- Review article
- Open access
- Published: 25 September 2019
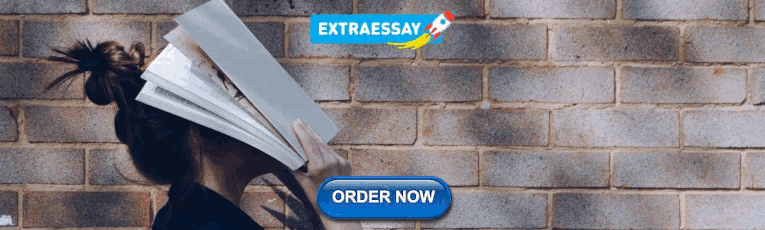
Use of Twitter across educational settings: a review of the literature
- Aqdas Malik ORCID: orcid.org/0000-0002-3517-8760 1 , 2 ,
- Cassandra Heyman-Schrum 3 &
- Aditya Johri 2
International Journal of Educational Technology in Higher Education volume 16 , Article number: 36 ( 2019 ) Cite this article
51k Accesses
53 Citations
118 Altmetric
Metrics details
The use of social media across the educational landscape is on the rise. Subsequently, the body of research on this topic is vibrant and growing. In this article, we present findings from a review of 103 peer-reviewed scientific studies published over the last decade (2007–2017) that address the use of Twitter for educational purposes across formal and informal settings. The majority of the studies reported in the literature are descriptive case studies carried out with students in North American and European higher education settings. Analysis of these studies signals Twitter as a useful tool for communication due to high accessibility, novelty, and real-time format. Students, teachers, and other stakeholders use it as a pedagogical tool to gain information, interact and engage with each other, participate in their respective communities of interests, and share their insights about specific topics. Moreover, Twitter has the potential to enhance students’ learning capabilities as well as improve their motivation and engagement due to its unique features and non-traditional teaching approach. Finally, our analysis advocates for carrying out further empirical studies focusing on digital trace data and inference, particularly in the developing countries.
Introduction
Since their introduction around a decade ago, social media platforms and applications have become steadily popular among public and used widely for entertainment, socialization, and information seeking and sharing purposes (Dolan, Conduit, Fahy, & Goodman, 2016 ; Quan-Haase & Young, 2010 ; Malik et al. 2016 ). Twitter, which can be categorized as a specific form of social media activity – microblogging, was established in 2006 and is one of the prominent social media platforms (others include Facebook, Instagram, and Youtube) across the globe (Alhabash & Ma, 2017 ). Recent statistics released by Twitter indicate that the network receives roughly 1 billion unique monthly visits and hosts around 313 million active users, with 82% being active mobile users (Twitter - Company, 2017 ). According to a recent study by Pew Research Center, Twitter is the fifth most popular social media platform among Americans. The study further points out that roughly one-quarter of online American adults use Twitter, with younger internet users being more active on Twitter when compared to older groups (Greenwood, Perrin, & Duggan, 2016 ).
Twitter’s microblogging feature allows users to publish their ideas and opinions in “real-time messaging” format by writing tweets limited to certain number of characters (initially 140 but now up to 280). Furthermore, by features such as hashtags, mentions, and replies, users can network and dialog with other Twitter users (Steckenbiller, 2016 ). Various aspects of Twitter practices has been researched in a number of domains including tourism (Sotiriadis & van Zyl, 2013 ), sports (Gibbs, O’Reilly, & Brunette, 2014 ), governance (Haro-de-Rosario, Sáez-Martín, & del Carmen Caba-Pérez, 2016 ), health information (Himelboim & Han, 2014 ; Malik et al. 2019 ), elections (Vergeer & Hermans, 2013 ), and activism (Johri et al. 2018 ; Malik et al. 2018 ). Besides using Twitter for entertainment and leisure, the platform is predominantly used for social interaction, information sharing, information seeking, self-documentation, and self-expression (Alhabash & Ma, 2017 ; Liu, Cheung, & Lee, 2010 ).
Even though social media platforms were not designed with the explicit purpose of supporting educational and other learning-related activities, their affordance for networking and content sharing have made them a natural fit for those purposes (Tess, 2013 ). Institutions of higher education, elementary and high schools, scholarly communities, as well as federal and state education agencies have actively embraced various social media platforms (Jordan, 2017 ; Wang, 2016 ). More recently, Twitter is being encompassed as an augmented scholarly communication tool for formal and informal learning. Students, scholars, and professionals from numerous academic domains use the network to connect and engage with peers and public to share discipline specific and other relevant information with an aim of pursuing their academic interests and goals (Holmberg & Thelwall, 2014 ; Veletsianos & Kimmons, 2016 ). A number of recent studies have also demonstrated the value, impact, and acceptance of Twitter in the context of education. For instance, Twitter has been reported to increase learning capabilities and communication (Bista, 2015 ; Carpenter, 2014 ; DeGroot, Young, & VanSlette, 2015 ). Similarly, Twitter is deemed helpful in enhancing engagement and collaboration among peers, teachers, and students (Desselle, 2017 ; Greenhalgh, Rosenberg, & Wolf, 2016 ; Osatuyi & Passerini, 2016 ). On the other hand, few studies have also highlighted the negative aspects of incorporating Twitter in the educational context. These studies have emphasized the inappropriate usage, overexposure, reputation, information overload, addiction, as well as other issues associated with the content and personal privacy (Cho & Rangel, 2017 ; Kinnison, Whiting, Magnier, & Mossop, 2017 ; Rinaldo, Tapp, & Laverie, 2011 ).
Given the exponentially increased usage by numerous entities, the potential of Twitter in education, and the wide range of studies carried out within the domain, there is a strong need to assess the state of scholarly research within the domain. Consequently, we aim to understand the usage of Twitter in various educational settings by systematically reviewing the prior research. More precisely this study examines the research on the use and perceptions of Twitter as a tool to support education and learning by various entities that include students, educationalists, and institutions. Additionally, the current review seeks to identify the obstacles associated with the usage and adoption of Twitter across different setups.
Search strategy
The process of article search and selection followed the PRISMA Statement (Preferred Reporting of Items for Systematic Reviews and Meta-Analyses) (Moher et al., 2009 ). As an initial step of the search strategy, we conducted search in a number of general as well as education focused scholarly databases including ERIC, EBSCOhost (Academic Search Complete), ProQuest, EditLib, Web of Science, ScienceDirect, and PsycINFO. All the studies identified through the database search were evaluated based on the topical relevance and established screening criteria. As an additional step, a non-systematic search was carried out for the articles that were not identified through initial systematic search. In this step, we carried out backward referencing for relevant literature in the text of the articles that were identified through systematic search criteria by using Google Scholar. All the searches were carried out between March 25, 2017, and April 3, 2017. We restricted our search for the last 10 years as Twitter was launched in 2006. During the search process, the following search terms were used: “twitter”, “learning”, “learn”, “education”, “educate”, and “educating”.
Inclusion and exclusion criteria
All the peer-reviewed studies published in scholarly journals during the last ten years (2007–2017) that asses the usage of Twitter in educational and learning context were included in the review. There was no restriction based on the methodology, geographical origin, or quality of the publication forum.
Studies assessing social media in general, other social media platforms (e.g. Facebook, YouTube etc.) as well as studies assessing Twitter together with other social media/learning/microblogging platforms (e.g. MOOC, EdModo) were excluded from the review. Furthermore, commentaries, editorials, letters, and newspaper articles were not accounted for the current review. Studies that were written in languages other than English, conference proceedings, book chapters, theses, and dissertations, and papers that were not accessible for full text were also excluded. A complete list of inclusion and exclusion criteria is outlined in Table 1 .
Study selection
The preliminary search carried out through scholarly databases yielded 2434 results, of which 1121 were excluded after title reviewing. The initial exclusion includes duplicate articles and those deemed clearly irrelevant based on the title of the paper. The remaining 1313 articles went through abstract reviewing, with the 708 articles that did not meet the inclusion criteria being excluded. 605 articles together with 15 articles manually selected by snowball search (backward referencing) methodology were assessed for full-text review. After performing the review of full-text based on eligibility criteria a total of 103 papers were selected for the final assessment. An overview of the articles selection process is illustrated as a PRISMA flow-chart in Fig. 1 .
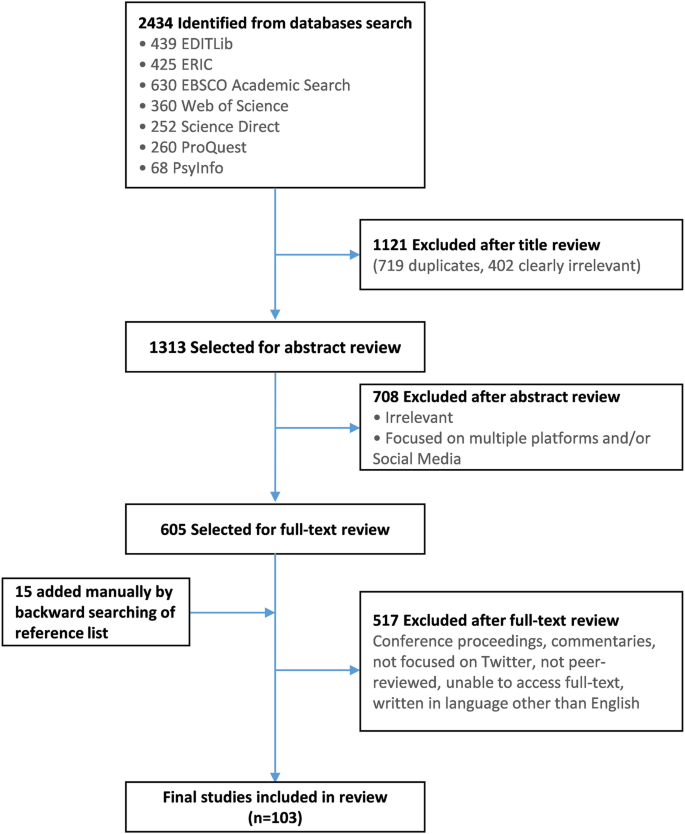
PRISMA flow-chart for Twitter in education
Data extraction
Information was collected on authors’ affiliation, publication forum, database sources, keywords, issues addressed, sampling characteristics, study context and domain as well as the setup country. Furthermore, research design and approach, data collection methods and analysis, and key findings (including limitations and future directions) were also collected. Later on, the data was cross-checked independently by two reviewers and any disagreements were resolved through discussion.
Descriptive features of the studies
Geographical distribution.
A majority of the studies ( N = 60) were carried out in the USA. Likewise, most of the authors ( N = 336) were affiliated with a USA based institution. Subsequently, authors from the UK ( N = 34), Canada (17) and Spain ( N = 15) contributed to these studies as well as the study setups in these countries. We also grouped the countries to assess the contributions region-wise; we found that none of the authors or the context of the study were from Africa or South America. Detailed information about the authors’ affiliation and study setup is presented in Table 2 .
Contextual distribution
In order to get a thorough understanding of the context, we identified the actual place of the study setup (see Table 3 ). The majority of the studies (80.6%) were carried out in higher educational institutes, including colleges and universities. Roughly one out of ten studies was carried out in schools (9.7%). The rest of the studies were carried out online, at a conference, or at another educational institute.
Temporal distribution
Analyzing the year of each publication indicates the growing interest of scholarly community towards the subject. There is a steady increase in the number of articles during the last five years (see Fig. 2 .). Extrapolating from the nine articles already published during first four months of 2017 suggests that the upward trend will continue.
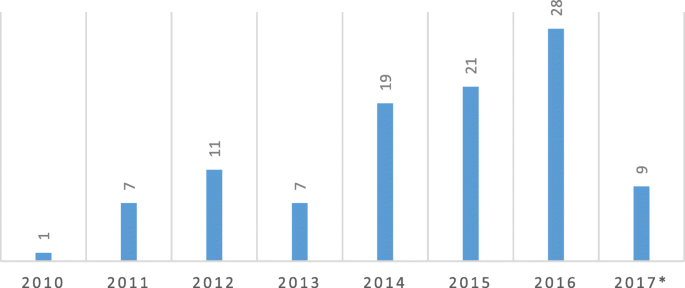
Number of articles by publication year (* until 3.4.2017)
Demographics of study participants
Students comprise an overwhelming majority of the study participants ( N = 74). Professors and teachers were subjects in 14 studies. Table 4 provides additional details of participant demographics.
We also identified the academic disciplines within which the studies were conducted. A large portion of the studies was conducted with participants within the applied sciences ( N = 26) and education ( N = 22) domain followed by participants in multidisciplinary fields ( N = 19). Looking further into sub-fields, interestingly, 21 studies were carried out within medicine. A detailed description of the study disciplines together with the number of respective studies is listed in Table 5 .
Slightly less than half ( N = 48) of these studies used mixed methods research approach. Meanwhile, 35 studies were quantitative, with the remaining 20 being qualitative in nature. With respect to research design, a majority of the studies were case studies ( N = 76) carried out with a confined set of participants e.g. students within a classroom. Digital trace data ( N = 12) and field surveys ( N = 11) were the second most used research designs, whereas, observational ( N = 2) and cross-sectional design ( N = 2) were adopted by the rest of the studies.
Twitter in classroom
Our review postulates that Twitter has been deemed a supportive tool within the classroom and has a strong potential as a technology-enabled learning instrument. Majority of the reviewed studies point out that implementing Twitter improved not only students learning, motivation, engagement, and communication but teaching as well, all of which leads towards creating a more resourceful classroom environment.
With the implementation of Twitter into educational settings, the key focus of the prior research has been on understanding if it helps students learn and if so, how? Most of the studies have found that Twitter is an effective learning tool, especially in the context of formal learning. Learning through digital technologies, especially via Twitter, has been recognized as one of the desired and enjoyable tool by students (Diug, Kendal, Ilic, et al., 2016 ; Hull & Dodd, 2017 ; Welch & Bonnan-White, 2012 ). Establishing a network for the students from which they can reach out and improve their learning experience is strongly facilitated by Twitter (Anthony & Jewell, 2017 ; Bledsoe, Harmeyer, & Wu, 2014 ; Hennessy, Kirkpatrick, Smith, & Border, 2016 ; Marín & Tur, 2014 ). As a platform, Twitter provides the space to improve their skill set, communicate with peers and teachers, think creatively, and at the same time have fun while learning (Al Harbi, 2016 ; Becker & Bishop, 2016 ; Bledsoe et al., 2014 ; Kassens, 2014 ; West, Moore, & Barry, 2015 ).
Twitter’s mixed media format (e.g. pictures, videos, and text) was also considered useful for conceptual learning (Buzzelli, Bissell, & Holdan, 2015 ; Mysko & Delgaty, 2015 ; Tur & Marín, 2015 ). It was also attributed to be highly effective for language learning as it provided more access to the language related resources and interesting ways to practice it (Fewell, 2014 ). For the most part, respondents in a number of studies reacted positively to its inclusion in their courses (Jones et al., 2016 ; Lowe & Laffey, 2011 ; Luo, 2016 ; Tur & Marín, 2015 ), however, to make learning more effective and efficient, teachers and educators must explain its use within the course and how they expect it to be used (Lackovic, Kerry, Lowe, & Lowe, 2017 ).
The platform is also deemed supportive for educators as it provides them opportunities to learn. Twitter enables them to learn more about innovative and effective teaching methods as they connect with other educators that was not likely otherwise (Davis, 2015 ). With its professional networking availability, Twitter also helps academics learn more about their fields from domain specialists (Cho & Rangel, 2017 ; Hitchcock & Young, 2016 ).
Motivation and engagement
Due to its novel format, introducing Twitter into classrooms frequently had the effect of increasing the motivation and engagement of the students and faculty involved. A number of studies point out that students often found the Twitter-based instructions and activities enjoyable, which increased their motivation and excitement for the course in general (Booth, 2015 ; Elavsky, Mislan, & Elavsky, 2011 ; Feliz, Ricoy, & Feliz, 2013 ; Kassens-Noor, 2012 ). As they learned more about Twitter, it helped them to engage better (Mercier, Rattray, & Lavery, 2015 ; Osatuyi & Passerini, 2016 ) that lead to increased motivation to participate within classroom (Ricoy & Feliz, 2016 ; Yakin & Tinmaz, 2013 ).
The online format also increased students motivation to participate by allowing them to post and share in a comfortable space, which in turn eased in-class discussions (Halpin, 2016 ; Luo & Franklin, 2015 ; Tiernan, 2014 ). Within the language learning context, the affordance for microblogging and involvement in a community increased their motivation to learn the language as well (Fewell, 2014 ; Kim, Park, & Baek, 2011 ). It also tended to help engage the students and staff together, allowing for a more holistic and active learning community (Junco, Heiberger, & Loken, 2011 ). Finally, for academics, Twitter’s comprehensive format engaged them further and renewed their professional vigor (Cho & Rangel, 2017 ; Jalali, Sherbino, Frank, & Sutherland, 2015 ). To sum up, the platform shows the potential to be used as an engagement tool in the future, but one must be aware that students’ motivation can be unpredictable especially if used voluntarily; hence, the implementation should be planned well ahead.
Communication affordance
Prior work also indicate that the use of Twitter in classrooms is supportive in increasing the communication not only among participants within classroom settings but with a larger professional community as well. Twitter amplifies communication among these entities by supporting collaboration and starting conversations (Carpenter, 2014 ; Junco, Elavsky, & Heiberger, 2013 ). Furthermore, its 140-character limit allowed students in practicing thoughtful communication more concisely (Lowe & Laffey, 2011 ). Within various classrooms, students regularly communicated through hashtags, relevant or interesting content, responding to one another, and tweets about the course contents, which increased communication among students and faculty (Hull & Dodd, 2017 ). In addition to the classroom effects, there was a positive correlation found between the amount of Twitter usage and peer communication (Evans, 2014 ). Throughout the studies, those students who actively participated gradually began to realize Twitter as a communication tool that can support their learning than just using it for social interactions (Helvie-Mason & Maben, 2017 ).
Moreover, Twitter also provides a convenient way to collaborate on assignments outside of class and to find intellectually stimulating interactions they would not have otherwise had (Gooding, Yinger, & Gregory, 2016 ; Hitchcock & Young, 2016 ; Juhary, 2016 ). According to Lin, Hoffman, & Borengasser, 2013 , the collaboration among students only occurred when prompted by a professor or authoritative figure, while another study by Osatuyi and Passerini ( 2016 ) found the exact opposite to be true. Due to the varied findings, this may be an important area to continue research.
For teachers, it also serves as a convenient means to regularly interact with other teachers who may be limited by distance in order to seek and share insights into teaching practices and informational resources (Carpenter, 2014 ; Carpenter, 2015 ; Carpenter & Krutka, 2015 ; Davis, 2015 ; Gonzalez & Gadbury-Amyot, 2016 ; Greenhalgh et al., 2016 ; Hsu & Ching, 2012 ). It also supports multidirectional communication which allows for the constant, real-time flow of information from the professional and educational sectors (Andrade, Castro, & Ferreira, 2012 ; Goff et al., 2016 ; Kassens, 2014 ; Segado-Boj, Domínguez, & Rodríguez, 2015 ; Wang, 2016 ). The platform also supported teachers in assessing difficulties that students faced with the topics at hand (Cohen & Duchan, 2012 ) and allowed the teachers to continue answering questions and sharing vital information even after the class has ended, therefore extending the pedagogical interaction beyond the class.
Whilst most of the research has been focused on the effects of Twitter on student learning and engagement, some studies have examined its effect on teachers and the effectiveness of using the platform for teaching. A number of studies have found that it is pedagogically supportive and appropriate. These studies endorse that Twitter not only supplements traditional learning material and research methods but also supports effective and appropriate use of social media (Andrade et al., 2012 ; Clarke & Nelson, 2012 ; Halpin, 2016 ; Menkhoff, Chay, Bengtsson, Woodard, & Gan, 2015 ; Rinaldo et al., 2011 ; Yakin & Tinmaz, 2013 ). Twitter is also realized as an important platform by teachers as it digitally connects them with students, allowing them to better understand the topics covered and shape the material they are teaching more effectively (Desselle, 2017 ; Gonzalez & Gadbury-Amyot, 2016 ; Hull & Dodd, 2017 ).
With regards to sharing information, Twitter has also shown potential to be far more effective than traditional teaching approaches (Kassens, 2014 ; Segado-Boj et al., 2015 ). The literature further lays out a number of techniques that can be applied by teachers to effectively engage students in learning through Twitter. For instance, in order to reach out to students and engage them in the classroom, teachers can assign students to compose regular tweets with hashtags, discuss interesting tweets, and promptly reply to students questions and other course information (Hull & Dodd, 2017 ; Pollard, 2014 ). However, when implementing Twitter as a tool for teaching, educators need to plan carefully in advance, be clear in their instructions and consider the limitations of the tool as well (Osgerby & Rush, 2015 ; Williams & Whiting, 2016 ).
Auxiliary support
Outside of the classroom, Twitter has provided many useful additional advantages to students and teachers alike, allowing for professional development and networking opportunities, co-curricular learning, support at conferences, greater information sharing, and overall accessibility.
Professional development and networking
One of the prominent findings in the literature was the positive effects that Twitter had on the professional development and peer-networking of the observed participants. The platform supported both students and educators to build connections, a community, and follow professionals in respective fields (Anthony & Jewell, 2017 ; Draper, Buzzelli, & Holdan, 2016 ; Jacquemin, Smelser, & Bernot, 2014 ; Mysko & Delgaty, 2015 ; Nicholson & Galguera, 2013 ). Twitter not only supports building peer networks but professional ones as well, in which a variety of scholarly information and resources can be shared and followed. This aspect has been experienced extensively, notably in the medical domain (nursing and surgical community), where the information shared is helpful for both students and professionals alike. For instance, Twitter facilitates the medical students and faculty to follow and connect to domain-specific and patients communities, current events, networking with peers and experts, and develop future aspirations (Booth, 2015 ; Goff et al., 2016 ; Jones et al., 2016 ; Reames, Sheetz, Englesbe, & Waits, 2016 ; Sinclair, McLoughlin, & Warne, 2015 ; Visser, Evering, & Barrett, 2014 ).
Teachers from a number of domains also reported using Twitter more for professional development than within classrooms, which reinforced their professional vigor and commitment to work (Cho & Rangel, 2017 ; Greenhalgh et al., 2016 ; Visser et al., 2014 ; Wesely, 2013 ). Research also points out that in recent years, Twitter has emerged as a platform for civic engagement and accessible political information, where scholars share information in order to promote their own practices and research agenda (Nicholson & Galguera, 2013 ; Veletsianos, 2012 ).
Students who used Twitter also found it helpful within the classroom, as it provided support through peer-networking (Hennessy et al., 2016 ). After getting familiar with Twitter in the classroom, some of the students continue further by using it as a career building tool. These students used the platform to follow and network with professionals in their respective fields, and anticipated using Twitter in the future for professional development along their careers (Carpenter, Tur, & Marín, 2016 ; Jacquemin et al., 2014 ; Tur, Marín-Juarros, & Carpenter, 2017 ; Waldrop & Wink, 2016 ). Another study by Nicholson and Galguera ( 2013 ) found that Twitter also aided students into the workforce transition. Whilst there are many positive reactions to the professional development provided by Twitter, a study by Lackovic et al. ( 2017 ), points out that experts and leading Twitter users dominate the platform, not allowing students to participate in the conversation which renders it far less effective. Overall, the majority of the studies have found that exposure to Twitter tends to improve participants opinions of its use as a tool for professional development and networking.
Information sharing
Twitter was also recognized useful for easy resource and information sharing to a wide breadth of people, more so than traditional classroom teaching methods (Goff et al., 2016 ; Kassens, 2014 ; Lin et al., 2013 ; Stephens & Gunther, 2016 ). Scholars covered a multitude of topics and at the same time promoted their own research and information about their classrooms. On the other hand, students shared relatively limited information, as they were typically the recipients of information (Kimmons & Veletsianos, 2016 ; Knight & Kaye, 2016 ; G. Veletsianos, 2012 ). Twitter is also deemed as one of the potential tools for disseminating information to public by many state education agencies (Kimmons, Veletsianos, & Woodward, 2017 ; Segado-Boj et al., 2015 ; Wang, 2016 ). However, despite Twitters’ affordance of two-way communication, the information tend to come in the form of a monologue by most of these institutions (Kimmons et al., 2017 ; Knight & Kaye, 2016 ).
Research also describe how Twitter use for information sharing purposes support users in various educational context. For instance, respondents used the platform for advocacy, keeping up with current issues, professional information, news, and exposure to new ideas (Camiel, Goldman-Levine, Kostka-Rokosz, & McCloskey, 2014 ; Greenhalgh et al., 2016 ; Nicholson & Galguera, 2013 ; Rehm & Notten, 2016 ). In classes, it provided an online forum that made sharing resources and information far easier and was considered a useful tool, especially in obtaining supplemental material (Desselle, 2017 ; Juhary, 2016 ; Lin et al., 2013 ; Stephens & Gunther, 2016 ). This led to students demonstrating improvement in classes with Twitter use than those without it (Fewell, 2014 ). Many of those who participated in the studies at hand enjoyed having access to a constant, rapid flow of information. This rapid communication allowed students to feel as though they had greater access to staff and could gain information from them in a timely manner (Cho & Rangel, 2017 ; Diug et al., 2016 ). Twitter also provided students a more comfortable atmosphere for information sharing. Students also felt more at ease with sharing information and opinions in person, also allowing students to comment on each other’s posts sharing further insights (Gooding et al., 2016 ; Lin et al., 2013 ; Sinclair et al., 2015 ; Tiernan, 2014 ; Wright, Frame, & Hartzler, 2014 ). With respect to the quality of information, the resources available on Twitter were considered more reliable and relevant. Classes that were taught how to properly use Twitter to find the information witnessed a significant decline in Google searches and an increase in the usage of reputable sources (Halpin, 2016 ).
Usage at the conferences
Though limited, research has also addressed the usage of Twitter at academic conferences. Twitter is often referred as intellectually stimulating and a worthy platform to disseminate new knowledge and information (Jalali et al., 2015 ). However, the use of Twitter in conference settings reported low uptake and usage, as well as several barriers attributed to its use. The study by Kimmons and Veletsianos ( 2016 ) indicates that few students and professors used Twitter at the conferences. Most of the professors who used Twitter at conferences reasoned it for networking purposes rather than communicating (Kimmons & Veletsianos, 2016 ; Li & Greenhow, 2015 ). Academics posted on the platform in order to cover information and connect with communities, meanwhile non-academic counterparts posted to positively promote topics and to critique periodically (Kimmons & Veletsianos, 2016 ).
Social aspects
The literature also emphasizes various social aspects associated with Twitter-based instruction that lead to a number of benefits. It allows for stronger community building and networking, as well as a reduction in shyness and isolation. Some of the literature also highlights demographic variation in the usage and adoption of Twitter for educational purposes.
Communities of practice
A benefit of integrating Twitter into the learning environment, especially in the classroom, is the sense of community it facilitates by supporting active collaboration and opportunities to communicate and share information inside and outside of the classroom (Becker & Bishop, 2016 ; Booth, 2015 ; Bull & Adams, 2012 ; Carpenter & Krutka, 2015 ; Lomicka & Lord, 2012 ). Twitter allows students to connect with each other as well as interact with instructors and professionals, which leads to the creation of social and professional support networks (Anthony & Jewell, 2017 ; Camiel et al., 2014 ; Cho & Rangel, 2017 ; Visser et al., 2014 ).
Twitter also facilitated a space for students to network and share information confidently with the community (Becker & Bishop, 2016 ; Mysko & Delgaty, 2015 ; Veletsianos, 2012 ). A number of students further report that Twitter’s hashtag feature is highly effective in building these communities, as it allows them to build connections and feel as though they belong to the community (Bledsoe et al., 2014 ). Courses that include Twitter usage have been found to have a significantly stronger sense of a classroom community and comfort with peers than those without it (Clarke & Nelson, 2012 ; Rohr & Costello, 2015 ; Ross, Banow, & Yu, 2015 ; Smith & Tirumala, 2012 ; Wright et al., 2014 ). Participation in the community increased their engagement, and the meaningfulness of what the students were learning (Bull & Adams, 2012 ; Evans, 2014 ; West et al., 2015 ). The sense of community in the online environment also enhanced students’ self-confidence. For instance, studies by Cohen and Duchan ( 2012 ) and Kinnison et al. ( 2017 ), report confidence facilitation via Twitter, as the platform allowed more timid students to participate as much as others.
For teachers, Twitter allowed them to create a new type of social network that digitally spanned farther distances. This created a unique experience that allowed them to interact and collaborate professionally with colleagues outside of their immediate communities (Davis, 2015 ; Greenhalgh et al., 2016 ; Munoz, Pellegrini-Lafont, & Cramer, 2014 ). Studies also report relationship improvement between instructors and students, as the platform promotes open and real-time communication (DeGroot et al., 2015 ; Diug et al., 2016 ; Domizi, 2013 ; Gonzalez & Gadbury-Amyot, 2016 ; Rehm & Notten, 2016 ). Furthermore, Twitter goes beyond simply connecting with classmates and support learners to follow and build a community for the purpose of learning. For instance, students studying a foreign language can interact with native speakers and know more about their culture. On Twitter they can practice with convenience outside of class while remaining in close contact with those who speak their first language (Fewell, 2014 ; Kim et al., 2011 ; Steckenbiller, 2016 ). Finally, Twitter is also supportive to educationalists other than students and teachers. For instance academic administrators use Twitter to increase their leadership by building a community that focuses on education (Sauers & Richardson, 2015 ).
Combatting shyness and isolation
In addition to creating a community, Twitter serves as a platform to help students combat isolation and shyness. Twitter supports the participation of shy students in a more active manner (Cohen & Duchan, 2012 ; Kinnison et al., 2017 ; Mercier et al., 2015 ; Tiernan, 2014 ). Many students expressed that they would not have shared their opinions otherwise if they had not been using Twitter (Fox & Varadarajan, 2011 ). Due to its capability to hold online conversations, it diminishes the sense of isolation in online classes which strongly supports the personal development of individual students (Munoz et al., 2014 ; Rehm & Notten, 2016 ). Similarly, the study by Wright ( 2010 ) also indicates that Twitter is helpful in reducing isolation and boosting self-confidence among the learning community.
Demographic variations
With regards to gender, males and females used Twitter equally. However, younger groups use and regard Twitter’s appropriateness for educational purposes far more than the older ones (Draper et al., 2016 ; Feliz et al., 2013 ). Furthermore, studies also reported differences between the usage of students, professors, academics, and scholars as well. Students tended to use Twitter less at conferences and for learning activities than others (e.g. Professors), but using it more for non-academic purposes (Kimmons & Veletsianos, 2016 ; Knight & Kaye, 2016 ; Li & Greenhow, 2015 ; Veletsianos & Kimmons, 2016 ). Students tended to exemplify their learning through Tweets and interactions, but not in an overt way (Prestridge, 2014 ) as they often refer to technology in a more general sense than professors and other professionals within academia (Veletsianos & Kimmons, 2016 ). Professors tended to use Twitter for specific academic purposes instead, often focusing on specific academic subjects (Knight & Kaye, 2016 ; Veletsianos & Kimmons, 2016 ). On the other hand, administrators in academia are found to have the majority of their usage focused on promoting community, educational issues and enhancing their leadership (Sauers & Richardson, 2015 ). Moreover, academic administrators appeared to focus on their own reputations rather than its utility to students, which can consist of posting Tweets of a wider variety and attempting to reach a larger community (Knight & Kaye, 2016 ).
Usage outcomes and perceptions
A number of studies also emphasize the outcomes and perceptions associated with the usage of Twitter in the educational context. Overall, most of the studies indicate positively about a number of aspects including retention rates, student grades, increased credibility for teachers, and the intentions of using Twitter in future. However, a few studies also point out concerns and adverse reactions as well as reasons behind hindering the usage and adoption associated with Twitter for educational purposes.
Performance outcomes (grading)
Although this aspect of using Twitter was only assessed in a few studies, most of them indicate that the platform supported students’ performance with respect to grading. Those students that used more of Twitter in their classes ended up with much higher assignment scores and grade point averages than the rest of the students (Diug et al., 2016 ; Junco et al., 2013 , 2011 ). Likewise, classes where Twitter was implemented as a supportive tool displayed higher learning as well as received higher grades than the counterparts (Clarke & Nelson, 2012 ; Diug et al., 2016 ; Gonzalez & Gadbury-Amyot, 2016 ). Due to limited research on the topic, a concrete decree cannot be established, hence this is one of the areas that merit further research.
A limited number of studies moreover focused on the retention rate once the use of Twitter was involved, but those that did found conflicting results. Some of these studies found that retention was significantly increased by the usage of Twitter in class, leading to an improved memory among students during testing (Blessing, Blessing, & Fleck, 2012 ; Stephens & Gunther, 2016 ). However, another study found no correlation between Twitter usage and increased retention in classes (Smith & Tirumala, 2012 ). This particular aspect has little research and would be therefore an important area to explore further.
Credibility
A handful of research has been conducted to assess the credibility of instructors with relation to Twitter usage. For instance, DeGroot et al. ( 2015 ), found that students perceived those professors more credible whose Twitter profile featured posts about education, professional information and resources. Another study, further found that there was higher credibility among those professors that shared more personal information or had a socially active Twitter profile (Johnson, 2011 ). Due to limited research on this topic, this is another interesting research avenue that merits future research.
Future use intentions
In general, respondents of various studies are quite positive about using the platform in the future. Most participants intended to continue using it for professional and teaching purposes, maintaining positive opinions about its usefulness (Carpenter et al., 2016 ; Carpenter & Krutka, 2015 ; Hitchcock & Young, 2016 ; Luo & Franklin, 2015 ; Marín & Tur, 2014 ). One of the key reason by students behind this is that they consider Twitter as a tool that encourages confidence and autonomous learning (Leis, 2014 ). Moreover, it encourages learning beyond the class content and many respondents desired to have more classes where Twitter is part of the curriculum (Menkhoff et al., 2015 ). Research also indicates that Twitter is also favored over other social media platforms by pre-service teachers. For instance, a study by Mills ( 2014 ), point out that many pre-service teachers were aspired by Twitter as an auxiliary pedagogical tool and exhibited a strong desire and intention to introduce it in their future classes.
Adverse perceptions and adoption obstacles
Through our review, we also found several recurring negative aspects to Twitter usage within educational context. Distraction, information overload, privacy, and limited space for expression were some of the frequently reported issues. With the overwhelming amount of tweets, it was difficult for the users to keep up with the relevant content (Bull & Adams, 2012 ; Cho & Rangel, 2017 ; Davis, 2015 ; Fox & Varadarajan, 2011 ; Gonzalez & Gadbury-Amyot, 2016 ; Lin et al., 2013 ). This paired with another major concern that Twitter was too much of a distraction in the classroom when it came to taking notes and participating in class discussions (Fox & Varadarajan, 2011 ; Mercier et al., 2015 ; Yakin & Tinmaz, 2013 ). The same concern is also raised by respondents of another study by Wright et al. ( 2014 ), who found that Twitter distracted in-class face to face discussions and prevented them from taking important notes (Wright et al., 2014 ). Another related perception surfaced that Twitter was not conducive to course discussions or participation as many students were unable to participate in discussions on Twitter due to the presence of more knowledgeable and dominant experts of the field (Jacquemin et al., 2014 ; Lackovic et al., 2017 ). Due to this conception among learners, they preferred using other social media platforms that are not as professional-oriented as Twitter. For instance, the study by Mills ( 2014 ), reported that respondents favored using other social media tools (e.g. Facebook) to Twitter for learning purposes. Furthermore, study by Segado-Boj et al. ( 2015 ), regard Twitter as a platform for disseminating research but not for teaching purposes, as it serves more of a research showcase than a platform for collaboration. The same concern is also raised by Jacquemin et al. ( 2014 ), who reported that for the classroom setting, Twitter can be a suitable tool for information sharing purposes but not conducive to course discussions.
Many studies also reported logistical issues with usage and implementation of Twitter. Most of the first-time users did not foresee the benefits of using Twitter for educational purposes. This negative perception was mostly related to higher learning curve that required effort and time to gain command over various syntactical features and communication style native to Twitter (Bull & Adams, 2012 ; Marr & DeWaele, 2015 ; Stephens & Gunther, 2016 ). Twitter was also seen as either too obtuse, or ineffective for formal discussion within the class, and unmanageable for larger classes (Jacquemin et al., 2014 ; Kassens, 2014 ). Due to learning curve, student resistance, and difficulty in judging the credibility of information, institutions find it challenging to expand the use and reach of Twitter (Kassens-Noor, 2012 ; Kimmons & Veletsianos, 2016 ; Mysko & Delgaty, 2015 ; Rinaldo et al., 2011 ).
In line with research on privacy aspects of social media (Malik et al. 2016 ), a number of studies also highlight students concerns over their right to privacy and how these concerns affected their Twitter use (DeGroot et al., 2015 ; Gonzalez & Gadbury-Amyot, 2016 ; Rinaldo et al., 2011 ). With the growing use of the internet for professional purposes, concerns associated with online reputation were also flagged (Cho & Rangel, 2017 ; Kinnison et al., 2017 ). There was also a concern for judgment and exposure within the class. Some students also displayed anxiety over grammatical correctness and the reactions from fellows and other users on the platforms (Kinnison et al., 2017 ). Similarly, another study also found that students used it infrequently and were unaware of the guidelines of using Twitter for educational purposes that led to anxiety towards its use and adoption (Gooding et al., 2016 ).
Moreover, the constricting nature of the 140 character limit (currently 280), was also considered challenging (Cohen & Duchan, 2012 ; Luo & Franklin, 2015 ). Due to restricted space to convey ideas through a tweet, the complexity and depth of students’ thoughts got confined, and placed an unfair burden on them (Bledsoe et al., 2014 ; Carpenter, 2015 ). According to Bull and Adams ( 2012 ), this tended to force content in an unoriginal, baseless direction. Finally, another concern raised by Mysko and Delgaty ( 2015 ) in terms of instruction and learning through Twitter is that it is difficult to judge the credibility of information and sources particularly for students, which is highly critical in educational context.
Implications for future research and practice
When looking at the structure of the prior research, we have found that there is a lack of diversity within the studies conducted, as most of them are case studies and oriented towards few disciplines. This has left us with data that cannot be comfortably generalized. As it’s not that long since Twitter got introduced in various educational settings, there has also been a severe lack of longitudinal studies. Similarly, there is a strong need as well as a desire within the academic community to further expand through exploiting digital trace data (netnography) and inferential data. With the greater amount of Twitter data and users currently accessible, both of these limitations can be addressed. With respect to studies carried out in various academic disciplines, there is a further need to conduct studies within arts and natural sciences as the research has so far concentrated on applied sciences and education domains.
We also observed some of the concerns with the tools that researchers have been using. While the use of Twitter for learning and engagement is important, we do not currently have a single holistic standard for learning as indicated by Carpenter et al., 2016 . Similarly there is a lack of standard measurements of engagement indices and analysis of the usefulness of pedagogical analytics specifically focusing on the platform (Junco et al., 2011 ; Menkhoff et al., 2015 ). In addition, numerous studies use self-reporting as their main form of data collection. In order to have more representative data, comparing the reported findings to the actual usage (monitored data) of the respondents can also be fruitful for the domain (Hull & Dodd, 2017 ). The overall push needs to use a more rigorous experimental design by collecting more quantitative data to support the current qualitative findings.
Next, the current understanding of Twitter in educational context has been confined that needs to further expanded. For instance, how Twitter can be more supportive for various learning contexts. Likewise, how Twitter can be promoted within these settings so that different entities are encouraged to adopt it for learning. There is also a strong need to further address the collaboration and connections formed on Twitter and how effective and lasting bonds can be formed over time in and outside the classroom. With so much negative press around the use of social media and the decline of cooperation and collaboration between students, this research could provide insight into how the use of social media can bring people together as opposed to creating barriers.
Investigating the use of Twitter for other educational settings than for learning purposes further can also be another valuable research avenue. For instance, Twitter can be a useful tool when planning a conference, but there is not enough evidence on how it affects the participants to have the extra influence (Jalali et al., 2015 ). In addition, there needs to be a further exploration of using Twitter as a backchannel and how this affects the participation and academic identity of individuals at conferences. The current research has another major limitation as the focus is usually towards a specific domain as the test subjects (for instance pre-service nurses and teachers) that limits the nuances of the platform used in other disciplines.
There is also a focus on comparing Twitter to the plethora of other teaching formats. The most basic of which is using Twitter versus not using it at all, or using the traditional method of teaching (Blessing et al., 2012 ; Luo, 2016 ; Veletsianos & Kimmons, 2016 ; Visser et al., 2014 ). A detailed understanding towards the reasons why some instructors choose to use it while others do not will be highly supportive. Subsequently, there is also the issue of Twitter versus other forms of social media. Within this comes the question of whether or not learning benefits by the use of social media in general. However, if it is assumed that it does then the studies must turn to explore two options, the benefits of using Twitter over other types of social media, or the combination of Twitter and social media (Carpenter, 2014 ; West et al., 2015 ).
While there can be drawbacks to using Twitter, preliminary data has found that it has the potential to support professional development for both students and teachers (Carpenter, 2014 ; Carpenter & Krutka, 2014 , 2015 ). This is another area that requires additional research in order to gain more reliable data, as most of the research has been self-reported. However, if we were able to confidently state that Twitter had a positive impact on professional development, the platform could be used in innovative ways such as supporting teachers in developing countries and improving their training in more cost-effective ways (Carpenter, 2015 ). With such benefits to the use of Twitter in education, the most important direction in which future research should traverse is to understand the barriers associated with Twitter use. For instance investigating the perceptions of non-users, or why some teachers (as well as learners) who see the educational benefit of using Twitter avoid using the tool. Once we better understand these barriers, we can prioritize preferred social media for educational purposes with sound reasoning. Based on this understanding, strategies can be developed to circumvent barriers to enable more productive implementation of technology in the classroom. Finally, most of the analyzed studies span North America and Europe, yet there is a strong need to conduct more studies around the topic in other parts of the globe, particularly within Asian and African countries where the adoption rate of social media is phenomenal.
Conclusions
We analyzed 103 studies related to the use of Twitter in education. We found clear positive impact of integrating Twitter within classrooms for teaching and learning purposes. In non-classroom learning and other scholarly contexts (e.g. institutional use and conferences), Twitter strongly supports professional following and networking that ultimately resulted in improved teaching, learning, and collaboration. In both contexts, Twitter provides affordances for strongly connecting and bonding with others and forming a community. The platform also supports communication among the participants due to its real-time format and novel features. Furthermore, it provides them a channel for professional development and networking with peers, professionals, and authorities in their respective fields, with whom they can connect, communicate, share relevant resources, and follow prominent figures. Finally, many studies also point out that integrating Twitter within coursework motivated learners to participate actively, as most of them perceived it to be a highly effective non-traditional learning tool.
Availability of data and materials
The datasets used and/or analyzed during the current study are available from the corresponding author on reasonable request.
Al Harbi, M. (2016). Effects of twitter-assisted learning on developing literacy skills and motivation for learning in EFL settings. Arab Journal for the Humanities , 34 (135), 268-292.
