Thank you for visiting nature.com. You are using a browser version with limited support for CSS. To obtain the best experience, we recommend you use a more up to date browser (or turn off compatibility mode in Internet Explorer). In the meantime, to ensure continued support, we are displaying the site without styles and JavaScript.
- View all journals
- Explore content
- About the journal
- Publish with us
- Sign up for alerts
- Review Article
- Published: 02 May 2023
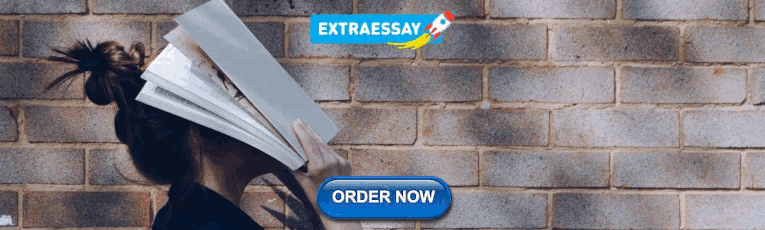
Climate change impacts on plant pathogens, food security and paths forward
- Brajesh K. Singh ORCID: orcid.org/0000-0003-4413-4185 1 , 2 ,
- Manuel Delgado-Baquerizo 3 , 4 ,
- Eleonora Egidi ORCID: orcid.org/0000-0002-1211-2355 1 ,
- Emilio Guirado ORCID: orcid.org/0000-0001-5348-7391 5 ,
- Jan E. Leach ORCID: orcid.org/0000-0001-7252-4397 6 ,
- Hongwei Liu ORCID: orcid.org/0000-0002-8200-8801 1 &
- Pankaj Trivedi ORCID: orcid.org/0000-0003-0173-2804 6
Nature Reviews Microbiology volume 21 , pages 640–656 ( 2023 ) Cite this article
54k Accesses
131 Citations
250 Altmetric
Metrics details
- Climate change
- Plant sciences
Plant disease outbreaks pose significant risks to global food security and environmental sustainability worldwide, and result in the loss of primary productivity and biodiversity that negatively impact the environmental and socio-economic conditions of affected regions. Climate change further increases outbreak risks by altering pathogen evolution and host–pathogen interactions and facilitating the emergence of new pathogenic strains. Pathogen range can shift, increasing the spread of plant diseases in new areas. In this Review, we examine how plant disease pressures are likely to change under future climate scenarios and how these changes will relate to plant productivity in natural and agricultural ecosystems. We explore current and future impacts of climate change on pathogen biogeography, disease incidence and severity, and their effects on natural ecosystems, agriculture and food production. We propose that amendment of the current conceptual framework and incorporation of eco-evolutionary theories into research could improve our mechanistic understanding and prediction of pathogen spread in future climates, to mitigate the future risk of disease outbreaks. We highlight the need for a science–policy interface that works closely with relevant intergovernmental organizations to provide effective monitoring and management of plant disease under future climate scenarios, to ensure long-term food and nutrient security and sustainability of natural ecosystems.
Similar content being viewed by others
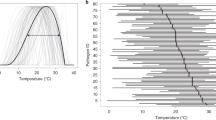
Plant pathogen infection risk tracks global crop yields under climate change
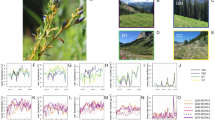
Predicting the effects of climate change on the cross-scale epidemiological dynamics of a fungal plant pathogen
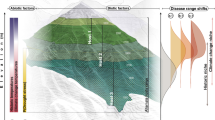
Nonlinear shifts in infectious rust disease due to climate change
Introduction.
Increasing incidence and severity of plant disease outbreaks poses significant and growing risks to primary productivity, global food security and biodiversity loss for many vulnerable areas of the world 1 , 2 , 3 , 4 , 5 , 6 , 7 . These disease outbreaks cause yield and ecological losses. For example, the annual crop yield loss caused by pathogens (microorganisms that cause diseases and constrain host health and productivity) and pests alone is estimated at US$220 billion 3 , 4 , 5 , 6 , directly impacting food security, regional economies and other linked socio-economic aspects. This is further exacerbated by post-harvest loss caused by pathogenic microorganisms such as Penicillium spp. and Xanthomonas euvesicatoria 1 . Furthermore, climate change poses an increased risk of intensification of plant diseases, putting at risk the world’s food supply and natural plant biodiversity 7 , 8 , 9 . It is postulated that any potential yield gains in the next five decades will be offset by climate change-mediated altered disease pressure caused by known and emerging pathogens 10 . Similarly, the spread of pathogens linked to climate change is considered one of the main threats to forest health globally 11 . Therefore, improved knowledge of climate change impacts on the molecular, epidemiological and ecological interactions between pathogens, plants and the associated microbial communities is needed to develop climate-resilient agricultural and natural ecosystems 4 , 6 .
Plants are infected by a diverse range of pathogens, including bacteria, fungi, oomycetes, viruses and nematodes, that differ in their lifestyles (biotrophs, deriving nutrients from living cells, to necrotrophs, deriving nutrients from dead cells), infection strategies (intracellular or extracellular) and target plant tissues (for example, xylem, phloem, roots or leaves) (Supplementary Table 1 ). A key challenge to predicting plant diseases in space and time is to understand how these different pathogens interact with, and respond to, multiple drivers of disease (for example, other pathogens, host/vectors, commensal microorganisms and environment), and how they jointly respond to climate change. Theoretically, climate change may facilitate plant infection in multiple ways including by altering pathogen evolution, changing host–pathogen interactions and vector physiology, and facilitating the emergence of new strains of pathogens, which in turn can break down host-plant resistance 7 , 12 , 13 . Climate change can also result in the range shifts of pathogens and hosts, which would increase the spread of plant disease into new areas 8 , 10 , 14 , 15 . Yet we have limited knowledge of how different components of climate change (for example, temperature and rainfall fluctuation) and their interaction with anthropogenic activities impact plant pathogens in both agricultural and natural ecosystems. For example, the abundance of fungal soil-borne plant pathogens is likely to increase in most natural ecosystems under projected climate change scenarios, with significant but unquantified consequences for primary productivity globally 14 . Similarly, variation in relative humidity affects the abundance and infectivity of pathogens 16 .
Climate change will expectedly increase plant diseases in crops. Firstly, globalization and international trade have intensified movement of crop pathogens between continents in the past few decades 17 , 18 , increasing the risk of transmission from disease-prevalent to disease-free regions. Plant species or cultivars that have not coevolved with the introduced pathogen in the new geographical location are likely to foster pathogen prevalence and disease outbreaks. An example of trade and transport as drivers of pathogen emergence is wilt disease of banana, also known as Panama disease, caused by the soil-borne fungus Fusarium oxysporum f. sp . cubense , which likely originated in Southeast Asia and then spread globally during the twentieth century 19 . Secondly, climate and ecological changes and modern land management practices dominated by monocultures and high-density crops likely facilitated the emergence and adaptation of plant pathogens able to disseminate beyond their normal geographical ranges. For example, soybean and wheat are extensively grown in high-density monocultures, and their yields are compromised by a plethora of pests and pathogens. Soybean rust caused by the fungus Phakopsora pachyrhizi and wheat blotch caused by the fungus Zymoseptoria tritici are among the most destructive diseases on these crops, and yield losses of more than 50% have been documented during severe epidemics 2 , 20 . Despite the complexity of natural ecosystems (for example, biodiversity interactions), climate change and the linked emergence and evolution of pathogens pose similar challenges for wild plant communities and productivity 21 . For example, global warming-associated range expansion of Phytophthora cinnamomi could have significant negative impact on indigenous plant communities in many parts of the world 22 , 23 . A further increase in disease burden as a result of climate change could have devastating consequences for many plant species, food production and security, ecosystem sustainability and social conflicts.
This Review discusses how plant pathogen loads and disease pressure are likely to change under future climate scenarios. We explore current and future impacts of climate change and land use intensification on pathogen biogeography, on interactions between the plant microbiome and plant pathogens, and on plant disease incidence and severity, and their collective influence on agriculture and primary production. We analyse possible mechanisms by which pathogen invasion affects the plant microbiome, and how this knowledge might be harnessed to mitigate the risk of disease outbreaks, via improved disease surveillance, predictive modelling and effective sustainable management strategies 8 , 12 . Finally, we propose different approaches that combine pathogen monitoring and policy frameworks to ensure the long-term sustainability of global food security and environmental sustainability.
Climate change and plant diseases
Predicting the impacts of climate change on plant disease is complex and challenging, as multiple aspects of plants, pathogens and the environment are involved. These factors include the distribution and abundance of taxa (geographical range, niche preference), their fitness and virulence, abiotic interactions, plant–microorganism evolutionary processes, host and vector biology, and environmental conditions. For instance, many soil opportunistic pathogens can cause disease outbreaks when environmental conditions become favourable for pathogen replication and vulnerable hosts are available 24 , 25 . Supplementary Table 1 summarizes the responses of several plant pathogens, the damage they cause to the plant and their geographical distributions 21 . Climate change can also indirectly affect plant–pathogen interactions through alterations in the biochemical, physiological, ecological and evolutionary processes of the plant host and/or pathogen 7 , 24 , 26 , 27 (Fig. 1 ). For example, prolonged drought causes water stress in forest trees, which results in increased susceptibility to infection by pathogens causing dieback disease from the genus Phytophthora, thus facilitating the occurrence of potentially new diseases 28 , 29 , 30 . Overall, the direct impacts of climate change are likely to vary depending on the pathogen, host identity and properties of biomes. Discussed in the following sections, there is limited but increasing evidence suggesting that climate change has a direct impact on pathogen virulence and disease development.
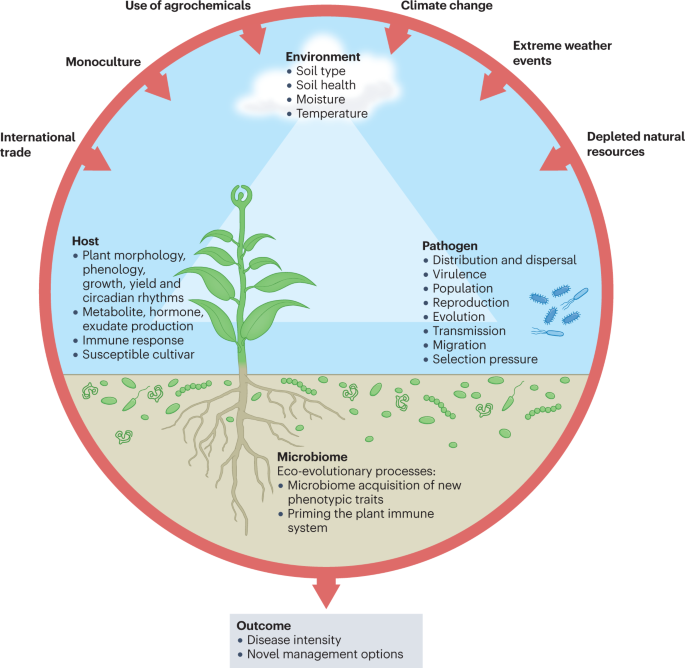
Intimate interactions among the plant, the environment, the soil and plant microbiomes, and invading pathogens impact the outcome of infection processes, disease severity and productivity of the plant. Environmental change and human activities (for example, global commodity and climate change) drive pathogen evolution and have increased disease threats to global crops. Genetically uniform crop monocultures and high planting density in modern agriculture have accelerated the emergence of virulent pathogens capable of overcoming disease-resistant crop varieties and promote the pathogen’s population size and genetic variability. Similarly, overreliance on pesticides has also fostered rapid emergence of new strains of pathogens. Pathogen transmission and anthropogenic pathogen movement due to, for example, international trade spreads pathogens to places free of natural enemies, and allows exchange of genetic material via horizontal gene transfer, facilitating adaptation to local hosts. Depletion of natural resources and natural landscapes has caused deterioration of the agroecosystem diversity. Emerging evidence suggests that soil and plant microbiomes influence the three angles of the disease paradigm — the host, the pathogen and the environment — by either facilitating or supressing pathogen attacks, by affecting plant physiology and immune response, and providing a line of defence and manipulating environmental conditions. For example, in disease-suppressive soils, indigenous microbiomes can reduce disease incidence, even in the presence of a pathogen, a susceptible host and a conductive environment. Explicit consideration of the role of the microbiome can improve our mechanistic understanding of disease outbreaks, which may lead to more effective prediction, monitoring and management of disease outbreaks. Better land management practices can improve overall soil health by influencing the diversity and functions of soil microbial communities, and could potentially be used to steer microbiomes that suppress diseases.
Elevated temperature
Climate warming can significantly impact aspects of the population dynamics of pathogens, such as overwintering and survival, population growth rates or the number of generations of polycyclic species. For example, a reduced diurnal temperature decreases the latency period of the coffee leaf rust pathogen Hemileia vastatrix , promoting rust epidemics in Central America 31 . Warming temperatures shorten the pathogen’s incubation period, resulting in increased abundance of the pathogen over a growing season. Higher temperature (along with high humidity) is linked to enhanced disease severity of potato blight pathogen ( Phytophthora infestans ) and phoma stem canker of oilseed rape. Increased mean winter temperatures enhanced the infection rates of American chestnut by fungal Phytophthora spp. causing extreme tree death events in North America 32 . Finally, a 30-year study reported the link between early snowmelt and higher snow blight ( Phacidium infestans ) infection in pine trees 33 .
Changes in global temperatures can profoundly impact the occurrence of pathogens in agricultural and natural ecosystems, increasing the risk of exposure to new pests and pathogens. Global warming is projected to increase the abundance of many fungal soil-borne plant pathogens, with significant consequences for primary productivity 14 . Warming temperatures can result in the development of new strains of pathogens that are better adapted and more virulent 7 , 12 , 13 , 19 . The severity of Fusarium head blight of wheat is likely to increase due to the shift from the milder Fusarium culmorum that prefers cool and wet conditions to the more aggressive Fusarium graminearum that prefers warm and humid conditions 34 . Similarly, more aggressive and temperature-tolerant novel strains of Puccinia striiformis have replaced older strains and are causing major outbreaks of wheat rust in the United States, Australia and Europe 35 , 36 . Warming temperatures can increase the range of many pathogens that are currently limited by requirements for overwintering, such as wheat stem rust caused by Puccinia graminis f. sp. tritici 37 . On the other hand, over a period of 30 years with a steady rise in summer temperatures, local extinction of Triphragmium ulmarie , the rust pathogen that infects Filipendula ulmaria (meadowsweet), was observed 38 . Other pathogens, such as Phytophthora infestans , are predicted to be little impacted by warming temperatures due to their lower thermal preferences 39 .
The molecular basis for why plants are more susceptible to pathogens at high temperatures is not well understood 13 . However, elevated temperatures can suppress plant immunity, leading to increased pathogen infection 24 . In Arabidopsis , production of salicylic acid, a hormone critical to plant defence, is suppressed at high temperatures 40 due to impaired activation of master immune transcription factors such as CBP60g (ref. 41 ). The CBP60g family transcription factors are widely conserved in plants 42 , and understanding their role in thermosensitive regulation of plant immunity provides clues for improved understanding of the warming effect on plant diseases. In rice, warm temperatures enhance expression of abscisic acid biosynthesis and responsive genes, and this is associated with increased susceptibility to bacterial blight disease; interestingly, suppression of the abscisic acid pathways was associated with resistance at elevated temperatures 43 . A recent study reported that induction of jasmonic acid biosynthesis and signalling genes by Magnaporthe oryzae results in enhanced susceptibility to rice blast disease in rice at warm temperatures 44 .
Elevated carbon dioxide
Varied disease incidence in conditions of increased carbon dioxide (CO 2 ) concentrations suggests pathogen and host-dependent responses to CO 2 . Elevated CO 2 levels increased the severity of powdery mildew on cucurbits caused by Sphaerotheca fuliginea 45 , as well as head blight and blotch on wheat caused by Fusarium spp. and Septoria tritici , respectively 46 , whereas the susceptibility of soybean towards the downy mildew pathogen Peronospora manshurica was reduced 47 . Similarly, changes in the leaf surface characteristics caused by elevated CO 2 treatment enhanced rust disease of aspen trees 48 but reduced the disease severity of brown spot disease of maple trees 49 . Atmospheric CO 2 impacts plant immune responses and hormone levels that can influence plant–pathogen interactions. For example, increased basal expression of jasmonic acid-responsive genes under elevated CO 2 enhanced resistance to the necrotrophic leaf pathogen Botrytis cinerea , but reduced the resistance to the hemi-biotrophic leaf pathogen Pseudomonas syringae pv. Tomato 50 . Reduction in the effectiveness of plant defence pathways under elevated CO 2 increased the susceptibility of wheat against the two major pathogens Z. tritici and F. graminearum that cause S. tritici blotch and Fusarium head blight, respectively 46 . Elevated CO 2 impacts tripartite biotic interactions between wheat, barley yellow dwarf virus (BYDV) and its aphid vector Rhopalosiphum padi . BYDV infection increased the aboveground nitrogen content of wheat growing under elevated CO 2 , as compared with non-infected plants, thereby reducing vector performance and phloem ingestion 51 . Elevated CO 2 clearly influences the outcome of plant–pathogen interactions, but currently no unifying framework exists to understand and predict its effects and consequences.
Climate change-induced variability in water availability
Variations in relative humidity and soil moisture are among the main drivers of abundance and infectivity of plant pathogens, and therefore climate-induced changes in humidity will likely impact future plant disease outbreaks 16 . Many fungal diseases require high humidity for spore germination and infection of their host plants 16 . High humidity generally promotes the virulence of pathogens infecting aerial plant tissues. Infection rates by Sclerotinia sclerotiorum in lettuce 52 and the stem rot pathogen Phytophthora sojae are higher under increased humidity 53 . Humidity-dependent expression of bacterial effectors that modify plant immune responses promotes establishment of P. syringae in the aqueous intercellular space (apoplast) of Arabidopsis leaves 54 . Higher humidity is also correlated with the increased production of the mycotoxin deoxynivalenol by F. graminearum , a pathogen infecting a range of grains, which results in significant economic losses and a reduction in food quality 55 , 56 . On the contrary, for M. oryzae , the causal agent of rice blast, and Streptomyces spp., causing bacterial scab in potato, lower moisture conditions increase pathogen numbers and disease severity 57 , 58 . Recent analyses suggest that an overall increase in relative humidity can increase the incidence of fungal-caused diseases in general 16 .
The impacts of drought on infection rates of pathogens and disease severity vary dramatically 59 . For example, diseases such as pea root rot (caused by Aphanomyces euteiches ), onion white rot ( Sclerotium cepivorum ), wheat take-all ( Gaeumannomyces graminis var. tritici ), wheat crown rot ( Fusarium spp.), brassica black leg ( Leptosphaeria maculans ) and grapevine black foot ( Ilyonectria/Dactylonectria spp.) increase in severity with the increase in the length and frequency of drought. On the other hand, drought reduced the severity of kiwifruit sclerotinia rot ( S. sclerotiorum ) and radiata pine red needle cast ( Phytophthora pluvialis ) 59 . Similar results were reported for the bacterial pathogen Xylella fastidiosa of grape 60 . In general, necrotrophs will accelerate drought-induced tree mortality by depleting tree resources as a result of repair and compartmentalization processes, whereas biotroph-caused diseases are expected to be less severe in drought because of the strong connection between pathogen performance and tree nutritional status. However, if biotrophs are able to invade stressed trees, they are expected to cause more severe drought-dependent impacts on trees because they deplete carbohydrate reserves important for tree drought tolerance 61 .
Drought-mediated shifts in the direction and strength of plant–pathogen interactions across an aridity gradient can modify disease range expansions in response to climate change 15 . For example, drought and higher rates of tree mortality in arid regions accelerate the decline in pine blister rust at low elevations, whereas lower alternate host occurrence at high elevations dampens infection probabilities, even as the climatic conditions become more hospitable. Drought can also result in the emergence of new pathogens that can withstand harsh environmental conditions and take advantage of the changes in plant physiology in response to stress. For example, drought favours the infection of chickpea plant by the dry root rot fungal pathogen Macrophomina phaseolina 62 . Drought-induced lowering of plant basal immune responses increased potato yellow vein virus infection and yellow vein disease symptoms 63 . These changes further modify host–virus–vector (greenhouse whitefly) interactions resulting in enhanced horizontal transmission of the virus.
Other variables and future scenarios
Although we have a limited understanding on the combined effects of multiple environmental factors on plant–pathogen interactions, a few studies have demonstrated that the combined effects are more pronounced than individual effects 64 , 65 , 66 and, in some cases, combinations of factors are required for outbreaks 16 , 67 . For example, an abnormally warm and humid pre-harvest season as a result of climate change was ascribed to the outbreak of M. oryzae triticum , the causal agent of wheat blast disease, in Bangladesh 68 . Similarly, high humidity and increased temperature promoted the disease incidence of B. cinerea in grape berries 69 . Altered climates (for example, increased temperature and soil moisture) can promote pathogen invasion and transmission across novel geographical and host ranges. In this respect, some fungal pathogens are more likely to spread in new regions of temperate and boreal biomes, as annual temperatures increase 14 , with disproportionally high negative impacts predicted on yield in Europe, China and some South American countries. Recent efforts have focused on understanding the global distribution of plant fungal pathogens under future climatic conditions 14 , but progress is limited by our knowledge of the current distribution of many important pathogens 5 , 70 (Supplementary Table 1 ). Modelling combined with experimental data suggested that the prevalence of key soil-borne fungal pathogens belonging to Alternaria, Fusarium, Venturia and Phoma genera will likely increase under projected global warming 14 . Further, our re-analysis of published global survey data 14 , 71 suggests that the relative abundance of some important soil-borne fungal taxa such as Penicillium spp., which damage fruit quality and production, are strongly associated with shifts in temperature and organic matter. Similarly, range expansion for Botryosphaeria dothidea and Neufusicoccum parvum resulting in more frequent and intensive disease outbreaks is predicted to be linked to climate change 72 . Conversely, the relative abundance of other soil-borne pathogens, such as the Oomycota taxa Phytophthora spp. and Pythium spp. 70 , may be highly sensitive to changes in soil pH associated with modifications in land use, and their distribution is likely to vary in response to climate change and land use intensification, with implications for food security worldwide (Fig. 2 ). Multiple environmental factors are known to interact with soil-borne pathogens, explaining complex patterns in the distribution of these microorganisms at a global scale (Fig. 2 ). Global shifts in the distribution of pathogens are concerning, as increasing evidence suggests that pathogens cause more damage in newly invaded regions and on new hosts than in their native region and hosts. As an example, ash dieback caused by the fungus Hymenoscyphus fraxineus causes minimal damage to ash native to Asia, where the pathogen originated, but has devastated European ash trees since invading Europe 30 years ago.
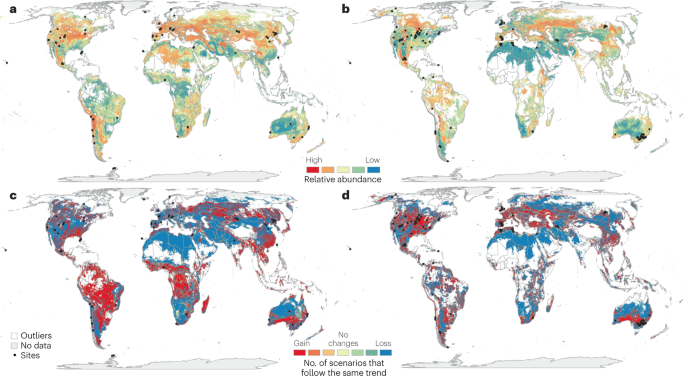
a , b , Current relative abundance of soil-borne potential plant pathogens: Phytophthora spp. and Pythium spp. (panel a ) and Penicillium sp. (panel b ). c , d , The projected change in their abundance under predicted future climates (2050): Phytophthora spp. and Pythium spp. (panel c ) and Penicillium sp. (panel d ) (also see Supplementary Table 1 ). Previously developed models 14 were implemented to project each map of the current and future relative abundance of plant pathogenic taxa worldwide. To implement these models, we performed exploratory correlation analyses to identify the most important factors associated with potential plant pathogen distributions from available data (see Supplementary Information). We used available data sets of climate variables, vegetation type, elevation and soil variables to identify the global distribution 14 , 141 . To perform projections of these pathogens in future climates, we used climatic and land use available data sets 142 , 143 , 144 , 145 . The prediction can be improved, as data from other locations will become available in the future. Areas of the projection away from the sampling points have been marked in white. The masking criterion was P < 0.01 to show the areas generated by the model in the projection that are closer to the sampling points (see Supplementary Information).
The interaction between climatic changes and evolutionary processes can also directly impact future pathogen outbreaks. Increases in frequency and intensities of extreme weather events can help spread pathogens to new locations, as for the case of soybean rust, which was introduced from Brazil into the United States by a hurricane 2 . Plant pathogens can also evolve to infect other plant species and/or become more virulent to overcome chemical and resistant cultivar control, or they may evolve into new pathogens by interspecific hybridization and extensive mutations. For example, a hybrid Phytophthora alni species that originated from the hybridization of Phytophthora uniformis and Phytophthora multiformis is the cause of severe decline of alder populations across Europe 73 . Overall, climate change will cause unknown shifts in the pathogen biology, host specificity and environmental favourability, making predictions challenging. Forecasting disease epidemic risks is also compounded by the interaction between agronomic practices (for example, agrochemicals, irrigation, plant variety), native and alternate host availability, connectivity and pathogen dispersal mechanisms (for example, airborne or vector-borne). Intensifying extreme weather events together with variations in land use and global trade are likely to further exacerbate pathogen transmission and disease incidence 74 . However, we have little knowledge about how these interactions among pathogen, biogeography, host and environment will influence disease management and efficacy of chemical, cultural and biological controls, key unknowns in securing food and environmental security for current and future generations.
Climate change, the plant microbiome and disease
Responses of the plant microbiome to climate change can also indirectly impact disease incidence. Plant microbiomes are likely impacted by climate change both via alterations in the starting inoculum from bulk soil or the rhizosphere 75 , 76 , 77 and by host responses, which include changes in host physiology, morphology, exudation patterns and immune responses 27 (Fig. 3 ). This is important because plant-associated microbiomes exert strong influences on host physiology, and contribute to the regulation of its metabolism, immune function and fitness in novel environments 25 , 27 , 78 , and play a key role in preventing the colonization and growth of pathogens. A range of mechanisms contribute to the control of plant pathogens by the plant microbiome, including the modulation of plant immune responses, competition with pathogens for resources and space, and/or production of antifungal effectors, lytic enzymes and secondary metabolites (including antibiotics, bacteriocins, toxins and siderophores) 25 . Plants can employ the ‘cry for help’ strategy that uses chemical stimuli for recruitment of beneficial microorganisms and traits from the soil, in order to enhance their capacity to combat pest-induced or pathogen-induced stresses 79 , 80 , 81 . The selective recruitment of beneficial microorganisms occurs through modulation of plant–microbiome signalling pathways 78 , 79 , 82 , altered root exudation patterns 81 , 83 , 84 and/or production of volatiles 85 . The most well-studied example of microbiota-mediated disease protection is disease-suppressive soil, where active microbiota contribute to disease reduction, even in the presence of the pathogen, susceptible host and favourable environmental conditions 80 , 86 , 87 . Complex ecological interactions and communication between plants and pathogens, between plant microbiomes and pathogens, and between plants and their microbiomes define disease outcomes but the specific mechanisms of interaction and the identity of communication molecules remain unclear 88 .
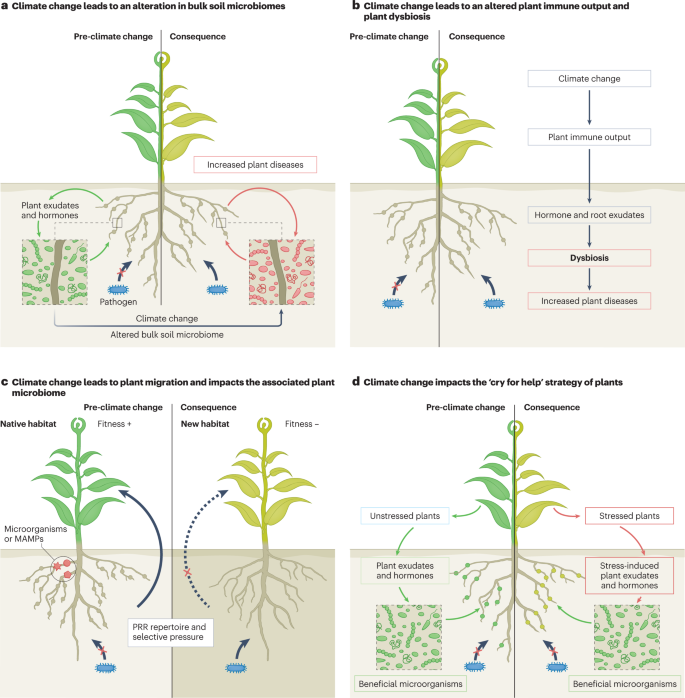
Four scenarios are proposed for microbiome responses to climate change. Green plants symbolize the healthy state of plants prior to climate change, whereas yellow plants indicate the effect of climate change and pathogen infection on plants. a , Plants employ an array of mechanisms that depend on optimal immune response, root exudates and hormonal balance to assemble complex microbiomes. Plant-associated microbiomes in soil, particularly in the rhizosphere, provide the first line of defence against pathogens. Climate change will likely alter the structure of the microbial reservoir in the bulk soil. This together with changes in the plant immunity may alter the rhizosphere microbiome assembly and change the first line of defence, which would allow the pathogen to breach. b , Plants maintain homeostasis of associated (leaf, root, stem, endophytes) microbiomes via tight and complex regulation of immune systems. Climate change can alter plant physiology and immune response, such as the production of the root exudates, volatile organic carbons and phytohormones, which may constrain the ability of plants to recruit and assemble beneficial microbiomes and promote dysbiosis of the plant leading to diseases. c , Plant migration to new locations (niche range shift) may interrupt the plant immune system and its mutualistic coevolution with indigenous soil microbiomes that support healthy plant growth and disease tolerance. As such, plant migration may expose them to local pathogens to which they are susceptible, whereas in some cases, migrating plants will escape local pathogens. Similarly, niche range shift in pathogens (along with evolutionary processes) can make the pathogen more transmissible and virulent in new regions in the absence of an effective immune response of local plants and resistance by local microflora. d , The ‘cry for help’ strategy of the plant, which refers to the plant recruiting beneficial microorganisms when under pathogen attack, is also likely be altered under climate change. Climate change may constrain the abilities of plants to produce signal molecules (for example, root exudates, volatiles and so on) to attract beneficial microorganisms and/or shift microbial composition and traits, or their ability to respond to these signals. Climate change could also reduce the burden of pathogen attacks where a shift in microbiomes has either enriched beneficial microorganisms or primed the plant immune response. MAMP, microorganism-associated molecular pattern; PRR, pattern recognition receptor.
Climate change variables (elevated CO 2 , warming and drought) can also increase root exudation and alter exudate composition in both crops and trees to attract beneficial microorganisms, which ultimately support plant growth 89 , 90 , 91 . Pathogens can overcome the first line of plant defence either directly, via competition with native microbial communities, or indirectly, by inducing changes in plant biology and physiology (for example, root exudation) 83 . The xylem-colonizing vascular wilt fungal pathogen Verticillium dahliae exploits effector proteins with antibacterial properties to manipulate the plant microbiota and colonize the host 92 . Pathogens can also modulate the plant microbiome by altering plant defence responses. For example, immune suppression by the wheat fungal pathogen Z. tritici causes fluctuations in the leaf microbial communities that render the plant vulnerable to further infections 93 . If the pathogen manages to displace a highly interacting keystone microbial species that facilitates interactions in the community, the entire microbial network may collapse, resulting in severe impairment of plant performance 94 . Members of the plant microbiome can themselves facilitate pathogen progression in some cases via signalling, metabolic interactions and weakening of the host immune response 95 , 96 . For example, the causal agent of olive knot disease, Pseudomonas savastanoi pv. savastanoi , exchanges quorum sensing signals with the native non-pathogenic strains of Pantoea agglomerans (an epiphyte, grows on the surface of the plant) and Erwinia toletana (an endophyte, lives within the plant) for increased colonization and disease incidence 97 . In many cases, shifts in the structure and function of plant microbiota have been observed for various plant–pathogen complexes 98 , 99 , 100 . A pathogen can co-occur with a range of members of plant microbiota 101 ; however, it is not yet clear whether observed changes in the microbial community composition contribute causally to pathogen colonization and disease.
As climate change can directly impact plant immune responses 24 , 40 , 102 , there is a possibility that plant microbial dysbiosis can facilitate pathogen invasion. For example, climate change-induced alteration in plant immune systems, which suppress pathogen invasion, can also negatively affect plant-microbiome assembly. For example, warming can increase or decrease microorganism-associated molecular pattern (MAMP)-triggered immunity and suppress plant effector-triggered immunity 26 , 103 , 104 . Similarly, production of salicylic acid is decreased under warming and drought. Climate-induced shifts in geographical distribution are suggested to have resulted in changes in the MAMP-induced selective pressure on pattern recognition receptor (PRR) repertoires, thereby impacting pathogen response, host fitness and the microbiome 105 . As the plant microbiome provides the first line of defence against pathogen invasion, changes in its structure and function can influence pathogen colonization. Plant immunity (including effector-triggered immunity, pattern-triggered immunity, RNA interference and defence hormone induction) has evolved not only to limit the growth of pathogens but also to maintain the homeostasis of the plant microbiome 105 . A recent study demonstrated the role of pattern-triggered immunity in preventing dysbiosis in the phyllosphere by regulating community structure and microbial abundances 106 . However, it remains to be evaluated whether plant immune-mediated alterations in the microbial community structure impact plant–pathogen interactions directly via shifting their overall response towards the incoming pathogen or indirectly via changes in plant fitness.
Although plant–microbiome–pathogen interactions will likely be altered in future climatic scenarios, we have limited knowledge to predict the overall directions and outcomes. We propose amendments to existing concepts (disease triangle and Koch’s postulates) and argue for explicit consideration of environmental and host microbiomes in disease concepts to obtain better mechanistic understanding of outbreaks for improved disease management. This can be further boosted by explicit inclusion of eco-evolutionary frameworks in future research, which will improve mechanistic knowledge and predictive models of pathogen invasion and disease outbreak (Box 1 ). For example, an experimental evolution study demonstrated that the relationship between a plant virus and its natural host can evolve from pathogenic to mutualistic under severe drought conditions 107 . As microbiomes are critical contributors to plant adaptation, we postulate that beneficial plant–microbiome interactions will evolve to maximize plant fitness against combined biotic and abiotic stresses under future climatic conditions 27 , 108 . For example, when exposed to soil-borne pathogens, the root-specific transcription factor MYB72 and the β-glucosidase BGLU42 regulate the synthesis and secretion of a coumarin molecule that inhibits pathogens but favours rhizobacteria, which induce systemic resistance 109 . Interestingly, coumarin accumulation is induced by osmotic and temperature stresses 110 , indicating a possible interplay of the plants’ ‘cry for help’ for selective recruitment of microbiota to tackle multiple stresses.
Box 1 Eco-evolutionary theories for prediction and management of plant diseases
Utilizing invasion ecology theory can help predict the success of new and emerging pathogens in new environments. Despite some variability, experimental and observational studies on plant communities have demonstrated that invasion success is linked to high dispersal ability, growth rate and resource use efficiency of alien species and/or to indigenous communities with low species diversity, high frequency of disturbances and rapid changes in resources 139 . Pathogen invasions are widespread and, similar to plant invasion, include dispersal, establishment, growth and spread, and impacts 140 . Ultimately, the success of pathogen invasion is likely influenced by the composition and diversity of the indigenous soil and plant microbial communities, where abiotic feedback (for example, pH and resource availability) and biotic feedback (for example, microbial–microbial interactions or impact of viruses and invertebrates; see the figure) modulate the rate and stability of colonization. Employing invasion theoretical frameworks can thus help in better predicting successful invasion by new pathogens, while also having the potential to support the development of disease management approaches, for example by providing tools to identify biological controls of pathogens 146 . However, it is important to note that microorganisms do have some distinct characteristics in terms of their physiology, genetics and behaviour. For example, unlike plant competition, microbial competition occurs via indirect interactions regulated by antibiotics, rapid gene acquisition and quorum sensing 147 . Explicit consideration of distinct microbial (pathogen) characteristics in an empirical framework can provide critical advancement in the understanding of pathogen invasion.
Other mechanisms that can help pathogen colonization include frequent disturbances (for example, increasing extreme weather events) and evolutionary processes. Frequent disturbances often alter indigenous communities, creating new niches and, hence, promoting invasion by alien species or niche occupation by less dominant species 140 . This is likely facilitated by increases in resource availability and decreased competition, as the abundance of the indigenous community decreases following disturbance (niche availability) 148 . Niche (complementarity) theory can help in identifying the relative contribution of microbial physiology, alteration in the indigenous community and disturbance-induced niche availability to the success of pathogen invasions. In addition, evolutionary processes can promote rapid diversification of pathogens that can promote a higher rate of colonization. Short generation times, large diversities and population sizes, and horizontal gene transfer can result in invading pathogens and/or indigenous populations of soil and plant microbial communities being able to rapidly acquire new phenotypes (for example, antibiotic biosynthetic genes, fungicide resistance genes) with direct impacts on the rate and success of pathogen colonization 147 . A system-based approach that addresses key eco-evolutionary mechanisms (pathogen ability versus habitat properties versus disturbance-induced niche availability) and identifies their relative contributions, combined with appropriate modelling tools, can significantly advance the discipline. For example, the use of random forest and structural equation models can identify the relative contribution of different factors, whereas the use of spatial individual-based models that incorporate an adaptive process, diversification and emergent behaviour can significantly improve our ability to predict the rate and success of pathogen invasion under different climate and environmental settings 149 , 150 , 151 . This framework can also be used to predict the success of microbial inoculants for pathogen controls.
The figure shows that pathogen (or any other invading or introduced microorganism) colonization involves invasion (dispersal ability), followed by microbial establishment, which requires the microorganisms to overcome biotic and abiotic constraints of the invaded environment, and to find a niche and manipulate the environment for proliferation and spread 140 . Effective colonization will have functional consequences. Ecological theories suggest that four ecological processes (dispersal, selection, drift and diversification) determine the success of colonization of new habitats by invading microorganisms.
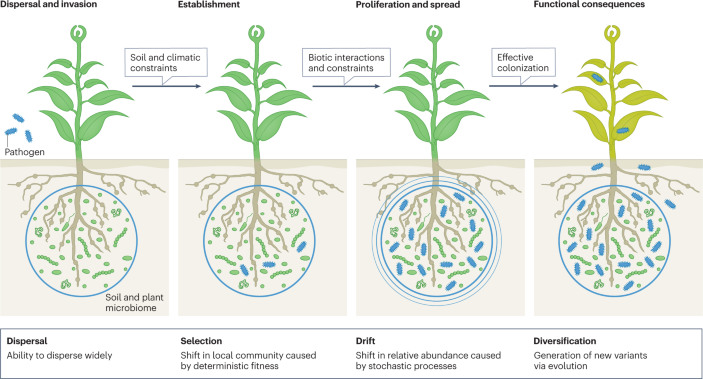
Paths forward
We propose three paths forward that address different but complementary disciplines of disease management.
Modern agriculture, pathogens and future mitigation strategies for sustainable land management
Pathogens are known to be highly sensitive to land management practices. For example, nitrogen and phosphorus fertilization consistently promote pathogenic over mutualistic fungi in grassland soils across four continents 111 . Conventional approaches to manage diseases rely on chemical fungicides and the use of disease-resistant varieties to control pathogens, but these methods have arguably reached their plateau, as in the case of soil-borne fungal pathogens which have increasingly become resistant to fungicides 112 . Currently, no effective chemical controls are available for diseases caused by widespread soil-borne pathogens such as Fusarium and Verticillium spp. in many crops 113 . Further, extensive use of chemical controls is increasingly discouraged due to both policy (for example, EU Green deals require 50% reduction in chemical pesticide use by 2030) and consumer demands, given their negative impacts on biodiversity including beneficial microorganisms, soil health and food quality (chemical residues), and ultimately human health 114 , 115 . Development of ecologically friendly chemicals needs prioritization, but these are currently expensive, and their development is time consuming. Also, there remains a risk: mid-term and long-term assessments have shown that chemicals initially considered environmentally friendly, such as organophosphorus and neonicotinoid, are in fact environmentally damaging.
The control of pathogens in natural ecosystems is addressed by different strategies at all levels. For example, maintaining species diversity in forest ecosystems could substantially reduce invasion of generalist plant pathogens 116 . Agroecosystems can adopt some of these approaches by promoting agrobiodiversity, intercropping and regular rotations of crops to improve resilience. However, cultural controls using rotations or fallow are economically challenging and are increasingly becoming ineffective, as pathogens evolve to be less sensitive to these practices. In the past, four or five non-cotton rotations were sufficient to reduce Fusarium and Verticillium wilt diseases in Australian cotton farms and allow profitable cotton production. Now, five to seven rotations are required for disease management 117 . Current strategies to address these problems include integrating disease resistance genes into crops by breeding (taking 10–20 years to release new crop-resistant cultivars) and through transgene cloning or gene editing (taking a few years but facing public and political concerns) 118 , 119 . In some cases, plant gene-mediated resistance can be overcome rapidly by evolving pathogens. The implementation of non-chemical tools such as biological control is complex, and outcomes are inconsistent 120 . Thus, fundamentally new approaches are needed to move beyond the current paradigm of disease management. Approaches that harness ecological and evolutionary interactions and other nature-based methods can provide future effective tools.
Technical innovations to monitor, manage and mitigate disease risks under global change
Current approaches to monitor, manage and mitigate disease risks are constrained by a focus on single-pathogen, single-crop and single-disease paradigms 121 . However, expanding studies that include other key aspects of disease, such as associated soil microbial and faunal communities and their interactions — a phytobiome approach 122 — can provide better scientific knowledge to drive improvement of predictive and management tools 123 , 124 . Similarly, integrating the biology and ecology of vectors and their response to climate change can improve predictability and risk associated with vector-borne pathogens (Box 2 ). However, accomplishing this is not trivial and will require a transformative shift in our approaches to plant diseases. For example, traditional notions such as the disease triangle would benefit by including emerging ecological concepts in host–pathogen interactions, such as the role of soil and plant (also vector) microbiomes (Fig. 1 ). Similarly, certain diseases can be caused by multiple microorganisms (for example, tomato pith necrosis, grapevine decline diseases 125 ) working in tandem and some of them cannot be isolated and re-introduced, limiting the effectiveness of the Koch postulates in establishing causative relationships between a microorganism and a disease 125 .
A transdisciplinary approach to understand pathogen biology and ecology from molecular to global scales is needed. Integrating available pathogen biology data with transport, trade, climate and geography can improve monitoring and predictive power of disease incidence. This can be further boosted by biochemical sensors, permanent observatories (airborne signals and vectors), satellite and remote sensing tools, artificial intelligence and the involvement of farmers and other volunteers to get an early report of disease, which can contribute towards effective surveillance 126 . Local data can be used to forecast the spread and severity of disease, and this can be upscaled to regional and global scales utilizing modelling and artificial intelligence tools (Box 2 ). These predictive tools would also contribute towards assessing the impact of disease on food production and other socio-economic indicators (jobs, income, mental health), thus supporting the development and implementation of effective mitigation tools 5 . In parallel, decision support systems based on systems biology approaches would be effective to manage disease risks, surveillance and forecasting. Such approaches should include precision agriculture, new eco-friendly chemicals and biologicals (for example, the microbiome and synthetic biology products), consideration of host genetic diversity and prediction of climate change on pathogens, host and vectors 121 . By integrating the impacts of climate change on agriculture and ecological sciences into predictive tools that also consider socio-economic information and international trade routes, better policies to effectively manage the risks of plant diseases could be developed.
A concerted effort involving technical innovations in microbiome, synthetic biology, precision agriculture and agroecological tools is needed to develop effective and sustainable solutions for plant disease managements (Box 3 ). Precision agricultural technologies based on drones, artificial intelligence and machine learning can identify disease early and assist effective and environmentally friendly targeted control measures, such as plant removal or precise dispersal of chemicals to plants. Recent advances in microbial (for example, microbiome engineering approaches), biochemical (volatiles and plant elicitors) and synthetic biology (synthetic microbial community (SynCom)) tools provide new pathways of disease management and reduced dependency on chemical controls 127 , 128 . In particular, there are emerging interests to harness plant and soil microbiomes to mitigate the negative consequences of climate change, varying from direct manipulation of microbiomes to indirect manipulation of their functions through changes in land management and farming practices, and the use of inoculants or biochemical products 27 . Beneficial plant microbiota can be harnessed to enhance plant fitness, reduce pathogen loads and prime plant defence signalling pathways 129 , 130 , 131 . Hence, microbial tools such as inoculants or microbiome engineering in situ promise optimized plant growth under the increasingly stressful conditions and pathogen attacks. Identifying beneficial seed and plant microbiota that can resist pathogen infection could, potentially, provide effective management tools as some of these microorganisms are inherited or actively recruited by the plant, and can hence improve the probability of successful host colonization and, ultimately, disease resistance 132 , 133 . Further, future plant breeding programmes should explicitly adopt a plant holobiont (host plus associate microbiomes) concept to ensure that new varieties can harness microbial symbionts to combat disease incidence 134 . Such tools can play an important role in mitigating deleterious consequences of pathogens on farm productivity and food availability under a climate change scenario 135 . However, systematic and coordinated studies are needed to advance understanding of the ecological and evolutionary processes that underpin interactions between plants, the associated microbiomes and pathogen invasions and how these are influenced by climate change, land management, agronomic practices and other ecosystem characteristics. This needs to be done with consideration of the climatic zone, crop and disease types, as well as management practices.
Box 2 Modelling future disease outbreaks
There is emerging consensus that climate change will increase disease risk and pest impacts in many parts of the world 10 , 152 . Crop models that included crop phenology and pathogen development show that the impact of climate change on crop losses would be host–pathogen dependent 39 , 153 , 154 . For example, shifts in planting dates due to cooler seasons will reduce the global risk of potato late blight by the end of this century 39 . On the other hand, climate change-mediated early anthesis in wheat will cause an increased incidence of Fusarium ear blight disease in China 153 . Mathematical models that combine epidemiology with spatial components and population heterogeneities are powerful tools to quantify the likelihood of success of management practices applied to invading pests and pathogens 155 , 156 , 157 , 158 . These models provide critical information on the most effective control measures, time and suitability of the intervention, site selection and cost balance to realistically manage emerging pests and pathogens. Unfortunately, the lack of robust quantitative and standardized data hinders efforts to make meaningful predictions to infer climate change impacts on disease epidemiology across crops, agroecosystems and regions 159 . Furthermore, although extreme weather events are predicted to increase in frequency and intensity in the future 160 , due to the lack of robust data sets we have limited modelling capability to predict the impact of these events on disease incidence and severity.
Disease epidemiology and dynamics depend on many factors including the interplay of the pathogen with the host plant, microbial communities, the changing environment and more. Linking information across scales (from genomes to landscapes) to predict disease outcomes in a rapidly changing world is a significant challenge 161 . However, many connections at intermediate scales are viable with integrated application of new systems biology approaches and powerful analytical and modelling techniques. For example, ‘omics’ combined with robust physiological/morphological/symptom training data sets can be used for predicting different aspects of plant–pathogen interactions including gene regulatory networks, pathogen effector proteins, pathogen adaptive strategies and genes involved in plant–pathogen interactions under different climate change scenarios. Genome-scale network reconstructions can model intracellular metabolism to predict virulence and pathogen–host interactions under a range of environmental and physiological conditions 162 , 163 , 164 . Such models are now being used to provide detailed insights into the interactions between the invading pathogen and the host-associated microbiome to predict disease incidence and interventions 165 . Ecological modelling approaches can provide information on the successful colonization of invading pathogens under a complex host-associated microbiome. For example, ‘game theory’ and the ‘Lotka–Volterra model’ can be expanded to describe microbial interactions related to pathogen colonization or extinction to predict disease emergence 166 . Statistical models can provide information on the direct and indirect impacts of environmental and biotic variables on disease incidence 167 , 168 , 169 , whereas dynamic network models allow incorporation of several aspects of disease epidemiology including molecular and cellular reactions, plant–vector–pathogen interactions, species interactions in the microbiome as well as international trade and social networks (reviewed elsewhere 170 ). Predictions of disease risks based on combinations of aerobiological models for inoculum transmission and crop-growth models offer a framework to quantify the impact of future climates on the risk of disease occurrence and spread 171 , 172 . However, most model development involves multidisciplinary knowledge integration, and the modelling approach should be transparent and flexible to allow users to select the level of details with which they would like to engage 126 . A model example is EMULSION, which couples a generic simulation engine to a domain-specific modelling language through structured text files, is readable by scientists from different fields (epidemiologists, biologists, economists) and allows those scientists to validate or revise assumptions at any stage of model development 173 .
Box 3 Technical innovations to better manage future disease outbreaks
Advancing fundamental knowledge of pathogen, vector and host biology, ecology and evolution is a key step forward to unravel the complexities of disease incidence and severity and the socio-economic impacts. How these variables will respond to climate change and the integration of such knowledge with climate and weather data, international trade and early pathogen detection can provide effective tools for forecasting and monitoring. These data can form the essential part of new integrated models along with big data analytics and artificial intelligence to predict regions at most risk of future disease outbreaks.
