
An official website of the United States government
The .gov means it’s official. Federal government websites often end in .gov or .mil. Before sharing sensitive information, make sure you’re on a federal government site.
The site is secure. The https:// ensures that you are connecting to the official website and that any information you provide is encrypted and transmitted securely.
- Publications
- Account settings
Preview improvements coming to the PMC website in October 2024. Learn More or Try it out now .
- Advanced Search
- Journal List
- Springer Nature - PMC COVID-19 Collection

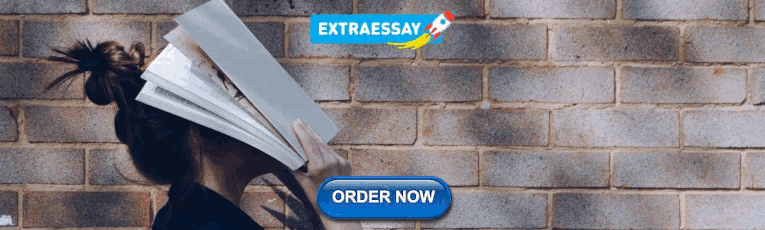
Causal Reasoning Meets Visual Representation Learning: A Prospective Study
School of Computer Science and Engineering, Sun Yat-sen University, Guangzhou, 510006 China
Yu-Shen Wei
Guan-bin li.
Visual representation learning is ubiquitous in various real-world applications, including visual comprehension, video understanding, multi-modal analysis, human-computer interaction, and urban computing. Due to the emergence of huge amounts of multimodal heterogeneous spatial/temporal/spatial-temporal data in the big data era, the lack of interpretability, robustness, and out-of-distribution generalization are becoming the challenges of the existing visual models. The majority of the existing methods tend to fit the original data/variable distributions and ignore the essential causal relations behind the multi-modal knowledge, which lacks unified guidance and analysis about why modern visual representation learning methods easily collapse into data bias and have limited generalization and cognitive abilities. Inspired by the strong inference ability of human-level agents, recent years have therefore witnessed great effort in developing causal reasoning paradigms to realize robust representation and model learning with good cognitive ability. In this paper, we conduct a comprehensive review of existing causal reasoning methods for visual representation learning, covering fundamental theories, models, and datasets. The limitations of current methods and datasets are also discussed. Moreover, we propose some prospective challenges, opportunities, and future research directions for benchmarking causal reasoning algorithms in visual representation learning. This paper aims to provide a comprehensive overview of this emerging field, attract attention, encourage discussions, bring to the forefront the urgency of developing novel causal reasoning methods, publicly available benchmarks, and consensus-building standards for reliable visual representation learning and related real-world applications more efficiently.
Acknowledgements
This work was supported in part by National Natural Science Foundation of China (Nos. 62002395, 61976250 and U1811463), the National Key R&D Program of China (No. 2021ZD0111601), the Guangdong Basic and Applied Basic Research Foundation, China (Nos. 2021A15150123 and 2020B1515020048).
Yang Liu received the B.Sc. degree in telecommunications engineering from Chang’an University, China in 2014, and the Ph.D. degree in telecommunications and information systems from Xidian University, China in 2019. He is currently a research associate professor working at School of Computer Science and Engineering, Sun Yat-sen University, China. He has authorized and co-authorized more than 20 papers in top-tier academic journals and conferences. He has been serving as a reviewer for numerous academic journals and conferences such as IEEE TIP, TNNLS, TMM, TCSVT, TCyb, CVPR, ICCV, AAAI, and ECCV. He is a member of IEEE and CSIG.
His research interests include video understanding, causal reasoning, and computer vision.
Yu-Shen Wei received the B.Sc. degree in computer science and technology from Sun Yat-sen University, China in 2020. He is currently a master student at School of Computer Science and Engineering, Sun Yat-sen University, China.
His current research interests include video understanding, computer vision and machine learning.
Hong Yan received the B.Sc. degree in computer science and technology from Nanchang University, China in 2020. He is currently a master student at School of Computer Science and Engineering, Sun Yat-sen University, China.
His research interests include video understanding, computer vision and machine learning.
