
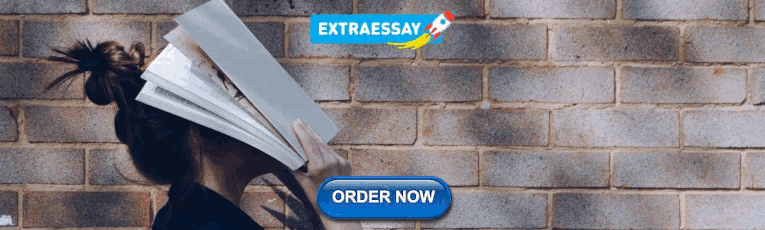
Systematic Review
- Library Help
- What is a Systematic Review (SR)?
Steps of a Systematic Review
- Framing a Research Question
- Developing a Search Strategy
- Searching the Literature
- Managing the Process
- Meta-analysis
- Publishing your Systematic Review
Forms and templates

Image: David Parmenter's Shop
- PICO Template
- Inclusion/Exclusion Criteria
- Database Search Log
- Review Matrix
- Cochrane Tool for Assessing Risk of Bias in Included Studies
• PRISMA Flow Diagram - Record the numbers of retrieved references and included/excluded studies. You can use the Create Flow Diagram tool to automate the process.
• PRISMA Checklist - Checklist of items to include when reporting a systematic review or meta-analysis
PRISMA 2020 and PRISMA-S: Common Questions on Tracking Records and the Flow Diagram
- PROSPERO Template
- Manuscript Template
- Steps of SR (text)
- Steps of SR (visual)
- Steps of SR (PIECES)
Adapted from A Guide to Conducting Systematic Reviews: Steps in a Systematic Review by Cornell University Library
Source: Cochrane Consumers and Communications (infographics are free to use and licensed under Creative Commons )
Check the following visual resources titled " What Are Systematic Reviews?"
- Video with closed captions available
- Animated Storyboard
- << Previous: What is a Systematic Review (SR)?
- Next: Framing a Research Question >>
- Last Updated: Mar 4, 2024 12:09 PM
- URL: https://lib.guides.umd.edu/SR
Have a language expert improve your writing
Run a free plagiarism check in 10 minutes, generate accurate citations for free.
- Knowledge Base
Methodology
- Systematic Review | Definition, Example, & Guide
Systematic Review | Definition, Example & Guide
Published on June 15, 2022 by Shaun Turney . Revised on November 20, 2023.
A systematic review is a type of review that uses repeatable methods to find, select, and synthesize all available evidence. It answers a clearly formulated research question and explicitly states the methods used to arrive at the answer.
They answered the question “What is the effectiveness of probiotics in reducing eczema symptoms and improving quality of life in patients with eczema?”
In this context, a probiotic is a health product that contains live microorganisms and is taken by mouth. Eczema is a common skin condition that causes red, itchy skin.
Table of contents
What is a systematic review, systematic review vs. meta-analysis, systematic review vs. literature review, systematic review vs. scoping review, when to conduct a systematic review, pros and cons of systematic reviews, step-by-step example of a systematic review, other interesting articles, frequently asked questions about systematic reviews.
A review is an overview of the research that’s already been completed on a topic.
What makes a systematic review different from other types of reviews is that the research methods are designed to reduce bias . The methods are repeatable, and the approach is formal and systematic:
- Formulate a research question
- Develop a protocol
- Search for all relevant studies
- Apply the selection criteria
- Extract the data
- Synthesize the data
- Write and publish a report
Although multiple sets of guidelines exist, the Cochrane Handbook for Systematic Reviews is among the most widely used. It provides detailed guidelines on how to complete each step of the systematic review process.
Systematic reviews are most commonly used in medical and public health research, but they can also be found in other disciplines.
Systematic reviews typically answer their research question by synthesizing all available evidence and evaluating the quality of the evidence. Synthesizing means bringing together different information to tell a single, cohesive story. The synthesis can be narrative ( qualitative ), quantitative , or both.
Here's why students love Scribbr's proofreading services
Discover proofreading & editing
Systematic reviews often quantitatively synthesize the evidence using a meta-analysis . A meta-analysis is a statistical analysis, not a type of review.
A meta-analysis is a technique to synthesize results from multiple studies. It’s a statistical analysis that combines the results of two or more studies, usually to estimate an effect size .
A literature review is a type of review that uses a less systematic and formal approach than a systematic review. Typically, an expert in a topic will qualitatively summarize and evaluate previous work, without using a formal, explicit method.
Although literature reviews are often less time-consuming and can be insightful or helpful, they have a higher risk of bias and are less transparent than systematic reviews.
Similar to a systematic review, a scoping review is a type of review that tries to minimize bias by using transparent and repeatable methods.
However, a scoping review isn’t a type of systematic review. The most important difference is the goal: rather than answering a specific question, a scoping review explores a topic. The researcher tries to identify the main concepts, theories, and evidence, as well as gaps in the current research.
Sometimes scoping reviews are an exploratory preparation step for a systematic review, and sometimes they are a standalone project.
Prevent plagiarism. Run a free check.
A systematic review is a good choice of review if you want to answer a question about the effectiveness of an intervention , such as a medical treatment.
To conduct a systematic review, you’ll need the following:
- A precise question , usually about the effectiveness of an intervention. The question needs to be about a topic that’s previously been studied by multiple researchers. If there’s no previous research, there’s nothing to review.
- If you’re doing a systematic review on your own (e.g., for a research paper or thesis ), you should take appropriate measures to ensure the validity and reliability of your research.
- Access to databases and journal archives. Often, your educational institution provides you with access.
- Time. A professional systematic review is a time-consuming process: it will take the lead author about six months of full-time work. If you’re a student, you should narrow the scope of your systematic review and stick to a tight schedule.
- Bibliographic, word-processing, spreadsheet, and statistical software . For example, you could use EndNote, Microsoft Word, Excel, and SPSS.
A systematic review has many pros .
- They minimize research bias by considering all available evidence and evaluating each study for bias.
- Their methods are transparent , so they can be scrutinized by others.
- They’re thorough : they summarize all available evidence.
- They can be replicated and updated by others.
Systematic reviews also have a few cons .
- They’re time-consuming .
- They’re narrow in scope : they only answer the precise research question.
The 7 steps for conducting a systematic review are explained with an example.
Step 1: Formulate a research question
Formulating the research question is probably the most important step of a systematic review. A clear research question will:
- Allow you to more effectively communicate your research to other researchers and practitioners
- Guide your decisions as you plan and conduct your systematic review
A good research question for a systematic review has four components, which you can remember with the acronym PICO :
- Population(s) or problem(s)
- Intervention(s)
- Comparison(s)
You can rearrange these four components to write your research question:
- What is the effectiveness of I versus C for O in P ?
Sometimes, you may want to include a fifth component, the type of study design . In this case, the acronym is PICOT .
- Type of study design(s)
- The population of patients with eczema
- The intervention of probiotics
- In comparison to no treatment, placebo , or non-probiotic treatment
- The outcome of changes in participant-, parent-, and doctor-rated symptoms of eczema and quality of life
- Randomized control trials, a type of study design
Their research question was:
- What is the effectiveness of probiotics versus no treatment, a placebo, or a non-probiotic treatment for reducing eczema symptoms and improving quality of life in patients with eczema?
Step 2: Develop a protocol
A protocol is a document that contains your research plan for the systematic review. This is an important step because having a plan allows you to work more efficiently and reduces bias.
Your protocol should include the following components:
- Background information : Provide the context of the research question, including why it’s important.
- Research objective (s) : Rephrase your research question as an objective.
- Selection criteria: State how you’ll decide which studies to include or exclude from your review.
- Search strategy: Discuss your plan for finding studies.
- Analysis: Explain what information you’ll collect from the studies and how you’ll synthesize the data.
If you’re a professional seeking to publish your review, it’s a good idea to bring together an advisory committee . This is a group of about six people who have experience in the topic you’re researching. They can help you make decisions about your protocol.
It’s highly recommended to register your protocol. Registering your protocol means submitting it to a database such as PROSPERO or ClinicalTrials.gov .
Step 3: Search for all relevant studies
Searching for relevant studies is the most time-consuming step of a systematic review.
To reduce bias, it’s important to search for relevant studies very thoroughly. Your strategy will depend on your field and your research question, but sources generally fall into these four categories:
- Databases: Search multiple databases of peer-reviewed literature, such as PubMed or Scopus . Think carefully about how to phrase your search terms and include multiple synonyms of each word. Use Boolean operators if relevant.
- Handsearching: In addition to searching the primary sources using databases, you’ll also need to search manually. One strategy is to scan relevant journals or conference proceedings. Another strategy is to scan the reference lists of relevant studies.
- Gray literature: Gray literature includes documents produced by governments, universities, and other institutions that aren’t published by traditional publishers. Graduate student theses are an important type of gray literature, which you can search using the Networked Digital Library of Theses and Dissertations (NDLTD) . In medicine, clinical trial registries are another important type of gray literature.
- Experts: Contact experts in the field to ask if they have unpublished studies that should be included in your review.
At this stage of your review, you won’t read the articles yet. Simply save any potentially relevant citations using bibliographic software, such as Scribbr’s APA or MLA Generator .
- Databases: EMBASE, PsycINFO, AMED, LILACS, and ISI Web of Science
- Handsearch: Conference proceedings and reference lists of articles
- Gray literature: The Cochrane Library, the metaRegister of Controlled Trials, and the Ongoing Skin Trials Register
- Experts: Authors of unpublished registered trials, pharmaceutical companies, and manufacturers of probiotics
Step 4: Apply the selection criteria
Applying the selection criteria is a three-person job. Two of you will independently read the studies and decide which to include in your review based on the selection criteria you established in your protocol . The third person’s job is to break any ties.
To increase inter-rater reliability , ensure that everyone thoroughly understands the selection criteria before you begin.
If you’re writing a systematic review as a student for an assignment, you might not have a team. In this case, you’ll have to apply the selection criteria on your own; you can mention this as a limitation in your paper’s discussion.
You should apply the selection criteria in two phases:
- Based on the titles and abstracts : Decide whether each article potentially meets the selection criteria based on the information provided in the abstracts.
- Based on the full texts: Download the articles that weren’t excluded during the first phase. If an article isn’t available online or through your library, you may need to contact the authors to ask for a copy. Read the articles and decide which articles meet the selection criteria.
It’s very important to keep a meticulous record of why you included or excluded each article. When the selection process is complete, you can summarize what you did using a PRISMA flow diagram .
Next, Boyle and colleagues found the full texts for each of the remaining studies. Boyle and Tang read through the articles to decide if any more studies needed to be excluded based on the selection criteria.
When Boyle and Tang disagreed about whether a study should be excluded, they discussed it with Varigos until the three researchers came to an agreement.
Step 5: Extract the data
Extracting the data means collecting information from the selected studies in a systematic way. There are two types of information you need to collect from each study:
- Information about the study’s methods and results . The exact information will depend on your research question, but it might include the year, study design , sample size, context, research findings , and conclusions. If any data are missing, you’ll need to contact the study’s authors.
- Your judgment of the quality of the evidence, including risk of bias .
You should collect this information using forms. You can find sample forms in The Registry of Methods and Tools for Evidence-Informed Decision Making and the Grading of Recommendations, Assessment, Development and Evaluations Working Group .
Extracting the data is also a three-person job. Two people should do this step independently, and the third person will resolve any disagreements.
They also collected data about possible sources of bias, such as how the study participants were randomized into the control and treatment groups.
Step 6: Synthesize the data
Synthesizing the data means bringing together the information you collected into a single, cohesive story. There are two main approaches to synthesizing the data:
- Narrative ( qualitative ): Summarize the information in words. You’ll need to discuss the studies and assess their overall quality.
- Quantitative : Use statistical methods to summarize and compare data from different studies. The most common quantitative approach is a meta-analysis , which allows you to combine results from multiple studies into a summary result.
Generally, you should use both approaches together whenever possible. If you don’t have enough data, or the data from different studies aren’t comparable, then you can take just a narrative approach. However, you should justify why a quantitative approach wasn’t possible.
Boyle and colleagues also divided the studies into subgroups, such as studies about babies, children, and adults, and analyzed the effect sizes within each group.
Step 7: Write and publish a report
The purpose of writing a systematic review article is to share the answer to your research question and explain how you arrived at this answer.
Your article should include the following sections:
- Abstract : A summary of the review
- Introduction : Including the rationale and objectives
- Methods : Including the selection criteria, search method, data extraction method, and synthesis method
- Results : Including results of the search and selection process, study characteristics, risk of bias in the studies, and synthesis results
- Discussion : Including interpretation of the results and limitations of the review
- Conclusion : The answer to your research question and implications for practice, policy, or research
To verify that your report includes everything it needs, you can use the PRISMA checklist .
Once your report is written, you can publish it in a systematic review database, such as the Cochrane Database of Systematic Reviews , and/or in a peer-reviewed journal.
In their report, Boyle and colleagues concluded that probiotics cannot be recommended for reducing eczema symptoms or improving quality of life in patients with eczema. Note Generative AI tools like ChatGPT can be useful at various stages of the writing and research process and can help you to write your systematic review. However, we strongly advise against trying to pass AI-generated text off as your own work.
If you want to know more about statistics , methodology , or research bias , make sure to check out some of our other articles with explanations and examples.
- Student’s t -distribution
- Normal distribution
- Null and Alternative Hypotheses
- Chi square tests
- Confidence interval
- Quartiles & Quantiles
- Cluster sampling
- Stratified sampling
- Data cleansing
- Reproducibility vs Replicability
- Peer review
- Prospective cohort study
Research bias
- Implicit bias
- Cognitive bias
- Placebo effect
- Hawthorne effect
- Hindsight bias
- Affect heuristic
- Social desirability bias
A literature review is a survey of scholarly sources (such as books, journal articles, and theses) related to a specific topic or research question .
It is often written as part of a thesis, dissertation , or research paper , in order to situate your work in relation to existing knowledge.
A literature review is a survey of credible sources on a topic, often used in dissertations , theses, and research papers . Literature reviews give an overview of knowledge on a subject, helping you identify relevant theories and methods, as well as gaps in existing research. Literature reviews are set up similarly to other academic texts , with an introduction , a main body, and a conclusion .
An annotated bibliography is a list of source references that has a short description (called an annotation ) for each of the sources. It is often assigned as part of the research process for a paper .
A systematic review is secondary research because it uses existing research. You don’t collect new data yourself.
Cite this Scribbr article
If you want to cite this source, you can copy and paste the citation or click the “Cite this Scribbr article” button to automatically add the citation to our free Citation Generator.
Turney, S. (2023, November 20). Systematic Review | Definition, Example & Guide. Scribbr. Retrieved March 21, 2024, from https://www.scribbr.com/methodology/systematic-review/
Is this article helpful?
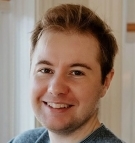
Shaun Turney
Other students also liked, how to write a literature review | guide, examples, & templates, how to write a research proposal | examples & templates, what is critical thinking | definition & examples, "i thought ai proofreading was useless but..".
I've been using Scribbr for years now and I know it's a service that won't disappoint. It does a good job spotting mistakes”
How to Do a Systematic Review: A Best Practice Guide for Conducting and Reporting Narrative Reviews, Meta-Analyses, and Meta-Syntheses
Affiliations.
- 1 Behavioural Science Centre, Stirling Management School, University of Stirling, Stirling FK9 4LA, United Kingdom; email: [email protected].
- 2 Department of Psychological and Behavioural Science, London School of Economics and Political Science, London WC2A 2AE, United Kingdom.
- 3 Department of Statistics, Northwestern University, Evanston, Illinois 60208, USA; email: [email protected].
- PMID: 30089228
- DOI: 10.1146/annurev-psych-010418-102803
Systematic reviews are characterized by a methodical and replicable methodology and presentation. They involve a comprehensive search to locate all relevant published and unpublished work on a subject; a systematic integration of search results; and a critique of the extent, nature, and quality of evidence in relation to a particular research question. The best reviews synthesize studies to draw broad theoretical conclusions about what a literature means, linking theory to evidence and evidence to theory. This guide describes how to plan, conduct, organize, and present a systematic review of quantitative (meta-analysis) or qualitative (narrative review, meta-synthesis) information. We outline core standards and principles and describe commonly encountered problems. Although this guide targets psychological scientists, its high level of abstraction makes it potentially relevant to any subject area or discipline. We argue that systematic reviews are a key methodology for clarifying whether and how research findings replicate and for explaining possible inconsistencies, and we call for researchers to conduct systematic reviews to help elucidate whether there is a replication crisis.
Keywords: evidence; guide; meta-analysis; meta-synthesis; narrative; systematic review; theory.
- Guidelines as Topic
- Meta-Analysis as Topic*
- Publication Bias
- Review Literature as Topic
- Systematic Reviews as Topic*

University Libraries
- Ohio University Libraries
- Library Guides
Evidence Synthesis Methods including Systematic Reviews (SRs)
- Timeline & Typical Workflow
- Home: SR Services & Intro
- Types of Reviews
- Project Management Resources
- Search Strategy Repositories
- Training Opportunities
- Scholarly Research Impact Guide
- Contact/Need help
- Protocol Agencies
- Study Appraisel Tools
- Cochrane Handbook
- PRISMA Checklist and scoping review information
- Joanna Briggs
- PROSPERO Register your health/medicine systematic review here
- Open Science Framework Register your non-health/medical systematic review here
- Campbell Collaboration Education, policy, and social sciences systematic reviews
- PRESS Peer Review of Electronic Search Strategies
- GRADE Grading of Recommendations Assessment, Development and Evaluation
- CASP Checklists
- EQUATOR Network Reporting guidelines for many study types, including qualitative studies.
Common Misconcetpions
- Time! This is at least a year-long process. Roughly 400 hours of work.
- You need at least two people, three would be better, on your research team.
- A systematic review may not be the appropriate methodology for you.
- You may be sifting through over 4,000 records.
- There are standards and guidelines that must be followed. Quality control.
- Librarians are masters at search strategies and manipulating databases; which is where you will be getting data for your study.
Protocols, guidelines, agencies, oh my!
The reason why systematic reviews are so highly respected and sought after is because they are rigorous, reproducible, transparent, and have standards. But these characteristics are only true if those standards/guidelines are followed.
Not all systematic reviews are created equally. Make sure yours is top notch so you do not perpetuate the problem.
Definitions:
- Guidelines : How to do systematic reviews or similar reviews. The rules and expectations; directions.
- Agency : Systematic review (or similar reviews: i.e. scoping review) authority. A group who creates guidelines and/or manages protocols. Cochrane is a good example or the Joanna Briggs Institute.
- Protocols : This is the map to your specific study that you create at the beginning of the process. In order to do a systematic review well, you must create a protocol with your research team which includes: the types of studies you will be gathering, what resources you will be searching, inclusion/exclusion criteria, etc. All of this information is often included when you register your review with an agency.
- Appraisal : If you are doing a traditional systematic review, you will need to appraise the studies to ensure they are good enough to include as your data. Good stuff in, good stuff out.
- ROBIS Tool Use to check your methodology for bias.
Preperation:
- Look to other systematic reviews that have been completed to compare.
- Who is on your research team? (2+ people)
- Locate the appropriate guidelines for said review type.
- Review PRISMA checklist for
- This will include the databases you will be searching, study inclusion/exclusion rules, etc.
- Check other registries (PROSPERO, Cochrane, etc.) to ensure no one else is doing this study.
- Register your review.
- Team needs to agree on citation manager that will be used, if team will be using an abstract tracking software, and where you wil be storing all of your shared documentation.
Timeline for a typical systematic review, provided by Cochrane's Handbook.
- << Previous: Types of Reviews
- Next: Project Management Resources >>
- Subject guides
- Systematic Review
- Getting started
Systematic Review: Getting started
- Manuals, documentation & PRISMA
- Develop question & key concepts
- Look for existing reviews
- Scoping searches & gold set
- Identify search terms
- Select databases & grey literature sources
- Develop criteria & protocol
- Run your search
- Limits & filters
- Review & test your search
- Save & manage your search results
- Database search translation
- Screening process steps
- Assess quality of your included studies
- Request a consultation
What is a systematic review?
We recommend that you closely follow the steps on this guide to create your systematic review. A systematic review (SR) is a type of literature review . Unlike other forms of review, where authors can include any articles they consider appropriate, a systematic review aims to remove the reviewer's bias as far as possible by following a clearly defined, transparent process. This Cochrane video gives a clear summary, but you should find examples and methodologies for your specific discipline as there are various approaches to systematic reviews that differ from those in Medicine.
Systematic review workflow
Before embarking on a systematic review
The production of SRs has been prolific. Since the mid-nineties published SRs have increased by 4676% (Brackett & Batten, 2020) , but concerns are held for their quality and usefulness. It is important to consider the following:
- Have you checked if an SR already exists on your topic? Check for protocols and published reviews.
- Do you have adequate time and resources, to commit around 12 months to the review?
- SRs should not be undertaken by just one person. Cochrane recommends multidisciplinary teams work best.
- To ensure rigour, follow established standards and guidelines.
- Is an SR the right review type for your topic and/or research question? You may find this decision tree helpful.
- Familiarise yourself with various review types by reading widely eg. (Sutton et al., 2019) .
- Broaden your knowledge about systematic reviews further, by reading as much as you can about the process and find examples of good reviews eg. (Bastian, 2021) .
Systematic review online courses
- Systematic reviews and meta-analysis: open and free Campbell Collaboration online course Systematic Reviews and Meta-Analysis: A Campbell Collaboration Online Course provides an overview of the steps involved in conducting a systematic (scientific) review of results of multiple quantitative studies. These steps include: problem formulation, searching for relevant literature, screening potentially eligible studies, coding and critically appraising studies, synthesizing results across studies using meta-analysis, reporting and disseminating results, and updating or re-analysis of data.
- << Previous: Home
- Next: Manuals, documentation & PRISMA >>
MSU Libraries
- Need help? Ask a Librarian
Systematic & Advanced Evidence Synthesis Reviews
- Our Services
- Choosing A Review Type
- Conducting A Review
- Systematic Reviews
- Scoping & Other Types of Advanced Reviews
Online Toolkits & Workbooks
Search strategies and citation chaining, citation management, deduplication, bibliography creation, and cite-while-you-write, screening results, creating prisma compliant flow charts, data analysis & abstraction, total workflow sr products, writing a manuscript.
- Contact Your Librarian For Help
This page lists commonly used software for Systematic Review's (SRs) and other advanced evidence synthesis reviews and should not be taken as MSU Libraries endorsing one program over another. The sections of the guide list fee-based as well as free and open-source software for different aspects of the review workflow. All-inclusive workflow products are listed in this section.
- Wanner, Amanda. 2019. Getting started with your systematic or scoping review: Workbook & Endnote Instructions. Open Science Framework. This is a librarian created workbook on OSF that includes a pretty comprehensive workbook that walks you through all the steps and stages of creating a systematic or scoping review.
- What review is right for you? This tool is designed to provide guidance and supporting material to reviewers on methods for the conduct and reporting of knowledge synthesis. As a pilot project, the current version of the tool only identifies methods for knowledge synthesis of quantitative studies. A future iteration will be developed for qualitative evidence synthesis.
- Systematic Review Toolkit The Systematic Review Toolbox is a web-based catalogue of tools that support various tasks within the systematic review and wider evidence synthesis process. The toolbox aims to help researchers and reviewers find the following: Software tools, Quality assessment / critical appraisal checklists, Reporting standards, and Guidelines.
It is highly recommended that researchers partner with the academic librarian for their specialty to create search strategies for systematic and advanced reviews. Many guidance organizations recognize the invaluable contributions of information professionals to creating search strategies - the bedrock of synthesis reviews.
- Visualising systematic review search strategies to assist information specialists
- Gusenbauer, M., & Haddaway, N. R. (2019). Which Academic Search Systems are Suitable for Systematic Reviews or Meta‐Analyses? Evaluating Retrieval Qualities of Google Scholar, PubMed and 26 other Resources. Research Synthesis Methods.
- Citation Chaser Forward citation chasing looks for all records citing one or more articles of known relevance; backward citation chasing looks for all records referenced in one or more articles. This tool automates this process by making use of the Lens.org API. An input article list can be used to return a list of all referenced records, and/or all citing records in the Lens.org database (consisting of PubMed, PubMed Central, CrossRef, Microsoft Academic Graph and CORE)
How do you track your integration and resourcing for projects that require systematic searching, like systematic or scoping reviews? What, where, and how should you be tracking? Using a tool like Air Table can help you stay organized.
- Airtable for Systematic Search Tracking Talk from Whitney Townsend at the University of Michigan - April 6, 2022
Having a software program that can store citations from databases, deduplicating your results, and automating the creation and formatting of citations and a bibliography using a cite-while-you-write plugin will save a lot of time when doing any literature review. The software listed below can do all of these functions which are not found in the fee-based total systematic review workflow products.
You could also do most of the components of an SR in these software including screening. Screening is easiest done in F1000 Workspace and the desktop version of Endnote because of their ability to share a library with a group of people and nested folder structures.
- Endnote Guide Endnote Online is free and has basic functionality like importing citations and cite-while-you-write for Microsoft Word. The desktop version of Endnote is a separate individual purchase and is more robust then the online version particularly for organization of citations and ease of use with large citation libraries.
- Mendeley Guide Mendeley has all the standard features of a citation manager with the addition of a social community of scholars. Mendeley can be sluggish with large file sizes of multiple thousands of citations and the free version has limited collaborative features.