Molecular profiling is the most reliable method to detect and track the spread of plant disease, Several methods are now available to rapidly extract DNA in as little as 1 min without using sophisticated laboratory instruments or expensive kits and chemicals 174 , 175 , and could be scaled up to improve disease tracking in situ. For example, smartphone-based diagnostic platforms are now available that perform isothermal nucleic acid amplification and are designed for rapid and inexpensive pathogen detection in plants 175 . Ultraportable platforms such as POCKET (point-of-care kit for the entire test 176 ) are inexpensive and will enable a versatile sample-to-answer approach for pathogen diagnostics. Additionally, miniaturized sequencing platforms such as MinION or SmidgION will significantly improve portable DNA/RNA analysis. Using the hand-on sequencing platform in cost-efficient whole-genome sequencing technologies (US$1 per gigabase 177 ) is critical to enable surveillance (identity of genotype and strain), thus expediting studies on the origin of outbreaks, tracking transmission and pathogen evolution.
Other methods, including hyperspectral imaging, volatile organic compound fingerprinting and remote sensing, have the potential to revolutionize the pathogen and disease surveillance sector. Hyperthermal and thermal imaging data sets provide unique signatures to differentiate the infection of olive trees by two different xylem-limited pathogens ( Xylella fastidiosa and Verticillium dahliae ) 178 . A smartphone-based volatile organic compound sensor can profile key plant metabolites at parts per million levels within 1 min, and have been used to detect Phytophthora infestans in both laboratory-inoculated and field-collected tomato leaves with more than 95% accuracy 179 . High-resolution imagery captured by remote sensing technologies (for example, satellites and drones) coupled with advanced machine learning approaches can capture subtle changes in plant chemistry and detect pathogens far earlier than symptoms become visible 180 , 181 . Drones are also useful to detect and monitor spores of plant pathogens 182 , 183 . Digital epidemiology, which translates and analyses information from media, newswires, official reports and crowdsourcing and disseminates the information through media (including websites, email lists and mobile alerts), should be integrated in tracking disease dynamics around the world 184 .
Data mining and big data analytics provide unique opportunities to advance our understanding of complex biological processes at a level of accuracy without precedence 126 . Integrating knowledge gathered through these approaches into collaborative international research programmes, such as the Centre for Agriculture and Bioscience International (CABI) Global Burden of Crop Loss initiative or the Group on Earth Observations Global Agricultural Monitoring GEOGLAM) Initiative’s Crop Monitor that collate diverse data sources to build predictive disease impact models, can potentially enable targeted responses to plant pathogens. Baseline data on the plant pathogen and microbial communities are required to generate global microbial maps and detect climate-mediated changes. Initiatives such as the Global Initiative of Crop Microbiome 130 and the Soil Biodiversity Observatory Network 185 are working towards profiling the soil/plant-associated microbiome globally.
Science–policy interface and social innovations
Plant diseases affect all four pillars of food security: access, availability, utilization and stability 136 . Additionally, plant diseases can significantly impact the sociopolitical stability of a region or country in the absence of an effective policy framework to monitor, manage and mitigate disease impacts. One of the best examples is Phytophthora blight of potato, which caused the Irish famine in 1859, triggering the death of two million people and mass migrations; this event was a devastating consequence of the lack of effective policies to manage disease-associated risks. Other examples include famine and death linked to brown spot disease in rice in Bengal, India, and the recent outbreak of coffee rust that caused a more than 50% loss in coffee productivity and led to hunger, poverty and mass migration from Central America 5 , 126 . Clearly, prioritizing evidence-based policy to monitor, manage and mitigate the impact of plant diseases is critical to maintain socio-economic well-being. For effective management of plant diseases, we therefore propose a knowledge hub and networks of science–policy interfaces.
Knowledge hub
Climate-induced range shifts and emerging pathogens threaten agricultural productivity, trade and access to international markets 137 . Effective surveillance, forecasting tools and policies would help mitigate these risks to food security and human well-being. The current monitoring of plant disease is coordinated mostly at the regional and national levels, with strong disparities between developing and developed countries 138 . These disparities and the lack of international coordination hamper a quick response to new emerging or fast-spreading diseases. A global approach, such as the recently proposed global surveillance system 138 , is urgently needed to continuously monitor and predict global hot spots of important plant diseases, and their socio-economic impacts. Such a system would allow real-time monitoring and quick response to mitigate risks of new emerging or fast-spreading diseases internationally, and could be modelled and upscaled by joining together existing regional surveillance systems with national and regional hubs (for example, USABlight.org for the monitoring of Phytophthora infestans ). This network could help assemble, analyse and store data, and provide evidence and tools for monitoring and predicting disease progression and risks. This global information is critical to establish regulatory frameworks that include effective phytosanitary and quarantine rules for international trade.
Science–policy–society interfaces
To be effective, networks such as the global surveillance system would require the establishment of science–policy–society interfaces (SPSIs) operating at local, regional and global scales. If in continuous engagement with other organizations that are actively operating at the interface of science, policy and society, these SPSIs would provide assessment, monitoring, forecasting and provision of recommendations for policy actions that consider science, non-traditional knowledge (for example, indigenous and private sectors) and socio-economic conditions. Already active organizations such as the Consultative Group for International Agricultural Research (One CGIAR) would be in a strong position to coordinate a larger knowledge network, given the broad skill base and locations of their institutions in developing countries where new data need to be generated. With adequate mandate and resources, One CGIAR could coordinate periodic assessments, forecasting and monitoring of plant diseases, in partnership with the Food and Agriculture Organization of the United Nations (FAO). This could be boosted by harnessing expertise from other organizations and the global research community, such as the Intergovernmental Panel on Climate Change (IPCC) and the International Platform for Biodiversity and Ecosystem Services (IPBES), which act upon climate change and biodiversity loss, respectively. In fact, a formal association with these intergovernmental bodies including One Health will be mutually beneficial (Box 4 ), allowing the SPSIs to use their data, resources and models to predict disease incidence linked to climate change, biodiversity loss and consequences for plant and human health. Similar to the COVID-19 pandemic, in a globalized economy and trade market, plant pathogen transmission is difficult to control or restrict to a particular region. Thus, a global effort is essential to manage socio-economic risks associated with the likely increases in plant disease outbreaks, especially in low-income countries. Plant disease control in developing countries will not only reduce risk of transmission into those areas but also contribute towards food and job security for local communities, with the potential to reduce illegal migration and national or regional conflicts.
Box 4 Plant diseases as an integral part of the One Health concept
The One Health concept consists of effective measures for surveillance, forecasting and mitigation of zoonotic diseases and can provide an important framework and infrastructure to better manage plant diseases 74 . Plant diseases and their control impact multiple dimensions of human health, including the availability and quality of food, food safety (mycotoxin-producing plant pathogens such as Aspergillus spp. and Fusarium spp.), vectors of human pathogens ( Escherichia coli , Salmonella spp., Listeria monocytogenes in lettuce and other vegetables and fruits), antimicrobial resistance (transfer of antimicrobial resistance from food microbiomes to human gut microorganisms) and exposure to harmful chemical pesticides (for example, organophosphate chronic exposure and their carcinogenic and genotoxic effects) 114 , 136 . Explicit consideration of plant diseases in the One Health concept can provide an effective framework to simultaneously mitigate risks of human health that are directly linked to plant health (for example, human infection, agrochemicals) and spread of plant diseases. The One Health concept poses a transdisciplinary approach to identify epidemics of human disease based on biological and evolutionary aspects of the pathogen and host, habitat characteristics and connectivity and surveillance of wildlife reservoirs. Potential constraints, challenges and solutions for plant diseases are fundamentally similar to human and animal pathogens, and employing common approaches could help in identifying, predicting, monitoring and managing emerging plant diseases. Similarly, the One Health concept provides a holistic framework encompassing human and veterinary medicines, analytical practices, social and conservation science, environmental science and policymaking to manage the outbreak and spread of zoonotic diseases. A similar framework is needed for plant disease to manage increasing risks associated with climate change. In addition, the adoption of One Health data sharing can overcome some cultural, economic and political constraints in plant diseases and can be facilitated through capacity building and data repositories. A framework that allows incorporation of multiple potential pathways that can impact public health is critical to systematically and holistically manage risks associated with public health.
Inclusion of plant health into One Health can also further strengthen the monitoring and managing of human health risks associated with food safety (for example, human pathogens in agricultural produce) 74 . This will help streamline regulatory frameworks, improve surveillance measures and enable development of effective tools for human health. Inclusion of plant disease in One Health can also contribute towards capacity building in low-income countries through the integration of activities with other intergovernmental agencies including those that impact global biodiversity (International Platform for Biodiversity and Ecosystem Services (IPBES)) and climate change (Intergovernmental Panel on Climate Change (IPCC)). Overall, inclusion of plant disease (health) in the One Health concept will be mutually beneficial for human health outcomes as well ensuring food security and environmental sustainability 74 .
Conclusion and future perspectives
The current research landscape lacks some key fundamental knowledge to exploit emerging tools to manage disease risks. A holistic solution will require significant expansions of our current knowledge beyond disease monitoring and chemical controls. We need to improve our understanding of pathogen, vector and host biological, ecological and evolutionary responses to climate change. This should include identification of pathobiomes (group of microorganisms and invertebrates that help or hamper infection and disease progression) and their response to climate change. The use of existing ecological theories (for example, invasion theory; co-existing theory/network theory for microbiome coalescence) could provide a strong framework to study and predict pathogen transmission in new regions or to new hosts, and how they interact with host and soil microbiomes 139 , 140 . Similarly, the integration of evolutionary processes (for example, new phenotype acquisition by pathogens or indigenous microflora via horizontal gene transfer or mutations) could advance our fundamental knowledge on the mechanisms of pathogenicity (Box 1 ). We also need an improved understanding of plant phenology (the study of seasonal changes in plants) and disease interactions. Different plant species are vulnerable to pathogens at different stages of plant growth. For example, Fusarium and Verticillium pathogens mainly infect the host at early stages of growth to cause wilt disease 117 . Similarly, for many diseases (for example, powdery mildews of grapevine and strawberry), young leaves are more susceptible to pathogen infection than the mature leaves. Given that climate change will likely impact plant growth and phenology, we need to address how these changes might affect the susceptibility of plants to disease, in order to develop targeted strategies for disease management.
Measuring pathogen movement through air and water systems is needed to forecast pathogen loads as a result of climate change-driven changes in weather, wind direction and extreme weather events. Individual and interactive effects of climate factors such as temperature, precipitation and drought on disease manifestation and their interaction in different climatic zones should also be considered 121 . To achieve this, it is possible to use permanent observatories that monitor pollutants and microorganisms, together with drone technologies capable of sampling at 100 m above the ground. Better tools for disease surveillance and management are needed. Remote sensing and drones, enhanced sensor-based technologies (for example, analysis of metagenomes or volatiles) and population genomics along with data mining of social networks can be refined with more accurate data to improve disease surveillances. There is a need for improved modelling tools that integrate climate, weather, epidemiological and socio-economic models for prediction of future outbreaks and effective risk management. Importantly, most of the attention has been placed on diseases of commercial crops, yet the role of wild and native plants, which can act as alternate hosts or barriers to pathogen spread, in disease incidence remains underexplored. Climate change is likely to have impact on the range shift of wild plants, and how this will affect plant disease and epidemics is not known 8 . Similarly, we have very little understanding on how plant-associated microbiomes, which play a critical role in disease progression or restriction, will respond to climate change and the consequences for pathogen infections. Finally, to achieve effective monitoring and management of plant diseases, socio-economic aspects must be considered. The implementation of effective new computational information systems that support organizations in decision-making (detection or decision support systems) need to be user-friendly and accessible to small-holder farmers who may not be technology savvy. Easy-to-use tools with substantial training will be needed to implement new approaches that have better predictive power and more effective management advice. In this respect, mobile phone-based applications could be a useful tool as most people are familiar with their operation. The lack of adoption of new tools remains a major challenge, mostly because of poor predictive power. However, agricultural scientists could adopt an approach similar to weather forecasting, that is largely accepted by most stakeholders including farming communities. Overall, collaborations with socio-economic and behavioural sciences can contribute towards the development of strategies that encourage greater adoption of all these tools, such as subsidies and/or insurance to cover crop failure.
Available scientific evidence and simulation models suggest that plant disease pressures will significantly increase as climate change intensifies, thus negatively impacting food safety and the sustainability of natural ecosystems. As the magnitude and mechanisms of these impacts remain largely uncertain, effective monitoring and management of plant pathogens should be one of the highest priorities to minimize disease, ensure food safety and environmental sustainability, and promote better socio-economic outcomes. This is made difficult by scientific knowledge gaps around the ecological and evolutionary response of pathogens, hosts and vectors to climate change, and the transmission and emergence of new pathogens under increasing intensity and frequency of extreme weather events and international trade. We propose amendment of existing concepts (disease triangle and Koch’s postulates) and incorporation of eco-evolutionary theories to improve the mechanistic understanding and prediction of disease outbreaks under future climatic conditions and in new regions. Concerted efforts to integrate and harness emerging tools (for example, genomics, satellite, digital, big data, machine learning) for early detection, monitoring and prediction of disease outbreaks will enable sustainable management of disease from local to global scales. These are not trivial goals and will require coordinated research and policy actions from all levels of relevant organizations. We believe that the formation of a dedicated knowledge hub–SPSI in partnership with existing intergovernmental bodies in the context of One Health could help achieve those goals. To improve the detection, monitoring and management of plant pathogens in the face of changing climate, it will be key that important stakeholders (research funding providers, policymakers, intergovernmental agencies) worldwide commit to dedicate more resources to research and SPSIs.
Tripathi, A. N., Tiwari, S. K. & Behera, T. K. in Postharvest Technology Ch. 5 (ed. Ahiduzzaman, M. D.) (IntechOpen, 2022).
Fones, H. N. et al. Threats to global food security from emerging fungal and oomycete crop pathogens. Nat. Food 1 , 332–342 (2020). This paper highlights the main knowledge gaps and proposes a research direction to address challenges associated with emerging crop fungal pathogens.
PubMed Google Scholar
Chakraborty, S. & Newton, A. C. Climate change, plant diseases and food security: an overview. Plant. Pathol. 60 , 2–14 (2011).
Google Scholar
Rohr, J. R. et al. Emerging human infectious diseases and the links to global food production. Nat. Sustain. 2 , 445–456 (2019).
PubMed PubMed Central Google Scholar
Ristaino, J. B. et al. The persistent threat of emerging plant disease pandemics to global food security. Proc. Natl Acad. Sci. USA https://doi.org/10.1073/pnas.2022239118 (2021). This paper proposes integrated research priorities that can potentially reduce the burden of future disease pandemics.
Article PubMed PubMed Central Google Scholar
van Dijk, M., Morley, T., Rau, M. L. & Saghai, Y. A meta-analysis of projected global food demand and population at risk of hunger for the period 2010–2050. Nat. Food 2 , 494–501 (2021).
Velasquez, A. C., Castroverde, C. D. M. & He, S. Y. Plant–pathogen warfare under changing climate conditions. Curr. Biol. 28 , R619–R634 (2018).
CAS PubMed PubMed Central Google Scholar
Burdon, J. J. & Zhan, J. Climate change and disease in plant communities. PLoS Biol. 18 , e3000949 (2020). This manuscript highlights the importance of plant–pathogen interactions and evolution on disease incidence under future climates.
Muluneh, M. G. Impact of climate change on biodiversity and food security: a global perspective—a review article. Agric. Food Secur. 10 , 36 (2021).
Chaloner, T. M., Gurr, S. J. & Bebber, D. P. Plant pathogen infection risk tracks global crop yields under climate change. Nat. Clim. Change 11 , 710–715 (2021). This paper predicts that the yield gain for 12 crops under future climates will be tempered by increased infection rates by plant pathogens.
Trumbore, S., Brando, P. & Hartmann, H. Forest health and global change. Science 349 , 814–818 (2015).
CAS PubMed Google Scholar
Newbery, F., Qi, A. & Fitt, B. D. L. Modelling impacts of climate change on arable crop diseases: progress, challenges and applications. Curr. Opin. Plant. Biol. 32 , 101–109 (2016).
Cohen, S. P. & Leach, J. E. High temperature-induced plant disease susceptibility: more than the sum of its parts. Curr. Opin. Plant Biol. 56 , 235–241 (2020).
Delgado-Baquerizo, M. et al. The proportion of soil-borne pathogens increases with warming at the global scale. Nat. Clim. Change 10 , 550–554 (2020). This paper provides the first global atlas of soil-borne plant pathogens and projects an overall increase in their relative abundance under future climates.
Dudney, J. et al. Nonlinear shifts in infectious rust disease due to climate change. Nat. Commun. 12 , 5102 (2021).
Romero, F. et al. Humidity and high temperature are important for predicting fungal disease outbreaks worldwide. N. Phytol. 234 , 1553–1556 (2022).
Brown, J. K. & Hovmøller, M. S. Aerial dispersal of pathogens on the global and continental scales and its impact on plant disease. Science 297 , 537–541 (2002).
Sikes, B. A. et al. Import volumes and biosecurity interventions shape the arrival rate of fungal pathogens. PLoS Biol. 16 , e2006025 (2018).
Fisher, M. C. et al. Threats posed by the fungal kingdom to humans, wildlife, and agriculture. mBio https://doi.org/10.1128/mBio.00449-20 (2020).
Goellner, K. et al. Phakopsora pachyrhizi , the causal agent of Asian soybean rust. Mol. Plant Pathol. 11 , 169–177 (2010).
Jeger, M. J. The impact of climate change on disease in wild plant populations and communities. Plant Pathol. 71 , 111–130 (2022).
Thompson, S. E., Levin, S. & Rodriguez-Iturbe, I. Rainfall and temperatures changes have confounding impacts on Phytophthora cinnamomi occurrence risk in the southwestern USA under climate change scenarios. Glob. Chang. Biol. 20 , 1299–1312 (2014).
Rigg, J. L., McDougall, K. L. & Liew, E. C. Y. Susceptibility of nine alpine species to the root rot pathogens Phytophthora cinnamomi and P. cambivora . Australas. Plant Pathol. 47 , 351–356 (2018).
CAS Google Scholar
Cheng, Y. T., Zhang, L. & He, S. Y. Plant–microbe interactions facing environmental challenge. Cell Host Microbe 26 , 183–192 (2019).
Trivedi, P., Leach, J. E., Tringe, S. G., Sa, T. & Singh, B. K. Plant–microbiome interactions: from community assembly to plant health. Nat. Rev. Microbiol. 18 , 607–621 (2020). This paper highlights different processes involved in plant-microbiome assembly and the beneficial traits that microbiomes provide to their host plants.
Desaint, H. et al. Fight hard or die trying: when plants face pathogens under heat stress. N. Phytol. 229 , 712–734 (2021).
Trivedi, P., Batista, B. D., Bazany, K. E. & Singh, B. K. Plant–microbiome interactions under a changing world: responses, consequences and perspectives. N. Phytol. 234 , 1951–1959 (2022). This paper proposes that the adaptation of plants to climate change will be driven by the plant microbiome in the short term (years to decades) whereas the eco-evolutionary response will determine the long-term (century to millennia) plant adaptation.
Desprez-Loustau, M.-L., Marçais, B., Nageleisen, L.-M., Piou, D. & Vannini, A. Interactive effects of drought and pathogens in forest trees. Ann. Sci. 63 , 597–612 (2006).
Ryu, M., Mishra, R. C., Jeon, J., Lee, S. K. & Bae, H. Drought-induced susceptibility for Cenangium ferruginosum leads to progression of Cenangium -dieback disease in Pinus koraiensis . Sci. Rep. 8 , 16368 (2018).
Hossain, M., Veneklaas, E. J., Hardy, G. & Poot, P. Tree host–pathogen interactions as influenced by drought timing: linking physiological performance, biochemical defence and disease severity. Tree Physiol. 39 , 6–18 (2019).
Toniutti, L. et al. Influence of environmental conditions and genetic background of Arabica coffee ( C. arabica L) on leaf rust ( Hemileia vastatrix ) pathogenesis. Front. Plant. Sci. 8 , 2025 (2017).
Gustafson, E. J., Miranda, B. R., Dreaden, T. J., Pinchot, C. C. & Jacobs, D. F. Beyond blight: Phytophthora root rot under climate change limits populations of reintroduced American chestnut. Ecosphere 13 , 18 (2022).
Barbeito, I., Brücker, R. L., Rixen, C. & Bebi, P. Snow fungi-induced mortality of Pinus cembra at the alpine treeline: evidence from plantations. Arct. Antarct. Alp. Res. 45 , 455–470 (2013).
Parikka, P., Hakala, K. & Tiilikkala, K. Expected shifts in Fusarium species’ composition on cereal grain in Northern Europe due to climatic change. Food Addit. Contam. A 29 , 1543–1555 (2012).
Walter, S. et al. Molecular markers for tracking the origin and worldwide distribution of invasive strains of Puccinia striiformis . Ecol. Evol. 6 , 2790–2804 (2016).
Vidal, T. et al. Success and failure of invasive races of plant pathogens: the case of Puccinia striiformis f. sp. tritici in France. Plant. Pathol. https://doi.org/10.1111/ppa.13581 (2022).
Article Google Scholar
Ma, L. et al. Effect of low temperature and wheat winter-hardiness on survival of Puccinia striiformis f. sp. tritici under controlled conditions. PLoS ONE 10 , e0130691 (2015).
Zhan, J., Ericson, L. & Burdon, J. J. Climate change accelerates local disease extinction rates in a long-term wild host–pathogen association. Glob. Chang. Biol. 24 , 3526–3536 (2018).
Sparks, A. H., Forbes, G. A., Hijmans, R. J. & Garrett, K. A. Climate change may have limited effect on global risk of potato late blight. Glob. Chang. Biol. 20 , 3621–3631 (2014).
Castroverde, C. D. M. & Dina, D. Temperature regulation of plant hormone signaling during stress and development. J. Exp. Bot. https://doi.org/10.1093/jxb/erab257 (2021).
Article PubMed Google Scholar
Kim, J. H. et al. Increasing the resilience of plant immunity to a warming climate. Nature 607 , 339–344 (2022).
Zheng, Q., Majsec, K. & Katagiri, F. Pathogen‐driven coevolution across the CBP60 plant immune regulator subfamilies confers resilience on the regulator module. N. Phytol. 233 , 479–495 (2022).
Cohen, S. P. et al. RNA-seq analysis reveals insight into enhanced rice Xa7 -mediated bacterial blight resistance at high temperature. PLoS ONE 12 , e0187625 (2017).
Qiu, J. et al. Warm temperature compromises JA-regulated basal resistance to enhance Magnaporthe oryzae infection in rice. Mol. Plant 15 , 723–739 (2022).
Khan, M. R. & Rizvi, T. F. Effect of elevated levels of CO 2 on powdery mildew development in five cucurbit species. Sci. Rep. https://doi.org/10.1038/s41598-020-61790-w (2020).
Vary, Z., Mullins, E., McElwain, J. C. & Doohan, F. M. The severity of wheat diseases increases when plants and pathogens are acclimatized to elevated carbon dioxide. Glob. Change Biol. 21 , 2661–2669 (2015). This paper provides empirical evidence that increases in CO 2 concentration will enhance the severity of fungal diseases in wheat.
Eastburn, D. M., Degennaro, M. M., Delucia, E. H., Dermody, O. & Mcelrone, A. J. Elevated atmospheric carbon dioxide and ozone alter soybean diseases at SoyFACE. Glob. Change Biol. 16 , 320–330 (2010).
Karnosky, D. F. et al. Interacting elevated CO 2 and tropospheric O 3 predisposes aspen ( Populus tremuloides Michx.) to infection by rust ( Melampsora medusae f. sp. tremuloidae ). Glob. Change Biol. 8 , 329–338 (2002).
Mcelrone, A. J., Reid, C. D., Hoye, K. A., Hart, E. & Jackson, R. B. Elevated CO 2 reduces disease incidence and severity of a red maple fungal pathogen via changes in host physiology and leaf chemistry. Glob. Change Biol. 11 , 1828–1836 (2005).
Zhou, Y., Van Leeuwen, S. K., Pieterse, C. M. J., Bakker, P. A. H. M. & Van Wees, S. C. M. Effect of atmospheric CO 2 on plant defense against leaf and root pathogens of Arabidopsis . Eur. J. Plant Pathol. 154 , 31–42 (2019).
Trębicki, P. et al. Virus infection mediates the effects of elevated CO 2 on plants and vectors. Sci. Rep. 6 , 22785 (2016).
Mamo, B. E. et al. Epidemiological characterization of lettuce drop ( Sclerotinia spp.) and biophysical features of the host identify soft stem as a susceptibility factor. PhytoFrontiers 1 , 182–204 (2021).
Tada, T., Tanaka, C., Katsube-Tanaka, T. & Shiraiwa, T. Effects of wounding and relative humidity on the incidence of Phytophthora root and stem rot in soybean seedlings. Phsiol. Mol. Plant Pathol. 116 , 101737 (2021).
Xin, X.-F. et al. Bacteria establish an aqueous living space in plants crucial for virulence. Nature 539 , 524–529 (2016).
Andersen, K. F., Madden, L. V. & Paul, P. A. Fusarium head blight development and deoxynivalenol accumulation in wheat as influenced by post-anthesis moisture patterns. Phytopathology 105 , 210–219 (2015).
Qiu, J. B., Dong, F., Yu, M. Z., Xu, J. H. & Shi, J. R. Effect of preceding crop on Fusarium species and mycotoxin contamination of wheat grains. J. Sci. Food Agric. 96 , 4536–4541 (2016).
Johansen, T. J., Dees, M. W. & Hermansen, A. High soil moisture reduces common scab caused by Streptomyces turgidiscabies and Streptomyces europaeiscabiei in potato. Acta Agric. Scand. B Soil Plant Sci. 65 , 193–198 (2015).
Bidzinski, P. et al. Transcriptional basis of drought-induced susceptibility to the rice blast fungus Magnaporthe oryzae . Front. Plant Sci. https://doi.org/10.3389/fpls.2016.01558 (2016).
Wakelin, S. A. et al. Climate change induced drought impacts on plant diseases in New Zealand. Australas. Plant Pathol. 47 , 101–114 (2018).
Choi, H.-K., Iandolino, A., da Silva, F. G. & Cook, D. R. Water deficit modulates the response of Vitis vinifera to the Pierce’s disease pathogen Xylella fastidiosa . Mol. Plant Microbe Interact. 26 , 643–657 (2013).
Oliva, J., Stenlid, J. & Martinez-Vilalta, J. The effect of fungal pathogens on the water and carbon economy of trees: implications for drought-induced mortality. N. Phytol. 203 , 1028–1035 (2014).
Rai, A., Irulappan, V. & Muthappa, S.-K. Dry root rot of chickpea: a disease favored by drought. Plant Dis. https://doi.org/10.1094/PDIS-07-21-1410-FE (2021).
Vasquez, D. F. et al. Drought as a modulator of plant–virus–vector interactions: effects on symptom expression, plant immunity and vector behaviour. Plant Pathol. 71 , 1282–1292 (2022).
Webb, K. M. et al. A benefit of high temperature: increased effectiveness of a rice bacterial blight disease resistance gene. N. Phytol. 185 , 568–576 (2010).
Cohen, S. P. & Leach, J. E. Abiotic and biotic stresses induce a core transcriptome response in rice. Sci. Rep. 9 , 6273 (2019).
Teshome, D. T., Zharare, G. E. & Naidoo, S. The threat of the combined effect of biotic and abiotic stress factors in forestry under a changing climate. Front. Plant Sci. https://doi.org/10.3389/fpls.2020.601009 (2020).
Sewelam, N., El-Shetehy, M., Mauch, F. & Maurino, V. G. Combined abiotic stresses repress defense and cell wall metabolic genes and render plants more susceptible to pathogen infection. Plants https://doi.org/10.3390/plants10091946 (2021).
Islam, M. T., Kim, K. H. & Choi, J. Wheat blast in Bangladesh: the current situation and future impacts. Plant Pathol. J. 35 , 1–10 (2019).
Ciliberti, N., Fermaud, M., Roudet, J. & Rossi, V. Environmental conditions affect Botrytis cinerea infection of mature grape berries more than the strain or transposon genotype. Phytopathology 105 , 1090–1096 (2015).
Juroszek, P. & von Tiedemann, A. Linking plant disease models to climate change scenarios to project future risks of crop diseases: a review. J. Plant Dis. Prot. 122 , 3–15 (2015). This paper highlights the importance of including several climate factors and multiple pathogen life stages in the modelling of pathogens, disease incidence and range shifts for reliable future predictions.
Guerra, C. A. et al. Global hotspots for soil nature conservation. Nature 610 , 693–698 (2022).
Batista, E., Lopes, A., Miranda, P. & Alves, A. Can species distribution models be used for risk assessment analyses of fungal plant pathogens? A case study with three Botryosphaeriaceae species. Eur. J. Plant Pathol. https://doi.org/10.1007/s10658-022-02587-7 (2022).
Mizeriene, G. et al. Patterns of genetic diversification in the invasive hybrid plant pathogen Phytophthora × alni and its parental species P. uniformis . Phytopathology 110 , 1959–1969 (2020).
Morris, C. E., Géniaux, G., Nédellec, C., Sauvion, N. & Soubeyrand, S. One Health concepts and challenges for surveillance, forecasting, and mitigation of plant disease beyond the traditional scope of crop production. Plant Pathol. 71 , 86–97 (2022).
Wang, P. et al. Shifts in microbial communities in soil, rhizosphere and roots of two major crop systems under elevated CO 2 and O 3 . Sci. Rep. 7 , 15019 (2017).
Santos-Medellín, C. et al. Prolonged drought imparts lasting compositional changes to the rice root microbiome. Nat. Plants 7 , 1065–1077 (2021).
Bazany, K. E., Wang, J. T., Delgado-Baquerizo, M., Singh, B. K. & Trivedi, P. Water deficit affects inter-kingdom microbial connections in plant rhizosphere. Environ. Microbiol. https://doi.org/10.1111/1462-2920.16031 (2022).
Liu, H., Brettell, L. E., Qiu, Z. & Singh, B. K. Microbiome-mediated stress resistance in plants. Trends Plant Sci. 25 , 733–743 (2020).
Berendsen, R. L. et al. Disease-induced assemblage of a plant-beneficial bacterial consortium. ISME J. 12 , 1496–1507 (2018). This paper provides empirical evidence that upon pathogen attack the plant recruits beneficial microorganisms on the roots to reduce disease impacts and also to promote the survival of offspring in subsequent years.
Carrion, V. J. et al. Pathogen-induced activation of disease-suppressive functions in the endophytic root microbiome. Science 366 , 606–612 (2019).
Rolfe, S. A., Griffiths, J. & Ton, J. Crying out for help with root exudates: adaptive mechanisms by which stressed plants assemble health-promoting soil microbiomes. Curr. Opin. Microbiol. 49 , 73–82 (2019).
Gao, M. et al. Disease-induced changes in plant microbiome assembly and functional adaptation. Microbiome 9 , 187 (2021).
Yuan, J. et al. Root exudates drive the soil-borne legacy of aboveground pathogen infection. Microbiome 6 , 156 (2018).
Kim, J. Y. et al. Cellular export of sugars and amino acids: role in feeding other cells and organisms. Plant. Physiol. 187 , 1893–1915 (2021).
Schulz-Bohm, K. et al. Calling from distance: attraction of soil bacteria by plant root volatiles. ISME J. 12 , 1252–1262 (2018).
Trivedi, P., Schenk, P. M., Wallenstein, M. D. & Singh, B. K. Tiny microbes, big yields: enhancing food crop production with biological solutions. Microb. Biotechnol. 10 , 999–1003 (2017).
Mendes, R. et al. Deciphering the rhizosphere microbiome for disease-suppressive bacteria. Science 332 , 1097–1100 (2011). This paper identifies soil microorganisms that are responsible for disease suppression traits of some soils.
Leach, J. E., Triplett, L. R., Argueso, C. T. & Trivedi, P. Communication in the phytobiome. Cell 169 , 587–596 (2017).
Wu, J. & Yu, S. Effect of root exudates of Eucalyptus urophylla and Acacia mearnsii on soil microbes under simulated warming climate conditions. BMC Microbiol. 19 , 224 (2019).
Wang, Q. et al. The effects of warming on root exudation and associated soil N transformation depend on soil nutrient availability. Rhizosphere 17 , 100263 (2021).
Ulrich, D. E. M. et al. Root exudate composition reflects drought severity gradient in blue grama ( Bouteloua gracilis ). Sci. Rep. 12 , 12581 (2022).
Snelders, N. C., Petti, G. C., van den Berg, G. C. M., Seidl, M. F. & Thomma, B. P. H. J. An ancient antimicrobial protein co-opted by a fungal plant pathogen for in planta mycobiome manipulation. Proc. Natl Acad. Sci. USA https://doi.org/10.1073/pnas.2110968118 (2021).
Seybold, H. et al. A fungal pathogen induces systemic susceptibility and systemic shifts in wheat metabolome and microbiome composition. Nat. Commun . 11 , 1910 (2020).
Agler, M. T. et al. Microbial hub taxa link host and abiotic factors to plant microbiome variation. PLoS Biol. 14 , e1002352 (2016).
Venturi, V. & da Silva, D. P. Incoming pathogens team up with harmless ‘resident’ bacteria. Trends Microbiol. 20 , 160–164 (2012).
Trivedi, P., Trivedi, C., Grinyer, J., Anderson, I. C. & Singh, B. K. Harnessing host-vector microbiome for sustainable plant disease management of phloem-limited bacteria. Front. Plant Sci. 7 , 1423 (2016).
Hosni, T. et al. Sharing of quorum-sensing signals and role of interspecies communities in a bacterial plant disease. ISME J. 5 , 1857–1870 (2011).
Trivedi, P. et al. Huanglongbing alters the structure and functional diversity of microbial communities associated with citrus rhizosphere. ISME J. 6 , 363–383 (2012).
Hamonts, K. et al. Field study reveals core plant microbiota and relative importance of their drivers. Environ. Microbiol. 20 , 124–140 (2018).
Lebreton, L. et al. Temporal dynamics of bacterial and fungal communities during the infection of Brassica rapa roots by the protist Plasmodiophora brassicae . PLoS ONE https://doi.org/10.1371/journal.pone.0204195 (2019).
Blaustein, R. A., Lorca, G. L., Meyer, J. L., Gonzalez, C. F. & Teplitski, M. Defining the core citrus leaf- and root-associated microbiota: factors associated with community structure and implications for managing huanglongbing (citrus greening) disease. Appl. Environ. Microbiol. https://doi.org/10.1128/aem.00210-17 (2017).
Saijo, Y. & Loo, E. P. Plant immunity in signal integration between biotic and abiotic stress responses. N. Phytol. 225 , 87–104 (2020).
Lebeis, S. L. et al. Plant microbiome. Salicylic acid modulates colonization of the root microbiome by specific bacterial taxa. Science 349 , 860–864 (2015).
Janda, M. et al. Temporary heat stress suppresses PAMP-triggered immunity and resistance to bacteria in Arabidopsis thaliana . Mol. Plant. Pathol. 20 , 1005–1012 (2019).
Hacquard, S., Spaepen, S., Garrido-Oter, R. & Schulze-Lefert, P. Interplay between innate immunity and the plant microbiota. Annu. Rev. Phytopathol. 55 , 565–589 (2017). This paper proposes that reciprocal interplays between the host immune system and the microbiota likely shape plant-microbiome assembly and promote microbial homeostasis.
Chen, Q. L. et al. Host identity determines plant associated resistomes. Environ. Pollut. 258 , 113709 (2020).
Gonzalez, R. et al. Plant virus evolution under strong drought conditions results in a transition from parasitism to mutualism. Proc. Natl Acad. Sci. USA https://doi.org/10.1073/pnas.2020990118 (2021).
Suryanarayanan, T. S. & Shaanker, R. U. Can fungal endophytes fast-track plant adaptations to climate change? Fungal Ecol. https://doi.org/10.1016/j.funeco.2021.101039 (2021).
Stringlis, I. A. et al. MYB72-dependent coumarin exudation shapes root microbiome assembly to promote plant health. Proc. Natl Acad. Sci. USA 115 , E5213–E5222 (2018).
Doll, S. et al. Accumulation of the coumarin scopolin under abiotic stress conditions is mediated by the Arabidopsis thaliana THO/TREX complex. Plant J. 93 , 431–444 (2018).
Lekberg, Y. et al. Nitrogen and phosphorus fertilization consistently favor pathogenic over mutualistic fungi in grassland soils. Nat. Commun. 12 , 3484 (2021).
Singh, B. K. & Trivedi, P. Microbiome and the future for food and nutrient security. Microb. Biotechnol. 10 , 50–53 (2017).
Arie, T. Fusarium diseases of cultivated plants, control, diagnosis, and molecular and genetic studies. J. Pestic. Sci. 44 , 275–281 (2019).
Yan, Z., Xiong, C., Liu, H. & Singh, B. K. Sustainable agricultural practices contribute significantly to One Health. J. Sustain. Agric. Env. https://doi.org/10.1002/sae2.12019 (2022).
Edlinger, A. et al. Agricultural management and pesticide use reduce the functioning of beneficial plant symbionts. Nat. Ecol. Evol. 6 , 1145–1154 (2022).
Haas, S. E., Hooten, M. B., Rizzo, D. M. & Meentemeyer, R. K. Forest species diversity reduces disease risk in a generalist plant pathogen invasion. Ecol. Lett. 14 , 1108–1116 (2011). This paper provides empirical evidence that higher plant diversity reduces disease risks by pathogen dilution.
Kirkby, K. A., Lonergan, P. A. & Allen, S. J. Three decades of cotton disease surveys NSW, Australia. Crop. Sci. Pasture Sci. 64 , 774–779 (2013).
Araki, M. & Ishii, T. Towards social acceptance of plant breeding by genome editing. Trends Plant Sci. 20 , 145–149 (2015).
Hickey, L. T. et al. Breeding crops to feed 10 billion. Nat. Biotechnol. 37 , 744–754 (2019).
Qiu, Z., Egidi, E., Liu, H., Kaur, S. & Singh, B. K. New frontiers in agriculture productivity: optimised microbial inoculants and in situ microbiome engineering. Biotechnol. Adv. 37 , 107371 (2019).
Jeger, M. et al. Global challenges facing plant pathology: multidisciplinary approaches to meet the food security and environmental challenges in the mid-twenty-first century. CABI Agric. Bio 2 , 20 (2021). This paper highlights the importance of multidisciplinary approaches to detect, predict, monitor and manage plant diseases.
APS. Phytobiomes: A Roadmap for Research and Translation (American Phytopathological Society, 2016).
Pozo, M. J., Zabalgogeazcoa, I., Vazquez de Aldana, B. R. & Martinez-Medina, A. Untapping the potential of plant mycobiomes for applications in agriculture. Curr. Opin. Plant Biol. 60 , 102034 (2021).
Trivedi, P., Mattupalli, C., Eversole, K. & Leach, J. E. Enabling sustainable agriculture through understanding and enhancement of microbiomes. N. Phytol. 230 , 2129–2147 (2021).
Lamichhane, J. R. & Venturi, V. Synergisms between microbial pathogens in plant disease complexes: a growing trend. Front. Plant Sci. 6 , 00385 (2015).
Garrett, K. A. et al. Climate change effects on pathogen emergence: artificial intelligence to translate big data for mitigation. Annu. Rev. Phytopathol. https://doi.org/10.1146/annurev-phyto-021021-042636 (2022).
Delgado-Baquerizo, M. Simplifying the complexity of the soil microbiome to guide the development of next-generation SynComs. J. Sustain. Agric. Environ. 1 , 9–15 (2022).
Liu, H. et al. Effective colonisation by a bacterial synthetic community promotes plant growth and alters soil microbial community. J. Sustain. Agric. Environ. 1 , 30–42 (2022).
Liu, H. et al. Inner plant values: diversity, colonization and benefits from endophytic bacteria. Front. Microbiol. 8 , 2552 (2017).
Singh, B. K., Trivedi, P., Egidi, E., Macdonald, C. A. & Delgado-Baquerizo, M. Crop microbiome and sustainable agriculture. Nat. Rev. Microbiol. 18 , 601–602 (2020).
Li, J., Wang, J., Liu, H., Macdonald, C. A. & Singh, B. K. Application of microbial inoculants significantly enhances crop productivity: a meta-analysis of studies from 2010 to 2020. J. Sustain. Agric. Environ. 1 , 216–225 (2022).
Matsumoto, H. et al. Bacterial seed endophyte shapes disease resistance in rice. Nat. Plants 7 , 60–72 (2021).
Berg, G. & Raaijmakers, J. M. Saving seed microbiomes. ISME J. 12 , 1167–1170 (2018).
Mendes, L. W., Raaijmakers, J. M., de Hollander, M., Mendes, R. & Tsai, S. M. Influence of resistance breeding in common bean on rhizosphere microbiome composition and function. ISME J. 12 , 212–224 (2018).
de Vries, F. T., Griffiths, R. I., Knight, C. G., Nicolitch, O. & Williams, A. Harnessing rhizosphere microbiomes for drought-resilient crop production. Science 368 , 270–274 (2020). This paper proposes to improve the mechanistic understanding of plant–microbial interactions for development of climate-resilient food production systems.
Rizzo, D. M., Lichtveld, M., Mazet, J. A. K., Togami, E. & Miller, S. A. Plant health and its effects on food safety and security in a One Health framework: four case studies. One Health Outlook 3 , 6 (2021).
Porfirio, L. L., Newth, D., Finnigan, J. J. & Cai, Y. Economic shifts in agricultural production and trade due to climate change. Palgrave Commun. 4 , 111 (2018).
Carvajal-Yepes, M. et al. A global surveillance system for crop diseases. Science 364 , 1237–1239 (2019). This paper calls for a global surveillance system to facilitate quick response to emerging disease outbreaks.
Blumenthal, D. M. Interactions between resource availability and enemy release in plant invasion. Ecol. Lett. 9 , 887–895 (2006).
Mallon, C. A., Elsas, J. D. V. & Salles, J. F. Microbial invasions: the process, patterns, and mechanisms. Trends Microbiol. 23 , 719–729 (2015). This paper suggests that the application of an invasion framework in product evaluation can improve the translation of microbial products, such as biocontrol and probiotic usages.
Feng, Y. et al. Temperature thresholds drive the global distribution of soil fungal decomposers. Glob. Change Biol. 28 , 2779–2789 (2022).
Hurtt, G. C. et al. Harmonization of land-use scenarios for the period 1500–2100: 600 years of global gridded annual land-use transitions, wood harvest, and resulting secondary lands. Clim. Change 109 , 117–161 (2011).
Lawrence, D. M. et al. The Land Use Model Intercomparison Project (LUMIP) contribution to CMIP6: rationale and experimental design. Geosci. Model. Dev. 9 , 2973–2998 (2016).
Dufresne, J. L. et al. Climate change projections using the IPSL-CM5 Earth System Model: from CMIP3 to CMIP5. Clim. Dyn. 40 , 2123–2165 (2013).
Hempel, S., Frieler, K., Warszawski, L., Schewe, J. & Piontek, F. A trend-preserving bias correction—the ISI-MIP approach. Earth Syst. Dyn. 4 , 219–236 (2013).
Kinnunen, M. et al. A conceptual framework for invasion in microbial communities. ISME J. 10 , 2773–2775 (2016).
Bewick, S., Staniczenko, P. P. A., Li, B., Karig, D. K. & Fagan, W. F. Invasion speeds in microbial systems with toxin production and quorum sensing. J. Theor. Biol. 420 , 290–303 (2017).
Costello, E. K., Stagaman, K., Dethlefsen, L., Bohannan, B. J. & Relman, D. A. The application of ecological theory toward an understanding of the human microbiome. Science 336 , 1255–1262 (2012).
Jayathilake, P. G. et al. A mechanistic individual-based model of microbial communities. PLoS ONE 12 , e0181965 (2017).
Daly, A. J. et al. Individual-based modelling of invasion in bioaugmented sand filter communities. Processes https://doi.org/10.3390/pr6010002 (2018).
Liu, X., Wang, M., Nie, Y. & Wu, X.-L. Successful microbial colonization of space in a more dispersed manner. ISME Commun. 1 , 68 (2021).
Deutsch, C. A. et al. Increase in crop losses to insect pests in a warming climate. Science 361 , 916–919 (2018).
Zhang, X. et al. Climate change increases risk of Fusarium ear blight on wheat in central China. Ann. Appl. Biol. 164 , 384–395 (2014).
Duku, C., Sparks, A. H. & Zwart, S. J. Spatial modelling of rice yield losses in Tanzania due to bacterial leaf blight and leaf blast in a changing climate. Clim. Change 135 , 569–583 (2016).
Cunniffe, N. J., Cobb, R. C., Meentemeyer, R. K., Rizzo, D. M. & Gilligan, C. A. Modeling when, where, and how to manage a forest epidemic, motivated by sudden oak death in California. Proc. Natl Acad. Sci. USA 113 , 5640–5645 (2016).
Hyatt-Twynam, S. R. et al. Risk-based management of invading plant disease. N. Phytol. 214 , 1317–1329 (2017).
Thompson, R. N., Gilligan, C. A. & Cunniffe, N. J. Control fast or control smart: when should invading pathogens be controlled? PLoS Comput. Biol. 14 , e1006014 (2018).
Mastin, A. J., Gottwald, T. R., van den Bosch, F., Cunniffe, N. J. & Parnell, S. Optimising risk-based surveillance for early detection of invasive plant pathogens. PLoS Biol. 18 , e3000863 (2020).
Bebber, D. P. et al. Many unreported crop pests and pathogens are probably already present. Glob. Chang. Biol. 25 , 2703–2713 (2019).
Diffenbaugh, N. S. Verification of extreme event attribution: using out-of-sample observations to assess changes in probabilities of unprecedented events. Sci. Adv. 6 , eaay2368 (2020).
Garrett, K. A., Dendy, S. P., Frank, E. E., Rouse, M. N. & Travers, S. E. Climate change effects on plant disease: genomes to ecosystems. Annu. Rev. Phytopathol. 44 , 489–509 (2006).
Botero, D. et al. Genome-scale metabolic model of Xanthomonas phaseoli pv. manihotis : an approach to elucidate pathogenicity at the metabolic level. Front. Genet. 11 , 837 (2020).
Gerlin, L. et al. Genome-scale investigation of the metabolic determinants generating bacterial fastidious growth. mSystems https://doi.org/10.1128/mSystems.00698-19 (2020).
Xu, N., Yang, Q., Yang, X., Wang, M. & Guo, M. Reconstruction and analysis of a genome-scale metabolic model for Agrobacterium tumefaciens . Mol. Plant Pathol. 22 , 348–360 (2021).
Heinken, A., Basile, A., Hertel, J., Thinnes, C. & Thiele, I. Genome-scale metabolic modeling of the human microbiome in the era of personalized medicine. Annu. Rev. Microbiol. 75 , 199–222 (2021).
Kim, M. S., Zhang, H. & Shim, W. B. Application of game theory to explore the dynamics of host−pathogen association in phytobiomes. Phytobiomes J. https://doi.org/10.1094/PBIOMES-04-18-0019-P (2018).
Trivedi, P. et al. Keystone microbial taxa regulate the invasion of a fungal pathogen in agro-ecosystems. Soil. Biol. Biochem. 111 , 10–14 (2017).
Delgado-Baquerizo, M. et al. Multiple elements of soil biodiversity drive ecosystem functions across biomes. Nat. Ecol. Evol. 4 , 210–220 (2020).
Liu, Q. et al. Rhizosphere fungal dynamics in sugarcane during different growth growth stages. Int. J. Mol. Sci. 24 , 5701 (2023).
Garrett, K. A. et al. Network analysis: a systems framework to address grand challenges in plant pathology. Annu. Rev. Phytopathol. 56 , 559–580 (2018). This paper argues that statistical tools have the potential to inform pathogen management.
Skelsey, P., Cooke, D. E., Lynott, J. S. & Lees, A. K. Crop connectivity under climate change: future environmental and geographic risks of potato late blight in Scotland. Glob. Chang. Biol. 22 , 3724–3738 (2016).
Newlands, N. K. Model-based forecasting of agricultural crop disease risk at the regional scale, integrating airborne inoculum, environmental, and satellite-based monitoring data. Front. Environ. Sci. https://doi.org/10.3389/fenvs.2018.00063 (2018).
Picault, S. et al. EMULSION: transparent and flexible multiscale stochastic models in human, animal and plant epidemiology. PLoS Comput. Biol. 15 , e1007342 (2019).
Zou, Y. et al. Nucleic acid purification from plants, animals and microbes in under 30 seconds. PLoS Biol. 15 , e2003916 (2017).
Paul, R., Ostermann, E., Gu, Z., Ristaino, J. B. & Wei, Q. DNA extraction from plant leaves using a microneedle patch. Curr. Protoc. Plant Biol. 5 , e20104 (2020).
Xu, H. et al. An ultraportable and versatile point-of-care DNA testing platform. Sci. Adv. 6 , eaaz7445 (2020).
Almogy, G. et al. Cost-efficient whole genome-sequencing using novel mostly natural sequencing-by-synthesis chemistry and open fluidics platform. Preprint at bioRxiv https://doi.org/10.1101/2022.05.29.493900 (2022).
Zarco-Tejada, P. J. et al. Divergent abiotic spectral pathways unravel pathogen stress signals across species. Nat. Commun. 12 , 6088 (2021).
Li, Z. et al. Non-invasive plant disease diagnostics enabled by smartphone-based fingerprinting of leaf volatiles. Nat. Plants 5 , 856–866 (2019).
McNish, I. G. & Smith, K. P. Oat crown rust disease severity estimated at many time points using multispectral aerial photos. Phytopathology 112 , 682–690 (2022).
Tanner, F. et al. Sensor-based phenotyping of above-ground plant–pathogen interactions. Plant Methods 18 , 35 (2022).
Schmale, D. G. III & Ross, S. D. Highways in the sky: scales of atmospheric transport of plant pathogens. Annu. Rev. Phytopathol. 53 , 591–611 (2015).
Vélez-Rodríguez, Z., Torres-Pratts, H. & Maldonado-Ramírez, S. L. Use of drones to recover fungal spores and pollen from the lower atmosphere. Caribb. J. Sci. 50 , 159–170 (2020).
O’Shea, J. Digital disease detection: a systematic review of event-based internet biosurveillance systems. Int. J. Med. Inf. 101 , 15–22 (2017).
Guerra, C. A. et al. Tracking, targeting, and conserving soil biodiversity. Science 371 , 239–241 (2021).
Download references
Acknowledgements
B.K.S. acknowledges funding from the Australian Research Council (DP210102081; DP230101448) for microbiome research. E.E. is supported by an Australian Research Council fellowship (DE210101822). E.G. acknowledges funding from Generalitat Valenciana and a European Social Fund grant (APOSTD/2021/188). M.D.-B. is supported by the Spanish Ministry of Science and Innovation (PID202-115813RA-100). P.T. and J.E.L.’s research is supported by the US National Science Foundation (no. 2120117). J.E.L. receives additional funding from the Foundation of Food and Agriculture (ICRC20-0000000084).
Author information
Authors and affiliations.
Hawkesbury Institute for the Environment, Western Sydney University, Penrith, New South Wales, Australia
Brajesh K. Singh, Eleonora Egidi & Hongwei Liu
Global Centre for Land-Based Innovation, Western Sydney University, Penrith, New South Wales, Australia
Brajesh K. Singh
Laboratorio de Biodiversidad y Funcionamiento Ecosistémico, Instituto de Recursos Naturales y Agrobiología de Sevilla (IRNAS), CSIC, Sevilla, Spain
Manuel Delgado-Baquerizo
Unidad Asociada CSIC-UPO (BioFun), Universidad Pablo de Olavide, Sevilla, Spain
Multidisciplinary Institute for Environment Studies ‘Ramon Margalef’, University of Alicante, Alicante, Spain
Emilio Guirado
Microbiome Newtork and Department of Agricultural Biology, Colorado State University, Fort Collins, CO, USA
Jan E. Leach & Pankaj Trivedi
You can also search for this author in PubMed Google Scholar
Contributions
All authors contributed equally to all aspects of the manuscript.
Corresponding author
Correspondence to Brajesh K. Singh .
Ethics declarations
Competing interests.
The authors declare no competing interests.
Peer review
Peer review information.
Nature Reviews Microbiology thanks Gabriele Berg, Hang-Wei Hu, Marcel van der Heijden and the other, anonymous, reviewer(s) for their contribution to the peer review of this work.
Additional information
Publisher’s note Springer Nature remains neutral with regard to jurisdictional claims in published maps and institutional affiliations.
Related links
Ash dieback: https://www.woodlandtrust.org.uk/trees-woods-and-wildlife/tree-pests-and-diseases/key-tree-pests-and-diseases/ash-dieback/
Supplementary information
Supplementary information, rights and permissions.
Springer Nature or its licensor (e.g. a society or other partner) holds exclusive rights to this article under a publishing agreement with the author(s) or other rightsholder(s); author self-archiving of the accepted manuscript version of this article is solely governed by the terms of such publishing agreement and applicable law.
Reprints and permissions
About this article
Cite this article.
Singh, B.K., Delgado-Baquerizo, M., Egidi, E. et al. Climate change impacts on plant pathogens, food security and paths forward. Nat Rev Microbiol 21 , 640–656 (2023). https://doi.org/10.1038/s41579-023-00900-7
Download citation
Accepted : 11 April 2023
Published : 02 May 2023
Issue Date : October 2023
DOI : https://doi.org/10.1038/s41579-023-00900-7
Share this article
Anyone you share the following link with will be able to read this content:
Sorry, a shareable link is not currently available for this article.
Provided by the Springer Nature SharedIt content-sharing initiative
This article is cited by
Comparative mineral and biochemical characterization of citrus reticulata fruits and leaves to citrus canker pathogens, xanthomonas axonopodis.
- Abdul Ghani
- Ihab Mohamed Moussa
BMC Plant Biology (2024)
Pine wilt disease: what do we know from proteomics?
- Joana M. S. Cardoso
- Bruno Manadas
- Luís Fonseca
The Egyptian wheat cultivar Gemmeiza-12 is a source of resistance against the fungus Zymoseptoria tritici
- Abdelrahman M Qutb
- Florence Cambon
- Graeme J Kettles
Sulfonate derivatives bearing an amide unit: design, synthesis and biological activity studies
- You-hua Liu
- Chang-kun Li
BMC Chemistry (2024)
The buzz about honey-based biosurveys
- Paton Vuong
- Anna Poppy Griffiths
- Parwinder Kaur
npj Biodiversity (2024)
Quick links
- Explore articles by subject
- Guide to authors
- Editorial policies
Sign up for the Nature Briefing: Microbiology newsletter — what matters in microbiology research, free to your inbox weekly.

