- Student Program
- Sign Up for Free
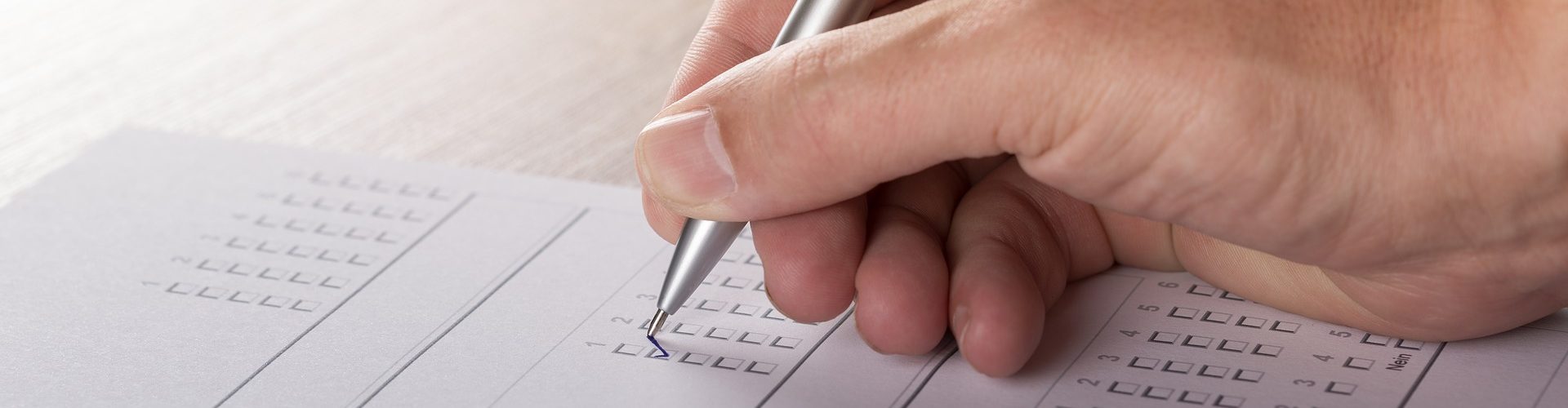
Data Collection Methods
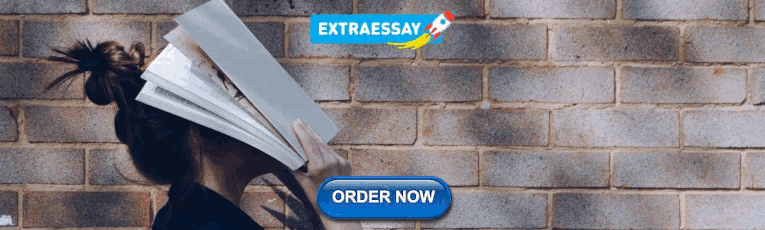
How to use the questionnaire method of data collection
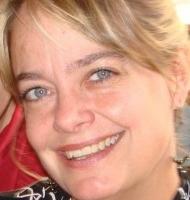
If there’s any area of your business or organization you want to improve — customer retention, marketing initiatives, increased sales, new product development, etc. — getting into the hearts and minds of your current customers and your desired audience is key.
And while there are many ways to gather this kind of intel, one of the best is the questionnaire method of data collection.
Surveys vs questionnaires
If you’ve done any research online about how to collect data from your audience, you’ve likely noticed people use the terms “survey” and “questionnaire” interchangeably. But are they the same thing?
Actually, they’re not.
Although both surveys and questionnaires can help you gather valuable insights into your audience, a questionnaire is one stand-alone method of data collection (among several), while a survey describes the entire process of collecting, aggregating, and analyzing responses from various methods of data collection — and questionnaires are among them.
Learn more about surveys vs questionnaires here , and discover which one you should use to collect data for your next project.
Create an online questionnaire or survey with Jotform to gather responses on any device.
Use cases for questionnaires
You can use the questionnaire method of data collection for a number of purposes:
- To determine what your market wants related to the product or service you provide (for market analysis)
- To get helpful feedback from customers after a purchase
- To get intel on customer demographics and preferences to use for product (or service) development
- To gauge the effectiveness of your customer service and monitor customer satisfaction
- To determine and inform marketing initiatives
- To improve business processes
A few helpful pointers on questionnaires
There’s more than one kind of questionnaire. Which approach you use will depend on your organization’s mission and offerings as well as the kind of information you’re seeking. Customer satisfaction surveys and product use satisfaction surveys are two types of questionnaires you might want to deploy in a business setting.
Your questionnaire can be structured or unstructured (or some combination of the two). For example, a structured questionnaire collects quantitative (numerical) data, while an unstructured questionnaire collects qualitative data (like personal preferences).
There are several question types. Three of the most widely used are open-ended questions (which collect qualitative data and allow respondents to answer more broadly), close-ended questions (i.e., those that require a yes or no response), and multiple-choice questions. Your questionnaire can include all three of these question types — in fact, varying the question type tends to keep respondents more engaged.
Qualities of a good questionnaire
To create a successful questionnaire, you must have in-depth knowledge and insight into your target market or audience first; that’s how you’ll know which questions to ask.
You also have to be clear on what you’re trying to measure — such as which business or operation areas you’re trying to improve (customer service, product development, marketing, etc.) — so you can design your questions to collect feedback in those specific areas.
Once you know what kind of actionable data you need to make improvements, you can incorporate a mix of qualitative and quantitative questions of the various types mentioned above to gather the data you want.
Here are other useful tips for designing an effective questionnaire:
- Make sure every participant sees the same questions.
- Ask neutral, non-leading questions.
- Employ several different question types so respondents stay engaged and complete the survey.
- Include at least a few open-ended questions to gain more in-depth insights into your customers’ habits and practices related to your product or service.
- Arrange your questions from easy to more challenging. For example, start with basic demographic questions before moving into multiple-choice or open-ended questions about the product or customer experience.
One of the biggest things to avoid when designing your questionnaire is making it too long — it needs to be long enough to get the data you require, but not so long that it’s annoying or difficult for respondents to complete.
We’ve all been there: You open an email that politely asks for feedback and promises that it won’t take more than 5–10 minutes. But once you start the questionnaire, you realize it’s really going to take closer to 30 minutes, and you think, “Oh, please. I don’t have time for that.” And you close the survey without finishing it.
Your questionnaire should contain the minimum number of questions that will get you the data you need — no more. You can always ask an open-ended question at the end, like, “Is there anything else you’d like to share that this questionnaire didn’t address?” Just make the response optional.
And be on the lookout for any bias in the questions you develop — you don’t want to reveal an opinion of the business or organization owner (or the person creating the questionnaire).
Successful organizations regularly collect data to help make good business decisions, create new products and services, improve processes and practices, and grow their companies. And as an added benefit, when you query your customers, you show them that their opinion matters. That builds goodwill that will go a long way in creating true customer loyalty.
Ready to survey your audience so your business or organization can enjoy some of the same benefits?
The questionnaire method of data collection is simple when you use powerful tools like Jotform’s questionnaire templates . Choose one template from more than 250 options and customize it with our drag-and-drop form builder — write your own questions, add rating scales and survey tables, and change the fonts and colors to make an engaging, effective questionnaire that’s uniquely yours.
Thank you for helping improve the Jotform Blog. 🎉
- Data Collection
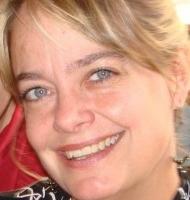
RECOMMENDED ARTICLES
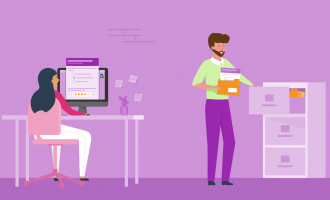
Qualitative vs quantitative data
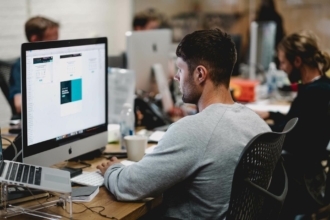
What is purposive sampling? An introduction
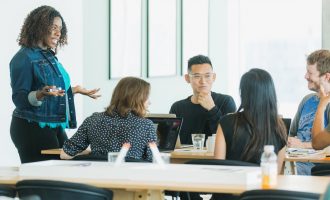
What are focus groups, and how do you conduct them?
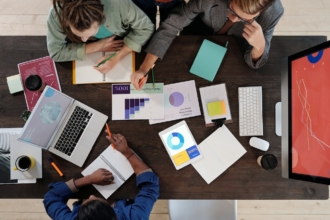
River sampling in market research: Definitions and examples
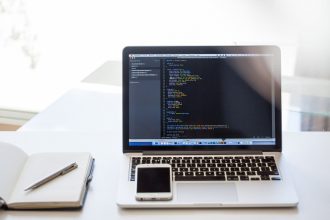
Quantitative data-collection methods
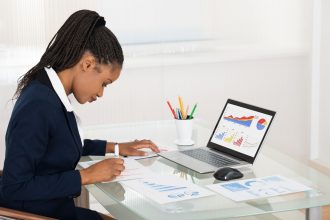
10 of the best data analysis tools
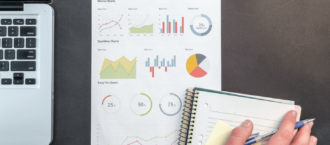
Why is data important to your business?
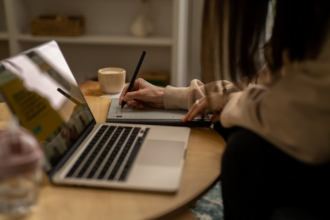
Understanding manual data entry
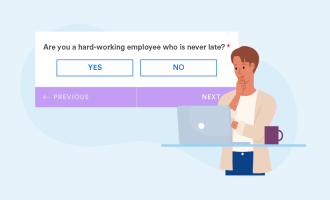
What is a double-barreled question, and how do you avoid it?
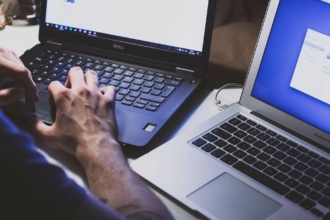
What is systematic sampling?
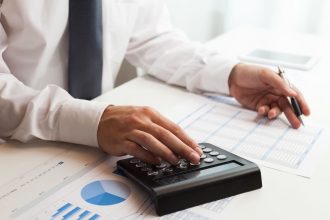
5 of the top data analytics tools for your business
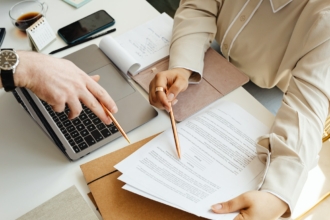
A guide on primary and secondary data-collection methods
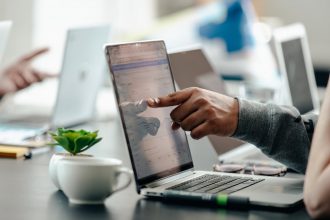
How to be GDPR compliant while collecting data
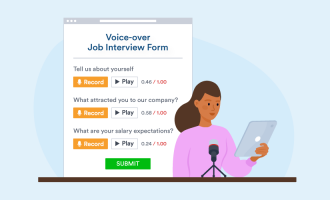
11 best voice recording software options
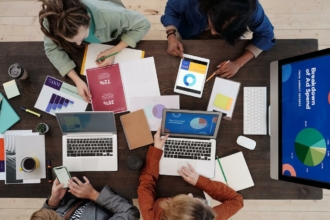
Automated data entry for optimized workflows
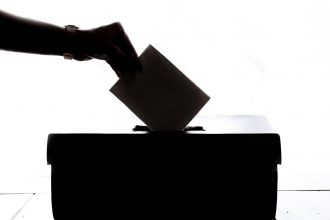
Types of sampling methods
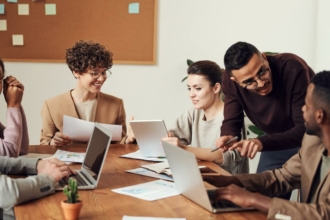
How small businesses can solve data-collection challenges
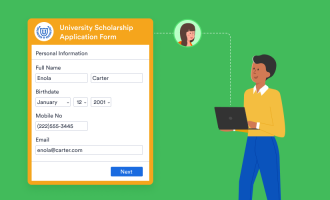
Benefits of data-collection: What makes a good data-collection form?
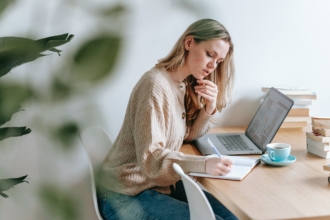
A comprehensive guide to types of research
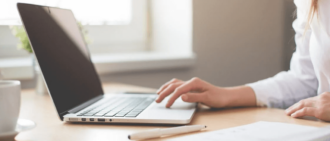
The 12 best Jotform integrations for managing collected data
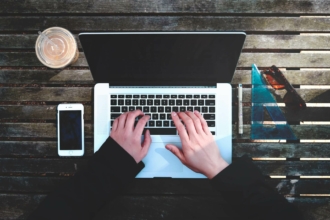
Population vs sample in research: What’s the difference?
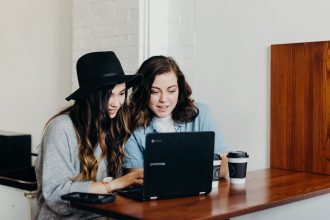
The 5 best data collection tools of 2024
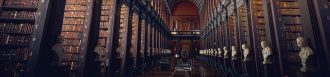
How to conduct an oral history interview
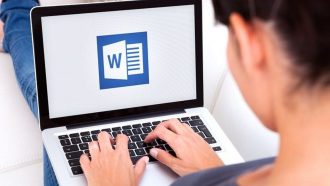
How to create a fillable form in Microsoft Word
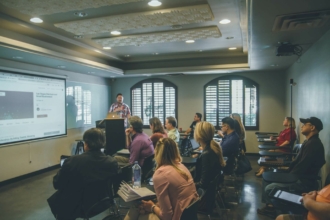
How to get started with business data collection
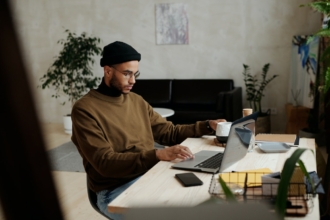
When to use focus groups vs surveys
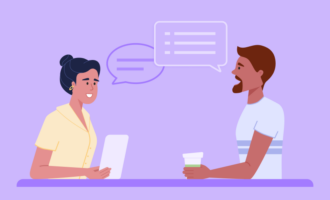
Qualitative data-collection methods
Send Comment :


An official website of the United States government
The .gov means it’s official. Federal government websites often end in .gov or .mil. Before sharing sensitive information, make sure you’re on a federal government site.
The site is secure. The https:// ensures that you are connecting to the official website and that any information you provide is encrypted and transmitted securely.
- Publications
- Account settings
Preview improvements coming to the PMC website in October 2024. Learn More or Try it out now .
- Advanced Search
- Journal List
- Perspect Clin Res
- v.14(3); Jul-Sep 2023
- PMC10405529
Designing and validating a research questionnaire - Part 1
Priya ranganathan.
Department of Anaesthesiology, Tata Memorial Centre, Homi Bhabha National Institute, Mumbai, Maharashtra, India
Carlo Caduff
1 Department of Global Health and Social Medicine, King’s College London, London, United Kingdom
Questionnaires are often used as part of research studies to collect data from participants. However, the information obtained through a questionnaire is dependent on how it has been designed, used, and validated. In this article, we look at the types of research questionnaires, their applications and limitations, and how a new questionnaire is developed.
INTRODUCTION
In research studies, questionnaires are commonly used as data collection tools, either as the only source of information or in combination with other techniques in mixed-method studies. However, the quality and accuracy of data collected using a questionnaire depend on how it is designed, used, and validated. In this two-part series, we discuss how to design (part 1) and how to use and validate (part 2) a research questionnaire. It is important to emphasize that questionnaires seek to gather information from other people and therefore entail a social relationship between those who are doing the research and those who are being researched. This social relationship comes with an obligation to learn from others , an obligation that goes beyond the purely instrumental rationality of gathering data. In that sense, we underscore that any research method is not simply a tool but a situation, a relationship, a negotiation, and an encounter. This points to both ethical questions (what is the relationship between the researcher and the researched?) and epistemological ones (what are the conditions under which we can know something?).
At the start of any kind of research project, it is crucial to select the right methodological approach. What is the research question, what is the research object, and what can a questionnaire realistically achieve? Not every research question and not every research object are suitable to the questionnaire as a method. Questionnaires can only provide certain kinds of empirical evidence and it is thus important to be aware of the limitations that are inherent in any kind of methodology.
WHAT IS A RESEARCH QUESTIONNAIRE?
A research questionnaire can be defined as a data collection tool consisting of a series of questions or items that are used to collect information from respondents and thus learn about their knowledge, opinions, attitudes, beliefs, and behavior and informed by a positivist philosophy of the natural sciences that consider methods mainly as a set of rules for the production of knowledge; questionnaires are frequently used instrumentally as a standardized and standardizing tool to ask a set of questions to participants. Outside of such a positivist philosophy, questionnaires can be seen as an encounter between the researcher and the researched, where knowledge is not simply gathered but negotiated through a distinct form of communication that is the questionnaire.
STRENGTHS AND LIMITATIONS OF QUESTIONNAIRES
A questionnaire may not always be the most appropriate way of engaging with research participants and generating knowledge that is needed for a research study. Questionnaires have advantages that have made them very popular, especially in quantitative studies driven by a positivist philosophy: they are a low-cost method for the rapid collection of large amounts of data, even from a wide sample. They are practical, can be standardized, and allow comparison between groups and locations. However, it is important to remember that a questionnaire only captures the information that the method itself (as the structured relationship between the researcher and the researched) allows for and that the respondents are willing to provide. For example, a questionnaire on diet captures what the respondents say they eat and not what they are eating. The problem of social desirability emerges precisely because the research process itself involves a social relationship. This means that respondents may often provide socially acceptable and idealized answers, particularly in relation to sensitive questions, for example, alcohol consumption, drug use, and sexual practices. Questionnaires are most useful for studies investigating knowledge, beliefs, values, self-understandings, and self-perceptions that reflect broader social, cultural, and political norms that may well diverge from actual practices.
TYPES OF RESEARCH QUESTIONNAIRES
Research questionnaires may be classified in several ways:
Depending on mode of administration
Research questionnaires may be self-administered (by the research participant) or researcher administered. Self-administered (also known as self-reported or self-completed) questionnaires are designed to be completed by respondents without assistance from a researcher. Self-reported questionnaires may be administered to participants directly during hospital or clinic visits, mailed through the post or E-mail, or accessed through websites. This technique allows respondents to answer at their own pace and simplifies research costs and logistics. The anonymity offered by self-reporting may facilitate more accurate answers. However, the disadvantages are that there may be misinterpretations of questions and low response rates. Significantly, relevant context information is missing to make sense of the answers provided. Researcher-reported (or interviewer-reported) questionnaires may be administered face-to-face or through remote techniques such as telephone or videoconference and are associated with higher response rates. They allow the researcher to have a better understanding of how the data are collected and how answers are negotiated, but are more resource intensive and require more training from the researchers.
The choice between self-administered and researcher-administered questionnaires depends on various factors such as the characteristics of the target audience (e.g., literacy and comprehension level and ability to use technology), costs involved, and the need for confidentiality/privacy.
Depending on the format of the questions
Research questionnaires can have structured or semi-structured formats. Semi-structured questionnaires allow respondents to answer more freely and on their terms, with no restrictions on their responses. They allow for unusual or surprising responses and are useful to explore and discover a range of answers to determine common themes. Typically, the analysis of responses to open-ended questions is more complex and requires coding and analysis. In contrast, structured questionnaires provide a predefined set of responses for the participant to choose from. The use of standard items makes the questionnaire easier to complete and allows quick aggregation, quantification, and analysis of the data. However, structured questionnaires can be restrictive if the scope of responses is limited and may miss potential answers. They also may suggest answers that respondents may not have considered before. Respondents may be forced to fit their answers into the predetermined format and may not be able to express personal views and say what they really want to say or think. In general, this type of questionnaire can turn the research process into a mechanical, anonymous survey with little incentive for participants to feel engaged, understood, and taken seriously.
STRUCTURED QUESTIONS: FORMATS
Some examples of close-ended questions include:
e.g., Please indicate your marital status:
- Prefer not to say.
e.g., Describe your areas of work (circle or tick all that apply):
- Clinical service
- Administration
- Strongly agree
- Strongly disagree.
- Numerical scales: Please rate your current pain on a scale of 1–10 where 1 is no pain and 10 is the worst imaginable pain
- Symbolic scales: For example, the Wong-Baker FACES scale to rate pain in older children
- Ranking: Rank the following cities as per the quality of public health care, where 1 is the best and 5 is the worst.
A matrix questionnaire consists of a series of rows with items to be answered with a series of columns providing the same answer options. This is an efficient way of getting the respondent to provide answers to multiple questions. The EORTC QLQ-C30 is an example of a matrix questionnaire.[ 1 ]
For a more detailed review of the types of research questions, readers are referred to a paper by Boynton and Greenhalgh.[ 2 ]
USING PRE-EXISTING QUESTIONNAIRES VERSUS DEVELOPING A NEW QUESTIONNAIRE
Before developing a questionnaire for a research study, a researcher can check whether there are any preexisting-validated questionnaires that might be adapted and used for the study. The use of validated questionnaires saves time and resources needed to design a new questionnaire and allows comparability between studies.
However, certain aspects need to be kept in mind: is the population/context/purpose for which the original questionnaire was designed similar to the new study? Is cross-cultural adaptation required? Are there any permission needed to use the questionnaire? In many situations, the development of a new questionnaire may be more appropriate given that any research project entails both methodological and epistemological questions: what is the object of knowledge and what are the conditions under which it can be known? It is important to understand that the standardizing nature of questionnaires contributes to the standardization of objects of knowledge. Thus, the seeming similarity in the object of study across diverse locations may be an artifact of the method. Whatever method one uses, it will always operate as the ground on which the object of study is known.
DESIGNING A NEW RESEARCH QUESTIONNAIRE
Once the researcher has decided to design a new questionnaire, several steps should be considered:
Gathering content
It creates a conceptual framework to identify all relevant areas for which the questionnaire will be used to collect information. This may require a scoping review of the published literature, appraising other questionnaires on similar topics, or the use of focus groups to identify common themes.
Create a list of questions
Questions need to be carefully formulated with attention to language and wording to avoid ambiguity and misinterpretation. Table 1 lists a few examples of poorlyworded questions that could have been phrased in a more appropriate manner. Other important aspects to be noted are:
Examples of poorly phrased questions in a research questionnaire
- Provide a brief introduction to the research study along with instructions on how to complete the questionnaire
- Allow respondents to indicate levels of intensity in their replies, so that they are not forced into “yes” or “no” answers where intensity of feeling may be more appropriate
- Collect specific and detailed data wherever possible – this can be coded into categories. For example, age can be captured in years and later classified as <18 years, 18–45 years, 46 years, and above. The reverse is not possible
- Avoid technical terms, slang, and abbreviations. Tailor the reading level to the expected education level of respondents
- The format of the questionnaire should be attractive with different sections for various subtopics. The font should be large and easy to read, especially if the questionnaire is targeted at the elderly
- Question sequence: questions should be arranged from general to specific, from easy to difficult, from facts to opinions, and sensitive topics should be introduced later in the questionnaire.[ 3 ] Usually, demographic details are captured initially followed by questions on other aspects
- Use contingency questions: these are questions which need to be answered only by a subgroup of the respondents who provide a particular answer to a previous question. This ensures that participants only respond to relevant sections of the questionnaire, for example, Do you smoke? If yes, then how long have you been smoking? If not, then please go to the next section.
TESTING A QUESTIONNAIRE
A questionnaire needs to be valid and reliable, and therefore, any new questionnaire needs to be pilot tested in a small sample of respondents who are representative of the larger population. In addition to validity and reliability, pilot testing provides information on the time taken to complete the questionnaire and whether any questions are confusing or misleading and need to be rephrased. Validity indicates that the questionnaire measures what it claims to measure – this means taking into consideration the limitations that come with any questionnaire-based study. Reliability means that the questionnaire yields consistent responses when administered repeatedly even by different researchers, and any variations in the results are due to actual differences between participants and not because of problems with the interpretation of the questions or their responses. In the next article in this series, we will discuss methods to determine the reliability and validity of a questionnaire.
Financial support and sponsorship
Conflicts of interest.
There are no conflicts of interest.
- Privacy Policy