Screening the titles, abstracts, and full text of your results is one of the most time consuming components of any review. There are easy-to-use free software for this process but they won't have features like automatically creating the flow charts and inter-rater reliability kappa coefficient that you need to report in your methodology section. You will have to do this by hand.
Deduplication of results before importation into one of these tools and screening should be done in a citation management program like Endnote, Mendeley, or Zotero.
- Abstrackr Created by Brown University, Abstrackr is one of the best known and easiest to use free tools for screening results.
- Colandr Colandr is an open source screening tool. Like Abstrackr, deduplication is best done in a citation manager and then results imported into Colandr. There is a learning curve to this tool but a vibrant user community does exist for troubleshooting.
- Rayyan Built by the Qatar Foundation. It is a free web-tool (Beta) designed to help researchers working on systematic reviews and other knowledge synthesis projects. It has simple interface and a mobile app for screening-on-the-go.
- PRISMA Diagram Generator Using the PRISMA Diagram Generator you can produce a diagram easily in any of 10 different formats. The official PRISMA website only has the format as a .docx or .pdf option. Using the generator the diagram is produced using the Open Source dot program (part of graphviz), and this tool provides the source for your diagram if you wish to further tweak your diagram.
- PRISMA 2020: R Package and ShinyApp This free, online tool makes use of the DiagrammeR R package to develop a customisable flow diagram that conforms to PRISMA 2020 standards. It allows the user to specify whether previous and other study arms should be included, and allows interactivity to be embedded through the use of mouseover tooltips and hyperlinks on box clicks.
Tools for data analysis can help you categorize results such as outcomes of studies and perform metanalyses. The SRDR tool may be the easiest to use and has frequent functionality updates.
- OpenMeta[Analyst] Developed by Brown University using an AHRQ grant, OpenMeta[Analyst] is a no-frills approach to data analysis.
- SRDR Developed by the AHRQ, The Systematic Review Data Repository (SRDR) is a powerful and easy-to-use tool for the extraction and management of data for systematic review or meta-analysis. It is also an open and searchable archive of systematic reviews and their data.
Data abstraction commonly refers to the extraction, synthesis, and structured visualization of evidence characteristics. Evidence tables/table shells/reading grids are the core way article extraction analyses are displayed. It lists all the included data sources and their characteristics according to your inclusion/exclusion criteria. Tools like Covidence have modules to create your own data extraction form and export a table when finished.
- OpenAcademics: Reading Grid Template
- The National Academies Press: Sample Literature Table Shells
- Campbell Collaboration: Data Extraction Tips
There are several fee-based products that are a one-stop-shop for systematic reviews. They complete all the steps from importing citations, deduplicating results, screening, bibliography management, and some even perform metanalyses. These are best used by teams that have grant or departmental funding because they can be rather expensive.
None of these tools offers a robust bibliography creation function or cite-while-you write option. You will still need to use a separate citation manager to do these aspects of review writing. We list commonly used citation management tools on this page.
- EPPI-Reviewer 4 EPPI-Reviewer 4 is a web-based software program for managing and analysing data in literature reviews. It has been developed for all types of systematic review (meta-analysis, framework synthesis, thematic synthesis etc) but also has features that would be useful in any literature review. It manages references, stores PDF files and facilitates qualitative and quantitative analyses such as meta-analysis and thematic synthesis. It also contains some new ‘text mining’ technology which is promising to make systematic reviewing more efficient. It also supports many different analytic functions for synthesis including meta-analysis, empirical synthesis and qualitative thematic synthesis. It does not have a bibliographic manager or cite-while-you-write feature.
- JBI-SUMARI Currently, this tool can only accept Endnote XML files for citation import. So you would need to download citations to Endnote, import them into SUMARI, and when screening is complete use Endnote as your bibliographic manager for any writing. SUMARI supports 10 review types, including reviews of effectiveness, qualitative research, economic evaluations, prevalence/incidence, aetiology/risk, mixed methods, umbrella/overviews, text/opinion, diagnostic test accuracy and scoping reviews. It facilitates the entire review process, from protocol development, team management, study selection, critical appraisal, data extraction, data synthesis and writing your systematic review report.
Using Excel
Some teams may choose to use Excel for their systematic review. This is not recommended because it can be extremely time consuming and is more prone to error. However, there is a basic template for Excel-based SR's online that is good quality and walks one through the entire workflow of completing an SR (excluding bibliography creation and citation management).
- PIECES Workbook This link will open an Excel workbook designed to help conduct, document, and manage a systematic review. Made by Margaret J. Foster, MS, MPH, AHIP Systematic Reviews Coordinator Associate Professor Medical Sciences Library, Texas A&M University
- Systematic Review Accelerator: Methods Wizard An tool to help you write consistent, reproducible methods sections according to common reporting structures.
- PRISMA Extensions Each PRISMA reporting extension has a manuscript checklist that lays out exactly how to write/report your review and what information to include.
- << Previous: Scoping & Other Types of Advanced Reviews
- Next: Contact Your Librarian For Help >>
- Last Updated: Feb 8, 2024 8:23 AM
- URL: https://libguides.lib.msu.edu/systematic_reviews

Systematic Reviews
What is a systematic review, sr workflow visualization, want to learn more.
- Talk with a Librarian
- Use recommended guidelines
- Develop Preliminary Research Question
- Develop Preliminary Team
- SR already available?
- Do You Have the Time?
- Workflow Management Tools
- SR Not the Right Fit? What then?
- Moving Forward with a/n SR
- Search Tools
- Search Strategy
- Screening and Selection
- Additional Resources & Reading
A systematic review is a comprehensive review of the literature conducted by a research team using systematic and transparent methods in accordance with reporting guidelines to answer a well-defined research question. It aims to identify and synthesize scholarly research published in commercial and/or academic sources as well as in grey (or gray) literature produced by individuals or organizations in order to reduce bias and provide all available evidence for informing practice and policy-making. Systematic reviews may also include a meta-analysis, a more quantitative process of synthesizing and visualizing data retrieved from various studies.
- Systematic Review Workflow This image provides a snapshot of the process involved in a systematic review.
Tsafnat, G., Glasziou, P., Choong, M.K. et al. Systematic review automation technologies . Syst Rev 3, 74 (2014). https://doi.org/10.1186/2046-4053-3-74
There are two options to engage:
1. Have you read through the other parts of this guide, but feel you just want to talk to someone about your ideas and this process? Please contact the Research Impact Team to set up a general consultation.
2. If you have a research plan developed already and you would like to include a librarian on your team, review the "Talk with a Librarian" tab and submit a proposal as directed.
- Next: Talk with a Librarian >>
- Last Updated: Jan 31, 2024 10:46 PM
- URL: https://researchguides.library.syr.edu/SR
- Print This Page
How to: systematic review literature searching
Searching literature is one of the most important elements of a systematic review. A well planned search strategy in the right databases ensures you have a robust list of results to whittle down as part of your PRISMA workflow. We’ve answered some of the common questions we get asked about searching literature as part of a systematic review. Take a look – have we missed anything?
How do you do a literature search for a systematic review?
The literature search element of a systematic review is the next step after you’ve created a well-defined question that the systematic review itself is trying to answer.
Best-practice is to perform your literature search across at least three separate databases. The two most common are Embase and MEDLINE, with the third (and fourth and fifth!) varying depending on the subject area of your systematic review. Additional databases can include sources of grey literature like ClinicalTrials.gov or databases of systematic reviews such as Cochrane (with a lot of Cochrane content being available in both Embase and MEDLINE).
Your literature search needs to use a well-constructed and thorough search strategy. Following a framework like PICO (Problem, Intervention, Comparison, Outcome) or SPIDER (Sample, Phenomenon of Interest, Design, Evaluation, Research type) can help you ensure you’re not missing anything. It can also help to use a database search platform that allows you to save and edit your searches. This can make it easier to demonstrate your search strategy is truly reproducible and exhaustive.
Your search strategy will also benefit from using Embase and MEDLINE’s thesauri to refine your search keywords. Using medical synonyms (like those built into Dialog ) can also be helpful, both for saving your time and ensuring you’re not missing out on any common synonyms for your search terms.
And as a final point, make sure you’re able to refine your results consistently across all the databases you’re using. This is easier if you’re using a single platform to search multiple databases as you can limit date ranges, publications, author, etc. with a single click, rather than trying to do this consistently across individual databases.
What databases are used for systematic reviews?
The databases you choose for your systematic review literature search should be as extensive as possible to avoid any potential publication bias. At the very least, most people agree that MEDLINE and Embase have to be included within your systematic review literature search. You should also choose specific databases related to the topic of your systematic review question (such as PsycINFO for psychological-focused studies or ESPICOM for systematic reviews looking at medical devices). Cochrane also recommends you include its Central Register of Controlled Trials (CENTRAL) database in your search if you want your systematic review to eventually appear in CENTRAL.
It is also a good idea to use grey literature within your list of sources. Some useful databases for grey literature and pre-print content include the U.S. National Library of Medicine’s ClinicalTrials.gov, ProQuest Dissertations and Theses and the Morressier database of conference posters.
What is PRISMA for systematic reviews?
PRISMA stands for Preferred Reporting Items for Systematic Reviews and Meta-Analyses and is a set of guidelines designed to improve the quality of systematic reviews.
PRISMA includes a 27 item checklist that covers everything from the report title and abstract to declaring competing interests and support (you can access it on PRISMA’s website here ). There is also a PRISMA workflow that can help narrow down the results of your systematic review literature searches in an objective and repeatable way (access the flowchart on PRISMA’s website here ).
How many databases should be searched for a systematic review?
At least three databases should be used for systematic review literature searches. As explained above, the most common are Embase and MEDLINE, with the third database being chosen based on the subject area of the systematic review.
Additional databases can be used to provide sources of grey literature, such as pre-print working papers, dissertations and theses and conference papers and posters.
What is grey literature for a systematic review?
Grey literature is any content that is considered unpublished in the sense it doesn’t appear through traditional publishing channels (i.e. peer-reviewed databases). As a result grey literature covers a very wide range of content types and sources and can include:
- Conference posters
- Conference abstracts and proceedings
- Pre-print / working papers
- Dissertations and theses
- Clinical studies
Can you include grey literature in a systematic review?
Grey literature can be a very important resource in systematic reviews as it can provide extra evidence and sources outside of the main databases and journals. While it can be harder to find grey literature (unless you’re searching databases that specifically contain grey literature content), it can help a systematic review be more balanced and thorough.
The main drawback with grey literature is ensuring the quality and objectivity of the content, as it won’t necessarily have been reviewed in the same way as content from the main publication sources.
How do you find grey literature for a systematic review?
There are a number of databases that contain grey literature, including ProQuest Dissertations & Theses, Publicly Available Content, ClinicalTrials.gov and Morressier. If you’re doing a systematic review literature search on Dialog these databases can be added alongside MEDLINE and Embase as part of your search process.
How do you develop a search term for a systematic review?
To develop a search strategy for a systematic review, we recommend the following approach:
- i.e. Can antibiotics help alleviate the symptoms of a sore throat?
- Choose the most appropriate databases (MEDLINE, Embase and SciSearch, for example) and identify the most cost-effective and efficient way to search and access this content (such as a database search platform like Dialog)
- P opulation/ P roblem – sore throat
- I ntervention – antibiotics
- C omparison – e.g. anti-inflammatories, placebo
- O utcomes – alleviation, therapeutic effect
- Then decide what content you will include and exclude
- Use thesauri to find preferred terms and explosions in MEDLINE and Embase
- Use thesaurus subheadings to refine terms in MEDLINE and Embase
- Use medical synonyms to increase recall of free text terms
Related posts
Unlocking u.k. research excellence: key insights from the research professional news live summit.
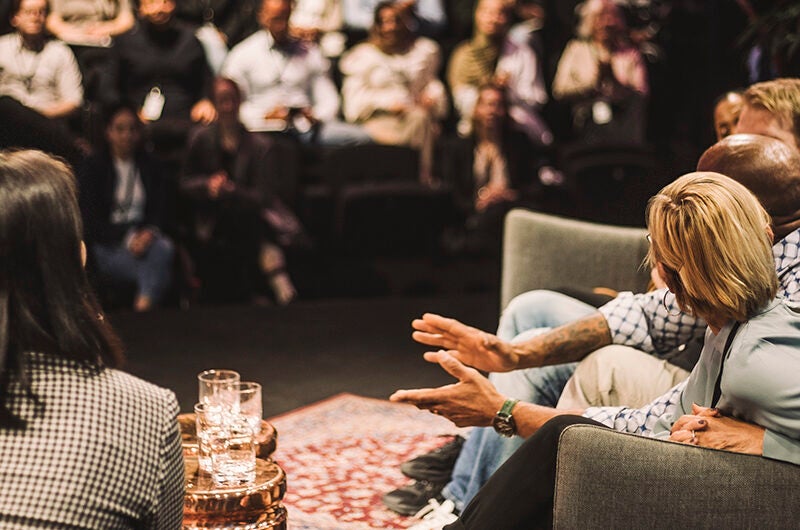
Rebates are likely driving U.S. payer coverage of GLP-1 agonists
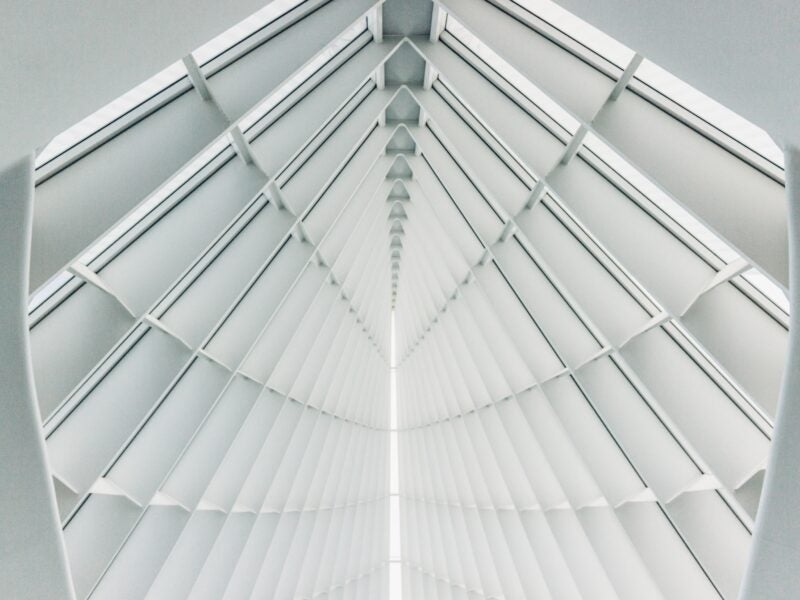
For better insights, assess research performance at the department level
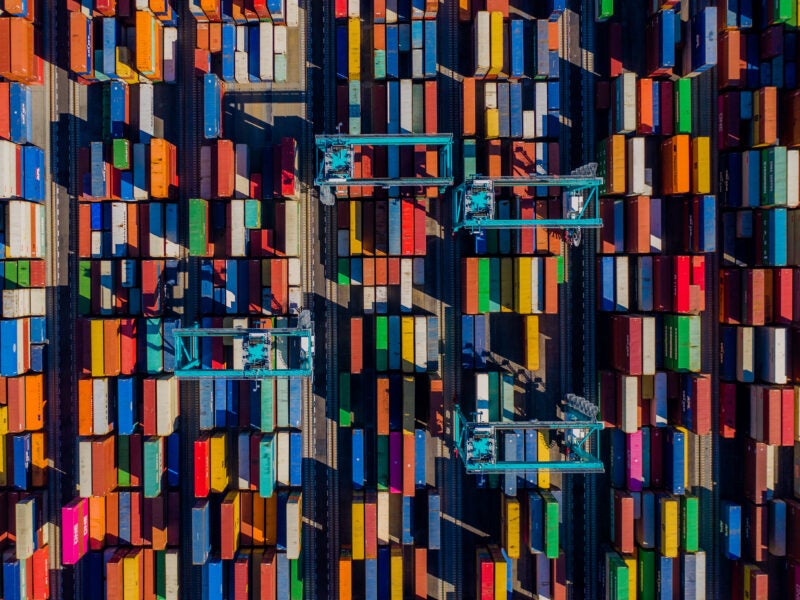
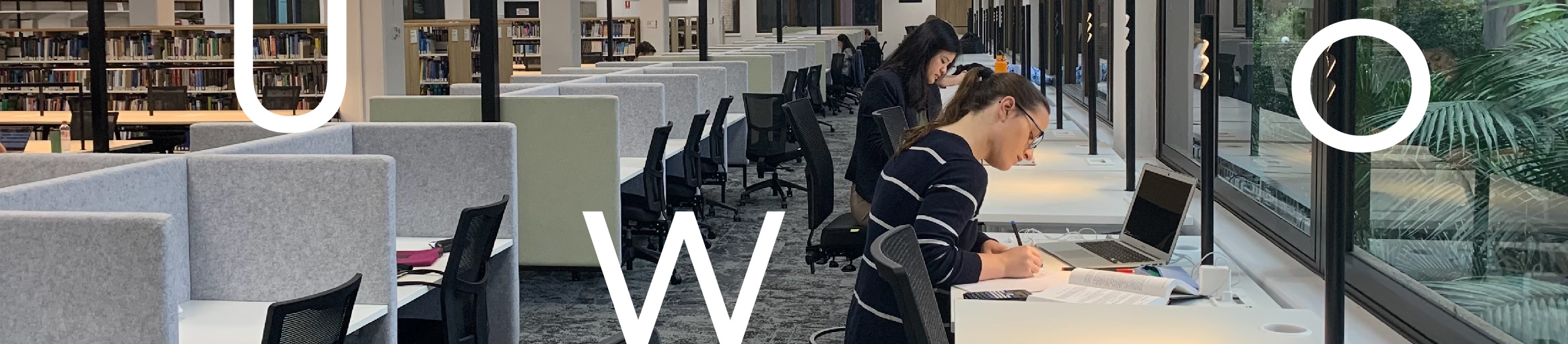
Help us improve our Library guides with this 5 minute survey . We appreciate your feedback!
- UOW Library
- Key guides for researchers
Systematic Review
- What is a systematic review?
- Five other types of systematic review
- How is a literature review different?
- Search tips for systematic reviews
- Controlled vocabularies
- Grey literature
- Transferring your search
- Documenting your results
- Support & contact
Frameworks for systematic reviews
Using a framework to structure your research question will assist you to structure the entire process - determine the scope of your review, provide a focus while searching for literature, help identify key concepts and guide your selection of papers for inclusion.
PICO framework
Use a framework like PICO when developing a good clinical research question:
PICO examples from the Cochrane Library
- Log into the Cochrane Library
- Enter your UOW username and password
- From the home page click “Advanced Search”
- Click on the “PICO search” tab and search for your topic
- Click on “Run search”
- Choose a review and click on the “ShowPICOs” drop-down menu
- You will then see the PICO attached to the systematic review.
PICO searching on Medline
- Advanced searching in Medline.
- Understanding Focus and Explode.
- Conduct a search strategy using the PICO framework.
- Searching using Medical Subject Headings.
- PICO Searches and Systematic Reviews (Academic skills and study support)
- Describes PICO Searches and systematic literature reviews.
PRISMA is an evidence-based minimum set of items for reporting in systematic reviews and meta-analyses.
PRISMA Checklist The 27 checklist items relate to the content of a systematic review and meta-analysis, which includes:
A PRISMA extension for scoping reviews, PRISMA-ScR , has been created to provide reporting guidance for this specific type of review. This extension is also intended to apply to evidence maps, as these share similarities with scoping reviews and involve a systematic search of a body of literature to identify knowledge gaps.
The PRISMA extension for scoping reviews contains 20 essential reporting items and 2 optional items to include when completing a scoping review. Scoping reviews serve to synthesize evidence and assess the scope of literature on a topic. Among other objectives, scoping reviews help determine whether a systematic review of the literature is warranted.
The SPIDER question format was adapted from the PICO tool to search for qualitative and mixed-methods research. Questions based on this format identify the following concepts:
- P henomenon of I nterest
- E valuation
- R esearch type.
Defining your question for finding qualitative research: SPIDER tool
Example: What are young parents’ experiences of attending antenatal education?
Search for ( S AND P of I AND ( D OR E ) AND R ) (Cooke, Smith, & Booth, 2012).
"Beyond PICO: the SPIDER tool for qualitative evidence synthesis" (Cooke, Smith, & Booth, 2012)
- Previous: Tools
- Next: Support & contact
- Last Updated: Feb 22, 2024 12:34 PM
- URL: https://uow.libguides.com/systematic-review
Insert research help text here
LIBRARY RESOURCES
Library homepage
Library SEARCH
A-Z Databases
STUDY SUPPORT
Learning Co-Op (academic skills support)
Referencing and citing
Digital Skills Hub
MORE UOW SERVICES
UOW homepage
Student support and wellbeing
IT Services
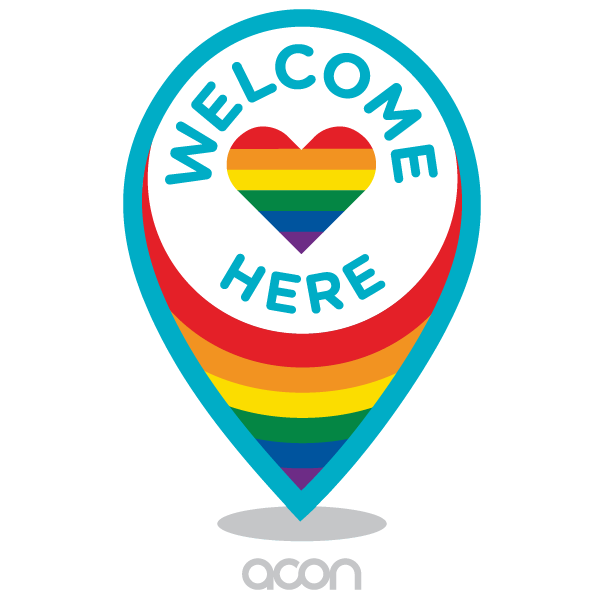
On the lands that we study, we walk, and we live, we acknowledge and respect the traditional custodians and cultural knowledge holders of these lands.
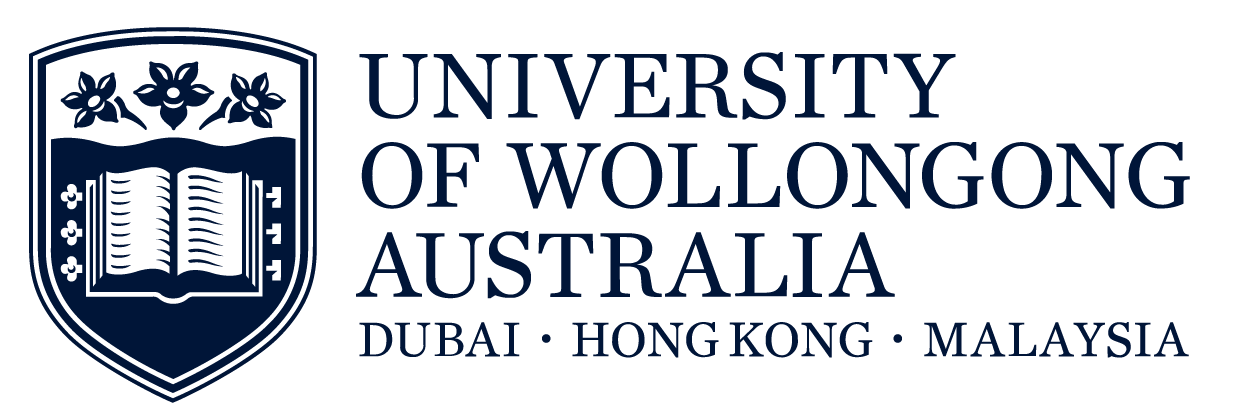
Copyright & disclaimer | Privacy & cookie usage

Systematic Reviews: Automating Your Workflows With DistillerSR
by Madhu Vijayakumar | Jun 19, 2023
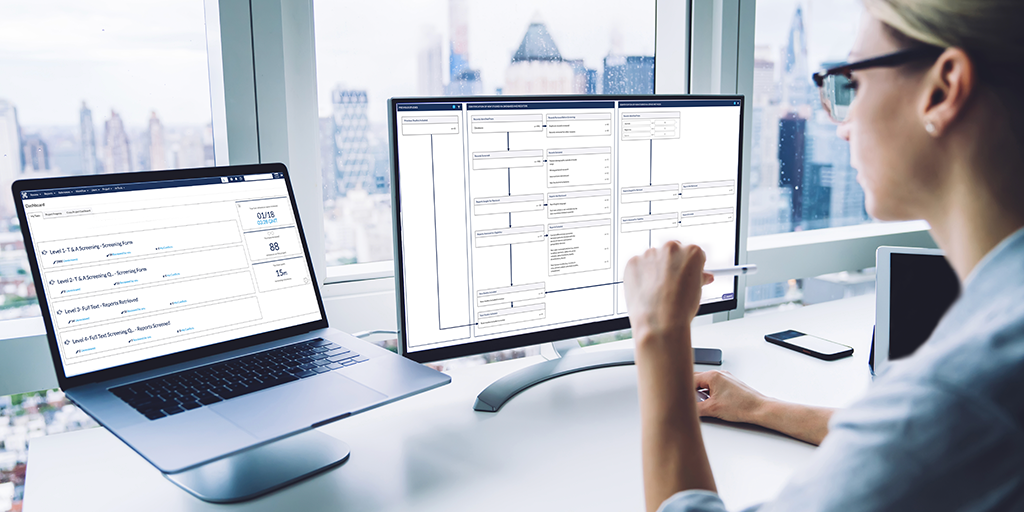
Collecting, evaluating and managing evidence is the most crucial part of research that drives policy and regulates medical technologies, and Systematic Reviews are the cornerstone of evidence-based research. A Systematic Review may contain tens of thousands of articles and papers and each of them may contain hundreds of data points. Given the growing volume of literature available for reviewers, using traditional methods to conduct Systematic reviews can be taxing.
