- Diversity & Inclusion
- Board of Directors
- Past Leadership
- Science Advocate Newsletter
- Press Releases
- Member Benefits
- AADOCR Community
- AADOCR Sections
- Scientific Groups & Networks
- Student Members (NSRG)
- 2024 Annual Meeting
- 2025 Annual Meeting
- Upcoming Events
- Webinars & Continuing Education
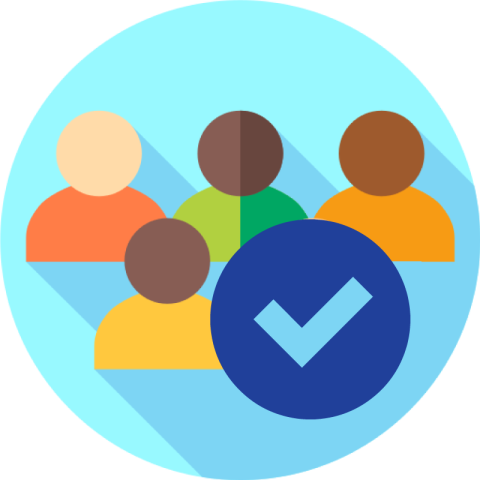
- Search Awards
- Access Journals
- Donate to AADOCR
- Contact AADOCR HQ
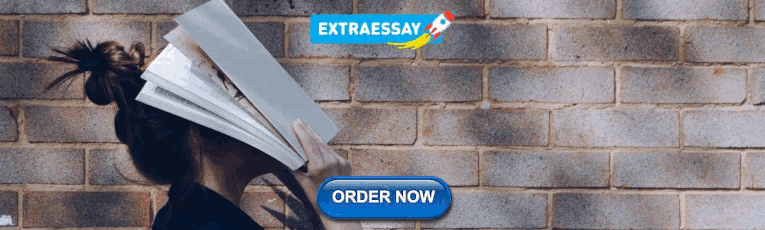
Journal of Dental Research 2020 Journal Impact Factor™ Over 6 For the First Time
Alexandria, Va., USA — The International and American Associations for Dental Research announce that the Journal of Dental Research ( JDR) 2-year Journal Impact Factor™ is over 6 for the first time at 6.116, ranking #5 of 91 journals in the “Dentistry, Oral Surgery & Medicine” category, and the JDR 5-year Journal Impact Factor™ is over 7 for the first time at 7.199 score, ranking #3 of 91 journals.
The JDR also ranks #1 of 91 journals in total citations and Eigenfactor, #2 in the new Journal Citation Index and #3 in Article Influence Score. This news comes from the 2021 Journal Citation Reports ™ (Clarivate™, 2020).
Full 2021 Journal Citation Reports ™ results for the JDR :
- 2-year Journal Impact Factor™: 6.116, ranking #5 of 91 journals
- 5-year Journal Impact Factor™: 7.199, ranking #3 of 91 journals
- Eigenfactor: 0.01683, ranking #1 of 91 journals
- Total citations: 26,197, ranking #1 of 91 journals
- Journal Citation Indicator: 2.39, ranking #2 of 91 journals
- Article Influence Score: 1.726, ranking #3 of 91 journals
The 2-year Journal Impact Factor™ is defined as citations to the journal in the Journal Citation Reports ™ year to items published in the previous two years, divided by the total number of scholarly items, also known as citable items, including articles and reviews published in the journal in the previous two years.
New in 2021 is the Journal Citation Indicator. Developed by the Institute for Scientific Information (ISI)™ at Clarivate™, this new metric represents the average category-normalized citation impact for papers published in the prior three-year period, providing a single journal-level metric that can be easily interpreted and compared across disciplines. The Journal Citation Indicator will be calculated for all journals in the Web of Science Core Collection – including those that do not have a Journal Impact Factor (JIF)™.
“I am thrilled to see the groundbreaking scores for the 2-year and 5-year Journal Impact Factor™ and to see the JDR continue to rank #1 in Eigenfactor and total citations,” said JDR Editor-in-Chief Nicholas Jakubovics. “For over 100 years the JDR has been a top journal in our field and we owe this achievement to the researchers who submit their best science to the JDR and also to all the current and former Editors, Associate Editors, reviewers and Editorial Board members that ensure the JDR continues to publish science of the highest quality.”
About the Journal of Dental Research
The IADR/AADR Journal of Dental Research ( JDR ) is a multidisciplinary journal dedicated to the dissemination of new knowledge in all sciences relevant to dentistry and the oral cavity and associated structures in health and disease. The JDR 2-year Journal Impact Factor™ is 6.116, ranking #5 of 91 journals in the “Dentistry, Oral Surgery & Medicine” category, and the JDR 5-year Journal Impact Factor™ is 7.199. The JDR ranks #1 of 91 journals in total citations at 26,197 and Eigenfactor at 0.01683. The JDR Editor-in-Chief is Nicholas Jakubovics, Newcastle University, England. Follow the JDR on Twitter at @JDentRes !
International Association for Dental Research
The International Association for Dental Research (IADR) is a nonprofit organization with over 10,000 individual members worldwide, with a mission to drive dental, oral and craniofacial research for health and well-being worldwide. To learn more, visit www.iadr.org . The American Association for Dental Research (AADR) is the largest Division of IADR with 3,100 members in the United States. To learn more, visit www.iadr.org/aadr .
We respect your right to privacy.
By continuing to explore our site, you are agreeing to our Terms of Service and Privacy Policy. Learn more about our terms and conditions here.
- Technical Support
- Find My Rep
You are here
Journal of Dental Research
Preview this book.
- Description
- Aims and Scope
- Editorial Board
- Abstracting / Indexing
- Submission Guidelines
The journal for dental, oral and craniofacial research. View the Journals Subscription Package for the Journal of Dental Research (JDR) and JDR Clinical & Translational Research, including Advances in Dental Research (ADR) and Critical Reviews in Oral Biology & Medicine , Vol 1-15. Journal of Dental Research (JDR) is a peer-reviewed scientific journal dedicated to the dissemination of new knowledge and information on all sciences relevant to dentistry and to the oral cavity and associated structures in health and disease.
JDR is published monthly, allowing for frequent dissemination of its leading content. Rapid publication from acceptance to print provides timely communication of the latest research to the oral and dental community.
- Original research in all fields of dental, oral and craniofacial sciences
- Biological, Clinical and Biomaterials & Bioengineering sections for original research articles
- Timely state-of-the-art review articles in the Critical Reviews in Oral Biology & Medicine (CROBM) section
- Erudite articles on noteworthy topics of research interest in the DISCOVERY!
- Leading meeting proceedings in the e-supplement, Advances in Dental Research (see below)
- Oral, dental and craniofacial researchers and clinical scientists
- Oral and dental policy-makers
- Dental educators
- Hard-tissue scientists
Additional JDR information
Notice : Please note that no issues were published in 1925, 1926, and 1936. Issue 15:4 and 24:4 were also not published. Volume and pagination sequencing skip over these breaks in publication; for example, Volume 6 was published in 1924 and Volume 7 in 1927.
Advances in Dental Research (ADR) is an e-supplement to JDR . Subscribers to J DR receive access to all new ADR content.
The journal Critical Reviews in Oral Biology & Medicine (CROBM) was merged into the JDR . Subscriptions to CROBM are no longer available, but all content from Volume 1, Issue 1 (1990) to Volume 15, Issue 1 (2004) is hosted online and accessible with your JDR subscription. Members of the International Association for Dental Research (IADR) receive complimentary online access to the JDR and reduced print subscription pricing. For more information about joining IADR please click here or if you are already a member and are trying to access JDR , please click here . This journal is a member of the Committee on Publication Ethics (COPE)
The Journal of Dental Research® (print ISSN: 0022-0345; online ISSN: 1544-0591) is published by the International & American Associations for Dental Research, and is dedicated to the dissemination of new knowledge and information on all sciences relevant to dentistry and to the oral cavity and associated structures in health and disease. The JDR publishes original research in all fields of dental, oral and craniofacial sciences.
- CABI: CAB Abstracts
- Chemical Abstracts Service (CAS)
- Clarivate Analytics: BIOSIS Previews
- Clarivate Analytics: Biological Abstracts
- Clarivate Analytics: Current Contents - Life Sciences
- Clarivate Analytics: Science Citation Index Expanded (SCIE)
- Mosby's Index
- PubMed: MEDLINE
The Journal of Dental Research ( JDR ) is a peer-reviewed scientific journal dedicated to the dissemination of new knowledge and information on all science relevant to dentistry and to the oral cavity and associated structures in health and disease. The Journal of Dental Research ’s broad readership consists of oral, dental and craniofacial researchers, clinical scientists, dentists, dental educators, and oral and dental policy-makers. The Journal is published monthly, allowing for frequent dissemination of its leading content. The Journal of Dental Research also offers OnlineFirst, by which forthcoming articles are published online before they are scheduled to appear in print. Average time from submission to first decision: 18 days.
Authors of all types of articles should be aware of the following guidelines when submitting to JDR . Please note that if you have been invited to submit an article for Advances in Dental Research , an e-supplement to JDR , please refer to the JDR Instructions for Authors.
The Journal of Dental Research has changed editorial styles to CSE (8th Edition). Submitted manuscripts should now be formatted in that style.
View Instructions to Authors Download a PDF of the Instructions to Authors Submit a manuscript
Journal of Dental Research Editorial Office: [email protected] +1.703-299.8080
- Read Online
- Sample Issues
- Current Issue
- Email Alert
- Permissions
- Foreign rights
- Reprints and sponsorship
- Advertising
Individual Subscription, Print Only
Individual, Single Print Issue
Institutional, Single Print Issue
To order single issues of this journal, please contact SAGE Customer Services at 1-800-818-7243 / 1-805-583-9774 with details of the volume and issue you would like to purchase.