Plant Disease Detection and Classification by Deep Learning—A Review
Ieee account.
- Change Username/Password
- Update Address
Purchase Details
- Payment Options
- Order History
- View Purchased Documents
Profile Information
- Communications Preferences
- Profession and Education
- Technical Interests
- US & Canada: +1 800 678 4333
- Worldwide: +1 732 981 0060
- Contact & Support
- About IEEE Xplore
- Accessibility
- Terms of Use
- Nondiscrimination Policy
- Privacy & Opting Out of Cookies
A not-for-profit organization, IEEE is the world's largest technical professional organization dedicated to advancing technology for the benefit of humanity. © Copyright 2024 IEEE - All rights reserved. Use of this web site signifies your agreement to the terms and conditions.
- Open access
- Published: 24 February 2021
Plant diseases and pests detection based on deep learning: a review
- Jun Liu ORCID: orcid.org/0000-0001-8769-5981 1 &
- Xuewei Wang 1
Plant Methods volume 17 , Article number: 22 ( 2021 ) Cite this article
127k Accesses
333 Citations
17 Altmetric
Metrics details
Plant diseases and pests are important factors determining the yield and quality of plants. Plant diseases and pests identification can be carried out by means of digital image processing. In recent years, deep learning has made breakthroughs in the field of digital image processing, far superior to traditional methods. How to use deep learning technology to study plant diseases and pests identification has become a research issue of great concern to researchers. This review provides a definition of plant diseases and pests detection problem, puts forward a comparison with traditional plant diseases and pests detection methods. According to the difference of network structure, this study outlines the research on plant diseases and pests detection based on deep learning in recent years from three aspects of classification network, detection network and segmentation network, and the advantages and disadvantages of each method are summarized. Common datasets are introduced, and the performance of existing studies is compared. On this basis, this study discusses possible challenges in practical applications of plant diseases and pests detection based on deep learning. In addition, possible solutions and research ideas are proposed for the challenges, and several suggestions are given. Finally, this study gives the analysis and prospect of the future trend of plant diseases and pests detection based on deep learning.
Plant diseases and pests detection is a very important research content in the field of machine vision. It is a technology that uses machine vision equipment to acquire images to judge whether there are diseases and pests in the collected plant images [ 1 ]. At present, machine vision-based plant diseases and pests detection equipment has been initially applied in agriculture and has replaced the traditional naked eye identification to some extent.
For traditional machine vision-based plant diseases and pests detection method, conventional image processing algorithms or manual design of features plus classifiers are often used [ 2 ]. This kind of method usually makes use of the different properties of plant diseases and pests to design the imaging scheme and chooses appropriate light source and shooting angle, which is helpful to obtain images with uniform illumination. Although carefully constructed imaging schemes can greatly reduce the difficulty of classical algorithm design, but also increase the application cost. At the same time, under natural environment, it is often unrealistic to expect the classical algorithms designed to completely eliminate the impact of scene changes on the recognition results [ 3 ]. In real complex natural environment, plant diseases and pests detection is faced with many challenges, such as small difference between the lesion area and the background, low contrast, large variations in the scale of the lesion area and various types, and a lot of noise in the lesion image. Also, there are a lot of disturbances when collecting plant diseases and pests images under natural light conditions. At this time, the traditional classical methods often appear helpless, and it is difficult to achieve better detection results.
In recent years, with the successful application of deep learning model represented by convolutional neural network (CNN) in many fields of computer vision (CV, computer-vision), for example, traffic detection [ 4 ], medical Image Recognition [ 5 ], Scenario text detection [ 6 ], expression recognition [ 7 ], face Recognition [ 8 ], etc. Several plant diseases and pests detection methods based on deep learning are applied in real agricultural practice, and some domestic and foreign companies have developed a variety of deep learning-based plant diseases and pests detection Wechat applet and photo recognition APP software. Therefore, plant diseases and pests detection method based on deep learning not only has important academic research value, but also has a very broad market application prospect.
In view of the lack of comprehensive and detailed discussion on plant diseases and pests detection methods based on deep learning, this study summarizes and combs the relevant literatures from 2014 to 2020, aiming to help researchers quickly and systematically understand the relevant methods and technologies in this field. The content of this study is arranged as follows: “ Definition of plant diseases and pests detection problem ” section gives the definition of plant diseases and pests detection problem; “ Image recognition technology based on deep learning ” section focuses on the detailed introduction of image recognition technology based on deep learning; “ Plant diseases and pests detection methods based on deep learning ” section analyses the three kinds of plant diseases and pests detection methods based on deep learning according to network structure, including classification, detection and segmentation network; “ Dataset and performance comparison ” section introduces some datasets of plant diseases and pests detection and compares the performance of the existing studies; “ Challenges ” section puts forward the challenges of plant diseases and pests detection based on deep learning; “ Conclusions and future directions ” section prospects the possible research focus and development direction in the future.
Definition of plant diseases and pests detection problem
Definition of plant diseases and pests
Plant diseases and pests is one kind of natural disasters that affect the normal growth of plants and even cause plant death during the whole growth process of plants from seed development to seedling and to seedling growth. In machine vision tasks, plant diseases and pests tend to be the concepts of human experience rather than a purely mathematical definition.
Definition of plant diseases and pests detection
Compared with the definite classification, detection and segmentation tasks in computer vision [ 9 ], the requirements of plant diseases and pests detection is very general. In fact, its requirements can be divided into three different levels: what, where and how [ 10 ]. In the first stage, “what” corresponds to the classification task in computer vision. As shown in Fig. 1 , the label of the category to which it belongs is given. The task in this stage can be called classification and only gives the category information of the image. In the second stage, “where” corresponds to the location task in computer vision, and the positioning of this stage is the rigorous sense of detection. This stage not only acquires what types of diseases and pests exist in the image, but also gives their specific locations. As shown in Fig. 1 , the plaque area of gray mold is marked with a rectangular box. In the third stage, “how” corresponds to the segmentation task in computer vision. As shown in Fig. 1 , the lesions of gray mold are separated from the background pixel by pixel, and a series of information such as the length, area, location of the lesions of gray mold can be further obtained, which can assist the higher-level severity level evaluation of plant diseases and pests. Classification describes the image globally through feature expression, and then determines whether there is a certain kind of object in the image by means of classification operation; while object detection focuses on local description, that is, answering what object exists in what position in an image, so in addition to feature expression, object structure is the most obvious feature that object detection differs from object classification. That is, feature expression is the main research line of object classification, while structure learning is the research focus of object detection. Although the function requirements and objectives of the three stages of plant diseases and pests detection are different, yet in fact, the three stages are mutually inclusive and can be converted. For example, the “where” in the second stage contains the process of “what” in the first stage, and the “how” in the third stage can finish the task of “where” in the second stage. Also, the “what” in the first stage can achieve the goal of the second and the third stages through some methods. Therefore, the problem in this study is collectively referred to as plant diseases and pests detection as conventions in the following text, and the terminology differentiates only when different network structures and functions are adopted.
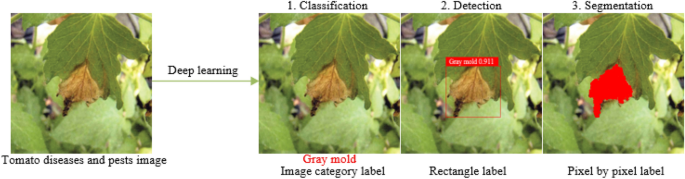
Comparison with traditional plant diseases and pests detection methods
To better illustrate the characteristics of plant diseases and pests detection methods based on deep learning, according to existing references [ 11 , 12 , 13 , 14 , 15 ], a comparison with traditional plant diseases and pests detection methods is given from four aspects including essence, method, required conditions and applicable scenarios. Detailed comparison results are shown in Table 1 .
Image recognition technology based on deep learning
Compared with other image recognition methods, the image recognition technology based on deep learning does not need to extract specific features, and only through iterative learning can find appropriate features, which can acquire global and contextual features of images, and has strong robustness and higher recognition accuracy.
Deep learning theory
The concept of Deep Learning (DL) originated from a paper published in Science by Hinton et al. [ 16 ] in 2006. The basic idea of deep learning is: using neural network for data analysis and feature learning, data features are extracted by multiple hidden layers, each hidden layer can be regarded as a perceptron, the perceptron is used to extract low-level features, and then combine low-level features to obtain abstract high-level features, which can significantly alleviate the problem of local minimum. Deep learning overcomes the disadvantage that traditional algorithms rely on artificially designed features and has attracted more and more researchers’ attention. It has now been successfully applied in computer vision, pattern recognition, speech recognition, natural language processing and recommendation systems [ 17 ].
Traditional image classification and recognition methods of manual design features can only extract the underlying features, and it is difficult to extract the deep and complex image feature information [ 18 ]. And deep learning method can solve this bottleneck. It can directly conduct unsupervised learning from the original image to obtain multi-level image feature information such as low-level features, intermediate features and high-level semantic features. Traditional plant diseases and pests detection algorithms mainly adopt the image recognition method of manual designed features, which is difficult and depends on experience and luck, and cannot automatically learn and extract features from the original image. On the contrary, deep learning can automatically learn features from large data without manual manipulation. The model is composed of multiple layers, which has good autonomous learning ability and feature expression ability, and can automatically extract image features for image classification and recognition. Therefore, deep learning can play a great role in the field of plant diseases and pests image recognition. At present, deep learning methods have developed many well-known deep neural network models, including deep belief network (DBN), deep Boltzmann machine (DBM), stack de-noising autoencoder (SDAE) and deep convolutional neural network (CNN) [ 19 ]. In the area of image recognition, the use of these deep neural network models to realize automate feature extraction from high-dimensional feature space offers significant advantages over traditional manual design feature extraction methods. In addition, as the number of training samples grows and the computational power increases, the characterization power of deep neural networks is being further improved. Nowadays, the boom of deep learning is sweeping both industry and academia, and the performance of deep neural network models are all significantly ahead of traditional models. In recent years, the most popular deep learning framework is deep convolutional neural network.
- Convolutional neural network
Convolutional Neural Networks, abbreviated as CNN, has a complex network structure and can perform convolution operations. As shown in Fig. 2 , the convolutional neural network model is composed of input layer, convolution layer, pooling layer, full connection layer and output layer. In one model, the convolution layer and the pooling layer alternate several times, and when the neurons of the convolution layer are connected to the neurons of the pooling layer, no full connection is required. CNN is a popular model in the field of deep learning. The reason lies in the huge model capacity and complex information brought about by the basic structural characteristics of CNN, which enables CNN to play an advantage in image recognition. At the same time, the successes of CNN in computer vision tasks have boosted the growing popularity of deep learning.
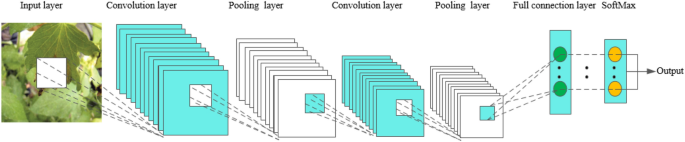
The basic structure of CNN
In the convolution layer, a convolution core is defined first. The convolution core can be considered as a local receptive field, and the local receptive field is the greatest advantage of the convolution neural network. When processing data information, the convolution core slides on the feature map to extract part of the feature information. After the feature extraction of the convolution layer, the neurons are input into the pooling layer to extract the feature again. At present, the commonly used methods of pooling include calculating the mean, maximum and random values of all values in the local receptive field [ 20 , 21 ]. After the data entering several convolution layers and pooling layers, they enter the full-connection layer, and the neurons in the full-connection layer are fully connected with the neurons in the upper layer. Finally, the data in the full-connection layer can be classified by the softmax method, and then the values are transmitted to the output layer for output results.
Open source tools for deep learning
The commonly used third-party open source tools for deep learning are Tensorflow [ 22 ], Torch/PyTorch [ 23 ], Caffe [ 24 ], Theano [ 25 ]. The different characteristics of each open source tool are shown in Table 2 .
The four commonly used deep learning third-party open source tools all support cross-platform operation, and the platforms that can be run include Linux, Windows, iOS, Android, etc. Torch/PyTorch and Tensorflow have good scalability and support a large number of third-party libraries and deep network structures, and have the fastest training speed when training large CNN networks on GPU.
Plant diseases and pests detection methods based on deep learning
This section gives a summary overview of plant diseases and pests detection methods based on deep learning. Since the goal achieved is completely consistent with the computer vision task, plant diseases and pests detection methods based on deep learning can be seen as an application of relevant classical networks in the field of agriculture. As shown in Fig. 3 , the network can be further subdivided into classification network, detection network and segmentation network according to the different network structures. As can be seen from Fig. 3 , this paper is subdivided into several different sub-methods according to the processing characteristics of each type of methods.
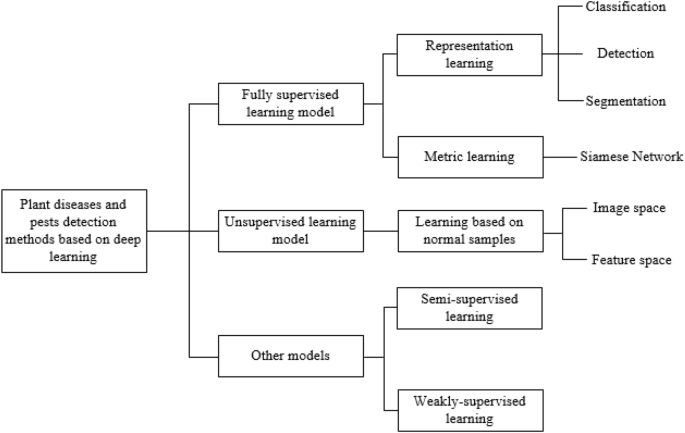
Framework of plant diseases and pests detection methods based on deep learning
Classification network
In real natural environment, the great differences in shape, size, texture, color, background, layout and imaging illumination of plant diseases and pests make the recognition a difficult task. Due to the strong feature extraction capability of CNN, the adoption of CNN-based classification network has become the most commonly used pattern in plant diseases and pests classification. Generally, the feature extraction part of CNN classification network consists of cascaded convolution layer + pooling layer, followed by full connection layer (or average pooling layer) + softmax structure for classification. Existing plant diseases and pests classification network mostly use the muture network structures in computer vision, including AlexNet [ 26 ], GoogleLeNet [ 27 ], VGGNet [ 28 ], ResNet [ 29 ], Inception V4 [ 30 ], DenseNets [ 31 ], MobileNet [ 32 ] and SqueezeNet [ 33 ]. There are also some studies which have designed network structures based on practical problems [ 34 , 35 , 36 , 37 ]. By inputting a test image into the classification network, the network analyses the input image and returns a label that classifies the image. According to the difference of tasks achieved by the classification network method, it can be subdivided into three subcategories: using the network as a feature extractor, using the network for classification directly and using the network for lesions location.
Using network as feature extractor
In the early studies on plant diseases and pests classification methods based on deep learning, many researchers took advantage of the powerful feature extraction capability of CNN, and the methods were combined with traditional classifiers [ 38 ]. First, the images are input into a pretrained CNN network to obtain image characterization features, and the acquired features are then input into a conventional machine learning classifier (e.g., SVM) for classification. Yalcin et al. [ 39 ] proposed a convolutional neural network architecture to extract the features of images while performing experiments using SVM classifiers with different kernels and feature descriptors such as LBP and GIST, the experimental results confirmed the effectiveness of the approach. Fuentes et al. [ 40 ] put forward the idea of CNN based meta architecture with different feature extractors, and the input images included healthy and infected plants, which were identified as their respective classes after going through the meta architecture. Hasan et al. [ 41 ] identified and classified nine different types of rice diseases by using the features extracted from DCNN model and input into SVM, and the accuracy achieved 97.5%.
Using network for classification directly
Directly using classification network to classify lesions is the earliest common means of CNN applied in plant diseases and pests detection. According to the characteristics of existing research work, it can be further subdivided into original image classification, classification after locating Region of Interest (ROI) and multi-category classification.
Original image classification. That is, directly put the collected complete plant diseases and pests image into the network for learning and training. Thenmozhi et al. [ 42 ] proposed an effective deep CNN model, and transfer learning is used to fine-tune the pre-training model. Insect species were classified on three public insect datasets with accuracy of 96.75%, 97.47% and 95.97%, respectively. Fang et al. [ 43 ] used ResNet50 in plant diseases and pests detection. The focus loss function was used instead of the standard cross-entropy loss function, and the Adam optimization method was used to identify the leaf disease grade, and the accuracy achieved 95.61%.
Classification after locating ROI. For the whole image acquired, we should focus on whether there is a lesion in a fixed area, so we often obtain the region of interest (ROI) in advance, and then input the ROI into the network to judge the category of diseases and pests. Nagasubramanian et al. [ 44 ] used a new three-dimensional deep convolution neural network (DCNN) and salience map visualization method to identify healthy and infected samples of soybean stem rot, and the classification accuracy achieved 95.73%.
Multi-category classification. When the number of plant diseases and pests class to be classified exceed 2 class, the conventional plant diseases and pests classification network is the same as the original image classification method, that is, the output nodes of the network are the number of plant diseases and pests class + 1 (including normal class). However, multi-category classification methods often use a basic network to classify lesions and normal samples, and then share feature extraction parts on the same network to modify or increase the classification branches of lesion categories. This approach is equivalent to preparing a pre-training weight parameter for subsequent multi-objective plant diseases and pests classification network, which is obtained by binary training between normal samples and plant diseases and pests samples. Picon et al. [ 45 ] proposed a CNN architecture to identify 17 diseases in 5 crops, which seamlessly integrates context metadata, allowing training of a single multi-crop model. The model can achieve the following goals: (a) obtains richer and more robust shared visual features than the corresponding single crop; (b) is not affected by different diseases in which different crops have similar symptoms; (c) seamlessly integrates context to perform crop conditional disease classification. Experiments show that the proposed model alleviates the problem of data imbalance, and the average balanced accuracy is 0.98, which is superior to other methods and eliminates 71% of classifier errors.
Using network for lesions location
Generally, the classification network can only complete the classification of image label level. In fact, it can also achieve the location of lesions and the pixel-by-pixel classification by combining different techniques and methods. According to the different means used, it can be further divided into three forms: sliding window, heatmap and multi-task learning network.
Sliding window. This is the simplest and intuitive method to achieve the location of lesion coarsely. The image in the sliding window is input into the classification network for plant diseases and pests detection by redundant sliding on the original image through a smaller size window. Finally, all sliding windows are connected to obtain the results of the location of lesion. Chen et al. [ 46 ] used CNN classification network based on sliding window to build a framework for characteristics automatic learning, feature fusion, recognition and location regression calculation of plant diseases and pests species, and the recognition rate of 38 common symptoms in the field was 50–90%.
Heatmap. This is an image that reflects the importance of each region in the image, the darker the color represents the more important. In the field of plant diseases and pests detection, the darker the color in the heatmap represents the greater the probability that it is the lesion. In 2017, Dechant et al. [ 47 ] trained CNN to make heatmap to show the probability of infection in each region in maize disease images, and these heatmaps were used to classify the complete images, dividing each image into containing or not containing infected leaves. At runtime, it takes about 2 min to generate a heatmap for an image (1.6 GB of memory) and less than one second to classify a set of three heatmaps (800 MB of memory). Experiments show that the accuracy is 96.7% on the test dataset. In 2019, Wiesner-Hanks et al. [ 48 ] used heatmap method to obtain accurate contour areas of maize diseases, the model can accurately depict lesions as low as millimeter scale from the images collected by UAVs, with an accuracy rate of 99.79%, which is the best scale of aerial plant disease detection achieved so far.
Multi-task learning network. If the pure classified network does not add any other skills, it could only realize the image level classification. Therefore, to accurately locate the location of plant diseases and pests, the designed network should often add an extra branch, and the two branches would share the results of the feature extracting. In this way, the network generally had the classification and segmentation output of the plant diseases and pests, forming a multi-task learning network. It takes into account the characteristics of both network. For segmentation network branches, each pixel in the image can be used as a training sample to train the network. Therefore, the multi-task learning network not only uses the segmentation branches to output the specific segmentation results of the lesions, but also greatly reduces the requirements of the classification network for samples. Ren et al. [ 49 ] constructed a Deconvolution-Guided VGNet (DGVGNet) model to identify plant leaf diseases which were easily disturbed by shadows, occlusions and light intensity. The deconvolution was used to guide the CNN classifier to focus on the real lesion sites. The test results show that the accuracy of disease class identification is 99.19%, the pixel accuracy of lesion segmentation is 94.66%, and the model has good robustness in occlusion, low light and other environments.
To sum up, the method based on classification network is widely used in practice, and many scholars have carried out application research on the classification of plant diseases and pests [ 50 , 51 , 52 , 53 ]. At the same time, different sub-methods have their own advantages and disadvantages, as shown in Table 3 .
Detection network
Object positioning is one of the most basic tasks in the field of computer vision. It is also the closest task to plant diseases and pests detections in the traditional sense. Its purpose is to obtain accurate location and category information of the object. At present, object detection methods based on deep learning emerge endlessly. Generally speaking, plant diseases and pests detection network based on deep learning can be divided into: two stage network represented by Faster R-CNN [ 54 ]; one stage network represented by SSD [ 55 ] and YOLO [ 56 , 57 , 58 ]. The main difference between the two networks is that the two-stage network needs to first generate a candidate box (proposal) that may contain the lesions, and then further execute the object detection process. In contrast, the one-stage network directly uses the features extracted in the network to predict the location and class of the lesions.
Plant diseases and pests detection based on two stages network
The basic process of two-stage detection network (Faster R-CNN) is to obtain the feature map of the input image through the backbone network first, then calculate the anchor box confidence using RPN and get the proposal. Then, input the feature map of the proposal area after ROIpooling to the network, fine-tune the initial detection results, and finally get the location and classification results of the lesions. Therefore, according to the characteristics of plant diseases and pests detection, common methods often improve on the backbone structure or its feature map, anchor ratio, ROIpooling and loss function. In 2017, Fuentes et al. [ 59 ] first used Faster R-CNN to locate tomato diseases and pests directly, combined with deep feature extractors such as VGG-Net and ResNet, the mAP value reached 85.98% in a dataset containing 5000 tomato diseases and pests of 9 categories. In 2019, Ozguven et al. [ 60 ] proposed a Faster R-CNN structure for automatic detection of beet leaf spot disease by changing the parameters of CNN model. 155 images were trained and tested. The results show that the overall correct classification rate of this method is 95.48%. Zhou et al. [ 61 ] presented a fast rice disease detection method based on the fusion of FCM-KM and Faster R-CNN. The application results of 3010 images showed that: the detection accuracy and time of rice blast, bacterial blight, and sheath blight are 96.71%/0.65 s, 97.53%/0.82 s and 98.26%/0.53 s respectively. Xie et al. [ 62 ] proposed a Faster DR-IACNN model based on the self-built grape leaf disease dataset (GLDD) and Faster R-CNN detection algorithm, the Inception-v1 module, Inception-ResNet-v2 module and SE are introduced. The proposed model achieved higher feature extraction ability, the mAP accuracy was 81.1% and the detection speed was 15.01FPS. The two-stage detection network has been devoted to improving the detection speed to improve the real-time and practicability of the detection system, but compared with the single-stage detection network, it is still not concise enough, and the inference speed is still not fast enough.
Plant diseases and pests detection based on one stage network
The one-stage object detection algorithm has eliminated the region proposal stage, but directly adds the detection head to the backbone network for classification and regression, thus greatly improving the inference speed of the detection network. The single-stage detection network is divided into two types, SSD and YOLO, both of which use the whole image as the input of the network, and directly return the position of the bounding box and the category to which it belongs at the output layer.
Compared with the traditional convolutional neural network, the SSD selects VGG16 as the trunk of the network, and adds a feature pyramid network to obtain features from different layers and make predictions. Singh et al. [ 63 ] built the PlantDoc dataset for plant disease detection. Considering that the application should predict in mobile CPU in real time, an application based on MobileNets and SSD was established to simplify the detection of model parameters. Sun et al. [ 64 ] presented an instance detection method of multi-scale feature fusion based on convolutional neural network, which is improved on the basis of SSD to detect maize leaf blight under complex background. The proposed method combined data preprocessing, feature fusion, feature sharing, disease detection and other steps. The mAP of the new model is higher (from 71.80 to 91.83%) than that of the original SSD model. The FPS of the new model has also improved (from 24 to 28.4), reaching the standard of real-time detection.
YOLO considers the detection task as a regression problem, and uses global information to directly predict the bounding box and category of the object to achieve end-to-end detection of a single CNN network. YOLO can achieve global optimization and greatly improve the detection speed while satisfying higher accuracy. Prakruti et al. [ 65 ] presented a method to detect pests and diseases on images captured under uncontrolled conditions in tea gardens. YOLOv3 was used to detect pests and diseases. While ensuring real-time availability of the system, about 86% mAP was achieved with 50% IOU. Zhang et al. [ 66 ] combined the pooling of spatial pyramids with the improved YOLOv3, deconvolution is implemented by using the combination of up-sampling and convolution operation, which enables the algorithm to effectively detect small size crop pest samples in the image and reduces the problem of relatively low recognition accuracy due to the diversity of crop pest attitudes and scales. The average recognition accuracy can reach 88.07% by testing 20 class of pests collected in real scene.
In addition, there are many studies on using detection network to identify diseases and pests [ 47 , 67 , 68 , 69 , 70 , 71 , 72 , 73 ]. With the development of object detection network in computer vision, it is believed that more and more new detection models will be applied in plant diseases and pests detection in the future. In summary, in the field of plant diseases and pests detection which emphasizes detection accuracy at this stage, more models based on two-stage are used, and in the field of plant diseases and pests detection which pursue detection speed more models based on one-stage are used.
Can detection network replace classification network? The task of detection network is to solve the location problem of plant diseases and pests. The task of classification network is to judge the class of plant diseases and pests. Visually, the hidden information of detection network includes the category information, that is, the category information of plant diseases and pests that need to be located needs to be known beforehand, and the corresponding annotation information should be given in advance to judge the location of plant diseases and pests. From this point of view, the detection network seems to include the steps of the classification network, that is, the detection network can answer “what kind of plant diseases and pests are in what place”. But there is a misconception, in which “what kind of plant diseases and pests” is given a priori, that is, what is labelled during training is not necessarily the real result. In the case of strong model differentiation, that is, when the detection network can give accurate results, the detection network can answer “what kind of plant diseases and pests are in what place” to a certain extent. However, in the real world, in many cases, it cannot uniquely reflect the uniqueness of plant diseases and pests categories, only can answer “what kind of plant diseases and pests may be in what place”, then the involvement of the classification network is necessary. Thus, the detection network cannot replace the classification network.
Segmentation network
Segmentation network converts the plant diseases and pests detection task to semantic and even instance segmentation of lesions and normal areas. It not only finely divides the lesion area, but also obtains the location, category and corresponding geometric properties (including length, width, area, outline, center, etc.). It can be roughly divided into: Fully Convolutional Networks (FCN) [ 74 ] and Mask R-CNN [ 75 ].
Full convolution neural network (FCN) is the basis of image semantics segmentation. At present, almost all semantics segmentation models are based on FCN. FCN first extracts and codes the features of the input image using convolution, then gradually restores the feature image to the size of the input image by deconvolution or up sampling. Based on the differences in FCN network structure, the plant diseases and pests segmentation methods can be divided into conventional FCN, U-net [ 76 ] and SegNet [ 77 ].
Conventional FCN. Wang et al. [ 78 ] presented a new method of maize leaf disease segmentation based on full convolution neural network to solve the problem that traditional computer vision is susceptible to different illumination and complex background, and the segmentation accuracy reached 96.26. Wang et al. [ 79 ] proposed a plant diseases and pests segmentation method based on improved FCN. In this method, a convolution layer was used to extract multi-layer feature information from the input maize leaf lesion image, and the size and resolution of the input image were restored by deconvolution operation. Compared with the original FCN method, not only the integrity of the lesion was guaranteed, but also the segmentation of small lesion area was highlighted, and the accuracy rate reached 95.87%.
U-net. U-net is not only a classical FCN structure, but also a typical encoder-decoder structure. It is characterized by introducing a layer-hopping connection, fusing the feature map in the coding stage with that in the decoding stage, which is beneficial to the recovery of segmentation details. Lin et al. [ 80 ] used U-net based convolutional neural network to segment 50 cucumber powdery mildew leaves collected in natural environment. Compared with the original U-net, a batch normalization layer was added behind each convolution layer, making the neural network insensitive to weight initialization. The experiment shows that the convolutional neural network based on U-net can accurately segment powdery mildew on cucumber leaves at the pixel level with an average pixel accuracy of 96.08%, which is superior to the existing K-means, Random-forest and GBDT methods. The U-net method can segment the lesion area in a complex background, and still has good segmentation accuracy and segmentation speed with fewer samples.
SegNet. It is also a classical encoder–decoder structure. Its feature is that the up-sampling operation in the decoder takes advantage of the index of the largest pooling operation in the encoder. Kerkech et al. [ 81 ] presented an image segmentation method for unmanned aerial vehicles. Visible and infrared images (480 samples from each range) were segmented using SegNet to identify four categories: shadows, ground, healthy and symptomatic grape vines. The detection rates of the proposed method on grape vines and leaves were 92% and 87%, respectively.
Mask R-CNN is one of the most commonly used image instance segmentation methods at present. It can be considered as a multitask learning method based on detection and segmentation network. When multiple lesions of the same type have adhesion or overlap, instance segmentation can separate individual lesions and further count the number of lesions. However, semantic segmentation often treats multiple lesions of the same type as a whole. Stewart et al. [ 82 ] trained a Mask R-CNN model to segment maize northern leaf blight (NLB) lesions in an unmanned aerial vehicle image. The trained model can accurately detect and segment a single lesion. At the IOU threshold of 0.50, the IOU between the baseline true value and the predicted lesion was 0.73, and the average accuracy was 0.96. Also, some studies combine the Mask R-CNN framework with object detection networks for plant diseases and pests detection. Wang et al. [ 83 ] used two different models, Faster R-CNN and ask R-CNN, in which Faster R-CNN was used to identify the class of tomato diseases and Mask R-CNN was used to detect and segment the location and shape of the infected area. The results showed that the proposed model can quickly and accurately identify 11 class of tomato diseases, and divide the location and shape of infected areas. Mask R-CNN reached a high detection rate of 99.64% for all class of tomato diseases.
Compared with the classification and detection network methods, the segmentation method has advantages in obtaining the lesion information. However, like the detection network, it requires a lot of annotation data, and its annotation information is pixel by pixel, which often takes a lot of effort and cost.
Dataset and performance comparison
This section first gives a brief introduction to the plant diseases and pests related datasets and the evaluation index of deep learning model, then compares and analyses the related models of plant diseases and pests detection based on deep learning in recent years.
Datasets for plant diseases and pests detection
Plant diseases and pests detection datasets are the basis for research work. Compared with ImageNet, PASCAL-VOC2007/2012 and COCO in computer vision tasks, there is not a large and unified dataset for plant diseases and pests detection. The plant diseases and pests dataset can be acquired by self-collection, network collection and use of public datasets. Among them, self-collection of image dataset is often obtained by unmanned aerial remote sensing, ground camera photography, Internet of Things monitoring video or video recording, aerial photography of unmanned aerial vehicle with camera, hyperspectral imager, near-infrared spectrometer, and so on. Public datasets typically come from PlantVillage, an existing well-known public standard library. Relatively, self-collected datasets of plant diseases and pests in real natural environment are more practical. Although more and more researchers have opened up the images collected in the field, it is difficult to compare them uniformly based on different class of diseases under different detection objects and scenarios. This section provides links to a variety of plant diseases and pests detection datasets in conjunction with existing studies. As shown in Table 4 .
Evaluation indices
Evaluation indices can vary depending on the focus of the study. Common evaluation indices include \(Precision\) , \(Recall\) , mean Average Precision (mAP) and the harmonic Mean F1 score based on \(Precision\) and \(Recall\) .
\(Precision\) and \(Recall\) are defined as:
In Formula ( 1 ) and Formula ( 2 ), TP (True Positive) is true-positive, predicted to be 1 and actually 1, indicating the number of lesions correctly identified by the algorithm. FP (False Positive) is false-positive, predicted to be 1 and actually 0, indicating the number of lesions incorrectly identified by the algorithm. FN (False Negative) is false-negative, predicted to be 0 and actually 1, indicating the number of unrecognized lesions.
Detection accuracy is usually assessed using mAP. The average accuracy of each category in the dataset needs to be calculated first:
In the above-mentioned formula, \(N\left( {class} \right)\) represents the number of all categories, \(Precision\left( j \right)\) and \(Recall\left( j \right)\) represents the precision and recall of class j respectively.
Average accuracy for each category is defined as mAP:
The greater the value of \(mAP\) , the higher the recognition accuracy of the algorithm; conversely, the lower the accuracy of the algorithm.
F1 score is also introduced to measure the accuracy of the model. F1 score takes into account both the accuracy and recall of the model. The formula is
Frames per second (FPS) is used to evaluate the recognition speed. The more frames per second, the faster the algorithm recognition speed; conversely, the slower the algorithm recognition speed.
Performance comparison of existing algorithms
At present, the research on plant diseases and pests based on deep learning involves a wide range of crops, including all kinds of vegetables, fruits and food crops. The tasks completed include not only the basic tasks of classification, detection and segmentation, but also more complex tasks such as the judgment of infection degree.
At present, most of the current deep learning-based methods for plant diseases and pests detection are applied on specific datasets, many datasets are not publicly available, there is still no single publicly available and comprehensive dataset that will allow all algorithms to be uniformly compared. With the continuous development of deep learning, the application performance of some typical algorithms on different datasets has been gradually improved, and the mAP, F1 score and FPS of the algorithms have all been increased.
The breakthroughs achieved in the existing studies are amazing, but due to the fact that there is still a certain gap between the complexity of the infectious diseases and pests images in the existing studies and the real-time field diseases and pests detection based on mobile devices. Subsequent studies will need to find breakthroughs in larger, more complex, and more realistic datasets.
Small dataset size problem
At present, deep learning methods are widely used in various computer vision tasks, plant diseases and pests detection is generally regarded as specific application in the field of agriculture. There are too few agricultural plant diseases and pests samples available. Compared with open standard libraries, self-collected data sets are small in size and laborious in labeling data. Compared with more than 14 million sample data in ImageNet datasets, the most critical problem facing plant diseases and pests detection is the problem of small samples. In practice, some plant diseases have low incidence and high cost of disease image acquisition, resulting in only a few or dozen training data collected, which limits the application of deep learning methods in the field of plant diseases and pests identification. In fact, for the problem of small samples, there are currently three different solutions.
Data amplification, synthesis and generation
Data amplification is a key component of training deep learning models. An optimized data amplification strategy can effectively improve the plant diseases and pests detection effect. The most common method of plant diseases and pests image expansion is to acquire more samples using image processing operations such as mirroring, rotating, shifting, warping, filtering, contrast adjustment, and so on for the original plant diseases and pests samples. In addition, Generative Adversarial Networks (GANs) [ 93 ] and Variational automatic encoder (VAE) [ 94 ] can generate more diverse samples to enrich limited datasets.
Transfer learning and fine-tuning classical network model
Transfer learning (TL) transfers knowledge learned from generic large datasets to specialized areas with relatively small amounts of data. When transfer learning develops a model for newly collected unlabeled samples, it can start with a training model by a similar known dataset. After fine-tuning parameters or modifying components, it can be applied to localized plant disease and pest detection, which can reduce the cost of model training and enable the convolution neural network to adapt to small sample data. Oppenheim et al. [ 95 ] collected infected potato images of different sizes, hues and shapes under natural light and classified by fine-tuning the VGG network. The results showed that, the transfer learning and training of new networks were effective. Too et al. [ 96 ] evaluated various classical networks by fine-tuning and contrast. The experimental results showed that the accuracy of Dense-Nets improved with the number of iterations. Chen et al. [ 97 ] used transfer learning and fine-tuning to identify rice disease images under complex background conditions and achieved an average accuracy of 92.00%, which proves that the performance of transfer learning is better than training from scratch.
Reasonable network structure design
By designing a reasonable network structure, the sample requirements can be greatly reduced. Zhang et al. [ 98 ] constructed a three-channel convolution neural network model for plant leaf disease recognition by combining three color components. Each channel TCCNN component is composed of three color RGB leaf disease images. Liu et al. [ 99 ] presented an improved CNN method for identifying grape leaf diseases. The model used a depth-separable convolution instead of a standard convolution to alleviate overfitting and reduce the number of parameters. For the different size of grape leaf lesions, the initial structure was applied to the model to improve the ability of multi-scale feature extraction. Compared with the standard ResNet and GoogLeNet structures, this model has faster convergence speed and higher accuracy during training. The recognition accuracy of this algorithm was 97.22%.
Fine-grained identification of small-size lesions in early identification
Small-size lesions in early identification.
Accurate early detection of plant diseases is essential to maximize the yield [ 36 ]. In the actual early identification of plant diseases and pests, due to the small size of the lesion object itself, multiple down sampling processes in the deep feature extraction network tend to cause small-scale objects to be ignored. Moreover, due to the background noise problem on the collected images, large-scale complex background may lead to more false detection, especially on low-resolution images. In view of the shortage of existing algorithms, the improvement direction of small object detection algorithm is analyzed, and several strategies such as attention mechanism are proposed to improve the performance of small target detection.
The use of attention mechanism makes resources allocated more rationally. The essence of attention mechanism is to quickly find region of interest and ignore unimportant information. By learning the characteristics of plant diseases and pests images, features can be separated using weighted sum method with weighted coefficient, and the background noise in the image can be suppressed. Specifically, the attention mechanism module can get a salient image, and seclude the object from the background, and the Softmax function can be used to manipulate the feature image, and combine it with the original feature image to obtain new fusion features for noise reduction purposes. In future studies on early recognition of plant diseases and pests, attention mechanisms can be used to effectively select information and allocate more resources to region of interest to achieve more accurate detection. Karthik et al. [ 100 ] applied attention mechanism on the residual network and experiments were carried out using the plantVillage dataset, which achieved 98% overall accuracy.
Fine-grained identification
First, there is a large difference within the class, that is, the visual characteristics of plant diseases and pests belonging to the same class are quite different. The reason is that the aforementioned external factors such as uneven illumination, dense occlusion, blurred equipment dithering and other interferences, resulting in different image samples belonging to the same kind of diseases and pests differ greatly. Plant diseases and pests detection in complex scenarios is a very challenging task of fine-grained recognition [ 101 ]. The existence of growth variations of diseases and pests results in distinct differences in the characterization of the same diseases and pests at different stages, forming the “intra-class difference” fine-grained characteristics.
Secondly, there is fuzziness between classes, that is, objects of different classes have some similarity. There are many detailed classifications of biological subspecies and subclasses of different kinds of diseases and pests, and there are some similarities of biological morphology and life habits among the subclasses, which lead to the problem of fine-grained identification of “inter-class similarity”. Barbedo believed that similar symptoms could be produced, which even phytopathologists could not correctly distinguish [ 102 ].
Thirdly, background disturbance makes it impossible for plant diseases and pests to appear in a very clean background in the real world. Background can be very complex and interfere with objects of interest, which makes plant diseases and pests detection more difficult. Some literature often ignores this issue because images are captured under controlled conditions [ 103 ].
Relying on the existing deep learning methods can not effectively identify the fine-grained characteristics of diseases and pests that exist naturally in the application of the above actual agricultural scenarios, resulting in technical difficulties such as low identification accuracy and generalization robustness, which has long restricted the performance improvement of decision-making management of diseases and pests by the Intelligent Agricultural Internet of Things [ 104 ]. The existing research is only suitable for fine-grained identification of fewer class of diseases and pests, can not solve the problem of large-scale, large-category, accurate and efficient identification of diseases and pests, and is difficult to deploy directly to the mobile terminals of smart agriculture.
Detection performance under the influence of illumination and occlusion
Lighting problems.
Previous studies have collected images of plant diseases and pests mostly in indoor light boxes [ 105 ]. Although this method can effectively eliminate the influence of external light to simplify image processing, it is quite different from the images collected under real natural light. Because natural light changes very dynamically, and the range in which the camera can accept dynamic light sources is limited, it is easy to cause image color distortion when above or below this limit. In addition, due to the difference of view angle and distance during image collection, the apparent characteristics of plant diseases and pests change greatly, which brings great difficulties to the visual recognition algorithm.
Occlusion problem
At present, most researchers intentionally avoid the recognition of plant diseases and pests in complex environments. They only focus on a single background. They use the method of directly intercepting the area of interest to the collected images, but seldom consider the occlusion problem. As a result, the recognition accuracy under occlusion is low and the practicability is greatly reduced. Occlusion problems are common in real natural environments, including blade occlusion caused by changes in blade posture, branch occlusion, light occlusion caused by external lighting, and mixed occlusion caused by different types of occlusion. The difficulties of plant diseases and pests identification under occlusion are the lack of features and noise overlap caused by occlusion. Different occlusion conditions have different degrees of impact on the recognition algorithm, resulting in false detection or even missed detection. In recent years, with the maturity of deep learning algorithms under restricted conditions, some researchers have gradually challenged the identification of plant diseases and pests under occluded conditions [ 106 , 107 ], and significant progress has been made, which lays a good foundation for the application of plant diseases and pests identification in real-world scenarios. However, occlusion is random and complex. The training of the basic framework is difficult and the dependence on the performance of hardware devices still exists, we should strengthen the innovation and optimization of the basic framework, including the design of lightweight network architecture. The exploration of GAN and other aspects should be enhanced, while ensuring the accuracy of detection, the difficulty of model training should be reduced. GAN has prominent advantages in dealing with posture changes and chaotic background, but its design is not yet mature, and it is easy to crash in learning and cause model uncontrollable problems during training. We should strengthen the exploration of network performance to make it easier to quantify the quality of the model.
Detection speed problem
Compared with traditional methods, deep learning algorithms have better results, but their computational complexity is also higher. If the detection accuracy is guaranteed, the model needs to fully learn the characteristics of the image and increase the computational load, which will inevitably lead to slow detection speed and can not meet the needs of real-time. In order to ensure the detection speed, it is usually necessary to reduce the amount of calculation. However, this will cause insufficient training and result in false or missed detection. Therefore, it is important to design an efficient algorithm with both detection accuracy and detection speed.
Plant diseases and pests detection methods based on deep learning include three main links in agricultural applications: data labeling, model training and model inference. In real-time agricultural applications, more attention is paid to model inference. Currently, most plant diseases and pests detection methods focus on the accuracy of recognition. Little attention is paid to the efficiency of model inference. In reference [ 108 ], to improve the efficiency of the model calculation process to meet the actual agricultural needs, a deep separable convolution structure model for plant leaf disease detection was introduced. Several models were trained and tested. The classification accuracy of Reduced MobileNet was 98.34%, the parameters were 29 times less than VGG, and 6 times less than MobileNet. This shows an effective compromise between delay and accuracy, which is suitable for real-time crop diseases diagnosis on resource-constrained mobile devices.
Conclusions and future directions
Compared with traditional image processing methods, which deal with plant diseases and pests detection tasks in several steps and links, plant diseases and pests detection methods based on deep learning unify them into end-to-end feature extraction, which has a broad development prospects and great potential. Although plant diseases and pests detection technology is developing rapidly, it has been moving from academic research to agricultural application, there is still a certain distance from the mature application in the real natural environment, and there are still some problems to be solved.
Plant diseases and pests detection dataset
Deep learning technology has made some achievements in the identification of plant diseases and pests. Various image recognition algorithms have also been further developed and extended, which provides a theoretical basis for the identification of specific diseases and pests. However, the collection of image samples in previous studies mostly come from the characterization of disease spots, insect appearance characteristics or the characterization of insect pests and leaves. Most of the research results are limited to the laboratory environment and are applicable only to the plant diseases and pests images obtained at the time. The main reason for this is that the growth of plants is cyclical, continuous, seasonal and regional. Similarly, the characteristics of the same disease or pest at different growing stages of crops are different. Images of different plant species vary from region to region. As a result, most of the existing research results are not universal. Even with a high recognition rate in a single trial, the validity of the data obtained at other times cannot be guaranteed.
Most of the existing studies are based on the images generated in the visible range, but the electromagnetic wave outside the visible range also contains a lot of information, so the comprehensive information such as visible light, near infrared, multi-spectral should be fused to achieve the acquisition of plant diseases and pests dataset. Future research should focus on multi-information fusion method to obtain and identify plant diseases and pests information.
In addition, image databases of different kinds of plant diseases and pests in real natural environments are still in the blank stage. Future research should make full use of the data information acquisition platform such as portable field spore auto-capture instrument, unmanned aerial vehicle aerial photography system, agricultural internet of things monitoring equipment, which performs large-area and coverage identification of farmland and makes up for the lack of randomness of image samples in previous studies. Also, it can ensures the comprehensiveness and accuracy of dataset, and improves the generality of the algorithm.
Early recognition of plant diseases and pests
In the application of plant diseases and pests identification, the manifestation symptoms are not obvious, so early diagnosis is very difficult whether it is by visual observation or computer interpretation. However, the research significance and demand of early diagnosis are greater, which is more conducive to the prevention and control of plant diseases and pests and prevent their spread and development. The best image quality can be obtained when the sunlight is sufficient, and taking pictures in cloudy weather will increase the complexity of image preprocessing and reduce the recognition effect. In addition, in the early stage of plant diseases and pests occurrence, even high-resolution images are difficult to analyze. It is necessary to combine meteorological and plant protection data such as temperature and humidity to realize the recognition and prediction of diseases and pests. By consulting the existing research literatures, there are few reports on the early diagnosis of plant diseases and pests.
Network training and learning
When plant diseases and pests are visually identified manually, it is difficult to collect samples of all plant diseases and pests types, and many times only healthy data (positive samples) are available. However, most of the current plant diseases and pests detection methods based on deep learning are supervised learning based on a large number of diseases and pests samples, so manual collection of labelled datasets requires a lot of manpower, so unsupervised learning needs to be explored. Deep learning is a black box, which requires a large number of labelled training samples for end-to-end learning and has poor interpretability. Therefore, how to use the prior knowledge of brain-inspired computing and human-like visual cognitive model to guide the training and learning of the network is also a direction worthy of studying. At the same time, deep models need a large amount of memory and are extremely time-consuming during testing, which makes them unsuitable for deployment on mobile platforms with limited resources. It is important to study how to reduce complexity and obtain fast-executing models without losing accuracy. Finally, the selection of appropriate hyper-parameters has always been a major obstacle to the application of deep learning model to new tasks, such as learning rate, filter size, step size and number, these hyper-parameters have a strong internal dependence, any small adjustment may have a greater impact on the final training results.
Interdisciplinary research
Only by more closely integrating empirical data with theories such as agronomic plant protection, can we establish a field diagnosis model that is more in line with the rules of crop growth, and will further improve the effectiveness and accuracy of plant diseases and pests identification. In the future, it is necessary to go from image analysis at the surface level to identification of the occurrence mechanism of diseases and pests, and transition from simple experimental environment to practical application research that comprehensively considers crop growth law, environmental factors, etc.
In summary, with the development of artificial intelligence technology, the research focus of plant diseases and pests detection based on machine vision has shifted from classical image processing and machine learning methods to deep learning methods, which solved the difficult problems that could not be solved by traditional methods. There is still a long distance from the popularization of practical production and application, but this technology has great development potential and application value. To fully explore the potential of this technology, the joint efforts of experts from relevant disciplines are needed to effectively integrate the experience knowledge of agriculture and plant protection with deep learning algorithms and models, so as to make plant diseases and pests detection based on deep learning mature. Also, the research results should be integrated into agricultural machinery equipment to truly land the corresponding theoretical results.
Availability of data and materials
For relevant data and codes, please contact the corresponding author of this manuscript.
Lee SH, Chan CS, Mayo SJ, Remagnino P. How deep learning extracts and learns leaf features for plant classification. Pattern Recogn. 2017;71:1–13.
Article Google Scholar
Tsaftaris SA, Minervini M, Scharr H. Machine learning for plant phenotyping needs image processing. Trends Plant Sci. 2016;21(12):989–91.
Article CAS PubMed Google Scholar
Fuentes A, Yoon S, Park DS. Deep learning-based techniques for plant diseases recognition in real-field scenarios. In: Advanced concepts for intelligent vision systems. Cham: Springer; 2020.
Google Scholar
Yang D, Li S, Peng Z, Wang P, Wang J, Yang H. MF-CNN: traffic flow prediction using convolutional neural network and multi-features fusion. IEICE Trans Inf Syst. 2019;102(8):1526–36.
Sundararajan SK, Sankaragomathi B, Priya DS. Deep belief cnn feature representation based content based image retrieval for medical images. J Med Syst. 2019;43(6):1–9.
Melnyk P, You Z, Li K. A high-performance CNN method for offline handwritten chinese character recognition and visualization. Soft Comput. 2019;24:7977–87.
Li J, Mi Y, Li G, Ju Z. CNN-based facial expression recognition from annotated rgb-d images for human–robot interaction. Int J Humanoid Robot. 2019;16(04):504–5.
Kumar S, Singh SK. Occluded thermal face recognition using bag of CNN(BoCNN). IEEE Signal Process Lett. 2020;27:975–9.
Wang X. Deep learning in object recognition, detection, and segmentation. Found Trends Signal Process. 2016;8(4):217–382.
Article CAS Google Scholar
Boulent J, Foucher S, Théau J, St-Charles PL. Convolutional neural networks for the automatic identification of plant diseases. Front Plant Sci. 2019;10:941.
Article PubMed PubMed Central Google Scholar
Kumar S, Kaur R. Plant disease detection using image processing—a review. Int J Comput Appl. 2015;124(2):6–9.
Martineau M, Conte D, Raveaux R, Arnault I, Munier D, Venturini G. A survey on image-based insect classification. Pattern Recogn. 2016;65:273–84.
Jayme GAB, Luciano VK, Bernardo HV, Rodrigo VC, Katia LN, Claudia VG, et al. Annotated plant pathology databases for image-based detection and recognition of diseases. IEEE Latin Am Trans. 2018;16(6):1749–57.
Kaur S, Pandey S, Goel S. Plants disease identification and classification through leaf images: a survey. Arch Comput Methods Eng. 2018;26(4):1–24.
CAS Google Scholar
Shekhawat RS, Sinha A. Review of image processing approaches for detecting plant diseases. IET Image Process. 2020;14(8):1427–39.
Hinton GE, Salakhutdinov R. Reducing the dimensionality of data with neural networks. Science. 2006;313(5786):504–7.
Liu W, Wang Z, Liu X, et al. A survey of deep neural network architectures and their applications. Neurocomputing. 2017;234:11–26.
Fergus R. Deep learning methods for vision. CVPR 2012 Tutorial; 2012.
Bengio Y, Courville A, Vincent P. Representation learning: a review and new perspectives. IEEE Trans Pattern Anal Mach Intell. 2013;35(8):1798–828.
Article PubMed Google Scholar
Boureau YL, Le Roux N, Bach F, Ponce J, Lecun Y. [IEEE 2011 IEEE international conference on computer vision (ICCV)—Barcelona, Spain (2011.11.6–2011.11.13)] 2011 international conference on computer vision—ask the locals: multi-way local pooling for image recognition; 2011. p. 2651–8.
Zeiler MD, Fergus R. Stochastic pooling for regularization of deep convolutional neural networks. Eprint Arxiv. arXiv:1301.3557 . 2013.
TensorFlow. https://www.tensorflow.org/ .
Torch/PyTorch. https://pytorch.org/ .
Caffe. http://caffe.berkeleyvision.org/ .
Theano. http://deeplearning.net/software/theano/ .
Krizhenvshky A, Sutskever I, Hinton G. Imagenet classification with deep convolutional networks. In: Proceedings of the conference neural information processing systems (NIPS), Lake Tahoe, NV, USA, 3–8 December; 2012. p. 1097–105.
Szegedy C, Liu W, Jia Y, Sermanet P, Reed S, Anguelov D, Erhan D, Vanhoucke V, Rabinovich A. Going deeper with convolutions. In: Proceedings of the 2015 IEEE conference on computer vision and pattern recognition, Boston, MA, USA, 7–12 June; 2015. p. 1–9.
Simonyan K, Zisserman A. Very deep convolutional networks for large-scale image recognition. arXiv. arXiv:1409.1556 . 2014.
Xie S, Girshick R, Dollár P, Tu Z, He K. Aggregated residual transformations for deep neural networks. arXiv. arXiv:1611.05431 . 2017.
Szegedy C, Ioffe S, Vanhoucke V, et al. Inception-v4, inception-resnet and the impact of residual connections on learning. In: Proceedings of the AAAI conference on artificial intelligence. 2016.
Huang G, Lrj Z, Maaten LVD, et al. Densely connected convolutional networks. In: IEEE conference on computer vision and pattern recognition. 2017. p. 2261–9.
Howard AG, Zhu M, Chen B, Kalenichenko D, Wang W, Weyand T, Andreetto M, Adam H. MobileNets: efficient convolutional neural networks for mobile vision applications. arXiv. arXiv:1704.04861 . 2017.
Iandola FN, Han S, Moskewicz MW, Ashraf K, Dally WJ, Keutzer K. SqueezeNet: AlexNet-level accuracy with 50 × fewer parameters and < 0.5 MB model size. arXiv. arXiv:1602.07360 . 2016.
Priyadharshini RA, Arivazhagan S, Arun M, Mirnalini A. Maize leaf disease classification using deep convolutional neural networks. Neural Comput Appl. 2019;31(12):8887–95.
Wen J, Shi Y, Zhou X, Xue Y. Crop disease classification on inadequate low-resolution target images. Sensors. 2020;20(16):4601.
Article PubMed Central Google Scholar
Thangaraj R, Anandamurugan S, Kaliappan VK. Automated tomato leaf disease classification using transfer learning-based deep convolution neural network. J Plant Dis Prot. 2020. https://doi.org/10.1007/s41348-020-00403-0 .
Atila M, Uar M, Akyol K, Uar E. Plant leaf disease classification using efficientnet deep learning model. Ecol Inform. 2021;61:101182.
Sabrol H, Kumar S. Recent studies of image and soft computing techniques for plant disease recognition and classification. Int J Comput Appl. 2015;126(1):44–55.
Yalcin H, Razavi S. Plant classification using convolutional neural networks. In: 2016 5th international conference on agro-geoinformatics (agro-geoinformatics). New York: IEEE; 2016.
Fuentes A, Lee J, Lee Y, Yoon S, Park DS. Anomaly detection of plant diseases and insects using convolutional neural networks. In: ELSEVIER conference ISEM 2017—The International Society for Ecological Modelling Global Conference, 2017. 2017.
Hasan MJ, Mahbub S, Alom MS, Nasim MA. Rice disease identification and classification by integrating support vector machine with deep convolutional neural network. In: 2019 1st international conference on advances in science, engineering and robotics technology (ICASERT). 2019.
Thenmozhi K, Reddy US. Crop pest classification based on deep convolutional neural network and transfer learning. Comput Electron Agric. 2019;164:104906.
Fang T, Chen P, Zhang J, Wang B. Crop leaf disease grade identification based on an improved convolutional neural network. J Electron Imaging. 2020;29(1):1.
Nagasubramanian K, Jones S, Singh AK, Sarkar S, Singh A, Ganapathysubramanian B. Plant disease identification using explainable 3D deep learning on hyperspectral images. Plant Methods. 2019;15(1):1–10.
Picon A, Seitz M, Alvarez-Gila A, Mohnke P, Echazarra J. Crop conditional convolutional neural networks for massive multi-crop plant disease classification over cell phone acquired images taken on real field conditions. Comput Electron Agric. 2019;167:105093.
Tianjiao C, Wei D, Juan Z, Chengjun X, Rujing W, Wancai L, et al. Intelligent identification system of disease and insect pests based on deep learning. China Plant Prot Guide. 2019;039(004):26–34.
Dechant C, Wiesner-Hanks T, Chen S, Stewart EL, Yosinski J, Gore MA, et al. Automated identification of northern leaf blight-infected maize plants from field imagery using deep learning. Phytopathology. 2017;107:1426–32.
Wiesner-Hanks T, Wu H, Stewart E, Dechant C, Nelson RJ. Millimeter-level plant disease detection from aerial photographs via deep learning and crowdsourced data. Front Plant Sci. 2019;10:1550.
Shougang R, Fuwei J, Xingjian G, Peishen Y, Wei X, Huanliang X. Deconvolution-guided tomato leaf disease identification and lesion segmentation model. J Agric Eng. 2020;36(12):186–95.
Fujita E, Kawasaki Y, Uga H, Kagiwada S, Iyatomi H. Basic investigation on a robust and practical plant diagnostic system. In: IEEE international conference on machine learning & applications. New York: IEEE; 2016.
Mohanty SP, Hughes DP, Salathé M. Using deep learning for image-based plant disease detection. Front Plant Sci. 2016;7:1419. https://doi.org/10.3389/fpls.2016.01419 .
Brahimi M, Arsenovic M, Laraba S, Sladojevic S, Boukhalfa K, Moussaoui A. Deep learning for plant diseases: detection and saliency map visualisation. In: Zhou J, Chen F, editors. Human and machine learning. Cham: Springer International Publishing; 2018. p. 93–117.
Chapter Google Scholar
Barbedo JG. Plant disease identification from individual lesions and spots using deep learning. Biosyst Eng. 2019;180:96–107.
Ren S, He K, Girshick R, Sun J. Faster R-CNN: towards real-time object detection with region proposal networks. IEEE Trans Pattern Anal Mach Intell. 2017;39(6):1137–49.
Liu W, Anguelov D, Erhan D, Szegedy C, Berg AC. SSD: Single shot MultiBox detector. In: European conference on computer vision. Cham: Springer International Publishing; 2016.
Redmon J, Divvala S, Girshick R, Farhadi A. You only look once: unified, real-time object detection. In: Proceedings of the IEEE conference on computer vision and pattern recognition. 2015.
Redmon J, Farhadi A. Yolo9000: better, faster, stronger. In: Proceedings of the IEEE conference on computer vision and pattern recognition. 2017. p. 6517–25.
Redmon J, Farhadi A. Yolov3: an incremental improvement. arXiv preprint. arXiv:1804.02767 . 2018.
Fuentes A, Yoon S, Kim SC, Park DS. A robust deep-learning-based detector for real-time tomato plant diseases and pests detection. Sensors. 2017;17(9):2022.
Ozguven MM, Adem K. Automatic detection and classification of leaf spot disease in sugar beet using deep learning algorithms. Phys A Statal Mech Appl. 2019;535(2019):122537.
Zhou G, Zhang W, Chen A, He M, Ma X. Rapid detection of rice disease based on FCM-KM and faster R-CNN fusion. IEEE Access. 2019;7:143190–206. https://doi.org/10.1109/ACCESS.2019.2943454 .
Xie X, Ma Y, Liu B, He J, Wang H. A deep-learning-based real-time detector for grape leaf diseases using improved convolutional neural networks. Front Plant Sci. 2020;11:751.
Singh D, Jain N, Jain P, Kayal P, Kumawat S, Batra N. Plantdoc: a dataset for visual plant disease detection. In: Proceedings of the 7th ACM IKDD CoDS and 25th COMAD. 2019.
Sun J, Yang Y, He X, Wu X. Northern maize leaf blight detection under complex field environment based on deep learning. IEEE Access. 2020;8:33679–88. https://doi.org/10.1109/ACCESS.2020.2973658 .
Bhatt PV, Sarangi S, Pappula S. Detection of diseases and pests on images captured in uncontrolled conditions from tea plantations. In: Proc. SPIE 11008, autonomous air and ground sensing systems for agricultural optimization and phenotyping IV; 2019. p. 1100808. https://doi.org/10.1117/12.2518868 .
Zhang B, Zhang M, Chen Y. Crop pest identification based on spatial pyramid pooling and deep convolution neural network. Trans Chin Soc Agric Eng. 2019;35(19):209–15.
Ramcharan A, McCloskey P, Baranowski K, Mbilinyi N, Mrisho L, Ndalahwa M, Legg J, Hughes D. A mobile-based deep learning model for cassava disease diagnosis. Front Plant Sci. 2019;10:272. https://doi.org/10.3389/fpls.2019.00272 .
Selvaraj G, Vergara A, Ruiz H, Safari N, Elayabalan S, Ocimati W, Blomme G. AI-powered banana diseases and pest detection. Plant Methods. 2019. https://doi.org/10.1186/s13007-019-0475-z .
Tian Y, Yang G, Wang Z, Li E, Liang Z. Detection of apple lesions in orchards based on deep learning methods of CycleGAN and YOLOV3-dense. J Sens. 2019. https://doi.org/10.1155/2019/7630926 .
Zheng Y, Kong J, Jin X, Wang X, Zuo M. CropDeep: the crop vision dataset for deep-learning-based classification and detection in precision agriculture. Sensors. 2019;19:1058. https://doi.org/10.3390/s19051058 .
Arsenovic M, Karanovic M, Sladojevic S, Anderla A, Stefanović D. Solving current limitations of deep learning based approaches for plant disease detection. Symmetry. 2019;11:21. https://doi.org/10.3390/sym11070939 .
Fuentes AF, Yoon S, Lee J, Park DS. High-performance deep neural network-based tomato plant diseases and pests diagnosis system with refinement filter bank. Front Plant Sci. 2018;9:1162. https://doi.org/10.3389/fpls.2018.01162 .
Jiang P, Chen Y, Liu B, He D, Liang C. Real-time detection of apple leaf diseases using deep learning approach based on improved convolutional neural networks. IEEE Access. 2019. https://doi.org/10.1109/ACCESS.2019.2914929 .
Long J, Shelhamer E, Darrell T. Fully convolutional networks for semantic segmentation. IEEE Trans Pattern Anal Mach Intell. 2015;39(4):640–51.
He K, Gkioxari G, Dollár P, Girshick R. Mask R-CNN. In: 2017 IEEE international conference on computer vision (ICCV). New York: IEEE; 2017.
Ronneberger O, Fischer P, Brox T. U-net: convolutional networks for biomedical image segmentation. In: International conference on medical image computing and computer-assisted intervention. Berlin: Springer; 2015. p. 234–41. https://doi.org/10.1007/978-3-319-24574-4_28 .
Badrinarayanan V, Kendall A, Cipolla R. Segnet: a deep convolutional encoder-decoder architecture for image segmentation. IEEE Trans Pattern Anal Mach Intell. 2019;39(12):2481–95.
Wang Z, Zhang S. Segmentation of corn leaf disease based on fully convolution neural network. Acad J Comput Inf Sci. 2018;1:9–18.
Wang X, Wang Z, Zhang S. Segmenting crop disease leaf image by modified fully-convolutional networks. In: Huang DS, Bevilacqua V, Premaratne P, editors. Intelligent computing theories and application. ICIC 2019, vol. 11643. Lecture Notes in Computer Science. Cham: Springer; 2019. https://doi.org/10.1007/978-3-030-26763-6_62 .
Lin K, Gong L, Huang Y, Liu C, Pan J. Deep learning-based segmentation and quantification of cucumber powdery mildew using convolutional neural network. Front Plant Sci. 2019;10:155.
Kerkech M, Hafiane A, Canals R. Vine disease detection in UAV multispectral images using optimized image registration and deep learning segmentation approach. Comput Electron Agric. 2020;174:105446.
Stewart EL, Wiesner-Hanks T, Kaczmar N, Dechant C, Gore MA. Quantitative phenotyping of northern leaf blight in UAV images using deep learning. Remote Sens. 2019;11(19):2209.
Wang Q, Qi F, Sun M, Qu J, Xue J. Identification of tomato disease types and detection of infected areas based on deep convolutional neural networks and object detection techniques. Comput Intell Neurosci. 2019. https://doi.org/10.1155/2019/9142753 .
Hughes DP, Salathe M. An open access repository of images on plant health to enable the development of mobile disease diagnostics through machine learning and crowdsourcing. Comput Sci. 2015.
Shah JP, Prajapati HB, Dabhi VK. A survey on detection and classification of rice plant diseases. In: IEEE international conference on current trends in advanced computing. New York: IEEE; 2016.
Prajapati HB, Shah JP, Dabhi VK. Detection and classification of rice plant diseases. Intell Decis Technol. 2017;11(3):1–17.
Barbedo JGA, Koenigkan LV, Halfeld-Vieira BA, Costa RV, Nechet KL, Godoy CV, Junior ML, Patricio FR, Talamini V, Chitarra LG, Oliveira SAS. Annotated plant pathology databases for image-based detection and recognition of diseases. IEEE Latin Am Trans. 2018;16(6):1749–57.
Brahimi M, Arsenovic M, Laraba S, Sladojevic S, Boukhalfa K, Moussaoui A. Deep learning for plant diseases: detection and saliency map visualisation. In: Zhou J, Chen F, editors. Human and machine learning. Human–computer interaction series. Cham: Springer; 2018. https://doi.org/10.1007/978-3-319-90403-0_6 .
Tyr WH, Stewart EL, Nicholas K, Chad DC, Harvey W, Nelson RJ, et al. Image set for deep learning: field images of maize annotated with disease symptoms. BMC Res Notes. 2018;11(1):440.
Thapa R, Snavely N, Belongie S, Khan A. The plant pathology 2020 challenge dataset to classify foliar disease of apples. arXiv preprint. arXiv:2004.11958 . 2020.
Wu X, Zhan C, Lai YK, Cheng MM, Yang J. IP102: a large-scale benchmark dataset for insect pest recognition. In: 2019 IEEE/CVF conference on computer vision and pattern recognition (CVPR). New York: IEEE; 2019.
Huang M-L, Chuang TC. A database of eight common tomato pest images. Mendeley Data. 2020. https://doi.org/10.17632/s62zm6djd2.1 .
Goodfellow I, Pouget-Abadie J, Mirza M, Xu B, Warde Farley D, Ozair S, Courville A, Bengio Y. Generative adversarial nets. In: Proceedings of the 2014 conference on advances in neural information processing systems 27. Montreal: Curran Associates, Inc.; 2014. p. 2672–80.
Pu Y, Gan Z, Henao R, et al. Variational autoencoder for deep learning of images, labels and captions [EB/OL]. 2016–09–28. arxiv:1609.08976 .
Oppenheim D, Shani G, Erlich O, Tsror L. Using deep learning for image-based potato tuber disease detection. Phytopathology. 2018;109(6):1083–7.
Too EC, Yujian L, Njuki S, Yingchun L. A comparative study of fine-tuning deep learning models for plant disease identification. Comput Electron Agric. 2018;161:272–9.
Chen J, Chen J, Zhang D, Sun Y, Nanehkaran YA. Using deep transfer learning for image-based plant disease identification. Comput Electron Agric. 2020;173:105393.
Zhang S, Huang W, Zhang C. Three-channel convolutional neural networks for vegetable leaf disease recognition. Cogn Syst Res. 2018;53:31–41. https://doi.org/10.1016/j.cogsys.2018.04.006 .
Liu B, Ding Z, Tian L, He D, Li S, Wang H. Grape leaf disease identification using improved deep convolutional neural networks. Front Plant Sci. 2020;11:1082. https://doi.org/10.3389/fpls.2020.01082 .
Karthik R, Hariharan M, Anand S, et al. Attention embedded residual CNN for disease detection in tomato leaves. Appl Soft Comput J. 2020;86:105933.
Guan W, Yu S, Jianxin W. Automatic image-based plant disease severity estimation using deep learning. Comput Intell Neurosci. 2017;2017:2917536.
Barbedo JGA. Factors influencing the use of deep learning for plant disease recognition. Biosyst Eng. 2018;172:84–91.
Barbedo JGA. Impact of dataset size and variety on the effectiveness of deep learning and transfer learning for plant disease classification. Comput Electron Agric. 2018;153:46–53.
Nawaz MA, Khan T, Mudassar R, Kausar M, Ahmad J. Plant disease detection using internet of thing (IOT). Int J Adv Comput Sci Appl. 2020. https://doi.org/10.14569/IJACSA.2020.0110162 .
Martinelli F, Scalenghe R, Davino S, Panno S, Scuderi G, Ruisi P, et al. Advanced methods of plant disease detection. A review. Agron Sustain Dev. 2015;35(1):1–25.
Liu J, Wang X. Early recognition of tomato gray leaf spot disease based on MobileNetv2-YOLOv3 model. Plant Methods. 2020;16:83.
Article CAS PubMed PubMed Central Google Scholar
Liu J, Wang X. Tomato diseases and pests detection based on improved Yolo V3 convolutional neural network. Front Plant Sci. 2020;11:898.
Kamal KC, Yin Z, Wu M, Wu Z. Depthwise separable convolution architectures for plant disease classification. Comput Electron Agric. 2019;165:104948.
Download references
Acknowledgements
Appreciations are given to the editors and reviewer of the Journal Plant Method.
This study was supported by the Facility Horticulture Laboratory of Universities in Shandong with Project Numbers 2019YY003, 2018YY016, 2018YY043 and 2018YY044; school level High-level Talents Project 2018RC002; Youth Fund Project of Philosophy and Social Sciences of Weifang College of Science and Technology with project numbers 2018WKRQZ008 and 2018WKRQZ008-3; Key research and development plan of Shandong Province with Project Number 2019RKA07012, 2019GNC106034 and 2020RKA07036; Research and Development Plan of Applied Technology in Shouguang with Project Number 2018JH12; 2018 innovation fund of Science and Technology Development centre of the China Ministry of Education with Project Number 2018A02013; 2019 basic capacity construction project of private colleges and universities in Shandong Province; and Weifang Science and Technology Development Programme with project numbers 2019GX081 and 2019GX082, Special project of Ideological and political education of Weifang University of science and technology (W19SZ70Z01).
Author information
Authors and affiliations.
Shandong Provincial University Laboratory for Protected Horticulture, Blockchain Laboratory of Agricultural Vegetables, Weifang University of Science and Technology, Weifang, 262700, Shandong, China
Jun Liu & Xuewei Wang
You can also search for this author in PubMed Google Scholar
Contributions
JL designed the research. JL and XW conducted the experiments and data analysis and wrote the manuscript. XW revised the manuscript. Both authors read and approved the final manuscript.
Corresponding author
Correspondence to Xuewei Wang .
Ethics declarations
Ethics approval and consent to participate.
Not applicable.
Consent for publication
Competing interests.
The authors declare that they have no competing interests.
Additional information
Publisher's note.
Springer Nature remains neutral with regard to jurisdictional claims in published maps and institutional affiliations.
Rights and permissions
Open Access This article is licensed under a Creative Commons Attribution 4.0 International License, which permits use, sharing, adaptation, distribution and reproduction in any medium or format, as long as you give appropriate credit to the original author(s) and the source, provide a link to the Creative Commons licence, and indicate if changes were made. The images or other third party material in this article are included in the article's Creative Commons licence, unless indicated otherwise in a credit line to the material. If material is not included in the article's Creative Commons licence and your intended use is not permitted by statutory regulation or exceeds the permitted use, you will need to obtain permission directly from the copyright holder. To view a copy of this licence, visit http://creativecommons.org/licenses/by/4.0/ . The Creative Commons Public Domain Dedication waiver ( http://creativecommons.org/publicdomain/zero/1.0/ ) applies to the data made available in this article, unless otherwise stated in a credit line to the data.
Reprints and permissions
About this article
Cite this article.
Liu, J., Wang, X. Plant diseases and pests detection based on deep learning: a review. Plant Methods 17 , 22 (2021). https://doi.org/10.1186/s13007-021-00722-9
Download citation
Received : 20 September 2020
Accepted : 13 February 2021
Published : 24 February 2021
DOI : https://doi.org/10.1186/s13007-021-00722-9
Share this article
Anyone you share the following link with will be able to read this content:
Sorry, a shareable link is not currently available for this article.
Provided by the Springer Nature SharedIt content-sharing initiative
- Deep learning
- Plant diseases and pests
- Classification
- Object detection
- Segmentation
Plant Methods
ISSN: 1746-4811
- Submission enquiries: [email protected]
ORIGINAL RESEARCH article
Detection and identification of plant leaf diseases using yolov4.

