We have a new app!
Take the Access library with you wherever you go—easy access to books, videos, images, podcasts, personalized features, and more.
Download the Access App here: iOS and Android . Learn more here!
- Remote Access
- Save figures into PowerPoint
- Download tables as PDFs

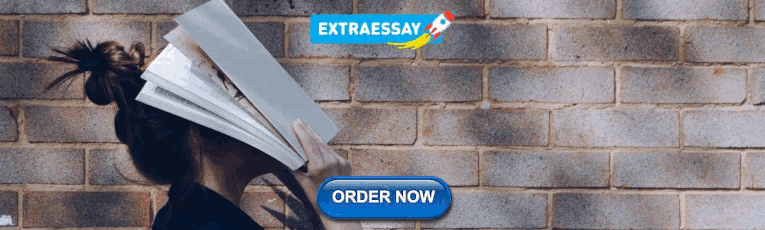
Chapter 14: Descriptive Research
- Download Chapter PDF
Disclaimer: These citations have been automatically generated based on the information we have and it may not be 100% accurate. Please consult the latest official manual style if you have any questions regarding the format accuracy.
Download citation file:
- Search Book
Jump to a Section
Introduction, developmental research.
- NORMATIVE STUDIES
- QUALITATIVE RESEARCH
- DESCRIPTIVE SURVEYS
- CASE STUDIES
- Full Chapter
- Supplementary Content
Descriptive research is designed to document the factors that describe characteristics, behaviors and conditions of individuals and groups. For example, researchers have used this approach to describe a sample of individuals with spinal cord injuries with respect to gender, age, and cause and severity of injury to see whether these properties were similar to those described in the past. 1 Descriptive studies have documented the biomechanical parameters of wheelchair propulsion, 2 and the clinical characteristics of stroke. 3 As our diagram of the continuum of research shows, descriptive and exploratory elements are commonly combined, depending on how the investigator conceptualizes the research question.
Descriptive studies document the nature of existing phenomena and describe how variables change over time. They will generally be structured around a set of guiding questions or research objectives to generate data or characterize a situation of interest. Often this information can be used as a basis for formulation of research hypotheses that can be tested using exploratory or experimental techniques. The descriptive data supply the foundation for classifying individuals, for identifying relevant variables, and for asking new research questions.
Descriptive studies may involve prospective or retrospective data collection, and may be designed using longitudinal or cross-sectional methods (see Chapter 13 ). Surveys and secondary analysis of clinical databases are often used as sources of data for descriptive analysis. Several types of research can be categorized as descriptive, including developmental research, normative research, qualitative research and case studies. The purpose of this chapter is to describe these approaches.
Concepts of human development, whether they are related to cognition, perceptual-motor control, communication, physiological change, or psychological processes, are important elements of a clinical knowledge base. Valid interpretation of clinical outcomes depends on our ability to develop a clear picture of those we treat, their characteristics and performance expectations under different conditions. Developmental research involves the description of developmental change and the sequencing of behaviors in people over time. Developmental studies have contributed to the theoretical foundations of clinical practice in many ways. For example, the classic descriptive studies of Gesell and Amatruda 4 and McGraw 5 provide the basis for much of the research on sequencing of motor development in infants and children. Erikson's studies of life span development have contributed to an understanding of psychological growth through old age. 6
Pop-up div Successfully Displayed
This div only appears when the trigger link is hovered over. Otherwise it is hidden from view.
Please Wait
Qualitative Descriptive Methods in Health Science Research
Affiliations.
- 1 College of Nursing & Health Innovation, Arizona State University, Phoenix, AZ, USA [email protected].
- 2 College of Nursing & Health Innovation, Arizona State University, Phoenix, AZ, USA.
- PMID: 26791375
- PMCID: PMC7586301
- DOI: 10.1177/1937586715614171
Objective: The purpose of this methodology paper is to describe an approach to qualitative design known as qualitative descriptive that is well suited to junior health sciences researchers because it can be used with a variety of theoretical approaches, sampling techniques, and data collection strategies.
Background: It is often difficult for junior qualitative researchers to pull together the tools and resources they need to embark on a high-quality qualitative research study and to manage the volumes of data they collect during qualitative studies. This paper seeks to pull together much needed resources and provide an overview of methods.
Methods: A step-by-step guide to planning a qualitative descriptive study and analyzing the data is provided, utilizing exemplars from the authors' research.
Results: This paper presents steps to conducting a qualitative descriptive study under the following headings: describing the qualitative descriptive approach, designing a qualitative descriptive study, steps to data analysis, and ensuring rigor of findings.
Conclusions: The qualitative descriptive approach results in a summary in everyday, factual language that facilitates understanding of a selected phenomenon across disciplines of health science researchers.
Keywords: qualitative analysis; qualitative descriptive; qualitative design; qualitative methodology; rigor.
© The Author(s) 2016.
- Data Collection / methods*
- Qualitative Research*
- Research Design*
- Statistics as Topic / methods
Grants and funding
- R01 NR010541/NR/NINR NIH HHS/United States
- T32 NR012718/NR/NINR NIH HHS/United States
Descriptive Research and Qualitative Research
Cite this chapter.
- Eunsook T. Koh 2 &
- Willis L. Owen 2
913 Accesses
18 Citations
Descriptive research is a study of status and is widely used in education, nutrition, epidemiology, and the behavioral sciences. Its value is based on the premise that problems can be solved and practices improved through observation, analysis, and description. The most common descriptive research method is the survey, which includes questionnaires, personal interviews, phone surveys, and normative surveys. Developmental research is also descriptive. Through cross-sectional and longitudinal studies, researchers investigate the interaction of diet (e.g., fat and its sources, fiber and its sources, etc.) and life styles (e.g., smoking, alcohol drinking, etc.) and of disease (e.g., cancer, coronary heart disease) development. Observational research and correlational studies constitute other forms of descriptive research. Correlational studies determine and analyze relationships between variables as well as generate predictions. Descriptive research generates data, both qualitative and quantitative, that define the state of nature at a point in time. This chapter discusses some characteristics and basic procedures of the various types of descriptive research.
This is a preview of subscription content, log in via an institution to check access.
Access this chapter
- Available as PDF
- Read on any device
- Instant download
- Own it forever
- Compact, lightweight edition
- Dispatched in 3 to 5 business days
- Free shipping worldwide - see info
- Durable hardcover edition
Tax calculation will be finalised at checkout
Purchases are for personal use only
Institutional subscriptions
Unable to display preview. Download preview PDF.
Achterberg C. Qualitative methods in nutritional education evaluation research. J Nutr Educ 1988; 20: 244
Article Google Scholar
Agar, M.H. The Professional Stranger: an Informal Introduction to Ethnography. Academic Press, New York: Academic Press 1980.
Google Scholar
Amstrong B., Doll R. Environmental factors and cancer incidence and mortality in different countries, with special reference to dietary practices. Int J Cancer, 1975; 15:617–631
Bang H.O., Dyerberg J., Hjorne, N. The composition of food consumed by Greenland Eskimos. Acta Med Scand 1976; 200: 69–75
Article CAS Google Scholar
Bailey, K.D. Methods of Social Research. New York: Free Press, Macmillan Publishing Co, Inc, 1978.
Beal V.A. The nutritional history in longitudinal research. J Am Dietet A 1967; 51: 526–531
Berdie D.R. Questionnaire length and response rate. J Appl Psychol 1973; 58:278–280
Berdie, D.R., Anderson J.F., Niebuhr, M.A. Questionnaires: Design and Use. Metuchen, N.J.: Scarescrow Press, 1986.
Buell P. Changing incidence of breast cancer in Japanese-American women. JNCI 1973; 51:1479–1483
CAS Google Scholar
Dyberg J., Bang H.O., Hjorne N. Fatty acid composition of the plasma lipids in Greenland Eskimos. Am J Clin Nutr 1975; 28: 958–961
Frank G. Life history model of adaptation to disability: the case of a congenital amputee. Soc Sci Med 1984; 19: 639–645
Fetterman, D.L. “A Walk Through the Wilderness: Learning to Find Your Way.” In Experiencing Fieldwork: An Inside View of Qualitative Research. Shaffir, W., Stebbins, R. eds. Newbury Park, CA: Sage, 1991.
Fieldhouse, P. Food & Nutrition: Customs & Culture. New York: Croom Helm, 1986
Fielding, N.G., Fielding, J.L. Linking Data. Beverly Hills, CA: Sage, 1986.
Firestone W.A. Meaning in method: The rhetoric of quantitative and qualitative research. Educational Researcher 1987; 16:16–21
Geertz, C. The interpretation of Cultures. New York: Basic Books, 1973.
Glaser B.G., Strauss, A.L. The Discovery of Grounded Theory: Strategies for Qualitative Research. New York: Aldine Publishing Co, 1967.
Goldberger, J.E. Goldberger on Pellagra. Baton Rouge, LA: Louisiana State University Press, 1964.
Greer J.G. What do open-ended questions measure? Public Opinion Quart 1988; 52:365–371
Gordis, L. Epidemiology. Philadelphia PA: Saunders, 1996.
Hammersley, M., Atkinson, P. Ethnography: Principles in Practice. London: Tavistock, 1983.
Headland, T.N., Pike, K.L., Harris, M. Emics and Etics: The Insider/Outsider Debate. Newbury Park, CA: Sage, 1990.
Hodge, R., Kress, G. Social Semiotics. Ithaca, NY: Cornell University Press, 1988.
Holbrook J.T., Patterson K.Y., Bodner J.E., Douglas L.W., Veillon C., Kelsey J.L. Mertz W, Smith J.C. Sodium and potassium intake and balance in adults consuming self selected diets. Am J Clin Nutr 1984; 40: 786–793
Human Nutrition Information Service, US Department of Agriculture, Food Consumption: Households in the United States, Spring 1977, Washington, D.E.: Government Printing Office, Publication H-1, 1982.
ICNND (International Committee on Nutrition for National Defense) Manual for Nutrition Surveys. Second edition. Superintendent of Documents. Washington, D.C.: U.S. Government Printing Office, 1963
Jacob E. Qualitative research traditions: A review. Rev Educ Res 1987; 57: 1–4
Jelliffe, D.B. The Assessment of the Nutritional Status of the Community. WHO Monograph 53. Geneva: World Health Organization, 1966.
Keys, A. Seven Countries: A Multivariate Analysis of Death and Corornary Heart Disease. Cambridge, MA: Harvard University Press, 1980.
Kittler, P.G., Sucher, K. Food and Culture in America. New York: Reinhold, 1989.
Kirk, J., Miller, M.L. Reliability and Validity in Qualitative Research. Beverly Hills, CA: Sage, 1986.
Koh E.T., Caples V. Nutrient intake of low-income, black families in Southwest Mississippi. J Am Dietet A 1979; 75:665–670
Kolonel, L.N., Hinds, M.W., Hankin, J.H. “Cancer Patterns Among Migrant and Native-Born Japanese in Hawaii in Relation to Smoking, Drinking, and Dietary Habits.” In Genetic and Environmental Factors in Experimental and Human Cancer. Gelboin, H.V. et al. eds. Tokyo, Japan: Science Press, 1980.
Kromann N., Green A. Epidemiological studies in the Upernavik district, Greenland: Incidence of some chronic disease 1950–1974. Acta Med Scand 1980; 401–405
Krueger, R. Focus Groups: A Practical Guide for Applied Rese arch. Newbury Park, CA: Sage, 1988.
Langness, L.L. Frank, G. Lives: An Anthropological Approach to Biography. Novato, CA: Chandler & Sharp, 1981.
Lind, J. (1753) A Treatise on the Scurvy. Reprinted Edinburgh: Edinburgh University Press, 1953.
Lock, L.F. “The Question of Quality in Qualitative Research.” In Proceedings of the 5th Measurement and Evaluation Symposium. Nelson, J.K. ed. Baton Rouge, LA: Louisiana State University Press, 1987.
Lofland, J., Lofland, L. Analyzing Social Settings: A Guide to Qualitative Research. Belmont, CA: Wadsworth, 1984.
Marshall, C; Rossman, G.B. Designing Qualitative Research. Newbury Park, CA: Sage, 1989.
Mauser, J.S., Kramer, S. Epidemiology — An Introductory Text. Philadelphia PA: Saunders, 1985.
McClendon M.J., O’Brien D.J. Question-order effects on the determinants of subjective well-being. Public Opinion Quart 1988; 52:351–364
McCraken, G. The Long Interview. Newbury Park, CA: Sage, 1988.
McMichael A.J., McCall M.G., Hartshorne J.M., Woodings T.L. Patterns of gastro-intestinal cancer in European migrants to Australia: The role of dietary changes. In J Cancer 1980; 25:431–437
Miles, M.B., Huberman, A.M. Qualitative Data Analysis: A Sourcebook of New Methods. (2nd ed.) Thousand Oaks, CA: Sage, 1994.
Montgomery A.C., Crittenden K.S. Improving coding reliability for open-ended questions. Public Opinion Quart 1977; 41: 235–243
National Center for Health Statistics: Plan and Operation of the HANES. Vital and Health Statistics, Series 1, Nos 10a and 10b, DHEW Pub No (HSM) 73–130. Washington, D.C.: U.S. Government Printing Office, 1973.
O’Brien T., Dugdale V. Questionnaire administration by computer. J Market Res Soc, 1978; 20:228–237
Okolo, E.N. Health Research Design and Methodology. Boca Raton, FL: CRC Press 1990.
Parkin, D.M., Muir, C.S., Whelan, S.L., Gao, Y.T., Ferlay, J., Powell, J. Cancer Incidence in Five Continents VI IARC Sci Publ 120, Lyon: International Agency for Research on Cancer, 1992.
Peterkin B.B., Rizek R.L., Tippett K.S. Nationwide food consumption survey, 1987. Nutr Today 1988; 23:18–24
Poe G.S., Seeman I., McLaughlin J., Mehl E., Dietz M. Don’t know boxes in factual questions in a mail questionnaire: Effects on level and quality of response. Public Opinion Quart 1988; 52: 212–222
Poikolainen K., Karkkainen P. Nature of questionnaire options affects estimates of alcohol intake. J Stud Alcohol 1985; 46: 219–222
Potter, J.D. Food, Nutrition and the Prevention of Cancer: A Global Perspective. Washington, D.C.: American Institute for Cancer Research, 1997.
Rose, G.A., Blackburn, H. Cardiovascular Survey Methods. Geneva, Switzerland: World Health Organization 1968.
Runcie, J.F. Experiencing Social Sresearch. Homewood, IL.: Dorsey Press, 1976.
Sandelowski, M. The Problem of Rigor in Qualitative Research. Adv Nurs Sci April 27–37, 1986.
Schutz R.W. Qualitative research: comments and controversies. Research Quarterly Exercise Sport, 1989; 60:30–35
Sheatsley, P.B. “Questionnaire Construction and Item Writing.” In Handbook of Survey Research. Rossi, P.H., Wright, J.D., Anderson, A.B. eds. Orlando, FL: Academic Press, 1983.
Sherry, B. “Epidemiologic Analytical Research.” In Research. Monsen, E.R. Chicago, IL: American Dietetic Association, 1992
Spradley, J. The Ethnographic Interview. Chicago, IL: Holtz, Rinehart & Winston, 1979.
Spradley, J.P. Participant Observation. New York: Holt, Rinehart & Winston, 1980.
Sudman, S., Bradburn, N.M. Asking Questions: A Practical Guide to Questionnaire Design. (2nd ed.) Washington, D.C.: Jossey-Bass Publisher, 1983.
Taylor, S.J., Bogdan, R. Introduction to Qualitative Research Methods. (2nd ed.) New York: Wiley, 1984.
Thomas, J.R. Nelson, J.K. Research Methods in Physical Activity. (3rd ed.), Champaign, IL: Human Kinetics, 1996.
Van Maanen, J. ed. Qualitative Methodology. Newbury Park, CA: Sage, 1983.
Willett, W. Nutritional Epidemiology. (2nd ed.) New York: Oxford University Press, 1998.
Book Google Scholar
Download references
Author information
Authors and affiliations.
University of Oklahoma Health Sciences Center, USA
Eunsook T. Koh & Willis L. Owen
You can also search for this author in PubMed Google Scholar
Rights and permissions
Reprints and permissions
Copyright information
© 2000 Springer Science+Business Media New York
About this chapter
Koh, E.T., Owen, W.L. (2000). Descriptive Research and Qualitative Research. In: Introduction to Nutrition and Health Research. Springer, Boston, MA. https://doi.org/10.1007/978-1-4615-1401-5_12
Download citation
DOI : https://doi.org/10.1007/978-1-4615-1401-5_12
Publisher Name : Springer, Boston, MA
Print ISBN : 978-1-4613-5535-9
Online ISBN : 978-1-4615-1401-5
eBook Packages : Springer Book Archive
Share this chapter
Anyone you share the following link with will be able to read this content:
Sorry, a shareable link is not currently available for this article.
Provided by the Springer Nature SharedIt content-sharing initiative
- Publish with us
Policies and ethics
- Find a journal
- Track your research
- Privacy Policy

Home » Descriptive Research Design – Types, Methods and Examples
Descriptive Research Design – Types, Methods and Examples
Table of Contents

Descriptive Research Design
Definition:
Descriptive research design is a type of research methodology that aims to describe or document the characteristics, behaviors, attitudes, opinions, or perceptions of a group or population being studied.
Descriptive research design does not attempt to establish cause-and-effect relationships between variables or make predictions about future outcomes. Instead, it focuses on providing a detailed and accurate representation of the data collected, which can be useful for generating hypotheses, exploring trends, and identifying patterns in the data.
Types of Descriptive Research Design
Types of Descriptive Research Design are as follows:
Cross-sectional Study
This involves collecting data at a single point in time from a sample or population to describe their characteristics or behaviors. For example, a researcher may conduct a cross-sectional study to investigate the prevalence of certain health conditions among a population, or to describe the attitudes and beliefs of a particular group.
Longitudinal Study
This involves collecting data over an extended period of time, often through repeated observations or surveys of the same group or population. Longitudinal studies can be used to track changes in attitudes, behaviors, or outcomes over time, or to investigate the effects of interventions or treatments.
This involves an in-depth examination of a single individual, group, or situation to gain a detailed understanding of its characteristics or dynamics. Case studies are often used in psychology, sociology, and business to explore complex phenomena or to generate hypotheses for further research.
Survey Research
This involves collecting data from a sample or population through standardized questionnaires or interviews. Surveys can be used to describe attitudes, opinions, behaviors, or demographic characteristics of a group, and can be conducted in person, by phone, or online.
Observational Research
This involves observing and documenting the behavior or interactions of individuals or groups in a natural or controlled setting. Observational studies can be used to describe social, cultural, or environmental phenomena, or to investigate the effects of interventions or treatments.
Correlational Research
This involves examining the relationships between two or more variables to describe their patterns or associations. Correlational studies can be used to identify potential causal relationships or to explore the strength and direction of relationships between variables.
Data Analysis Methods
Descriptive research design data analysis methods depend on the type of data collected and the research question being addressed. Here are some common methods of data analysis for descriptive research:
Descriptive Statistics
This method involves analyzing data to summarize and describe the key features of a sample or population. Descriptive statistics can include measures of central tendency (e.g., mean, median, mode) and measures of variability (e.g., range, standard deviation).
Cross-tabulation
This method involves analyzing data by creating a table that shows the frequency of two or more variables together. Cross-tabulation can help identify patterns or relationships between variables.
Content Analysis
This method involves analyzing qualitative data (e.g., text, images, audio) to identify themes, patterns, or trends. Content analysis can be used to describe the characteristics of a sample or population, or to identify factors that influence attitudes or behaviors.
Qualitative Coding
This method involves analyzing qualitative data by assigning codes to segments of data based on their meaning or content. Qualitative coding can be used to identify common themes, patterns, or categories within the data.
Visualization
This method involves creating graphs or charts to represent data visually. Visualization can help identify patterns or relationships between variables and make it easier to communicate findings to others.
Comparative Analysis
This method involves comparing data across different groups or time periods to identify similarities and differences. Comparative analysis can help describe changes in attitudes or behaviors over time or differences between subgroups within a population.
Applications of Descriptive Research Design
Descriptive research design has numerous applications in various fields. Some of the common applications of descriptive research design are:
- Market research: Descriptive research design is widely used in market research to understand consumer preferences, behavior, and attitudes. This helps companies to develop new products and services, improve marketing strategies, and increase customer satisfaction.
- Health research: Descriptive research design is used in health research to describe the prevalence and distribution of a disease or health condition in a population. This helps healthcare providers to develop prevention and treatment strategies.
- Educational research: Descriptive research design is used in educational research to describe the performance of students, schools, or educational programs. This helps educators to improve teaching methods and develop effective educational programs.
- Social science research: Descriptive research design is used in social science research to describe social phenomena such as cultural norms, values, and beliefs. This helps researchers to understand social behavior and develop effective policies.
- Public opinion research: Descriptive research design is used in public opinion research to understand the opinions and attitudes of the general public on various issues. This helps policymakers to develop effective policies that are aligned with public opinion.
- Environmental research: Descriptive research design is used in environmental research to describe the environmental conditions of a particular region or ecosystem. This helps policymakers and environmentalists to develop effective conservation and preservation strategies.
Descriptive Research Design Examples
Here are some real-time examples of descriptive research designs:
- A restaurant chain wants to understand the demographics and attitudes of its customers. They conduct a survey asking customers about their age, gender, income, frequency of visits, favorite menu items, and overall satisfaction. The survey data is analyzed using descriptive statistics and cross-tabulation to describe the characteristics of their customer base.
- A medical researcher wants to describe the prevalence and risk factors of a particular disease in a population. They conduct a cross-sectional study in which they collect data from a sample of individuals using a standardized questionnaire. The data is analyzed using descriptive statistics and cross-tabulation to identify patterns in the prevalence and risk factors of the disease.
- An education researcher wants to describe the learning outcomes of students in a particular school district. They collect test scores from a representative sample of students in the district and use descriptive statistics to calculate the mean, median, and standard deviation of the scores. They also create visualizations such as histograms and box plots to show the distribution of scores.
- A marketing team wants to understand the attitudes and behaviors of consumers towards a new product. They conduct a series of focus groups and use qualitative coding to identify common themes and patterns in the data. They also create visualizations such as word clouds to show the most frequently mentioned topics.
- An environmental scientist wants to describe the biodiversity of a particular ecosystem. They conduct an observational study in which they collect data on the species and abundance of plants and animals in the ecosystem. The data is analyzed using descriptive statistics to describe the diversity and richness of the ecosystem.
How to Conduct Descriptive Research Design
To conduct a descriptive research design, you can follow these general steps:
- Define your research question: Clearly define the research question or problem that you want to address. Your research question should be specific and focused to guide your data collection and analysis.
- Choose your research method: Select the most appropriate research method for your research question. As discussed earlier, common research methods for descriptive research include surveys, case studies, observational studies, cross-sectional studies, and longitudinal studies.
- Design your study: Plan the details of your study, including the sampling strategy, data collection methods, and data analysis plan. Determine the sample size and sampling method, decide on the data collection tools (such as questionnaires, interviews, or observations), and outline your data analysis plan.
- Collect data: Collect data from your sample or population using the data collection tools you have chosen. Ensure that you follow ethical guidelines for research and obtain informed consent from participants.
- Analyze data: Use appropriate statistical or qualitative analysis methods to analyze your data. As discussed earlier, common data analysis methods for descriptive research include descriptive statistics, cross-tabulation, content analysis, qualitative coding, visualization, and comparative analysis.
- I nterpret results: Interpret your findings in light of your research question and objectives. Identify patterns, trends, and relationships in the data, and describe the characteristics of your sample or population.
- Draw conclusions and report results: Draw conclusions based on your analysis and interpretation of the data. Report your results in a clear and concise manner, using appropriate tables, graphs, or figures to present your findings. Ensure that your report follows accepted research standards and guidelines.
When to Use Descriptive Research Design
Descriptive research design is used in situations where the researcher wants to describe a population or phenomenon in detail. It is used to gather information about the current status or condition of a group or phenomenon without making any causal inferences. Descriptive research design is useful in the following situations:
- Exploratory research: Descriptive research design is often used in exploratory research to gain an initial understanding of a phenomenon or population.
- Identifying trends: Descriptive research design can be used to identify trends or patterns in a population, such as changes in consumer behavior or attitudes over time.
- Market research: Descriptive research design is commonly used in market research to understand consumer preferences, behavior, and attitudes.
- Health research: Descriptive research design is useful in health research to describe the prevalence and distribution of a disease or health condition in a population.
- Social science research: Descriptive research design is used in social science research to describe social phenomena such as cultural norms, values, and beliefs.
- Educational research: Descriptive research design is used in educational research to describe the performance of students, schools, or educational programs.
Purpose of Descriptive Research Design
The main purpose of descriptive research design is to describe and measure the characteristics of a population or phenomenon in a systematic and objective manner. It involves collecting data that describe the current status or condition of the population or phenomenon of interest, without manipulating or altering any variables.
The purpose of descriptive research design can be summarized as follows:
- To provide an accurate description of a population or phenomenon: Descriptive research design aims to provide a comprehensive and accurate description of a population or phenomenon of interest. This can help researchers to develop a better understanding of the characteristics of the population or phenomenon.
- To identify trends and patterns: Descriptive research design can help researchers to identify trends and patterns in the data, such as changes in behavior or attitudes over time. This can be useful for making predictions and developing strategies.
- To generate hypotheses: Descriptive research design can be used to generate hypotheses or research questions that can be tested in future studies. For example, if a descriptive study finds a correlation between two variables, this could lead to the development of a hypothesis about the causal relationship between the variables.
- To establish a baseline: Descriptive research design can establish a baseline or starting point for future research. This can be useful for comparing data from different time periods or populations.
Characteristics of Descriptive Research Design
Descriptive research design has several key characteristics that distinguish it from other research designs. Some of the main characteristics of descriptive research design are:
- Objective : Descriptive research design is objective in nature, which means that it focuses on collecting factual and accurate data without any personal bias. The researcher aims to report the data objectively without any personal interpretation.
- Non-experimental: Descriptive research design is non-experimental, which means that the researcher does not manipulate any variables. The researcher simply observes and records the behavior or characteristics of the population or phenomenon of interest.
- Quantitative : Descriptive research design is quantitative in nature, which means that it involves collecting numerical data that can be analyzed using statistical techniques. This helps to provide a more precise and accurate description of the population or phenomenon.
- Cross-sectional: Descriptive research design is often cross-sectional, which means that the data is collected at a single point in time. This can be useful for understanding the current state of the population or phenomenon, but it may not provide information about changes over time.
- Large sample size: Descriptive research design typically involves a large sample size, which helps to ensure that the data is representative of the population of interest. A large sample size also helps to increase the reliability and validity of the data.
- Systematic and structured: Descriptive research design involves a systematic and structured approach to data collection, which helps to ensure that the data is accurate and reliable. This involves using standardized procedures for data collection, such as surveys, questionnaires, or observation checklists.
Advantages of Descriptive Research Design
Descriptive research design has several advantages that make it a popular choice for researchers. Some of the main advantages of descriptive research design are:
- Provides an accurate description: Descriptive research design is focused on accurately describing the characteristics of a population or phenomenon. This can help researchers to develop a better understanding of the subject of interest.
- Easy to conduct: Descriptive research design is relatively easy to conduct and requires minimal resources compared to other research designs. It can be conducted quickly and efficiently, and data can be collected through surveys, questionnaires, or observations.
- Useful for generating hypotheses: Descriptive research design can be used to generate hypotheses or research questions that can be tested in future studies. For example, if a descriptive study finds a correlation between two variables, this could lead to the development of a hypothesis about the causal relationship between the variables.
- Large sample size : Descriptive research design typically involves a large sample size, which helps to ensure that the data is representative of the population of interest. A large sample size also helps to increase the reliability and validity of the data.
- Can be used to monitor changes : Descriptive research design can be used to monitor changes over time in a population or phenomenon. This can be useful for identifying trends and patterns, and for making predictions about future behavior or attitudes.
- Can be used in a variety of fields : Descriptive research design can be used in a variety of fields, including social sciences, healthcare, business, and education.
Limitation of Descriptive Research Design
Descriptive research design also has some limitations that researchers should consider before using this design. Some of the main limitations of descriptive research design are:
- Cannot establish cause and effect: Descriptive research design cannot establish cause and effect relationships between variables. It only provides a description of the characteristics of the population or phenomenon of interest.
- Limited generalizability: The results of a descriptive study may not be generalizable to other populations or situations. This is because descriptive research design often involves a specific sample or situation, which may not be representative of the broader population.
- Potential for bias: Descriptive research design can be subject to bias, particularly if the researcher is not objective in their data collection or interpretation. This can lead to inaccurate or incomplete descriptions of the population or phenomenon of interest.
- Limited depth: Descriptive research design may provide a superficial description of the population or phenomenon of interest. It does not delve into the underlying causes or mechanisms behind the observed behavior or characteristics.
- Limited utility for theory development: Descriptive research design may not be useful for developing theories about the relationship between variables. It only provides a description of the variables themselves.
- Relies on self-report data: Descriptive research design often relies on self-report data, such as surveys or questionnaires. This type of data may be subject to biases, such as social desirability bias or recall bias.
About the author
Muhammad Hassan
Researcher, Academic Writer, Web developer
You may also like

Questionnaire – Definition, Types, and Examples

Case Study – Methods, Examples and Guide

Observational Research – Methods and Guide

Quantitative Research – Methods, Types and...