- DEIAB Statement
- Board of Directors
- Past Leadership
- Global Research Update
- Press Releases
- Member Benefits
- Regions/Divisions/Sections
- Scientific Groups & Networks
- IADR Community
- 2024 IADR General Session
- 2025 IADR General Session
- Upcoming Events
- Webinars & Continuing Education
- Event Policies
- Policy Statement
- Declarations
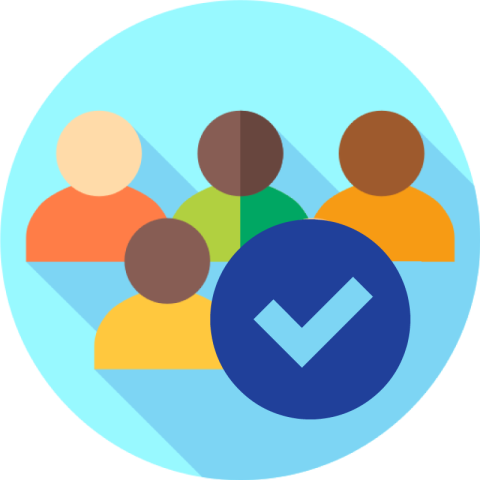
- Search Awards
- Access Journals
- Contact IADR GHQ
- Give to IADR
Journal of Dental Research Announces New Impact Factor™
Alexandria, VA – The International Association for Dental, Oral, and Craniofacial Research and American Association for Dental, Oral, and Craniofacial Research announced the Journal of Dental Research (JDR) 2-Year Journal Impact Factor™ is now 7.6, ranking it #3 of 91 journals in the “Dentistry, Oral Surgery & Medicine” category.
The JDR 5-year Journal Impact Factor™ is also 7.6, with an Immediacy Index of 1.1 and an article Influence score of 1.638. The JDR once again ranked #1 of 91 journals in total citations, with a total of 25,849 in 2022, and ranked #3 in Eigenfactor with a score of 0.01345.
The 2-year Journal Impact Factor™ is defined as citations to the journal in the Journal Citation Reports™ (Clarivate™, 2023) year to items published in the previous two years, divided by the total number of scholarly items, also known as citable items, including articles and reviews published in the journal in the previous two years.
About the Journal of Dental Research The IADR/AADOCR Journal of Dental Research (JDR) is a multidisciplinary journal dedicated to the dissemination of new knowledge in all sciences relevant to dentistry and the oral cavity and associated structures in health and disease. The JDR Editor-in-Chief is Nicholas Jakubovics, Newcastle University, England. Follow the JDR on Twitter at @JDentRes!
About IADR The International Association for Dental, Oral, and Craniofacial Research (IADR) is a nonprofit organization with a mission to drive dental, oral, and craniofacial research for health and well-being worldwide. IADR represents the individual scientists, clinician-scientists, dental professionals, and students based in academic, government, non-profit, and private-sector institutions who share our mission. Learn more at www.iadr.org .
About AADOCR The American Association for Dental, Oral, and Craniofacial Research (AADOCR) is a nonprofit organization with a mission to drive dental, oral, and craniofacial research to advance health and well-being. AADOCR represents the individual scientists, clinician-scientists, dental professionals, and students based in academic, government, non-profit, and private-sector institutions who share our mission. AADOCR is the largest division of IADR. Learn more at www.aadocr.org .
We respect your right to privacy.
By continuing to explore our site, you are agreeing to our Terms of Service and Privacy Policy. Learn more about our terms and conditions here.
- All subject areas
- Agricultural and Biological Sciences
- Arts and Humanities
- Biochemistry, Genetics and Molecular Biology
- Business, Management and Accounting
- Chemical Engineering
- Computer Science
- Decision Sciences
- Earth and Planetary Sciences
- Economics, Econometrics and Finance
- Engineering
- Environmental Science
- Health Professions
- Immunology and Microbiology
- Materials Science
- Mathematics
- Multidisciplinary
- Neuroscience
- Pharmacology, Toxicology and Pharmaceutics
- Physics and Astronomy
- Social Sciences
- All subject categories
- Dental Assisting
- Dental Hygiene
- Dentistry (miscellaneous)
- Oral Surgery
- Orthodontics
- Periodontics
- All regions / countries
- Asiatic Region
- Eastern Europe
- Latin America
- Middle East
- Northern America
- Pacific Region
- Western Europe
- ARAB COUNTRIES
- IBEROAMERICA
- NORDIC COUNTRIES
- Afghanistan
- Bosnia and Herzegovina
- Brunei Darussalam
- Czech Republic
- Dominican Republic
- Netherlands
- New Caledonia
- New Zealand
- Papua New Guinea
- Philippines
- Puerto Rico
- Russian Federation
- Saudi Arabia
- South Africa
- South Korea
- Switzerland
- Syrian Arab Republic
- Trinidad and Tobago
- United Arab Emirates
- United Kingdom
- United States
- Vatican City State
- Book Series
- Conferences and Proceedings
- Trade Journals

- Citable Docs. (3years)
- Total Cites (3years)

Follow us on @ScimagoJR Scimago Lab , Copyright 2007-2024. Data Source: Scopus®

Cookie settings
Cookie Policy
Legal Notice
Privacy Policy

- News Releases
Journal of Dental Research 2020 Journal Impact Factor™ over 6 for the first time
International Association for Dental, Oral, and Craniofacial Research
Alexandria, Va., USA -- The International and American Associations for Dental Research announce that the Journal of Dental Research (JDR) 2-year Journal Impact Factor™ is over 6 for the first time at 6.116, ranking #5 of 91 journals in the "Dentistry, Oral Surgery & Medicine" category, and the JDR 5-year Journal Impact Factor™ is over 7 for the first time at 7.199 score, ranking #3 of 91 journals.
The JDR also ranks #1 of 91 journals in total citations and Eigenfactor, #2 in the new Journal Citation Index and #3 in Article Influence Score. This news comes from the 2021 Journal Citation Reports™ (Clarivate™, 2020).
Full 2021 Journal Citation Reports™ results for the JDR:
The 2-year Journal Impact Factor™ is defined as citations to the journal in the Journal Citation Reports™ year to items published in the previous two years, divided by the total number of scholarly items, also known as citable items, including articles and reviews published in the journal in the previous two years.
New in 2021 is the Journal Citation Indicator. Developed by the Institute for Scientific Information (ISI)™ at Clarivate™, this new metric represents the average category-normalized citation impact for papers published in the prior three-year period, providing a single journal-level metric that can be easily interpreted and compared across disciplines. The Journal Citation Indicator will be calculated for all journals in the Web of Science Core Collection - including those that do not have a Journal Impact Factor (JIF)™.
"I am thrilled to see the groundbreaking scores for the 2-year and 5-year Journal Impact Factor™ and to see the JDR continue to rank #1 in Eigenfactor and total citations," said JDR Editor-in-Chief Nicholas Jakubovics. "For over 100 years the JDR has been a top journal in our field and we owe this achievement to the researchers who submit their best science to the JDR and also to all the current and former Editors, Associate Editors, reviewers and Editorial Board members that ensure the JDR continues to publish science of the highest quality."
The IADR/AADR Journal of Dental Research (JDR) is a multidisciplinary journal dedicated to the dissemination of new knowledge in all sciences relevant to dentistry and the oral cavity and associated structures in health and disease. The JDR 2-year Journal Impact Factor™ is 6.116, ranking #5 of 91 journals in the "Dentistry, Oral Surgery & Medicine" category, and the JDR 5-year Journal Impact Factor™ is 7.199. The JDR ranks #1 of 91 journals in total citations at 26,197 and Eigenfactor at 0.01683. The JDR Editor-in-Chief is Nicholas Jakubovics, Newcastle University, England. Follow the JDR on Twitter at @JDentRes!
International Association for Dental Research
The International Association for Dental Research (IADR) is a nonprofit organization with over 10,000 individual members worldwide, with a mission to drive dental, oral and craniofacial research for health and well-being worldwide. To learn more, visit http://www.iadr.org . The American Association for Dental Research (AADR) is the largest Division of IADR with 3,100 members in the United States. To learn more, visit http://www.iadr.org/aadr .
Journal of Dental Research
Disclaimer: AAAS and EurekAlert! are not responsible for the accuracy of news releases posted to EurekAlert! by contributing institutions or for the use of any information through the EurekAlert system.
Original Source
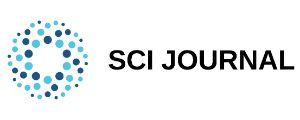
Journal of Dental Research Impact Factor & Key Scientometrics
Journal of dental research overview, impact factor.
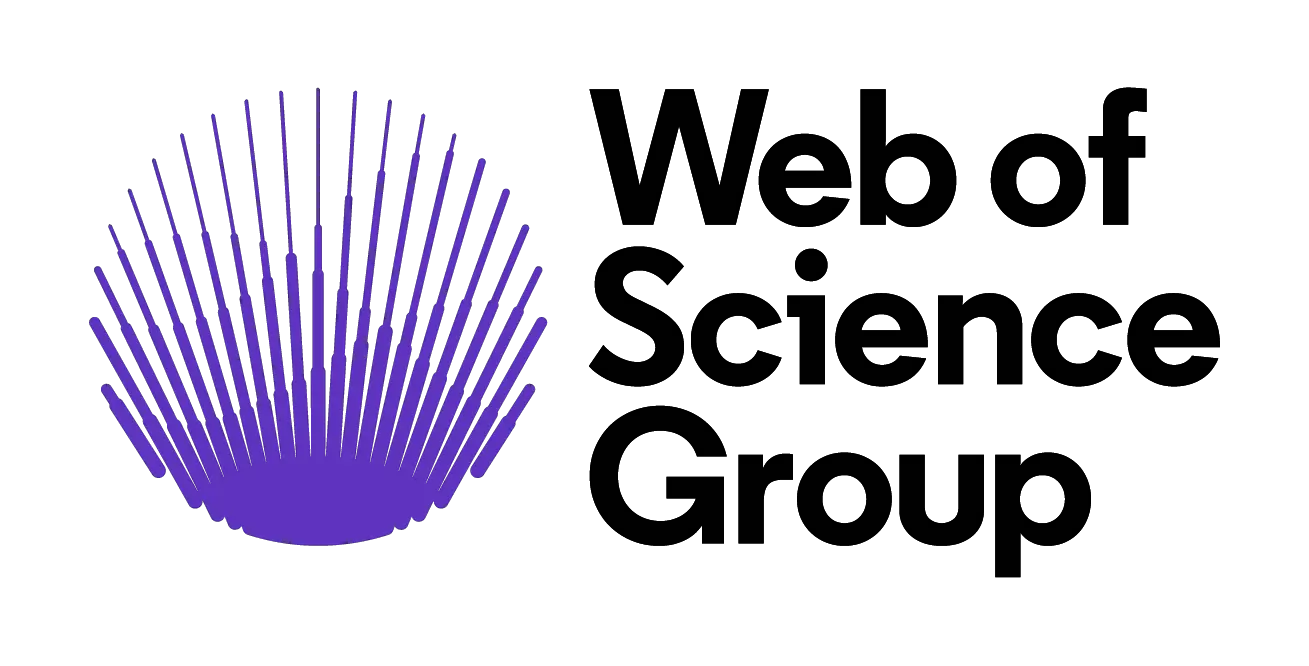
I. Basic Journal Info

Journal ISSN: 220345
Publisher: sage publications, history: 1919-1924, 1927-1935, 1937-ongoing, journal hompage: link, how to get published:, research categories, scope/description:.