Research conducted needs to be auditable, reproducible and stand up to scrutiny by relevant departments or Regulatory Bodies. Adding on to this, reviews are usually conducted on strict budgets, so reviewers need to be mindful of avoiding duplicate work or errors which may prove costly for the organization.
To overcome the above challenges, libraries, medical journals and experts swear by setting up a protocol to define the rationale, hypothesis, and methods of the project before the review has begun to streamline your systematic reviews. But how can you execute them in practice?
100% Configurable Workflows That Work The Way You Do
Systematic Reviews are generally conducted by teams which may consist of reviewers with varying levels of expertise working in collaboration, possibly from different geographical locations. Each department at every stage of product development has its own goals and workflows that address specific requirements. For example, the protocol at the discovery stage may have a protocol designed to identify opportunities in the market for a product while the Regulatory Affairs department may have one focused on obtaining necessary approvals from government agencies. Reviewing references yield better results when reviewers have clear, unambiguous instructions on where to start, what to include vs. exclude, and whom to engage.
To ensure reviewers in your organization are able to effectively collaborate in real time to screen, extract and present their error-free evidence in a standard report, automation is the way to go. According to Pulse of the Medical Devices Market Report, 73% of reviewers who use literature review software trust the quality of their data vs 37% who use spreadsheets, an error-prone, time consuming and resource intensive method. Here is how you can configure your protocol workflows and streamline your Systematic Review projects.
Setting Up Your Research Projects
When conducting a Systematic Review that has participants across geographical boundaries, reviewers need access to the right steps or levels and be able to see what needs to be done. It may sound simple, but when it comes to practicality, reviews done using spreadsheets are plagued by miscommunication, duplication of efforts and unclear project goals.
Your research protocol is a work plan, a step-by-step outline for knowledge synthesis. DistillerSR helps you bring your research protocol to life by easy configuration that is scalable and secure.
By setting up a project with well-planned levels, clearly defined inclusion and exclusion criteria, and assigning reviewers, you can eliminate all of the above challenges, arbitrary inclusion or elimination references,and any risk of bias. The non-linear workflow levels also helps avoid “screening fatigue” for reviewers. DistillerSR gives you the ability to easily configure even the most complex workflows that work for your organization.
Reviewers have ready access to the references they need to work on and DistillerSR takes care of the smooth transitioning of references across levels. Be it Dual Reviews, Conditional Workflows, Conflict Resolution or Quality Control operations, leave it to DistillerSR to manage it behind-the-scenes.
Reduce Your Literature Review Time By Half
AI-Powered Screening
Systematic Reviews by nature require a complete and exhaustive study of the current body of literature available on a topic which may sometimes be tens of thousands. Screening such a massive body of references takes up a significant portion of time in a systematic review. With the increasing volume of available references, it becomes an immense task which is laborious and time-consuming to not only thoroughly search a paper to identify relevant articles but also to do so correctly and prevent further delays.
DistillerSR helps you increase screening efficiency by stepping-in as a smart assistant. Using advanced Natural Language Processing, DistillerSR’s AI can learn your inclusion and exclusion pattern and automatically rearranges the reference set so that the more relevant ones are at the top, making it easier for you to include them.
DistillerSR’s AI can also detect duplicates even with different formatting, classify references for triaging and even check for references that may be erroneously excluded.
Efficient and Error-free Data Extraction
Spreadsheets have undeniably improved systematic reviews by enabling reviewers to digitally store, tabulate and share data. However, they still require extensive work, error-prone and essentially outdated. Any errors in data managed in spreadsheets are difficult to trace and rectify in a timely manner and hardly a step above manual methods.
“By learning my inclusion and exclusion pattern and reordering references based on relevance, DistillerSR’s AI enabled a more efficient overall review process and faster literature review completion rates.”
Shelley Jambresic, Senior Clinical Evaluation Manager at Geistlich Pharma AG
Data extraction with DistillerSR on the other hand, is strengthened by high configurability, extensive audit log, and version control providing the necessary stability and trustability. Reviewers can preemptively avoid transcription errors and the data is easily auditable. CuratorCR , a module of DistillerSR, provides reviewers with access to full-texts that were already purchased by the organization and to previously collected data that can be reused in the current project. This tremendously improves data quality and consistency apart from helping you save time and reducing costs.
Auto-generated PRISMA 2020 Flow-Diagram
Another DistillerSR advantage for your Systematic Review projects is the PRISMA 2020 Flow Diagram. It provides users the ability to automatically generate their PRISMA flow diagrams and its calculated numbers based on the decisions made and captured when using DistillerSR to conduct their screening. The most comprehensive and highly configurable PRISMA 2020 flow diagram is generated at the click of a button, saving you several days of work.
Automating your review process offers extensive benefits to reviewers. It helps you conduct smarter reviews that are transparent, audit-ready, and regulatory-compliant. If you want to achieve dramatically improved efficiencies in your Systematic Reviews, Request a Free Demo and see how DistillerSR can be easily configured to your preferred systematic review type.
DistillerSR® Inc. is the market leader in AI-enabled literature review automation software and creator of DistillerSR™. More than 300 of the world’s leading research organizations, including over 60 percent of the largest pharmaceutical and medical device companies, trust DistillerSR to securely produce transparent, audit-ready and regulatory compliant literature reviews faster and more accurately than any other method. With more organizations using DistillerSR to automate their systematic reviews, healthcare researchers can make informed and time-sensitive health policy decisions, clinical practice guidelines and regulatory submissions, and deliver better overall research.

Madhumitha Vijayakumar is the Product Marketing Manager for DistillerSR. Having been a software developer early on in her career and then a marketer who has worked with people in almost every timezone, she believes in the power of story telling to bridge the gap between customers and the business solutions they seek. She is also an avid reader and a full-time mom.
View all posts
Stay in Touch with Our Quarterly Newsletter
Recent posts.
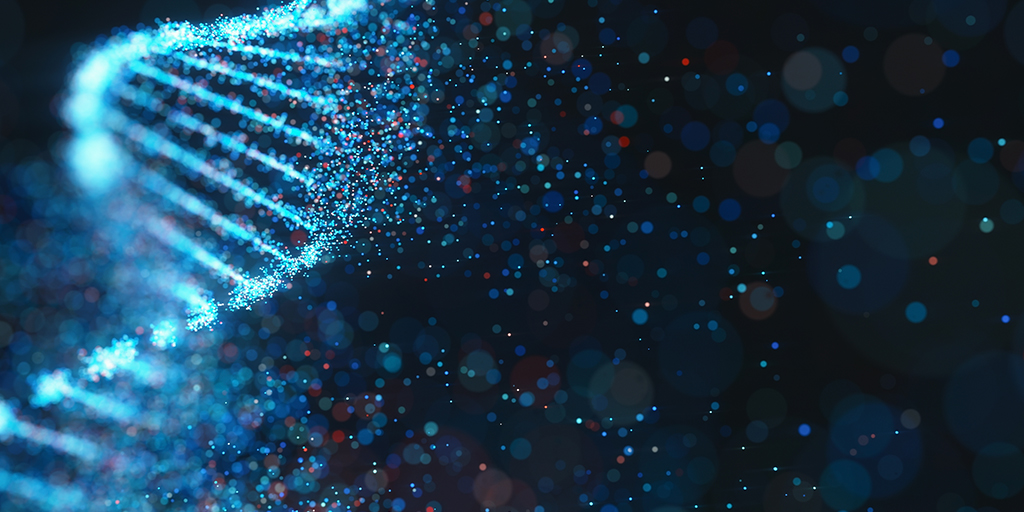
2023 Evidence Matters MedTech Roundtable Recap: AI and Enterprise Evidence Management Driving Innovation
At the 2023 Evidence Matters MedTech Roundtable, Dr. Matthew M. Cooper, Vice-President, Medical Affairs, Corporate Health & Safety at 3M Health Care, Chris Manrodt, Head of Data Strategy - IGTD at Philips, Dr. Matthias Fink, Senior Clinical Consultant at AKRA Team...
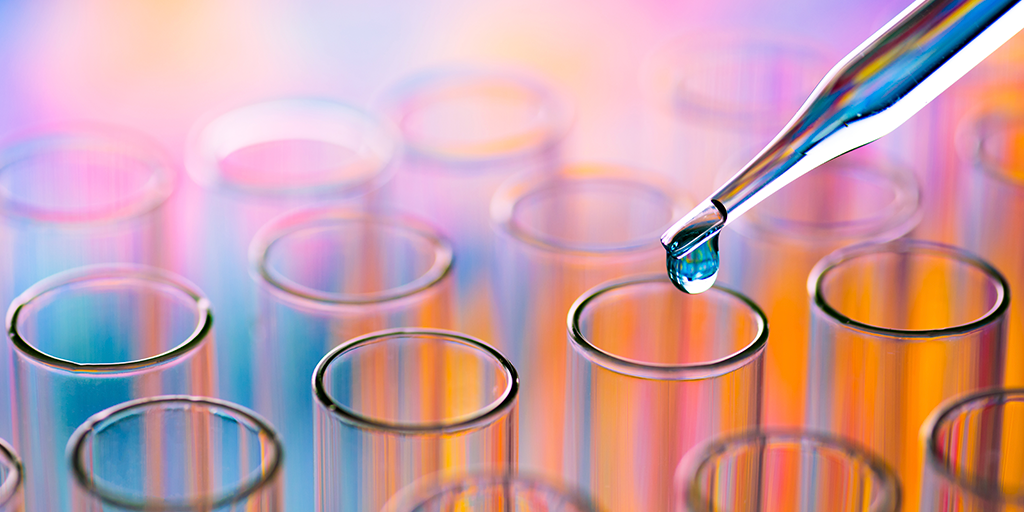
Webinar Recap: Abbott Diagnostics and AKRA Team on More Confident IVD Regulatory Submissions (RAPS Western Canada Chapter event)
In a recent webinar, DistillerSR customer Dr. Victoria Samonte, Medical Director of Global Medical Operations at Abbott Diagnostics and Dr. Bassil Akra, CEO and Founder at the AKRA Team GmbH were joined by Michelle Zaharic, CEO at MLZ Biotech Consulting and IVD Lead...
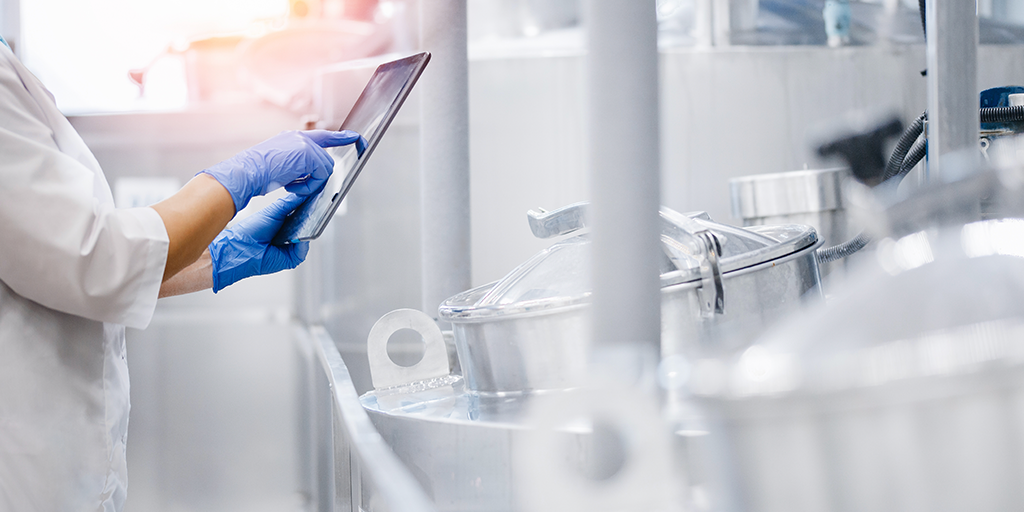
Webinar Recap: ICON and Oracle Share Literature Surveillance Best Practices for Pharmacovigilance
In a recent webinar, DistillerSR customer Andrew Purchase, Director of Pharmacovigilance, UK QPPV, Site Head (Swansea, UK) at ICON was joined by Michael-Braun Boghos, Senior Director Safety Strategy at Oracle Life Science, and moderated by Peter O’Blenis, DistillerSR...
- Oracle Mode
- Oracle Mode – Advanced
- Exploration Mode
- Simulation Mode
- Simulation Infrastructure
- Uncategorized
- Seven ways to integrate ASReview…
Seven ways to integrate ASReview in your systematic review workflow
Systematic reviewing using software implementing Active Learning (AL) is relatively new. Many users (and reviewers) have to get familiar with the many different ways how AL can be used in practice. In this blog post, we discuss seven ways meant to inspire users.
- Use ASReview with a single screener .
- Use ASReview with multiple screeners.
- Switch models for hard-to-find papers.
- Add more data because a reviewer asks you to.
- Screen data from a narrow search and apply active learning to a comprehensive search.
- Quality check for incorrectly excluded papers due to screening fatigue .
- Use random reading because you like the looks of the software.
1. Use ASReview with a single screener
Let’s assume you conducted a systematic search in multiple databases, the records you have found in the different databases were merged into one dataset, the data was de-duplicated, and as many abstracts as possible were retrieved ( why is this so important? ). You found 10,000 potentially relevant records, and you want to screen the records based on predefined inclusion/exclusion criteria. You also have chosen a stopping rule .
You already know about ten records that you already know are relevant (for example, from asking experts in the field). To warm up the model, you can use five records as prior knowledge , while the other five will be used as validation records to check if the software can find these records. After selecting the ten randomly chosen irrelevant records and deciding on the active learning model , you can start screening until you reach your stopping criterium . The goal is to screen fewer records than exist in your dataset, and simulation studies have shown you can skip up to 95% of the records (e.g., Van de Schoot et al., 2022 ), but this highly depends on your dataset and inclusion/exclusion criteria (e.g., Harmsen et al., 2022 ). After deciding to stop screening, you can export the results (i.e., the partly labeled data and the project file containing the technical information to reproduce the entire process) and publish them on, for example, the Open Science Framework. As a final step, you can mark the project as finished in ASReview.
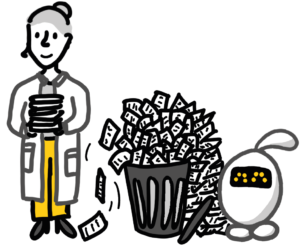
2. Use ASReview with multiple screeners
Again, let’s assume you found 10,000 potentially relevant records, and you want to screen the records based on predefined inclusion/exclusion criteria using multiple screeners, let’s say two researchers. Then there are numerous options:
Both researchers install the software locally, upload the same data and select identical records as prior knowledge. Both researchers train the same active learning model and independently start screening records for relevance. After both researchers are done screening- because each fulfills the pre-specified stopping criterium , the results, containing the labeling decisions and the ranking of the unseen records, are exported as a RIS, CSV, or XLSX file. Both files can be merged in, for example, Excel or R ). Now, both screeners can discuss differences in labeling decisions and compute the similarity (e.g., Kappa), just like with a classical PRISMA-based reviewing. The only difference is that there might be records only seen by one of the two researchers or records not seen at all.
Tip: One screener can set up the project and export the project file. The second screener can import the project file instead of setting up a new project. This will make sure that both screeners start with the same priors and project setup.
Both screeners use the same data, but they use a different set of records as prior knowledge. After screening until the stopping criterium has been reached, both screeners merge the results to check if the same relevant papers have been found independent of the initial training set.
A similar procedure can be applied for different settings for the active learning model (e.g., use two other feature extractors or classifiers) to check if they find the same set of relevant independent of the chosen model.
Research A starts with screening, and Reviewer B takes over if the screening time for A is done. If B is done, then A takes over again. You can export the project file and share it with a colleague who can import the file in ASReview to continue screening where the first researchers stopped (officially supported). Alternatively, ASReview can be put on a server (not officially supported but successfully implemented by some users).
Tip: Collaborate with researchers in different time zones, so screening can continue 24 hours per day!
3. Switch models for hard-to-find papers
Based on a simulation study by Teijema et al. (2022 ), we strongly advise switching to a different model after reaching your predefined stopping criterium. Especially switching from a simple and fast model (e.g., TF-IDF with NB or logistic regression) to a more advanced and computational intense model (e.g., doc2vec or sBert with a neural network or even a custom model designed specifically for your data added via a template ) can be beneficial in finding records that are more difficult to be identified by the simpler models. Be aware of the longer training time of advanced feature extraction techniques during the warm-up phase; see Table 1 of Teijema et al. for an indication of the time you need to wait before the first iteration is done training.
Procedure :
- Start Screening round 1.
- Use the information on the analytics page to determine whether you reached your stopping criterium .
- Export your data and project file.
- Start a new project for screening round 2.
- Upload your partly labeled dataset ; the labels of your first round of screening will be automatically recognized as prior knowledge.
- Select a different model than you used on screening round 1.
- Screen for another round until you reach your stopping criterium again.
- Export your data and the project file.
- Mark your project as finished.
- Make both the project file and dataset for both screening rounds available, for example, the Open Science Framework.
The Mega Meta project is an example where we applied the procedure mentioned above. The team first screened a dataset containing >165K records using TF-IDF and logistic regression. After reaching the stopping criterium, the labeling decisions of the first round were used to optimize the hyperparameters of a neural network. Using the optimized hyperparameters, a 17-layer CNN model was used as a model for the partly-labeled data of the first round. The output was screened for another round until the stopping criterium was reached. 290 extra papers were screened, and 24 additional relevant papers were found. Their workflow is described in Brouwer et al. (2022) .
4. Add more data because a reviewer asks you to
Working on a systematic review project (or meta-analysis) is a long process and by the time reviewers are reading your paper, your literature search is outdated. Often it is Reviewer #2 who asks you to update your search 🙁
A way to deal with this request is to re-run your search and add the newly found and unlabeled records to your labeled dataset. Make sure to have a column in the dataset containing the labels (`0` for excluded papers and `1` for the included papers, and leave blank for the new records). Import the file into ASReview and the labeled records will be detected as prior knowledge.
Tip: When using reference managing software the labels can be stored in the N1 field .
For example, Berk et al. ( 2022 ) performed a classical search and screening process, identifying 638 records, of which 18 were deemed relevant. Then, the search was updated after 6 months. The labels of the first search were used as training data for the second search. Then, the 53 records were screened using ASReview, and seven additional relevant records were identified.
5. Screen data from a narrow search and apply active learning to a comprehensive search
Since a single literature search can easily result in thousands of publications that have to be read and screened for relevance, literature screening is time-consuming. As truly relevant papers are very sparse (often <5%) this is an extremely imbalanced data problem. When answering a research question is urgent, as, with the COVID-19 crisis, it is even more challenging to provide a review that is both fast and comprehensive. Therefore, scholars often develop narrower searches, however, this increases the risk of missing relevant studies.
To avoid having to make harsh decisions in the search phase, you could spend an equal amount of screening time starting with a much larger dataset. That is, you can broaden the search query and identify many more papers than you would have been willing to screen with a more narrow search. Since you always screen the most likely relevant paper as predicted by the model, you will screen the most likely relevant papers in the larger dataset.
You could first perform a classical search and use all the decisions made to train a model for a larger dataset found with a broader search.
For example, Mohseni et al. ( 2022 ) broadened the search terms after an initial search strategy. The original search identified 996 articles, of which 93 were deemed relevant after the manual screening. The broader search yielded 3477 records, and after screening the first 996 abstracts with ASReview they found 28 additional relevant abstracts of which 3 met the final full-text inclusion criteria, which were otherwise missed using the standard search strategy.
Similarly, Savas et al. ( 2022 ) screened papers from 2010 and onward, which yielded 1155 records, of which 30 were deemed relevant. Subsequently, a new search was performed, including papers published before 2010. This search yielded an additional 4.305 records. The labels of the screening phase of the first search were used as training data for the second search and five papers appeared to be found to meet the inclusion criteria.
6. Quality check for excluded records
Due to screening fatigue, you might have accidentally excluded a relevant paper. To check for such incorrectly excluded but relevant papers, you can ask a second screener to screen your excluded papers using the relevant records as training data.
- Screener A finishes the screening process (with or without using active learning / ASReview).
- In reference manager software (or excel), select the included and excluded records, but remove all unseen records from the data. Add a column with the label `1` for the relevant records. Select 10 records you are sure should be excluded and add the label `0`. Remove any labels for the other excluded records you want to check.
- Decide on a stopping rule.
- Start a project in ASReview, import the partly labeled dataset (the prior knowledge will be automatically detected), and train a model.
- Ask a second screener to screen the data (maybe just for 1-2 hours). This person will screen the initially excluded records but rank-ordered based on relevance scores.
- After the stopping criterium has been reached, mark the project as finished and export the data and project file.
In Brouwer et al. (2022) this procedure was used, and, in total, 388 labels originally determined as irrelevant and predicted by the machine learning model as most likely relevant were assessed by a topic expert, and 95 labels were converted back to relevant
7. Use random reading because you like the looks of the software
Maybe you don’t want to use active learning, but you do want to use the software because it looks great! Or, because there is a hidden gaming mode… No worries, you can always select random as the query strategy.
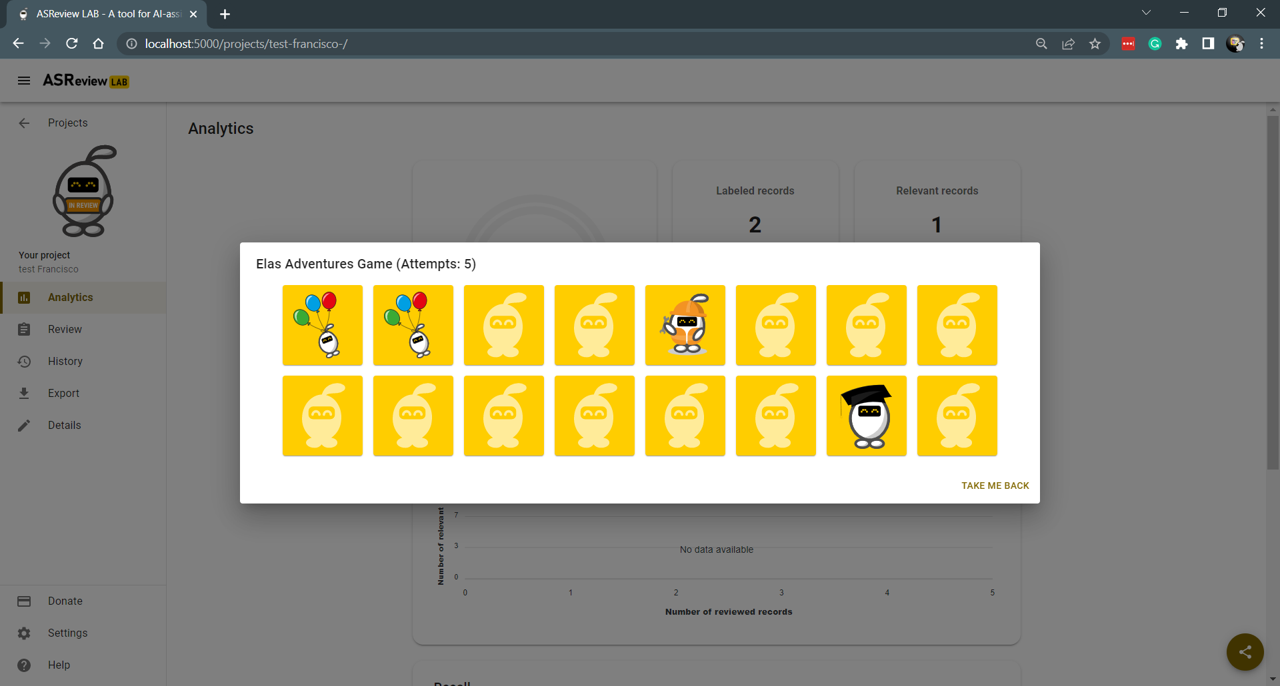
Related posts
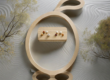

- Ask a Librarian
Artificial Intelligence (AI)
Ai for systematic review.
- How to Cite AI Generated Content
- Prompt Design
- Resources for Educators
- Purdue AI Resources
- AI and Ethics
- Publisher Policies
- Selected Journals in AI
Various AI tools are invaluable throughout the systematic review or evidence synthesis process. While the consensus acknowledges the significant utility of AI tools across different review stages, it's imperative to grasp their inherent biases and weaknesses. Moreover, ethical considerations such as copyright and intellectual property must be at the forefront.
- Application ChatGPT in conducting systematic reviews and meta-analyses
- Are ChatGPT and large language models “the answer” to bringing us closer to systematic review automation?
- Artificial intelligence in systematic reviews: promising when appropriately used
- Harnessing the power of ChatGPT for automating systematic review process: methodology, case study, limitations, and future directions
- In-depth evaluation of machine learning methods for semi-automating article screening in a systematic review of mechanistic
- Tools to support the automation of systematic reviews: a scoping review
- The use of a large language model to create plain language summaries of evidence reviews in healthcare: A feasibility study
- Using artificial intelligence methods for systematic review in health sciences: A systematic review
AI Tools for Systematic Review
- DistillerSR Securely automate every stage of your literature review to produce evidence-based research faster, more accurately, and more transparently at scale.