Alhabash, S., & Ma, M. (2017). A tale of four platforms: Motivations and uses of facebook, twitter, instagram, and snapchat among college students. Social Media+ Society , 3 (1) https://doi.org/10.1177/2056305117691544 .
Article Google Scholar
Andrade, A., Castro, C., & Ferreira, S. A. (2012). Cognitive communication 2.0 in higher education: To tweet or not to tweet? Electronic Journal of E-Learning , 10 (3), 293–305.
Google Scholar
Anthony, B., & Jewell, J. R. (2017). Students’ perceptions of using twitter for learning in social work courses. Journal of Technology in Human Services , 35 (1), 38–48.
Becker, R., & Bishop, P. (2016). “Think bigger about science”: Using twitter for learning in the middle grades. Middle School Journal , 47 (3), 4–16.
Bista, K. (2015). Is twitter a pedagogical tool in higher education? Perspectives of education graduate students. Journal of the Scholarship of Teaching and Learning , 15 (2), 83.
Bledsoe, T. S., Harmeyer, D., & Wu, S. F. (2014). Utilizing twitter and #hashtags toward enhancing student learning in an online course environment. International Journal of Distance Education Technologies , 12 (3), 75–83.
Blessing, S. B., Blessing, J. S., & Fleck, B. K. B. (2012). Using twitter to reinforce classroom concepts. Teaching of Psychology , 39 (4), 268–271. https://doi.org/10.1177/0098628312461484 .
Booth, R. G. (2015). Happiness, stress, a bit of vulgarity, and lots of discursive conversation: A pilot study examining nursing students’ tweets about nursing education posted to twitter. Nurse Education Today , 35 (2), 322–327. https://doi.org/10.1016/j.nedt.2014.10.012 .
Article MathSciNet Google Scholar
Bull, P. H., & Adams, S. (2012). Learning technologies: Tweeting in a high school social studies class. I-Manager’s Journal of Educational Technology , 8 (4), 26.
Buzzelli, A., Bissell, J., & Holdan, G. (2015). Analyzing Twitter’s impact on student engagement in college instruction. International Journal of Information and Communication Technology Education , 12 (2), 3–14.
Camiel, L. D., Goldman-Levine, J. D., Kostka-Rokosz, M. D., & McCloskey, W. W. (2014). Twitter as a medium for pharmacy students’ personal learning network development. Currents in Pharmacy Teaching and Learning , 6 (4), 463–470.
Carpenter, J. (2014). Twitter’s capacity to support collaborative learning. International Journal of Social Media and Interactive Learning Environments , 2 (2), 103–118.
Carpenter, J. (2015). Preservice teachers’ microblogging: Professional development via twitter. Contemporary Issues in Technology and Teacher Education , 15 (2), 209–234.
Carpenter, J., & Krutka, D. G. (2014). How and why educators use twitter: A survey of the field. Journal of Research on Technology in Education , 46 (4), 414–434. https://doi.org/10.1080/15391523.2014.925701 .
Carpenter, J., & Krutka, D. G. (2015). Engagement through microblogging: Educator professional development via twitter. Professional Development in Education , 41 (4), 707–728. https://doi.org/10.1080/19415257.2014.939294 .
Carpenter, J., Tur, G., & Marín, V. I. (2016). What do U.S. and Spanish pre-service teachers think about educational and professional use of twitter? A comparative study. Teaching and Teacher Education , 60 , 131–143. https://doi.org/10.1016/j.tate.2016.08.011 .
Cho, V., & Rangel, V. S. (2017). The dynamic roots of school leaders’ twitter use. JSL Vol 26-N5, 26 , 837.
Clarke, T. B., & Nelson, C. L. (2012). Classroom community, pedagogical effectiveness, and learning outcomes associated with twitter use in undergraduate marketing courses. Journal for Advancement of Marketing Education , 20 (2), 29-38.
Cohen, A., & Duchan, G. (2012). The usage characteristics of twitter in the learning process. Interdisciplinary Journal of E-Learning and Learning Objects , 8 (1), 149–163.
Davis, K. (2015). Teachers’ perceptions of twitter for professional development. Disability and Rehabilitation , 37 (17), 1551–1558.
DeGroot, J. M., Young, V. J., & VanSlette, S. H. (2015). Twitter use and its effects on student perception of instructor credibility. Communication Education , 64 (4), 419–437. https://doi.org/10.1080/03634523.2015.1014386 .
Desselle, S. P. (2017). The use of twitter to facilitate engagement and reflection in a constructionist learning environment. Currents in Pharmacy Teaching and Learning , 9 (2), 185–194. https://doi.org/10.1016/j.cptl.2016.11.016 .
Diug, B., Kendal, E., Ilic, D., et al. (2016). Evaluating the use of twitter as a tool to increase engagement in medical education. Education for Health , 29 (3), 223.
Dolan, R., Conduit, J., Fahy, J., & Goodman, S. (2016). Social media engagement behaviour: A uses and gratifications perspective. Journal of Strategic Marketing , 24 (3–4), 261–277.
Domizi, D. P. (2013). Microblogging to foster connections and community in a weekly graduate seminar course. TechTrends , 57 (1), 43.
Draper, J., Buzzelli, A. A., & Holdan, E. G. (2016). Patterns of twitter usage in one cohort-based doctoral program. International Journal of Doctoral Studies , 11 , 163-183.
Elavsky, C. M., Mislan, C., & Elavsky, S. (2011). When talking less is more: Exploring outcomes of Twitter usage in the large-lecture hall. Learning, Media and Technology , 36 (3), 215–233. https://doi.org/10.1080/17439884.2010.549828 .
Evans, C. (2014). Twitter for teaching: Can social media be used to enhance the process of learning?: Twitter for teaching. British Journal of Educational Technology , 45 (5), 902–915. https://doi.org/10.1111/bjet.12099 .
Feliz, T., Ricoy, C., & Feliz, S. (2013). Analysis of the use of twitter as a learning strategy in master’s studies. Open Learning: The Journal of Open, Distance and e-Learning , 28 (3), 201–215. https://doi.org/10.1080/02680513.2013.870029 .
Fewell, N. (2014). Social networking and language learning with twitter. Research Papers in Language Teaching and Learning , 5 (1), 223.
Fox, B. I., & Varadarajan, R. (2011). Use of twitter to encourage interaction in a multi-campus pharmacy management course. American Journal of Pharmaceutical Education , 75 (5), 88.
Gibbs, C., O’Reilly, N., & Brunette, M. (2014). Professional team sport and twitter: Gratifications sought and obtained by followers. International Journal of Sport Communication , 7 (2), 188–213. https://doi.org/10.1123/IJSC.2014-0005 .
Goff, D. A., Jones, C., Toney, B., Nwomeh, B. C., Bauer, K., & Ellison, E. C. (2016). Use of twitter to educate and engage surgeons in infectious diseases and antimicrobial stewardship. Infectious Diseases in Clinical Practice , 24 (6), 324–327.
Gonzalez, S. M., & Gadbury-Amyot, C. C. (2016). Using twitter for teaching and learning in an oral and maxillofacial radiology course. Journal of Dental Education , 80 (2), 149–155.
Gooding, L. F., Yinger, O. S., & Gregory, D. (2016). #music students: College music students’ twitter use and perceptions. Update: Applications of Research in Music Education , 34 (2), 45–53.
Greenhalgh, S. P., Rosenberg, J. M., & Wolf, L. G. (2016). For all intents and purposes: Twitter as a foundational technology for teachers. E-Learning and Digital Media , 13 (1–2), 81–98. https://doi.org/10.1177/2042753016672131 .
Greenwood, S., Perrin, A., & Duggan, M. (2016). Social media update 2016 Retrieved August 14, 2017, from http://www.pewinternet.org/2016/11/11/social-media-update-2016/ .
Halpin, P. A. (2016). Using twitter in a nonscience major science class increases students’ use of reputable science sources in class discussions. Journal of College Science Teaching , 45 (6), 71.
Haro-de-Rosario, A., Sáez-Martín, A., & del Carmen Caba-Pérez, M. (2016). Using social media to enhance citizen engagement with local government: Twitter or Facebook? New Media & Society. https://doi.org/10.1177/1461444816645652 .
Helvie-Mason, L., & Maben, S. (2017). Twitter-vism: Student narratives and perceptions of learning from an undergraduate research experience on twitter activism. Teaching Journalism & Mass Communication , 7 (1), 47.
Hennessy, C. M., Kirkpatrick, E., Smith, C. F., & Border, S. (2016). Social media and anatomy education: Using twitter to enhance the student learning experience in anatomy: Use of twitter in anatomy education. Anatomical Sciences Education , 9 (6), 505–515. https://doi.org/10.1002/ase.1610 .
Himelboim, I., & Han, J. Y. (2014). Cancer talk on twitter: Community structure and information sources in breast and prostate cancer social networks. Journal of Health Communication , 19 (2), 210–225. https://doi.org/10.1080/10810730.2013.811321 .
Hitchcock, L. I., & Young, J. A. (2016). Tweet, tweet!: Using live twitter chats in social work education. Social Work Education , 35 (4), 457–468. https://doi.org/10.1080/02615479.2015.1136273 .
Holmberg, K., & Thelwall, M. (2014). Disciplinary differences in twitter scholarly communication. Scientometrics , 101 (2), 1027–1042. https://doi.org/10.1007/s11192-014-1229-3 .
Hsu, Y.-C., & Ching, Y.-H. (2012). Mobile microblogging: Using twitter and mobile devices in an online course to promote learning in authentic contexts. The International Review of Research in Open and Distributed Learning , 13 (4), 211–227.
Hull, K., & Dodd, J. E. (2017). Faculty use of twitter in higher education teaching. Journal of Applied Research in Higher Education , 9 (1), 91–104. https://doi.org/10.1108/JARHE-05-2015-0038 .
Jacquemin, S. J., Smelser, L. K., & Bernot, M. J. (2014). Twitter in the higher education classroom: A student and faculty assessment of use and perception. Journal of College Science Teaching , 43 (6), 22–27.
Jalali, A., Sherbino, J., Frank, J., & Sutherland, S. (2015). Social media and medical education: Exploring the potential of twitter as a learning tool. International Review of Psychiatry , 27 (2), 140–146. https://doi.org/10.3109/09540261.2015.1015502 .
Johnson, K. A. (2011). The effect of Twitter posts on students’ perceptions of instructor credibility. Learning, Media and Technology , 36 (1), 21–38. https://doi.org/10.1080/17439884.2010.534798 .
Johri, A., Karbasian, H., Malik, A., Handa, R., & Purohit, H. (2018). How Diverse Users and Activities Trigger Connective Action via Social Media: Lessons from the Twitter Hashtag Campaign# ILookLikeAnEngineer. In Proceedings of HICSS . Hawaii.
Jones, R., Kelsey, J., Nelmes, P., Chinn, N., Chinn, T., & Proctor-Childs, T. (2016). Introducing twitter as an assessed component of the undergraduate nursing curriculum: Case study. Journal of Advanced Nursing , 72 (7), 1638–1653. https://doi.org/10.1111/jan.12935 .
Jordan, K. (2017). Examining the UK higher education sector through the network of institutional accounts on twitter. First Monday , 22 (5). https://doi.org/10.5210/fm.v22i5.7133 .
Juhary, J. (2016). Revision through twitter: Do tweets affect students’ performance? International Journal of Emerging Technologies in Learning (IJET) , 11 (04), 4. https://doi.org/10.3991/ijet.v11i04.5124 .
Junco, R., Elavsky, C., & Heiberger, G. (2013). Putting twitter to the test: Assessing outcomes for student collaboration, engagement and success: Twitter collaboration & engagement. British Journal of Educational Technology , 44 (2), 273–287. https://doi.org/10.1111/j.1467-8535.2012.01284.x .
Junco, R., Heiberger, G., & Loken, E. (2011). The effect of twitter on college student engagement and grades: Twitter and student engagement. Journal of Computer Assisted Learning , 27 (2), 119–132. https://doi.org/10.1111/j.1365-2729.2010.00387.x .
Kassens, A. L. (2014). Tweeting your way to improved #writing, #reflection, and #community. The Journal of Economic Education , 45 (2), 101–109. https://doi.org/10.1080/00220485.2014.889937 .
Kassens-Noor, E. (2012). Twitter as a teaching practice to enhance active and informal learning in higher education: The case of sustainable tweets. Active Learning in Higher Education , 13 (1), 9–21.
Kim, E.-Y., Park, S.-M., & Baek, S.-H. (2011). Twitter and implications for its use in EFL learning. Multimedia Assisted Language Learning , 14 (2), 113–137.
Kimmons, R., & Veletsianos, G. (2016). Education scholars’ evolving uses of twitter as a conference backchannel and social commentary platform: Twitter backchannel use. British Journal of Educational Technology , 47 (3), 445–464. https://doi.org/10.1111/bjet.12428 .
Kimmons, R., Veletsianos, G., & Woodward, S. (2017). Institutional uses of twitter in US higher education. Innovative Higher Education , 42 (2), 97–111.
Kinnison, T., Whiting, M., Magnier, K., & Mossop, L. (2017). Evaluating #VetFinals: Can twitter help students prepare for final examinations? Medical Teacher , 39 (4), 436–443. https://doi.org/10.1080/0142159X.2017.1296561 .
Knight, C. G., & Kaye, L. K. (2016). ‘To tweet or not to tweet?’ A comparison of academics’ and students’ usage of twitter in academic contexts. Innovations in Education and Teaching International , 53 (2), 145–155. https://doi.org/10.1080/14703297.2014.928229 .
Lackovic, N., Kerry, R., Lowe, R., & Lowe, T. (2017). Being knowledge, power and profession subordinates: Students’ perceptions of twitter for learning. The Internet and Higher Education , 33 , 41–48. https://doi.org/10.1016/j.iheduc.2016.12.002 .
Leis, A. (2014). Encouraging autonomy through the use of a social networking system. JALT CALL Journal , 10 (1), 69–80.
Li, J., & Greenhow, C. (2015). Scholars and social media: Tweeting in the conference backchannel for professional learning. Educational Media International , 52 (1), 1–14. https://doi.org/10.1080/09523987.2015.1005426 .
Lin, M.-F. G., Hoffman, E. S., & Borengasser, C. (2013). Is social media too social for class? A case study of twitter use. TechTrends , 57 (2), 39.
Liu, I., Cheung, C., & Lee M. (2010). Understanding Twitter usage: What drive people continue to tweet . Proceedings of Pacific Asia Conference on Information Systems. Taipei, Taiwan.
Lomicka, L., & Lord, G. (2012). A tale of tweets: Analyzing microblogging among language learners. System , 40 (1), 48–63. https://doi.org/10.1016/j.system.2011.11.001 .
Lowe, B., & Laffey, D. (2011). Is twitter for the birds? Using twitter to enhance student learning in a marketing course. Journal of Marketing Education , 33 (2), 183–192.
Luo, T. (2016). Enabling microblogging-based peer feedback in face-to-face classrooms. Innovations in Education and Teaching International , 53 (2), 156–166. https://doi.org/10.1080/14703297.2014.995202 .
Luo, T., & Franklin, T. (2015). Tweeting and blogging: Moving towards education 2.0. International Journal on E-Learning , 14 (2), 235-258.
Malik, A., Dhir, A., & Nieminen, M. (2016). Uses and gratifications of digital photo sharing on Facebook. Telematics and Informatics, 33 (1), 129–138.
Malik, A., Hiekkanen, K., & Nieminen, M. (2016). Privacy and trust in Facebook photo sharing: age and gender differences. Program, 50 (4):462–480.
Malik, A., Johri, A., Handa, R., Karbasian, H., & Purohit, H. (2018). How social media supports hashtag activism through multivocality: A case study of# ILookLikeanEngineer. First Monday, 23 (11). https://doi.org/10.5210/fm.v23i11.9181 .
Malik, A., Li, Y., Karbasian, H., Hamari, J., & Johri, A. (2019). Live, love, Juul: User and content analysis of Twitter posts about Juul. American Journal of Health Behavior, 43 (2), 326–336.
Marín, V. I., & Tur, G. (2014). Student teachers’ attitude towards twitter for educational aims. Open Praxis , 6 (3), 275–285.
Marr, J., & DeWaele, C. S. (2015). Incorporating twitter within the sport management classroom: Rules and uses for effective practical application. Journal of Hospitality, Leisure, Sport & Tourism Education , 17 , 1–4. https://doi.org/10.1016/j.jhlste.2015.05.001 .
Menkhoff, T., Chay, Y. W., Bengtsson, M. L., Woodard, C. J., & Gan, B. (2015). Incorporating microblogging (“tweeting”) in higher education: Lessons learnt in a knowledge management course. Computers in Human Behavior , 51 , 1295–1302. https://doi.org/10.1016/j.chb.2014.11.063 .
Mercier, E., Rattray, J., & Lavery, J. (2015). Twitter in the collaborative classroom: Micro-blogging for in-class collaborative discussions. International Journal of Social Media and Interactive Learning Environments , 3 (2), 83–99.
Mills, M. (2014). Effect of faculty member’s use of twitter as informal professional development during a preservice teacher internship. Education , 14 (4), 451–467.
Moher, D., Liberati, A., Tetzlaff, J., Altman, D. G., Group, P, et al. (2009). Preferred reporting items for systematic reviews and meta-analyses: The PRISMA statement. PLoS Medicine , 6 (7), e1000097.
Munoz, L. R., Pellegrini-Lafont, C., & Cramer, E. (2014). Using social Media in Teacher Preparation Programs: Twitter as a means to create social presence. Penn GSE Perspectives on Urban Education , 11 (2), 57–69.
Mysko, C., & Delgaty, L. (2015). How and why are students using twitter for# MEDED? Integrating twitter into undergraduate medical education to promote active learning. Annual Review of Education, Communication & Language Sciences , 12 , 24-52.
Nicholson, J., & Galguera, T. (2013). Integrating new literacies in higher education: A self-study of the use of twitter in an education course. Teacher Education Quarterly , 40 (3), 7–26.
Osatuyi, B., & Passerini, K. (2016). Twittermania: Understanding how social media technologies impact engagement and academic performance of a new generation of learners. CAIS , 39 , 23.
Osgerby, J., & Rush, D. (2015). An exploratory case study examining undergraduate accounting students’ perceptions of using twitter as a learning support tool. The International Journal of Management Education , 13 (3), 337–348. https://doi.org/10.1016/j.ijme.2015.10.002 .
Pollard, E. A. (2014). Tweeting on the backchannel of the jumbo-sized lecture hall: Maximizing collective learning in a world history survey. The History Teacher , 47 (3), 329–354.
Prestridge, S. (2014). A focus on students’ use of twitter–their interactions with each other, content and interface. Active Learning in Higher Education , 15 (2), 101–115.
Quan-Haase, A., & Young, A. L. (2010). Uses and gratifications of social media: A comparison of Facebook and instant messaging. Bulletin of Science, Technology & Society , 30 (5), 350–361.
Reames, B. N., Sheetz, K. H., Englesbe, M. J., & Waits, S. A. (2016). Evaluating the use of twitter to enhance the educational experience of a medical school surgery clerkship. Journal of Surgical Education , 73 (1), 73–78.
Rehm, M., & Notten, A. (2016). Twitter as an informal learning space for teachers!? The role of social capital in twitter conversations among teachers. Teaching and Teacher Education , 60 , 215–223. https://doi.org/10.1016/j.tate.2016.08.015 .
Ricoy, M.-C., & Feliz, T. (2016). Twitter as a learning community in higher education. Journal of Educational Technology & Society , 19 (1), 237.
Rinaldo, S. B., Tapp, S., & Laverie, D. A. (2011). Learning by tweeting: Using twitter as a pedagogical tool. Journal of Marketing Education , 33 (2), 193–203. https://doi.org/10.1177/0273475311410852 .
Rohr, L., & Costello, J. (2015). Student perceptions of twitters’ effectiveness for assessment in a large enrollment online course. Online Learning , 19 (4), n4.
Ross, H. M., Banow, R., & Yu, S. (2015). The use of twitter in large lecture courses: Do the students see a benefit? Contemporary Educational Technology , 6 (2), 126–139.
Sauers, N. J., & Richardson, J. W. (2015). Leading by following: An analysis of how K-12 school leaders use twitter. NASSP Bulletin , 99 (2), 127–146.
Segado-Boj, F., Domínguez, M. Á. C., & Rodríguez, C. C. (2015). Use of twitter among Spanish communication-area faculty: Research, teaching and visibility. First Monday , 20 (6) https://doi.org/10.5210/fm.v20i6.5602 .
Sinclair, W., McLoughlin, M., & Warne, T. (2015). To twitter to woo: Harnessing the power of social media (SoMe) in nurse education to enhance the student’s experience. Nurse Education in Practice , 15 (6), 507–511. https://doi.org/10.1016/j.nepr.2015.06.002 .
Smith, J. E., & Tirumala, L. N. (2012). Twitter’s effects on student learning and social presence perceptions. Teaching Journalism & Mass Communication , 2 (1), 212.
Sotiriadis, M. D., & van Zyl, C. (2013). Electronic word-of-mouth and online reviews in tourism services: The use of twitter by tourists. Electronic Commerce Research; New York , 13 (1), 103–124. https://doi.org/10.1007/s10660-013-9108-1 .
Steckenbiller, C. (2016). Am kürzeren Ende der Sonnenallee in 140 characters or less: Using twitter as a creative approach to literature in the intermediate German classroom. Die Unterrichtspraxis/Teaching German , 49 (2), 147–160.
Stephens, T. M., & Gunther, M. E. (2016). Twitter, millennials, and nursing education research. Nursing Education Perspectives , 37 (1), 23–27.
Tess, P. A. (2013). The role of social media in higher education classes (real and virtual)–a literature review. Computers in Human Behavior , 29 (5), A60–A68.
Tiernan, P. (2014). A study of the use of twitter by students for lecture engagement and discussion. Education and Information Technologies , 19 (4), 673–690. https://doi.org/10.1007/s10639-012-9246-4 .
Tur, G., & Marín, V. I. (2015). Enhancing learning with the social media: Student teachers’ perceptions on twitter in a debate activity. Journal of New Approaches in Educational Research , 4 (1), 46.
Tur, G., Marín-Juarros, V., & Carpenter, J. (2017). Using twitter in higher education in Spain and the USA. Comunicar , 25 (51). https://doi.org/10.3916/C51-2017-02 .
Twitter - Company. (2017). Retrieved August 14, 2017, from https://about.twitter.com/en_us.html .
Veletsianos, G. (2012). Higher education scholars’ participation and practices on twitter: Scholars’ participation and practices on twitter. Journal of Computer Assisted Learning , 28 (4), 336–349. https://doi.org/10.1111/j.1365-2729.2011.00449.x .
Veletsianos, G., & Kimmons, R. (2016). Scholars in an increasingly open and digital world: How do education professors and students use twitter? The Internet and Higher Education , 30 , 1–10. https://doi.org/10.1016/j.iheduc.2016.02.002 .
Vergeer, M., & Hermans, L. (2013). Campaigning on twitter: Microblogging and online social networking as campaign tools in the 2010 general elections in the Netherlands. Journal of Computer-Mediated Communication , 18 (4), 399–419.
Visser, R. D., Evering, L. C., & Barrett, D. E. (2014). #TwitterforTeachers: The implications of twitter as a self-directed professional development tool for K–12 teachers. Journal of Research on Technology in Education , 46 (4), 396–413. https://doi.org/10.1080/15391523.2014.925694 .
Waldrop, J., & Wink, D. (2016). Twitter: An application to encourage information seeking among nursing students. Nurse Educator , 41 (3), 160–163. https://doi.org/10.1097/NNE.0000000000000235 .
Wang, Y. (2016). US state education agencies’ use of twitter: Mission accomplished? SAGE Open , 6 (1). https://doi.org/10.1177/2158244015626492 .
Welch, B. K., & Bonnan-White, J. (2012). Twittering to increase student engagement in the university classroom. Knowledge Management & E-Learning: An International Journal (KM&EL) , 4 (3), 325–345.
Wesely, P. M. (2013). Investigating the community of practice of world language educators on twitter. Journal of Teacher Education , 64 (4), 305–318.
West, B., Moore, H., & Barry, B. (2015). Beyond the tweet: Using twitter to enhance engagement, learning, and success among first-year students. Journal of Marketing Education , 37 (3), 160–170.
Williams, D., & Whiting, A. (2016). Exploring the relationship between student engagement, twitter, and a learning management system: A study of undergraduate marketing students. International Journal of Teaching and Learning in Higher Education , 28 (3), 302–313.
Wright, N. (2010). Twittering in teacher education: Reflecting on practicum experiences. Open Learning: The Journal of Open and Distance Learning , 25 (3), 259–265. https://doi.org/10.1080/02680513.2010.512102 .
Wright, K. J., Frame, T. R., & Hartzler, M. L. (2014). Student perceptions of a self-care course taught exclusively by team-based learning and utilizing twitter. Currents in Pharmacy Teaching and Learning , 6 (6), 842–848. https://doi.org/10.1016/j.cptl.2014.07.003 .
Yakin, I., & Tinmaz, H. (2013). Using twitter as an instructional tool: A case study in higher education. TOJET: The Turkish Online Journal of Educational Technology , 12 (4), 209-218.
Download references
Acknowledgements
Not applicable.
The work presented here is supported in part by U.S. National Science Foundation Award#: 1424444, 1707837, 1712129, and 1741754. Dr. Malik partly contributed to this work as a postdoctoral scholar at GMU and Cassie's contribution was supported by a NSF REU. Any opinions, findings, and conclusions or recommendations expressed in this material are those of the authors and do not necessarily reflect the views of the funding agencies.
Author information
Authors and affiliations.
Department of Computer Science, Aalto University, Espoo, 02150, Finland
Aqdas Malik
Department of Information Sciences & Technology, George Mason University, Fairfax, VA, 22030, USA
Aqdas Malik & Aditya Johri
William & Mary, Williamsburg, VA, 23187-8795, USA
Cassandra Heyman-Schrum
You can also search for this author in PubMed Google Scholar
Contributions
AM planned and lead all the phases of the manuscript. CH supported in data analysis, and contributed in writing the manuscript. All authors read and approved the final manuscript.
Authors’ information
Corresponding author.
Correspondence to Aqdas Malik .
Ethics declarations
Competing interests.
The authors declare that they have no competing interests.
Additional information
Publisher’s note.
Springer Nature remains neutral with regard to jurisdictional claims in published maps and institutional affiliations.
Rights and permissions
Open Access This article is distributed under the terms of the Creative Commons Attribution 4.0 International License ( http://creativecommons.org/licenses/by/4.0/ ), which permits unrestricted use, distribution, and reproduction in any medium, provided you give appropriate credit to the original author(s) and the source, provide a link to the Creative Commons license, and indicate if changes were made.
Reprints and permissions
About this article
Cite this article.
Malik, A., Heyman-Schrum, C. & Johri, A. Use of Twitter across educational settings: a review of the literature. Int J Educ Technol High Educ 16 , 36 (2019). https://doi.org/10.1186/s41239-019-0166-x
Download citation
Received : 26 March 2019
Accepted : 01 August 2019
Published : 25 September 2019
DOI : https://doi.org/10.1186/s41239-019-0166-x
Share this article
Anyone you share the following link with will be able to read this content:
Sorry, a shareable link is not currently available for this article.
Provided by the Springer Nature SharedIt content-sharing initiative
- Social media

An official website of the United States government
The .gov means it’s official. Federal government websites often end in .gov or .mil. Before sharing sensitive information, make sure you’re on a federal government site.
The site is secure. The https:// ensures that you are connecting to the official website and that any information you provide is encrypted and transmitted securely.
- Publications
- Account settings
Preview improvements coming to the PMC website in October 2024. Learn More or Try it out now .
- Advanced Search
- Journal List
- J Med Internet Res
- v.24(11); 2022 Nov

Ethical and Methodological Considerations of Twitter Data for Public Health Research: Systematic Review
Courtney takats.
1 City University of New York School of Public Health, New York City, NY, United States
Rachel Wormer
Dari goldman, heidi e jones, diana romero, associated data.
Supplementary tables.
Full data extraction sheet.
Much research is being carried out using publicly available Twitter data in the field of public health, but the types of research questions that these data are being used to answer and the extent to which these projects require ethical oversight are not clear.
This review describes the current state of public health research using Twitter data in terms of methods and research questions, geographic focus, and ethical considerations including obtaining informed consent from Twitter handlers.
We implemented a systematic review, following PRISMA (Preferred Reporting Items for Systematic Reviews and Meta-Analyses) guidelines, of articles published between January 2006 and October 31, 2019, using Twitter data in secondary analyses for public health research, which were found using standardized search criteria on SocINDEX, PsycINFO, and PubMed. Studies were excluded when using Twitter for primary data collection, such as for study recruitment or as part of a dissemination intervention.
We identified 367 articles that met eligibility criteria. Infectious disease (n=80, 22%) and substance use (n=66, 18%) were the most common topics for these studies, and sentiment mining (n=227, 62%), surveillance (n=224, 61%), and thematic exploration (n=217, 59%) were the most common methodologies employed. Approximately one-third of articles had a global or worldwide geographic focus; another one-third focused on the United States. The majority (n=222, 60%) of articles used a native Twitter application programming interface, and a significant amount of the remainder (n=102, 28%) used a third-party application programming interface. Only one-third (n=119, 32%) of studies sought ethical approval from an institutional review board, while 17% of them (n=62) included identifying information on Twitter users or tweets and 36% of them (n=131) attempted to anonymize identifiers. Most studies (n=272, 79%) included a discussion on the validity of the measures and reliability of coding (70% for interreliability of human coding and 70% for computer algorithm checks), but less attention was paid to the sampling frame, and what underlying population the sample represented.
Conclusions
Twitter data may be useful in public health research, given its access to publicly available information. However, studies should exercise greater caution in considering the data sources, accession method, and external validity of the sampling frame. Further, an ethical framework is necessary to help guide future research in this area, especially when individual, identifiable Twitter users and tweets are shared and discussed.
Trial Registration
PROSPERO CRD42020148170; https://www.crd.york.ac.uk/prospero/display_record.php?RecordID=148170
Introduction
Since its launch in 2006, Twitter has become one of the most popular social media sites as a platform that allows users to post and interact with short messages known as tweets. According to a 2019 survey by Pew Research Center [ 1 ], 1 in 5 (23%) adults in the United States report using Twitter. While Twitter users are not representative of the general population (users tend to be younger, more educated, and located in urban or suburban areas) [ 2 ], the volume of publicly available tweets allows for research to be conducted on large data sets, eschewing a common perceived limitation of small samples.
Public health researchers have identified “big data” from Twitter as a new wellspring from which research can be conducted [ 3 ]. However, the utility of these data depends on the appropriateness of the research questions and the methodological approaches used in sampling and analyzing the data. Previous systematic reviews have explored how Twitter data have been used. A systematic review by Sinnenberg et al [ 4 ] of 137 articles using Twitter in health research between 2010 and 2015 found that the main research questions explored with Twitter data involved content analysis, surveillance, engagement, recruitment, intervention, and network analysis. Similarly, a scoping review from 2020 [ 5 ] found 92 articles that fell within 6 domains: surveillance, event detection, pharmacovigilance, forecasting, disease tracking, and geographic identification. Additional systematic reviews of social media, beyond Twitter alone, have examined specific domains, for instance, exploring how these data, including Twitter, are being used for public health surveillance [ 6 - 8 ] or pharmacovigilance [ 9 - 11 ].
While social media provides new opportunities for data sources in research, some unique obstacles are also present. For instance, the presence of spam and noisy data can make it difficult for researchers to identify a legitimate signal for the research topic in question [ 12 ]. To navigate this issue, researchers sometimes opt to employ traditional manual coding of content; however, this can be a nonideal solution given the size of the data sets and the time and effort required for these analyses [ 13 ]. Other teams have used natural language processing (NLP) or machine learning approaches, which present their own problems; one study [ 14 ] found that among the algorithms built to classify emotions, the highest performing model had an accuracy of 65%. The landscape of social media necessitates understanding of the mechanisms and limitations of the platforms, as well as adaptations to the requirements of this landscape.
In addition to the research questions and methodological approaches used with Twitter data, the extent to which social media data are in general considered public, and what this means for ethical research oversight are unclear. There is substantial literature discussing the ethics of using social media data for public health research, but clear ethical guidelines have not been established [ 15 - 24 ].
The need for these guidelines is increasingly pressing, as leveraging social media for public health research raises questions about privacy and anonymity; properly deidentifying user data requires the researchers to understand an “increasingly networked, pervasive, and ultimately searchable dataverse” [ 18 ]. Information shared on social media can often be intensely personal; hence, anonymity would be even more important for research involving sensitive data such as health conditions and disease [ 23 ]. This is particularly relevant for the field of public health, since the data collected and analyzed for public health research will often fall into these more sensitive categories.
Beyond the questions of user anonymity, when conducting research on more sensitive health information, traditional research protocols center the importance of informed consent among participants. However, there are currently no established guidelines for the expectation of consent when leveraging publicly available social media data. Some theorists in the realm of internet research ethics have proposed an assessment model that determines the need for consent based on possibility of pain or discomfort. They further suggest that this assessment should consider the vulnerability of the population being studied and the sensitivity of the topics [ 22 ].
In the systematic review by Sinnenberg et al [ 4 ], approximately one-third of the 137 articles included therein mentioned ethical board approval. Given that Twitter usage has changed dramatically in recent years [ 25 ], this systematic review is an updated examination of both ethical considerations and research questions or methodologies across all domains of public health research using Twitter.
We sought to investigate the methodological and ethical aspects of using Twitter data for public health research from 2006, when Twitter was launched, to 2019 [ 26 ]. Specifically, we describe the measures being used in Twitter research, the extent to which they are validated and reliable, and the extent to which ethical oversight is included in studies using publicly available tweets.
This review followed the PRISMA (Preferred Reporting Items for Systematic Reviews and Meta-Analyses) guidelines [ 27 , 28 ] and was registered with PROSPERO (CRD42020148170).
Eligibility Criteria
The database search was limited to peer-reviewed public health studies originally written in English, which were published between January 2006 and October 31, 2019, and used social media data to explore a public health research question. The social media platforms included in the search were Twitter and Sina Weibo (China’s version of Twitter), Facebook, Instagram, YouTube, Tumblr, or Reddit.
Studies were excluded if they were systematic or literature reviews, marketing or sales research, only investigated organizational-level tweets, investigated tweets from conferences in disciplines other than public health, or included primary data collection asking participants about their social media use. We excluded articles that focused on organizations disseminating information to the public (evaluation of social media dissemination and analysis of organizational- or institutional-level social media data) or testing interventions that used social media as a method (intervention study using social media), as our research question was not related to interventions using social media platforms as a tool but rather explored how existing social media data are being used in secondary analyses in public health research.
Given the volume of studies identified, separate analyses were conducted on Facebook and YouTube; thus, this systematic review focuses solely on Twitter. Studies that included Twitter and other social media platforms were included, but only Twitter findings were extracted.
Information Sources
We searched PubMed, SocINDEX, and PsycINFO for articles about social media and public health after consulting with our institutional librarian on the best approaches to the search.
The search strategy consisted of the Boolean search term: ((“Social media” OR twitter OR tweet* OR facebook OR instagram OR youtube OR tumblr OR reddit OR “web 2.0” OR “public comments” OR hashtag*) AND (“public health” OR “health research” OR “community health” OR “population health”)).
Study Selection
Three authors reviewed abstracts for eligibility in a 2-step process, with each abstract reviewed by 2 authors independently. A first screen was performed on the basis of the title and abstract; if deemed ineligible, the study was excluded from further screening. Disagreements were resolved through discussion and consensus. Full texts of the remaining articles were retrieved for the second screen and reasons for exclusion were coded and ranked by the priority of exclusion criteria for cases in which more than one exclusion criterion was applied ( Figure 1 ). Disagreements about inclusion and exclusion criteria were resolved through discussion and consensus.

PRISMA (Preferred Reporting Items for Systematic Reviews and Meta-Analyses) flowchart for systematic review of methodological approaches and ethical considerations for public health research using Twitter data, 2006-2019.
Data Collection Process
Data were extracted using a standardized data extraction spreadsheet, which was developed a priori and refined during the data extraction process. This refinement resulted in the removal of data elements; new data elements were not added. To establish consistency in extractions, 2 reviewers independently extracted data from the same 5 articles and compared the results. This process continued during weekly meetings, in which papers of varying complexity were discussed until consensus was reached. No studies were excluded on the basis of their quality.
The data items in this review categorized information about the study within 4 domains: (1) study characteristics: public health topic, year, and country of publication; (2) study design and results: sample size, Twitter data extraction method, operationalization (ie, which data points were collected from social media posts and how researchers quantified these data), methodologic and analytic approaches, primary results, and descriptions of linking or account data; (3) ethical considerations: ethical approval, discussion of informed consent, and general discussion of ethical issues; and (4) risk of bias or methodological checks: quality assessment, validity, reliability, and accuracy checks implemented. We defined methodological approach as the overall objective of a research project coupled with the operationalization of methods to fulfill this objective.
Quality assessment metrics were adapted from existing quality assessment tools used for systematic reviews [ 29 - 31 ]. The specific quality assessment metrics were the following: whether the stated research question matches the data-defined research question, the presence of a clearly defined objective or hypothesis, validity of measures, reliability of measures, validation of computer algorithms, whether the data analysis is sufficiently grounded, whether findings logically flow from the analysis and address the research questions, and the presence of a clear description of limitations. A study was considered to have addressed validity if the measures used were based on validated measures, previous studies, or existing frameworks. A study addressed reliability if manual coding efforts incorporated checks or assessed intercoder reliability, descriptions of reliability were not expected for studies that only used machine learning. Accuracy checks were described if manual checks were performed by researchers or validation of computer algorithms used for studies using machine learning algorithms and NLP.
Summary Measures
The summary measures related to methods and study design include the following: the frequency of studies by topic, geographic focus, year of publication, analytic approach, sampling approach, and overall methodological approach or objective of the study (ie, surveillance, content exploration, sentiment mining, network science, and model development and testing). The summary measures related to ethical considerations include the frequency of studies that sought institutional review board (IRB) review or approval, included informed consent from Twitter handlers, discussed ethical considerations within the paper, and reported identifying results (ie, verbatim tweets). For quality assessment, we present information on the validity and reliability of measures used; a full summary of quality assessments is provided in Multimedia Appendix 1 .
Our search resulted in 6657 unique studies for review, of which 730 required full-text review ( Figure 1 ). We identified 539 studies across all social media platforms; 367 used Twitter data forming the analytic sample for this review ( Multimedia Appendix 2 for the full list of included articles with all data extraction fields; for readability of text, references are only included when details of specific articles are provided as contextual examples).
Study Characteristics
Public health research topics.
The most common public health topics among the articles reviewed were communicable diseases (eg, influenza, Ebola, and Zika; n=80, 22%), substance use (n=66, 18%), health promotion (n=63, 17%), chronic disease (eg, cancer; n=48, 13%), and environmental health (n=48, 13%; Multimedia Appendix 1 ).
Year of Publication
The year of publication for the articles in this review ranged from 2010 to 2019. A sharp increase in the number of Twitter articles was observed from 2012 to 2017 ( Figure 2 ). Two preprint articles on October 31, 2019, were included in the count for 2019 [ 32 , 33 ].

Number of articles published by year for systematic review of methodological approaches and ethical considerations for public health research using Twitter data, 2006-2019.
Geographic Focus
Most studies analyzed tweets originating from the United States (n=158, 43%) or worldwide (n=134, 36%); only 75 (20%) of them focused on non-US regions or countries. Of the articles that had a global geographic focus, 23 (17%) of them collected geotags and reported on geospatial metrics within the body of the article. Despite having a worldwide focus, these 23 articles demonstrated a bias toward the United States, western Europe (namely the United Kingdom), Canada, and Australia; the majority of the data collected in these studies were posts originating in these countries, with a distinct minority representing other regions or countries.
Study Design and Results
Sample size and unit of analysis.
Of the 367 articles reviewed here, 355 (97%) used individual tweets as the unit of analysis and 11 (3%) used Twitter accounts (or “handles”) as the unit of analysis. One article (0.3%) used keywords as the unit of analysis, as the study sought to identify keywords that would help researchers detect influenza epidemics via Twitter [ 34 ].
There was a wide range of sample sizes. For studies with tweets as the unit of analysis (n=353), the number of analyzed tweets ranged from 82 [ 35 ] to 2.77 billion [ 36 ] (median=74,000), with 90 papers having a sample size larger than 1 million. Similarly, for studies using Twitter handles as the unit of analysis (n=11), the sample size ranged from 18 [ 37 ] to 217,623 [ 32 ].
Methods for Accessing Data
To pull data from Twitter, most studies used application programming interfaces (APIs) that were developed by Twitter (eg, Gardenhose and Firehose) and could be integrated into statistical software packages. Third-party APIs (eg, Twitonomy and Radian6) were also used frequently, either through contracting with a commercial vendor, purchasing tweets that match specified criteria, or using software developed by an entity outside of Twitter. Most studies either mentioned that they used an API without indicating the specific type (37%) or did not mention their method of tweet accession (13%; Table 1 ). Of papers that identified the API used, purposive and random sampling were equally employed. However, only 22 (7%) articles explicitly mentioned whether the API used was purposive or random in its sampling technique; when the API was named (eg, decahose, search API, and Gardenhose) but the sampling type was not noted in the article, we looked up the sampling technique in use by the API.
Frequency of studies by access method and data source from a systematic review of methodological approaches and ethical considerations for public health research using Twitter data, 2006-2019.
a Accession methods and sampling type are differentiated as random or purposive in accordance with reports from the articles’ authors or Twitter.
We also found that the description of the sampling method was often not described. For instance, some Twitter APIs are purposive in nature (eg, Twitter Search API) and some are random (Twitter Firehose API) or systematic (some REST APIs). Many studies did not specify what type of sampling was used to extract tweets from Twitter or did not fully explain retrieval limitations (eg, how it might affect the sample population if only a certain number of tweets could be retrieved daily through an API).
Methodological Approach
As seen in Table 2 , the most common methodological approaches were as follows: thematic exploration (eg, describing the themes of conversations about e-cigarettes on Twitter) [ 38 ], sentiment mining (eg, assessing if tweets about vaccines are positive, negative, or neutral) [ 39 ], and surveillance (eg, tracking the patterns of information spread about an Ebola outbreak) [ 40 ]. Less common methodological approaches were tool evaluation (eg, using Twitter data to predict population health indices) [ 41 ] and network science (eg, examining health information flows) [ 42 ]. Different methodological approaches tended to be pursued for different topics. For example, most infectious disease research was in the domain of surveillance, whereas research about mental health and experiences with the health care system was more conducive to thematic exploration and sentiment mining.
Frequency of studies by methodological approach and analytical technique from a systematic review of methodological approaches and ethical considerations for public health research using Twitter data, 2006-2019.
a Multiple responses were allowed.