--------------------------------
Best Academic Tools
- Academic Writing Tools
- Proofreading Tools
- Academic Search Engines
- Project Management Tools
- Survey Tools for Research
- Transcription Tools
- Reference Management Software
- AI-Based Summary Generators
- Academic Social Network Sites
- Plagiarism Checkers
- Science Communication Tools
- Jasper AI Review
II. Science Citation Report (SCR)
Journal of dental research scr impact factor, journal of dental research scr journal ranking, journal of dental research scimago sjr rank.
SCImago Journal Rank (SJR indicator) is a measure of scientific influence of scholarly journals that accounts for both the number of citations received by a journal and the importance or prestige of the journals where such citations come from.
Journal of Dental Research Scopus 2-Year Impact Factor Trend
Journal of dental research scopus 3-year impact factor trend, journal of dental research scopus 4-year impact factor trend, journal of dental research impact factor history.
- 2022 Impact Factor 7.719 7.337 7.602
- 2021 Impact Factor 7.347 7.545 7.682
- 2020 Impact Factor 4.894 5.495 5.859
- 2019 Impact Factor 4.871 5.285 5.648
- 2018 Impact Factor 4.995 5.362 5.643
- 2017 Impact Factor 5.19 5.59 5.638
- 2016 Impact Factor 4.851 5.067 5.167
- 2015 Impact Factor 4.918 5.291 5.398
- 2014 Impact Factor 4.459 NA NA
- 2013 Impact Factor 4.222 NA NA
- 2012 Impact Factor 4.211 NA NA
- 2011 Impact Factor 3.641 NA NA
- 2010 Impact Factor 3.553 NA NA
- 2009 Impact Factor 3.433 NA NA
- 2008 Impact Factor 3.156 NA NA
- 2007 Impact Factor 3.356 NA NA
- 2006 Impact Factor 3.175 NA NA
- 2005 Impact Factor 2.705 NA NA
- 2004 Impact Factor 2.536 NA NA
- 2003 Impact Factor 2.075 NA NA
- 2002 Impact Factor 1.729 NA NA
- 2001 Impact Factor 1.693 NA NA
- 2000 Impact Factor 1.863 NA NA
See what other people are reading
HIGHEST PAID JOBS
- Highest Paying Nursing Jobs
- Highest Paying Non-Physician Jobs
- Highest Paying Immunology Jobs
- Highest Paying Microbiology Jobs
LATEX TUTORIALS
- LaTeX Installation Guide – Easy to Follow Steps to Install LaTeX
- 6 Easy Steps to Create Your First LaTeX Document
- How to Use LaTeX Paragraphs and Sections
- How to Use LaTeX Packages with Examples
MUST-READ BOOKS
- Multidisciplinary
- Health Science
Impact factor (IF) is a scientometric factor based on the yearly average number of citations on articles published by a particular journal in the last two years. A journal impact factor is frequently used as a proxy for the relative importance of a journal within its field. Find out more: What is a good impact factor?
III. Other Science Influence Indicators
Any impact factor or scientometric indicator alone will not give you the full picture of a science journal. There are also other factors such as H-Index, Self-Citation Ratio, SJR, SNIP, etc. Researchers may also consider the practical aspect of a journal such as publication fees, acceptance rate, review speed. ( Learn More )
Journal of Dental Research H-Index
The h-index is an author-level metric that attempts to measure both the productivity and citation impact of the publications of a scientist or scholar. The index is based on the set of the scientist's most cited papers and the number of citations that they have received in other publications
Journal of Dental Research H-Index History
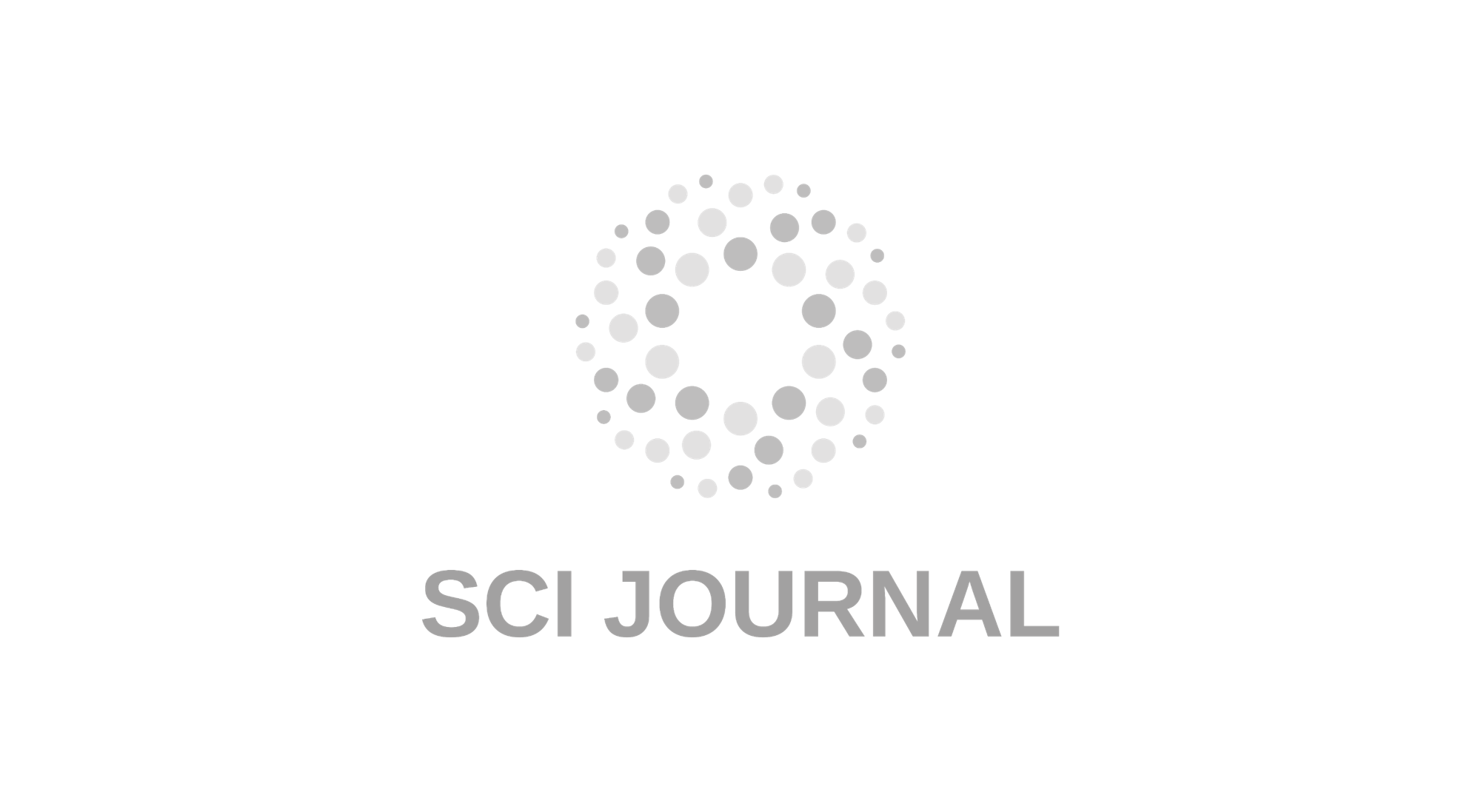
scijournal.org is a platform dedicated to making the search and use of impact factors of science journals easier.
Click through the PLOS taxonomy to find articles in your field.
For more information about PLOS Subject Areas, click here .
Loading metrics
Open Access
Peer-reviewed
Research Article
Analysis of factors influencing the degree of accidental injury of bicycle riders considering data heterogeneity and imbalance
Roles Conceptualization, Data curation, Methodology, Writing – review & editing
Affiliation School of Automobile and Transportation, Xihua University, Chengdu, China
Roles Conceptualization, Methodology, Validation
* E-mail: [email protected]
Affiliations School of Automobile and Transportation, Xihua University, Chengdu, China, Vehicle Measurement Control and Safety Key Laboratory of Sichuan Province, Xihua University, Chengdu, China

Roles Data curation, Investigation, Software
Affiliations School of Automobile and Transportation, Xihua University, Chengdu, China, Faculty of Engineering and Technology, Sichuan Sanhe College of Professionals, Luzhou, China
Roles Supervision, Validation
Affiliation Computer and Mathematical Sciences, The University of Adelaide, North Terrace Adelaide, Adelaide, Australia
- Xinchi Dong,
- Daowen Zhang,
- Chaojian Wang,
- Tianshu Zhang
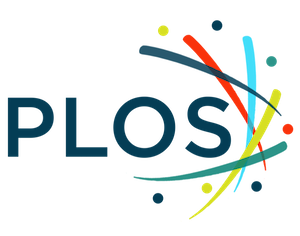
- Published: May 14, 2024
- https://doi.org/10.1371/journal.pone.0301293
- Reader Comments
Bicycle safety has emerged as a pressing concern within the vulnerable transportation community. Numerous studies have been conducted to identify the significant factors that contribute to the severity of cyclist injuries, yet the findings have been subject to uncertainty due to unobserved heterogeneity and class imbalance. This research aims to address these issues by developing a model to examine the impact of key factors on cyclist injury severity, accounting for data heterogeneity and imbalance. To incorporate unobserved heterogeneity, a total of 3,895 bicycle accidents were categorized into three homogeneous sub-accident clusters using Latent Class Cluster Analysis (LCA). Additionally, five over-sampling techniques were employed to mitigate the effects of data imbalance in each accident cluster category. Subsequently, Bayesian Network (BN) structure learning algorithms were utilized to construct 32 BN models after pairing the accident data from the four accident cluster types before and after sampling. The optimal BN models for each accident cluster type provided insights into the key factors associated with cyclist injury severity. The results indicate that the key factors influencing serious cyclist injuries vary heterogeneously across different accident clusters. Female cyclists, adverse weather conditions such as rain and snow, and off-peak periods were identified as key factors in several subclasses of accident clusters. Conversely, factors such as the week of the accident, characteristics of the trafficway, the season, drivers failing to yield to the right-of-way, distracted cyclists, and years of driving experience were found to be key factors in only one subcluster of accident clusters. Additionally, factors such as the time of the crash, gender of the cyclist, and weather conditions exhibit varying levels of heterogeneity across different accident clusters, and in some cases, exhibit opposing effects.