Qualitative Research Methods

Explanatory Research – Types, Methods, Guide
- Open access
- Published: 19 April 2024
A scoping review of continuous quality improvement in healthcare system: conceptualization, models and tools, barriers and facilitators, and impact
- Aklilu Endalamaw 1 , 2 ,
- Resham B Khatri 1 , 3 ,
- Tesfaye Setegn Mengistu 1 , 2 ,
- Daniel Erku 1 , 4 , 5 ,
- Eskinder Wolka 6 ,
- Anteneh Zewdie 6 &
- Yibeltal Assefa 1
BMC Health Services Research volume 24 , Article number: 487 ( 2024 ) Cite this article
674 Accesses
Metrics details
The growing adoption of continuous quality improvement (CQI) initiatives in healthcare has generated a surge in research interest to gain a deeper understanding of CQI. However, comprehensive evidence regarding the diverse facets of CQI in healthcare has been limited. Our review sought to comprehensively grasp the conceptualization and principles of CQI, explore existing models and tools, analyze barriers and facilitators, and investigate its overall impacts.
This qualitative scoping review was conducted using Arksey and O’Malley’s methodological framework. We searched articles in PubMed, Web of Science, Scopus, and EMBASE databases. In addition, we accessed articles from Google Scholar. We used mixed-method analysis, including qualitative content analysis and quantitative descriptive for quantitative findings to summarize findings and PRISMA extension for scoping reviews (PRISMA-ScR) framework to report the overall works.
A total of 87 articles, which covered 14 CQI models, were included in the review. While 19 tools were used for CQI models and initiatives, Plan-Do-Study/Check-Act cycle was the commonly employed model to understand the CQI implementation process. The main reported purposes of using CQI, as its positive impact, are to improve the structure of the health system (e.g., leadership, health workforce, health technology use, supplies, and costs), enhance healthcare delivery processes and outputs (e.g., care coordination and linkages, satisfaction, accessibility, continuity of care, safety, and efficiency), and improve treatment outcome (reduce morbidity and mortality). The implementation of CQI is not without challenges. There are cultural (i.e., resistance/reluctance to quality-focused culture and fear of blame or punishment), technical, structural (related to organizational structure, processes, and systems), and strategic (inadequate planning and inappropriate goals) related barriers that were commonly reported during the implementation of CQI.
Conclusions
Implementing CQI initiatives necessitates thoroughly comprehending key principles such as teamwork and timeline. To effectively address challenges, it’s crucial to identify obstacles and implement optimal interventions proactively. Healthcare professionals and leaders need to be mentally equipped and cognizant of the significant role CQI initiatives play in achieving purposes for quality of care.
Peer Review reports
Continuous quality improvement (CQI) initiative is a crucial initiative aimed at enhancing quality in the health system that has gradually been adopted in the healthcare industry. In the early 20th century, Shewhart laid the foundation for quality improvement by describing three essential steps for process improvement: specification, production, and inspection [ 1 , 2 ]. Then, Deming expanded Shewhart’s three-step model into ‘plan, do, study/check, and act’ (PDSA or PDCA) cycle, which was applied to management practices in Japan in the 1950s [ 3 ] and was gradually translated into the health system. In 1991, Kuperman applied a CQI approach to healthcare, comprising selecting a process to be improved, assembling a team of expert clinicians that understands the process and the outcomes, determining key steps in the process and expected outcomes, collecting data that measure the key process steps and outcomes, and providing data feedback to the practitioners [ 4 ]. These philosophies have served as the baseline for the foundation of principles for continuous improvement [ 5 ].
Continuous quality improvement fosters a culture of continuous learning, innovation, and improvement. It encourages proactive identification and resolution of problems, promotes employee engagement and empowerment, encourages trust and respect, and aims for better quality of care [ 6 , 7 ]. These characteristics drive the interaction of CQI with other quality improvement projects, such as quality assurance and total quality management [ 8 ]. Quality assurance primarily focuses on identifying deviations or errors through inspections, audits, and formal reviews, often settling for what is considered ‘good enough’, rather than pursuing the highest possible standards [ 9 , 10 ], while total quality management is implemented as the management philosophy and system to improve all aspects of an organization continuously [ 11 ].
Continuous quality improvement has been implemented to provide quality care. However, providing effective healthcare is a complicated and complex task in achieving the desired health outcomes and the overall well-being of individuals and populations. It necessitates tackling issues, including access, patient safety, medical advances, care coordination, patient-centered care, and quality monitoring [ 12 , 13 ], rooted long ago. It is assumed that the history of quality improvement in healthcare started in 1854 when Florence Nightingale introduced quality improvement documentation [ 14 ]. Over the passing decades, Donabedian introduced structure, processes, and outcomes as quality of care components in 1966 [ 15 ]. More comprehensively, the Institute of Medicine in the United States of America (USA) has identified effectiveness, efficiency, equity, patient-centredness, safety, and timeliness as the components of quality of care [ 16 ]. Moreover, quality of care has recently been considered an integral part of universal health coverage (UHC) [ 17 ], which requires initiatives to mobilise essential inputs [ 18 ].
While the overall objective of CQI in health system is to enhance the quality of care, it is important to note that the purposes and principles of CQI can vary across different contexts [ 19 , 20 ]. This variation has sparked growing research interest. For instance, a review of CQI approaches for capacity building addressed its role in health workforce development [ 21 ]. Another systematic review, based on random-controlled design studies, assessed the effectiveness of CQI using training as an intervention and the PDSA model [ 22 ]. As a research gap, the former review was not directly related to the comprehensive elements of quality of care, while the latter focused solely on the impact of training using the PDSA model, among other potential models. Additionally, a review conducted in 2015 aimed to identify barriers and facilitators of CQI in Canadian contexts [ 23 ]. However, all these reviews presented different perspectives and investigated distinct outcomes. This suggests that there is still much to explore in terms of comprehensively understanding the various aspects of CQI initiatives in healthcare.
As a result, we conducted a scoping review to address several aspects of CQI. Scoping reviews serve as a valuable tool for systematically mapping the existing literature on a specific topic. They are instrumental when dealing with heterogeneous or complex bodies of research. Scoping reviews provide a comprehensive overview by summarizing and disseminating findings across multiple studies, even when evidence varies significantly [ 24 ]. In our specific scoping review, we included various types of literature, including systematic reviews, to enhance our understanding of CQI.
This scoping review examined how CQI is conceptualized and measured and investigated models and tools for its application while identifying implementation challenges and facilitators. It also analyzed the purposes and impact of CQI on the health systems, providing valuable insights for enhancing healthcare quality.
Protocol registration and results reporting
Protocol registration for this scoping review was not conducted. Arksey and O’Malley’s methodological framework was utilized to conduct this scoping review [ 25 ]. The scoping review procedures start by defining the research questions, identifying relevant literature, selecting articles, extracting data, and summarizing the results. The review findings are reported using the PRISMA extension for a scoping review (PRISMA-ScR) [ 26 ]. McGowan and colleagues also advised researchers to report findings from scoping reviews using PRISMA-ScR [ 27 ].
Defining the research problems
This review aims to comprehensively explore the conceptualization, models, tools, barriers, facilitators, and impacts of CQI within the healthcare system worldwide. Specifically, we address the following research questions: (1) How has CQI been defined across various contexts? (2) What are the diverse approaches to implementing CQI in healthcare settings? (3) Which tools are commonly employed for CQI implementation ? (4) What barriers hinder and facilitators support successful CQI initiatives? and (5) What effects CQI initiatives have on the overall care quality?
Information source and search strategy
We conducted the search in PubMed, Web of Science, Scopus, and EMBASE databases, and the Google Scholar search engine. The search terms were selected based on three main distinct concepts. One group was CQI-related terms. The second group included terms related to the purpose for which CQI has been implemented, and the third group included processes and impact. These terms were selected based on the Donabedian framework of structure, process, and outcome [ 28 ]. Additionally, the detailed keywords were recruited from the primary health framework, which has described lists of dimensions under process, output, outcome, and health system goals of any intervention for health [ 29 ]. The detailed search strategy is presented in the Supplementary file 1 (Search strategy). The search for articles was initiated on August 12, 2023, and the last search was conducted on September 01, 2023.
Eligibility criteria and article selection
Based on the scoping review’s population, concept, and context frameworks [ 30 ], the population included any patients or clients. Additionally, the concepts explored in the review encompassed definitions, implementation, models, tools, barriers, facilitators, and impacts of CQI. Furthermore, the review considered contexts at any level of health systems. We included articles if they reported results of qualitative or quantitative empirical study, case studies, analytic or descriptive synthesis, any review, and other written documents, were published in peer-reviewed journals, and were designed to address at least one of the identified research questions or one of the identified implementation outcomes or their synonymous taxonomy as described in the search strategy. Based on additional contexts, we included articles published in English without geographic and time limitations. We excluded articles with abstracts only, conference abstracts, letters to editors, commentators, and corrections.
We exported all citations to EndNote x20 to remove duplicates and screen relevant articles. The article selection process includes automatic duplicate removal by using EndNote x20, unmatched title and abstract removal, citation and abstract-only materials removal, and full-text assessment. The article selection process was mainly conducted by the first author (AE) and reported to the team during the weekly meetings. The first author encountered papers that caused confusion regarding whether to include or exclude them and discussed them with the last author (YA). Then, decisions were ultimately made. Whenever disagreements happened, they were resolved by discussion and reconsideration of the review questions in relation to the written documents of the article. Further statistical analysis, such as calculating Kappa, was not performed to determine article inclusion or exclusion.
Data extraction and data items
We extracted first author, publication year, country, settings, health problem, the purpose of the study, study design, types of intervention if applicable, CQI approaches/steps if applicable, CQI tools and procedures if applicable, and main findings using a customized Microsoft Excel form.
Summarizing and reporting the results
The main findings were summarized and described based on the main themes, including concepts under conceptualizing, principles, teams, timelines, models, tools, barriers, facilitators, and impacts of CQI. Results-based convergent synthesis, achieved through mixed-method analysis, involved content analysis to identify the thematic presentation of findings. Additionally, a narrative description was used for quantitative findings, aligning them with the appropriate theme. The authors meticulously reviewed the primary findings from each included material and contextualized these findings concerning the main themes1. This approach provides a comprehensive understanding of complex interventions and health systems, acknowledging quantitative and qualitative evidence.
Search results
A total of 11,251 documents were identified from various databases: SCOPUS ( n = 4,339), PubMed ( n = 2,893), Web of Science ( n = 225), EMBASE ( n = 3,651), and Google Scholar ( n = 143). After removing duplicates ( n = 5,061), 6,190 articles were evaluated by title and abstract. Subsequently, 208 articles were assessed for full-text eligibility. Following the eligibility criteria, 121 articles were excluded, leaving 87 included in the current review (Fig. 1 ).
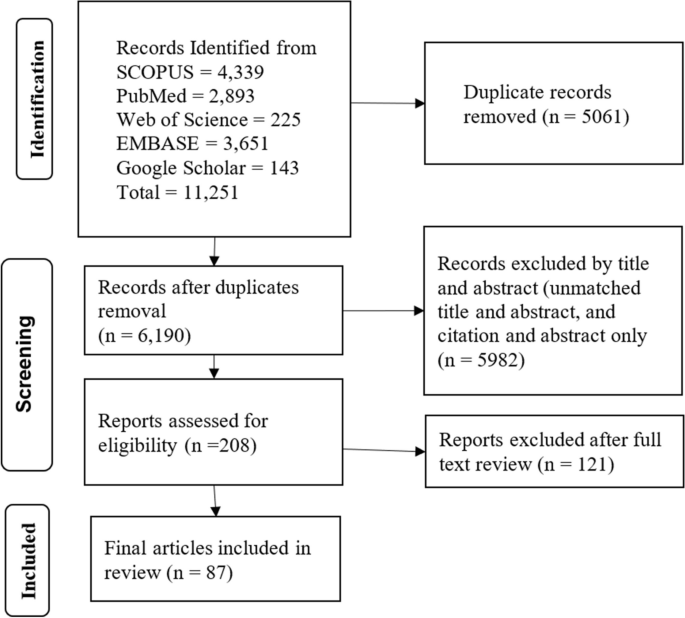
Article selection process
Operationalizing continuous quality improvement
Continuous Quality Improvement (CQI) is operationalized as a cyclic process that requires commitment to implementation, teamwork, time allocation, and celebrating successes and failures.
CQI is a cyclic ongoing process that is followed reflexive, analytical and iterative steps, including identifying gaps, generating data, developing and implementing action plans, evaluating performance, providing feedback to implementers and leaders, and proposing necessary adjustments [ 31 , 32 , 33 , 34 , 35 , 36 , 37 , 38 ].
CQI requires committing to the philosophy, involving continuous improvement [ 19 , 38 ], establishing a mission statement [ 37 ], and understanding quality definition [ 19 ].
CQI involves a wide range of patient-oriented measures and performance indicators, specifically satisfying internal and external customers, developing quality assurance, adopting common quality measures, and selecting process measures [ 8 , 19 , 35 , 36 , 37 , 39 , 40 ].
CQI requires celebrating success and failure without personalization, leading each team member to develop error-free attitudes [ 19 ]. Success and failure are related to underlying organizational processes and systems as causes of failure rather than blaming individuals [ 8 ] because CQI is process-focused based on collaborative, data-driven, responsive, rigorous and problem-solving statistical analysis [ 8 , 19 , 38 ]. Furthermore, a gap or failure opens another opportunity for establishing a data-driven learning organization [ 41 ].
CQI cannot be implemented without a CQI team [ 8 , 19 , 37 , 39 , 42 , 43 , 44 , 45 , 46 ]. A CQI team comprises individuals from various disciplines, often comprising a team leader, a subject matter expert (physician or other healthcare provider), a data analyst, a facilitator, frontline staff, and stakeholders [ 39 , 43 , 47 , 48 , 49 ]. It is also important to note that inviting stakeholders or partners as part of the CQI support intervention is crucial [ 19 , 38 , 48 ].
The timeline is another distinct feature of CQI because the results of CQI vary based on the implementation duration of each cycle [ 35 ]. There is no specific time limit for CQI implementation, although there is a general consensus that a cycle of CQI should be relatively short [ 35 ]. For instance, a CQI implementation took 2 months [ 42 ], 4 months [ 50 ], 9 months [ 51 , 52 ], 12 months [ 53 , 54 , 55 ], and one year and 5 months [ 49 ] duration to achieve the desired positive outcome, while bi-weekly [ 47 ] and monthly data reviews and analyses [ 44 , 48 , 56 ], and activities over 3 months [ 57 ] have also resulted in a positive outcome.
Continuous quality improvement models and tools
There have been several models are utilized. The Plan-Do-Study/Check-Act cycle is a stepwise process involving project initiation, situation analysis, root cause identification, solution generation and selection, implementation, result evaluation, standardization, and future planning [ 7 , 36 , 37 , 45 , 47 , 48 , 49 , 50 , 51 , 53 , 56 , 57 , 58 , 59 , 60 , 61 , 62 , 63 , 64 , 65 , 66 , 67 , 68 , 69 , 70 ]. The FOCUS-PDCA cycle enhances the PDCA process by adding steps to find and improve a process (F), organize a knowledgeable team (O), clarify the process (C), understand variations (U), and select improvements (S) [ 55 , 71 , 72 , 73 ]. The FADE cycle involves identifying a problem (Focus), understanding it through data analysis (Analyze), devising solutions (Develop), and implementing the plan (Execute) [ 74 ]. The Logic Framework involves brainstorming to identify improvement areas, conducting root cause analysis to develop a problem tree, logically reasoning to create an objective tree, formulating the framework, and executing improvement projects [ 75 ]. Breakthrough series approach requires CQI teams to meet in quarterly collaborative learning sessions, share learning experiences, and continue discussion by telephone and cross-site visits to strengthen learning and idea exchange [ 47 ]. Another CQI model is the Lean approach, which has been conducted with Kaizen principles [ 52 ], 5 S principles, and the Six Sigma model. The 5 S (Sort, Set/Straighten, Shine, Standardize, Sustain) systematically organises and improves the workplace, focusing on sorting, setting order, shining, standardizing, and sustaining the improvement [ 54 , 76 ]. Kaizen principles guide CQI by advocating for continuous improvement, valuing all ideas, solving problems, focusing on practical, low-cost improvements, using data to drive change, acknowledging process defects, reducing variability and waste, recognizing every interaction as a customer-supplier relationship, empowering workers, responding to all ideas, and maintaining a disciplined workplace [ 77 ]. Lean Six Sigma, a CQI model, applies the DMAIC methodology, which involves defining (D) and measuring the problem (M), analyzing root causes (A), improving by finding solutions (I), and controlling by assessing process stability (C) [ 78 , 79 ]. The 5 C-cyclic model (consultation, collection, consideration, collaboration, and celebration), the first CQI framework for volunteer dental services in Aboriginal communities, ensures quality care based on community needs [ 80 ]. One study used meetings involving activities such as reviewing objectives, assigning roles, discussing the agenda, completing tasks, retaining key outputs, planning future steps, and evaluating the meeting’s effectiveness [ 81 ].
Various tools are involved in the implementation or evaluation of CQI initiatives: checklists [ 53 , 82 ], flowcharts [ 81 , 82 , 83 ], cause-and-effect diagrams (fishbone or Ishikawa diagrams) [ 60 , 62 , 79 , 81 , 82 ], fuzzy Pareto diagram [ 82 ], process maps [ 60 ], time series charts [ 48 ], why-why analysis [ 79 ], affinity diagrams and multivoting [ 81 ], and run chart [ 47 , 48 , 51 , 60 , 84 ], and others mentioned in the table (Table 1 ).
Barriers and facilitators of continuous quality improvement implementation
Implementing CQI initiatives is determined by various barriers and facilitators, which can be thematized into four dimensions. These dimensions are cultural, technical, structural, and strategic dimensions.
Continuous quality improvement initiatives face various cultural, strategic, technical, and structural barriers. Cultural dimension barriers involve resistance to change (e.g., not accepting online technology), lack of quality-focused culture, staff reporting apprehensiveness, and fear of blame or punishment [ 36 , 41 , 85 , 86 ]. The technical dimension barriers of CQI can include various factors that hinder the effective implementation and execution of CQI processes [ 36 , 86 , 87 , 88 , 89 ]. Structural dimension barriers of CQI arise from the organization structure, process, and systems that can impede the effective implementation and sustainability of CQI [ 36 , 85 , 86 , 87 , 88 ]. Strategic dimension barriers are, for example, the inability to select proper CQI goals and failure to integrate CQI into organizational planning and goals [ 36 , 85 , 86 , 87 , 88 , 90 ].
Facilitators are also grouped to cultural, structural, technical, and strategic dimensions to provide solutions to CQI barriers. Cultural challenges were addressed by developing a group culture to CQI and other rewards [ 39 , 41 , 80 , 85 , 86 , 87 , 90 , 91 , 92 ]. Technical facilitators are pivotal to improving technical barriers [ 39 , 42 , 53 , 69 , 86 , 90 , 91 ]. Structural-related facilitators are related to improving communication, infrastructure, and systems [ 86 , 92 , 93 ]. Strategic dimension facilitators include strengthening leadership and improving decision-making skills [ 43 , 53 , 67 , 86 , 87 , 92 , 94 , 95 ] (Table 2 ).
Impact of continuous quality improvement
Continuous quality improvement initiatives can significantly impact the quality of healthcare in a wide range of health areas, focusing on improving structure, the health service delivery process and improving client wellbeing and reducing mortality.
Structure components
These are health leadership, financing, workforce, technology, and equipment and supplies. CQI has improved planning, monitoring and evaluation [ 48 , 53 ], and leadership and planning [ 48 ], indicating improvement in leadership perspectives. Implementing CQI in primary health care (PHC) settings has shown potential for maintaining or reducing operation costs [ 67 ]. Findings from another study indicate that the costs associated with implementing CQI interventions per facility ranged from approximately $2,000 to $10,500 per year, with an average cost of approximately $10 to $60 per admitted client [ 57 ]. However, based on model predictions, the average cost savings after implementing CQI were estimated to be $5430 [ 31 ]. CQI can also be applied to health workforce development [ 32 ]. CQI in the institutional system improved medical education [ 66 , 96 , 97 ], human resources management [ 53 ], motivated staffs [ 76 ], and increased staff health awareness [ 69 ], while concerns raised about CQI impartiality, independence, and public accountability [ 96 ]. Regarding health technology, CQI also improved registration and documentation [ 48 , 53 , 98 ]. Furthermore, the CQI initiatives increased cleanliness [ 54 ] and improved logistics, supplies, and equipment [ 48 , 53 , 68 ].
Process and output components
The process component focuses on the activities and actions involved in delivering healthcare services.
Service delivery
CQI interventions improved service delivery [ 53 , 56 , 99 ], particularly a significant 18% increase in the overall quality of service performance [ 48 ], improved patient counselling, adherence to appropriate procedures, and infection prevention [ 48 , 68 ], and optimised workflow [ 52 ].
Coordination and collaboration
CQI initiatives improved coordination and collaboration through collecting and analysing data, onsite technical support, training, supportive supervision [ 53 ] and facilitating linkages between work processes and a quality control group [ 65 ].
Patient satisfaction
The CQI initiatives increased patient satisfaction and improved quality of life by optimizing care quality management, improving the quality of clinical nursing, reducing nursing defects and enhancing the wellbeing of clients [ 54 , 76 , 100 ], although CQI was not associated with changes in adolescent and young adults’ satisfaction [ 51 ].
CQI initiatives reduced medication error reports from 16 to 6 [ 101 ], and it significantly reduced the administration of inappropriate prophylactic antibiotics [ 44 ], decreased errors in inpatient care [ 52 ], decreased the overall episiotomy rate from 44.5 to 33.3% [ 83 ], reduced the overall incidence of unplanned endotracheal extubation [ 102 ], improving appropriate use of computed tomography angiography [ 103 ], and appropriate diagnosis and treatment selection [ 47 ].
Continuity of care
CQI initiatives effectively improve continuity of care by improving client and physician interaction. For instance, provider continuity levels showed a 64% increase [ 55 ]. Modifying electronic medical record templates, scheduling, staff and parental education, standardization of work processes, and birth to 1-year age-specific incentives in post-natal follow-up care increased continuity of care to 74% in 2018 compared to baseline 13% in 2012 [ 84 ].
The CQI initiative yielded enhanced efficiency in the cardiac catheterization laboratory, as evidenced by improved punctuality in procedure starts and increased efficiency in manual sheath-pulls inside [ 78 ].
Accessibility
CQI initiatives were effective in improving accessibility in terms of increasing service coverage and utilization rate. For instance, screening for cigarettes, nutrition counselling, folate prescription, maternal care, immunization coverage [ 53 , 81 , 104 , 105 ], reducing the percentage of non-attending patients to surgery to 0.9% from the baseline 3.9% [ 43 ], increasing Chlamydia screening rates from 29 to 60% [ 45 ], increasing HIV care continuum coverage [ 51 , 59 , 60 ], increasing in the uptake of postpartum long-acting reversible contraceptive use from 6.9% at the baseline to 25.4% [ 42 ], increasing post-caesarean section prophylaxis from 36 to 89% [ 62 ], a 31% increase of kangaroo care practice [ 50 ], and increased follow-up [ 65 ]. Similarly, the QI intervention increased the quality of antenatal care by 29.3%, correct partograph use by 51.7%, and correct active third-stage labour management, a 19.6% improvement from the baseline, but not significantly associated with improvement in contraceptive service uptake [ 61 ].
Timely access
CQI interventions improved the time care provision [ 52 ], and reduced waiting time [ 62 , 74 , 76 , 106 ]. For instance, the discharge process waiting time in the emergency department decreased from 76 min to 22 min [ 79 ]. It also reduced mean postprocedural length of stay from 2.8 days to 2.0 days [ 31 ].
Acceptability
Acceptability of CQI by healthcare providers was satisfactory. For instance, 88% of the faculty, 64% of the residents, and 82% of the staff believed CQI to be useful in the healthcare clinic [ 107 ].
Outcome components
Morbidity and mortality.
CQI efforts have demonstrated better management outcomes among diabetic patients [ 40 ], patients with oral mucositis [ 71 ], and anaemic patients [ 72 ]. It has also reduced infection rate in post-caesarean Sect. [ 62 ], reduced post-peritoneal dialysis peritonitis [ 49 , 108 ], and prevented pressure ulcers [ 70 ]. It is explained by peritonitis incidence from once every 40.1 patient months at baseline to once every 70.8 patient months after CQI [ 49 ] and a 63% reduction in pressure ulcer prevalence within 2 years from 2008 to 2010 [ 70 ]. Furthermore, CQI initiatives significantly reduced in-hospital deaths [ 31 ] and increased patient survival rates [ 108 ]. Figure 2 displays the overall process of the CQI implementations.
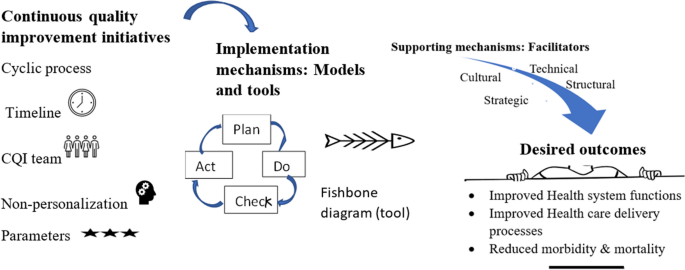
The overall mechanisms of continuous quality improvement implementation
In this review, we examined the fundamental concepts and principles underlying CQI, the factors that either hinder or assist in its successful application and implementation, and the purpose of CQI in enhancing quality of care across various health issues.
Our findings have brought attention to the application and implementation of CQI, emphasizing its underlying concepts and principles, as evident in the existing literature [ 31 , 32 , 33 , 34 , 35 , 36 , 39 , 40 , 43 , 45 , 46 ]. Continuous quality improvement has shared with the principles of continuous improvement, such as a customer-driven focus, effective leadership, active participation of individuals, a process-oriented approach, systematic implementation, emphasis on design improvement and prevention, evidence-based decision-making, and fostering partnership [ 5 ]. Moreover, Deming’s 14 principles laid the foundation for CQI principles [ 109 ]. These principles have been adapted and put into practice in various ways: ten [ 19 ] and five [ 38 ] principles in hospitals, five principles for capacity building [ 38 ], and two principles for medication error prevention [ 41 ]. As a principle, the application of CQI can be process-focused [ 8 , 19 ] or impact-focused [ 38 ]. Impact-focused CQI focuses on achieving specific outcomes or impacts, whereas process-focused CQI prioritizes and improves the underlying processes and systems. These principles complement each other and can be utilized based on the objectives of quality improvement initiatives in healthcare settings. Overall, CQI is an ongoing educational process that requires top management’s involvement, demands coordination across departments, encourages the incorporation of views beyond clinical area, and provides non-judgemental evidence based on objective data [ 110 ].
The current review recognized that it was not easy to implement CQI. It requires reasonable utilization of various models and tools. The application of each tool can be varied based on the studied health problem and the purpose of CQI initiative [ 111 ], varied in context, content, structure, and usability [ 112 ]. Additionally, overcoming the cultural, technical, structural, and strategic-related barriers. These barriers have emerged from clinical staff, managers, and health systems perspectives. Of the cultural obstacles, staff non-involvement, resistance to change, and reluctance to report error were staff-related. In contrast, others, such as the absence of celebration for success and hierarchical and rational culture, may require staff and manager involvement. Staff members may exhibit reluctance in reporting errors due to various cultural factors, including lack of trust, hierarchical structures, fear of retribution, and a blame-oriented culture. These challenges pose obstacles to implementing standardized CQI practices, as observed, for instance, in community pharmacy settings [ 85 ]. The hierarchical culture, characterized by clearly defined levels of power, authority, and decision-making, posed challenges to implementing CQI initiatives in public health [ 41 , 86 ]. Although rational culture, a type of organizational culture, emphasizes logical thinking and rational decision-making, it can also create challenges for CQI implementation [ 41 , 86 ] because hierarchical and rational cultures, which emphasize bureaucratic norms and narrow definitions of achievement, were found to act as barriers to the implementation of CQI [ 86 ]. These could be solved by developing a shared mindset and collective commitment, establishing a shared purpose, developing group norms, and cultivating psychological preparedness among staff, managers, and clients to implement and sustain CQI initiatives. Furthermore, reversing cultural-related barriers necessitates cultural-related solutions: development of a culture and group culture to CQI [ 41 , 86 ], positive comprehensive perception [ 91 ], commitment [ 85 ], involving patients, families, leaders, and staff [ 39 , 92 ], collaborating for a common goal [ 80 , 86 ], effective teamwork [ 86 , 87 ], and rewarding and celebrating successes [ 80 , 90 ].
The technical dimension barriers of CQI can include inadequate capitalization of a project and insufficient support for CQI facilitators and data entry managers [ 36 ], immature electronic medical records or poor information systems [ 36 , 86 ], and the lack of training and skills [ 86 , 87 , 88 ]. These challenges may cause the CQI team to rely on outdated information and technologies. The presence of barriers on the technical dimension may challenge the solid foundation of CQI expertise among staff, the ability to recognize opportunities for improvement, a comprehensive understanding of how services are produced and delivered, and routine use of expertise in daily work. Addressing these technical barriers requires knowledge creation activities (training, seminar, and education) [ 39 , 42 , 53 , 69 , 86 , 90 , 91 ], availability of quality data [ 86 ], reliable information [ 92 ], and a manual-online hybrid reporting system [ 85 ].
Structural dimension barriers of CQI include inadequate communication channels and lack of standardized process, specifically weak physician-to-physician synergies [ 36 ], lack of mechanisms for disseminating knowledge and limited use of communication mechanisms [ 86 ]. Lack of communication mechanism endangers sharing ideas and feedback among CQI teams, leading to misunderstandings, limited participation and misinterpretations, and a lack of learning [ 113 ]. Knowledge translation facilitates the co-production of research, subsequent diffusion of knowledge, and the developing stakeholder’s capacity and skills [ 114 ]. Thus, the absence of a knowledge translation mechanism may cause missed opportunities for learning, inefficient problem-solving, and limited creativity. To overcome these challenges, organizations should establish effective communication and information systems [ 86 , 93 ] and learning systems [ 92 ]. Though CQI and knowledge translation have interacted with each other, it is essential to recognize that they are distinct. CQI focuses on process improvement within health care systems, aiming to optimize existing processes, reduce errors, and enhance efficiency.
In contrast, knowledge translation bridges the gap between research evidence and clinical practice, translating research findings into actionable knowledge for practitioners. While both CQI and knowledge translation aim to enhance health care quality and patient outcomes, they employ different strategies: CQI utilizes tools like Plan-Do-Study-Act cycles and statistical process control, while knowledge translation involves knowledge synthesis and dissemination. Additionally, knowledge translation can also serve as a strategy to enhance CQI. Both concepts share the same principle: continuous improvement is essential for both. Therefore, effective strategies on the structural dimension may build efficient and effective steering councils, information systems, and structures to diffuse learning throughout the organization.
Strategic factors, such as goals, planning, funds, and resources, determine the overall purpose of CQI initiatives. Specific barriers were improper goals and poor planning [ 36 , 86 , 88 ], fragmentation of quality assurance policies [ 87 ], inadequate reinforcement to staff [ 36 , 90 ], time constraints [ 85 , 86 ], resource inadequacy [ 86 ], and work overload [ 86 ]. These barriers can be addressed through strengthening leadership [ 86 , 87 ], CQI-based mentoring [ 94 ], periodic monitoring, supportive supervision and coaching [ 43 , 53 , 87 , 92 , 95 ], participation, empowerment, and accountability [ 67 ], involving all stakeholders in decision-making [ 86 , 87 ], a provider-payer partnership [ 64 ], and compensating staff for after-hours meetings on CQI [ 85 ]. The strategic dimension, characterized by a strategic plan and integrated CQI efforts, is devoted to processes that are central to achieving strategic priorities. Roles and responsibilities are defined in terms of integrated strategic and quality-related goals [ 115 ].
The utmost goal of CQI has been to improve the quality of care, which is usually revealed by structure, process, and outcome. After resolving challenges and effectively using tools and running models, the goal of CQI reflects the ultimate reason and purpose of its implementation. First, effectively implemented CQI initiatives can improve leadership, health financing, health workforce development, health information technology, and availability of supplies as the building blocks of a health system [ 31 , 48 , 53 , 68 , 98 ]. Second, effectively implemented CQI initiatives improved care delivery process (counselling, adherence with standards, coordination, collaboration, and linkages) [ 48 , 53 , 65 , 68 ]. Third, the CQI can improve outputs of healthcare delivery, such as satisfaction, accessibility (timely access, utilization), continuity of care, safety, efficiency, and acceptability [ 52 , 54 , 55 , 76 , 78 ]. Finally, the effectiveness of the CQI initiatives has been tested in enhancing responses related to key aspects of the HIV response, maternal and child health, non-communicable disease control, and others (e.g., surgery and peritonitis). However, it is worth noting that CQI initiative has not always been effective. For instance, CQI using a two- to nine-times audit cycle model through systems assessment tools did not bring significant change to increase syphilis testing performance [ 116 ]. This study was conducted within the context of Aboriginal and Torres Strait Islander people’s primary health care settings. Notably, ‘the clinics may not have consistently prioritized syphilis testing performance in their improvement strategies, as facilitated by the CQI program’ [ 116 ]. Additionally, by applying CQI-based mentoring, uptake of facility-based interventions was not significantly improved, though it was effective in increasing community health worker visits during pregnancy and the postnatal period, knowledge about maternal and child health and exclusive breastfeeding practice, and HIV disclosure status [ 117 ]. The study conducted in South Africa revealed no significant association between the coverage of facility-based interventions and Continuous Quality Improvement (CQI) implementation. This lack of association was attributed to the already high antenatal and postnatal attendance rates in both control and intervention groups at baseline, leaving little room for improvement. Additionally, the coverage of HIV interventions remained consistently high throughout the study period [ 117 ].
Regarding health care and policy implications, CQI has played a vital role in advancing PHC and fostering the realization of UHC goals worldwide. The indicators found in Donabedian’s framework that are positively influenced by CQI efforts are comparable to those included in the PHC performance initiative’s conceptual framework [ 29 , 118 , 119 ]. It is clearly explained that PHC serves as the roadmap to realizing the vision of UHC [ 120 , 121 ]. Given these circumstances, implementing CQI can contribute to the achievement of PHC principles and the objectives of UHC. For instance, by implementing CQI methods, countries have enhanced the accessibility, affordability, and quality of PHC services, leading to better health outcomes for their populations. CQI has facilitated identifying and resolving healthcare gaps and inefficiencies, enabling countries to optimize resource allocation and deliver more effective and patient-centered care. However, it is crucial to recognize that the successful implementation of Continuous Quality Improvement (CQI) necessitates optimizing the duration of each cycle, understanding challenges and barriers that extend beyond the health system and settings, and acknowledging that its effectiveness may be compromised if these challenges are not adequately addressed.
Despite abundant literature, there are still gaps regarding the relationship between CQI and other dimensions within the healthcare system. No studies have examined the impact of CQI initiatives on catastrophic health expenditure, effective service coverage, patient-centredness, comprehensiveness, equity, health security, and responsiveness.
Limitations
In conducting this review, it has some limitations to consider. Firstly, only articles published in English were included, which may introduce the exclusion of relevant non-English articles. Additionally, as this review follows a scoping methodology, the focus is on synthesising available evidence rather than critically evaluating or scoring the quality of the included articles.