Across the 3 most common study methodological approaches (thematic exploration, sentiment mining, and surveillance), approximately one-third of the papers (36%) used machine learning ( Table 2 ). Machine learning here is defined as an application of algorithms and statistical modeling to reveal patterns and relationships in data without explicit instruction (eg, to identify the patterns of dissemination related to Zika virus–related information on Twitter) [ 43 ]. This can be contrasted to NLP, which necessitates explicit instruction; often, NLP is used to identify and classify words or phrases from a predefined list in large data sets (eg, to identify the most common key topics used by Twitter users regarding the opioid epidemic) [ 44 ]. Of the articles reviewed, NLP was more prevalent in sentiment mining than in other types of methodological approaches.
Ethical Considerations
Presence of identifying information.
Just under half (n=174, 47%) of the articles reviewed did not contain any identifying information of Twitter accounts or tweets, 36% (n=131) of them contained anonymized account information or paraphrased tweets, and 17% (n=62) of them contained direct quotes of tweets or identifiable information such as Twitter handles or account names ( Table 3 ). Of the 62 articles that included verbatim tweets or identifying information about the user, one-third (n=21, 34%) of them included a discussion of ethics in the paper (eg, Berry et al [ 45 ]).
Frequency of studies by ethics-related factors from a systematic review of methodological approaches and ethical considerations for public health research using Twitter data, 2006-2019.
a Note that 3 articles included both an extensive discussion of ethics as well as details regarding their anonymization process.
b The denominator for the articles that discussed ethics is 109.
Less than half of the articles (n=173, 47%) indicated that they did not use any of the metadata (eg, username, demographics, and geolocation) associated with the tweet ( Multimedia Appendix 1 ). Approximately one-third of the articles (n=110, 30%) used geographic information associated with the tweet, and a much smaller number of articles (n=15, 4%) included photos associated with the account or health information (such as illness disclosure or mentions of medications taken). Of the articles analyzing tweets from either the United States or another specific region or country (n=233), 37% (n=86) of them used geotags of Twitter accounts to identify the location of the tweets; of the articles that did not specify a geographic region (n=134), 17% (n=23) of them used geotagging.
Though research on infectious disease and health promotion were most likely to include user metadata in their data analyses, linked health information was most often used in papers about infectious disease and mental health, often in the form of medical self-disclosures.
IRB Approval and Informed Consent
Just under one-third of the articles reviewed (n=119; 32%) explicitly stated that those studies sought and received IRB review or approval ( Table 3 ). The majority (n=226, 61%) of them did not mention IRB approval, although many of these articles included statements about the nature of Twitter posts being publicly available. Only a small subset (n=23, 6%) of studies explicitly stated that IRB approval was not necessary.
Among those that sought IRB approval (n=119), over half (n=68, 57%) of them were granted exemptions; just under half (n=49, 41%) of them did not specify the type of approval received. Two studies [ 46 , 47 ] received full IRB approval. One of them [ 46 ] retrospectively examined existing public data about health beliefs regarding the human papillomavirus and was approved with a waiver of consent owing to its retrospective design. The other study [ 47 ] had 2 parts: study 1 consisted of a survey of self-reported stress following a school lockdown, and study 2 consisted of data mining of community-level rumor generation during the lockdown on Twitter. The survey necessitated informed consent as it involved human participants; hence, the full scope of the study (parts 1 and 2) had to undergo IRB review. None of the studies using only Twitter data sought informed consent, even when including identifying information from Twitter handlers or tweets. Over two-thirds of the articles (n=258, 70%) did not include a discussion of ethics or privacy concerns.
Additionally, 53 (49%) articles discussed the anonymization of data used in their study either by omitting usernames and Twitter handles [ 48 ] or by providing only paraphrased tweets to prevent exact-match searching [ 49 ]. Only 5 studies included specific and extensive discussions around the ethical implications of social media research and went beyond disclaimer statements about the publicly available nature of tweets. One study [ 50 ] described consulting guidelines for internet research from various organizations and researchers, while another [ 51 ] included a long “ethical considerations” section that described needing to “weigh threats to safety and privacy against benefits gained by using novel approaches to study suicide,” and acknowledged vulnerable populations and risks of stigma and discrimination. Another study [ 52 ] raised the challenge of social media research given the lack of relevant ethical frameworks.
Risk of Bias in Individual Studies
We found that 270 (74%) articles included a clear description of the validity of measures; 21 (6%) articles were purely exploratory in nature and collected only counts of tweets, so we deemed them exempt from an assessment of validity of measures; 76 (21%) articles did not include efforts at establishing measurement validity. Further, of the 264 articles involving human coding, 184 (70%) included a description of intercoder reliability and quality assurance checks, while 80 (30%) did not. Similarly, 235 articles involved computer algorithms or automated coding, of which 165 (70%) explicitly described accuracy checks or validation of the algorithms, while 70 (39%) did not.
In addition to concerns about validity and reliability of measures, one of the main sources of bias was the sampling frame. The self-selection of Twitter users was discussed in most of the studies, with 85% (n=314) of them describing this as a potential limitation.
Principal Findings
We saw evidence of a steep increase in publications using Twitter data after 2012, which may be due to Twitter releasing its native standard (version 1.1) API in 2012, which made mining of its data much more accessible to the general public without the need for complex coding capabilities [ 53 ]. The prevalence of research using “big data” from Twitter is increasing and will likely continue to do so in the coming years [ 50 ].
Infectious disease was the most common topic of the research papers, which may indicate a burgeoning interest in using social media to detect disease outbreaks. It is likely that a review of studies using Twitter data that picks up from where this study left off (ie, after October 31, 2019) would support this finding given the onset of the COVID-19 pandemic in late 2019.
There are some major considerations that this review highlights for the future of public health research using Twitter data. Most of the research focused on Twitter users in the United States; this includes the articles with a global focus that demonstrated a bias toward the anglophone world. Three articles appeared to genuinely have a representative global scope; interestingly, two of these were about the Zika virus. This indicates the data scraped from Twitter tends to be heavily focused on the United States and English-speaking settings.
Another major consideration is that of the accession method used to build a data set. Most of the studies examined in this review used APIs or variations thereof; only 10 studies used alternative accession methods. Those 10 studies used data either extracted from Twitter for previous studies or hosted in pre-existing databases. Of the remaining studies that used an API, only 22 studies explained whether the API used was purposive or random in nature. This is of interest because the sampling technique of APIs has been called into question in previous papers [ 54 , 55 ]. In particular, the Twitter Streaming API is considered to produce less representative samples and should be approached with caution; this API is susceptible to intentional or accidental bias based on inclusion and exclusion criteria selected for a particular study [ 56 ]. Owing to the “black box” nature (ie, lack of documentation of the sampling approach) of native Twitter APIs, it cannot be determined that data retrieved using Twitter APIs are truly random [ 57 , 58 ].
In addition to the aforementioned obstacles, there are questions about the accuracy of algorithms using machine learning and NLP. A little less than half of the papers reviewed for this systematic review involved surveillance and prediction, and approximately one-sixth of them evaluated new tools or frameworks in the realm of Twitter data. Machine learning was commonly used for these methodological approaches. However, a previous evaluation of the efficacy of using various machine learning algorithms to automatically identify emotions expressed on Twitter found that the highest performing algorithm achieved an accuracy rate of 65% [ 14 ]. Another recent article found that machine learning was not effective in making meaningful predictions about users’ mental health from language use on social media; further, Twitter metadata and language use was not specific to any one mental health condition [ 59 ].
This raises concerns about the overall use of social media data for research, as data science in general and public health research in particular use data to make insights; these data “then get acted upon and the decisions impact people’s lives” [ 20 ]. Hence, conscientious planning is advised when using publicly available social media data for the purpose of public health research.
Discussion of Ethics
Given that slightly over one-third of studies anonymized Tweets or Twitter users, many researchers seem to think that there are ethical considerations when using these data, even if they are publicly available. Nevertheless, the majority of projects did not seek IRB review or approval. This contradiction suggests an implicit understanding that while there are no international or place-specific ethical guidelines around research using social media data, there is something unique about the nature of this research that distinguishes it from truly public data.
International ethical standards for biomedical and public health research already exist, and these standards often continue to influence the national guidelines that develop within a given country [ 60 - 62 ]. Given the global scope of social media, it may be most prudent for guidelines to be established on an international scale and then adapted to place-specific committees and ethics boards. However, this is complicated by the ever-evolving landscape of social media use and data agreements. The field of research ethics has yet to fully address the introduction of new media as sources of data; even before a comprehensive international framework is introduced, it may be advisable for institutions and regions to enact their own interim frameworks to mitigate possible harm and preserve user privacy and anonymity to the extent possible.
Limitations
This systematic review has a number of limitations. Owing to the iterative nature of data extraction for a large number of articles included, it is possible that there were differences in how data were coded as we refined our process. However, we attempted to minimize this concern through weekly research team meetings during the extraction process. Another limitation is that because we only examined articles originally published in English, we may be underestimating the number of articles that were conducting research in a specific geographic area other than the United States. The influence of this underestimation should be minimal; however, as most leading journals for health research are published in English [ 63 ]. One final limitation is that the literature review spanned from 2010 to 2019, so we are not capturing changes since then, which may have taken place in the approach to ethics or methodology in research using social media data since then. This is an evolving field of research; hence, we anticipate that standards and norms may have also evolved.
Comparison With Prior Work
Similar to Sinnenberg et al’s [ 4 ] review, this study examined whether ethics board approvals were sought when using social media data for public health research, finding equivalent proportions of articles that obtained IRB approval. Our study further explored whether there were other types of ethical considerations (eg, ethical discussion) present in the body of the articles. We also assessed the presence and use of identifiable information such as personal health information, verbatim Tweets, and user account metadata. In both this review and in that of Sinnenberg et al [ 4 ], many articles noted that the public nature of tweets allows researchers to observe the content. This presents a clear need for an ethical guideline framework for researchers using Twitter, especially when including identifying information.
Twitter data appear to be an increasingly important source of data in public health research. However, attention needs to be paid to sampling constraints, ethical considerations involved in using these data, and the specific methodologies to be used to ensure the rigorous conduct of this research.
Acknowledgments
We would like to thank Sarah Pickering, MPH, Jessie Losch, MPH, and Rebecca Berger, MPH, graduate students at the City University of New York (CUNY) School of Public Health, who contributed to refinement of the data extraction forms, data extraction, and quality assessments. This study was partially funded by an anonymous private foundation. The foundation did not play any role in implementation of the systematic review or manuscript preparation. The authors have no financial disclosures to report.
Abbreviations
Multimedia appendix 1, multimedia appendix 2.
Conflicts of Interest: None declared.
Sentiment analysis using Twitter data: a comparative application of lexicon- and machine-learning-based approach
- Original Article
- Open access
- Published: 09 February 2023
- Volume 13 , article number 31 , ( 2023 )
Cite this article
You have full access to this open access article
- Yuxing Qi 1 &
- Zahratu Shabrina 2 , 3
13k Accesses
13 Citations
1 Altmetric
Explore all metrics
Avoid common mistakes on your manuscript.
1 Introduction
Social media platform such as Twitter provides a space where users share their thoughts and opinion as well as connect, communicate, and contribute to certain topics using short, 140 characters posts, known as tweets . This can be done through texts, pictures, and videos, etc., and users can interact using likes, comments, and reposts buttons. According to Twitter ( https://investor.twitterinc.com ), the platform has more than 206 million daily active users in 2022, which is defined as the number of logged accounts that can be identified by the platform and where ads can be shown. As more people contribute to social media, the analysis of information available online can be used to reflect on the changes in people's perceptions, behavior, and psychology (Alamoodi et al. 2021 ). Hence, using Twitter data for sentiment analysis has become a popular trend. The growing interest in social media analysis has brought more attention to Natural Languages Processing (NLP) and Artificial Intelligence (AI) technologies related to text analysis.
Using text analysis, it is possible to determine the sentiments and attitudes of certain target groups. Much of the available literature focuses on texts in English but there is a growing interest in multilanguage analysis (Arun and Srinagesh 2020a ; Dashtipour et al. 2016 ; Lo et al. 2017 ). Text analysis can be done by extracting subjective comments toward a certain topic using different sentiments such as Positive, Negative, and Neutral (Arun and Srinagesh 2020b ). One of the topical interests would be related to the Coronavirus (Covid-19), which is a novel disease that was first discovered in late 2019. The rapid spread of Covid-19 worldwide has affected many countries, leading to changes in people’s lifestyles, such as wearing masks on public transportation and maintaining social distancing. Sentiment analysis can be implemented to social media data to explore changes in people’s behavior, emotions, and opinions such as by dividing the spread trend of Covid-19 into three stages and exploring people’s negative sentiments toward Covid-19 based on topic modeling and feature extraction (Boon-Itt and Skunkan 2020 ). Previous studies have retrieved tweets based on certain hashtags (#) used to categorize content based on certain topics such as “#stayathome” and “#socialdistancing” to measure their frequency (Saleh et al. 2021 ). Another study has used the Word2Vec technique and machine learning models, such as Naive Bayes, SVC, and Decision Tree, to explore the sentimental changes of students during the online learning process as various learning activities were moved online due to the pandemic (Mostafa 2021 ).
In this paper, we implement social media data analysis to explore sentiments toward Covid-19 in England. This paper aims to examine the sentiments of tweets using various methods including lexicon and machine learning approaches during the third lockdown period in England as a case study. Those who just started dealing with NLP should be able to use this paper to help select the appropriate method for their NLP analysis. Empirically, the case study also contributes to our understanding of the sentiments related to the UK national lockdown. In many countries, the implementation of policies and plans related to Covid-19 often sparked widespread discussion on Twitter. Tweet data can reflect the public sentiments on the Covid-19 pandemic, therefore providing an alternative source for guiding the government’s policies. The UK has experienced three national lockdowns since the outbreak of Covid-19, and people have expressed their opinions on Covid-19-related topics, such as social restrictions, vaccination plans, and school reopening, etc., all of which are worthy of exploring and analyzing. In addition, few existing studies focus on the UK or England, especially the change in people’s attitudes toward Covid-19 during the third lockdown.
2 Sentiment analysis approaches
In applying sentiment analysis, the key process is classifying extracted data into sentiment polarities such as positive, neutral, and negative classes. A wide range of emotions can also be considered which is the focus of the emerging fields of affective computing and sentiment analysis (Cambria 2016 ). There are various ways to separate sentiments according to different research topics, for example in political debates, sentiments can be divided further into satisfied and angry (D’Andrea et al. 2015 ). Sentiment analysis with ambivalence handling can be incorporated to account for a finer-grained results and characterize emotions into such detailed categories such as anxiety, sadness, anger, excitement, and happiness (Wang et al. 2015 , 2020 ).
Sentiment analysis is generally done to text data, although it can also be used to analyze data from devices that utilize audio- or audio-visual formats such as webcams to examine expression, body movement, or sounds known as multimodal sentiment analysis (Soleymani et al. 2017 ; Yang et al. 2022 ; Zhang et al. 2020 ). Multimodal sentiment analysis expands text-based analysis into something more complex that opens possibilities in the use of NLP for various purposes. Advancement of NLP is also rapidly growing driven by various research, for example in neural network (Kim 2014 ; Ray and Chakrabarti 2022 ). An example would be the implementation of Neurosymbolic AI that combines deep learning and symbolic reasoning, which is thought to be a promising method in NLP for understanding reasonings (Sarker et al. 2021 ). This indicates the wide possibilities of the direction of NLP research.
There are three main methods to detect and classify emotions expressed in text, which are lexicon-based, machine-learning-based approaches, and hybrid techniques. The lexicon-based approach uses the polarity of words, while the machine learning method sees texts as a classification problem and can be further divided into unsupervised, semi-supervised, and supervised learning (Aqlan et al. 2019 ). Figure 1 shows the classification of methods that can be used for sentiment analysis, and in practical applications, machine learning methods and lexicon-based methods could be used in combination.
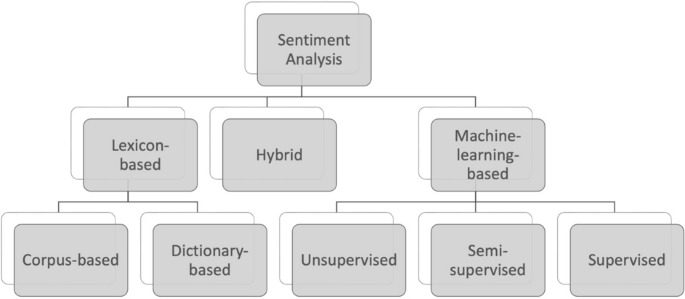
Sentiment analysis approaches
When dealing with large text data such as those from Twitter, it is important to do the data pre-processing before starting the analysis. This includes replacing upper-case letters, removing useless words or links, expanding contractions, removing non-alphabetical characters or symbols, removing stop words, and removing duplicate datasets. Beyond the basic data cleaning, there is a further cleaning process that should be implemented as well including tokenization, stemming, lemmatization, and Part of Speech (POS) tagging. Tokenization splits texts into smaller units and turns them into a list of tokens. This helps to make it convenient to calculate the frequency of each word in the text and analyze their sentiment polarity. Stemming and lemmatization replace words with their root word. For example, the word “feeling” and “felt” can be mapped to their stem word: “feel” using stemming. Lemmatization, on the other hand, uses the context of the words. This can reduce the dimensionality and complexity of a bag of words, which also improves the efficiency of searching the word in the lexicon when applying the lexicon-based method. POS Tagging can automatically tag the POS of words in the text, such as nouns, verbs, and adjectives, etc., which is useful for feature selection and extraction (Usop et al. 2017 ).
2.1 Lexicon-based approach
The core idea of the lexicon-based method is to (1) split the sentences into a bag of words, then (2) compare them with the words in the sentiment polarity lexicon and their related semantic relations, and (3) calculate the polarity score of the whole text. These methods can effectively determine whether the sentiment of the text is positive, negative, or neutral (Zahoor and Rohilla 2020 ). The lexicon-based approach performs the task of tagging words with semantic orientation either using dictionary-based or corpus-based approaches. The former is simpler, and we can determine the polarity score of words or phrases in the text using a sentiment dictionary with opinion words.
2.1.1 Lexicon-based approaches with built-in library
Examples of the most popular lexicon-based sentiment analysis models in Python are TextBlob and VADER. TextBlob is a Python library based on the Natural Language Toolkit (NLTK) that calculates the sentiment score for texts. An averaging technique is applied to each word to obtain the sentiment polarity scores for the entire text (Oyebode and Orji 2019 ). The words recorded in the TextBlob lexicon have their corresponding polarity score, subjectivity score, and intensity score. Additionally, there may be different records for the same word, so the sentiment score of the word is the average value of the polarity of all records containing them. The sentiment polarity scores produced are between [− 1, 1], in which − 1 refers to negative sentiment and + 1 refers to positive sentiment.
VADER (Valence Aware Dictionary and sEntiment Reasoner) is a lexicon and rule-based tool for sentiment analysis with a well-established sentiment lexicon (Hutto and Gilbert 2014 ). Compared to the TextBlob library, there are more corpora related to the language of social media, which may work better on a social media-type text that often contains non-formal language. From the results, the positive, negative, neutral, and compound values of tweets are presented, and the sentiment orientation is determined based on the compound score. There are several main steps of compound score calculation. Firstly, each word in the sentiment lexicon is given its corresponding scores of positive, negative, and neutral sentiments, ranging from − 4 to 4 from the most “negative” to the most “positive.” Heuristic rules are then applied when handling punctuation, capitalization, degree modifiers, contrastive conjunctions, and negations, which boosts the compound score of a sentence. The scores of all words in the text are standardized to (− 1, 1) using the formula below:
where x represents the sum of Valence scores of sentiment words, and α is a normalization constant. The compound score is obtained by calculating the scores of all standardized lexicons in the range of − 1 (most negative) to 1 (most positive). The specific classification criteria for both TextBlob and VADER are shown in Table 1 .
2.1.2 Lexicon-based approach with SentiWordNet
SentiWordNet is a lexical opinion resource that operates on the WordNet Database, which contains a set of lemmas with a synonymous interface called “synset” (Baccianella et al. 2010 ). Each synset corresponds to the positive and negative polarity scores. The value range of Pos(s) and Neg(s) is between 0 and 1. The process of SentiWordNet analysis is shown in Fig. 2 .
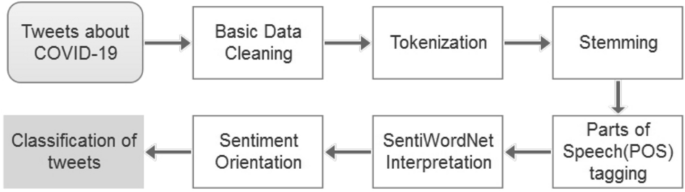
Process of SentiWordNet-based approaches
There are several steps in applying the SentiWordNet-based approach. The first steps are data pre-processing including applying basic data cleaning, tokenization, stemming, and POS tagging. These steps can improve the time spent searching the words in the SentiWordNet database. For a given lemma that contains n meanings in the tweet, only the polarity score with the most common meaning is considered (the first one). The formula is as follows:
We can count the positive and negative terms in each tweet and calculate their sentiment polarity scores (Guerini et al. 2013 ). The sentiment score of each word or specific term in the SentiWordNet lexicon can be calculated by applying Eq. ( 4 ):
The SynsetScore then computes the absolute value of the maximum positive score and the maximum negative score of the word. For a term containing several synsets, the calculation is as follows:
where n is a count number, the total score would be recorded as 0 if this term is not in SentiWordNet. The symbol k indicates how many synsets are contained in this term, and if there are negations in front of this term, then, this sentiment value is reserved. Finally, we can add the sentiment scores of all terms to get the sentiment score of the tweets using the formula below:
where p is a clean tweet with m positive terms and n negative terms. PosScore( p ) is the final score of all the positive terms, while NegScore( p ) represents the negative terms, and SentiScore( s ) is the final sentiment score of tweets (Bonta et al. 2019 ).
2.2 Machine learning approach
The machine learning approaches can construct classifiers to complete sentiment classification by extracting feature vectors, which mainly includes steps including data collecting and cleaning, extracting features, training data with the classifier, and analyzing results (Adwan et al. 2020 ). The dataset needs to be divided into a training and a test dataset using machine learning methods. The training sets aim to enable the classifier to learn the text features, and the test dataset evaluates the performance of the classifier.
The role of classifiers (e.g., Naïve Bayes classifier, Support Vector Machine, Logistic classifier, and Random Forest classifier.) is to classify text into different defined classes. As one of the most common methods for text classification, machine learning is widely used by researchers. In addition, the performance of the same classifier for different types of text may differ greatly, so the feature vectors of each type of text should be trained separately. To increase the robustness of the model, a two-stage support vector machine classifier can be used, which can effectively process the influence of noise data on classification (Barbosa and Feng 2010 ). In the subsequent process, it is necessary to vectorize the tweets data and divide the labeled tweets data into a training set (80%) and a test set (20%), and then, the sentiment labels can be predicted by training different classification models. The overall process is shown in Fig. 3 below:
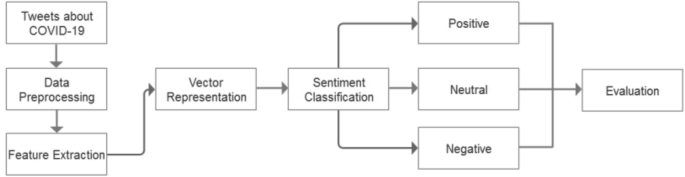
Main process of machine-learning-based approaches
2.2.1 Feature representation
The common methods of text feature representation can be divided into two categories: frequency-based embeddings (e.g., Count vector, Hashing Vectorizer, and TF–IDF) and pre-trained word embedding (e.g., Word2Vec, Glove, and Bert) (Naseem et al. 2021 ). In this paper, the following three feature representation models are mainly used:
Bag of words ( BoW ) converts textual data to numerical data with a fixed-length vector by counting the frequency of each word in tweets. In Python, CountVectorizer() works on calculating terms frequency, in which a sparse matrix of clean tokens is built.
Term frequency–inverse document frequency ( TF–IDF ) measures the relevance between a word and the entire text and evaluates the importance of the word in the tweet dataset. In Python, TfidfVectorizer() can obtain a TF–IDF matrix by calculating the product of the word frequency metric and inverse document frequency metric of each word from clean tweets.
Word2Vec generates a vector space according to all tweet corpus, and each word is represented in the form of a vector in this space. In the vector space, words with similar meanings will be closer together, so this method is more effective for dealing with semantic relations. In Python, the text embedding method can be implemented with the Word2Vec model in the Gensim library, and many different hyperparameters can be adjusted to optimize the word embedding model, such as setting various corpus (sentences), trying different training algorithms (skip-grams/sg), and adjusting the maximum distance between the current word and the predicted word in a sentence (window).
2.2.2 Classification models
Sentiment classification is the process of predicting users’ tweets as positive, negative, and neutral based on the feature representation of tweets. The classifiers in the supervised machine learning methods, such as a random forest, can classify and predict unlabeled text by training a large number of sentiment-labeled tweets. The classification models used in this paper are as follows:
2.2.2.1 Random forest
The results of the random forest algorithm are based on the prediction results of multiple decision trees, and the classification of new data points is determined by a voting mechanism (Breiman 2001 ). Increasing the number of trees can increase the accuracy of the results. There are several steps in applying random forest for text processing (Kamble and Itkikar 2018 ). First, we select n random tweet records from the dataset as the sample dataset and build a decision tree for each sample. We then get the predicted classification results of each decision tree. Then, we take the majority vote for each prediction of the decision trees. The sentiment orientation will be assigned to the category with the most votes. To evaluate the results, we can split the dataset into a training part to build the forest and a test part to calculate the error rate (al Amrani et al. 2018 ).
2.2.2.2 Multinomial Naïve Bayes
This model is based on the Naïve Bayes Theorem, which calculates the probability of multiple categories from many observations, and the category with the maximum probability is assigned to the text. Hence, the model can effectively solve the problem of text classification with multiple classes. The formula using Bayes Theorem to predict the category label based on text features (Kamble and Itkikar 2018 ) is as follows:
where p (label) represents the prior probability of label p , and (feature/label) is the prior probability of the features with a given classifying label. To implement this technique, firstly, we calculate the prior probability for known category labels. Then, we obtain the likelihood probability with each feature for different categories and calculate the posterior probability with the formulas of the Bayes theorem. Lastly, we select the category with the highest probability as the label of the input tweet.
2.2.2.3 Support vector classification (SVC)
The purpose of this model is to determine linear separators in the vector space and facilitate the separation of different categories of input vector data. After the hyperplane is obtained, the extracted text features can be put into the classifier to predict the results. Additionally, the core idea is to find a line closest to the support vectors. The steps in implementing SVC include calculating the distance between the nearest support vectors, which is also called the margin, maximizing the margin to obtain an optimal hyperplane between support vectors from given data, and using this hyperplane as a decision boundary to segregate the support vectors.
2.2.3 Hyperparameters optimization
Hyperparameters can be considered as the settings of machine learning models, and they need to be tuned for ensuring better performance of models. There are many approaches to hyperparameter tuning, including Grid Search, Random Search, and automated hyperparameter optimization. In this study, Grid Search and Random Search are considered. The result may not be the global optimal solution of a classification model, but it is the optimal hyperparameters within the range of these grid values.
In applying Grid Search, we build a hyperparameter values grid, train a model with each combination of hyperparameter values, and evaluate every position of the grid. For Random Search, we build a grid of hyperparameter values and then, train a model with combinations randomly selected, which means not all the values can be tried. For this paper, this latter approach is more feasible because although the results of the Grid Search optimization method might be more accurate, it is inefficient and costs more time when compared with the random search approach.
3 Data and methods
This paper focuses on tweets that were geotagged from the main UK cities during the third national Covid-19 lockdown. The cities are Greater London, Bristol, South Hampton, Birmingham, Manchester, Liverpool, Newcastle, Leeds, Sheffield, and Nottingham. Since the total number of tweets in each city is positively correlated with the urban population size and density, the number of tweets varies widely among these different cities. To collect more tweets to represent the perception of most people in England toward the Covid-19 pandemic, the selection criteria for major cities are based on the total population and density to improve the validity of the data (Jiang et al. 2016 ).
We divide the data collection time frame into three different stages of the third national lockdown in 2021. The timeline of the third national lockdown in England is from 6 January 2021 to 18 July 2021 as can be seen in Fig. 4 . During this period, we selected several critical time points for research and analysis in stages according to the plan of lifting the lockdown in England, and the duration of each stage is about two months. The different stages are Stage 1 on January 6 until March 7, 2021, when England enters the third national lockdown, Stage 2 on March 8 until May 16, 2021, when the government implemented steps 1 and step 2 of lifting the lockdowns and Stage 3 on May 17 until July 18, 2021, when the government implemented step 3 of lifting the lockdown and easing most Covid-19 restrictions in the UK.
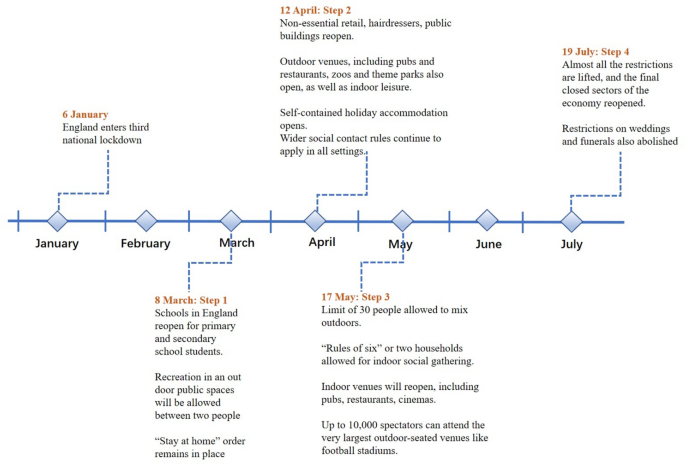
Detailed timeline of the third national lockdown in 2021
The tweets are extracted using Twint and Twitter Academic API, as these scraping tools can help facilitate the collection of tweets with geo-location, which helps in applying geographical analysis. However, users who are willing to disclose their geographic location when sending tweets only account for 1% of the total users (Sloan and Morgan 2015 ), and the location-sharing option is off by default. Therefore, the data collected by Twint and Twitter academic API are merged to obtain more tweets.
To filter the tweets related to Covid-19, we used keywords including “corona” or “covid” in the searching configuration of Twint or the query field of Twitter academic API, thus extracting the tweets and hashtags containing the search terms. In Twint, 1000 tweets can be fetched in each city per day, which avoids large bias in sentiment analysis due to uneven data distribution, but, in most cases, the number of tweets from a city for one day cannot reach this upper limit. Moreover, cities in the major cities list are used as a condition for filtering tweets from different geographic regions.
A total of 77,332 unique tweets were collected in three stages crawled from January 6 to July 18, 2021 (stage 1: 29,923; stage 2: 24,689; and stage 3: 22,720 tweets). The distribution of the number of tweets in each city is shown in Fig. 5 a. Most of the tweets originate from London, Manchester, Birmingham, and Liverpool, and there are far more tweets in London (37,678) than in other cities. The number of tweets obtained in some cities, such as Newcastle, is much lower than the number of tweets in London, with only 852 tweets collected in six months. Figure 5 shows the distribution of data at each stage with the first stage having the most data while the third stage has the least amount of data. Additionally, at each stage, London has the largest proportion of data, with Newcastle having the least, linear to the total population and density of the area.
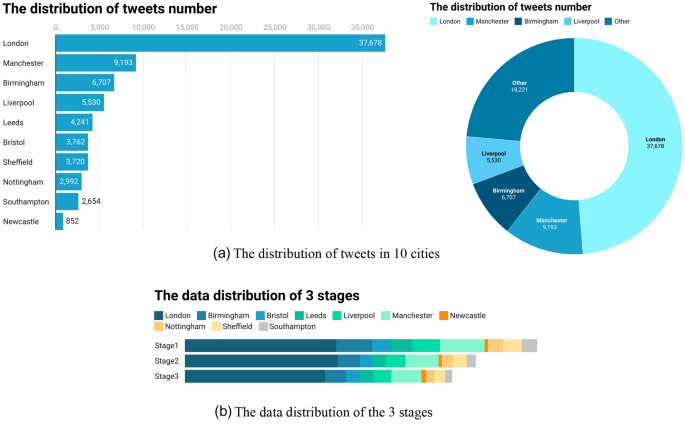
Distribution of collected tweets based on the selected cities and different stages
Since most raw tweets are unstructured and informal, which may affect the word polarity or text feature extraction, the data were pre-processed before sentiment analysis (Naseem et al. 2021 ). We implemented a basic data-cleaning process as follows:
Replacing upper-case letters to avoid recognizing the same word as different words because of capitalization.
Removing hashtags (#topic), mentioned usernames (@username), and all the links that start with “www,” “http,” and “https.” Removing stop words and short words (less than two characters). The stop words are mostly very common in the text but hardly contain any sentiment polarity. However, in sentiment analysis, “not” and “no” should not be listed as stop words, because removing these negations would change the real meaning of entire sentences.
Reducing repeated characters from some words. Some users will type repeated characters to express their strong emotions, so these words that are not in the lexicons should be converted into their corresponding correct words. For example: “sooooo goooood” becomes “so good.”
Expanding contractions in tweets such as “isn't” or “don't” as these will become meaningless letters or words after punctuations have been removed. Therefore, all contractions in the tweets are expanded into their formal forms, such as “isn’t” become “is not.”
Clearing all non-alphabetical characters or symbols including punctuation, numbers, and other special symbols that may affect the feature extraction of the text.
Removing duplicated or empty tweets and creating a clean dataset.
Converting emojis to their real meaning as many Twitter users use emojis in their tweets to express their sentiments and emotions. Hence, using the demojize() function in the emoji module of Python and transforming emojis into their true meaning may improve the accuracy of the sentiment analysis (Tao and Fang 2020 ).
In addition, for some sentiment analysis approaches, such as SentiWordNet-based analysis, further cleaning is essential, including stemming and POS Tagging.
In this study, strategies for text cleaning, polarity calculation, and sentiment classification model are designed and optimized using two different approaches to sentiment analysis: lexicon and machine-learning-based techniques. We then compared the results of the different methods and compare their output and prediction accuracy. The machine-learning-based approaches require labels with the tweets data, but the constraint is that it often takes too much time to manually annotate a large amount of data. Hence, 3000 tweets are randomly sampled in this paper, with the average number of tweets in each sentiment category of about 1000. To save the time spent on labeling, the classification results of the TextBlob or VADER method are used as the labels of the sample data (Naseem et al. 2021 ). We then manually check whether the classification of the VADER or TextBlob method is correct and modify it when necessary.
4 Results and discussion
4.1 lexicon-based approach.
From Fig. 6 , the results obtained by TextBlob and VADER tools are similar, showing that positive sentiments appear more than negative sentiments. However, the number of neutral sentiments from the VADER method is lower. This might be because the VADER lexicon can efficiently handle the type of language used by social media users such as by considering the use of slang, Internet buzzwords, and abbreviations. On the other hand, TextBlob works better with formal language usage. Moreover, the results from the analysis using the SentiWordNet show a high proportion of negative sentiments. This might be due to some of the social media expressions of positive emotions that are not comprehensively recorded in the dictionary. Additionally, due to its limited coverage of domain-specific words, some words may be assigned wrong scores, which would cause a large deviation in sentiment scores. Only the most common meaning of each word is considered in SentiWordNet-based calculation; therefore, some large bias might occur. Consequently, the results of the VADER method are more convincing in this experiment. According to the comparison of public sentiment toward “Covid-19” and the “Covid-19 vaccine,” the classification results of all three approaches show that more people have positive sentiments than negative, indicating that most people expect the vaccine to have a good impact on Covid-19.
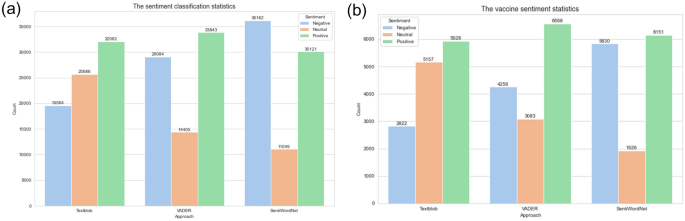
a Sentiment classification statistics, b vaccine sentiment statistics
After using the lexicon-based approaches with TextBlob, VADER, and SentiWordNet-based methods, the sentiment scores and their classification results were obtained for each tweet. In this study, the three sentiment categories of positive, negative, and neutral sentiment correspond to 1, − 1, and 0, respectively, and we filter out the tweets in each city with their corresponding sentiment values (positive: 1, negative: − 1; and neutral: 0). The proportion of positive and negative sentiments in each city at each stage was calculated to compare how the sentiments change and to examine the differences in people’s perception of Covid-19 between these different cities.
Figure 7 a indicates the results of using TextBlob in the three stages. In most cities in Fig. 7 a, the proportion of positive sentiments at each stage is between 38 and 50%. Southampton and Manchester show a steady decline, while Sheffield is the only city where the proportion of positive sentiments increased in all three stages. Considering the entire period, Newcastle has the largest proportion of positive emotions, peaking at the second stage (about 50%), and Southampton was the lowest. For negative sentiments, the trend of Sheffield was different from other cities, which rise first and then fall. In addition, for most cities, the proportion of negative sentiments in the second stage is the lowest, and the proportion of negative sentiments in most cities is between 20 and 30%.
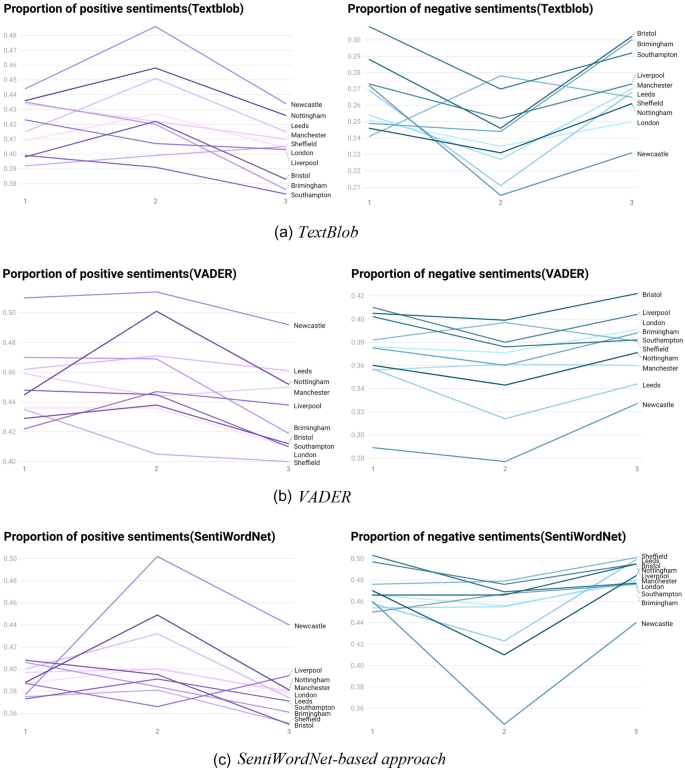
Results of the various lexicon-based approaches
The results of VADER shown in Fig. 7 b are similar to those of TextBlob. The proportion of positive sentiment in most cities is 40–50%, showing a trend of increasing first and then falling, except for Sheffield. Additionally, most of the negative sentiments account for between 30 and 40%. Moreover, the changes in the proportion of positive emotions in Manchester and Leeds are relatively flat, and the proportion of negative sentiments in Manchester also changes smoothly. However, Nottingham has a large change in positive sentiments at each stage, with a difference of about 6% between the highest and lowest values, and Newcastle has a wide range of negative sentiments proportion.
Based on the results of the SentiWordNet-based approach shown in Fig. 7 c, the proportion of negative sentiments in each city is higher when compared with the previous two methods. Most of the negative sentiments are in the range of 40–50%, while the proportion of positive emotions is mostly between 36 and 46%. In terms of the trend of change, the percentage of Birmingham’s positive sentiment is declining, while the percentage of Liverpool’s positive sentiments trend is the opposite of other cities, which decreased first and then, increased.
Overall, according to the results of the three approaches, for most cities, the proportion of positive sentiments first rises and then, decreases. This is in contrast with the proportion of negative sentiments that decline from the first stage to the second stage and then, start to increase. The number of Covid-19 deaths and confirmed cases could be an indicator that can quantify the severity of the pandemic. Meanwhile, the increase in the number of people vaccinated with the Covid-19 vaccine can reduce the speed of the virus spreading among the population, thereby reducing the impact of the pandemic on people’s lives.
Figure 8 shows the changes in the number of deaths and confirmed cases, and the number of new vaccines given. It shows that after peaking at the beginning of the third national lockdown, the number of deaths began to decline and became stable after April 2021. In addition, the number of newly confirmed cases in 2021 shows a downward trend from January to May but has increased significantly since June. Moreover, from the perspective of vaccination, the peak period of vaccination in 2021 is mainly in April and May, while after June, the vaccination volume drops greatly. Furthermore, combined with the previous results of sentiment analysis, from the first stage to the second stage, the positive sentiment proportion increases in most cities. This might be related to the improved situation of the Covid-19 pandemic as well as the increased number of vaccinations. However, there is a drop in positive sentiments from stage two to stage three, and the negative proportion increases. This might be due to the overall sentiment toward the vaccine’s protection rate and a large amount of new confirmed cases at the time. Overall, it might be that the public feels that the third lockdown policy and vaccination have not achieved the expected effect on the control of Covid-19 in England; hence, the number of negative sentiments has an upward trend after the second stage. More analysis is needed to explain the change in the sentiment trends more accurately.
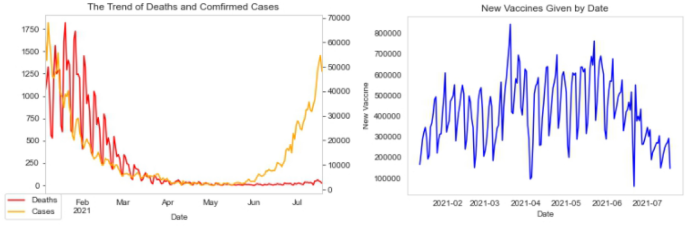
Trend of deaths, confirmed cases, and vaccines
4.2 Machine-learning-based approach
In this paper, supervised learning approaches also need to be considered because unsupervised lexicon-based approaches cannot quantitatively analyze the results of sentiment classification. This part shows the classification performance of the three models (the proportion of the train dataset compared with the test dataset is 8:2) under different feature representation models (BoW, TF–IDF, and Word2Vec) and the optimization training on the models.
4.2.1 The hyperparameters of classification models
Each classification model needs to extract the text features of tweets and vectorize them before training, and the feature vectors of different forms may show different performances in the same classification model. Therefore, before the training of feature vectors, RandomizedSearchCV() is used to optimize the hyperparameters in the classifier. In the optimization process, the hyperparameters that are expected to be optimized can be selected with various options, and the result would be the optimal solution for the hyperparameters grid. Table 2 (a) presents the optimal parameters of the random forest classifier, and Table 2 (b) shows the optimal hyperparameters of the Multinomial Naive Bayes (MNB) classifier and the Support Vector Machine (SVC) classifier.
4.2.2 The evaluation results of classifiers
These models classify all tweets into three categories, which are negative, positive, and neutral. The following Table 3 shows their performance with different feature representations.