Guan-Bin Li received the Ph.D. degree from the University of Hong Kong, China in 2016. He is currently an associate professor in School of Computer Science and Engineering, Sun Yat-Sen University, China. He is a recipient of ICCV 2019 Best Paper Nomination Award. He has authorized and co-authorized on more than 70 papers in top-tier academic journals and conferences. He serves as an area chair for the conference of VIS-APP. He has been serving as a reviewer for numerous academic journals and conferences such as TPAMI, IJCV, TIP, TMM, TCyb, CVPR, ICCV, ECCV and NeurIPS.
His research interests include computer vision, image processing, and machine learning.
Liang Lin received the Ph. D. degree from Beijing Institute of Technology, China in 2008. He is a full professor of computer science at Sun Yat-sen University, China. He served as the executive director and distinguished scientist of SenseTime Group from 2016 to 2018, leading the R&D teams for cutting-edge technology transferring. He has authored or co-authored more than 200 papers in leading academic journals and conferences, and his papers have been cited by more than 21 000 times. He is an associate editor of IEEE Transactions on Neural Networks and Learning Systems and IEEE Transactions on Human-Machine Systems , and served as area Chairs for numerous conferences such as CVPR, ICCV, SIGKDD and AAAI. He is the recipient of numerous awards and honors including Wu Wen-Jun Artificial Intelligence Award, the First Prize of China Society of Image and Graphics, ICCV Best Paper Nomination in 2019, Annual Best Paper Award by Pattern Recognition (Elsevier) in 2018, Best Paper Dimond Award in IEEE ICME 2017, Google Faculty Award in 2012. His supervised Ph.D. students received ACM China Doctoral Dissertation Award, CCF Best Doctoral Dissertation and CAAI Best Doctoral Dissertation. He is a fellow of IET/IAPR.
His research interests include artificial intelligence, computer vision, machine learning, multimedia, and NLP/Dialogue.
Contributor Information
Yang Liu, Email: nc.ude.usys.liam@658yuil .
Yu-Shen Wei, Email: nc.ude.usys.2liam@8hsyiew .
Hong Yan, Email: nc.ude.usys.2liam@63hnay .
Guan-Bin Li, Email: nc.ude.usys.liam@nibnaugil .
Liang Lin, Email: gro.eeei@gnailnil .

Causal reasoning meets visual representation learning: A prospective study
Beijing Zhongke Journal Publising Co. Ltd.
Overview of the structure of this paper, including the discussion of related methods, datasets, challenges, and the relations among causal reasoning, visual representation learning, and their integration
Credit: Beijing Zhongke Journal Publising Co. Ltd.
With the emergence of huge amounts of heterogeneous multi-modal data, including images, videos, texts/languages, audios, and multi-sensor data, deep learning-based methods have shown promising performance for various computer vision and machine learning tasks, e.g., the visual comprehension, video understanding, visual-linguistic analysis, and multi-modal fusion, etc. However, the existing methods rely heavily upon fitting the data distributions and tend to capture the spurious correlations from different modalities, and thus fail to learn the essential causal relations behind the multi-modal knowledge that have a good generalization and cognitive abilities. Inspired by the fact that most of the data in computer vision society are independent and identically distributed (i.i.d.), a substantial body of literature adopted data augmentation, pre-training, self-supervision, and novel architectures to improve the robustness of the state-of-the-art deep neural network architectures. However, it has been argued that such strategies only learn correlation-based patterns (statistical dependencies) from data and may not generalize well without the guarantee of the i.i.d setting.
Due to the powerful ability of to uncover the underlying structural knowledge about data generating processes that allow interventions and generalize well across different tasks and environments, causal reasoning offers a promising alternative to correlation learning. Recently, causal reasoning has attracted increasing attention in a myriad of high-impact domains of computer vision and machine learning, such as interpretable deep learning, causal feature selection, visual comprehension, visual robustness, visual question answering, and video understanding. A common challenge of these causal methods is how to build a strong cognitive model that can fully discover causality and spatial-temporal relations.
In this paper, researchers aim to provide a comprehensive overview of causal reasoning for visual representation learning, attract attention, encourage discussions, and bring to the forefront the urgency of developing novel causality-guided visual representation learning methods. Although there are some surveys about causal reasoning, these works are intended for general representation learning tasks such as deconfounding, out-of-distribution (OOD) generalization, and debiasing. Differently, this paper focuses on the systematic and comprehensive survey of related works, datasets, insights, future challenges and opportunities for causal reasoning, visual representation learning, and their integration. To present the review more concisely and clearly, this paper selects and cites related work by considering their sources, publication years, impact, and the cover of different aspects of the topic surveyed in this paper. Overall, the main contributions of this paper are given as follows.