- Rayyan A web-tool designed to help researchers working on systematic reviews, scoping reviews and other knowledge synthesis projects, by dramatically speeding up the process of screening and selecting studies.
- RobotReviewer A machine learning system aiming which aims to automate evidence synthesis.
- << Previous: AI Tools
- Next: How to Cite AI Generated Content >>
- Last Edited: Mar 21, 2024 1:34 PM
- URL: https://guides.lib.purdue.edu/ai
systematic-reviewpy 0.0.1
pip install systematic-reviewpy Copy PIP instructions
Released: Jan 25, 2023
A Python framework for systematic Review.
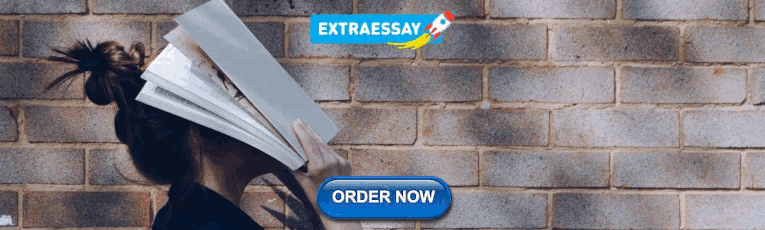
Project links
- Bug Reports
- Bug Tracker
- Say Thanks!
- Open issues:
View statistics for this project via Libraries.io , or by using our public dataset on Google BigQuery
License: MIT License
Author: Chandravesh Chaudhari
Tags browser, automation, systematic review, research papers, Bibliometric analysis
Requires: Python >=3.8
Maintainers
Classifiers
- End Users/Desktop
- Science/Research
- OSI Approved :: MIT License
- OS Independent
- Python :: 3.8
- Internet :: WWW/HTTP :: Browsers
- Software Development :: Build Tools
Project description
An open-source Python framework for systematic review based on PRISMA : systematic-reviewpy
Introduction, installation, contribution, future improvements.
The main objective of the Python framework is to automate systematic reviews to save reviewers time without creating constraints that might affect the review quality. The other objective is to create an open-source and highly customisable framework with options to use or improve any parts of the framework. python framework supports each step in the systematic review workflow and suggests using checklists provided by Preferred Reporting Items for Systematic Reviews and Meta-Analyses (PRISMA).
The packages systematic-reviewpy and browser-automationpy are part of Research paper An open-source Python framework for systematic review based on PRISMA created by Chandravesh chaudhari , Doctoral candidate at CHRIST (Deemed to be University), Bangalore, India under supervision of Dr. Geetanjali purswani .
- supported file types: ris, json, and pandas IO
- supports the complete workflow for systematic reviews.
- supports to combine multiple databases citations.
- supports searching words with boolean conditions and filter based on counts.
- browser automation using browser-automationpy
- validation of downloaded articles.
- contains natural language processing techniques such as stemming and lemmatisation for text mining.
- sorting selected research papers based on database.
- generating literature review excel or csv file.
- automatically generates analysis tables and graphs.
- automatically generates workflow diagram.
- generate the ASReview supported file for Active-learning Screening
Significance
- Automate monotonous tasks
- Never makes mistakes
- Provides replicable results
This project is available at PyPI . For help in installation check instructions
Dependencies
- rispy - A Python 3.6+ reader/writer of RIS reference files.
- pandas - A Python package that provides fast, flexible, and expressive data structures designed to make working with "relational" or "labeled" data both easy and intuitive.
- browser-automationpy
- pdftotext - Simple PDF text extraction
- PyMuPDF - PyMuPDF (current version 1.19.2) - A Python binding with support for MuPDF, a lightweight PDF, XPS, and E-book viewer, renderer, and toolkit.
Important links
- [Documentation](documentation link)
- [Quick tour](tutorial file link)
- Project maintainer (feel free to contact)
all kinds of contributions are appreciated.
- [Improving readability of documentation](documentation link)
- Feature Request
- Reporting bugs
- Contribute code
- Asking questions in discussions
Graphical User Interface
Project details
Release history release notifications | rss feed.
Jan 25, 2023
Download files
Download the file for your platform. If you're not sure which to choose, learn more about installing packages .
Source Distribution
Uploaded Jan 25, 2023 Source
Built Distribution
Uploaded Jan 25, 2023 Python 3
Hashes for systematic-reviewpy-0.0.1.tar.gz
Hashes for systematic_reviewpy-0.0.1-py3-none-any.whl.
- português (Brasil)
Supported by

An official website of the United States government
The .gov means it’s official. Federal government websites often end in .gov or .mil. Before sharing sensitive information, make sure you’re on a federal government site.
The site is secure. The https:// ensures that you are connecting to the official website and that any information you provide is encrypted and transmitted securely.
- Publications
- Account settings
Preview improvements coming to the PMC website in October 2024. Learn More or Try it out now .
- Advanced Search
- Journal List

Operating Room Performance Optimization Metrics: a Systematic Review
Anne m. schouten.
1 Biomedical Engineering Department, Technical University of Delft, Mekelweg 5, 2628 CD Delft, the Netherlands
Steven M. Flipse
2 Science Education and Communication Department, Technical University of Delft, Mekelweg 5, 2628 CD Delft, the Netherlands
Kim E. van Nieuwenhuizen
3 Gynecology Department, Leiden University Medical Center, Albinusdreef 2, 2333 ZA Leiden, the Netherlands
Frank Willem Jansen
Anne c. van der eijk.
4 Operation Room Centre, Leiden University Medical Center, Albinusdreef 2, 2333 ZA Leiden, the Netherlands
John J. van den Dobbelsteen
Associated data.
Literature proposes numerous initiatives for optimization of the Operating Room (OR). Despite multiple suggested strategies for the optimization of workflow on the OR, its patients and (medical) staff, no uniform description of ‘optimization’ has been adopted. This makes it difficult to evaluate the proposed optimization strategies. In particular, the metrics used to quantify OR performance are diverse so that assessing the impact of suggested approaches is complex or even impossible. To secure a higher implementation success rate of optimisation strategies in practice we believe OR optimisation and its quantification should be further investigated. We aim to provide an inventory of the metrics and methods used to optimise the OR by the means of a structured literature study. We observe that several aspects of OR performance are unaddressed in literature, and no studies account for possible interactions between metrics of quality and efficiency. We conclude that a systems approach is needed to align metrics across different elements of OR performance, and that the wellbeing of healthcare professionals is underrepresented in current optimisation approaches.
Supplementary Information
The online version contains supplementary material available at 10.1007/s10916-023-01912-9.
Operating Room (OR) performance optimization is investigated from many angles and numerous different strategies are proposed. Think hereby of new systems based on data analysis that enable more efficient OR scheduling. However, many of these promising initiatives that are meant to improve the OR do not seem to land in practice [ 1 ]. Suggested changes do not always fit the overall workflow of the OR, or they solve the targeted problem ineffectively. Creating a support base amongst the people that implement or work with the innovation also tends to be problematic [ 2 ]. To enable improvement of OR performance with innovations that fit well in practice, it should be clear what is exactly meant with the term OR performance. Furthermore, to know if an innovation improves overall OR performance, one must know how to measure the overall performance. Below, we discuss what OR performance means according to literature and which elements it contains. Next, to investigate how to measure OR performance we make an inventory of the metrics used in literature to measure OR performance. Finally, to investigate how the field approaches OR performance optimization, we collected studies on this topic and addressed what methods were used and what aspect of OR performance the research focussed on. Besides perspectives on patients and healthcare professionals, we also consider economic perspectives on the OR on hospital budgets.
Pressures for change in the OR
The OR comprises a complex environment with multi-layered social interactions, unpredictability and a low tolerance for mistakes [ 3 ]. Irregularities in the workflow are often triggered by a combination of factors such as demanding caseloads, pressure to perform complex tasks and conflicting priorities. This can result in increased mental strain and stress amongst the healthcare professionals [ 4 ].
Irregularities in workflow on the OR also impact patients. Approximately 60% of the patients visit the OR at some point during their hospital stay [ 5 ]. Undergoing hospital admission and an operation makes many people experience emotions such as nervousness, agitation and uncertainty. Irregularities in the process can worsen this [ 6 ].
Accounting for about 35% to 40% of the costs, the OR is a large contributor to a hospital’s finances, also being one of the most costly units [ 7 – 9 ]. Over the past years, healthcare costs have increased and diminishing returns have prompted healthcare administrators to alleviate institutional costs through reductions in budget allocations [ 10 ].
Partly driven by the increasing demands for care on the one hand, and constrained resources on the other, a technological evolution has taken place over the last decades. This has played an important role in the development of surgery and resulted in dramatic changes in working conditions within the OR [ 11 ]. But healthcare professionals are not always prepared for this transformation of their work. Healthcare professionals are reported to lack preparation for radical (technical) changes in their work [ 12 ].
Despite the growing influx of new healthcare professionals, the sector experiences a major exodus of healthcare professionals. Causes are the heavy workload and a lack of autonomy. The limited autonomy of healthcare professionals in their daily work is appointed as a long-standing issue [ 12 ]. Research from the Dutch doctors organisation De Jonge Dokter has interviewed 622 young doctors about their work. About 50% of the interviewees has thought about quitting their job due to high work pressure, emotional pressure and working culture [ 13 ].
The impact of the workflow of the OR on patients, pressure on healthcare professionals that work on the OR, the vast changing work environment and economical constraints make that optimizing the OR is high on the academic agenda. However, the high expectations of patients, interactions between different professionals, unpredictability and complex surgical case scheduling make managing and changing the system difficult. Attempts to resort to commonly used industrial principles to increase factors such as efficiency have been demonstrated to easily fail due to these (and possibly other) particular characteristics of the OR [ 7 ]: human factors have too great of an impact to standardize and automate certain OR processes. Another complicating factor is the divergent perspectives on OR performance optimization.
OR performance optimization metrics
The metrics used to quantify OR performance optimization reported in literature are diverse [ 14 ]. Many articles focus on the efficiency aspect of OR performance optimization, some focus more on the quality aspects. For example, the work of Bellini et al. [ 7 ] speaks of the optimization related factor efficiency in the sense of more precise scheduling and limiting waste of resources. Costa Jr. et al. (2015) speak of both efficiency and optimization, hereby focussing on resources and time management. Sandbaek et al. [ 16 ] refer to OR efficiency as maximizing throughput and OR utilization while minimizing overtime and waiting time, without additional resources. Tanaka et al. (2011) assesses OR performance using indicators such as the number of operations, the procedural fees per OR, the total utilization time per OR and total fees per OR. Rothstein & Raval [ 3 ] refer to the metrics of OR efficiency based on the Canadian Paediatric Wait Times Project: off-hours surgery, same-day cancellation rate, first case start-time accuracy, OR use, percentage of unplanned closures, case duration accuracy, turnover time and excess staffing costs. Alternatively, Arakelian, Gunningberg and Larsson (2008) emphasize that apart from cost-effectiveness, work in the OR should be organized to fulfil the demands on patient safety and high-quality care. From their perspective, OR departments must create efficient ways of planning and processing the work, while at the same time maintaining the quality of care. These authors also show that there are diverging perspectives among OR personnel on what efficiency and productivity entail.
The previous paragraphs illustrate that when speaking about OR performance optimization, different terminology is being used. Furthermore, although many studies focus on how to optimize or monitor certain aspects of the OR, studies on the impact of these changes on the quality and efficiency of the hospital as a whole appear to be lacking. This may lead to uncertain optimisation strategies that are difficult to substantiate with supporting evidence [ 15 ]. Table Table1 1 summarizes both quality and efficiency aspects of OR workflow and strives to align the methods and metrics to assess OR performance in terms of 1. Patient safety 2. Quality of care 3. Cost-effectiveness and 4. Healthcare professional well-being.
OR performance includes four aspects: 1. Patient safety 2. Quality of care 3. Cost-effectiveness 4. Well-being healthcare professionals
A systematic literature review was conducted to make an inventory of metrics for optimization of the OR in literature. We used the search engines Scopus, Web of Science and PubMed with the search terms: “Operation Room” AND Optimization and Workflow AND Optimization AND Hospital. We limited the search to articles that discuss ways to optimize the OR as a system, not the performed medical interventions themselves. Furthermore, articles that were not written in the English language or did not belong to the category healthcare or medicine were excluded.
An inventory of the topics of the articles was made by filtering out 1. the focus/aim of the study, 2. the method used and 3. the conclusion. Optimization strategies in other hospital departments might be transferable to the OR as well. Therefore, to gain insight in the distribution of optimization strategies on the different departments of the hospital, the articles were analysed by labelling the operational department the research is focussed on, which topic was investigated, and which method was used. After creating this overview, only data about the OR was used. To obtain OR performance metrics a second analysis was conducted: OR performance characteristics from the articles were split into aspects with their corresponding metrics.
Coding nodes
Overall categories for departments, topics and methods were identified based on the first 50 articles, as the authors felt a saturation rate for new categories was reached. The remaining articles were then labelled within these categories. To illustrate, Table Table2 2 shows two sections that were both labelled as the metric T_3 and two sections that were labelled as the method M_8.
Sections from articles that were labelled as T_3 and sections that were labelled as M_8, to illustrate when these sections fall in the same category
Coding the articles
Some articles mention multiple topics or methods. If multiple topics were mentioned, the article was labelled for the topic which had the most emphasis. This is illustrated in Example 1, where both the topics patient throughput and costs are mentioned. However, the emphasis is on patient throughput. For topics the article was therefore labelled as T_3: Optimize patient flow.
When labelling the articles for their method, it occurred that an article investigates an optimization possibility and method by means of a literature study. The method of the article was then labelled as literature study. In Example 2, the article investigates how workflow can be improved by identifying the potential failures of the system by means of a management tool. However, the effects of the management system on workflow are investigated by a literature study. For methods the article was therefore labelled as M_1: Literature study. In the results the coded articles are displayed in 3 sunburst graphs: the first shows the distribution of methods and topics of all hospital departments, the second contains the distribution of methods and topics on the OR. To elucidate the OR data, the third graph shows a selection with the biggest categories of the second sunburst (categories with N ≥ 3). Some of the smaller categories are illustrated with examples in the text.
The labelling was performed independently by two of the authors. Discrepancies were discussed and adjusted by obtaining consensus.
Example 1: “In most hospitals, patients move through their operative day in a linear fashion, starting at registration and finishing in the recovery room. Given this pattern, only 1 patient may occupy the efforts of the operating room team at a time. By processing patients in a parallel fashion, operating room efficiency and patient throughput are increased while costs remain stable” [ 18 ].
Example 2: “Failure mode and effects analysis (FMEA) is a valuable reliability management tool that can pre-emptively identify the potential failures of a system and assess their causes and effects, thereby preventing them from occurring. The use of FMEA in the healthcare setting has become increasingly popular over the last decade, being applied to a multitude of different areas. The objective of this study is to review comprehensively the literature regarding the application of FMEA for healthcare risk analysis” [ 21 ].
In this section, the results of the inventory of OR performance metrics and the addressed OR performance topics in literature are shown.
Review statistics
Figure 1 shows the search engines, search terms and number of papers found.

Literature search method used to make an inventory of the current literature on OR optimization
OR performance metrics
Based on Table Table1, 1 , the characteristics of OR performance (efficiency and quality) have been split up into aspects and were then further specified into metrics (Table (Table3 3 ).
Characteristics, aspects, and metrics of OR performance as reported in literature
Addressed OR performance topics in literature
Table Table4 4 shows the categories used to analyse the articles, the corresponding labels and their names. In Appendix 1, Supp. Tab. 1, the topic category and their corresponding sources are presented.
The categories used to analyse the articles, corresponding labels and names
Distribution of the labels
Appendix 2, Supp. Figure 1 , shows a sunburst graph that illustrates the distribution of the labels per department (D_x). To give an overview that represents the distribution of departments in a hospital, only the data from the second search criteria (Workflow AND Optimization AND Hospital) was included in this graph. The inner circle contains the different departments, namely the OR, ER (emergency room), outpatient clinic, patient clinic and the hospital in general. The middle circle shows the corresponding methods, the outer circle shows the topics. Most articles focus on the OR (D_1, N = 16). The ER receives less attention (D_2, N = 2). There were no articles that were labelled for outpatient clinic (D_3).
In Appendix 2, Supp. Figure 2 zooms in on methods and corresponding topics of just the OR. This graph includes all OR data from both search criteria and shows the methods, topics and number of articles in each category. In Fig. 2 , a selection of the OR sunburst graph is displayed. This selection contains the most frequent combination of period, method and topic (N ≥ 3). Most articles have the aim to optimize scheduling (N = 7), workflow tracking (N = 5) and patient flow (N = 4) by computational means such as machine learning.

Selection of the sunburst graph, showing the seven main categories
All data was then stratified by the means of a bar chart. Figure 3 shows the data while looking at different methods per topic. Computational methods (M_8) are used most frequently (N = 41).

Bar chart with the different methods per topic
The methods that were used the least are experiments with the medical staff (M_7, N = 2) and system engineering (M_10, N = 2). The most investigated topics are patient flow (T_10, N = 18), OR scheduling (T_3, N = 17) and workflow tracking systems (T_5, N = 15).
In this study we addressed what methods were used in other studies, what aspect of OR performance they focussed on, and for which department the effects were to be relevant. We aimed to investigate how the field approaches OR performance optimization and to create an overview of OR performance metrics for the categories of patient safety, quality of care, cost-effectiveness, and the well-being of healthcare professionals.
Most studies focused on patient safety, quality of care and cost-effectiveness. This might be explained by the fact that healthcare has a central focus on patient wellbeing and clinical outcome measures. One striking result from this study is that the well-being of healthcare professionals is largely ignored in OR optimization studies. Ill-performing in these areas may contribute to staff shortages. Therefore, we deliberately added the well-being of healthcare professionals as a crucial aspect of OR performance as we feel that this is a subject that must be taken in account in OR optimisation.
By taking all four categories within OR performance as a starting point for the delineation of the ways to measure OR performance, we strive to create an all-encompassing overview of relevant metrics in literature. More metrics were found for efficiency than for quality aspects. This was as expected because efficiency tends to be easier to measure than quality aspects. Furthermore, quality metrics are often subjective. For instance, well-being of healthcare professionals, is linked to the metric autonomy (freedom to make your own choices, plan your workday etc.) . This is a capacity that is difficult to quantify in a valid and reliable way.
Considering the research topics addressed in literature it was found that most articles have the aim to optimize OR scheduling, Workflow tracking or Patient flow by computational means, such as machine learning. Thanks to greater computing power, as well as the growing availability of large amounts of data, machine learning holds the promise to make sense of complex modelling tasks [ 24 ]. Topics such as OR scheduling, Workflow tracking and Patient flow fit this picture. They are suitable for computational simulations and optimizations of complex systems such as the OR that are characterised by high variability in the timing and alignment of processes.
Categories that involve experiments with healthcare professionals (such as interventions in practice) were only limitedly represented in the literature. With AI on the rise, it seems a logical choice to use simulations to test optimizations instead of occupying the (often overworked) healthcare professionals. However, although simulated efficacy trials have generated many possible interventions to improve healthcare, their impact on practice and policy is limited so far [ 25 ]. Establishing and conveying the credibility of computational modelling and simulation outcomes is a delicate task [ 26 ] and the step from simulation to implementation in practice turns out to be a difficult one.
Kessler & Glasgow point out that healthcare research must deal with “wicked” problems that are multilevel, multiply determined, complex and interacting. Research tends to isolate, decontextualize and simplify issues in order to be able to investigate them. Consequently, the small number of studies with representative populations, staff and settings that substantiate optimisation approaches is in sheer contrast with the large number of papers that promote the potential of computational methods.
Overall, similar to what Fong et al. (2016) report, timepoints, cost, methodology and outcome measures were inconsistent across the studies in this review, and it appears that multiple metrics can fit a topic. Nevertheless, the topics of the articles cited in this review give insightful handles of how to structure OR performance metrics. Increasing awareness about these topics and metrics amongst the people who work with them is therefore of value.
Awareness should also be increased about the definitions of the concepts of OR performance [ 17 ]. It is important to realize that the term “ OR performance” only describes a snapshot in time but extents across all topics. Some studies talk about performance, but do not always specify if there is change in this performance. Change can only be measured over time. When doing so, clear criteria are required to determine if the change is also an improvement. In one context something might be an improvement, in another it might worsen the situation [ 27 ].
The ideal scenario would be to optimize an OR performance topic for all the metrics from Table Table3. 3 . However, this may not always be attainable. A sensible approach is to apply relevant metrics both at the beginning of your project and after your intervention in the system, and to evaluate the impact on all four categories of OR performance. By prioritizing and assigning weights to metrics acceptable ranges for the optimisation outcomes could be defined. When looking at optimization in this way, it should comprise two elements: improvement on a (set of) metric(s) and an improvement of the total system after your intervention.
This approach is illustrated in Fig. 4 , where on the left the hypothetical optimization of one metric is shown, and on the right the same change of the metric is shown, together with another metric of the system. When, for example, one chooses to optimize OR performance by increasing the metric Number of operations per OR per month , you aim for point A in Fig. 4 . However, Fig. 4 also illustrates that an increase along one metric could mean a (unintended) decrease on another. When taking other metrics into account you can see it is actually point B you are aiming for. Therefore, measuring every metric before and after your intervention to monitor the impact on the total system is essential for a thorough validation of its appropriateness.

Two elements of OR performance optimization: optimize for a (set of) metric(s) and improve or conserve the overall balance of the metrics
Modelling the metrics
Optimizing the system for a certain metric while also considering the other metrics should be part of the optimization strategy. Practical execution of this strategy is a roadmap with design steps in which the metrics are incorporated. In the following paragraphs this idea will be illustrated with Fig. 5 and an example scenario for an optimization goal.

Suggestion for a research setup in which the whole system is taken into account by incorporating an analysis of the metrics. Based on the PDCA Cycle of Deming
Figure 5 shows an example of the main steps of a research approach (aim, method, data collection, results, conclusion) with an emphasis on the phase between method and data collection. The approach is based on the Plan-Do-Check-Act method of Deming [ 28 ]. In a fictitious scenario the aim of the project is to improve the well-being of healthcare professionals on the OR. This is the first step of the model in Fig. 5 . In second step it is determined that the method used to achieve the aim will be increasing the metric autonomy of the healthcare professionals. A questionnaire amongst the staff involved shows that the healthcare professionals would like to have more autonomy over when they work. A more open work schedule is therefore suggested.
In the third step of the model the most important metrics that could be affected by this change are listed by the researchers:
- Excess staffing costs (often caused by over- or under-utilization of the OR).
- OR utilization
- Quality of care and patient safety
In the fourth step the selected metrics are combined in logical sets as system optimisation metrics with assigned weights and acceptance ranges. As an example, we could look at optimizing OR utilization. In this case the constant consists of the metric off time per OR per month and the utility time per OR per month (see Eq. 1 ).
Ranges of the constant are then given scores and weights to calculate the optimal value (Table (Table5). 5 ). For example, if T* were to be greater than 1, there would be more off time per OR than utility time. That is an undesirable scenario. This range is therefore given a score of -1 and a weight of 2.
The constant T* describes OR utility. To create insight in what values of T* are desirable and which are not, scores and weights are assigned to ranges of values of T*
When T* is low there is a high utility rate of the OR’s. When T* is high there is a low utility rate. A more complete overview would be created when also plotting financial metrics and metrics concerning the well-being of the medical staff.
After data has been collected in step 5 of the model, the results of step 6 can be compared in step 7. One can then evaluate whether the intended innovation will improve the system in such a way that it is worth the investment. And if not, consider carefully based on the metric overviews where adjustments to the intervention are required.
Limitations
This study has limitations. A major focus of this paper is the importance of seeing the whole picture when doing research. We have given examples of possibilities to bring this way of doing research into practice. However, despite the broad view of this study, we did not cover all aspects of healthcare. We looked at just the OR. Following our own philosophy, we want to stress that an even broader scope is relevant for successful optimization in healthcare. There is an intricate interplay between the different departments of a hospital. Increasing the efficiency of the OR might, for example, cause trouble in the timetable of the PACU (Post Anaesthesia Care Unit).
Concluding remarks
In this study it was found that there are many different perspectives and approaches used to optimize OR performance. The metrics used to optimize OR performance are diverse. Based on our inventory of the metrics and methods used in literature we conclude that part of the crucial aspects of OR performance, such as the wellbeing of healthcare professionals, are underrepresented in the research field. The lack of studies that account for possible interactions between metrics of quality and efficiency have limited the impact of optimisation approaches. Too much focus on one metric potentially deteriorates other elements of the system you try to optimize. To obtain profitable OR optimization, a systems approach that aligns metrics across functions and better representation of the wellbeing of healthcare professionals are needed.
Future research
An informative topic to investigate further is to test the effect of awareness of metrics when optimizing OR metrics in practice. The hypothesis here is that more awareness of OR performance metrics and their correlations amongst researchers could lead to better optimizing strategies. In this context, the model in Fig. 5 might also be tested. Does it increase awareness? Do researchers use different approaches with the model than without? Does this lead to better outcomes?