- 1 Department of Computer Sciences, College of Computer and Information Sciences, Princess Nourah bint Abdulrahman University, Riyadh, Saudi Arabia
- 2 Department of Computer Science, College of Computer and Information Science, King Saud University, Riyadh, Saudi Arabia
- 3 Department of Biology, College of Science, Imam Abdulrahman Bin Faisal University, Dammam, Saudi Arabia
Detecting plant leaf diseases accurately and promptly is essential for reducing economic consequences and maximizing crop yield. However, farmers’ dependence on conventional manual techniques presents a difficulty in accurately pinpointing particular diseases. This research investigates the utilization of the YOLOv4 algorithm for detecting and identifying plant leaf diseases. This study uses the comprehensive Plant Village Dataset, which includes over fifty thousand photos of healthy and diseased plant leaves from fourteen different species, to develop advanced disease prediction systems in agriculture. Data augmentation techniques including histogram equalization and horizontal flip were used to improve the dataset and strengthen the model’s resilience. A comprehensive assessment of the YOLOv4 algorithm was conducted, which involved comparing its performance with established target identification methods including Densenet, Alexanet, and neural networks. When YOLOv4 was used on the Plant Village dataset, it achieved an impressive accuracy of 99.99%. The evaluation criteria, including accuracy, precision, recall, and f1-score, consistently showed high performance with a value of 0.99, confirming the effectiveness of the proposed methodology. This study’s results demonstrate substantial advancements in plant disease detection and underscore the capabilities of YOLOv4 as a sophisticated tool for accurate disease prediction. These developments have significant significance for everyone involved in agriculture, researchers, and farmers, providing improved capacities for disease control and crop protection.
1 Introduction
Plant diseases present a crucial obstacle to the growth of agriculture in every country, resulting in significant yearly financial losses ( Mitra, 2021 ). Plant disease detection has developed into a substantial area of study in pattern recognition and contemporary agricultural development due to developments in machine learning technology ( Roy and Bhaduri, 2021 ; Albattah et al., 2022 ; Sanida et al., 2023 ). Early plant disease identification approaches used a support vector machine (SVM) ( Rahman et al., 2023 ; Thangavel et al., 2023 ), artificial neural network (ANN) ( Attallah, 2023 ), and SVM method for disease diagnosis under segmented plant disease spots. These techniques are used to manually isolate the affected area of an image, after which the K-means clustering method is implemented ( Javidan et al., 2023 ).
With the advancement of AI technology, agricultural detection based on AI is now widely utilized for tasks including predicting crop production, processing weed identification, and finding plant diseases ( Albattah et al., 2022 ). Moreover, the process of machine learning-based disease detection involves several steps. Firstly, the dataset undergoes preprocessing to ensure its suitability for analysis. Following this, feature extraction algorithms are employed to identify and extract relevant features from regions of interest in the images, specifically focusing on disease-affected areas of plant leaves. Subsequently, the extracted feature information is transmitted to the classifier, where model parameters are derived. Finally, the system accepts the identified categories of diseases, along with their respective severity levels, integrating this crucial information into the output for further analysis or action. Moreover, leveraging image recognition through machine learning methodologies holds significant promise for enhancing the generalization ability of models. Specifically, in the domain of detecting and identifying plant leaf diseases using YOLOv4, the term “model generalization ability” pertains to the model’s proficiency in accurately identifying and categorizing diseases in plant leaves across a broad spectrum of scenarios, including instances not encountered during training. This capability enables the model to effectively discern subtle patterns and characteristics indicative of various leaf diseases, thereby contributing to more reliable and robust disease detection systems ( Singh et al., 2017 ). When there are fewer classes, it is easier to distinguish between their characteristics. Moreover, the categories can only be identified within minimal visual settings. Researching a fast, end-to-end plant disease detection system is vital since it will be necessary to meet the demands of large-scale planting.
Further, early identification and management of plant diseases is an essential part of crop harvesting, since it helps to minimize development problems and lowers the need for pesticides. As a result, the environmental damage brought on by pesticide use is reduced, supporting sustainable agricultural practices ( Perveen et al., 2023 ). Many ML techniques have been used for plant and disease categorization and detection.Where such approaches, however, perform less well and more slowly when detecting diseases in real time ( Peng and Wang, 2022 ), likely due to the problematic image preprocessing and feature extraction stages. The fact that classic ML methods are unsuitable for real-world detection scenarios with complex backgrounds and non-uniform surfaces is another major disadvantage of these methods. With several applications, deep learning has lately achieved a substantial breakthrough in this area of computer vision ( Wang et al., 2021 ).
Additionally, it has been used for picture segmentation, crop recognition, and automated agriculture technology, including the classification of crops and fruits ( Dhinesh et al., 2019 ). Models based on convolutional neural networks (CNNs) have gained popularity due to their improved accuracy in object detection ( Sangeetha et al., 2022 ). CNNs can save time on preprocessing because they automatically extract features from the input images. The ability to identify crop diseases has made great strides in recent years ( Dhinesh et al., 2019 ; Sangeetha et al., 2022 ). There are now two distinct kinds of CNN-based object detectors: those with a single detection stage and those with two. One of the most popular two-stage detectors is the region convolution neural network (RCNN), which consists of the fast/faster RCNN and the mask-RCNN ( Sangeetha et al., 2022 ). These models have had significant effects on automated agriculture management ( Chen et al., 2022 ), crop and fruit detection ( Eunice et al., 2022 ), and yield and growth assessment ( Hassan et al., 2021 ). Although these models cannot recognize high-resolution images in real-time, faster R-CNN, consisting of region proposal networks (RPN) ( Chen and Wu, 2023 ) and classification networks, considerably decrease detection time. By combining target categorization and localization into a regression problem, the recently suggested You Only Look Once (YOLO) ( Soeb et al., 2023 ) method simplifies the problem. Due to its lack of RPN, YOLO employs regression to directly locate targets in the image, significantly improving its detection speed. High precision, accuracy, and detection speed are hallmarks of modern object detection technologies like YOLOv4 ( Xinming and Tang, 2023 ), which can perform several real-time object recognition applications.
This work ( Chuanlei et al., 2017 ) concentrates on rust and scab detection in apple leaves, which are susceptible to two dangerous and prevalent fungal infections. Real-time early disease identification of apple leaves is challenging due to factors such as the fine-grained multi-scale distribution, the similarity of colour texture between illnesses and background, the diversity of diseases’ morphology, and the occurrence of many diseases on the same leaf. A significant gap exists between the current model and real-time illness diagnosis on mobile computing devices since existing disease detection algorithms pay off accuracy for real-time detection speed ( Arathi and Dulhare, 2023 ).
Plant leaves have many different features, such as differences in size, shape, color, and growth conditions, but effectively identifying and categorizing diseases is still quite difficult. Brightness fluctuations that occur during the process of capturing leaf image aggravate this issue and challenge detection strategies. This paper presents a novel approach that leverages the YOLOv4 architecture to achieve remarkable accuracy on the Plant Village dataset. It is shown that the used methodology is robust against a range of input sample distortions, including noise, blurring, rotation, contrast changes, lighting adjustments, color inconsistencies, and brightness swings. The outcomes highlight how well the recommended approach works to improve plant leaf disease identification and detection.
To create a framework for plant disease detection and classification, the following processes must be completed: data collection, model training, and multiple-class categorization of plant leaf disease. Figure 1 provides an overview of the proposed framework. Leaf disease items can be found and identified with the Yolov4 method.
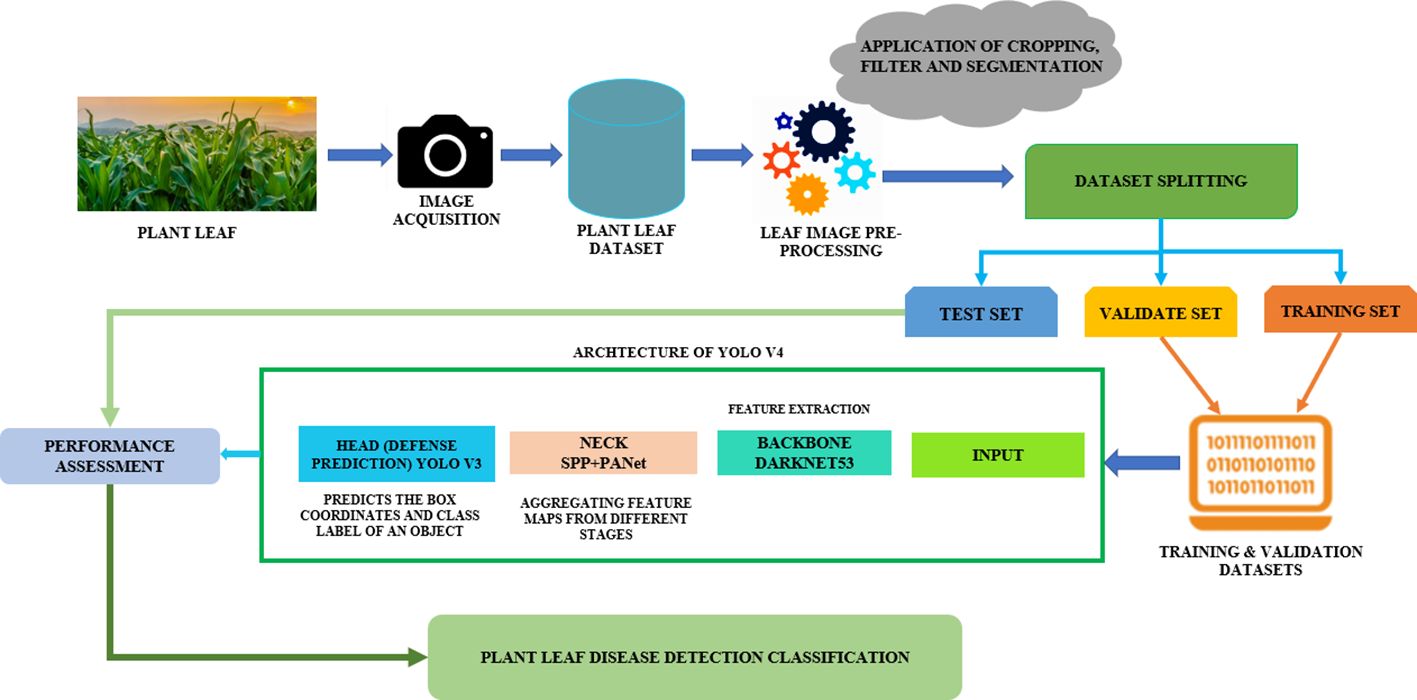
Figure 1 Implementing the Yolov4 disease detection and identification framework in plant leaves.
The following are the primary contributions of this research paper:
● This study applies YOLOv4, a cutting-edge object detection framework, to plant pathology. The work improves plant leaf disease identification accuracy and efficiency by seamlessly incorporating YOLOv4, providing a fresh method to address agricultural concerns.
● The study achieves high precision in recognizing and classifying various plant leaf diseases through thorough image annotation and data preprocessing. The suggested methodology’s robustness provides accurate disease diagnosis, assisting farmers and researchers in timely intervention and crop management.
● The study uses the widely accepted Plant Village dataset, which helps validate and benchmark the proposed model. This dataset selection improves the generalizability of the findings, allowing for more excellent applications in plant pathology research.
● The research provides valuable insights into the practical implementation of the YOLOv4 architecture for detecting plant leaf disease. The precise methodology can help researchers and practitioners implement and adapt this technology in real-world agricultural contexts.
The fundamental structure of this paper is as follows: An overview of the topic is given in the first part. The literature on diagnosing leaf diseases is reviewed in the section 2. The dataset structure and the evaluation criteria for the experiments are presented in the 3 section. The methodology portion is covered in section 4, and a comparison of the findings is covered in section 5. The discussion portion is covered under Section 6. Finally, a summary concludes in section 7, where the paper is closed up.
2 Literature review
In the context of precision agriculture, there is a strong need for sophisticated techniques that improve detection efficiency because plant diseases pose significant risks to crop health and the field ( Attallah, 2023 ; Rahman et al., 2023 ; Thangavel et al., 2023 ). In computer vision for plant disease identification, deep learning—specifically, the YOLOv4 architecture—has proven to be a potent tool. Deep learning holds great potential to overcome obstacles associated with various illness kinds and environmental variables ( Javidan et al., 2023 ). Previous research has demonstrated the drawbacks of standard approaches. The release of YOLOv4 improves object identification speed and accuracy, which makes it an excellent option for applications in plant pathology ( Singh et al., 2017 ). An in-depth analysis of the current research environment is provided by this literature review, which sets the stage for a later investigation into the use of YOLOv4 in identifying and detecting plant leaf diseases ( Perveen et al., 2023 ).
By addressing the fundamental restrictions that are present in the approaches that are currently in use, Peng et al ( Peng and Wang, 2022 ). have made great achievements in the advancement of plant disease identification. Predefined recognition categories and the significant demand for image annotation are two examples of these restrictions that are particularly difficult to overcome. The authors have methodically separated the PlantVillage dataset into two sub-datasets, which they have referred to as PlantVillage-A and PlantVillage-B. This allowed them to use the PlantVillage dataset as a solid foundation for training and evaluation. Remarkable accuracy rates of 98% for YOLOv5 and 97.84% for ResNet were achieved by their proposed strategy, which indicates a significant change from the standard approaches that have been historically utilized. Not only does this achievement serve as a testimonial to the effectiveness of the technique in minimizing the inadequacies of previous methodologies, but it also serves to enhance the precision and adaptability of leaf disease identification. A full re-evaluation of recognition categories and an improved method to picture annotation are both components of the strategy that has been presented.
Further, Chen et al. (2022) introduced an improved model based on the YOLOv5 network to precisely identify diseases in the difficult conditions of various natural habitats, significantly contributing to plant disease recognition and using the PlantVillage dataset—especially the rubber tree disease database with 2375 images—the suggested model targets anthracnose and powdery mildew. The model achieves an impressive 86.5% accuracy rate, highlighting its usefulness in improving methods for identifying plant diseases.
Moreover, Eunice et al. (2022) address the critical imperative of early plant disease diagnosis for optimizing food production and reducing economic losses. Leveraging the advancements in deep learning, the study introduces a robust leaf disease detection model for agricultural applications. The focus lies on utilizing CNN-based pre-trained models, specifically DenseNet-121 and VGG-16, with meticulous hyperparameter fine-tuning. The PlantVillage dataset, comprising 54,305 samples, serves as the comprehensive foundation partitioned for training, validation, and testing. Impressively, the proposed model achieves an exceptional accuracy of 99.81%, underscoring its effectiveness in enhancing plant disease identification accuracy and its potential for substantial impact on agricultural practices and crop management strategies ( Hassan et al., 2021 ; Chen and Wu, 2023 ; Soeb et al., 2023 ; Xinming and Tang, 2023 ).
Likewise, Chuanlei et al. (2017) contributed to the critical domain of apple leaf disease identification within computer vision, addressing the challenge of adequate representation of diseased leaf images. Leveraging the PlantVillage dataset, comprising ninety images, the study employs a methodology centred on a Support Vector Machine (SVM) classifier. Impressively, the proposed model achieves an accuracy surpassing 95% on the PlantVillage dataset, showcasing the efficacy of the developed apple leaf disease recognition method ( Arathi and Dulhare, 2023 ; Bin Naeem et al., 2023 ; Xu et al., 2023 ). This work holds substantial promise for advancing computer vision applications in precision agriculture and plant health monitoring ( Vengaiah and Konda, 2023 ; Terentev et al., 2023 ; Liu et al., 2023 ).
Further, Kaur et al. (2022) contributed significantly to the imperative task of plant disease identification for sustainable agriculture. Acknowledging the challenges of manual monitoring, the research focuses on grapevines, targeting four prevalent diseases: Leaf blight, Black rot, stable, and Black measles ( Taheri-Garavand et al., 2021 ; Sharma et al., 2023 ). With the overarching need for automated disease characterization in agriculture, the study utilizes the PlantVillage dataset, emphasizing grapevine health with 2115 images. The proposed Hybrid Convolutional Neural Network emerges as a robust solution, achieving a remarkable accuracy of 98.7%. This work stands at the forefront of precision agriculture, offering a comprehensive approach to automatic and accurate leaf disease recognition in grape plants ( Mustafa et al., 2020 ).
Moreover, Jasim et al ( Jasim and Al-Tuwaijari, 2020 ). contributed to precision agriculture with their research using Image Processing and Deep Learning Techniques. The study leverages the comprehensive Plant Village dataset comprising 20,636 images to address the critical task of detecting and classifying plant leaf diseases. Further, it Employs deep-learning techniques, particularly Convolutional Neural Networks (CNN), where the research advances the state-of-the-art in disease identification ( Liu and Wang, 2021 ). Using CNN allowed for the classification of 15 distinct classes, encompassing various diseases such as bacteria and fungi, along with a category for healthy leaves. The proposed model attained an accuracy of 90.029%, underscoring its efficacy in the automated detection and classification of plant leaf diseases, thereby contributing significantly to advancing precision agriculture methodologies.
To sum up, this study has advanced plant pathology significantly. The study effectively solves the crucial need for precise and effective plant leaf disease detection and classification using the YOLOv4 architecture. The research shows that picture annotation, data preparation, and model training, that is used to diagnose various diseases through creative integration and rigorous methods reliably. High precision is achieved with the help of the YOLOv4 model, demonstrating the model’s potential for practical application in agriculture. This approach facilitates prompt interventions and sustainable crop management practices, contributing to the evolving field of precision agriculture.
Table 1 contains a list of previous references along with plant types, techniques, limitations and outcomes.
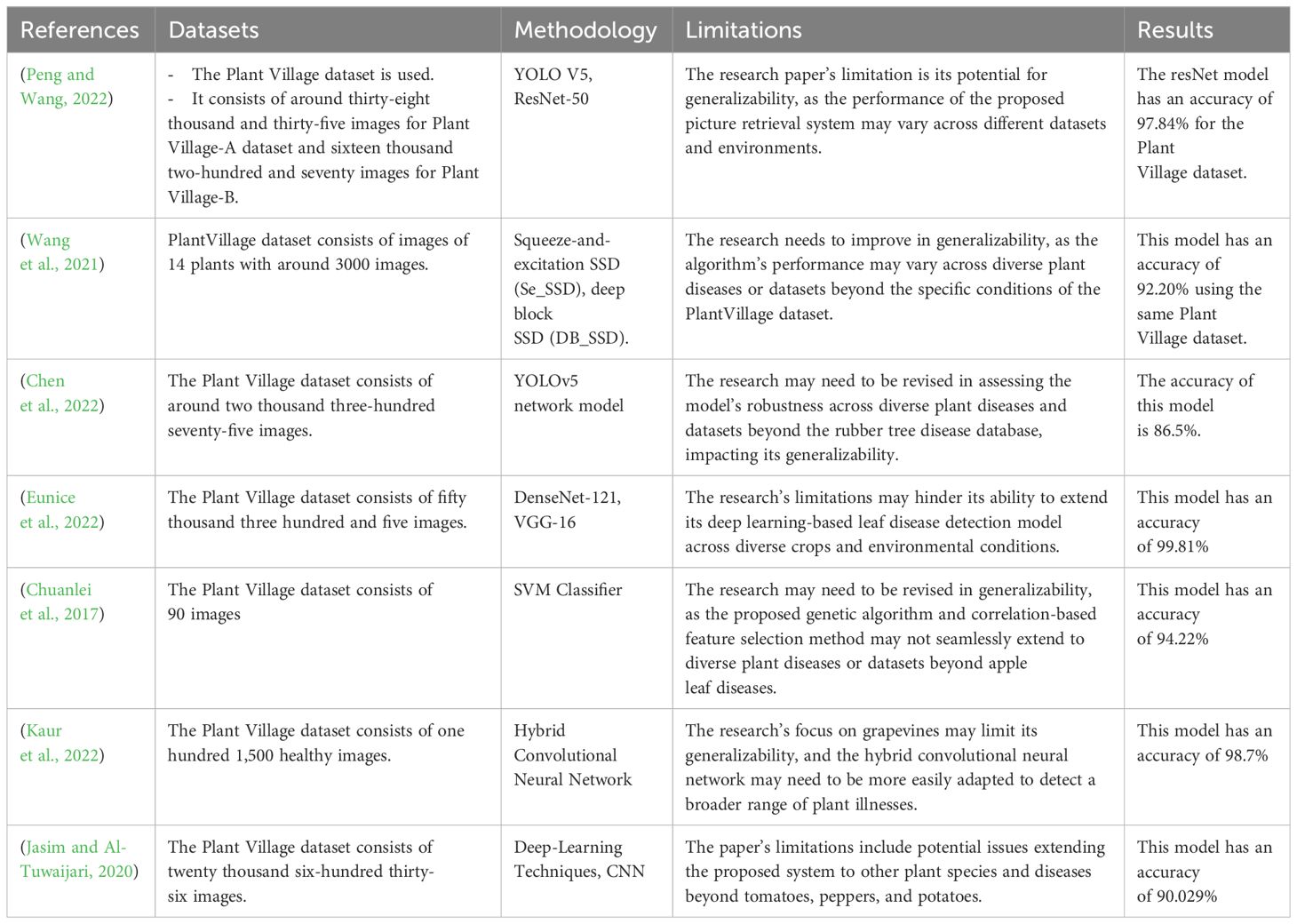
Table 1 List of past references with datasets, methodology, and results.
The review concludes by highlighting the development of plant disease identification approaches and highlighting the usefulness and accessibility of YOLOv4 in addressing the drawbacks of conventional methods. Molecular techniques, hyperspectral imaging, and traditional computer vision have all been necessary. However, YOLOv4 presents a viable path toward precise and effective illness detection. Its versatility and intuitive interface offer prospects for broad implementation in farming environments. With the advancement of technology, crop health monitoring can be improved by incorporating YOLOv4 into plant disease control strategies, which will increase agricultural output and sustainability.
3 Data collection
Over 50,000 images of healthy and diseased plant leaves from 14 distinct plant species are included in the publicly accessible image resource called “Plant Village.” The dataset was produced to help develop computer vision algorithms for plant disease identification on an automated basis.
Each image in the dataset is annotated with the corresponding plant species and whether a disease is present. The pictures in the collection were taken using a smartphone camera, and they come in various lighting, backgrounds, and orientations.
The Plant Village dataset, compiled by scholars at Pennsylvania State University, is accessible for download on the official website. Utilized in a number of programs and studies to identify plant diseases, the dataset has contributed to the advancement of research in this field.
Datasets for strawberries, tomatoes, and potatoes are gathered from the plant village dataset. The datasets for mango and bean were collected from the website.
3.1 Data description
Each dataset is divided into two primary categories: healthy and diseased. As shown in Table 2 , additional categories exist in the disease dataset for each Plant leaf.
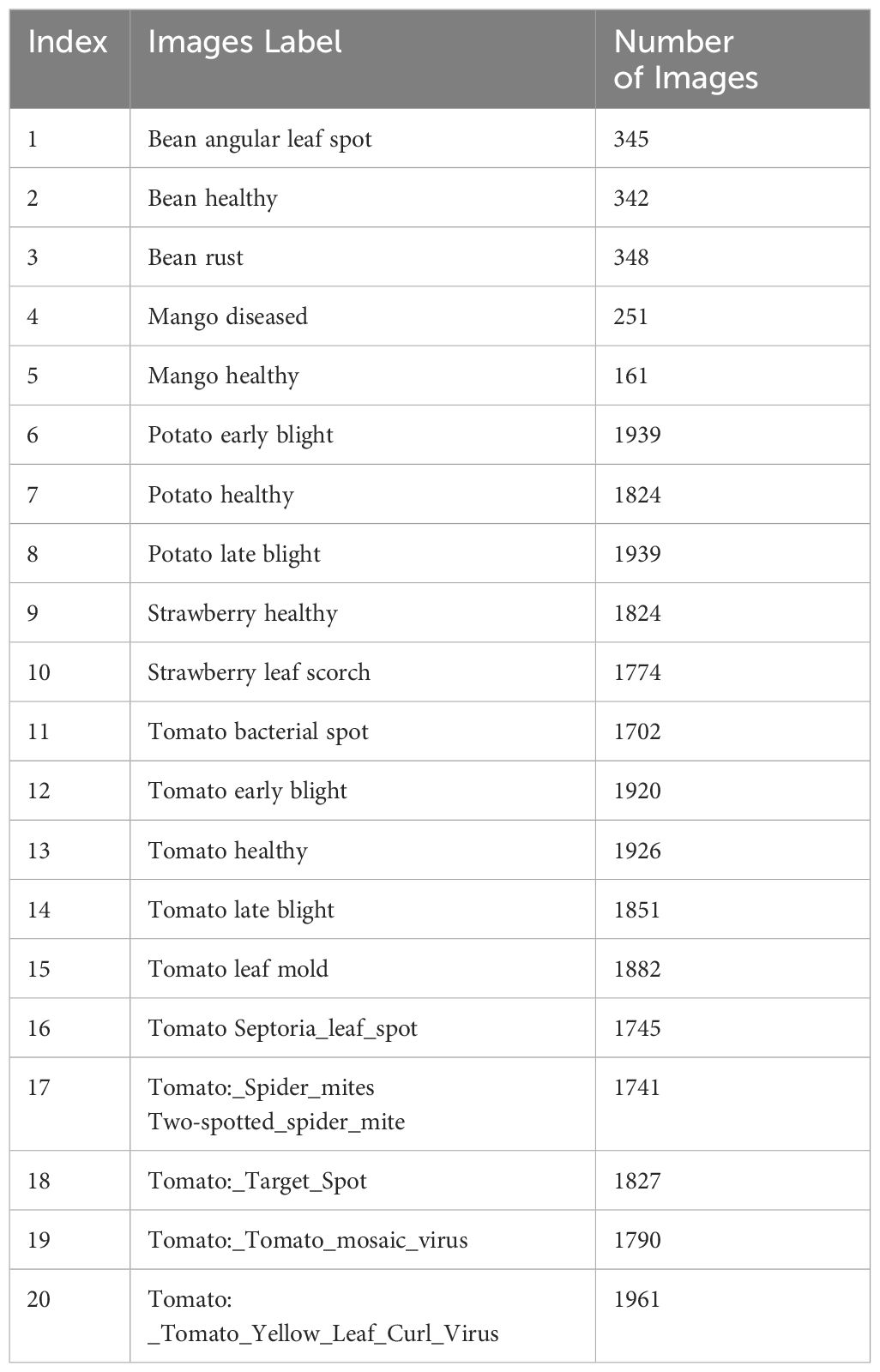
Table 2 Plants images classification.
This collection includes five groups of images, and the disease is catalogued in 20 volumes. The leaf disease datasets of some fruit and vegetable plants are more comprehensive than those of others, which are only healthy or diseased. Figure 2 shows how the distribution of the dataset is represented visually:
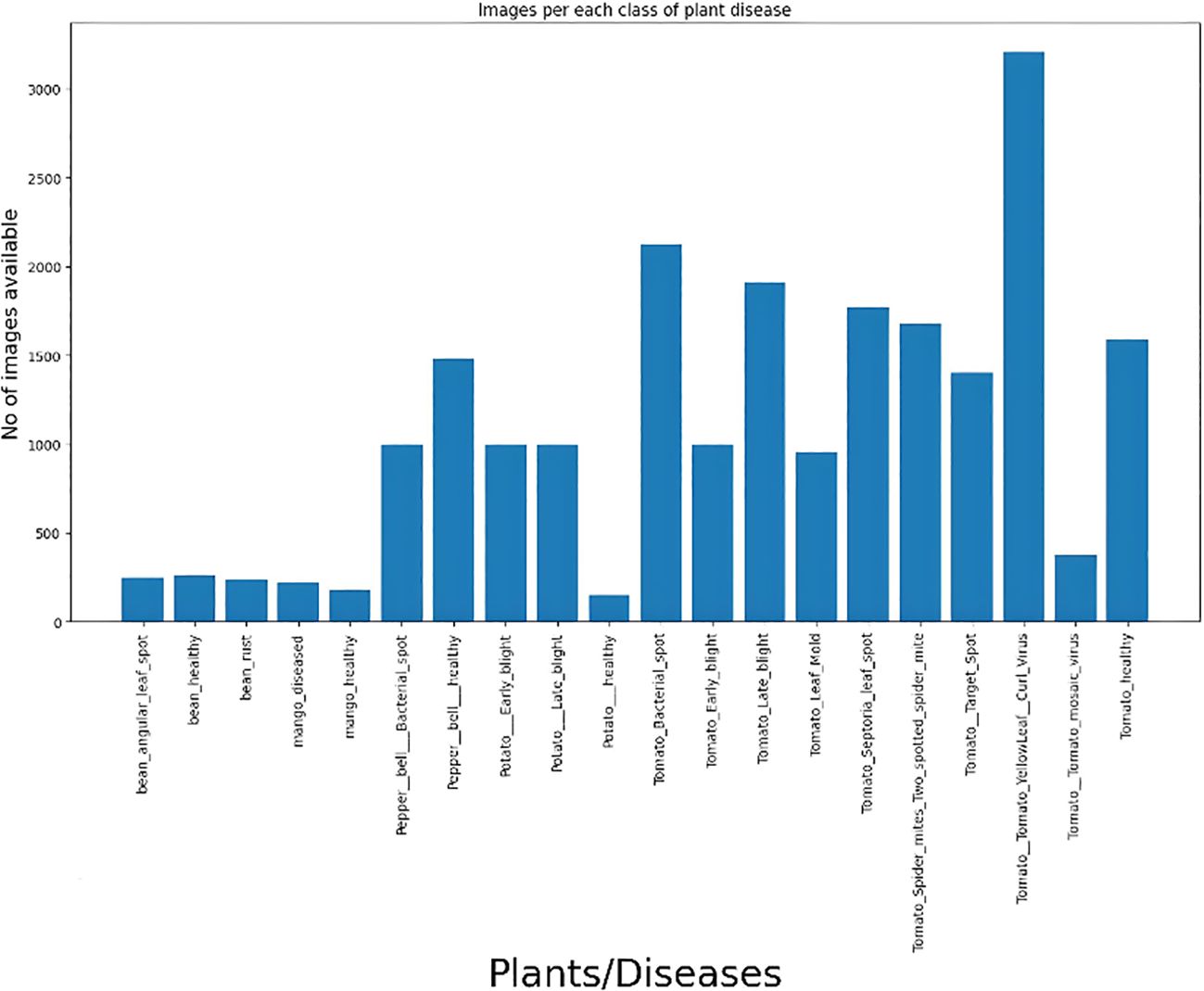
Figure 2 Image size distribution.
3.2 Data visualization
Visualising the image of each fruit is an essential pre-data preparation phase in this process. The integration of widely recognized and popular datasets is simplified by the torch-vision.datasets class, which also simplifies the process for unique datasets. Making use of the torch-vision.datasets subclass. ImageFolder enhances the efficiency of image data importing by organizing the data beforehand. After the data has been imported, it is crucial to normalize the data in order to improve its suitability for neural networks. Normalization is the process of transforming pixel values from the range (0,255) to (0,1) for each image. By dividing each value by 255, this normalization procedure generates a torch tensor from the entire array of pixel values. The underlying reasoning for normalizing inputs is illustrated in Figure 3 , which utilizes a plant leaf to demonstrate how this methodology improves the performance of neural networks.
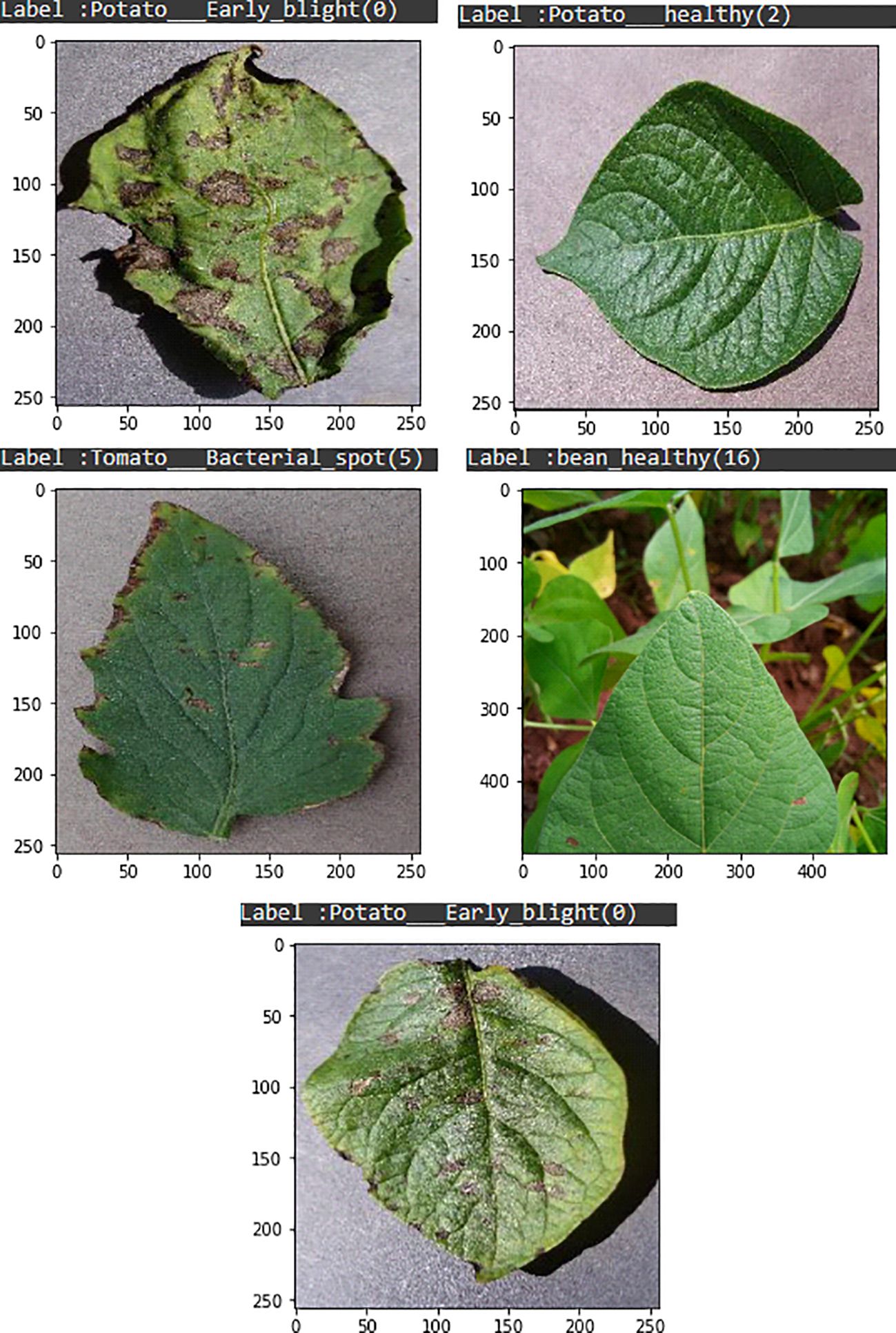
Figure 3 Plants leaf visualization.
3.3 Images annotation
To characterize an image’s contents or qualities, metadata or labels are typically added to the image. It is frequently done to teach computer vision algorithms to recognize particular objects, characteristics, or properties inside an image.
Various Information can be added to an image by image annotation, including text descriptions, polygons, points, and bounding boxes around objects. In contrast, polygons can be used to define an object’s shape and bounding boxes are frequently used to identify and pinpoint the locations of objects within an image.
Image annotation is crucial in many ML applications, including object identification, image classification, and image segmentation. Developers can train ML models to spot image patterns by annotating a dataset. This technology can be applied to various tasks, such as facial recognition, self-driving cars, medical imaging, etc.
3.3.1 Process for detecting plant diseases from images
3.3.1.1 bounding box.
oRectangular bounding boxes are employed to enclose targeted portions of the plant, with particular emphasis on affected areas like leaves.
oThe green hue for these bounding boxes accentuates the segmentation of leaves and facilitates the visual differentiation of annotated regions.
3.3.1.2 Segmentation and disease identification
oSegmentation entails dividing the image into smaller pieces and assigning labels to indicate the existence or non-existence of illness.
oThe presence of green colour-bounding boxes on leaves aids in the segmentation process, enabling accurate detection of disease-affected regions on the plant.
3.3.1.3 Plant feature annotation for landmarks
oLandmark annotation entails identifying and labelling significant points on the plant, such as the lowermost part of the stem, the leaves’ ends, or the fruits’ placements.
oThe presence of green-coloured bounding boxes aids in precisely identifying these landmarks on leaves, hence offering intricate details about distinct plant characteristics.
3.3.1.4 Annotation of attributes for detailed information
oAttribute annotation involves assigning labels to photos that provide Supplementary Information about the plant, such as its species, the specific illness, and the degree of severity.
oBy employing green color-bounding boxes, the annotations effectively capture distinct characteristics on leaves, hence augmenting the dataset’s informational depth.
3.3.1.5 Image tagging for categorization
oImage tagging is assigning descriptive tags to photos to classify them according to attributes such as illness type, location, or plant species.
oGreen colour-coded bounding boxes enhance the precision of picture tagging, streamline the classification of images, and optimize the organization of the dataset.
3.3.1.6 Training of machine learning (ML) algorithms
oPrecise picture annotation, which involves the application of green colour-bounding boxes on leaves, allows machine learning algorithms to acquire knowledge about specific symptoms and patterns linked to different plant illnesses.
oThe acquired expertise enables ML models, like the YOLOv4 model, to accurately categorize novel photos for disease diagnosis.
Employing the OpenCV bounding box method, including green-coloured bounding boxes specifically on leaves, guarantees a targeted and visually discernible marking of areas impacted by illness. The rigorous procedure of annotating significantly enhances the efficacy of training models for plant disease identification. As seen in Figure 4 , which also displays the image number, disease kind, and output images, the output images are as follows.
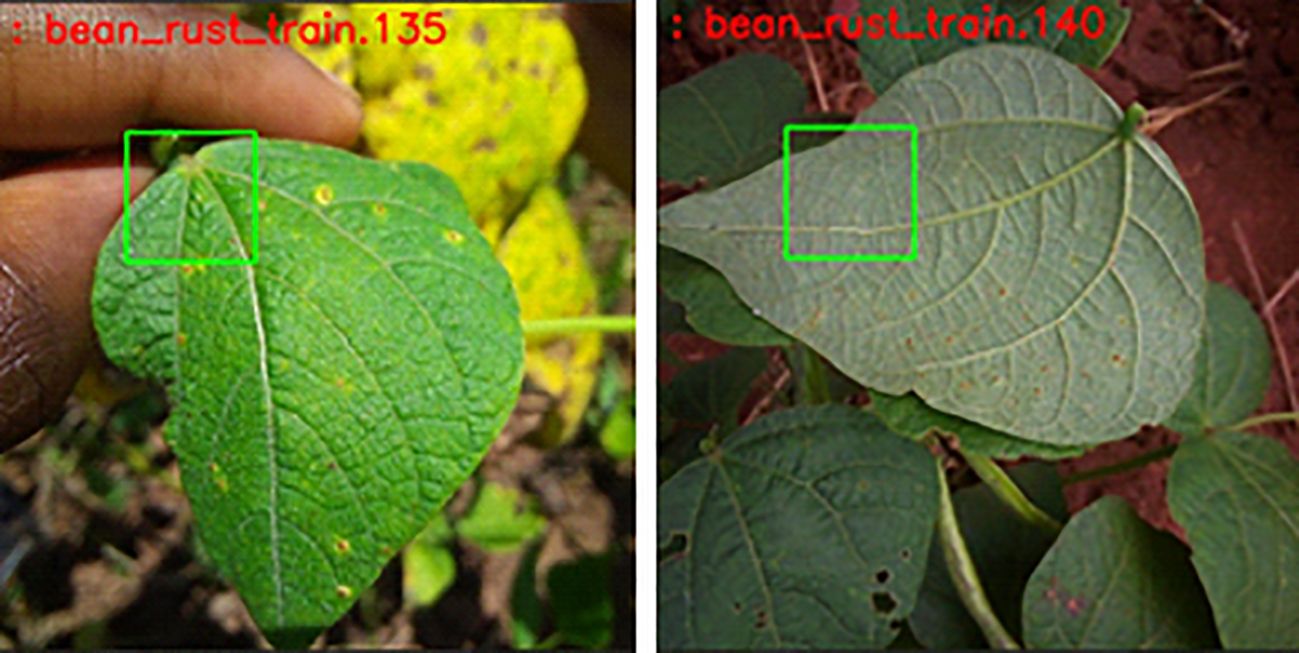
Figure 4 Images annotation.
4 Model design
4.1 yolo v4.
Modern real-time object identification technology is used by YOLOv4 (You Only Look Once, Version 4). It was created by a research team at the University of Washington under the direction of Alexey Bochkovskiy and is an upgrade over its forerunner, YOLOv3.
With single-pass image processing and real-time prediction of bounding boxes and class probabilities for several objects, YOLOv4 uses DNN architecture. To do this, the image is divided into a grid of cells, and each cell’s likelihood of containing an item, its bounding box coordinates, and class probabilities are predicted. To increase the precision and speed of object recognition, YOLOv4 employs some cutting-edge approaches, including weighted residual connections, mish activation functions, and spatial pyramid pooling.
On some object detection benchmarks, including COCO and KITTI, YOLOv4 has achieved cutting-edge performance. It is widely employed in many applications, such as robots, self-driving cars, and surveillance. Since the YOLOv4 code is open-source and accessible on GitHub, developers can use and alter it for their projects.
4.2 Yolo V4 custom training
The critical steps in developing a bespoke YOLOv4 model include the preparation of the dataset, the determination of training parameters, and the implementation of model training. A concise overview of these methodologies is provided below:
4.2.1 Prepare the dataset
Creating a dataset with labeled photos is the initial stage. Bounding boxes, class labels, and images of the items that one wants to detect must all be included in the dataset. Applications such as VoTT, LabelImg, and YOLOv4 Label can be utilized to annotate the data.
4.2.1 Generate the YOLOv4 configuration file
Constructing a YOLOv4 configuration file that specifies the model architecture, hyperparameters, and training settings is the following step. The default configuration file from the YOLOv4 repository may be modified to meet specific requirements.
4.2.3 Download pre-trained weights
One potential method for streamlining the training process is to utilize pre-trained weights designed for the YOLOv4 architecture. The Darknet framework, which YOLOv4 employs, possesses pre-trained weights.
4.2.4 Train the model
After obtaining the configuration file and the dataset, the model can start to be trained. The YOLOv4 model can be introduced on a special dataset using the Darknet framework. Throughout training, the model will improve its recognition of the objects in the dataset.
4.2.5 Evaluate the model
After training on a validation dataset, the model’s performance is evaluated. Metrics like accuracy, precision, recall, and F1-score is used to assess the model’s performance.
4.2.6 Test the model
It can then be tested on a test dataset to evaluate its performance with brand-new, untested data. Moreover, our research utilized a data splitting scheme of 90% for training and 10% for testing. Specifically, 90% of the training set was designated for training purposes, while the remaining 10% was employed for validation. These proportions guarantee rigorous training and evaluation of the model.
Moreover, due to the heavy computational tasks involved, training a GPU model takes a lot of resilience. During training, the GPU does a huge number of complicated calculations over and over again, going through huge datasets to find the best settings for the model. For each epoch, the GPU has to constantly process and update millions of factors, making changes to them to make the model more accurate. The need for endurance comes from the fact that training sessions can last for hours, days, or even weeks, based on the size and complexity of the dataset. Keeping a steady level of steadiness and computational performance over long periods of time is therefore necessary to make sure that a high-quality YOLOv4 model is trained well Figure 5 .
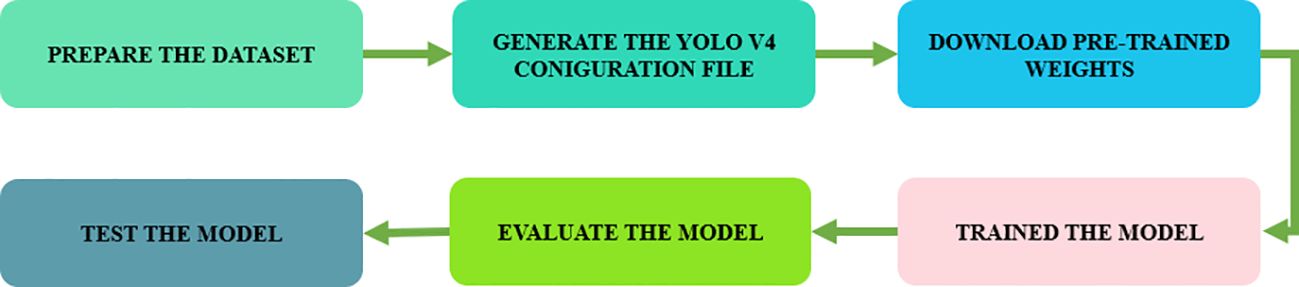
Figure 5 Flowchart for Training a Yolo V4 custom model.
4.3 Revolutionizing plant disease detection with YOLOv4
This multidisciplinary method of disease prediction, especially with reference to plant leaf diseases utilizing YOLOv4, integrates remote sensing and statistical regression approaches through the use of breakthroughs in computer vision, agriculture, and data science. The data that remote sensing instruments, such as satellite and drone imagery, give for monitoring crop health and spotting disease outbreaks can be beneficial to large-scale agricultural areas. With the use of these approaches, high-resolution pictures can be obtained in order to detect any minute changes in a plant’s characteristics that might point to a disease.
Statistical regression techniques are critical for data analysis, trend identification, and the establishment of links between various environmental factors and sickness prevalence. Regression models can be used to quantify the impact of temperature, humidity, and soil conditions on the spread of plant diseases. Scientists have a comprehensive understanding of the complex interaction between environmental factors and the dynamics of sickness through the combination of multiple methodologies.
YOLOv4, a state-of-the-art object recognition method in computer vision, is being used to identify plant leaf disease. YOLOv4 is a fantastic real-time image processing program that locates and recognizes objects in pictures with exceptional accuracy. Its accuracy, quickness, and ability to recognize multiple items at once make it a dependable choice for plant disease diagnosis in agricultural contexts.
Compared to earlier methods, YOLOv4 offers improved efficiency and accuracy in identifying plant leaves afflicted with disease. Its ability to recognize objects allows it to identify areas impacted by disease, which makes it possible to implement more targeted intervention strategies. The real-time processing capabilities of YOLOv4 enable prompt action to halt the spread of illnesses and enhance disease detection speed.
A complete and novel approach to plant leaf disease detection is provided by combining YOLOv4, statistical regression, and remote sensing. The benefits of these methods can be connected to improving disease prediction and control in agriculture in terms of timeliness, accuracy, and efficiency.
4.4 Yolo V4 for plant disease detection
4.4.1 image annotation.
The first step is to annotate images by the disease of the particular leaf. Annotated images are as follows in Figure 6 :
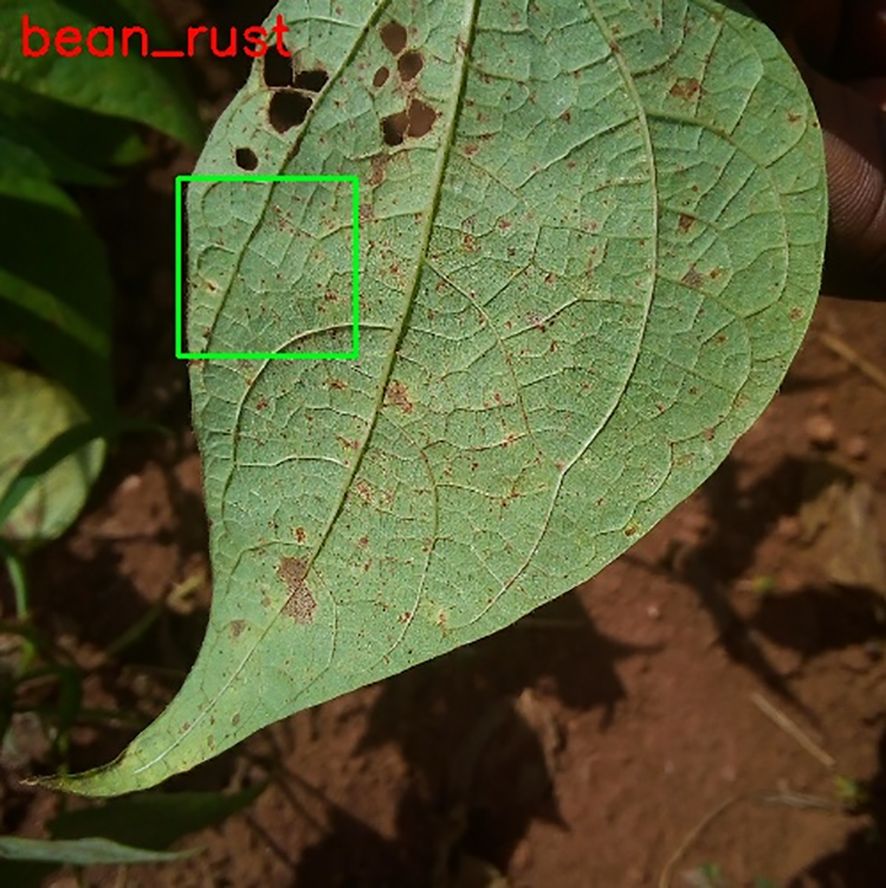
Figure 6 Annotated image.
Image labeling is a laborious and time-consuming undertaking that demands undivided attention in order to individually label each image. This procedure is accelerated through the use of a Python iteration, which facilitates the management of numerous images simultaneously. A thorough examination of the images enabled the accurate determination of bounding box ratios. Despite sincere endeavors, it is widely recognized that accurate annotations are crucial for preserving the integrity and dependability of the dataset in preparation for subsequent scientific investigations and research. Ensuring accurate annotation of images are crucial for preserving the practicality and integrity of academic projects.
4.4.2 Images labels
It is critical to bear in mind that labels must be created for the images. The name of the folder should specify the type of malady or food contained within. The Python code executing the procedure is displayed in Table 3 .
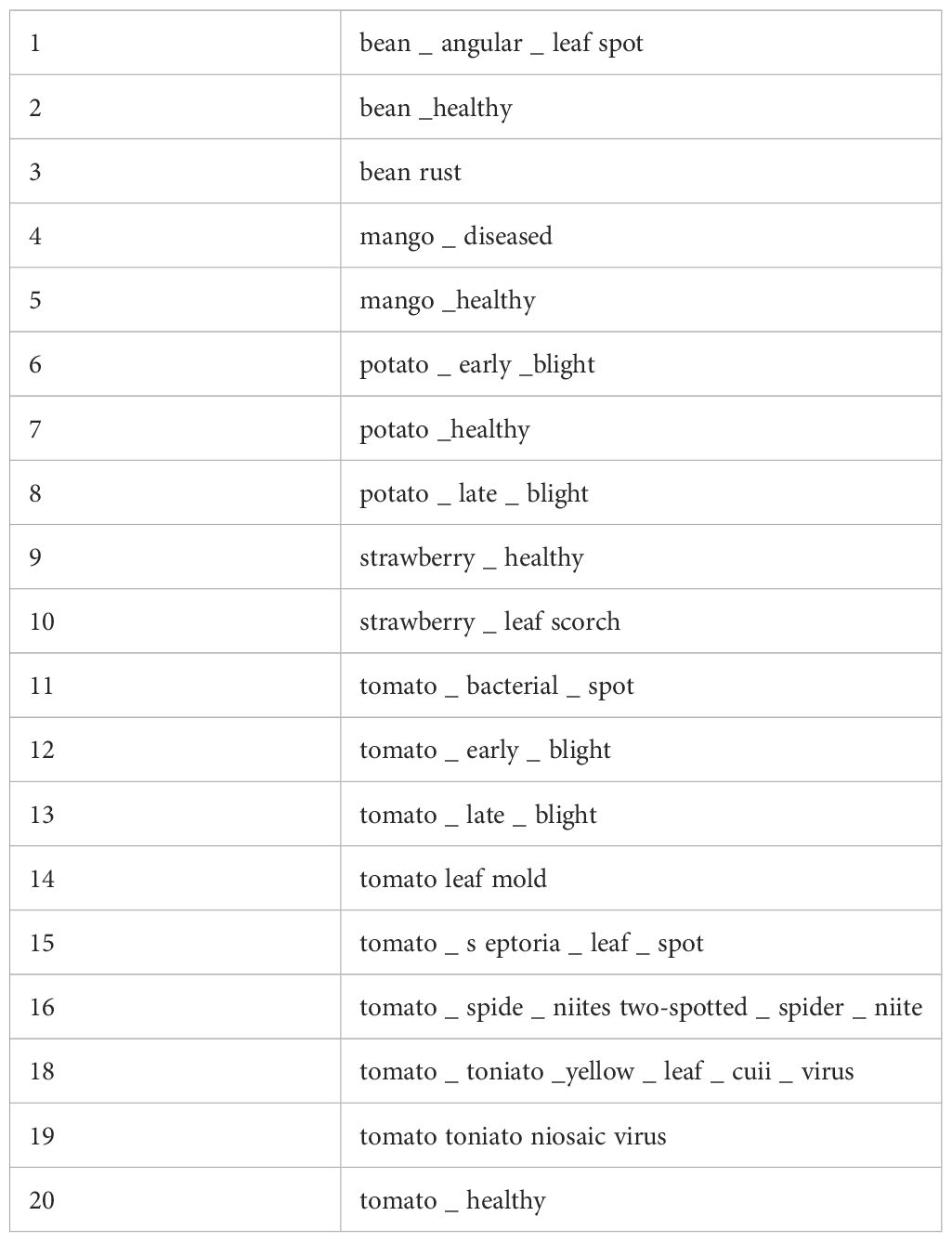
Table 3 Plants leaves labels.
4.5 Object data preparation
The next step is to store the number of classes, train and test text file path, and backup folder training in the text file, which will be used to generate image paths and train those images in Yolov4 custom training.
● classes = 20
● train = data/train.txt
● valid = data/test.txt
● names = data/obj.names
● backup =/mydrive/yolov4/training
4.6 Generate the Yolov4 configuration file
To generate the YOLOv4 custom file, obtain the original YOLO-custom configuration file from the Darknet repository. Personalize this file by altering specific attributes. Modify the number of classes in all YOLO layers to 20, and fine-tune the number of filters in the final convolution layer preceding the YOLO layer to 75. These modifications customize the arrangement to the unique demands of the desired object detection assignment. After making the necessary modifications to the class count and filter configuration, the customized YOLOv4 file is now prepared for implementation. These changes have been made to enhance the performance of the file in the intended application.
4.6.1 Darknet
The Darknet neural network framework was created by Joseph Redmon. It is based on C/CUDA and is utilized in computer vision applications for object identification and image classification. Because of its speed, efficiency, and versatility, the darknet has gained popularity in both business and academic circles. Due to its open-source nature and ease of use, a wide range of users can utilize the framework. Darknet is a very useful tool with a wide range of applications since it can identify items and categorize photographs. The structure of the software is both practical and versatile, which greatly contributes to its wide usage. Darknet is a very powerful computer vision tool that performs exceptionally well on challenges using neural networks. Because of this, it is a very useful tool for practitioners and scholars in the field.
4.6.2 Customizable network architecture
Darknet allows users to define and train their neural network architectures, which can be optimized for specific computer vision tasks. This flexibility is beneficial when pre-trained models do not perform well or when a new application requires a unique architecture.
4.6.3 Support for multiple platforms
Darknet can be run on various platforms, including Linux, Windows, and MacOS. Additionally, it can be compiled to run on GPUs, which significantly speeds up training and inference times.
4.6.4 Integration with OpenCV
Darknet is designed to work seamlessly with OpenCV. This popular computer vision library provides a range of image processing and analysis functions. This integration allows users to easily preprocess images and extract features for their neural network models.
4.6.5 Pre-trained models
Darknet provides pre-trained models for various computer vision tasks, including object detection and image classification. These models can be used as a starting point for new applications or fine-tuned for specific use cases.
Overall, Darknet is a robust computer vision framework that offers flexibility and speed. Its popularity in the research community and industry is a testament to its effectiveness, and it continues to be widely used and developed today.
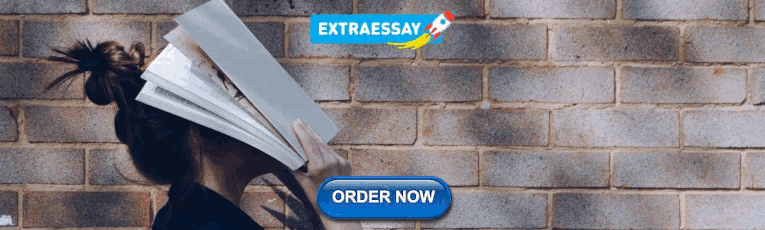
4.6.6 Cloning
To clone DarknetDarknet for custom YOLO v4 training, follow these steps:
4.6.7 Install Git
If Git is not installed on the system, install it from the official Git website.
4.6.8 Clone Darknet
Open a terminal window and navigate to the directory where DarknetDarknet is to be cloned. Then, enter the following command to clone the Darknet repository:
- git clone https://github.com/AlexeyAB/darknet.git .
4.6.9 Yolo V4 custom weights
Download Pre-Trained YOLO v4 Weights:
To use YOLO v4, the pre-trained weights need to be downloaded. This can be done by running the following command from within the Darknet directory:
https://github.com/mzakariah/plant-disease-detection-using-yolov4 .
4.7 Yolo V4 training
4.7.1 customize configuration files.
In the Darknet directory, navigate to the CFG folder. Here, several configuration files for different YOLO versions can be found. For YOLO v4, the “yolov4.cfg” file should be used as a starting point and customized to suit the needs.
4.7.2 Prepare training data
To train a custom YOLO v4 model, the training data must be prepared in the YOLO annotation format. This involves creating text files for each image that contain the object annotations and their corresponding class labels in Supplementary Table 1 .
5.1 Train the model
Formulating and training a novel YOLOv4 model to precisely identify and detect diseases in plant leaves was the principal aim of this investigation. Both gathering training data and modifying configuration files were critical components of the training method. Table 4 provides a detailed description of the YOLOv4 training parameters utilized in this investigation. Notably, substantial assistance from the Darknet directory was used in the training process.
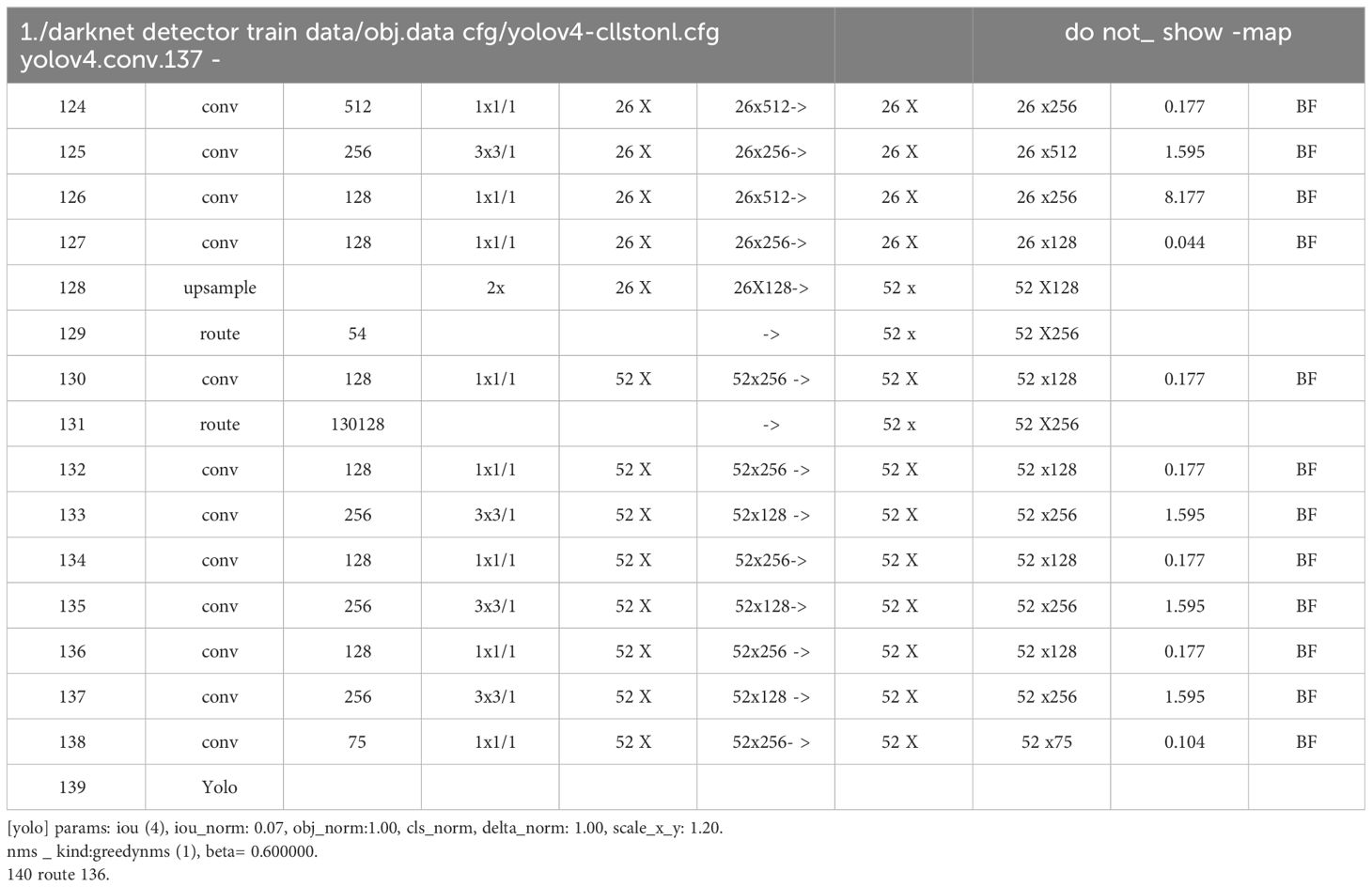
Table 4 Yolo V4 training.
Using a batch size of 64 and 16 subdivisions, the YOLOv4 model exhibited efficient learning and optimization. A resolution of 416 by 416 pixels characterizes the input image. During the training process, the learning rate was maintained at 0.001, which promoted the convergence of the model. To ensure a well-generalized model and prevent overfitting, the training procedure was confined to a batch limit of 6.0. After the YOLOv4 model underwent practical training, additional functions were assessed through further testing. The testing process incorporated a wide range of plant leaf photographs showcasing different symptoms of diseases. The algorithm computed the subsequent metrics—accuracy, precision, recall, and F1 score—to evaluate the model’s resilience to obstacles and its capacity to implement acquired knowledge in novel circumstances. The ability of the model to precisely identify and localize plant leaf diseases was fundamental to its practical efficacy. Using metrics that are frequently applied to object detection models, the outcomes of the model’s assessment on a separate test dataset were analyzed. It was evident that the YOLOv4 model exhibited superior performance in identifying and detecting plant leaf diseases when compared to established benchmarks.
Furthermore, by ensuring transparency and replicability via stringent protocols and standards, the groundwork is laid for subsequent developments in precision agriculture research and implementation strategies.
5.2 Evaluation metrics
The YOLOv4 model, designed for detecting and identifying plant leaf diseases, performs excellently in essential evaluation criteria. The model demonstrates exceptional precision in discriminating between damaged and healthy plant leaves. The model’s accuracy of 0.99 indicates its strong capability to identify instances accurately. In contrast, a precision of 0.99 means the minimal occurrence of false positives, where healthy leaves are mistakenly recognized as diseased.
Furthermore, the model attains a recall rate of 0.99, demonstrating its high level of competence in accurately identifying most confirmed sickness cases. The F1 score, a composite measure of precision and recall, achieves an impressive value of 0.99. The combination of these indicators highlights the YOLOv4 model’s strength and dependability in accurately detecting and classifying plant leaf diseases. The model’s exceptional performance across various measures demonstrates its usefulness and potential for practical use in agriculture and plant pathology. Table 5 shows the values of evaluation metrics, and overall, the performance value of accuracy, recall, f1-score and precision is 0.99.
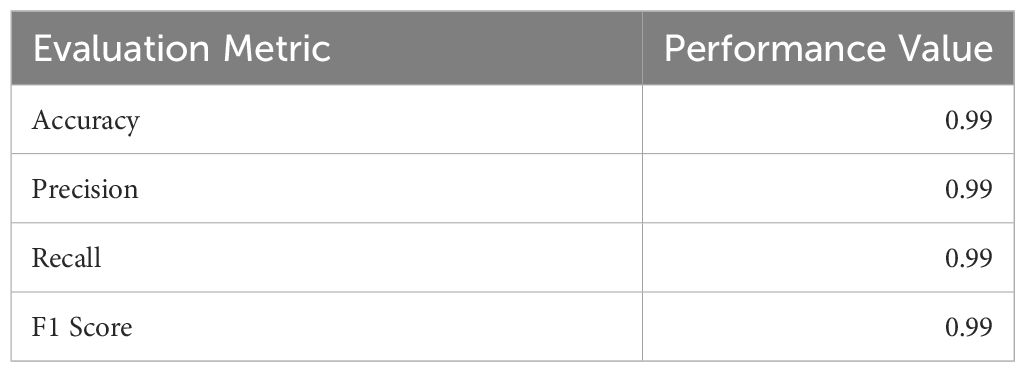
Table 5 Evaluation metrics.
Furthermore, in the framework for detecting plant leaf diseases employing YOLOv4, the confusion matrix is an essential component for evaluating the performance of the model. The matrix provides a comprehensive examination of the model’s forecasts, enabling a meticulous assessment of its efficacy. This matrix incorporates four critical metrics, namely false positives (FP), true negatives (TN), and true positives (TP).
TP denotes instances in which the model detects unhealthy regions on plant foliage with precision. The precise designation for regions devoid of leaf diseases is TN. On the contrary, FP occurs when the model erroneously classifies healthy regions as diseased. In contrast, false negatives (FN) transpire when the algorithm fails to identify diseased sections accurately.
An in-depth summary of the model’s accuracy in binary classification—that is, its ability to discern between diseased plant leaves and those that are healthy—is given by the way these metrics interact in the confusion matrix. The model performs well, as evidenced by significant values of TP and TN, which highlight its capacity to identify both positive and negative cases accurately. Higher FP and FN values, on the other hand, can point to areas that require improvement and highlight potential misclassifications that might compromise the model’s accuracy in actual plant disease detection scenarios. The TN, TP, FN, and FP confusion matrix is a crucial instrument for evaluating the precision and overall efficacy of the YOLOv4 model in the diagnosis of plant leaf diseases.
5.3 Training performance
The graph, which provides the results shown in Figure 7 , generates training performance.
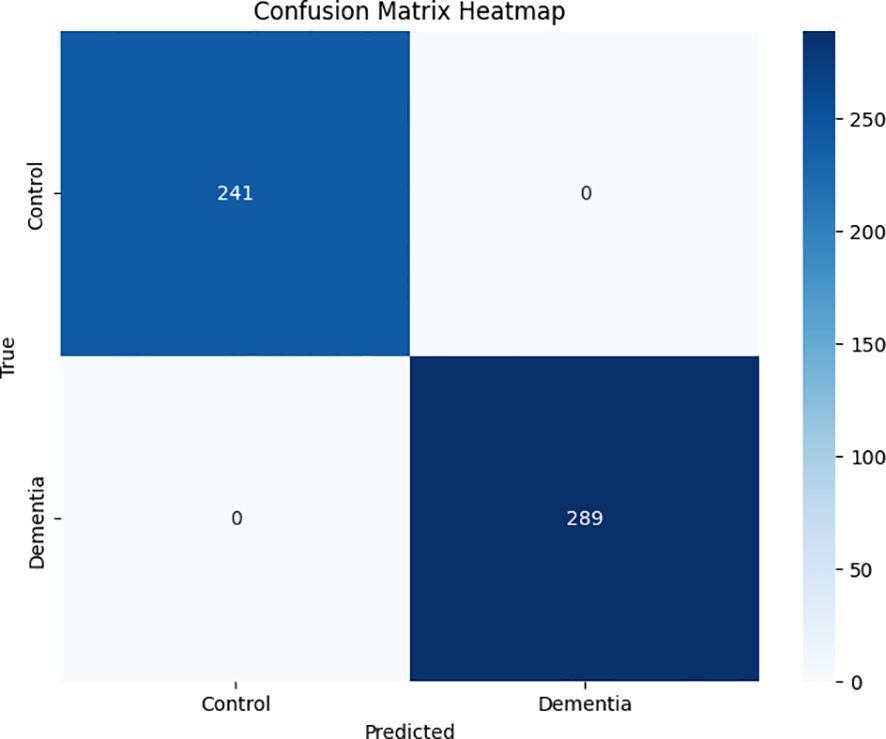
Figure 7 Confusion matrix.
● Where, loss of performance, Normalizer: (i.e., 0.07, obj: 1.00, cls: 1.00) Region 161 Avg (IOU: 0.323502), count: 17, class_loss = 7.521840, iou_loss = 5.702097, total_loss = 13.223937.
● v3 (iou loss, Normalizer: (ie: 0.07, obj: 1.00, cls: 1.00) Region 139 Avg (IOU: 0.000000), count: 11, class_loss = 7.750000, iou_loss = 0.000005, total_loss = 7.750005.
● v3 (iou loss, Normalizer: (iou: 0.07, obj: 1.00, cls: 1.00) Region 150 Avg (IOU: 0.396017), count: 15, class_loss = 6.526371, iou_loss = 26.913261, total_loss = 33.439632.