Continuous quality improvement is investigated as a continuous and ongoing intervention, where the implementation time can vary across different cycles. The CQI team and implementation timelines were critical elements of CQI in different models. Among the commonly used approaches, the PDSA or PDCA is frequently employed. In most CQI models, a wide range of tools, nineteen tools, are commonly utilized to support the improvement process. Cultural, technical, structural, and strategic barriers and facilitators are significant in implementing CQI initiatives. Implementing the CQI initiative aims to improve health system blocks, enhance health service delivery process and output, and ultimately prevent morbidity and reduce mortality. For future researchers, considering that CQI is context-dependent approach, conducting scale-up implementation research about catastrophic health expenditure, effective service coverage, patient-centredness, comprehensiveness, equity, health security, and responsiveness across various settings and health issues would be valuable.
Availability of data and materials
The data used and/or analyzed during the current study are available in this manuscript and/or the supplementary file.
Shewhart WA, Deming WE. Memoriam: Walter A. Shewhart, 1891–1967. Am Stat. 1967;21(2):39–40.
Article Google Scholar
Shewhart WA. Statistical method from the viewpoint of quality control. New York: Dover; 1986. ISBN 978-0486652320. OCLC 13822053. Reprint. Originally published: Washington, DC: Graduate School of the Department of Agriculture, 1939.
Moen R, editor Foundation and History of the PDSA Cycle. Asian network for quality conference Tokyo. https://www.deming.org/sites/default/files/pdf/2015/PDSA_History_Ron_MoenPdf . 2009.
Kuperman G, James B, Jacobsen J, Gardner RM. Continuous quality improvement applied to medical care: experiences at LDS hospital. Med Decis Making. 1991;11(4suppl):S60–65.
Article CAS PubMed Google Scholar
Singh J, Singh H. Continuous improvement philosophy–literature review and directions. Benchmarking: An International Journal. 2015;22(1):75–119.
Goldstone J. Presidential address: Sony, Porsche, and vascular surgery in the 21st century. J Vasc Surg. 1997;25(2):201–10.
Radawski D. Continuous quality improvement: origins, concepts, problems, and applications. J Physician Assistant Educ. 1999;10(1):12–6.
Shortell SM, O’Brien JL, Carman JM, Foster RW, Hughes E, Boerstler H, et al. Assessing the impact of continuous quality improvement/total quality management: concept versus implementation. Health Serv Res. 1995;30(2):377.
CAS PubMed PubMed Central Google Scholar
Lohr K. Quality of health care: an introduction to critical definitions, concepts, principles, and practicalities. Striving for quality in health care. 1991.
Berwick DM. The clinical process and the quality process. Qual Manage Healthc. 1992;1(1):1–8.
Article CAS Google Scholar
Gift B. On the road to TQM. Food Manage. 1992;27(4):88–9.
CAS PubMed Google Scholar
Greiner A, Knebel E. The core competencies needed for health care professionals. health professions education: A bridge to quality. 2003:45–73.
McCalman J, Bailie R, Bainbridge R, McPhail-Bell K, Percival N, Askew D et al. Continuous quality improvement and comprehensive primary health care: a systems framework to improve service quality and health outcomes. Front Public Health. 2018:6 (76):1–6.
Sheingold BH, Hahn JA. The history of healthcare quality: the first 100 years 1860–1960. Int J Afr Nurs Sci. 2014;1:18–22.
Google Scholar
Donabedian A. Evaluating the quality of medical care. Milbank Q. 1966;44(3):166–206.
Institute of Medicine (US) Committee on Quality of Health Care in America. Crossing the Quality Chasm: A New Health System for the 21st Century. Washington (DC): National Academies Press (US). 2001. 2, Improving the 21st-century Health Care System. Available from: https://www.ncbi.nlm.nih.gov/books/NBK222265/ .
Rubinstein A, Barani M, Lopez AS. Quality first for effective universal health coverage in low-income and middle-income countries. Lancet Global Health. 2018;6(11):e1142–1143.
Article PubMed Google Scholar
Agency for Healthcare Reserach and Quality. Quality Improvement and monitoring at your fingertips USA,: Agency for Healthcare Reserach and Quality. 2022. Available from: https://qualityindicators.ahrq.gov/ .
Anderson CA, Cassidy B, Rivenburgh P. Implementing continuous quality improvement (CQI) in hospitals: lessons learned from the International Quality Study. Qual Assur Health Care. 1991;3(3):141–6.
Gardner K, Mazza D. Quality in general practice - definitions and frameworks. Aust Fam Physician. 2012;41(3):151–4.
PubMed Google Scholar
Loper AC, Jensen TM, Farley AB, Morgan JD, Metz AJ. A systematic review of approaches for continuous quality improvement capacity-building. J Public Health Manage Pract. 2022;28(2):E354.
Hill JE, Stephani A-M, Sapple P, Clegg AJ. The effectiveness of continuous quality improvement for developing professional practice and improving health care outcomes: a systematic review. Implement Sci. 2020;15(1):1–14.
Candas B, Jobin G, Dubé C, Tousignant M, Abdeljelil AB, Grenier S, et al. Barriers and facilitators to implementing continuous quality improvement programs in colonoscopy services: a mixed methods systematic review. Endoscopy Int Open. 2016;4(02):E118–133.
Peters MD, Marnie C, Colquhoun H, Garritty CM, Hempel S, Horsley T, et al. Scoping reviews: reinforcing and advancing the methodology and application. Syst Reviews. 2021;10(1):1–6.
Arksey H, O’Malley L. Scoping studies: towards a methodological framework. Int J Soc Res Methodol. 2005;8(1):19–32.
Tricco AC, Lillie E, Zarin W, O’Brien KK, Colquhoun H, Levac D, et al. PRISMA extension for scoping reviews (PRISMA-ScR): checklist and explanation. Ann Intern Med. 2018;169(7):467–73.
McGowan J, Straus S, Moher D, Langlois EV, O’Brien KK, Horsley T, et al. Reporting scoping reviews—PRISMA ScR extension. J Clin Epidemiol. 2020;123:177–9.
Donabedian A. Explorations in quality assessment and monitoring: the definition of quality and approaches to its assessment. Health Administration Press, Ann Arbor. 1980;1.
World Health Organization. Operational framework for primary health care: transforming vision into action. Geneva: World Health Organization and the United Nations Children’s Fund (UNICEF); 2020 [updated 14 December 2020; cited 2023 Nov Oct 17]. Available from: https://www.who.int/publications/i/item/9789240017832 .
The Joanna Briggs Institute. The Joanna Briggs Institute Reviewers’ Manual :2014 edition. Australia: The Joanna Briggs Institute. 2014:88–91.
Rihal CS, Kamath CC, Holmes DR Jr, Reller MK, Anderson SS, McMurtry EK, et al. Economic and clinical outcomes of a physician-led continuous quality improvement intervention in the delivery of percutaneous coronary intervention. Am J Manag Care. 2006;12(8):445–52.
Ade-Oshifogun JB, Dufelmeier T. Prevention and Management of Do not return notices: a quality improvement process for Supplemental staffing nursing agencies. Nurs Forum. 2012;47(2):106–12.
Rubenstein L, Khodyakov D, Hempel S, Danz M, Salem-Schatz S, Foy R, et al. How can we recognize continuous quality improvement? Int J Qual Health Care. 2014;26(1):6–15.
O’Neill SM, Hempel S, Lim YW, Danz MS, Foy R, Suttorp MJ, et al. Identifying continuous quality improvement publications: what makes an improvement intervention ‘CQI’? BMJ Qual Saf. 2011;20(12):1011–9.
Article PubMed PubMed Central Google Scholar
Sibthorpe B, Gardner K, McAullay D. Furthering the quality agenda in Aboriginal community controlled health services: understanding the relationship between accreditation, continuous quality improvement and national key performance indicator reporting. Aust J Prim Health. 2016;22(4):270–5.
Bennett CL, Crane JM. Quality improvement efforts in oncology: are we ready to begin? Cancer Invest. 2001;19(1):86–95.
VanValkenburgh DA. Implementing continuous quality improvement at the facility level. Adv Ren Replace Ther. 2001;8(2):104–13.
Loper AC, Jensen TM, Farley AB, Morgan JD, Metz AJ. A systematic review of approaches for continuous quality improvement capacity-building. J Public Health Manage Practice. 2022;28(2):E354–361.
Ryan M. Achieving and sustaining quality in healthcare. Front Health Serv Manag. 2004;20(3):3–11.
Nicolucci A, Allotta G, Allegra G, Cordaro G, D’Agati F, Di Benedetto A, et al. Five-year impact of a continuous quality improvement effort implemented by a network of diabetes outpatient clinics. Diabetes Care. 2008;31(1):57–62.
Wakefield BJ, Blegen MA, Uden-Holman T, Vaughn T, Chrischilles E, Wakefield DS. Organizational culture, continuous quality improvement, and medication administration error reporting. Am J Med Qual. 2001;16(4):128–34.
Sori DA, Debelew GT, Degefa LS, Asefa Z. Continuous quality improvement strategy for increasing immediate postpartum long-acting reversible contraceptive use at Jimma University Medical Center, Jimma, Ethiopia. BMJ Open Qual. 2023;12(1):e002051.
Roche B, Robin C, Deleaval PJ, Marti MC. Continuous quality improvement in ambulatory surgery: the non-attending patient. Ambul Surg. 1998;6(2):97–100.
O’Connor JB, Sondhi SS, Mullen KD, McCullough AJ. A continuous quality improvement initiative reduces inappropriate prescribing of prophylactic antibiotics for endoscopic procedures. Am J Gastroenterol. 1999;94(8):2115–21.
Ursu A, Greenberg G, McKee M. Continuous quality improvement methodology: a case study on multidisciplinary collaboration to improve chlamydia screening. Fam Med Community Health. 2019;7(2):e000085.
Quick B, Nordstrom S, Johnson K. Using continuous quality improvement to implement evidence-based medicine. Lippincotts Case Manag. 2006;11(6):305–15 ( quiz 16 – 7 ).
Oyeledun B, Phillips A, Oronsaye F, Alo OD, Shaffer N, Osibo B, et al. The effect of a continuous quality improvement intervention on retention-in-care at 6 months postpartum in a PMTCT Program in Northern Nigeria: results of a cluster randomized controlled study. J Acquir Immune Defic Syndr. 2017;75(Suppl 2):S156–164.
Nyengerai T, Phohole M, Iqaba N, Kinge CW, Gori E, Moyo K, et al. Quality of service and continuous quality improvement in voluntary medical male circumcision programme across four provinces in South Africa: longitudinal and cross-sectional programme data. PLoS ONE. 2021;16(8):e0254850.
Article CAS PubMed PubMed Central Google Scholar
Wang J, Zhang H, Liu J, Zhang K, Yi B, Liu Y, et al. Implementation of a continuous quality improvement program reduces the occurrence of peritonitis in PD. Ren Fail. 2014;36(7):1029–32.
Stikes R, Barbier D. Applying the plan-do-study-act model to increase the use of kangaroo care. J Nurs Manag. 2013;21(1):70–8.
Wagner AD, Mugo C, Bluemer-Miroite S, Mutiti PM, Wamalwa DC, Bukusi D, et al. Continuous quality improvement intervention for adolescent and young adult HIV testing services in Kenya improves HIV knowledge. AIDS. 2017;31(Suppl 3):S243–252.
Le RD, Melanson SE, Santos KS, Paredes JD, Baum JM, Goonan EM, et al. Using lean principles to optimise inpatient phlebotomy services. J Clin Pathol. 2014;67(8):724–30.
Manyazewal T, Mekonnen A, Demelew T, Mengestu S, Abdu Y, Mammo D, et al. Improving immunization capacity in Ethiopia through continuous quality improvement interventions: a prospective quasi-experimental study. Infect Dis Poverty. 2018;7:7.
Kamiya Y, Ishijma H, Hagiwara A, Takahashi S, Ngonyani HAM, Samky E. Evaluating the impact of continuous quality improvement methods at hospitals in Tanzania: a cluster-randomized trial. Int J Qual Health Care. 2017;29(1):32–9.
Kibbe DC, Bentz E, McLaughlin CP. Continuous quality improvement for continuity of care. J Fam Pract. 1993;36(3):304–8.
Adrawa N, Ongiro S, Lotee K, Seret J, Adeke M, Izudi J. Use of a context-specific package to increase sputum smear monitoring among people with pulmonary tuberculosis in Uganda: a quality improvement study. BMJ Open Qual. 2023;12(3):1–6.
Hunt P, Hunter SB, Levan D. Continuous quality improvement in substance abuse treatment facilities: how much does it cost? J Subst Abuse Treat. 2017;77:133–40.
Azadeh A, Ameli M, Alisoltani N, Motevali Haghighi S. A unique fuzzy multi-control approach for continuous quality improvement in a radio therapy department. Qual Quantity. 2016;50(6):2469–93.
Memiah P, Tlale J, Shimabale M, Nzyoka S, Komba P, Sebeza J, et al. Continuous quality improvement (CQI) institutionalization to reach 95:95:95 HIV targets: a multicountry experience from the Global South. BMC Health Serv Res. 2021;21(1):711.
Yapa HM, De Neve JW, Chetty T, Herbst C, Post FA, Jiamsakul A, et al. The impact of continuous quality improvement on coverage of antenatal HIV care tests in rural South Africa: results of a stepped-wedge cluster-randomised controlled implementation trial. PLoS Med. 2020;17(10):e1003150.
Dadi TL, Abebo TA, Yeshitla A, Abera Y, Tadesse D, Tsegaye S, et al. Impact of quality improvement interventions on facility readiness, quality and uptake of maternal and child health services in developing regions of Ethiopia: a secondary analysis of programme data. BMJ Open Qual. 2023;12(4):e002140.
Weinberg M, Fuentes JM, Ruiz AI, Lozano FW, Angel E, Gaitan H, et al. Reducing infections among women undergoing cesarean section in Colombia by means of continuous quality improvement methods. Arch Intern Med. 2001;161(19):2357–65.
Andreoni V, Bilak Y, Bukumira M, Halfer D, Lynch-Stapleton P, Perez C. Project management: putting continuous quality improvement theory into practice. J Nurs Care Qual. 1995;9(3):29–37.
Balfour ME, Zinn TE, Cason K, Fox J, Morales M, Berdeja C, et al. Provider-payer partnerships as an engine for continuous quality improvement. Psychiatric Serv. 2018;69(6):623–5.
Agurto I, Sandoval J, De La Rosa M, Guardado ME. Improving cervical cancer prevention in a developing country. Int J Qual Health Care. 2006;18(2):81–6.
Anderson CI, Basson MD, Ali M, Davis AT, Osmer RL, McLeod MK, et al. Comprehensive multicenter graduate surgical education initiative incorporating entrustable professional activities, continuous quality improvement cycles, and a web-based platform to enhance teaching and learning. J Am Coll Surg. 2018;227(1):64–76.
Benjamin S, Seaman M. Applying continuous quality improvement and human performance technology to primary health care in Bahrain. Health Care Superv. 1998;17(1):62–71.
Byabagambi J, Marks P, Megere H, Karamagi E, Byakika S, Opio A, et al. Improving the quality of voluntary medical male circumcision through use of the continuous quality improvement approach: a pilot in 30 PEPFAR-Supported sites in Uganda. PLoS ONE. 2015;10(7):e0133369.
Hogg S, Roe Y, Mills R. Implementing evidence-based continuous quality improvement strategies in an urban Aboriginal Community Controlled Health Service in South East Queensland: a best practice implementation pilot. JBI Database Syst Rev Implement Rep. 2017;15(1):178–87.
Hopper MB, Morgan S. Continuous quality improvement initiative for pressure ulcer prevention. J Wound Ostomy Cont Nurs. 2014;41(2):178–80.
Ji J, Jiang DD, Xu Z, Yang YQ, Qian KY, Zhang MX. Continuous quality improvement of nutrition management during radiotherapy in patients with nasopharyngeal carcinoma. Nurs Open. 2021;8(6):3261–70.
Chen M, Deng JH, Zhou FD, Wang M, Wang HY. Improving the management of anemia in hemodialysis patients by implementing the continuous quality improvement program. Blood Purif. 2006;24(3):282–6.
Reeves S, Matney K, Crane V. Continuous quality improvement as an ideal in hospital practice. Health Care Superv. 1995;13(4):1–12.
Barton AJ, Danek G, Johns P, Coons M. Improving patient outcomes through CQI: vascular access planning. J Nurs Care Qual. 1998;13(2):77–85.
Buttigieg SC, Gauci D, Dey P. Continuous quality improvement in a Maltese hospital using logical framework analysis. J Health Organ Manag. 2016;30(7):1026–46.
Take N, Byakika S, Tasei H, Yoshikawa T. The effect of 5S-continuous quality improvement-total quality management approach on staff motivation, patients’ waiting time and patient satisfaction with services at hospitals in Uganda. J Public Health Afr. 2015;6(1):486.
PubMed PubMed Central Google Scholar
Jacobson GH, McCoin NS, Lescallette R, Russ S, Slovis CM. Kaizen: a method of process improvement in the emergency department. Acad Emerg Med. 2009;16(12):1341–9.
Agarwal S, Gallo J, Parashar A, Agarwal K, Ellis S, Khot U, et al. Impact of lean six sigma process improvement methodology on cardiac catheterization laboratory efficiency. Catheter Cardiovasc Interv. 2015;85:S119.
Rahul G, Samanta AK, Varaprasad G A Lean Six Sigma approach to reduce overcrowding of patients and improving the discharge process in a super-specialty hospital. In 2020 International Conference on System, Computation, Automation and Networking (ICSCAN) 2020 July 3 (pp. 1-6). IEEE
Patel J, Nattabi B, Long R, Durey A, Naoum S, Kruger E, et al. The 5 C model: A proposed continuous quality improvement framework for volunteer dental services in remote Australian Aboriginal communities. Community Dent Oral Epidemiol. 2023;51(6):1150–8.
Van Acker B, McIntosh G, Gudes M. Continuous quality improvement techniques enhance HMO members’ immunization rates. J Healthc Qual. 1998;20(2):36–41.
Horine PD, Pohjala ED, Luecke RW. Healthcare financial managers and CQI. Healthc Financ Manage. 1993;47(9):34.
Reynolds JL. Reducing the frequency of episiotomies through a continuous quality improvement program. CMAJ. 1995;153(3):275–82.
Bunik M, Galloway K, Maughlin M, Hyman D. First five quality improvement program increases adherence and continuity with well-child care. Pediatr Qual Saf. 2021;6(6):e484.
Boyle TA, MacKinnon NJ, Mahaffey T, Duggan K, Dow N. Challenges of standardized continuous quality improvement programs in community pharmacies: the case of SafetyNET-Rx. Res Social Adm Pharm. 2012;8(6):499–508.
Price A, Schwartz R, Cohen J, Manson H, Scott F. Assessing continuous quality improvement in public health: adapting lessons from healthcare. Healthc Policy. 2017;12(3):34–49.
Gage AD, Gotsadze T, Seid E, Mutasa R, Friedman J. The influence of continuous quality improvement on healthcare quality: a mixed-methods study from Zimbabwe. Soc Sci Med. 2022;298:114831.
Chan YC, Ho SJ. Continuous quality improvement: a survey of American and Canadian healthcare executives. Hosp Health Serv Adm. 1997;42(4):525–44.
Balas EA, Puryear J, Mitchell JA, Barter B. How to structure clinical practice guidelines for continuous quality improvement? J Med Syst. 1994;18(5):289–97.
ElChamaa R, Seely AJE, Jeong D, Kitto S. Barriers and facilitators to the implementation and adoption of a continuous quality improvement program in surgery: a case study. J Contin Educ Health Prof. 2022;42(4):227–35.
Candas B, Jobin G, Dubé C, Tousignant M, Abdeljelil A, Grenier S, et al. Barriers and facilitators to implementing continuous quality improvement programs in colonoscopy services: a mixed methods systematic review. Endoscopy Int Open. 2016;4(2):E118–133.
Brandrud AS, Schreiner A, Hjortdahl P, Helljesen GS, Nyen B, Nelson EC. Three success factors for continual improvement in healthcare: an analysis of the reports of improvement team members. BMJ Qual Saf. 2011;20(3):251–9.
Lee S, Choi KS, Kang HY, Cho W, Chae YM. Assessing the factors influencing continuous quality improvement implementation: experience in Korean hospitals. Int J Qual Health Care. 2002;14(5):383–91.
Horwood C, Butler L, Barker P, Phakathi S, Haskins L, Grant M, et al. A continuous quality improvement intervention to improve the effectiveness of community health workers providing care to mothers and children: a cluster randomised controlled trial in South Africa. Hum Resour Health. 2017;15(1):39.
Hyrkäs K, Lehti K. Continuous quality improvement through team supervision supported by continuous self-monitoring of work and systematic patient feedback. J Nurs Manag. 2003;11(3):177–88.
Akdemir N, Peterson LN, Campbell CM, Scheele F. Evaluation of continuous quality improvement in accreditation for medical education. BMC Med Educ. 2020;20(Suppl 1):308.
Barzansky B, Hunt D, Moineau G, Ahn D, Lai CW, Humphrey H, et al. Continuous quality improvement in an accreditation system for undergraduate medical education: benefits and challenges. Med Teach. 2015;37(11):1032–8.
Gaylis F, Nasseri R, Salmasi A, Anderson C, Mohedin S, Prime R, et al. Implementing continuous quality improvement in an integrated community urology practice: lessons learned. Urology. 2021;153:139–46.
Gaga S, Mqoqi N, Chimatira R, Moko S, Igumbor JO. Continuous quality improvement in HIV and TB services at selected healthcare facilities in South Africa. South Afr J HIV Med. 2021;22(1):1202.
Wang F, Yao D. Application effect of continuous quality improvement measures on patient satisfaction and quality of life in gynecological nursing. Am J Transl Res. 2021;13(6):6391–8.
Lee SB, Lee LL, Yeung RS, Chan J. A continuous quality improvement project to reduce medication error in the emergency department. World J Emerg Med. 2013;4(3):179–82.
Chiang AA, Lee KC, Lee JC, Wei CH. Effectiveness of a continuous quality improvement program aiming to reduce unplanned extubation: a prospective study. Intensive Care Med. 1996;22(11):1269–71.
Chinnaiyan K, Al-Mallah M, Goraya T, Patel S, Kazerooni E, Poopat C, et al. Impact of a continuous quality improvement initiative on appropriate use of coronary CT angiography: results from a multicenter, statewide registry, the advanced cardiovascular imaging consortium (ACIC). J Cardiovasc Comput Tomogr. 2011;5(4):S29–30.
Gibson-Helm M, Rumbold A, Teede H, Ranasinha S, Bailie R, Boyle J. A continuous quality improvement initiative: improving the provision of pregnancy care for Aboriginal and Torres Strait Islander women. BJOG: Int J Obstet Gynecol. 2015;122:400–1.
Bennett IM, Coco A, Anderson J, Horst M, Gambler AS, Barr WB, et al. Improving maternal care with a continuous quality improvement strategy: a report from the interventions to minimize preterm and low birth weight infants through continuous improvement techniques (IMPLICIT) network. J Am Board Fam Med. 2009;22(4):380–6.
Krall SP, Iv CLR, Donahue L. Effect of continuous quality improvement methods on reducing triage to thrombolytic interval for Acute myocardial infarction. Acad Emerg Med. 1995;2(7):603–9.
Swanson TK, Eilers GM. Physician and staff acceptance of continuous quality improvement. Fam Med. 1994;26(9):583–6.
Yu Y, Zhou Y, Wang H, Zhou T, Li Q, Li T, et al. Impact of continuous quality improvement initiatives on clinical outcomes in peritoneal dialysis. Perit Dial Int. 2014;34(Suppl 2):S43–48.
Schiff GD, Goldfield NI. Deming meets Braverman: toward a progressive analysis of the continuous quality improvement paradigm. Int J Health Serv. 1994;24(4):655–73.
American Hospital Association Division of Quality Resources Chicago, IL: The role of hospital leadership in the continuous improvement of patient care quality. American Hospital Association. J Healthc Qual. 1992;14(5):8–14,22.
Scriven M. The Logic and Methodology of checklists [dissertation]. Western Michigan University; 2000.
Hales B, Terblanche M, Fowler R, Sibbald W. Development of medical checklists for improved quality of patient care. Int J Qual Health Care. 2008;20(1):22–30.
Vermeir P, Vandijck D, Degroote S, Peleman R, Verhaeghe R, Mortier E, et al. Communication in healthcare: a narrative review of the literature and practical recommendations. Int J Clin Pract. 2015;69(11):1257–67.
Eljiz K, Greenfield D, Hogden A, Taylor R, Siddiqui N, Agaliotis M, et al. Improving knowledge translation for increased engagement and impact in healthcare. BMJ open Qual. 2020;9(3):e000983.
O’Brien JL, Shortell SM, Hughes EF, Foster RW, Carman JM, Boerstler H, et al. An integrative model for organization-wide quality improvement: lessons from the field. Qual Manage Healthc. 1995;3(4):19–30.
Adily A, Girgis S, D’Este C, Matthews V, Ward JE. Syphilis testing performance in Aboriginal primary health care: exploring impact of continuous quality improvement over time. Aust J Prim Health. 2020;26(2):178–83.
Horwood C, Butler L, Barker P, Phakathi S, Haskins L, Grant M, et al. A continuous quality improvement intervention to improve the effectiveness of community health workers providing care to mothers and children: a cluster randomised controlled trial in South Africa. Hum Resour Health. 2017;15:1–11.
Veillard J, Cowling K, Bitton A, Ratcliffe H, Kimball M, Barkley S, et al. Better measurement for performance improvement in low- and middle-income countries: the primary Health Care Performance Initiative (PHCPI) experience of conceptual framework development and indicator selection. Milbank Q. 2017;95(4):836–83.
Barbazza E, Kringos D, Kruse I, Klazinga NS, Tello JE. Creating performance intelligence for primary health care strengthening in Europe. BMC Health Serv Res. 2019;19(1):1006.
Assefa Y, Hill PS, Gilks CF, Admassu M, Tesfaye D, Van Damme W. Primary health care contributions to universal health coverage. Ethiopia Bull World Health Organ. 2020;98(12):894.
Van Weel C, Kidd MR. Why strengthening primary health care is essential to achieving universal health coverage. CMAJ. 2018;190(15):E463–466.
Download references
Acknowledgements
Not applicable.
The authors received no fund.
Author information
Authors and affiliations.
School of Public Health, The University of Queensland, Brisbane, Australia
Aklilu Endalamaw, Resham B Khatri, Tesfaye Setegn Mengistu, Daniel Erku & Yibeltal Assefa
College of Medicine and Health Sciences, Bahir Dar University, Bahir Dar, Ethiopia
Aklilu Endalamaw & Tesfaye Setegn Mengistu
Health Social Science and Development Research Institute, Kathmandu, Nepal
Resham B Khatri
Centre for Applied Health Economics, School of Medicine, Grifth University, Brisbane, Australia
Daniel Erku
Menzies Health Institute Queensland, Grifth University, Brisbane, Australia
International Institute for Primary Health Care in Ethiopia, Addis Ababa, Ethiopia
Eskinder Wolka & Anteneh Zewdie
You can also search for this author in PubMed Google Scholar
Contributions
AE conceptualized the study, developed the first draft of the manuscript, and managing feedbacks from co-authors. YA conceptualized the study, provided feedback, and supervised the whole processes. RBK provided feedback throughout. TSM provided feedback throughout. DE provided feedback throughout. EW provided feedback throughout. AZ provided feedback throughout. All authors read and approved the final manuscript.
Corresponding author
Correspondence to Aklilu Endalamaw .
Ethics declarations
Ethics approval and consent to participate.
Not applicable because this research is based on publicly available articles.
Consent for publication
Competing interests.
The authors declare that they have no competing interests.
Additional information
Publisher’s note.
Springer Nature remains neutral with regard to jurisdictional claims in published maps and institutional affiliations.
Supplementary Information
Supplementary material 1., supplementary material 2., rights and permissions.
Open Access This article is licensed under a Creative Commons Attribution 4.0 International License, which permits use, sharing, adaptation, distribution and reproduction in any medium or format, as long as you give appropriate credit to the original author(s) and the source, provide a link to the Creative Commons licence, and indicate if changes were made. The images or other third party material in this article are included in the article's Creative Commons licence, unless indicated otherwise in a credit line to the material. If material is not included in the article's Creative Commons licence and your intended use is not permitted by statutory regulation or exceeds the permitted use, you will need to obtain permission directly from the copyright holder. To view a copy of this licence, visit http://creativecommons.org/licenses/by/4.0/ . The Creative Commons Public Domain Dedication waiver ( http://creativecommons.org/publicdomain/zero/1.0/ ) applies to the data made available in this article, unless otherwise stated in a credit line to the data.
Reprints and permissions
About this article
Cite this article.
Endalamaw, A., Khatri, R.B., Mengistu, T.S. et al. A scoping review of continuous quality improvement in healthcare system: conceptualization, models and tools, barriers and facilitators, and impact. BMC Health Serv Res 24 , 487 (2024). https://doi.org/10.1186/s12913-024-10828-0
Download citation
Received : 27 December 2023
Accepted : 05 March 2024
Published : 19 April 2024
DOI : https://doi.org/10.1186/s12913-024-10828-0
Share this article
Anyone you share the following link with will be able to read this content:
Sorry, a shareable link is not currently available for this article.
Provided by the Springer Nature SharedIt content-sharing initiative
- Continuous quality improvement
- Quality of Care
BMC Health Services Research
ISSN: 1472-6963
- General enquiries: [email protected]
- Open access
- Published: 27 April 2024
Advancing social care integration in health systems with community health workers: an implementation evaluation based in Bronx, New York
- Kevin P. Fiori 1 , 2 ,
- Samantha Levano 1 , 2 ,
- Jessica Haughton 1 , 2 ,
- Renee Whiskey-LaLanne 1 , 2 ,
- Andrew Telzak 1 , 2 ,
- Hemen Muleta 1 ,
- Kavita Vani 3 ,
- Earle C. Chambers 2 &
- Andrew Racine 1
BMC Primary Care volume 25 , Article number: 140 ( 2024 ) Cite this article
Metrics details
In recent years, health systems have expanded the focus on health equity to include health-related social needs (HRSNs) screening. Community health workers (CHWs) are positioned to address HRSNs by serving as linkages between health systems, social services, and the community. This study describes a health system’s 12-month experience integrating CHWs to navigate HRSNs among primary care patients in Bronx County, NY.
We organized process and outcome measures using the RE-AIM (Reach, Effectiveness, Adoption, Implementation, Maintenance) implementation framework domains to evaluate a CHW intervention of the Community Health Worker Institute (CHWI). We used descriptive and inferential statistics to assess RE-AIM outcomes and socio-demographic characteristics of patients who self-reported at least 1 HRSN and were referred to and contacted by CHWs between October 2022 and September 2023.
There were 4,420 patients who self-reported HRSNs in the standardized screening tool between October 2022 and September 2023. Of these patients, 1,245 were referred to a CHW who completed the first outreach attempt during the study period. An additional 1,559 patients self-reported HRSNs directly to a clinician or CHW without being screened and were referred to and contacted by a CHW. Of the 2,804 total patients referred, 1,939 (69.2%) were successfully contacted and consented to work with a CHW for HRSN navigation. Overall, 78.1% ( n = 1,515) of patients reported receiving social services. Adoption of the CHW clinician champion varied by clinical team (median 22.2%; IQR 13.3–39.0%); however, there was no difference in referral rates between those with and without a clinician champion ( p = 0.50). Implementation of CHW referrals via an electronic referral order appeared successful (73.2%) and timely (median 11 days; IQR 2–26 days) compared to standard CHWI practices. Median annual cost per household per CHW for the intervention was determined to be $184.02 (IQR $134.72 – $202.12).
Conclusions
We observed a significant proportion of patients reporting successful receipt of social services following engagement with an integrated CHW model. There are additional implementation factors that require further inquiry and research to understand barriers and enabling factors to integrate CHWs within clinical teams.
Peer Review reports
Addressing the ways in which social needs influence health outcomes is critical to improving the health, well-being, and quality of life of communities and individuals. Health-related social needs (HRSNs) are defined as the self-reported individual experiences of social risk factors at a particular moment in time and may include needs such as housing stability, food security, or transportation access [ 1 ]. HRSNs are distinct from the broader, structural social determinants of health (SDoH), which are defined by the World Health Organization as “the conditions in which people are born, grow, work, live, and age, and the wider set of forces and systems shaping the conditions of daily life” [ 2 ]. In recent years, health systems have expanded their focus from access and quality of health care to include screening for and addressing HRSNs, which can influence more than 50% of a patient’s health [ 3 ]. Increasing clinical teams’ awareness of HRSNs is an important first step in addressing health disparities, as outlined by the National Academies of Sciences, Engineering, and Medicine (NASEM) [ 1 ]. Health systems have primarily integrated awareness activities by screening patients for HSRNs [ 4 ].
There remain clear gaps in how to best provide assistance once patients report HRSNs [ 5 ]. Health systems have demonstrated variability in linking patients with HRSNs to appropriate resources and social service providers, also known as “social prescribing” [ 6 ]. These decisions have recently shifted from the health system to regulatory agencies, which have identified HRSN screening and referral as an emerging priority. The Centers for Medicare & Medicaid Services (CMS) released new health equity measures, which mandate reporting and screening of key SDoH domains in inpatient settings in 2024 [ 7 ]. Meanwhile, the Joint Commission now requires health systems to screen patients for HRSNs as well as to address the HRSNs identified through a defined action plan [ 8 ].
Social prescribing activities may include referrals to onsite resources or community-based organizations, and involve nurses, social workers, student volunteers, or community health workers [ 6 ]. Community health workers (CHWs) are frontline public health workers with deep understanding of, and trust within local communities [ 9 ]. CHWs often serve as intermediaries between health care institutions, social services, and the community because they are uniquely positioned to facilitate access to resources, improve cultural competence of care delivery, strengthen patient self-sufficiency, and support communities in addressing the underlying causes of health disparities [ 10 ].
There is evidence supporting the effectiveness of CHW interventions in the US in improving patient care, reducing cost of care, and advancing health equity [ 11 ]; however, few studies have evaluated the impact of CHWs on HRSNs in real world practice. Previous randomized control trials (RCTs) examining CHW social prescribing interventions have reported reduced hospitalizations [ 12 ], improved health-related quality of life [ 13 ], improved access to primary care after hospitalization [ 14 ], and improved child health status [ 15 ] when compared to usual care. Additional CHW interventions have focused evaluations on the resolution of social needs [ 15 ] and connection to essential social services [ 16 , 17 , 18 ]. There is a demonstrated gap in understanding how a large health system can successfully integrate an enterprise wide CHW program into clinical practice. This study’s objective was to describe and evaluate a health system’s 12-month experience integrating CHWs within clinical teams to address HRSNs among primary care patients in a large, multi-cultural, resource-constrained system in Bronx County, NY.
Intervention
The Community Health Worker Institute (CHWI) at Montefiore Medical Center strives to improve health equity by optimizing the integration of CHWs within clinical care teams using a learning health system approach [ 19 ]. CHWs serve as a bridge between social and clinical care by addressing HRSNs and improving access to healthcare for disadvantaged populations. The CHWI centralizes recruitment, training, continuing professional development, and deployment of CHWs embedded within the health system.
Montefiore Medical Center primarily serves patients from Bronx County, New York, which is home to more than 1.3 million people [ 20 ]. It is one of the most diverse counties in the US, with 56.6% of its residents identifying as Hispanic and 44.3% as non-Hispanic Black. Bronx County consistently ranks among the least healthy counties in New York State in measures of health factors and health outcomes [ 3 ]. In spite of these challenges, the Bronx also has many assets and resources including access to higher education, healthcare facilities, open spaces, and community- and faith-based organizations [ 21 ].
The CHWI builds on an initial pilot, the Community Linkage to Care (CLC) program, which modeled standardized HRSN screening and CHW referral support in our health system in 2017 [ 22 ]. As part of the CLC program, the health system implemented a 10-item HRSN screening tool adapted from the Health Leads Toolkit (Supplemental Fig. 1) [ 23 ]. In 2022, the health system centralized CHW operations within the CHWI structure, representing a novel and enhanced investment towards CHW integration within the health system.
The CHWI was developed in partnership with key stakeholders including community-based organizations, CHW workforce pipeline programs, clinical teams, existing CHWs, and CHW networks. Leadership and management of the CHWI include former CHWs, which integrates their perspective into operations and implementation. The CHWI has also taken the steps to establish a Community Advisory Board (CAB) to represent the interests of patient representatives, local community leaders, and partners from the social service providers’ network. We utilize these established relationships to ensure the CHWI is meeting the needs of our catchment population, involve the community in CHW recruitment, and co-design new programs and proposals.
The CHWI has collaborated with multi-sector partners to recruit socially connected community residents who share the lived experiences of our patients. These CHWs are full-time employees with competitive salaries and robust union benefits packages who receive training, supervision, and mentorship immediately upon hire. Implementation of the CHWI includes a training plan composed of standards and guidelines for hiring and deployment, a comprehensive training curriculum, an accredited apprenticeship program with a local community college, a detailed scope of practice, and a peer-management structure. The supportive supervision structure is a key component of the CHWI, meeting with individual CHWs weekly and in groups monthly to review successes and challenges, provide mentorship, share bi-directional feedback, support professional development, and foster retention.
The CHWs employed by the CHWI provide social service navigation and referral support to patients with HRSNs within their assigned clinical practices. If a patient self-reports at least one HRSN during a clinical encounter, clinicians discuss the need or review the results of the screening tool, if screened, with the patient and their family members and ask whether they are interested in receiving assistance with their HRSN(s). If assistance is requested, clinicians send an electronic referral order within the health system’s electronic health record (EHR) to facilitate connection to CHWs. If assistance is requested but a CHW is not available, clinical teams utilize the health system’s EHR-supported social service directory to find available resources to refer patients to in their local community.
After receiving an electronic referral order from a clinician, CHWs contact the patient and/or their family member to collect household and demographic information, re-screen for HRSNs, confirm consent for assistance, and begin HRSN navigation. During HRSN navigation encounters, CHWs screen patients for eligibility criteria for public benefits programs, complete or assist with benefits applications, refer patients to community-based organizations, and provide direct benefits on site, when available. After CHWs complete in-person or telephone navigation encounters with patients, they conduct outreach with other clinical and community-based care team members and document connection to social services and resolution of HRSNs. CHWs conduct ongoing follow-up with each patient until their HRSN is resolved, no more progress can be made, the patient is disconnected, or the patient is no longer interested in receiving assistance from the CHW.
In prior work, we have demonstrated the successful reach and adoption of the HRSN screening arm of the previous CLC program [ 24 ]. This study aims to evaluate the centralized CHW referral component of the enhanced CHWI program.