Citation: Dong X, Zhang D, Wang C, Zhang T (2024) Analysis of factors influencing the degree of accidental injury of bicycle riders considering data heterogeneity and imbalance. PLoS ONE 19(5): e0301293. https://doi.org/10.1371/journal.pone.0301293
Editor: Quan Yuan, Tsinghua University, CHINA
Received: May 22, 2023; Accepted: March 12, 2024; Published: May 14, 2024
Copyright: © 2024 Dong et al. This is an open access article distributed under the terms of the Creative Commons Attribution License , which permits unrestricted use, distribution, and reproduction in any medium, provided the original author and source are credited.
Data Availability: All relevant data are within the manuscript and its Supporting information files.
Funding: We have amended the Financial Disclosure to read: the Project of National Automobile Accident In-depth Investigation System (202248), the Project of Vehicle Measurement Control and Safety Key Laboratory of Sichuan Province in China (QCCK2021-011).
Competing interests: The authors have declared that no competing interests exist.
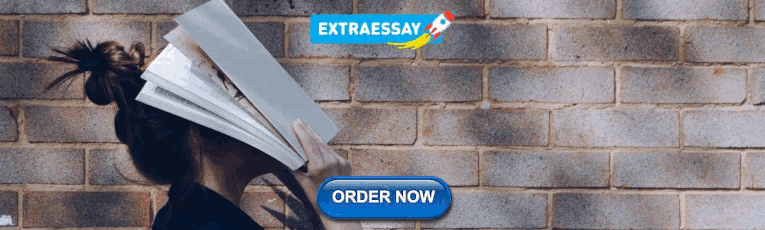
1 Introduction
Bicycle travel is considered a sustainable and low-carbon mode of transportation with numerous socio-economic and personal health benefits [ 1 ]. However, cyclists are particularly vulnerable road users, lacking the protection afforded by motorists, and therefore, they are at a higher risk of sustaining fatal injuries in collisions [ 2 ]. Ensuring the safety of cyclists has become a major concern for traffic engineers and policymakers [ 3 ]. According to the World Health Organization (WHO), over 1.35 million people worldwide will lose their lives in road traffic crashes during 2020, with approximately 41,000 of these fatalities involving cyclists, accounting for 3% of global road traffic deaths [ 4 ]. Moreover, data on traffic fatalities from the National Highway Traffic Safety Administration (NHTSA) in 2018 revealed an alarming increase in cyclist deaths, reaching a nearly two-decade high [ 5 ]. With the rise of sustainable urban transportation and the growing popularity of bike-sharing programs, the use of bicycles on urban roads has surged, making bicycle safety a critically important issue for cyclists [ 6 ]. Therefore, it is imperative to investigate the factors that contribute to the severity of injuries in road bicycle accidents and develop targeted measures to mitigate the occurrence and reduce the severity of these incidents.
In general, researchers base their work on traffic accident data and use statistical methods or machine learning methods to identify the key factors that influence accident severity. In particular, traffic accidents are complex multifactorial events that occur under different conditions with a complex occurrence process, and the pattern of factor effects on accidents is not fully revealed, and significant unobserved heterogeneity can also exist. Because accidents are episodic events with a much lower number of serious and fatal categories than minor injuries, accident data usually suffer from category imbalance [ 7 ]. It is worth noting that if the problem of data heterogeneity and imbalance is ignored when constructing an analytical model of factors, it may lead to incorrect parameter estimation of the models, reduce the ability of the models to identify risk factors, or even draw biased inferences for safety analysis [ 8 ]. In addition, most of the existing research methods are based on derivative classes of parametric models with specific assumptions such as Logit and Probit, and the non-collinearity requirement of independent variables in this class of methods can limit the introduction of logically relevant variables, failing to fully explore risk factors [ 9 ].
Therefore, the present study aimed to reduce the impact of heterogeneity and imbalance among accident data on the analysis results as well as to achieve a quantitative analysis of accident factors. The rest of the paper is organized as follows: section 2 provides a literature review that summarizes previous studies in terms of content and methodology; Section 3 explains the study data; Section 4 describes the methodology used; Sections 5 and 6 provide an analysis and discussion of the results of the model calculations, respectively; and, finally, the main findings of this study are summarized in section 7.
2 Literature review
At present, scholars have explored various factors influencing the severity of cyclist injuries based on traffic accident data, which can be broadly classified into two categories, namely, micro factors and macro factors. Micro factors include cyclist factors and driver factors (age and gender), whereas macro factors include bicycle factors, vehicle factors (vehicle type and collision location), road factors (road speed limit and road conditions), environmental factors (weather and light conditions), and time factors (season and holidays) [ 10 ]. Fewer studies have performed macroscopic analysis, focusing on the numerical safety effects of socioeconomic, demographic, commuting, and infrastructure factors on injury severity or accident rates, which can provide a scientific basis for decision-makers to reduce cycling risks at the planning level.
In order to explore the relationship between different factors and traffic accidents, many scholars have applied regression analysis-type methods and confirmed that there are statistical associations between driver, vehicle, road, and environmental factors and traffic accident risk [ 11 – 13 ]. However, this methodology makes it difficult to identify specific influencing factors and their expressions and ignores the diversity of influencing factors and the unobservability of some factors [ 14 ]. In addition, when the regression analysis method is used to evaluate the frequency of traffic accidents, the discrete distribution of traffic accident data and the limited sample size, which are common in traffic accident data, may also affect the effectiveness of data fitting [ 15 ].
To this end, researchers have used machine learning models such as Decision Tree (DT) models, BN models, Neural Network (NN) models, and Support Vector Machines (SVM) for traffic accident rate prediction and analysis and accident risk evaluation, taking advantage of the strengths of machine learning models in dealing with small-sample, nonlinear, and high-dimensional traffic accident data [ 16 – 18 ]. Although these models have strong predictive and analytical capabilities, they are weak in explaining the differences between risk factors, ignoring the degree of influence of different risk factors on accident risk evaluation, and may also lead to biased estimation of traffic accident risk evaluation. Meanwhile, accident categories in accident data are unbalanced, and for dichotomous accidents, there are fewer fatal accidents than non-fatal accidents, which challenges the performance of machine learning models. Since machine learning algorithms inherently assume that the data categories are balanced, machine learning models built on unbalanced data are usually biased toward the majority of the categories in classification, resulting in samples from the minority of the categories being susceptible to misclassification. In addition, Ignoring the imbalanced nature of the data in the modeling process can also lead to incorrect estimation of the model parameters and failure to fully explore the relationship between factors and injury severity. There are two main types of solutions to the imbalance problem as follows: algorithm-level methods and data-level methods. The algorithm-level approach is mainly cost-sensitive learning, which defines a "cost matrix" to assign different misclassification costs to the desired category, but it is difficult to obtain the best misclassification costs for each category [ 19 ]. Therefore, scholars mostly use the data-level approach, i.e., data resampling. Domestic and foreign scholars have studied the influencing factors of accident categories using different resampling techniques combined with machine learning algorithms, such as Decision Trees (DT) and XG boost, and they have found that resampling effectively improves the classification performance of models in a few classes and the ability to identify risk factors.
Heterogeneity refers to the fact that the influence of certain factors incorporated into the model on the accident consequences is not constant in all cases, but may vary randomly, and it is one of the main problems of the modeling process. If the heterogeneity of the data is ignored in the modeling process, it can lead to incorrect estimation of model parameters or even to wrong inferences [ 20 ]. Because traditional statistical and machine learning methods cannot adequately observe the heterogeneity of data, models that can account for unobserved heterogeneity have been introduced into traffic accident analysis, in which random parameters Logit and LCA are widely used [ 21 ].
Although the heterogeneity of the data can be observed with the random parameter Logit model, the method presupposes that the random parameters obey a normal or uniform distribution and ignores the possibility that the random parameters follow a complex, multistate distribution. To overcome this drawback, the entire dataset can be divided into sub-datasets of different clusters, which maximizes both the heterogeneity effect of the inter-group dataset and the homogeneity of the intra-group dataset. Thus, potential category analysis based on the idea of clustering has been applied to the field of traffic safety analysis. Lin Z et al. [ 22 ] based on NHTSA bicycle crash data, the entire dataset was divided into seven sub-datasets using LCA, and models were constructed for the overall dataset and the seven sub-datasets. The sub-models had better-fit superiority than the individual models developed using the entire dataset. Some of the variables had significant effects only in specific groups, and the same variable may have different levels of effect in different groups.
Therefore, the present study proposed a two-stage method integrating LCA, over-sampling, and BN based on the Crash Report Sampling System (CRSS) database from 2016–2020, considering bicycle accidents as the research object. It is intended to reduce the influence of data heterogeneity and imbalance from the method level and realize the quantitative analysis of accident causal factors. From the application level, the focus of bicycle accident prevention is clarified, and then precise prevention and control strategies are implemented.