Another direction is the continuous measuring of OR performance metrics to be able to monitor unintended interactions, in ways that not put a burden on healthcare professionals (i.e., increasing administrative tasks). Furthermore, technology can speed up and smoothen processes within the OR, but the impact on perioperative processes might not have been considered. An interesting way to put these thoughts to practice is investigating how the increase of technology on the OR has influenced the work of healthcare professionals such as OR nurses and supporting department.
Below is the link to the electronic supplementary material.
Acknowledgements
Not Applicable
Abbreviations
Authors' contributions.
J.D. and F.J. and A.S. conceived of the presented idea. K.N. verified selection of the articles. A.S., S.F., J.D. and A.E. contributed to the design and implementation of the research, to the analysis of the results and to the writing of the manuscript.
Medical Delta.
Declarations
Not Applicable.
The authors declare that they have no competing interests.
Not applicable.
Publisher's Note
Springer Nature remains neutral with regard to jurisdictional claims in published maps and institutional affiliations.
SYSTEMATIC REVIEW article
Promoting mental health in children and adolescents through digital technology: a systematic review and meta-analysis.
- Faculty of Artificial Intelligence in Education, Central China Normal University, Wuhan, China
Background: The increasing prevalence of mental health issues among children and adolescents has prompted a growing number of researchers and practitioners to explore digital technology interventions, which offer convenience, diversity, and proven effectiveness in addressing such problems. However, the existing literature reveals a significant gap in comprehensive reviews that consolidate findings and discuss the potential of digital technologies in enhancing mental health.
Methods: To clarify the latest research progress on digital technology to promote mental health in the past decade (2013–2023), we conducted two studies: a systematic review and meta-analysis. The systematic review is based on 59 empirical studies identified from three screening phases, with basic information, types of technologies, types of mental health issues as key points of analysis for synthesis and comparison. The meta-analysis is conducted with 10 qualified experimental studies to determine the overall effect size of digital technology interventions and possible moderating factors.
Results: The results revealed that (1) there is an upward trend in relevant research, comprising mostly experimental and quasi-experimental designs; (2) the common mental health issues include depression, anxiety, bullying, lack of social emotional competence, and mental issues related to COVID-19; (3) among the various technological interventions, mobile applications (apps) have been used most frequently in the diagnosis and treatment of mental issues, followed by virtual reality, serious games, and telemedicine services; and (4) the meta-analysis results indicated that digital technology interventions have a moderate and significant effect size ( g = 0.43) for promoting mental health.
Conclusion: Based on these findings, this study provides guidance for future practice and research on the promotion of adolescent mental health through digital technology.
Systematic review registration: https://inplasy.com/inplasy-2023-12-0004/ , doi: 10.37766/inplasy2023.12.0004 .
1 Introduction
In recent years, the mental health status of children and adolescents (6–18 years old) has been a matter of wide societal concern. The World Health Organization noted that one in seven adolescents suffers from mental issues, accounting for 13% of the global burden of disease in this age group ( World Health Organization, 2021 ). In particular, the emergence of COVID-19 has led to an increase in depression, anxiety, and other psychological symptoms ( Jones et al., 2021 ; Shah et al., 2021 ). There is thus an urgent need to monitor and diagnose the mental health of teenagers.
The development of digital technology has brought about profound socio-economic changes; it also provides new opportunities for mental health diagnosis and intervention ( Goodyear and Armour, 2018 ; Giovanelli et al., 2020 ). First, digital technology breaks the constraints of time and space. It not only provides adolescents with mental health services at a distance but also enables real-time behavioral monitoring for the timely acquisition of dynamic data on adolescents’ mental health ( Naslund et al., 2017 ). Second, due to the still-developing stage of mental health resource building, traditional intervention methods may not be able to meet the increasing demand for mental health services among children and adolescents ( Villarreal, 2018 ; Aschbrenner et al., 2019 ). In addition, as digital natives in the information age, adolescents have the ability to use digital technology proficiently, and social media, such as the internet, has long been integrated into all aspects of adolescents’ lives ( Uhlhaas and Torous, 2019 ). However, it is worth noting that excessive reliance on digital technology (e.g., internet and smartphone addiction) are also common triggers of mental problems among youth ( Wacks and Weinstein, 2021 ). Therefore, we must be aware of the risks posed by digital technology to better utilize it for promoting the mental health of young people.
Mental health, sometimes referred to as psychological health in the literature, encompasses three different perspectives: pathological orientation, positive orientation, and complete orientation ( Keyes, 2009 ). Pathological orientation refers to whether patients exhibit symptoms of mental issues, including internalized mental disorders (e.g., depression and anxiety) and behavioral dysfunctions (e.g., aggression, self-harm) as well as other mental illnesses. Studies have indicated that both internalizing and externalizing disorders belong to different dimensions of mental disorders ( Scott et al., 2020 ), and internalizing symptoms often occur simultaneously with externalizing behaviors ( Essau and de la Torre-Luque, 2023 ). The positive orientation suggests that mental health is a positive mental state, characterized by a person’s ability to fully participate in various activities and to express positive and negative emotions ( Kenny et al., 2016 ). The complete orientation integrates pathological and positive orientation ( Antaramian et al., 2010 ), suggesting that mental health means the absence of mental issues and the presence of subjective well-being ( Suldo and Shaffer, 2008 ). The development of social emotional abilities helps to promote subjective well-being for adolescents during social, emotional, and cognitive development ( Cejudo et al., 2019 ). Adolescents with mental health issues may thus exhibit pathological symptoms or lack of subjective well-being due to a lack of social emotional abilities. In this study, mental health is defined as a psychological state advocated by the complete orientation.
Promoting mental health using digital technology involves providing help through digital tools such as computers, tablets, or phones with internet-based programs ( Hollis et al., 2017 ). Currently, various digital technologies have been tested to address mental health issues in young individuals, including apps, video games, telemedicine, chatbots, and virtual reality (VR). However, the impact of digital technology interventions is affected by various factors ( Piers et al., 2023 ). Efficacy varies based on the kind of mental health issues. Individuals with mental illness related to COVID-19 may profit more from digital interventions than those experiencing depression and anxiety. Moreover, studies reveal that several mental health conditions in young people deteriorate with age, particularly anxiety and suicide attempts ( Tang et al., 2019 ). The impact of digital technology interventions may therefore differ depending on the adolescent’s age. Having psychological problems usually indicates that people are in an unhealthy mental state for a long time, so an enduring intervention may have greater efficacy than a short-term one. Earlier studies have also suggested that the outcomes of treatment are linked to its duration, with patients receiving long-term treatment experiencing better results ( Grist et al., 2019 ).
Although more digital technologies are being used to treat mental health issues, the most important clinical findings have come from strict randomized controlled trials ( Mohr et al., 2018 ). It is still unclear how these interventions affect long-term care or how they would function in real-world settings ( Folker et al., 2018 ). There is much relevant empirical research, but it is scattered, and there is a need for systematic reviews in this area. In previous studies about technology for mental health, Grist et al. (2019) analyzed how digital interventions affect teenagers with depression and anxiety, but their study only considered mental disorders, without considering other mental health issues. Cheng et al. (2019) examined serious games and their application of gamification elements to enhance mental health; however, they overlooked various technological approaches beyond serious games and did not give adequate consideration to the diverse types and features of technology. Eisenstadt et al. (2021) reviewed how mobile apps can help adults between 18 and 45 years of age improve their emotional regulation, mental health, and overall well-being; however, they did not investigate the potential benefits of apps for teenagers.
The present study reviews research from the past decade on digital technology for promoting adolescent mental health. A systematic literature review and meta-analysis are used to explore which types and features of technology can enhance mental health. We believe that the present study makes a meaningful contribution to scholarship because it is among the earliest to report on the impact of technology-enhanced mental health interventions and has revealed crucial influencing factors that merit careful consideration during both research and practical implementation. The following three research questions guided our systematic review and meta-analysis:
1. What is the current status of global research on digital technology for promoting children and adolescent mental health?
2. What digital technology characteristics support the development of mental health among children and adolescents?
3. How effective is digital technology in promoting the mental health of children and adolescents? What factors have an impact on the effectiveness of digital technology interventions?
2 Study 1: systematic literature review
2.1.1 study design.
This study used the systematic literature review method to analyze the relevant literature on the promotion of mental health through digital technology. It followed the Preferred Reporting Items for Systematic Reviews and Meta-Analysis statement for the selection and use of research methods. The protocol for this study was registered with INPLASY (2023120004). Standardized systematic review protocol is used to strictly identify, screen, analyze, and integrate literature ( Bearman et al., 2012 ). To clarify the research issues, systematic literature reviews typically comprise the following six key procedures: planning, literature search, literature assessment, data extraction, data synthesis, and review composition ( Lacey and Matheson, 2011 ).
2.1.2 Literature search
To access high-quality empirical research literature from the past decade, this study selected SCIE and SSCI index datasets from the Web of Science core database and Springer Link. Abstracts containing the English search terms “mental health or psychological health or psychological wellbeing” AND “technology or technological or technologies or digital media” AND “K-12 or teenager or children or adolescents or youth” were retrieved. The search period spanned from January 1, 2013, to July 1, 2023, and 1,032 studies were obtained. To ensure the relevance of the studies to the research question, the relevant inclusion and exclusion criteria were developed based on the 1,032 studies retrieved. The specific criteria are listed in Table 1 .
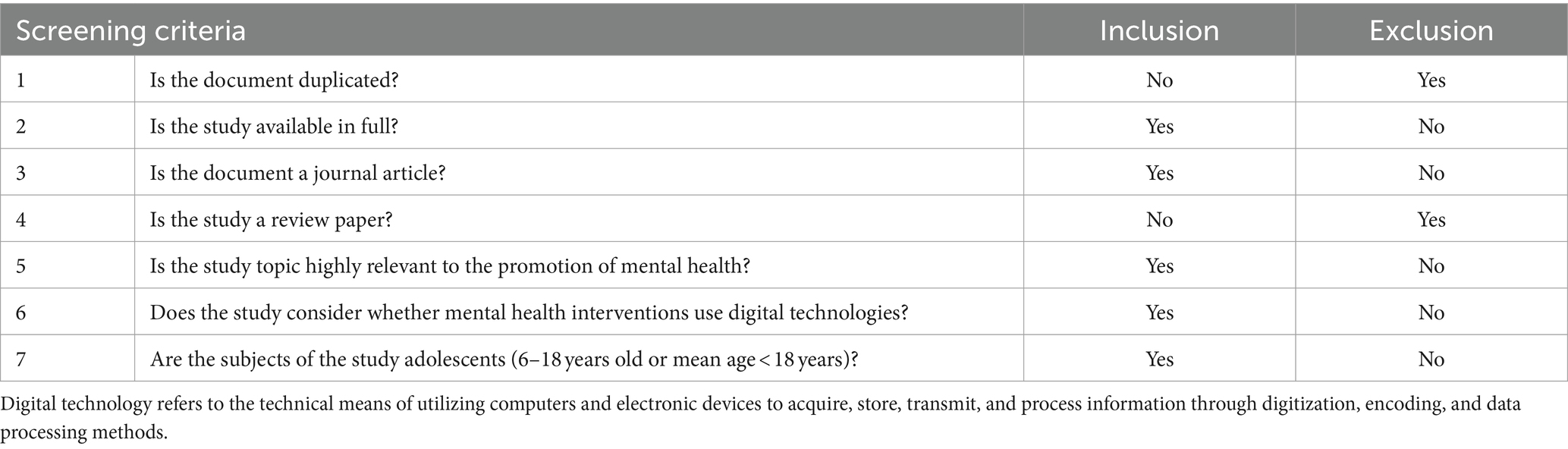
Table 1 . Literature screening criteria.
In this study, we followed a systematic literature review approach and screened the retrieved studies based on the above selection criteria. We conducted three rounds of screening and supplemented new studies through snowballing, ultimately including 59 effective sample documents. The specific process is shown in Figure 1 .
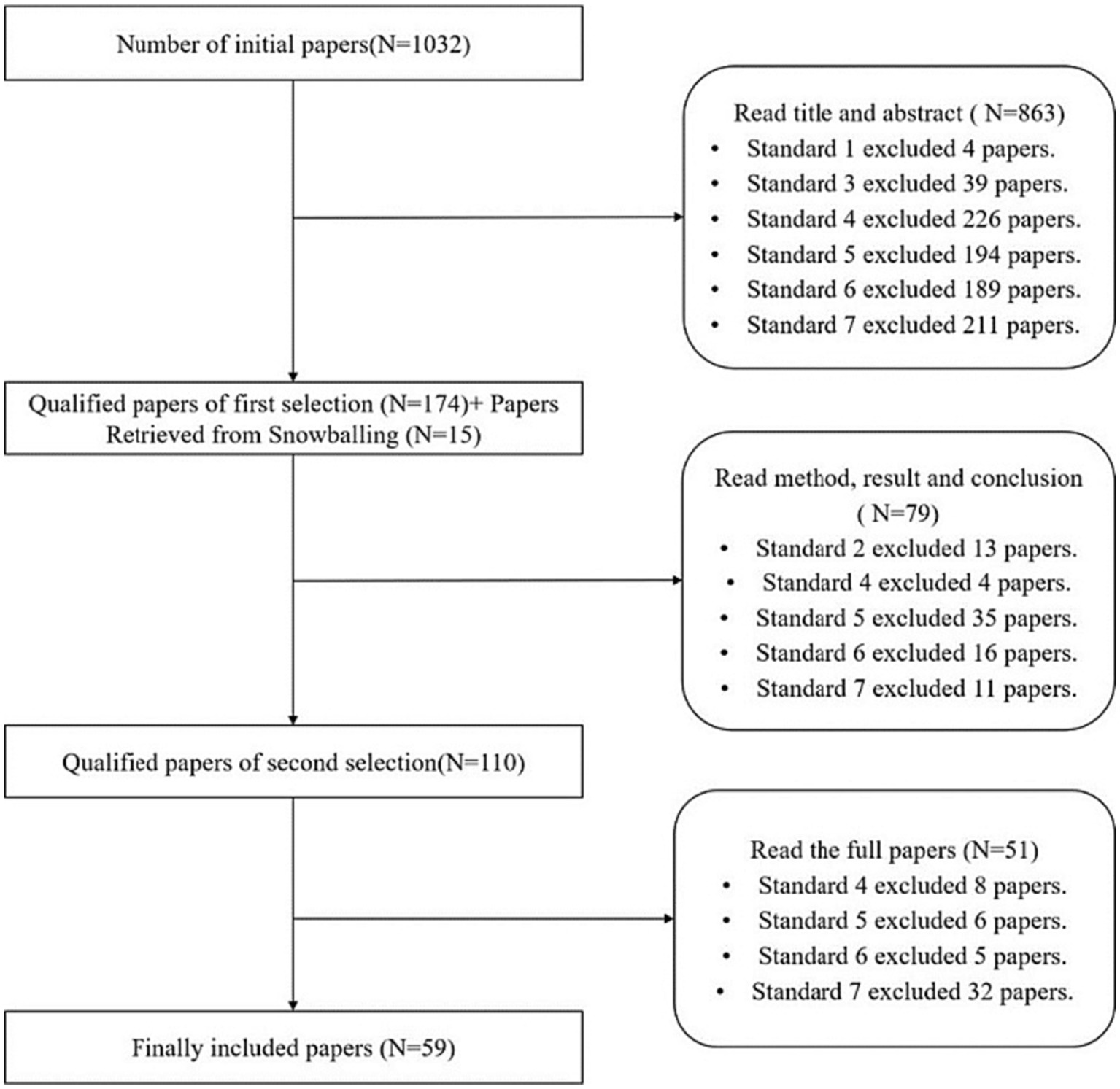
Figure 1 . Screening process and results.
2.1.3 Coding protocol
To extract key information from the included papers, we systematically analyzed 59 studies on the basis of reading the full text. Our coding protocol encompassed the following aspects: (a) basic information about the study, including the first author, publication year, publication region, study type, study object, and intervention duration; (b) the type of technology used in the study, including apps, chatbots, serious games, VR/AR, short messaging service (SMS), telemedicine services, and others; (c) mental health issues, including depression and anxiety, mental illness, bullying, lack of social and emotional competence, mental health issues caused by COVID-19, and other mental health issues; and (d) experimental data (mean, sample size, standard deviation or p -value, t -value, etc.). By capturing basic study information, we establish a foundation for comparing and contextualizing the selected studies. The type of technology used is crucial as it reflects the innovative approaches and their technical affordances. Mental health issues are the core focus that dictates the objectives of the technological interventions as well as their suitability and relevance. Experimental data provides quantifiable evidence to support the effectiveness claims and lays a foundation for the meta-analysis. Together, these four coding aspects offer a holistic view for a comprehensive understanding and analysis of the existing literature. The document coding was completed jointly by the researchers after confirming the coding rules and details through multiple rounds of negotiation. Problems arising in the coding process were intensively discussed to ensure consistency and accuracy of the coding.
2.2 Results and discussion
2.2.1 study and sample characteristics.
As shown in Figure 2 , in terms of the time of publication, the number of studies has gradually increased from 2013 to 2021 along with the development of digital technology. The proportion of studies published in the past 5 years (2019–2023) accounted for 76.3% of the total (45/59), with a peak in 2021 with 15 papers. Social isolation, school suspension, and reduced extracurricular activities caused by COVID-19 may exacerbate mental health issues among children and adolescents, which has attracted more researchers to explore the application of digital technology to mental health treatment.
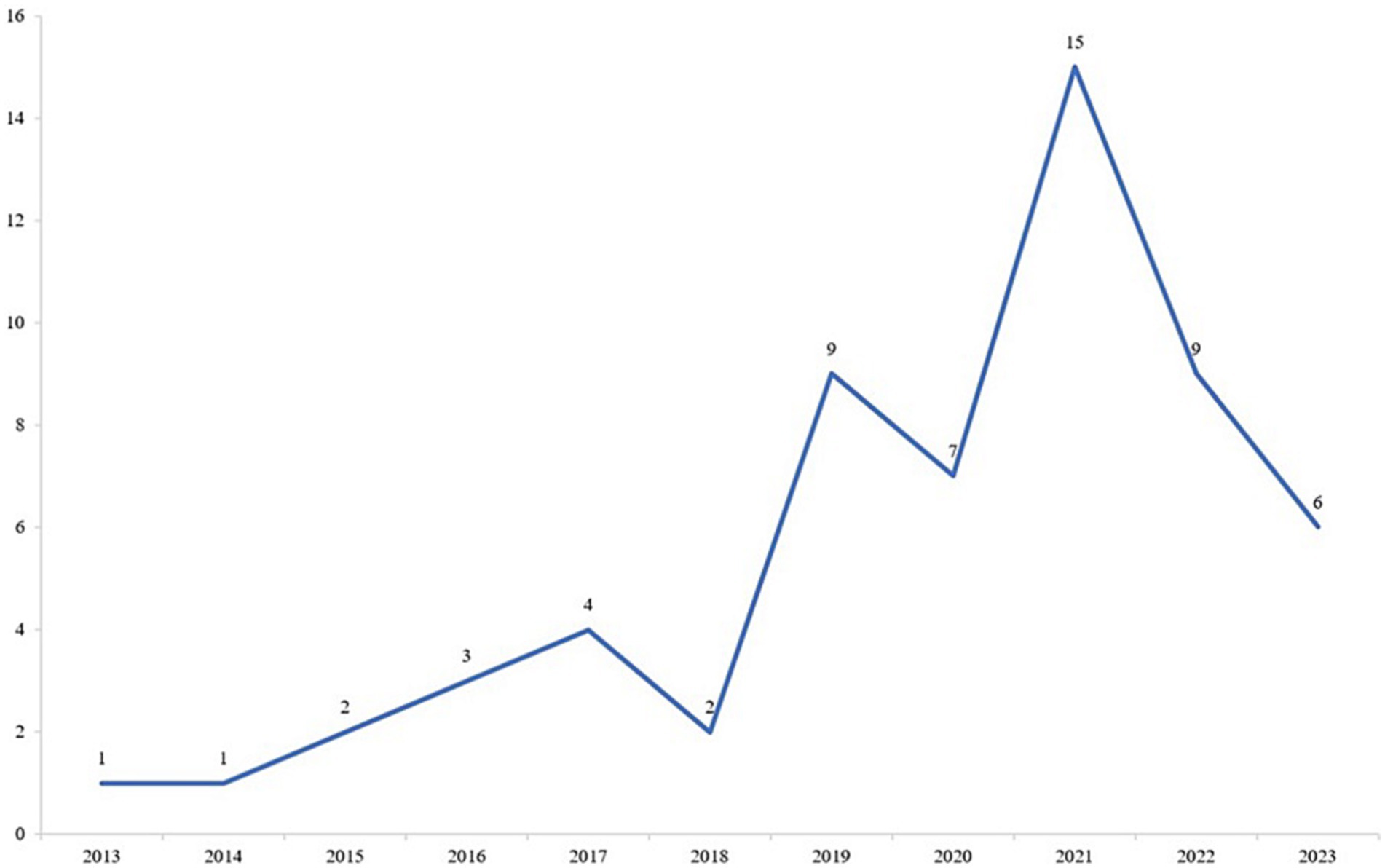
Figure 2 . Trend in the number of studies published in the past decade.
From the perspective of published journals, all of the studies were published in 41 kinds of journals, but two fields were clear leaders: 46 studies (77.97%) were published in medical journals, followed by psychological journals (13.56%). Table 2 shows the source distribution and types of the sample studies. Looking at the country of the first author, the largest number of articles came from the Americas, including the United States and Canada, accounting for 40.7%, followed by European countries, including the United Kingdom and Finland. Only one article came from the African region. In terms of the research types, experimental research was the main type, followed by mixed research, and the number of investigation- and design-based research was relatively small.
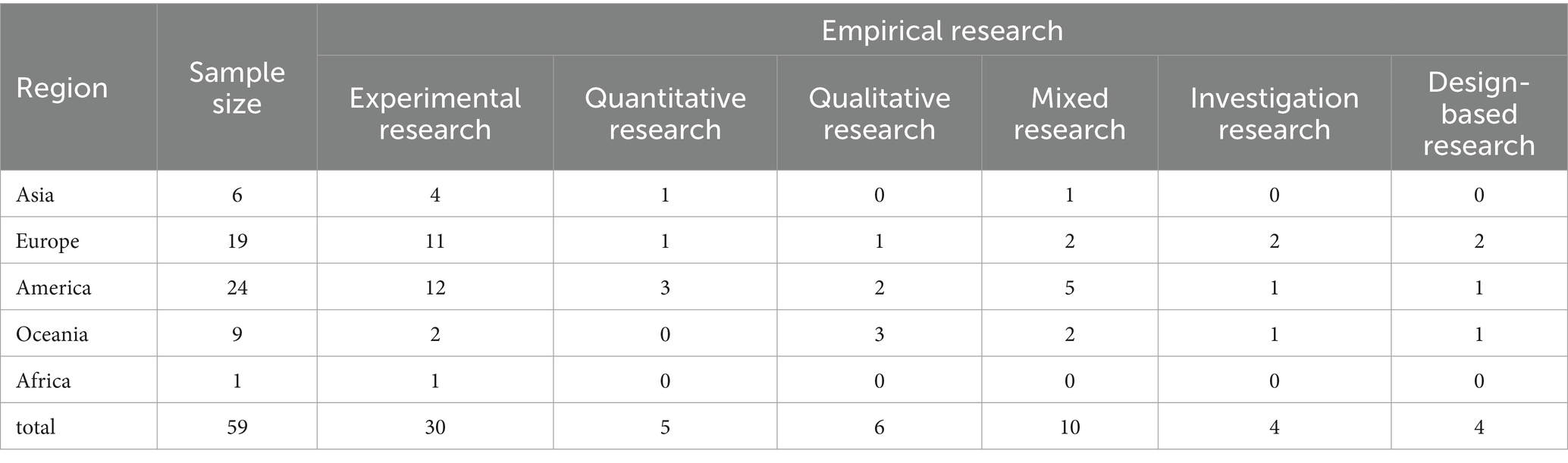
Table 2 . Coding results for sample studies.
Looking more specifically at the research objects, the age range varied from 6 to 18 years. Overall, adolescents aged 13–18 years received more attention, while only six articles considered the younger age group aged 6–12 years. In addition, by coding the sample size of the studies, we found that the quality and size of the studies varied, ranging from small pilot studies or case studies to large-scale cluster studies. For example, Orlowski et al. (2016) conducted a qualitative study on adolescents with experience of seeking help in mental health care institutions in rural Australia; in their study, 10 adolescents with an average age of 18 years were recruited for semi-structured interviews to determine their attitudes and views on the use of technology as a mental health care tool. Another large-scale, randomized controlled trial is planned to enroll 10,000 eighth graders to investigate whether cognitive behavioral therapy (CBT) provided by a smartphone app can prevent depression ( Werner-Seidler et al., 2020 ).
2.2.2 Mental health issues and technology interventions
Based on the coding results, we present the total number of studies that correspond to both mental health issues and technological interventions in Figure 3 . Our findings indicate that apps represent the most prevalent form of digital technology, particularly in addressing depression and anxiety. Telemedicine services also rank highly in terms of utilization. Contrarily, there are comparatively fewer apps involving virtual reality (VR), augmented reality (AR), chatbots, and serious games. Below, we delve into the specifics of digital technology application and its unique affordances, tailored to distinct mental health issues.