Study design & data sources
This retrospective, cross-sectional study utilized data collected and managed through Research Electronic Data Capture (REDCap) tools to assess the integration of CHWs across the health system. REDCap is a secure, web-based software platform hosted at Albert Einstein College of Medicine, which is designed to support data capture for research studies [ 25 , 26 ]. The CHWI REDCap database is a tool designed for CHWs to routinely collect data on patient demographics, referral information, outreach encounters, social need services provided, and key program outcomes. This database was internally developed and has been continually updated by the CHWI team as part of our learning health system approach. Since the launch of CHWI, we have added new services offered in the community, integrated REDCap data access groups for CHW training, automated data entry processes for follow-up encounters, linked family members through unique record numbers, and streamlined reports to track patients with ongoing follow-up needed.
Additional data was extracted from the EHR to identify patients eligible to be referred to CHWs through HRSN screening and electronic referral order databases. All EHR data was extracted using Microsoft SQL Server, version 18, to query data from the Epic Electronic Health Record Data Warehouse.
Study population
Patients were eligible for inclusion in the analysis if they were referred to a CHW for HRSN service navigation and support within participating clinical teams and the first outreach was attempted by a CHW between October 2022 and September 2023. This time period reflects when the CHWI deployed its first cohort of CHWs within the system. Patients were initially referred to a CHW if they met the following eligibility criteria: (1) self-reported HRSNs by a standardized screening tool and requested assistance from a clinician, (2) self-reported HRSNs during the clinician visit and requested assistance from a clinician without being screened, or (3) self-reported HRSNs and requested assistance directly from the CHW, if on-site, without being screened. If the patient requested assistance directly from the CHW, the CHW would meet with the patient, contact the patient’s primary clinician, and request that the clinician retroactively submit an electronic referral order. We focused on an initial 12-month period to account for seasonality with additional follow-up outreach and outcomes for the patient after September 2023 being excluded from the analysis.
Study measures
We organized both process and outcome measures using the RE-AIM (Reach, Effectiveness, Adoption, Implementation, Maintenance) implementation framework domains (Table 1 ) [ 27 ]. This framework facilitated and organized assessments of implementation with the study period. We utilized data from five ambulatory pediatric and five ambulatory internal medicine clinical teams that were actively participating in this initiative during the study period. All process and outcome measures were focused on these ten clinical teams.
We defined reach as the (1) proportion of patients with self-reported HRSNs in the screening tool who were referred to and contacted by a CHW (i.e., eligibility criteria 1 only) and (2) the total number of patients who self-reported HRSNs by any means and were referred to and contacted by a CHW (i.e., eligibility criteria 1, 2, and 3). Patients were eligible to be referred to a CHW if they self-reported HRSNs with or without a screener; therefore, the second reach measure estimates the absolute coverage of the CHWI program. We utilized the first reach measure to estimate the potential drop-off between initial self-report of HRSNs and first attempted contact by the CHW because the screening tool provides the only standardized documentation of patients with self-reported HRSNs who may decline assistance from a CHW prior to the clinician electronic referral order.
To determine the proportion of patients eligible to be referred and contacted, we matched patients with self-reported HRSNs in the screening tool from the clinical teams of interest between October 2022 and September 2023 with patients contacted by CHWs from those clinical teams during the same time period using unique identifiers. For the second reach measure, we calculated the total number of patients contacted by CHWs from these clinical teams during the study period, since not all patients referred may have been screened using the standardized tool.
For the total patients referred and contacted, we further described their referral status based on their stage in the initial contact and consent process. We reported socio-demographic covariates, including age at referral, gender, race/ethnicity, and preferred spoken language, which were collected and documented by CHWs in the CHWI REDCap Database.
Effectiveness
We defined our primary effectiveness measure as the proportion of patients assisted with HRSNs for which the CHW connected (i.e., received) or equipped the patient with the tools to connect to at least one social service on their own. These outcomes are both considered successful because the CHW completed all necessary steps, per program workflows, to connect the patient to available services. We also defined a secondary effectiveness measure as the proportion of patients connected to at least one social service who resolved or made progress on at least one HRSN. This secondary measure was self-reported by the patient and only answered by those connected to at least one social service, not those equipped to complete connection on their own. These measures were based on internally standardized definitions for all social needs and services (Supplemental Table 1).
Adoption of the intervention was measured according to two measures, (1) the proportion patients with self-reported HRSNs in the screening tool who were referred to and contacted by a CHW by each clinical team and (2) the proportion of clinical teams establishing a clinician champion as recommended by the intervention. Clinician champions were defined as “full-time clinicians based at practice who serve as a clinical contact, mentor, and/or coach to support CHW team integration and lead performance improvement initiatives” and have been previously demonstrated to increase screening rates in our health system [ 22 , 28 ]. In other studies, clinician champions have demonstrated their ability to influence the behavior of other clinicians, challenge institutional norms, leverage professional relationships, cultivate a learning environment, and optimize existing workflows [ 29 , 30 ]. This role was implemented alongside the CHWI peer management structure so that CHWs would have an advocate within the clinical team to facilitate the HRSN screening and referral process. We used two sample T-tests to estimate whether the proportion of patients referred and contacted differed for clinical teams with and without clinician champions present.
Implementation
Implementation measures included fidelity measures of the extent to which clinical teams and CHWs adhered to recommended, established workflows. These measures included (1) the proportion of patients with electronic referral orders who were referred to and contacted by a CHW, and (2) the median time, in days, between the clinician’s electronic referral order and the first CHW outreach attempt. It was an operational expectation by the CHWI for CHWs to contact patients within 7 days of the electronic referral order by a clinician. The CHWI also encouraged clinicians to complete a warm handoff, or transfer of care, with the CHW after the on-site clinical visit as part of their standardized workflow. Due to potential delays in the documentation of the electronic referral orders in the EHR, we confirmed the utilization of a "warm handoff" in the CHWI REDCap Database and assigned the time between the order date and first outreach as 0 days for these cases. We excluded all other observations when the first CHW outreach attempt was dated prior to the electronic referral order date.
We did not assess time between the HRSN screen and electronic referral order since this is dependent solely on the clinician placing an order. To determine the proportion of patients with electronic referral orders contacted, we matched the EHR electronic referral order and CHWI REDCap databases using unique identifiers.
Maintenance
Maintenance was defined as the cost to sustain the CHWI intervention over time given the need to establish an estimated ongoing annual cost per beneficiary. This was measured by estimating the median annual cost to the health system per patient referred to a CHW. We first determined the annual patient count for each CHW and annual cost per CHW based on standardized CHWI salary estimates and time contributed by each CHW during the annualized study period. We then calculated the annual cost to the health system per patient for each CHW by dividing the annual patient count by the annual cost per CHW. Next, we calculated the weighted median annual cost to the health system per patient across the study period, with weights based on the proportion of months that the CHW participated in the intervention. We excluded observations for CHWs if they contributed partial data due to mid-month deployment or departure, provided only supplemental coverage to the clinical teams of interest, or demonstrated abnormal data due to performance concerns.
We used both descriptive and inferential statistics to summarize socio-demographic characteristics of patients referred and RE-AIM process and outcome measures. All descriptive and inferential statistics were conducted in SAS version 9.4. Weighted estimates for the Maintenance measure were calculated using PROC MEANS in SAS. All research was approved by the Albert Einstein College of Medicine Institutional Review Board (2017–8434).
Between October 2022 and September 2023, 25,996 unique patients were screened for HRSNs within the ten participating clinical teams using the screening tool, with 4,420 (17.0%) reporting at least one unmet HRSN. Of the patients who self-reported HRSNs in the screening tool, 1,782 were referred by clinicians via electronic referral order and 1,245 were successfully referred to and contacted by (i.e., completed the first outreach attempt) CHWs integrated within these clinical teams during the study period. An additional 1,559 patients self-reported HRSNs directly to a clinician (i.e., eligibility criteria 2) or CHW (i.e., eligibility criteria 3) and were referred to CHWs who attempted the first outreach.
We summarized the socio-demographic characteristics of the total referred population ( n = 2,804) (Table 2 ). Most patients referred were between 0 and 5 years of age (27.7%) followed by those between 30 and 64 years (23.9%). There were more women (55.7%) than men referred (43.8%). Referred patients most identified as Hispanic (42.4%) or Non-Hispanic Black (28.2%), with many patients declining to report their race or ethnicity (22.0%). Most patients preferred English as their primary language (74.3%) followed by Spanish (21.6%). There were 2,993 total HRSNs reported with the most reported HRSNs identified as housing security (24.7%), food security, (20.3%) and financial benefits (16.9%), respectively (Table 3 ).
There were 2,804 total patients referred from these clinical teams with the first outreach attempt completed by CHWs between October 2022 and September 2023. These patients were referred to a total of 28 full-time employed CHWs, who each contributed a median of 3.0 months (IQR 2.0–6.5 months) during the 12-month study period. We collected demographic data for 23 of the 28 CHWs included in the analysis. Most of the CHWs identified as Black or African American (65.2%) followed by other race (13.0%). Almost half (47.8%) of the CHWs identified as Hispanic or Latino. Most CHWs were between the ages of 25 to 34 years (65.2%) followed by those between 35 and 44 years (13.0%). Almost all CHWs identified as female (95.7%).
CHWs completed 12,376 total outreach encounters with these patients, with 84.9% of outreach attempts completed by phone, 7.7% in person, and the remaining by alternative or unknown methods. This includes 7,509 HRSN navigation encounters, with most navigation encounters (52.3%) reported as 15 min or less in duration. CHWs conducted HRSN navigation with patients and their families over a median of 31 days (IQR 8–70 days) across the study period.
Of the patients referred with attempted contacted, 1,939 (69.2%) were successfully contacted and consented to work with a CHW to address self-reported HRSNs. An additional 124 (4.4%) patients were successfully contacted but have yet to consent to CHW assistance, 329 (11.7%) were successfully contacted but declined CHW assistance, 336 (12.0%) were disconnected after three or more unsuccessful initial outreach attempts, and 76 (2.7%) patients were still awaiting successful initial contact by a CHW (i.e., have not three or more initial outreach attempts) at the time of data analysis (Fig. 1 ).
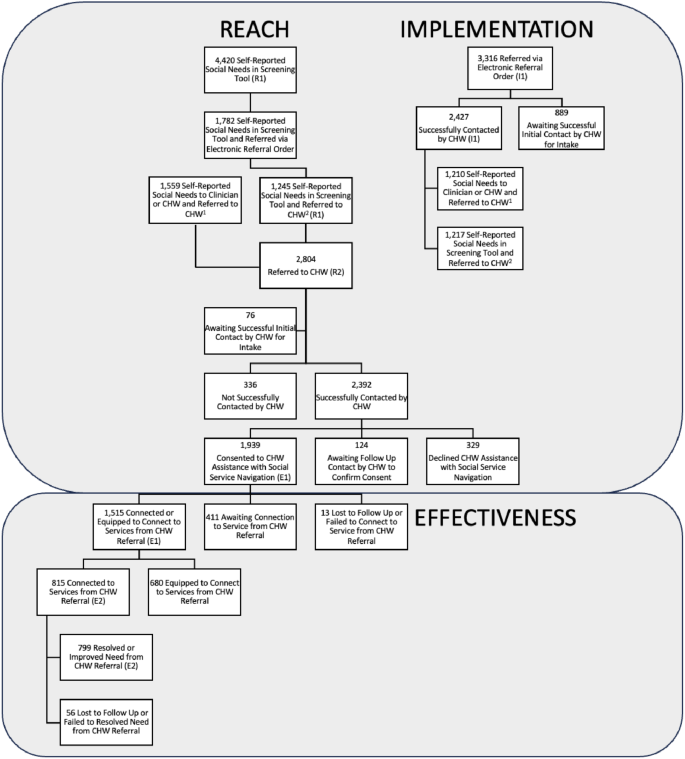
Reach and effectiveness of the community health worker institute in connecting patients with self-reported health-related social needs to services, October 2022- September 2023. 1 1,210 of 1,559 patients identified through direct report of HRSNs to a clinician or CHW were referred via electronic referral order during the study period. 2 1,217 of 1,245 patients identified through the screening tool were referred via electronic referral order during the study period
We measured CHW effectiveness through patient reported connection to social services. Overall, 1,515 (78.1%) of the 1,939 patients were connected (i.e., received) ( n = 835) or equipped to connect ( n = 680) to social services (Supplemental Table 1). Meanwhile, 13 (0.7%) patients failed to connect to services or were lost to follow-up before services could be confirmed. The remaining 411 (21.2%) patients are still actively working with a CHW at the time of study end period (Fig. 1 ).
Of the 815 patients who were connected to a social service (i.e., received only), 779 (93.3%) reported that their HRSN was improved or fully resolved. Approximately 56 (6.7%) patients reported that they failed to make progress on their HRSN or were not able to document progress because they were disconnected from care.
In our health system, every clinical team approached during the study period integrated CHWs into their team for HSRN navigation. Of the ten clinical teams, adoption of the referral component of the intervention varied with a range of 6.4–72.4% of eligible patients referred to a CHW, with a median referral rate of 27.8% (IQR 14.9–44.6%) (Table 4 ). We also measured adoption of a key aspect of the intervention, the adoption of a clinician champion within the team. Approximately 80% ( n = 8) of clinical teams had recruited or retained a clinician champion during the study period to collaborate with the CHWI program team, educate other clinicians on the referral process, and discuss barriers and facilitators to implementation. There was no difference in the average rate of referral usage between clinical teams with (80%) and without (20%) a clinician champion present ( p = 0.50).
There were 3,316 patients with electronic referral orders sent by clinicians, after self-reporting HRSNs in the screening tool or directly to a clinician or CHW, between October 2022 and September 2023. CHWs completed the first outreach attempt for 2,427 (73.2%) of these patients, who are included in our study sample, with the remaining 889 patients (26.8%) still awaiting initial outreach by a CHW at the time of data analysis. Of the 2,427 patients contacted, 1,210 were initially identified through direct report of HRSNs to a clinician or CHW while 1,217 were identified through the screening tool (Fig. 1 ). There were 377 patients, of the total 2,804 referred patients in the study sample, who were excluded in this assessment because their electronic referral orders were sent outside of the study period.
We measured the time between the patient’s electronic referral order and first outreach attempt by the CHW to better understand implementation of the intervention by CHWs. The median time for CHWs to first contact the patient was 11 days (IQR 2–26 days) after the electronic referral order, compared to standard CHWI expectation of 7 days. There were 273 patients whose electronic referral order and first outreach attempt were reassigned to the same day because their clinicians completed a warm handoff with the CHW, as confirmed in the CHWI REDCap Database. There were 392 patients with electronic referral orders sent after the CHW’s first outreach attempt that were excluded from this study.
There were 12 CHWs included in our assessment of median annual cost to the health system per patient to sustain the CHWI intervention. After applying analytic weights, calculated based on the number of months contributed by each CHW to the intervention, we determined that the median annual cost per patient was $184.02 (IQR $134.72 – $202.12).
The CHWI reached over 2,800 patients in its initial 12-month roll-out period and was effective in linking nearly 80% of patients assisted to resources. Adoption of the CHWI intervention components varied by participating clinical team, with no difference in referral rates between clinical teams with and without a clinician champion present. Implementation of CHW referrals via the electronic referral order from clinicians and initial contact by CHWs was overall successful and timely. Maintaining CHWs in our health system will require more sustainable funding as adoption of the program expands; however, the median cost per patient provides a baseline cost estimate to prepare for future reimbursement and value-based payment models.
This evaluation expands and adds to existing knowledge of assessing real-world implementation of social prescribing interventions. In 2022, the RE-AIM framework was utilized to evaluate a similar ambulatory social care program, which reached 34% of patients who screened positive for HRSNs and connected 75% of participants to social services [ 31 ]. This program is comparable in scale to the catchment population of the CHWI but limited to pediatric settings. Additional programs focused on CHWs and HRSNs have demonstrated varying estimates of reach but at smaller scale [ 16 ] and within different settings [ 32 ].
Preliminary results from the largest HRSN screening and referral program in the US, the American Health Communities (AHC) Model, have demonstrated a much higher acceptance rate, reaching closer to 80% of eligible participants [ 33 ]. This model, however, has also suggested that HRSN navigation alone is not effective in increasing connection to social services or resolving social needs [ 33 ]. There were significant barriers noted by beneficiaries and service providers in accessing or confirming connection to services that likely contributed to the effectiveness of HRSN navigation. Additionally, services accessed were not always enough to meet the needs of the beneficiaries. Recommendations from this study include assessing and investing in local community service provider capacity and exploring additional mechanisms, other than addressing HRSNs, through which navigation programs may contribute to health outcomes.
Our experience has suggested that effective integration of CHWs within health systems is challenging as the role is often novel with ambiguous roles and scope. Prior to engagement with CHWs, clinical teams may struggle with understanding the CHW role or experience conflict while transitioning from traditional care models [ 34 ]. In a qualitative study in Chicago, higher levels of CHW integration were found in clinical teams with greater alignment in CHW purpose and value perspectives across administrators, clinicians, and CHWs [ 35 ]. Additional studies indicated that communication channels, internal and external trainings, community expertise, and opportunities for CHW connection were most valuable to CHW integration [ 36 , 37 ]. Despite the finding that clinician champions were not associated with adoption of CHW referrals in our study, these staff members have increased access and opportunity to educate other clinicians on the purpose and value of the CHWI referral program. Clinician and administrative champions were identified as key facilitators to CHW integration in a study in Minnesota, along with a culture of innovation, prioritization of nonmedical determinants of health, and sustainable reimbursement strategies [ 38 ].
Few studies have successfully demonstrated the direct economic impact of CHW interventions. In a recent analysis as part of a RCT in Pennsylvania, the CHW program demonstrated an annual return of $2.47 for every dollar invested annually by Medicaid, which further incentivizes state Medicaid programs and health systems to invest in CHW programs to improve health outcomes, address HRSNs, and lower costs [ 39 ]. Several states have started utilizing CHW services to address population health needs and have authorized their payments through Medicaid programs [ 40 ]. In 2023, New York State (NYS) Medicaid announced that health care providers would be reimbursed for CHW services. This funding was initially limited to pregnant and postpartum individuals but will be expanded to children and adults with HRSNs in 2024, which will directly impact the maintenance of the CHWI [ 41 ].
In NYS, CHWs will be reimbursed at a rate of $35.00 per Medicaid member for individual education and training sessions, with 12 annual sessions allowed for adults and 24 for children [ 42 ]. The CHWI developed its maintenance measure based on the number of patients served per year rather than the number of sessions administered, given that it does not currently limit the number of sessions per patient. Additionally, the CHWI does not administer group navigation sessions, for which Medicaid also offers rates of reimbursement [ 42 ]. These are factors that the CHWI model may need to consider adapting or may need to advocate against as non-practical elements of the Medicaid-reimbursement model. There are additional opportunities for sustainable funding in NYS to expand the CHWI, including the Sect. 1115 waiver and shift from Fee for Service towards Value Based Payment models [ 40 ]. As new models are released and updated, it is important that we continue to compare our baseline cost estimates to determine the need for future adaptation and advocacy work.
Limitations
This study has several limitations to address. First, patients with self-reported HRSNs identified in the screening tool are not representative of all active patients with HRSNs in the health system. Although universal screening is recommended, clinical teams have the discretion to screen their patient population based on pre-defined intervals or eligibility criteria. Additionally, patients who do complete the screen may not self-report HRSNs due to a lack of trust in the health care system [ 43 ]. As previously mentioned, patients may also self-report HRSNs directly to the clinician or CHW without being screened; however, there is currently no documentation of these patients unless the patient requests assistance and the clinician sends an electronic referral order or the CHW completes the first outreach attempt.
There are also limitations in documenting patients who self-report HRSNs in the screening tool but do not request assistance with HRSNs. We are able to document rates of acceptance for these patients; however, this measure is not widely utilized with significant missing data observed [ 44 ]. We also do not have data available for patients who requested assistance and were referred to the EHR-supported social service directory when a CHW was not available.
There are additional limitations related to the workflow between clinicians and CHWs. The health system recommends, but does not require, that clinicians screen patients for HRSNs prior to completing an electronic referral order. Therefore, not all patients referred will be identified as eligible in the EHR screening database. Additionally, there are challenges in matching the EHR database with the REDCap CHWI database due to lag times between screening, electronic referral order, and first CHW contact dates.
Finally, data collection for HRSN screening and CHW referrals were conducted by non-research staff as part of routine service delivery. Although data entry safeguards were utilized and data were regularly reviewed by investigators, there is potential for misclassification bias and data entry errors. Our process and outcome measures have specific limitations given the nature of social service referrals. We define success according to the CHWs ability to facilitate connection to services rather than the patient’s actual receipt of benefits, which is challenged by the barriers and limitations of the social service industry. This definition may overestimate the “true” magnitude of our interventions effect on HRSNs. On the other hand, we define effectiveness as unsuccessful when patients are disconnected from CHWs, which may underestimate that same effect.
We conducted an implementation evaluation of a real-world CHW intervention aimed at connecting patients with HRSNs to social services. Despite significant proportions of patients both connecting to social services and reporting progress or resolution of HRSNs after receipt of CHW assistance, there is more optimization work ahead. We need to better understand why a meaningful proportion of patients with HRSNs either decline assistance or are not successfully connected to CHW navigation services. Furthermore, we need more rigorous costing assessments to understand how health systems can sustain this workforce. The shift of health systems to improve social care integration portends improved health outcomes and reduced health disparities, but there is more research and learning required to achieve these goals.
Data availability
The de-identified datasets used and/or analyzed during the current study are available from the corresponding author on reasonable request.
Abbreviations
health-related social nee
social determinants of health
community health worker
randomized control trial
Community Health Worker Institute
Community Linkage to Care
electronic health record
Research Electronic Data Capture
Integrating Social Care into the Delivery of Health Care. Moving Upstream to improve the nation’s health. Washington (DC): The National Academies of Sciences, Engineering, and Medicine; 2019.
Google Scholar
Solar O, Irwin A. A conceptual framework for action on the social determinants of health. Social Determinants of Health Discussion Paper 2 (Policy and Practice). Geneva: World Health Organization; 2010.
County Health Rankings Model: Health Factors: University of Wisconsin Population Health Institute. 2023 [ https://www.countyhealthrankings.org/explore-health-rankings/county-health-rankings-model/health-factors? .
Whitman A, Lew ND, Chappel A, Aysola V, Zuckerman R, Sommers BD. Addressing Social Determinants of Health: Examples of Successful Evidence-Based Strategies and Current Federal Efforts. 2022.
Kreuter MW, Thompson T, McQueen A, Garg R. Addressing Social needs in Health Care settings: evidence, challenges, and Opportunities for Public Health. Annu Rev Public Health. 2021;42:329–44.
Article PubMed PubMed Central Google Scholar
Gottlieb L, Cottrell EK, Park B, Clark KD, Gold R, Fichtenberg C. Advancing Social prescribing with implementation science. J Am Board Fam Med. 2018;31(3):315–21.
Article PubMed Google Scholar
The CMS Framework for Health Equity. (2022–2032). Baltimore, MD: Centers for Medicare & Medicaid Services; 2022.
New Requirements to Reduce Health Care Disparities. The Joint Commission; 2022.
APHA. Community Health Workers. APHA; [ https://www.apha.org/apha-communities/member-sections/community-health-workers .
Brown O, Kangovi S, Wiggins N, Alvarado CS. Supervision Strategies and Community Health Worker Effectiveness in Health Care Settings. NAM Perspect. 2020;2020.
Knowles M, Crowley AP, Vasan A, Kangovi S. Community Health Worker Integration with and Effectiveness in Health Care and Public Health in the United States. Annu Rev Public Health. 2023;44:363–81.
Kangovi S, Mitra N, Norton L, Harte R, Zhao X, Carter T, et al. Effect of Community Health Worker support on clinical outcomes of low-income patients across Primary Care facilities: a Randomized Clinical Trial. JAMA Intern Med. 2018;178(12):1635–43.
Patel MI, Kapphahn K, Wood E, Coker T, Salava D, Riley A et al. Effect of a Community Health worker-led intervention among low-income and Minoritized patients with Cancer: a Randomized Clinical Trial. J Clin Oncol. 2023:JCO2300309.
Kangovi S, Mitra N, Grande D, White ML, McCollum S, Sellman J, et al. Patient-centered community health worker intervention to improve posthospital outcomes: a randomized clinical trial. JAMA Intern Med. 2014;174(4):535–43.
Gottlieb LM, Hessler D, Long D, Laves E, Burns AR, Amaya A, et al. Effects of Social needs screening and In-Person Service Navigation on Child Health: a Randomized Clinical Trial. JAMA Pediatr. 2016;170(11):e162521.
Schechter SB, Lakhaney D, Peretz PJ, Matiz LA. Community Health Worker Intervention To Address Social Determinants of Health for Children Hospitalized with Asthma. Hosp Pediatr. 2021;11(12):1370–6.
Matiz LA, Leong S, Peretz PJ, Kuhlmey M, Bernstein SA, Oliver MA, et al. Integrating community health workers into a community hearing health collaborative to understand the social determinants of health in children with hearing loss. Disabil Health J. 2022;15(1):101181.
Flike K, Means RH, Chou J, Shi L, Hayman LL. Bridges to elders: a program to improve outcomes for older women experiencing homelessness. Health Promot Pract. 2023:15248399231192992.
Olsen L, Aisner D, McGinnis JM, editors. The Learning Healthcare System: Workshop Summary. Washington (DC)2007.
: QuickFacts, County B. New York 2022 [ https://www.census.gov/quickfacts/fact/table/bronxcountynewyork/PST045222 .
MMC. Community Health Needs AssessmentImplementation Strategy Report and Community Service Plan 2022–2024. 2022.
Fiori KP, Rehm CD, Sanderson D, Braganza S, Parsons A, Chodon T, et al. Integrating Social Needs Screening and Community Health Workers in Primary Care: the Community linkage to Care Program. Clin Pediatr (Phila). 2020;59(6):547–56.
HealthLeads. The Health Leads Screening Toolkit 2023 [ https://healthleadsusa.org/news-resources/the-health-leads-screening-toolkit/ .
Fiori KP, Heller CG, Flattau A, Harris-Hollingsworth NR, Parsons A, Rinke ML, et al. Scaling-up social needs screening in practice: a retrospective, cross-sectional analysis of data from electronic health records from Bronx County, New York, USA. BMJ Open. 2021;11(9):e053633.
Harris PA, Taylor R, Minor BL, Elliott V, Fernandez M, O’Neal L, et al. The REDCap consortium: building an international community of software platform partners. J Biomed Inf. 2019;95:103208.
Article Google Scholar
Harris PA, Taylor R, Thielke R, Payne J, Gonzalez N, Conde JG. Research electronic data capture (REDCap)--a metadata-driven methodology and workflow process for providing translational research informatics support. J Biomed Inf. 2009;42(2):377–81.
Glasgow RE, Harden SM, Gaglio B, Rabin B, Smith ML, Porter GC, et al. RE-AIM planning and evaluation Framework: adapting to New Science and Practice with a 20-Year review. Front Public Health. 2019;7:64.
Berman RS, Nguyen HT, Levano SR, Fiori KP. Clinician Champions’ Influence on Social Needs Screening Volumes in Pediatric Practices. Clin Pediatr (Phila). 2023:99228231200404.
Bunce AE, Gruss I, Davis JV, Cowburn S, Cohen D, Oakley J, et al. Lessons learned about the effective operationalization of champions as an implementation strategy: results from a qualitative process evaluation of a pragmatic trial. Implement Sci. 2020;15(1):87.
Bonawitz K, Wetmore M, Heisler M, Dalton VK, Damschroder LJ, Forman J, et al. Champions in context: which attributes matter for change efforts in healthcare? Implement Sci. 2020;15(1):62.
DeCamp LR, Yousuf S, Peters C, Cruze E, Kutchman E. Assessing strengths, challenges, and Equity Via pragmatic evaluation of a Social Care Program. Acad Pediatr. 2023.
Foster AA, Daly CJ, Leong R, Stoll J, Butler M, Jacobs DM. Integrating community health workers within a pharmacy to address health-related social needs. J Am Pharm Assoc (2003). 2023;63(3):799–806 e3.
Renaud J, McClellan SR, DePriest K, Witgert K, O’Connor S, Abowd Johnson K, et al. Addressing Health-Related Social needs Via Community resources: lessons from Accountable Health communities. Health Aff (Millwood). 2023;42(6):832–40.
Washburn DJ, Callaghan T, Schmit C, Thompson E, Martinez D, Lafleur M. Community health worker roles and their evolving interprofessional relationships in the United States. J Interprof Care. 2022;36(4):545–51.
McCarville EE, Martin MA, Pratap PL, Pinsker E, Seweryn SM, Peters KE. Understanding the relationship between care team perceptions about CHWs and CHW integration within a US health system, a qualitative descriptive multiple embedded case study. BMC Health Serv Res. 2022;22(1):1587.
Allen CG, Escoffery C, Satsangi A, Brownstein JN. Strategies to improve the Integration of Community Health Workers Into Health Care teams: a little fish in a Big Pond. Prev Chronic Dis. 2015;12:E154.
Malcarney MB, Pittman P, Quigley L, Horton K, Seiler N. The Changing Roles of Community Health Workers. Health Serv Res. 2017;52(Suppl 1Suppl 1):360–82.
Rogers EA, Manser ST, Cleary J, Joseph AM, Harwood EM, Call KT. Integrating Community Health Workers Into Medical Homes. Ann Fam Med. 2018;16(1):14–20.
Kangovi S, Mitra N, Grande D, Long JA, Asch DA. Evidence-based Community Health Worker Program addresses Unmet Social needs and generates positive return on investment. Health Aff (Millwood). 2020;39(2):207–13.
Haldar S, Hinton E. State Policies for Expanding Medicaid Coverage of Community Health Worker (CHW) Services: KFF; 2023 [ https://www.kff.org/medicaid/issue-brief/state-policies-for-expanding-medicaid-coverage-of-community-health-worker-chw-services/ .
Assembly Bill AC, STATE OF NEW YORK. 2023–2024 Legislative Session Sess. (2023).
NYCDOH. Community Health Worker Services Policy Manual. 2023.
Armstrong K, Rose A, Peters N, Long JA, McMurphy S, Shea JA. Distrust of the health care system and self-reported health in the United States. J Gen Intern Med. 2006;21(4):292–7.
Shi M, Fiori K, Kim RS, Gao Q, Umanski G, Thomas I, et al. Social Needs Assessment and Linkage to Community Health Workers in a large Urban Hospital System. J Prim Care Community Health. 2023;14:21501319231166918.
Download references
Acknowledgements
The authors would like to acknowledge the role of many CHWI program partners including team members from the Montefiore Office of Community and Population Health; Montefiore Medical Group leadership and analytics team for developing the screening tool and integrating it within the electronic health record; Hostos Community College for partnering with CHWI to train CHWs and build sustainable healthcare careers for local community members; CHWI programs team for recruiting, training, managing, and leading a skilled and insightful team of CHWs; CHWs for always going above and beyond to find resources and assist our patients; and the staff and patients at Montefiore Health System for supporting this new initiative.
Support for this work was provided by the New York Health Foundation, Doris Duke Charitable Foundation (2023 − 0258) and the Harold and Muriel Block Institute for Clinical and Translational Research at Einstein and Montefiore (UL1TR002556).
Author information
Authors and affiliations.
Department of Pediatrics, Albert Einstein College of Medicine, 1300 Morris Park Avenue, Bronx, NY, 10461, USA
Kevin P. Fiori, Samantha Levano, Jessica Haughton, Renee Whiskey-LaLanne, Andrew Telzak, Hemen Muleta & Andrew Racine
Department of Family and Social Medicine, Albert Einstein College of Medicine, 1300 Morris Park Avenue, Bronx, NY, 10461, USA
Kevin P. Fiori, Samantha Levano, Jessica Haughton, Renee Whiskey-LaLanne, Andrew Telzak & Earle C. Chambers
Department of Obstetrics & Gynecology and Women’s Health, Albert Einstein College of Medicine, 1300 Morris Park Avenue, Bronx, NY, 10461, USA
Kavita Vani
You can also search for this author in PubMed Google Scholar
Contributions
KPF contributed to the study design; SL analyzed the data; SL contributed to literature review; SL, KPF, and JH contributed to writing of the manuscript. KPF, SL, JH, RW, AT, HM, KV, ECC, and AR read, reviewed, and approved the final manuscript.
Corresponding author
Correspondence to Kevin P. Fiori .
Ethics declarations
Ethics approval and consent to participate.
All research was approved by the Albert Einstein College of Medicine Institutional Review Board (2017–8434). The Albert Einstein College of Medicine Institutional Review Board granted our study a waiver of informed consent since this was a retrospective, cross-sectional analysis of data routinely collected by the health system.
Consent for publication
Not applicable.
Conflicts of interest
The authors have no conflicts of interest to disclose.
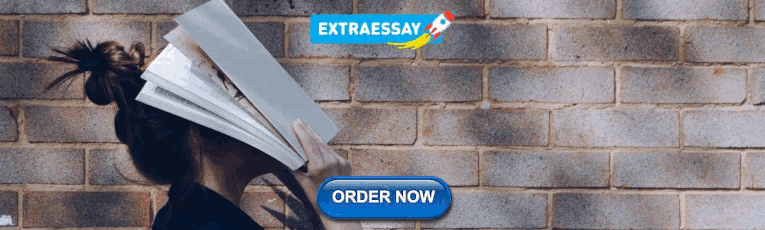
Additional information
Publisher’s note.
Springer Nature remains neutral with regard to jurisdictional claims in published maps and institutional affiliations.
Electronic supplementary material
Below is the link to the electronic supplementary material.
Supplementary Material 1
Rights and permissions.
Open Access This article is licensed under a Creative Commons Attribution 4.0 International License, which permits use, sharing, adaptation, distribution and reproduction in any medium or format, as long as you give appropriate credit to the original author(s) and the source, provide a link to the Creative Commons licence, and indicate if changes were made. The images or other third party material in this article are included in the article’s Creative Commons licence, unless indicated otherwise in a credit line to the material. If material is not included in the article’s Creative Commons licence and your intended use is not permitted by statutory regulation or exceeds the permitted use, you will need to obtain permission directly from the copyright holder. To view a copy of this licence, visit http://creativecommons.org/licenses/by/4.0/ . The Creative Commons Public Domain Dedication waiver ( http://creativecommons.org/publicdomain/zero/1.0/ ) applies to the data made available in this article, unless otherwise stated in a credit line to the data.
Reprints and permissions
About this article
Cite this article.
Fiori, K.P., Levano, S., Haughton, J. et al. Advancing social care integration in health systems with community health workers: an implementation evaluation based in Bronx, New York. BMC Prim. Care 25 , 140 (2024). https://doi.org/10.1186/s12875-024-02376-7
Download citation
Received : 09 February 2024
Accepted : 09 April 2024
Published : 27 April 2024
DOI : https://doi.org/10.1186/s12875-024-02376-7
Share this article
Anyone you share the following link with will be able to read this content:
Sorry, a shareable link is not currently available for this article.
Provided by the Springer Nature SharedIt content-sharing initiative
- Health related social needs
- Community health workers
- Health equity
- Primary health care
- Social determinants of health
- Social care integration
BMC Primary Care
ISSN: 2731-4553
- General enquiries: [email protected]
Europe PMC requires Javascript to function effectively.
Either your web browser doesn't support Javascript or it is currently turned off. In the latter case, please turn on Javascript support in your web browser and reload this page.
Search life-sciences literature (43,980,279 articles, preprints and more)
- Free full text
- Citations & impact
- Similar Articles
An overview of the qualitative descriptive design within nursing research.
Author information, affiliations, orcids linked to this article.
- Doyle L | 0000-0002-0153-8326
- Brady A | 0000-0002-7112-6810
- McCann M | 0000-0002-7925-6396
Journal of Research in Nursing : JRN , 18 Dec 2019 , 25(5): 443-455 https://doi.org/10.1177/1744987119880234 PMID: 34394658 PMCID: PMC7932381
Free full text in Europe PMC
Abstract
Methods and results, conclusions, free full text .