In this paper, Accuracy, Precision, and Recall are selected as evaluation indicators, measuring the performance of each classification model. Before calculating them, the values of the confusion matrix need to be known, and they are TP (True Positive), TN (True Negative), FP (False Positive), and FN (False Negative). Accuracy shows the proportion of the number of correct observations to the total observations using the formula below:
Precision is the proportion of positive observations that correctly estimates the total number of positive predictions using the formula:
Recall refers to the proportion of actual positive observations that are identified correctly calculated using:
The F1 Score is a comprehensive evaluation and balance of precision and recall values, which can be calculated as follows:
According to the classification results of the three models, the performance of these classifiers for tweets with negative labels is poor, especially for the Random Forest Classifier, which has a low ability to recognize negative tweets, though the prediction precision is high. The reason for this may be that the labels are annotated manually, and unsupervised learning methods are different from the real sentiment expression of tweets. For the overall prediction, the SVC model has the best prediction ability with an accuracy of 0.71. Additionally, the F1 values of each label show that the SVC model has a good ability to classify the three categories of sentiments.
The accuracy of the three models is relatively high with the TF–IDF method, all above 60%. However, similar to the experimental results using the BoW feature representation, in Random Forest Classifier, the recall value of the negative category is very low, indicating that there are many negative tweets in the test dataset that have not been identified. This may be caused by the imbalanced distribution of data in each category, or the category contains some wrong data that would affect the training results. Moreover, these three models have the best predictive effect on the positive category, with an F1 score above 0.7. In summary, the performance of the SVC model is the best and the accuracy is higher than 70% in our study.
The prediction results of the three classifiers with Word2Vec are not as good as the previous two feature representation models, especially for the identification of negative sentiments. The reasons for the poor performance are that the Word2Vec embedding method needs to group semantically similar words, which requires a large amount of data, and it is difficult to extract sufficient text feature vectors from a small dataset. Moreover, compared with the Multinomial Naïve Bayes classifier, the SVC model and Random Forest classifier have better prediction performance, and their values of accuracy are 0.56 and 0.53, respectively.
5 Conclusion
In conclusion, this paper extracts data regarding Covid-19 from people in the main cities of England on Twitter and separates it into three different stages. First, we perform data cleaning and use unsupervised lexicon-based approaches to classify the sentiment orientations of the tweets at each stage. Then, we apply the supervised machine learning approaches using a sample of annotated data to train the Random Forest classifier, Multinomial Naïve Bayes classifier, and SVC, respectively. From lexicon-based approaches, the three stages of public sentiment changes about the Covid-19 pandemic can be found. For most cities, the proportion of positive sentiments increases first and then drops, while the proportion of negative sentiments changed in a different direction. In addition, by analyzing the number of deaths and confirmed cases as well as vaccination situations, it could be concluded that the increase in confirmed cases and the decrease in vaccination volume might be the reason for the increase in negative sentiments, even though further research is needed to confirm this inference.
For supervised machine learning classifiers, the Random Search method is applied to optimize the hyperparameters of each model. The SVC results using BoW and TF–IDF feature models have the best performance, and their classification accuracy is as high as 71%. Due to the insufficiency of training data, the prediction accuracy of classifiers with the Word2Vec embedding method is low. Consequently, applying machine learning approaches to sentiment analysis can accurately extract text features without being restricted by lexicons.
It is important to note that this paper only collects the opinions of people in England on Twitter about Covid-19; thus, the result should be interpreted by considering this limitation. To obtain a more convincing conclusion, we can increase the data size by incorporating longer timeline, wider geographies, or by collecting data via other social media platforms while also considering the data protection policy. In addition, large-scale manually annotated datasets can be created for training machine learning models to improve their classification ability. Moreover, deep learning approaches can be used for model training, and this can be compared with different machine learning models. Furthermore, the Random Search method can only find the optimal parameters within a certain range, so exploring how to select model hyperparameters efficiently can further improve the stability of machine learning models. However, despite all the limitations, this study has provided contributions in advancing our understanding of the use of various NLP methods.
For lexicon-based approaches, the existing lexicon is modified to better fit the language habits of modern social media, improving the accuracy of this approach. Additionally, an annotated dataset can be created to compare the difference between predicted results and real results. Research on Covid-19 can be based on time series so that the changes in people’s attitudes and perceptions can be analyzed over some time. Moreover, further studies can combine the sentiment classification results with other factors such as deaths and vaccination rates and establish a regression model to analyze which factors contribute to the sentiment changes. Overall, the paper has showcased different methods of conducting sentiment analysis with SVC using BoW or TF–IDF outperformed the model accuracy overall.
6 The codes of the project
The main codes of this project have uploaded to GitHub, and here is the link: https://github.com/Yuxing-Qi/Sentiment-analysis-using-Twitter-data .
Adwan OY, Al-Tawil M, Huneiti AM, Shahin RA, Abu Zayed AA, Al-Dibsi RH (2020) Twitter sentiment analysis approaches: a survey. Int J Emerg Technol Learn. https://doi.org/10.3991/ijet.v15i15.14467
Article Google Scholar
al Amrani Y, Lazaar M, el Kadirp KE (2018) Random forest and support vector machine based hybrid approach to sentiment analysis. Proc Comput Sci. https://doi.org/10.1016/j.procs.2018.01.150
Alamoodi AH, Zaidan BB, Zaidan AA, Albahri OS, Mohammed KI, Malik RQ, Almahdi EM, Chyad MA, Tareq Z, Albahri AS, Hameed H, Alaa M (2021) Sentiment analysis and its applications in fighting COVID-19 and infectious diseases: a systematic review. Expert Syst Appl. https://doi.org/10.1016/j.eswa.2020.114155
Aqlan AAQ, Manjula B, Lakshman Naik R (2019) A study of sentiment analysis: Concepts, techniques, and challenges. In Lecture notes on data engineering and communications technologies, vol 28. https://doi.org/10.1007/978-981-13-6459-4_16
Arun K, Srinagesh A (2020a) Multi-lingual Twitter sentiment analysis using machine learning. Int J Electr Comput Eng. https://doi.org/10.11591/ijece.v10i6.pp5992-6000
Arun K, Srinagesh A (2020b) Multi-lingual Twitter sentiment analysis using machine learning. Int J Electr Comput Eng. https://doi.org/10.11591/ijece.v10i6.pp5992-6000
Baccianella S, Esuli A, Sebastiani F (2010) SENTIWORDNET 3.0: an enhanced lexical resource for sentiment analysis and opinion mining. In: Proceedings of the 7th international conference on language resources and evaluation, LREC 2010
Barbosa L, Feng J (2010) Robust sentiment detection on twitter from biased and noisy data. In: Coling 2010—23rd international conference on computational linguistics, proceedings of the conference, 2
Bonta V, Kumaresh N, Janardhan N (2019) A comprehensive study on Lexicon based approaches for sentiment analysis. Asian J Comput Sci Technol 8(S2):1–6. https://doi.org/10.51983/ajcst-2019.8.s2.2037
Boon-Itt S, Skunkan Y (2020) Public perception of the COVID-19 pandemic on Twitter: sentiment analysis and topic modeling study. JMIR Public Health and Surveillance, 6(4), e21978. https://doi.org/10.2196/21978
Breiman L (2001) Random forests. Mach Learn. https://doi.org/10.1023/A:1010933404324
Cambria E (2016) Affective computing and sentiment analysis. IEEE Intell Syst. https://doi.org/10.1109/MIS.2016.31
D’Andrea A, Ferri F, Grifoni P, Guzzo T (2015) Approaches, tools and applications for sentiment analysis implementation. Int J Comput Appl. https://doi.org/10.5120/ijca2015905866
Dashtipour K, Poria S, Hussain A, Cambria E, Hawalah AYA, Gelbukh A, Zhou Q (2016) Multilingual sentiment analysis: state of the art and independent comparison of techniques. Cogn Comput. https://doi.org/10.1007/s12559-016-9415-7
Guerini M, Gatti L, Turchi M (2013) Sentiment analysis: how to derive prior polarities from SentiWordNet. In: EMNLP 2013—2013 conference on empirical methods in natural language processing, proceedings of the conference
Hutto CJ, Gilbert E (2014) VADER: a parsimonious rule-based model for sentiment analysis of social media text. In: Proceedings of the 8th international conference on weblogs and social media, ICWSM 2014. https://doi.org/10.1609/icwsm.v8i1.14550
Jiang B, Ma D, Yin J, Sandberg M (2016) Spatial distribution of city Tweets and their densities. Geogr Anal. https://doi.org/10.1111/gean.12096
Kamble SS, Itkikar PAR (2018) Study of supervised machine learning approaches for sentiment analysis. Int Res J Eng Technol (IRJET) 05(04)
Kim Y (2014) Convolutional neural networks for sentence classification. In: EMNLP 2014—2014 conference on empirical methods in natural language processing, proceedings of the conference. https://doi.org/10.3115/v1/d14-1181
Lo SL, Cambria E, Chiong R, Cornforth D (2017) Multilingual sentiment analysis: from formal to informal and scarce resource languages. Artif Intell Rev. https://doi.org/10.1007/s10462-016-9508-4
Mostafa L (2021) Egyptian student sentiment analysis using Word2vec during the coronavirus (Covid-19) pandemic. In Proceedings of the International Conference on Advanced Intelligent Systems and Informatics 2020 (pp. 195-203). Springer International Publishing. https://doi.org/10.1007/978-3-030-58669-0_18
Naseem U, Razzak I, Khushi M, Eklund PW, Kim J (2021) COVIDSenti: a large-scale benchmark Twitter data Set for COVID-19 sentiment analysis. IEEE Trans Comput Soc Syst. https://doi.org/10.1109/TCSS.2021.3051189
Oyebode O, Orji R (2019) Social media and sentiment analysis: the Nigeria presidential election 2019. In: 2019 IEEE 10th annual information technology, electronics and mobile communication conference, IEMCON 2019. https://doi.org/10.1109/IEMCON.2019.8936139
Ray P, Chakrabarti A (2022) A mixed approach of deep learning method and rule-based method to improve aspect level sentiment analysis. Appl Comput Inform. https://doi.org/10.1016/j.aci.2019.02.002
Saleh SN, Lehmann CU, McDonald SA, Basit MA, Medford RJ (2021) Understanding public perception of coronavirus disease 2019 (COVID-19) social distancing on Twitter. Infect Control Hosp Epidemiol 42(2):131–138. https://doi.org/10.1017/ice.2020.406
Sarker MK, Zhou L, Eberhart A, Hitzler P (2021) Neuro-symbolic artificial intelligence. AI Commun. https://doi.org/10.3233/AIC-210084
Article MathSciNet Google Scholar
Sloan L, Morgan J (2015) Who tweets with their location? Understanding the relationship between demographic characteristics and the use of geoservices and geotagging on twitter. PLoS ONE. https://doi.org/10.1371/journal.pone.0142209
Soleymani M, Garcia D, Jou B, Schuller B, Chang SF, Pantic M (2017) A survey of multimodal sentiment analysis. Image vis Comput. https://doi.org/10.1016/j.imavis.2017.08.003
Tao J, Fang X (2020) Toward multi-label sentiment analysis: a transfer learning based approach. J Big Data. https://doi.org/10.1186/s40537-019-0278-0
Usop ES, Isnanto RR, Kusumaningrum R (2017) Part of speech features for sentiment classification based on Latent Dirichlet allocation. In: Proceedings—2017 4th international conference on information technology, computer, and electrical engineering, ICITACEE 2017, 2018-January. https://doi.org/10.1109/ICITACEE.2017.8257670
Wang Z, Ho SB, Cambria E (2020) Multi-level fine-scaled sentiment sensing with ambivalence handling. Int J Uncertain Fuzziness Knowl-Based Syst. https://doi.org/10.1142/S0218488520500294
Wang Z, Joo V, Tong C, Chan D (2015) Issues of social data analytics with a new method for sentiment analysis of social media data. In: Proceedings of the international conference on cloud computing technology and science, CloudCom, 2015-February(February). https://doi.org/10.1109/CloudCom.2014.40
Yang B, Shao B, Wu L, Lin X (2022) Multimodal sentiment analysis with unidirectional modality translation. Neurocomputing. https://doi.org/10.1016/j.neucom.2021.09.041
Zahoor S, Rohilla R (2020) Twitter sentiment analysis using lexical or rule based approach: a case study. In: ICRITO 2020—IEEE 8th international conference on reliability, Infocom technologies and optimization (trends and future directions). https://doi.org/10.1109/ICRITO48877.2020.9197910
Zhang Y, Rong L, Song D, Zhang P (2020) A survey on multimodal sentiment analysis. In Moshi Shibie yu Rengong Zhineng/pattern recognition and artificial intelligence, vol 33, issue 5. https://doi.org/10.16451/j.cnki.issn1003-6059.202005005
Download references
Author information
Authors and affiliations.
Centre for Urban Science and Progress, King’s College London, London, UK
Department of Geography, King’s College, London, UK
Zahratu Shabrina
Regional Innovation, Graduate School, Universitas Padjadjaran, Bandung, Indonesia
You can also search for this author in PubMed Google Scholar
Contributions
Z.S. and Y.Q. conceived the presented idea. Y.Q. conducted the data gathering, analysis, and drafted the main manuscript. Z.S. wrote and edited the final version of the manuscript and supervised the project. All authors provided critical feedback and helped shape the research, analysis, and manuscript.
Corresponding author
Correspondence to Zahratu Shabrina .
Ethics declarations
Competing interests.
The authors declare no competing interests.
Additional information
Publisher's note.
Springer Nature remains neutral with regard to jurisdictional claims in published maps and institutional affiliations.
Rights and permissions
Open Access This article is licensed under a Creative Commons Attribution 4.0 International License, which permits use, sharing, adaptation, distribution and reproduction in any medium or format, as long as you give appropriate credit to the original author(s) and the source, provide a link to the Creative Commons licence, and indicate if changes were made. The images or other third party material in this article are included in the article's Creative Commons licence, unless indicated otherwise in a credit line to the material. If material is not included in the article's Creative Commons licence and your intended use is not permitted by statutory regulation or exceeds the permitted use, you will need to obtain permission directly from the copyright holder. To view a copy of this licence, visit http://creativecommons.org/licenses/by/4.0/ .
Reprints and permissions
About this article
Qi, Y., Shabrina, Z. Sentiment analysis using Twitter data: a comparative application of lexicon- and machine-learning-based approach. Soc. Netw. Anal. Min. 13 , 31 (2023). https://doi.org/10.1007/s13278-023-01030-x
Download citation
Received : 01 June 2022
Revised : 16 January 2023
Accepted : 20 January 2023
Published : 09 February 2023
DOI : https://doi.org/10.1007/s13278-023-01030-x
Share this article
Anyone you share the following link with will be able to read this content:
Sorry, a shareable link is not currently available for this article.
Provided by the Springer Nature SharedIt content-sharing initiative
- Find a journal
- Publish with us
- Track your research
Click through the PLOS taxonomy to find articles in your field.
For more information about PLOS Subject Areas, click here .
Loading metrics
Open Access
Peer-reviewed
Research Article
Academic information on Twitter: A user survey
Roles Conceptualization, Data curation, Formal analysis, Investigation, Methodology, Project administration, Resources, Software, Validation, Visualization, Writing – original draft, Writing – review & editing
* E-mail: [email protected]
Affiliations School of Library and Information Science, University of South Carolina, Columbia, South Carolina, United States of America, Department of Preventive Medicine, Northwestern University Feinberg School of Medicine, Chicago, Illinois, United States of America

Roles Conceptualization, Data curation, Methodology, Software, Writing – review & editing
Affiliation Statistical Cybermetrics Research Group, School of Mathematics and Computer Science, University of Wolverhampton, Wolverhampton, United Kingdom
Roles Formal analysis, Writing – review & editing
Affiliation Department of Preventive Medicine, Northwestern University Feinberg School of Medicine, Chicago, Illinois, United States of America
Roles Conceptualization, Funding acquisition, Investigation, Project administration, Resources, Supervision, Writing – original draft, Writing – review & editing
- Ehsan Mohammadi,
- Mike Thelwall,
- Mary Kwasny,
- Kristi L. Holmes
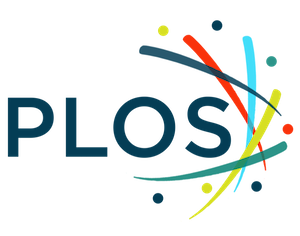
- Published: May 17, 2018
- https://doi.org/10.1371/journal.pone.0197265
- Reader Comments
Although counts of tweets citing academic papers are used as an informal indicator of interest, little is known about who tweets academic papers and who uses Twitter to find scholarly information. Without knowing this, it is difficult to draw useful conclusions from a publication being frequently tweeted. This study surveyed 1,912 users that have tweeted journal articles to ask about their scholarly-related Twitter uses. Almost half of the respondents (45%) did not work in academia, despite the sample probably being biased towards academics. Twitter was used most by people with a social science or humanities background. People tend to leverage social ties on Twitter to find information rather than searching for relevant tweets. Twitter is used in academia to acquire and share real-time information and to develop connections with others. Motivations for using Twitter vary by discipline, occupation, and employment sector, but not much by gender. These factors also influence the sharing of different types of academic information. This study provides evidence that Twitter plays a significant role in the discovery of scholarly information and cross-disciplinary knowledge spreading. Most importantly, the large numbers of non-academic users support the claims of those using tweet counts as evidence for the non-academic impacts of scholarly research.
Citation: Mohammadi E, Thelwall M, Kwasny M, Holmes KL (2018) Academic information on Twitter: A user survey. PLoS ONE 13(5): e0197265. https://doi.org/10.1371/journal.pone.0197265
Editor: Sergi Lozano, Institut Català de Paleoecologia Humana i Evolució Social (IPHES), SPAIN
Received: September 29, 2017; Accepted: April 30, 2018; Published: May 17, 2018
Copyright: © 2018 Mohammadi et al. This is an open access article distributed under the terms of the Creative Commons Attribution License , which permits unrestricted use, distribution, and reproduction in any medium, provided the original author and source are credited.
Data Availability: Data cannot be made publicly available due to the respect the privacy of participants and as Northwestern University IRB committee policy requires that the data should only be shared with qualified researchers. Therefore, the data can be shared with qualified scholars upon request. Data access requests can be sent to [email protected] or [email protected] .
Funding: This work was supported by the National Institutes of Health's National Center for Advancing Translational Sciences in grant UL1TR001422 awarded to Northwestern University. The funder had no role in study design, data collection and analysis, decision to publish, or preparation of the manuscript.
Competing interests: The authors have declared that no competing interests exist.
Introduction
Scholars use a range of different types of social web platforms in their professional activities. Twitter is one of the most popular microblogging platforms in many countries, allowing users to broadcast short messages. Academics use Twitter to communicate scientific messages at real-time events, like conferences, but also for more routine information sharing [ 1 ]. Since Twitter is used for academic purposes and counts of tweets from Altmetric.com and elsewhere seem to be increasingly consulted for research monitoring [ 2 ],[ 3 ],[ 4 ], it is important to understand the typical motivations for using Twitter in scholarly communication. In response, previous studies have surveyed a small snowball sample of 28 Twitter-active academics [ 5 ] and 613 academics with a Twitter account in Physics, Biology, Chemistry, Computer Science, Philosophy, English, Sociology, and Anthropology at 68 U.S. universities [ 6 ]. Since tweet counts may be useful as evidence of the impact of research outside of academia (i.e., the extent to which non-academics find a study to be useful), a broader perspective is needed, including non-academic users. In response, this paper reports the first survey of a large and diverse sample of Twitter users inside and outside academia and from around the world to discover who tweets academic publications and why. This contextual information is important to help interpret the meaning of tweet counts as a scholarly impact indicator. Users were selected on the basis that they had tweeted at least one journal article.
The main previous evidence for the value of Tweet counts as impact indicators for academic papers is that they have statistically significant, but small, positive correlations with citation counts in various disciplines, including the medical sciences [ 7 ], papers published in arXiv [ 8 ], and ecology [ 9 ]. These positive associations suggest that the number of times a publication has been shared on Twitter relates in some way to its scholarly impact, but it is difficult to interpret tweet counts confidently because of the low correlations and the possibility that tweets might reflect non-academic impacts. There have also been small scale studies of academic Twitter users, reviewed below, but no investigations of non-academic users. The survey in this article will help to clarify why anyone tweets academic research so that tweet counts can be interpreted with more confidence for the type of impact that they reflect, if any.
The search behavior of Twitter users has studied before but only from a general perspective [ 10 ],[ 11 ] and little is known about how users find scholarly information. It is therefore not clear whether tweeting academic articles is useful in the sense of helping others to find them.
Literature review
Scholarly communication in the digital age includes formal and informal uses of the internet to discuss, publish and disseminate scientific discoveries [ 12 ]. In the current paper this definition is expanded to cover non-academics accessing, discussing, and sharing academic information. This study focuses on Twitter as a new channel for dissemination of scholarly articles because it is the most widely used general social media site for publication sharing [ 13 ]. This section first discusses general reasons for using Twitter in academic contexts. There is no relevant research on academic tweeting (i.e. tweeting scientific articles) by non-academic users, so this issue is not covered. It then focuses on counts of tweets about academic publications as an indicator for research evaluations.
Types of people using Twitter in a scholarly context
Twitter seems to be used by a substantial minority of academics, although this varies by country. Nothing is known about the extent to which non-academics use Twitter to share scholarly information. About a third (35%) of higher education professionals in the USA were Twitter users in 2010 [ 14 ]. Uptake can vary by discipline, with Twitter being among the most popular platforms in the bibliometric community [ 15 ]. Internationally, Twitter is banned in some countries and can be second choice to other microblogging services, such as Sina Weibo in China.
Information discovery on Twitter in academia
Few studies have investigated how people discover scholarly or other information on Twitter. A user study with 20 faculty and students found that facts and links helped to make a tweet useful [ 16 ]. A survey of Microsoft employees showed that they sometimes tweeted questions to get factual knowledge, often related to technology [ 17 ]. In addition to asking questions and noticing tweets in their feed, users can also search Twitter for a specific piece of information [ 10 ]. When users search Twitter, they are typically looking for time-dependent recent information, such as breaking news [ 11 ]. In the context of scholarly information, this news might be the publication of new relevant articles or information about an ongoing conference talk, for example. Nevertheless, very little is known about how Twitter is used to actively seek scholarly information.
Motivations for tweeting in a scholarly context
There are many reasons that academics cite research on Twitter. A survey of 28 tweeting academics in 2010 found that they cited peer-reviewed publications as an integral part of their activities on Twitter [ 5 ].
Scholars also use Twitter for purposes other than sharing articles. An online survey of faculty and students of a UK university found that academics use Twitter to increase their professional reputation [ 18 ]. An analysis of 68,232 tweets from 37 astrophysicists revealed that they used Twitter to communicate with co-workers, science communicators, other scholars, and related organizations or associations [ 19 ]. A study of ten academic disciplines on Twitter found substantial disciplinary differences in usage patterns. For example, cognitive science and digital humanities scholars hosted discussions while economists were more likely to share web links[ 20 ]. In contrast, digital humanities scholars use Twitter to get updated information, develop their professional skills, and expand their networks [ 1 ]. Most recently, a survey of 515 Twitter users who identified themselves as scientists found that they mainly used Twitter to communicate with researchers and share their publications with the public[ 21 ]. An analysis of tweets from 45 academics found that they also share information about their teaching activities and request help or give suggestions[ 22 ].
Types of scholarly content shared on Twitter by academics
At conferences, Twitter is sometimes used to disseminate quotes, notes, and presentations [ 23 ]. An analysis of shared links via tweets of three conferences revealed that blog entries, slides shows were among top types of information resources were exchanges [ 24 ]. A study of the Social Sciences and Humanities Research Council of Canada (SSHRC) doctoral fellowship recipients showed that they mainly share news links in their tweets even when chatting about scientific subjects [ 25 ]. A multidisciplinary analysis of scholars on Twitter indicates that they disseminated informal scientific related resource including magazine papers, blog posts, newspaper links [ 20 ].
Academic topics discussed by non-academics on Twitter
Microblogging services like Twitter may provide a new way for scholars to interact with the public since many non-academics use Twitter and it is suitable for information sharing [ 26 ]. Twitter therefore has the potential to supplement or replace existing science communication methods, such as science magazines, public lectures and newspaper stories. Nevertheless, since Twitter is partly a connection-based network, it is not clear whether it can be effective for communication between researchers and non-academics because they may not be part of the same networks. Moreover, the lay public may not search Twitter for scholarly information but may instead consult Wikipedia or the mass media.
There have been systematic attempts by scholars to communicate with the public, indicating a belief that this is worthwhile and possible. For example, one study demonstrated that scholars used Twitter to educate the public in the Flint water crisis in Michigan [ 27 ].
A content analysis of 72,469 tweets related to more than 900 scientific news stories revealed that climate change generated the most discussion and there was a complex Twitter ecosystem of contributors that included mass media, celebrity activists, and politicians. Whilst individual scholars and articles did not seem to be important on Twitter for discussions of these news stories, major scientific organizations like @NASA, scientific projects like @marsCuriosity, and prominent scholarly bloggers like literary and cultural commentator Maria Popova (@brainpicker) were important [ 28 ]. Thus, it seems possible that typical researchers and papers have little or no impact on the public through Twitter but individual prominent organizations and individuals can be heard. They presumably achieve this through a long-term high-effort strategy of building Twitter networks through quality appropriate content. This would not be practical for most scholars.
Twitter and other social media have been successfully used to engage the public as part of The European Space Agency's comet-chasing Rosetta mission. For example, the hashtag #WakeUpRosetta trended on Twitter at one stage [ 29 ]. This illustrates that individual important news stories can engage public attention, and suggests that Twitter might be useful for real-time scientific event monitoring and as part of a wider social and mass media engagement strategy.
From the perspective of journals in a given field, an analysis of academic articles from several conservation studies journals demonstrated that mass media news stories were the most important factor for informing the public about an article, leading to increased sharing on Twitter and Facebook [ 30 ]. This confirms that Twitter alone may not be enough to communicate effectively with the public for typical research.
Scholars liking, saving, and retweeting scholarly content
Liking, saving, and retweeting are important parts of the Twitter information ecosystem. Whilst liking and retweeting help to promote tweets so that they are more easily found by other users, saving helps a user to retrieve a tweet later.
Retweeting can be used to help disseminate academic information. A study of tweets from 447 active scholars from ten disciplines showed that scholars retweeted more (20%-42% of all tweets) than typical users and that biochemists were the most active retweeters [ 20 ]. In support of this, Social Sciences and Humanities Research Council doctoral awardees retweeted in 37% of their tweets, although a lower figure (13%) was found for 37 selected Twitter astrophysicists [ 19 ]. A study of academic conference tweeters and retweeters found that they had common interests [ 31 ] rather than being separate classes of users. Participants at three conferences tended to retweet users with similar opinions and professions [ 24 ].
Some projects have studied motivations for favoriting tweets (replaced in 2015 by liking tweets), although not in a scholarly context. A mainly crowdsourced (Tellwut) survey of 606 tweeters in 2013/4 found that half favorited tweets. Users favorited a tweet to help them to find it later or to show approval of the tweet [ 32 ] [ 33 ]. There seems to be no research focusing on reasons for favoriting academic tweets.
Tweeting academic publications and research impact
Information about scholarly uses of Twitter is helpful to interpret tweet counts as impact evidence for tweeted publications. Traditionally, citation-based indicators have been used to help evaluate scholarly outputs, but are slow to accumulate and are unable to reflect non-academic impacts [ 34 ] [ 35 ]. In response, altmetrics have been developed as academic-related indicators derived from social web data, such as Twitter, Mendeley, and blogs, to give earlier evidence of impact or evidence of non-academic impacts [ 36 ].
Many publications are mentioned on Twitter. At least 39% of papers submitted to arXiv.org in 2012 have been tweeted at least once [ 37 ] and 10% of 1.4 million publications indexed in both PubMed and Web of Science between 2010 and 2012 have been tweeted[ 38 ]. More articles are tweeted than mentioned on any other social media platform, according to Altmetric.com [ 7 ], although their data may underestimate the numbers of Mendeley readers because this company collects Mendeley information only for articles that are mentioned in at least one other source.
The logical first step for evaluating an impact indicator, such as tweet counts, is to assess whether it correlates with citation counts [ 39 ]. This is more straightforward than questionnaires and can give large scale evidence of the relationship between the new indicator and citation counts, which are better understood. Most Twitter studies have found very weak correlations, however. Using Altmetric.com data, an early study found a very low negative correlation between tweets and citation counts [ 7 ]. The reason posited for the negative correlation was the rapid increase in Twitter uptake at the time so that new, uncited aritcles were tweeted more than older, cited articles from the same year. A study of publications indexed in both PubMed and Web of Science between 2010 and 2012 found low positive correlations between citations and tweets for articles, with disciplinary variations[ 38 ]. For arXiv.org repository papers 2011–2012, downloads and early citation counts correlate moderately with Twitter mentions for academic papers [ 8 ]. Weak or moderate correlations between Twitter mentions and citations were found in another study [ 40 ], and strong correlations for twenty ecology journals [ 9 ]. Using a different approach, tweets of academic articles have been shown to predict future citations, although only for one online journal in the early years of Twitter [ 41 ]. Despite some stronger findings, the overall picture is therefore of a very weak relationship between tweet counts and citation counts. This low correlation suggests that people do not tweet articles for the same reason that they cite them. It it is important to investigate these new reasons thoroughly.
Another way to investigate the value of tweeting academic research is to apply content analysis to tweets. Using this approach, most tweets reflect the title of the papers or brief summaries, with very little scholarly discussion [ 42 ]. This gives little insight into why an article was tweeted, but rules out critical analyses as a major factor, despite it occurring occasionally [ 43 ].
Research questions
This study investigates key aspects of academic information sharing on Twitter to better understand (a) the context in which academic research is tweeted, and (b) the context in which Twitter is used to find academic information. The research questions cover gaps identified by the literature review.
- What types of people (occupation; broad disciplinary area; age; gender) tweet academic research?
- How is Twitter used to find academic-related information?
- Why is Twitter used to communicate scholarly information? Does the answer depend on academic discipline, gender, age, and occupation?
- What types of scholarly content is shared on Twitter? Does the answer depend on academic discipline, gender, age, and occupation?
- Why are scholarly tweets liked, saved, or retweeted?
Methodology
Although many previous studies have analyzed published tweets, not all tweets originate from a person. Twitter bots create automatic tweets that are difficult to distinguish from human-authored posts [ 44 ]. Machines create a substantial number of tweets of academic papers [ 45 ], undermining evidence about the value of Twitter from studies that interpret tweets at face value. A survey approach was used to bypass this problem by focusing on human users and to get richer background information.
Twitter had 310 million users in 2017 [ 46 ], making it difficult to randomly sample academic-related content and users [ 23 ]. In theory, all public tweets could be purchased and data mined to extract a large set of users that generate academic-related tweets. This method is prohibitively costly but a practical alternative would be to use the free Twitter API to randomly sample 1% of all tweets for a specified period and data mine these for academic content. This approach would be undesirable because Twitter does not publicize the methods used to select the permitted 1% of free tweets. Moreover, any data mining attempt to identify academic-related tweeters would introduce its own hidden biases. In response, a novel approach was developed to identify users who shared scientific information through Twitter. A list of Twitter accounts that have tweeted at least one academic paper between January 2011 and December 2015 was obtained from the altmetric data provider Altmetric.com from their ongoing Twitter monitoring of tweets that link to academic domains (e.g., publisher websites) using the commercial Twitter PowerTrack API, giving 4.5 million unique Twitter accounts. Whilst the requirement to have tweeted an academic article is a biasing feature, it is a transparent selection criterion and matches the goal of understanding how academic publication sharing occurs on Twitter.
Twitter’s anti-spam filters made it impossible to tweet a large sample of academic tweeting users directly and so an email survey was used instead. To obtain users’ email addresses, the sample was restricted to users with web links in their Twitter profiles. This is likely to bias the sample towards academic users because these seem most likely to have a public page containing contact information. Such pages are automatically generated for academics by many universities, for example. This additional source of unknown bias is undesirable but there does not seem to be a better alternative. The data mining approach rejected above would also need this step.
Using the data from Altmetric.com, 1,771,520 web links were harvested from the 4.5 million Twitter accounts (i.e., 39%). These links included personal or professional web pages as well as irrelevant targets, such as YouTube videos. The 1,771,520 websites were automatically downloaded and emails extracted from them, when present, producing 57,125 email addresses (1.3% of the original sample) from a range of different domains ( Table 1 ). There were very few emails from all other domains (e.g., .net, .org, .de), and so these were excluded to focus on the main domains. All contact information obtained from the web and Twitter for this study is public data. The data collection methods met terms of service for the websites.
- PPT PowerPoint slide
- PNG larger image
- TIFF original image
https://doi.org/10.1371/journal.pone.0197265.t001
A questionnaire was developed and refined through numerous pilot tests. This survey received institutional review board (IRB) approval from Northwestern University. A copy of survey questionnaire is available at https://figshare.com/s/caaa37d468b7302cb611 . The questionnaire was sent to 57,125 Twitter users through Survey Monkey in early July 2016, with a reminder sent to non-responding users in late July. Approximately 94% of the invitations were not opened and 1617 emails bounced, giving a low response rate (2%, n = 1105). Some survey invitation emails may have been marked as spam and not received by the intended recipients. Several users emailed to confirm the genuineness of the survey, suggesting that some recipients were reluctant to open the survey link in case it connected to a malicious site.
To increase the response rate, in mid-August 2016, the survey was distributed through Northwestern University, as a trusted academic mail server, to the 53,809 Twitter users who had not opened the Survey Monkey link. Users who had initially opted out of the survey or with bounced email addresses were excluded. A reminder was sent to non-responding invitations in early September. From this, 3,045 additional emails bounced and 113 additional users opted out. This step gave 807 additional responses.
Overall, 1,912 (4%) users completed the survey, with 4,662 (8%) email invitations bouncing and 707 (1%) of users opting out ( Table 1 ). The response rates for academic email addresses (.edu: 11%, .ac.uk: 9%) were higher than for the other domains. The email addresses in commercial domains (Gmail, .com) may not have been the primary or active email accounts of the Twitter users, causing the lower response rates. Alternatively, non-academic users and non-English speakers may have been more reluctant to reply. Most tweets are posted by 20% of all Twitter accounts [ 47 ], so the majority of people surveyed may have been occasional tweeters or former users that did not want to bother with a questionnaire about something that was not important to them. The overall response rate, while low, is normal for email questionnaires [ 48 ] and web surveys with response rates regularly being lower than 10% [ 49 ]. The total number of responses is higher than in other similar surveys with 28 responses [ 5 ] and 613 participants [ 6 ].
Descriptive statistics are presented as counts and percentages. Chi-square tests were used to assess associations between categorical covariates and responses, Cochran-Armitage trend tests between ordinal covariates and responses, and Wilcoxon rank sum tests for comparisons between skewed continuous covariates and responses. All analyses were performed in SAS v. 9.4, and a nominal 5% type I error level was used.
The main results are given in the appendices and this section summarizes the key findings.
Who tweets scholarly information?
Based on the IP addresses of the participants, the survey respondents were 64% from North America, 17% from the United Kingdom, 10% from Europe, 5% from Asia, 2% from South America, 2% from Oceania, and 1% from Africa. This agrees with the overall geographical distribution of Twitter users [ 50 ]. There was a slight gender bias in favor of males (55%). The most common age range was 31–40 years old (31%), while 25% were 41–50, 19% were 21–30, 16% were 51–60, 8% were older than 60, and 1% were under 21. Survey participants tend to be younger and male, which is consistent with previous studies [ 51 ],[ 52 ],[ 6 ].
Most participants defined their disciplines as social science (37%) or humanities (22%), with smaller numbers from Engineering/Technology (15%), Natural Sciences (13%), Medical/Health Sciences (11%), and Agricultural Sciences (1%). For the non-academic respondents, these disciplines presumably associated with their highest qualification or occupation. People from these broad disciplinary areas presumably share an information culture to some extent, irrespective of whether they are academics or not. From across academia overall, the first two categories are overrepresented. The survey respondents mostly worked in academic institutions (55%), but almost half worked outside, in industry and professional organizations (41%) or government (4%). Thus, the survey has successfully recruited large numbers of non-academic participants (see also Fig 1 ).
https://doi.org/10.1371/journal.pone.0197265.g001
The academic faculty members were assistant professor/lecturer or senior lecturer (40%), associate professor/reader (25%), professor (25%), instructor/teaching assistant (8%), and adjunct faculty (3%).
Finding academic-related tweets
Tweets can be found from a user’s Twitter home page, with the Twitter search box and or through general search engines, such as Google and Bing. Most of the respondents (73%, n = 1218 out of 1658 that answered the question) stated that they found tweets by following specific Twitter accounts. Half (50%, n = 826) used hashtags and some used keyword searching through Twitter (43%, n = 718) or web search engines (20%, n = 332). A few used third-party applications, such as TweetDeck (11%, n = 186), and other feeds (5%, n = 82) to find scholarly tweets.
Almost three quarters (73%) of survey participants found academic tweets by following accounts, with insufficient evidence to claim (population) differences between academic disciplines ( p = .205), and genders ( p = .205). Account following was more likely to be done by researchers (78%, p<0.001), people in academia (79%, p<0.001), faculty (79%, p<0.001), and younger people (76–77% for 21–40, p = .013). Journalists (62%, p = .001) and older people (for 60+: 64%, p = .013) were least likely to use it. Thus, following accounts is particularly important in an academic context although it is common for all (see S1 Table ).
Half of all respondents (50%) searched by hashtags, with insufficient evidence to conclude that there are disciplinary (p = .633), researcher status (p = .814), or work status (p = .831) differences. Hashtags are more often used in academia (53%, p = .024), by females (56%, p<0.001), by younger people (21–30: 58%, p<0.001), and by people that had joined Twitter a longer time ago (8+ years: 57%, p<0.001).
The main disciplinary differences for methods to find tweets concern querying in Twitter or commercial search engines. Humanities scholars used keyword searches in Twitter (48%) much more than did natural scientists (32%). People involved in engineering or technology used commercial search engines (25%) more than did natural scientists (17%). The underlying difference here is that natural scientists are less likely to actively search for tweets than are users from other disciplines.
Non-researchers are more likely to search in Twitter (46% vs. 43%) or commercial search engines (22% vs. 18%) than are researchers, but they otherwise find tweets in similar ways.
There are differences between sectors in all methods used to find tweets, except for 3 rd party apps. People in academia are the most likely to follow accounts (79%, compared to 70% for government, 67% for commercial/professional, p<0.001) and hashtags (53%, 48%, 46%, p = .024). Government users are most likely to use a commercial search engine (15%, 28%, 26%, p<0.001), whereas professional/commercial users are most likely to use Twitter keyword searches (40%, 36%, 48%, p = .002). Thus, more active searching is more characteristic of non-academic users.
The only statistically significant difference in methods to find tweets is that females are more likely to follow hashtags (56% vs. 45%, p<0.001). Hashtags probably perform a communicative function often in academia, such as by allowing conference participants to interact online.
There are age differences in all methods used to find tweets, except for 3 rd party apps. The biggest difference is that older users (60+: 31%) were almost twice as likely to find tweets with a commercial search engine than were younger users (<21: 16%).
Reasons for using Twitter in academic settings
The survey respondents that gave a reason for using Twitter (n = 1811) used it to obtain real-time information (73%, n = 1323), share real-time information (66%, n = 1198), expand their professional networks (64%, n = 1150), contribute to wider conversations (54%, n = 985) promote organizations (55%, n = 995), communicate about academic events (52%, n = 949), communicate the results of scientific publications to the public (47%, n = 856) and peers (43%, n = 773), and for teaching (16%, n = 292) (see S2 Table ).
There were no statistically significant differences between disciplines in terms of the extent to which respondents obtained ( p = .535) or shared ( p = .390) real-time information, or used Twitter to expand their professional network ( p = .650). In Social Sciences, Twitter is more likely to be used in teaching. In Humanities, it is more likely to be used to contribute to wider conversations, a natural humanities role. In Engineering/Technology, Twitter tends to be used less for everything, particularly for communicating results and about academic events. In Natural Sciences, it is less likely to be used to contribute to wider conversations. In Medical/Health Sciences, Twitter tends to be used more for most things except teaching, and is particularly well used for communicating results to peers and the public and for communicating about academic events (see S2 Table ).
Unsurprisingly, researchers are more likely to communicate research results but non-researchers are more likely to tweet promoting organizations. Similarly, in terms of work sector, the use of Twitter is higher in academia for most things except promoting organizations, where is it more common in industry. Government workers are the most likely to Tweet to contribute to wider conversations. Nevertheless, journalists use Twitter more for finding and sharing real-time information and for contributing to wider conversations, which fits their job role. Managers use it the most for promoting their organization (see S2 Table ).
More males used Twitter to promote their organizations and communicate about academic events than did females ( p = .001). There were no other significant gender differences in the other reasons for using Twitter in scholarly contexts (see S2 Table ).
Age was a statistically significant factor for obtaining real-time information ( p = .001), communicating the results of academic publications to peers ( p = .001) and the public ( p = .001), communicating about academic events ( p = .001), teaching ( p = .003) and promoting their organization ( p = .001). In general, people aged 31–40 tended to have more reasons for using Twitter and people under 21 tended to have the fewest reasons (see S2 Table ).
What types of scholarly content are shared through Twitter?
Survey participants shared many different types of academic-related materials on Twitter, including research articles and other published works (77%, n = 1281), research related news (68%, n = 1135), blog posts (66%, n = 1097), lay summaries of research for the public (42%, n = 703), videos and images (33%, n = 548), policy announcements (31%, n = 508), and presentation slides (20%, n = 338) ( S3 Table ). Since participants were selected on the basis that they had tweeted an academic article, the 77% figure above suggests that many users (23%) had forgotten some of their tweets.
Social scientists tended to share the most, including publications, research-related news and policy announcements. Humanities scholars tended to share the least, including publications, slides, and research-related news. In Engineering/Technology, slides were shared the most, presumably reflecting the importance of conferences in many engineering fields. In Medical/Health Sciences, policy announcements were frequently shared ( S3 Table ).
Unsurprisingly, researchers shared the most publications and research-related news. Interestingly, however, non-researchers shared the most videos and images, blog posts, and lay summaries. Thus, there seems to be a niche role for non-researchers in helping to communicate research in non-standard ways. This is broadly echoed by the work sector results. The government and industry/professional sectors tend to share similarly, except that government workers share more research-related news. In terms of occupation, researchers and faculty tended to share publications, presentations, and research-related news the most and lay summaries, videos, and images the least ( S3 Table ).
There were only minor gender differences in sharing, with males sending to share presentations more (perhaps due to their use in male-dominated engineering fields), whereas females shared research-related news and lay summaries more. The age range 31–50 was the most active for sharing, including publications, research-related news. People with older Twitter accounts also tended to share more, including presentations, videos and images, and blog posts ( S3 Table ).