Firstly, this paper presents the basic concepts of causality, the structural causal model (SCM), the independent causal mechanism (ICM) principle, causal inference, and causal intervention. Then, based on the analysis, this paper further gives some directions for conducting causal reasoning on visual representation learning tasks. Note that this paper is supposedto be the first that proposes the potential research directions for causal visual representation learning.
Secondly, a prospective review is introduced to systematically and structurally review the existing works according to their efforts in the above-pointed directions for conducting causal visual representation learning more efficiently. Researchers focus on the relation between visual representation learning and causal reasoning and provide a better understanding of why and how existing causal reasoning methods can be helpful in visual representation learning, as well as providing inspiration for future research and studies.
Thirdly, this paper explores and discusses future research areas and open problems related to using causal reasoning methods to tackle visual representation learning. This can encourage and support the broadening and deepening of research in the related fields.
Section 2 provides the preliminaries, which include five parts. The first part is the basic concepts of causality. Causal learning is different from statistical learning, which aims to discover causal relationships beyond statistical relations. Learning causality requires machine learning methods not only to predict the outcome of i.i.d. experiments but also to reason from a causal perspective. The second part is the SCM which considers the formulation of a causality style. The third part is the ICM principle that describes the independence of causal mechanisms. The fourth part is causal inference whose purpose is to estimate the outcome shift (or effect) of different treatments. The last part is causal intervention which aims to capture the causal effects of interventions (i.e., variables), and take advantage of causal relations in datasets to improve model performance and generalization ability.
Traditional feature learning methods usually learn the spurious correlation introduced by confounders. This will reduce the robustness of models and make models hard to generalize across domains. Causal reasoning, a learning paradigm that reveals the real causality from the outcome, overcomes the essential defect of correlation learning and learns robust, reusable, and reliable features. In Section 3, researchers review the recent representative causal reasoning methods for general feature learning, which mainly consist of three main paradigms: 1) structural causal model (SCM) embedded, 2) applying causal intervention/counterfactual, and 3) Markov boundary (MB) based feature selection.
Visual representation learning has made great progress in recent years, which can utilize spatial or/and temporal information to complete specific tasks, including visual understanding (object detection, scene graph generation, visual grounding, visual commonsense reasoning), action detection and recognition, and visual question answering, etc. In Section 4, researchers introduce these representative visual learning tasks and discuss the existing challenges and necessity of applying causal reasoning to visual representation learning.
According to the above-discussed visual representation learning methods, the current machine learning, especially representation learning, faces several challenges: 1) lack of interpretability, 2) poor generalization ability, and 3) over-reliance on correlations of data distribution. Causal reasoning offers a promising alternative to address these challenges. The discovery of causality helps to uncover the causal mechanism behind the data, allowing the machine to understand better why and to make decisions through intervention or counterfactual reasoning. In Section 5, researchers summarize some recent approaches for causal visual representation learning. The causal visual representation learning is an emerging research topic and has appeared since the 2020s. The related tasks can be roughly categorized into several main aspects: 1) causal visual understanding, 2) causal visual robustness, and 3) causal visual question answering. In this section, researchers discuss these three representative causal visual representations learning tasks.
Correlation-based models may perform well in existing datasets, not because these models have a strong reasoning capability, but because these datasets cannot fully support the evaluation of the models′ reasoning capability. Spurious correlations in these datasets can be exploited by the model to cheat, which means that the model just concentrates on superficial correlation learning, not real causal reasoning, only approximating the distribution of the dataset. For example, in the VQA v1.0 dataset for the VQA task, the model simply answers “yes” when seeing the question “Do you see a ···”, which will achieve nearly 90% accuracy. Due to this shortcoming in current datasets, researchers need to build benchmarks that can evaluate the true causal reasoning capability of models. In Section 6, researchers take image question answering benchmarks and video question answering benchmarks as examples to analyze the current research situation of related causal reasoning datasets and give some future directions.
Section 7 proposes and discusses some future research directions. Causal reasoning with visual representation learning has a variety of applications. Modeling causal reasoning for a variety of tasks can achieve a better perception of the real world. In this section, researchers introduce the applications from five aspects: image/video analysis, explainable artificial intelligence, recommendation system, human-computer dialog and interaction, and crowd intelligence analysis.