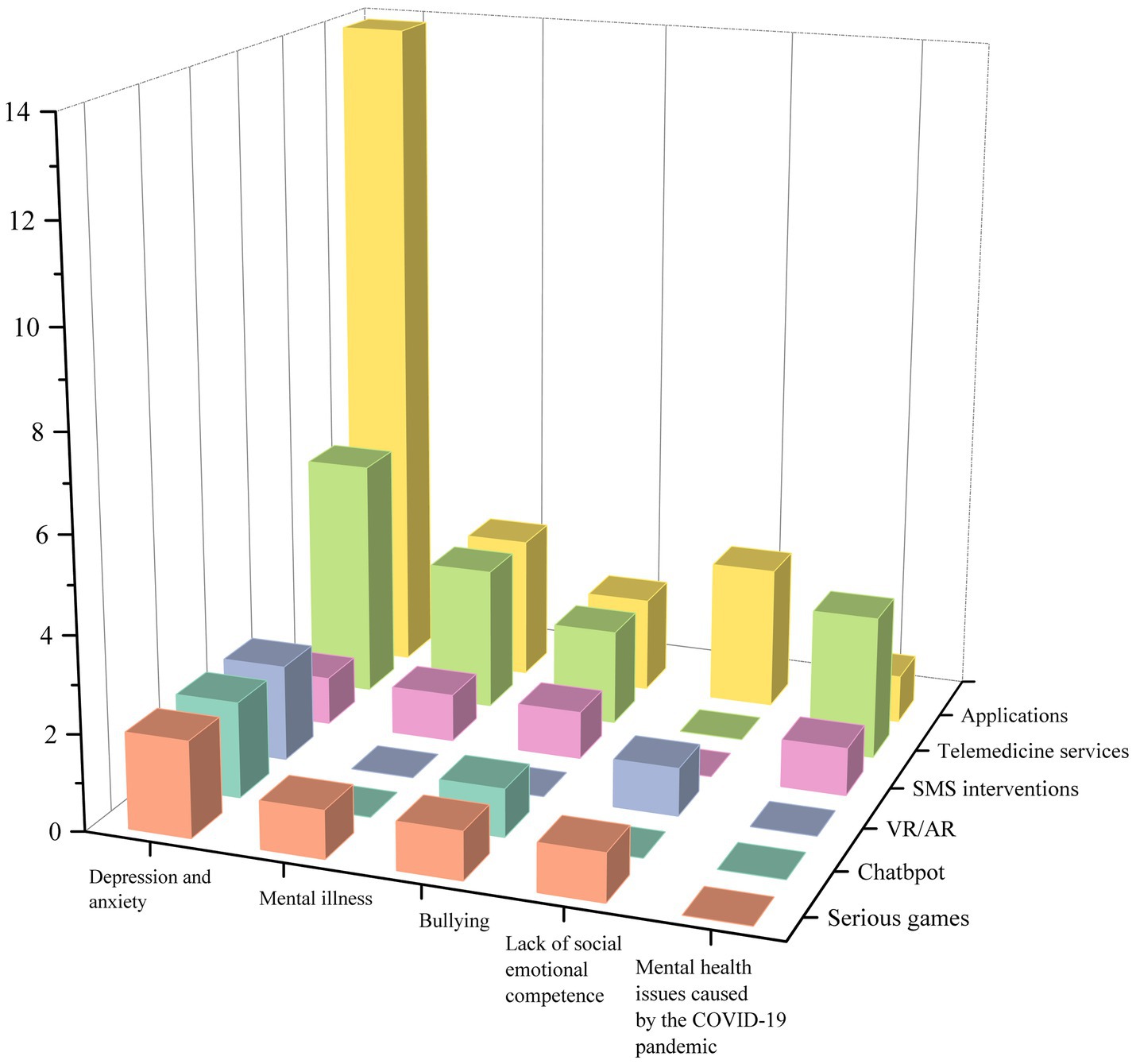
Figure 3 . Numbers of studies by mental health issues and technology interventions.
2.2.2.1 Depression and anxiety
Depression and anxiety in adolescents have become increasingly common, and their presence may signal the beginning of long-term mental health issues, with approximately one in five people experiencing a depressive episode before the age of 18 years ( Lewinsohn et al., 1993 ). This has a range of adverse consequences, including social dysfunction, substance abuse, and suicidal tendency. From the 59 articles considered here, 29 studies used digital technology to treat depression- and anxiety-related symptoms in adolescents. Among the many types of digital technology considered, 19 studies used apps or educational websites as intervention tools, accounting for 76%, followed by serious games, chatbots, and VR with two articles each.
Apps are a broad concept, but they typically refer to software that can be downloaded from app stores to mobile devices such as phones or tablets. Due to characteristics such as their clear structure, ease of use, accessibility, strong privacy, interactivity, and multi-modularity, apps and educational websites are commonly used as tools for technological interventions. For example, Gladstone et al. (2015) developed an interactive website called CATCH-IT to prevent depression in adolescents; the site includes 14 optional modules. The course design of each module applies educational design theories, such as attracting learners’ attention, reviewing content, enhancing memory, and maintaining transfer. Apps and websites can also combine CBT with digital technology. The theoretical framework of CBT is rooted in a core assumption that depression is caused and maintained by unhelpful cognitions and behaviors. Treatment thus focuses on improving the function of these areas by applying skill-based behavioral strategies ( Wenzel, 2017 ). Multiple studies have incorporated CBT’s emphasis on reducing cognitive errors and strengthening positive behavior into their designs by, for example, using fictional storylines to help participants correct irrational thought patterns during reflective tasks, thereby improving patients’ depression conditions ( Stasiak et al., 2014 ; Topooco et al., 2019 ; Neumer et al., 2021 ).
In addition to the intervention methods involving apps and websites, serious games have also become a prospect for treating depression due to their interesting and interactive characteristics. Low-intensity human support combined with smartphone games may potentially reduce the resource requirements of traditional face-to-face counseling. Games contain complete storylines and competitive and cooperative tasks between peers in the form of levels that encourage adolescents to reflect on quizzes at the end of each challenge ( Gonsalves et al., 2019 ). Game designs tend to use flow theory, which emphasizes the dynamic matching of game challenges and the user’s own skill level ( Csikszentmihalyi, 2014 ). During game design, it is necessary to provide users with an easy-to-use and interesting gaming experience, as well as appropriate difficulty challenges, clear rules and goals, and instant feedback, which will help them relax and relieve stress, concentrate on changing cognitive processes, and improve their mood.
Two articles also consider the use of chatbots in interventions. Chatbots act as a dialog agent ( Mariamo et al., 2021 ), which makes the intervention process more interactive. Establishing a relationship of trust between adolescents and chatbots may also help lead to better results in depression and anxiety treatment. Chatbot functions are typically integrated into apps ( Werner-Seidler et al., 2020 ) and tend to be developed as part of the program rather than as a separate technological tool.
In recent years, with the gradual marketization of head-mounted VR devices, VR technology has been increasingly applied to mental health interventions. Studies have shown that the effectiveness of VR apps is often attributed to the distraction created by immersive environments, which produce an illusion of being in a virtual world, thus reducing users’ awareness of painful stimuli in the real world ( Ahmadpour et al., 2020 ). In the treatment of depression and anxiety for adolescents, active distraction supported by VR can engage users in games or cognitive tasks to redirect their attention to virtual objects and away from negative stimuli. Studies have also shown that, in addition to providing immersion, VR should create a pleasant emotional experience (e.g., the thrill of riding a roller coaster) and embed narrative stories (e.g., adventure and exploration) to meet adolescents’ need for achievement ( Ahmadpour et al., 2019 ).
2.2.2.2 Mental illness
In this study, we define mental illness as neurological developmental problems other than depression and anxiety. Among the 59 reviewed articles, 10 were coded as mental illness, including obsessive-compulsive disorder, attention-deficit/hyperactivity disorder, conduct disorder, oppositional defiant disorder, personality disorder, drug addiction, bipolar disorder, and non-suicidal self-injury. For the treatment of mental illness, mobile apps based on CBT appeared twice in 10 articles, while other technology types included SMS intervention, serious games, remote video conferencing, and mobile sensing technology.
Similar to apps for treating depression and anxiety, adolescent patients believe that the apps have good usability and ease of use and can encourage them to share their thoughts, feelings, and behavioral information more openly and honestly while protecting their privacy ( Adams et al., 2021 ). However, due to the severe condition of patients with mental illness, the apps not only are used independently by patients but also serves as a bridge between therapists and patients. Therapists can thus closely monitor treatment progress through behavioral records, which can provide direct feedback to both patients and therapists ( Babiano-Espinosa et al., 2021 ).
SMS interventions send specific content text messages to patients. As a longitudinal intervention method, it is convenient, easy to operate, and low cost. For example, Owens and Charles (2016) sent text messages to adolescents with non-suicidal self-injury behaviors in an attempt to reduce their self-mutilation behaviors. The ultimate effect seemed to be unsatisfactory, as interventions for adolescents with self-mutilation behaviors may be better applied in schools and adolescents’ service agencies, which can help them control their self-mutilation behaviors in the early stages and prevent such behaviors from escalating.
There are also studies that have designed six serious games based on CBT frameworks to treat typical developmental disorders in adolescents, including attention-deficit/hyperactivity disorder, conduct disorder, and oppositional defiant disorder ( Ong et al., 2019 ). In the safe environment provided by the game world, the research subjects shape the behavior of the characters in the context through rule learning and task repetition, which allows them to master emotional management strategies and problem-solving skills. In addition to interventions, digital technology can also be used to evaluate treatment effectiveness and the type of disease. Orr et al. (2023) used mobile sensing technology and digital phenotyping to quantify people’s behavioral data in real time, thereby allowing diagnosis and evaluation of diseases.
2.2.2.3 Bullying
Bullying generally includes traditional bullying and cyberbullying. Traditional bullying usually manifests as direct physical violence or threats of abuse against victims, as well as indirect methods such as spreading rumors and social exclusion. Cyberbullying is defined as intentional harm to others through computers, mobile phones, and other electronic devices. Data show that, as of 2021, the proportion of adolescents who have experienced cyberbullying in the United States may be as high as 45.5% ( Patchin, 2021 ), which indicates that it has become a serious social problem. Among the nine articles on the topic of bullying and cyberbullying, three used SMS intervention methods, and two used mobile apps; chatbots, technology-supported courses, and CBT-based telemedicine services were also used in the mental health treatment for patients who had been bullied and cyberbullied.
The SMS intervention for bullying implemented personalized customization, and the automatic SMS content can be customized based on the subjects’ previous questionnaire or completed self-report status ( Ranney et al., 2019 ). The subjects are required to rate their feelings at the end of the day and report whether they were bullied that day. The psychotherapist then made adjustments based on their actual situation, and if necessary, the psychotherapist would also contact specific subjects to provide offline psychological counseling services ( Ranney et al., 2019 ). In addition to having similar functions as the SMS intervention ( Kutok et al., 2021 ), mobile apps can provide opportunities for personalized learning, where a variety of learning methods can be applied (e.g., providing therapist guidance, conducting meetings, and conducting family practice activities) to promote the acquisition of mental health skills ( Davidson et al., 2019 ). Furthermore, for adolescents, touchscreen learning, interactive games, and video demonstrations can enhance their enthusiasm for participating in the treatment process.
Chatbots with specific names and images were also used to guide research subjects through a series of online tasks in the form of conversations, including watching videos involving bullying and cyberbullying among adolescents, provoking self-reflection through questions and suggestions, and providing constructive strategic advice ( Gabrielli et al., 2020 ). Digital technology-supported courses and CBT-based telemedicine services both make full use of the convenience of technology, effectively addressing the time- and location-based limitations of traditional face-to-face treatment. Digital courses can be implemented on a large scale in schools through teacher training, and compared with professional medical services, such courses have a wider target audience and can play a scientific and preventive role in bullying and cyberbullying. Telemedicine services refer to the use of remote communication technology to provide psychological services ( Joint Task Force for the Development of Telepsychology Guidelines for Psychologists, 2013 ). For families with severely troubled adolescents, telemedicine allows parents and children to meet together, increasing the flexibility of timing, and one-on-one video services can help to build a closer relationship between patients and therapists.
2.2.2.4 Lack of social emotional competence
In research, social emotional competence typically refers to the development of emotional intelligence in adolescents ( De la Barrera et al., 2021 ), which also includes personal abilities (self-awareness and self-management), interpersonal relationships (social awareness and interpersonal skills), and cognitive abilities (responsible decision-making) ( Collaborative for Academic, Social, and Emotional Learning, 2020 ). It is an important indicator for measuring the mental health level of adolescents. People with positive social emotional intelligence are less likely to experience mental health issues such as depression, anxiety, and behavioral disorders. Using digital technology to promote social emotional development is becoming increasingly common, and in six intervention studies on social emotional competence, apps, serious games, VR technology, and SMS interventions were used.
The studies considered all emphasized the importance of interactive design in digital technology to enhance social and emotional skills, as interactive technology can increase students’ engagement, resulting in positive learning experiences. For example, Cherewick et al. (2021) designed a smartphone app that can be embedded with multimedia learning materials, allowing adolescents to watch social and emotional skill–related learning videos autonomously and complete topic reflection activities with family/peers after school. The app also has rich teaching interaction functions, allowing teachers to evaluate and share course and learning materials, which can provide pleasant learning experiences to students while also improving the flexibility of teaching. In addition to teacher–student interaction, another paper also mentioned the importance of human–computer interaction for developing social emotional competence. The fun and interactivity of the app are the key to attracting adolescents to download and use it, and it can also have a positive effect on improving students’ self-management and decision-making skills ( Kenny et al., 2016 ).
Unlike the treatment of depression and anxiety, the application of VR in the cultivation of social emotional competence not only relies on its highly immersive characteristics but also emphasizes the positive effects of multi-sensory experiences on emotional regulation. By utilizing various sensor devices and visualization devices, adolescents are provided with ideal visual, auditory, and tactile guidance and regulation, which can enhance their emotional regulation abilities and relieve psychological stress ( Wu et al., 2022 ). Existing studies have integrated dance and music into virtual scenes ( Liu et al., 2021 ), using virtual harmonic music therapy to allow users to relax physically and mentally while enjoying music, thereby reducing stress and anxiety. VR technology is also highly adaptable and generalizable, which can help in building diverse scenes that meet the psychological expectations of patients based on the characteristics of the different treatment objects.
2.2.2.5 Mental health issues caused by the COVID-19 pandemic
The global outbreak of COVID-19 created severe challenges for the mental health of adolescents. Factors such as lack of social contact, lack of personal space at home, separation from parents and relatives, and concerns about academics and the future have exacerbated mental health risks, leading to increased loneliness, pain, social isolation, mental disorders, and symptoms of anxiety, depression, and stress. The reports from five studies indicated that the COVID-19 pandemic has exacerbated mental health issues in adolescents. During the pandemic, technology—which is not limited by time and space—became the preferred method of treatment. Apps, remote health services, and online training courses were used in research. The apps were resource-oriented and evidence-based interventions that allowed patients to interact with therapists through remote conferencing and encouraged patients to self-reflect and express themselves after the conference to improve their mental condition ( Gómez-Restrepo et al., 2022 ). Remote health services combined CBT and dialectical behavior therapy with professional counselors engaging in online communication with patients for several weeks. This was in line with research that indicates that the establishment of a positive relationship between therapists and patients is the foundation for obtaining good effect ( Zepeda et al., 2021 ).
2.2.2.6 Other mental health issues
In addition to the common mental health issues mentioned above, there were also interventions mentioned in the literature for improving body image anxiety, mental issues caused by hospitalization, and reading disabilities through digital technology means. Due to its high-immersion and simulation characteristics, VR technology was selected for improving mental health issues such as loneliness, disconnection from peers, and academic anxiety caused by hospitalization ( Thabrew et al., 2022 ). Immersive VR experience technology used 360° panoramic live broadcast and VR headphones to enable hospitalized adolescents to indirectly participate in social activities through cameras in school or home environments, as well as to contact peers and teachers through methods such as text messages; such interventions are conducive to improving social inclusion, social connectivity, and happiness. Furthermore, two studies mentioned body image anxiety, especially targeting female audiences, and the research integrated body image CBT techniques into serious games and chatbots ( Mariamo et al., 2021 ; Matheson et al., 2021 ), using interesting interactive exploration and free dialog forms to help adolescents gain a correct understanding of body image and solve body image anxiety issues.
Another study used eye-tracking technology to treat children with reading disabilities ( Davidson et al., 2019 ). The researcher developed a reading evaluation platform called Lexplore, which used eye-tracking technology to monitor children’s eye movements when reading to determine the cognitive processes behind each child’s individual reading style and then design appropriate strategies to improve their reading difficulties.
3 Study 2: meta-analysis
To explore the effect of digital technology in promoting mental health, this study used a meta-analysis to assess 10 papers. It includes both experimental and quasi-experimental research studies. CMA3.0 (Comprehensive Meta-Analysis 3.0) was used, and the meta-analysis process consisted of five phases.
Phase 1: Literature screening, based on the prior stage of literature information coding. Relevant literature was filtered using the following criteria for meta-analysis: (a) the study must compare “technical intervention” and “traditional intervention”; (b) the study should report complete data that can generate the effect amount (e.g., average, sample size, standard deviation or t -values, p -values, etc.); and (c) the dependent variables in the study should contain at least one aspect of mental health.
Phase 2: Effect size calculation. In the case of a large sample size, there is little difference between Cohen’s d, Glass, and Hedges’ g values, but Cohen’s d can significantly overestimate the effect size for studies with a small sample ( Hedges, 1981 ). Therefore, Hedges’ g was used as the effect size indicator in this study.
Phase 3: Model selection. Meta-analyses include fixed- and random-effects models. Different models may produce different effect sizes. Due to the differences in sample size, experimental procedures, and methods among the initial studies included in the meta-analysis, the estimated average effect values may not be completely consistent with the true population effect values, which results in sample heterogeneity. This study used the method proposed by Borenstein et al. (2009) to establish fixed- and random-effects models to eliminate the influence of sample heterogeneity. When the heterogeneity test ( Q value) results were significant, the random-effects model was used; otherwise, the fixed-effects model was used.
Phase 4: Testing of main effects and moderating effects. Based on the selected model, a test of the main effects was conducted. Meanwhile, if heterogeneity was present, a test of moderating effects could be conducted.
Phase 5: Publication bias test. Publication bias is a common systematic error in meta-analyses and refers to a tendency for significantly significant research results to be more likely to be published than non-significant results. This study used a funnel plot to visually assess publication bias qualitatively and then further quantitatively assessed publication bias using Begg’s rank correlation method and the trim and fill method.
3.2 Results and discussion
3.2.1 inclusion and coding results.
For the studies that met the requirements of the meta-analysis, detailed classification was carried out based on the following variables one-by-one on the basis of the systematic review coding: (a) basic information (authors, year, sample size); (b) age stage, which is divided into three categories: primary school, junior high school, and senior high school; (c) mental health issues, including depression, bullying, and mental health issues caused by COVID-19; (d) technology type, including app, telemedicine, and chatbots; (e) intervention duration, coded as short-term for interventions less than a month and long-term for intervention that lasted more than a month; and (f) effect size. The coding results are shown in Table 3 .
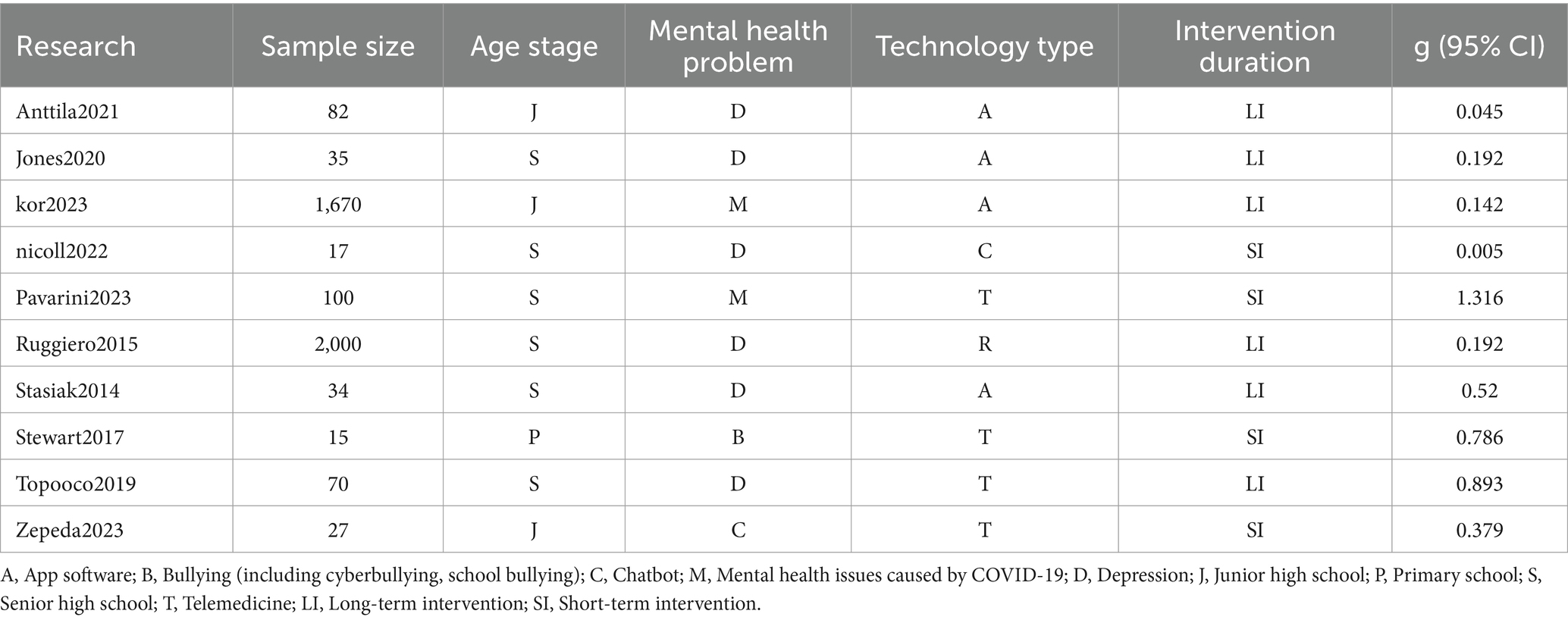
Table 3 . Research coding results included in meta-analysis.
3.2.2 The overall effect of digital technology on mental health outcomes
According to the results of the heterogeneity test in Table 4 , the Q test is significant ( p < 0.001), which indicates that there is significant heterogeneity among the samples. The random-effects model was therefore selected as the more reasonable option. The pooled effect size is 0.43. According to the criteria proposed by Cohen (1992) , 0.2, 0.5, and 0.8 are considered the boundaries of small, medium, and large effect sizes, respectively. It can be seen that the effect size for the promotion of mental health by digital technology is moderate and significant. At the same time, the lower limit of the 95% confidence interval is greater than 0 for each study, which indicates that the probability of the effect size being caused by chance is very small. In addition, the I 2 value is 78.164, which indicates that the heterogeneity between studies is high. Important moderating variables therefore may exist ( Higgins and Green, 2008 ), and additional moderating effect tests need to be conducted.

Table 4 . Overall effect of technology on mental health.
3.2.3 Moderating effect test
Moderating effect tests were conducted on four variables: age stage, mental health issues, technology type, and intervention duration. As shown in Table 5 , among the four moderating variables, only the age stage has a significant moderating effect ( p < 0.05). In particular, the effect size is the largest for the primary school stage, followed by the senior high school stage with a moderate promoting effect. In addition, although the effect size for the junior high school stage is small, it is still significant, which may be related to the limited number of studies considering this population. The results also indicate that the moderating effects of mental health issues, technology type, and intervention duration are not significant. However, it can be seen that digital technology methods have the largest effect size for treating psychological problems caused by COVID-19, while compared with apps and chatbots, remote medical services can achieve better effects. In terms of treatment duration, the effect size for short-term interventions is greater than that for long-term interventions.
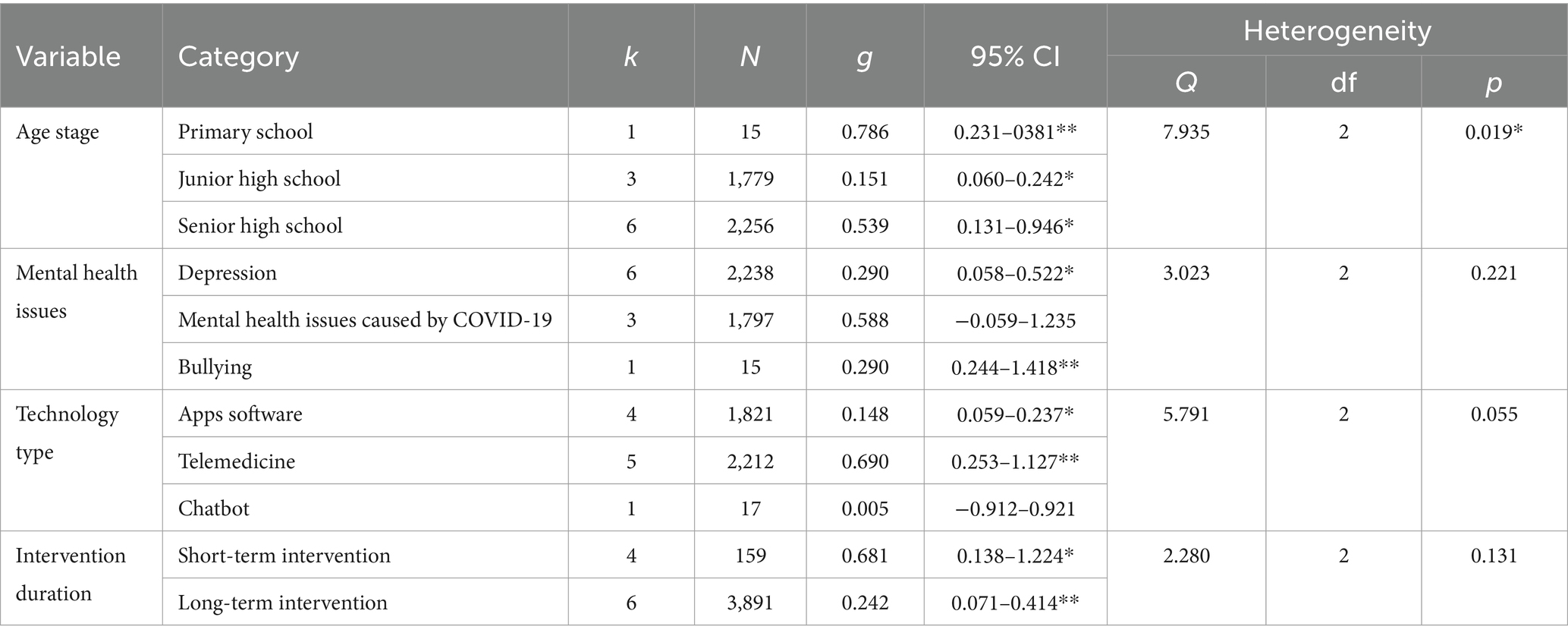
Table 5 . Regulatory effect test of technology (random-effect model).