The role of Twitter in scholarly practice
Several questions were asked about the use of Twitter in scholarly activities. Most respondents agreed or strongly agreed (81%, n = 1333) that tweeting academic articles disseminates scholarly information to the public, representing a broad consensus ( Fig 2 ), despite a lack of evidence that the public read academic tweets in most fields (health and astronomy are exceptions). Most respondents also agreed or strongly agreed (79%, n = 1307) that Twitter has changed the way that academic knowledge can be read and disseminated. Almost as many respondents agreed or strongly agreed (76%, n = 1249) that Twitter facilitates knowledge flows from one academic discipline to another.
https://doi.org/10.1371/journal.pone.0197265.g002
Although respondents agreed that Twitter could be used to communicate scientific knowledge, they were split about whether it could be used to measure scholarly impact. Only half (52%, n = 864) agreed or strongly agreed, with nearly one-third (29%, n = 479) being neutral.
User activities and influences in Twitter in scientific contexts
Two thirds of survey respondents (66%) tweeted at least weekly. This was most common in Social Sciences (69%) and least common in Humanities and Engineering/Technology (62%). Journalists were the most likely to tweet at least weekly (80%) and professionals (58%) the least likely. Males (68%) and people aged 51–60 (70%) were more frequent tweeters, as were people with an account over 8 years old (71%) ( S4 Table ).
Users have several engagement options available on Twitter: liking (formerly known as favoriting), saving , and retweeting content. Most respondents (71%, n = 1197) like a tweet to inform the authors that their tweets were interesting. Forty-three percent (n = 725) like tweets to help them to be found in the future. Only a fifth (21%, n = 352) liked tweets to disseminate them ( Table 2 ).
https://doi.org/10.1371/journal.pone.0197265.t002
Unsurprisingly, academic tweets were usually retweeted to disseminate them (85%, n = 1428) but also sometimes (42%, n = 710) to tell the author that their tweet was interesting, for future access (21%, n = 351) and to inform the author that they had read the tweet (15%, n = 235).
Limitations
The results have several limitations. The survey sample is limited to Twitter users with personal webpage links in their Twitter profiles. These are presumably more likely to be academics or professionals than people that are unemployed or working in government or industry. The sample is also restricted to people that have current email addresses in their webpages that can be extracted using web-mining methods. Thus, the original sample is a limited and probably biased subset of people that have tweeted academic articles. The low response rate is typical of online surveys and is likely to bias the results to the attitudes of people that have a greater interest in the use of Twitter within research. This may explain the high numbers of social sciences and humanities scholars. It probably also biases the results towards more active users.
The numerous statistical tests greatly increase the likelihood that at least one finds spurious evidence of differences when none are present (i.e., a high familywise type 1 error likelihood). The results should therefore be interpreted cautiously, especially when the p value is close to 0.05. As with all surveys, some respondents may misinterpret the questions. Moreover, social network sites and typical methods of use change over time, adding extra uncertainty to the results.
The survey suggests that most people who tweet academic information work in academic institutions, with other groups of users outside academia such professionals (engineers, physicians, and lawyers), but with many managers and journalists also tweeting academic information. This is consistent with previous research [ 31 ],[ 13 ], but probably over-represents academia due to the survey sampling method (emails from home page URLs in Twitter profiles). In this context, the relatively high proportion of non-academic tweeters is a surprising and important finding. No previous survey of Twitter use for scholarly communication has attempted to identify non-academic tweeters of academic research. For people seeking to use Twitter to disseminate information to the wider public, this is the most substantial evidence yet that this may work.
Within academia, the level of Twitter use varies by academic rank, with assistant professors among the top users, followed by associate professors, corroborating previous research [ 6 ]. Most respondents tweeting scholarly information were from the social sciences or humanities, agreeing with previous studies [ 20 ],[ 53 ],[ 13 ].
In terms of gender, the greater number of males responding to the survey may reflect similar gender imbalances in science [ 54 ] and perhaps also for Twitter use in academia [ 55 ].
Following specific accounts and hashtags are the primary means used to find academic information on Twitter. Users prefer this to searching on Twitter or via a web search engine. This probably reflects the way in which most people use Twitter [ 56 ] rather than being specific to science. Thus social ties are important on Twitter for finding information, and the same may also be said for hashtags, since these are human-generated communication channels [ 10 ].
For the second research question, the primary motivations for using Twitter in scholarly contexts are to get and share real-time information, and to develop connections. Twitter is also used to participate in online conversations, increase the visibility of organizations, disseminate activities related to academic events, share scholarly findings with peers and the public, and for educational purposes. These findings are consistent with previous studies about the reasons for using Twitter in general [ 57 ] and academic purposes in particular [ 58 ],[ 18 ]. There were statistically significant differences by discipline, employment sector, and occupation for many of these motivating factors.
Scientific publications, academic news, and blog posts are the most common information sources shared on Twitter. Some respondents also share lay summaries of research, videos, images, policy documents, and presentations. These findings are in agreement with an earlier study that used link analysis [ 13 ]. There were statistically significant differences by discipline, employment sector, occupation, age, and gender. For instance, faculty shared research articles more than other users while professionals tweeted scholarly videos the most. These differences are broadly consistent with what might be expected.
The consensus (81%) that tweeting academic articles disseminates scholarly information to the public is given some credence by the substantial number of non-academics responding to this survey. Perhaps related to this, most respondents (52%) thought that Twitter mentions of academic publications can be a research impact metric. Given the low correlations between Twitter mentions of scientific articles and citation counts found in previous studies[ 38 ],[ 8 ],[ 59 ], it is possible that tweets reflect non-academic impacts to a wider extent than previously thought. This supports previously unsubstantiated claims that Twitter mentions of academic papers could reflect their societal impact,which is an important issue in research evaluation [ 60 ].
There was agreement (81%) that Twitter has changed the way that scientific knowledge can be read and spread, including between disciplines (76%). Although previous studies have found evidence of cross-disciplinary knowledge transfer for other social web sites [ 61 ],[ 62 ] this is the first large-scale evidence for Twitter.
Most participants composed, replied to, liked and retweeted tweets. The level of activity varied based on the respondents’ occupation and gender, with males more actively tweeting than females. Researchers and faculty members at academic institutions more actively tweeted scholarly information than other users.
Conclusions
The results have several practical implications. For information sharing, Twitter is particularly important in the social sciences and humanities. Thus, researchers in these areas should be encouraged to investigate whether they can benefit from using the service, if they are not already doing so. It is possible that other disciplines rely on effective alternative communication means, such as conferences, preprint archives, or professional online networks such as LinkedIn.
Since users rely on social connections to find information on Twitter [ 10 ], people that seek to disseminate information on Twitter should not rely on others finding it through keyword searches and should try to build up followers or use appropriate hashtags instead. This will be unsurprising to experienced users.
The dissemination of science to wider audiences is an increasingly important task in academia. People that use Twitter to communicate research agree that it can perform this role, and this is supported by the proportion of non-academics responding to the survey (46% chose Government or Industry/Professional rather than Academic as their work sector) that also do this, despite the likely bias of the survey towards academics (because email addresses were extracted from personal pages to find respondents). This gives the strongest evidence yet that Twitter may be successful in this role and that Twitter-based indicators may also help to reveal the wider impacts of scholarly publications–although not in formal evaluations [ 63 ].
Finally, since messages shared on Twitter are not always conveyed effectively [ 31 ],[ 42 ],[ 64 ] and the current study has found a substantial number of non-academics that are interested enough in research to tweet papers, it is now increasingly important for academics to be able to write tweets that are understandable by a lay audience.
Supporting information
S1 table. methods used to find academic tweets based on academic discipline, age, gender and current job..
https://doi.org/10.1371/journal.pone.0197265.s001
S2 Table. Primary reasons for scholarly use of Twitter by academic discipline, gender, age and occupation.
https://doi.org/10.1371/journal.pone.0197265.s002
S3 Table. Types of scientific content shared by users on Twitter by discipline, gender, age and job.
https://doi.org/10.1371/journal.pone.0197265.s003
S4 Table. The level of scholarly activity on Twitter by discipline, gender, age and occupation.
https://doi.org/10.1371/journal.pone.0197265.s004
Acknowledgments
Thank you to William H. Dutton and Karen Gutzman for assistance with piloting the survey; and Altmetric.com for providing the dataset of Twitter mentions of academic papers. This research was supported, in part, by the National Institutes of Health’s National Center for Advancing Translational Sciences grant number UL1TR001422. The content is solely the responsibility of the authors and does not necessarily represent the official views of the National Institutes of Health. No additional external funding was received for this study.
- View Article
- Google Scholar
- 3. Sugimoto CR. Tenure can withstand Twitter”: we need policies that promote science communication and protect those who engage. In: Impact of Social Sciences Blog [Internet]. 2016. Available: http://blogs.lse.ac.uk/impactofsocialsciences/2016/04/11/tenure-can-withstand-twitter-thoughts-on-social-media-and-academic-freedom/
- PubMed/NCBI
- 6. Bowman TD. Investigating the use of affordances and framing techniques by scholars to manage personal and professional impressions on Twitter. Indiana University. 2015.
- 11. Teevan J, Ramage D, Morris MR. #TwitterSearch: a comparison of microblog search and web search. WSDM ‘11 Proceedings of the fourth ACM international conference on Web search and data mining. New York, New York, USA: ACM Press; 2011. pp. 35–44. https://doi.org/10.1145/1935826.1935842
- 14. Faculty Focus. Twitter in Higher Education 2010: Usage Habits and Trends of Today’s College Faculty [Internet]. 2010. Available: http://www.facultyfocus.com/free-reports/twitter-in-higher-education-2010-usage-habits-and-trends-of-todays-college-faculty
- 26. Puschmann C. (Micro)Blogging Science? Notes on Potentials and Constraints of New Forms of Scholarly Communication. Opening Science. Cham: Springer International Publishing; 2014. pp. 89–106. https://doi.org/10.1007/978-3-319-00026-8_6
- 37. Haustein S, Bowman T, Macaluso B, Sugimoto C. Measuring Twitter activity of arXiv e-prints and published papers. altmetrics14: expanding impacts and metrics. altmetrics14: expanding impacts and metrics An ACM Web Science Conference 2014 Workshop Bloomington,. Bloomington, IN; 2014. https://doi.org/10.6084/m9.figshare.1041514
- 46. Twitter. It’s what’s happening [Internet]. 2017. Available: https://about.twitter.com/company
- 50. Java A, Song X, Finin T, Tseng B. Why we twitter: Understanding Microblogging Usage Usage and Communities. Proceedings of the 9th WebKDD and 1st SNA-KDD 2007 workshop on Web mining and social network analysis—WebKDD/SNA-KDD ‘07. New York, New York, USA: ACM Press; 2007. pp. 56–65. https://doi.org/10.1145/1348549.1348556
- 52. Greenwood S, Perrin A, Duggan M. Social Media Update 2016 [Internet]. 2016. Available: http://www.pewinternet.org/2016/11/11/social-media-update-2016
- 56. Teevan J, Ramage D, Morris MR. #TwitterSearch: a comparison of microblog search and web search. fourth ACM Int Conf Web search data Min—WSDM ‘11. 2011; 35. https://doi.org/10.1145/1935826.1935842
- 57. Zhao D, Rosson MB. How and Why People Twitter: The Role that Micro-blogging Plays in Informal Communication at Work. Proceedinfs of the ACM 2009 international conference on Supporting group work—GROUP ‘09. New York, New York, USA: ACM Press; 2009. p. 243. https://doi.org/10.1145/1531674.1531710
Twitter and Research: A Systematic Literature Review Through Text Mining
Ieee account.
- Change Username/Password
- Update Address
Purchase Details
- Payment Options
- Order History
- View Purchased Documents
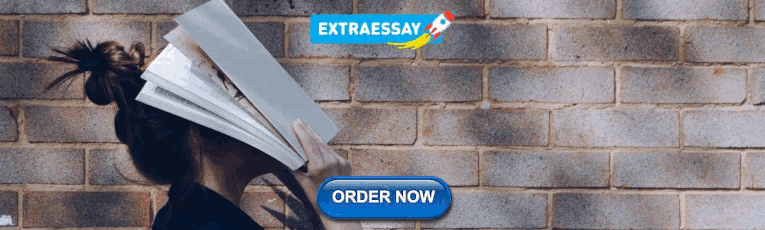
Profile Information
- Communications Preferences
- Profession and Education
- Technical Interests
- US & Canada: +1 800 678 4333
- Worldwide: +1 732 981 0060
- Contact & Support
- About IEEE Xplore
- Accessibility
- Terms of Use
- Nondiscrimination Policy
- Privacy & Opting Out of Cookies
A not-for-profit organization, IEEE is the world's largest technical professional organization dedicated to advancing technology for the benefit of humanity. © Copyright 2024 IEEE - All rights reserved. Use of this web site signifies your agreement to the terms and conditions.
Suggestions or feedback?
MIT News | Massachusetts Institute of Technology
- Machine learning
- Social justice
- Black holes
- Classes and programs
Departments
- Aeronautics and Astronautics
- Brain and Cognitive Sciences
- Architecture
- Political Science
- Mechanical Engineering
Centers, Labs, & Programs
- Abdul Latif Jameel Poverty Action Lab (J-PAL)
- Picower Institute for Learning and Memory
- Lincoln Laboratory
- School of Architecture + Planning
- School of Engineering
- School of Humanities, Arts, and Social Sciences
- Sloan School of Management
- School of Science
- MIT Schwarzman College of Computing
Study: On Twitter, false news travels faster than true stories
Press contact :, media download.
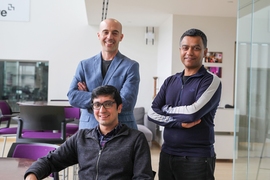
*Terms of Use:
Images for download on the MIT News office website are made available to non-commercial entities, press and the general public under a Creative Commons Attribution Non-Commercial No Derivatives license . You may not alter the images provided, other than to crop them to size. A credit line must be used when reproducing images; if one is not provided below, credit the images to "MIT."
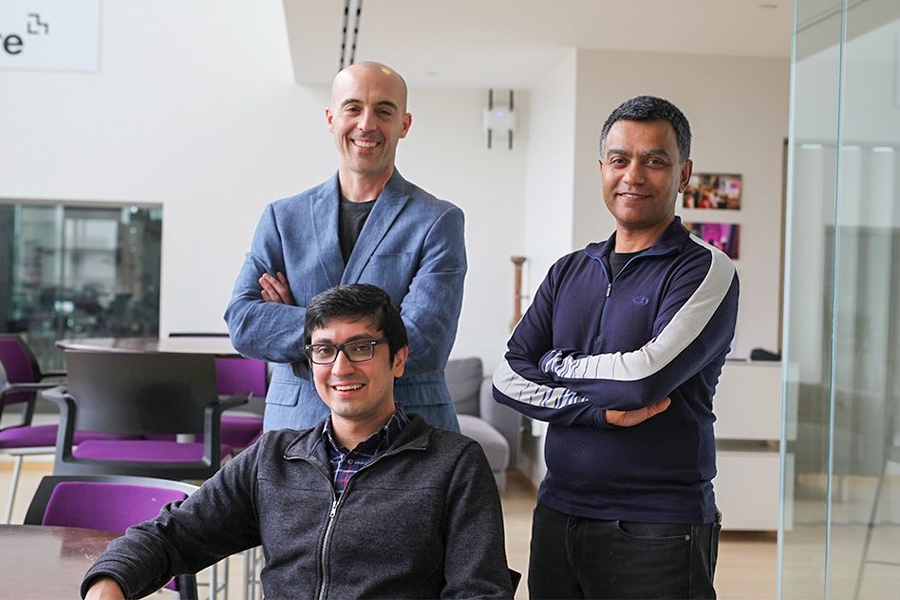
Previous image Next image
A new study by three MIT scholars has found that false news spreads more rapidly on the social network Twitter than real news does — and by a substantial margin.
“We found that falsehood diffuses significantly farther, faster, deeper, and more broadly than the truth, in all categories of information, and in many cases by an order of magnitude,” says Sinan Aral, a professor at the MIT Sloan School of Management and co-author of a new paper detailing the findings.
“These findings shed new light on fundamental aspects of our online communication ecosystem,” says Deb Roy, an associate professor of media arts and sciences at the MIT Media Lab and director of the Media Lab’s Laboratory for Social Machines (LSM), who is also a co-author of the study. Roy adds that the researchers were “somewhere between surprised and stunned” at the different trajectories of true and false news on Twitter.
Moreover, the scholars found, the spread of false information is essentially not due to bots that are programmed to disseminate inaccurate stories. Instead, false news speeds faster around Twitter due to people retweeting inaccurate news items.
“When we removed all of the bots in our dataset, [the] differences between the spread of false and true news stood,”says Soroush Vosoughi, a co-author of the new paper and a postdoc at LSM whose PhD research helped give rise to the current study.
The study provides a variety of ways of quantifying this phenomenon: For instance, false news stories are 70 percent more likely to be retweeted than true stories are. It also takes true stories about six times as long to reach 1,500 people as it does for false stories to reach the same number of people. When it comes to Twitter’s “cascades,” or unbroken retweet chains, falsehoods reach a cascade depth of 10 about 20 times faster than facts. And falsehoods are retweeted by unique users more broadly than true statements at every depth of cascade.
The paper, “The Spread of True and False News Online,” is published today in Science .
Why novelty may drive the spread of falsity
The genesis of the study involves the 2013 Boston Marathon bombings and subsequent casualties, which received massive attention on Twitter.
“Twitter became our main source of news,” Vosoughi says. But in the aftermath of the tragic events, he adds, “I realized that … a good chunk of what I was reading on social media was rumors; it was false news.” Subsequently, Vosoughi and Roy — Vosoughi’s graduate advisor at the time — decided to pivot Vosoughi’s PhD focus to develop a model that could predict the veracity of rumors on Twitter.
Subsequently, after consultation with Aral — another of Vosoughi’s graduate advisors, who has studied social networks extensively — the three researchers decided to try the approach used in the new study: objectively identifying news stories as true or false, and charting their Twitter trajectories. Twitter provided support for the research and granted the MIT team full access to its historical archives. Roy served as Twitter’s chief media scientist from 2013 to 2017.
To conduct the study, the researchers tracked roughly 126,000 cascades of news stories spreading on Twitter, which were cumulatively tweeted over 4.5 million times by about 3 million people, from the years 2006 to 2017.
To determine whether stories were true or false, the team used the assessments of six fact-checking organizations (factcheck.org, hoax-slayer.com, politifact.com, snopes.com, truthorfiction.com, and urbanlegends.about.com), and found that their judgments overlapped more than 95 percent of the time.
Of the 126,000 cascades, politics comprised the biggest news category, with about 45,000, followed by urban legends, business, terrorism, science, entertainment, and natural disasters. The spread of false stories was more pronounced for political news than for news in the other categories.
The researchers also settled on the term “false news” as their object of study, as distinct from the now-ubiquitous term “fake news,” which involves multiple broad meanings.
The bottom-line findings produce a basic question: Why do falsehoods spread more quickly than the truth, on Twitter? Aral, Roy, and Vosoughi suggest the answer may reside in human psychology: We like new things.
“False news is more novel, and people are more likely to share novel information,” says Aral, who is the David Austin Professor of Management. And on social networks, people can gain attention by being the first to share previously unknown (but possibly false) information. Thus, as Aral puts it, “people who share novel information are seen as being in the know.”
The MIT scholars examined this “novelty hypothesis” in their research by taking a random subsample of Twitter users who propagated false stories, and analyzing the content of the reactions to those stories.
The result? “We saw a different emotional profile for false news and true news,” Vosoughi says. “People respond to false news more with surprise and disgust,” he notes, whereas true stories produced replies more generally characterized by sadness, anticipation, and trust.
So while the researchers “cannot claim that novelty causes retweets” by itself, as they state in the paper, the surprise people register when they see false news fits with the idea that the novelty of falsehoods may be an important part of their propagation.
Directions for further research
While the three researchers all think the magnitude of the effect they found is highly significant, their views on its civic implications vary slightly. Aral says the result is “very scary” in civic terms, while Roy is a bit more sanguine. But the scholars agree it is important to think about ways to limit the spread of misinformation, and they hope their result will encourage more research on the subject.
On the first count, Aral notes, the recognition that humans, not bots, spread false news more quickly suggests a general approach to the problem.
“Now behavioral interventions become even more important in our fight to stop the spread of false news,” Aral says. “Whereas if it were just bots, we would need a technological solution.”
Vosoughi, for his part, suggests that if some people are deliberately spreading false news while others are doing so unwittingly, then the phenomenon is a two-part problem that may require multiple tactics in response. And Roy says the findings may help create “measurements or indicators that could become benchmarks” for social networks, advertisers, and other parties.
The MIT scholars say it is possible that the same phenomenon occurs on other social media platforms, including Facebook, but they emphasize that careful studies are needed on that and other related questions.
In that vein, Aral says, “science needs to have more support, both from industry and government, in order to do more studies.”
For now, Roy says, even well-meaning Twitter users might reflect on a simple idea: “Think before you retweet.”
Share this news article on:
Press mentions, marketplace.
Prof. Sinan Aral speaks with Marketplace reporter Molly Wood about the proliferation of fake news. “If platforms like Facebook are to be responsible for the spread of known falsities, then they could use policies, technologies or algorithms to reduce or dampen the spread of this type of news, which may reduce the incentive to create it in the first place,” Aral explains.
The Guardian
Researchers from the Media Lab and Sloan found that humans are more likely than bots to be “responsible for the spread of fake news,” writes Paul Chadwick for The Guardian. “More openness by the social media giants and greater collaboration by them with suitably qualified partners in tackling the problem of fake news is essential.”
The Washington Post
In an op-ed for The Washington Post, Megan McArdle shares her thoughts on research from the Media Lab and Sloan that identifies “fake news” as traveling six times faster than factual news. “The difference between social media and ‘the media’ is that the gatekeeper model…does care more about the truth than ‘the narrative,’” McArdle writes.
Jordan Webber of The Guardian addresses the rise of “fake news”, citing research from the Media Lab and Sloan. “I believe that social media is a turning point in human communication,” said Sloan Prof. Sinan Aral. “I believe it is having dramatic effect on our democracies, our politics, even our health.”
Robin Young and Femi Oke of WBUR’s Here and Now highlight research from Sloan and the Media Lab that shows how quickly false news travels the internet. “We [also] found that false political news traveled farther, faster, deeper, and more broadly than any other type of false news,” says Prof. Sinan Aral.
The New York Times
Prof. Sinan Aral writes for The New York Times about research he co-authored with Postdoc Soroush Vousaghi and Associate Prof. Deb Roy, which found that false news spreads “disturbingly” faster than factual news. “It could be, for example, that labeling news stories, in much the same way we label food, could change the way people consume and share it,” writes Aral.
Scientific American
Larry Greenemeier of Scientific American writes about a study from researchers at Sloan and the Media Lab that finds “false news” is “70% more likely to be retweeted than information that faithfully reports actual events.” “Although it is tempting to blame automated “bot” programs for this,” says Greenemeier, “human users are more at fault.”
The Atlantic
Researchers from Sloan and the Media Lab examined why false news spreads on Twitter more quickly than factual information. “Twitter bots amplified true stories as much as they amplified false ones,” writes Robinson Meyer for The Atlantic . “Fake news prospers, the authors write, ‘because humans, not robots, are more likely to spread it.’”
Previous item Next item
Related Links
- Paper: "The spread of true and false news online"
- Project: The Spread of True and False Information Online
- Soroush Vosoughi
- School of Architecture and Planning
Related Topics
- MIT Sloan School of Management
- Social media
- Technology and society
Related Articles
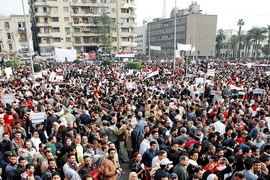
Street signs
![research paper on twitter “[W]hen you send location data as a secondary piece of information, it is extremely simple for people with very little technical knowledge to find out where you work or live,” Ilaria Liccardi says.](https://news.mit.edu/sites/default/files/styles/news_article__archive/public/images/201605/MIT-No-Privacy.jpg?itok=XWehmJJY)
We know where you live
More mit news.
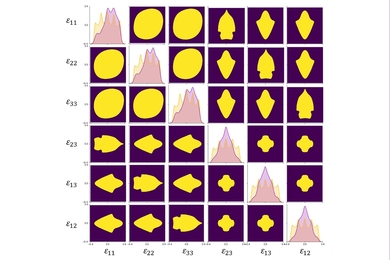
A first-ever complete map for elastic strain engineering
Read full story →
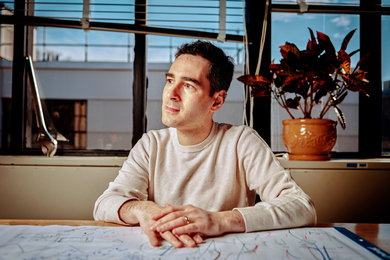
“Life is short, so aim high”
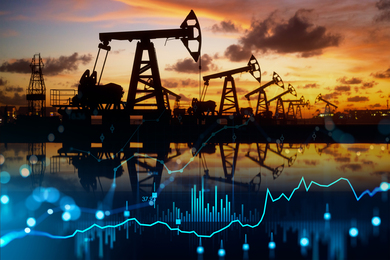
Shining a light on oil fields to make them more sustainable
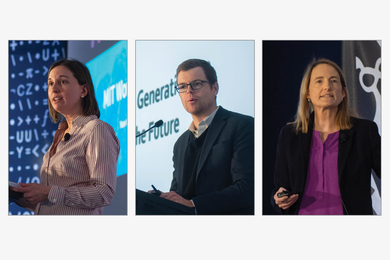
MIT launches Working Group on Generative AI and the Work of the Future
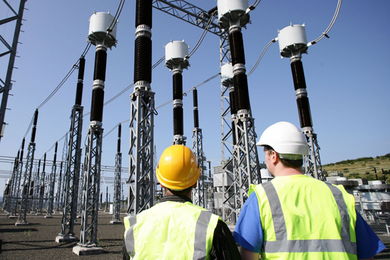
Atmospheric observations in China show rise in emissions of a potent greenhouse gas
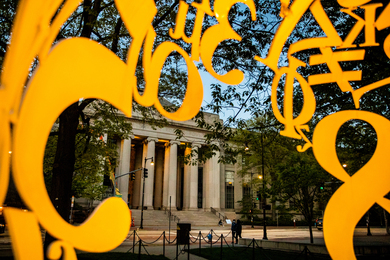
Second round of seed grants awarded to MIT scholars studying the impact and applications of generative AI
- More news on MIT News homepage →
Massachusetts Institute of Technology 77 Massachusetts Avenue, Cambridge, MA, USA
- Map (opens in new window)
- Events (opens in new window)
- People (opens in new window)
- Careers (opens in new window)
- Accessibility
- Social Media Hub
- MIT on Facebook
- MIT on YouTube
- MIT on Instagram
- Open access
- Published: 17 April 2023
Twitter sentiment analysis using hybrid gated attention recurrent network
- Nikhat Parveen 1 , 2 ,
- Prasun Chakrabarti 3 ,
- Bui Thanh Hung 4 &
- Amjan Shaik 2 , 5
Journal of Big Data volume 10 , Article number: 50 ( 2023 ) Cite this article
3544 Accesses
8 Citations
Metrics details
This article has been updated
Sentiment analysis is the most trending and ongoing research in the field of data mining. Nowadays, several social media platforms are developed, among that twitter is a significant tool for sharing and acquiring peoples’ opinions, emotions, views, and attitudes towards particular entities. This made sentiment analysis a fascinating process in the natural language processing (NLP) domain. Different techniques are developed for sentiment analysis, whereas there still exists a space for further enhancement in accuracy and system efficacy. An efficient and effective optimization based feature selection and deep learning based sentiment analysis is developed in the proposed architecture to fulfil it. In this work, the sentiment 140 dataset is used for analysing the performance of proposed gated attention recurrent network (GARN) architecture. Initially, the available dataset is pre-processed to clean and filter out the dataset. Then, a term weight-based feature extraction termed Log Term Frequency-based Modified Inverse Class Frequency (LTF-MICF) model is used to extract the sentiment-based features from the pre-processed data. In the third phase, a hybrid mutation-based white shark optimizer (HMWSO) is introduced for feature selection. Using the selected features, the sentiment classes, such as positive, negative, and neutral, are classified using the GARN architecture, which combines recurrent neural networks (RNN) and attention mechanisms. Finally, the performance analysis between the proposed and existing classifiers is performed. The evaluated performance metrics and the gained value for such metrics using the proposed GARN are accuracy 97.86%, precision 96.65%, recall 96.76% and f-measure 96.70%, respectively.
Introduction
Sentiment Analysis (SA) uses text analysis, NLP (Natural Language Processing), and statistics to evaluate the user’s sentiments. SA is also called emotion AI or opinion mining [ 1 ]. The term ‘sentiment’ refers to feelings, thoughts, or attitudesexpressed about a person, situation, or thing. SA is one of the NLP techniques used to identify whether the obtained data or information is positive, neutral or negative. Business experts frequently use it to monitor or detect sentiments to gauge brand reputation, social data and understand customer needs [ 2 , 3 ]. Over recent years, the amount of information uploaded or generated online has rapidly increased due to the enormous number of Internet users [ 4 , 5 ].
Globally, with the emergence of technology, social media sites [ 6 , 7 ] such as Twitter, Instagram, Facebook, LinkedIn, YouTube etc.,have been used by people to express their views or opinions about products, events or targets. Nowadays, Twitter is the global micro-blogging platform greatly preferred by users to share their opinions in the form of short messages called tweets [ 8 ]. Twitterholds 152 M (million) daily active users and 330 M monthly active users,with 500 M tweets sent daily [ 9 ]. Tweets often effectively createa vast quantity of sentiment data based on analysis. Twitter is an effective OSN (online social network) for disseminating information and user interactions. Twitter sentiments significantly influence diverse aspects of our lives [ 10 ]. SA and text classification aims at textual information extraction and further categorizes the polarity as positive (P), negative (N) or neutral (Ne).
NLP techniques are often used to retrieve information from text or tweet content. NLP-based sentiment classification is the procedure in which the machine (computer) extracts the meaning of each sentence generated by a human. Manual analysis of TSA (Twitter Sentiment Analysis) is time-consuming and requires more experts for tweet labelling. Hence, to overcome these challenges automated model is developed. The innovations of ML (Machine learning) algorithms [ 11 , 12 ],such as SVM (Support Vector Machine), MNB (Multinomial Naïve Bayes), LR (Logistic Regression), NB (Naïve Bayes) etc., have been used in the analysis of online sentiments. However, these methods illustrated good performance, but these approaches are very slow and need more time to perform the training process.
DL model is introduced to classify Twitter sentiments effectively. DL is the subset of ML that utilizes multiple algorithms to solve complicated problems. DL uses a chain of progressive events and permits the machine to deal with vast data and little human interaction. DL-based sentiment analysis offers accurate results and can be applied to various applications such as movie recommendations, product predictions, emotion recognition [ 13 , 14 , 15 ],etc. Such innovations have motivated several researchers to introduce DL in Twitter sentiment analysis.
SA (Sentiment Analysis) is deliberated with recognizing and classifying the polarity or opinions of the text data. Nowadays, people widely share their opinions and sentiments on social sites. Thus, a massive amount of data is generated online, and effectively mining the online data is essential for retrieving quality information. Analyzing online sentiments can createa combined opinion on certain products. Moreover, TSA (Twitter Sentiment Analysis) is challenging for multiple reasons. Short texts (tweets), owing to the maximum character limit, is a major issue. The presence of misspellings, slang and emoticons in the tweets requires an additional pre-processing step for filtering the raw data. Also, selecting a new feature extraction model would be challenging,further impacting sentiment classification. Therefore, this work aims to develop a new feature extraction and selection approach integrated with a hybrid DL classification model for accurate tweet sentiment classification. The existing research works [ 16 , 17 , 18 , 19 , 20 , 21 ] focus on DL-based TSA, which haven’t attained significant results because of smaller dataset usage and slower manual text labelling. However, the datasets with unwanted details and spaces also reduce the classification algorithm’s efficiency. Further, the dimension occupied by extracted features also degrades the efficiency of a DL approach. Hence, to overcome such issues, this work aims to develop a successful DL algorithm for performing Twitter SA. Pre-processing is a major contributor to this architecture as it can enhance DL efficiency by removing unwanted details from the dataset. This pre-processing also reduces the processing time of a feature extraction algorithm. Followed to that, an optimization-based feature selection process was introduced, which reduces the effort of analyzing irrelevant features. However, unlike existing algorithms, the proposed GARN can efficiently analyse the text-based features. Further, combining the attention mechanism with DL has enhanced the overall efficiency of the proposed DL algorithm. As attention mechanism have the greater ability to learn the selected features by reducing the complexity of model. This merit causes the attention mechanism to integrate with RNN and achieved effective performance.
The major objectives of the proposed research are:
To introduce a new deep model Hybrid Mutation-based White Shark Optimizer with a Gated Attention Recurrent Network (HMWSO-GARN) for Twitter sentiment analysis.
The feature set can be extracted with the new Term weighting-based feature extraction (TW-FE) approach named Log Term Frequency-based Modified Inverse Class Frequency (LTF-MICF) is used and compared with traditional feature extraction models.
To identify the polarity of tweets with the bio-inspired feature selection and deep classification model.
To evaluate the performance using different metrics and compare it with traditional DL procedures on TSA.
Related works
Some of the works related to dl-based twitter sentiment analysis are:.
Alharbi et al. [ 16 ] presented the analysis of Twitter sentiments using a DNN (deep neural network) based approach called CNN (Convolutional Neural Network). The classification of tweets was processed based on dual aspects, such as using social activities and personality traits. The sentiment (P, N or Ne) analysis was demonstrated with the CNN model, where the input layer involves the feature lists and the pre-trained word embedding (Word2Vec). The dual datasets used for processing were SemEval-2016_1 and SemEval-2016_2. The accuracy obtained by CNN was 88.46%, whereas the existing methods achieved less accuracy than CNN. The accuracy of existing methods is LSTM (86.48%), SVM (86.75%), KNN (k-nearest neighbour) (82.83%), and J48 (85.44%), respectively.
Tam et al. [ 17 ] developed a Convolutional Bi-LSTM model based on sentiment classification on Twitter data. Here, the integration of CNN-Bi-LSTM was characterized byextracting local high-level features. The input layer gets the text input and slices it into tokens. Each token was transformed into NV (numeric values). Next, the pre-trained WE (word embedding), such as GloVe and W2V (word2vector), were used to create the word vector matrix. The important words were extracted using the CNN model,and the feature set was further minimized using the max-pooling layer. The Bi-LSTM (backwards, forward) layers were utilized to learn the textual context. The dense layer (DeL) was included after the Bi-LSTM layer to interconnect the input data with output using weights. The performance was experimented using datasets TLSA (Twitter Label SA) and SST-2 (Stanford Sentiment Treebank). The accuracy with the TLSA dataset was (94.13%) and (91.13%) with the SST-2 dataset.
Chugh et al. [ 18 ] developed an improved DL model for information retrieval and classification of sentiments. The hybridized optimization algorithm SMCA was the integration of SMO (Spider Monkey Optimization) and CSA (Crow Search Algorithm). The presented DRNN (DeepRNN) was trained using the algorithm named SMCA. Here, the sentiment categorization was processed with DeepRNN-SMCA and the information retrieval was done with FuzzyKNN. The datasets used were the mobile reviews amazon dataset and telecom tweets dataset. Forsentiment classification, the accuracy obtained on the first dataset was (0.967), andthe latter was gained (0.943). The performance with IR (information retrieval) on dataset 1 gained (0.831) accuracy and dataset 2 obtained (0.883) accuracy.
Alamoudi et al. [ 19 ] performed aspect-based SA and sentiment classification aboutWE (word embeddings) and DL. The sentiment categorization involves both ternary and binary classes. Initially, the YELP review dataset was prepared and pre-processed for classification. The feature extraction was modelled with TF-IDF, BoW and Glove WE. Initially, the NB and LR were used for first set feature (TF-IDF, BoW features) modelling; then, the Glove features were modelled using diverse models such as ALBERT, CNN, and BERT for the ternary classification. Next, aspect and sentence-based binary SA was executed. The WE vector for sentence and aspect was done with the Glove approach. The similarity among aspects and sentence vectors was measured using cosine similarity, and binary aspects were classified. The highest accuracy (98.308%) was obtained when executed with the ALBERT model on aYELP 2-class dataset, whereas the BERT model gained (89.626%) accuracy with a YELP 3-class dataset.
Tan et al. [ 20 ] introduced a hybrid robustly optimized BERT approach (RoBERTa) with LSTM for analyzing the sentiment data with transformer and RNN. The textual data was processed with word embedding, and tokenization of the subwordwas characterized with the RoBERTa model. The long-distance Tm (temporal) dependencies were encoded using the LSTM model. The DA (data augmentation) based on pre-trained word embedding was developed to synthesize multiple lexical samples and present the minority class-based oversampling. Processing of DA solves the problem of an imbalanced dataset with greater lexical training samples. The Adam optimization algorithm was used to perform hyperparameter tuning,leading to greater results with SA. The implementation datasets were Sentiment140,Twitter US Airline,and IMDb datasets. The overall accuracy gained with these datasets was 89.70%, 91.37% and 92.96%, respectively.
Hasib et al. [ 21 ] proposed a novel DL-based sentiment analysis of Twitter data for the US airline service. The Twitter tweet is collected from the Kaggle dataset: crowdflowerTwitter US airline sentiment. Two models are used for feature extraction:DNN and convolutional neural network (CNN). Before applying four layers, the tweets are converted to metadata and tf-idf. The four layers of DNN aretheinput, covering, and output layers. CNN for feature extraction is by the following phases; data pre-processing, embedded features, CNN and integration features. The overall precision is 85.66%, recall is 87.33%, and f1-score is 87.66%, respectively. Sentiment analysis was used to identify the attitude expressed using text samples. To identify such attitudes, a novel term weighting scheme was developed by Carvalho and Guedes in [ 24 ], which was an unsupervised weighting scheme (UWS). It can process the input without considering the weighting factor. The SWS (Supervised Weighting Schemes) was also introduced, which utilizes the class information related to the calculated term weights. It had shown a more promising outcome than existing weighting schemes.
Learning from online courses are considered as the mainstream of learning domain. However, it was identified that analysing the users comments are considered as the major key for enhancing the efficiency and quality of online courses. Therefore, identifying sentiments from the user’s comments were considered as the efficient process for enhancing the learning process of online course. By taking this as major goal, an ensemble learning architecture was introduced by Pu et al. in [ 34 ] which utilizes glove, and Word2Vec for obtaining vector representation. Then, the extraction of deep features was achieved using CNN (Convolutional neural network) and bidirectional long and short time network (Bi-LSTM). The integration of suggested models were achieved using ensemble multi-objective gray wolf optimization (MOGWO). It achieves 91% f1-score value.
The sentiment dictionaries use binary sentiment analysis like BERT, word2vec and TF-IDF were used to convert movie and product review into vectors. Three-way decision in binary sentiment analysis separates the data sample into uncertain region (UNC), positive (POS) region and Negative (NEG) region. UNC got benefit from this three-way decision model and enhances the effect of binary sentiment analysis process. For the optimal feature selection, Chen, J et al. [ 35 ] developed a three-way decision model which get the optimal features representation of positive and negative domains for sentiment analysis. Simulation was done in both Amazon and IMDB database to show the effectiveness of the proposed methodology.
The advancements in biga data analytics (BDA) model is obtained by the people who generate large amount of data in their day-to-day live. The linguistic based tweets, feature extraction and sentimental texts placed between the tweets are analysed by the sentimental analysis (SA) process. In this article, Jain, D.K et al. [ 36 ] developed a model which contains pre-processing, feature extraction, feature selection and classification process. Hadoop Map Reduce tool is used to manage the big data, then pre-processing method is initiated to remove the unwanted words from the text. For feature extraction, TF-IDF vector is utilized and Binary Brain Storm Optimization (BBSO) is used to select the relevant features from the group of vectors. Finally, the incidence of both positive and negative sentiments is classified using Fuzzy Cognitive Maps (FCMs). Table 1 shows the comparative analysis of Twitter sentiment analysis using DL techniques.
Problem statement
There are many problems related to twitter sentiment analysis using DL techniques. The author in [ 16 ] has used the DL model and performed the sentiment classification from Twitter data. To classify such data, this method analysed each user’s behavioural information. However, this method has faced struggles in interpreting exact tweet words from the massive tweet corpus; due to this, the efficiency of a classification algorithm has been reduced.ConvBiLSTM was introduced in [ 17 ], which used glove and word2vec-based features for sentiment classification. However, the extracted features are not sufficient to achieve satisfactory accuracy. Then, processing time reduction was considered a major objective in [ 18 ], which utilizes DeepRNN for sentiment classification. But it fails to reduce the dimension occupied by the extracted features. This makes several valuable featuresfall within the local optimum. DL and word embedding processes were combined in [ 19 ], which utilizes Yelp reviews for processing. It has shown efficient performance for two classes but fails to provide better accuracy for three-class classification. Recently, a hybrid LSTM architecture was developed in [ 20 ], which has shown flexible processing over sentiment classification and takes a huge amount of time to process large datasets. DNN-based feature extraction and CNN-based sentiment classification were performed in [ 21 ], which haven’t shown more efficient performance than other algorithms. Further, it also concentrated only on 2 classes.
Few of the existing literatures fails to achieve efficient processing time, complexity and accuracy due to the availability of large dataset. Further, the extraction of low-level and unwanted features reduces the efficiency of classifier. Further, the usage of all extracted features occupies large dimension. These demerits makes the existing algorithms not suitable for efficient processing. This shortcomings open a research space for efficient combined algorithm for twitter data analysis. To overcome such issue, the proposed architecture has combined RNN and attention mechanism. The features required for classification is extracted using LTF-MICF which provides features for twitter processing. Then, the dimension occupied by huge extracted features are reduced using HMWSO algorithm. This algorithm has the ability to process the features in less time complexity and shows better optimal feature selection process. This selected features has enhanced the performance of proposed classifier over the large dataset and also achieved efficient accuracy with less misclassification error rate.
Proposed methodology
For sentiment classification of Twitter tweets, a DL technique of gated attention recurrent network (GARN) is proposed. The Twitter dataset (Sentiment140 dataset) with sentiment tweets that the public can access is initially collected and given as input. After collecting data, the next stage is pre-processing the tweets. In the pre-processing stage, tokenization, stopwords removal, stemming, slang and acronym correction, removal of numbers, punctuations &symbol removal, removal of uppercase and replacing with lowercase, character &URL, hashtag & user mention removal are done. Now the pre-processed dataset act as input for the next process. Based on term frequency, a term weight is allocated for each term in the dataset using the Log Term Frequency-based Modified Inverse Class Frequency (LTF-MICF) extraction technique. Next, Hybrid Mutation based White Shark Optimizer (HMWSO) is used to select optimal term weight. Finally, the output of HMWSO is fed into the gated attention recurrent network (GARN) for sentiment classification with three different classes. Figure 1 shows a diagrammatic representation of the proposed methodology.