3 Data description and preprocessing
The National Highway Traffic Safety Administration (NHTSA) has collected motor vehicle traffic crash data since the early 1970s to support its mission to reduce motor vehicle traffic crashes, injuries, and deaths on our Nation’s trafficways. NHTSA uses data from many sources, including the Crash Report Sampling System (CRSS) [ 23 ]. CRSS is a sample of police-reported crashes involving all types of motor vehicles, pedestrians, and cyclists, ranging from property damage-only crashes to those that result in fatalities. To investigate the relationship between the severity of cyclist injuries and the influencing factors, the present study searched the CRSS database for bicycle accidents occurring in urban areas from 2016 to 2020. The inclusion criteria were as follows:
- The 5-year crash data provide a larger sample size, while the shorter time span allows controlling for changes in road and traffic conditions nationwide and reducing the effect of temporal instability [ 24 ]; and
- Because the sample size of BSV crashes was small and BMV crashes were the main type of fatal bicycle accidents, BMV crashes were mainly considered in the present study. In addition, only collisions involving a motor vehicle (motorcycles were excluded due to the small number) and a pedal bike were screened for one-to-one driver-cyclist correspondence to reduce the influence of other potential risk factors. The exclusion criteria were as follows: (a) variables not relevant to the present study; (b) when missing values were higher than 30%; and (c) when the number of crashes with a given value was more than 95% of the total number of crashes.
Data for 3895 bicycle accidents in urban areas were obtained, and the original database classified the accident severity into the following five classes:
No Apparent Injury is a situation where there is no reason to believe that the person received any bodily harm from the motor vehicle crash. There is no physical evidence of injury and the person does not report any change in normal function (1.41%).
Possible Injury is any injury reported or claimed that is not a fatal injury, suspected serious injury, or suspected minor injury. Examples include momentary loss of consciousness, possible concussion, claim of injury limping, complaint of pain, or nausea (38.64%).
Minor Injury is any injury that is evident at the scene of the crash, other than fatal or serious injuries. Examples include a lump on the head, abrasions, bruises, and minor lacerations (cuts on the skin surface with minimal bleeding and no exposure of deeper tissue/muscle) (47.88%).
Serious Injury is any injury other than fatal that results in one or more of the following (10.99%).
- Severe laceration resulting in exposure of underlying tissues/muscle/organs or resulting in significant loss of blood
- Broken or distorted extremity (arm or leg)
- Crush injuries
- Suspected skull, chest, or abdominal injury other than bruises or minor lacerations
- Significant burns (second and third-degree burns over 10 percent or more of the body)
- Unconsciousness when taken from the crash scene
Fatal Injury is any injury that results in death within 30 days after the motor vehicle crash in which the injury occurred (1.08%).
Due to the small percentage of uninjured accidents and fatal accidents, the present study combined uninjured, possibly injured, and slightly injured as non-serious injuries, and the remaining accident categories were combined as serious injuries. Referring to related studies on the severity of cyclist injuries and combining the field characteristics of CRSS, 25 variables were selected from five aspects, namely, time characteristics, vehicle characteristics, road characteristics, environmental characteristics, and driver/cyclist characteristics. The final values of the variables and their coding are listed in Table 1 .
- PPT PowerPoint slide
- PNG larger image
- TIFF original image
https://doi.org/10.1371/journal.pone.0301293.t001
4 Methodology
The purpose of the present study was to model bicycle accident crash data and explore the influence of key factors of each type of accident cluster on the severity of cyclist injuries while considering data heterogeneity and imbalance. The following methods were used in the present study: (1) an LCA model was used to manage data heterogeneity; (2) ROS, SMOTE, and ADASYN methods were used to contend with data imbalance; and (3) the sampled accident data were paired with a BN model, and five evaluation indicators, such as Accuracy, G-mean, and area under the curve (AUC) values, were used to select the best BN model.
4.1 Latent Class Cluster Analysis (LCA)
LCA is a probabilistic model-based clustering analysis method that achieves its basic requirement of local independence by assuming the existence of a latent variable used to explain the association between explicit variables. LCA has several advantages over traditional cluster analysis as follows: (1) in LCA, the number of clusters does not need to be predetermined, and the optimal number of clusters can be determined based on various goodness-of-fit metrics [ 25 ]; and (2) LCA can handle various types of variables, including nominal, continuous, count, and categorical variables, without a standardization process.
In this paper, the coded independent variable data were stored in CSV format and imported into LatentGold 4.5 software for LCA. However, LCA does not know the most appropriate number of clusters from the beginning. The most appropriate number can be found by testing different models with different numbers of clusters. The allocation error of assigning crashes to potential classes can be minimized by choosing the optimal number of clusters [ 25 ]. This number can be determined by a number of methods for measuring allocation accuracy. We determined the optimal number of classifications based on fitting metrics, among which the commonly used metrics were Bayesian information criteria (BIC), Akaike information criterion (AIC), consistent Akaike information criterion (CAIC), and entropy [ 26 ]. The number of clusters with the smallest scores for AIC, BIC, and CAIC is considered to be the most appropriate number of clusters. In addition, increasing the number of clusters may not always result in a minimum information criterion. Therefore, calculating the percentage reduction in BIC values between different models is preferred [ 27 ]. An entropy measure between 0 and 1 indicates the quality of the clustering solution. It is essentially a weighted average of the posterior affiliation probabilities for each case. The closer the entropy value is to 1 indicates better clustering [ 25 , 28 ].
4.2 ROS, SMOTE, and ADASYN sampling
Oversampling balances the minority samples with the majority samples by replicating or synthesizing the minority samples. Oversampling methods have the advantage of improving model performance without causing information loss, and the main oversampling methods include ROS, SMOTE, and ADASYN [ 29 ], which are used to manage the imbalance problem of self-accident data sets.
The basic principle of random oversampling is to randomly select and replicate a small number of class samples until they are comparable to the majority of class samples. This method solves the problem of information loss due to oversampling.
The basic concept of ADASYN is to assign different weights to the minority class samples according to their learning difficulty, in which the more difficult minority class samples will generate more synthetic data compared to the easier minority class samples.
Among the constraint-based methods, the Three Phase Dependency Analysis (TPDA), which was proposed by Cheng J et al. [ 31 ] in 2002, was used, which can adapt not only to the case of known node ordering, but also to the case of unknown node ordering.
In the present study, the scoring-based search method had the following two parts: the search operator and the scoring function. The search operator uses the widely used and computationally efficient hill climbing (HC) algorithm, and the scoring function uses the BDeu scoring function proposed by Buntine [ 32 ] in 1991. After determining the BN structure, the robust expectation maximization (EM) algorithm was used for parameter estimation.
5.1 Clustering results
The BIC, AIC, and CAIC values gradually decreased with the increase in cluster number ( Fig 1 ). In general, lower IC values indicate better models. However, when analyzing large sample data, each IC value often does not reach the minimum value as the number of clusters increases. In this case, analyzing the rate of decline of each IC value is preferred. In addition, it has been argued that BIC is more reliable than AIC and CAIC when the amount of data is large. If the rate of decline of IC values is less than 1% and the entropy value is higher than 0.9, the number of clusters is considered to be the optimal number of clusters. In the present study, the decline rate of each IC value was lower than 1% in the M4 model, and the entropy value of the M3 model reached 0.9463, which is greater than 0.9, which indicates the clear separation between clusters and satisfactory fitness of the model. Considering the clustering effect of each model, the number of clusters in the present study was determined to be 3 [ 33 ].
https://doi.org/10.1371/journal.pone.0301293.g001
LCA divided the overall bicycle accident data into three homogeneous traffic accident clusters, and the three accident clusters were defined as C1, C2, and C3 accident clusters. The category probabilities and conditional probabilities of the accident clusters are shown in Table 2 .
https://doi.org/10.1371/journal.pone.0301293.t002
After determining the number of clusters, it was necessary to identify the characteristic variables to determine the important characteristics of each type of accident cluster. Regarding accident cluster C1, 91.93% of the accidents occurred at intersections, 86.13% of the accidents had traffic control devices, 91.58% of the accidents were on dry roads, 38.64% of the vehicles were turning right at the time of the accident, 51.02% of the cyclists were on the Sidewalk/Crosswalk/Driveway Access, and 48.67% were the Forward. The main characteristics of the accidents were described as BMV accidents at intersections with traffic control devices. Similarly, the C2 accident cluster was described as non-intersection BMV accidents without traffic signal control devices, and the C3 accident cluster was described as BMV accidents on off-road/dedicated side streets without traffic control devices.
5.2 Results of the construction of the BMV BN model
The original accident clusters (OD accident clusters) as well as the C1, C2, and C3 accident clusters all had different degrees of category imbalance problems. To reduce the impact of accident data category imbalance, ROS, SMOTE, and ADASYN were used to resample various accident clusters. In Fig 2 , OD, C1, C2, and C3 represent the corresponding accident clusters without balancing treatment; OD_X represents the balancing data obtained after sampling OD data using X. For example, OD_SMOTE indicates the balancing data obtained after sampling OD data by SMOTE. It can be seen from Fig 2 : the problem of imbalance of accident categories is basically solved after all kinds of accident groups are sampled.
https://doi.org/10.1371/journal.pone.0301293.g002
In this study, different Bayesian classifiers are developed based on the treated and untreated datasets, and five measures are employed for the performance evaluation. To obtain the best BN model for each type of accident cluster, four data (including one unprocessed original unbalanced data, and three sampled and processed balanced data) of each accident cluster were combined with TPDA and HC. All BN models were then generated. Finally, 5-fold cross-validation was utilized to obtain the accuracy, sensitivity, specificity, G-mean, and AUC values of each model, and the optimal BN model for each type of accident cluster was selected based on these results. The optimal BN models for the C1, C2, C3, and OD accident clusters were the BMV_C1, BMV_C2, BMV_C3, and BMV_OD models, respectively. The modeling process is shown in Fig 3 .