Home » Data Collection – Methods Types and Examples
Data Collection – Methods Types and Examples
Table of Contents

Data Collection
Definition:
Data collection is the process of gathering and collecting information from various sources to analyze and make informed decisions based on the data collected. This can involve various methods, such as surveys, interviews, experiments, and observation.
In order for data collection to be effective, it is important to have a clear understanding of what data is needed and what the purpose of the data collection is. This can involve identifying the population or sample being studied, determining the variables to be measured, and selecting appropriate methods for collecting and recording data.
Types of Data Collection
Types of Data Collection are as follows:
Primary Data Collection
Primary data collection is the process of gathering original and firsthand information directly from the source or target population. This type of data collection involves collecting data that has not been previously gathered, recorded, or published. Primary data can be collected through various methods such as surveys, interviews, observations, experiments, and focus groups. The data collected is usually specific to the research question or objective and can provide valuable insights that cannot be obtained from secondary data sources. Primary data collection is often used in market research, social research, and scientific research.
Secondary Data Collection
Secondary data collection is the process of gathering information from existing sources that have already been collected and analyzed by someone else, rather than conducting new research to collect primary data. Secondary data can be collected from various sources, such as published reports, books, journals, newspapers, websites, government publications, and other documents.
Qualitative Data Collection
Qualitative data collection is used to gather non-numerical data such as opinions, experiences, perceptions, and feelings, through techniques such as interviews, focus groups, observations, and document analysis. It seeks to understand the deeper meaning and context of a phenomenon or situation and is often used in social sciences, psychology, and humanities. Qualitative data collection methods allow for a more in-depth and holistic exploration of research questions and can provide rich and nuanced insights into human behavior and experiences.
Quantitative Data Collection
Quantitative data collection is a used to gather numerical data that can be analyzed using statistical methods. This data is typically collected through surveys, experiments, and other structured data collection methods. Quantitative data collection seeks to quantify and measure variables, such as behaviors, attitudes, and opinions, in a systematic and objective way. This data is often used to test hypotheses, identify patterns, and establish correlations between variables. Quantitative data collection methods allow for precise measurement and generalization of findings to a larger population. It is commonly used in fields such as economics, psychology, and natural sciences.
Data Collection Methods
Data Collection Methods are as follows:
Surveys involve asking questions to a sample of individuals or organizations to collect data. Surveys can be conducted in person, over the phone, or online.
Interviews involve a one-on-one conversation between the interviewer and the respondent. Interviews can be structured or unstructured and can be conducted in person or over the phone.
Focus Groups
Focus groups are group discussions that are moderated by a facilitator. Focus groups are used to collect qualitative data on a specific topic.
Observation
Observation involves watching and recording the behavior of people, objects, or events in their natural setting. Observation can be done overtly or covertly, depending on the research question.
Experiments
Experiments involve manipulating one or more variables and observing the effect on another variable. Experiments are commonly used in scientific research.
Case Studies
Case studies involve in-depth analysis of a single individual, organization, or event. Case studies are used to gain detailed information about a specific phenomenon.
Secondary Data Analysis
Secondary data analysis involves using existing data that was collected for another purpose. Secondary data can come from various sources, such as government agencies, academic institutions, or private companies.
How to Collect Data
The following are some steps to consider when collecting data:
- Define the objective : Before you start collecting data, you need to define the objective of the study. This will help you determine what data you need to collect and how to collect it.
- Identify the data sources : Identify the sources of data that will help you achieve your objective. These sources can be primary sources, such as surveys, interviews, and observations, or secondary sources, such as books, articles, and databases.
- Determine the data collection method : Once you have identified the data sources, you need to determine the data collection method. This could be through online surveys, phone interviews, or face-to-face meetings.
- Develop a data collection plan : Develop a plan that outlines the steps you will take to collect the data. This plan should include the timeline, the tools and equipment needed, and the personnel involved.
- Test the data collection process: Before you start collecting data, test the data collection process to ensure that it is effective and efficient.
- Collect the data: Collect the data according to the plan you developed in step 4. Make sure you record the data accurately and consistently.
- Analyze the data: Once you have collected the data, analyze it to draw conclusions and make recommendations.
- Report the findings: Report the findings of your data analysis to the relevant stakeholders. This could be in the form of a report, a presentation, or a publication.
- Monitor and evaluate the data collection process: After the data collection process is complete, monitor and evaluate the process to identify areas for improvement in future data collection efforts.
- Ensure data quality: Ensure that the collected data is of high quality and free from errors. This can be achieved by validating the data for accuracy, completeness, and consistency.
- Maintain data security: Ensure that the collected data is secure and protected from unauthorized access or disclosure. This can be achieved by implementing data security protocols and using secure storage and transmission methods.
- Follow ethical considerations: Follow ethical considerations when collecting data, such as obtaining informed consent from participants, protecting their privacy and confidentiality, and ensuring that the research does not cause harm to participants.
- Use appropriate data analysis methods : Use appropriate data analysis methods based on the type of data collected and the research objectives. This could include statistical analysis, qualitative analysis, or a combination of both.
- Record and store data properly: Record and store the collected data properly, in a structured and organized format. This will make it easier to retrieve and use the data in future research or analysis.
- Collaborate with other stakeholders : Collaborate with other stakeholders, such as colleagues, experts, or community members, to ensure that the data collected is relevant and useful for the intended purpose.
Applications of Data Collection
Data collection methods are widely used in different fields, including social sciences, healthcare, business, education, and more. Here are some examples of how data collection methods are used in different fields:
- Social sciences : Social scientists often use surveys, questionnaires, and interviews to collect data from individuals or groups. They may also use observation to collect data on social behaviors and interactions. This data is often used to study topics such as human behavior, attitudes, and beliefs.
- Healthcare : Data collection methods are used in healthcare to monitor patient health and track treatment outcomes. Electronic health records and medical charts are commonly used to collect data on patients’ medical history, diagnoses, and treatments. Researchers may also use clinical trials and surveys to collect data on the effectiveness of different treatments.
- Business : Businesses use data collection methods to gather information on consumer behavior, market trends, and competitor activity. They may collect data through customer surveys, sales reports, and market research studies. This data is used to inform business decisions, develop marketing strategies, and improve products and services.
- Education : In education, data collection methods are used to assess student performance and measure the effectiveness of teaching methods. Standardized tests, quizzes, and exams are commonly used to collect data on student learning outcomes. Teachers may also use classroom observation and student feedback to gather data on teaching effectiveness.
- Agriculture : Farmers use data collection methods to monitor crop growth and health. Sensors and remote sensing technology can be used to collect data on soil moisture, temperature, and nutrient levels. This data is used to optimize crop yields and minimize waste.
- Environmental sciences : Environmental scientists use data collection methods to monitor air and water quality, track climate patterns, and measure the impact of human activity on the environment. They may use sensors, satellite imagery, and laboratory analysis to collect data on environmental factors.
- Transportation : Transportation companies use data collection methods to track vehicle performance, optimize routes, and improve safety. GPS systems, on-board sensors, and other tracking technologies are used to collect data on vehicle speed, fuel consumption, and driver behavior.
Examples of Data Collection
Examples of Data Collection are as follows:
- Traffic Monitoring: Cities collect real-time data on traffic patterns and congestion through sensors on roads and cameras at intersections. This information can be used to optimize traffic flow and improve safety.
- Social Media Monitoring : Companies can collect real-time data on social media platforms such as Twitter and Facebook to monitor their brand reputation, track customer sentiment, and respond to customer inquiries and complaints in real-time.
- Weather Monitoring: Weather agencies collect real-time data on temperature, humidity, air pressure, and precipitation through weather stations and satellites. This information is used to provide accurate weather forecasts and warnings.
- Stock Market Monitoring : Financial institutions collect real-time data on stock prices, trading volumes, and other market indicators to make informed investment decisions and respond to market fluctuations in real-time.
- Health Monitoring : Medical devices such as wearable fitness trackers and smartwatches can collect real-time data on a person’s heart rate, blood pressure, and other vital signs. This information can be used to monitor health conditions and detect early warning signs of health issues.
Purpose of Data Collection
The purpose of data collection can vary depending on the context and goals of the study, but generally, it serves to:
- Provide information: Data collection provides information about a particular phenomenon or behavior that can be used to better understand it.
- Measure progress : Data collection can be used to measure the effectiveness of interventions or programs designed to address a particular issue or problem.
- Support decision-making : Data collection provides decision-makers with evidence-based information that can be used to inform policies, strategies, and actions.
- Identify trends : Data collection can help identify trends and patterns over time that may indicate changes in behaviors or outcomes.
- Monitor and evaluate : Data collection can be used to monitor and evaluate the implementation and impact of policies, programs, and initiatives.
When to use Data Collection
Data collection is used when there is a need to gather information or data on a specific topic or phenomenon. It is typically used in research, evaluation, and monitoring and is important for making informed decisions and improving outcomes.
Data collection is particularly useful in the following scenarios:
- Research : When conducting research, data collection is used to gather information on variables of interest to answer research questions and test hypotheses.
- Evaluation : Data collection is used in program evaluation to assess the effectiveness of programs or interventions, and to identify areas for improvement.
- Monitoring : Data collection is used in monitoring to track progress towards achieving goals or targets, and to identify any areas that require attention.
- Decision-making: Data collection is used to provide decision-makers with information that can be used to inform policies, strategies, and actions.
- Quality improvement : Data collection is used in quality improvement efforts to identify areas where improvements can be made and to measure progress towards achieving goals.
Characteristics of Data Collection
Data collection can be characterized by several important characteristics that help to ensure the quality and accuracy of the data gathered. These characteristics include:
- Validity : Validity refers to the accuracy and relevance of the data collected in relation to the research question or objective.
- Reliability : Reliability refers to the consistency and stability of the data collection process, ensuring that the results obtained are consistent over time and across different contexts.
- Objectivity : Objectivity refers to the impartiality of the data collection process, ensuring that the data collected is not influenced by the biases or personal opinions of the data collector.
- Precision : Precision refers to the degree of accuracy and detail in the data collected, ensuring that the data is specific and accurate enough to answer the research question or objective.
- Timeliness : Timeliness refers to the efficiency and speed with which the data is collected, ensuring that the data is collected in a timely manner to meet the needs of the research or evaluation.
- Ethical considerations : Ethical considerations refer to the ethical principles that must be followed when collecting data, such as ensuring confidentiality and obtaining informed consent from participants.
Advantages of Data Collection
There are several advantages of data collection that make it an important process in research, evaluation, and monitoring. These advantages include:
- Better decision-making : Data collection provides decision-makers with evidence-based information that can be used to inform policies, strategies, and actions, leading to better decision-making.
- Improved understanding: Data collection helps to improve our understanding of a particular phenomenon or behavior by providing empirical evidence that can be analyzed and interpreted.
- Evaluation of interventions: Data collection is essential in evaluating the effectiveness of interventions or programs designed to address a particular issue or problem.
- Identifying trends and patterns: Data collection can help identify trends and patterns over time that may indicate changes in behaviors or outcomes.
- Increased accountability: Data collection increases accountability by providing evidence that can be used to monitor and evaluate the implementation and impact of policies, programs, and initiatives.
- Validation of theories: Data collection can be used to test hypotheses and validate theories, leading to a better understanding of the phenomenon being studied.
- Improved quality: Data collection is used in quality improvement efforts to identify areas where improvements can be made and to measure progress towards achieving goals.
Limitations of Data Collection
While data collection has several advantages, it also has some limitations that must be considered. These limitations include:
- Bias : Data collection can be influenced by the biases and personal opinions of the data collector, which can lead to inaccurate or misleading results.
- Sampling bias : Data collection may not be representative of the entire population, resulting in sampling bias and inaccurate results.
- Cost : Data collection can be expensive and time-consuming, particularly for large-scale studies.
- Limited scope: Data collection is limited to the variables being measured, which may not capture the entire picture or context of the phenomenon being studied.
- Ethical considerations : Data collection must follow ethical principles to protect the rights and confidentiality of the participants, which can limit the type of data that can be collected.
- Data quality issues: Data collection may result in data quality issues such as missing or incomplete data, measurement errors, and inconsistencies.
- Limited generalizability : Data collection may not be generalizable to other contexts or populations, limiting the generalizability of the findings.
About the author
Muhammad Hassan
Researcher, Academic Writer, Web developer
You may also like

Delimitations in Research – Types, Examples and...

Research Process – Steps, Examples and Tips

Research Design – Types, Methods and Examples

Institutional Review Board – Application Sample...

Evaluating Research – Process, Examples and...