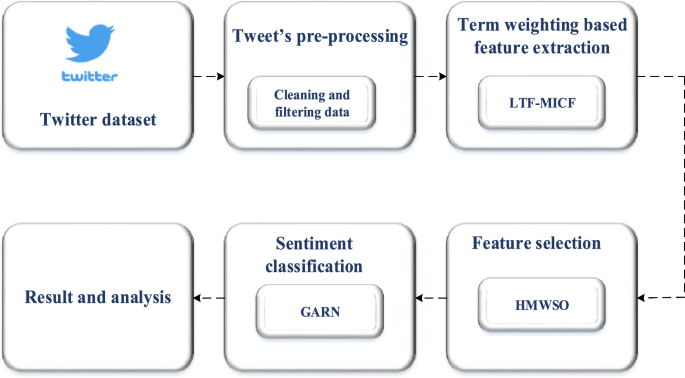
Architecture diagram
Tweets pre-processing
Pre-processing is converting the long data into short text to perform other processes such as classification, detecting unwanted news, sentiment analysis etc., as Twitter users use different styles to post their tweets. Some may post the tweet in abbreviations, symbols, URLs, hashtags, and punctuations. Also, tweets may consist of emojis, emoticons, or stickers to express the user’s sentiments and feelings. Sometimes the tweets may be in a hybrid form,such as adding abbreviations, symbols and URLs. So these kinds of symbols, abbreviations, and punctuations should be removed from the tweet toclassify the dataset further. The features to be removed from the tweet dataset are tokenization, stopwords removal, stemming, slag and acronym correction, removal of numbers, punctuation and symbol removal, noise removal, URL, hashtags, replacing long characters, upper case to lower case, and lemmatization.
Tokenization
Tokenization [ 28 ] is splitting a text cluster into small words, symbols, phrases and other meaningful forms known as tokens. These tokens are considered as input for further processing. Another important use of tokenization is that it can identify meaningful words.The tokenization challenge depends only on the type of language used. For example, in languages such as English and French, some words may be separated by white spaces. Other languages, such as Chinese and Thai words,are not separated. The tokenization process is carried out in the NLTK Python library. In this phase, the data is processed in three forms: convert the text document into word counts. Secondly,data cleansing and filtering occur, andfinally, the document is split into tokens or words.
The example provided below illustrates the original tweet before and after performing tokenization:
Before tokenization
DLis a technology which trains the machineto behave naturally like a human being.
After tokenization
Deep, learning, is, a, technology, which, train, the, machine, to, behave, naturally, like, a, human, being.
Numerous tools are available to tokenize a text document. Some of them are as follows;
NLTK word tokenize
Nlpdotnet tokenizer
TextBlob word tokenize
Mila tokenizer
Pattern word tokenize
MBSP word tokenize
Stopwords removal
Stopword removal [ 28 ] is a process of removing frequently used words with meaningless in a text document. Stopwords such as are, this, that, and, so are frequently occurring words in a sentence. These words are also termed pronouns, articles and prepositions. Such words are not used forfurther processing, so removing those words is required. If such words are not removed, the sentence seems heavy and becomes less important for the analyst.Also, they are not considered keywords in Twitter analysis applications. Many methods exist to remove stopwords from a document; they are.
Classic method
Mutual information (MI) method
Term based random sampling (TBRS) method
Removing stopwords from a pre-compiled list is performed using a classic-based method. Z-methods are known as Zipf’s law-based methods. In Z-methods, three removal processes occur: removing the most frequently used words, removing the words which occur once in a sentence, and removing words with a document frequency of low inverse. In the mutual MI method, the information with low mutual will be removed. In the TBRS method, the words are randomly chosen from the document and given rank for a particular term using the Kullback–Leibler divergence formula, which is represented as;
where \(Q_{l} (t)\) is the normalized term frequency (NTF) of the term \(t\) within a mass \(l\) , and NTF is denoted as \(Q(t)\) of term \(t\) in the entire document. Finally, using this equation, the least terms are considered a stopword list from which the duplications are removed.
Removing prefixesand suffixes from a word is performed using the stemming method. It can also be defined as detecting the root and stem of a word and removing them. For example, processed word processing can be stemmed from a single word as a process [ 28 ]. The two points to be considered while performing stemming are: the words with different meanings must be kept separate, and the words of morphological forms will contain the same meaning and must be mapped with a similar stem. There are stemming algorithms to classify the words. The algorithms are divided into three methods: truncating, statistical, and mixed methods. Truncating method is the process of removing a suffix from a plural word. Some rules must be carried out to remove suffixes from the plurals to convert the plural word into the singular form.
Different stemmer algorithms are used under the truncating method. Some algorithms are Lovins stemmer, porters stemmer, paice and husk stemmer, and Dawson stemmer. Lovins stemmer algorithm is used to remove the lengthy suffix from a word. The drawback of using this stemmer is that it consumes more time to process. Porter’s stemmer algorithm removes suffixes from a word by applying many rules. If the applied rule is satisfied, the suffix is automatically removed. The algorithm consists of 60 rules and is faster than theLovins algorithm. Paice and husk is an iterative algorithm that consists of 120 rules to remove the last character of the suffixed word. This algorithm performs two operations, namely, deletion and replacement. The Dawson algorithm keeps the suffixed words in reverse order by predicting their length and the last character. In statistical methods, some algorithms are used: N-gram stemmer, HMM stemmer, and YASS stemmer. In a mixed process, the inflectional and derivational methods are used.
Slang and acronym correction
Users typically use acronyms and slang to limit the characters in a tweet posted on social media [ 29 ]. The use of acronyms and slangis an important issue because the users do not have the same mindset to make the acronym in the same full form, and everyone considers the tweet in different styles or slang. Sometimes, the acronym posted may possess other meanings or be associated with other problems. So, interpreting these kinds of acronyms and replacing them with meaningful words should be done so the machine can easily understand the acronym’s meaning.
An example illustrates the original tweet with acronyms and slang before and after removal.
Before removal : ROM permanently stores information in the system, whereas RAM temporarily stores information in the system.
After removal : Read Only Memory permanently store information in the system, whereas Random Access Memory temporarily store information in the system.
Removal of numbers
Removal of numbers in the Twitter dataset is a process of deleting the occurrence of numbers between any words in a sentence [ 29 ].
An example illustrates the original tweet before and after removing numbers.
Before removal : My ink “My Way…No Regrets” Always Make Happiness Your #1 Priority.
After removal : My ink “My Way … No Regrets” Always Make Happiness Your # Priority.
Once removed, the tweet will no longer contain any numbers.
Punctuation and symbol removal
The punctuation and symbols are removed in this stage. Punctuations such as ‘.’, ‘,’, ‘?’, ‘!’, and ‘:’ are removed from the tweet [ 29 , 30 ].
An example illustrates the original tweet before and after removing punctuation marks.
After removal : My ink My Way No Regrets Always Make Happiness Your Priority.
After removal, the tweet will not contain any punctuation. Symbol removal is the process of removing all the symbols from the tweet.
An example illustrates the original tweet before and after removing symbols.
After removal : wednesday addams as a disney princess keeping it.
After removal, there would not be any symbols in the tweet.
Removal of uppercase into lowercase character
In this process of removal or deletion, all the uppercase charactersare replaced with lowercase characters [ 30 ].
An example illustrates the original tweet before and after removing uppercase characters into lowercase characters.
After removal : my ink my way no regrets always make happiness your priority.
After removal, the tweet will no longer contain capital letters.
URL, hashtag & user mention removal
For clear reference,Twitter users post tweets with various URLs and hashtags [ 29 , 30 ]. This information ishelpful for the people but mostly noise, which cannot be used for further processes. The example provided below illustrates the original tweet with URL, hashtag and user mention before removal and after removal:
Before removal : This gift is given by #ahearttouchingpersonfor securing @firstrank. Click on the below linkto know more https://tinyurl.com/giftvoucher .
After removal : This is a gift given by a heart touching person for securing first rank. Click on the below link to know more.
Term weighting-based feature extraction
After the pre-processing, the pre-processed data is extracted in text documents based on the term weighting \(T_{w}\) [ 22 ]. A new term weighting scheme,Log term frequency-based modified inverse class frequency (LTF-MICF), is employed in this research paper for feature extraction based on term weight. The technique integrates two different term weighting schemes: log term frequency (LTF) and modified inverse class frequency (MICF). The frequently occurring terms in the document are known as term frequency \(^{f} T\) . But, \(^{f} T\) alone is insufficient because the frequently occurring terms will possess heavyweight in the document. So, the proposed hybrid feature extraction technique can overcome this issue. Therefore, \(^{f} T\) is integrated with MICF, an effective \(T_{w}\) approach. Inverse class frequency \(^{f} C_{i}\) is the inverse ratio of the total class of terms that occurs on training tweets to the total classes. The algorithm for the TW-FE technique is shown in algorithm 1 [ 22 ].
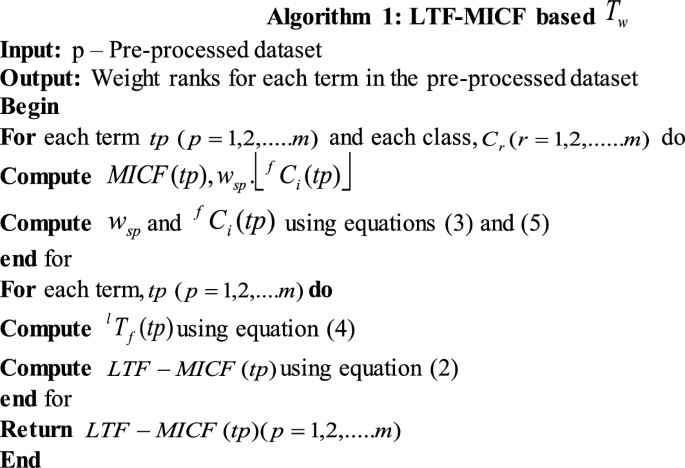
Two steps are involved in calculating LTF \(^{l} T_{f}\) . The first step is to calculate the \(^{f} T\) of each term in the pre-processed dataset. The second step is, applying log normalization to the output of the computed \(^{f} T\) data. The modified version of \(^{f} C_{i}\) , the MICF is calculated for each term in the document. MICF is said to be executed then;each term in the document should have different class-particular ranks, which should possess differing contributions to the total term rank. It is necessary to assign dissimilar weights for dissimilar class-specific ranks. Consequently, the sum of the weights of all class-specific ranks is employed as the total term rank. The proposed formula for \(T_{w}\) using LTF-based MICF is represented as follows [ 22 ];
where a specific weighting factor is denoted \(w_{sp}\) for each \(tp\) for class \(C_{r}\) , which can be clearly represented as;
The method used to assign a weight for a given dataset is known as the weighting factor (WF). Where the number of tweets \(s_{i}\) in class \(C_{r}\) which contains pre-processed terms \(tp\) is denoted as \(s_{i} \mathop{t}\limits^{\rightharpoonup}\) . The number of \(s_{i}\) in other classes, which contains \(tp\) is denoted as \(s_{i} \mathop{t}\limits^{\leftarrow}\) . The number of \(s_{i}\) in-class \(C_{r}\) , which do not possess, \(tp\) is denoted as \(s_{i} \overset{\lower0.5em\hbox{$\smash{\scriptscriptstyle\frown}$}}{t}\) . The number of \(s_{i}\) in other classes, which do not possess, \(tp\) is denoted as \(s_{i} \tilde{t}\) . To eliminate negative weights, the constant ‘1’ is used. In extreme cases, to avoid a zero-denominator issue, the minimal denominator is set to ‘1’ if \(s_{i} \mathop{t}\limits^{\leftarrow}\) = 0 or \(s_{i} \overset{\lower0.5em\hbox{$\smash{\scriptscriptstyle\frown}$}}{t}\) = 0. The formula for \(^{l} T_{f} (tp)\) and \(^{f} C_{i} (tp)\) can be presented as follows [ 22 ];
where raw count of \(tp\) on \(s_{i}\) is denoted as \(^{f} T(tp,s_{i} )\) , i.e., the total times of \(tp\) occurs on \(s_{i}\) .
where \(r\) refers to the total number of classes in \(s_{i}\) , and \(C(tp)\) is the total number of classes in \(tp\) . The dataset features are represented as \(f_{j} = \left\{ {f_{1} ,f_{2} ,..........f_{3} ,......f_{m} } \right\}\) after \(T_{w}\) , where the number of weighted terms in the pre-processed dataset is denoted as \(f_{1} ,f_{2} ,...f_{3} ,...f_{m}\) respectively. The computed rank values of each term in the text document of tweets are used for performing the further process.
Feature selection
The existence of irrelevant features in the data can reduce the accuracy level of the classification process and make the model to learn those irrelevant features. This issue is termed as the optimization issue. This issue can be ignored only by taking optimal solutions from the processed dataset. Therefore, a feature selection algorithm named White shark optimizer with a hybrid mutation strategy is utilized to achieve a feature selection process.
White Shark Optimizer (WSO)
WSO is proposed based on the behaviour of the white shark while foraging [ 23 ]. Great white shark in the ocean catches prey by moving the waves and other features to catch prey kept deep in the ocean. Since the white shark catch prey based on three behaviours, namely: (1) the velocity of the shark in catching the prey, (2) searching for the best optimal food source, (3) the movement of other sharks toward the shark, which is near to the optimal food source. The initial white shark population is represented as;
where \(W_{q}^{p}\) is the initial parameters of the \(p_{th}\) white shark in the \(q_{th}\) dimension. The upper and lower bounds in the \(q_{th}\) dimension are denoted as \(up_{q}\) and \(lb_{q}\) , respectively. Whereas \(r\) denotes a random number in the range [0, 1].
The white shark’s velocity is to locate the prey based on the motion of the sea wave is represented as [ 23 ];
where \(s = 1,2,....m\) is the index of a white shark with a population size of \(m\) . The new velocity of \(p_{th}\) shark is denoted as \(vl_{s + 1}^{p}\) in \((s + 1)_{th}\) step. The initial speed of the \(p_{th}\) shark in the \(s_{th}\) step is denoted as \(vl_{s}^{p}\) . The global best position achieved by any \(p_{th}\) shark in \(s_{th}\) step is denoted as \(W_{{gbest_{s} }}\) . The initial position of the \(p_{th}\) shark in \(s_{th}\) step is denoted as \(W_{s}^{p}\) . The best position of the \(p_{th}\) shark and the index vector on attainingthe best position are denoted as \(W_{best}^{{vl_{s}^{p} }}\) and \(vc^{i}\) . Where \(C_{1}\) and \(C_{2}\) in the equation is defined as the creation of uniform random numbers of the interval [1, 0]. \(F_{1}\) and \(F_{2}\) are the force of the shark to control the effect of \(W_{{gbest_{s} }}\) and \(W_{best}^{{vl_{s}^{p} }}\) on \(W_{s}^{p}\) . \(\mu\) represents to analyze the convergence factor of the shark. The index vector of the white shark is represented as;
where \(rand(1,t)\) is a random numbers vector obtained with a uniform distribution in the interval [0, 1].The forces of the shark to control the effect are represented as follows;
The initial and maximum sum of the iteration is denoted as \(u\) and \(U\) , whereas the white shark’s current and sub-ordinate velocities are denoted as \(F_{\min }\) and \(F_{\max }\) . The convergence factor is represented as;
where \(\tau\) is defined as the acceleration coefficient. The strategy for updating the position of the white shark is represented as follows;
The new position of the \(p_{th}\) shark in \((s + 1)\) iteration, \(\neg\) represent the negation operator, \(c\) and \(d\) represents the binary vectors. The search space lower and upper bounds are denoted as \(lo\) and \(ub\) . \(W_{0}\) and \(fr\) denotes the logical vector and frequency at which the shark moves. The binary and logic vectors are expressed as follows;
The frequency at which the white shark moves is represented as;
\(fr_{\max }\) and \(fr_{\min }\) represents the maximum and minimum frequency rates. The increase in force at each iteration is represented as;
where \(MV\) represents the weight of the terms in the document.
The best optimal solution is represented as;
where the position updation following the food source of \(p_{th}\) the white shark is denoted as \(W_{s + 1}^{\prime p}\) . The \({\text{sgn}} (r_{2} - 0.5)\) produce 1 or −1 to modify the search direction. The food source and shark distance \(\vec{D}is_{w}\) and the strength of the white shark following other sharks close to the food source \(Str_{sns}\) is formulated as follows;
The initial best optimal solutions are kept constant, and the position of other sharks is updated according to these two constant optimal solutions. The fish school behaviour of the sharks is formulated as follows;
The weight factor \(^{j} we\) is represented as;
where \(^{q} fit\) is defined as the fitness of each term in the text document. The expansion of the equation is represented as;
The concatenation of hybrid mutation \(HM\) is applied to the WSO for a faster convergence process. Thus, the hybrid mutation applied with the optimizer is represented as;
whereas \(G_{a} (\mu ,\sigma )\) and \(C_{a} (\mu ,\sigma )\) represents an arbitrary number of both Gaussian and Cauchy distribution. \((\mu ,\sigma )\) and \((\mu^{\prime},\sigma^{\prime})\) represents the mean and variance function of both Gaussian and Cauchy distributions. \(D_{1}\) and \(D_{2}\) represents the coefficients of Gaussian \(^{t + 1} GM\) along with Cauchy \(^{t + 1} CM\) mutation. On applying these two hybrid mutation operators, a new solution is produced that is represented as;
whereas \(^{p}_{we}\) represents the weight vector and \(PS\) represents the size of the population. The selected features from the extracted features are represented as \(Sel(p = 1,2,...m)\) . The WSO output is denoted as \((sel) = \left\{ {sel^{1} ,sel^{2} ,.....sel^{m} } \right.\left. {} \right\}\) ,which is a new sub-group of terms in the dataset. At the same time, \(m\) denotes a new number of each identical feature. Finally, the feature selection stage provides a dataset document with optimal features.
Gated attention recurrent network (GARN) classifier
GARN is a hybrid network of Bi-GRU with an attention mechanism. Many problems occur due to the utilization of recurrent neural network (RNNs) because it employs old information rather than the current information for classification. To overcome this problem, a bidirectional recurrent neural network (BRNN) model is proposed, which can utilize both old and current information. So, to perform both the forward and reverse functions, two RNNs are employed. The output will be connected to a similar output layer to record the feature sequence. Based on the BRNN model, another bidirectional gated recurrent unit (Bi-GRU) model is introduced, which replaces the hidden layer of the BRNN with a single GRU memory unit. Here, the hybridization of both Bi-GRU with attention is considered agated attention recurrent network (GARN) [ 25 ] and its structure is given in Fig. 2 .
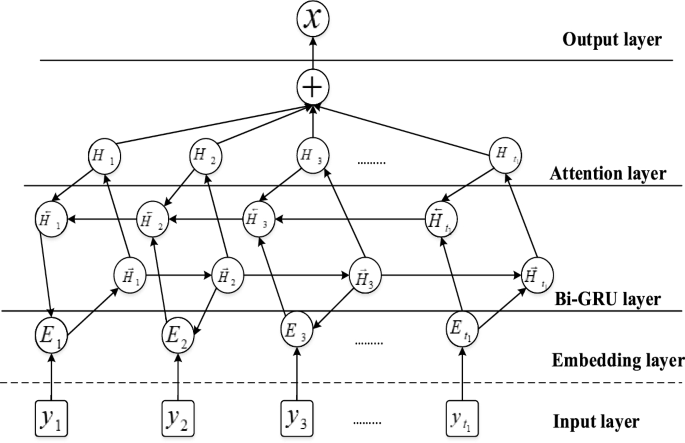
Structure of GARN
Consider an m-dimensional input data as \((y_{1} ,y_{2} ,....,y_{m} )\) . The hidden layer in the BGRU produces an output \(H_{{t_{1} }}\) at a time interval \(t_{1}\) is represented as;
where the weight factor for two connecting layers is denoted as \(w_{e}\) , \(c\) is the bias vector, \(\sigma\) represents the activation function, positive and negative outputs of GRU is denoted as \(\vec{H}_{{t_{1} }}\) and \(\overleftarrow {H} _{{t_{1} }}\) , \(\oplus\) is a bitwise operator.
Attention mechanism
In sentiment analysis, the attention module is very important to denote the correlation between the terms in a sentence and the output [ 26 ]. For direct simplification, an attention model is used in this proposal named as feed-forward attention model. This simplification is to produce a single vector \(\nu\) from the total sequence represented as;
Where \(\beta\) is a learning function and is identified using \(H_{{t_{1} }}\) . From the above Eq. 34 , the attention mechanism produces a fixed length for the embedding layer in a BGRU model for every single vector \(\nu\) by measuring the average weight of the data sequence \(H\) . The structure for attention mechanism is shown in Fig. 3 . Therefore, the final sub-set for the classification is obtained from:
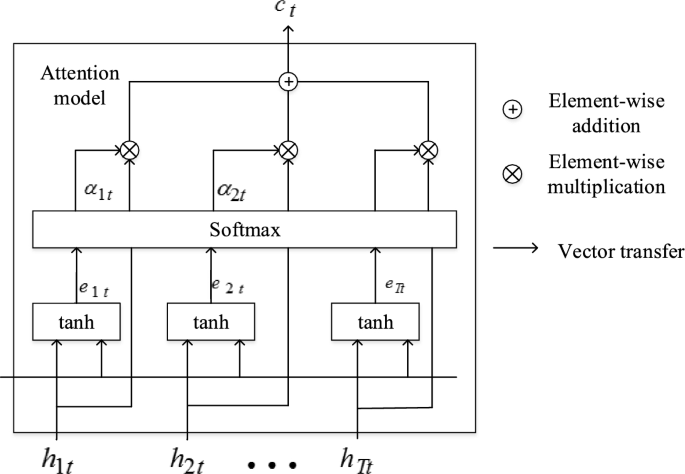
Structure of attention mechanism
Sentiment classification
Twitter sentiment analysis is formally a classification problem. The proposed approach classifies the sentiment data into three classes: positive, negative and neutral. For classification, the softmax classifier is used to classify the output in the hidden layer \(H^{\# }\) is represented as;
where \(w_{e}\) is the weight factor, \(c\) is a bias vector and \(H^{\# }\) is the output of the last hidden layer. Also, the cross-entropy is evaluated as a loss function represented as;
The total number of samples is denoted as, \(n\) . The real category of the sentence is denoted as \(sen_{j}\) ,the sentence with the predictive category is denoted as \(x_{j}\) , and the \(L2\) regular item is denoted as \(\lambda ||\theta ||^{2}\) .
Results and discussion
This section briefly describes the performance metrics like accuracy, precision, recall and f-measure. The overall analysis of the Twitter sentiment classification with pre-processing, feature extraction, feature selection and classification are also analyzed and discussed clearly. Results on comparing the existing and trending classifiers with term weighting schemes in bar graphs and tables are included. Finally, a small discussion about the overall workflow concluded the research by importing the analyzed performance metrics. The sentiment is an expression from individuals based on an opinion on any subject. Tweet-based analysis of sentiment mainly focuses on detecting positive and negative sentiments. So, it is necessary to enhance the classification classes in which a neutral class is added to the datasets.
The dataset utilized in our proposed work is Sentiment 140, gathered from [ 27 ], which contains 1,600,000tweets extracted from Twitter API. The score values for each tweet as, for positive tweets, the rank value is 4.Similarly, for negative tweets rank value is 0, and for neutral tweets, the rank value is 2.The total number of positive tweets in a dataset is 20832, neutral tweets are 18318, negative tweets are 22542, and irrelevant tweets are 12990. From the entire dataset, 70%is used for training, 15% for testing and 15% for validation. Table 2 shows the system configuration of the designed classifier.
Performance metrics
In this proposed method, 4 different weight schemes are compared with other existing,proposed classifiers in which the performance metrics are precision, f1-score, recall and accuracy. Four notations, namely, true-positive \((t_{p} )\) , true-negative \((t_{n} )\) , false-positive \((f_{p} )\) and false-negative, \((f_{n} )\) are particularly utilized to measure the performance metrics.
Accuracy \((A_{c} )\)
Accuracy is the dataset’s information accurately being classified by the proposed classifier. The accuracy value for the proposed method is obtained using Eq. 39 .
Precision \((P_{r} )\)
Precision is defined as the number of terms accurately identified positive to the total identified positively. The precision value for the proposed method is obtained using Eq. 40 .
Recall \((R_{e} )\)
The recall is defined as the percentage of accurately identified positive observations to the total observations in the dataset. The recall value for the proposed method is obtained using Eq. 41 .
F1-score \((F_{s} )\)
F1-score is defined as the average weight of recall and precision. The f1-score value for the proposed method is obtained using Eq. 42 .
Analysis of Twitter sentiments using GARN
The research paper mainly focuses on classifying Twitter sentiments in the form of three classes, namely, positive, negative and neutral. The data are collected using Twitter api. After collecting data, it is given as input for pre-processing. The unwanted symbols are removed in the pre-processing technique, giving a new pre-processed dataset. Now, the pre-processed dataset is given as an input to extract the required features. These features are extracted from the pre-processed dataset using a novel technique known as the log term frequency-based modified inverse class frequency (LTF-MICF) model, which integrates two-weight schemes, LTF and MICF. Here, the required features are extracted in which the extracted features are given as input to select an optimal feature subset. The optimized feature subset is selected using a hybrid mutation-based white shark optimizer (HMWSO). The mutation is referred to as the Cauchy mutation and the Gaussian mutation. Finally, with the selected feature sub-set as input, the sentiments are classified under three classes using a classifier named gated recurrent attention recurrent network (GARN), which is a hybridization of Bi-GRU with an attention mechanism.
The evaluated value of the proposed GARN is preferred for classifying the sentiments of Twitter tweets. The suggested GARN model is implemented in the Python environment, and the sentiment140 Twitter dataset is utilized for training the proposed model. To evaluate the efficiency of the classifier, the proposed classifier is compared with existing classifiers, namely, CNN (Convolutional neural network), DBN (Deep brief neural network), RNN (Recurrent neural network), and Bi-LSTM (Bi-directional long short term memory). Along with these classifiers, the proposed term weighting scheme (LTF-MICF) with the existing term weighting schemes TF (Term Frequency), TF-IDF (Term-frequency-inverse document frequency), TF-DFS (Term-frequency-distinguishing feature selector), and W2V (Word to vector) are also analyzed. The performance was evaluated for both sentiment classification with an optimizer and without using an optimizer. The metrics evaluated are accuracy, precision, recall and f1-score, respectively.The existing methods implemented and proposed (GARU) are Bi-GRU, RNN, Bi-LSTM, and CNN. The simulation parameters used for processing the proposed and existing methods are discussed in Table 3 . This comparative analysis is performed to show the efficiency of a proposed over the other related existing algorithms.
Figure 4 compares the accuracy of the GARN with the existing classifiers. The accuracy obtained by existing Bi-GRU, Bi-LSTM, RNN, and CNN for the LTF-MICF is 96.93%, 95.79%, 94.59% and 91.79%. In contrast, the proposed GARN classifier achieves an accuracy of 97.86% and is considered the best classifier with the LTF-MICF term weight scheme for classifyingTwitter sentiments. But when the proposed classifier is compared with other term weighting schemes,TF-DFS, TF-IDF, TF and W2V, the accuracy obtained is 97.53%, 97.26%, 96.73% and 96.12%. Therefore, the term weight scheme withthe GARN classifier is the best solution for classification problems. Table 4 contains the accuracy values attained by four existing classifiers and the proposed classifier with four existing term weight schemes and proposed term weight scheme.
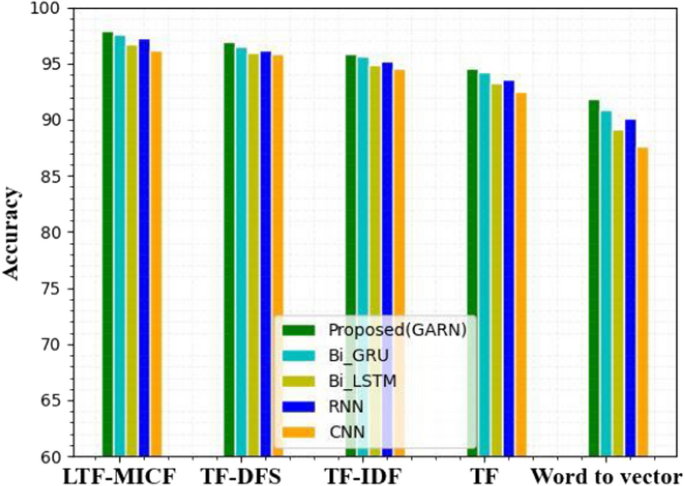
Accuracy of the classifiers with term weight schemes
Figure 5 shows the precision performance analysis with the proposed and four existing classifiers for different term weight schemes. The precision of all existing classifiers with other term weight schemes is less than the proposed term weighting scheme. In Bi-GRU, the precision obtained by TF-DFS, TF-IDF, TF and W2V is 94.51%, 94.12%, 93.76% and 93.59%. But, when Bi-GRU is compared with the LTF-MICF term weight scheme, the precision level is increased by 95.22%. The precision achieved by the suggested method GARN with TF-DFS, TF-IDF, TF and W2V is 96.03%, 95.67%, 94.90% and 93.90%. Whereas, when the GARN classifier is compared with the suggested term weighting scheme LTF-MICF the precision achieved is 96.65%, which is considered the best classifier with the best term weighting scheme. Figure 5 shows that the GARN classifier with the LTF-MICF term weighting scheme achieved the highest precision level compared with other classifiers and term weighting schemes.Table 5 indicates the precision performance analysis for existing and proposed classifiers with term weight schemes.
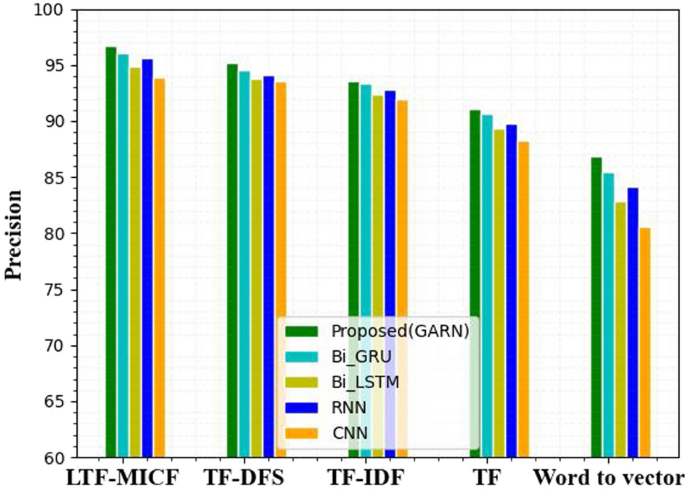
Precision of the classifiers with term weight schemes
The analysis graph of Fig. 6 shows the f-measure of the four prevalent classifiers and suggested classifiers with different term weight schemes. The f-measure of all the prevalent classifier with other term weight schemes are minimum compared to the suggested term weighting scheme. In Bi-LSTM, the f-measure gained with TF-DFS, TF-IDF, TF and W2V is93.34%, 92.77%, 92.28% and 91.89%. Compared with LTF-MICF, the f-measure level is improved by 95.22%. The f-measure derived by the advance GARN with TF-DFS, TF-IDF, TF and W2V is 96.10%, 95.65%, 94.90% and 94.00%. When GARN is compared with the advanced LTF-MICF scheme, the f-measure grows by 96.70%, which is considered the leading classifier with the supreme term weighting scheme. Therefore, from Fig. 6 , the GARN model with the LTF-MICF scheme achieved the greatest f-measure level compared with other DL models and term weighting schemes.Table 6 indicates the performance analysis of the f-measure for both prevalent and suggested classifiers with term weight schemes.
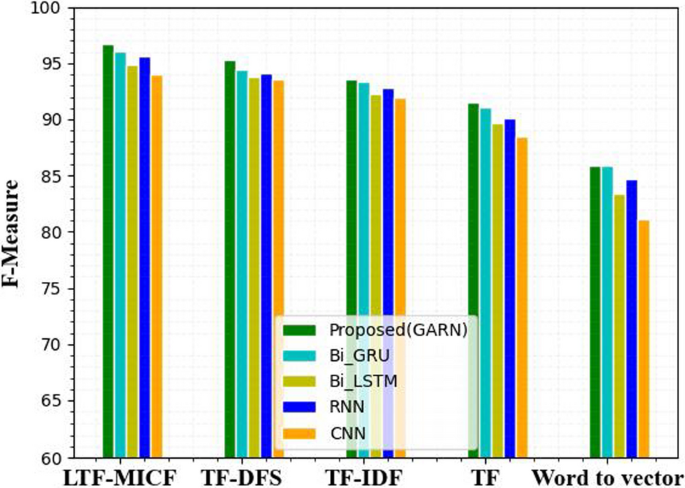
F-measure of the classifiers with term weight schemes
Figure 7 illustrates the recall of the four previously discovered DL models andthe recommended model of dissimilar term weight schemes. The recall of the previously discovered classifier with other term weight schemes is reduced compared to the novel term weighting scheme. In RNN, the recall procured with TF-DFS, TF-IDF, TF and W2V is 91.83%, 90.65%, 90.36% and 89.04%. In comparison with LTF-MICF, the recall value is raised by 92.25%. The recall acquired by the invented GARN with TF-DFS, TF-IDF, TF and W2V is 96.23%, 95.77%, 94.09% and 94.34%. Comparing GARN with the advanced LTF-MICF scheme maximizes recall by 96.76%,which is appraised as the prime classifier with an eminent term weighting scheme. Therefore, from Fig. 7 , the GARN model with the LTF-MICF scheme securedextraordinaryrecallvalue when differentiated from other DL models and term weighting schemes. Table 7 indicates the recall performance analysis for the previously discovered and recommended classifiers with term weight schemes.
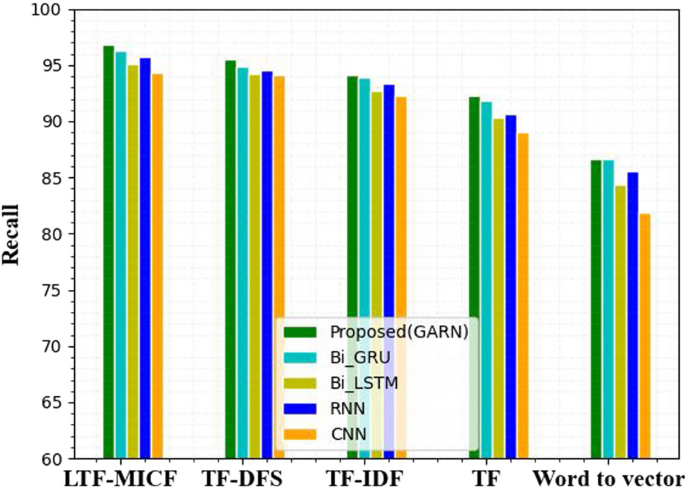
Recall of the classifiers with term weight schemes
The four stages employed to implement this proposed work are Twitter data collection, tweet pre-processing, term weighting-based feature extraction, feature selection and classification of sentiments present in the tweet. Initially, the considered tweet sentiment dataset is subjected to pre-processing.Here, tokenization, stemming, punctuations, symbols, numbers, hashtags, and acronyms are removed. After removal, a clean pre-processed dataset is obtained. The performance achieved by proposed and existing methods for solving proposed objective is discussed in Table 8 .
Using this pre-processed dataset, a term weighting-based feature extraction is done using an integrated terms weight scheme such as LTF and MICF as a novel term weighting scheme technique named LTF-MICF technique. An optimization algorithm, HMWSO, with two hybrid mutation techniques, namely Cauchy and Gaussian mutation, is chosen for feature selection. Finally, the GARN classifier is used for the classification of Twitter sentiments. The sentiments are classified as positive, negative and neutral. The performance of existing classifiers with term weighting schemes and the proposed classifier with term weighting schemes are analyzed. The performance comparison between the proposed and existing methods is shown in Table 9 . The existing details are collected from previous works developed for sentiment analysis from theTwitter dataset.
Many DL techniques use only a single feature extraction technique, namely term frequency (TF) and distinguishing feature selector (DFS), which will not accurately extract the features. The proposed methods without optimization can diminish the proposed model’s accuracy level. The feature extraction technique used in our proposed work will perform greatly because it can extract features from frequently occurring terms in the document. The proposed work uses an optimization algorithm to increase the accuracy level of the designed model.The achieved results are shown in Fig. 8 .
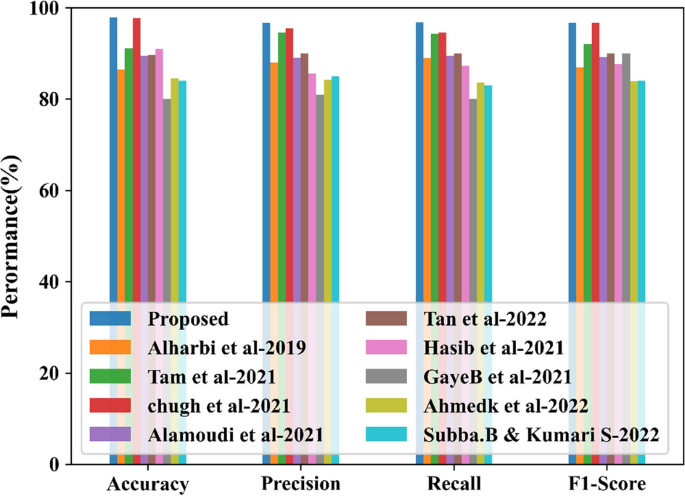
Performance comparison between proposed and existing methods
The accuracy comparison by varying the total selected features is described in Fig. 9 (a). The ROC curve of proposed model is discussed in Fig. 9 (b). The ROC is evaluated using FPR (False positive rate), and TPR (True positive rate). The AUC (Area under curve) obtained for proposed is found to be 0.989. It illustrates that the proposed model has shown efficient accuracy with less error rate.
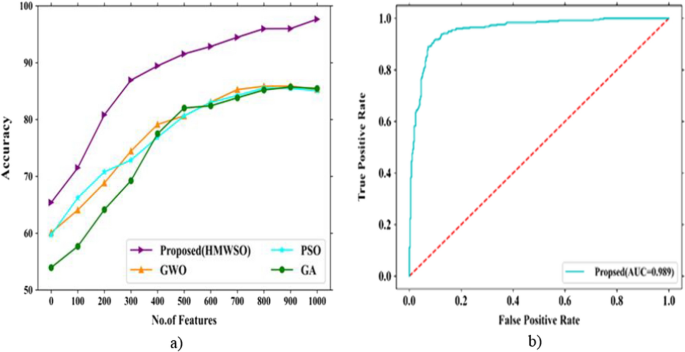
a Accuracy vs no of features b ROC curve
Ablation study
The ablation study for the proposed model is discussed in Table 10 . In this the performance of overall architecture is described, further the comparative analysis between existing techniques also described in Table 10 . Among all the techniques the proposed GARN has attained efficient performance than other algorithms. The hybridized methods are separately analysed and the results achieved by such techniques are also analysed which indicates that the integrating of all methods have improved the overall efficiency than applying the techniques in separate manner. Along with that, the ablation study for feature selection process is also evaluated and the obtained results are provided in Table 10 .The existing classification and feature selection methods taken for comparison are GRN (Gated recurrent network), ARN (Attention based recurrent network), RNN (Recurrent neural network), WSO, and MO (Mutation optimization).
The computational complexity of proposed model is defined below:The complexity of attention model is \(O\left( {n^{2} \cdot d} \right)\) , for recurrent network it is \(O\left( {n \cdot d^{2} } \right)\) , and the complexity of gated recurrent is found to be \(O\left( {k \cdot n \cdot d^{2} } \right)\) . The total complexity of proposed GARN is \(O\left( {k \cdot n^{2} \cdot d} \right)\) . This complexity shows that the proposed model has obtained efficient performance by reducing the system complexity. However, using the model separately won’t provide satisfactory performance. However, integration of such models has attained efficient performance than other existing methods.
GARN is preferred in this research to find the various opinions of Twitter online platform users. The implementation was carried out by utilizing the Sentiment 140 dataset. The performance of the leading GARN classifier is compared with other DL models Bi-GRU, Bi-LSTM, RNN and CNN for four performance metrics: accuracy, precision, f-measure and recall centred with four-term weighting schemes LTF-MICF, TF-DFS, TF-IDF, TF and W2V. The evaluation shows that the leading GARN DL technique reached the target level for Twitter sentiment classification. Additionally, while applying the suggested term weighting scheme-based feature extraction technique LTF-MICF with the leading GARN classifier gained an efficient result for tweet feature extraction. With the Twitter dataset, the GARN accuracy on applying LTF-MICF is 97.86%. The accuracy value attained by the proposed classifier is the highest of all the existing classifiers. Finally, the suggested GARN classifier is regarded as an effective DL classifier for Twitter sentiment analysis and other sentiment analysis applications. The proposed model has attained satisfactory result but it haven’t attained required level. This is because the proposed architecture fails to provide equal importance to the selected features. Due to this, few of the important features get lost, this has reduced the efficient performance of proposed model.Therefore as a future scope, an effective DL technique with the best feature selection method for classifying visual sentiment classification by utilizing all the selected features will be introduced. Further, this method is analysed using the small dataset, therefore in future large data with challenging images will be used to analyse the performance of present architecture.
Availability of data and materials
In this work, the dataset utilized in our proposed work contains 1,600,000 with score values for each tweets as, for positive tweets the rank value is 4 similarly for negative tweets rank value is 0 and for neutral tweets the rank value is 2 are collected using twitter api.
Change history
12 july 2023.
The typo in affiliation has been corrected.
Abbreviations
Deep Learning
- Gated recurrent attention network
Log Term Frequency-based Modified Inverse Class Frequency
Hybrid mutation based white shark optimizer
- Recurrent neural network
Natural Language Processing
Support Vector Machine
Naïve Bayes
Twitter Sentiment Analysis
Convolutional Neural Network
Term based random sampling
Saberi B, Saad S. Sentiment analysis or opinion mining: a review. Int J Adv Sci Eng Inf Technol. 2017;7(5):1660–6.
Article Google Scholar
Medhat W, Hassan A, Korashy H. Sentiment analysis algorithms and applications: a survey. Ain Shams Eng J. 2014;5(4):1093–113.
Drus Z, Khalid H. Sentiment analysis in social media and its application: systematic literature review. Procedia Comput Sci. 2019;161:707–14.
Zeglen E, Rosendale J. Increasing online information retention: analyzing the effects. J Open Flex Distance Learn. 2018;22(1):22–33.
Google Scholar
Qian Yu, Deng X, Ye Q, Ma B, Yuan H. On detecting business event from the headlines and leads of massive online news articles. Inf Process Manage. 2019;56(6): 102086.
Osatuyi B. Information sharing on social media sites. Comput Hum Behav. 2013;29(6):2622–31.
Neubaum, German. Monitoring and expressing opinions on social networking sites–Empirical investigations based on the spiral of silence theory. PhD diss., Dissertation, Duisburg, Essen, Universität Duisburg-Essen, 2016, 2016.
Karami A, Lundy M, Webb F, Dwivedi YK. Twitter and research: a systematic literature review through text mining. IEEE Access. 2020;8:67698–717.