https://doi.org/10.1371/journal.pone.0301293.g003
Because both the G-mean and AUC values represent the comprehensive performance of the model and can be reasonably evaluated even if the data are in an imbalance state, the one with the highest G-mean and AUC value in the C1, C2, C3, and OD accident clusters was used as the optimal BN model. Table 3 shows that the C1_ROS_HC model had the highest G-mean and AUC value in the C1 accident group, which indicated that the C1_ROS_HC model was the optimal BMV_C1 model. Similarly, the C2_SMOTE_TPDA, C3_ROS_HC, and OD_SMOTE_TPDA models were the optimal BMV_C2, BMV_C3, and BMV_OD models, respectively. Tables 3 – 6 show the various evaluation metrics for different BN models in different accident clusters. Figs 4 – 7 show the BN plots for the BMV_C1, BMV_C2, BMV_C3, and BMV_OD models.
https://doi.org/10.1371/journal.pone.0301293.g004
https://doi.org/10.1371/journal.pone.0301293.g005
https://doi.org/10.1371/journal.pone.0301293.g006
https://doi.org/10.1371/journal.pone.0301293.g007
https://doi.org/10.1371/journal.pone.0301293.t003
https://doi.org/10.1371/journal.pone.0301293.t004
https://doi.org/10.1371/journal.pone.0301293.t005
https://doi.org/10.1371/journal.pone.0301293.t006
5.3 Key elements of the BMV BN model
Interdependencies between different variables can be easily established based on the BN theory. Similar to other researchers studying traffic accidents, we are more interested in the key factors that influence the serious injuries (both Serious Injury and Fatal Injury) to cyclists. Thus, the present study focused only on the factors that have a direct dependence on serious injuries and are considered as the key factors that influence serious injuries to cyclists [ 7 ]. Figs 8 – 11 show the key factors affecting the serious injuries of cyclists in the BMV_C1, BMV_C2, BMV_C3, and BMV_OD models, respectively, as well as the key factors affecting the serious injuries to cyclists in the BN model constructed based on the same BN algorithm but without the balanced treatment algorithm.
https://doi.org/10.1371/journal.pone.0301293.g008
https://doi.org/10.1371/journal.pone.0301293.g009
https://doi.org/10.1371/journal.pone.0301293.g010
https://doi.org/10.1371/journal.pone.0301293.g011
To investigate which values of the key factors contribute to the probability of a cyclist sustaining a serious injury (hereinafter referred to as the "serious injury rate"), based on the optimal BN model of different accident groups, each value of each key factor is used as "evidence" (i.e., the probability of occurrence of one value of the variable is set to 100% and the probability of occurrence of the rest of the values is set to 0%) in GeNIe 4.0, and the serious injury rate of each node under the evidence is observed. Based on the optimal BN model for different accident clusters, each value of each key factor is used as "evidence" in GeNIe 4.0 software (i.e., the probability of occurrence of a certain value of the variable is set to 100%, and the probability of occurrence of the rest of the values is set to 0%), and the serious injury rate of the seriously injured node is observed under each evidence.
Figs 12 – 15 show the values of all key factors in the BMV_C1, BMV_C2, BMV_C3, and BMV_OD models for which the serious injury rate was higher than the non-serious injury rate.
https://doi.org/10.1371/journal.pone.0301293.g012
https://doi.org/10.1371/journal.pone.0301293.g013
https://doi.org/10.1371/journal.pone.0301293.g014
https://doi.org/10.1371/journal.pone.0301293.g015
6 Discussion
6.1 analysis of key factors in the bmv model, 6.1.1 time of crash characteristics..
In the C1 accident cluster, accidents occurring on weekends and in the morning peak increased the rate of serious injuries to cyclists by 5% and 8.8%, respectively. This is consistent with Kim JK’s [ 34 ] study that both drivers and cyclists during rush hour tend to drive and cycle more aggressively, resulting in severe injuries to cyclists.
In the C3 accident cluster, accidents occurring in the fall and winter seasons increased the rate of serious injury to cyclists by 6.6% and 6.1%, respectively, which was similar to previous studies [ 35 ]. Similar to the C1 accident cluster, accidents occurring in the morning peak increased the rate of serious injury to cyclists by 7.4%, but unlike the C1 accident cluster, accidents occurring in the off-peak hours also increased the rate of serious injury to cyclists by 3.4% in the C3 accident cluster. Combined with the characteristics of the C3 accident cluster. The potential reason is that most of the accidents in the C3 accident cluster occur on dedicated feeder roads, i.e., roads and intersections where commercial centers, residences, parking lots, etc., enter arterials, which have little to do with peak hours.
6.1.2 Driver and cyclist characteristics.
In the C1 accident cluster, female cyclists were more likely to be seriously injured compared to male cyclists, which may be explained by 99% of the C1 accident clusters occurring at and near intersections. Some studies have shown that female cyclists are more likely to be involved in dangerous conflicts at intersections than male cyclists [ 36 ]. In particular, cyclists’ failure to yield and traffic control device violations increased the rate of serious injuries to cyclists by 10.4% and 9.4%, respectively. Moreover, older cyclists were more likely to be seriously injured, with a 9.6% increase in the probability of serious injury for cyclists over 65 years of age. The potential reason may be due to decreases in physical fitness and hazard perception that occur with age, and injuries tend to be more severe in collisions.
In the C2 accident cluster, failure to yield to cyclists increased the rate of serious injuries by 9.2%. Moreover, drivers aged 18 to 25, drivers aged 26 to 40, and male drivers increased the rate of serious injuries to cyclists by 9.9%, 9%, and 3.4%, respectively. These results may be attributed to the majority of accidents in this accident cluster occurring on non-intersection general roadways, and drivers aged 18 to 40 years and male drivers drive more aggressively and faster on general roadways, leading to more serious accidents [ 37 ]. Unlike the C1 accident cluster, the C2 accident cluster had a 2.7% increase in the probability of serious injuries for male cyclists. The reason for this may be that most of the C2 accident clusters occurred on common non-intersection roadways with better riding conditions, and male cyclists are more likely to engage in risky riding behaviors than female cyclists when traveling on this roadway [ 35 ]. Of note, there is no reliable conclusion on the effect of cyclist gender on injury severity. Li et al. [ 38 ] concluded that male cyclists have more driving experience and are calmer than female in t accidents, which indicates that female cyclists are more likely to cause serious injuries in accidents. Therefore, further research is needed to clarify these inconsistent findings. Finally, an obstructed field of view of the driver increased the serious injury rate of cyclists by 20.2%, which agreed with the findings of previous studies on BMV accidents.
In the C3 accident clusters, male drivers increased the probability of serious injury to cyclists by 4.4%, and driver failure and distracted cyclists increased the rate of serious injury to cyclists by 20% and 21.1%, respectively. The majority of accidents in the C3 accident cluster involved cyclists about to exit a dedicated slip road, and 81.19% of C3 accident clusters did not have traffic control devices. Because slip roads are narrower than normal intersections, drivers traveling on the main road before the accident are less likely to notice cyclists.
6.1.3 Vehicle characteristics.
In the C1 and C2 accident clusters, the vehicle travel status straight ahead increased the serious injury rate of cyclists by 9.2% and 5.7%, respectively, which may have been attributed to the higher impact energy from the collision due to the faster speed of the straight-ahead vehicle compared to the steering and start/stop states.
In the C2 accident clusters, passenger cars increased the serious injury rate of cyclists by 5% because most of the crashes in this cluster occurred at non-intersections where there are no blind spots in the field of view. The speed of passenger cars at non-intersections is fast, causing both the cyclist and the driver to have time to avoid the crash before the crash. The rate of serious injury to cyclists increased by 10.7% when the collision location was at the front of the car. One possible explanation is that bicycles are more like to crash in frontal collisions, resulting in more serious accidents, especially on non-intersection roadways where traffic speeds are higher [ 35 ]. This type of accident may be addressed by improving the active and passive safety technology of vehicles, such as automatic emergency braking (AEB) systems and hood pop-up technology, and cyclists are also required to wear helmets and protective devices [ 39 ].
In the C3 accident cluster, the rate of serious injuries to cyclists increased by 13.8% when the vehicle type was a truck/bus, which was due to the large mass of the truck/bus and the kinetic energy of the vehicle before the crash. Driving age of 6 to 10 years caused an increase in the probability of serious injury to cyclists. These results may be explained by drivers with 6 to 10 years of driving experience having a higher threshold of risk acceptance and are instead prone to overconfidence and overdriving behavior.
6.1.4 Road characteristics.
The results of the C2 accident cluster indicated that the probability of a cyclist being seriously injured while riding on dry pavement increased by 1.9%, and the probability of a cyclist being seriously injured while riding in the carriageway increased by 1.8%. In contrast, the probability of being seriously injured while riding in the bike lane/shoulder decreased. Thus, these findings suggest that roadways should be designed to achieve as much separation as possible between the machine and non-machine as well as to arrange machine and non-machine separation zones.
6.1.5 Environment attributes.
In the C1 accident cluster, the rate of serious injuries to cyclists increased by 5.7% when accidents occurred in bad weather, such as rain/snow, which is attributed to rain/snow reducing visibility, ultimately affecting the risk perception and reaction ability of drivers and cyclists. In particular, some studies have also shown that bad weather, such as rain/snow, reduces the probability of cyclist injury or death [ 36 ], which is attributed to a risk compensation effect mechanism [ 38 ]. The absence of lighting at night increased the probability of serious injury by 8.3%, and the probability of serious injury increased by 4.3% even with lighting at night, which is due to the lack of light at night and the fact that most bicycles do not have lights similar to electric two-wheelers and are not easily detected by drivers. In contrast to most studies, the present study found that when the traffic control device was a traffic signal, it increased the rate of serious injuries to cyclists by 4.1%. Moreover, 91.13% of the accidents in the C1 accident cluster occurred at intersections. It has been noted that the probability of serious injury increases when traffic signal lights are present at intersections, which may be due to high traffic flow, leading to an increased risk for cyclists [ 40 ].
In the C2 accident cluster, clear weather increased the probability of serious injury to cyclists by 3% due to similar reasons as those for C2 accidents occurring at non-intersections where drivers are more likely to speed in clear weather. Similar to the findings for the C1 accident cluster, both no lighting at night and lighting at night increased the probability of serious injury to cyclists in the C2 accident cluster.