Research Questions – Types, Examples and Writing...
Quantitative Research: Questionnaire Design and Data Collection
- First Online: 18 November 2021
Cite this chapter
- Kerstin Kurzhals 2
Part of the book series: Gabler Theses ((GT))
926 Accesses
1 Citations
Following the introduction and discussion of the general research design and the presentation of the results from the qualitative research, this chapter specifies the quantitative research method applied for testing the conceptual model and hypotheses. The chapter starts with a critical examination of the data collection method, a self-administered online survey, chosen for the quantitative research part, and justifies its use. This is followed by a discussion of the questionnaire design, which incorporates the levels of measurement, theory and statistical analysis, an operationalisation of the measurement constructs and scales used, as well as the pre-test of the survey instrument.
This is a preview of subscription content, log in via an institution to check access.
Access this chapter
- Available as EPUB and PDF
- Read on any device
- Instant download
- Own it forever
- Compact, lightweight edition
- Dispatched in 3 to 5 business days
- Free shipping worldwide - see info
Tax calculation will be finalised at checkout
Purchases are for personal use only
Institutional subscriptions
In their study Pavlou and El Sawy (2011) focus on the group level, explicitly the NPD unit’s attributes, not the firm’s attributes are addressed.
CINT is a professional panel data provider that was conducted for target sampling as described in 5.4.2 .
Author information
Authors and affiliations.
Department of Strategic and Applied Management, Coventry University Business School, Coventry, UK
Kerstin Kurzhals
You can also search for this author in PubMed Google Scholar
Corresponding author
Correspondence to Kerstin Kurzhals .
5.1 Electronic supplementary material
Below is the link to the electronic supplementary material.
Supplementary file1 (PDF 1802 kb)
Rights and permissions.
Reprints and permissions
Copyright information
© 2021 The Author(s), under exclusive license to Springer Fachmedien Wiesbaden GmbH, part of Springer Nature
About this chapter
Kurzhals, K. (2021). Quantitative Research: Questionnaire Design and Data Collection. In: Resource Recombination in Firms from a Dynamic Capability Perspective. Gabler Theses. Springer Gabler, Wiesbaden. https://doi.org/10.1007/978-3-658-35666-8_5
Download citation
DOI : https://doi.org/10.1007/978-3-658-35666-8_5
Published : 18 November 2021
Publisher Name : Springer Gabler, Wiesbaden
Print ISBN : 978-3-658-35665-1
Online ISBN : 978-3-658-35666-8
eBook Packages : Business and Management Business and Management (R0)
Share this chapter
Anyone you share the following link with will be able to read this content:
Sorry, a shareable link is not currently available for this article.
Provided by the Springer Nature SharedIt content-sharing initiative
- Publish with us
Policies and ethics
- Find a journal
- Track your research
- Skip to main content
- Skip to primary sidebar
- Skip to footer
- QuestionPro

- Solutions Industries Gaming Automotive Sports and events Education Government Travel & Hospitality Financial Services Healthcare Cannabis Technology Use Case NPS+ Communities Audience Contactless surveys Mobile LivePolls Member Experience GDPR Positive People Science 360 Feedback Surveys
- Resources Blog eBooks Survey Templates Case Studies Training Help center

Home Surveys
Survey Data Collection: Definition, Methods with Examples and Analysis
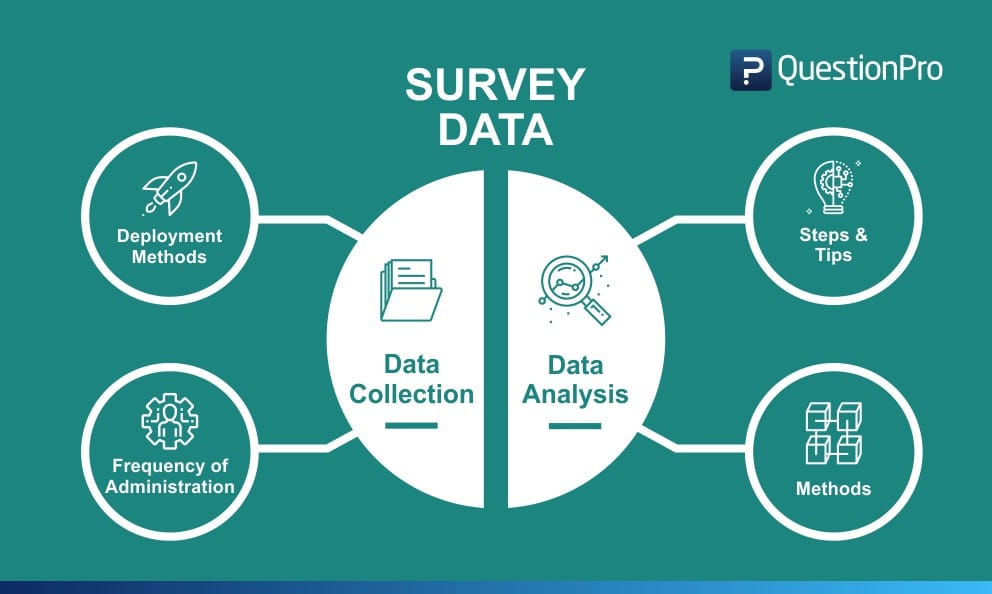
Survey Data: Definition
Survey data is defined as the resultant data that is collected from a sample of respondents that took a survey . This data is comprehensive information gathered from a target audience about a specific topic to conduct research. There are many methods used for survey data collection and statistical analysis .
Various mediums are used to collect feedback and opinions from the desired sample of individuals. While conducting survey research, researchers prefer multiple sources to gather data such as online surveys , telephonic surveys, face-to-face surveys, etc. The medium of collecting survey data decides the sample of people that are to be reached out to, to reach the requisite number of survey responses.
LEARN ABOUT: Survey Sample Sizes
Factors of collecting survey data such as how the interviewer will contact the respondent (online or offline), how the information is communicated to the respondents etc. decide the effectiveness of gathered data.
LEARN ABOUT: telephone survey
Survey Data Collection Methods with Examples
The methods used to collect survey data have evolved with the change in technology. From face-to-face surveys, telephonic surveys to now online and email surveys , the world of survey data collection has changed with time. Each survey data collection method has its pros and cons, and every researcher has a preference for gathering accurate information from the target sample.
The survey response rates for each of these data collection methods will differ as their reach and impact are always different. Different ways are chosen according to specific target population characteristics and the intent to examine human nature under various situations.
Types of survey data based on deployment methods:
There are four main survey data collection methods – Telephonic Surveys, Face-to-face Surveys, and Online Surveys.
- Online Surveys
Online surveys are the most cost-effective and can reach the maximum number of people in comparison to the other mediums. The performance of these surveys is much more widespread than the other data collection methods. In situations where there is more than one question to be asked to the target sample, certain researchers prefer conducting online surveys over the traditional face-to-face or telephone surveys.
Online surveys are effective and therefore require computational logic and branching technologies for exponentially more accurate survey data collection vs any other traditional means of surveying. They are straightforward in their implementation and take a minimum time of the respondents. The investment required for survey data collection using online surveys is also negligible in comparison to the other methods. The results are collected in real-time for researchers to analyze and decide corrective measures.
A very good example of an online survey is a hotel chain using an online survey to collect guest satisfaction metrics after a stay or an event at the property.
Learn more: Quality Of Life Survey Questions + Sample Questionnaire Template
Online surveys are safe and secure to conduct. As there is no in-person interaction or any direct form of communication, they are quite useful in times of global crisis. For instance, many organizations moved to contactless surveys during the pandemic. It helped them ensure that the employees are not experiencing any COVID-19 symptoms before they come to the office.
Learn more: Contactless Health-Screen Survey Questions + Sample Questionnaire Template
- Face-to-face Surveys
Gaining information from respondents via face-to-face mediums is much more effective than the other mediums because respondents usually tend to trust the surveyors and provide honest and clear feedback about the subject in-hand.
Researchers can easily identify whether their respondents are uncomfortable with the asked questions and can be extremely productive in case there are sensitive topics involved in the discussion. This online data collection method demands more cost-investment than in comparison to the other methods. According to the geographic segmentation or psychographic segmentation , researchers must be trained to gain accurate information.
For example, a job evaluation survey is conducted in person between an HR or a manager with the employee. This method works best face-to-face as the data collection can collect as accurate information as possible.
LEARN ABOUT: Workforce Planning Model
- Telephone Surveys
Telephone surveys require much lesser investment than face-to-face surveys. Depending on the required reach, telephone surveys cost as much or a little more than online surveys. Contacting respondents via the telephonic medium requires less effort and manpower than the face-to-face survey medium.
If interviewers are located at the same place, they can cross-check their questions to ensure error-free questions are asked to the target audience. The main drawback of conducting telephone surveys is that establishing a friendly equation with the respondent becomes challenging due to the bridge of the medium. Respondents are also highly likely to choose to remain anonymous in their feedback over the phone as the reliability associated with the researcher can be questioned.
For example, if a retail giant would like to understand purchasing decisions, they can conduct a telephonic, motivation, and buying experience survey to collect data about the entire purchasing experience.
LEARN ABOUT: Anonymous Surveys
- Paper Surveys
The other commonly used survey method is paper surveys. These surveys can be used where laptops, computers, and tablets cannot go, and hence they use the age-old method of data collection ; pen and paper. This method helps collect survey data in field research and helps strengthen the number of responses collected and the validity of these responses.
A popular example or use case of a paper survey is a fast-food restaurant survey, where the fast-food chain would like to collect feedback on its patrons’ dining experience.
Types of survey data based on the frequency at which they are administered:
Surveys can be divided into 3 distinctive types on the basis of the frequency of their distribution. They are:
- Cross-Sectional Surveys
Cross-sectional surveys is an observational research method that analyzes data of variables collected at one given point of time across a sample population or a pre-defined subset. The survey data from this method helps the researcher understand what the respondent feels at a certain point. It helps measure opinions in a particular situation.
For example, if the researcher would like to understand movie rental habits, a survey can be conducted across demographics and geographical locations. The cross-sectional study , for example, can help understand that males between 21-28 rent action movies and females between 35-45 rent romantic comedies. This survey data helps for the basis of a longitudinal study .
LEARN ABOUT: Real Estate Surveys
- Longitudinal Surveys
Longitudinal surveys are those surveys that help researchers to make an observation and collect data over an extended period of time. This survey data can be qualitative or quantitative in nature, and the survey creator does not interfere with the survey respondents.
For example, a longitudinal study can be carried out for years to help understand if mine workers are more prone to lung diseases. This study takes a year and discounts any pre-existing conditions.
- Retrospective Surveys
In retrospective surveys, researchers ask respondents to report events from the past. This survey method offers in-depth survey data but doesn’t take as long to complete. By deploying this kind of survey, researchers can gather data based on past experiences and beliefs of people.
For example, if hikers are asked about a certain hike – the conditions of the hiking trail, ease of hike, weather conditions, trekking conditions, etc. after they have completed the trek, it is a retrospective study.
LEARN ABOUT: Powerful Survey Generator
Survey Data Analysis
After the survey data has been collected, this data has to be analyzed to ensure it aids towards the end research objective. There are different ways of conducting this research and some steps to follow. They are as below:
Survey Data Analysis: Steps and Tips
There are four main steps of survey data analysis :
- Understand the most popular survey research questions: The survey format questions should align with the overall purpose of the survey. That is when the collected data will be effective in helping researchers. For example, if a seminar has been conducted, the researchers will send out a post-seminar feedback survey. The primary goal of this survey will be to understand whether the attendees are interested in attending future seminars. The question will be: “How likely are you to attend future seminars?” – Data collected for this question will decide the likelihood of success of future seminars.
- Filter obtained results using the cross-tabulation technique: Understand the various categories in the target audience and their thoughts using cross-tabulation format. For example, if there are business owners, administrators, students, etc. who attend the seminar, the data about whether they would prefer attending future seminars or not can be represented using cross-tabulation.
- Evaluate the derived numbers: Analyzing the gathered information is critical. How many of the attendees are of the opinion that they will be attending future seminars and how many will not – these facts need to be evaluated according to the results obtained from the sample.
- Draw conclusions: Weave a story with the collected and analyzed data. What was the intention of the survey research, and how does the survey data suffice that objective? – Understand that and develop accurate, conclusive results.
Survey Data Analysis Methods
Conducting a survey without having access to the resultant data and the inability to drawing conclusions from the survey data is pointless. When you conduct a survey, it is imperative to have access to its analytics. It is tough to analyze using traditional survey methods like pen and paper and also requires additional manpower. Survey data analysis becomes much easier when using advanced online data collection methods with an online survey platform such as market research survey software or customer survey software.
LEARN ABOUT: Top 12 Tips to Create A Good Survey
Statistical analysis can be conducted on the survey data to make sense of the data that has been collected. There are multiple data analysis methods of quantitative data . Some of the commonly used types are:
- Cross-tabulation: Cross-tabulation is the most widely used data analysis methods. It uses a basic tabulation framework to make sense of data. This statistical analysis method helps tabulate data into easily understandable rows and columns, and this helps draw parallels between different research parameters. It contains data that is mutually exclusive or have some connection with each other.
- Trend analysis: Trend analysis is a statistical analysis method that provides the ability to look at survey-data over a long period of time. This method helps plot aggregated response data over time allows drawing a trend line of the change, if any, of perceptions over time about a common variable.
- MaxDiff analysis: The MaxDiff analysis method is used to gauge what a customer prefers in a product or a service across multiple parameters. For example, a product’s feature list, the difference with the competition, ease of use and likert scale , pricing, etc. form the basis for maxdiff analysis. In a simplistic form, this method is also called the “best-worst” method. This method is very similar to conjoint analysis, but it is much easier to implement and can be interchangeably used.
LEARN ABOUT: System Usability Scale
- Conjoint analysis: As mentioned above, conjoint analysis is similar to maxdiff analysis, only differing in its complexity and the ability to collect and analyze advance survey data. This method analyzes each parameter behind a person’s purchasing behavior. By using conjoint analysis, it is possible to understand what exactly is important to a customer and the aspects that are evaluated before purchase.
- TURF analysis: TURF analysis or Total Unduplicated Reach and Frequency analysis, is a statistical research methodology that assesses the total market reach of a product or service or a mix of both. This method is used by organizations to understand the frequency and the avenues at which their messaging reaches customers and prospective customers. This helps them tweak their go-to-market strategies.
- Gap analysis: Gap analysis uses a side-by-side matrix question type that helps measure the difference between expected performance and actual performance. This statistical method for survey data helps understand the things that have to be done to move performance from actual to planned performance.
- SWOT analysis: SWOT analysis , another widely used statistical method, organizes survey data into data that represents the strength, weaknesses, opportunities, and threats of an organization or product or service that provides a holistic picture of competition. This method helps to create effective business strategies.
- Text analysis: Text analysis is an advanced statistical method where intelligent tools make sense of and quantify or fashion qualitative and open-ended data into easily understandable data. This method is used when the survey data is unstructured.
MORE LIKE THIS
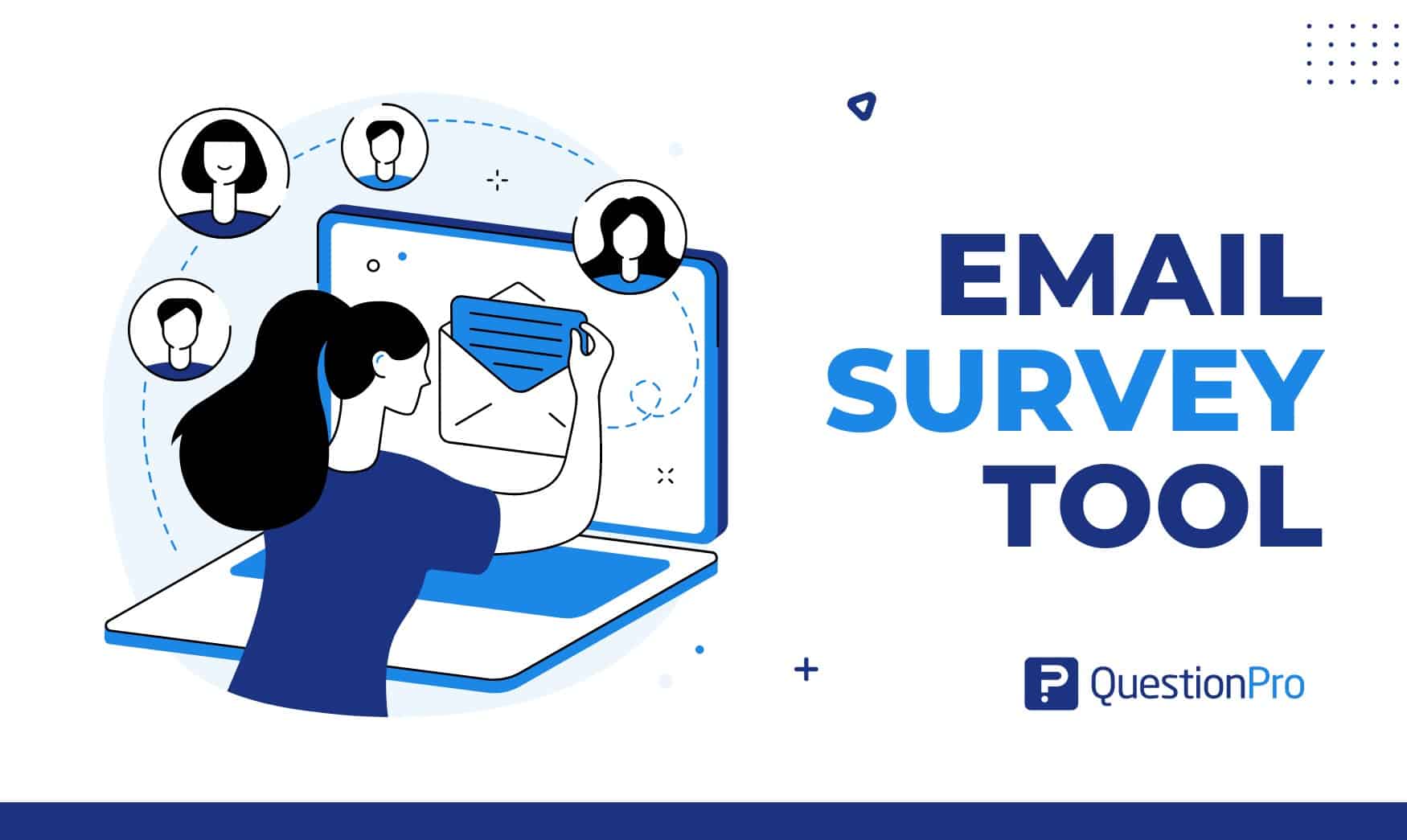
The Best Email Survey Tool to Boost Your Feedback Game
May 7, 2024
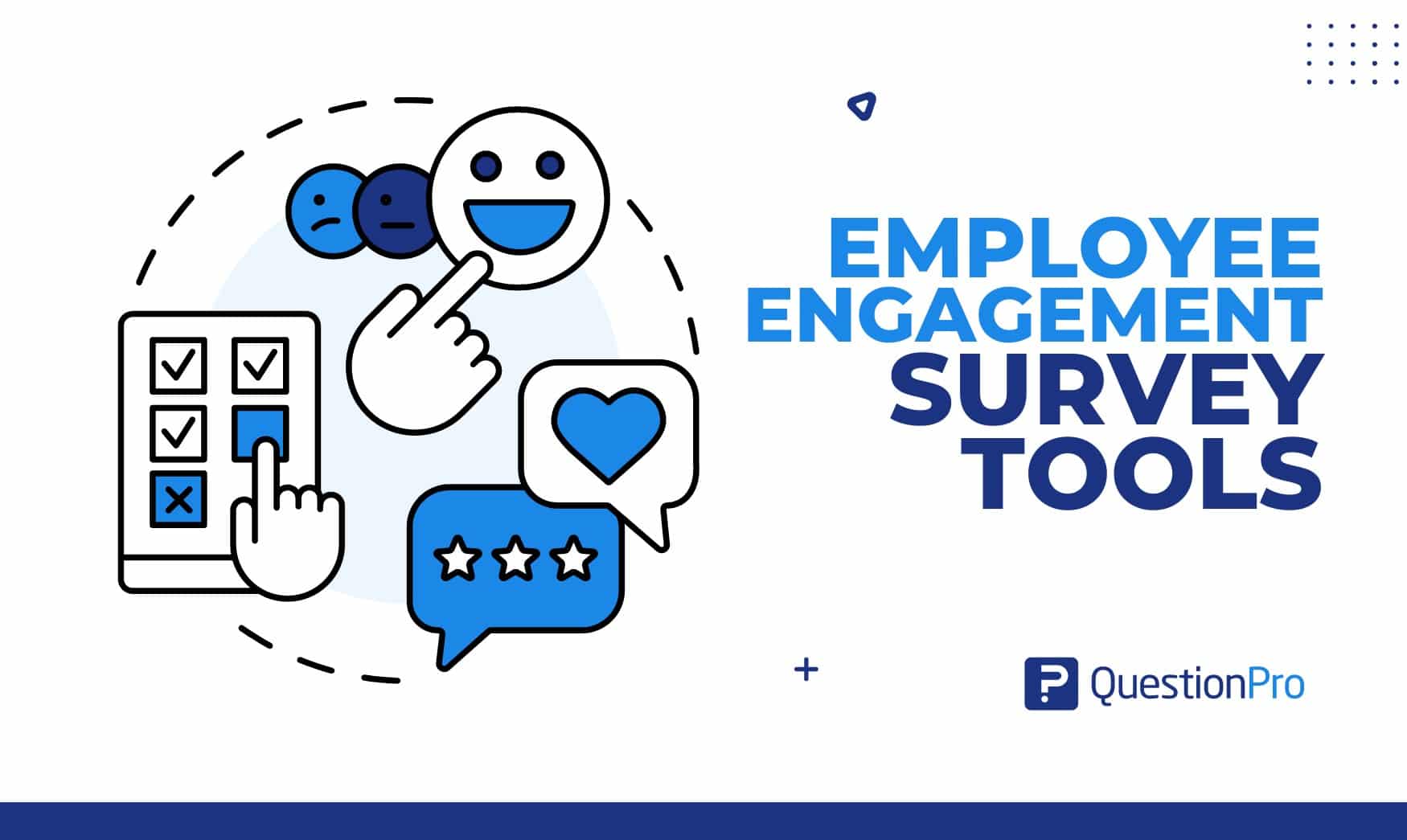
Top 10 Employee Engagement Survey Tools
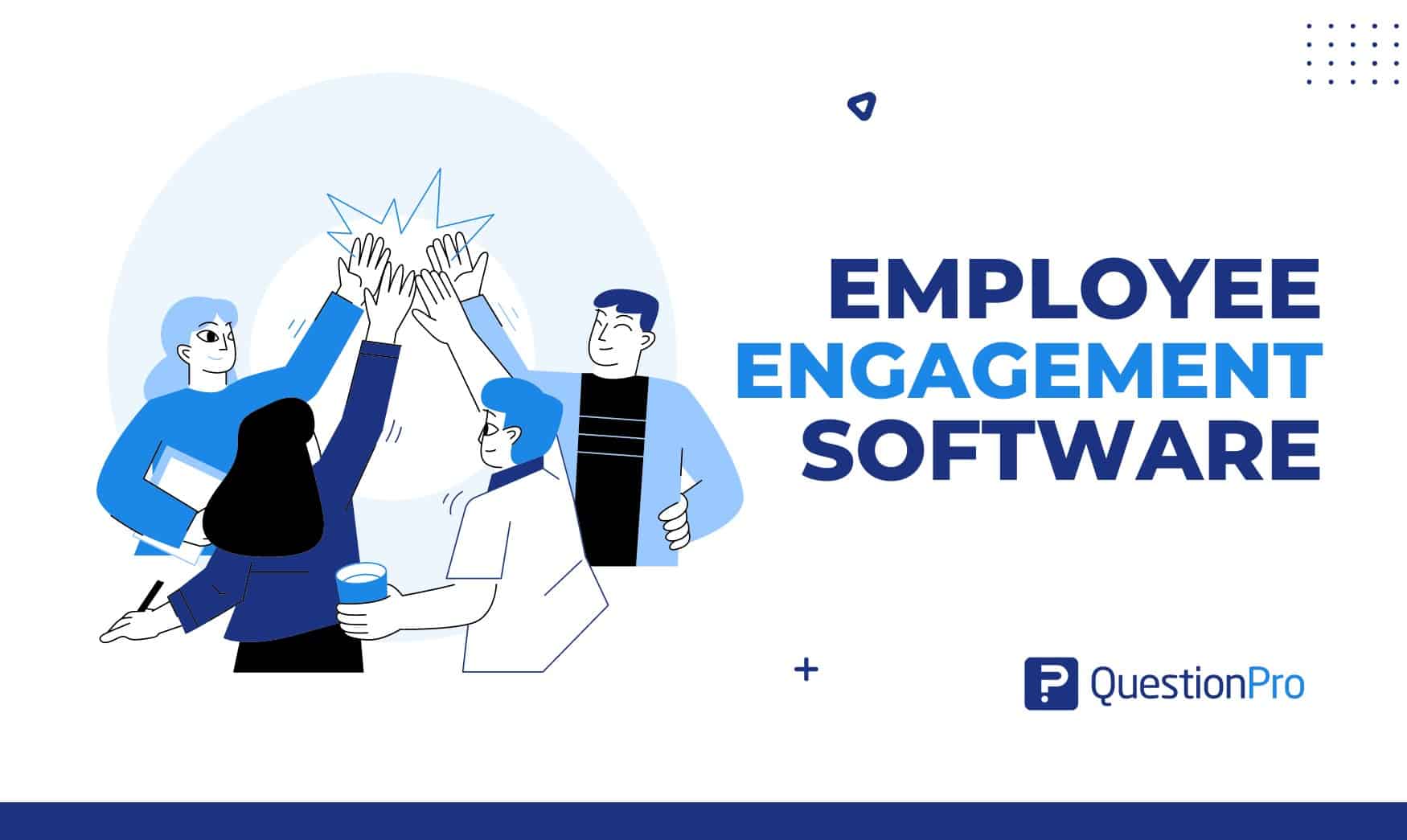
Top 20 Employee Engagement Software Solutions
May 3, 2024
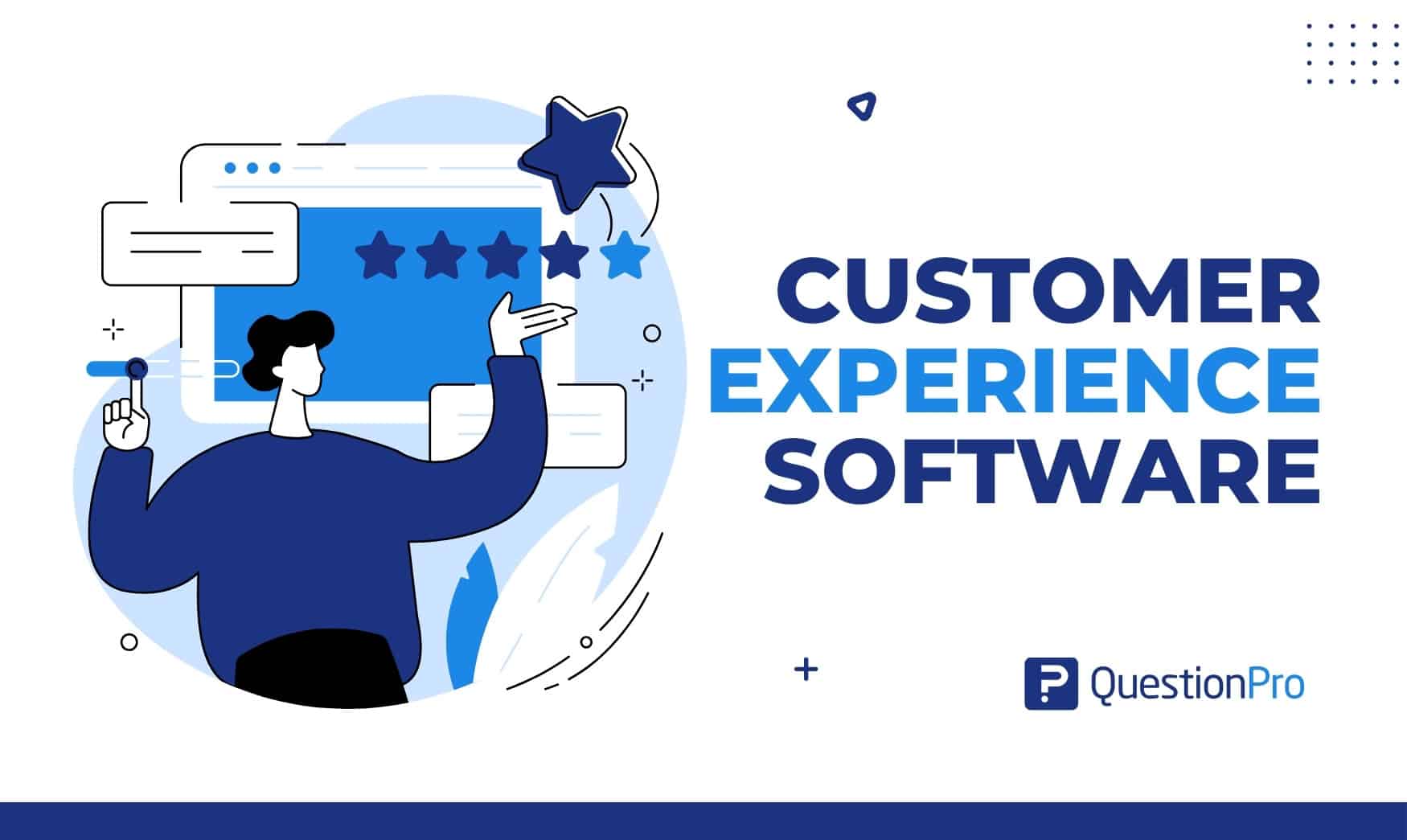
15 Best Customer Experience Software of 2024
May 2, 2024
Other categories
- Academic Research
- Artificial Intelligence
- Assessments
- Brand Awareness
- Case Studies
- Communities
- Consumer Insights
- Customer effort score
- Customer Engagement
- Customer Experience
- Customer Loyalty
- Customer Research
- Customer Satisfaction
- Employee Benefits
- Employee Engagement
- Employee Retention
- Friday Five
- General Data Protection Regulation
- Insights Hub
- Life@QuestionPro
- Market Research
- Mobile diaries
- Mobile Surveys
- New Features
- Online Communities
- Question Types
- Questionnaire
- QuestionPro Products
- Release Notes
- Research Tools and Apps
- Revenue at Risk
- Survey Templates
- Training Tips
- Uncategorized
- Video Learning Series
- What’s Coming Up
- Workforce Intelligence
Accessibility
Ask antea group, what are you looking for.
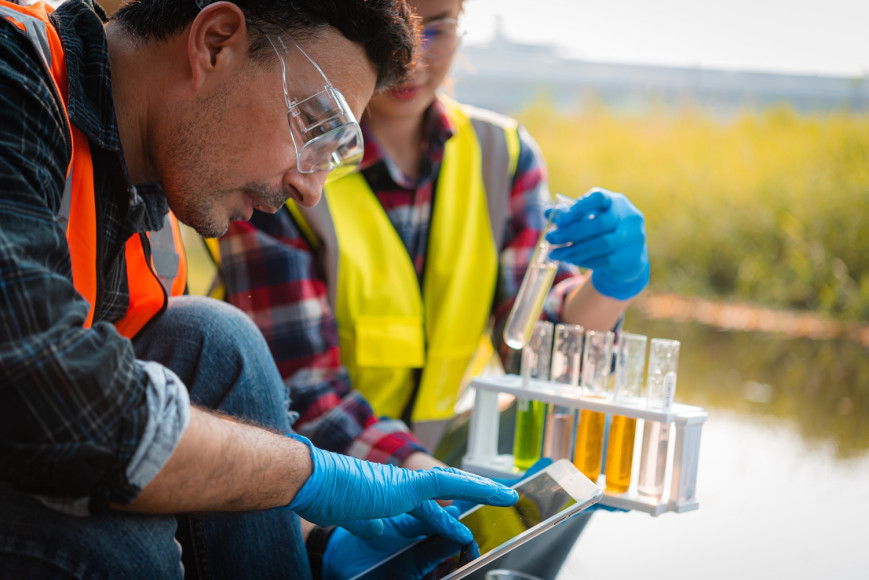
Digging Deeper: EPA's Dive into PFAS Pollution through Data Collection
- Tuesday May 14th 2024
Per- and polyfluoroalkyl substances (PFAS) have been the subject of growing concern due to their persistence in the environment and potential health risks. Now, the Environmental Protection Agency (EPA) is taking a significant step forward in understanding and addressing PFAS contamination through a comprehensive data gathering initiative involving publicly owned treatment works (POTWs) and industrial facilities.
In a recent Federal Register notice, the EPA announced its plans to collect data on PFAS discharges from thousands of upstream industrial facilities, as well as collecting data on the presence of these chemicals in POTW influent, effluent, and sewage sludge. This initiative lays the groundwork for potential Clean Water Act limits on PFAS across various sectors and sources.
Why is This Data Collection Effort Necessary?
According to the EPA, there is currently limited publicly accessible data on PFAS discharges to POTWs, the relative contributions of residential, commercial, and industrial sources, and the fate of PFAS in wastewater treatment processes. By gathering comprehensive data, the EPA aims to fill these knowledge gaps and inform future regulatory actions.
The EPA's initiative involves gathering basic data from 400 POTWs through a questionnaire, the selection of approximately 200 to 300 POTWs based on questionnaire responses, and the collection of sampling data from said subset of 200 to 300 POTWs. According to the EPA, this data will be instrumental in developing technology-based effluent limitations guidelines (ELGs) for different industry sectors and informing risk assessments and management options.
One of the key findings driving this initiative is that most POTWs currently lack processes and technologies to effectively remove PFAS from wastewater. As a result, PFAS are discharged into surface waters or accumulate in sewage sludge, posing potential risks depending on sewage sludge management practices.
The data collected will not only help identify and prioritize industrial sources of PFAS, but will also establish a national dataset of sewage sludge characteristics. This dataset will be crucial for informing future risk assessments and regulatory decisions regarding sewage sludge management.
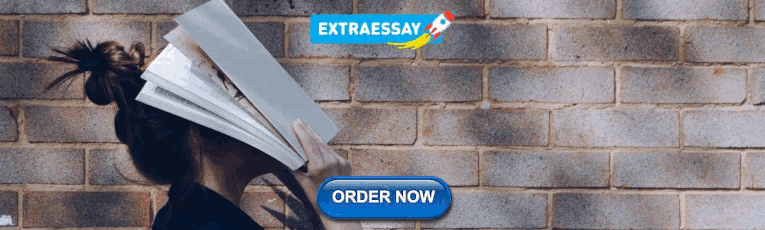
EPA Data Collection Methodology
The EPA's approach involves a two-phase sampling program, with selected POTWs collecting and analyzing samples of industrial user effluent, domestic wastewater influent, POTW influent, POTW effluent, and sewage sludge for specific PFAS and ancillary parameters.
To ensure the success of this initiative, the EPA has consulted extensively with stakeholders, including representatives from the POTW industry, state regulators, and environmental agencies. This collaborative approach ensures that the data collection efforts are comprehensive and relevant to the needs of all stakeholders involved. The EPA hopes to begin collecting sampling data in 2024 and 2025.
How to Respond if Your Organization Receives a Directive to Sample
If you operate an industrial facility and are selected as part of this program for the collection of effluent samples, there are a few key items to keep in mind:
- Determine the correct location for the collection of effluent samples. Ideally, this will be an easy location to access and will be located as close as possible to the connection with the municipal sanitary sewer.
- Collect an influent water sample as well as it is not uncommon to observe PFAS in water coming into a facility from municipal-supplied water sources.
- Collect quality assurance samples. Matrix spike and matrix spike duplicates, duplicate samples, and blank samples are great ways to validate and confirm data integrity.
- Sample collection should be completed by a trained professional experienced with potential PFAS sources to eliminate doubt in the sample results and prevent potential cross contamination.
For more guidance, check out our recent blog post . While many of the above items may not be required as part of this program and likely will not be required to be reported to the EPA (such as quality assurance samples and influent water samples), the collection of these samples will help validate potential sources of PFAS and data integrity.
In conclusion, the EPA's ambitious data gathering initiative marks a significant step forward in addressing PFAS contamination in water treatment systems. By collecting comprehensive data from POTWs and industrial facilities, the EPA aims to better understand the sources and fate of PFAS, paving the way for informed regulatory decisions and effective management strategies to protect public health and the environment.
Have questions? Reach out to our team of experts today to get them answered !
Want more news and insights like this.
Sign up for our monthly e-newsletter, The New Leaf. Our goal is to keep you updated, educated, and even a bit entertained as it relates to all things EHS and sustainability.
Share this page
Have any questions?
Contact us to discuss your environment, health, safety, and sustainability needs today.