Moreover, training the YOLOv4 model for plant leaf disease detection and identification requires careful parameter adjustment to achieve the best results. Selecting the right batch size and epochs is crucial for efficient dataset learning. When model accuracy falls short, changes are needed. By adding epochs, the model may train more and possibly recognize complicated visual patterns. By allowing the model to extract relevant dataset features, altering the learning rate can improve performance.
In accordance with the YOLOv4 model configuration, parameters like epochs size, batch size and learning rate are left at their default parameters. These default settings are usually fine for everyday use unless there are unique requirements. By balancing these parameters and making modifications, the YOLOv4 model can learn and detect plant leaf illnesses. To examine, following specifics are presented:
5.3.1 Region, normalizer, and loss specifics
● Loss: This value signifies the inaccuracy or divergence between the model’s predicted output and the true labels present in the ground.
● Normalizer: These values are employed to standardize various loss function components, generally to achieve a balance in the significance of distinct features such as localization, object presence, and classification. It is probable that it pertains to particular areas of interest contained within the input image. The average IOU (Intersection over Union) quantifies the degree of overlap that exists between the ground truth boxes and the predicted bounding boxes. It is utilized to assess the precision of object localization.
● Count: The quantity of identified instances within the specified region.
5.3.2 Dissection of components of loss
● Class loss is the loss that is inherent in the classification assignment, which consists of identifying the class of the detected object (which, in this instance, is the presence or absence of disease on the leaf).
● IOU Loss: This value represents the loss associated with the precision of bounding box forecasts. It evaluates the degree of overlap between the predicted and ground-truth bounding boxes.
5.3.3 Analysis and revision of the results
● The initial entry signifies the identification of 17 instances in Region 161, accompanied by an average IOU of 0.323502. Significant portions of the total loss (12.22) are attributable to class loss (7.52) and IOU loss (5.70).
● The average IOU of 0.00 for the second entry, which appears to be for Region 139, indicates weak localization accuracy. There are eleven in total, and the loss is 7.75, which is driven primarily by class loss due to the insignificance of the IOU loss.
● Region 150 is the subject of the third entry, which has a mean IOU of 0.39 and 15 instances were identified. The considerable IOU loss of 26.913261 contributes significantly to the overall loss of 33.43, which indicates inadequate localization performance despite respectable class loss.
In general, the model appears to be functioning satisfactorily in terms of classification (as measured by class loss). However, certain regions exhibit challenges with localization (as indicated by IOU loss), which could potentially impact the overall efficacy of the leaf disease detection system. Additional refinement and training might be required to enhance the precision of the model, specifically with regard to the accurate localization of diseased regions.
5.4 Model testing
There are numerous techniques to evaluate or forecast the new image. Two strategies were chosen, and Figure 8 illustrates the test image and the test image for prediction, respectively.
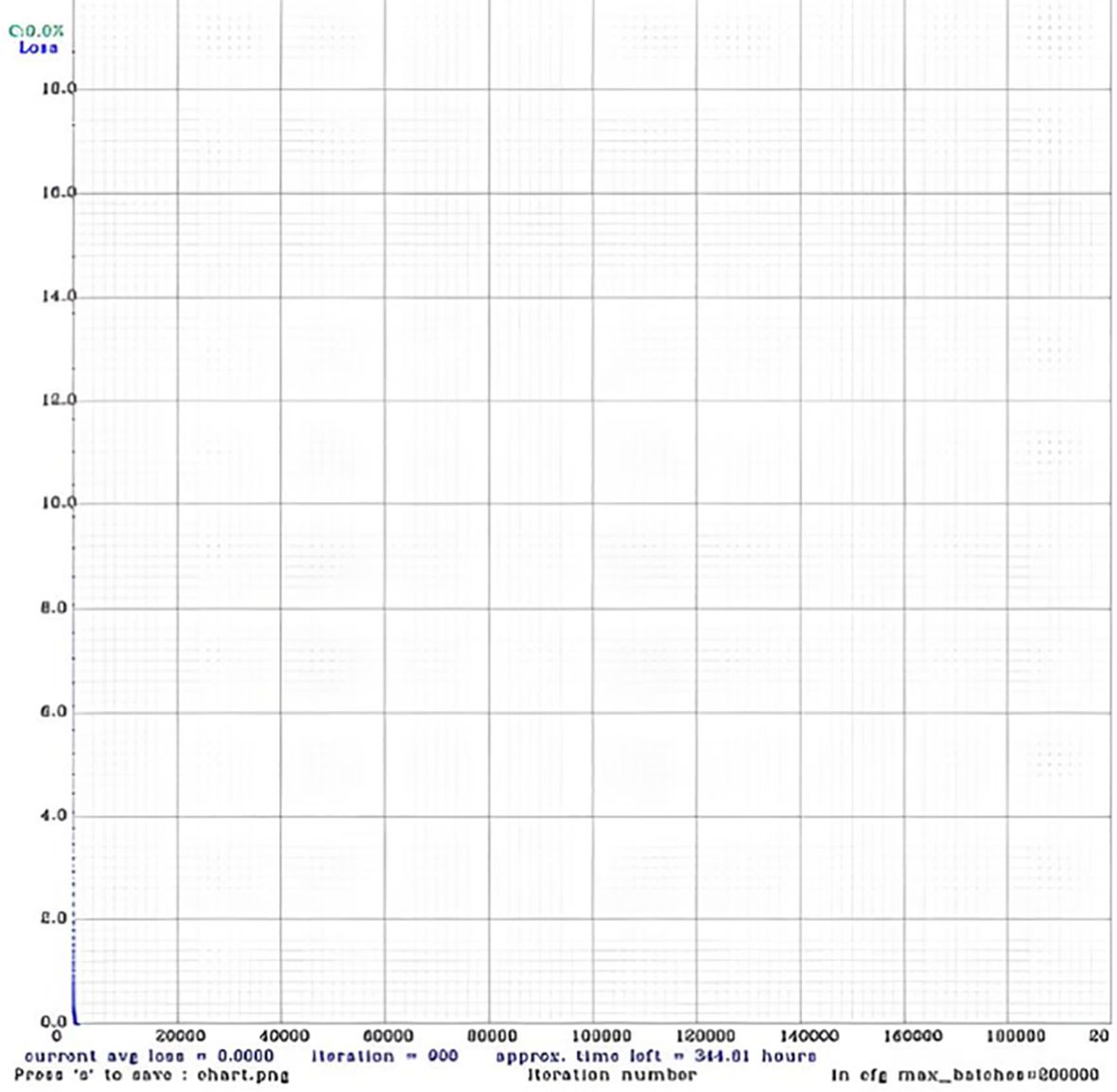
Figure 8 Loss of performance.
An essential test image that is critical for precise prediction in the identification of plant leaf diseases is illustrated in Figure 9 . The accuracy of predictions is significantly impacted by the composition of the dataset, the grade of images, and the precision of annotations. By meticulously annotating high-quality images, model learning is improved, leading to an overall enhancement in performance. A meticulously curated dataset that includes a wide range of scenarios guarantees that the model is both flexible and resilient. The interconnectedness of these components emphasizes the significance of accurate model performance, high-quality images, and precise annotations in order to achieve the most favorable outcomes in predicting plant leaf diseases.
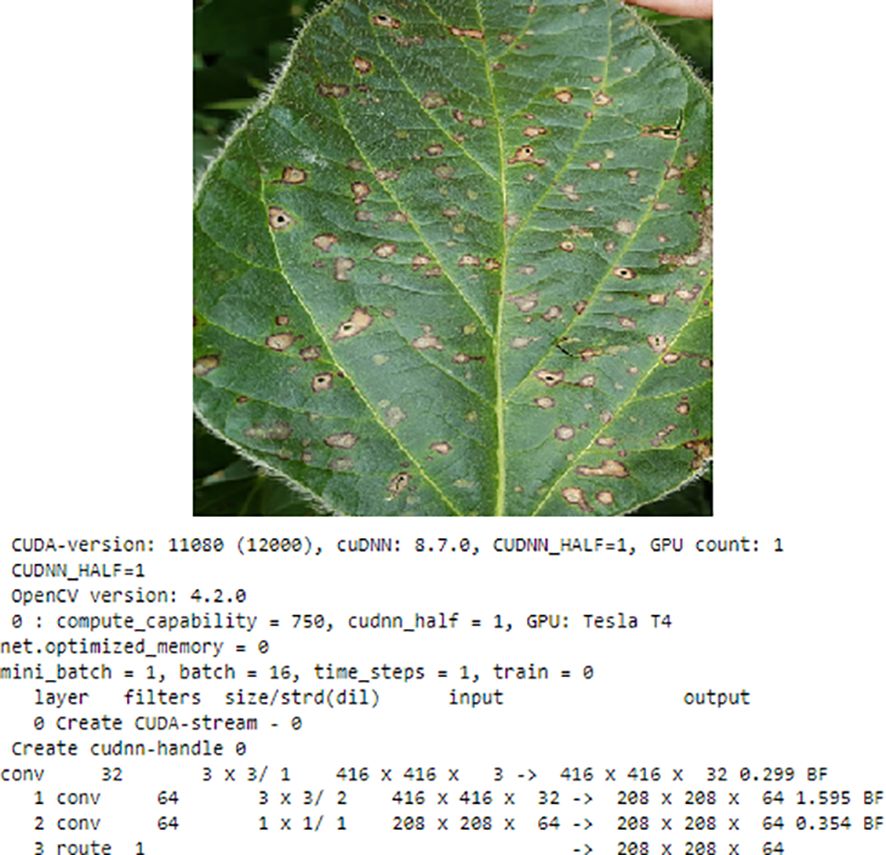
Figure 9 Live webcam snapshot for testing.
5.5 Model evaluation
The objective of this segment is to assess the effectiveness of our model by employing test images that were generated from the data. The methodology entails the implementation of a trained model for the purpose of forecasting plant leaf maladies, followed by an assessment of the predictions’ accuracy. In order to assess the precision of the predictions, test images will be utilized to implement our trained model. Following this, the anticipated classifications will be appended to the corresponding images as shown in Figure 10 .
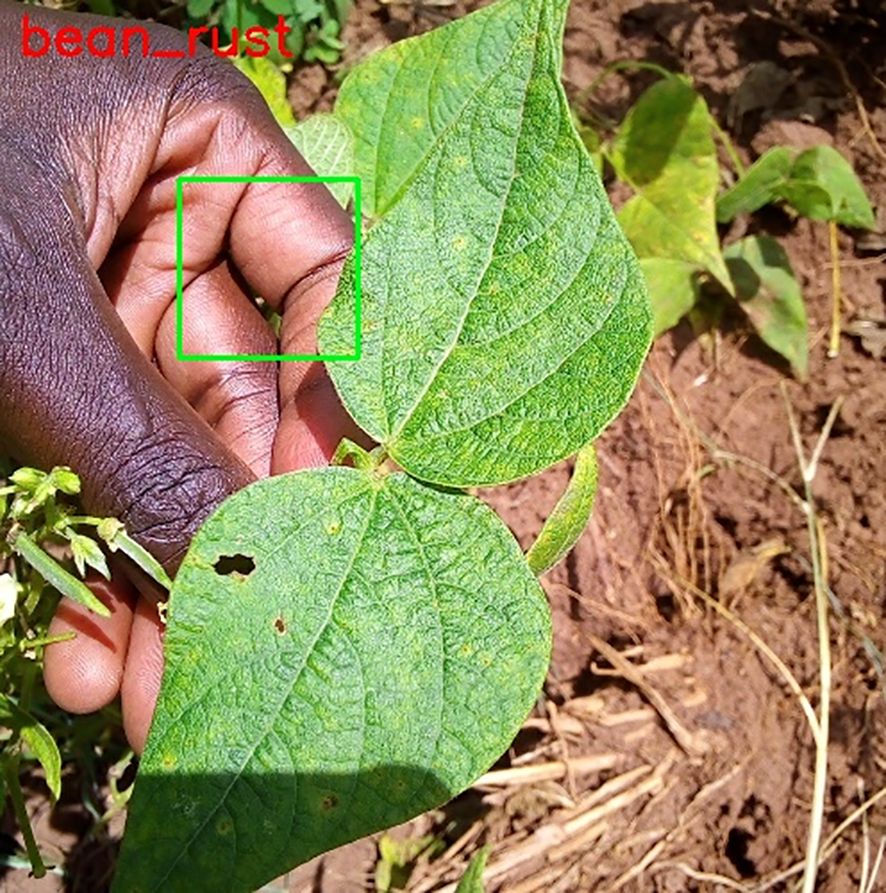
Figure 10 Test image for prediction.
As depicted in Figure 10 , an anticipated designation has been allocated to each image of the plant. It is worth mentioning that the annotation of the projected square aligns precisely with the area actually impacted by illness in specific samples. In other instances, however, misplacement appears to have occurred. This observation implies that the model has been trained and has acquired a certain degree of capability in producing predictions that are accurate. Nonetheless, the efficacy of the model could be enhanced, especially in situations involving inconsistencies. Employing a confusion matrix chart would present a more favorable approach to assess the performance of the model, given that it furnishes a comprehensive synopsis through the inclusion of accurate predictions in conjunction with true-false and true-negative forecasts.
The Confusion Matrix Heatmap, which shows the results of the assessed test images, is shown in Figure 11 . Although YOLOv4 exhibits remarkable effectiveness in precisely detecting illnesses of plant leaves, it is crucial to recognize its occasional limitations. Notably, even with the model’s 99% overall accuracy, a little early-stage infection on a tomato leaf was misclassified as healthy. This disparity highlights the difficulties that come with complex disease patterns and the continuous need for dataset enhancement and improvement. Thorough annotations and a large image bank with various illness presentations are essential to strengthen the model’s robustness. Transfer learning and fine-tuning are two strategies that can improve YOLOv4’s performance, especially in tough settings like cases of misclassification. This example demonstrates how crucial it is to continuously improve models in order to ensure that they can be adjusted to the ever-changing complexity of various plant diseases and generate consistent and trustworthy diagnosis results.
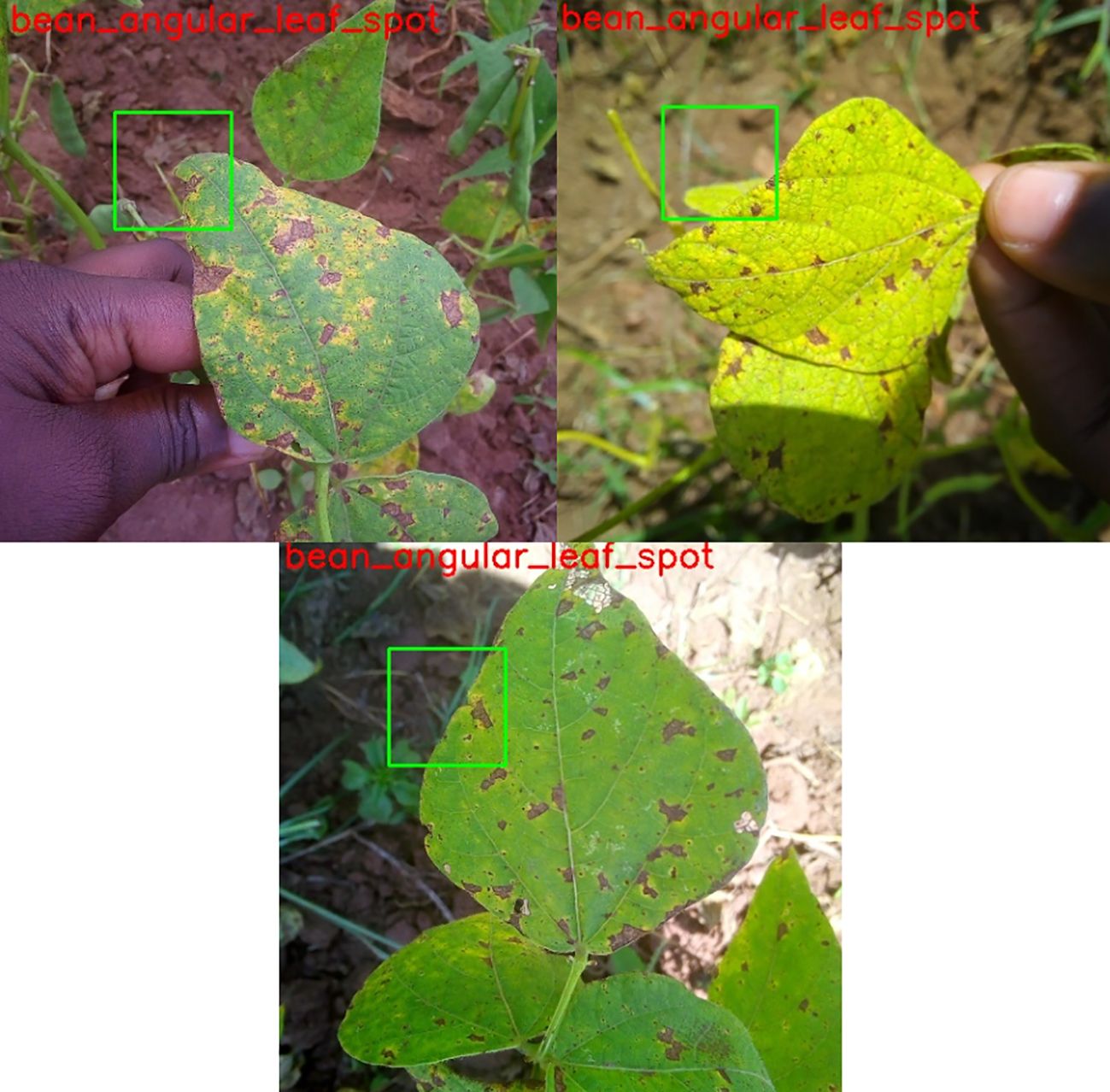
Figure 11 Evaluated predicted disease classes of test images.
5.6 Model evaluation on new plant disease dataset
The Yolov4 model was tested on a new dataset with more classes and different plant leaves to see how well it was able to generalize and stay strong. This dataset has over 87K RGB images of healthy and damaged crop leaves. It is split into 38 groups. The whole dataset is split into two parts: training and validation sets. The directory layout is kept the same. After that, a new directory with 33 test pictures is created to help with the prediction.
Moreover, there are 38 distinct plant species with several illnesses in this collection. There are fourteen distinct plant species and twenty-six distinct disease species. Figure 12 shows the image distribution for each of the 38 classes.
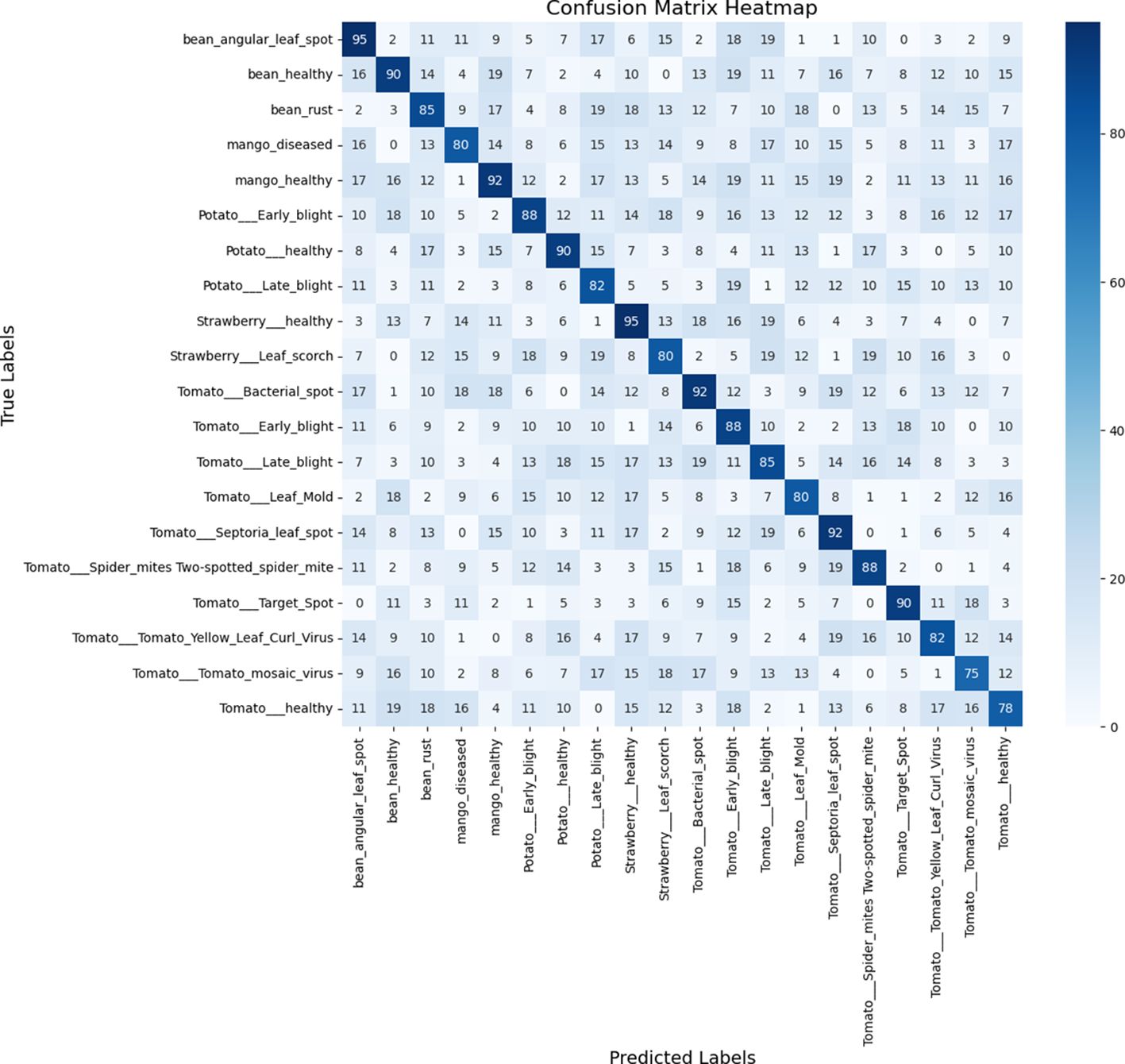
Figure 12 Confusion matrix heatmap of evaluated test images.
The graphic shows that there is a very uniform distribution of images across all classes, ensuring equal training for each class and fair learning for the model. Unequal distribution of photos, either oversampled or undersampled, might lead to overfitting in the model, causing it to perform poorly on classes with fewer images. Here are some sample images shown in Figure 13 .
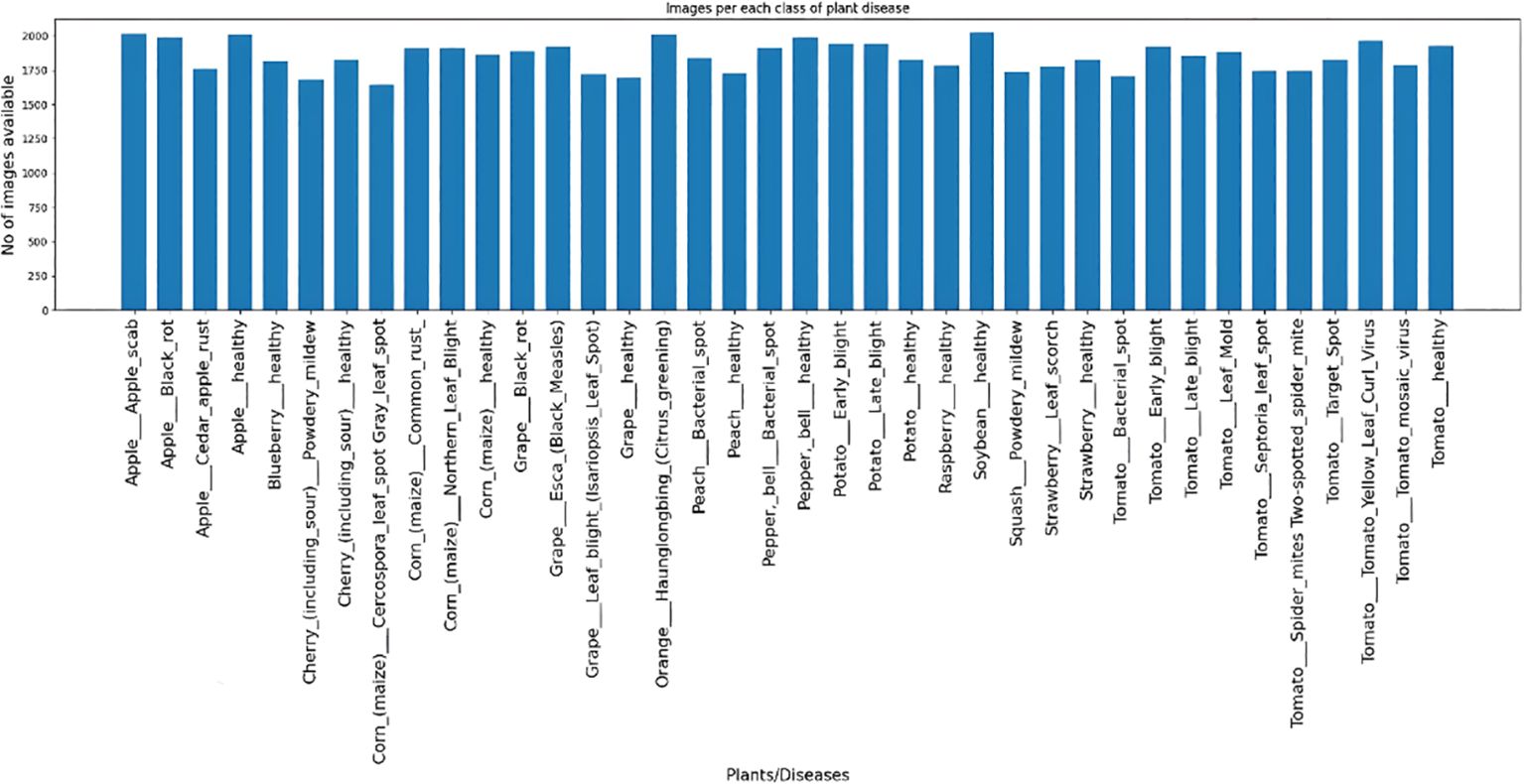
Figure 13 Images distribution of new plant disease dataset.
All the images are scaled, tagged, and supplemented. Necessary processes include creating object data, object names, cloning Darknet, and custom configuring Yolov4 for this dataset. Upon completing the training of this dataset, the accuracy reached about 98% for all image classes. Yolov4 demonstrates its effectiveness by producing high-quality results across several datasets containing about 38 classes. Increasing the number of classes typically leads to a decline in model classification accuracy. However, in this instance, the model demonstrated strong robustness by producing outstanding results when tested on a new dataset containing more photos, other plants, and disease classes.
5.7 Comparative analysis
Table 6 comparison study shows that all four systems have identified plant diseases with a respectable degree of accuracy using DL.
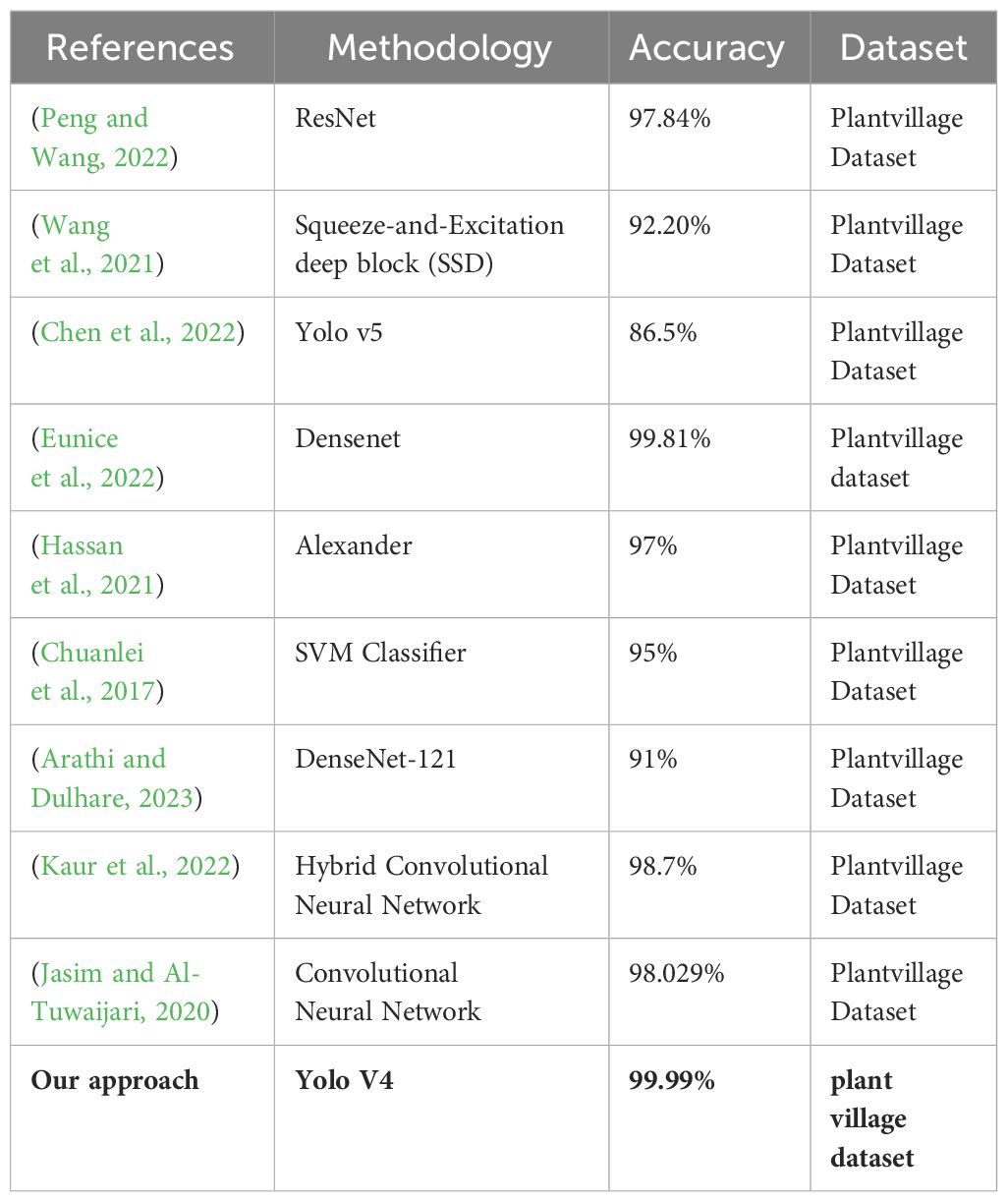
Table 6 Related work for comparison analysis.
Regarding plant disease classification with the Plantvillage dataset, our new Yolo V4-based method leads the way with an outstanding 99.99% accuracy. Other well-known models perform noticeably worse when applying this achievement to the same dataset. The most notable design, ResNet ( Peng and Wang, 2022 ), achieved 97.84% accuracy, followed by squeeze-and-excitation deep block (SSD) ( Wang et al., 2021 ) at 92.20% and Yolo v5 ( Chen et al., 2022 ) with a noteworthy but lesser accuracy of 86.5%.
Densenet ( Eunice et al., 2022 ), which is renowned for its dense connections, attained an astounding accuracy of 99.81%; nonetheless, it is not as precise as our Yolo V4-based method, which is revolutionary. Analogously, 98.7% accuracy was reported by Alexander ( Hassan et al., 2021 ), 95% by the SVM classifier ( Chuanlei et al., 2017 ), 91% by DenseNet-121 ( Arathi and Dulhare, 2023 ), 98.7% by hybrid convolutional neural networks ( Kaur et al., 2022 ), and 98.029% by convolutional neural networks ( Jasim and Al-Tuwaijari, 2020 ). Although these models show many ways to classify plant diseases, they can only partially equal the extraordinary accuracy our suggested strategy can accomplish.
The Yolo V4-based approach surpasses all others, setting a new standard in the market. This demonstrates the efficacy of Yolo V4 in the Plantvillage dataset and the importance of selecting the right model architecture to get the best precision in diagnosing agricultural diseases.
Our method on the Plant Village dataset—which uses the YOLO v4 architecture—achieved perfect accuracy. However, the precise figure is not provided. A well-performing architecture for object detection tasks has been demonstrated for YOLO v4 on some datasets.
Overall, it is evident that all four methods have successfully detected plant diseases using deep learning techniques, with Densenet having the best results on the Plant Village dataset. The performance and accuracy of deep learning models can be significantly impacted by various datasets, model topologies, and hyperparameters; it is crucial to keep this in mind. Therefore, it is imperative to carefully assess and compare multiple approaches in the context of the current problem and dataset.
5.8 Core contributions
Figure 14 depicts the core contributions of the study:
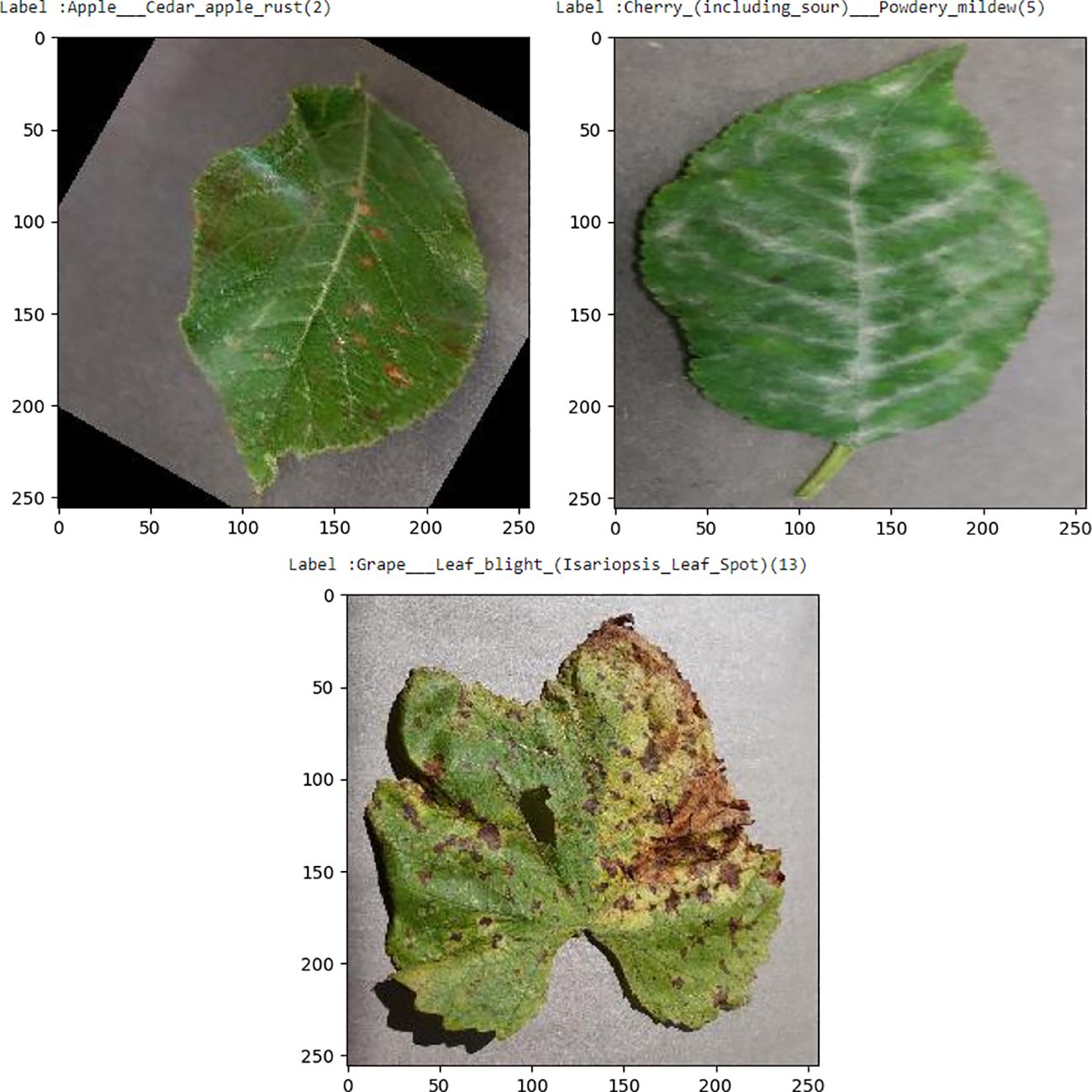
Figure 14 Sample images of different plants diseases of new plant disease dataset.
5.8.1 Innovative image retrieval method
Presented a novel image retrieval technique for open-set plant leaf disease diagnosis. Using tiny annotated pictures, this technique enables the simultaneous creation, location, and diagnosis of leaf diseases, improving accuracy even for situations never before observed.
5.8.2 Enhancements to YOLOv4 architecture
YOLOv4 architecture was enhanced with an emphasis on improving the identification of minute items in plant leaf photos. These improvements strengthen the model’s overall resilience, especially when it comes to the detection of plant leaf diseases.
5.8.3 Versatile model performance
Conducted a thorough investigation of model configurations and hyperparameter settings, demonstrating the adaptability and durability of the YOLOv4 architecture. The model performed well in adequately identifying and categorizing various plant leaf diseases.
5.8.4 Boarder implications for food security
Emphasized the broader ramifications of our findings about sustainable agriculture and food security. Our work promotes cutting-edge computer vision technology, which helps guarantee a more reliable and secure global food supply.
5.8.5 Future research
Outlined future research directions and acknowledged its limitations, such as expanding our methodology to cover a broader range of plant diseases and datasets, investigating new deep learning techniques, and tackling newly identified issues.
All techniques considered, our research raises the bar for plant disease diagnosis, providing workable answers that can be used immediately and opening the door for more advancements in the field.
5.9 Novel model design
The work offered unique approaches utilizing a proprietary Yolov4 model, advanced image processing techniques, and custom annotation for detecting plant diseases. The same is also seen in Supplementary Figure 1 .
5.9.1 Utilization of Yolov4 architecture
Implemented the state-of-the-art YOLOv4 architecture for plant disease identification and detection. This architecture was specially modified to address the difficulties associated with plant disease identification, and it is well-known for its effectiveness in real-time object detection.
5.9.2 Image dataset selection
The utilization of the “Plant Village” dataset is notable for its innovative nature stemming from its diverse characteristics. In contrast to conventional datasets that may concentrate exclusively on particular diseases or restricted plant species, “Plant Village” comprises in excess of fifty thousand images that depict both healthy and afflicted foliage on fourteen species of diverse plants. The extensive range of topics covered guarantees a thorough depiction of common agricultural obstacles. The utilization of images captured by smartphones enables the democratization of data collection, which in turn promotes extensive engagement and instantaneous updates. The extensive scope and inclusivity of the dataset not only substantiate its pertinence to agricultural environments but also augment its practicality in driving groundbreaking investigations and advancing knowledge in the field of plant pathology.
5.9.3 Data augmentation techniques
A novel methodology is introduced herein for enhancing the diagnosis of plant leaf maladies, employing YOLOv4 alongside distinctive data augmentation techniques. Our approach capitalizes on innovative methodologies, notably spatial-temporal transformations, to generate dynamic variations in leaf images, thereby enhancing the adaptability of the model. Through the augmentation of the dataset with a diverse range of elements, this method surpasses conventional approaches, bolstering the model’s ability to extrapolate across various environmental conditions. By adding changes in both space and time to the dataset, its resilience is increased, leading to a YOLOv4 model that can accurately identify plant leaf diseases and shows higher adaptability.
5.9.4 Performance contrast with traditional algorithms
Compared and evaluated the YOLOv4 algorithm’s performance against established target identification algorithms like Densenet, Alexanet, and neural networks. The study offers a thorough assessment, emphasizing YOLOv4’s superiority in identifying plant leaf diseases.
The YOLOv4-based solution demonstrated outstanding performance by achieving a remarkable accuracy of 99.99% on the Plant Village dataset. The exceptional accomplishment of achieving high precision showcases the impressive performance of the YOLOv4 framework in effectively detecting and categorizing illnesses affecting plant leaves.
The work’s novel contribution lies in the successful utilization of the YOLOv4 architecture for identifying plant diseases, showcasing exceptional precision across diverse datasets. Advanced computer vision methods are essential for maintaining worldwide food security, and this study emphasizes their importance with practical applications for the agricultural industry. The study centres on the expedient and punctual identification of plant diseases by implementing state-of-the-art computer vision techniques, specifically the YOLOv4 model. The practical practicality of utilizing a variety of datasets representing different plant species is highlighted.
6 Discussion
The study paper investigates the application of YOLOv4 for the detection and classification of plant leaf diseases. The recommended images retrieval technique is thoroughly examined for its practicality and dependability. The study highlights the crucial need for adjustments in the recognition system to align with the unique application context, with a particular focus on the importance of leaf detection for accurate retrieval of plant diseases. There are issues with finding the best balance between the need for more precise detection models and the usage of higher image resolutions in complex and changing scenarios. Hence, it is crucial to strike a cautious equilibrium between the rate of detection and the effectiveness of the application.
The study relies on the utilization of the Plant Village dataset, comprising over 50,000 photos taken with a smartphone camera. The photographs illustrate both the foliage in good condition and the foliage that has been harmed by a total of fourteen distinct plant types. The researchers meticulously categorized records of diseases affecting fruits and vegetables into 20 different categories, resulting in a diverse compendium. YOLOv4, developed by the University of Washington, is a highly efficient technology for real-time object detection. The system employs a complex neural network architecture to precisely predict the positions of bounding boxes and the probability of different classes. The model attains high performance by using sophisticated techniques such as weighted residual connections, mish activation functions, and spatial pyramid pooling. These methodologies produce state-of-the-art results in several item recognition metrics and improve the model’s applicability in different settings.
Researchers now have the opportunity to utilize the capabilities of YOLOv4 by accessing its source code on GitHub. The study outlines a systematic procedure for developing a personalized YOLOv4 model. This involves preparing the dataset, specifying training parameters, and training the model using the Darknet framework. To begin, the process entails annotating and tagging photos using software applications such as LabelImg, YOLOv4 Label. Next, the process involves obtaining pre-trained weights for the Darknet Framework and creating a YOLOv4 configuration file. The Darknet framework is a versatile tool that enables the development and training of neural network architectures, facilitates compatibility with OpenCV, and provides access to pre-trained models for various computer vision tasks.
The training procedure includes a validation dataset to assess the model’s performance, highlighting the importance of high-quality photos, accurate annotations, and well-maintained image databases in order to achieve optimal prediction accuracy. The study emphasizes the correlation between the quality of the input data and the efficiency and performance of the model. The statement underscores the crucial importance of thorough data preparation in ensuring the strength and reliability of the trained YOLOv4 model.
The project aims to perform a comparative analysis to evaluate the effectiveness of four different approaches that employ deep learning algorithms for the purpose of diagnosing plant diseases. Using the AlexNet architecture, the initial benchmark achieves an impressive accuracy of 97% on the Plant Village dataset. The second approach, employing the DenseNet architecture, attains exceptional performance with a 99% accuracy rate. The third method, which combines a convolutional neural network with feature reduction, achieves a precision rate of 98.7%. Densenet is the most accurate architecture for disease diagnosis on the Plant Village dataset, but YOLOv4, although not the most accurate, is widely recognized for its frequent usage.
Moreover, when it comes to YOLOv4 plant leaf disease identification, hyperparameters have a significant impact. For a model to be accurate, batch size, learning rate, and epoch adjustments must be made optimally. The batch size, which is 64 with 16 subdivisions, affects generalization and convergence performance. Model convergence is ensured at a learning rate of 0.001, which strikes a balance between training time and accuracy. Effective disease detection requires avoiding overfitting, which is achieved by careful epoch selection. This emphasizes how important it is to carefully test and validate ideas across a variety of datasets in order to find the right balance in agricultural applications between generalization, training efficiency, and accuracy.
Further, the research fosters a discerning viewpoint within the scientific community by examining and comparing various approaches to diagnosing plant diseases. This enhances understanding of the intricacies of these methods and their suitability in multiple situations. The evaluation metrics exhibit outstanding performance, with accuracy, precision, recall, and the F1 score all attaining a value of 0.99. The measurements demonstrate the effectiveness of the proposed YOLOv4-based approach in detecting plant leaf diseases, thereby affirming the reliability of the strategy.
In conclusion, the research offers a thorough review of employing YOLOv4 for identifying plant leaf diseases, highlighting the viability and limitations of the picture retrieval method. The study highlights the need for more efforts to enhance the equilibrium between detection accuracy and processing speed. The comparison research confirms that YOLOv4 is effective in actual situations but does not achieve the highest level of precision. In summary, the research provides useful insights in the field, allowing progress in detecting plant illnesses using deep learning approaches.
6.1 Future directions
Following are the future improvements which can be taken in after undergoing this specific study for plant disease detection. The Supplementary Figure 2 should be expanded towards more diseases of plants:
6.1.1 Extensions of different plant diseases and plants datasets
Extend the application of the suggested methodology to include more plant diseases than were initially thought of. Add a variety of datasets that reflect various crops and geographical areas to improve the model’s generalization ability.
6.1.2 Integration of multimodal data
Investigate multimodal data integration by merging image data with additional pertinent sensor data (such as spectral or environmental data). This method could lead to a more precise identification of diseases and a more thorough understanding of the variables affecting plant health.
6.1.3 Real-time implementation and edge computing
Examine whether the model can be implemented in real-time situations, especially in edge computing. In agricultural settings, creating a version of the model optimized for edge device deployment can help with on-site disease diagnosis and prompt intervention.
6.1.4 Continuous model improvement with online learning
Establish a framework for online learning that enables the model to evolve and improve over time. It can maintain long-term efficacy and remain relevant to changing illness patterns by adding fresh data and adjusting the model parameters.
6.1.5 Examine model interpretability and explainability
Prioritize enhancing the model predictions’ interpretability and explainability. Gaining the trust of end users, including farmers and agricultural practitioners, and promoting the implementation of the technology in practical situations depend on their awareness of how the model makes its judgments.
6.1.6 Collaboration with agriculture expert
Work with subject matter experts to further validate and improve the model such as plant pathologists and agronomists. Their knowledge can improve the model’s performance in actual agricultural environments and aid in the creation of more contextually relevant features.
6.1.7 Implementation of transferable model architecture
Consider transferability when designing the model architecture. Ensure the model is more flexible and scalable by ensuring that the Information acquired from training on one set of plant diseases can be efficiently transferred to new illnesses with little extra training.
6.1.8 User-friendly interface for end-users
Provide an interface that is easy for end users, such as farmers and agricultural extension agents. To encourage user acceptance and adoption, the interface should make the model’s predictions, diagnostic data, and illness management advice easily accessible.
6.1.9 Integration of environmental context
Incorporate soil health and weather information into the environmental context for diagnosing diseases. Comprehending the dynamic relationship between environmental variables and disease incidence can produce more comprehensive and precise forecasts.
6.1.10 Benchmarking against emerging technologies
Benchmark the established model against new developments in plant disease diagnostics regularly. Keeping up with developments guarantees that the approach will always be at the cutting edge of innovation and provide cutting-edge results.
By addressing these prospective avenues, the research can contribute to the ongoing advancement of innovative and essential techniques for diagnosing plant diseases. Ultimately, this will enhance agricultural methodologies and ensure global food security.
7 Conclusion
The study focuses on addressing the complex issue of open-set detection of plant leaf diseases by incorporating an image retrieval method into the YOLOv4 framework. The method uses brief annotated photos to achieve both the identification and detection of leaf diseases at the same time, demonstrating significant progress in accuracy, flexibility, and reliability. Improving YOLOv4 to better detect small details significantly enhances the precision of diagnosing leaf diseases. It demonstrated exceptional performance by achieving a 99.99% accuracy rate when validating the Plant Village dataset, outperforming other models in its category. The investigation’s effectiveness depends significantly on the smooth incorporation of the Plant Village dataset and the YOLOv4 architecture to accurately identify and classify different types of plant leaf diseases. The technique demonstrates outstanding proficiency in accurately identifying plant leaf diseases via picture labeling, data preparation, and intensive model training. YOLOv4’s success in identifying and diagnosing unhealthy areas in plant leaf images is a direct outcome of extensive testing on hyperparameter configurations and model designs, leading to continuous enhancement of its performance. This study significantly enhances the utilization of deep learning methods for detecting plant illnesses, highlighting the adaptability of YOLOv4 in this specific area.
Future study seeks to broaden the approaches by include a wider range of plant diseases and datasets. Enhancing the system’s performance will require investigating alternative deep-learning methods. Advancements in deep learning technology allow for a more thorough examination of plant diseases, leading to more precise and comprehensive diagnoses. By implementing advanced techniques and adding more data, the model’s robustness will be strengthened, making it ideal for many situations. The methodology will be enhanced through a thorough investigation of incorporating modern deep learning techniques, including transfer learning, ensemble models, and attention mechanisms. The system’s ability to detect new and intricate plant illnesses will be improved by using transfer learning to utilize pre-trained models created from extensive datasets. Ensemble models will be investigated to improve the resilience and adaptability of the approaches to different datasets and environmental situations by combining predictions from many models. The model will incorporate attention approaches based on human cognitive processes to better identify important qualities and enhance its ability to detect subtle patterns associated with different plant diseases.
Moreover, it is crucial to include more datasets that encompass various geographical regions, temperatures, and plant species to guarantee the model’s adaptability and usefulness. Forming partnerships with agricultural research institutes and organizations helps streamline the gathering and dissemination of varied datasets, promoting a cooperative strategy in tackling the worldwide issue of plant diseases. The research highlights the crucial requirement for sophisticated computer vision technologies to guarantee food security and develop sustainable agriculture. Combining YOLOv4 and photo retrieval allows for precise and quick identification of plant diseases. This technology provides a reliable and effective way to identify new leaf diseases with no training required. This discovery places the study at the forefront of the confluence between deep learning and plant pathology, making major contributions to generating strong and adaptable solutions for the issues in modern agriculture. Continual improvements and fine-tuning of procedures are being made to promote the role of technology in protecting global food production and supporting agricultural sustainability in the future.
Data availability statement
The original contributions presented in the study are included in the article/ Supplementary Material . Further inquiries can be directed to the corresponding author.
Author contributions
MZ: Conceptualization, Data curation, Formal analysis, Investigation, Methodology, Writing – original draft. EA: Funding acquisition, Methodology, Project administration, Resources, Writing – review & editing. AA: Conceptualization, Supervision, Validation, Visualization, Writing – review & editing.
The author(s) declare financial support was received for the research, authorship, and/or publication of this article. The authors extend their appreciation to the Deputyship for Research & Innovation, Ministry of Education in Saudi Arabia for funding this research work through the project number RI-44-0618.
Acknowledgments
The authors extend their appreciation to the Deputyship for Research & Innovation, Ministry of Education in Saudi Arabia for funding this research work through the project number RI-44-0618.
Conflict of interest
The authors declare that the research was conducted in the absence of any commercial or financial relationships that could be construed as a potential conflict of interest.
Publisher’s note
All claims expressed in this article are solely those of the authors and do not necessarily represent those of their affiliated organizations, or those of the publisher, the editors and the reviewers. Any product that may be evaluated in this article, or claim that may be made by its manufacturer, is not guaranteed or endorsed by the publisher.
Supplementary material
The Supplementary Material for this article can be found online at: https://www.frontiersin.org/articles/10.3389/fpls.2024.1355941/full#supplementary-material
Abbreviations
PLF, Plant Leaf Disease; SVM, Support Vector Machine; CNN, Convolution Neural Network; RPN, Region Proposal Networks; DL, Deep Learning; SSCNN, Self-Structured CNN; SPP, Spatial Pyramid Pooling; RS, Remote Sensing; ANN, Artificial Neural Network; ML, Machine Learning; RCNN, Region Convolution Neural Network; YOLO, You Only Look Once; PDDNN, Plant Disease Detection Neural Network; KNN, K-Nearest Neighbour; DNN, Deep Neural Network.
Albattah, W., Nawaz, M., Javed, A., Masood, M., Albahli, S. (2022). A novel deep learning method for detection and classification of plant diseases. Complex Intelligent Syst. , 1–18. doi: 10.1007/s40747-021-00536-1
CrossRef Full Text | Google Scholar
Arathi, B., Dulhare, U. N. (2023). “Classification of cotton leaf diseases using transfer learning-denseNet-121,” in Proceedings of Third International Conference on Advances in Computer Engineering and Communication Systems: ICACECS 2022. (Hyderabad, India) 393–405.
Google Scholar
Attallah, O. (2023). Tomato leaf disease classification via compact convolutional neural networks with transfer learning and feature selection. Horticulture 9, 149. doi: 10.3390/horticulturae9020149
Bin Naeem, A., Senapati, B., Chauhan, A. S., Kumar, S., Gavilan, J. C. O., Abdel-Rehim, W. M. F. (2023). Deep learning models for cotton leaf disease detection with VGG-16. Int. J. Intelligent Syst. Appl. Eng. 11, 550–556.
Chen, Y., Wu, Q. (2023). Grape leaf disease identification with sparse data via generative adversarial networks and convolutional neural networks. Precis Agric. 24, 235–253. doi: 10.1007/s11119-022-09941-z
Chen, Z., Ruhui, W., Yiyan, L., Chuyu, L., Siyu, C., Zhineng, Y., et al. (2022). Plant disease recognition model based on improved YOLOv5. Agronomy 12, 365. doi: 10.3390/agronomy12020365
Chuanlei, Z., Shanwen, Z., Jucheng, Y., Yancui, S., Jia, C. (2017). Apple leaf disease identification using genetic algorithm and correlation-based feature selection method. Int. J. Agric. Biol. Eng. 10, 74–83.
Dhinesh, E., Jagan, A., et al. (2019). “Detection of leaf disease using principal component analysis and linear support vector machine,” in 2019 11th International Conference on Advanced Computing (ICoAC). (Chennai, India) 350–355.
Eunice, J., Popescu, D. E., Chowdary, M. K., Hemanth, J. (2022). Deep learning-based leaf disease detection in crops using images for agricultural applications. Agronomy 12, 2395.
Hassan, S. M., Maji, A. K., Jasiński, M., Leonowicz, Z., Jasińska, E. (2021). Identification of plant-leaf diseases using CNN and transfer-learning approach. Electron. (Basel) 10, 1388. doi: 10.3390/electronics10121388
Jasim, M. A., Al-Tuwaijari, J. M. (2020). “Plant leaf diseases detection and classification using image processing and deep learning techniques,” in 2020 International Conference on Computer Science and Software Engineering (CSASE). (Duhok, Iraq) 259–265.
Javidan, S. M., Banakar, A., Vakilian, K. A., Ampatzidis, Y. (2023). Diagnosis of grape leaf diseases using automatic K-means clustering and machine learning. Smart Agric. Technol. 3, 100081. doi: 10.1016/j.atech.2022.100081
Kaur, P., Shilpi, H., Rajeev, T., Shuchi, U., Surbhi, B., Arwa, M., et al. (2022). Recognition of leaf disease using the hybrid convolutional neural network by applying feature reduction. Sensors 22, 575. doi: 10.3390/s22020575
PubMed Abstract | CrossRef Full Text | Google Scholar
Liu, G., Peng, J., El-Latif, A. A. A. (2023). SK-mobileNet: A lightweight adaptive network based on complex deep transfer learning for plant disease recognition. Arab J. Sci. Eng. 48, 1661–1675. doi: 10.1007/s13369-022-06987-z
Liu, J., Wang, X. (2021). Plant diseases and pests detection based on deep learning: a review. Plant Methods 17, 1–18. doi: 10.1186/s13007-021-00722-9
Mitra, D. (2021). Emerging plant diseases: research status and challenges. Emerging Trends Plant Pathol. 1–17.
Mustafa, M. S., Husin, Z., Tan, W. K., Mavi, M. F., Farook, R. S. M. (2020). Development of an automated hybrid intelligent system for herbs plant classification and early herbs plant disease detection. Neural Comput. Appl. 32, 11419–11441. doi: 10.1007/s00521-019-04634-7
Peng, Y., Wang, Y. (2022). Leaf disease image retrieval with object detection and deep metric learning. Front. Plant Sci. 13, 963302. doi: 10.3389/fpls.2022.963302
Perveen, K., Sanjay, K., Sahil, K., Mukesh, S., Najla, A. A., Shanzeh, B., et al. (2023). Multidimensional attention-based CNN model for identifying apple leaf disease. J. Food Qual 2023, 1–12. doi: 10.1155/2023/9504186
Rahman, S. U., Alam, F., Ahmad, N., Arshad, S. (2023). Image processing based system for the detection, identification and treatment of tomato leaf diseases. Multimed Tools Appl. 82, 9431–9445. doi: 10.1007/s11042-022-13715-0
Roy, A. M., Bhaduri, J. (2021). A deep learning enabled multi-class plant disease detection model based on computer vision. Ai 2, 413–428. doi: 10.3390/ai2030026
Sangeetha, K., Rima, P., Kumar, P., Preethees, S. (2022). “Apple leaf disease detection using deep learning,” in 2022 6th International Conference on Computing Methodologies and Communication (ICCMC). (Erode, India) 1063–1067.
Sanida, M. V., Sanida, T., Sideris, A., Dasygenis, M. (2023). An efficient hybrid CNN classification model for tomato crop disease. Technol. (Basel) 11, 10. doi: 10.3390/technologies11010010
Sharma, R. P., Dharavath, R., Edla, D. R. (2023). IoFT-FIS: Internet of farm things based prediction for crop pest infestation using optimized fuzzy inference system. Internet Things 21, 100658. doi: 10.1007/978-3-031-33808-3
Singh, M. K., Chetia, S., Singh, M. (2017). Detection and classification of plant leaf diseases in image processing using MATLAB. Int. J. Life Sci. Res. 5, 120–124.
Soeb, M. J. A., Fahad, J., Tahmina, A., Muhammad, R., Fahim, M., Aney, P., et al. (2023). Tea leaf disease detection and identification based on YOLOv7 (YOLO-T). Sci. Rep. 13, 6078, 2023. doi: 10.1038/s41598-023-33270-4
Taheri-Garavand, A., Nasiri, A., Fanourakis, D., Fatahi, S., Omid, M., Nikoloudakis, N. (2021). Automated in situ seed variety identification via deep learning: a case study in chickpea. Plants 10, 1406. doi: 10.3390/plants10071406
Terentev, A., Vladimir, B., Ekaterina, S., Dmitriy, E., Danila, E., Dmitriy, K., et al. (2023). Hyperspectral remote sensing for early detection of wheat leaf rust caused by puccinia triticina. Agriculture 13, 1186. doi: 10.3390/agriculture13061186
Thangavel, K. D., Seerengasamy, U., Palaniappan, S., Sekar, R. (2023). Prediction of factors for Controlling of Green House Farming with Fuzzy based multi-class Support Vector Machine. Alexandria Eng. J. 62, 279–289.
Vengaiah, C., Konda, S. R. (2023). Improving tomato leaf disease detection with denseNet-121 architecture. Int. J. Intelligent Syst. Appl. Eng. 11, 442–448.
Wang, J., Yu, L., Yang, J., Dong, H. (2021). Dba_ssd: A novel end-to-end object detection algorithm applied to plant disease detection. Information 12, 474. doi: 10.3390/info12110474
Xinming, W., Tang, S. (2023). Comparative study on Leaf disease identification using Yolo v4 and Yolo v7 algorithm. AgBioForum , 25, 1.
Xu, L., Cao, B., Ning, S., Zhang, W., Zhao, F. (2023). Peanut leaf disease identification with deep learning algorithms. Mol. Breed. 43, 25. doi: 10.1007/s11032-023-01370-8
Keywords: plant leaf disease, leaf disease detection, object detection, deep-learning, YOLO v4, darknet
Citation: Aldakheel EA, Zakariah M and Alabdalall AH (2024) Detection and identification of plant leaf diseases using YOLOv4. Front. Plant Sci. 15:1355941. doi: 10.3389/fpls.2024.1355941
Received: 14 December 2023; Accepted: 27 March 2024; Published: 22 April 2024.
Reviewed by:
Copyright © 2024 Aldakheel, Zakariah and Alabdalall. This is an open-access article distributed under the terms of the Creative Commons Attribution License (CC BY) . The use, distribution or reproduction in other forums is permitted, provided the original author(s) and the copyright owner(s) are credited and that the original publication in this journal is cited, in accordance with accepted academic practice. No use, distribution or reproduction is permitted which does not comply with these terms.
*Correspondence: Eman Abdullah Aldakheel, [email protected]
Disclaimer: All claims expressed in this article are solely those of the authors and do not necessarily represent those of their affiliated organizations, or those of the publisher, the editors and the reviewers. Any product that may be evaluated in this article or claim that may be made by its manufacturer is not guaranteed or endorsed by the publisher.
Journal of Plant Diseases and Protection
Scientific Journal of the "Deutsche Phytomedizinische Gesellschaft" (DPG) - the German Society for Plant Protection and Plant Health
- It covers a wide range of interests for the global plant protection community with relevance to European plant health and protection.
- This journal bridges the gap between lab research, field application, and legislative framework.
- It is the official scientific journal of the Deutsche Phytomedizinische Gesellschaft (DPG) the German Society for Plant Protection and Plant Health.
- Offers “Topic collections” on coherent themes often arising from international conferences.
- It encourages the submission of manuscripts on virtually all aspects of plant diseases, pathogenesis, biology, and molecular biology of various pests and diseases.
- Falko Feldmann,
- Noemi Messmer
Societies and partnerships
Latest issue
Volume 131, Issue 3
Latest articles
Chemical constituents from the aerial parts of rhododendron thymifolium and its bioactivities against tribolium castaneum , lasioderma serricorne , and ditylenchus destructor.
- Jun-Yu Liang
- Ying-Ying Yang
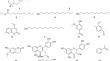
First report of Stemphylium vesicarium causing leaf blight of onion in Slovakia
- Miriam Kádasi Horáková
- Marek Barta
- Katarína Pastirčáková
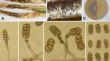
The 20th International Reinhardsbrunn Symposium: Modern Fungicides and Antifungal Compounds
- Holger B. Deising
Physiological responses of grapevine ( Vitis vinifera var. ‘Pinot gris’) affected by different flavescence dorée genotypes: dynamics through the development of phytoplasma infection
- Dino Davosir
- Martina Šeruga Musić
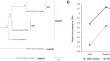
Study of phytopathogenic fungi of Ipomoea hederifolia L.: an invasive weed in agricultural crops in Brazil
- Lisandro Pieroni
- Edivaldo Velini
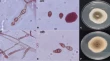
Journal updates
Reviewer acknowledgements, jpdp: a historical insight into the journal, journal information.
- CAB Abstracts
- Chemical Abstracts Service (CAS)
- Engineering Village – GEOBASE
- Google Scholar
- Japanese Science and Technology Agency (JST)
- Norwegian Register for Scientific Journals and Series
- OCLC WorldCat Discovery Service
- Pathway Studio
- Science Citation Index Expanded (SCIE)
- TD Net Discovery Service
- UGC-CARE List (India)
Rights and permissions
Editorial policies
© Deutsche Phytomedizinische Gesellschaft
- Find a journal
- Publish with us
- Track your research