Antonakaki D, Fragopoulou P, Ioannidis S. A survey of Twitter research: data model, graph structure, sentiment analysis and attacks. Expert Syst Appl. 2021;164: 114006.
Birjali M, Kasri M, Beni-Hssane A. A comprehensive survey on sentiment analysis: approaches, challenges and trends. Knowl-Based Syst. 2021;226: 107134.
Yadav N, Kudale O, Rao A, Gupta S, Shitole A. Twitter sentiment analysis using supervised machine learning. Intelligent data communication technologies and internet of things. Singapore: Springer; 2021. p. 631–42.
Book Google Scholar
Jain PK, Pamula R, Srivastava G. A systematic literature review on machine learning applications for consumer sentiment analysis using online reviews. Comput Sci Rev. 2021;41:100413.
Pandian AP. Performance Evaluation and Comparison using Deep Learning Techniques in Sentiment Analysis. Journal of Soft Computing Paradigm (JSCP). 2021;3(02):123–34.
Gandhi UD, Kumar PM, Babu GC, Karthick G. Sentiment analysis on Twitter data by using convolutional neural network (CNN) and long short term memory (LSTM). Wirel Pers Commun. 2021;17:1–10.
Kaur H, Ahsaan SU, Alankar B, Chang V. A proposed sentiment analysis deep learning algorithm for analyzing COVID-19 tweets. Inf Syst Front. 2021;23(6):1417–29.
Alharbi AS, de Doncker E. Twitter sentiment analysis with a deep neural network: an enhanced approach using user behavioral information. Cogn Syst Res. 2019;54:50–61.
Tam S, Said RB, Özgür Tanriöver Ö. A ConvBiLSTM deep learning model-based approach for Twitter sentiment classification. IEEE Access. 2021;9:41283–93.
Chugh A, Sharma VK, Kumar S, Nayyar A, Qureshi B, Bhatia MK, Jain C. Spider monkey crow optimization algorithm with deep learning for sentiment classification and information retrieval. IEEE Access. 2021;9:24249–62.
Alamoudi ES, Alghamdi NS. Sentiment classification and aspect-based sentiment analysis on yelp reviews using deep learning and word embeddings. J Decis Syst. 2021;30(2–3):259–81.
Tan KL, Lee CP, Anbananthen KSM, Lim KM. RoBERTa-LSTM: a hybrid model for sentiment analysis with transformer and recurrent neural network. IEEE Access. 2022;10:21517–25.
Hasib, Khan Md, Md Ahsan Habib, Nurul Akter Towhid, Md Imran Hossain Showrov. A Novel Deep Learning based Sentiment Analysis of Twitter Data for US Airline Service. In 2021 International Conference on Information and Communication Technology for Sustainable Development (ICICT4SD), pp. 450–455. IEEE. 2021.
Zhao H, Liu Z, Yao X, Yang Q. A machine learning-based sentiment analysis of online product reviews with a novel term weighting and feature selection approach. Inf Process Manage. 2021;58(5): 102656.
Braik M, Hammouri A, Atwan J, Al-Betar MA, Awadallah MA. White Shark Optimizer: a novel bio-inspired meta-heuristic algorithm for global optimization problems. Knowl-Based Syst. 2022;243: 108457.
Carvalho F. Guedes, GP. 2020. TF-IDFC-RF: a novel supervised term weighting scheme. arXiv preprint arXiv:2003.07193 .
Zeng L, Ren W, Shan L. Attention-based bidirectional gated recurrent unit neural networks for well logs prediction and lithology identification. Neurocomputing. 2020;414:153–71.
Niu Z, Yu Z, Tang W, Wu Q, Reformat M. Wind power forecasting using attention-based gated recurrent unit network. Energy. 2020;196: 117081.
https://www.kaggle.com/datasets/kazanova/sentiment140
Ahuja R, Chug A, Kohli S, Gupta S, Ahuja P. The impact of features extraction on the sentiment analysis. Procedia Comput Sci. 2019;152:341–8.
Gupta B, Negi M, Vishwakarma K, Rawat G, Badhani P, Tech B. Study of Twitter sentiment analysis using machine learning algorithms on Python. Int J Comput Appl. 2017;165(9):29–34.
Ikram A, Kumar M, Munjal G. Twitter Sentiment Analysis using Machine Learning. In 2022 12th International Conference on Cloud Computing, Data Science & Engineering (Confluence) pp. 629–634. IEEE. 2022.
Gaye B, Zhang D, Wulamu A. A Tweet sentiment classification approach using a hybrid stacked ensemble technique. Information. 2021;12(9):374.
Ahmed K, Nadeem MI, Li D, Zheng Z, Ghadi YY, Assam M, Mohamed HG. Exploiting stacked autoencoders for improved sentiment analysis. Appl Sci. 2022;12(23):12380.
Subba B, Kumari S. A heterogeneous stacking ensemble based sentiment analysis framework using multiple word embeddings. Comput Intell. 2022;38(2):530–59.
Pu X, Yan G, Yu C, Mi X, Yu C. Sentiment analysis of online course evaluation based on a new ensemble deep learning mode: evidence from Chinese. Appl Sci. 2021;11(23):11313.
Chen J, Chen Y, He Y, Xu Y, Zhao S, Zhang Y. A classified feature representation three-way decision model for sentiment analysis. Appl Intell. 2022;1:1–13.
Jain DK, Boyapati P, Venkatesh J, Prakash M. An intelligent cognitive-inspired computing with big data analytics framework for sentiment analysis and classification. Inf Process Manage. 2022;59(1): 102758.
Download references
Acknowledgements
Not applicable.
Authors did not receive any funding for this study.
Author information
Authors and affiliations.
Department of Computer Science & Engineering, Koneru Lakshmaiah Education Foundation, Guntur-Dt, Vaddeswaram, Andhra Pradesh, India
Nikhat Parveen
Ton Duc Thang University, Ho Chi Minh, Vietnam
Nikhat Parveen & Amjan Shaik
ITM SLS Baroda University, Vadodara, Gujarat, India
Prasun Chakrabarti
Data Science Laboratory, Faculty of Information Technology, Industrial University of Ho Chi Minh, Ho Chi Minh, Vietnam
Bui Thanh Hung
Department of Computer Science & Engineering, St.Peter’s Engineering College, Hyderabad, India
Amjan Shaik
You can also search for this author in PubMed Google Scholar
Contributions
NP and PC has found the proposed algorithms and obtained the datasets for the research and explored different methods discussed and contributed to the modification of study objectives and framework. Their rich experience was instrumental in improving our work. BTH and AS has done the literature survey of the paper and contributed writing the paper. All authors contributed to the editing and proofreading. All authors read and approved the final manuscript.
Corresponding author
Correspondence to Nikhat Parveen .
Ethics declarations
Ethics approval and consent to participate, consent for publication, competing interests.
The authors declare that they have no Competing interests.
Additional information
Publisher's note.
Springer Nature remains neutral with regard to jurisdictional claims in published maps and institutional affiliations.
Rights and permissions
Open Access This article is licensed under a Creative Commons Attribution 4.0 International License, which permits use, sharing, adaptation, distribution and reproduction in any medium or format, as long as you give appropriate credit to the original author(s) and the source, provide a link to the Creative Commons licence, and indicate if changes were made. The images or other third party material in this article are included in the article's Creative Commons licence, unless indicated otherwise in a credit line to the material. If material is not included in the article's Creative Commons licence and your intended use is not permitted by statutory regulation or exceeds the permitted use, you will need to obtain permission directly from the copyright holder. To view a copy of this licence, visit http://creativecommons.org/licenses/by/4.0/ .
Reprints and permissions
About this article
Cite this article.
Parveen, N., Chakrabarti, P., Hung, B.T. et al. Twitter sentiment analysis using hybrid gated attention recurrent network. J Big Data 10 , 50 (2023). https://doi.org/10.1186/s40537-023-00726-3
Download citation
Received : 30 June 2022
Accepted : 03 April 2023
Published : 17 April 2023
DOI : https://doi.org/10.1186/s40537-023-00726-3
Share this article
Anyone you share the following link with will be able to read this content:
Sorry, a shareable link is not currently available for this article.
Provided by the Springer Nature SharedIt content-sharing initiative
- Deep learning
- Term weight-feature extraction
- White shark optimizer
- Twitter sentiment
- Natural language processing
Thank you for visiting nature.com. You are using a browser version with limited support for CSS. To obtain the best experience, we recommend you use a more up to date browser (or turn off compatibility mode in Internet Explorer). In the meantime, to ensure continued support, we are displaying the site without styles and JavaScript.
- View all journals
- Explore content
- About the journal
- Publish with us
- Sign up for alerts
- NATURE BRIEFING
- 27 March 2024
Daily briefing: Tweeting about your paper doesn’t boost citations
- Flora Graham
You can also search for this author in PubMed Google Scholar
Hello Nature readers, would you like to get this Briefing in your inbox free every day? Sign up here .
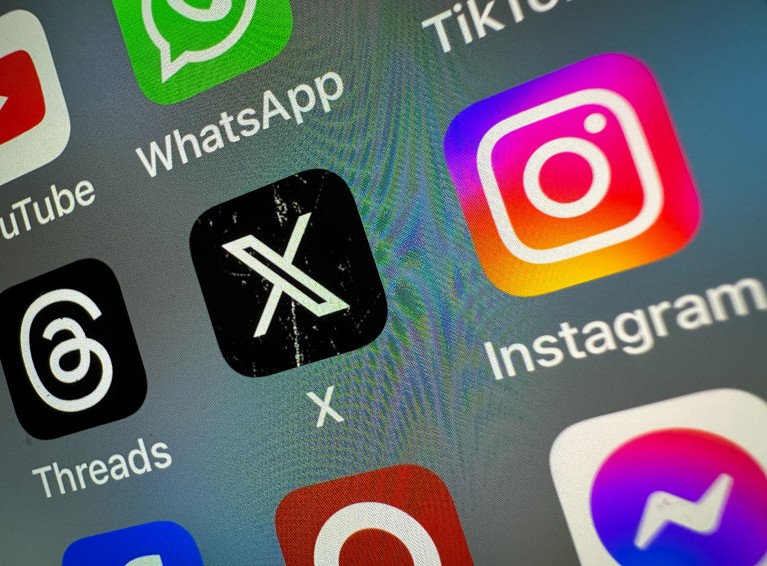
Even before recent complaints about X's declining quality, posting a paper on the social media platform did not translate to a boost in citations. Credit: Matt Cardy/Getty
Tweeting your paper doesn’t boost citations
Posting about a paper on X (formerly Twitter) seems to boost engagement but doesn’t translate into a bump in citations . A group of 11 researchers, each with at least several thousand followers, tweeted about a combined 110 articles between late 2018 and early 2020. In the short term, this increased the papers’ downloads and their Altmetric scores (a measure of how many people have looked at and are talking about it). But three years later, the citation rates for the tweeted papers weren’t significantly different to those of 440 control articles.
Nature | 4 min read
Reference: PLoS ONE paper
US lawsuit challenges abortion-pill access
Members of the US Supreme Court expressed scepticism yesterday about arguments from a group of anti-abortion organizations and physicians seeking to restrict use of the abortion drug mifepristone in the United States . Over the past eight years, the US Food and Drug Administration expanded the drug’s usage limit from 7 to 10 weeks of pregnancy and allowed it to be sent by post. If the court invalidates those actions, mifepristone access would be restricted nationwide. Reproductive health researchers say that the case has no scientific merit, because mifepristone has proved to be safe and effective. A decision is expected in June.
Nature | 6 min read
Hub for humans’ journey out of Africa found
After Homo sapiens expanded out of Africa 70,000 years ago, they seem to have paused for some 20,000 years before colonizing Europe and Asia. Now researchers think they know where. Looking at ancient and modern DNA, and the environment of the time, scientists have pinpointed the Persian Plateau — which in this definition encompasses Iran, the United Arab Emirates, Kuwait and parts of Oman — as the perfect place. Finding local archaeological evidence to confirm this could be difficult. “There's very little work being done there because of geopolitics,” says archaeologist and study co-author Michael Petraglia.
ABC News | 4 min read
Reference: Nature Communications paper
Engineers assess Baltimore bridge collapse
The Francis Scott Key Bridge would have been designed to survive a collision with a ship — but the sheer size of modern cargo vessels might surpass what was planned for, say engineers . Yesterday, the bridge in Baltimore catastrophically failed after one of its supports was struck by the 300-metre cargo ship ‘Dali’. The shocking speed of the collapse was due in part to its ‘continuous truss’ design, specialists say. “The collision of a vessel as large as the Dali container ship will have far exceeded the design loads for the slender concrete piers that support the truss structure, and once the pier is damaged you can see from the videos that the entire truss structure collapses very rapidly,” says structural engineer Andrew Barr.
The Independent | 7 min read
Features & opinion
Questioning the ‘mother tree’.
In 1997, ecologist Suzanne Simard made the cover of Nature with the discovery of a subterranean network of roots and fungal filaments through which, it was suggested, trees were exchanging resources. Simard’s ideas, further expressed in her hit scientific memoir Finding the Mother Tree , resonated deeply with many. But some ecologists think our fascination with the ‘wood wide web’ has outstripped the scientific evidence that underpins it.
Nature | 16 min read
Visa obstacles hobble global research
Of the ten speakers from low- and middle-income countries invited to a panel in Portugal last month, only four were able to get visas — and Ghanaian herpetologist Sandra Owusu-Gyamfi wasn’t one of them. “My experience left me feeling demoralized, embarrassed and insulted,” she writes. Her visa fees, flights and other costs were not refundable. Visa issues also come at a cost to global efforts to prevent further biodiversity loss . “Our participation is not a matter of simply ticking the inclusivity boxes, but a deliberate effort to ensure that the voices of people for whom some of these conservation policies are formulated are heard, and their opinions sought,” writes Owusu-Gyamfi.
Nature | 5 min read
Image of the day
Bubbles can be made considerably more stable by suspending them in the air using sound waves. This could reduce the need for surfactants that help them keep from popping when they’re used in industrial processes. Using ultrasonic waves, researchers kept soap stable for up to 15 minutes — longevity that’s previously only been achieved under microgravity conditions, for instance on the International Space Station. The bubbles tended to rotate a few times per second, maybe because of the way the sound waves moved around them. ( Nature Research Highlight | 3 min read , Nature paywall)
Reference : Droplet paper (Credit: X. Ji et al./Droplet ( CC-BY 4.0 DEED ))
Quote of the day
“the reason i think i’ve become obsessed with tuberculosis is because it’s such a glaring example of global injustice. this is a disease that is curable, it’s preventable. and yet, it remains the deadliest infectious disease in the world.”.
Best-selling US author John Green is using his passionate YouTube following to help him pressure pharma companies to lower the cost of TB diagnosis and treatment in low-and middle income countries. ( STAT | 6 min read )
Read more: John Green on why “the deadliest infectious disease isn’t a science problem. It’s a money problem” . (The Washington Post | 6 min read)
doi: https://doi.org/10.1038/d41586-024-00958-0
The 8 April total solar eclipse (visible in parts of the United States, Canada and Mexico, you lucky devils) will be more than just a visual phenomenon. The NASA-funded Eclipse Soundscapes Project is collecting multi-sensory observations and recorded sound data from community scientists on the day. Another effort, GLOBE Eclipse , asks volunteers to document air temperature and clouds during the event. As for me, I want to hear about the vibe.
Send your vibe-checks — plus any other feedback on this newsletter — to [email protected] .
Thanks for reading,
Flora Graham, senior editor, Nature Briefing
With contributions by Gemma Conroy and Katrina Krämer
Want more? Sign up to our other free Nature Briefing newsletters:
• Nature Briefing: Anthropocene — climate change, biodiversity, sustainability and geoengineering
• Nature Briefing: AI & Robotics — 100% written by humans, of course
• Nature Briefing: Cancer — a weekly newsletter written with cancer researchers in mind
• Nature Briefing: Translational Research covers biotechnology, drug discovery and pharma
Related Articles
Daily briefing: Weird new electron behaviour thrills physicists
Daily briefing: Pregnancy advances your ‘biological’ age
Daily briefing: How PhD assessment needs to change
Daily briefing: COVID ‘brain fog’ linked to brain inflammation
Tenure-track Assistant Professor in Ecological and Evolutionary Modeling
Tenure-track Assistant Professor in Ecosystem Ecology linked to IceLab’s Center for modeling adaptive mechanisms in living systems under stress
Umeå, Sweden
Umeå University
Faculty Positions in Westlake University
Founded in 2018, Westlake University is a new type of non-profit research-oriented university in Hangzhou, China, supported by public a...
Hangzhou, Zhejiang, China
Westlake University
Postdoctoral Fellowships-Metabolic control of cell growth and senescence
Postdoctoral positions in the team Cell growth control by nutrients at Inst. Necker, Université Paris Cité, Inserm, Paris, France.
Paris, Ile-de-France (FR)
Inserm DR IDF Paris Centre Nord
Zhejiang Provincial Hospital of Chinese Medicine on Open Recruitment of Medical Talents and Postdocs
Director of Clinical Department, Professor, Researcher, Post-doctor
The First Affiliated Hospital of Zhejiang Chinese Medical University
Sir Run Run Shaw Hospital, School of Medicine, Zhejiang University, Warmly Welcomes Talents Abroad
“Qiushi” Distinguished Scholar, Zhejiang University, including Professor and Physician
No. 3, Qingchun East Road, Hangzhou, Zhejiang (CN)
Sir Run Run Shaw Hospital Affiliated with Zhejiang University School of Medicine
Sign up for the Nature Briefing newsletter — what matters in science, free to your inbox daily.
Quick links
- Explore articles by subject
- Guide to authors
- Editorial policies
This paper is in the following e-collection/theme issue:
Published on 29.3.2024 in Vol 26 (2024)
#ProtectOurElders: Analysis of Tweets About Older Asian Americans and Anti-Asian Sentiments During the COVID-19 Pandemic
Authors of this article:

Original Paper
- Reuben Ng 1, 2 , PhD ;
- Nicole Indran 1 , BSocSci (Hons)
1 Lee Kuan Yew School of Public Policy, National University of Singapore, Singapore, Singapore
2 Lloyd's Register Foundation Institute for the Public Understanding of Risk, National University of Singapore, Singapore, Singapore
Corresponding Author:
Reuben Ng, PhD
Lee Kuan Yew School of Public Policy
National University of Singapore
469C Bukit Timah Road
Singapore, 259772
Phone: 65 66013967
Email: [email protected]
Background: A silver lining to the COVID-19 pandemic is that it cast a spotlight on a long-underserved group. The barrage of attacks against older Asian Americans during the crisis galvanized society into assisting them in various ways. On Twitter, now known as X, support for them coalesced around the hashtag #ProtectOurElders. To date, discourse surrounding older Asian Americans has escaped the attention of gerontologists—a gap we seek to fill. Our study serves as a reflection of the level of support that has been extended to older Asian Americans, even as it provides timely insights that will ultimately advance equity for them.
Objective: This study explores the kinds of discourse surrounding older Asian Americans during the COVID-19 crisis, specifically in relation to the surge in anti-Asian sentiments. The following questions guide this study: What types of discourse have emerged in relation to older adults in the Asian American community and the need to support them? How do age and race interact to shape these discourses? What are the implications of these discourses for older Asian Americans?
Methods: We retrieved tweets (N=6099) through 2 search queries. For the first query, we collated tweets with the hashtag #ProtectOurElders. For the second query, we collected tweets with an age-based term, for example, “elderly” or “old(er) adults(s)” and either the hashtag #StopAAPIHate or #StopAsianHate. Tweets were posted from January 1, 2020, to August 1, 2023. After applying the exclusion criteria, the final data set contained 994 tweets. Inductive and deductive approaches informed our qualitative content analysis.
Results: A total of 4 themes emerged, with 50.1% (498/994) of posts framing older Asian Americans as “vulnerable and in need of protection” (theme 1). Tweets in this theme either singled them out as a group in need of protection because of their vulnerable status or discussed initiatives aimed at safeguarding their well-being. Posts in theme 2 (309/994, 31%) positioned them as “heroic and resilient.” Relevant tweets celebrated older Asian Americans for displaying tremendous strength in the face of attack or described them as individuals not to be trifled with. Tweets in theme 3 (102/994, 10.2%) depicted them as “immigrants who have made selfless contributions and sacrifices.” Posts in this section referenced the immense sacrifices made by older Asian Americans as they migrated to the United States, as well as the systemic barriers they had to overcome. Posts in theme 4 (85/994, 8.5%) venerated older Asian Americans as “worthy of honor.”
Conclusions: The COVID-19 crisis had the unintended effect of garnering greater support for older Asian Americans. It is consequential that support be extended to this group not so much by virtue of their perceived vulnerability but more so in view of their boundless contributions and sacrifices.
Introduction
Not unlike other public health crises, the COVID-19 pandemic brought with it a disconcerting onslaught of racism and xenophobia [ 1 ]. The number of anti-Asian hate crimes in the United States quadrupled in 2021, escalating from the already significant uptick it experienced in 2020, when the COVID-19 outbreak was declared a global pandemic [ 2 ]. In the Asian American and Pacific Islanders (AAPI) community, those aged 60 years or older accounted for 7.3% of the 2808 self-reported incidents in 2020 [ 3 ]. Though not a particularly large figure, underreporting in this community is fairly common [ 4 ]. Moreover, older adults have reported being physically assaulted and having to deal with civil rights violations more than the general AAPI community [ 3 ]. When the crisis first emerged, older Asian Americans were beleaguered by increased economic insecurity [ 5 ] and poorer health outcomes [ 6 ] due to a confluence of structural inequities [ 5 ].
A silver lining to the COVID-19 pandemic is that it cast a spotlight on a long-underserved group. The barrage of attacks against older Asian Americans galvanized both individuals and organizations into assisting them in various ways, such as by distributing safety whistles and meal vouchers [ 7 ]. On Twitter, now known as X, support for them coalesced around the hashtag #ProtectOurElders [ 4 ]. The objective of this study is to explore the kinds of discourse surrounding older Asian Americans during the COVID-19 crisis, specifically in relation to the surge in anti-Asian sentiments.
Dating back to the nineteenth century, one of the most pervasive stereotypes of Asian Americans is that they are a high-achieving demographic [ 8 ]. While seemingly innocuous, this myth of them as a “model minority” has been criticized as highly problematic. Not only does it run counter to their lived realities—plenty of evidence has exposed the widespread inequalities confronted by various subgroups within the community [ 8 , 9 ]—it also delegitimizes their struggles and feeds the misconception that they require no assistance whatsoever [ 5 ].
Racial discrimination is well known to be a key social determinant of health [ 6 , 10 ]. Among Asian Americans in the United States, experiences of discrimination are linked to poorer mental health outcomes, including anxiety, depression, hypertension, and elevated blood pressure [ 10 ]. Racism may exacerbate health issues brought about by the aging process, such as the onset of chronic diseases or functional impairment [ 11 ], rendering older Asian Americans more susceptible to detrimental health outcomes.
Studies have indicated that social support has a positive impact on both the mental and physical health of older adults [ 12 ]. Social support likewise serves as a protective buffer against the negative effects of racial discrimination on one’s health [ 13 , 14 ]. The role of social support may be especially critical for Asian Americans. Although the Asian American populace includes a diverse array of ethnicities, cultures, and languages, collectivism appears to be a cultural orientation shared among many Asian American groups [ 15 ]. Evidence revealed that social support improved health outcomes among Asian Americans during the start of the pandemic, when anti-Asian sentiments were rampant [ 14 ].
It is widely acknowledged that in Asian societies, attitudes toward older adults are typically informed by values of respect and filial piety [ 11 , 16 ]. Old age bespeaks knowledge and wisdom, and younger people are expected to honor and respect their older counterparts [ 11 ]. Despite concerns that such values have eroded, there is evidence that they continue to resonate with Asian Americans [ 17 ]. One study concluded that Asian Americans are twice as likely as the general population to care for their parents [ 18 ]. Even so, ageism has been discovered to be pan-cultural [ 19 ]. A meta-analysis comparing Western and Eastern attitudes toward older adults revealed that Easterners actually harbored more negative views of older adults than Westerners [ 20 ]. In this analysis, Western countries included anglophone countries in the West such as Australia, Canada, the United Kingdom, and the United States, as well as Western European countries like Switzerland and France. Eastern countries covered countries in different regions of Asia, such as East Asia, South Asia, and Southeast Asia [ 20 ].
First proposed in 2002, the stereotype content model maintains that people stereotype others on the basis of warmth and competence [ 21 ]. The dimension of warmth includes qualities such as friendliness and sincerity, while the dimension of competence includes traits such as intelligence and skillfulness [ 21 ]. According to the stereotype content model, perceptions of social groups can be categorized into four clusters: (1) warm and competent, (2) incompetent and cold, (3) competent and cold, and (4) warm and incompetent. These 4 combinations of stereotypes produce distinct emotional responses among those who hold them. Groups stereotyped as warm and competent elicit admiration. Those evaluated as incompetent and cold elicit contempt. Groups stereotyped as competent and cold evoke envy. Those evaluated as warm but incompetent evoke pity [ 21 ].
A large body of work has evinced that older adults are generally stereotyped as warm but incompetent [ 21 ]. Although they elicit feelings of admiration occasionally, they predominantly evoke pity. Evidence attests to the universality of these stereotypes in both individualistic and collectivistic societies [ 19 ]. The evaluation of older adults as warm but lacking in competence may lend itself to benevolent ageism—a paternalistic form of prejudice founded on the assumption that older adults are helpless or pitiful [ 22 ]. Benevolent ageism has intensified over the course of the pandemic owing to recurring depictions of older adults as an at-risk group [ 23 ].
Asian Americans—older or otherwise—are one of the most underresearched ethnic groups in peer-reviewed literature [ 24 , 25 ]. In spite of the discomfiting rise in violence directed at them during the COVID-19 outbreak, discourse surrounding older adults from the Asian American community has escaped the attention of gerontologists. Most social media analyses conducted before and during the pandemic have focused on the discursive construction of the older population as a whole [ 26 - 28 ]. Other social media analyses have concentrated on the general Asian American population [ 29 - 31 ]. This study is therefore conceptually significant in that it is the first to dissect the content of tweets posted about older Asian Americans during the COVID-19 crisis.
At the heart of the concept of intersectionality is the notion that various social positions—such as race, age, gender, and socioeconomic status—interact to shape the types of biases one confronts [ 32 ]. From an intersectional standpoint, age and race may converge in ways that worsen the experience of discrimination for older Asian Americans [ 33 ]. In addition to being part of a racial group that faces more systemic challenges compared to White people, older Asian Americans also face age-related hurdles [ 34 ]. In terms of practical significance, this study serves as a reflection of the level of support being extended to older Asian Americans, even as it provides timely insights that will ultimately advance equity for them.
This study pivots around the following questions: What types of discourse have emerged in relation to older Asian Americans and the need to support them? How do age and race interact to shape these discourses? What are the implications of these discourses for older Asian Americans?
We retrieved the data using version 2 of Twitter’s application programming interface (API) [ 35 ], which was accessed through Twitter’s academic research product track [ 36 ]. Compared to what was achievable with the standard version 1.1 API, the version 2 API grants users a higher monthly tweet cap and access to more precise filters [ 37 ].
To build an extensive data set, we collected the tweets using 2 search queries. For both queries, “retweets” were excluded, and only English tweets posted from January 1, 2020, to August 1, 2023, were collated. We excluded retweets to avoid including duplicate content in the data set, which could skew the significance of particular topics. Tweets collected through the first query (n=1549) contained the hashtag #ProtectOurElders. For the second query (n=4550), we gathered tweets that met the following inclusion criteria: (1) contained either the hashtag #StopAAPIHate or #StopAsianHate; (2) included “elder,” “elderly,” “old(er) adult(s),” “old(er) people,” “old(er) person(s),” “senior(s),” “aged,” “old folk(s),” “grandparent(s),” “grandfather(s),” “grandmother(s),” “grandpa,” or “grandma.” The 2 queries yielded a total of 6099 tweets.
We removed posts that were (1) contextually irrelevant, that is, discussed content not pertaining to anti-Asian attacks, such as tweets related to getting vaccinated to protect older people, or tweets related to protecting older adults from cybercrime (n=1384); (2) repeated in the 2 queries (n=20); (3) incorrectly retrieved by the API, that is, they did not fulfill the inclusion criteria of either search query (n=258); and (4) informative, factual, or descriptive (eg, tweets that were newspaper headlines) or that brought up the older person in a tangential fashion (eg, tweets that mentioned older Asian Americans alongside several other groups; n=3443). After applying the aforementioned exclusion criteria, the data set consisted of 994 tweets. Figure 1 provides a flowchart of the data collection process.

Tweet Content Coding
Consistent with past research [ 27 , 38 - 41 ], the codebook was designed through both deductive and inductive modes of reasoning [ 42 ]. Analyses led by a directed or deductive approach begin with the identification of an initial set of codes based on previous literature [ 43 ]. Conversely, in inductive content analyses, codes are derived directly from the data [ 43 ]. We used both deductive and inductive approaches to make sure certain pertinent assumptions guided the analysis while also being aware that new categories would surface inductively [ 42 ].
To create a preliminary codebook, we first identified a set of categories based on previous literature regarding the perceptions of older adults in Asia [ 44 ]. The content analysis was subsequently conducted in several stages, with each tweet read twice by 2 researchers trained in gerontology to ensure familiarity with and immersion in the data [ 43 ]. The goal of the first reading was to ascertain the validity of the initial set of categories as well as to generate codes systematically across the whole data set. Each researcher modified the codebook independently until all variables were refined and clearly defined. During this first reading, a new category was added whenever a post featured a particular trait that could not be suitably coded into any of the existing categories and which was recurrent in the data. During the second reading, the 2 coders had frequent discussions where any discrepancies were reviewed and adjudicated to ensure rigor in the analysis. At this point, both coders discussed what the codes meant, confirmed the relevance of the codes to the research question, and identified areas of significant overlap to finalize the coding rubric.
The percentage agreement between the 2 raters was 92.5% with a weighted Cohen κ of 0.89 (P<.001), indicating high interrater reliability. A total of 4 themes emerged from the whole process. The frequency of each theme was identified after the analysis. As mentioned in past scholarship, categories in a content analysis need not be mutually exclusive, although they should be internally homogeneous (ie, coherent within themes) and externally heterogeneous (ie, distinct from each other) as far as possible [ 27 , 45 ].
Ethical Considerations
Ethical approval was not deemed necessary for this study, as all the data used were publicly available and anonymized.
Summary of Insights From Content Analysis of Tweets
A total of 4 themes emerged from our content analysis of 994 tweets. Half of the posts (498/994, 50.1%) were filed under the theme “vulnerable and in need of protection” (theme 1). Tweets in this theme either singled out older Asian Americans as a group in need of protection because of their vulnerable status or discussed initiatives aimed at safeguarding their well-being. The theme “heroic and resilient” (theme 2) was present in 31.1% (309/994) of the posts. Relevant tweets celebrated older Asian Americans for displaying tremendous strength in the face of attack or described them as individuals not to be trifled with. The theme “immigrants who have made selfless contributions and sacrifices” (theme 3) appeared in 10.2% (102/994) of the posts. Posts in this section referenced the immense sacrifices made by older Asian Americans as they migrated to the United States, as well as the systemic barriers they had to overcome. Theme 4 “worthy of honor” (85/994, 8.5%) consisted of tweets that venerated older Asian Americans. Textbox 1 provides a summary of the themes.
Vulnerable and in need of protection (498/994, 50.1%)
- “Isn't it so cowardly that they attack the elderly mostly? Not that violence is acceptable for any age, but to hurt the defenseless only means they got loose screws. #StopAsianHate”
- “Conducting walking patrols everyday to protect our elders and community #StopAAPIHate #HateisaVirus #StopAsianHate #SFChinatown #SafeNeighborhood #ProtectOurElders #TogetherWeCan”
Heroic and resilient (309/994, 31.1%)
- “Underestimating the terror wrought by old Chinese ladies with sticks was his first mistake #grannygoals #StopAsianHate”
- “Don't mess with Asian grandmas. But also sad this is happening. #StopAsianHate #StopAAPIHate”
Immigrants who have made selfless contributions and sacrifices (102/994, 10.2%)
- “Come to America they said..
It's the land of Opportunities they said...
Feeling so sad seeing this video 2 underage over privileged girls get to do this to a man ,a father ,a grandfather and not even have their identities revealed ...devastating
#MuhammadAnwar #StopAsianHate”
- “These are my grandparents. They came to America to build a new life. (That's my dad on the right wearing a tie.) My grandfather was a very well respected doctor in the Chinese community. America is built on the backbone of hard-working immigrants. #StopAsianHate”
Worthy of honor (85/994, 8.5%)
- “What's been shocking to me about these increased attacks on #AAPI is how often the elderly have been the focus. It’s such a shock because one thing that has been common amongst #AAPI culture is the reverence/respect of elders. #StopAAPIHate #StopAsianHate”
- “It really makes me weak and cry seeing videos of those elderly being hit and hurt. We, Asians, value and esteem our elderly. We even live with them in the same house, take care of them. I can't imagine how someone can simply push them. Just like that. #StopAsianHate”
Theme 1: Vulnerable and in Need of Protection
The vulnerability of older adults was a throughline in this category (498/994, 50.1%). Although concern was directed at the entire Asian American population, older adults were singled out as deserving of more sympathy because of their advanced age. Adjectives commonly used to frame them include “infirm,” “weak,” “defenseless,” and “powerless.” A person described them as lacking “the strength to even unclasp a grip.” Sympathy for older adults was magnified in view of other challenges they had been confronting since the outbreak of COVID-19. For instance, one poster expressed sorrow over how older Asian Americans had to grapple with the “fear of getting attacked” on top of “already [being] really afraid of COVID-19 because it disproportionately affects” them.
What made the act “especially egregious” in the eyes of many was the fact that assailants targeted older adults of all people. Users lambasted attackers for their “coward[ice],” asserting that they should have “picked on someone [their] own size” instead of attacking “people who can’t even defend themselves.” Several posters insisted that it was incumbent upon society to “be watchdogs” for older adults since they are more vulnerable.
A large number of tweets featured a call-to-action aimed at mobilizing members of the Twitter community to assist older Asian Americans. Fundraising campaigns were conducted to raise money for “alarms and pepper spray” for older Asian Americans. Others lobbied for donations to causes that deliver food to this group. The following tweet is one such example: “Wondering how you can support elderly Asians and show you will not tolerate #Asianhate? Join me in making a contribution to @heartofdinner, which brings food to elderly Asians in NYC so they can eat safely in their homes #StopAsianHateCrimes #StopAAPIHate.” The Twitter audience was also invited to escort older persons who walk alone: “United Peace Collaborative protects the #SF Chinatown community with daily walking patrols, providing protection & assistance to the elderly & residents. Please join us & volunteer!”
There were many tweets concerning the suite of initiatives aimed at supporting older Asian Americans. The Yellow Whistle—a campaign involving the distribution of free whistles for Asian Americans to signal danger in the event of an assault—was held up as one such example to “keep older Asian Americans safe.” Select community partners also received plaudits for their “wonderful work in distributing and training use of the alarms to” older persons.
Theme 2: Heroic and Resilient
Tweets in this theme (309/994, 31.1%) mainly revolved around a high-profile incident in San Francisco in which Xiao Zhen Xie, an older woman of Asian descent, put her assailant on a stretcher in an unexpected turn of events. She earned kudos from the Twitter community for “hold[ing] her ground,” “fighting back,” and sending him “to the hospital with his face bloodied.” Many saluted her for being “feisty,” “resilient,” and “[as] tough as nails,” dubbing her a “hero” who made them feel “#HonoredToBeAsian.” One user used the hashtag “#GrannyGoals,” quipping that the attacker made a “mistake” “underestimating the terror” that “old Chinese ladies” could wreak. Xiao Zhen Xie was also applauded for “refusing to be a statistic” as well as for defying the image of older adults as a group most expect “not to fight back.”
This episode involving Xiao Zhen Xie set in motion a series of tweets in which users warned others not to get on their grandparents’ bad side. A user cautioned that the incident was a lesson to everyone not to “mess with ahjummas, lolas, and all the elderly Asian women.” Another claimed that Asian grandmothers possess a special kind of “Asian grandma strength.” Some took the opportunity to underline the importance of not belittling older adults, with one in particular commenting on how his or her grandparents embodied grit and “toughness” because they “lived through war.”
Besides Xiao Zhen Xie, a few other older Asian Americans were celebrated for their resilience. A Filipina immigrant, Vilma Kari, was lauded for saying she “forgives” and “prays” for her attacker. A handful of tweets focused on a group of older Asian Americans who made the headlines for having filmed a music video in which they condemned the racially motivated acts of violence targeting their community.
Theme 3: Immigrants Who Have Made Selfless Contributions and Sacrifices
Members of the Twitter community frequently shared stories of their grandparents’ immigration (102/994, 10.2%). A common thread running through these posts was that their forefathers made immense sacrifices, uprooting themselves to move to the United States in order that their children might receive “the best education they can get” and “enjoy a “better future.” A user portrayed his or her grandmother as a “fighter” who “worked two to three jobs” while struggling to acculturate in a new society at a time when she knew “very little English.”
Attention was drawn to how the string of attacks against Asian Americans was ironic given the national ethos of the country commonly touted as the “American dream.” A few posters implied that labeling the United States as a “land of opportunity” was a misnomer: “Come to America,’ they said... ‘It’s the land of opportunities,’ they said...” A user said that the Asian “elderly did not escape communism” only to become a target of racism.
Tweets in this theme also discussed the burden of racism that older Asian Americans had endured before the COVID-19 pandemic. Users commented on their grandparents’ day-to-day experiences of racial discrimination. A handful were dismayed by how their grandparents were survivors of “prejudice and xenophobia” during World War II when they were forcibly relocated to Japanese internment camps. Others bemoaned that their older family members were “imprisoned for being the wrong-colored Americans.” One user deplored the fact that his or her grandfather “could not come to [the United States] because of his race” due to the Chinese Exclusion Act of 1882, a law that suspended Chinese immigration for 10 years and declared Chinese immigrants ineligible for naturalization. Another poster pinpointed how his or her grandfather felt compelled to dress in an “extremely patriotic” manner in order to camouflage his Asian identity and better assimilate into America.
Users considered older Asian Americans as foundational to the growth of America and foregrounded the need to acknowledge that “America is built on the backbone of hardworking immigrants,” who “made 90%” of what society has. Examples of contributions made by those of Asian ancestry include how they “oversaw” the construction of the transcontinental railroad in the “Old West” as well as their “service in the #442RCT (442nd Infantry Regiment)”—a highly decorated infantry regiment that mainly comprised second-generation American soldiers of Japanese descent who served in World War II. One user mentioned Chien-Shiung Wu, a groundbreaking Chinese American physicist whose scientific accomplishments were a core part of “U.S. WW II efforts” and that “helped win Nobel Prizes for Americans,” without which the “country would be so much worse off.” Artworks inspired by “hustling, elderly Asian folks” were also broadcasted under a hashtag that deified them as “#ChinatownGods.”
Several attempts were made to deconstruct the myth of the model minority. Individuals were aggrieved at how the looming specter of anti-Asian violence compounded the plight of older Asian Americans, who had already been dealt multiple blows during the COVID-19 crisis. These posters raised awareness of how many of them are in “precarious living situations” or “working in low-wage jobs.” Some pleaded for the Asian American community to be seen and understood, as captured in the following tweet: “See what’s happening to our elderly and community. Understand us. Understand why no matter how model of a minority we seem to be... we are just like you. #StopAsianHate #StopAAPIHate #StandWithAsians.”
Theme 4: Worthy of Honor
Many users (85/994, 8.5%) were outraged at how older adults appeared to be prime targets of violence against the Asian American community, perceiving these acts as a flagrant transgression of Asian cultural mores that “revere” them as “the most important people” in society. Some tweets exalted them as wellsprings of “wisdom” and “thoughtful guidance”—one user even likened them to “gold”—to “value and esteem.” Tweets in this theme also alluded to how deference to the older community was practically nonnegotiable in the Asian household. A poster tweeted, “No one should be assaulted, especially the elderly. I grew up respecting my elders. You never even argued with them ... They pass on wisdom.”
Values of collectivism were prized by certain users. These posters made reference to the notion of intergenerational reciprocity by stressing that younger people had an obligation to “protect” the older generation in return. The idea of solidarity was also raised. For instance, some viewed the attack of an older adult—related or otherwise—as an affront to the entire Asian community: “Many are saying ‘she could've been MY grandma.’ To that I say, she is ALL OUR GRANDMAS. Fight hate, love justice, stand with our elders always. #ForTheLoveOfLolas #StopAsianHate #StopAAPIHate #StopAsianHateCrimes.”
This study serves as a substantive first step in understanding discourses surrounding older Asian Americans. In our content analysis of tweets posted about the rash of attacks targeting them during the COVID-19 crisis, 4 main discourses surfaced. The first positioned them as “vulnerable and in need of protection” (theme 1). The second characterized them as “heroic and resilient” (theme 2). The third portrayed them as “immigrants who have made selfless contributions and sacrifices” (theme 3), and the fourth extolled them as “worthy of honor” (theme 4).
Our findings demonstrate an outpouring of support for the older Asian American community, which manifested itself in various local initiatives such as the distribution of safety whistles and the delivery of food. Scholars have drawn attention to how social support is particularly crucial for those in their later years [ 12 ] as well as those who experience racial discrimination [ 13 , 14 ]. The fact that older Asian Americans are finally being given support and assistance is therefore a step in the right direction.
However, even well-intentioned acts may be met with negative repercussions. In the wake of the COVID-19 crisis, older adults were reduced to a uniform group of at-risk individuals [ 46 ]. Assumptions of their vulnerability led to paternalistic behaviors, which denied them their autonomy [ 23 ]. Our results indicate that the rise in violence toward older Asian Americans sparked much-needed dialogue regarding their everyday struggles. Nevertheless, an unfortunate corollary is that this may have predisposed them to being recipients of benevolent prejudice on the basis of both age and race. Older Asian Americans may have been viewed as especially defenseless or vulnerable, perhaps more so than the general older population. This was made amply clear in the findings, where half of the tweets branded older Asian Americans as “weak” and “powerless.”
Notwithstanding concerns that Asian values of respect and filial piety have become irrelevant in the face of modernization [ 17 ], findings from themes 2-4 show emphatically that older adults retain their revered status, at least among some in the Asian American community. Tweets in theme 2 featured users enthusing over the way Xiao Zhen Xie held her ground when she was attacked in San Francisco, which led to deliberations on the strength and tenacity of older Asian women in general. Discourses of gratitude emerged in theme 3 as users ruminated over the sacrifices their forefathers had made in migrating to the United States, as well as the attendant systemic challenges they had to navigate. Posts in theme 4 indicate that users perceived the violence against older Asian Americans as a contravention of cultural norms, which emphasize the importance of honoring older adults. These provide a countervailing force to the various ageist tropes that came to the fore during the COVID-19 pandemic, such as the #BoomerRemover hashtag, which saw the lives of older people being discounted [ 27 , 28 ].