Kipp Sande Senior Project Manager Contact me
Related content
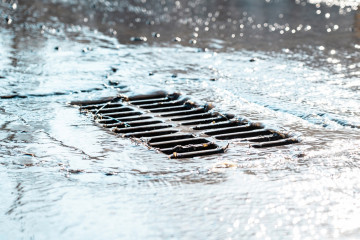
Navigating Stormwater Permits: What Manufacturers Need to Know
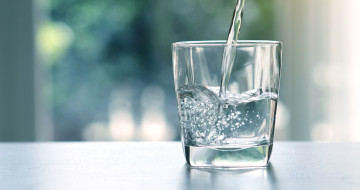
EPA Finalizes a PFAS National Primary Drinking Water Regulation (NPDWR)
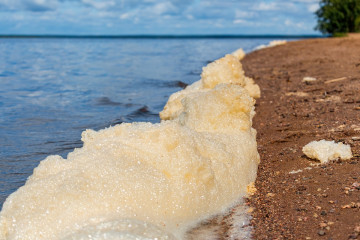
Regulating PFAS in Stormwater: What You Need to Know
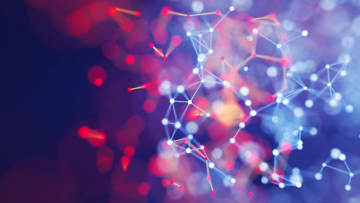
PFAS Management Support
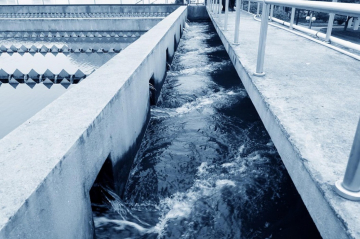
Wastewater Management
You are using an old version of edge..
Please note some functionalities might not be supported in this version of Edge.
This website uses cookies
Select the type of cookies you want to accept
More information
https://www.inogenalliance.com
Have a language expert improve your writing
Run a free plagiarism check in 10 minutes, automatically generate references for free.
- Knowledge Base
- Methodology
- Questionnaire Design | Methods, Question Types & Examples
Questionnaire Design | Methods, Question Types & Examples
Published on 6 May 2022 by Pritha Bhandari . Revised on 10 October 2022.
A questionnaire is a list of questions or items used to gather data from respondents about their attitudes, experiences, or opinions. Questionnaires can be used to collect quantitative and/or qualitative information.
Questionnaires are commonly used in market research as well as in the social and health sciences. For example, a company may ask for feedback about a recent customer service experience, or psychology researchers may investigate health risk perceptions using questionnaires.
Table of contents
Questionnaires vs surveys, questionnaire methods, open-ended vs closed-ended questions, question wording, question order, step-by-step guide to design, frequently asked questions about questionnaire design.
A survey is a research method where you collect and analyse data from a group of people. A questionnaire is a specific tool or instrument for collecting the data.
Designing a questionnaire means creating valid and reliable questions that address your research objectives, placing them in a useful order, and selecting an appropriate method for administration.
But designing a questionnaire is only one component of survey research. Survey research also involves defining the population you’re interested in, choosing an appropriate sampling method , administering questionnaires, data cleaning and analysis, and interpretation.
Sampling is important in survey research because you’ll often aim to generalise your results to the population. Gather data from a sample that represents the range of views in the population for externally valid results. There will always be some differences between the population and the sample, but minimising these will help you avoid sampling bias .
Prevent plagiarism, run a free check.
Questionnaires can be self-administered or researcher-administered . Self-administered questionnaires are more common because they are easy to implement and inexpensive, but researcher-administered questionnaires allow deeper insights.
Self-administered questionnaires
Self-administered questionnaires can be delivered online or in paper-and-pen formats, in person or by post. All questions are standardised so that all respondents receive the same questions with identical wording.
Self-administered questionnaires can be:
- Cost-effective
- Easy to administer for small and large groups
- Anonymous and suitable for sensitive topics
But they may also be:
- Unsuitable for people with limited literacy or verbal skills
- Susceptible to a nonreponse bias (most people invited may not complete the questionnaire)
- Biased towards people who volunteer because impersonal survey requests often go ignored
Researcher-administered questionnaires
Researcher-administered questionnaires are interviews that take place by phone, in person, or online between researchers and respondents.
Researcher-administered questionnaires can:
- Help you ensure the respondents are representative of your target audience
- Allow clarifications of ambiguous or unclear questions and answers
- Have high response rates because it’s harder to refuse an interview when personal attention is given to respondents
But researcher-administered questionnaires can be limiting in terms of resources. They are:
- Costly and time-consuming to perform
- More difficult to analyse if you have qualitative responses
- Likely to contain experimenter bias or demand characteristics
- Likely to encourage social desirability bias in responses because of a lack of anonymity
Your questionnaire can include open-ended or closed-ended questions, or a combination of both.
Using closed-ended questions limits your responses, while open-ended questions enable a broad range of answers. You’ll need to balance these considerations with your available time and resources.
Closed-ended questions
Closed-ended, or restricted-choice, questions offer respondents a fixed set of choices to select from. Closed-ended questions are best for collecting data on categorical or quantitative variables.
Categorical variables can be nominal or ordinal. Quantitative variables can be interval or ratio. Understanding the type of variable and level of measurement means you can perform appropriate statistical analyses for generalisable results.
Examples of closed-ended questions for different variables
Nominal variables include categories that can’t be ranked, such as race or ethnicity. This includes binary or dichotomous categories.
It’s best to include categories that cover all possible answers and are mutually exclusive. There should be no overlap between response items.
In binary or dichotomous questions, you’ll give respondents only two options to choose from.
White Black or African American American Indian or Alaska Native Asian Native Hawaiian or Other Pacific Islander
Ordinal variables include categories that can be ranked. Consider how wide or narrow a range you’ll include in your response items, and their relevance to your respondents.
Likert-type questions collect ordinal data using rating scales with five or seven points.
When you have four or more Likert-type questions, you can treat the composite data as quantitative data on an interval scale . Intelligence tests, psychological scales, and personality inventories use multiple Likert-type questions to collect interval data.
With interval or ratio data, you can apply strong statistical hypothesis tests to address your research aims.
Pros and cons of closed-ended questions
Well-designed closed-ended questions are easy to understand and can be answered quickly. However, you might still miss important answers that are relevant to respondents. An incomplete set of response items may force some respondents to pick the closest alternative to their true answer. These types of questions may also miss out on valuable detail.
To solve these problems, you can make questions partially closed-ended, and include an open-ended option where respondents can fill in their own answer.
Open-ended questions
Open-ended, or long-form, questions allow respondents to give answers in their own words. Because there are no restrictions on their choices, respondents can answer in ways that researchers may not have otherwise considered. For example, respondents may want to answer ‘multiracial’ for the question on race rather than selecting from a restricted list.
- How do you feel about open science?
- How would you describe your personality?
- In your opinion, what is the biggest obstacle to productivity in remote work?
Open-ended questions have a few downsides.
They require more time and effort from respondents, which may deter them from completing the questionnaire.
For researchers, understanding and summarising responses to these questions can take a lot of time and resources. You’ll need to develop a systematic coding scheme to categorise answers, and you may also need to involve other researchers in data analysis for high reliability .
Question wording can influence your respondents’ answers, especially if the language is unclear, ambiguous, or biased. Good questions need to be understood by all respondents in the same way ( reliable ) and measure exactly what you’re interested in ( valid ).
Use clear language
You should design questions with your target audience in mind. Consider their familiarity with your questionnaire topics and language and tailor your questions to them.
For readability and clarity, avoid jargon or overly complex language. Don’t use double negatives because they can be harder to understand.
Use balanced framing
Respondents often answer in different ways depending on the question framing. Positive frames are interpreted as more neutral than negative frames and may encourage more socially desirable answers.
Use a mix of both positive and negative frames to avoid bias , and ensure that your question wording is balanced wherever possible.
Unbalanced questions focus on only one side of an argument. Respondents may be less likely to oppose the question if it is framed in a particular direction. It’s best practice to provide a counterargument within the question as well.
Avoid leading questions
Leading questions guide respondents towards answering in specific ways, even if that’s not how they truly feel, by explicitly or implicitly providing them with extra information.
It’s best to keep your questions short and specific to your topic of interest.
- The average daily work commute in the US takes 54.2 minutes and costs $29 per day. Since 2020, working from home has saved many employees time and money. Do you favour flexible work-from-home policies even after it’s safe to return to offices?
- Experts agree that a well-balanced diet provides sufficient vitamins and minerals, and multivitamins and supplements are not necessary or effective. Do you agree or disagree that multivitamins are helpful for balanced nutrition?
Keep your questions focused
Ask about only one idea at a time and avoid double-barrelled questions. Double-barrelled questions ask about more than one item at a time, which can confuse respondents.
This question could be difficult to answer for respondents who feel strongly about the right to clean drinking water but not high-speed internet. They might only answer about the topic they feel passionate about or provide a neutral answer instead – but neither of these options capture their true answers.
Instead, you should ask two separate questions to gauge respondents’ opinions.
Strongly Agree Agree Undecided Disagree Strongly Disagree
Do you agree or disagree that the government should be responsible for providing high-speed internet to everyone?
You can organise the questions logically, with a clear progression from simple to complex. Alternatively, you can randomise the question order between respondents.
Logical flow
Using a logical flow to your question order means starting with simple questions, such as behavioural or opinion questions, and ending with more complex, sensitive, or controversial questions.
The question order that you use can significantly affect the responses by priming them in specific directions. Question order effects, or context effects, occur when earlier questions influence the responses to later questions, reducing the validity of your questionnaire.
While demographic questions are usually unaffected by order effects, questions about opinions and attitudes are more susceptible to them.
- How knowledgeable are you about Joe Biden’s executive orders in his first 100 days?
- Are you satisfied or dissatisfied with the way Joe Biden is managing the economy?
- Do you approve or disapprove of the way Joe Biden is handling his job as president?
It’s important to minimise order effects because they can be a source of systematic error or bias in your study.
Randomisation
Randomisation involves presenting individual respondents with the same questionnaire but with different question orders.
When you use randomisation, order effects will be minimised in your dataset. But a randomised order may also make it harder for respondents to process your questionnaire. Some questions may need more cognitive effort, while others are easier to answer, so a random order could require more time or mental capacity for respondents to switch between questions.
Follow this step-by-step guide to design your questionnaire.
Step 1: Define your goals and objectives
The first step of designing a questionnaire is determining your aims.
- What topics or experiences are you studying?
- What specifically do you want to find out?
- Is a self-report questionnaire an appropriate tool for investigating this topic?
Once you’ve specified your research aims, you can operationalise your variables of interest into questionnaire items. Operationalising concepts means turning them from abstract ideas into concrete measurements. Every question needs to address a defined need and have a clear purpose.
Step 2: Use questions that are suitable for your sample
Create appropriate questions by taking the perspective of your respondents. Consider their language proficiency and available time and energy when designing your questionnaire.
- Are the respondents familiar with the language and terms used in your questions?
- Would any of the questions insult, confuse, or embarrass them?
- Do the response items for any closed-ended questions capture all possible answers?
- Are the response items mutually exclusive?
- Do the respondents have time to respond to open-ended questions?
Consider all possible options for responses to closed-ended questions. From a respondent’s perspective, a lack of response options reflecting their point of view or true answer may make them feel alienated or excluded. In turn, they’ll become disengaged or inattentive to the rest of the questionnaire.
Step 3: Decide on your questionnaire length and question order
Once you have your questions, make sure that the length and order of your questions are appropriate for your sample.
If respondents are not being incentivised or compensated, keep your questionnaire short and easy to answer. Otherwise, your sample may be biased with only highly motivated respondents completing the questionnaire.
Decide on your question order based on your aims and resources. Use a logical flow if your respondents have limited time or if you cannot randomise questions. Randomising questions helps you avoid bias, but it can take more complex statistical analysis to interpret your data.
Step 4: Pretest your questionnaire
When you have a complete list of questions, you’ll need to pretest it to make sure what you’re asking is always clear and unambiguous. Pretesting helps you catch any errors or points of confusion before performing your study.
Ask friends, classmates, or members of your target audience to complete your questionnaire using the same method you’ll use for your research. Find out if any questions were particularly difficult to answer or if the directions were unclear or inconsistent, and make changes as necessary.
If you have the resources, running a pilot study will help you test the validity and reliability of your questionnaire. A pilot study is a practice run of the full study, and it includes sampling, data collection , and analysis.
You can find out whether your procedures are unfeasible or susceptible to bias and make changes in time, but you can’t test a hypothesis with this type of study because it’s usually statistically underpowered .
A questionnaire is a data collection tool or instrument, while a survey is an overarching research method that involves collecting and analysing data from people using questionnaires.
Closed-ended, or restricted-choice, questions offer respondents a fixed set of choices to select from. These questions are easier to answer quickly.
Open-ended or long-form questions allow respondents to answer in their own words. Because there are no restrictions on their choices, respondents can answer in ways that researchers may not have otherwise considered.
A Likert scale is a rating scale that quantitatively assesses opinions, attitudes, or behaviours. It is made up of four or more questions that measure a single attitude or trait when response scores are combined.
To use a Likert scale in a survey , you present participants with Likert-type questions or statements, and a continuum of items, usually with five or seven possible responses, to capture their degree of agreement.
You can organise the questions logically, with a clear progression from simple to complex, or randomly between respondents. A logical flow helps respondents process the questionnaire easier and quicker, but it may lead to bias. Randomisation can minimise the bias from order effects.
Questionnaires can be self-administered or researcher-administered.
Researcher-administered questionnaires are interviews that take place by phone, in person, or online between researchers and respondents. You can gain deeper insights by clarifying questions for respondents or asking follow-up questions.
Cite this Scribbr article
If you want to cite this source, you can copy and paste the citation or click the ‘Cite this Scribbr article’ button to automatically add the citation to our free Reference Generator.
Bhandari, P. (2022, October 10). Questionnaire Design | Methods, Question Types & Examples. Scribbr. Retrieved 14 May 2024, from https://www.scribbr.co.uk/research-methods/questionnaire-design/
Is this article helpful?
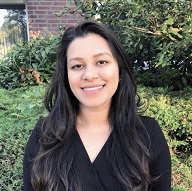
Pritha Bhandari
Other students also liked, doing survey research | a step-by-step guide & examples, what is a likert scale | guide & examples, reliability vs validity in research | differences, types & examples.
Call or Text the Maternal Mental Health Hotline
Parents: don’t struggle alone
The National Maternal Mental Health Hotline provides free, confidential mental health support. Pregnant people, moms, and new parents can call or text any time, every day.
Start a call: 1-833-TLC-MAMA (1-833-852-6262)
Text now: 1-833-TLC-MAMA (1-833-852-6262)
Use TTY: Use your preferred relay service or dial 711 , then 1-833-852-6262 .
Learn more about the Hotline
- Data & Research
- National Survey of Children's Health (NSCH)
National Survey of Children’s Health Questionnaires, Datasets, and Supporting Documents
The Data Resource Center for Child & Adolescent Health provides an easy to use Interactive Data Query for all years of the NSCH and a Guide to NSCH changes across survey years.
Guidance for data users
How-to instructions for using the NSCH data.
Historically enhanced 2016-2020 National Survey of Children’s Health (NSCH) data files were released in April 2024 and are available on the NSCH datasets page . This data release is a continuation of the improvements that were made to the 2021 NSCH data set released in October 2023. These revised datasets should be used when combining or comparing with the 2022 NSCH. Please read the weighting revisions technical document (PDF) .
- NSCH Enhancement Technical Document (PDF)
- Guide to Multiply Imputed Data Analysis (PDF)
- Guide to Multi-Year Analysis (PDF)
- 2023 Household Screener (PDF - 3 MB) | 2023 Household Screen Spanish (PDF - 1 MB)
- 2023 Topical Questionnaire (Children, 0-5 years) (PDF - 7 MB) | 2023 Topical Questionnaire (Children, 0-5 years) Spanish (PDF - 4 MB)
- 2023 Topical Questionnaire (Children, 6-11 years) (PDF - 6 MB) | 2023 Topical Questionnaire (Children, 6-11 years) Spanish (PDF - 3 MB)
- 2023 Topical Questionnaire (Children, 12-17 years) (PDF - 6 MB) | 2023 Topical Questionnaire (Children, 12-17 years) Spanish (PDF - 4 MB)
- 2022 Household Screener (PDF - 3 MB) | 2022 Household Screen Spanish (PDF - 2 MB)
- 2022 Topical Questionnaire (Children, 0-5 years) (PDF - 8 MB) | 2022 Topical Questionnaire (Children, 0-5 years) Spanish (PDF - 5 MB)
- 2022 Topical Questionnaire (Children, 6-11 years) (PDF - 4 MB) | 2022 Topical Questionnaire (Children, 6-11 years) Spanish (PDF - 4 MB)
- 2022 Topical Questionnaire (Children, 12-17 years) (PDF - 4 MB) | 2022 Topical Questionnaire (Children, 12-17 years) Spanish (PDF - 4 MB)
Methodology and data user FAQs
- 2022 FAQs (PDF)
- 2022 Source and Accuracy Statement (PDF)
- 2022 Survey and Sampling Administration Map (PDF - 620 KB)
- 2022 Methodology Report (PDF)
Variable lists and frequencies
- 2022 Screener Variable List (PDF)
- 2022 Topical Variable List (PDF)
- 2022 Screener PUF Frequencies (PDF)
- 2022 Topical PUF Frequencies (PDF)
- 2022 Guide to Topics and Questions Asked (PDF - 645 KB)
Note: See Sample Size footnote
- 2022 Interactive Data Query – Data Resource Center
- 2022 NSCH Data
- 2021 Household Screener (PDF - 1 MB) | 2021 Household Screener Spanish (PDF - 1 MB)
- 2021 Topical Questionnaire (Children, 0-5 years) (PDF - 1 MB) | 2021 Topical Questionnaire (Children, 0-5 years Spanish (PDF - 1 MB)
- 2021 Topical Questionnaire (Children, 6-11 years) (PDF - 771 KB) | 2021 Topical Questionnaire (Children, 6-11 years Spanish (PDF - 873 KB)
- 2021 Topical Questionnaire (Children, 12-17 years) (PDF - 742 KB) | 2021 Topical Questionnaire (Children, 12-17 years) Spanish (PDF - 858 KB)
- 2021 FAQs (PDF)
- 2021 Source and Accuracy Statement (PDF)
- 2021 Survey and Sampling Administration (PDF - 624 KB)
- 2021 Methodology Report (PDF)
- 2021 Non-Response Bias Analysis (PDF)
- 2021 Screener Variable List (PDF)
- 2021 Topical Variable List (PDF)
- 2021 Screener PUF Frequencies (PDF)
- 2021 Topical PUF Frequencies (PDF)
- 2021 Guide to Topics and Questions Asked
- 2021 Interactive Data Query – Data Resource Center
- 2021 Revised NSCH Data
Revised 2021 NSCH data files were released on October 2, 2023, and are available now on the 2021 Data Release page . Please read : weighting revisions technical document (PDF)
- 2020 Household Screener (PDF - 1 MB) | 2020 Household Screener Spanish (PDF - 1 MB)
- 2020 Topical Questionnaire (Children, 0-5 years) (PDF - 1 MB) | 2020 Topical Questionnaire (Children, 0-5 years) Spanish (PDF - 2 MB)
- 2020 Topical Questionnaire (Children, 6-11 years) (PDF - 800 KB) | 2020 Topical Questionnaire (Children, 6-11 years) Spanish (PDF - 998 KB)
- 2020 Topical Questionnaire (Children, 12-17 years) (PDF - 761 KB) | 2020 Topical Questionnaire (Children, 12-17 years) Spanish (PDF - 1 MB)
- 2020 FAQs (PDF)
- 2020 Source and Accuracy Statement (PDF)
- 2020 Survey and Sampling Administration Map (PDF - 600 KB)
- 2020 Methodology Report (PDF)
- 2020 Non-Response Bias Analysis (PDF)
- 2020 Screener Variable List (PDF)
- 2020 Topical Variable List (PDF)
- 2020 Screener PUF Frequencies (PDF)
- 2020 Topical PUF Frequencies (PDF)
- 2020 Guide to Topics and Questions Asked (PDF - 495 KB)
- 2020 Interactive Data Query – Data Resource Center
- 2020 NSCH Data
- 2019 Household Screener (PDF - 1 MB) | 2020 Household Screener Spanish (PDF - 622 KB)
- 2019 Topical Questionnaire (Children, 0-5 years) (PDF - 724 KB) | 2019 Topical Questionnaire (Children, 0-5 years)Spanish (PDF - 759 KB)
- 2019 Topical Questionnaire (Children, 6-11 years) (PDF - 801 KB) | 2019 Topical Questionnaire (Children, 6-11 years) Spanish (PDF - 811 KB)
- 2019 Topical Questionnaire (Children, 12-17 years) (PDF - 760 KB) | 2019 Topical Questionnaire (Children, 12-17 years) Spanish (PDF - 784 KB)
- 2019 FAQs (PDF)
- 2019 Source and Accuracy Statement (PDF)
- 2019 Survey and Sampling Administration Map (PDF - 626 KB)
- 2019 Methodology Report (PDF)
- 2019 Non-Response Bias Analysis (PDF)
- 2019 Screener Variable List (PDF)
- 2019 Topical Variable List (PDF)
- NSCH Crosswalk 2016-2019 (XLSX)
- 2019 Screener PUF Frequencies (PDF)
- 2019 Topical PUF Frequencies (PDF)
- 2019 Guide to Topics and Questions Asked (PDF - 595 KB)
- Interactive Data Query – Data Resource Center
- 2019 NSCH Data
- 2018 Household Screener (PDF - 1 MB) | 2018 Household Screener Spanish (PDF - 1 MB)
- 2018 Topical Questionnaire (Children, 0-5 years) (PDF - 1 MB) | 2018 Topical Questionnaire (Children, 0-5 years Spanish (PDF - 1 MB)
- 2018 Topical Questionnaire (Children, 6-11 years) (PDF - 1 MB) | 2018 Topical Questionnaire (Children, 6-11 years) Spanish (PDF - 1 MB)
- 2018 Topical Questionnaire (Children, 12-17 years) (PDF - 1 MB) | 2018 Topical Questionnaire (Children, 12-17 years) Spanish (PDF - 1 MB)
- 2018 FAQs (PDF)
- 2018 Source and Accuracy Statement (PDF)
- 2018 Survey and Sampling Administration Map (PDF - 944 KB)
- 2018 Methodology Report (PDF)
- 2018 Non-Response Bias Analysis (PDF)
- 2018 Screener Variable List (PDF)
- 2018 Topical Variable List (PDF)
- 2016-2018 NSCH Crosswalk (XLSX)
- 2018 Screener PUF Frequencies (PDF)
- 2018 Topical PUF Frequencies (PDF)
- 2018 Guide to Topics & Questions Asked (PDF - 572 KB)
Note: See Sample Size footnote .
- Interactive Data Query - Data Resource Center
- 2018 NSCH Data
- 2017 Household Screener (PDF - 1 MB) | 2017 Household Screener Spanish (PDF - 1 MB)
- 2017 Topical Questionnaire (Children, 0-5 years) (PDF - 1 MB) | 2017 Topical Questionnaire (Children, 0-5 years) Spanish (PDF - 1 MB)
- 2017 Topical Questionnaire (Children, 6-11 years) (PDF - 1 MB) | 2017 Topical Questionnaire (Children, 6-11 years) Spanish (PDF - 1 MB)
- 2017 Topical Questionnaire (Children, 12-17 years) (PDF - 1 MB) | 2017 Topical Questionnaire (Children, 12-17 years) Spanish (PDF - 1 MB)
- 2017 FAQs (PDF)
- 2017 Source and Accuracy Statement (PDF - 1 MB)
- 2017 Survey and Sampling Administration Map (PDF - 804 KB)
- 2017 Methodology Report (PDF - 9 MB)
- 2017 Multiple Imputation Data Guide (PDF - 80 KB)
- 2017 Non-Response Bias Analysis (PDF - 1 MB)
- 2017 Multi-Year Analysis Guide (PDF - 811 KB)
- 2017 Screener Variable List (PDF)
- 2017 Topical Variable List (PDF)
- 2017 Screener PUF Frequencies (PDF)
- 2017 Topical PUF Frequencies (PDF)
- 2017 Guide to Topics & Questions Asked (PDF - 966 KB)
- 2017 NSCH Data
- 2016 Household Screener (PDF - 1 MB) | 2016 Household Screener Spanish (PDF - 1 MB)
- 2016 Topical Questionnaire (Children, 0-5 years) (PDF - 1 MB) | 2016 Topical Questionnaire (Children, 0-5 years) Spanish (PDF - 1 MB)
- 2016 Topical Questionnaire (Children, 6-11 years) (PDF - 1 MB) | 2016 Topical Questionnaire (Children, 6-11 years)Spanish (PDF - 1 MB)
- 2016 Topical Questionnaire (Children, 12-17 years) (PDF - 1 MB) | 2016 Topical Questionnaire (Children, 12-17 years Spanish (PDF - 1 MB)
- 2016 FAQs (PDF - 720 KB)
- 2016 Source and Accuracy Statement (PDF - 480 KB)
- 2016 Survey and Sampling Administration Map (PDF - 771 KB)
- 2016 Methodology Report (PDF - 8 MB)
- 2016 NSCH Guide to Analysis with Multiple Imputed Data (PDF - 539 KB)
- 2016 Non-Response Bias Analysis (PDF - 1 MB)
- 2016 Screener Variable List (PDF)
- 2016 Topical Variable List (PDF)
- 2016 Screener PUF Frequencies (PDF)
- 2016 Topical PUF Frequencies (PDF)
Supplemental documents
- 2016 Insurance (PDF - 180 KB)
- 2016 Geography (PDF - 98 KB)
- 2016 Interactive Data Guide
- 2016 Guide to Topics & Questions Asked (PDF - 355 KB)
Title V changes
- NPM and NOM Content Changes in 2016 (PDF - 201 KB)
- 2016 NSCH Data
A note on sample size
In both individual year and multi-year analyses, the NSCH sample size may be limited for smaller populations (e.g., American Indian (AI) /Alaska Native (AN)) and state-level subgroups or rare outcomes (e.g., adolescent children with special health care needs (CSHCN) or autism in a particular state).
Small sample sizes may produce unstable estimates.
To minimize misinterpretation, we recommend only presenting statistics with a sample size or unweighted denominator of 30 or more.
Additionally, if the 95% confidence interval width exceeds 20 percentage points or 1.2 times the estimate (≈ relative standard error>30%), we recommend flagging for poor reliability and/or presenting a measure of statistical reliability (e.g., confidence intervals or statistical significance testing) to promote appropriate interpretation.
Thank you for visiting nature.com. You are using a browser version with limited support for CSS. To obtain the best experience, we recommend you use a more up to date browser (or turn off compatibility mode in Internet Explorer). In the meantime, to ensure continued support, we are displaying the site without styles and JavaScript.
- View all journals
- My Account Login
- Explore content
- About the journal
- Publish with us
- Sign up for alerts
- Data Descriptor
- Open access
- Published: 03 May 2024
A dataset for measuring the impact of research data and their curation
- Libby Hemphill ORCID: orcid.org/0000-0002-3793-7281 1 , 2 ,
- Andrea Thomer 3 ,
- Sara Lafia 1 ,
- Lizhou Fan 2 ,
- David Bleckley ORCID: orcid.org/0000-0001-7715-4348 1 &
- Elizabeth Moss 1
Scientific Data volume 11 , Article number: 442 ( 2024 ) Cite this article
595 Accesses
8 Altmetric
Metrics details
- Research data
- Social sciences
Science funders, publishers, and data archives make decisions about how to responsibly allocate resources to maximize the reuse potential of research data. This paper introduces a dataset developed to measure the impact of archival and data curation decisions on data reuse. The dataset describes 10,605 social science research datasets, their curation histories, and reuse contexts in 94,755 publications that cover 59 years from 1963 to 2022. The dataset was constructed from study-level metadata, citing publications, and curation records available through the Inter-university Consortium for Political and Social Research (ICPSR) at the University of Michigan. The dataset includes information about study-level attributes (e.g., PIs, funders, subject terms); usage statistics (e.g., downloads, citations); archiving decisions (e.g., curation activities, data transformations); and bibliometric attributes (e.g., journals, authors) for citing publications. This dataset provides information on factors that contribute to long-term data reuse, which can inform the design of effective evidence-based recommendations to support high-impact research data curation decisions.
Similar content being viewed by others
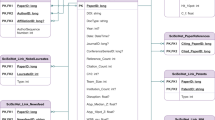
SciSciNet: A large-scale open data lake for the science of science research
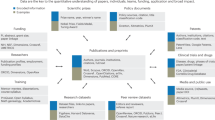
Data, measurement and empirical methods in the science of science
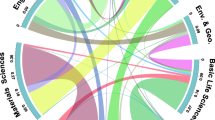
Interdisciplinarity revisited: evidence for research impact and dynamism
Background & summary.
Recent policy changes in funding agencies and academic journals have increased data sharing among researchers and between researchers and the public. Data sharing advances science and provides the transparency necessary for evaluating, replicating, and verifying results. However, many data-sharing policies do not explain what constitutes an appropriate dataset for archiving or how to determine the value of datasets to secondary users 1 , 2 , 3 . Questions about how to allocate data-sharing resources efficiently and responsibly have gone unanswered 4 , 5 , 6 . For instance, data-sharing policies recognize that not all data should be curated and preserved, but they do not articulate metrics or guidelines for determining what data are most worthy of investment.