They also discuss how causal reasoning benefits various real-world applications.
Some researchers have successfully implemented causal reasoning for visual representation learning to discover causality and visual relations. However, causal reasoning for visual representation learning is still in its infancy stage, and many issues remain unsolved. Therefore, Section 8 highlights several possible research directions and open problems to inspire further extensive and in-depth research on this topic. Potential research directions for causal visual representation learning can be summarized as: 1) more reasonable causal relation modeling; 2) more precise approximation of intervention distributions; 3) more proper counterfactual synthesizing process; 4) large-scale benchmarks and evaluation pipeline.
This paper has provided a comprehensive survey on causal reasoning for visual representation learning. Researchers hope that this survey can help attract attention, encourage discussions, and bring to the forefront the urgency of developing novel causal reasoning methods, publicly available benchmarks, and consensus-building standards for reliable visual representation learning and related real-world applications more efficiently.
See the article:
Causal Reasoning Meets Visual Representation Learning: A Prospective Study
http://doi.org/10.1007/s11633-022-1362-z
Disclaimer: AAAS and EurekAlert! are not responsible for the accuracy of news releases posted to EurekAlert! by contributing institutions or for the use of any information through the EurekAlert system.

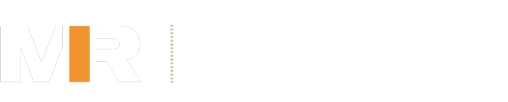
- Published Online
- Current Issue
- Special Issue
- Selected Papers
- Aims and Scopes
- Index information
- Editorial Board
- Springer Page
- Guide for Authors
- Submission Template
- Copyright Agreement
- Guide for Referees
- Referees Acknowledgement
Causal Reasoning Meets Visual Representation Learning: A Prospective Study
- Yang Liu ,
- Yu-Shen Wei ,
- Hong Yan ,
- Guan-Bin Li ,
Export File
You can copy and paste references from this page.
Causal Reasoning Meets Visual Representation Learning: A Prospective Study
We're not able to analyze this paper right now due to high demand.
Please check back later (sorry!).
Sign up or log in to have Emergent Mind summarize this paper with GPT-4 for free.
We ran into a problem analyzing this paper.
Please try again later (sorry!).
Get summaries of trending AI papers delivered straight to your inbox
Unsubscribe anytime.
- Causality-based Cross-Modal Representation Learning for Vision-and-Language Navigation
- Towards Causal Representation Learning
- CausalVLR: A Toolbox and Benchmark for Visual-Linguistic Causal Reasoning
- Cross-Modal Causal Relational Reasoning for Event-Level Visual Question Answering
- Causal models in string diagrams
> cs > arXiv:2204.12037
- Other formats
Current browse context:
Change to browse by:, references & citations, dblp - cs bibliography, computer science > computer vision and pattern recognition, title: causal reasoning meets visual representation learning: a prospective study.
Abstract: Visual representation learning is ubiquitous in various real-world applications, including visual comprehension, video understanding, multi-modal analysis, human-computer interaction, and urban computing. Due to the emergence of huge amounts of multi-modal heterogeneous spatial/temporal/spatial-temporal data in big data era, the lack of interpretability, robustness, and out-of-distribution generalization are becoming the challenges of the existing visual models. The majority of the existing methods tend to fit the original data/variable distributions and ignore the essential causal relations behind the multi-modal knowledge, which lacks unified guidance and analysis about why modern visual representation learning methods easily collapse into data bias and have limited generalization and cognitive abilities. Inspired by the strong inference ability of human-level agents, recent years have therefore witnessed great effort in developing causal reasoning paradigms to realize robust representation and model learning with good cognitive ability. In this paper, we conduct a comprehensive review of existing causal reasoning methods for visual representation learning, covering fundamental theories, models, and datasets. The limitations of current methods and datasets are also discussed. Moreover, we propose some prospective challenges, opportunities, and future research directions for benchmarking causal reasoning algorithms in visual representation learning. This paper aims to provide a comprehensive overview of this emerging field, attract attention, encourage discussions, bring to the forefront the urgency of developing novel causal reasoning methods, publicly available benchmarks, and consensus-building standards for reliable visual representation learning and related real-world applications more efficiently.