An official website of the United States government
The .gov means it’s official. Federal government websites often end in .gov or .mil. Before sharing sensitive information, make sure you’re on a federal government site.
The site is secure. The https:// ensures that you are connecting to the official website and that any information you provide is encrypted and transmitted securely.
- Publications
- Account settings
Preview improvements coming to the PMC website in October 2024. Learn More or Try it out now .
- Advanced Search
- Journal List
- Sensors (Basel)
- PMC10223612

Plant Disease Detection and Classification: A Systematic Literature Review
1 Department of Computer Science and Engineering, Lovely Professional University, Phagwara 144411, Punjab, India
Usha Mittal
Ankita wadhawan, jimmy singla.
2 School of Engineering and Technology, CT University, Ludhiana 142024, Punjab, India
N.Z Jhanjhi
3 School of Computer Science, SCS, Taylor’s University, Subang Jaya 47500, Malaysia
Rania M. Ghoniem
4 Department of Information Technology, College of Computer and Information Sciences, Princess Nourah bint Abdulrahman University, P.O. Box 84428, Riyadh 11671, Riyadh, Saudi Arabia
Sayan Kumar Ray
Abdelzahir abdelmaboud.
5 Department of Information Systems, King Khalid University, Abha 61913, Muhayel Aseer, Saudi Arabia
Associated Data
Data will be available on request.
A significant majority of the population in India makes their living through agriculture. Different illnesses that develop due to changing weather patterns and are caused by pathogenic organisms impact the yields of diverse plant species. The present article analyzed some of the existing techniques in terms of data sources, pre-processing techniques, feature extraction techniques, data augmentation techniques, models utilized for detecting and classifying diseases that affect the plant, how the quality of images was enhanced, how overfitting of the model was reduced, and accuracy. The research papers for this study were selected using various keywords from peer-reviewed publications from various databases published between 2010 and 2022. A total of 182 papers were identified and reviewed for their direct relevance to plant disease detection and classification, of which 75 papers were selected for this review after exclusion based on the title, abstract, conclusion, and full text. Researchers will find this work to be a useful resource in recognizing the potential of various existing techniques through data-driven approaches while identifying plant diseases by enhancing system performance and accuracy.
1. Introduction
Agricultural biodiversity is foundational to providing food and raw materials to humans. When pathogenic organisms such as fungi, bacteria, and nematodes; the soil pH; temperature extremes; changes in the amount of moisture and humidity in the air; and other factors continuously disrupt a plant, it can develop a disease. Various plant diseases can impact the growth, function, and structures of plants and crops, which automatically affect the people who are dependent on them. The majority of farmers still use manual methods to identify plant illnesses, since it is challenging to do so early on and has a negative impact on productivity. To overcome this, many deep learning (DL), image processing, and machine learning (ML) techniques are being developed, by which the detection of disease in a plant is performed by images of plant leaves.
Image processing is utilized to improve the quality of images in order to extract valuable information from them; because of this feature, image processing techniques are utilized in many areas, such as color processing, remote sensing, and pattern recognition, of the medical and agricultural fields. Images of plant leaves can be used to identify disease using image processing techniques that are appropriate, effective, and dependable. In image processing techniques, various stages are involved—image acquisition, image pre-processing, feature extraction, image segmentation, and classification. In this study, we examined papers that use image processing approaches. For an instance, Malathy et al. [ 1 ] claim that 97% classification accuracy can be achieved for illness detection using image processing techniques, which is highest compared to other publications.
Machine learning (ML) involves the concept of loss function, which makes it more effective than image processing. The loss function lets us know how the proposed models will function via predictions. Models can predict outcomes more correctly when machine learning (ML), a type of artificial intelligence (AI), is used without explicit guidance. Models which are trained using ML improve their performance based on experience. Due to its nature, ML is applicable in many fields, some of which are medical diagnosis, speech recognition, product recommendation, self-driving cars, virtual assistants (such as Alexa and Siri), prediction of traffic (for example—in Google Maps), and agriculture. ML approaches can be implemented in various manners to develop new algorithms for detecting and classifying diseases that occur in plants at an early stage. We reviewed the state-of-the-art literature in this field, and found that Rumpf et al. [ 2 ], Dubey et al. [ 3 ], Ramesh et al. [ 4 ], Behera et al. [ 5 ], Tulshan et al. [ 6 ], Wahab et al. [ 7 ], etc., are utilizing the concept of ML.
Deep learning (DL) networks learn by spotting intricate patterns in the data with which they work. By building computational models that are composed of numerous processing layers, the networks can produce various degrees of abstraction to explain the data. Although DL is a form of ML, it is more adaptable than ML. While feature extraction and classification are carried out separately in ML, they are combined by utilizing numerous processing layers in DL. When working with unstructured data, automatic feature generation, superior self-learning capabilities, and support for distributed and parallel algorithms are all areas in which it outperforms ML. Various DL approaches can be utilized in agriculture for detecting diseases in plants from leaves, such as recurrent neural networks (RNNs) and convolutional neural networks (CNNs). The proposed paper reviewed the state-of-the-art works by Sladojevic et al. [ 8 ], Fujita et al. [ 9 ], Brahimi et al. [ 10 ], Fuentes et al. [ 11 ], Cap et al. [ 12 ], Ma et al. [ 13 ], Sardogan et al. [ 14 ], Adedoja et al. [ 15 ], Geetharamani et al. [ 16 ], Zhang et al. [ 17 ], Sharma et al. [ 18 ], Coulibaly et al. [ 19 ], Ji et al. [ 20 ], Marzougui et al. [ 21 ], Shrestha et al. [ 22 ], Selvam et al. [ 23 ], Jadhav et al. [ 24 ], Lijo [ 25 ], Sun et al. [ 26 ], Sujatha et al. [ 27 ], Abbas et al. [ 28 ], Divakar et al. [ 29 ], Chowdhury et al. [ 30 ], Akshai et al. [ 31 ], Kibriya et al. [ 32 ], B.V. et al. [ 33 ], Pandian et al. [ 34 ], etc., which has been conducted by utilizing deep learning and convolutional neural networks, which is a DL technique. CNN is a kind of feed-forward neural network whose power lies in the convolutional layer; there is less need to pre-process data in CNN.
This paper is divided into six different sections. Section 1 is the introduction itself. The remaining sections of the paper are arranged as follows. Section 2 describes the research methodology utilized for finding and analyzing the available existing research, research questions, and research criteria. The literature review of previously published studies is described in Section 3 . Section 4 discusses the challenges of the existing approaches. Overall, observation and comparison are provided in Section 5 , and this paper is concluded in Section 6 .
This paper’s significance lies in its discussion of many methods that have been created to identify plant illnesses from their leaves in the domains of ML, image processing, and DL.
2. Methodology
This section presents information regarding the planning and selection criteria for selecting relevant papers for this review.
2.1. Planning
The investigation included compiling a number of journal and conference articles that were released between 2010 to 2022. First, keyword-based searches were made in scientific databases such as IEEE Xplore, SCOPUS Indexed Journal, and Google Scholar (academic search engines). In Table 1 , the list of searched keywords is provided.
Number of papers extracted utilizing each keyword.
Using different keywords, 182 papers were extracted on which inclusion and exclusion operations were performed.
2.2. Conduction
This phase focuses on reviewing and summing up the selection criteria for assessing existing models based on ML, image processing techniques, and DL, including CNN, in terms of effective disease detection in different crops and plants using different datasets. In Figure 1 , the entire research method utilized to produce this study is shown.