Despite the potential for innovation and advancement that data sharing holds, the best strategies to prioritize datasets for preparation and archiving are often unclear. Some datasets are likely to have more downstream potential than others, and data curation policies and workflows should prioritize high-value data instead of being one-size-fits-all. Though prior research in library and information science has shown that the “analytic potential” of a dataset is key to its reuse value 7 , work is needed to implement conceptual data reuse frameworks 8 , 9 , 10 , 11 , 12 , 13 , 14 . In addition, publishers and data archives need guidance to develop metrics and evaluation strategies to assess the impact of datasets.
Several existing resources have been compiled to study the relationship between the reuse of scholarly products, such as datasets (Table 1 ); however, none of these resources include explicit information on how curation processes are applied to data to increase their value, maximize their accessibility, and ensure their long-term preservation. The CCex (Curation Costs Exchange) provides models of curation services along with cost-related datasets shared by contributors but does not make explicit connections between them or include reuse information 15 . Analyses on platforms such as DataCite 16 have focused on metadata completeness and record usage, but have not included related curation-level information. Analyses of GenBank 17 and FigShare 18 , 19 citation networks do not include curation information. Related studies of Github repository reuse 20 and Softcite software citation 21 reveal significant factors that impact the reuse of secondary research products but do not focus on research data. RD-Switchboard 22 and DSKG 23 are scholarly knowledge graphs linking research data to articles, patents, and grants, but largely omit social science research data and do not include curation-level factors. To our knowledge, other studies of curation work in organizations similar to ICPSR – such as GESIS 24 , Dataverse 25 , and DANS 26 – have not made their underlying data available for analysis.
This paper describes a dataset 27 compiled for the MICA project (Measuring the Impact of Curation Actions) led by investigators at ICPSR, a large social science data archive at the University of Michigan. The dataset was originally developed to study the impacts of data curation and archiving on data reuse. The MICA dataset has supported several previous publications investigating the intensity of data curation actions 28 , the relationship between data curation actions and data reuse 29 , and the structures of research communities in a data citation network 30 . Collectively, these studies help explain the return on various types of curatorial investments. The dataset that we introduce in this paper, which we refer to as the MICA dataset, has the potential to address research questions in the areas of science (e.g., knowledge production), library and information science (e.g., scholarly communication), and data archiving (e.g., reproducible workflows).
We constructed the MICA dataset 27 using records available at ICPSR, a large social science data archive at the University of Michigan. Data set creation involved: collecting and enriching metadata for articles indexed in the ICPSR Bibliography of Data-related Literature against the Dimensions AI bibliometric database; gathering usage statistics for studies from ICPSR’s administrative database; processing data curation work logs from ICPSR’s project tracking platform, Jira; and linking data in social science studies and series to citing analysis papers (Fig. 1 ).
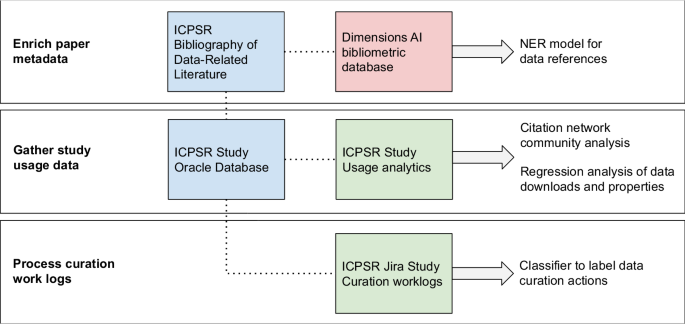
Steps to prepare MICA dataset for analysis - external sources are red, primary internal sources are blue, and internal linked sources are green.
Enrich paper metadata
The ICPSR Bibliography of Data-related Literature is a growing database of literature in which data from ICPSR studies have been used. Its creation was funded by the National Science Foundation (Award 9977984), and for the past 20 years it has been supported by ICPSR membership and multiple US federally-funded and foundation-funded topical archives at ICPSR. The Bibliography was originally launched in the year 2000 to aid in data discovery by providing a searchable database linking publications to the study data used in them. The Bibliography collects the universe of output based on the data shared in each study through, which is made available through each ICPSR study’s webpage. The Bibliography contains both peer-reviewed and grey literature, which provides evidence for measuring the impact of research data. For an item to be included in the ICPSR Bibliography, it must contain an analysis of data archived by ICPSR or contain a discussion or critique of the data collection process, study design, or methodology 31 . The Bibliography is manually curated by a team of librarians and information specialists at ICPSR who enter and validate entries. Some publications are supplied to the Bibliography by data depositors, and some citations are submitted to the Bibliography by authors who abide by ICPSR’s terms of use requiring them to submit citations to works in which they analyzed data retrieved from ICPSR. Most of the Bibliography is populated by Bibliography team members, who create custom queries for ICPSR studies performed across numerous sources, including Google Scholar, ProQuest, SSRN, and others. Each record in the Bibliography is one publication that has used one or more ICPSR studies. The version we used was captured on 2021-11-16 and included 94,755 publications.
To expand the coverage of the ICPSR Bibliography, we searched exhaustively for all ICPSR study names, unique numbers assigned to ICPSR studies, and DOIs 32 using a full-text index available through the Dimensions AI database 33 . We accessed Dimensions through a license agreement with the University of Michigan. ICPSR Bibliography librarians and information specialists manually reviewed and validated new entries that matched one or more search criteria. We then used Dimensions to gather enriched metadata and full-text links for items in the Bibliography with DOIs. We matched 43% of the items in the Bibliography to enriched Dimensions metadata including abstracts, field of research codes, concepts, and authors’ institutional information; we also obtained links to full text for 16% of Bibliography items. Based on licensing agreements, we included Dimensions identifiers and links to full text so that users with valid publisher and database access can construct an enriched publication dataset.
Gather study usage data
ICPSR maintains a relational administrative database, DBInfo, that organizes study-level metadata and information on data reuse across separate tables. Studies at ICPSR consist of one or more files collected at a single time or for a single purpose; studies in which the same variables are observed over time are grouped into series. Each study at ICPSR is assigned a DOI, and its metadata are stored in DBInfo. Study metadata follows the Data Documentation Initiative (DDI) Codebook 2.5 standard. DDI elements included in our dataset are title, ICPSR study identification number, DOI, authoring entities, description (abstract), funding agencies, subject terms assigned to the study during curation, and geographic coverage. We also created variables based on DDI elements: total variable count, the presence of survey question text in the metadata, the number of author entities, and whether an author entity was an institution. We gathered metadata for ICPSR’s 10,605 unrestricted public-use studies available as of 2021-11-16 ( https://www.icpsr.umich.edu/web/pages/membership/or/metadata/oai.html ).
To link study usage data with study-level metadata records, we joined study metadata from DBinfo on study usage information, which included total study downloads (data and documentation), individual data file downloads, and cumulative citations from the ICPSR Bibliography. We also gathered descriptive metadata for each study and its variables, which allowed us to summarize and append recoded fields onto the study-level metadata such as curation level, number and type of principle investigators, total variable count, and binary variables indicating whether the study data were made available for online analysis, whether survey question text was made searchable online, and whether the study variables were indexed for search. These characteristics describe aspects of the discoverability of the data to compare with other characteristics of the study. We used the study and series numbers included in the ICPSR Bibliography as unique identifiers to link papers to metadata and analyze the community structure of dataset co-citations in the ICPSR Bibliography 32 .
Process curation work logs
Researchers deposit data at ICPSR for curation and long-term preservation. Between 2016 and 2020, more than 3,000 research studies were deposited with ICPSR. Since 2017, ICPSR has organized curation work into a central unit that provides varied levels of curation that vary in the intensity and complexity of data enhancement that they provide. While the levels of curation are standardized as to effort (level one = less effort, level three = most effort), the specific curatorial actions undertaken for each dataset vary. The specific curation actions are captured in Jira, a work tracking program, which data curators at ICPSR use to collaborate and communicate their progress through tickets. We obtained access to a corpus of 669 completed Jira tickets corresponding to the curation of 566 unique studies between February 2017 and December 2019 28 .
To process the tickets, we focused only on their work log portions, which contained free text descriptions of work that data curators had performed on a deposited study, along with the curators’ identifiers, and timestamps. To protect the confidentiality of the data curators and the processing steps they performed, we collaborated with ICPSR’s curation unit to propose a classification scheme, which we used to train a Naive Bayes classifier and label curation actions in each work log sentence. The eight curation action labels we proposed 28 were: (1) initial review and planning, (2) data transformation, (3) metadata, (4) documentation, (5) quality checks, (6) communication, (7) other, and (8) non-curation work. We note that these categories of curation work are very specific to the curatorial processes and types of data stored at ICPSR, and may not match the curation activities at other repositories. After applying the classifier to the work log sentences, we obtained summary-level curation actions for a subset of all ICPSR studies (5%), along with the total number of hours spent on data curation for each study, and the proportion of time associated with each action during curation.
Data Records
The MICA dataset 27 connects records for each of ICPSR’s archived research studies to the research publications that use them and related curation activities available for a subset of studies (Fig. 2 ). Each of the three tables published in the dataset is available as a study archived at ICPSR. The data tables are distributed as statistical files available for use in SAS, SPSS, Stata, and R as well as delimited and ASCII text files. The dataset is organized around studies and papers as primary entities. The studies table lists ICPSR studies, their metadata attributes, and usage information; the papers table was constructed using the ICPSR Bibliography and Dimensions database; and the curation logs table summarizes the data curation steps performed on a subset of ICPSR studies.
Studies (“ICPSR_STUDIES”): 10,605 social science research datasets available through ICPSR up to 2021-11-16 with variables for ICPSR study number, digital object identifier, study name, series number, series title, authoring entities, full-text description, release date, funding agency, geographic coverage, subject terms, topical archive, curation level, single principal investigator (PI), institutional PI, the total number of PIs, total variables in data files, question text availability, study variable indexing, level of restriction, total unique users downloading study data files and codebooks, total unique users downloading data only, and total unique papers citing data through November 2021. Studies map to the papers and curation logs table through ICPSR study numbers as “STUDY”. However, not every study in this table will have records in the papers and curation logs tables.
Papers (“ICPSR_PAPERS”): 94,755 publications collected from 2000-08-11 to 2021-11-16 in the ICPSR Bibliography and enriched with metadata from the Dimensions database with variables for paper number, identifier, title, authors, publication venue, item type, publication date, input date, ICPSR series numbers used in the paper, ICPSR study numbers used in the paper, the Dimension identifier, and the Dimensions link to the publication’s full text. Papers map to the studies table through ICPSR study numbers in the “STUDY_NUMS” field. Each record represents a single publication, and because a researcher can use multiple datasets when creating a publication, each record may list multiple studies or series.
Curation logs (“ICPSR_CURATION_LOGS”): 649 curation logs for 563 ICPSR studies (although most studies in the subset had one curation log, some studies were associated with multiple logs, with a maximum of 10) curated between February 2017 and December 2019 with variables for study number, action labels assigned to work description sentences using a classifier trained on ICPSR curation logs, hours of work associated with a single log entry, and total hours of work logged for the curation ticket. Curation logs map to the study and paper tables through ICPSR study numbers as “STUDY”. Each record represents a single logged action, and future users may wish to aggregate actions to the study level before joining tables.
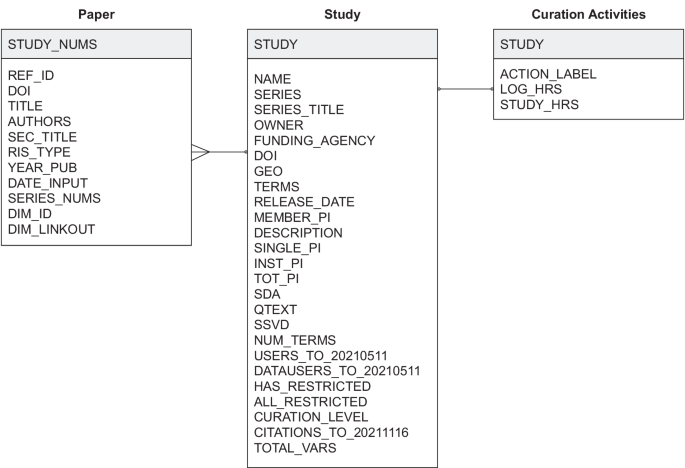
Entity-relation diagram.
Technical Validation
We report on the reliability of the dataset’s metadata in the following subsections. To support future reuse of the dataset, curation services provided through ICPSR improved data quality by checking for missing values, adding variable labels, and creating a codebook.
All 10,605 studies available through ICPSR have a DOI and a full-text description summarizing what the study is about, the purpose of the study, the main topics covered, and the questions the PIs attempted to answer when they conducted the study. Personal names (i.e., principal investigators) and organizational names (i.e., funding agencies) are standardized against an authority list maintained by ICPSR; geographic names and subject terms are also standardized and hierarchically indexed in the ICPSR Thesaurus 34 . Many of ICPSR’s studies (63%) are in a series and are distributed through the ICPSR General Archive (56%), a non-topical archive that accepts any social or behavioral science data. While study data have been available through ICPSR since 1962, the earliest digital release date recorded for a study was 1984-03-18, when ICPSR’s database was first employed, and the most recent date is 2021-10-28 when the dataset was collected.
Curation level information was recorded starting in 2017 and is available for 1,125 studies (11%); approximately 80% of studies with assigned curation levels received curation services, equally distributed between Levels 1 (least intensive), 2 (moderately intensive), and 3 (most intensive) (Fig. 3 ). Detailed descriptions of ICPSR’s curation levels are available online 35 . Additional metadata are available for a subset of 421 studies (4%), including information about whether the study has a single PI, an institutional PI, the total number of PIs involved, total variables recorded is available for online analysis, has searchable question text, has variables that are indexed for search, contains one or more restricted files, and whether the study is completely restricted. We provided additional metadata for this subset of ICPSR studies because they were released within the past five years and detailed curation and usage information were available for them. Usage statistics including total downloads and data file downloads are available for this subset of studies as well; citation statistics are available for 8,030 studies (76%). Most ICPSR studies have fewer than 500 users, as indicated by total downloads, or citations (Fig. 4 ).
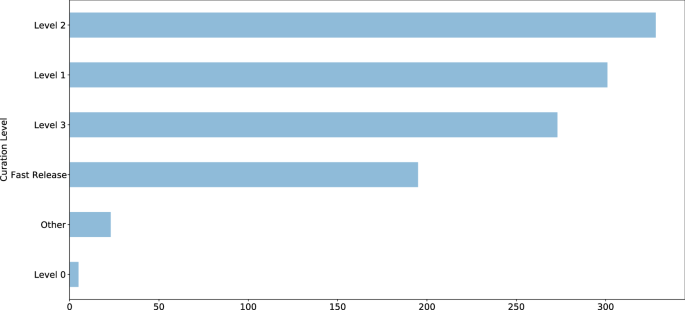
ICPSR study curation levels.
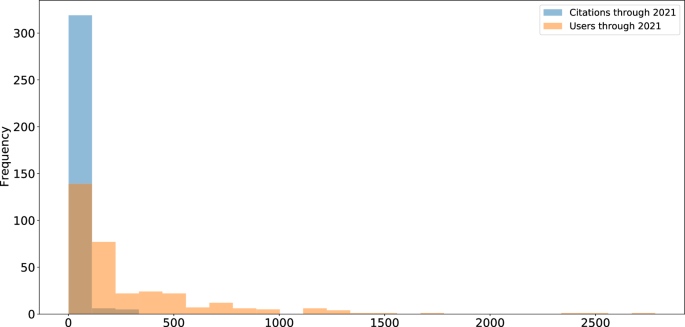
ICPSR study usage.
A subset of 43,102 publications (45%) available in the ICPSR Bibliography had a DOI. Author metadata were entered as free text, meaning that variations may exist and require additional normalization and pre-processing prior to analysis. While author information is standardized for each publication, individual names may appear in different sort orders (e.g., “Earls, Felton J.” and “Stephen W. Raudenbush”). Most of the items in the ICPSR Bibliography as of 2021-11-16 were journal articles (59%), reports (14%), conference presentations (9%), or theses (8%) (Fig. 5 ). The number of publications collected in the Bibliography has increased each decade since the inception of ICPSR in 1962 (Fig. 6 ). Most ICPSR studies (76%) have one or more citations in a publication.
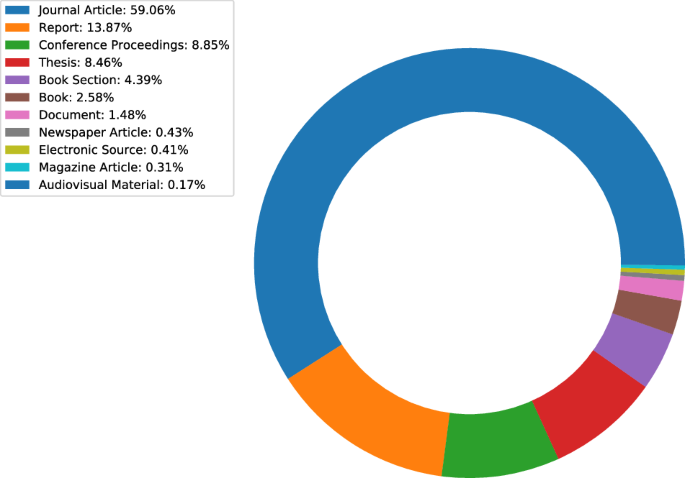
ICPSR Bibliography citation types.
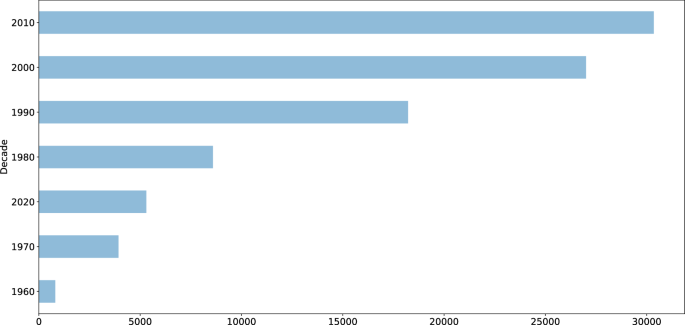
ICPSR citations by decade.
Usage Notes
The dataset consists of three tables that can be joined using the “STUDY” key as shown in Fig. 2 . The “ICPSR_PAPERS” table contains one row per paper with one or more cited studies in the “STUDY_NUMS” column. We manipulated and analyzed the tables as CSV files with the Pandas library 36 in Python and the Tidyverse packages 37 in R.
The present MICA dataset can be used independently to study the relationship between curation decisions and data reuse. Evidence of reuse for specific studies is available in several forms: usage information, including downloads and citation counts; and citation contexts within papers that cite data. Analysis may also be performed on the citation network formed between datasets and papers that use them. Finally, curation actions can be associated with properties of studies and usage histories.
This dataset has several limitations of which users should be aware. First, Jira tickets can only be used to represent the intensiveness of curation for activities undertaken since 2017, when ICPSR started using both Curation Levels and Jira. Studies published before 2017 were all curated, but documentation of the extent of that curation was not standardized and therefore could not be included in these analyses. Second, the measure of publications relies upon the authors’ clarity of data citation and the ICPSR Bibliography staff’s ability to discover citations with varying formality and clarity. Thus, there is always a chance that some secondary-data-citing publications have been left out of the bibliography. Finally, there may be some cases in which a paper in the ICSPSR bibliography did not actually obtain data from ICPSR. For example, PIs have often written about or even distributed their data prior to their archival in ICSPR. Therefore, those publications would not have cited ICPSR but they are still collected in the Bibliography as being directly related to the data that were eventually deposited at ICPSR.
In summary, the MICA dataset contains relationships between two main types of entities – papers and studies – which can be mined. The tables in the MICA dataset have supported network analysis (community structure and clique detection) 30 ; natural language processing (NER for dataset reference detection) 32 ; visualizing citation networks (to search for datasets) 38 ; and regression analysis (on curation decisions and data downloads) 29 . The data are currently being used to develop research metrics and recommendation systems for research data. Given that DOIs are provided for ICPSR studies and articles in the ICPSR Bibliography, the MICA dataset can also be used with other bibliometric databases, including DataCite, Crossref, OpenAlex, and related indexes. Subscription-based services, such as Dimensions AI, are also compatible with the MICA dataset. In some cases, these services provide abstracts or full text for papers from which data citation contexts can be extracted for semantic content analysis.
Code availability
The code 27 used to produce the MICA project dataset is available on GitHub at https://github.com/ICPSR/mica-data-descriptor and through Zenodo with the identifier https://doi.org/10.5281/zenodo.8432666 . Data manipulation and pre-processing were performed in Python. Data curation for distribution was performed in SPSS.
He, L. & Han, Z. Do usage counts of scientific data make sense? An investigation of the Dryad repository. Library Hi Tech 35 , 332–342 (2017).
Article Google Scholar
Brickley, D., Burgess, M. & Noy, N. Google dataset search: Building a search engine for datasets in an open web ecosystem. In The World Wide Web Conference - WWW ‘19 , 1365–1375 (ACM Press, San Francisco, CA, USA, 2019).
Buneman, P., Dosso, D., Lissandrini, M. & Silvello, G. Data citation and the citation graph. Quantitative Science Studies 2 , 1399–1422 (2022).
Chao, T. C. Disciplinary reach: Investigating the impact of dataset reuse in the earth sciences. Proceedings of the American Society for Information Science and Technology 48 , 1–8 (2011).
Article ADS Google Scholar
Parr, C. et al . A discussion of value metrics for data repositories in earth and environmental sciences. Data Science Journal 18 , 58 (2019).
Eschenfelder, K. R., Shankar, K. & Downey, G. The financial maintenance of social science data archives: Four case studies of long–term infrastructure work. J. Assoc. Inf. Sci. Technol. 73 , 1723–1740 (2022).
Palmer, C. L., Weber, N. M. & Cragin, M. H. The analytic potential of scientific data: Understanding re-use value. Proceedings of the American Society for Information Science and Technology 48 , 1–10 (2011).
Zimmerman, A. S. New knowledge from old data: The role of standards in the sharing and reuse of ecological data. Sci. Technol. Human Values 33 , 631–652 (2008).
Cragin, M. H., Palmer, C. L., Carlson, J. R. & Witt, M. Data sharing, small science and institutional repositories. Philosophical Transactions of the Royal Society A: Mathematical, Physical and Engineering Sciences 368 , 4023–4038 (2010).
Article ADS CAS Google Scholar
Fear, K. M. Measuring and Anticipating the Impact of Data Reuse . Ph.D. thesis, University of Michigan (2013).
Borgman, C. L., Van de Sompel, H., Scharnhorst, A., van den Berg, H. & Treloar, A. Who uses the digital data archive? An exploratory study of DANS. Proceedings of the Association for Information Science and Technology 52 , 1–4 (2015).
Pasquetto, I. V., Borgman, C. L. & Wofford, M. F. Uses and reuses of scientific data: The data creators’ advantage. Harvard Data Science Review 1 (2019).
Gregory, K., Groth, P., Scharnhorst, A. & Wyatt, S. Lost or found? Discovering data needed for research. Harvard Data Science Review (2020).
York, J. Seeking equilibrium in data reuse: A study of knowledge satisficing . Ph.D. thesis, University of Michigan (2022).
Kilbride, W. & Norris, S. Collaborating to clarify the cost of curation. New Review of Information Networking 19 , 44–48 (2014).
Robinson-Garcia, N., Mongeon, P., Jeng, W. & Costas, R. DataCite as a novel bibliometric source: Coverage, strengths and limitations. Journal of Informetrics 11 , 841–854 (2017).
Qin, J., Hemsley, J. & Bratt, S. E. The structural shift and collaboration capacity in GenBank networks: A longitudinal study. Quantitative Science Studies 3 , 174–193 (2022).
Article PubMed PubMed Central Google Scholar
Acuna, D. E., Yi, Z., Liang, L. & Zhuang, H. Predicting the usage of scientific datasets based on article, author, institution, and journal bibliometrics. In Smits, M. (ed.) Information for a Better World: Shaping the Global Future. iConference 2022 ., 42–52 (Springer International Publishing, Cham, 2022).
Zeng, T., Wu, L., Bratt, S. & Acuna, D. E. Assigning credit to scientific datasets using article citation networks. Journal of Informetrics 14 , 101013 (2020).
Koesten, L., Vougiouklis, P., Simperl, E. & Groth, P. Dataset reuse: Toward translating principles to practice. Patterns 1 , 100136 (2020).
Du, C., Cohoon, J., Lopez, P. & Howison, J. Softcite dataset: A dataset of software mentions in biomedical and economic research publications. J. Assoc. Inf. Sci. Technol. 72 , 870–884 (2021).
Aryani, A. et al . A research graph dataset for connecting research data repositories using RD-Switchboard. Sci Data 5 , 180099 (2018).
Färber, M. & Lamprecht, D. The data set knowledge graph: Creating a linked open data source for data sets. Quantitative Science Studies 2 , 1324–1355 (2021).
Perry, A. & Netscher, S. Measuring the time spent on data curation. Journal of Documentation 78 , 282–304 (2022).
Trisovic, A. et al . Advancing computational reproducibility in the Dataverse data repository platform. In Proceedings of the 3rd International Workshop on Practical Reproducible Evaluation of Computer Systems , P-RECS ‘20, 15–20, https://doi.org/10.1145/3391800.3398173 (Association for Computing Machinery, New York, NY, USA, 2020).
Borgman, C. L., Scharnhorst, A. & Golshan, M. S. Digital data archives as knowledge infrastructures: Mediating data sharing and reuse. Journal of the Association for Information Science and Technology 70 , 888–904, https://doi.org/10.1002/asi.24172 (2019).
Lafia, S. et al . MICA Data Descriptor. Zenodo https://doi.org/10.5281/zenodo.8432666 (2023).
Lafia, S., Thomer, A., Bleckley, D., Akmon, D. & Hemphill, L. Leveraging machine learning to detect data curation activities. In 2021 IEEE 17th International Conference on eScience (eScience) , 149–158, https://doi.org/10.1109/eScience51609.2021.00025 (2021).
Hemphill, L., Pienta, A., Lafia, S., Akmon, D. & Bleckley, D. How do properties of data, their curation, and their funding relate to reuse? J. Assoc. Inf. Sci. Technol. 73 , 1432–44, https://doi.org/10.1002/asi.24646 (2021).
Lafia, S., Fan, L., Thomer, A. & Hemphill, L. Subdivisions and crossroads: Identifying hidden community structures in a data archive’s citation network. Quantitative Science Studies 3 , 694–714, https://doi.org/10.1162/qss_a_00209 (2022).
ICPSR. ICPSR Bibliography of Data-related Literature: Collection Criteria. https://www.icpsr.umich.edu/web/pages/ICPSR/citations/collection-criteria.html (2023).
Lafia, S., Fan, L. & Hemphill, L. A natural language processing pipeline for detecting informal data references in academic literature. Proc. Assoc. Inf. Sci. Technol. 59 , 169–178, https://doi.org/10.1002/pra2.614 (2022).
Hook, D. W., Porter, S. J. & Herzog, C. Dimensions: Building context for search and evaluation. Frontiers in Research Metrics and Analytics 3 , 23, https://doi.org/10.3389/frma.2018.00023 (2018).
https://www.icpsr.umich.edu/web/ICPSR/thesaurus (2002). ICPSR. ICPSR Thesaurus.
https://www.icpsr.umich.edu/files/datamanagement/icpsr-curation-levels.pdf (2020). ICPSR. ICPSR Curation Levels.
McKinney, W. Data Structures for Statistical Computing in Python. In van der Walt, S. & Millman, J. (eds.) Proceedings of the 9th Python in Science Conference , 56–61 (2010).
Wickham, H. et al . Welcome to the Tidyverse. Journal of Open Source Software 4 , 1686 (2019).
Fan, L., Lafia, S., Li, L., Yang, F. & Hemphill, L. DataChat: Prototyping a conversational agent for dataset search and visualization. Proc. Assoc. Inf. Sci. Technol. 60 , 586–591 (2023).
Download references
Acknowledgements
We thank the ICPSR Bibliography staff, the ICPSR Data Curation Unit, and the ICPSR Data Stewardship Committee for their support of this research. This material is based upon work supported by the National Science Foundation under grant 1930645. This project was made possible in part by the Institute of Museum and Library Services LG-37-19-0134-19.
Author information
Authors and affiliations.
Inter-university Consortium for Political and Social Research, University of Michigan, Ann Arbor, MI, 48104, USA
Libby Hemphill, Sara Lafia, David Bleckley & Elizabeth Moss
School of Information, University of Michigan, Ann Arbor, MI, 48104, USA
Libby Hemphill & Lizhou Fan
School of Information, University of Arizona, Tucson, AZ, 85721, USA
Andrea Thomer
You can also search for this author in PubMed Google Scholar
Contributions
L.H. and A.T. conceptualized the study design, D.B., E.M., and S.L. prepared the data, S.L., L.F., and L.H. analyzed the data, and D.B. validated the data. All authors reviewed and edited the manuscript.
Corresponding author
Correspondence to Libby Hemphill .
Ethics declarations
Competing interests.
The authors declare no competing interests.
Additional information
Publisher’s note Springer Nature remains neutral with regard to jurisdictional claims in published maps and institutional affiliations.
Rights and permissions
Open Access This article is licensed under a Creative Commons Attribution 4.0 International License, which permits use, sharing, adaptation, distribution and reproduction in any medium or format, as long as you give appropriate credit to the original author(s) and the source, provide a link to the Creative Commons licence, and indicate if changes were made. The images or other third party material in this article are included in the article’s Creative Commons licence, unless indicated otherwise in a credit line to the material. If material is not included in the article’s Creative Commons licence and your intended use is not permitted by statutory regulation or exceeds the permitted use, you will need to obtain permission directly from the copyright holder. To view a copy of this licence, visit http://creativecommons.org/licenses/by/4.0/ .
Reprints and permissions
About this article
Cite this article.
Hemphill, L., Thomer, A., Lafia, S. et al. A dataset for measuring the impact of research data and their curation. Sci Data 11 , 442 (2024). https://doi.org/10.1038/s41597-024-03303-2
Download citation
Received : 16 November 2023
Accepted : 24 April 2024
Published : 03 May 2024
DOI : https://doi.org/10.1038/s41597-024-03303-2
Share this article
Anyone you share the following link with will be able to read this content:
Sorry, a shareable link is not currently available for this article.
Provided by the Springer Nature SharedIt content-sharing initiative
Quick links
- Explore articles by subject
- Guide to authors
- Editorial policies
Sign up for the Nature Briefing newsletter — what matters in science, free to your inbox daily.