Submission history
Link back to: arXiv , form interface , contact .

Causal Reasoning Meets Visual Representation Learning: A Prospective Study [article]
Preserved fulltext.

Other Versions
Search code, repositories, users, issues, pull requests...
Provide feedback.
We read every piece of feedback, and take your input very seriously.
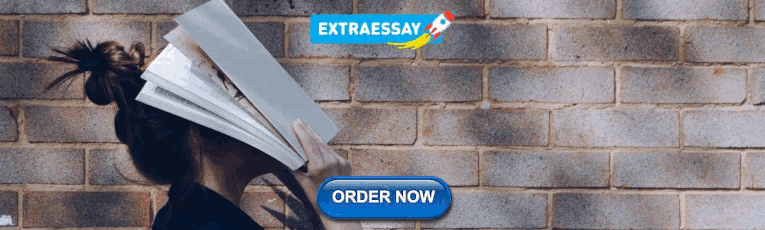
Saved searches
Use saved searches to filter your results more quickly.
To see all available qualifiers, see our documentation .
- Notifications
[MIR 2022] Causal Reasoning Meets Visual Representation Learning: A Prospective Study
YangLiu9208/MIR
Folders and files, repository files navigation, causal reasoning meets visual representation learning: a prospective study.
Machine Intelligence Research (MIR) 2022 A Review paper for causal reasoning and visual representation learning
Homepage: https://yangliu9208.github.io/home/
Visual representation learning is ubiquitous in various real-world applications, including visual comprehension, video understanding, multi-modal analysis, human-computer interaction, and urban computing. Due to the emergence of huge amounts of multimodal heterogeneous spatial/temporal/spatial-temporal data in the big data era, the lack of interpretability, robustness, and out-of-distribution generalization are becoming the challenges of the existing visual models. The majority of the existing methods tend to fit the original data/variable distributions and ignore the essential causal relations behind the multi-modal knowledge, which lacks unified guidance and analysis about why modern visual representation learning methods easily collapse into data bias and have limited generalization and cognitive abilities. Inspired by the strong inference ability of human-level agents, recent years have therefore witnessed great effort in developing causal reasoning paradigms to realize robust representation and model learning with good cognitive ability. In this paper, we conduct a comprehensive review of existing causal reasoning methods for visual representation learning, covering fundamental theories, models, and datasets. The limitations of current methods and datasets are also discussed. Moreover, we propose some prospective challenges, opportunities, and future research directions for benchmarking causal reasoning algorithms in visual representation learning. This paper aims to provide a comprehensive overview of this emerging field, attract attention, encourage discussions, bring to the forefront the urgency of developing novel causal reasoning methods, publicly available benchmarks, and consensus-building standards for reliable visual representation learning and related real-world applications more efficiently.

Media Report
WeChat Official Account Tencent News BAAI Newsletter Sina Weibo MIR Official Website Twitter Facebook ScienceNet
If you have any question about this paper, feel free to reach me ( [email protected] )
Subscribe to the PwC Newsletter
Join the community, edit social preview.

Add a new code entry for this paper
Remove a code repository from this paper, mark the official implementation from paper authors, add a new evaluation result row.
- BENCHMARKING
- OUT-OF-DISTRIBUTION GENERALIZATION
- REPRESENTATION LEARNING
- VIDEO UNDERSTANDING
Remove a task

Add a method
Remove a method, edit datasets, causal reasoning meets visual representation learning: a prospective study.
26 Apr 2022 · Yang Liu , Yushen Wei , Hong Yan , Guanbin Li , Liang Lin · Edit social preview
Visual representation learning is ubiquitous in various real-world applications, including visual comprehension, video understanding, multi-modal analysis, human-computer interaction, and urban computing. Due to the emergence of huge amounts of multi-modal heterogeneous spatial/temporal/spatial-temporal data in big data era, the lack of interpretability, robustness, and out-of-distribution generalization are becoming the challenges of the existing visual models. The majority of the existing methods tend to fit the original data/variable distributions and ignore the essential causal relations behind the multi-modal knowledge, which lacks unified guidance and analysis about why modern visual representation learning methods easily collapse into data bias and have limited generalization and cognitive abilities. Inspired by the strong inference ability of human-level agents, recent years have therefore witnessed great effort in developing causal reasoning paradigms to realize robust representation and model learning with good cognitive ability. In this paper, we conduct a comprehensive review of existing causal reasoning methods for visual representation learning, covering fundamental theories, models, and datasets. The limitations of current methods and datasets are also discussed. Moreover, we propose some prospective challenges, opportunities, and future research directions for benchmarking causal reasoning algorithms in visual representation learning. This paper aims to provide a comprehensive overview of this emerging field, attract attention, encourage discussions, bring to the forefront the urgency of developing novel causal reasoning methods, publicly available benchmarks, and consensus-building standards for reliable visual representation learning and related real-world applications more efficiently.