An overview of the qualitative descriptive design within nursing research
Louise doyle.
Associate Professor in Mental Health Nursing, School of Nursing and Midwifery, Trinity College Dublin, Ireland
Catherine McCabe
Associate Professor in General Nursing, School of Nursing and Midwifery, Trinity College Dublin, Ireland
Brian Keogh
Assistant Professor in Mental Health Nursing, School of Nursing and Midwifery, Trinity College Dublin, Ireland
Annemarie Brady
Chair of Nursing and Chronic Illness, School of Nursing and Midwifery, Trinity College Dublin, Ireland
Qualitative descriptive designs are common in nursing and healthcare research due to their inherent simplicity, flexibility and utility in diverse healthcare contexts. However, the application of descriptive research is sometimes critiqued in terms of scientific rigor. Inconsistency in decision making within the research process coupled with a lack of transparency has created issues of credibility for this type of approach. It can be difficult to clearly differentiate what constitutes a descriptive research design from the range of other methodologies at the disposal of qualitative researchers.
This paper provides an overview of qualitative descriptive research, orientates to the underlying philosophical perspectives and key characteristics that define this approach and identifies the implications for healthcare practice and policy.
Using real-world examples from healthcare research, the paper provides insight to the practical application of descriptive research at all stages of the design process and identifies the critical elements that should be explicit when applying this approach.
By adding to the existing knowledge base, this paper enhances the information available to researchers who wish to use the qualitative descriptive approach, influencing the standard of how this approach is employed in healthcare research.
Introduction
Qualitative descriptive approaches to nursing and healthcare research provide a broad insight into particular phenomena and can be used in a variety of ways including as a standalone research design, as a precursor to larger qualitative studies and commonly as the qualitative component in mixed-methods studies. Despite the widespread use of descriptive approaches within nursing research, there is limited methodological guidance about this type of design in research texts or papers. The lack of adequate representation in research texts has at times resulted in novice researchers using other more complex qualitative designs including grounded theory or phenomenology without meeting the requirements of these approaches ( Lambert and Lambert, 2012 ), or having an appropriate rationale for use of these approaches. This suggests there is a need to have more discussion about how and why descriptive approaches to qualitative research are used. This serves to not only provide information and guidance for researchers, but to ensure acceptable standards in how this approach is applied in healthcare research.
Rationale for qualitative descriptive research
The selection of an appropriate approach to answer research questions is one of the most important stages of the research process; consequently, there is a requirement that researchers can clearly articulate and defend their selection. Those who wish to undertake qualitative research have a range of approaches available to them including grounded theory, phenomenology and ethnography. However, these designs may not be the most suitable for studies that do not require a deeply theoretical context and aim to stay close to and describe participants’ experiences. The most frequently proposed rationale for the use of a descriptive approach to is to provide straightforward descriptions of experiences and perceptions ( Sandelowski, 2010 ), particularly in areas where little is known about the topic under investigation. A qualitative descriptive design may be deemed most appropriate as it recognises the subjective nature of the problem, the different experiences participants have and will present the findings in a way that directly reflects or closely resembles the terminology used in the initial research question ( Bradshaw et al., 2017 ). This is particularly relevant in nursing and healthcare research, which is commonly concerned with how patients experience illness and associated healthcare interventions. The utilisation of a qualitative descriptive approach is often encouraged in Master’s level nurse education programmes as it enables novice clinical nurse researchers explore important healthcare questions that have direct implications and impact for their specific healthcare setting (Colorafi and Evans, 2016). As a Master’s level project is often the first piece of primary research undertaken by nurses, the use of a qualitative descriptive design provides an excellent method to address important clinical issues where the focus is not on increasing theoretical or conceptual understanding, but rather contributing to change and quality improvement in the practice setting ( Chafe, 2017 ).
This design is also frequently used within mixed-methods studies where qualitative data can explain quantitative findings in explanatory studies, be used for questionnaire development in exploratory studies and validate and corroborate findings in convergent studies ( Doyle et al., 2016 ). There has also been an increase in the use of qualitative descriptive research embedded in large-scale healthcare intervention studies, which can serve a number of purposes including identifying participants’ perceptions of why an intervention worked or, just as importantly, did not work and how the intervention might be improved ( Doyle et al., 2016 ). Using qualitative descriptive research in this manner can help to make the findings of intervention studies more clinically meaningful.
Philosophical and theoretical influences
Qualitative descriptive research generates data that describe the ‘who, what, and where of events or experiences’ from a subjective perspective ( Kim et al., 2017 , p. 23). From a philosophical perspective, this approach to research is best aligned with constructionism and critical theories that use interpretative and naturalistic methods ( Lincoln et al., 2017 ). These philosophical perspectives represent the view that reality exists within various contexts that are dynamic and perceived differently depending on the subject, therefore, reality is multiple and subjective ( Lincoln et al., 2017 ). In qualitative descriptive research, this translates into researchers being concerned with understanding the individual human experience in its unique context. This type of inquiry requires flexible research processes that are inductive and dynamic but do not transform the data beyond recognition from the phenomenon being studied ( Ormston et al., 2014 ; Sandelwoski 2010). Descriptive qualitative research has also been aligned with pragmatism ( Neergaard et al., 2009 ) where decisions are made about how the research should be conducted based on the aims or objectives and context of the study ( Ormston et al., 2014 ). The pragmatist researcher is not aligned to one particular view of knowledge generation or one particular methodology. Instead they look to the concepts or phenomena being studied to guide decision making in the research process, facilitating the selection of the most appropriate methods to answer the research question ( Bishop, 2015 ).
Perhaps linked to the practical application of pragmatism to research, that is, applying the best methods to answer the research question, is the classification of qualitative descriptive research by Sandelowski ( 2010 , p. 82) into a ‘distributed residual category’. This recognises and incorporates uncertainty about the phenomena being studied and the research methods used to study them. For researchers, it permits the use of one or more different types of inquiry, which is essential when acknowledging and exploring different realities and subjective experiences in relation to phenomena ( Long et al., 2018 ). Clarity, in terms of the rationale for the phenomenon being studied and the methods used by the researcher, emerges from the qualitative descriptive approach because the data gathered continue to remain close to the phenomenon throughout the study ( Sandelowski, 2010 ). For this to happen a flexible approach is required and this is evident in the practice of ‘borrowing’ elements of other qualitative methodologies such as grounded theory, phenomenology and ethnography ( Vaismoradi et al., 2013 ).
Regarded as a positive aspect by many researchers who are interested in studying human nature and phenomenon, others believe this flexibility leads to inconsistency across studies and in some cases complacency by researchers. This can result in vague or unexplained decision making around the research process and subsequent lack of credibility. Accordingly, nurse researchers need to be reflexive, that is, clear about their role and position in terms of the phenomena being studied, the context, the theoretical framework and all decision-making processes used in a qualitative descriptive study. This adds credibility to both the study and qualitative descriptive research.
Methods in qualitative descriptive research
As with any research study, the application of descriptive methods will emerge in response to the aims and objectives, which will influence the sampling, data collection and analysis phases of the study.
Most qualitative research aligns itself with non-probability sampling and descriptive research is no different. Descriptive research generally uses purposive sampling and a range of purposive sampling techniques have been described ( Palinkas et al., 2015 ). Many researchers use a combination of approaches such as convenience, opportunistic or snowball sampling as part of the sampling framework, which is determined by the desired sample and the phenomena being studied.
Purposive sampling refers to selecting research participants that can speak to the research aims and who have knowledge and experience of the phenomenon under scrutiny ( Ritchie et al., 2014 ). When purposive sampling is used in a study it delimits and narrows the study population; however, researchers need to remember that other characteristics of the sample will also affect the population, such as the location of the researcher and their flexibility to recruit participants from beyond their base. In addition, the heterogeneity of the population will need to be considered and how this might influence sampling and subsequent data collection and analysis ( Palinkas et al ., 2015 ). Take, for example, conducting research on the experience of caring for people with Alzheimer’s disease (AD). For the most part AD is a condition that affects older people and experiences of participants caring for older people will ultimately dominate the sample. However, AD also affects younger people and how this will impact on sampling needs to be considered before recruitment as both groups will have very different experiences, although there will be overlap. Teddlie and Fu (2007) suggest that although some purposive sampling techniques generate representative cases, most result in describing contrasting cases, which they argue are at the heart of qualitative analysis. To achieve this, Sandelowski (2010) suggests that maximum variation sampling is particularly useful in qualitative descriptive research, which may acknowledge the range of experiences that exist especially in healthcare research. Palinkas et al . (2015) describe maximum variation sampling as identifying shared patterns that emerge from heterogeneity. In other words, researchers attempt to include a wide range of participants and experiences when collecting data. This may be more difficult to achieve in areas where little is known about the substantive area and may depend on the researcher’s knowledge and immersion within the subject area.
Sample size will also need to be considered and although small sample sizes are common in qualitative descriptive research, researchers need to be careful they have enough data collected to meet the study aims ( Ritchie et al., 2014 ). Pre-determining the sample size prior to data collection may stifle the analytic process, resulting in too much or too little data. Traditionally, the gold standard for sample size in qualitative research is data saturation, which differs depending on the research design and the size of the population ( Fusch and Ness, 2015 ). Data saturation is reached ‘when there is enough information to replicate the study, when the ability to obtain additional new information has been attained, and when further coding is no longer feasible’ ( Fusch and Ness, 2015 , p. 1408). However, some argue that although saturation is often reported, it is rarely demonstrated in qualitative descriptive research reports ( Caelli et al., 2003 ; Malterud et al., 2016 ). If data saturation is used to determine sample size, it is suggested that greater emphasis be placed on demonstrating how saturation was reached and at what level to provide more credibility to sample sizes ( Caelli et al., 2003 ). Sample size calculation should be an estimate until saturation has been achieved through the concurrent processes of data collection and analysis. Where saturation has not been achieved, or where sample size has been predetermined for resource reasons, this should be clearly acknowledged. However, there is also a movement away from the reliance on data saturation as a measure of sample size in qualitative research ( Malterud et al., 2016 ). O’Reilly and Parker (2012) question the appropriateness of the rigid application of saturation as a sample size measure arguing that outside of Grounded Theory, its use is inconsistent and at times questionable. Malterud et al. (2016) focus instead on the concept of ‘information power’ to determine sample size. Here, they suggest sample size is determined by the amount of information the sample holds relevant to the actual study rather than the number of participants ( Malterud et al., 2016 ). Some guidance on specific sample size depending on research design has been provided in the literature; however, these are sometimes conflicting and in some cases lack evidence to support their claims ( Guest et al., 2006 ). This is further complicated by the range of qualitative designs and data collection approaches available.
Data collection
Data collection methods in qualitative descriptive research are diverse and aim to discover the who, what and where of phenomena ( Sandelowski, 2000 ). Although semi-structured individual face-to-face interviews are the most commonly used data collection approaches ( Kim et al ., 2017 ), focus groups, telephone interviews and online approaches are also used.
Focus groups involve people with similar characteristics coming together in a relaxed and permissive environment to share their thoughts, experiences and insights ( Krueger and Casey, 2009 ). Participants share their own views and experiences, but also listen to and reflect on the experiences of other group members. It is this synergistic process of interacting with other group members that refines individuals’ viewpoints to a deeper and more considered level and produces data and insights that would not be accessible without the interaction found in a group (Finch et al., 2014). Telephone interviews and online approaches are gaining more traction as they offer greater flexibility and reduced costs for researchers and ease of access for participants. In addition, they may help to achieve maximum variation sampling or examine experiences from a national or international perspective. Face-to-face interviews are often perceived as more appropriate than telephone interviews; however, this assumption has been challenged as evidence to support the use of telephone interviews emerges ( Ward et al., 2015 ). Online data collection also offers the opportunity to collect synchronous and asynchronous data using instant messaging and other online media ( Hooley et al., 2011 ). Online interviews or focus groups conducted via Skype or other media may overcome some of the limitations of telephone interviews, although observation of non-verbal communication may be more difficult to achieve ( Janghorban et al., 2014 ). Open-ended free-text responses in surveys have also been identified as useful data sources in qualitative descriptive studies ( Kim et al . , 2017 ) and in particular the use of online open-ended questions, which can have a large geographical reach ( Seixas et al., 2018 ). Observation is also cited as an approach to data collection in qualitative descriptive research ( Sandelowski, 2000 ; Lambert and Lambert, 2012 ); however, in a systematic review examining the characteristics of qualitative research studies, observation was cited as an additional source of data and was not used as a primary source of data collection ( Kim et al. , 2017 ).
Data analysis and interpretation
According to Lambert and Lambert (2012) , data analysis in qualitative descriptive research is data driven and does not use an approach that has emerged from a pre-existing philosophical or epistemological perspective. Within qualitative descriptive research, it is important analysis is kept at a level at which those to whom the research pertains are easily able to understand and so can use the findings in healthcare practice ( Chafe, 2017 ). The approach to analysis is dictated by the aims of the research and as qualitative descriptive research is generally explorative, inductive approaches will commonly need to be applied although deductive approaches can also be used ( Kim et al . , 2017 ).
Content and thematic analyses are the most commonly used data analysis techniques in qualitative descriptive research. Vaismoradi et al . (2013) argue that content and thematic analysis, although poorly understood and unevenly applied, offer legitimate ways of a lower level of interpretation that is often required in qualitative descriptive research. Sandelowski (2000) indicated that qualitative content analysis is the approach of choice in descriptive research; however, confusion exists between content and thematic analysis, which sometimes means researchers use a combination of the two. Vaismoradi et al. (2013) argue there are differences between the two and that content analysis allows the researchers to analyse the data qualitatively as well as being able to quantify the data whereas thematic analysis provides a purely qualitative account of the data that is richer and more detailed. Decisions to use one over the other will depend on the aims of the study, which will dictate the depth of analysis required. Although there is a range of analysis guidelines available, they share some characteristics and an overview of these, derived from some key texts ( Sandleowski, 2010 ; Braun and Clark, 2006 ; Newell and Burnard, 2006), is presented in Table 1 . Central to these guidelines is an attempt by the researcher to immerse themselves in the data and the ability to demonstrate a consistent and systematic approach to the analysis.
Common characteristics of descriptive qualitative analysis.
Coding in qualitative descriptive research can be inductive and emerge from the data, or a priori where they are based on a pre-determined template as in template analysis. Inductive codes can be ‘in vivo’ where the researcher uses the words or concepts as stated by the participants ( Howitt, 2019 ), or can be named by the researcher and grouped together to form emerging themes or categories through an iterative systematic process until the final themes emerge. Template analysis involves designing a coding template, which is designed inductively from a subset of the data and then applied to all the data and refined as appropriate ( King, 2012 ). It offers a standardised approach that may be useful when several researchers are involved in the analysis process.
Within qualitative research studies generally, the analysis of data and subsequent presentation of research findings can range from studies with a relatively minimal amount of interpretation to those with high levels of interpretation ( Sandelowski and Barroso, 2003 ). The degree of interpretation required in qualitative descriptive research is contentious. Sandelowski (2010) argues that although descriptive research produces findings that are ‘data-near’, they are nevertheless interpretative. Sandelowski (2010) reports that a common misconception in qualitative descriptive designs is that researchers do not need to include any level of analysis and interpretation and can rely solely on indiscriminately selecting direct quotations from participants to answer the research question(s). Although it is important to ensure those familiar with the topic under investigation can recognise their experiences in the description of it ( Kim et al . , 2017 ), this is not to say that there should be no transformation of data. Researchers using a qualitative descriptive design need to, through data analysis, move from un-interpreted participant quotations to interpreted research findings, which can still remain ‘data-near’ ( Sandeklwoski, 2010 ). Willis et al. (2016) suggest that researchers using the qualitative descriptive method might report a comprehensive thematic summary as findings, which moves beyond individual participant reports by developing an interpretation of a common theme. The extent of description and/or interpretation in a qualitative descriptive study is ultimately determined by the focus of the study (Neergard et al ., 2009).
As with any research design, ensuring the rigor or trustworthiness of findings from a qualitative descriptive study is crucial. For a more detailed consideration of the quality criteria in qualitative studies, readers are referred to the seminal work of Lincoln and Guba (1985) in which the four key criteria of credibility, dependability, confirmability and transferability are discussed. At the very least, researchers need to be clear about the methodological decisions taken during the study so readers can judge the trustworthiness of the study and ultimately the findings ( Hallberg, 2013 ). Being aware of personal assumptions and the role they play in the research process is also an important quality criterion (Colorafi and Evans, 2016) and these assumptions can be made explicit through the use of researcher reflexivity in the study ( Bradshaw et al., 2017 ).
Challenges in using a qualitative descriptive design
One of the challenges of utilising a qualitative descriptive design is responding to the charge that many qualitative designs have historically encountered, which is that qualitative designs lack the scientific rigor associated with quantitative approaches ( Vaismoradi et al . , 2013 ). The descriptive design faces further critique in this regard as, unlike other qualitative approaches such as phenomenology or grounded theory, it is not theory driven or oriented ( Neergaard et al ., 2009 ). However, it is suggested that this perceived limitation of qualitative descriptive research only holds true if it is used for the wrong purposes and not primarily for describing the phenomenon ( Neergaard et al ., 2009 ). Kahlke (2014) argues that rather than being atheoretical, qualitative descriptive approaches require researchers to consider to what extent theory will inform the study and are sufficiently flexible to leave space for researchers to utilise theoretical frameworks that are relevant and inform individual research studies. Kim et al. (2017) reported that most descriptive studies reviewed did not identify a theoretical or philosophical framework, but those that did used it to inform the development of either the interview guide or the data analysis framework, thereby identifying the potential use of theory in descriptive designs.
Another challenge around the use of qualitative descriptive research is that it can erroneously be seen as a ‘quick fix’ for researchers who want to employ qualitative methods, but perhaps lack the expertise or familiarity with qualitative research ( Sandelowski, 2010 ). Kim et al. (2017) report how in their review fewer than half of qualitative descriptive papers explicitly identified a rationale for choosing this design, suggesting that in some cases the rationale behind its use was ill considered. Providing a justification for choosing a particular research design is an important part of the research process and, in the case of qualitative descriptive research, a clear justification can offset concerns that a descriptive design was an expedient rather than a measured choice. For studies exploring participants’ experiences, which could be addressed using other qualitative designs, it also helps to clearly make a distinction as to why a descriptive design was the best choice for the research study ( Kim et al ., 2017 ). Similarly, there is a perception that the data analysis techniques most commonly associated with descriptive research – thematic and content analysis are the ‘easiest’ approaches to qualitative analysis; however, as Vaismoradi et al . (2013) suggest, this does not mean they produce low-quality research findings.
As previously identified, a further challenge with the use of qualitative descriptive methods is that as a research design it has limited visibility in research texts and methodological papers ( Kim et al ., 2017 ). This means that novice qualitative researchers have little guidance on how to design and implement a descriptive study as there is a lack of a ‘methodological rulebook’ to guide researchers ( Kahlke, 2014 ). It is also suggested that this lack of strict boundaries and rules around qualitative descriptive research also offers researchers flexibility to design a study using a variety of data collection and analysis approaches that best answer the research question ( Kahlke, 2014 ; Kim et al . , 2017 ). However, should researchers choose to integrate methods ‘borrowed’ from other qualitative designs such as phenomenology or grounded theory, they should do so with the caveat that they do not claim they are using designs they are not actually using ( Neergaard et al . , 2009 ).
Examples of the use of qualitative descriptive research in healthcare
Findings from qualitative descriptive studies within healthcare have the potential to describe the experiences of patients, families and health providers, inform the development of health interventions and policy and promote health and quality of life ( Neergaard et al ., 2009 ; Willis et al ., 2016 ). The examples provided here demonstrate different ways qualitative descriptive methods can be used in a range of healthcare settings.
Simon et al. (2015) used a qualitative descriptive design to identify the perspectives of seriously ill, older patients and their families on the barriers and facilitators to advance care planning. The authors provided a rationale for using a descriptive design, which was to gain a deeper understanding of the phenomenon under investigation. Data were gathered through nine open-ended questions on a researcher-administered questionnaire. Responses to all questions were recorded verbatim and transcribed. Using descriptive, interpretative and explanatory coding that transformed raw data recorded from 278 patients and 225 family members to more abstract ideas and concepts ( Simon et al. , 2015 ), a deeper understanding of the barriers and facilitators to advance care planning was developed. Three categories were developed that identified personal beliefs, access to doctors and interaction with doctors as the central barriers and facilitators to advance care planning. The use of a qualitative descriptive design facilitated the development of a schematic based on these three themes, which provides a framework for use by clinicians to guide improvement in advance care planning.
Focus group interviews are a common data collection method in qualitative descriptive studies and were the method of choice in a study by Pelentsov et al. (2015), which sought to identify the supportive care needs of parents whose child has a rare disease. The rationale provided for using a qualitative descriptive design was to obtain a ‘straight description of the phenomena’ and to provide analysis and interpretation of the findings that remained data-near and representative of the responses of participants. In this study, four semi-structured focus group interviews were conducted with 23 parents. The data from these focus groups were then subjected to a form of thematic analysis during which emerging theories and inferences were identified and organised into a series of thematic networks and ultimately into three global themes. These themes identified that a number of factors including social isolation and lack of knowledge on behalf of healthcare professionals significantly affected how supported parents felt. Identifying key areas of the supportive needs of parents using qualitative description provides direction to health professionals on how best to respond to and support parents of children with a rare disease.
The potential for findings from a qualitative descriptive study to impact on policy was identified in a study by Syme et al. (2016) , who noted a lack of guidance and policies around sexual expression management of residents in long-term care settings. In this study, 20 directors of nursing from long-term care settings were interviewed with a view to identifying challenges in addressing sexual expression in these settings and elicit their recommendations for addressing these challenges in practice and policy. Following thematic analysis, findings relating to what directors of nursing believed to be important components of policy to address sexual expression were identified. These included providing educational resources, having a person-centred care delivery model when responding to sexual expression and providing guidance when working with families. Findings from this qualitative descriptive study provide recommendations that can then feed in to a broader policy on sexual expression in long-term care settings.
The final example of the use of a qualitative descriptive study comes from a mixed-methods study comprising a randomised control trial and a qualitative process evaluation. He et al. (2015) sought to determine the effects of a play intervention for children on parental perioperative anxiety and to explore parents’ perceptions of the intervention. Parents who had children going for surgery were assigned to a control group or an intervention group. The intervention group took part in a 1-hour play therapy session with their child whereas the control group received usual care. Quantitative findings identified there was no difference in parents’ anxiety levels between the intervention and control group. However, qualitative findings identified that parents found the intervention helpful in preparing both themselves and their child for surgery and perceived a reduction in their anxiety about the procedure thereby capturing findings that were not captured by the quantitative measures. In addition, in the qualitative interviews, parents made suggestions about how the play group could be improved, which provides important data for the further development of the intervention.
These examples across a range of healthcare settings provide evidence of the way findings from qualitative descriptive research can be directly used to more fully understand the experiences and perspectives of patients, their families and healthcare providers in addition to guiding future healthcare practice and informing further research.
Qualitative research designs have made significant contributions to the development of nursing and healthcare practices and policy. The utilisation of qualitative descriptive research is common within nursing research and is gaining popularity with other healthcare professions. This paper has identified that the utilisation of this design can be particularly relevant to nursing and healthcare professionals undertaking a primary piece of research and provides an excellent method to address issues that are of real clinical significance to them and their practice setting. However, the conundrum facing researchers who wish to use this approach is its lack of visibility and transparency within methodological papers and texts, resulting in a deficit of available information to researchers when designing such studies. By adding to the existing knowledge base, this paper enhances the information available to researchers who wish to use the qualitative descriptive approach, thus influencing the standard in how this approach is employed in healthcare research. We highlight the need for researchers using this research approach to clearly outline the context, theoretical framework and concepts underpinning it and the decision-making process that informed the design of their qualitative descriptive study including chosen research methods, and how these contribute to the achievement of the study’s aims and objectives. Failure to describe these issues may have a negative impact on study credibility. As seen in our paper, qualitative descriptive studies have a role in healthcare research providing insight into service users and providers’ perceptions and experiences of a particular phenomenon, which can inform healthcare service provision.
Key points for policy, practice and/or research
Despite its widespread use, there is little methodological guidance to orientate novice nurse researchers when using the qualitative descriptive design. This paper provides this guidance and champions the qualitative descriptive design as appropriate to explore research questions that require accessible and understandable findings directly relevant to healthcare practice and policy.
This paper identifies how the use of a qualitative descriptive design gives direct voice to participants including patients and healthcare staff, allowing exploration of issues of real and immediate importance in the practice area.
This paper reports how within qualitative descriptive research, the analysis of data and presentation of findings in a way that is easily understood and recognised is important to contribute to the utilisation of research findings in nursing practice.
As this design is often overlooked in research texts despite its suitability to exploring many healthcare questions, this paper adds to the limited methodological guidance and has utility for researchers who wish to defend their rationale for the use of the qualitative descriptive design in nursing and healthcare research.
Louise Doyle (PhD, MSc, BNS, RNT, RPN) is an Associate Professor in Mental Health Nursing at the School of Nursing and Midwifery, Trinity College Dublin. Her research interests are in the area of self-harm and suicide and she has a particular interest and expertise in mixed-methods and qualitative research designs.
Catherine McCabe (PhD, MSc, BNS, RNT, RGN) is an Associate Professor in General Nursing at the School of Nursing and Midwifery, Trinity College Dublin. Her research interests and expertise are in the areas of digital health (chronic disease self-management and social/cultural wellbeing), cancer, dementia, arts and health and systematic reviews.
Brian Keogh (PhD, MSc, BNS, RNT, RPN) is an Assistant Professor in Mental Health Nursing at the School of Nursing and Midwifery, Trinity College Dublin. His main area of research interest is mental health recovery and he specialises in qualitative research approaches with a particular emphasis on grounded theory.
Annemarie Brady (PhD, MSc, BNS, RNT, RPN) is Chair of Nursing and Chronic Illness and Head of School of Nursing and Midwifery at Trinity College Dublin. Her research work has focused on the development of healthcare systems and workforce solutions to respond to increased chronic illness demands within healthcare. She has conducted a range of mixed-method research studies in collaboration with health service providers to examine issues around patient-related outcomes measures, workload measurement, work conditions, practice development, patient safety and competency among healthcare workers.
Margaret McCann (PhD, MSc, BNS, RNT, RGN) is an Assistant Professor in General Nursing at the School of Nursing and Midwifery, Trinity College Dublin. Research interests are focused on chronic illness management, the use of digital health and smart technology in supporting patient/client education, self-management and independence. Other research interests include conducting systematic reviews, infection prevention and control and exploring patient outcomes linked to chronic kidney disease.
Declaration of conflicting interests
The author(s) declared no potential conflicts of interest with respect to the research, authorship and/or publication of this article.
Ethical approval was not required for this paper as it is a methodological paper and does not report on participant data.
The author(s) received no financial support for the research, authorship and/or publication of this article.
Louise Doyle https://orcid.org/0000-0002-0153-8326
Margaret McCann https://orcid.org/0000-0002-7925-6396
- Bishop FL. (2015) Using mixed methods in health research: Benefits and challenges . British Journal of Health Psychology 20 : 1–4. [ Abstract ] [ Google Scholar ]
- Bradshaw C, Atkinson S, Doody O. (2017) Employing a qualitative description approach in health care research . Global Qualitative Nursing Research 4 : 1–8. [ Europe PMC free article ] [ Abstract ] [ Google Scholar ]
- Braun V, Clarke V. (2006) Using thematic analysis in psychology . Qualitative Research in Psychology 3 : 77–101. [ Google Scholar ]
- Caelli K, Ray L, Mill J. (2003) ‘Clear as mud’: Toward greater clarity in generic qualitative research . International Journal of Qualitative Methods 2 : 1–13. [ Google Scholar ]
- Chafe R. (2017) The Value of Qualitative Description in Health Services and Policy Research . Healthcare Policy 12 : 12–18. [ Europe PMC free article ] [ Abstract ] [ Google Scholar ]
- Doyle L, Brady AM, Byrne G. (2016) An overview of mixed methods research–revisited . Journal of Research in Nursing 21 : 623–635. [ Google Scholar ]
- Finch H, Lewis J, Turley C. Ritchie J, Lewis J, McNaughton Nicholls C, Ormston R, editors. Focus Groups . Qualitative Research Practice. A Guide for Social Science Students and Researchers , London: Sage, pp. 211–242. [ Google Scholar ]
- Fusch PI, Ness LR. (2015) Are we there yet? Data saturation in qualitative research . The Qualitative Report 20 : 1408–1416. [ Google Scholar ]
- Guest G, Bunce A, Johnson L. (2006) How many interviews are enough? An experiment with data saturation and variability . Field Methods 18 : 59–82. [ Google Scholar ]
- Hallberg L. (2013) Quality criteria and generalization of results from qualitative studies . International Journal of Qualitative Studies in Health and Well-being 8 : 1. [ Europe PMC free article ] [ Abstract ] [ Google Scholar ]
- He HG, Zhu LX, Chan WCS, et al.(2015) A mixed-method study of effects of a therapeutic play intervention for children on parental anxiety and parents’ perceptions of the intervention . Journal of Advanced Nursing 71 ( 7 ): 1539–1551. [ Abstract ] [ Google Scholar ]
- Hooley T, Wellens J, Marriott J. (2011) What is Online research? Using the Internet for Social Science Research , London: Bloomsbury Academic. [ Google Scholar ]
- Howitt D. (2019) Introduction to Qualitative Methods in Psychology: Putting Theory into Practice , (4th edition). Harlow: Pearson Education Limited. [ Google Scholar ]
- Janghorban R, Roudsari RL, Taghipour A. (2014) Skype interviewing: The new generation of online synchronous interview in qualitative research . International Journal of Qualitative Studies on Health and Wellbeing 9 . [ Europe PMC free article ] [ Abstract ] [ Google Scholar ]
- Kahlke RM. (2014) Generic qualitative approaches: Pitfalls and benefits of methodological mixology . International Journal of Qualitative Methods 13 : 37–52. [ Google Scholar ]
- Kim H, Sefcik JS, Bradway C. (2017) Characteristics of qualitative descriptive studies: A systematic review . Research in Nursing & Health 40 : 23–42. [ Europe PMC free article ] [ Abstract ] [ Google Scholar ]
- King N. (2012) Doing Template Analysis . In: Symon G, Cassell C, editors. (eds) Qualitative Organizational Research: Core Methods and Current Challenges , Los Angeles, CA: Sage. [ Google Scholar ]
- Krueger RA, Casey MA. (2009) Focus Groups: A Practical Guide for Applied Research , 4th ed. Thousand Oaks, CA: Sage. [ Google Scholar ]
- Lambert VA, Lambert CE. (2012) Qualitative descriptive research: An acceptable design . Pacific Rim International Journal of Nursing Research 16 : 255–256. [ Google Scholar ]
- Lincoln YS, Guba EG. (1985) Naturalistic Inquiry , Newbury Park, CA: Sage. [ Google Scholar ]
- Lincoln YS, Lynham SA, Guba EG. (2017) Paradigmatic Controversies, Contradictions and Emerging Confluences . In: NK Denzin, YS Guba, editors. (ed) The Sage Handbook of Qualitative Research , (5th edition). Thousand Oaks, CA: Sage. [ Google Scholar ]
- Long KM, McDermott F, Meadows GN. (2018) Being pragmatic about healthcare complexity: Our experiences applying complexity theory and pragmatism to health services research . BMC Medicine 16 : 94. [ Europe PMC free article ] [ Abstract ] [ Google Scholar ]
- Malterud K, Siersma VD, Guassora AD. (2016) Sample size in qualitative interview studies: Guided by information power . Qualitative Health Research 26 ( 13 ): 1753–1760. [ Abstract ] [ Google Scholar ]
- Neergaard MA, Olesen F, Andersen RS, et al.(2009) Qualitative description – the poor cousin of health research? BMC Medical Research Methodology 9 . [ Europe PMC free article ] [ Abstract ] [ Google Scholar ]
- Newell R, Burnard P. (2011) Research for Evidence Based Practice , Oxford: Blackwell Publishing. [ Google Scholar ]
- O’Reilly M, Parker N. (2012) ‘Unsatisfactory Saturation’: A critical exploration of the notion of saturated sample sizes in qualitative research . Qualitative Research 13 ( 2 ): 190–197. [ Google Scholar ]
- Ormston R, Spencer L, Barnard M, et al.(2014) The foundations of qualitative research . In: Ritchie J, Lewis J, McNaughton Nicholls C, Ormston R, editors. (eds) Qualitative Research Practice. A Guide for Social Science Students and Researchers , London: Sage, pp. 1–25. [ Google Scholar ]
- Palinkas LA, Horwitz SM, Green CA, et al.(2015) Purposeful sampling for qualitative data collection and analysis in mixed method implementation research . Administration and Policy in Mental Health and Mental Health Services Research 42 : 533–544. [ Europe PMC free article ] [ Abstract ] [ Google Scholar ]
- Pelentsov LL, Fielder AL, Esterman AJ. (2016) The supportive care needs of parents with a child with a rare disease: A qualitative descriptive study . Journal of Pediatric Nursing 31 ( 3 ): e207–e218. [ Abstract ] [ Google Scholar ]
- Ritchie J, Lewis J, Elam G, et al.(2014) Designing and selecting samples . In: Ritchie J, Lewis J, McNaughton Nicholls C, Ormston R, editors. (eds) Qualitative Research Practice. A Guide for Social Science Students and Researchers , London: Sage, pp. 111–145. [ Google Scholar ]
- Sandelowski M. (2000) Whatever happened to qualitative description? Research in Nursing & Health 23 : 334–340. [ Abstract ] [ Google Scholar ]
- Sandelowski M. (2010) What’s in a name? Qualitative description revisited . Research in Nursing & Health 33 : 77–84. [ Abstract ] [ Google Scholar ]
- Sandelowski M, Barroso J. (2003) Classifying the findings in qualitative studies . Qualitative Health Research 13 : 905–923. [ Abstract ] [ Google Scholar ]
- Seixas BV, Smith N, Mitton C. (2018) The qualitative descriptive approach in international comparative studies: Using online qualitative surveys . International Journal of Health Policy Management 7 ( 9 ): 778–781. [ Europe PMC free article ] [ Abstract ] [ Google Scholar ]
- Simon J, Porterfield P, Bouchal SR, et al.(2015) ‘Not yet’ and ‘just ask’: Barriers and facilitators to advance care planning – a qualitative descriptive study of the perspectives of seriously ill, older patients and their families . BMJ Supportive & Palliative Care 5 : 54–62. [ Abstract ] [ Google Scholar ]
- Syme ML, Lichtenberg P, Moye J. (2016) Recommendations for sexual expression management in long-term care: A qualitative needs assessment . Journal of Advanced Nursing 72 ( 10 ): 2457–2467. [ Europe PMC free article ] [ Abstract ] [ Google Scholar ]
- Teddlie C, Yu F. (2007) Mixed methods sampling: A typology with examples . Journal of Mixed Methods Research 1 : 77–100. [ Google Scholar ]
- Vaismoradi M, Turunen H, Bondas T. (2013) Content analysis and thematic analysis: Implications for conducting a qualitative descriptive study . Nursing & Health Sciences 15 : 398–405. [ Abstract ] [ Google Scholar ]
- Ward K, Gott M, Hoare K. (2015) Participants’ views of telephone interviews within a grounded theory study . Journal of Advanced Nursing 71 : 2775–2785. [ Abstract ] [ Google Scholar ]
- Willis DG, Sullivan-Bolyai S, Knafl K, et al.(2016) Distinguishing features and similarities between descriptive phenomenological and qualitative descriptive research . Western Journal of Nursing Research 38 : 1185–1204. [ Abstract ] [ Google Scholar ]
Full text links
Read article at publisher's site: https://doi.org/10.1177/1744987119880234
Citations & impact
Impact metrics, citations of article over time, alternative metrics.