Numbers, Facts and Trends Shaping Your World
Read our research on:
Full Topic List
Regions & Countries
- Publications
- Our Methods
- Short Reads
- Tools & Resources
Read Our Research On:
Teens and Video Games Today
- Methodology
Table of Contents
- Who plays video games?
- How often do teens play video games?
- What devices do teens play video games on?
- Social media use among gamers
- Teen views on how much they play video games and efforts to cut back
- Are teens social with others through video games?
- Do teens think video games positively or negatively impact their lives?
- Why do teens play video games?
- Bullying and violence in video games
- Appendix A: Detailed charts
- Acknowledgments
The analysis in this report is based on a self-administered web survey conducted from Sept. 26 to Oct. 23, 2023, among a sample of 1,453 dyads, with each dyad (or pair) comprised of one U.S. teen ages 13 to 17 and one parent per teen. The margin of sampling error for the full sample of 1,453 teens is plus or minus 3.2 percentage points. The margin of sampling error for the full sample of 1,453 parents is plus or minus 3.2 percentage points. The survey was conducted by Ipsos Public Affairs in English and Spanish using KnowledgePanel, its nationally representative online research panel.
The research plan for this project was submitted to an external institutional review board (IRB), Advarra, which is an independent committee of experts that specializes in helping to protect the rights of research participants. The IRB thoroughly vetted this research before data collection began. Due to the risks associated with surveying minors, this research underwent a full board review and received approval (Approval ID Pro00073203).
KnowledgePanel members are recruited through probability sampling methods and include both those with internet access and those who did not have internet access at the time of their recruitment. KnowledgePanel provides internet access for those who do not have it and, if needed, a device to access the internet when they join the panel. KnowledgePanel’s recruitment process was originally based exclusively on a national random-digit dialing (RDD) sampling methodology. In 2009, Ipsos migrated to an address-based sampling (ABS) recruitment methodology via the U.S. Postal Service’s Delivery Sequence File (DSF). The Delivery Sequence File has been estimated to cover as much as 98% of the population, although some studies suggest that the coverage could be in the low 90% range. 4
Panelists were eligible for participation in this survey if they indicated on an earlier profile survey that they were the parent of a teen ages 13 to 17. A random sample of 3,981 eligible panel members were invited to participate in the study. Responding parents were screened and considered qualified for the study if they reconfirmed that they were the parent of at least one child ages 13 to 17 and granted permission for their teen who was chosen to participate in the study. In households with more than one eligible teen, parents were asked to think about one randomly selected teen, and that teen was instructed to complete the teen portion of the survey. A survey was considered complete if both the parent and selected teen completed their portions of the questionnaire, or if the parent did not qualify during the initial screening.
Of the sampled panelists, 1,763 (excluding break-offs) responded to the invitation and 1,453 qualified, completed the parent portion of the survey, and had their selected teen complete the teen portion of the survey, yielding a final stage completion rate of 44% and a qualification rate of 82%. The cumulative response rate accounting for nonresponse to the recruitment surveys and attrition is 2.2%. The break-off rate among those who logged on to the survey (regardless of whether they completed any items or qualified for the study) is 26.9%.
Upon completion, qualified respondents received a cash-equivalent incentive worth $10 for completing the survey. To encourage response from non-Hispanic Black panelists, the incentive was increased from $10 to $20 on Oct 5, 2023. The incentive was increased again on Oct. 10, from $20 to $40; then to $50 on Oct. 17; and to $75 on Oct. 20. Reminders and notifications of the change in incentive were sent for each increase.
All panelists received email invitations and any nonresponders received reminders, shown in the table. The field period was closed on Oct. 23, 2023.
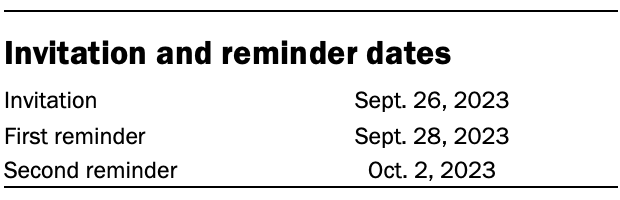
The analysis in this report was performed using separate weights for parents and teens. The parent weight was created in a multistep process that begins with a base design weight for the parent, which is computed to reflect their probability of selection for recruitment into the KnowledgePanel. These selection probabilities were then adjusted to account for the probability of selection for this survey, which included oversamples of non-Hispanic Black and Hispanic parents. Next, an iterative technique was used to align the parent design weights to population benchmarks for parents of teens ages 13 to 17 on the dimensions identified in the accompanying table, to account for any differential nonresponse that may have occurred.
To create the teen weight, an adjustment factor was applied to the final parent weight to reflect the selection of one teen per household. Finally, the teen weights were further raked to match the demographic distribution for teens ages 13 to 17 who live with parents. The teen weights were adjusted on the same teen dimensions as parent dimensions with the exception of teen education, which was not used in the teen weighting.
Sampling errors and tests of statistical significance take into account the effect of weighting. Interviews were conducted in both English and Spanish.
In addition to sampling error, one should bear in mind that question wording and practical difficulties in conducting surveys can introduce error or bias into the findings of opinion polls.
The following table shows the unweighted sample sizes and the error attributable to sampling that would be expected at the 95% level of confidence for different groups in the survey:
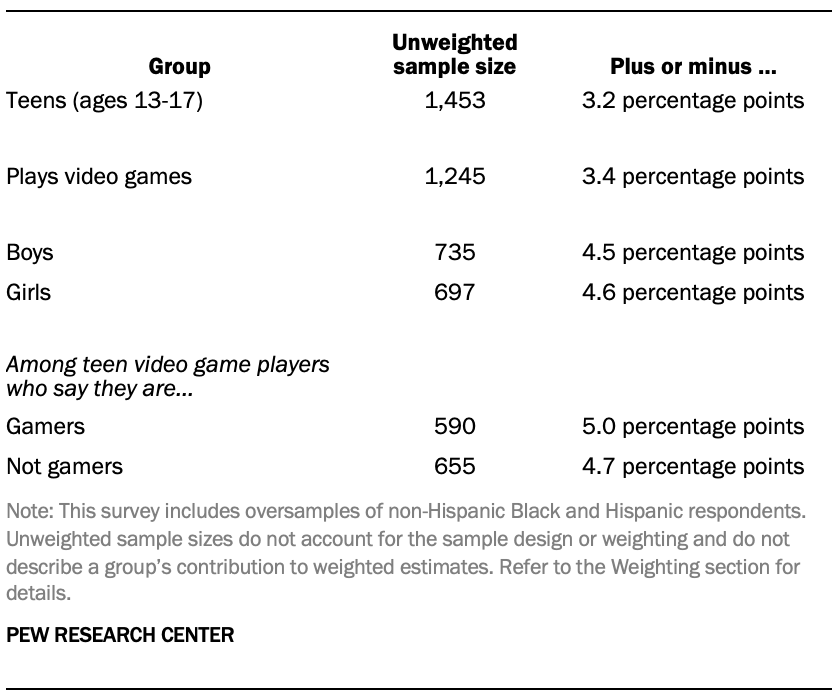
Sample sizes and sampling errors for subgroups are available upon request.
Dispositions and response rates
The tables below display dispositions used in the calculation of completion, qualification and cumulative response rates. 5
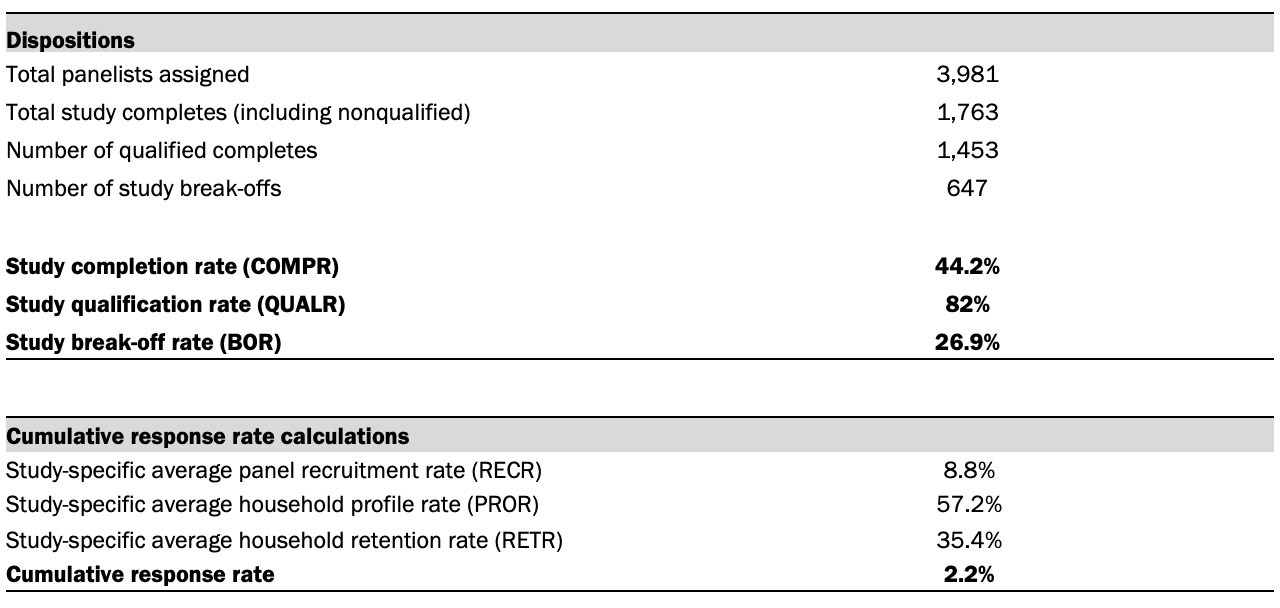
© Pew Research Center, 2023
- AAPOR Task Force on Address-based Sampling. 2016. “AAPOR Report: Address-based Sampling.” ↩
- For more information on this method of calculating response rates, refer to: Callegaro, Mario, and Charles DiSogra. 2008. “Computing response metrics for online panels.” Public Opinion Quarterly. ↩
Sign up for our weekly newsletter
Fresh data delivery Saturday mornings
Sign up for The Briefing
Weekly updates on the world of news & information
- Friendships
- Online Harassment & Bullying
- Teens & Tech
- Teens & Youth
How Teens and Parents Approach Screen Time
Teens and internet, device access fact sheet, teens and social media fact sheet, teens, social media and technology 2023, what the data says about americans’ views of artificial intelligence, most popular, report materials.
1615 L St. NW, Suite 800 Washington, DC 20036 USA (+1) 202-419-4300 | Main (+1) 202-857-8562 | Fax (+1) 202-419-4372 | Media Inquiries
Research Topics
- Age & Generations
- Coronavirus (COVID-19)
- Economy & Work
- Family & Relationships
- Gender & LGBTQ
- Immigration & Migration
- International Affairs
- Internet & Technology
- Methodological Research
- News Habits & Media
- Non-U.S. Governments
- Other Topics
- Politics & Policy
- Race & Ethnicity
- Email Newsletters
ABOUT PEW RESEARCH CENTER Pew Research Center is a nonpartisan fact tank that informs the public about the issues, attitudes and trends shaping the world. It conducts public opinion polling, demographic research, media content analysis and other empirical social science research. Pew Research Center does not take policy positions. It is a subsidiary of The Pew Charitable Trusts .
Copyright 2024 Pew Research Center