The entire method of research utilized to produce this study.
By conducting a keyword search, 182 papers on plant disease detection and classification from sources such as IEEE Xplore, SCOPUS Indexed Journal, and Google Scholar were retrieved that were published in the last 12 years from 2010 to 2022. Three stages made up the exclusion process. The retrieved data were then decreased to 164 based on their titles; publications were then eliminated based on their abstracts and conclusions; and, finally, 75 papers were found after reading the entire text. Figure 2 and Figure 3 represent the number of papers reviewed by year from 2010 to 2022.

Inclusion and exclusion techniques utilized in this review.

Number of papers, by year, from 2010 to 2022.
For the purpose of writing a systematic review, ten research questions were framed, which are specified in Table 2 , and a complete evaluation procedure was conducted by monitoring the existing models for the purpose of addressing research questions.
Research questions and their motives.
3. Related Work
On the basis of the data obtained from the chosen studies, the research methodology findings in this section provide answers to the research questions listed above. These automated models require significant training time, but once they are trained, they are incredibly accurate at spotting early-stage plant diseases and enabling farmers to take preventative action to lessen the effects of disease on productivity. Figure 4 shows various parameters that were considered for review. The approach that was utilized for conducting this literature review included data acquisition, pre-processing techniques, techniques for augmenting data, techniques for extracting features, different features that were extracted, techniques utilized for identification and classification, how the quality of images was enhanced, and techniques utilized for reducing overfitting of the models. The research questions (RQ1 to RQ10) listed in Table 2 are discussed in Section 3.1 , Section 3.2 , Section 3.3 , Section 3.4 , Section 3.5 , Section 3.6 , Section 3.7 , Section 3.8 , Section 3.9 and Section 3.10 .

Parameters considered for the literature review.
3.1. Discussion for RQ1: What Are the Main Sources for Collecting Data on Plants?
The primary step in the identification and categorization of leaf disease is the acquisition of plant leaf imaging data. Images of plant leaves can be taken manually using a camera device, or image data can be acquired from open-source repositories. This section involves discussion on different sources from which image data was collected by various researchers for their work. A total of 46 publications, which were identified as the sources of the data for this section, were taken into consideration for analysis.
Rumpf et al. [ 2 ] utilized images of sugar beet leaves that were cultivated in plastic pots on a commercial substrate, namely Klassmann–Deilmann GmbH (Germany), in an environment with 60 percent relative humidity, a 23 °C daytime temperature, and a 20 °C nighttime temperature. In addition to weekly fertilization with 100 mL of a Poly Crescal 0.2 percent solution, plants were watered as needed. To identify and categorize plant diseases, the study used hyperspectral reflectance-based approaches. Wang et al. [ 35 ] utilized backpropagation networks on a dataset of 185 images—acquired using a digital camera—to identify two distinct instances of wheat and grape illnesses. The collection included 50 wheat stripe rust images, 35 grape powdery mildew images, and 50 images each of grape downy mildew and wheat leaf rust. Husin et al. [ 36 ] utilized LABVIEW IMAQ Vision to collect a dataset of 107 images of chili leaves, 21 of which were healthy and 86 of which were diseased. Sannakki et al. [ 37 ] acquired images of grape leaves with a 16.1 Megapixel Nikon Coolpix P510 digital camera from various locations, including Pune, Bijapur, and Sangali, under the guidance of experts for identification. For system testing and training, the captured images were employed. The captured images were all saved in the common .jpg format. To provide a diversified environment, several images were acquired from the internet. Images were taken of leaves afflicted by powdery mildew and downy mildew, two of the most common illnesses in India.
Es-saddy et al. [ 38 ], under the guidance of an expert, utilized a digital camera to collect image data from a number of farms. The dataset’s size was increased by downloading images from the internet and using a variety of environments. The images include leaf damage from thrips, Tuta Absoluta, and leaf miners (pest insects). Sladojevic et al. [ 8 ] acquired a dataset by searching for plants’ names and diseases. Images were collected from the internet and divided into 15 distinct categories, out of which 13 represented plant diseases to be visually identified from leaves, and the remaining 2 represented healthy leaves and background images.
Fujita et al. [ 9 ] utilized two separate datasets that were created using cucumber leaf images provided by the Research Center of Saitama Agricultural Technology, Japan. Dataset 1 contained 7320 images, including images of leaves that were affected by seven distinct diseases and images of healthy leaves, whereas dataset 2 contained 7520 images. Dyrmann et al. [ 39 ] utilized images acquired from a real environment and six different publicly available datasets for their work. A total of 10,213 images representing 22 different species in terms of changes in illumination, resolution, and soil types were captured with hand-held mobile phones.
Mohanty et al. [ 40 ] acquired data from a publicly available dataset (PlantVillage dataset which is available on kaggle) of 54,306 images, which were categorized into 38 distinct classes of diseased and healthy leaves that were gathered under controlled circumstances. Durmus et al. [ 41 ] utilized the PlantVillage dataset to acquire images of 10 different tomato leaf classes, out of which 9 were disease-affected leaf images and 1 was a healthy leaf image. Brahimi et al. [ 10 ] utilized an open-access repository (PlantVillage) of more than 50,000 images to gather data on around 14,800 images of tomato leaves that were afflicted by 9 distinct diseases. Fuentes et al. [ 11 ] used basic camera devices to collect data of over 5000 images from several tomato fields in Korea while taking lighting, temperature, humidity, and location into consideration. Liu et al. [ 42 ] utilized a digital camera, namely, BM-500 GE/BB-500 GE, to collect a dataset of 1053 apple leaf images from 2 apple experiment stations in China. Four distinct diseases, namely, rust, brown spot, Alternaria leaf spot, and mosaic, could be seen in the acquired images.
Ma et al. [ 13 ] acquired image data from two open-access repositories and a digital camera. Powdery mildew, anthracnose, target leaf spots, and downy mildew were some of the symptoms represented in the dataset, which was obtained from two open-access repositories ( https://plantvillage.org/and https:// www.forestryimages.org/ (accessed on 14 January 2023)). Some image data were also taken using a Nikon Coolpix S3100 (digital camera) from an agricultural scientific innovation base greenhouse in Tianjin (China) under field conditions. Sardogan et al. [ 14 ] utilized a public dataset called the PlantVillage dataset to obtain 500 images of tomato leaves, including 4 distinct kinds of diseased leaves (late blight, septoria leaf spot, bacterial spot, and yellow leaf curl) and images of healthy ones, of which 100 were used for testing and 400 for training. Cap et al. [ 12 ] utilized image data provided by the Saitama Agricultural Technology Research Center in Japan, which included over 60,000 images of cucumber leaves. Behera et al. [ 5 ] acquired images of four different diseased orange samples, including those affected by melanoses, brown rot, stubborn, and citrus canker, from a dataset of the division of Agriculture and Natural Resources at the University of California. Geetharamani et al. [ 16 ] acquired image data from the PlantVillage dataset, which contains about 54,000 images divided into 38 different groups, to train and test the proposed CNN model.
Atila et al. [ 43 ] and Too et al. [ 44 ] obtained image data from the open-source dataset, PlantVillage, which includes around 54,000 images of 14 distinct plant species. Wahab et al. [ 7 ] using a series of camera movements that had been pre-programmed, which enabled them to capture images from multiple orientations and heights. Image data of a chili plant was acquired and recorded in Matlab. KC et al. [ 45 ] acquired data from the publicly accessible PlantVillage dataset, which consists of over 82,000 images divided into 55 separate classes. Both diseased and healthy leaf were including images for the purpose of training and testing the proposed model. Haque et al. [ 46 ] collected ten thousand images using a Nikon D7200 DSLR camera, under various conditions, for four different types of guavas: fruit canker, anthracnose, fruit rot (disease-impacted), and healthy guava. Sahithya et al. [ 47 ] acquired ladies’ finger leaf images using a 1584 × 3456 resolution digital camera.
Chen et al. [ 48 ] acquired image data of roughly 1000 images of rice and maize leaves damaged by various diseases given by the Xiamen, China-based Fujian Institute of Subtropical Botany. The image dataset was taken under various lighting circumstances and cluttered field background conditions and stored in .jpg format. Marzougui et al. [ 21 ] captured 500 images in total, 250 of which were of healthy leaves, while the other shots, which were all taken with a camera against a consistent background, were of diseased leaves. Ponnusamy et al. [ 49 ] collected image data of around 300 healthy and diseased tomato leaves from agricultural fields for a disease diagnosis model based on YOLO, considering the camera quality, impact of the number of leaves in the frame, exposure, and zoom level. Nanehkaran et al. [ 50 ] gathered about 1000 diseased leaf images of three distinct crops—rice, maize, and cucumber—in diverse manners. While images of damaged maize and rice leaves were taken from research farms in Xiamen (China), image data pertaining to cucumber leaves affected by various diseases were retrieved from the internet. All the collected images were stored in .jpg format.
Pham et al. [ 51 ] utilized a 3096 × 3096 resolution camera to acquire a dataset of roughly 450 images of mango leaves from Giang Province, Vietnam. The dataset included three types of disease-infected leaves (powdery mildew, anthracnose, and gall midge) and one category of healthy leaves. The CNN model suggested by Selvam et al. [ 23 ] was trained and tested using image data of around 1085 lady finger leaves (healthy, disease-affected, and impacted by the overuse of fertilizers) collected from two villages in the Tiruvannamalai district. Jadhav et al. [ 24 ] gathered soybean-related image data from a number of soybean fields in Kolhapur district, Maharashtra, India. A total of 1199 photos were utilized for training the suggested CNN models, and roughly 80 images were used to test them. Sun et al. [ 26 ] collected image data from the open-source PlantVillage dataset, of which 80% were used for training and 20% for testing the proposed model. Lijo [ 25 ] acquired 10,000 diseased and healthy images of potato, mango, strawberry, grape, tomato, and pepper leaves from the plant village dataset. Both bacterial and fungal illnesses were included in this research. Chakraborty et al. [ 52 ] utilized images of 13 plant species and 17 kinds of illnesses, comprising approximately 2600 images, which were obtained from PlantDoc.
Abbas et al. [ 28 ] acquired diseased and healthy tomato leaf images from the open-source PlantVillage dataset. Wang et al. [ 53 ] acquired 3000 leaf images of various species, both healthy and disease-affected, that were gathered from the PlantVillage dataset. Divakar et al. [ 29 ] acquired image data that contained images of both diseased and healthy apple leaves, which were downloaded from a publicly accessible dataset on Kaggle. Chowdhury et al. [ 30 ] and Gonzalez-Huitron et al. [ 54 ] acquired image data of around 18,100 tomato leaves from the PlantVillage dataset. It was composed of ten classes, of which nine represented various disease-affected leaves and one contained healthy leaves. Akshai et al. [ 31 ] acquired images of about 4060 grape leaves, including both healthy and diseased leaves of various categories, from the PlantVillage dataset. Kibriya et al. [ 32 ] acquired around 10,000 tomato leaf images from the PlantVillage dataset, out of which 30% were utilized to test the suggested model, whereas 70% were used for training it.
B.V. et al. [ 33 ] for the purpose of identifying potato and tomato leaf diseases, utilized a subset of tomato and potato leaf images from the publicly accessible PlantVillage dataset. Jain et al. [ 55 ] acquired image data for three different crops—maize, grapes, and rice—from the New Plant Diseases Dataset, which is available on Kaggle. Sujatha et al. [ 27 ] gathered images of citrus leaves using DSLR cameras under the direction of experts. Pandian et al. [ 34 ] acquired about 55,448 images of both healthy and diseased leaves of various plant species from a freely accessible dataset. Vallabhajosyula et al. [ 56 ] acquired RGB images of 14 distinct types of crop species and 38 classifications of damaged leaves from the open-source dataset PlantVillage, which was downloaded from Kaggle. Table 3 represents a summarized view of the different data acquisition sources utilized by the reviewed studies. Table 4 provides information about the sources in the real environment from which images were gathered by various researchers for their work.
Summarized view of data acquisition source.
Real Environment description.
Observation 1
This observation is purely framed on the basis of discussions for RQ1 (3.1): 51% of the research under consideration acquired image data from publicly accessible datasets, while 44% employed digital cameras or other devices to collect images from the real environment, and the other 5% obtained their image data from other online sources. The primary publicly accessible datasets used in the evaluated studies were PlantVillage, PlantDoc, and other public datasets. All of the data acquisition sources are depicted in Figure 5 .

Usage of various data acquisition sources.
3.2. Discussion for RQ2: What Different Pre-Processing Techniques Are Applied?
For further processing, image data were pre-processed utilizing a number of different techniques. This section involves a discussion on various pre-processing techniques that have been employed by various researchers in their work. By using “Pre-processing techniques” as a filter, 34 papers were identified for this section, of which 26 papers were chosen for analysis.
Sannakki et al. [ 37 ] pre-processed images using anisotropic diffusion to produce space-variant and non-linear changes to the original images. Khirade et al. [ 57 ] utilized various image pre-processing techniques, including image smoothing, clipping, image enhancement, color conversion, and histogram equalization, to eliminate noise from the images. Rastogi et al. [ 58 ], prior to training and testing the proposed model, pre-processed the image data that were gathered during the image acquisition phase by resizing and cropping operations. Es-saddy et al. [ 38 ] first downsized the images into a standard size using a resizing operation, and then the noise was eliminated from them using a median filter to enhance their quality. Sladojevic et al. [ 8 ] performed two pre-processing operations, including resizing, where the image was scaled into 256 × 256 pixels, and cropping, which was performed to define the regions of interest in plant leaves for improved feature extraction.
Singh et al. [ 59 ] carried out several operations during the pre-processing stage to improve the quality of the image, including the clipping operation to extract the relevant image regions and the use of smoothing filters to improve the image’s smoothness. In order to increase the image’s contrast, image enhancement was used. In the study by Krithika et al. [ 60 ], during the pre-processing stage, pixels from grape leaf images’ edges were deleted, and RGB data collected during the data acquisitionphase were transformed into the HSV and CIELAB color spaces. Ferentinos [ 61 ] performed image size reduction and cropping as part of the pre-processing operations that were performed on collected image data to make the images 256 × 256 pixels. Ramesh et al. [ 4 ] pre-processed the collected images to make them all the same size. Behera et al. [ 5 ] utilized two techniques for pre-processing images. The first technique used was image enhancement, which increased the contrast in the images and drew attention to any hidden details that may have been there, while another technique used was CIELAB color space, which shortened the computing time. Francis et al. [ 62 ] downsized images to 64 × 64 pixels using the resizing and cropping pre-processing techniques.
Devaraj et al. [ 63 ] utilized different MATLAB algorithms throughout the pre-processing step to downsize images, improve the contrast, and transform the RGB images into greyscale. Wahab et al. [ 7 ] utilized MATLAB’s reb2gray function in the pre-processing step to convert RGB format images into grayscale while retaining luminance and removing hue and saturation. Howlader et al. [ 64 ] used Python code in pre-processing for the purpose of scaling all of the acquired images to 256 × 256 pixels. Sharma et al. [ 18 ] performed different pre-processing operations on images to enhance the quality of an image by eliminating noise from it. This was performed by enhancing compactness, changing brightness, extracting noise, and converting to another color space. Sahithya et al. [ 47 ] performed a resizing operation on the image to convert all of the images of the same standard size. Jadhav et al. [ 24 ] converted images into two different dimensions for AlexNet and GoogleNet. For AlexNet, a total of 649 images of soybean were pre-processed into dimensions of 227 × 227 × 3, whereas 550 images of soybean leaf samples underwent the same pre-processing for the proposed GoogleNet framework, resulting in dimensions of 224 × 224 × 3. Chen et al. [ 48 ], for the purpose of creating images of the same size, blackened shorter sides of images during the pre-processing step. Pham et al. [ 51 ], during the pre-processing stage, downscaled the images to a lower resolution, and pixel intensities were adjusted using contrast enhancement. Lijo [ 25 ] scaled images to 256 × 256 pixels during the pre-processing stage.
Chowdhury et al. [ 30 ] utilized various pre-processing operations. Operations such as resizing and normalization were carried out in the pre-processing step. All collected images were downsized to 224 × 224 for various EfficientNet approaches, while they were all converted to 256 × 256 for U-net segmentation techniques. In addition to resizing, the means and standard deviations of the images in the dataset were computed in order to normalize the z-score data. Kibriya et al. [ 32 ] utilized two distinct image processing methods, namely, resizing and denoising. The images were denoised using the Gaussian Blur filter, and all of the collected data were scaled to 225 × 225. Chouhan et al. [ 65 ] pre-processed image data using the resizing, restoration, and image enhancement techniques. Malathy et al. [ 1 ] pre-processed image data are utilizing image resizing and image restoration, which lessen image noise and improve the image’s sharpness.
Jain et al. [ 55 ] improved the images’ quality by employing a 3 × 3 Gaussian filter to remove noise from the image during pre-processing. Ashwinkumar et al. [ 66 ] utilized bilateral filtering, a non-linear filtering technique, during the pre-processing stage to enhance the quality of an image by eradicating noise from the acquired image data. Table 5 shows a summarized view of the various pre-processing methods applied in different reviewed studies.
Summarized view of various pre-processing techniques.
Observation 2
This observation is solely based on RQ2 (3.2) discussions. The studies under evaluation included a variety of pre-processing methods, including scaling, clipping, smoothing, anisotropic diffusion, cropping, denoising, CIELAB color space, contrast improvement, converting RGB images to greyscale, increasing compactness, restoration, and normalizing. Figure 6 shows how various pre-processing methods are generally utilized. First, 30% of the papers that were examined used resizing to pre-process images, while the image improvement, cropping, and denoising operations were utilized by 30% of examined studies combinedly, 10% of each respectively. In the publications that were reviewed, 4% of the restoration, color conversion, clipping, and smoothing procedures were used individually, while 3% of the other pre-processing approaches were utilized individually.

Usage graph of different pre-processing techniques (% in descending order).
3.3. Discussion for RQ3: What Different Techniques Are Used for Data Augmentation?
Various data augmentation approaches can be utilized to enhance the dataset’s image count in order to improve accuracy. In this section, techniques utilized by various researchers in their works to increase the size of dataset have been discussed. Twenty-eight papers were selected which used data augmentation for this section, and are currently being considered for analysis.
Sladojevic et al. [ 8 ] utilized three different operations, namely, rotations, 3 × 3 transformation matrix-based perspective transformation, and affine transformations for augmenting images. Fujita et al. [ 9 ] utilized three different augmentation techniques—image shifting, mirroring, and image rotation—to expand the dataset. Dyrmann et al. [ 39 ] utilized rotation and mirroring techniques to expand the training dataset to 50,864 images (eight times the number of original images). Fuentes et al. [ 11 ] increased the image count in the training dataset through the use of the two image augmentation approaches, namely, geometrical transformation and intensity transformations. While procedures including image scaling, cropping, rotation, and horizontal flipping were carried out during geometrical transformation, intensity transformation dealt with noise, color, brightness enhancement, and contrast. Ma et al. [ 13 ] utilized the rotation and flipping operations to increase the amount of image data. Images in the dataset were rotated by 90, 180, and 270 degrees during the rotation process, but during the flipping operation, images were flipped in both the horizontal and vertical directions. Cap et al. [ 12 ] increased the dataset’s image count utilizing cropping (from the center) and rotation (clockwise) operations.
Kobayashi et al. [ 67 ] utilized several augmentation techniques, including rotation, shear conversion, cutout, and horizontal and vertical direction, to expand the size of the dataset in order to improve detection accuracy. Geetharamani et al. [ 16 ] utilized augmentation operations such as flipping, principal component analysis, rotation, scaling, noise injection, and gamma correction to expand the dataset’s size to approximately 61,400 images. Zhang et al. [ 17 ] utilized intensity transformations and geometric transformations to increase the number of images. There were five approaches used for the intensity transformations: contrast enhancement, color jittering, PCA jittering, blur (radial), and brightness enhancement. Images were enlarged, cropped, rotated, and flipped in geometric transformations (horizontally and vertically). Adedoja et al. [ 15 ] utilized different combinations of data augmentation techniques, including RandomRotate, RandomFlip, and RandomLighting, which added to images so that they could be evaluated from various perspectives. KC et al. [ 45 ] augmented the image data using cropping, flipping, shifting, rotating, and combining these techniques.
Haque et al. [ 46 ] applied several augmentation methods, including flipping (horizontal flip), zooming, shifting (height and breadth), rotating, nearest fill, and shearing, to lessen the overfitting of the guava images in the dataset. Coulibaly et al. [ 19 ] utilized four different operations by which images were augmented, namely, rescale, flipping, shift, and zoom. Ji et al. [ 20 ] increased the number of images of grape leaves with the aid of various data augmentation techniques, including rotation, zooming, flipping, shearing, and color changing. Chen et al. [ 48 ] utilized rotation, flip, scaling, and translation operations to increase the amount of image data in the utilized dataset. Kannan E et al. [ 68 ] utilized two different operations to increase the size of the dataset. Using RandomResizedCrop, where images were cropped to a size between 0.08 and 1; RandomRotation by 30 degrees; and both of these techniques together, the dataset was increased by fourfold.
Marzougui et al. [ 21 ] utilized the “Keras Image Data Generator” class, and operations such as flip, rotation, and shift were carried out to increase the amount of image data. Images were rotated by 30 degrees and flipped horizontally, the fill mode was set to nearest, and shift operations were carried out both vertically and horizontally for better results. Selvam et al. [ 23 ] performed five different augmentation operations, namely, rotation, flipping (horizontally), shear, zoom, and shift (height, width), to increase the count of images of lady’s finger leaves. Lijo [ 25 ] utilized rotation, contrast enhancement, brightness enhancement, and noise reduction to increase the amount of image data. Divakar et al. [ 29 ] utilized the synthetic minority oversampling technique (SMOTE) to increase the count of images in the dataset in a balanced manner. Chowdhury et al. [ 30 ] performed three affine transformation operations—scaling, rotation (clockwise and anticlockwise), and translation (5% to 20% vertically and horizontally)—for the purpose of increasing image data.
Akshai et al. [ 31 ], to enhance the size of the dataset while reducing overfitting, utilized different augmentation techniques, such as rotation, shifting, and zooming. Gonzalez-Huitron et al. [ 54 ] performed horizontal flipping and four-angle rotation throughout the augmentation process. B.V. et al. [ 33 ] utilized the flip operation for the purpose of increasing the count of images in the dataset. Chelleapandi et al. [ 69 ] carried out five different data augmentation operations, including rotation, filling, flipping, zooming, and shearing, using the Keras library to enhance the dataset. Pandian et al. [ 34 ] utilized neural style transfer, position and color augmentation, deep convolutional generative adversarial network, and principal component analysis to increase the number of images from 55,448 to 234,008. Vallabhajosyula et al. [ 56 ] performed four different augmentation approaches— scaling, translation, rotation, and image enhancement—to increase the size of the dataset and to reduce overfitting. Table 6 shows a summarized view of the various data augmentation techniques utilized in different evaluated studies.
Summarized view of different data augmentation techniques.
Observation 3
This observation is purely based on discussions of RQ3 (3.3). Numerous data augmentation methods, such as rotation, mirroring, cropping, flipping, PCA (color augmentation), zooming, shifting, scaling, RandomRotate, translation, etc., were used in the reviewed studies for increasing the dataset’s image count. The overall utilization of the various augmentation techniques used in the reviewed studies is shown in Figure 7 .

The deployment percentage for various augmentation methods.
From the figure, it is clearly evident that rotation was much more frequently utilized for increasing the dataset’s image count than other methods (21%), while flipping came in second, with a value of 10%. Mirroring was employed for augmentation in 8% of the investigations, while zooming and shearing were utilized in 7% of the research, respectively. Additionally, 2% of the evaluated studies utilized affine transformation, mirroring, geometrical transformation, intensity transformation, cropping, PCA, RandomRotate, and translation, whereas only 1% of the other 20 techniques were used individually.
3.4. Discussion for RQ4: What Kinds of Feature Extraction Methods Are Employed?
This section involves a discussion of various feature extraction methods that were utilized by various researchers in their works. Twenty-six papers were found by using feature extraction techniques for filtering, of which nineteen papers are utilized for analysis in this section.
Husin et al. [ 36 ] take color space into account; using this, the illumination from images can be reduced, allowing for an effective determination of whether a leaf is from a chili plant or not. Images were extracted for information pertaining to color matching, color identification, and color information. Dubey et al. [ 3 ] utilized the color coherence vector, global color histogram, complete local binary pattern, and local binary pattern methods for retrieving/extracting features. Sannakki et al. [ 37 ] utilized the color co-occurrence method for extracting texture features. Rastogi et al. [ 58 ] utilized the gray level co-occurrence matrix (GLCM) for extracting features. Es-saddy et al. [ 38 ] extracted three distinct categories of features, namely, shape, color, and texture. While textural features were retrieved using GLCM, color features were extracted using the color histogram, color structure descriptor, and color moments (skewness, mean, and standard deviation). The complexity, area, circularity, and perimeter were used as shape features. Singh et al. [ 59 ] extracted features using the color co-occurrence approach. Krithika et al. [ 60 ] extracted texture features by utilizing GLCM. Ramesh et al. [ 4 ] utilized the histogram of oriented gradients (HOG) as a feature extraction method for creating feature vectors.
Behera et al. [ 5 ] utilized GLCM for extracting textural features. Tulshan et al. [ 6 ] and Devaraj et al. [ 63 ], using GLCM, retrieved the relevant features needed for classification. Kumari et al. [ 70 ] extracted features from the segmented cluster that contained the leaf segment afflicted by the disease after converting the images to greyscale. Color and texture (extracted using GLCM), two distinct types of features, were retrieved from images in the works by Wahab et al. [ 7 ] and Sahithya et al. [ 47 ]. Chen et al. [ 71 ] utilized RESNET18 (CNN) and a task-adaptive procedure for extracting features. Chouhan et al. [ 65 ] utilized scale-invariant feature transform for extracting features. Jain et al. [ 55 ] extracted two main kinds of information from the images: texture features and color features. To extract color features, the skewness, standard deviation, kurtosis, and mean of the color moment equation were used. With the use of a GLCM, the second class of features was extracted. Pandian et al. [ 34 ] utilized several optimal convolutional layers for extracting features. Ashwinkumar et al. [ 66 ] utilized the MobileNet model, which is based on CNNs, for extracting the necessary information from the images. Table 7 shows a summarized view of the various feature extraction techniques applied in different reviewed studies.
Summarized view of different feature extraction techniques.
Observation 4
This discussion is purely based on RQ4 (3.4). The evaluated studies utilized various approaches for extracting features, namely, GLCM, HOG, color co-occurrence, global color histogram, etc.
Figure 8 makes it clear that 43% of the examined research used GLCM to extract features, with color coherence vector coming in second place, with 14% of the total. Additionally, only 4% of the examined research used the global color histogram and local binary pattern, whereas 5% of the studies used the complete local binary pattern, color histogram, color structure descriptor, color moments, HOG, RESNET18, and task-adaptive process. The overall utilization of the different feature extraction techniques in the reviewed studies is shown in Figure 8 .

Utilization of different feature extraction techniques in % (% in descending order).
3.5. Discussion for RQ5: What Are the Typical Attributes That Are Used or Extracted?
This section contains information about different features that were extracted during the feature extraction process. Using extracted features to filter the pool of publications, 20 papers were found for this section, out of which 14 were used for analysis and are represented in Table 8 .
Summary of different extracted features.
Observation 5
This observation is purely based on the discussion for RQ5 (3.5). The reviewed studies extracted various features during the feature extraction stage, namely, color features, shape features, correlation, texture features, energy, variance, mean, geometrical features, and standard deviation. Figure 9 shows the utilization, in percentages, of the different extracted features used in the evaluated studies.

Utilization of various extracted features in % (% in descending order).
The chart shows that 32% of the evaluated studies extracted texture features, 17% color features, and 12% shape features during the feature extraction stage. This indicates that the majority of the examined studies extracted texture features. In addition, 6% of the research that was reviewed extracted each feature, namely correlation, homogeneity, energy, and contrast, while 3% of the studies retrieved feature vectors, variance, mean, geometrical features, and standard deviation.
3.6. Discussion for RQ6: What Automated Systems Have Been Implemented for Identifying and Categorizing Plant Diseases?
This section involves discussion on different machine learning- and deep learning-based approaches that were utilized by various researchers in their works for the identification and classification of diseases. By sifting through them using existing automated algorithms created for identifying and categorizing plant diseases, 45 publications were found to be relevant to this subject. For analysis, 37 papers were considered.
Rumpf et al. [ 2 ] utilized an SVM on hyperspectral data for the purpose of identifying illnesses from sugar beet plant leaves, such as powdery mildew, sugar beet rust, and cercospora leaf spot. Wang et al. [ 35 ] employed backpropagation networks (BPNN) to recognize two distinct diseases in grape leaves and two different types of diseases in wheat. To identify disease in chili leaves, image processing techniques were utilized by Husin et al. [ 36 ]. Dubey et al. [ 3 ] employed multi-class SVM for recognizing and categorizing three diseases, namely, apple rot, apple blotch, and apple scab, which affect apples. Mahlein et al. [ 72 ] examined the leaves of sugar beet plants to identify three different plant illnesses using spectral disease indices. Sannakki et al. [ 37 ] utilized a feed-forward back propagation neural network (BPNN) for identifying powdery mildew and downy mildew from grape leaves. Es-saddy et al. [ 38 ] employed a serial combination of two support vector machines for identifying different types of damage to leaves by Tuta absoluta, leaf miners, and thrips (pest insects), along with late blight and powdery mildew (pathogen symptoms). Fujita et al. [ 9 ] used a CNN to identify seven distinct illnesses from cucumber leaves using CNN. Durmus et al. [ 41 ] employed two types of deep learning models, namely, SqueezeNet and AlexNet, for detecting illnesses, including leaf mold, bacterial spot, early blight, septoria leaf spot, mosaic virus, target spot, late blight, yellow leaf curl, and spider mites, from tomato leaves. Brahimi et al. [ 10 ] utilized CNN to identify nine distinct diseases from tomato leaves.
Liu et al. [ 42 ] employed AlexNet’s deep CNN to recognize mosaic, rust, alternaria leaf spot, and brown spot in apples. Ferentinos [ 61 ] employed DL-based CNN models for identifying diseases in 25 distinct plant species. Ramesh et al. [ 4 ] utilized the random forest as a classifier in order to detect diseases in papaya leaves. Ma et al. [ 13 ] utilized deep CNN to identify downy mildew, anthracnose, powdery mildew, and target leaf spots from cucumber leaves. Sardogan et al. [ 14 ] employed a CNN model based on learning vector quantization (LVQ) to detect and categorize four distinct illnesses in tomato leaves. Behera et al. [ 5 ] utilized SVM with K-means clustering to identify four different diseases in oranges (brown rot, citrus canker, stubborn, and melanoses), while fuzzy logic was used to determine the severity of each disease. Geetharamani et al. [ 16 ] deployed a nine-layer deep CNN to detect illnesses in 13 different plant species. Francis et al. [ 62 ] employed a CNN for the purpose of identifying illness from leaves of the tomato and apple species. Kumari et al. [ 70 ] applied image processing techniques and neural networks for the purpose of identifying illnesses in cotton and tomato leaves.
Zhang et al. [ 17 ] utilized a CNN with GAP (global average pooling) for detecting several diseases from cucumber leaves, such as gray mold, anthracnose, powdery mildew, downy mildew, black spot, and angular leaf spot. Wahab et al. [ 7 ] employed SVM (support vector machine) to locate the cucumber mosaic virus in the chili leaf plant. Adedoja et al. [ 15 ] employed NASNet for the identification of diseases. Howlader et al. [ 64 ] utilized deep CNN to detect several illnesses, including algal leaf spots, rust, and whitefly, from guava leaves, while Haque et al. [ 46 ] employed a convolutional neural network for detecting fruit rot, anthracnose, and fruit canker from the same species. Sahithya et al. [ 47 ] utilized a support vector machine (SVM) and an artificial neural network (ANN) for detecting three different illnesses from lady finger leaves, including powdery mildew, leaf spots, and yellow mosaic vein.
Coulibaly et al. [ 19 ] utilized transfer learning with feature extraction to identify mildew in pearl millet. Ji et al. [ 20 ] employed UnitedModel (CNN) for the purpose of diagnosing three diseases from grape leaves, namely isariopsis, black rot, and esca. Jadhav et al. [ 24 ] employed two types of CNNs, AlexNet and GoogleNet, for the purpose of detecting three different illnesses, namely, frogeye leaf spot, brown spot, and bacterial blight, from soybean leaves. Sun et al. [ 26 ] applied the DM (discount momentum) deep learning optimizer for the purpose of identifying diseases of 26 distinct classes from 14 different crops. Shrestha et al. [ 22 ] utilized a CNN for the detection of different diseases from three different species—potato, tomato, and bell pepper—including early blight and late blight from potato, bell paper bacterial spot, tomato target spot, tomato mosaic virus, tomato yellow leaf curl virus, tomato bacterial spot, late blight and early blight from tomato, tomato leaf mold, tomato spider mites, and tomato septoria leaf spot. Bedi et al. [ 73 ] employed a hybrid technique based on CNN and convolutional autoencoders for the purpose of identifying bacterial spot disease from peach leaves.
Abbas et al. [ 28 ] applied DenseNet to synthetic images produced by the Conditional Generative Adversarial Network in order to detect various diseases from tomato leaves. Chen et al. [ 71 ] utilized LFM-CNAPS (local feature matching conditional neural adaptive processes), which was developed on the basis of meta-learning, for the detection of 60 distinct diseases from 26 different plants. Akshai et al. [ 31 ] employed three distinct models based on convolutional neural networks, namely, VGG, DenseNet, and ResNet, for detecting the black rot, leaf blight, and esca diseases from grape leaves. Kibriya et al. [ 32 ] utilized GoogleNet and VGG16 for the purpose of identifying three different diseases in tomato leaves. Sujatha et al. [ 27 ] utilized three ML and three DL approaches to classify diseases. Ashwinkumar et al. [ 66 ] employed optimal mobile network-based CNN for identifying leaf mold, early blight, target spot, and late blight from the leaves of tomatoes. Table 9 shows a summarized view of the different classification techniques utilized by various researchers for classifying plant diseases.
Summary of different classification techniques.
Observation 6
This observation is solely based on the RQ6 discussion (3.6). As shown in Figure 10 , the evaluated studies utilized numerous approaches for classifying plant diseases, including SVM, BPNN, multi-class SVM, SqueezeNet, AlexNet, ANN, VGG-19, ResNet, DenseNet, and others. The figure illustrates that while SVM was employed in five studies for identifying plant diseases, eight of the evaluated publications utilized convolutional neural networks. Secondly, three studies that were examined used DCNN and AlexNet. Third, the VGG16 model, DenseNet, and GoogleNet were utilized in two reviewed studies. Additionally, all other diagnosis methods, such as backpropagation, multi-class SVM, feed-forward BPNN, two SVMs, SqueezeNet, CNN with GAP, CNN based on LVQ, NasNet, DM optimizer, autoencoders, VGG, ResNet, inception-v3, and optimal mobile network-based CNN, were each used once by the evaluated studies.

Various techniques utilized for classification.
3.7. Discussion for RQ7: What Analytical Techniques Are Employed for Improving Image Quality?
This section involves discussion on the techniques that were utilized by various researchers for improving the quality of the images. By filtering them using methods for improving image quality, 16 publications were found for this section. Eleven papers were finally considered for the study.
Wang et al. [ 35 ] denoised images of wheat and grape leaves with diseased symptoms using a median filter to improve the image quality. Thangadurai et at. [ 74 ] utilized two techniques for image enhancement, namely, color conversion and histogram equalization, to improve the quality of the images. Images from RGB sources were changed to greyscale using color conversion. The images became clearer after histogram equalization. Khirade et al. [ 57 ] enhanced the image quality by histogram equalization. Es-saddy et al. [ 38 ] and Singh et al. [ 59 ] increased the quality of the images during the pre-processing stage. Krithika et al. [ 60 ] utilized the following formula during the pre-processing step to improve the greyscale images:
S k,l = (T k,l − min(T))/(max(T) − min(T)), where T is the original pixel value, S is the new pixel value, and (k,l) are the indices of the pixels.
Tulshan et al. [ 6 ] enhanced the quality of the images that were taken from the dataset, which were utilized as inputs during the pre-processing stage. Malathy et al. [ 1 ] performed pre-processing following image data collection to increase the image quality. Cap et al. [ 75 ] utilized LeafGAN, which significantly improved the quality of the images generated during the data augmentation stage, which also improved the proposed model’s overall performance. Vallabhajosyula et al. [ 56 ] focused on the images’ brightness and contrast during the pre-processing stage, which boosted their quality. Ashwinkumar et al. [ 66 ] employed a bilateral filter to enhance the quality of the images by removing noise from them. In the pre-processing stage, plant leaf images were used as the input images for a bilateral filter, which improved the image quality by eliminating noise. Table 10 represents a summarized view of the various techniques applied in different studies for improving the quality of the images.
Summary of different techniques utilized for improving image quality.
Observation 7
This observation is purely based on the basis of discussion for RQ7 (3.7). In the evaluated studies, the quality of the images was improved using various filters, namely, bilateral and median, by histogram equalization during pre-processing and color conversion. Figure 11 was created on the basis of the information in Table 9 .

Percentages of techniques used for enhancing image quality.
3.8. Discussion for RQ8: What Are the Techniques Utilized for Reducing/Removing Overfitting?
When there is a significant discrepancy in the accuracy values which a model produces for training and testing datasets, it is said to overfit. In this section, the different techniques which were employed by different authors to reduce overfitting are discussed. Twenty-four publications were found for this section after filtering them using techniques to reduce overfitting. Finally, 21 papers were taken into account for analysis.
Sladojevic et al. [ 8 ] introduced some distortion to the images during augmentation to prevent overfitting. Fujita et al. [ 9 ] utilized rotation and flipping operations for data augmentation to lessen overfitting. Durmus et al. [ 41 ] applied activation function layers to increase the model’s non-linearity, while dropout layers and pooling layers were used to lessen overfitting. Fuentes et al. [ 11 ] performed extensive data augmentation in order to prevent overfitting. Liu et al. [ 42 ], by using image processing approaches (expanding the training dataset’s image count), response-normalizing layers (which enabled local normalization), and swapping out some fully connected layers for convolution layers, lessened the overfitting of the model. Ma et al. [ 13 ] minimized overfitting by expanding the dataset of cucumber leaf images using data augmentation techniques. Geetharamani et al. [ 16 ] introduced distorted images to the training dataset during image transformation to avoid overfitting. Francis et al. [ 62 ] avoided overfitting by setting the dropout value at 0.25. Ji et al. [ 20 ] employed several approaches, including an early stop mechanism, data augmentation techniques, and dropout, to minimize the overfitting of the model. Howlader et al. [ 64 ] mitigated overfitting by using the ReLU activation function and data augmentation approaches. The formula for the ReLU activation function was given as:
F(N) = Max(0, N), where N refers to the number of neurons.
Coulibaly et al. [ 19 ] utilized the concept of an early stopping strategy to reduce overfitting. Lijo [ 25 ], Abbas et al. [ 28 ], Pandian et al. [ 34 ], Chen et al. [ 48 ], Vallabhajosyula et al. [ 56 ], and Kannan E et al. [ 68 ] reduced the overfitting of the model using data augmentation techniques. Bedi et al. [ 73 ] employed the concept of early halting, and the patience value was set to 5 to prevent model overfitting. Wang et al. [ 53 ] utilized 1 × 1 convolution to decrease overfitting. Chen et al. [ 71 ] utilized forward propagation in order to avoid overfitting. Chowdhury et al. [ 30 ] utilized GAP for the purpose of reducing overfitting. Table 11 shows a summarized view of the various techniques applied in different research for reducing or removing overfitting.
Summary of ways for reducing or removing overfitting.
Observation 8
This observation is purely based on the basis of discussion for RQ8 (3.8). In the evaluated studies, overfitting was reduced by various approaches, such as by adding distortion to images, data augmentation, global average pooling (GAP), response-normalizing layers, pooling layers, the early stop mechanism, etc. Figure 12 shows various approaches utilized for reducing the overfitting of the model. Figure 12 shows that in various evaluated studies, overfitting was reduced by data augmentation.

Techniques utilized for reducing overfitting.
3.9. Discussion for RQ9: What Are the Different Plant Species That the Evaluated Research Is Based on, and What Classes of Diseases Have Been Found by the Evaluated Studies?
This discussion focuses on the disease classes that were discovered in the specific plant species on which the utilized reviewed studies were based.
The model used by Rumpf et al. [ 2 ] and Mahlein et al. [ 72 ] diagnosed three diseases, namely, sugar beet rust, Cercospora leaf spot, and powdery mildew, from the leaves of sugar beet plants. Wang et al. [ 35 ] suggested an approach which detected diseases from two species, namely, grapes and wheat. Downy and powdery mildew were detected in grapes, whereas leaf rust and stripe rust were found in wheat. Dubey et al. [ 3 ] suggested an approach which diagnosed apple rot, apple scab, and apple blotch from images of apples [ 3 ]. The proposed model of Sannakki et al. [ 37 ] detected two distinct classes of mildew, namely, powdery and downy, from images of grape leaves. Es-saady et al. [ 38 ] diagnosed diseases caused by pest insects (thrips, leaf miners, tutaabsoluta) and pathogens (early and late blight, powdery mildew) from leaf images. Fujita et al. [ 9 ] proposed a model which identified a total of seven distinct classes of diseases from cucumber leaf images, of which four classes were caused by mosaic viruses, including zucchini yellow, cucumber mosaic virus, watermelon mosaic virus, and kyuri green mottle mosaic virus. Three classes were caused by other viruses, including melon yellow spot virus, cucurbit chlorotic yellows virus, and papaya ring spot virus.
Durmus et al. [ 41 ] and Brahimi et al. [ 10 ] proposed a model which was utilized for identifying nine classes of diseases, namely, leaf mold, early and late blight, yellow leaf curl virus, bacterial spot, septoria leaf spot, mosaic virus, target spot, and spider mites, from images of tomato leaves. Liu et al. [ 42 ], using the AlexNet-based model, diagnosed four distinct classes of diseases, namely, rust, alternaria leaf spot, mosaic, and brown spot, from the leaves of apples. Ferentinos [ 61 ] suggested an approach for the purpose of recognizing 58 kinds of diseases from leaf images of 25 different plant species. Ramesh et al. [ 4 ] presented a method for identifying healthy and unhealthy papaya leaves. Ma et al. [ 13 ] proposed a model which diagnosed four distinct categories of cucumber diseases, namely, target leaf spots, downy and powdery mildew, and anthracnose, from cucumber leaf images. Sardogan et al. [ 14 ] presented a model for identifying four groups of diseases from images of tomato leaves, including septoria spot, bacterial spot, tellow curved, and late blight. Behera et al. [ 5 ] proposed an approach for detecting brown rot, citrus canker, melanoses, and stubbornness from images of oranges. Geetharamani et al. [ 16 ] suggested a technique for identifying 38 classes from images of the leaves of 13 distinct plant species. Francis et al. [ 62 ] suggested an approach for categorizing healthy and diseased leaves of two species, namely, apple and tomato.
Ji et al. [ 20 ] presented a UnitedModel for diagnosing three classes of grape diseases, namely, isariopsis leaf spot, esca, and black rot, from images of grape leaves. Kumari et al. [ 70 ] proposed a model for diagnosing diseases from images of cotton and tomato leaves. It identified two classes of cotton diseases, target spot and bacterial leaf spot, and two species of tomato diseases, namely, leaf mold and septoria leaf spot. Zhang et al. [ 17 ] suggested an approach which detected six distinct classes of cucumber diseases. The proposed model of Wahab et al. [ 7 ] identified cucumber mosaic virus from images of leaves of the chili plant. Haque et al. [ 46 ] presented a methodology for diagnosing fruit rot, anthracnose, and fruit canker from images of guava, whereas Howlader et al. [ 64 ] proposed an approach for identifying rust, algal leaf spot, and whitefly from images of guava leaves. Sahithya et al. [ 47 ] diagnosed three distinct classes of diseases, namely, powdery mildew, leaf spot, and yellow mosaic vein, from images of lady finger leaves. Coulibaly et al. [ 19 ] presented an approach for the purpose of identifying mildew in pearl millet. Jadhav et al. [ 24 ] proposed a methodology which identified brown spots, bacterial blight, and frogeye leaf spots from images of soybean leaves.
Kannan E et al. [ 68 ] diagnosed diseases, namely, yellow leaf curl, septoria leaf spot, early blight, mosaic virus, and bacterial spot, from tomato leaf images. Sun et al. [ 26 ] proposed a model which identified 26 classes of disease from the leaves of 14 plant species. Pham et al. [ 51 ] identified three types of diseases from images of mango leaves, including powdery mildew, gall midge, and anthracnose. Shrestha et al. [ 22 ] proposed a model that diagnosed twelve classes of disease from the leaves of three species, namely, potato (two classes), tomato (nine classes), and bell pepper (one class). The 12 classes of diseases included late and early blight (potato); bell pepper bacterial spot; and, in tomato plants, yellow leaf curl virus, target spot, mosaic virus, septoria leaf spot, early blight, bacterial spot, leaf mold, late blight, and spider mites. Bedi et al. [ 73 ] presented a model which detected bacterial spots from images of peach leaves. Vallabhajosyula et al. [ 56 ] proposed a deep ensemble neural network to diagnose 38 classes of diseases from 14 plant species. Abbas et al. [ 28 ] suggested a methodology for identifying nine classes of diseases from images of tomato leaves, namely, yellow leaf curl virus, bacterial spot, septoria leaf spot, two-spotted spider mite, target spot, early blight, leaf mold, late blight, and mosaic virus. Chen et al. [ 71 ] suggested an approach which diagnosed 60 distinct classes of diseases from the leaf images of 26 plant species.
Akshai et al. [ 31 ] proposed CNN-based models, which they utilized for diagnosing black rot, leaf blight, and esca from images of grape leaves, which were acquired from the plant village dataset. Malathy et al. [ 1 ] proposed a CNN for diagnosing diseases, namely, bitter rot, powdery mildew, and sooty blotch, from images of apples. Kibriya et al. [ 32 ] diagnosed early and late blight and bacterial spot, whereas Ashwinkumar et al. [ 66 ] proposed a model for identifying leaf mold, early and late blight, and target spot from images of tomato leaves.
Table 12 shows a summarized view of the plant species and classes of diseases detected and classified by the reviewed studies.
Summary of plant species and classes of detected and classified diseases.
Observation 9
This observation is purely framed on the basis of the discussion for RQ9 (3.9). As per Figure 13 , it is evident that the evaluated studies mostly worked on classifying tomato diseases (13 evaluated studies). Secondly, the number of evaluated studies classifying diseases for the apple and grape species was equal, at seven. Four evaluated studies were conducted to classify diseases in cucumber, orange, peach, pepper, potato, and soybean, whereas two reviewed studies included guava and sugar beet. Lastly, diseases in chili, papaya, cotton, wheat, pearl millet, etc., were diagnosed by one reviewed study. Figure 13 depicts the various species for which diagnoses were made by the evaluated studies.

Species for which diagnosis was performed.
3.10. Discussion for RQ10: What Is the Accuracy of Existing Plant Disease Detection and Classification Approaches?
This section focuses on accuracy of the existing approaches that were proposed by the evaluated studies.
Rumpf et al. [ 2 ], for the diagnosis of several diseases in sugar beet, proposed an SVM model based on hyperspectral reflectance which offered accuracy levels greater than 86%. Wang et al. [ 35 ] used a model for predicting two different grape diseases with an accuracy of 97.14%, while two types of wheat diseases were detected with 100% accuracy using BPNN and image processing technologies. Dubey et al. [ 3 ] proposed an approach that attained 93% accuracy in identifying various diseases in apples, namely, apple rot, apple scab, and apple blotch. Mahlein et al. [ 72 ] proposed a model for detecting sugar beet diseases which achieved accuracy rates for sugar beet rust, powdery mildew, and Cercospora leaf spot of 87%, 85%, and 92%, respectively. Sannakki et al. [ 37 ] proposed a model which achieved 100% accuracy in identifying two different grape illnesses by utilizing the hue feature. Es-saddy et al. [ 38 ] proposed a model that attained an accuracy of 87.80%. The proposed CNN model of Fujita et al. [ 9 ] attained 82.3% accuracy for detecting various cucumber diseases. Durmus et al. [ 41 ] used AlexNet and SqueezeNet, two DL-based models, which attained 95.65 and 94.3 percent accuracy, respectively, whereas a 99.18% accuracy rate was attained by Brahimi et al. [ 10 ], who used CNN for identifying the same illnesses from tomato leaves.
Liu et al. [ 42 ] used an AlexNet-based model which attained 97.62% accuracy for identifying different apple diseases. Ferentinos [ 61 ] proposed a CNN model that obtained an accuracy of 99.53% for detecting 58 classes of diseases. Ramesh et al. [ 4 ] proposed a random forest classifier that provided an accuracy of 70% for detecting healthy and unhealthy papaya leaves. Ma et al. [ 13 ] attained an accuracy of 93.4% using the proposed deep CNN to identify various kinds of cucumber leaf diseases. Sardogan et al. [ 14 ] achieved 86% accuracy in the detection of septoria spot, bacterial spot, yellow curved, and late blight from tomato leaves by utilizing CNN and learning vector quantization. Behera et al. [ 5 ] proposed SVM with k-means clustering obtained an accuracy of 90% for detecting orange diseases. Geetharamani et al. [ 16 ] attained an accuracy of 96.46% for identifying diseases from the leaves of 13 different species of plants. Wahab et al. [ 7 ] proposed model provided an accuracy of 57.1% for detecting cucumber mosaic diseases from chili leaves. Francis et al. [ 62 ] suggested a CNN model which achieved 87% accuracy in the identification of diseases in apple and tomato leaf species. Ji et al. [ 20 ] proposed a UnitedModel, which attained a test accuracy of 98.57%.
The proposed approach of Kumari et al. [ 70 ] attained 90% accuracy in identifying bacterial leaf spots and 80% in diagnosing target spots from cotton leaves, whereas it provided an accuracy of 100% in identifying two distinct classes of tomato diseases from its leaves. Zhang et al. [ 17 ] proposed a model which attained 94.65% accuracy for detecting downy mildew, anthracnose, black spot, powdery mildew, angular leaf spot, and gray mold diseases using a convolutional neural network with global average pooling from cucumber leaves. Haque et al. [ 46 ] proposed a CNN which achieved an accuracy of 95.61% for diagnosing diseases from guava, whereas the suggested deep CNN model of Howlader et al. [ 64 ] attained an accuracy of 98.74% in identifying fruit rot, anthracnose, and fruit canker from guava leaves. Sahithya et al. [ 47 ] proposed SVM and ANN models for identifying various diseases from lady finger leaves, which showed variation when tested using datasets with and without noise images. SVM provided an accuracy of 85% when noise was present in the images and 92% when tested for images without noise, whereas ANN provided 97% accuracy when noise was present in the images and 98% in images without noise. Coulibaly et al. [ 19 ] attained an accuracy of 95% in diagnosing mildew from pearl millet. Jadhav et al. [ 24 ] proposed an AlexNet which attained 98.75% accuracy, whereas GoogleNet attained 96.25% accuracy for identifying diseases from soybean leaves. Kannan E et al. [ 68 ] proposed a CNN model that obtained an accuracy of 97% in detecting diseases, namely, early blight, mosaic virus, septoria leaf spot, yellow leaf curl, and bacterial spot, from tomato leaves.
Sun et al. [ 26 ] proposed a discount momentum deep learning optimizer which attained an accuracy of 97% for detecting 26 classes of diseases. Pham et al. [ 51 ] suggested a model which achieved a testing accuracy of 85.45% for identifying diseases from mango leaves. Shrestha et al. [ 22 ] obtained an accuracy of 88.8% using the proposed CNN model for diagnosing diseases in tomato, potato, and bell pepper leaves. The research of Sujatha et al. [ 27 ] revealed that when it comes to identifying citrus plants, DL models showed a superior performance to ML models. Different ML models, such as SVM, stochastic gradient descent, and random forest, achieved accuracies of 87%, 86.5%, and 76.8%, respectively. In contrast, three DL models, namely, Inception-v3, VGG-16, and VGG-19, provided disease detection accuracies of 89%, 89.5%, and 87.4%, respectively, for the same species. Bedi et al. [ 73 ] suggested an approach based on CNN and convolutional autoencoders which attained an accuracy of 98.38%. Vallabhajosyula et al. [ 56 ] proposed a deep ensemble neural network technique which obtained 99.99% accuracy when the performance was assessed using the PlantVillage dataset. The accuracies of the C-GAN model, provided by Abbas et al. [ 28 ], for five classes, seven classes, and ten classes of tomato leaf images were 99.51%, 98.65%, and 97.11%, respectively.
Chen et al. [ 71 ] suggested a model which attained an accuracy of 93.9% for identifying 60 classes of diseases from 26 different plant species. Akshai et al. [ 31 ] proposed a DenseNet model which achieved 98.27% accuracy for diagnosing black rot, leaf blight, and esca from images of grape leaves. Kibriya et al. [ 32 ] proposed two models for identifying diseases from tomato leaves, namely, GoogleNet and VGG16. GoogleNet obtained an accuracy of 99.23%, whereas VGG-16 attained 98% accuracy. Malathy et al. [ 1 ] proposed a CNN which obtained an accuracy of 97% for diagnosing diseases from images of apples. Ashwinkumar et al. [ 66 ] suggested an optimal mobile network-based CNN, which achieved an accuracy of 98.7% for detecting various diseases, namely, late blight, target spot, leaf mold, and early blight, from images of tomato leaves.
Observation 10
This observation is solely based on the discussion of RQ10. Three categories were used to classify the accuracy levels attained by the various reviewed studies. The accuracies achieved by various existing models are compared in Figure 14 in three classes: ≤85%, 86–90%, and >90%. It was found that 73% of the evaluated studies offered plant disease diagnosis accuracy of more than 90%, while 14% offered accuracies of between 86 and 90%. The percentage of the examined studies with accuracy levels of 85% or less was only 13%.