Article citations
Life course perspectives of aging with schizophrenia spectrum disorders in psychiatric and long-term care facilities..
Walker VG , Harrison TC
Gerontologist , 64(5):gnad149, 01 May 2024
Cited by: 0 articles | PMID: 37930229
Sustaining the nursing workforce - exploring enabling and motivating factors for the retention of returning nurses: a qualitative descriptive design.
Yamamoto K , Nasu K , Nakayoshi Y , Takase M
BMC Nurs , 23(1):248, 17 Apr 2024
Cited by: 0 articles | PMID: 38627792 | PMCID: PMC11022350
Healthcare team resilience during COVID-19: a qualitative study.
Ambrose JW , Catchpole K , Evans HL , Nemeth LS , Layne DM , Nichols M
BMC Health Serv Res , 24(1):459, 12 Apr 2024
Cited by: 0 articles | PMID: 38609968 | PMCID: PMC11010334
Comprehension of informed consent and voluntary participation in registration cohorts for phase IIb HIV vaccine trial in Dar Es Salaam, Tanzania: a qualitative descriptive study.
Iseselo MK , Tarimo EAM
BMC Med Ethics , 25(1):29, 13 Mar 2024
Cited by: 0 articles | PMID: 38481301 | PMCID: PMC10935914
Experiences of caring for women with cervical cancer: A qualitative study among male partners in Dar es Salaam, Tanzania.
Gosse RA , Msengi EA , Chona EZ , Ambikile JS
Health Expect , 27(2):e14038, 01 Apr 2024
Cited by: 1 article | PMID: 38561909 | PMCID: PMC10985225
Similar Articles
To arrive at the top five similar articles we use a word-weighted algorithm to compare words from the Title and Abstract of each citation.
Avoiding and identifying errors in health technology assessment models: qualitative study and methodological review.
Chilcott J , Tappenden P , Rawdin A , Johnson M , Kaltenthaler E , Paisley S , Papaioannou D , Shippam A
Health Technol Assess , 14(25):iii-iv, ix-xii, 1-107, 01 Jan 2010
Cited by: 29 articles | PMID: 20501062
Review Books & documents Free full text in Europe PMC
The Effectiveness of Integrated Care Pathways for Adults and Children in Health Care Settings: A Systematic Review.
Allen D , Gillen E , Rixson L
JBI Libr Syst Rev , 7(3):80-129, 01 Jan 2009
Cited by: 20 articles | PMID: 27820426
The future of Cochrane Neonatal.
Soll RF , Ovelman C , McGuire W
Early Hum Dev , 150:105191, 12 Sep 2020
Cited by: 5 articles | PMID: 33036834
Sampling issues in qualitative research.
Higginbottom GM
Nurse Res , 12(1):7-19, 01 Jan 2004
Cited by: 78 articles | PMID: 15493211
Using qualitative Health Research methods to improve patient and public involvement and engagement in research.
Rolfe DE , Ramsden VR , Banner D , Graham ID
Res Involv Engagem , 4:49, 13 Dec 2018
Cited by: 35 articles | PMID: 30564459 | PMCID: PMC6293564
Europe PMC is part of the ELIXIR infrastructure
This paper is in the following e-collection/theme issue:
Published on 24.4.2024 in Vol 8 (2024)
Patient Satisfaction With the Health Care Services of a Government-Financed Health Protection Scheme in Bangladesh: Cross-Sectional Study
Authors of this article:

Original Paper
- Md Zahid Hasan 1, 2 , MSS ;
- Md Golam Rabbani 1 , MSS ;
- Orin Akter 1 , MSS ;
- Gazi Golam Mehdi 1 , MSS ;
- Mohammad Wahid Ahmed 1 , MSS ;
- Sayem Ahmed 3 , PhD ;
- Mahbub Elahi Chowdhury 1 , PhD
1 Health Systems and Population Studies Division, icddr,b, Dhaka, Bangladesh
2 Leeds Institute of Health Sciences, University of Leeds, Leeds, United Kingdom
3 Health Economics and Health Technology Assessment, School of Health & Wellbeing, University of Glasgow, Glasgow, United Kingdom
Corresponding Author:
Md Zahid Hasan, MSS
Health Systems and Population Studies Division
68, Shaheed Tajuddin Ahmed Sarani
Dhaka, 1212
Phone: 880 01673163613
Email: [email protected]
Background: Since 2016, the government of Bangladesh has been piloting a health protection scheme known as Shasthyo Surokhsha Karmasuchi (SSK), which specifically targets households living below the poverty line. This noncontributory scheme provides enrolled households access to inpatient health care services for 78 disease groups. Understanding patients’ experiences with health care utilization from the pilot SSK scheme is important for enhancing the quality of health care service delivery during the national-level scale-up of the scheme.
Objective: We aimed to evaluate patient satisfaction with the health care services provided under the pilot health protection scheme in Bangladesh.
Methods: A cross-sectional survey was conducted with the users of the SSK scheme from August to November 2019. Patients who had spent a minimum of 2 nights at health care facilities were selected for face-to-face exit interviews. During these interviews, we collected information on patients’ socioeconomic characteristics, care-seeking experiences, and level of satisfaction with various aspects of health care service delivery. To measure satisfaction, we employed a 5-point Likert scale (very satisfied, 5; satisfied, 4; neither satisfied nor dissatisfied, 3; dissatisfied, 2; very dissatisfied, 1). Descriptive statistics, statistical inferential tests ( t -test and 1-way ANOVA), and linear regression analyses were performed.
Results: We found that 55.1% (241/438) of users were either very satisfied or satisfied with the health care services of the SSK scheme. The most satisfactory indicators were related to privacy maintained during diagnostic tests (mean 3.91, SD 0.64), physicians’ behaviors (mean 3.86, SD 0.77), services provided at the registration booth (mean 3.86, SD 0.62), confidentiality maintained regarding diseases (mean 3.78, SD 0.72), and nurses’ behaviors (mean 3.60, SD 0.83). Poor satisfaction was identified in the interaction of patients with providers about illness-related information (mean 2.14, SD 1.40), availability of drinking water (mean 1.46, SD 0.76), cleanliness of toilets (mean 2.85, SD 1.04), and cleanliness of the waiting room (mean 2.92, SD 1.09). Patient satisfaction significantly decreased by 0.20 points for registration times of 16-30 minutes and by 0.32 points for registration times of >30 minutes compared with registration times of ≤15 minutes. Similarly, patient satisfaction significantly decreased with an increase in the waiting time to obtain services. However, the satisfaction of users significantly increased if they received a complete course of medicines and all prescribed diagnostic services.
Conclusions: More than half of the users were satisfied with the services provided under the SSK scheme. However, there is scope for improving user satisfaction. To improve the satisfaction level, the SSK scheme implementation authorities should pay attention to reducing the registration time and waiting time to obtain services and improving the availability of drugs and prescribed diagnostic services. The authorities should also ensure the supply of drinking water and enhance the cleanliness of the facility.
Introduction
Globally, more than half of the population encounters difficulties in accessing essential health care services, with the majority residing in low- and middle-income countries (LMICs) [ 1 ]. These nations experience substantial challenges in financing health care [ 2 - 5 ]. Consequently, health care financing in these countries heavily relies on out-of-pocket spending by households, leading to increased financial distress on families during their illness [ 2 , 3 , 6 ]. In many instances, the most affected are those in poverty, and they lack access to health care services when they are unwell [ 7 ]. Similar to other LMICs, out-of-pocket spending for health care in Bangladesh is notably high. Recent evidence indicates that 68.5% of the total health care expenditure is shouldered by households through out-of-pocket payments [ 8 ]. Another recent study reported that such high out-of-pocket payments resulted in 24.6% of households experiencing catastrophic health expenditure when estimated using the 10% threshold of the budget share method. Furthermore, in 2016, over 8.5 million people were pushed into poverty due to health care expenses [ 9 ]. Moreover, the incidence of catastrophic health expenditure is more concentrated among the poorest households (16.5%) compared to the richest (9.2%) [ 10 ]. To reduce the burden of health care among the population and progress toward universal health coverage, the Government of Bangladesh has developed the Health Care Financing Strategy 2012–2032, intending to provide financial protection for health care to all citizens by 2032 [ 11 ]. As a component of this strategy, the Health Economics Unit of the Ministry of Health and Family Welfare of the Government of Bangladesh has been implementing a social health protection scheme known as “Shasthyo Surokhsha Karmasuchi (SSK)” since 2016. Although there is a comprehensive plan to cover the entire population of the country within the financing scheme, the current implementation is limited to a noncontributory scheme focusing on the below-poverty-line population. The scheme is being piloted in 3 subdistricts: Kalihati, Madhupur, and Ghatail under Tangail District. The scheme has enrolled almost 1,00,000 households that have access to inpatient health care services from Upazila Health Complexes (UzHCs) of the respective Upazilas (subdistricts) and district hospitals. Participation in the scheme is mandatory for households identified as being below the poverty line, and their enrollment is noncontributory, meaning that these enrolled households are not required to pay any fees for services. Notably, the scheme does not offer purchasing services to the above-poverty-line population. The government established a pool fund, allocating BDT 1000 (US$12) per household per year as a premium (BDT 84.5 = US$ 1, August 2019, Bangladesh Bank). This measure ensures access to inpatient health care services for the enrolled below-poverty-line households, covering 78 different disease groups. The annual coverage limit for each household is BDT 50,000 (US $592). Under the scheme, inpatient health care is delivered through UzHCs, serving as the first access point for the insured beneficiaries to receive health care services. Through a structured referral system, the beneficiaries can also access services at the Tangail District Hospital. The scheme ensures that insured patients receive free diagnostic services and medicines through hospitals, contracted diagnostic centers, and pharmacies. The SSK management authority, scheme operator, hospitals, contracted diagnostic centers, and pharmacies play crucial roles in the implementation of the scheme [ 12 ].
Although the scheme provides free inpatient care services to the member households, the health care utilization under the SSK scheme is notably low. A study revealed that less than half of the beneficiary households used health care services under the SSK scheme [ 13 ]. Several factors may contribute to this low utilization rate. For instance, quality of care might be a significant factor among the various important determinants of health service utilization. Quality of service is recognized as one of the key components in achieving universal health coverage by its definition [ 14 ]. Traditionally, the quality of health care services was primarily assessed based on professional practice standards. However, in the recent decades, patients’ perceptions of health care have emerged as an important indicator for evaluating the quality of health care services. Various studies have demonstrated that health service utilization is closely linked with users’ perceptions of the quality of health care provided [ 15 - 17 ]. Consequently, patient satisfaction is considered as an important aspect of performance improvement of the delivered health care services, alongside clinical effectiveness. It is a multidimensional aspect where patients’ perceptions and attitudes shape their overall health care–seeking experience [ 18 , 19 ]. Several factors, including registration time and process, waiting time to obtain health care services, interpersonal communication, and availability of basic amenities within health care facilities, can influence patient satisfaction with health care services [ 20 - 23 ]. Increased utilization and satisfaction of any insurance scheme are associated with improved quality of health care services. However, the literature provides mixed evidence. For example, a study in India found no significant difference in satisfaction levels between insured and uninsured hospitalized patients [ 24 ]. Conversely, regarding the overall quality of care provided under the National Health Insurance Scheme of Ghana, a significant portion of insured patients reported higher satisfaction compared with uninsured patients [ 25 ]. Evidence from Nigeria indicated that most patients were satisfied with the service delivery of their national health insurance scheme [ 26 - 28 ]. In Ethiopia, a study revealed that approximately 55% of enrollees were satisfied with the community-based health insurance scheme [ 29 ], whereas another study from the same country indicated that over 90% of households were satisfied with the community-based health insurance scheme [ 30 ]. Different Vietnamese studies have reported poor satisfaction among beneficiaries regarding service coverage and quality of care under national health insurance [ 31 , 32 ]. A recent study conducted on a self-financed health insurance scheme in Bangladesh showed that, overall, members of the scheme were satisfied with the health care services; however, their satisfaction levels could be improved in several aspects of health care service delivery [ 33 ].
Despite the pilot implementation of the SSK scheme since 2016 and its low utilization, no research has been conducted on service users’ experiences and levels of satisfaction with the scheme. Gaining a better understanding of beneficiaries’ experiences and levels of satisfaction with the health care service provided under the pilot SSK scheme is crucial. This insight can help identify the gaps in the quality of health care services provided. Such evidence will be useful for the key stakeholders of the health protection scheme, allowing them to make necessary changes in the service delivery process and related aspects to enhance the quality of health care services provided under the scheme. As a result, this study was conducted to address 2 central research questions: (1) What is the level of satisfaction among the beneficiaries of the SSK scheme? and (2) What are the factors influencing their satisfaction level? In addressing these research questions, this study aimed to assess patients’ levels of satisfaction with the services offered by the SSK scheme in Bangladesh.
Study Design
A cross-sectional exit patient survey was designed to gain insights into the experiences of insured patients with various aspects of the service delivery process and the quality of services provided under the pilot SSK scheme. Every second patient who had been admitted for at least 2 nights at a scheme-designated facility was selected and interviewed at the time of discharge.
Study Setting and Sample
The study was conducted in the UzHCs of Kalihati, Madhupur, and Ghatail Upazilas (subdistricts), and Tangail District Hospital of Tangail District. Insured inpatients were interviewed after discharge from the health care facilities. The survey of the respondents took place between August 18 and November 16, 2019, on working days, from Saturday to Thursday. Every second discharged inpatient from the male and female wards was interviewed. To ensure the quality of the data, a maximum of 4 patients were interviewed each day at an SSK hospital. A total of 438 discharged inpatients aged 18 years or older were interviewed from 3 UzHCs (Kalihati, n=128; Madhupur, n=176; and Ghatail, n=134) and Tangail District Hospital (n=88).
Data Collection Process
A semistructured questionnaire was designed and pretested before data collection. Face-to-face interviews were conducted with the insured patients and, in certain cases, with attendants of patients at the time of discharge. An attendant was considered as a respondent when the patient was not involved with the various dimensions of the service delivery process during the inpatient episode owing to the physical condition.
The questionnaire covered demographic and socioeconomic details of the respondents and households, health care utilization, and various dimensions of satisfaction related to the SSK scheme. These dimensions included the registration process at the SSK booth, the dignity of patients during treatment, clear communication with health care providers, privacy during treatment, the quality of basic amenities, the availability of drugs and supplies, and the availability of prescribed diagnostic services.
Four experienced research assistants were employed for patient recruitment and conducting the interviews. Prior to the interviews, written informed consent was obtained from all participants, and their participation was entirely voluntary. Completed interviews were cross-checked among the interviewers and further reviewed by the supervisor to ensure data quality and to address any associated issues, if needed, during the data collection.
Study Variables
We collected information on various background characteristics of the patients, including age, sex, education level, current employment status, current marital status, and family size. For measuring satisfaction levels, we considered several dimensions of health care delivery under the SSK scheme.
The first dimension was hospitalization-related factors. It included self-reported illnesses and length of stay. Self-reported illnesses were categorized into 3 groups: communicable, noncommunicable, and others (ie, obstetrics and injury). Communicable diseases encompass illnesses caused by viruses or bacteria that spread through contact, bodily fluids, blood products, insect bites, or the air. Noncommunicable diseases, on the other hand, are those that do not transmit between individuals and often necessitate long-term treatment.
The second dimension was service utilization–related aspects. It included waiting time for registration, waiting time to obtain health care services, behavior of health care providers (including physicians, nurses, and other staff, such as ward boys and cleaners), interaction of health care providers with patients, privacy during diagnostic services, and confidentiality of the health care provided.
The third dimension was facility environment and basic amenity–related factors. It included cleanliness of health facilities, waiting rooms, and toilets, and availability of drinking water.
The satisfaction measurement items demonstrated a satisfactory level of internal consistency, as indicated by an overall Cronbach α coefficient of 0.77 out of 1.0 [ 34 ].
Satisfaction Measurements
Patient satisfaction was measured with a collective outcome of 14 different items. The selection of items for measurements was devised based on a literature review of patient satisfaction with the insurance scheme as well as previous systematic reviews [ 26 , 27 , 33 , 35 - 40 ]. The existing literature has examined various aspects of health service delivery from the patients’ viewpoints, encompassing domains such as patient-provider interactions, the physical environment, and internal management processes. We selected items that revolved around these domains as they encompassed the most influential satisfaction constructs. The 14 items are presented in Textbox 1 .
Each considered item was rated on a 5-point Likert scale (very satisfied, 5; satisfied, 4; neither satisfied nor dissatisfied, 3; dissatisfied, 2; very dissatisfied, 1). The total satisfaction score of respondents for all items ranged from a minimum of 14 to a maximum of 70. Furthermore, we included an item in the questionnaire to assess the overall satisfaction (on a scale of 5) with the services at the SSK facility.
Satisfaction items
- How will you rate the behavior of the authority of Shasthyo Surokhsha Karmasuchi (SSK) at the registration booth?
- What is your opinion about the time taken for completing registration?
- What is your opinion about the waiting time before consultation with the service provider?
- How will you rate the behavior of the service provider during your treatment at this hospital?
- How will you rate the behavior of nurses during your treatment at this hospital?
- How will you rate the behavior of the aya/ward boy during your treatment at this hospital?
- How will you rate the interaction with the service provider about your illness and treatment?
- How will you rate the doctor’s attitude toward listening to your problems?
- How will you rate the privacy maintained during diagnostic tests?
- What is your opinion about the privacy maintained during consultation?
- What is your opinion about the cleanliness of this hospital?
- How will you rate the cleanliness of the waiting room of this hospital?
- How will you rate the cleanliness of the toilets of this hospital?
- What is your opinion regarding the availability of drinking water in the hospital?
Statistical Analysis
We analyzed the data using Stata version 16 (StataCorp) [ 41 ]. We performed both descriptive analysis and statistical inferential tests to measure the association between dependent and independent variables. In the descriptive analysis, background characteristics of the study participants and health care facility utilization–related characteristics were presented in terms of frequency (n) and percentage (%) with 95% CIs. Moreover, we performed a t -test for variables with 2 categories and 1-way ANOVA for variables with more than 2 categories to test the significant differences in average satisfaction levels across the demographic and socioeconomic characteristics related to the SSK scheme.
To identify factors associated with patients’ average scores for satisfaction with the services under the SSK scheme, a linear regression analysis was performed. We estimated the satisfaction level for each patient by taking the average of the reported satisfaction levels in the 14 items. In the univariate unadjusted regression model, the dependent variable was the mean satisfaction score and the independent variables were age, gender, education, employment status, marital status, family size, self-reported illness, length of hospitalization, registration time, waiting time to obtain services, status of receiving drugs and supplies, and status of receiving diagnostic services. However, in the multivariate regression model, we included independent variables that had a significant association with the satisfaction score (ie, P values ≤.05) in the univariate regression models. We considered P values of <.05 as statistically significant in our analysis.
Ethics Approval
This study was approved by the Research Review Committee and Ethical Review Committee of the icddr,b (protocol#: PR-17047). Participants in the study were recruited and interviewed after obtaining written informed consent, and their participation was voluntary.
Descriptive Statistics
A total of 438 patients aged 18 years and above were interviewed at the studied facilities ( Table 1 ), and 60.1% (263/438) of the patients were female. According to education level, 60.9% (267/438) of patients had no education, whereas 24.2% (106/438) and 14.8% (65/438) had primary and secondary levels of education, respectively. Moreover, 67.8% (297/438) of patients were not involved with income generation. In terms of marital status, 83.3% (365/438) of patients were married. Moreover, 54.1% (237/438) were from a household consisting of more than 4 members.
According to self-reported diseases, 62.6% (274/438) of patients reported the reason for hospitalization as noncommunicable disease, 30.8% (135/438) reported the reason as communicable disease, and 7.0% (29/438) reported the reason as other health problems (ie, obstetrics and injury). Regarding the length of hospitalization, 47.5% (208/438) of patients were admitted for 3-4 days, 34.5% (151/438) were admitted for 2 days, and 18.0% (79/438) were admitted for more than 4 days. Among the respondents, 66.2% (290/438) mentioned that they had completed their registration process within 15 minutes, and 58.9% (258/438) waited for 15 minutes or less to get services. The majority of patients (347/438, 79.2%) received all prescribed medicines and supplies free from the SSK pharmacy. Regarding laboratory services, 74.4% (326/438) of patients reported that they received diagnostic services as prescribed. More details of the descriptive statistics are shown in Table 1 .
Level of Satisfaction by Different Items
Patient satisfaction with the items considered while using the SSK scheme is shown in Table 2 . A total of 14 satisfaction items were used to examine patient satisfaction. The highest average score on satisfaction was related to “privacy maintained during diagnostic tests” (mean 3.91, SD 0.64), followed by “physicians’ behaviors” (mean 3.86, SD 0.77), “services at the SSK registration booth” (mean 3.86, SD 0.62), “confidentiality maintained about diseases” (mean 3.78, SD 0.72), and “services from nurses” (mean 3.6, SD 0.83). Among service-related items, a lower level of satisfaction was reported for the interaction of service providers with patients (mean 2.14, SD 1.4). Among the items in the environment and basic amenities domain, comparatively higher satisfaction was found for the cleanliness of the health facility (mean 3.43, SD 0.76), followed by the cleanliness of the waiting room (mean 2.92, SD 1.09) and toilets (mean 2.85, SD 1.04). The lowest level of satisfaction was reported for the availability of drinking water (mean 1.46, SD 0.76).
a SSK: Shasthyo Surokhsha Karmasuchi.
Overall Patient Satisfaction With Health Care Services at SSK Facilities
Considering the response to the overall patient satisfaction with the services at SSK facilities, 8.5% (37/438) reported being very satisfied and 46.6% (204/438) reported being satisfied with the services received under the SSK scheme. On the other hand, 31.3% (137/438) of respondents reported feeling neither satisfied nor dissatisfied. Moreover, 8.9% (39/438) were dissatisfied and 4.8% (21/438) were very dissatisfied ( Multimedia Appendix 1 ).
Patient Satisfaction by Socioeconomic and Hospital Service Utilization Characteristics
Patient satisfaction levels significantly varied across different groups of age, sex, marital status, illness type, registration time, waiting time, status of receiving drugs, and status of getting diagnostic tests ( Table 3 ). Patients aged between 45 and 64 years were comparatively more satisfied (mean 3.28, 95% CI 3.21-3.34) with services under the SSK scheme, and the difference in the satisfaction level across the age groups was statistically significant ( P <.001). Male patients were significantly ( P =.01) more satisfied (mean 3.24, 95% CI 3.17-3.31) than female patients. Married and widowed, divorced, or separated individuals were more satisfied than unmarried individuals, and the difference was statistically significant ( P <.001). However, there was no significant difference in satisfaction by education level, employment status, or household size.
Patients with noncommunicable diseases had a higher satisfaction level (mean 3.22, 95% CI 3.17-3.28) than patients with other illnesses, and the difference in the satisfaction level was statistically significant ( P =.008). Satisfaction scores decreased with increases in the length of hospitalization, registration time, and waiting time. The satisfaction level was significantly ( P =.006) higher among patients who received all prescribed drugs from the scheme (mean 3.20, 95% CI 3.15-3.26). Similarly, the satisfaction level was higher among patients who received all prescribed diagnostic or laboratory services compared with other groups (mean 3.22, 95% CI 3.17-3.27), and the difference in the satisfaction level across the groups was statistically significant ( P <.001).
a One-way ANOVA.
Determinants of Patient Satisfaction With Services Provided Under the SSK Scheme
Our analysis demonstrated noteworthy associations between satisfaction scores and various factors ( Table 4 ). The satisfaction score was significantly higher by 0.13 points in patients aged between 45 and 64 years than in patients aged between 18 and 44 years. Additionally, the satisfaction score was significantly higher by 0.34 points in married patients than in unmarried patients. Moreover, the satisfaction score was significantly higher by 0.15 points in patients seeking care for noncommunicable diseases than in patients seeking care for communicable diseases. We found a significant negative association of the satisfaction score with extended registration and waiting time for obtaining services. Conversely, a positive association was observed with the status of receiving all drugs, supplies, and diagnostic services. The satisfaction score was significantly lower by 0.18 points in patients with a registration time of 16-30 minutes and by 0.33 points in patients with a registration time of >30 minutes than in patients with a registration time of ≤15 minutes. Similarly, the satisfaction score was significantly lower by 0.30 points in patients who waited for 16-30 minutes to obtain services and by 0.36 points in patients who waited for >30 minutes to obtain services than in patients who waited for ≤15 minutes to obtain services. Moreover, the satisfaction score was significantly higher by 0.13 points in patients who received the complete course of prescribed medicines from the SSK pharmacy than in patients who received partial medicines and supplies. Likewise, the satisfaction score was significantly higher by 0.26 points in patients who received partial diagnostic services and by 0.28 points in patients who received full diagnostic services than in patients who were not prescribed diagnostic services.
a The dependent variable is the average satisfaction score of 14 items.
b The number of observations was 438, R-square value was 0.319, and adjusted R-square value was 0.293.
c N/A: not applicable.
Principal Results and Comparison With Prior Work
We found that 55.1% (241/438) of patients were either very satisfied or satisfied with the services provided by the SSK health protection scheme. The mean satisfaction score was 3.17 out of 5, which means that, on average, the satisfaction level among the patients was slightly above the level of neither satisfied nor dissatisfied. Regarding the 14 considered items for measuring satisfaction, most of the patients were either very satisfied or satisfied with services at the SSK center (368/438, 84.0%), physicians’ behaviors (366/438, 83.6%), and privacy maintained during diagnostic services (297/364, 81.6%). On the other hand, majority of the patients were either very dissatisfied or dissatisfied with the availability of drinking water (409/438, 93.4%) and interaction with health care providers (293/438, 66.9%) regarding the illness. In multiple regression analysis, we found that receiving prescribed drugs and diagnostic services, the waiting time for registration, and the waiting time for getting treatment were the strongest predictors of patient satisfaction.
Health financing schemes are becoming popular to maintain and improve the health of the population in LMICs [ 2 , 6 , 42 ]. The SSK health protection scheme has been introduced to increase the access of the poor population to inpatient health care services and ensure financial protection against expenditure to alleviate poverty or extreme poverty induced by out-of-pocket payments for health care in Bangladesh. Although several studies have been conducted on patient satisfaction with health care utilization in different settings in Bangladesh [ 33 , 43 - 46 ], patient satisfaction with services under the SSK health protection scheme has not been studied thus far. The mean satisfaction score in our study was higher than that in a study conducted to assess satisfaction with the service quality of UzHCs among the uninsured population (3.17 vs 2.75) [ 44 ]. The SSK scheme provides health care to members through selected UzHCs; however, compared with nonmembers, insured patients are supposed to receive all prescribed medicines and diagnostic services from private providers contracted by the scheme [ 13 ]. The situation is different for other UzHCs where the SSK scheme is not being implemented. The availability of medicines and diagnostic services under the SSK scheme might have increased the satisfaction level among the insured patients.
Our study showed that patient satisfaction was the highest regarding the privacy and confidentiality maintained by providers during diagnostic tests and the patients’ diseases. The finding is similar to that in a study conducted in Bangladesh [ 33 ] among the beneficiaries of a community-based health insurance scheme. Another study conducted among adult patients at a general hospital in Ethiopia also reported that patient privacy and confidentiality maintained by health care providers were significantly associated with higher satisfaction levels [ 47 ]. Our study found that patients were satisfied with providers’ behaviors, particularly physicians’ and nurses’ behaviors, which influenced the overall level of patient satisfaction. Although not directly comparable, the proportion of patients satisfied with the behavior of providers was higher than the proportion reported in a study conducted in rural Bangladesh (84% vs 69%) [ 45 ]. Previous studies have also reported that the behavior of health care providers toward patients is directly connected with patient satisfaction [ 33 , 43 , 48 ].
Regarding interactions with health care providers, our study found that two-thirds of patients were not satisfied. This might be the result of patients not knowing about their illnesses from physicians during their treatment episodes. It is evident from the literature that patients’ satisfaction levels are influenced by healthy interpersonal communication with health care providers as this maintains a better physician-patient relationship [ 43 ]. A previous study conducted in Bangladesh showed that more than half of the surveyed patients could not ask questions to their providers about their illness [ 49 ]. However, as all patients in our study were inpatients and stayed at the facility for at least 2 days, it is unlikely that patients could not ask their providers about their illness.
Patient experiences with the cleanliness of health facilities and toilets and the availability of drinking water were not positive. Previous studies revealed that the health facility environment and cleanliness were crucial aspects of patient satisfaction [ 33 , 50 - 52 ]. Moreover, evidence indicates that since environmental contamination is directly connected with nosocomial infection, the physical environment can lead to the dissatisfaction of patients at health facilities instead of increasing satisfaction [ 33 , 50 - 52 ].
We found that patient age was significantly associated with the level of satisfaction. Another study conducted in Bangladesh [ 44 ] reported significant variation in the average satisfaction score across patient age, which is similar to our findings. Two other studies conducted among beneficiaries of health insurance schemes also reported similar findings that age was significantly associated with the level of satisfaction [ 30 , 53 ]. Lower waiting times for registration and health care were significantly associated with patient satisfaction. The findings are consistent with the findings that prolonged waiting times for registration and services are associated with lower client satisfaction [ 54 , 55 ]. Patients who received care for noncommunicable diseases were significantly more satisfied than patients having communicable diseases. This might be because people having noncommunicable diseases require regular medications, which are common and available through the contracted pharmacy. Such availability of medicines might have increased patient satisfaction. Similarly, SSK beneficiaries who received all prescribed medicines and diagnostic services were significantly more satisfied. According to the benefits package of the SSK scheme, patients should receive all prescribed medicines and diagnostic services for 78 disease groups. However, 20.8% (91/438) of patients reported that they received partial medicines and 8.7% (38/438) reported that they received partial diagnostic services. It might have happened that some of the prescribed medicines or diagnostic tests were not correlated with the 78 disease categories and therefore were not provided under the scheme. However, evidence indicates that medicines and diagnostic tests are associated with higher out-of-pocket expenditure and lead to falling into poverty [ 9 , 56 , 57 ]. Scheme beneficiaries are provided free essential medicines and free diagnostic services, and they have a low chance of incurring treatment costs and experience low risks of catastrophic health expenditure, impoverishment, and further impoverishment [ 9 ], thus increasing their satisfaction with the services under the scheme. However, other variables, such as education level, employment status, family size, and length of hospitalization, were not significantly associated with satisfaction levels. This might be because the SSK scheme targets the below-poverty-line population having relatively similar socioeconomic characteristics; thus, their perceptions of satisfaction do not vary across these factors. These findings are consistent with the findings of other studies conducted in India [ 24 ] and Turkey [ 37 ].
This is the first study to explore patient satisfaction with the pilot SSK scheme in Bangladesh. Furthermore, we included patients from all 4 facilities under the SSK scheme rather than selecting them purposively. The findings of this study will help SSK implementation authorities to understand the patient experience of the service delivery process and the quality of health care provided under the SSK scheme.
Limitations
The design of this study was observational in nature, which did not allow us to establish any causal inference with satisfaction and other characteristics under the SSK scheme without a control group. The study only focused on the point of view of the beneficiaries, and we did not explore the providers’ views in this context. The survey collected self-reported satisfaction information from patients, which is highly susceptible to social desirability bias as patients might give responses that please health care providers instead of truly reflecting their satisfaction. However, we interviewed patients at hospital premises in the absence of any providers to minimize such bias.
Conclusions
Our findings demonstrate that more than half of the patients were overall satisfied with the services provided under the SSK scheme. However, there is room for improvement in several dimensions, such as the cleanliness of the waiting room and toilets and the availability of drinking water. Furthermore, attention should be paid to minimizing the waiting time for registration and accessing health care services, and improving providers’ skills on interaction with patients. The results of this study could help stakeholders make necessary changes in the identified determinants of satisfaction related to health service delivery of the SSK scheme. Such changes will enhance the quality of services as well as increase utilization of the scheme in the target population, ultimately advancing progress toward achieving universal health coverage.
Acknowledgments
This study received support from the United States Agency for International Development (USAID) under the terms of USAID’s Research for Decision Makers (RDM) activity cooperative agreement number AID-388-A-17-00006. The views expressed herein do not necessarily reflect the views of the US Government or USAID. The icddr,b acknowledges with gratitude the commitment of the USAID to its research efforts and funding for this study. icddr,b is also thankful to the Governments of Bangladesh, Canada, Sweden, and the United Kingdom for providing core and unrestricted support. Furthermore, the authors are grateful to Health Economics Unit, Ministry of Health and Family Welfare of the Government of Bangladesh, for its cooperation during this study. All authors declared that they had insufficient or no funding to support open access publication of this manuscript, including from affiliated organizations or institutions, funding agencies, or other organizations. JMIR Publications provided article processing fee (APF) support for the publication of this article.
Data Availability
The data sets generated or analyzed during this study are available from the corresponding author upon reasonable request.
Authors' Contributions
MZH, MGR, and MEC contributed to conceptualizing, analyzing, writing, revising, and finalizing the manuscript with the support of OA, SA, GGM, and MWA. All authors have read, revised, and approved the final version of the manuscript.
Conflicts of Interest
None declared.
Overall satisfaction with the inpatient care services under the Shasthyo Surokhsha Karmasuchi (SSK) scheme.
- Thirteenth general programme of work, 2019–2023: promote health, keep the world safe, serve the vulnerable. World Health Organization. URL: https://www.who.int/publications/i/item/thirteenth-general-programme-of-work-2019-2023 [accessed 2024-03-19]
- Asante A, Price J, Hayen A, Jan S, Wiseman V. Equity in Health Care Financing in Low- and Middle-Income Countries: A Systematic Review of Evidence from Studies Using Benefit and Financing Incidence Analyses. PLoS One. 2016;11(4):e0152866. [ FREE Full text ] [ CrossRef ] [ Medline ]
- Puchalski Ritchie LM, Khan S, Moore JE, Timmings C, van Lettow M, Vogel JP, et al. Low- and middle-income countries face many common barriers to implementation of maternal health evidence products. J Clin Epidemiol. Aug 2016;76:229-237. [ FREE Full text ] [ CrossRef ] [ Medline ]
- McIntyre D, Thiede M, Dahlgren G, Whitehead M. What are the economic consequences for households of illness and of paying for health care in low- and middle-income country contexts? Soc Sci Med. Feb 2006;62(4):858-865. [ FREE Full text ] [ CrossRef ] [ Medline ]
- The world health report: health systems financing: the path to universal coverage. World Health Organization. URL: https://apps.who.int/iris/handle/10665/44371 [accessed 2024-03-19]
- Wagstaff A, Eozenou P, Smitz M. Out-of-pocket expenditures on health: A global stocktake. World Bank Res Obs. 2020;35(2):123-157. [ FREE Full text ] [ CrossRef ]
- Poverty and Health. The World Bank. 2014. URL: https://www.worldbank.org/en/topic/health/brief/poverty-health [accessed 2024-03-19]
- Bangladesh National Health Accounts 1997-2020 (BNHA-VI). Dhaka, Bangladesh. Health Economics Unit, Health Services Division, Ministry of Health and Family Welfare; 2022.
- Ahmed S, Ahmed M, Hasan M, Mehdi G, Islam Z, Rehnberg C, et al. Assessing the incidence of catastrophic health expenditure and impoverishment from out-of-pocket payments and their determinants in Bangladesh: evidence from the nationwide Household Income and Expenditure Survey 2016. Int Health. Jan 19, 2022;14(1):84-96. [ FREE Full text ] [ CrossRef ] [ Medline ]
- Khan J, Ahmed S, Evans T. Catastrophic healthcare expenditure and poverty related to out-of-pocket payments for healthcare in Bangladesh-an estimation of financial risk protection of universal health coverage. Health Policy Plan. Oct 01, 2017;32(8):1102-1110. [ CrossRef ] [ Medline ]
- Expanding Social Protection for Health: Towards Universal Coverage, Health Care Financing Strategy 2012-2032. Ministry of Health & Family Welfare. URL: http://oldweb.heu.gov.bd/pdf/Health%20Care%20Financing%20Strategy%202012-2032.pdf [accessed 2024-03-19]
- Ahmed S, Hasan MZ, Ahmed MW, Dorin F, Sultana M, Islam Z, et al. Evaluating the implementation related challenges of Shasthyo Suroksha Karmasuchi (health protection scheme) of the government of Bangladesh: a study protocol. BMC Health Serv Res. Jul 16, 2018;18(1):552. [ FREE Full text ] [ CrossRef ] [ Medline ]
- Hasan MZ, Ahmed MW, Mehdi GG, Khan JAM, Islam Z, Chowdhury ME, et al. Factors affecting the healthcare utilization from Shasthyo Suroksha Karmasuchi scheme among the below-poverty-line population in one subdistrict in Bangladesh: a cross sectional study. BMC Health Serv Res. Jul 08, 2022;22(1):885. [ FREE Full text ] [ CrossRef ] [ Medline ]
- Universal health coverage (UHC). World Health Organization. URL: https://www.who.int/news-room/fact-sheets/detail/universal-health-coverage-(uhc) [accessed 2024-03-19]
- Bhatia J, Cleland J. Health-care seeking and expenditure by young Indian mothers in the public and private sectors. Health Policy Plan. Mar 2001;16(1):55-61. [ CrossRef ] [ Medline ]
- Painter T, Diaby K, Matia D, Lin L, Sibailly T, Kouassi M, et al. Women's reasons for not participating in follow up visits before starting short course antiretroviral prophylaxis for prevention of mother to child transmission of HIV: qualitative interview study. BMJ. Sep 04, 2004;329(7465):543. [ FREE Full text ] [ CrossRef ] [ Medline ]
- Haddad S, Fournier P, Machouf N, Yatara F. What does quality mean to lay people? Community perceptions of primary health care services in Guinea. Soc Sci Med. Aug 1998;47(3):381-394. [ CrossRef ] [ Medline ]
- Cleary P, Edgman-Levitan S, Roberts M, Moloney T, McMullen W, Walker J, et al. Patients evaluate their hospital care: a national survey. Health Aff (Millwood). 1991;10(4):254-267. [ CrossRef ] [ Medline ]
- Woodring S, Polomano RC, Haagen BF, Haack MM, Nunn RR, Miller GL, et al. Development and testing of patient satisfaction measure for inpatient psychiatry care. J Nurs Care Qual. 2004;19(2):137-148. [ CrossRef ] [ Medline ]
- Preyde M, Crawford K, Mullins L. Patients' satisfaction and wait times at Guelph General Hospital Emergency Department before and after implementation of a process improvement project. CJEM. May 11, 2012;14(3):157-168. [ CrossRef ] [ Medline ]
- Abolfotouh M, Al-Assiri M, Alshahrani R, Almutairi Z, Hijazi R, Alaskar A. Predictors of patient satisfaction in an emergency care centre in central Saudi Arabia: a prospective study. Emerg Med J. Jan 2017;34(1):27-33. [ FREE Full text ] [ CrossRef ] [ Medline ]
- Renzi C, Picardi A, Abeni D, Agostini E, Baliva G, Pasquini P, et al. Association of dissatisfaction with care and psychiatric morbidity with poor treatment compliance. Arch Dermatol. Mar 01, 2002;138(3):337-342. [ CrossRef ] [ Medline ]
- Sran M, Pyra T, Chen L, Holroyd B, McCabe C. P116: A scoping review of factors affecting patient satisfaction with care in North American adult emergency departments. CJEM. May 15, 2017;19(S1):S117. [ CrossRef ]
- Devadasan N, Criel B, Van Damme W, Lefevre P, Manoharan S, Van der Stuyft P. Community health insurance schemes and patient satisfaction: evidence from India. Indian J Med Res. Jan 2011;133(1):40-49. [ FREE Full text ] [ Medline ]
- Fenny AP, Enemark U, Asante FA, Hansen KS. Patient satisfaction with primary health care - a comparison between the insured and non-insured under the National Health Insurance Policy in Ghana. Glob J Health Sci. Apr 01, 2014;6(4):9-21. [ FREE Full text ] [ CrossRef ] [ Medline ]
- Daramola OE, Adeniran A, Akande TM. Patients’ satisfaction with the quality of services accessed under the National Health Insurance Scheme at a Tertiary Health Facility in FCT Abuja, Nigeria. Journal of Community Medicine and Primary Health Care. 2018;30(2):90-97. [ FREE Full text ]
- Iloh G, Ofoedu J, Njoku P, Odu F, Ifedigbo C, Iwuamanam K. Evaluation of patients' satisfaction with quality of care provided at the National Health Insurance Scheme clinic of a tertiary hospital in South- Eastern Nigeria. Niger J Clin Pract. 2012;15(4):469-474. [ CrossRef ] [ Medline ]
- Garba M, Gadanya M, Iliyasu Z, Gajida A. Comparative study of patients' satisfaction between national health insurance scheme-insured and un-insured patients attending a Northern Nigerian tertiary hospital. Niger J Basic Clin Sci. 2018;15(2):118. [ CrossRef ]
- Mitiku Kebede K, Geberetsadik SM. Household satisfaction with community-based health insurance scheme and associated factors in piloted Sheko district; Southwest Ethiopia. PLoS One. May 13, 2019;14(5):e0216411. [ FREE Full text ] [ CrossRef ] [ Medline ]
- Badacho AS, Tushune K, Ejigu Y, Berheto TM. Household satisfaction with a community-based health insurance scheme in Ethiopia. BMC Res Notes. Aug 30, 2016;9(1):424. [ FREE Full text ] [ CrossRef ] [ Medline ]
- Le N, Groot W, Tomini SM, Tomini F. Working Paper Series. Accounting & Finance. 1984;24(1):75-75. [ CrossRef ]
- Quynh NTN, Dhar N. A study of satisfaction among poor patients holding health insurance card with health care services at two district public hospitals in Vietnam. Health and Population - Perspectives and Issues. 2014;37:50-56. [ FREE Full text ]
- Sarker AR, Sultana M, Ahmed S, Mahumud RA, Morton A, Khan JAM. Clients' Experience and Satisfaction of Utilizing Healthcare Services in a Community Based Health Insurance Program in Bangladesh. Int J Environ Res Public Health. Aug 02, 2018;15(8):1637. [ FREE Full text ] [ CrossRef ] [ Medline ]
- Taber KS. The Use of Cronbach’s Alpha When Developing and Reporting Research Instruments in Science Education. Res Sci Educ. Jun 7, 2017;48(6):1273-1296. [ CrossRef ]
- Schoenfelder T, Klewer J, Kugler J. Determinants of patient satisfaction: a study among 39 hospitals in an in-patient setting in Germany. Int J Qual Health Care. Oct 2011;23(5):503-509. [ CrossRef ] [ Medline ]
- Ng J, Luk B. Patient satisfaction: Concept analysis in the healthcare context. Patient Educ Couns. Apr 2019;102(4):790-796. [ FREE Full text ] [ CrossRef ] [ Medline ]
- Ali Jadoo SA, Wan Puteh SE, Ahmed Z, Jawdat A. Level of patients' satisfaction toward National Health Insurance in Istanbul City-Turkey. BMC Public Health. Nov 27, 2012;12(S2):A5. [ CrossRef ]
- Manzoor F, Wei L, Hussain A, Asif M, Shah SIA. Patient Satisfaction with Health Care Services; An Application of Physician's Behavior as a Moderator. Int J Environ Res Public Health. Sep 09, 2019;16(18):3318. [ FREE Full text ] [ CrossRef ] [ Medline ]
- Andaleeb SS, Siddiqui N, Khandakar S. Patient satisfaction with health services in Bangladesh. Health Policy Plan. Jul 25, 2007;22(4):263-273. [ CrossRef ] [ Medline ]
- Almeida RSD, Bourliataux-Lajoinie S, Martins M. Satisfaction measurement instruments for healthcare service users: a systematic review. Cad Saude Publica. Jan 2015;31(1):11-25. [ FREE Full text ] [ CrossRef ] [ Medline ]
- StataCorp. Stata Statistical Software: Release 16. College Station, TX. StataCorp LLC; 2019.
- Acharya A, Vellakkal S, Kalita S, Taylor F, Satija A, Burke M, et al. Do Social health insurance schemes in developing country settings improve health outcomes and reduce the impoverishing effect of healthcare payments for the poorest people? UK Government. URL: https://assets.publishing.service.gov.uk/media/57a08b2540f0b652dd000b18/FINAL-Q40-Social-Health-Insurance-Protocol-DFID-LSHTM.pdf [accessed 2024-03-19]
- Adhikary G, Shawon MSR, Ali MW, Shamsuzzaman M, Ahmed S, Shackelford KA, et al. Factors influencing patients' satisfaction at different levels of health facilities in Bangladesh: Results from patient exit interviews. PLoS One. 2018;13(5):e0196643. [ FREE Full text ] [ CrossRef ] [ Medline ]
- Rumi MH, Makhdum N, Rashid MH, Muyeed A. Patients' Satisfaction on the Service Quality of Upazila Health Complex in Bangladesh. J Patient Exp. Aug 11, 2021;8:23743735211034054. [ FREE Full text ] [ CrossRef ] [ Medline ]
- Mendoza Aldana J, Piechulek H, Al-Sabir A. Client satisfaction and quality of health care in rural Bangladesh. Bull World Health Organ. 2001;79(6):512-517. [ FREE Full text ] [ Medline ]
- Nuri NN, Sarker M, Ahmed HU, Hossain MD, Beiersmann C, Jahn A. Experience and perceived quality of care of patients and their attendants in a specialized mental hospital in Bangladesh. Int J Ment Health Syst. 2019;13:46. [ FREE Full text ] [ CrossRef ] [ Medline ]
- Aga TB, Ferede YM, Mekonen EG. Satisfaction and associated factors towards inpatient health care services among adult patients at Pawie General Hospital, West Ethiopia. PLoS One. 2021;16(4):e0249168. [ FREE Full text ] [ CrossRef ] [ Medline ]
- Crow R, Gage H, Hampson S, Hart J, Kimber A, Storey L, et al. The measurement of satisfaction with healthcare: implications for practice from a systematic review of the literature. Health Technol Assess. 2002;6(32):1-244. [ FREE Full text ] [ CrossRef ] [ Medline ]
- Boquiren V, Hack T, Beaver K, Williamson S. What do measures of patient satisfaction with the doctor tell us? Patient Educ Couns. Jun 10, 2015.:1465-1473. [ FREE Full text ] [ CrossRef ] [ Medline ]
- Sun J, Hu G, Ma J, Chen Y, Wu L, Liu Q, et al. Consumer satisfaction with tertiary healthcare in China: findings from the 2015 China National Patient Survey. Int J Qual Health Care. Apr 01, 2017;29(2):213-221. [ CrossRef ] [ Medline ]
- Beggs C, Knibbs L, Johnson G, Morawska L. Environmental contamination and hospital-acquired infection: factors that are easily overlooked. Indoor Air. Oct 2015;25(5):462-474. [ CrossRef ] [ Medline ]
- Becker F, Sweeney B, Parsons K. Ambulatory facility design and patients' perceptions of healthcare quality. HERD. Jul 01, 2008;1(4):35-54. [ CrossRef ] [ Medline ]
- Mohammed S, Sambo MN, Dong H. Understanding client satisfaction with a health insurance scheme in Nigeria: factors and enrollees experiences. Health Res Policy Syst. May 25, 2011;9(1):20. [ FREE Full text ] [ CrossRef ] [ Medline ]
- Anderson RT, Camacho FT, Balkrishnan R. Willing to wait?: the influence of patient wait time on satisfaction with primary care. BMC Health Serv Res. Feb 28, 2007;7:31. [ FREE Full text ] [ CrossRef ] [ Medline ]
- Al-Harajin R, Al-Subaie S, Elzubair A. The association between waiting time and patient satisfaction in outpatient clinics: Findings from a tertiary care hospital in Saudi Arabia. J Fam Community Med. 2019;26(1):17. [ CrossRef ]
- Pavel MS, Chakrabarty S, Gow J. Cost of illness for outpatients attending public and private hospitals in Bangladesh. Int J Equity Health. Oct 10, 2016;15(1):167. [ FREE Full text ] [ CrossRef ] [ Medline ]
- Ghosh S. Catastrophic Payments and Impoverishment Due to Out-of-Pocket Health Spending. Economic and Political Weekly. 2011.:63-70. [ FREE Full text ]
Abbreviations
Edited by A Mavragani; submitted 10.06.23; peer-reviewed by MA Islam, S Bidmon; comments to author 13.09.23; revised version received 07.10.23; accepted 22.11.23; published 24.04.24.
©Md Zahid Hasan, Md Golam Rabbani, Orin Akter, Gazi Golam Mehdi, Mohammad Wahid Ahmed, Sayem Ahmed, Mahbub Elahi Chowdhury. Originally published in JMIR Formative Research (https://formative.jmir.org), 24.04.2024.
This is an open-access article distributed under the terms of the Creative Commons Attribution License (https://creativecommons.org/licenses/by/4.0/), which permits unrestricted use, distribution, and reproduction in any medium, provided the original work, first published in JMIR Formative Research, is properly cited. The complete bibliographic information, a link to the original publication on https://formative.jmir.org, as well as this copyright and license information must be included.