An official website of the United States government, Department of Justice.
Here's how you know
Official websites use .gov A .gov website belongs to an official government organization in the United States.
Secure .gov websites use HTTPS A lock ( Lock A locked padlock ) or https:// means you’ve safely connected to the .gov website. Share sensitive information only on official, secure websites.
FY 2024 National Survey of Victim Service Providers (NSVSP)
Download PDF, 403.58 KB
BJS seeks a data collection agent to administer the 2024 National Survey of Victim Service Providers (NSVSP). The NSVSP is part of BJS’s Victim Services Statistical Research Program, an effort to develop a statistical infrastructure around victim services and address major gaps in our knowledge about the availability and use of services to support victims of crime or abuse. As a follow-up to the 2023 National Census of Victim Service Providers (NCVSP), the NSVSP will collect more detailed information on services provided, staffing, and organizational constraints from a representative sample of victim service providers.

Cohort Profile: Health and Attainment of Pupils in a Primary Education National-Cohort (HAPPEN). A hybrid total population cohort in Wales, UK
- Find this author on Google Scholar
- Find this author on PubMed
- Search for this author on this site
- For correspondence: [email protected]
- ORCID record for Hope E Jones
- ORCID record for Michaela L James
- ORCID record for Emily K Marchant
- ORCID record for Amrita Bandyopadhyay
- ORCID record for Gareth Stratton
- ORCID record for Sinead Brophy
- Info/History
- Preview PDF
Purpose: HAPPEN is a primary school national cohort which brings together education, health and wellbeing research in line with the Curriculum for Wales framework for health and wellbeing. Health, education and social care data of primary school children are linked and held in the Secure Anonymised Information Linkage (SAIL) Databank. In addition, school-aged children can take part in the HAPPEN Survey throughout the academic year to inform design and implementation of the Health and Wellbeing curriculum area based on their pupils' needs. There are over 600 schools registered to take part in the HAPPEN Survey. The linkage of health and education records from the HAPPEN national cohort with the HAPPEN Survey responses gives enriched cohort depth and detail which can be used to extrapolate to other schools in Wales. We present the descriptive data available in HAPPEN, and the future expansion plans. Participants: The HAPPEN cohort includes 37,902 primary-aged school children from 2016-July 2023. Of this number, 28,019 can be linked in SAIL with their anonymised linkage field (ALF). In addition, to date (May 2024), HAPPEN Survey data has been captured from over 45,000 children which can in turn be linked to the electronic data. The survey is completed on an ongoing basis and continues to rise by 7000-8000 responses annually. Findings to date: The child cohort is 49% girls, 47% boys (3% prefer not to state their gender and 1% of this data is missing) and 14% are from an ethnic minority background (10% prefer not to state their ethnicity). Initial findings have explored the impact of Covid-19 on wellbeing and play opportunities. As well as a longitudinal exploration of wellbeing throughout the years. Future plans: HAPPEN is an ongoing dynamic cohort of data collection. Access to the cohort is available through SAIL or HDRUK gateway applications. Ongoing research includes the evaluation of interventions for primary school children such as natural experiment methods, non-means tested free school meal roll-out in Wales, interventions to improve physical literacy including changes to the built environment and interventions to improve health and wellbeing of primary school children.
Competing Interest Statement
The authors have declared no competing interest.
Funding Statement
This work is ESRC funded through ADR.
Author Declarations
I confirm all relevant ethical guidelines have been followed, and any necessary IRB and/or ethics committee approvals have been obtained.
The details of the IRB/oversight body that provided approval or exemption for the research described are given below:
This study involves human participants, HAPPEN has been granted ethical approval by Swansea Universitys School of Medicine Ethics Board (ref: 7933). Participants give informed assent to participate in the study before taking part.
I confirm that all necessary patient/participant consent has been obtained and the appropriate institutional forms have been archived, and that any patient/participant/sample identifiers included were not known to anyone (e.g., hospital staff, patients or participants themselves) outside the research group so cannot be used to identify individuals.
I understand that all clinical trials and any other prospective interventional studies must be registered with an ICMJE-approved registry, such as ClinicalTrials.gov. I confirm that any such study reported in the manuscript has been registered and the trial registration ID is provided (note: if posting a prospective study registered retrospectively, please provide a statement in the trial ID field explaining why the study was not registered in advance).
I have followed all appropriate research reporting guidelines, such as any relevant EQUATOR Network research reporting checklist(s) and other pertinent material, if applicable.
Data Availability
Data is available upon reasonable request. Researchers can apply for data access by submitting a research application to the SAIL team. The SAIL website provides information on the application process (https://saildatabank.com/data/apply-to-work-with-the-data/). All proposals to use SAIL data are subject to review by an independent Information Governance Review Panel (IGRP). This projects approval code is 0916. Before any data can be accessed, approval must be given by the IGRP. To use HAPPEN data you need to provide a safe researcher training certificate, a signed data access agreement and IGRP approval.
View the discussion thread.
Thank you for your interest in spreading the word about medRxiv.
NOTE: Your email address is requested solely to identify you as the sender of this article.