Theoretical Contribution and Implications
Findings from this study show that during the COVID-19 pandemic, age and race interfaced in complex ways to shape discourses on older Asian Americans. Specifically, our content analysis demonstrates that the stereotypes of warmth and incompetence, which are often thought to shape evaluations of older adults, cannot be applied indiscriminately to older Asian Americans as a subcategory of the older demographic. Theme 1, which positions older Asian Americans as vulnerable and in need of protection, does indeed align with traditional evaluations of older adults as warm and incompetent. However, the remaining themes celebrate older Asian Americans for their numerous contributions to society, the sacrifices they have made, and their unwavering resilience during the pandemic, all of which challenge the stereotype of incompetence under the stereotype content model. These findings add complexity to the commonly held notion of older adults as a pitiful social group by highlighting that older Asian Americans evoke not just pity but also admiration. The stereotype content model should therefore be expanded or modified in a way that accounts for attitudes toward older adults of different ethnicities.
Additionally, gerontological scholarship would benefit from a cross-cultural analysis of benevolent ageism. At present, little is known about how displays of benevolent ageism are affected by cultural norms of parental respect and filial piety and the extent to which these norms affect one’s perception of an older adult’s competence. Several studies have been conducted to make sense of ageism in different cultures [ 47 , 48 ], but there has been limited research on the cross-cultural differences in benevolent ageism specifically. The ways in which evaluations of older Asian Americans may be complicated by the deeply ingrained myth of the model minority as well as the pandemic-induced rise in anti-Asian hate are important avenues for future study.
This study has a number of implications for policy and practice. First, although care toward one’s parents or grandparents is not the prerogative of Asians [ 49 ], Asia’s adherence to collectivism nonetheless offers a useful learning point for the West. Many of the posters were Asian Americans, who held older adults in high regard, whether related or otherwise. Fostering a cultural emphasis on solidarity and interconnectedness in the West may promote respect not only for one’s parents but also for older adults outside of one’s family [ 44 ]. Second, ongoing efforts to reframe aging [ 50 ] could highlight the need to respect older adults, not in a way that advances their supremacy or absolves them from wrongdoing, but in a way that teaches society to view them as people whose experience may render them wise and worth learning from. Educators could also incorporate lessons on age-related stereotypes in schools to guard against the formation of ageist beliefs [ 51 ].
Third, current moves to redress the longstanding omission of Asian American history from national curricula [ 52 ] should ensure that students in every state are taught about the sacrifices, struggles, and contributions of older Asian Americans. Public campaigns could be organized as well to raise awareness of the aforementioned. This will help counter the myth of the model minority and get more people to acknowledge older Asian Americans as a significant part of America’s social fabric. Fourth, our findings underscore the need to reflect on the diversity of the older population in terms of socioeconomic status. Older adults—particularly those from the baby boomer generation—have been stereotyped as having made significant financial gains compared to their predecessors, at times even seen as stealing resources from the young [ 53 ]. However, as highlighted by some of the Twitter users as well as scholars, many older Asian Americans are in dire economic straits [ 5 ]. Rectifying the structural inequities that have contributed to their immiseration should hence be a key component of the agenda moving forward.
There are limitations inherent in this study. First, we acknowledge that Twitter users might not be representative of the wider population and that only publicly available tweets were included in the data set. Some of the users whose tweets were included in the study appeared to be Asian Americans, who are likely to be more passionate about supporting individuals in their community. Relatedly, as we did not collect information regarding users’ demographics—not all users publish demographic information, and there are certain limitations to using publicly provided demographic information on social media [ 54 ]—we could not contextualize the motivations of those whose tweets were included in the analysis. Ultimately, social support for older Asian Americans—whether from the Asian American community or society as a whole—has important implications for their well-being [ 14 ]. Subsequent research could focus on conducting interviews among individuals from different ethnic groups to tease out any differences in the level of support extended to older Asian Americans.
Second, we queried the hashtag #StopAAPIHate as a way to understand sentiments toward Asian Americans, even though the term “AAPI” refers to 2 different racial groups: Asian Americans and Pacific Islanders. As the tweets analyzed paid more attention to older Asian Americans, we were not able to offer insight into the types of discourses that emerged in relation to older Pacific Islanders. Future studies are needed to expound on such discourses. Third, it is vital to highlight that both the Asian American community and the older population are heterogeneous. The Asian American community encompasses numerous ethnicities, all with distinct languages, cultures, immigration histories, values, and beliefs [ 34 ]. The older demographic, too, is a diverse group composed of people with vastly different health trajectories [ 55 ]. Given the brevity of the tweets uploaded, we were unable to assess how discourses on older Asian Americans vary across different ethnicities. Finally, we collected only textual data, although tweets often contain visual elements such as photos, videos, and GIFs. This is a drawback that can be overcome in the future when multimodal techniques are developed to analyze both textual and visual content on Twitter.
Another direction for future inquiry involves an assessment of how discourses surrounding older Asian Americans have changed over time. The level of support shown to this group is likely to fluctuate over time, depending on the frequency at which anti-Asian attacks are reported in the news as well as other types of news being covered. Sentiment and narrative analyses [ 56 - 58 ] could be performed to glean such insights.
Even as older Asian Americans contended with a rise in racism alongside other struggles during the COVID-19 pandemic, our findings reveal that the crisis had the unintended effect of garnering greater support for this group. In the future, it is important that support be extended to older Asian Americans not so much by virtue of their perceived vulnerability but more so in view of their boundless contributions and sacrifices.
Acknowledgments
The authors would like to thank L Liu for preprocessing the data. We gratefully acknowledge support from the Social Science Research Council SSHR Fellowship (MOE2018-SSHR-004). The funder had no role in study design, data collection, analysis, writing, or the decision to publish this study.
Data Accessibility
Data are publicly available on Twitter [ 59 ].
Authors' Contributions
RN designed the study, developed the methodology, analyzed the data, wrote the paper, acquired the funding. RN and NI analyzed the data and wrote the paper.
Conflicts of Interest
None declared.
- Elias A, Ben J, Mansouri F, Paradies Y. Racism and nationalism during and beyond the COVID-19 pandemic. Ethn Racial Stud. 2021;44(5):783-793. [ CrossRef ]
- Yamauchi N. Anti-Asian hate crimes quadrupled in U.S. last year. Nikkei Asia. 2022. URL: https://asia.nikkei.com/Spotlight/Society/Anti-Asian-hate-crimes-quadrupled-in-U.S.-last-year [accessed 2022-02-16]
- Turton N. Stop AAPI Hate: new data on anti-Asian hate incidents against elderly and total national incidents in 2020. Stop AAPI Hate. 2021. URL: https://stopaapihate.org/wp-content/uploads/2021/04/Stop-AAPI-Hate-Press-Statement-Bay-Area-Elderly-Incidents-210209.pdf [accessed 2024-02-23]
- Huang J. How the #ProtectOurElders movement helped create a wave of first-time Asian American Activists. LAist. 2021. URL: https://laist.com/news/how-protectourelders-helped-created-a-wave-of-first-time-asian-american-activists [accessed 2022-04-03]
- Ma KPK, Bacong AM, Kwon SC, Yi SS, Ðoàn LN. The impact of structural inequities on older Asian Americans during COVID-19. Front Public Health. 2021;9:690014. [ FREE Full text ] [ CrossRef ] [ Medline ]
- Chen JA, Zhang E, Liu CH. Potential impact of COVID-19-related racial discrimination on the health of Asian Americans. Am J Public Health. 2020;110(11):1624-1627. [ CrossRef ] [ Medline ]
- Lee E. Asian American community rallies to support elders. AARP. 2021. URL: https://www.aarp.org/home-family/friends-family/info-2021/asian-american-support-communities.html [accessed 2022-02-16]
- Yi V, Museus S. Model minority myth. In: Smith AD, Hou X, Stone J, Dennis RM, Rizova P, editors. The Wiley Blackwell Encyclopedia of Race, Ethnicity, and Nationalism. Oxford, UK. John Wiley & Sons, Ltd; 2015.
- Li G, Wang L. Model Minority Myth Revisited: An Interdisciplinary Approach to Demystifying Asian American Educational Experiences. Charlotte, NC. Information Age Publishing; 2008.
- Paradies Y, Ben J, Denson N, Elias A, Priest N, Pieterse A, et al. Racism as a determinant of health: a systematic review and meta-analysis. PLoS One. 2015;10(9):e0138511. [ FREE Full text ] [ CrossRef ] [ Medline ]
- Min J, Moon A. Older Asian Americans. In: Berkman B, D'Ambruoso S, editors. Handbook of Social Work in Health and Aging. New York, NY. Oxford University Press; 2006.
- Antonucci TC, Ajrouch KJ, Birditt KS. The convoy model: explaining social relations from a multidisciplinary perspective. Gerontologist. 2014;54(1):82-92. [ FREE Full text ] [ CrossRef ] [ Medline ]
- Ajrouch KJ, Reisine S, Lim S, Sohn W, Ismail A. Perceived everyday discrimination and psychological distress: does social support matter? Ethn Health. 2010;15(4):417-434. [ FREE Full text ] [ CrossRef ] [ Medline ]
- Lee S, Waters SF. Asians and Asian Americans’ experiences of racial discrimination during the COVID-19 pandemic: impacts on health outcomes and the buffering role of social support. Stig Health. Feb 2021;6(1):70-78. [ CrossRef ]
- Markus HR, Kitayama S. The cultural construction of self and emotion: implications for social behavior. In: Emotion and Culture: Empirical Studies of Mutual Influence. Washington, DC. American Psychological Association; 1994;89-130.
- Ingersoll-Dayton B, Saengtienchai C. Respect for the elderly in Asia: stability and change. Int J Aging Hum Dev. 1999;48(2):113-130. [ CrossRef ] [ Medline ]
- Harrington B. "It's more us helping them instead of them helping us": how class disadvantage motivates Asian American college students to help their parents. J Fam Issues. 2022;44(7):1773-1795. [ CrossRef ]
- Montenegro X. Caregiving among Asian Americans and Pacific Islanders age 50+. AARP Research. 2014. URL: https://www.aarp.org/pri/topics/ltss/family-caregiving/caregiving-asian-americans-pacific-islanders/ [accessed 2024-02-23]
- Cuddy AJC, Norton MI, Fiske ST. This old stereotype: the pervasiveness and persistence of the elderly stereotype. J Social Issues. 2005;61(2):267-285. [ CrossRef ]
- North MS, Fiske ST. Modern attitudes toward older adults in the aging world: a cross-cultural meta-analysis. Psychol Bull. 2015;141(5):993-1021. [ CrossRef ] [ Medline ]
- Fiske ST, Cuddy AJC, Glick P, Xu J. A model of (often mixed) stereotype content: competence and warmth respectively follow from perceived status and competition. J Pers Soc Psychol. 2002;82(6):878-902. [ CrossRef ]
- Cary LA, Chasteen AL, Remedios J. The ambivalent ageism scale: developing and validating a scale to measure benevolent and hostile ageism. Gerontologist. 2017;57(2):e27-e36. [ FREE Full text ] [ CrossRef ] [ Medline ]
- Vervaecke D, Meisner B. Caremongering and assumptions of need: the spread of compassionate ageism during COVID-19. Gerontologist. 2021;61(2):159-165. [ FREE Full text ] [ CrossRef ] [ Medline ]
- Yi SS. Taking action to improve Asian American Health. Am J Public Health. 2020;110(4):435-437. [ CrossRef ] [ Medline ]
- Ðoàn LN, Takata Y, Sakuma KLK, Irvin VL. Trends in clinical research including Asian American, Native Hawaiian, and Pacific Islander participants funded by the US National Institutes of Health, 1992 to 2018. JAMA Netw Open. 2019;2(7):e197432. [ FREE Full text ] [ CrossRef ] [ Medline ]
- Levy BR, Chung PH, Bedford T, Navrazhina K. Facebook as a site for negative age stereotypes. Gerontologist. 2014;54(2):172-176. [ FREE Full text ] [ CrossRef ] [ Medline ]
- Sipocz D, Freeman JD, Elton J. "A toxic trend?": generational conflict and connectivity in Twitter discourse under the #BoomerRemover hashtag. Gerontologist. 2021;61(2):166-175. [ FREE Full text ] [ CrossRef ] [ Medline ]
- Skipper AD, Rose DJ. #BoomerRemover: COVID-19, ageism, and the intergenerational twitter response. J Aging Stud. 2021;57:100929. [ FREE Full text ] [ CrossRef ] [ Medline ]
- Hswen Y, Xu X, Hing A, Hawkins JB, Brownstein JS, Gee GC. Association of "#covid19" versus "#chinesevirus" with Anti-Asian sentiments on Twitter: march 9-23, 2020. Am J Public Health. 2021;111(5):956-964. [ FREE Full text ] [ CrossRef ] [ Medline ]
- Nguyen TT, Criss S, Dwivedi P, Huang D, Keralis J, Hsu E, et al. Exploring U.S. shifts in Anti-Asian sentiment with the emergence of COVID-19. Int J Environ Res Public Health. 2020;17(19):7032. [ FREE Full text ] [ CrossRef ] [ Medline ]
- Darling-Hammond S, Michaels EK, Allen AM, Chae DH, Thomas MD, Nguyen TT, et al. After "The China Virus" went viral: racially charged coronavirus coverage and trends in bias against Asian Americans. Health Educ Behav. 2020;47(6):870-879. [ FREE Full text ] [ CrossRef ] [ Medline ]
- Krekula C, Nikander P, Wilińska M. Multiple marginalizations based on age: gendered ageism and beyond. In: Ayalon L, Tesch-Römer C, editors. Contemporary Perspectives on Ageism. Cham, Switzerland. Springer Open; 2018;33-50.
- Gutterman AS. Ageism, race and ethnicity. SSRN Journal. Preprint posted online on December 8 2021. 2021. [ FREE Full text ] [ CrossRef ]
- Kim G, Wang SY, Park S, Yun SW. Mental health of Asian American older adults: contemporary issues and future directions. Innov Aging. 2020;4(5):igaa037. [ FREE Full text ] [ CrossRef ] [ Medline ]
- Twitter API v2: early access. Twitter. 2021. URL: https://developer.twitter.com/en/docs/twitter-api/early-access [accessed 2021-10-13]
- Tornes A, Trujillo L. Enabling the future of academic research with the Twitter API. Twitter Developer Platform Blog. 2021. URL: https://blog.twitter.com/developer/en_us/topics/tools/2021/enabling-the-future-of-academic-research-with-the-twitter-api [accessed 2021-10-13]
- Barrie C, Ho JC. academictwitteR: an R package to access the Twitter Academic Research Product Track v2 API endpoint. JOSS. 2021;6(62):3272. [ FREE Full text ] [ CrossRef ]
- Ng R, Indran N. Does age matter? Tweets about gerontocracy in the United States. J Gerontol B Psychol Sci Soc Sci. 2023;78(11):1870-1878. [ CrossRef ] [ Medline ]
- Ng R, Indran N. Innovations for an aging society through the lens of patent data. Gerontologist. 2024;64(2). [ FREE Full text ] [ CrossRef ] [ Medline ]
- Ng R, Indran N. Not too old for TikTok: how older adults are reframing aging. Gerontologist. 2022;62(8):1207-1216. [ FREE Full text ] [ CrossRef ] [ Medline ]
- Ng R, Indran N. Age advocacy on Twitter over 12 years. Gerontologist. 2024;64(1). [ CrossRef ] [ Medline ]
- Armat MR, Assarroudi A, Rad M, Sharifi H, Heydari A. Inductive and deductive: ambiguous labels in qualitative content analysis. TQR. 2018;23(1):219-221. [ FREE Full text ] [ CrossRef ]
- Hsieh HF, Shannon SE. Three approaches to qualitative content analysis. Qual Health Res. 2005;15(9):1277-1288. [ CrossRef ] [ Medline ]
- Hwang KK. Filial piety and loyalty: two types of social identification in Confucianism. Asian J of Social Psycho. 2002;2(1):163-183. [ CrossRef ]
- Bengtsson M. How to plan and perform a qualitative study using content analysis. NursingPlus Open. 2016;2:8-14. [ FREE Full text ] [ CrossRef ]
- Ayalon L. There is nothing new under the sun: ageism and intergenerational tension in the age of the COVID-19 outbreak. Int Psychogeriatr. 2020;32(10):1221-1224. [ FREE Full text ] [ CrossRef ] [ Medline ]
- Ng R, Lim-Soh JW. Ageism linked to culture, not demographics: evidence from an 8-billion-word corpus across 20 countries. J Gerontol B Psychol Sci Soc Sci. 2021;76(9):1791-1798. [ FREE Full text ] [ CrossRef ] [ Medline ]
- Löckenhoff CE, De Fruyt F, Terracciano A, McCrae RR, De Bolle M, Costa PT, et al. Perceptions of aging across 26 cultures and their culture-level associates. Psychol Aging. 2009;24(4):941-954. [ FREE Full text ] [ CrossRef ] [ Medline ]
- Lim AJ, Lau CYH, Cheng CY. Applying the Dual Filial Piety Model in the United States: a comparison of filial piety between Asian Americans and Caucasian Americans. Front Psychol. 2021;12:786609. [ FREE Full text ] [ CrossRef ] [ Medline ]
- Sweetland J, Volmert A, O'Neil M. Finding the frame: an empirical approach to reframing aging and ageism. FrameWorks Institute. Washington, DC.; 2017. URL: https://www.frameworksinstitute.org/wp-content/uploads/2020/05/aging_research_report_final_2017.pdf [accessed 2024-02-23]
- Russell ER, Thériault ÉR, Colibaba A. Facilitating age-conscious student development through lecture-based courses on aging. Can J Aging. 2022;41(2):283-293. [ FREE Full text ] [ CrossRef ] [ Medline ]
- Chavez N. New Jersey becomes second state to require Asian American history to be taught in schools. CNN. 2022. URL: https://www.cnn.com/2022/01/18/us/new-jersey-schools-asian-american-history/index.html [accessed 2022-04-11]
- Ng R, Indran N. Hostility toward baby Boomers on TikTok. Gerontologist. 2022;62(8):1196-1206. [ FREE Full text ] [ CrossRef ] [ Medline ]
- Sloan L. Who Tweets in the United Kingdom? Profiling the Twitter population using the British Social Attitudes Survey 2015. Soc Media Soc. 2017;3(1):205630511769898. [ FREE Full text ] [ CrossRef ]
- Diehl M, Smyer MA, Mehrotra CM. Optimizing aging: a call for a new narrative. Am Psychol. 2020;75(4):577-589. [ FREE Full text ] [ CrossRef ] [ Medline ]
- Ng R, Indran N, Suarez P. Communicating risk perceptions through Batik art. JAMA. 2023;330(9):790-791. [ CrossRef ] [ Medline ]
- Ng R, Indran N. Reframing aging: foregrounding familial and occupational roles of older adults is linked to decreased ageism over two centuries. J Aging Soc Policy. 2023.:1-18. [ CrossRef ] [ Medline ]
- Ng R, Indran N. Impact of old age on an occupation's image over 210 years: an age premium for doctors, lawyers, and soldiers. J Appl Gerontol. 2023;42(6):1345-1355. [ CrossRef ] [ Medline ]
- Twitter. 2021. URL: https://twitter.com/ [accessed 2021-10-13]
Abbreviations
Edited by A Mavragani; submitted 19.01.23; peer-reviewed by A Atalay, A Bacong; comments to author 22.02.23; revised version received 12.03.23; accepted 14.09.23; published 29.03.24.
©Reuben Ng, Nicole Indran. Originally published in the Journal of Medical Internet Research (https://www.jmir.org), 29.03.2024.
This is an open-access article distributed under the terms of the Creative Commons Attribution License (https://creativecommons.org/licenses/by/4.0/), which permits unrestricted use, distribution, and reproduction in any medium, provided the original work, first published in the Journal of Medical Internet Research, is properly cited. The complete bibliographic information, a link to the original publication on https://www.jmir.org/, as well as this copyright and license information must be included.
Help | Advanced Search
Computer Science > Digital Libraries
Title: can chatgpt predict article retraction based on twitter mentions.
Abstract: Detecting problematic research articles timely is a vital task. This study explores whether Twitter mentions of retracted articles can signal potential problems with the articles prior to retraction, thereby playing a role in predicting future retraction of problematic articles. A dataset comprising 3,505 retracted articles and their associated Twitter mentions is analyzed, alongside 3,505 non-retracted articles with similar characteristics obtained using the Coarsened Exact Matching method. The effectiveness of Twitter mentions in predicting article retraction is evaluated by four prediction methods, including manual labelling, keyword identification, machine learning models, and ChatGPT. Manual labelling results indicate that there are indeed retracted articles with their Twitter mentions containing recognizable evidence signaling problems before retraction, although they represent only a limited share of all retracted articles with Twitter mention data (approximately 16%). Using the manual labelling results as the baseline, ChatGPT demonstrates superior performance compared to other methods, implying its potential in assisting human judgment for predicting article retraction. This study uncovers both the potential and limitation of social media events as an early warning system for article retraction, shedding light on a potential application of generative artificial intelligence in promoting research integrity.
Submission history
Access paper:.
- Other Formats
References & Citations
- Google Scholar
- Semantic Scholar
BibTeX formatted citation

Bibliographic and Citation Tools
Code, data and media associated with this article, recommenders and search tools.
- Institution
arXivLabs: experimental projects with community collaborators
arXivLabs is a framework that allows collaborators to develop and share new arXiv features directly on our website.
Both individuals and organizations that work with arXivLabs have embraced and accepted our values of openness, community, excellence, and user data privacy. arXiv is committed to these values and only works with partners that adhere to them.
Have an idea for a project that will add value for arXiv's community? Learn more about arXivLabs .
Institutional Review Board (IRB)
The Institutional Review Board (IRB) reviews research involving human participants to ensure that the rights and welfare of human participants are protected. The IRB has the authority to approve, require modifications, disapprove, suspend, terminate and observe the consenting process for research that falls within its scope of review, as specified by federal regulations and institutional policy. UT Austin has two IRBs: a Social Behavioral and Educational Research IRB (SBERIRB) and a Health Sciences IRB (HSIRB).
The HRPP and the UT IRBs have been accredited by the Association for the Accreditation of Human Research Participants (AAHRPP) since 2006.
Activities Requiring IRB Review
UT’s IRB’s provide ethical oversight to all activities that meet the regulatory definitions of research involving human subjects conducted by UT faculty, staff or students.
Research requires IRB review under Department of Health and Human Services (DHHS) regulations if the project is a systematic investigation designed to develop or contribute to generalizable knowledge AND involves living individuals about whom an investigator:
- Obtains information or biospecimens through intervention or interactions with the individuals, and uses, studies or analyzes the information or biospecimens
- Obtains, uses, studies, analyzes or generates identifiable private information or identifiable biospecimens
Additionally, research requires IRB review under FDA regulations if the project meets the definition of a clinical investigation — any experiment that involves one or more human subjects and an FDA-regulated test article (drug, device, biologic, human food additive, electronic product) other than in the course of standard medical practice — AND involves a human subject defined as an individual who is or becomes a participant in research, either as a recipient of the test article or as a control. A subject may either be a healthy individual or a patient.
If a research project meets either two scenarios above, IRB review and determination is required prior to beginning any research activities involving human subjects.
If an activity does not meet the regulatory definition of “research,” no IRB review is required. Similarly, if an activity is research, but it does not involve human subjects, the research does not require IRB review. Failure to meet either definition means that the activity is not human subjects research.
For help determining if research meets these regulatory definitions before submitting to the IRB, contact the IRB staff at [email protected] or online chat.
Determining When to Submit Activities to the IRB
Examples of activities that may or may not be human subjects research NIH Decision Tool: Am I Doing Human Subjects Research? HRP-310 – Worksheet: Human Research Determination
PI Eligibility
The principal investigator (PI) of a human research study is the individual with ultimate responsibility for the conduct of the activities described in the IRB submission and for protecting the rights and welfare of human participants involved in the research. The PI must be available to devote adequate time and attention to the study to ensure its responsible conduct.
- Tenure-track faculty
- Research assistant, associate or full professor
- Non-tenure-track faculty who are paid UT employees
- Directors, chairs, deans, VPs or AVPs
- Affiliate of clinical faculty when all of the research will be conducted in a UT, Dell or Seton facility
- Research scientists
- Research associates
- Faculty instructors
- Emeritus faculty
- Adjunct faculty
- Visiting faculty and scholars
- Postdoctoral fellows
- Research assistants
- Graduate students
- Undergraduate students
Faculty Sponsors
A faculty sponsor, who is eligible to serve as a PI, is required for all students conducting human subjects research and should oversee the conduct of student conducted research. Students and their faculty sponsors are responsible for human subjects protections. The faculty sponsor is typically the student’s dissertation or thesis chair; however, this is not always the case. The sponsor will provide valuable recommendations about experimental designs aimed at reducing the risk to human subjects.
In the UTRMS-IRB system, the faculty sponsor will be listed as the PI and the student investigator should be designated as the PI Proxy. The PI Proxy will be able to submit actions in the UTRMS-IRB system.
Review Process
In the UT Research Management Suite – IRB Module, researchers can see at a glance where their submission is within the review process. At the top of the study action workspace is the workflow.
The RMS system uses “states” to classify where a submission is in the process. The following table defines the possible study states for a new study submission during the course of its review.
Pre-Submission The application is under preparation by the PI/submission preparer. The study will remain in this state until the PI/PI Proxy clicks Submit.
Pre-Review The submission is in the queue / is being reviewed by the assigned IRB Analyst.
Clarifications Requested Pre-Review The IRB Analyst has requested changes or clarification. The PI, PI Proxy and Primary Contact will receive an email notification advising them of the request. Details regarding the request can be found in the study workspace.
IRB Review The submission is currently being reviewed by an IRB member.
Clarifications Requested IRB Review The IRB member has requested changes or clarifications regarding the submission under review.
Post Review The IRB letter advising the PI of the IRB member or IRB committee’s decision is being prepared.
Modifications Required The IRB has requested additional modifications to secure final approval. The PI, PI Proxy and Primary contact will receive an email notification advising them of this request.
Review Complete The PI will receive the final approval/determination letter and IRB review is complete.
Departmental Review
Some academic areas have a departmental review process for research submitted to the IRB. Researchers must follow all departmental requirements for review and approval, if applicable. For questions about the rules and procedures for departmental review or the applicability of this information to a submission, please contact the appropriate department.
IRB Review Types
Depending on the type of research conducted, it may be exempt, expedited or require full board review. The type of review is determined by risk level and categories as defined by federal regulations. Minimal risk is defined by federal regulations as the probability and magnitude of harm or discomfort anticipated in the research are not greater in and of themselves than those ordinarily encountered in daily life or during the performance of routine physical or psychological examinations or tests [45 CFR 46.102(j)].
Federal regulations identify several different categories of minimal risk research as being exempt from the regulations. This does not mean that they are exempt from IRB review, only that some of the federal requirements that apply to non-exempt studies are not applicable to studies deemed exempt. For example, exempt studies are not required to obtain written informed consent and are not required to submit modifications prior to implementation (unless they may affect the exempt status of the study).
Exempt reviews are performed administratively by the IRB Minimal Risk Research Team. For detailed information on the exempt categories and exempt submissions, refer to the IRB Policies and Procedures Section 5.4 Exempt Research . FDA regulated studies are not eligible for exemption under these categories.
Unlike exempt review, expedited review falls under the full scope of the regulations and is reviewed by a designated IRB member reviewer. Expedited studies must fall into one of the Expedited Review Categories .
All expedited studies must adhere to the requirements for informed consent or its waiver or alteration. Expedited studies may or may not be required to undergo continuing review. All modifications must be approved by the IRB prior to implementation, unless they are necessary for the immediate safety of participants.
Studies that are not eligible for expedited review (do not meet the definition of minimal risk and/or do not fit into an expedited category) must be reviewed by the convened IRB.
All full board studies must adhere to the requirements for informed consent or its waiver or alteration. Full board studies must undergo continuing review at least annually. All modifications must be approved by the IRB prior to their implementation, unless they are necessary for the immediate safety of participants.
Please email [email protected] with questions or to set up a consultation. Include information about the proposed research study or UT IRB Study Number (if available) along with questions to facilitate an efficient response.
Electronic Submission
All new human subjects research applications must be submitted electronically via the UT Research Management Suite – IRB Module (UTRMS-IRB) . If a study was originally approved in the legacy system, historical study documents and IRB determinations can be accessed in IRBaccess .
Submitting to the IRB
All new human subjects research must be reviewed by the IRB prior to the commencement of any study activity. The IRB Application Guide will assist UT Austin faculty, staff and students who are planning to conduct research involving human subjects.
Once IRB approval or determination has been granted, researchers must follow IRB Policies and Procedures for follow-on submissions during the course of their research study to remain in compliance. Examples of follow-on submissions include modifications, continuing reviews (when applicable), and reportable new information reports.
- See the step-by-step instructions on submitting new studies via UTRMS.
- Forms and templates are available for download via the UTRMS-IRB Library, “Templates” tab .
- Guidance documents help with questions during the submission process.
IRB Dates, Deadlines and Fees IRB Reliance Frequently Asked Questions
The University of Chicago The Law School
Whose judicial data is it, anyway, in his latest research project, aziz huq focuses on making public data from courts more accessible.
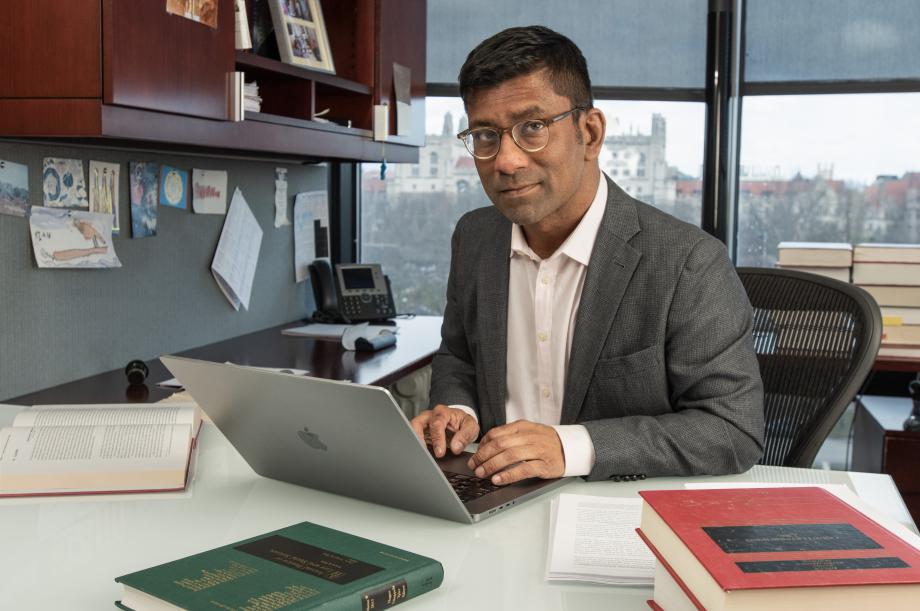
Editor’s Note: This story is the first in an occasional series on research projects currently in the works at the Law School.
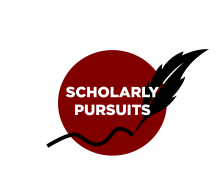
Every court case and judicial proceeding generates an enormous amount of data, some of which is either non-public or difficult to access.
What to do with that data is a question that Aziz Z. Huq, the Frank and Bernice J. Greenberg Professor at the Law School has been pondering lately. Huq is coauthoring a paper with Northwestern Law School Professor (and former Chicago Law School Public Fellow) Zachary D. Clopton that they hope will begin a thoughtful discussion of who should control this judicial data and who should have access to it.
If currently hidden data were made accessible and affordable, Huq explains, attorneys and researchers could use it to help find answers to a wide range of constitutional and public policy questions. For example:
- W hen is the provision of legal counsel effective, unnecessary, or sorely needed?
- When and where is litigation arising and what are the barriers to court access?
- Are judges consistent when they determine in forma pauperis status?
- Do judges ’ sentencing decisions reflect defendants ’ observed race, ethnicity, or gender?
- Are any state and local governments infringing on civil rights though their policing or municipal court systems?
According to Huq and Clopton, judicial data could be used to help clarify the law in ways that advance legality and judicial access, reveal shortfalls in judicial practice, and enable the provision of cheaper and better access to justice.
That potential has increased dramatically with the advent of AI and large language models (LLMs), such as ChatGPT.
“I had been writing about public law and technology, especially AI, for about five years. I became curious recently about why, of all the branches of government, only courts have been left largely to their own devices when it comes to collecting, archiving, and releasing information about its work,” said Huq.
While the legislative and executive branches have an extensive body of constitutional, statutory, and regulatory provisions channeling Congress and executive branch information—and countless public debates about transparency and opacity in and around both elected branches—the federal judiciary still relies on ad hoc procedures to determine what data to collect, preserve, and make available.
As a result, Huq and Clopton believe that “a lot of valuable data is either lost or stored in a way that makes it hard to use for the public good.”
Meanwhile, the authors note that large commercial firms such as Westlaw (owned by the Thomson Reuters Corporation), Lexis (owned by the RELX Group), and Bloomberg are moving to become the de facto data managers and gatekeepers who decide on the public flow of this information and who capture much of its value.
“At minimum, these developments should be the subject of more public discussion and scholarly debate,” said Huq. “Until now, however, one of the biggest obstacles to having that discussion is a lack of information about what data is at stake. It became apparent that we didn’t know why we knew what we knew, and we didn’t know what we didn’t know.”
The Scope of the Data
There were no studies about the full scope and depth of judicial data currently being preserved by the various courts’ disparate procedures—and no certainty about what other data could be preserved if there was a concerted effort to do so.
To fill that gap, Huq and Clopton drew on primary sources and previous scholarship, and then supplement ed that research with anonymized interviews with selected judicial staff and judges.
They quickly discovered that, with no regulatory framework to guide them, institutional practices varied widely among federal courts. Different courts save different types of data, organize it differently, and make different types available to the public.
Even significant judicial data that has been collected is often kept just out of reach. For example, the cover sheets that are filed in every civil case contain a treasure trove of useful information, such as the court’s basis of jurisdiction, the type of relief sought, and the nature of the suit .
“A comprehensive database of civil cover sheets,” the authors write, “would be an extremely valuable source of insight into the timing, cyclicality, substance, and distribution of civil litigation in federal courts.”
Defective Delivery of Data
While federal courts make some data available via the Public Access to Court Electronic Records (PACER) database, that archive is neither comprehensive nor easy to use, and with a 10 cents per page public access fee, expensive, especially for large research projects. Moreover, its search capabilities are limited; PACER does not allow the user to search by judge and does not permit full-text or natural-language searches.
The Federal Judicial Center ’ s Integrated Database suffers from similar defects, as do the courts’ various statistical reports.
Huq and Clopton’s paper demonstrates how these database design choices — kludgy interfaces, limited search options, requiring downloads to proceed page-by-page and at a fee — have the effect of partly privatizing this info by driving the public to commercial firms, who then get to decide what data they want to make available and at what price.
Data Should Generally Be Open, Not Opaque
In the authors’ view, openness and transparency are critical ingredients for making an institution that all Americans would recognize as a true “court.”
“To be clear,” Huq said, “we are not saying the courts must disclose everything. We recognize that there are privacy and other interests at stake and there needs to be some balance and debate around them. But we do believe there are some things we could all agree that the courts could be required to do now. So, our article focuses on that low-hanging fruit and seeks to provoke a conversation rather than partisanship.”
Huq and Clopton’s article will be published this summer by the Stanford Law Review .
Charles Williams is a freelance writer based in South Bend, Indiana.
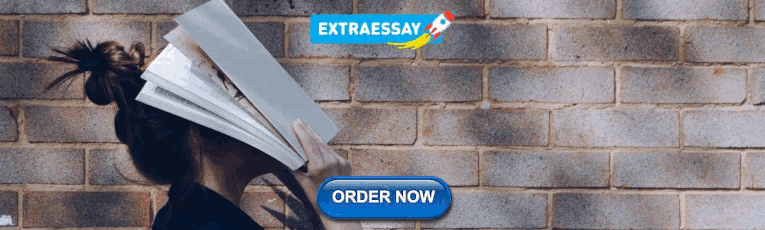
COMMENTS
In answer to RQ1, Table 3 shows an increasing interest in researching the potential of Twitter as a predictive system, with the highest research activity in 2020 and 2021, according to the number of publications. The evolution of artificial intelligence (AI) and machine learning, as represented in the case of Sentiment analysis as an NLP technique applied to the massive volume of texts ...
The use of social media across the educational landscape is on the rise. Subsequently, the body of research on this topic is vibrant and growing. In this article, we present findings from a review of 103 peer-reviewed scientific studies published over the last decade (2007-2017) that address the use of Twitter for educational purposes across formal and informal settings. The majority of the ...
The History and Demographics of Twitter. Even in internet terms, the history of Twitter is short, yet it has also been eventful. The site was launched in June 2006, offering users the ability to send 140 character messages (tweets) which would be, by default, publicly available to other users who chose to follow them (Sagolla Citation 2009).Subsequent developments on this basic service ...
This study collected relevant papers from three databases and applied text mining and trend analysis to detect semantic patterns and explore the yearly development of research themes across a ...
Twitter is a valuable science communication and marketing tool for academic journals to increase web-based visibility, promote research, and translate science to lay and scientific audiences. Four key Twitter strategies are implemented by medical journals: tweeting the title and link of the article, infographics, podcasts, and hosting monthly ...
Thus, a paper on "social media research" topic has an average additional e 1.278 = 3.589 Twitter mentions in the first year than papers on other topics (H1). For papers from the US and G11 countries, the expected additional Twitter mentions are 1.353 and 1.738 respectively (H2).
Social media is opening up new avenues for disseminating research outputs. While prior literature points to the essential role of Twitter in this context, evidence on what determines variation in researchers´ Twitter engagement remains scarce. In this account-level study of Twitter usage, we consider how research productivity, research quality, and participation in academic conferences relate ...
All social media platforms can be used to conduct social science research, but Twitter is the most popular as it provides its data via several Application Programming Interfaces, which allows qualitative and quantitative research to be conducted with its members. As Twitter is a huge universe, both in number of users and amount of data, sampling is generally required when using it for research ...
The prevalence of research using "big data" from Twitter is increasing and will likely continue to do so in the coming years . Infectious disease was the most common topic of the research papers, which may indicate a burgeoning interest in using social media to detect disease outbreaks.
Twitter sentiment analysis technology provides methods for polling public opinion on events or products. The majority of current research is aimed at obtaining sentiment features by analyzing ...
It is important to note that this paper only collects the opinions of people in England on Twitter about Covid-19; thus, the result should be interpreted by considering this limitation. To obtain a more convincing conclusion, we can increase the data size by incorporating longer timeline, wider geographies, or by collecting data via other ...
A guide to Twitter for researchers. A search in Google can show you a long list of academics explaining how Twitter has benefited their research, before and after publication. As a researcher, here is how Twitter can be a useful tool to you: Connect you with other academics in your field. Source for ideas by asking questions ("crowdsourcing")
As social media platforms or microblogging, Twitter allows for the free exchange of thoughts, at the national and international level, among individuals interested in comparable fields of ...
This article explores how Twitter has emerged as a signifier of contemporary protest. Using the concept of 'social media imaginaries', a derivative of the broader field of 'media imaginaries', our analysis seeks to offer new insights into activists' relation to and conceptualisation of social media and how it shapes their digital media practices.
Twitter has become a major social media platform and has attracted considerable interest among researchers in sentiment analysis. Research into Twitter Sentiment Analysis (TSA) is an active subfield of text mining. TSA refers to the use of computers to process the subjective nature of Twitter data, including its opinions and sentiments. In this research, a thorough review of the most recent ...
Although counts of tweets citing academic papers are used as an informal indicator of interest, little is known about who tweets academic papers and who uses Twitter to find scholarly information. Without knowing this, it is difficult to draw useful conclusions from a publication being frequently tweeted. This study surveyed 1,912 users that have tweeted journal articles to ask about their ...
Researchers have collected Twitter data to study a wide range of topics. This growing body of literature, however, has not yet been reviewed systematically to synthesize Twitter-related papers. The existing literature review papers have been limited by constraints of traditional methods to manually select and analyze samples of topically related papers. The goals of this retrospective study ...
Nature obtained the e-mail addresses of thousands of scientists who were identified through a social-media research project as having tweeted about papers on which they were a corresponding author 1.
Instead, false news speeds faster around Twitter due to people retweeting inaccurate news items. "When we removed all of the bots in our dataset, [the] differences between the spread of false and true news stood,"says Soroush Vosoughi, a co-author of the new paper and a postdoc at LSM whose PhD research helped give rise to the current study.
Sentiment analysis is the most trending and ongoing research in the field of data mining. Nowadays, several social media platforms are developed, among that twitter is a significant tool for sharing and acquiring peoples' opinions, emotions, views, and attitudes towards particular entities. This made sentiment analysis a fascinating process in the natural language processing (NLP) domain.
Tweeting your paper doesn't boost citations. Posting about a paper on X (formerly Twitter) seems to boost engagement but doesn't translate into a bump in citations. A group of 11 researchers ...
Research into Twitter Sentiment Analysis (TSA) is an active subfield of text mining. ... T able 1 displays the abbreviation descriptions mentioned in this paper. To gain a better. understanding of ...
Background: A silver lining to the COVID-19 pandemic is that it cast a spotlight on a long-underserved group. The barrage of attacks against older Asian Americans during the crisis galvanized society into assisting them in various ways. On Twitter, now known as X, support for them coalesced around the hashtag #ProtectOurElders. To date, discourse surrounding older Asian Americans has escaped ...
Detecting problematic research articles timely is a vital task. This study explores whether Twitter mentions of retracted articles can signal potential problems with the articles prior to retraction, thereby playing a role in predicting future retraction of problematic articles. A dataset comprising 3,505 retracted articles and their associated Twitter mentions is analyzed, alongside 3,505 non ...
Click the hamburger menu to open the sidebar. Select Alerts to open a new page. Click the red Create alert button and insert the keywords for which Google Scholar should look. Select Update ...
Sentiment analysis is a natural language processing. techniques to quantify an expressed opinion or sentimen t. within a selection of tweets [8]. 2014 International Conference on Information ...
GTC— Powering a new era of computing, NVIDIA today announced that the NVIDIA Blackwell platform has arrived — enabling organizations everywhere to build and run real-time generative AI on trillion-parameter large language models at up to 25x less cost and energy consumption than its predecessor. The Blackwell GPU architecture features six ...
The Institutional Review Board (IRB) reviews research involving human participants to ensure that the rights and welfare of human participants are protected. The IRB has the authority to approve, require modifications, disapprove, suspend, terminate and observe the consenting process for research that falls within its scope of review, as ...
The basic information and knowledge to sentiment analysis of twitter are briefly discussed in this review paper. Sentiment Analysis can be viewed as a field of text mining, NLP (Natural Language ...
Editor's Note: This story is the first in an occasional series on research projects currently in the works at the Law School. Every court case and judicial proceeding generates an enormous amount of data, some of which is either non-public or difficult to access. What to do with that data is a question that Aziz Z. Huq, the Frank and Bernice J. Greenberg Professor at the Law School has been ...