Classification accuracies of the evaluated studies.
4. Challenges in Existing Approaches
These discussions were solely based on the literature that was reviewed for plant diseases; the conclusions might be different for applications of image processing, ML, and DL in other fields.
- The analysis of disease classification can be impacted by environmental factors such as temperature and humidity;
- It is difficult to identify appropriate and unhealthy portions of leaves because disease symptoms are not well defined;
- Some models were unable to identify a certain stage of a plant leaf disease;
- Some models failed to extract the desired impacted area from images with intricate backgrounds;
- Several of the methods discussed in this review study were trained using the publicly available PlantVillage dataset, but they fell short when put to the test against a real-world environment.
5. Overall Observation and Comparison
This section involves overall observation and comparison. The overall observation was framed on the basis of Observations 1 to 10, as shown in Figure 15 . The comparison section involves a comparison of various parameters, as shown in Figure 16 .

Flowchart showing how the observations were framed.

Flowchart showing how the comparison was framed.
5.1. Overall Observation
The majority of the reviewed studies obtained image data from publicly available datasets, as is evident from Observation 1. Secondly, Observation 2 indicates that resizing was utilized for pre-processing the images, whereas Observation 3 reflects the size of the dataset, or, simply, that the count of images in the dataset was increased using rotation operation during the data augmentation stage in most of the evaluated studies. Thirdly, Observation 4 indicates that during feature extraction, GLCM was widely utilized, and Observation 5 reflects the texture features extracted by most of the evaluated studies. The plant diseases were classified using CNN in many of the publications that were reviewed, as demonstrated by Observation 6. In the majority of the studies that were analyzed, the quality of the images was improved during pre-processing, as shown by Observation 7, while Observation 8 reveals that data augmentation helped to decrease the overfitting of the models. Last but not least, Observation 10 demonstrates that the majority of the reviewed studies offered accuracy levels greater than or equal to 90%.
5.2. Comparison
Table 13 involves a comparison of various reviewed papers on the basis of the species evaluated, the techniques used for identification, the disease identified, the performance measures, and their value.
Comparison of various reviewed papers.
6. Conclusions and Future Scope
Diverse available techniques using ML, DL, and image processing were surveyed in this research to determine their applicability to diagnosing illnesses in various plant species. By looking into the field of agriculture for this effort, 75 pertinent articles were selected for this review. Attention was particularly paid to the data sources, pre-processing methods, feature extraction methods, data augmentation methods, utilized models, and general effectiveness of proposed models. The results showed that most existing models have a modest capacity to process original image data in its unstructured state. For the purpose of separating the desired impacted area from the complicated background of an image, identification techniques based on different approaches required systematic engineering and expert design abilities.
This survey’s objective was to encourage researchers to use various image processing, ML, and DL approaches for identifying and categorizing plant diseases. Most of the reviewed studies worked on images of single leaves for disease detection; in future work, multiple leaves in a single frame may be used for disease detection. These images could be captured in diversified environmental conditions (temperature, humidity, etc.), for the purpose of reducing the impact of environmental conditions on disease detection, and new approaches could be developed which provide detail regarding the stage of the disease. Moreover, in the future, plant disease detection approaches can be integrated with drones and mobile applications to detect diseases in their early stages in large agricultural fields.
Acknowledgments
The authors extend their appreciation to the Deanship of Scientific Research at King Khalid University for funding this work through a large research groups project under grant number (RGP.2/175/44).
Funding Statement
The authors extend their appreciation to the Deanship of Scientific Research at King Khalid University for funding this work through large Research Groups Project under grant number (RGP.2/175/44).
Author Contributions
All authors carried out the review of existing literature and searched for gaps in the existing work. All authors prepared questionnaires for conducting the review and helped to draft the manuscript. All authors have read and agreed to the published version of the manuscript.
Institutional Review Board Statement
Not applicable.
Informed Consent Statement
Data availability statement, conflicts of interest.
The authors declare no conflict of interest.
Disclaimer/Publisher’s Note: The statements, opinions and data contained in all publications are solely those of the individual author(s) and contributor(s) and not of MDPI and/or the editor(s). MDPI and/or the editor(s) disclaim responsibility for any injury to people or property resulting from any ideas, methods, instructions or products referred to in the content.

Two decades of studies suggest health benefits associated with plant-based diets
But researchers caution against broad diet recommendations until remaining knowledge gaps are filled.
Vegetarian and vegan diets are generally associated with better status on various medical factors linked to cardiovascular health and cancer risk, as well as lower risk of cardiovascular diseases, cancer, and death, according to a new review of 49 previously published papers. Angelo Capodici and colleagues present these findings in the open-access journal PLOS ONE on May 15, 2024.
Prior studies have linked certain diets with increased risk of cardiovascular disease and cancer. A diet that is poor in plant products and rich in meat, refined grains, sugar, and salt is associated with higher risk of death. Reducing consumption of animal-based products in favor of plant-based products has been suggested to lower the risk of cardiovascular disease and cancer. However, the overall benefits of such diets remain unclear.
To deepen understanding of the potential benefits of plant-based diets, Capodici and colleagues reviewed 48 papers published between January 2000 and June 2023 that themselves compiled evidence from multiple prior studies. Following an "umbrella" review approach, they extracted and analyzed data from the 48 papers on links between plant-based diets, cardiovascular health, and cancer risk.
Their analysis showed that, overall, vegetarian and vegan diets have a robust statistical association with better health status on a number of risk factors associated with cardiometabolic diseases, cancer, and mortality, such as blood pressure, management of blood sugar, and body mass index. Such diets are associated with reduced risk of ischemic heart disease, gastrointestinal and prostate cancer, and death from cardiovascular disease.
However, among pregnant women specifically, those with vegetarian diets faced no difference in their risk of gestational diabetes and hypertension compared to those on non-plant-based diets.
Overall, these findings suggest that plant-based diets are associated with significant health benefits. However, the researchers note, the statistical strength of this association is significantly limited by the many differences between past studies in terms of the specific diet regimens followed, patient demographics, study duration, and other factors. Moreover, some plant-based diets may introduce vitamin and mineral deficiencies for some people. Thus, the researchers caution against large-scale recommendation of plant-based diets until more research is completed.
The authors add: "Our study evaluates the different impacts of animal-free diets for cardiovascular health and cancer risk showing how a vegetarian diet can be beneficial to human health and be one of the effective preventive strategies for the two most impactful chronic diseases on human health in the 21st century."
- Diet and Weight Loss
- Diseases and Conditions
- Colon Cancer
- Endangered Plants
- Veterinary Medicine
- Colorectal cancer
- Ovarian cancer
- Polyphenol antioxidant
- Stomach cancer
- Cervical cancer
- HPV vaccine
- Breast cancer
Story Source:
Materials provided by PLOS . Note: Content may be edited for style and length.
Journal Reference :
- Angelo Capodici, Gabriele Mocciaro, Davide Gori, Matthew J. Landry, Alice Masini, Francesco Sanmarchi, Matteo Fiore, Angela Andrea Coa, Gisele Castagna, Christopher D. Gardner, Federica Guaraldi. Cardiovascular health and cancer risk associated with plant based diets: An umbrella review . PLOS ONE , 2024; 19 (5): e0300711 DOI: 10.1371/journal.pone.0300711
Cite This Page :
Explore More
- Treating Cataracts and Other Eye Conditions
- Early Arrival of Palaeolithic People On Cyprus
- Networks Regulating Gene Function in Human Brain
- Birth of Universe's Earliest Galaxies
- Why the Brain Can Robustly Recognize B&W Images
- Birth Control Pill for Men?
- Intriguing World Sized Between Earth, Venus
- Billions of Orphan Stars Revealed
- Massive Catalog of Strange Worlds
- Mental Disorders May Spread Thru Social Networks
Trending Topics
Strange & offbeat.

- Weird But True
- Sex & Relationships
- Viral Trends
- Human Interest
- Fashion & Beauty
- Food & Drink
- Health Care
- Men’s Health
- Women’s Health
- Mental Health
- Health & Wellness Products
- Personal Care Products
trending now in Lifestyle

Bride and groom blasted for 15 outrageous wedding-day rules for...

Ozempic dupe costs just $5 at your local gas station — and Gen...

Plus-size influencer rips airport worker who allegedly refused to...

Guy Fieri reveals the key piece of fitness gear that helped him...

I used to work for the Queen — now I live in a bug-infested...

This Asian nation grappling with invasive raccoon population...

I'm a doctor — here's my 'game-changer' trick to quickly fall...

Dear Abby: My husband is creating a hostile home for me and my...
These diets are best for lowering risk of diseases and cancer: study.
- View Author Archive
- Follow on Twitter
- Get author RSS feed
Thanks for contacting us. We've received your submission.

Turns out, vegan and vegetarian diets are un-beet-able when it comes to lowering the risk of cardiovascular diseases and cancer, new research has found .
Dr. Angelo Capodici, of the University of Bologna in Italy, and his colleagues reviewed 48 papers published between January 2000 and June 2023 that investigated the link between plant-based diets, cardiovascular health and cancer risk. They found “significant” health benefits in plant-based diets.

“Our umbrella review seems consistent with other primary evidence that links the consumption of red processed meats to an increased risk of cancers of the gastrointestinal tract,” Capodici and his team wrote in their findings, published Wednesday in PLOS One .
The World Health Organization’s International Agency for Research on Cancer classified processed meat as “carcinogenic to humans” in 2015 , noting that there is “sufficient evidence from epidemiological studies that eating processed meat causes colorectal cancer.” IARC also declared red meat as “probably carcinogenic to humans.”
Nevertheless, Capodici warned that “caution should be paid” before making a large-scale recommendation for plant-based diets because of limitations to the studies and potential vitamin and mineral deficiencies associated with these eating plans.
The pros of eating a diet rich in fruits, vegetables, nuts, seeds, oils, whole grains, legumes and beans have long been espoused.
Capodici and crew said lower blood pressure, better blood sugar management and a healthier body mass index are some outcomes of vegetarian and vegan diets.
They did point out that people who tend to follow these diets are “more prone to engage in healthy lifestyles,” such as regular exercise, avoidance of sugar-sweetened beverages and abstinence from alcohol and tobacco, which also reduces the risk of heart disease.
They also noted that pregnant women who adopted vegetarian diets did not lower their risk of developing gestational diabetes and hypertension compared to women who ate meat.

Get the latest breakthroughs in medicine, diet & nutrition tips and more.
Subscribe to our weekly Post Care newsletter!
Thanks for signing up!
Please provide a valid email address.
By clicking above you agree to the Terms of Use and Privacy Policy .
Never miss a story.
And vegans risk developing anemia due to a lack of vitamin B12, an essential nutrient naturally found in animal products. Vegans are encouraged to eat grains fortified with vitamin B12 or take a daily supplement .
Capodici’s team advised that more research is needed into the effects of vegetarian and vegan diets — they say the studies they analyzed differed in dietary patterns, sample size and participant demographics, among other factors.
Share this article:
Antioxidants
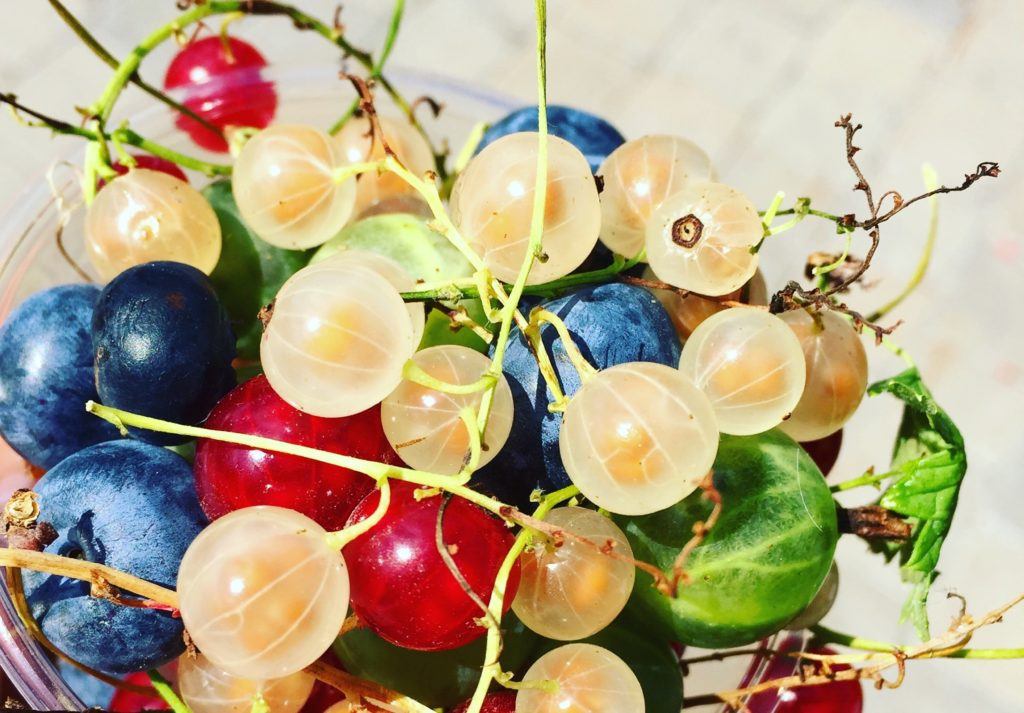
Often used as a marketing buzzword, learn about the role of antioxidants beyond the hype, and some of the research on health and disease prevention.
Jump to: – What are antioxidants? – Health benefits of antioxidants: what’s the buzz? – Studies of antioxidant supplements and disease prevention – Antioxidants in food – Bottom line on antioxidants and disease prevention
What are antioxidants?
The body’s trillion or so cells face formidable threats, from lack of food to infection with a virus. Another constant threat comes from chemicals called free radicals. In very high levels, they are capable of damaging cells and genetic material. The body generates free radicals as the inevitable byproducts of turning food into energy. Free radicals are also formed after exercising or exposure to cigarette smoke, air pollution, and sunlight. [1]
Free radicals come in many shapes, sizes, and chemical configurations. What they all share is a voracious appetite for electrons, stealing them from any nearby substances that will yield them. This electron theft can radically alter the “loser’s” structure or function. Free radical damage can change the instructions coded in a strand of DNA. It can make a circulating low-density lipoprotein (LDL, sometimes called bad cholesterol) molecule more likely to get trapped in an artery wall. Or it can alter a cell’s membrane, changing the flow of what enters the cell and what leaves it. An excessive chronic amount of free radicals in the body causes a condition called oxidative stress, which may damage cells and lead to chronic diseases. [2]
We aren’t defenseless against free radicals. The body, long used to this relentless attack, makes many molecules that quench free radicals as surely as water douses fire. We also extract free-radical fighters from food. These defenders are labeled “antioxidants.” They work by generously giving electrons to free radicals without turning into electron-scavenging substances themselves. They are also involved in mechanisms that repair DNA and maintain the health of cells.
There are hundreds, probably thousands, of different substances that can act as antioxidants. The most familiar ones are vitamin C , vitamin E , beta-carotene , and other related carotenoids, along with the minerals selenium and manganese. They’re joined by glutathione, coenzyme Q10, lipoic acid, flavonoids, phenols, polyphenols, phytoestrogens, and many more. Most are naturally occurring, and their presence in food is likely to prevent oxidation or to serve as a natural defense against the local environment.
But using the term “antioxidant” to refer to substances is misleading. It is really a chemical property, namely, the ability to act as an electron donor. Some substances that act as antioxidants in one situation may be pro-oxidants—electron grabbers—in a different situation. Another big misconception is that antioxidants are interchangeable. They aren’t. Each one has unique chemical behaviors and biological properties. They almost certainly evolved as parts of elaborate networks, with each different substance (or family of substances) playing slightly different roles. This means that no single substance can do the work of the whole crowd.
Health benefits of antioxidants: what’s the buzz?
Antioxidants came to public attention in the 1990s, when scientists began to understand that free radical damage was involved in the early stages of artery-clogging atherosclerosis. It was also linked to cancer , vision loss, and a host of other chronic conditions. Some studies showed that people with low intakes of antioxidant-rich fruits and vegetables were at greater risk for developing these chronic conditions than were people who ate plenty of those foods. Clinical trials began testing the impact of single substances in supplement form, especially beta-carotene and vitamin E, as weapons against chronic diseases.
Even before the results of these trials were in, the media and the supplement and food industries began to hype the benefits of “antioxidants.” Frozen berries, green tea, and other foods labeled as being rich in antioxidants began popping up in stores. Supplement makers touted the disease-fighting properties of all sorts of antioxidants.
The research results were mixed, but most did not find the hoped-for benefits. Most research teams reported that vitamin E and other antioxidant supplements didn’t protect against heart disease or cancer. [3] One study even showed that taking beta-carotene supplements actually increased the chances of developing lung cancer in smokers. On the other hand, some trials reported benefits; for example, after 18 years of follow-up, the Physicians’ Health Study found that taking beta-carotene supplements was associated with a modest reduction in the rate of cognitive decline. [4]
These mostly disappointing results haven’t stopped food companies and supplement sellers from banking on antioxidants. Antioxidants are still added to breakfast cereals, sports bars, energy drinks, and other processed foods , and they are promoted as additives that can prevent heart disease, cancer, cataracts, memory loss, and other conditions.
Often the claims have stretched and distorted the data: While it’s true that the package of antioxidants, minerals , fiber , and other substances found naturally in fruits , vegetables , and whole grains helps prevent a variety of chronic diseases , it is unlikely that high doses of antioxidant supplements can accomplish the same feat.
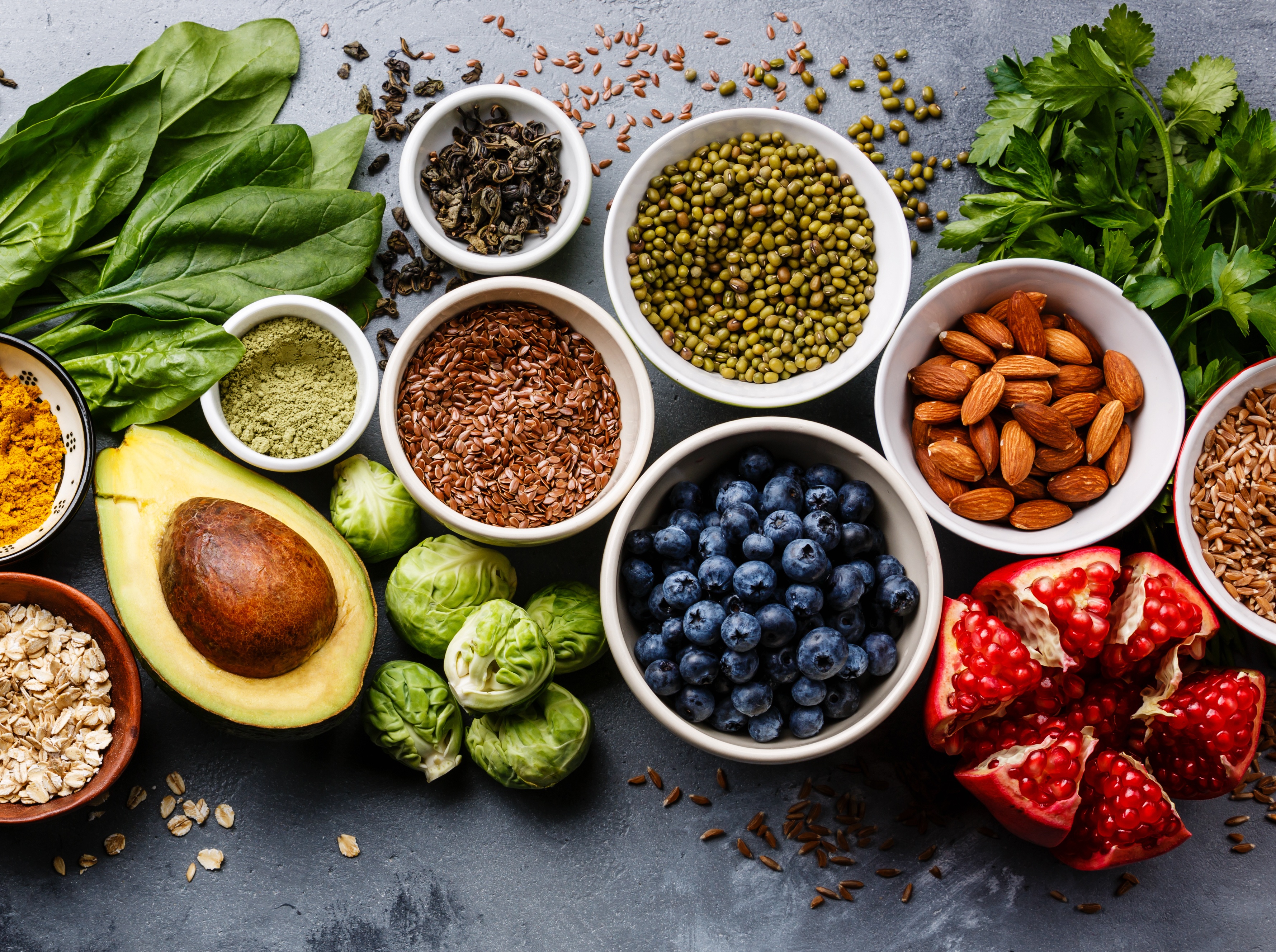
Antioxidant foods hyped to super-status
Antioxidant supplements and disease prevention: little supportive evidence.
Randomized placebo-controlled trials, which can provide the strongest evidence, offer little support that taking vitamin C, vitamin E, beta-carotene, or other single antioxidants provides substantial protection against heart disease, cancer, or other chronic conditions. The results of the largest trials have been mostly negative.
Vitamin E, beta-carotene, and other antioxidants in supplement form aren’t the silver bullet against heart disease and stroke that researchers were hoping for. A modest effect of vitamin E has been found in some studies but more research is needed.
- In the Women’s Health Study, 39,876 initially healthy women took 600 IU of natural source vitamin E or a placebo every other day for 10 years. At the study’s end, the rates of major cardiovascular events and cancer were no lower among those taking vitamin E than those taking the placebo. However, the trial did observe a significant 24% reduction in total cardiovascular mortality. Although this was not a primary endpoint for the trial, it nevertheless represents an important outcome. [6]
- Earlier large vitamin E trials, conducted among individuals with previously diagnosed coronary disease or at high risk for it, generally showed no benefit. In the Heart Outcomes Prevention Evaluation (HOPE) trial, the rates of major cardiovascular events were essentially the same in the vitamin E (21.5%) and placebo (20.6%) groups, although participants taking vitamin E had higher risks of heart failure and hospitalization for heart failure. [7] In the Gruppo Italiano per lo Studio della Sopravvivenza nell’Infarto Miocardico (GISSI) trial, the results were mixed but mostly showed no preventive effects after more than three years of treatment with vitamin E among 11,000 heart attack survivors. [8] However, some studies suggest potential benefits among certain subgroups. A recent trial of vitamin E in Israel, for example, showed a marked reduction in coronary heart disease among people with type 2 diabetes who have a common genetic predisposition for greater oxidative stress. [9]
- Beta-carotene, meanwhile, did not provide any protection against heart disease or stroke, as demonstrated by the Physicians’ Health Study. [10]
- What about combinations? In the Supplementation en Vitamines et Mineraux Antioxydants (SU.VI.MAX) study, 13,017 French men and women took a single daily capsule that contained 120 mg vitamin C, 30 mg vitamin E, 6 mg beta-carotene, 100 mcg selenium, and 20 mg zinc, or a placebo, for seven and a half years. The vitamins had no effect on overall rates of cardiovascular disease. [11]
- In the Women’s Antioxidant Cardiovascular Study, vitamin E, vitamin C, and beta-carotene had similar effects as a placebo on myocardial infarction, stroke, coronary revascularization, and cardiovascular death, although there was a modest and significant benefit for vitamin E among women with existing cardiovascular disease. [12]
A 2014 study from the Journal of Respiratory Research found that different isoforms of vitamin E (called tocopherols) had opposing effects on lung function. [13] The study analyzed data from the Coronary Artery Risk Development in Young Adults (CARDIA) cohort and measured serum levels of alpha- and gamma-tocopherol in 4,526 adults. Lung function was tested using spirometric parameters: higher parameters are indicative of increased lung function, while lower parameters are indicative of decreased lung function. The study found that higher serum levels of alpha-tocopherol were associated with higher spirometric parameters and that high serum levels of gamma-tocopherol were associated with lower spirometric parameters. Though the study was observational in nature, it confirmed the mechanistic pathway of alpha- and gamma-tocopherol in mice studies. [14]
When it comes to cancer prevention, the picture remains inconclusive for antioxidant supplements. Few trials have gone on long enough to provide an adequate test for cancer.
- In the long-term Physicians’ Health Study, cancer rates were similar among men taking beta-carotene and among those taking a placebo. [10] Other trials have also largely shown no effect, including HOPE. [7]
- The SU.VI.MAX randomized placebo-controlled trial showed a reduction in cancer risk and all-cause mortality among men taking an antioxidant cocktail (low doses of vitamins C and E, beta-carotene, selenium, and zinc) but no apparent effect in women, possibly because men tended to have low blood levels of beta-carotene and other vitamins at the beginning of the study. [11]
- A randomized trial of selenium in people with skin cancer demonstrated significant reductions in cancer and cancer mortality at various sites, including colon, lung, and prostate. [15] The effects were strongest among those with low selenium levels at baseline.
- A six-year trial, the Age-Related Eye Disease Study (AREDS), found that a combination of vitamin C, vitamin E, beta-carotene, and zinc offered some protection against the development of advanced age-related macular degeneration, but not cataracts, in people who were at high risk of the disease. [16,17]
- Lutein, a naturally occurring carotenoid found in green, leafy vegetables such as spinach and kale, may protect vision. However, relatively short trials of lutein supplementation for age-related macular degeneration have yielded conflicting findings. [18,19] A follow-up trial to the AREDS, the AREDS2, examined lutein/zeaxanthin supplementation on late age-related macular degeneration (AMD) in men and women for up to five years. [20] It found a favorable but not significant effect of the supplements on AMD.
- A Cochrane review of 19 randomized controlled trials compared antioxidant vitamin/mineral supplements (multivitamin, vitamin E, lutein, zeaxanthin, zinc) with placebo or no intervention in people with AMD. [21] The participants were generally well-nourished. The study found that people taking the vitamins were less likely to progress to late-stage AMD and vision loss. However, the study authors noted that taking lutein and zeaxanthin alone or vitamin E alone did not have a beneficial effect on these eye conditions.
- The Selenium and Vitamin E Cancer Prevention Trial (SELECT) Eye Endpoints Study, which followed 11,267 men for a mean of five years, did not find that vitamin E and selenium supplements, in combination or alone, protected from age-related cataracts. [22]
- The Physicians’ Health Study II, a randomized trial giving 50 mg beta-carotene supplements or a placebo to 5,956 men older than 65 years, found that longer-term supplementation for at least 15 years provided cognitive benefits. [4]
- The Prevention of Alzheimer’s Disease by Vitamin E and Selenium (PREADViSE) trial followed more than 3,700 men ages 60 and older for six years. It did not find that antioxidant supplements of vitamin E or selenium, alone or in combination, protected against dementia compared with a placebo. [23]
- A meta-analysis of 68 antioxidant supplement trials found that taking beta-carotene and vitamin A and E supplements increased the risk of dying. [24] Although healthy participants were included in 21 of the trials, most of the studies included people who already had some type of serious illness. It was also difficult to compare interventions because the types of supplements, the dosages taken, and the length of time they were taken varied widely.
- The same authors conducted another systematic review of 78 randomized clinical trials on antioxidant supplements including beta-carotene, vitamin A, vitamin C, vitamin E, and selenium (alone or in combination). [25] Again, the majority of trials included people with various established diseases. The study found that both people who were healthy and those with diseases taking beta-carotene and vitamin E supplements had a higher rate of death. The duration of the studies varied widely from one month to 12 years, with varying dosages.
If antioxidants were harmless, it wouldn’t much matter if you took them “just in case.” A few studies, though, have raised the possibility that taking antioxidant supplements, either single agents or combinations, could interfere with health.
- The first inkling came in a large trial of beta-carotene conducted among men in Finland who were heavy smokers, and therefore at high risk for developing lung cancer. The trial was stopped early when researchers saw a significant increase in lung cancer among those taking the supplement compared to those taking the placebo. [26]
- In another trial among heavy smokers and people exposed to asbestos, beta-carotene was combined with vitamin A. Again, an increase in lung cancer was seen in the supplement group. [27] Not all trials of beta-carotene show this harmful effect, however. In the Physicians’ Health Study, which included few active smokers, no increase in lung cancer or any other adverse affect was seen even after 18 years of follow-up. [10]
- In the SU.VI.MAX trial, rates of skin cancer were higher in women who were assigned to take vitamin C, vitamin E, beta-carotene, selenium, and zinc. [28]
- Vitamin E supplements were found to significantly increase the risk of prostate cancer by 17% in healthy men compared with those who took a placebo. These results came from the Selenium and Vitamin E Cancer Prevention Trial (SELECT) that followed 35,533 men for up to 12 years. [29]
High-dose antioxidant supplements can also interfere with medicines. Vitamin E supplements can have a blood-thinning effect and increase the risk of bleeding in people who are already taking blood-thinning medicines. Some studies have suggested that taking antioxidant supplements during cancer treatment might interfere with the effectiveness of the treatment. Inform your doctor if starting supplements of any kind. [1]
Antioxidants in food
One possible reason why many studies on antioxidant supplements do not show a health benefit is because antioxidants tend to work best in combination with other nutrients, plant chemicals, and even other antioxidants.
For example, a cup of fresh strawberries contains about 80 mg of vitamin C, a nutrient classified as having high antioxidant activity. But a supplement containing 500 mg of vitamin C (667% of the RDA) does not contain the plant chemicals (polyphenols) naturally found in strawberries like proanthocyanins and flavonoids, which also possess antioxidant activity and may team up with vitamin C to fight disease. Polyphenols also have many other chemical properties besides their ability to serve as antioxidants. There is a question if a nutrient with antioxidant activity can cause the opposite effect with pro-oxidant activity if too much is taken. This is why using an antioxidant supplement with a single isolated substance may not be an effective strategy for everyone.
Differences in the amount and type of antioxidants in foods versus those in supplements might also influence their effects. For example, there are eight chemical forms of vitamin E present in foods. However, vitamin E supplements typically only include one form, alpha-tocopherol. [1]
Epidemiological prospective studies show that higher intakes of antioxidant-rich fruits, vegetables, and legumes are associated with a lower risk of chronic oxidative stress-related diseases like cardiovascular diseases , cancer, and deaths from all causes. [30-33] A plant-based diet is believed to protect against chronic oxidative stress-related diseases. [2] It is not clear if this protective effect is due to the antioxidants, other substances in the foods, or a combination of both. The following are nutrients with antioxidant activity and the foods in which they are found:
- Vitamin C : Broccoli, Brussels sprouts , cantaloupe, cauliflower, grapefruit, leafy greens (turnip, mustard, beet, collards), honeydew, kale , kiwi, lemon, orange, papaya, snow peas, strawberries, sweet potato , tomatoes, and bell peppers (all colors)
- Vitamin E : Almonds , avocado, Swiss chard, leafy greens (beet, mustard, turnip), peanuts, red peppers, spinach (boiled), and sunflower seeds
- Carotenoids including beta-carotene and lycopene: Apricots, asparagus, beets, broccoli, cantaloupe, carrots, bell peppers, kale , mangos, turnip and collard greens, oranges, peaches, pink grapefruit, pumpkin, winter squash , spinach, sweet potato , tangerines, tomatoes, and watermelon
- Selenium: Brazil nuts, fish, shellfish, beef, poultry, barley, brown rice
- Zinc : Beef, poultry, oysters, shrimp, sesame seeds, pumpkin seeds, chickpeas , lentils , cashews, fortified cereals
- Phenolic compounds: Quercetin ( apples , red wine, onions), catechins ( tea , cocoa , berries), resveratrol ( red and white wine , grapes, peanuts, berries), coumaric acid (spices, berries), anthocyanins (blueberries, strawberries)
Bottom line on antioxidants and disease prevention
Excessive free radicals contribute to chronic diseases including cancer, heart disease, cognitive decline, and vision loss. This doesn’t automatically mean that substances with antioxidant properties will fix the problem, especially if they are taken out of their natural context. The studies so far are inconclusive but generally don’t provide strong evidence that antioxidant supplements have a substantial impact on disease. Keep in mind that most of the trials conducted have had fundamental limitations due to their relatively short duration and inclusion of people with existing disease. At the same time, abundant evidence suggests that eating whole in fruits , vegetables , and whole grains —all rich in networks of naturally occurring antioxidants and their helper molecules—provides protection against many scourges of aging.
- National Center for Complementary and Integrative Health (NCCIH). Antioxidants: In Depth. https://nccih.nih.gov/health/antioxidants/introduction.htm Accessed 7/1/19.
- Carlsen MH, Halvorsen BL, Holte K, Bøhn SK, Dragland S, Sampson L, Willey C, Senoo H, Umezono Y, Sanada C, Barikmo I. The total antioxidant content of more than 3100 foods, beverages, spices, herbs and supplements used worldwide. Nutrition journal . 2010 Dec;9(1):3.
- Semba RD, Ferrucci L, Bartali B, Urpí-Sarda M, Zamora-Ros R, Sun K, Cherubini A, Bandinelli S, Andres-Lacueva C. Resveratrol levels and all-cause mortality in older community-dwelling adults. JAMA internal medicine . 2014 Jul 1;174(7):1077-84.
- Grodstein F, Kang JH, Glynn RJ, Cook NR, Gaziano JM. A randomized trial of beta carotene supplementation and cognitive function in men: the Physicians’ Health Study II. Archives of internal medicine . 2007 Nov 12;167(20):2184-90.
- USDA Oxygen Radical Absorbance Capacity (ORAC) of Selected Foods, Release 2 (2010). http://www.orac-info-portal.de/download/ORAC_R2.pdf Accessed 7/1/2019.
- Lee IM, Cook NR, Gaziano JM, Gordon D, Ridker PM, Manson JE, Hennekens CH, Buring JE. Vitamin E in the primary prevention of cardiovascular disease and cancer: the Women’s Health Study: a randomized controlled trial. JAMA . 2005 Jul 6;294(1):56-65.
- Lonn E, Bosch J, Yusuf S, Sheridan P, Pogue J, Arnold JM, Ross C, Arnold A, Sleight P, Probstfield J, Dagenais GR. Effects of long-term vitamin E supplementation on cardiovascular events and cancer: a randomized controlled trial. JAMA . 2005 Mar;293(11):1338-47.
- GISSI-Prevenzione Investigators. Dietary supplementation with n-3 polyunsaturated fatty acids and vitamin E after myocardial infarction: results of the GISSI-Prevenzione trial. The Lancet . 1999 Aug 7;354(9177):447-55.
- Milman U, Blum S, Shapira C, Aronson D, Miller-Lotan R, Anbinder Y, Alshiek J, Bennett L, Kostenko M, Landau M, Keidar S. Vitamin E supplementation reduces cardiovascular events in a subgroup of middle-aged individuals with both type 2 diabetes mellitus and the haptoglobin 2-2 genotype: a prospective double-blinded clinical trial. Arteriosclerosis, thrombosis, and vascular biology . 2008 Feb 1;28(2):341-7.
- Hennekens CH, Buring JE, Manson JE, Stampfer M, Rosner B, Cook NR, Belanger C, LaMotte F, Gaziano JM, Ridker PM, Willett W. Lack of effect of long-term supplementation with beta carotene on the incidence of malignant neoplasms and cardiovascular disease. New England Journal of Medicine . 1996 May 2;334(18):1145-9.
- Hercberg S, Galan P, Preziosi P, Bertrais S, Mennen L, Malvy D, Roussel AM, Favier A, Briançon S. The SU. VI. MAX Study: a randomized, placebo-controlled trial of the health effects of antioxidant vitamins and minerals. Archives of internal medicine . 2004 Nov 22;164(21):2335-42.
- Cook NR, Albert CM, Gaziano JM, Zaharris E, MacFadyen J, Danielson E, Buring JE, Manson JE. A randomized factorial trial of vitamins C and E and beta carotene in the secondary prevention of cardiovascular events in women: results from the Women’s Antioxidant Cardiovascular Study. Archives of internal medicine . 2007 Aug 13;167(15):1610-8.
- Marchese ME, Kumar R, Colangelo LA, Avila PC, Jacobs DR, Gross M, Sood A, Liu K, Cook-Mills JM. The vitamin E isoforms α-tocopherol and γ-tocopherol have opposite associations with spirometric parameters: the CARDIA study. Respiratory research . 2014 Dec;15(1):31.
- Berdnikovs S, Abdala-Valencia H, McCary C, Somand M, Cole R, Garcia A, Bryce P, Cook-Mills JM. Isoforms of vitamin E have opposing immunoregulatory functions during inflammation by regulating leukocyte recruitment. The Journal of Immunology . 2009 Apr 1;182(7):4395-405.
- Duffield-Lillico AJ, Reid ME, Turnbull BW, Combs GF, Slate EH, Fischbach LA, Marshall JR, Clark LC. Baseline characteristics and the effect of selenium supplementation on cancer incidence in a randomized clinical trial: a summary report of the Nutritional Prevention of Cancer Trial. Cancer Epidemiology and Prevention Biomarkers . 2002 Jul 1;11(7):630-9.
- Age-Related Eye Disease Study Research Group. A randomized, placebo-controlled, clinical trial of high-dose supplementation with vitamins C and E, beta carotene, and zinc for age-related macular degeneration and vision loss: AREDS report no. 8. Archives of ophthalmology . 2001 Oct;119(10):1417.
- Age-Related Eye Disease Study Research Group. A randomized, placebo-controlled, clinical trial of high-dose supplementation with vitamins C and E and beta carotene for age-related cataract and vision loss: AREDS report no. 9. Archives of Ophthalmology . 2001 Oct;119(10):1439.
- Richer S, Stiles W, Statkute L, Pulido J, Frankowski J, Rudy D, Pei K, Tsipursky M, Nyland J. Double-masked, placebo-controlled, randomized trial of lutein and antioxidant supplementation in the intervention of atrophic age-related macular degeneration: the Veterans LAST study (Lutein Antioxidant Supplementation Trial). Optometry-Journal of the American Optometric Association . 2004 Apr 1;75(4):216-29.
- Bartlett HE, Eperjesi F. Effect of lutein and antioxidant dietary supplementation on contrast sensitivity in age-related macular disease: a randomized controlled trial. European journal of clinical nutrition . 2007 Sep;61(9):1121.
- Chew EY, Clemons TE, SanGiovanni JP, Danis RP, Ferris FL, Elman MJ, Antoszyk AN, Ruby AJ, Orth D, Bressler SB, Fish GE. Secondary analyses of the effects of lutein/zeaxanthin on age-related macular degeneration progression: AREDS2 report No. 3. JAMA ophthalmology . 2014 Feb 1;132(2):142-9.
- Evans JR, Lawrenson JG. Antioxidant vitamin and mineral supplements for slowing the progression of age‐related macular degeneration. Cochrane Database of Systematic Reviews . 2017(7).
- Christen WG, Glynn RJ, Gaziano JM, Darke AK, Crowley JJ, Goodman PJ, Lippman SM, Lad TE, Bearden JD, Goodman GE, Minasian LM. Age-related cataract in men in the selenium and vitamin e cancer prevention trial eye endpoints study: a randomized clinical trial. JAMA ophthalmology . 2015 Jan 1;133(1):17-24.
- Kryscio RJ, Abner EL, Caban-Holt A, Lovell M, Goodman P, Darke AK, Yee M, Crowley J, Schmitt FA. Association of antioxidant supplement use and dementia in the prevention of Alzheimer’s disease by vitamin E and selenium trial (PREADViSE). JAMA neurology . 2017 May 1;74(5):567-73.
- Bjelakovic G, Nikolova D, Gluud LL, Simonetti RG, Gluud C. Mortality in randomized trials of antioxidant supplements for primary and secondary prevention: systematic review and meta-analysis. JAMA . 2007 Feb 28;297(8):842-57.
- Bjelakovic G, Nikolova D, Gluud LL, Simonetti RG, Gluud C. Antioxidant supplements for prevention of mortality in healthy participants and patients with various diseases. Cochrane database of systematic reviews . 2012(3).
- Albanes D, Heinonen OP, Taylor PR, Virtamo J, Edwards BK, Rautalahti M, Hartman AM, Palmgren J, Freedman LS, Haapakoski J, Barrett MJ. α-Tocopherol and β-carotene supplements and lung cancer incidence in the Alpha-Tocopherol, Beta-Carotene Cancer Prevention Study: effects of base-line characteristics and study compliance. JNCI: Journal of the National Cancer Institute . 1996 Nov 6;88(21):1560-70.
- Omenn GS, Goodman GE, Thornquist MD, Balmes J, Cullen MR, Glass A, Keogh JP, Meyskens Jr FL, Valanis B, Williams Jr JH, Barnhart S. Effects of a combination of beta carotene and vitamin A on lung cancer and cardiovascular disease. New England journal of medicine . 1996 May 2;334(18):1150-5.
- Hercberg S, Ezzedine K, Guinot C, Preziosi P, Galan P, Bertrais S, Estaquio C, Briançon S, Favier A, Latreille J, Malvy D. Antioxidant supplementation increases the risk of skin cancers in women but not in men. The Journal of nutrition . 2007 Sep 1;137(9):2098-105.
- Klein EA, Thompson IM, Tangen CM, Crowley JJ, Lucia MS, Goodman PJ, Minasian LM, Ford LG, Parnes HL, Gaziano JM, Karp DD. Vitamin E and the risk of prostate cancer: the Selenium and Vitamin E Cancer Prevention Trial (SELECT). JAMA . 2011 Oct 12;306(14):1549-56.
- Joshipura KJ, Hu FB, Manson JE, Stampfer MJ, Rimm EB, Speizer FE, Colditz G, Ascherio A, Rosner B, Spiegelman D, Willett WC. The effect of fruit and vegetable intake on risk for coronary heart disease. Annals of internal medicine . 2001 Jun 19;134(12):1106-14.
- Bhupathiraju SN, Wedick NM, Pan A, Manson JE, Rexrode KM, Willett WC, Rimm EB, Hu FB. Quantity and variety in fruit and vegetable intake and risk of coronary heart disease. The American journal of clinical nutrition . 2013 Oct 2;98(6):1514-23.
- Aune D, Giovannucci E, Boffetta P, Fadnes LT, Keum N, Norat T, Greenwood DC, Riboli E, Vatten LJ, Tonstad S. Fruit and vegetable intake and the risk of cardiovascular disease, total cancer and all-cause mortality—a systematic review and dose-response meta-analysis of prospective studies. International journal of epidemiology . 2017 Jun 1;46(3):1029-56.
- Miller V, Mente A, Dehghan M, Rangarajan S, Zhang X, Swaminathan S, Dagenais G, Gupta R, Mohan V, Lear S, Bangdiwala SI. Fruit, vegetable, and legume intake, and cardiovascular disease and deaths in 18 countries (PURE): a prospective cohort study. The Lancet . 2017 Nov 4;390(10107):2037-49.
Terms of Use
The contents of this website are for educational purposes and are not intended to offer personal medical advice. You should seek the advice of your physician or other qualified health provider with any questions you may have regarding a medical condition. Never disregard professional medical advice or delay in seeking it because of something you have read on this website. The Nutrition Source does not recommend or endorse any products.
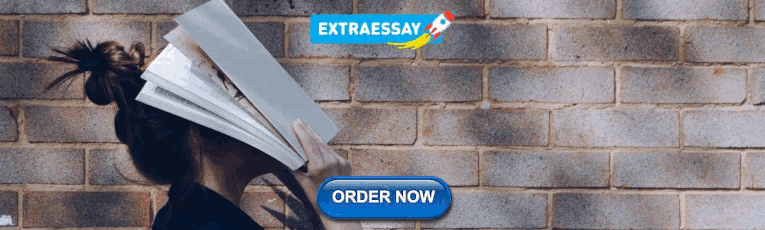
IMAGES
VIDEO
COMMENTS
Plant Disease is the leading international journal for rapid reporting of research on new, emerging, and established plant diseases. The journal publishes papers that describe translational and applied research focusing on practical aspects of disease diagnosis, development, and management in agricultural and horticultural crops.
There are some research papers previously presented to summarize the research based on agriculture (including plant disease recognition) by DL [43,54], but they lacked some of the recent developments in terms of visualization techniques implemented along with the DL and modified/cascaded version of famous DL models, which were used for plant ...
The National Academy of Sciences recently published an ambitious agricultural research agenda that emphasized the need for breakthrough technology for the early and rapid detection and prevention of plant diseases ().Emerging plant diseases are diseases that 1) have increased in either incidence, geographical, or host range; 2) have changed pathogenesis; 3) have newly evolved; or 4) have been ...
a wider plantation size, thus reducing crop production. Therefore, many smart agricultural practices are. deployed to control plant diseases and pests. Most of these approaches, for example, use ...
Among the 57 papers, 16 papers were selected for specific analysis based on the research object (plant disease) and research method (CNN). On this basis, the most recent research in 2022 is ...
Plant disease outbreaks pose significant risks to global food security and environmental sustainability worldwide, and result in the loss of primary productivity and biodiversity that negatively ...
Here, we demonstrate the technical feasibility using a deep learning approach utilizing 54,306 images of 14 crop species with 26 diseases (or healthy) made openly available through the project PlantVillage ( Hughes and Salathé, 2015 ). An example of each crop—disease pair can be seen in Figure 1. Figure 1.
At the same time, an early and deep understanding of plant disease epidemiology is needed to tackle future challenges ahead and to relate directly with the disease control strategies. Comprehensively, the Special Issue collected 13 original contributions (1 review, 1 perspective, and 11 research papers).
This review provides the research progress of deep learning technology in the field of crop leaf disease identification in recent years. In this paper, we present the current trends and challenges for the detection of plant leaf disease using deep learning and advanced imaging techniques.
The Crop Disease dataset comprises photos of 14 crops affected by 27 diseases, whereas the Plant-Pathology-2020 dataset provides images of plant leaves damaged by 38 diseases. All of these datasets are widely utilized by the research community and contribute to the creation and evaluation of DL models for plant disease detection.
The present shortcomings and limitations of offered detection of plant disease models are discussed and presented. In the year of 2020, the authors "MonuBhagat et al.", proposed a paper related to plant leaf disease detection with respect to classical Support Vector Classification methodology [4]. In this paper the authors illustrated such ...
Plant diseases and pests detection is a very important research content in the field of machine vision. It is a technology that uses machine vision equipment to acquire images to judge whether there are diseases and pests in the collected plant images [].At present, machine vision-based plant diseases and pests detection equipment has been initially applied in agriculture and has replaced the ...
For this, in this work, a deep learning-based [ 9, 10, 11] framework is designed to classify the Tomato and Potato leaf diseases. Here, CNN [ 12, 13] based model is developed for the classification of Tomato, Potato, and Bell pepper plant diseases. Kaur et al. [ 14] employed a CNN-based framework to detect diseases in tomato, potato, and grape ...
The following are the primary contributions of this research paper: This study applies YOLOv4, a cutting-edge object detection framework, to plant pathology. ... promoting a cooperative strategy in tackling the worldwide issue of plant diseases. The research highlights the crucial requirement for sophisticated computer vision technologies to ...
This section discusses many research articles to show how previous scholars have handled the issue and used diverse methodologies. The count of diseases in plants has been growing exponentially, thus creating situations like never seen before (Turkoglu et al. 2022).To forecast plant diseases and address the aforementioned issues, researchers have employed a range of strategies and algorithms ...
Plant Diseases Impact Food Security. During the global food crisis of 2007/2008 food price spikes occurred, pushing millions into hunger (11, 12).These price spikes were subsequently associated with political unrest and food riots in the Middle East and Africa (2, 12).This prompted the former US Secretary of State, John Kerry, to remark that "access to food and agriculture needs to be the ...
Journal of Plant Diseases and Protection is an international scientific journal dedicated to applied scientific aspects of plant pathology, plant health, and plant protection. It covers a wide range of interests for the global plant protection community with relevance to European plant health and protection. This journal bridges the gap between ...
Plant Disease Detection using CNN. Emma Harte. School of Computing. National College of Ireland. Mayor Street, IFSC, Dublin 1. Dublin, Ireland. [email protected]. Abstract — Plant ...
The plant disease identification and classification method based on the FL-EfficientNet network proposed in this paper solves the problem of imbalance in the number of samples of different kinds of plant diseases by introducing the Focal loss function in the task of multi-class plant disease classification, and effectively improves the accuracy ...
The research papers for this study were selected using various keywords from peer-reviewed publications from various databases published between 2010 and 2022. A total of 182 papers were identified and reviewed for their direct relevance to plant disease detection and classification, of which 75 papers were selected for this review after ...
A public dataset of 54,306 images o f healthy and diseased plant leaves has be en used to train a deep convolutional neu ral network to. identify 14 crops and 26 diseases. An accuracy of 99.35% ...
New Phytologist is an international journal owned by the New Phytologist Foundation publishing original research in plant science and its ... The nucleotide-binding domain of NRC-dependent disease resistance proteins is sufficient to activate downstream helper NLR oligomerization and immune signaling ... Search for more papers by this author ...
Feature papers represent the most advanced research with significant potential for high impact in the field. A Feature Paper should be a substantial original Article that involves several techniques or approaches, provides an outlook for future research directions and describes possible research applications.
To deepen understanding of the potential benefits of plant-based diets, Capodici and colleagues reviewed 48 papers published between January 2000 and June 2023 that themselves compiled evidence ...
A multidimensional therapeutic approach to inflammation using phytoextracts is described, however, further research is needed to provide more information about the effectiveness of herbal extracts, as well as their combinations, in people with inflammatory joint diseases. Medicinal plants and their secondary metabolites are increasingly used in the treatment of diseases in complex therapy.
Researchers found "significant" health benefits in plant-based diets after reviewing 48 papers published between January 2000 and June 2023. ... cardiovascular diseases and cancer, new research ...
Antioxidant supplements and disease prevention: little supportive evidence. Randomized placebo-controlled trials, which can provide the strongest evidence, offer little support that taking vitamin C, vitamin E, beta-carotene, or other single antioxidants provides substantial protection against heart disease, cancer, or other chronic conditions.