Citation Manager Formats
- EndNote (tagged)
- EndNote 8 (xml)
- RefWorks Tagged
- Ref Manager
- Tweet Widget
- Facebook Like
- Google Plus One
- Addiction Medicine (323)
- Allergy and Immunology (627)
- Anesthesia (163)
- Cardiovascular Medicine (2363)
- Dentistry and Oral Medicine (287)
- Dermatology (206)
- Emergency Medicine (378)
- Endocrinology (including Diabetes Mellitus and Metabolic Disease) (833)
- Epidemiology (11755)
- Forensic Medicine (10)
- Gastroenterology (701)
- Genetic and Genomic Medicine (3722)
- Geriatric Medicine (348)
- Health Economics (632)
- Health Informatics (2388)
- Health Policy (929)
- Health Systems and Quality Improvement (894)
- Hematology (340)
- HIV/AIDS (780)
- Infectious Diseases (except HIV/AIDS) (13298)
- Intensive Care and Critical Care Medicine (767)
- Medical Education (365)
- Medical Ethics (104)
- Nephrology (398)
- Neurology (3482)
- Nursing (197)
- Nutrition (522)
- Obstetrics and Gynecology (672)
- Occupational and Environmental Health (661)
- Oncology (1818)
- Ophthalmology (535)
- Orthopedics (218)
- Otolaryngology (286)
- Pain Medicine (232)
- Palliative Medicine (66)
- Pathology (445)
- Pediatrics (1030)
- Pharmacology and Therapeutics (426)
- Primary Care Research (418)
- Psychiatry and Clinical Psychology (3169)
- Public and Global Health (6128)
- Radiology and Imaging (1275)
- Rehabilitation Medicine and Physical Therapy (743)
- Respiratory Medicine (825)
- Rheumatology (379)
- Sexual and Reproductive Health (372)
- Sports Medicine (322)
- Surgery (400)
- Toxicology (50)
- Transplantation (172)
- Urology (145)
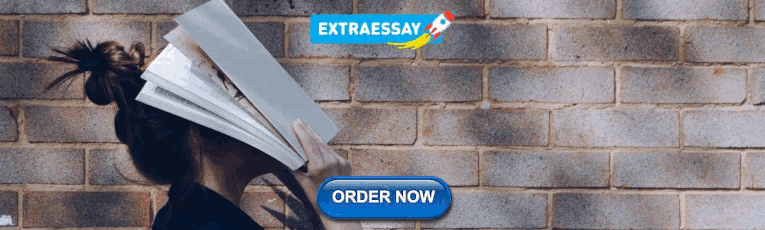
IMAGES
VIDEO
COMMENTS
Questionnaires vs. surveys. A survey is a research method where you collect and analyze data from a group of people. A questionnaire is a specific tool or instrument for collecting the data.. Designing a questionnaire means creating valid and reliable questions that address your research objectives, placing them in a useful order, and selecting an appropriate method for administration.
A questionnaire is defined a market research instrument that consists of questions or prompts to elicit and collect responses from a sample of respondents. This article enlists 21 questionnaire templates along with samples and examples. It also describes the different types of questionnaires and the question types that are used in these ...
Definition: A Questionnaire is a research tool or survey instrument that consists of a set of questions or prompts designed to gather information from individuals or groups of people. It is a standardized way of collecting data from a large number of people by asking them a series of questions related to a specific topic or research objective.
A survey is a research method used for collecting data from a pre-defined group of respondents to gain information and insights on various topics of interest. What is it? The instrument of data collection: Process of collecting and analyzing that data: Characteristic: Subset of survey: Consists of questionnaire and survey design, logic and data ...
A questionnaire is an important instrument in a research study to help the researcher collect relevant data regarding the research topic. It is significant to ensure that the design of the ...
questions to gather data from respondents. Questions are the translated form of what researchers need for their study which can be addressed using the answers of the respondents. A questionnaire, as the main and the most dominant way of collecting primary and quantitative data, makes the process of data collection standardized together
Data collection is the procedure of collecting, measuring, and analyzing accurate insights for research using standard validated techniques. Put simply, data collection is the process of gathering information for a specific purpose. It can be used to answer research questions, make informed business decisions, or improve products and services.
Abstract. Survey methodologies, usually using questionnaires, are among the most popular in. the social sciences, but they are also among the most mis-used. The ir popularity in. small-scale ...
You can use the questionnaire method of data collection for a number of purposes: To determine what your market wants related to the product or service you provide (for market analysis) To get helpful feedback from customers after a purchase. To get intel on customer demographics and preferences to use for product (or service) development.
Survey research means collecting information about a group of people by asking them questions and analysing the results. To conduct an effective survey, follow these six steps: Determine who will participate in the survey. Decide the type of survey (mail, online, or in-person) Design the survey questions and layout. Distribute the survey.
However, the quality and accuracy of data collected using a questionnaire depend on how it is designed, used, and validated. In this two-part series, we discuss how to design (part 1) and how to use and validate (part 2) a research questionnaire. It is important to emphasize that questionnaires seek to gather information from other people and ...
2. Define the target respondents and methods to reach them. The researcher should clearly define the target, study populations from which she/ he collects data and information. Main methods of reaching the respondents are: personal contact, interview, mail/Internet-based questionnaires, telephone interview. Data Collection Tools. 3.
One of the main stages in a research study is data collection that enables the researcher to find answers to research questions. Data collection is the process of collecting data aiming to gain insights regarding the research topic. There are different types of data and different data collection methods accordingly.
One of the main stages in a research study is data collection that enables the researcher to find answers to research questions. Data collection is the process of collecting data aiming to gain ...
Table of contents. Step 1: Define the aim of your research. Step 2: Choose your data collection method. Step 3: Plan your data collection procedures. Step 4: Collect the data. Frequently asked questions about data collection.
Survey is defined as the act of examining a process or questioning a selected sample of individuals to obtain data about a service, product, or process. Data collection surveys collect information from a targeted group of people about their opinions, behavior, or knowledge. Common types of example surveys are written questionnaires, face-to ...
Surveys involve asking questions to a sample of individuals or organizations to collect data. Surveys can be conducted in person, over the phone, or online. ... Research: When conducting research, data collection is used to gather information on variables of interest to answer research questions and test hypotheses.
The chapter starts with a critical examination of the data collection method, a self-administered online survey, chosen for the quantitative research part, and justifies its use. This is followed by a discussion of the questionnaire design, which incorporates the levels of measurement, theory and statistical analysis, an operationalisation of ...
Survey Data: Definition. Survey data is defined as the resultant data that is collected from a sample of respondents that took a survey. This data is comprehensive information gathered from a target audience about a specific topic to conduct research.There are many methods used for survey data collection and statistical analysis.
The EPA's initiative involves gathering basic data from 400 POTWs through a questionnaire, the selection of approximately 200 to 300 POTWs based on questionnaire responses, and the collection of sampling data from said subset of 200 to 300 POTWs. ... and blank samples are great ways to validate and confirm data integrity. Sample collection ...
Questionnaires vs surveys. A survey is a research method where you collect and analyse data from a group of people. A questionnaire is a specific tool or instrument for collecting the data.. Designing a questionnaire means creating valid and reliable questions that address your research objectives, placing them in a useful order, and selecting an appropriate method for administration.
Attention. Historically enhanced 2016-2020 National Survey of Children's Health (NSCH) data files were released in April 2024 and are available on the NSCH datasets page.This data release is a continuation of the improvements that were made to the 2021 NSCH data set released in October 2023.These revised datasets should be used when combining or comparing with the 2022 NSCH.
This paper introduces a dataset developed to measure the impact of archival and data curation decisions on data reuse. The dataset describes 10,605 social science research datasets, their curation ...
A sample is a subset of individuals from a larger population. Sampling means selecting the group that you will actually collect data from in your research. For example, if you are researching the opinions of students in your university, you could survey a sample of 100 students.
Living with Wildfire Series. This is the 20 th report in an on-going series of Rocky Mountain Research Station "Research Notes" that detail Wildfire Research (WiRē) Team projects with fire departments and communities. To date, WiRē has helped 23 partner communities complete 26,358 property-level wildfire risk assessments and implement 8,153 household surveys of perceived wildfire risk to homes.
The research plan for this project was submitted to an external institutional review board (IRB), Advarra, which is an independent committee of experts that specializes in helping to protect the rights of research participants. The IRB thoroughly vetted this research before data collection began.
BJS seeks a data collection agent to administer the 2024 National Survey of Victim Service Providers (NSVSP). The NSVSP is part of BJS's Victim Services Statistical Research Program, an effort to develop a statistical infrastructure around victim services and address major gaps in our knowledge about the availability and use of services to support victims of crime or abuse.
Purpose: HAPPEN is a primary school national cohort which brings together education, health and wellbeing research in line with the Curriculum for Wales framework for health and wellbeing. Health, education and social care data of primary school children are linked and held in the Secure Anonymised Information Linkage (SAIL) Databank. In addition, school-aged children can take part in the ...