin cancer detection has been established.
Acknowledgments
This work is technically supported by the Vellore Institute of Technology, Effat University, LINEACT CESI, Cracow University of Technology, and Polish Academy of Sciences. The authors are thankful to the Effat University, Cracow University of Technology and Polish Academy of Sciences for financially supporting this paper.
List of abbreviations used in this manuscript along with their full form.
Funding Statement
This research received no external funding.
Author Contributions
Conceptualization, N.M., K.S. and S.M.Q.; methodology, N.M., K.S. and S.M.Q.; implementation, N.M. and K.S.; validation, K.S., S.M.Q. and P.P.; investigation, N.M., K.S. and S.M.Q.; resources, K.S., S.M.Q. and P.P.; writing—original draft preparation, N.M. and K.S.; writing—review and editing, S.M.Q. and P.P.; visualization, N.M. and K.S.; supervision, K.S. and S.M.Q.; project administration, K.S., S.M.Q. and P.P.; funding acquisition, S.M.Q. and P.P. All authors have read and agreed to the published version of the manuscript.
Conflicts of Interest
Regarding the publication of this paper, the authors affirm that there are no conflict of interest.
Disclaimer/Publisher’s Note: The statements, opinions and data contained in all publications are solely those of the individual author(s) and contributor(s) and not of MDPI and/or the editor(s). MDPI and/or the editor(s) disclaim responsibility for any injury to people or property resulting from any ideas, methods, instructions or products referred to in the content.
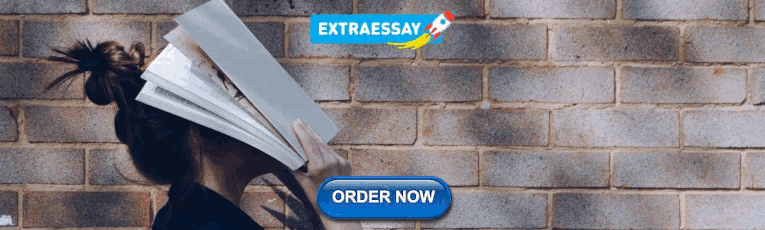
IMAGES
VIDEO
COMMENTS
This paper presents a detailed systematic review of deep learning techniques for the early detection of skin cancer. Research papers published in well-reputed journals, relevant to the topic of skin cancer diagnosis, were analyzed. Research findings are presented in tools, graphs, tables, techniques, and frameworks for better understanding.
This research paper introduces a comprehensive methodology to handle the complex issues related to skin cancer detection. This research presents a novel skin cancer recognition model based on ...
Skin cancer is one the most dangerous types of cancer and is one of the primary causes of death worldwide. The number of deaths can be reduced if skin cancer is diagnosed early. Skin cancer is mostly diagnosed using visual inspection, which is less accurate. Deep-learning-based methods have been proposed to assist dermatologists in the early and accurate diagnosis of skin cancers. This survey ...
This paper presents. a detailed systematic review of deep learning techniques for the early detection of skin cancer. Research papers published in well-reputed journals, relevant to the topic of ...
Studies suggest that in the area of skin cancer detection and image classification, there exists a plethora of research papers. A detailed survey of these methods is available in Refs. [1, 7, 8]. Each of these papers [1, 7, 8] used the then available state-of-art methods and claim performance improvements.
Identifying melanoma at the early stages of diagnosis is imperative as early detection can exponentially increase one's chances of cure. The paper first proposes a literature survey of multiple methods used for performing skin cancer classification. Our methodology consists of using Convolutional Neural Network (CNN) to identify and diagnose the skin cancer using the IS IC dataset containing ...
Recent advances in artificial intelligence (AI) in dermatology have demonstrated the potential to improve the accuracy of skin cancer detection. These capabilities may augment current diagnostic processes and improve the approach to the management of skin cancer. To explain this technology, we discuss fundamental terminology, potential benefits, and limitations of AI, and commercial ...
This paper presents a detailed systematic review of deep learning techniques for the early detection of skin cancer. Research papers published in well-reputed journals, relevant to the topic of skin cancer diagnosis, were analyzed. Research findings are presented in tools, graphs, tables, techniques, and frameworks for better understanding.
This paper presents a detailed systematic review of deep learning techniques for the early detection of skin cancer. Research papers published in well-reputed journals, relevant to the topic of skin cancer diagnosis, were analyzed. Research findings are presented in tools, graphs, tables, techniques, and frameworks for better understanding. ...
Through evaluation of research on skin cancer detection using ANN and CNN, the effectiveness and performance of these techniques in early and efficient diagnosis of skin cancer were established. ... ScienceDirect and Web of Science. 13 papers showed that skin cancer lesions were detected using CNN. Convolutional neural networks proved to ...
To avoid such incidents, there here has been exhaustive research conducted by the research community all over the world to build highly accurate automated tools for skin cancer detection. In this paper, we introduce a novel approach of combining machine learning and deep learning techniques to solve the problem of skin cancer detection.
Skin cancer is among the most common types of cancer, and quick identification considerably enhances the odds of survival. The purpose of this work is to develop cutting-edge deep learning models that can classify images of skin cells and accurately detect cases of skin cancer. The strength of deep learning algorithms is utilized in this research, which uses a cloud-based architecture. These ...
This article presents research on automated skin cancer detection using image processing and machine learning techniques. This paper focuses on melanoma, the deadliest form of skin cancer, and highlights the limitations of clinical observation. This study uses Convolutional Neural Networks (CNNs) and feature extraction to accurately classify skin cancers as malignant or benign. The proposed ...
to detect skin cancer and to distinguish benign skin cancer from melanoma. This paper presents a detailed systematic review of deep learning techniques for the early detection of skin cancer. Research papers published in well-reputed journals, relevant to the topic of skin cancer diagnosis, were analyzed. Research findings are presented in ...
This study allows us to recognize melanoma skin cancer. In this research paper, we've examined and reviewed many state of the art methods for the detection of skin cancer. ... Automatic skin cancer detection in dermoscopy images based on ensemble lightweight deep learning network. IEEE Access 8:99633-99647. Article Google Scholar Kwasigroch ...
In this paper, we have tried to evaluate the chance of deep learning algorithm namely Convolutional Neural Network (CNN) to detect skin cancer classifying benign and malignant mole. We have discussed recent studies that use different models of deep learning on practical datasets to develop the classification process. ... The dataset we use for ...
Challenges of skin cancer detection. There are some difficulties to detect skin cancer that can be attributed to variation in image types and sources (Al-Masni et al., 2018, Aljanabi et al., 2018). The variation in the appearance of human skin color makes skin cancer detection harder and complex (Naji, Jalab, & Kareem, 2019).
Researchers from the Karolinska Institute, the Karolinska University Hospital and the University of Nicosia contributed to the research. The study was funded by the National Institutes of Health (grants K24AR075060 and R01AR082109), Radiumhemmet Research, the Swedish Cancer Society and the Swedish Research Council.
The research includes a comparison on widely used datasets and prevalent review papers discussing skin cancer diagnosis using Artificial Intelligence. The authors of this study aim to set this review as a benchmark for further studies in the field of skin cancer diagnosis by also including limitations and benefits of historical approaches.