In the C3 accident cluster, inclement weather, such as rain/snow, increased the probability of serious injury to cyclists. Combining the three accident clusters showed that bad weather, such as rain/snow, increased the probability of serious injury accidents at intersections and dedicated branch intersections, while sunny days increased the probability of serious injury accidents at non-intersection general roads. These findings confirmed the heterogeneity of weather condition factors, further explaining why weather effects are controversial in previous studies on BMV accidents.
There were additional similarities between the OD accident cluster and the three sub-accident clusters, but these similarities were not further analyzed. Unlike the three sub-accident clusters, roadway type was only found to be a key factor in the OD accident cluster, and the results showed that accidents occurring near intersections or at non-intersections increased the probability of serious injury to cyclists by 3.7% and 5.8%, respectively. These findings were consistent with the majority of previous studies showing that non-intersection roadways are more dangerous than intersection roadways. These results suggested that the division of the entire data into three accident clusters by LCA explains the heterogeneity of roadway types in the OD accident clusters.
6.2 Heterogeneity analysis
We next evaluated the differences in critical factors for different accident clusters ( Table 7 ).
https://doi.org/10.1371/journal.pone.0301293.t007
A comparative analysis of the key factors for the OD incident cluster and the three sub-accident clusters in Table 7 revealed four meaningful results.
First, some factors were identified as critical only in sub-accident clusters but not in the OD accident cluster. For example, accident week and cyclists’ violation of traffic control device were identified as critical factors only in the C1 accident cluster, in which the accident occurring on a weekend and the cyclist failing to yield increased the probability of serious injury to the cyclist by 5% and 9.4%, respectively. In addition, roadway cross-section type was a key factor only in the C2 accident cluster, in which cyclists riding in the carriageway (motorway) increased the probability of serious injury to cyclists and riding in the bike lane/shoulder (non-motorway) reduced the probability of serious injury to cyclists. Similarly, season, driver failure to yield, distracted cyclist riding, and age of driving were key factors only in the C3 accident cluster. These findings reaffirmed the necessity and importance of using LCA to classify accident data into homogeneous sub-data, which not only explores the key factors hidden in OD accident clusters to provide more comprehensive information but also provides insight into the differences of key factors in different accident clusters to reveal the heterogeneity of the data. Moreover, most of the BN models constructed based on homogeneous sub-data outperformed the BN models constructed based on OD accident clusters in terms of G-mean and AUC values (Section 5.3).
Second, some factors were identified as key factors in both OD accident clusters and sub-accident clusters. For example, the driver’s gender and vehicle type were key factors in the OD, C2, and C3 accident clusters, while the cyclists’ gender, cyclists’ failure to yield, and pre-event movement were key factors for the OD, C1, and C2 accident clusters. In addition, some factors were identified as key factors only in the OD accident cluster and a specific class of sub-accident clusters. For example, the driver’s age, the view was blocked, and the crash location were only critical factors for OD accident clusters and C2 accident clusters. For these factors, the use of LCA helps to clarify their influence patterns on bicycles and accidents, providing the basis for sub-models to explain in more detail the factors influencing serious injuries to cyclists.
Third, some of the factors were identified as key factors in all three sub-accident clusters, but their effects on cyclist injury severity were not consistent and even sometimes opposite. In the C1 accident cluster, "female" cyclists were more likely to be seriously injured than male cyclists, whereas male cyclists were more likely to be seriously injured than female cyclists in the C2 accident cluster. In the C1 and C3 accident clusters, rain/snow and other bad weather cyclists were more likely to be seriously injured, whereas sunny weather cyclists were more likely to be seriously injured than female cyclists in the C2 accident cluster. In the C1 accident cluster, cyclists were less likely to be seriously injured during "off-peak" hours, while cyclists were more likely to be seriously injured during off-peak hours in the C3 accident cluster.
Fourth, although the use of LCA to divide the overall accident data into homogeneous sub-data revealed some new factors, several key factors of the OD accident clusters may have been overlooked, i.e., some of the factors were key factors in the OD accident clusters but not in the sub-accident clusters. For example, roadway type was a key factor only in the OD accident cluster, in which cyclists were vulnerable to serious injury at non-intersections and near intersections but not at intersections vulnerable to non-serious injuries.
6.3 Analysis of data before and after sampling
Compared to the BN model constructed based on unbalanced data, the BN model constructed based on balanced data did not show significant improvement in overall correctness and sensitivity but had substantial improvement in Specificity, G-mean, and AUC values (Tables 3 – 6 ). This phenomenon may be explained because machine learning methods inherently assume that the data are balanced and subject to the dominant effect of the majority class, and models constructed based on the original unbalanced data will be biased toward the majority class in terms of prediction results, resulting in poor classification accuracy or even invalid models for the minority class. For example, the overall correctness and sensitivity of the C1_HC model and C1_TPDA model were higher than 0.9, but the specificity index was 0, i.e., the classification accuracy of the minority class was 0. This type of classifier is called a foolish classifier. Thus, these findings illustrated that the overall correctness index cannot represent the validity of the model, indicating that the sensitivity and specificity indexes need to be considered.
With the same BN algorithm, the BN model constructed based on the balanced dataset identified more key factors affecting serious injuries (Figs 8 – 11 ). For example, the week of the accident, time period, cyclists’ gender, cyclists’ age, cyclists’ failure to yield, cyclists’ violation of traffic control devices, vehicle driving status, weather conditions, lighting conditions, and traffic control devices (a total of 10 key factors) were identified as critical factors in the C1_ROS_HC model constructed based on the balanced dataset. Some researchers have found that these factors influence serious injuries to cyclists [ 20 ]. However, only vehicle driving status was found to be a critical factor in the C1_HC model constructed based on the unbalanced data set. These findings suggested that the categories of accident data in an unbalanced state may mask the normal relationship between influencing factors and injury severity, leading to misleading research findings. Therefore, these results indicated that appropriate data balancing techniques must be used to reduce the effects of unbalanced category data.
6.4 Suggestions on prevention and control of bicycle accidents
Based on the above analysis of different accident groups, this section puts forward targeted prevention and control recommendations for bicycle accidents and different accident groups from four aspects: people, vehicles, roads, and environment:
- Prevention and control recommendations for people ①Improve the safety awareness of drivers and cyclists and reduce the occurrence of unsafe behaviors. During off-peak hours at intersections or in bad weather conditions such as rain/snow, it is especially important to prevent the behaviors of "cyclists failing to yield the right-of-way" and "cyclists violating traffic control devices"; and prevent the behaviors of "male" cyclists failing to yield the right-of-way at non-intersections. male" cyclists failing to yield and "male drivers aged 26–40" speeding on non-intersections; and "male drivers failing to yield" and "distracted cyclists" on dedicated slip roads. ②Optimize the driving test system and make the test more difficult. Driving simulators can be used to increase the form of "emergency preparedness test" and "night driving test". ③With the emergence of the aging problem, it is necessary to publicize and encourage the elderly over 65 years old to use free public transportation.
- Suggestions for prevention and control of vehicles ① Enhance the safety inspection of vehicles, especially the vehicle braking system, lighting system and other components, to ensure that the vehicles meet the requirements in terms of safety. ② Enhance the active and passive safety technologies of automobiles to improve the safety performance of automobiles. ③ Allow trucks/buses to make appropriate vehicle modifications, such as adding additional mirrors or reflectors to reduce the impact of blind spots in the field of vision.
- Prevention and control suggestions for roads ① Improve the planning and design of roads. From the analysis of the C2 accident group, it can be seen that cyclists driving in the motor vehicle lane will increase the probability of cyclists being seriously injured, therefore, in the road design and planning, non-intersections should try to avoid mixing of motor vehicle and non-motorized traffic and try to set up "colored non-motorized lanes" as shown in Fig 16 . to maximize the attention of motorists and reduce traffic conflicts. ②Enhance the roadway maintenance and safety hazard investigation. Focus on roadway drainage at intersections and dedicated side streets, and promptly clean roadways with standing water or snow. ③ Appropriately increase the roadway’s fault tolerance. Cyclists and drivers will inevitably make mistakes, in the road design can be appropriate to set aside some space for correcting errors, such as appropriate widening of the road surface, from the objective level to increase the reaction time of drivers and cyclists. ④ In the morning rush hour and weekend hours, the focus should be on the driving safety of intersection sections, while in the fall and winter seasons and off-peak hours, the focus should be on the driving safety of dedicated feeder roads.
- Suggestions for prevention and control of the environment ①Improve lighting conditions on roads and strengthen the management of lighting facilities. From the analysis of influencing factors, it can be seen that serious injuries are likely to occur in the environment without lighting at night. To address this problem, we can increase the number of street lamps and improve the coverage and brightness of street lamps in accident-prone areas. ② Optimize transportation infrastructure. As nighttime travel or inclement weather such as rain/snow can affect drivers’ ability to see the crosswalk markings, "smart crosswalks" as shown in Fig 17 . It can be popularized near intersections and dedicated branch intersections.③ Enhance the warning and reminder of bad weather.
https://doi.org/10.1371/journal.pone.0301293.g016
https://doi.org/10.1371/journal.pone.0301293.g017
7 Conclusions
In the current paper, we propose a two-stage method integrating LCA, resampling, and BN to investigate the effects of different influencing factors on the injury severity of bicyclists in bicycle accidents when the data are heterogeneous and unbalanced, and the key factors are mined from the characteristics of the time of the accident, the characteristics of the driver and the bicyclist, the characteristics of the vehicle, and the characteristics of the roadway to analyze the heterogeneity, and the results show that:
- In the C1 accident group "BMV accidents at intersections with traffic control devices", accidents occurring on weekends and in the morning rush hour will increase the serious injury rate of cyclists by 5% and 8.8%, respectively; female cyclists are more prone to serious injuries than male cyclists; the vehicle traveling in a straight line will increase the serious injury rate of cyclists by 9.2%; and the vehicle driving status will increase the serious injury rate of cyclists by 9.2%, respectively. serious injury rate will increase by 9.2%; when the accident occurs in bad weather such as rain/snow, the serious injury rate of cyclists will increase by 5.7%.