Code Edit Add Remove Mark official
Tasks edit add remove, datasets edit.

Results from the Paper Edit
Methods edit add remove.
Subscribe to Updates
Get the latest creative news from FooBar about art, design and business.
By signing up, you agree to the our terms and our Privacy Policy agreement.

Causal Reasoning Meets Visual Representation Learning: A Prospective Study
This paper provides an overview of the use of causal reasoning in computer vision and machine learning tasks, such as visual comprehension, video understanding, visual-linguistic analysis, and multi-modal fusion. It discusses the challenges of building a strong cognitive model that can fully discover causality and spatial-temporal relations, and provides an overview of the recent advances in causal reasoning for visual tasks.
Related Posts
How good is google bard’s visual understanding an empirical study on open challenges, meet advanced reasoning benchmark (arb): a new benchmark to evaluate large language models, why ai in retail requires cause-and-effect reasoning, causal ai makes for more accurate decision-making, causal ai quantifies cv risk, providing patient-specific goals, reveleer launches prospective risk adjustment enhancements, leveraging ai to close care gaps proactively.
Type above and press Enter to search. Press Esc to cancel.
Please Select below Reasons. —Please choose an option— Not Related Wrong Section Copyright Issue
Sign In or Register
Welcome back.
Login below or Register Now .
Register Now!
Already registered? Login .
A password will be e-mailed to you.
Report Post
« »
Your Email:
Please tell us why do you think this post is inappropriate and shouldn't be there:
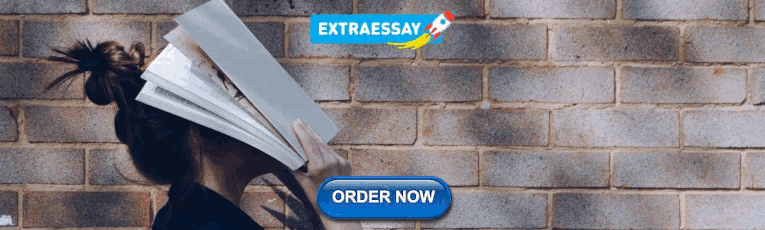
IMAGES
VIDEO
COMMENTS
Causal Reasoning Meets Visual Representation Learning: A Prospective Study. Visual representation learning is ubiquitous in various real-world applications, including visual comprehension, video understanding, multi-modal analysis, human-computer interaction, and urban computing. Due to the emergence of huge amounts of multi-modal heterogeneous ...
This paper aims to provide a comprehensive overview of this emerging field, attract attention, encourage discussions, bring to the forefront the urgency of developing novel causal reasoning methods, publicly available benchmarks, and consensus-building standards for reliable visual representation learning and related real-world applications ...
The majority of the existing methods tend to fit the original data/variable distributions and ignore the essential causal relations behind the multi-modal knowledge, which lacks unified guidance and analysis about why modern visual representation learning methods easily collapse into data bias and have limited generalization and cognitive ...
paper further gives some directions for conducting causal reasoning on visual representation learning tasks. Note that to the best of our knowledge, this paper is the first that proposes the potential research directions for causal visual representation learning. Secondly, a prospective review is introduced to sys-
This paper conducts a comprehensive review of existing causal reasoning methods for visual representation learning, covering fundamental theories, models, and datasets, and proposes some prospective challenges, opportunities, and future research directions for benchmarking causal reasoning algorithms inVisual representation learning. Visual representation learning is ubiquitous in various real ...
In this paper, we conduct a comprehensive review of existing causal reasoning methods for visual representation learning, covering fundamental theories, models, and datasets. The limitations of current methods and datasets are also discussed. Moreover, we propose some prospective challenges, opportunities, and future research directions for ...