3.2.4 Publication bias test
This study used funnel plots, Begg’s test, and the trim and fill method for the publication bias test. As shown in Figure 4 , the distribution of effect values in the study shows uneven and asymmetric distribution on both sides of the mean effect value, which initially suggests the possibility of publication bias. Begg’s test was thus used for further testing. Begg’s test is a method of quantitatively identifying bias using a rank correlation test, and it applies to studies with a small sample. The result of Begg’s test shows that t = 0.267, p = 0.283, Z = 1.01 < 1.96, which indicates that there is no obvious publication bias. Finally, the censoring method was used to censor the literature on both sides of the effect value, and this revealed that the effect value was still significant. In summary, there is negligible publication bias.
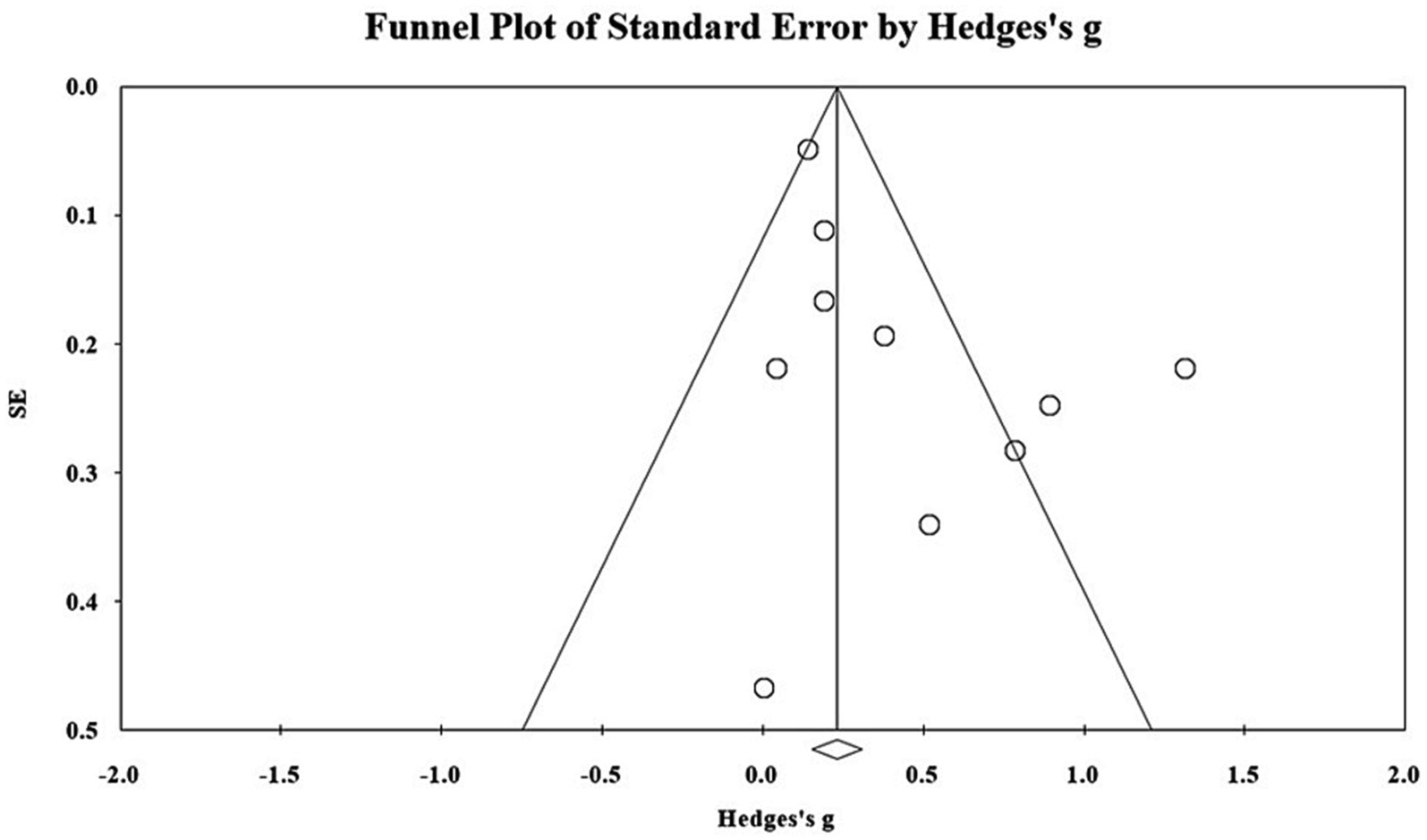
Figure 4 . Distributions of effect sizes for mental health treatment outcomes.
4 Conclusion and implications
4.1 summary of key findings.
This study made a systematic review and meta-analysis of 59 studies on digital technology promoting adolescents’ mental health over the past decade. Based on the investigation of current research, the types and characteristics of the commonly used technology interventions for different mental health issues were analyzed, and the actual effects and potential regulatory variables of digital technology in promoting mental health were investigated in the meta-analysis. The main findings are outlined below.
• Over the past decade, especially between 2013 and 2021, the number of studies on digital technology promoting adolescents’ mental health has generally shown an upward trend, with nearly 80% of the literature being published in medical journals.
• Digital technology is most commonly used to intervene in the mental health issues of adolescents aged 13–18 years, and children in the younger age group (6–12 years old) receive relatively less attention.
• Depression and anxiety disorders are the mental health issues that received the most research attention, followed by obsessive-compulsive disorder, attention-deficit hyperactivity disorder, conduct disorder, and other mental illnesses. There were also studies on, in decreasing order of the number of studies, bullying, social emotional competence deficiency, and mental health issues caused by COVID-19, dyslexia, and adolescent body image anxiety.
• Apps with convenience, ease of use, interactivity, and remote communication were most commonly used to treat mental health issues. Serious games, remote health services, and text message intervention were less often used, and only three studies used VR, which is difficult to realize for mental health treatment.
• Digital technology plays a significant role in promoting the treatment of mental health issues of adolescents, especially in primary and senior high school.
4.2 Interpretation and insights
The findings of this study highlight the nuanced role played by digital technology in promoting mental health for children and adolescents. While technology has broadened the scope of mental health interventions with innovative apps and programs, it should be viewed as a complement to traditional face-to-face approaches, not a replacement ( Aguilera, 2015 ), as they cannot replicate the personal connection and empathy provided by a trained mental health professional. Moreover, different technologies vary in effectiveness for specific mental health issues, emphasizing the need for careful evaluation of their benefits and limitations. For instance, virtual reality, cognitive behavioral therapy apps, and online support platforms have shown promise for in addressing depression and anxiety, but their effects vary depending on individual needs and contexts, suggesting the non-uniform efficacy of digital technologies across mental health conditions.
Furthermore, this study also draws attention to the limited incorporation of digital technology in mental health education, especially among children aged 6 to 12. Given the significance of this developmental stage, where emotional management, relationships, and mental health knowledge are crucial, innovative digital approaches that draw upon the unique affordances of mobile apps, online courses, and virtual reality are warranted to deliver interactive and personalized learning experiences. Nevertheless, this innovation poses challenges and risks, including addiction to virtual environments and a reduction in social activities, which can also negatively impact the mental health of youth ( Taylor et al., 2020 ). Therefore, striking a balance between harnessing technology’s potential and mitigating its risks is essential, emphasizing the need for responsible and targeted use of digital tools in mental healthcare and education.
4.3 Implication for practice and future research
Based on the results of the systematic review and meta-analysis, this study puts forward the relevant implications for practice and research. First, for mental health education service personnel, we suggest that the first step is to fully utilize the characteristics of digital technology and select the most appropriate digital intervention tools for different mental health issues. For example, apps are more suitable for the treatment of depression, anxiety, and mental illnesses. When facing adolescents who have been bullied, text message interventions may be a good choice. In addition, serious games and VR could play a greater role in developing adolescents’ social emotional competence.
Second, for mental health counselors or school mental health workers, it is necessary to consider learner characteristics and intervention duration, among other factors. In contrast to previous research results ( Tang et al., 2019 ), we found that the regulating effect of age was significant, so therapists need to implement personalized technical interventions for adolescents at different age stages. Short-term interventions seem to induce a greater effect size, so lengthy interventions should be avoided, as they are more likely to cause marginal effect and develop technical immunity for the youth population.
Third, for technology intervention developers, it is important to recognize that not all practitioners (e.g., psychologists, therapists) are technology savvy. In the process of designing mental health apps and VR interventions, it is necessary to provide sufficient technical support, such as instructional manuals and tutorial videos, to reduce the potential digital divide. It is also essential to arrange for appropriate technical personnel to provide safeguard services and training continuously, ensuring the personal safety and cybersecurity of practitioners and patients during intervention sessions.
For researchers, we suggest that, first, more empirical studies are needed to report first-hand experimental results. Most of the existing studies only described the experimental scheme and lacked key research results. It is hoped that future research will report the results as comprehensively as possible to improve the credibility and reliability of meta-analytical results. Second, the number of studies on moderating effects in the meta-analysis was relatively small. For example, there was only one study on the primary school population. Future research needs to focus on people who have paid less attention to existing studies and thus enhance the understanding of technology interventions in mental health. Finally, there have been few studies that analyze cost-effectiveness, which is key to determining whether technical interventions can be normalized and sustainable. Future studies need to conduct sufficient investigation and report on the cost-effectiveness of digital technology interventions, including the development and maintenance costs of VR ( Kraft, 2020 ).
Data availability statement
The raw data supporting the conclusions of this article will be made available by the authors, without undue reservation.
Author contributions
TC: Data curation, Formal analysis, Investigation, Visualization, Writing – original draft. JO: Formal analysis, Investigation, Writing – original draft. GL: Formal analysis, Writing – review & editing. HL: Conceptualization, Methodology, Supervision, Writing – review & editing.
The author(s) declare that no financial support was received for the research, authorship, and/or publication of this article.
Conflict of interest
The authors declare that the research was conducted in the absence of any commercial or financial relationships that could be construed as a potential conflict of interest.
Publisher’s note
All claims expressed in this article are solely those of the authors and do not necessarily represent those of their affiliated organizations, or those of the publisher, the editors and the reviewers. Any product that may be evaluated in this article, or claim that may be made by its manufacturer, is not guaranteed or endorsed by the publisher.
Adams, Z., Grant, M., Hupp, S., Scott, T., Feagans, A., Phillips, M. L., et al. (2021). Acceptability of an mHealth app for youth with substance use and mental health needs: iterative, mixed methods design. JMIR Form. Res. 5:e30268. doi: 10.2196/30268
PubMed Abstract | Crossref Full Text | Google Scholar
Aguilera, A. (2015). Digital technology and mental health interventions: opportunities and challenges. Arbor 191:a210. doi: 10.3989/arbor.2015.771n1012
Crossref Full Text | Google Scholar
Ahmadpour, N., Randall, H., Choksi, H., Gao, A., Vaughan, C., and Poronnik, P. (2019). Virtual reality interventions for acute and chronic pain management. Int. J. Biochem. Cell Biol. 114:105568. doi: 10.1016/j.biocel.2019.105568
Ahmadpour, N., Weatherall, A. D., Menezes, M., Yoo, S., Hong, H., and Wong, G. (2020). Synthesizing multiple stakeholder perspectives on using virtual reality to improve the periprocedural experience in children and adolescents: survey study. J. Med. Internet Res. 22:e19752. doi: 10.2196/19752
Antaramian, S. P., Huebner, E. S., Hills, K. J., and Valois, R. F. (2010). A dual-factor model of mental health: toward a more comprehensive understanding of youth functioning. Am. J. Orthopsychiatry 80, 462–472. doi: 10.1111/j.1939-0025.2010.01049.x
Aschbrenner, K. A., Naslund, J. A., Tomlinson, E. F., Kinney, A., Pratt, S. I., and Brunette, M. F. (2019). Adolescents' use of digital technologies and preferences for mobile health coaching in public mental health settings. Front. Public Health 7:178. doi: 10.3389/fpubh.2019.00178
Babiano-Espinosa, L., Wolters, L. H., Weidle, B., Compton, S. N., Lydersen, S., and Skokauskas, N. (2021). Acceptability and feasibility of enhanced cognitive behavioral therapy (eCBT) for children and adolescents with obsessive-compulsive disorder. Child Adolesc. Psychiatry Ment. Health 15:47. doi: 10.1186/s13034-021-00400-7
Bearman, M., Smith, C. D., Carbone, A., Slade, S., Baik, C., Hughes-Warrington, M., et al. (2012). Systematic review methodology in higher education. High. Educ. Res. Dev. 31, 625–640. doi: 10.1080/07294360.2012.702735
Borenstein, M., Hedges, L. V., Higgins, J. P. T., and Rothstein, H. R. (2009). Introduction to meta-analysis Wiley.
Google Scholar
Cejudo, J., López-Delgado, M. L., and Losada, L. (2019). Effectiveness of the videogame “Spock” for the improvement of the emotional intelligence on psychosocial adjustment in adolescents. Comput. Hum. Behav. 101, 380–386. doi: 10.1016/j.chb.2018.09.028
Cheng, V. W. S., Davenport, T., Johnson, D., Vella, K., and Hickie, I. B. (2019). Gamification in apps and technologies for improving mental health and well-being: systematic review. JMIR Mental Health 6:e13717. doi: 10.2196/13717
Cherewick, M., Lebu, S., Su, C., Richards, L., Njau, P. F., and Dahl, R. E. (2021). Study protocol of a distance learning intervention to support social emotional learning and identity development for adolescents using interactive mobile technology. Front. Public Health 9:623283. doi: 10.3389/fpubh.2021.623283
Cohen, J. (1992). A power primer. Psychol. Bull. 112, 155–159. doi: 10.1037/0033-2909.112.1.155
Collaborative for Academic, Social, and Emotional Learning (2020), What is the CASEL framework? A framework creates a foundation for applying evidence-based SEL strategies to your community. Available at: https://casel.org/fundamentals-of-sel/what-is-the-casel-framework/
Csikszentmihalyi, M. (2014). “Learning, “flow,” and happiness” in Applications of flow in human development and education (Dordrecht: Springer), 153–172.
Davidson, T. M., Bunnell, B. E., Saunders, B. E., Hanson, R. F., Danielson, C. K., Cook, D., et al. (2019). Pilot evaluation of a tablet-based application to improve quality of care in child mental health treatment. Behav. Ther. 50, 367–379. doi: 10.1016/j.beth.2018.07.005
De la Barrera, U., Postigo-Zegarra, S., Mónaco, E., Gil-Gómez, J.-A., and Montoya-Castilla, I. (2021). Serious game to promote socioemotional learning and mental health (emoTIC): a study protocol for randomised controlled trial. BMJ Open 11:e052491. doi: 10.1136/bmjopen-2021-052491
Eisenstadt, M., Liverpool, S., Infanti, E., Ciuvat, R. M., and Carlsson, C. (2021). Mobile apps that promote emotion regulation, positive mental health, and well-being in the general population: systematic review and meta-analysis. JMIR Mental Health 8:e31170. doi: 10.2196/31170
Essau, C. A., and de la Torre-Luque, A. (2023). Comorbidity between internalising and externalising disorders among adolescents: symptom connectivity features and psychosocial outcome. Child Psychiatry Hum. Dev. 54, 493–507. doi: 10.1007/s10578-021-01264-w
Folker, A. P., Mathiasen, K., Lauridsen, S. M., Stenderup, E., Dozeman, E., and Folker, M. P. (2018). Implementing internet-delivered cognitive behavior therapy for common mental health disorders: a comparative case study of implementation challenges perceived by therapists and managers in five European internet services. Internet Interv. 11, 60–70. doi: 10.1016/j.invent.2018.02.001
Gabrielli, S., Rizzi, S., Carbone, S., and Donisi, V. (2020). A chatbot-based coaching intervention for adolescents to promote life skills: pilot study. JMIR Hum. Factors 7:e16762. doi: 10.2196/16762
Giovanelli, A., Ozer, E. M., and Dahl, R. E. (2020). Leveraging technology to improve health in adolescence: a developmental science perspective. J. Adolesc. Health 67, S7–S13. doi: 10.1016/j.jadohealth.2020.02.020
Gladstone, T. G., Marko-Holguin, M., Rothberg, P., Nidetz, J., Diehl, A., DeFrino, D. T., et al. (2015). An internet-based adolescent depression preventive intervention: study protocol for a randomized control trial. Trials 16:203. doi: 10.1186/s13063-015-0705-2
Gómez-Restrepo, C., Sarmiento-Suárez, M. J., Alba-Saavedra, M., Bird, V. J., Priebe, S., and van Loggerenberg, F. (2022). Adapting DIALOG+ in a school setting-a tool to support well-being and resilience in adolescents living in postconflict areas during the COVID-19 pandemic: protocol for a cluster randomized exploratory study. JMIR Res. Protoc. 11:e40286. doi: 10.2196/40286
Gonsalves, P. P., Hodgson, E. S., Kumar, A., Aurora, T., Chandak, Y., Sharma, R., et al. (2019). Design and development of the "POD adventures" smartphone game: a blended problem-solving intervention for adolescent mental health in India. Front. Public Health 7:238. doi: 10.3389/fpubh.2019.00238
Goodyear, V. A., and Armour, K. M. (2018). Young people’s perspectives on and experiences of health-related social media, apps, and wearable health devices. Soc. Sci. 7:137. doi: 10.3390/socsci7080137
Grist, R., Croker, A., Denne, M., and Stallard, P. (2019). Technology delivered interventions for depression and anxiety in children and adolescents: a systematic review and meta-analysis. Clin. Child. Fam. Psychol. Rev. 22, 147–171. doi: 10.1007/s10567-018-0271-8
Hedges, L. V. (1981). Distribution theory for glass's estimator of effect size and related estimators. J. Educ. Stat. 6, 107–128. doi: 10.3102/10769986006002107
Higgins, J. P., and Green, S. (2008). Cochrane handbook for systematic reviews of interventions: Cochrane book series . Hoboken, New Jersey: Wiley.
Hollis, C., Falconer, C. J., Martin, J. L., Whittington, C., Stockton, S., Glazebrook, C., et al. (2017). Annual research review: digital health interventions for children and young people with mental health problems – a systematic and meta-review. J. Child Psychol. Psychiatry 58, 474–503. doi: 10.1111/jcpp.12663
Joint Task Force for the Development of Telepsychology Guidelines for Psychologists (2013). Guidelines for the practice of telepsychology. Am. Psychol. 68, 791–800. doi: 10.1037/a0035001
Jones, E. A. K., Mitra, A. K., and Bhuiyan, A. R. (2021). Impact of COVID-19 on mental health in adolescents: a systematic review. Int. J. Environ. Res. Public Health 18:2470. doi: 10.3390/ijerph18052470
Kenny, R., Dooley, B., and Fitzgerald, A. (2016). Developing mental health mobile apps: exploring adolescents’ perspectives. Health Informatics J. 22, 265–275. doi: 10.1177/1460458214555041
Keyes, C. L. M. (2009). “Toward a science of mental health” in The Oxford handbook of positive psychology (Oxford: Oxford University Press), 88–96.
Kraft, M. A. (2020). Interpreting effect sizes of education interventions. Educ. Res. 49, 241–253. doi: 10.3102/0013189x20912798
Kutok, E. R., Dunsiger, S., Patena, J. V., Nugent, N. R., Riese, A., Rosen, R. K., et al. (2021). A cyberbullying media-based prevention intervention for adolescents on instagram: pilot randomized controlled trial. JMIR Mental Health 8:e26029. doi: 10.2196/26029
Lacey, F. M., and Matheson, L. (2011). Doing your literature review: Traditional and systematic techniques . London: Sage.
Lewinsohn, P. M., Hops, H., Roberts, R. E., Seeley, J. R., and Andrews, J. A. (1993). Adolescent psychopathology: I. Prevalence and incidence of depression and other DSM-III—R disorders in high school students. J. Abnorm. Psychol. 102, 133–144. doi: 10.1037/0021-843x.102.1.133
Liu, T.-C., Lin, Y.-C., Wang, T.-N., Yeh, S.-C., and Kalyuga, S. (2021). Studying the effect of redundancy in a virtual reality classroom. Educ. Technol. Res. Dev. 69, 1183–1200. doi: 10.1007/s11423-021-09991-6
Mariamo, A., Temcheff, C. E., Léger, P.-M., Senecal, S., and Lau, M. A. (2021). Emotional reactions and likelihood of response to questions designed for a mental health chatbot among adolescents: experimental study. JMIR Hum. Factors 8:e24343. doi: 10.2196/24343
Matheson, E. L., Smith, H. G., Amaral, A. C. S., Meireles, J. F. F., Almeida, M. C., Mora, G., et al. (2021). Improving body image at scale among Brazilian adolescents: study protocol for the co-creation and randomised trial evaluation of a chatbot intervention. BMC Public Health 21:2135. doi: 10.1186/s12889-021-12129-1
Mohr, D. C., Riper, H., and Schueller, S. M. (2018). A solution-focused research approach to achieve an implementable revolution in digital mental health. JAMA Psychiatry 75, 113–114. doi: 10.1001/jamapsychiatry.2017.3838
Naslund, J. A., Aschbrenner, K. A., Kim, S. J., McHugo, G. J., Unützer, J., Bartels, S. J., et al. (2017). Health behavior models for informing digital technology interventions for individuals with mental illness. Psychiatr. Rehabil. J. 40, 325–335. doi: 10.1037/prj0000246
Neumer, S.-P., Patras, J., Holen, S., Lisøy, C., Askeland, A. L., Haug, I. M., et al. (2021). Study protocol of a factorial trial ECHO: optimizing a group-based school intervention for children with emotional problems. BMC Psychol. 9:97. doi: 10.1186/s40359-021-00581-y
Ong, J. G., Lim-Ashworth, N. S., Ooi, Y. P., Boon, J. S., Ang, R. P., Goh, D. H., et al. (2019). An interactive mobile app game to address aggression (regnatales): pilot quantitative study. JMIR Ser Games 7:e13242. doi: 10.2196/13242
Orlowski, S., Lawn, S., Antezana, G., Venning, A., Winsall, M., Bidargaddi, N., et al. (2016). A rural youth consumer perspective of technology to enhance face-to-face mental health services. J. Child Fam. Stud. 25, 3066–3075. doi: 10.1007/s10826-016-0472-z
Orr, M., MacLeod, L., Bagnell, A., McGrath, P., Wozney, L., and Meier, S. (2023). The comfort of adolescent patients and their parents with mobile sensing and digital phenotyping. Comput. Hum. Behav. 140:107603. doi: 10.1016/j.chb.2022.107603
Owens, C., and Charles, N. (2016). Implementation of a text-messaging intervention for adolescents who self-harm (TeenTEXT): a feasibility study using normalisation process theory. Child Adolesc. Psychiatry Ment. Health 10:14. doi: 10.1186/s13034-016-0101-z
Patchin, J. W. (2021). 2021 Cyberbullying Data. Available at: https://cyberbullying.org/2021-cyberbullying-data
Piers, R., Williams, J. M., and Sharpe, H. (2023). Review: can digital mental health interventions bridge the ‘digital divide’ for socioeconomically and digitally marginalised youth? A systematic review. Child Adolesc. Ment. Health 28, 90–104. doi: 10.1111/camh.12620
Ranney, M. L., Patena, J. V., Dunsiger, S., Spirito, A., Cunningham, R. M., Boyer, E., et al. (2019). A technology-augmented intervention to prevent peer violence and depressive symptoms among at-risk emergency department adolescents: protocol for a randomized control trial. Contemp. Clin. Trials 82, 106–114. doi: 10.1016/j.cct.2019.05.009
Scott, L. N., Victor, S. E., Kaufman, E. A., Beeney, J. E., Byrd, A. L., Vine, V., et al. (2020). Affective dynamics across internalizing and externalizing dimensions of psychopathology. Clin. Psychol. Sci. 8, 412–427. doi: 10.1177/2167702619898802
Shah, S. M. A., Mohammad, D., Qureshi, M. F. H., Abbas, M. Z., and Aleem, S. (2021). Prevalence, psychological responses and associated correlates of depression, anxiety and stress in a global population, during the coronavirus disease (COVID-19) pandemic. Community Ment. Health J. 57, 101–110. doi: 10.1007/s10597-020-00728-y
Stasiak, K., Hatcher, S., Frampton, C., and Merry, S. N. (2014). A pilot double blind randomized placebo controlled trial of a prototype computer-based cognitive behavioural therapy program for adolescents with symptoms of depression. Behav. Cogn. Psychother. 42, 385–401. doi: 10.1017/s1352465812001087
Suldo, S. M., and Shaffer, E. J. (2008). Looking beyond psychopathology: the dual-factor model of mental health in youth. School Psychol. Rev. 37, 52–68. doi: 10.1080/02796015.2008.12087908
Tang, X., Tang, S., Ren, Z., and Wong, D. F. K. (2019). Prevalence of depressive symptoms among adolescents in secondary school in mainland China: a systematic review and meta-analysis. J. Affect. Disord. 245, 498–507. doi: 10.1016/j.jad.2018.11.043
Taylor, C. B., Ruzek, J. I., Fitzsimmons-Craft, E. E., Sadeh-Sharvit, S., Topooco, N., Weissman, R. S., et al. (2020). Using digital technology to reduce the prevalence of mental health disorders in populations: time for a new approach. J. Med. Internet Res. 22:e17493. doi: 10.1177/2167702619859336
Thabrew, H., Chubb, L. A., Kumar, H., and Fouché, C. (2022). Immersive reality experience technology for reducing social isolation and improving social connectedness and well-being of children and young people who are hospitalized: open trial. JMIR Pediatr. Parent. 5:e29164. doi: 10.2196/29164
Topooco, N., Byléhn, S., Dahlström Nysäter, E., Holmlund, J., Lindegaard, J., Johansson, S., et al. (2019). Evaluating the efficacy of internet-delivered cognitive behavioral therapy blended with synchronous chat sessions to treat adolescent depression: randomized controlled trial. J. Med. Internet Res. 21:e13393. doi: 10.2196/13393
Uhlhaas, P., and Torous, J. (2019). Digital tools for youth mental health. NPJ Digit. Med. 2:104. doi: 10.1038/s41746-019-0181-2
Villarreal, V. (2018). Mental health collaboration: a survey of practicing school psychologists. J. Appl. Sch. Psychol. 34, 1–17. doi: 10.1080/15377903.2017.1328626
Wacks, Y., and Weinstein, A. M. (2021). Excessive smartphone use is associated with health problems in adolescents and young adults. Front. Psych. 12:669042. doi: 10.3389/fpsyt.2021.669042
Wenzel, A. (2017). Basic strategies of cognitive behavioral therapy. Psychiatr. Clin. North Am. 40, 597–609. doi: 10.1016/j.psc.2017.07.001
Werner-Seidler, A., Huckvale, K., Larsen, M. E., Calear, A. L., Maston, K., Johnston, L., et al. (2020). A trial protocol for the effectiveness of digital interventions for preventing depression in adolescents: the future proofing study. Trials 21:2. doi: 10.1186/s13063-019-3901-7
World Health Organization (2021). Mental health of adolescents. Available at: https://www.who.int/news-room/fact-sheets/detail/adolescent-mental-health (Accessed December 14, 2023).