- In accident group C2, "Non-intersection BMV accidents without traffic signal control," failure to yield to a bicyclist increases the rate of serious injury by 9.2%; male bicyclists increase the probability of serious injury by 2.7%; when the vehicle is traveling in a straight line, it increases the rate of serious injury by 5.7%; passenger vehicles increase the rate of serious injury by 5.7%; and when the vehicle is traveling in a straight line, it increases the rate of serious injury by 5.7%.; passenger vehicles increase the rate of serious injury to cyclists by 5%; cyclists are 1.9% more likely to be seriously injured when riding on dry pavement and 1.8% more likely to be seriously injured when riding in a driveway; and sunny days increase the probability of serious injury to cyclists by 3%.
- In accident group C3, "off-road/private sidewalk BMV accidents without traffic control devices," accidents occurring in the fall and winter seasons increase the rate of serious injury to bicyclists by 6.6% and 6.1%, respectively; a male driver increases the probability of serious injury to a bicyclist by 4.4%, and a driver who fails to comply with the rules, or who is distracted from riding, increases the probability of serious injury to a bicyclist by 1.8%; sunny weather increases the probability of serious injury to a bicyclist by 3%. distracted riding increases the rate of serious injuries to cyclists by 20% and 21.1%, respectively; when the vehicle type is truck/bus, the rate of serious injuries to cyclists increases by 13.8%; and inclement weather such as rain/snow increases the probability of serious injuries to cyclists.
In addition, the results of the key factor heterogeneity analysis showed that female cyclists, inclement weather such as rain/snow, and off-peak were key factors in several subcategories of accident groups, but had opposite effects on the severity of cyclist injuries in different subcategories.
Supporting information
S1 dataset..
https://doi.org/10.1371/journal.pone.0301293.s001
S1 Table. The complete distribution of featured variables describing cluster characteristics.
https://doi.org/10.1371/journal.pone.0301293.s002
S2 Table. Evaluation index of different BN models in the C1-C3 accident cluster.
https://doi.org/10.1371/journal.pone.0301293.s003
- View Article
- PubMed/NCBI
- Google Scholar
- 4. World Health Organization, 2020. Cyclist Safety: An Information Resource for Decision- Makers and Practitioners.
- 5. National Highway Traffic Safety Administration, 2020. Bicyclists and other cyclists: 2018 data. (Traffic Safety Facts. Report No. DOT HS 812 884). Washington, DC.
- 23. Crash Report Sampling System | NHTSA
- 31. Cheng J, Bell D A, Liu W. Learning belief networks from data: An information theory based approach[C]//Proceedings of the sixth international conference on Information and knowledge management. 1997: 325–331.
- 32. Buntine Wray. Theory Refinement on Bayesian Networks. Elsevier eBooks. 1991 Jul 13;52–60.
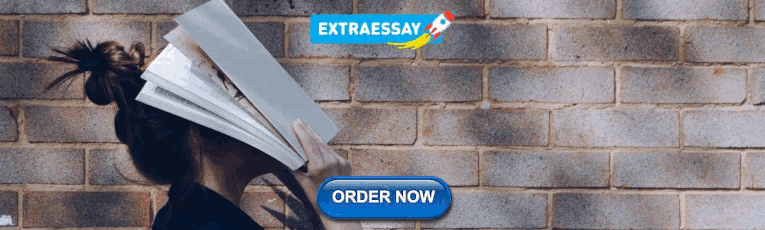
IMAGES
VIDEO
COMMENTS
The Effect of Patient's Age and duration and Frequency of Tissue Abusive Habit on Severity of Oral Sub Mucous Fibrosis. Gadkari, Tejal Rajkumar; Bhadage, Chetan Jalandar; Bhoosreddy, Ajay Ramesh; More. Journal of Dental Research and Review. 10 (3):119-122, Jul-Sep 2023. Abstract.
Journal of Dental Research (JDR) is a peer-reviewed scientific journal dedicated to the dissemination of new knowledge and information, encompassing all areas of clinical research in the dental, oral and craniofacial sciences. Average time … | View full journal description. This journal is a member of the Committee on Publication Ethics (COPE).
Table of contents for Journal of Dental Research, 103, 5, May 01, 2024 ... Impact Factor: 7.6 / 5-Year Impact Factor: 7.6 . JOURNAL HOMEPAGE. SUBMIT PAPER. Previous issue. Next issue. ... Restricted access Review article First published April 15, 2024 pp. 467-476. xml GET ACCESS.
Alexandria, VA, USA, June 29, 2022 - The International Association for Dental Research and American Association for Dental, Oral, and Craniofacial Research announced that the Journal of Dental Research (JDR) 2-Year Journal Impact Factor™ rose to 8.924, the highest in the journal's history.JDR now ranks #3 of 92 journals in the "Dentistry, Oral Surgery & Medicine" category, and the ...
The Journal of Dental Research (JDR) is a multidisciplinary journal dedicated to the dissemination of new knowledge in all sciences relevant to dentistry and the oral cavity and associated structures in health and disease. The JDR 2-year Journal Impact Factor™ is 8.924, ranking #3 of 92 journals in the "Dentistry, Oral Surgery & Medicine ...
About the Journal of Dental Research The IADR/AADR Journal of Dental Research (JDR) is a multidisciplinary journal dedicated to the dissemination of new knowledge in all sciences relevant to dentistry and the oral cavity and associated structures in health and disease.The JDR 2-year Journal Impact Factor™ is 6.116, ranking #5 of 91 journals in the "Dentistry, Oral Surgery & Medicine ...
The journal for dental, oral and craniofacial research. View the Journals Subscription Package for the Journal of Dental Research (JDR) and JDR Clinical & Translational Research, including Advances in Dental Research (ADR) and Critical Reviews in Oral Biology & Medicine, Vol 1-15. Journal of Dental Research (JDR) is a peer-reviewed scientific journal dedicated to the dissemination of new ...
Table of contents for Journal of Dental Research, 100, 10, Sep 01, 2021 ... Impact Factor: 7.6 / 5-Year Impact Factor: 7.6 . JOURNAL HOMEPAGE. SUBMIT PAPER. Previous issue. Next issue. Volume 100 Issue 10, September 2021. ... Reviews Critical Reviews in Oral Biology & Medicine.
Alexandria, VA - The International Association for Dental, Oral, and Craniofacial Research and American Association for Dental, Oral, and Craniofacial Research announced the Journal of Dental Research (JDR) 2-Year Journal Impact Factor™ is now 7.6, ranking it #3 of 91 journals in the "Dentistry, Oral Surgery & Medicine" category.. The JDR 5-year Journal Impact Factor™ is also 7.6 ...
Scope. Journal of Dental Research (JDR) is a peer-reviewed scientific journal dedicated to the dissemination of new knowledge and information on all sciences relevant to dentistry and to the oral cavity and associated structures in health and disease. JDR is published monthly, allowing for frequent dissemination of its leading content.
Get access to Journal of Dental Research and Review details, impact factor, Journal Ranking, H-Index, ISSN, Citescore, Scimago Journal Rank (SJR). Check top authors, submission guidelines, Acceptance Rate, Review Speed, Scope, Publication Fees, Submission Guidelines at one place. Improve your chances of getting published in Journal of Dental Research and Review with Researcher.Life.
International Scientific Journal & Country Ranking. SCImago Institutions Rankings SCImago Media Rankings SCImago Iber SCImago Research Centers Ranking SCImago Graphica Ediciones Profesionales de la Información
Impact Factor: 7.6 / 5-Year Impact Factor: 7.6 . JOURNAL HOMEPAGE. SUBMIT PAPER. Previous issue. ... Reviews Critical Reviews in Oral Biology & Medicine. Oral Cancer Screening: Past, Present, and Future ... Journal of Dental Research ISSN: 0022-0345; Online ISSN: 1544-0591; About Sage;
Aims & Scope. Dentistry Review is an international, open access, peer-reviewed dental journal. We publish high quality, review-based research that is useful to the practicing clinicians. The freely available papers published in Dentistry Review will improve the oral health of populations worldwide. The Journal scope is focused on topics of use ...
The IADR/AADR announce that the Journal of Dental Research (JDR) 2-year Journal Impact Factor™ is over 6 for the first time at 6.116, ranking #5 of 91 journals in the "Dentistry, Oral Surgery ...
About the journal. Official journal of the Japanese Association for Dental Science. Production and Hosting by Elsevier Ltd. on behalf of the Japanese Association for Dental Science. The Japanese Dental Science Review is published by the Japanese Association for Dental Science aiming to introduce the modern aspects of …. View full aims & scope.
Journal of Dental Research Impact Factor History. 2-year 3-year 4-year. 2022 Impact Factor . 7.719 7.337 7.602. 2021 Impact Factor . 7.347 7.545 7.682. 2020 Impact Factor . 4.894 5.495 5.859. ... SNIP, etc. Researchers may also consider the practical aspect of a journal such as publication fees, acceptance rate, review speed.
The digital economy may accelerate the upgrading of industrial structures and boost regional innovation output, effectively contributing to China's green economic transformation. The impact of the digital economy on developing the urban green economy is analyzed using data from 280 cities across China from 2010-2019. Using a fixed-effects model and the Spatial Durbin model, the digital ...
The increase in cancer survival rates has put a focus on ensuring fertility preservation procedures for cancer patients. Ovarian tissue cryopreservation presents the only option for prepubertal girls and patients who require immediate start of treatment and, therefore, cannot undergo controlled ovarian stimulation. We aimed to provide an assessment of stem cells' impact on cryopreserved ...
Table of contents for Journal of Dental Research, 102, 6, Jun 01, 2023 ... Impact Factor: 7.6 / 5-Year Impact Factor: 7.6 . JOURNAL HOMEPAGE. SUBMIT PAPER. Previous issue. Next issue. Volume 102 Issue 6, June 2023. ... Open Access Review article First published March 15, 2023 pp. 589-598.
Bicycle safety has emerged as a pressing concern within the vulnerable transportation community. Numerous studies have been conducted to identify the significant factors that contribute to the severity of cyclist injuries, yet the findings have been subject to uncertainty due to unobserved heterogeneity and class imbalance. This research aims to address these issues by developing a model to ...