In this paper, we conduct a comprehensive review of existing causal reasoning methods for visual representation learning, covering fundamental theories, models, and datasets. The limitations of ...
Yang Liu, Yu-Shen Wei, Hong Yan, Guan-Bin Li, Liang Lin. Causal Reasoning Meets Visual Representation Learning: A Prospective Study[J]. Machine Intelligence Research, 2022, 19(6): 485-511. DOI: 10.1007/s11633-022-1362-z. Yang Liu, Yu-Shen Wei, Hong Yan, Guan-Bin Li, Liang Lin. Causal Reasoning Meets Visual Representation Learning: A Prospective ...
For a detailed review of causal inference and visual representation learning, please refer to our review paper [16]. ... the benchmark results. Finally, we showcase representative studies on selected baselines. ... Guan-Bin Li, and Liang Lin. Causal reasoning meets visual representation learning: A prospective study. Machine Intelligence ...
Causal Reasoning Meets Visual Representation Learning: A Prospective Study. Visual representation learning is ubiquitous in various real-world applications, including visual comprehension, video understanding, multi-modal analysis, human-computer interaction, and urban computing. Due to the emergence of huge amounts of multi-modal heterogeneous ...
Moreover, researchers propose some prospective challenges, opportunities, and future research directions for benchmarking causal reasoning algorithms in visual representation learning.
Causal Reasoning Meets Visual Representation Learning: A Prospective Study. doi: 10.1007/s11633-022-1362-z. Yang Liu , Yu-Shen Wei , Hong Yan , Guan-Bin Li , Liang Lin. School of Computer Science and Engineering, Sun Yat-sen University, Guangzhou 510006, China. More Information.
Causal Reasoning Meets Visual Representation Learning: A Prospective Study (2204.12037) Published Apr 26, 2022 Abstract. Visual representation learning is ubiquitous in various real-world applications, including visual comprehension, video understanding, multi-modal analysis, human-computer interaction, and urban computing. ... we conduct a ...
Causal reasoning meets visual representation learning: A prospective study. by Beijing Zhongke Journal Publising Co. Overview of the structure of this paper, including the discussion of related methods, datasets, challenges, and the relations among causal reasoning, visual representation learning, and their integration.
In this paper, we conduct a comprehensive review of existing causal reasoning methods for visual representation learning, covering fundamental theories, models, and datasets. The limitations of current methods and datasets are also discussed. Moreover, we propose some prospective challenges, opportunities, and future research directions for ...
This paper aims to provide a comprehensive overview of this emerging field, attract attention, encourage discussions, bring to the forefront the urgency of developing novel causal reasoning methods, publicly available benchmarks, and consensus-building standards for reliable visual representation learning and related real-world applications ...
This paper aims to provide a comprehensive overview of this emerging field, attract attention, encourage discussions, bring to the forefront the urgency of developing novel causal reasoning methods, publicly available benchmarks, and consensus-building standards for reliable visual representation learning and related real-world applications ...
Causal Reasoning Meets Visual Representation Learning: A Prospective Study. Visual representation learning is ubiquitous in various real-world applications, including visual comprehension, video understanding, multi-modal analysis, human-computer interaction, and urban computing. Due to the emergence of huge amounts of multi-modal heterogeneous ...
Causal reasoning meets visual representation learning: A prospective study Y Liu, YS Wei, H Yan, GB Li, L Lin Machine Intelligence Research 19 (6), 485-511 , 2022
This paper provides an overview of the use of causal reasoning in computer vision and machine learning tasks, such as visual comprehension, video understanding, visual-linguistic analysis, and multi-modal fusion. It discusses the challenges of building a strong cognitive model that can fully discover causality and spatial-temporal relations, and provides an overview of the recent advances
Causal reasoning meets visual representation learning: A prospective study November 22 2023 Overview of the structure of this paper, including the discussion of related methods, datasets, challenges, and the relations among causal reasoning, visual representation learning, and their integration. Credit: Beijing Zhongke Journal Publising Co.
[16] Yang Liu, Yu-Shen Wei, Hong Yan, Guan-Bin Li, and Liang Lin. Causal reasoning meets visual representation learning: A prospective study. Machine Intelligence Research, pages 1-27, 2022. [17] Yang Liu, Yushen Wei, Hong Yan, Guanbin Li, and Liang Lin. Causal reasoning with spatial-temporal representation learning: A prospective study.