Wu, B., Zheng, C., and Huang, B. (2022). Influence of science education on mental health of adolescents based on virtual reality. Front. Psychol. 13:895196. doi: 10.3389/fpsyg.2022.895196
Zepeda, M., Deighton, S., Markova, V., Madsen, J., and Racine, N. (2021). iCOPE with COVID-19: A brief telemental health intervention for children and adolescents during the COVID-19 pandemic. PsyArXiv . doi: 10.31234/osf.io/jk32s
Keywords: children and adolescents, digital technology, systematic literature review, meta-analysis, mental health issues
Citation: Chen T, Ou J, Li G and Luo H (2024) Promoting mental health in children and adolescents through digital technology: a systematic review and meta-analysis. Front. Psychol . 15:1356554. doi: 10.3389/fpsyg.2024.1356554
Received: 15 December 2023; Accepted: 29 February 2024; Published: 12 March 2024.
Reviewed by:
Copyright © 2024 Chen, Ou, Li and Luo. This is an open-access article distributed under the terms of the Creative Commons Attribution License (CC BY) . The use, distribution or reproduction in other forums is permitted, provided the original author(s) and the copyright owner(s) are credited and that the original publication in this journal is cited, in accordance with accepted academic practice. No use, distribution or reproduction is permitted which does not comply with these terms.
*Correspondence: Heng Luo, [email protected]
Disclaimer: All claims expressed in this article are solely those of the authors and do not necessarily represent those of their affiliated organizations, or those of the publisher, the editors and the reviewers. Any product that may be evaluated in this article or claim that may be made by its manufacturer is not guaranteed or endorsed by the publisher.
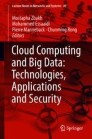
International Conference of Cloud Computing Technologies and Applications
CloudTech 2017: Cloud Computing and Big Data: Technologies, Applications and Security pp 241–263 Cite as
Workflow Scheduling Issues and Techniques in Cloud Computing: A Systematic Literature Review
- Samadi Yassir 6 ,
- Zbakh Mostapha 6 &
- Tadonki Claude 7
- Conference paper
- First Online: 28 July 2018
1154 Accesses
8 Citations
Part of the book series: Lecture Notes in Networks and Systems ((LNNS,volume 49))
One of the most challenging issues in cloud computing is workflow scheduling. Workflow applications have a complex structure and many discrete tasks. Each task may include entering data, processing, accessing software, or storage functions. For these reasons, the workflow scheduling is considered to be an NP-hard problem. Then, efficient scheduling algorithms are required for selection of best suitable resources for workflow execution. In this paper, we conduct a SLR (Systematic literature review) of workflow scheduling strategies that have been proposed for cloud computing platforms to help researchers systematically and objectively gather and aggregate research evidences about this topic. Then, we present a comparative analysis of the studied strategies. Finally, we highlight workflow scheduling issues for further research. The findings of this review provide a roadmap for developing workflow scheduling models, which will motivate researchers to propose better workflow scheduling algorithms for service consumers and/or utility providers in cloud computing.
- Workflow Scheduling
- Cloud Computing Environment
- Workflow Applications
- Heterogeneous Earliest Finish Time (HEFT)
- HEFT Algorithm
These keywords were added by machine and not by the authors. This process is experimental and the keywords may be updated as the learning algorithm improves.
This is a preview of subscription content, log in via an institution .
Buying options
- Available as PDF
- Read on any device
- Instant download
- Own it forever
- Available as EPUB and PDF
- Compact, lightweight edition
- Dispatched in 3 to 5 business days
- Free shipping worldwide - see info
Tax calculation will be finalised at checkout
Purchases are for personal use only
Vaquero, L.M., Rodero-Merino, L., Caceres, J., Lindner, M.: A break in the clouds: towards a cloud definition. ACM SIGCOMM Comput. Commun. Rev. 39 (1), 50–55 (2008)
Article Google Scholar
Rimal, B.P., Choi, E.: A service oriented taxonomical spectrum, cloudy challenges and opportunities of cloud computing. Int. J. Commun Syst 25 (6), 796–819 (2012)
Chen, H., Zhu, X., Qiu, D., Liu, L., Du, Z.: Scheduling for workflows with security-sensitive intermediate data by selective tasks duplication in clouds. IEEE Trans. Parallel Distrib. Syst. 28 (9), 2674–2688 (2017)
Wang, C., Ren, K., Wang, J.: Secure and practical outsourcing of linear programming in cloud computing. In: 2011 Proceedings IEEE INFOCOM, pp. 820–828, April 2011
Google Scholar
Wei, L., Zhu, H., Cao, Z., Dong, X., Jia, W., Chen, Y., Vasilakos, A.V.: Security and privacy for storage and computation in cloud computing. Inf. Sci. 258 , 371–386 (2014)
Wieczorek, M., Hoheisel, A., Prodan, R.: Towards a general model of the multi-criteria workflow scheduling on the grid. Future Gen. Comput. Syst. 25 (3), 237–256 (2009)
Zhao, Y., Chen, L., Li, Y., Tian, W.: Efficient task scheduling for Many Task Computing with resource attribute selection. China Commun. 11 (12), 125–140 (2014)
Pinedo, M.L.: Scheduling: Theory, Algorithms, and Systems. Springer, New York (2008)
MATH Google Scholar
Bittencourt, L.F., Sakellariou, R., Madeira, E.R.: Dag scheduling using a lookahead variant of the heterogeneous earliest finish time algorithm. In: 2010 18th Euromicro International Conference on Parallel, Distributed and Network-Based Processing (PDP), pp. 27–34, February 2010
Kwok, Y.-K., Ahmad, I.: Static scheduling algorithms for allocating directed task graphs to multiprocessors. ACM Comput. Surv. 31 (4), 406–471 (1999)
Keele, S.: Guidelines for performing systematic literature reviews in software engineering. In: Technical report, Ver. 2.3 EBSE Technical Report. EBSE. sn. (2007)
Kitchenham, B., Brereton, O.P., Budgen, D., Turner, M., Bailey, J., Linkman, S.: Systematic literature reviews in software engineering? A systematic literature review. Inf. Softw. Technol. 51 (1), 7–15 (2009)
Abdelkader, D.M., Omara, F.: Dynamic task scheduling algorithm with load balancing for heterogeneous computing system. Egypt. Inf. J. 13 (2), 135–145 (2012)
Deelman, E., Gannon, D., Shields, M., Taylor, I.: Workflows and e-science: an overview of workflow system features and capabilities. Future Gen. Comput. Syst. 25 , 528–540 (2009)
Du, Y., Li, X.: Application of workflow technology to current dispatching order system. Int. J. Comput. Sci. Netw. Secur. 8 (3), 59–61 (2008)
MathSciNet Google Scholar
Workflow Process Definition Interface: Workflow Management Coalition Workflow Standard Workflow Process Definition Interface–XML Process Definition Language (2002)
Berriman, G.B., Deelman, E., Good, J.C., Jacob, J.C., Katz, D.S., Kesselman, C., Laity, A.C., Prince, T.A., Singh, G., Su, M.: Montage: a grid-enabled engine for delivering custom science-grade mosaics on demand. In: SPIE Conference on Astronomical Telescopes and Instrumentation (2004)
Graves, R., et al.: CyberShake: a physics-based seismic hazard model for Southern California. Pure Appl. Geophys. 168 (3–4), 367–381 (2010)
Ye, C.X., Lu, J.: IGrid task scheduling based on improved genetic algorithm. Comput. Sci. 37 (7), 233–235 (2007)
Bittencourt, L.F., Madeira, E.R.M.: HCOC: a cost optimization algorithm for workflow scheduling in hybrid clouds. J. Internet Serv. Appl. 2 (3), 207–227 (2011)
Zeng, L., Veeravalli, B., Li, X.: SABA: a security-aware and budget-aware workflow scheduling strategy in clouds. J. Parallel Distrib. Comput. 75 , 141–151 (2015)
Zheng, W., Sakellariou, R.: Budget-deadline constrained workflow planning for admission control. J. Grid Comput. 11 (4), 633–651 (2013)
Zhao, L., Ren, Y., Sakurai, K.: Reliable workflow scheduling with less resource redundancy. Parallel Comput. 39 (10), 567–585 (2013)
Article MathSciNet Google Scholar
Wang, X., Yeo, C.S., Buyya, R., Su, J.: Optimizing the makespan and reliability for workflow applications with reputation and a look-ahead genetic algorithm. Future Gen. Comput. Syst. 27 (8), 1124–1134 (2011)
Zhang, C., Chang, E.C., Yap, R.H.: Tagged-MapReduce: a general framework for secure computing with mixed-sensitivity data on hybrid clouds. In: 2014 14th IEEE/ACM International Symposium on Cluster, Cloud and Grid Computing (CCGrid), pp. 31–40, May 2014
Zhou, H.Z., Huang, K.C., Wang, F.J.: Dynamic resource provisioning for interactive workflow applications on cloud computing platform. In: Russia-Taiwan Symposium on Methods and Tools of Parallel Processing, pp. 115–125. Springer, Heidelberg (2010)
Chapter Google Scholar
Ludtke, S., Baldwin, P., Chiu, W.: EMAN: semi automated software for high resolution single-particle reconstructions. J. Struct. Biol. 128 , 82–97 (1999)
Mandal, A., Kennedy, K., Koelbel, C., Marin, G., Crummey, J.M., Liu, B., Johnsson, L.: Scheduling strategies for mapping application workflows onto the gird. In: The IEEE Symposium on High Performance Distributed Computing (HPDC 2014), pp. 125–134 (2005)
Tan, W., Sun, Y., Li, L.X., Lu, G., Wang, T.: A trust service-oriented scheduling model for workflow applications in cloud computing. IEEE Syst. J. 8 (3), 868–878 (2014)
Malawski, M., Figiela, K., Bubak, M., Deelman, E., Nabrzyski, J.: Scheduling multilevel deadline-constrained scientific workflows on clouds based on cost optimization. Sci. Program. 2015 , 1–13 (2015)
Niu, S.H., Ong, S.K., Nee, A.Y.C.: An improved intelligent water drops algorithm for achieving optimal job-shop scheduling solutions. Int. J. Prod. Res. 50 (15), 4192–4205 (2012)
Samadi, Y., Zbakh, M., Tadonki, C.: E-HEFT: Enhancement Heterogeneous Earliest Finish Time algorithm for Task Scheduling based on Load Balancing in Cloud Computing (unpublished)
Liu, L., Zhang, M., Buyya, R., Fan, Q.: Deadline-constrained coevolutionary genetic algorithm for scientific workflow scheduling in cloud computing. Concurr. Comput.: Pract. Exp. 29 (5), e3942 (2017)
Chen, W., da Silva, R.F., Deelman, E., Sakellariou, R.: Using imbalance metrics to optimize task clustering in scientific workflow executions. Future Gen. Comput. Syst. 46 , 69–84 (2015)
Kumar, M.S., Gupta, I., Jana, P.K.: Forward load aware scheduling for data-intensive workflow applications in cloud system. In: International Conference on Information Technology (ICIT), pp. 93–98 (2016)
Casas, I., Taheri, J., Ranjan, R., Wang, L., Zomaya, A.Y.: A balanced scheduler with data reuse and replication for scientific workflows in cloud computing systems. Future Gen. Comput. Syst. 74 , 168–178 (2017)
Poola, D., Ramamohanarao, K., Buyya, R.: Enhancing reliability of workflow execution using task replication and spot instances. ACM Trans. Auton. Adapt. Syst. 10 (4), 30 (2016)
Rehani, N., Garg, R.: Reliability-aware workflow scheduling using monte carlo failure estimation in cloud. In: Proceedings of International Conference on Communication and Networks, pp. 139–153. Springer, Singapore (2017)
Xie, G., Zeng, G., Chen, Y., Bai, Y., Zhou, Z., Li, R., Li, K.: Minimizing redundancy to satisfy reliability requirement for a parallel application on heterogeneous service-oriented systems. IEEE Trans. Serv. Comput. PP (99), 1–11 (2017)
Samadi, Y., Zbakh, M., Tadonki, C.: DT-MG: Many-to-One Matching Game for Tasks Scheduling towards Resources Optimization in Cloud Computing (unpublished)
Duan, H., Chen, C., Min, G.: Y. Wu.: Energy-aware scheduling of virtual machines in heterogeneous cloud computing systems. Future Gen. Comput. Syst. (2016). https://doi.org/10.1016/j.future.2016.02.016
Yassa, S., Chelouah, R., Kadima, H., Granado, B.: Multi-objective approach for energy-aware workflow scheduling in cloud computing environments. Sci. World J. 2013 , 1–13 (2013)
Li, Z.J., Ge, J.D., Yang, H.J., Huang, L.G., Hu, H.Y., Hu, H., Luo, B.: A security and cost aware scheduling algorithm for heterogeneous tasks of scientific workflow in clouds. Future Gen. Comput. Syst. 65 , 140–152 (2016)
Arunarani, A.R., Manjula, D., Sugumaran, V.: FFBAT: A security and cost-aware workflow scheduling approach combining firefly and bat algorithms. Concurrency and Computation: Practice and Experience, 29(24) (2017)
Garcia Garcia, A., Blanquer Espert, I., Hernandez Garcia, V.: SLA-driven dynamic cloud resource management. Future Gener. Comput. Syst. (ISSN: 0167-739X), 31, 1–11 (2014)
Garg, S.K., Toosi, A.N., Gopalaiyengar, S.K., Buyya, R.: SLA-based virtual machine management for heterogeneous workloads in a cloud datacenter. Journal of Network and Computer Applications 45 , 108–120 (2014)
Wang, W.J., Chang, Y.S., Lo, W.T., Lee, Y.K.: Adaptive scheduling for parallel tasks with QoS satisfaction for hybrid cloud environments. The Journal of Supercomputing 66 (2), 783–811 (2013)
Y. Samadi, M. Zbakh, and C. Tadonki.: Graph-based Model and Algorithm for Minimizing Big Data Movement in a Cloud Environment, International Journal of High Performance Computing and Networking (2018)
Download references
Author information
Authors and affiliations.
National School of Computer Science and Systems Analysis, Mohamed V University, Rabat, Morocco
Samadi Yassir & Zbakh Mostapha
Mines ParisTech - PSL Research University Centre de Recherche en Informatique (CRI), Paris, France
Tadonki Claude
You can also search for this author in PubMed Google Scholar
Corresponding author
Correspondence to Samadi Yassir .
Editor information
Editors and affiliations.
ENSIAS College of Engineering, Mohammed V University, Agdal, Rabat, Morocco
Mostapha Zbakh
Mohammed Essaaidi
Department of Computer Science, Polytechnic of Mons, Mons, Belgium
Pierre Manneback
Department of Electrical Engineering and Computer Science, University of Stavanger, Stavanger, Norway
Chunming Rong
Rights and permissions
Reprints and permissions
Copyright information
© 2019 Springer Nature Switzerland AG
About this paper
Cite this paper.
Yassir, S., Mostapha, Z., Claude, T. (2019). Workflow Scheduling Issues and Techniques in Cloud Computing: A Systematic Literature Review. In: Zbakh, M., Essaaidi, M., Manneback, P., Rong, C. (eds) Cloud Computing and Big Data: Technologies, Applications and Security. CloudTech 2017. Lecture Notes in Networks and Systems, vol 49. Springer, Cham. https://doi.org/10.1007/978-3-319-97719-5_16
Download citation
DOI : https://doi.org/10.1007/978-3-319-97719-5_16
Published : 28 July 2018
Publisher Name : Springer, Cham
Print ISBN : 978-3-319-97718-8
Online ISBN : 978-3-319-97719-5
eBook Packages : Intelligent Technologies and Robotics Intelligent Technologies and Robotics (R0)
Share this paper
Anyone you share the following link with will be able to read this content:
Sorry, a shareable link is not currently available for this article.
Provided by the Springer Nature SharedIt content-sharing initiative
- Publish with us
Policies and ethics
- Find a journal
- Track your research
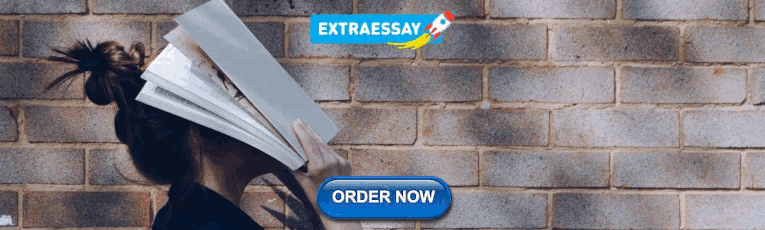
IMAGES
VIDEO
COMMENTS
Image by TraceyChandler. Steps to conducting a systematic review. Quick overview of the process: Steps and resources from the UMB HSHSL Guide. YouTube video (26 min); Another detailed guide on how to conduct and write a systematic review from RMIT University; A roadmap for searching literature in PubMed from the VU Amsterdam; Alexander, P. A. (2020).
A review is an overview of the research that's already been completed on a topic. What makes a systematic review different from other types of reviews is that the research methods are designed to reduce bias. The methods are repeatable, and the approach is formal and systematic: Formulate a research question. Develop a protocol.
Literature reviews establish the foundation of academic inquires. However, in the planning field, we lack rigorous systematic reviews. In this article, through a systematic search on the methodology of literature review, we categorize a typology of literature reviews, discuss steps in conducting a systematic literature review, and provide suggestions on how to enhance rigor in literature ...
The best reviews synthesize studies to draw broad theoretical conclusions about what a literature means, linking theory to evidence and evidence to theory. This guide describes how to plan, conduct, organize, and present a systematic review of quantitative (meta-analysis) or qualitative (narrative review, meta-synthesis) information.
This systematic review provides a comprehensive overview of the LBD workflow by answering nine research questions related to the major components of the LBD workflow (i.e., input, process, output, and evaluation). With regards to the input component, we discuss the data types and data sources used in the literature.
When choosing systematic literature review software, it's important to think about your unique challenges and review workflow. However, be warned! In this case, relying on a feature matrix can be misleading , so it's best to do extensive research about what review software best meets your needs before buying.
Method details Overview. A Systematic Literature Review (SLR) is a research methodology to collect, identify, and critically analyze the available research studies (e.g., articles, conference proceedings, books, dissertations) through a systematic procedure [12].An SLR updates the reader with current literature about a subject [6].The goal is to review critical points of current knowledge on a ...
The reason why systematic reviews are so highly respected and sought after is because they are rigorous, reproducible, transparent, and have standards. But these characteristics are only true if those standards/guidelines are followed. Not all systematic reviews are created equally. Make sure yours is top notch so you do not perpetuate the problem.
A systematic review (SR) is a type of literature review. Unlike other forms of review, where authors can include any articles they consider appropriate, a systematic review aims to remove the reviewer's bias as far as possible by following a clearly defined, transparent process. This Cochrane video gives a clear summary, but you should find ...
The Systematic Review Toolbox is a web-based catalogue of tools that support various tasks within the systematic review and wider evidence synthesis process. The toolbox aims to help researchers and reviewers find the following: Software tools, Quality assessment / critical appraisal checklists, Reporting standards, and Guidelines.
A systematic review is a comprehensive review of the literature conducted by a research team using systematic and transparent methods in accordance with reporting guidelines to answer a well-defined research question. It aims to identify and synthesize scholarly research published in commercial and/or academic sources as well as in grey (or ...
Searching literature is one of the most important elements of a systematic review. A well planned search strategy in the right databases ensures you have a robust list of results to whittle down as part of your PRISMA workflow. We've answered some of the common questions we get asked about searching literature as part of a systematic review.
The PRISMA extension for scoping reviews contains 20 essential reporting items and 2 optional items to include when completing a scoping review. Scoping reviews serve to synthesize evidence and assess the scope of literature on a topic. Among other objectives, scoping reviews help determine whether a systematic review of the literature is ...
A Systematic Literature Review of Workflows, Methodologies, Reproducibility, and Tools Adam Tutko [email protected] University of Tennessee Knoxville, TN Audris Mockus [email protected] ... Systematic literature reviews [12] are best suited to address our research questions: i.e., to summarize the state of the art and ...
Reduce Your Literature Review Time By Half . AI-Powered Screening. Systematic Reviews by nature require a complete and exhaustive study of the current body of literature available on a topic which may sometimes be tens of thousands. Screening such a massive body of references takes up a significant portion of time in a systematic review.
In this blog post, we discuss seven ways meant to inspire users. Use ASReview with a single screener. Use ASReview with multiple screeners. Switch models for hard-to-find papers. Add more data because a reviewer asks you to. Screen data from a narrow search and apply active learning to a comprehensive search.
A preliminary assessment of workflow research literature revealed a wide range of workflow-related research questions and varying approaches to workflow study. We determined that a systematic literature review was an appropriate and necessary technique to understand the depth and breadth of workflow research.
Securely automate every stage of your literature review to produce evidence-based research faster, more accurately, and more transparently at scale. Rayyan A web-tool designed to help researchers working on systematic reviews, scoping reviews and other knowledge synthesis projects, by dramatically speeding up the process of screening and ...
The main objective of the Python framework is to automate systematic reviews to save reviewers time without creating constraints that might affect the review quality. The other objective is to create an open-source and highly customisable framework with options to use or improve any parts of the framework. python framework supports each step in ...
A systematic literature review was conducted to make an inventory of metrics for optimization of the OR in literature. We used the search engines Scopus, Web of Science and PubMed with the search terms: "Operation Room" AND Optimization and Workflow AND Optimization AND Hospital.
More specifically, our contributions are; (1) being the first systematic literature review that covers every component of the LBD workflow, (2) shedding light on components in
This study used the systematic literature review method to analyze the relevant literature on the promotion of mental health through digital technology. It followed the Preferred Reporting Items for Systematic Reviews and Meta-Analysis statement for the selection and use of research methods. The protocol for this study was registered with ...
In this paper, we conduct a SLR (Systematic literature review) of workflow scheduling strategies that have been proposed for cloud computing platforms to help researchers systematically and objectively gather and aggregate research evidences about this topic. Then, we present a comparative analysis of the studied strategies.
However, integrating cultural data is not a trivial task; therefore, this work performs a systematic literature review on data aggregation workflows, in order to answer five questions: What are the projects? What are the planned steps? Which technologies are used? Are the steps performed manually, automatically, or semi-automatically?