An official website of the United States government
The .gov means it’s official. Federal government websites often end in .gov or .mil. Before sharing sensitive information, make sure you’re on a federal government site.
The site is secure. The https:// ensures that you are connecting to the official website and that any information you provide is encrypted and transmitted securely.
- Publications
- Account settings
Preview improvements coming to the PMC website in October 2024. Learn More or Try it out now .
- Advanced Search
- Journal List
- Healthcare (Basel)

A Systematic Review on Healthcare Analytics: Application and Theoretical Perspective of Data Mining
Md saiful islam.
1 Mechanical and Industrial Engineering, Northeastern University, Boston, MA 02115, USA; [email protected] (M.S.I.); [email protected] (M.M.H.); [email protected] (X.W.); [email protected] (H.D.G.)
Md Mahmudul Hasan
Xiaoyi wang, hayley d. germack.
2 National Clinician Scholars Program, Yale University School of Medicine, New Haven, CT 06511, USA
3 Bouvé College of Health Sciences, Northeastern University, Boston, MA 02115, USA
Md Noor-E-Alam
Associated data.
The growing healthcare industry is generating a large volume of useful data on patient demographics, treatment plans, payment, and insurance coverage—attracting the attention of clinicians and scientists alike. In recent years, a number of peer-reviewed articles have addressed different dimensions of data mining application in healthcare. However, the lack of a comprehensive and systematic narrative motivated us to construct a literature review on this topic. In this paper, we present a review of the literature on healthcare analytics using data mining and big data. Following Preferred Reporting Items for Systematic Reviews and Meta-Analyses (PRISMA) guidelines, we conducted a database search between 2005 and 2016. Critical elements of the selected studies—healthcare sub-areas, data mining techniques, types of analytics, data, and data sources—were extracted to provide a systematic view of development in this field and possible future directions. We found that the existing literature mostly examines analytics in clinical and administrative decision-making. Use of human-generated data is predominant considering the wide adoption of Electronic Medical Record in clinical care. However, analytics based on website and social media data has been increasing in recent years. Lack of prescriptive analytics in practice and integration of domain expert knowledge in the decision-making process emphasizes the necessity of future research.
1. Introduction
Healthcare is a booming sector of the economy in many countries [ 1 ]. With its growth, come challenges including rising costs, inefficiencies, poor quality, and increasing complexity [ 2 ]. U.S. healthcare expenditures increased by 123% between 2010 and 2015—from $2.6 trillion to $3.2 trillion [ 3 ]. Inefficient—non-value added tasks (e.g., readmissions, inappropriate use of antibiotics, and fraud)—constitutes 21–47% of this enormous expenditure [ 4 ]. Some of these costs were associated with low quality care—researchers found that approximately 251,454 patients in the U.S. die each year due to medical errors [ 5 ]. Better decision-making based on available information could mitigate these challenges and facilitate the transition to a value-based healthcare industry [ 4 ]. Healthcare institutions are adopting information technology in their management system [ 6 ]. A large volume of data is collected through this system on a regular basis. Analytics provides tools and techniques to extract information from this complex and voluminous data [ 2 ] and translate it into information to assist decision-making in healthcare.
Analytics is the way of developing insights through the efficient use of data and application of quantitative and qualitative analysis [ 7 ]. It can generate fact-based decisions for “planning, management, measurement, and learning” purposes [ 2 ]. For instance, the Centers for Medicare and Medicaid Services (CMS) used analytics to reduce hospital readmission rates and avert $115 million in fraudulent payment [ 8 ]. Use of analytics—including data mining, text mining, and big data analytics—is assisting healthcare professionals in disease prediction, diagnosis, and treatment, resulting in an improvement in service quality and reduction in cost [ 9 ]. According to some estimates, application of data mining can save $450 billion each year from the U.S. healthcare system [ 10 ]. In the past ten years, researchers have studied data mining and big data analytics from both applied (e.g., applied to pharmacovigilance or mental health) and theoretical (e.g., reflecting on the methodological or philosophical challenges of data mining) perspectives.
In this review, we systematically organize and summarize the published peer-reviewed literature related to the applied and theoretical perspectives of data mining. We classify the literature by types of analytics (e.g., descriptive, predictive, prescriptive), healthcare application areas (i.e., clinical decision support, mental health), and data mining techniques (i.e., classification, sequential pattern mining); and we report the data source used in each review paper which, to our best knowledge, has never done before.
Motivation and Scope
There is a large body of recently published review/conceptual studies on healthcare and data mining. We outline the characteristics of these studies—e.g., scope/healthcare sub-area, timeframe, and number of papers reviewed—in Table 1 . For example, one study reviewed awareness effect in type 2 diabetes published between 2001 and 2005, identifying 18 papers [ 11 ]. This current review literature is limited—most of the papers listed in Table 1 did not report the timeframe and/or number of papers reviewed (expressed as N/A).
Characteristics of existing review/conceptual studies on the related topics.
N/A represents Not Reported.
There is no comprehensive review available which presents the complete picture of data mining application in the healthcare industry. The existing reviews (16 out of 21) are either focused on a specific area of healthcare, such as clinical medicine (three reviews) [ 16 , 17 , 19 ], adverse drug reaction signal detection (two reviews) [ 25 , 26 ], big data analytics (four reviews) [ 8 , 10 , 22 , 24 ], or the application and performance of data mining algorithms (five reviews) [ 9 , 13 , 14 , 20 , 21 ]. Two studies focused on specific diseases (diabetes [ 11 ], skin diseases [ 18 ]). To the best of our knowledge, none of these studies present the universe of research that has been done in this field. These studies are also limited in the rigor of their methodology except for four articles [ 11 , 16 , 22 , 25 ], which provide key insights including the timeframe covered in the study, database search, and literature inclusion or exclusion criteria, but they are limited in their scope of topics covered (see Table 1 ).
Beyond condensing the applied literature, our review also adds to the body of theoretical reviews in the analytics literature. Current theoretical reviews are limited to methodological challenges and techniques to overcome those challenges [ 15 , 16 , 27 ] and application and impact of big data analytics in healthcare [ 23 ]. In summary, the current reviews listed in Table 1 lacks in (1) width of coverage in terms of application areas, (2) breadth of data mining techniques, (3) assessment of literature quality, and (4) systematic selection and analysis of papers. In this review, we aim to fill the above-mentioned gaps. We add to this literature by covering the applied and theoretical perspective of data mining and big data analytics in healthcare with a more comprehensive and systematic approach.
2. Methodology
The methodology of our review followed the checklist proposed by the Preferred Reporting Items for Systematic Reviews and Meta-Analyses (PRISMA) [ 28 ]. We assessed the quality of the selected articles using JBI Critical Appraisal Checklist for analytical cross sectional studies [ 29 ] and Critical Appraisal Skills Programme (CASP) qualitative research checklist [ 30 ].
2.1. Input Literature
Selected literature and their selection process for the review are described in this section. Initially a two phase advance keyword search was conducted on the database Web of Science and one phase (Phase 2) search in PubMed and Google Scholar with time filter 1 January 2005 to 31 December 2016 in “All Fields”. Journal articles written in English was added as additional filters. Keywords listed in Table 2 were used in different phases. The complete search procedure was conducted using the following procedure:

Preferred Reporting Items for Systematic Reviews and Meta-Analyses (PRISMA) flow chart [ 28 ] illustrating the literature search process.
- Exclusion criteria: This included articles reporting on results of: qualitative study, survey, focus group study, feasibility study, monitoring device, team relationship measurement, job satisfaction, work environment, “what-if” analysis, data collection technique, editorials or short report, merely mention data mining, and articles not published in international journals . Duplicates were removed (33 articles). Finally, 117 articles were retained for the review. Figure 1 provides a PRISMA [ 28 ] flow diagram of the review process and Supplementary Information File S1 (Table S1) provides the PRISMA checklist.
Keywords for database search.
1 A logical operator used between the keywords during database search. 2 Cancer was listed independently because other dominant associations have the word “disease” associated with them (i.e., heart disease, skin disease, mental disease etc.).
2.2. Quality Assessment and Processing Steps
The full text of each of the 117 articles was reviewed separately by two researchers to eliminate bias [ 28 ]. To assess the quality of the cross sectional studies, we applied the JBI Critical Appraisal Checklist for Analytical Cross Sectional Studies [ 29 ]. For theoretical papers, we applied the Critical Appraisal Skills Programme (CASP) qualitative research checklist [ 30 ]. We modified the checklist items, as not all items specified in the JBI or CASP checklists were applicable to studies on healthcare analytics ( Supplementary Materials Table S2 ). We evaluated each article’s quality based on inclusion of: (1) clear objective and inclusion criteria; (2) detailed description of sample population and variables; (3) data source (e.g., hospital, database, survey) and format (e.g., structured Electronic Medical Record (EMR), International Classification of Diseases code, unstructured text, survey response); (4) valid and reliable data collection; (5) consideration of ethical issues; (6) detailed discussion of findings and implications; (7) valid and reliable measurement of outcomes; and (8) use of an appropriate data mining tool for cross-sectional studies and (1) clear statement of aims; (2) appropriateness of qualitative methodology; (3) appropriateness of research design; (4) clearly stated findings; and (5) value of research for the theoretical papers. Summary characteristics from any study fulfilling these criteria were included in the final data aggregation ( Supplementary Materials Table S3 ).
To summarize the body of knowledge, we adopted the three-step processing methodology outlined by Levy and Ellis [ 31 ] and Webster and Watson [ 32 ] ( Figure 2 ). During the review process, information was extracted by identifying and defining the problem, understanding the solution process and listing the important findings (“Know the literature”). We summarized and compared each article with the articles associated with the similar problems (“Comprehend the literature”). This simultaneously ensured that any irrelevant information was not considered for the analysis. The summarized information was stored in a spreadsheet in the form of a concept matrix as described by Webster and Watson [ 32 ]. We updated the concept matrix periodically, after completing every 20% of the articles which is approximately 23 articles, to include new findings (“Apply”). Based on the concept matrix, we developed a classification scheme (see Figure 3 ) for further comparison and contrast. We established an operational definition (see Table 3 ) for each class and same class articles were separated from the pool (“Analyze and Synthesis”). We compared classifications between researchers and we resolved disagreements (on six articles) by discussion. The final classification provided distinguished groups of articles with summary, facts, and remarks made by the reviewers (“Evaluate”).

Three stages of effective literature review process, adapted from Levy and Ellis [ 31 ].

Classification scheme of the literature.
Operational definition of the classes.
* Most of the definitions listed in this table are well established in literature and well know. Therefore, we did not use any specific reference. However, for some classes, specifically for types of analytics and data, varying definitions are available in the literature. We cited the sources of those definitions.
2.3. Results
The network diagram of selected articles and the keywords listed by authors in Figure 4 represents the outcome of the methodological review process. We elaborate on the resulting output in the subsequent sections using the structure of the developed classification scheme ( Figure 3 ). We also report the potential future research areas.

Visualization of high-frequency keywords of the reviewed papers. The white circles symbolize the articles and the blue circles represent keywords. The keywords that occurred only once are eliminated as well as the corresponding articles. The size of the blue circles and the texts represent how often that keyword is found. The size of the white circles is proportional to the number of keywords used in that article. The links represents the connections between the keywords and the articles. For example, if a blue circle has three links (e.g., Decision-Making) that means that keyword was used in three articles. The diagram is created with the open source software Gephi [ 34 ].
2.3.1. Methodological Quality of the Studies
Out of 117 papers included in this review, 92 applied analytics and 25 were qualitative/conceptual. The methodological quality of the analytical studies (92 out of 117) were evaluated by a modified version of 8 yes/no questions suggested in JBI Critical Appraisal Checklist for Analytical Cross Sectional Studies [ 29 ]. Each question contains 1 point (1 if the answer is Yes or 0 for No). The score achieved by each paper is provided in the final column of Supplementary Materials Table S3 . On average, each paper applying analytics scored 7.6 out of 8, with a range of 6–8 points. Major drawbacks were the absence of data source and performance measure of data mining algorithms. Out of 92 papers, 23 did not evaluate or mention the performance of the applied algorithms and eight did not mention the source of the data. However, all the papers in healthcare analytics had a clear objective and a detailed discussion of sample population and variables. Data used in each paper was either de-identified/anonymized or approved by institute’s ethical committee to ensure patient confidentiality.
We applied the Critical Appraisal Skills Programme (CASP) qualitative research checklist [ 30 ] to evaluate the quality of the 25 theoretical papers. Five questions (out of ten) in that checklist were not applicable to the theoretical studies. Therefore, we evaluated the papers in this section in a five-point scale (1 if the answer is Yes or 0 for No). Papers included in this review showed high methodological quality as 21 papers (out of 25) scored 5. The last column in the Supplementary Materials Table S3 provides the score achieved by individual papers.
2.3.2. Distribution by Publication Year
The distribution of articles published related to data mining and big data analytics in healthcare across the timeline of the study (2005–2016) is presented in Figure 5 . The distribution shows an upward trend with at least two articles in each year and more than ten articles in the last four years. Additionally, this trend represents the growing interest of government agencies, healthcare practitioners, and academicians in this interdisciplinary field of research. We anticipate that the use of analytics will continue in the coming years to address rising healthcare costs and need of improved quality of care.

Distribution of publication by year (117 articles).
2.3.3. Distribution by Journal
Articles published in 74 different journals were included in this study. Table 4 lists the top ten journals in terms of number of papers published. Expert System with Application was the dominant source of literature on data mining application in healthcare with 7 of the 117 articles. Journals were interdisciplinary in nature and spanned computational journals like IEEE Transection on Information Technology in Biomedicine to policy focused journal like Health Affairs . Articles published in Expert System with Application, Journal of Medical Systems, Journal of the American Medical Informatics Association, Healthcare Informatics Research were mostly related to analytics applied in clinical decision-making and healthcare administration. On the other hand, articles published in Health Affairs were predominantly conceptual in nature addressing policy issues, challenges, and potential of this field.
Top 10 journals on application of data mining in healthcare.
3. Healthcare Analytics
Out of 117 articles, 92 applied analytics for decision-making in healthcare. We discuss the types of analytics, the application area, the data, and the data mining techniques used in these articles and summarize them in Supplementary Materials Table S4 .
3.1. Types of Analytics
We identified three types of analytics in the literature: descriptive (i.e., exploration and discovery of information in the dataset), predictive (i.e., prediction of upcoming events based on historical data) and prescriptive (i.e., utilization of scenarios to provide decision support). Five of the 92 studies employed both descriptive and predictive analytics. In Figure 6 , which displays the percentage of healthcare articles using each analytics type, we show that descriptive analytics is the most commonly used in healthcare (48%). Descriptive analytics was dominant in all the application areas except in clinical decision support. Among the application areas, pharmacovigilance studies only used descriptive analytics as this application area is focused on identifying an association between adverse drug effects with medication. Predictive analytics was used in 43% articles. Among application areas, clinical decision support had the highest application of predictive analytics as many studies in this area are involved in risk and morbidity prediction of chest pain, heart attack, and other diseases. In contrast, use of prescriptive analytics was very uncommon (only 9%) as most of these studies were focused on either a specific population base or a specific disease scenario. However, some evidence of prescriptive analytics was found in public healthcare, administration, and mental health (see Supplementary Materials Table S4 ). These studies create a data repository and/or analytical platform to facilitate decision-making for different scenarios.

Types of analytics used in literature. ( a ) Percentage of analytics type; ( b ) Analytics type by application area.
3.2. Types of Data
To identify types of data, we adopted the classification scheme identified by Raghupathi and Raghupathi [ 23 ] which takes into account the nature (i.e., text, image, number, electronic signal), source, and collection method of data together. Table 3 provides the operational definitions of taxonomy adopted in this paper. Figure 7 a presents the percentage of data type used and Figure 7 b, the number of usage by application area. As expected, human generated (HG) data, including EMR, Electronic Health Record (HER), and Electronic Patient Record (EPR), is the most commonly (77%) used form. Web or Social media (WS) data is the second dominant (11%) type of data, as increasingly more people are using social media now and ongoing digital revolution in the healthcare sector [ 35 ]. In addition, recent development in Natural Language Processing (NLP) techniques is making the use of WS data easier than before [ 36 ]. The other three types of data (SD, BT, and BM) consist of only about 12% of total data usage, but popularity and market growth of wearable personal health tracking devices [ 37 ] may increase the use of SD and BM data.

Percentage of data type used ( a ) and type of data used by application area ( b ).
3.3. Data Mining Techniques
Data mining techniques used in the articles reviewed include classification, clustering, association, anomaly detection, sequential pattern mining, regression, and data warehousing. While elaborate description of each technique and available algorithms is out of scope of this review, we report the frequency of each technique and its sector wise distribution in Figure 8 a,b, respectively. Among the articles included in the review, 57 used classification techniques to analyze data. Association and clustering were used in 21 and 18 articles, respectively. Use of other techniques was less frequent.

Utilization of data mining techniques, ( a ) by percentage and ( b ) by application area.
A high proportion (8 out of 9) of pharmacovigilance papers used association. Use of classification was dominant in every sector except pharmacovigilance ( Figure 8 b). Data warehousing was mostly used in healthcare administration ( Figure 8 b).
We delved deeper into classification as it was utilized in the majority (57 out of 92) of the papers. There are a number of algorithms used for classification, which we present in a word cloud in Figure 9 . Support Vector Machine (SVM), Artificial Neural Network (ANN), Logistic Regression (LR), Decision Tree (DT), and DT based algorithms were the most commonly used. Random Forest (RF), Bayesian Network and Fuzzy-based algorithms were also often used. Some papers (three papers) introduced novel algorithms for specific applications. For example, Yeh et al. [ 38 ] developed discrete particle swarm optimization based classification algorithm to classify breast cancer patients from a pool of general population. Self-organizing maps and K-means were the most commonly used clustering algorithm in healthcare. Performance (e.g., accuracy, sensitivity, specificity, area under the ROC curve, positive predictive value, negative predictive value etc.) of each of these algorithms varied by application and data type. We recommend applying multiple algorithms and choosing the one which achieves the best accuracy.

Word cloud [ 39 ] with classification algorithms.
4. Application of Analytics in Healthcare
Table 3 provides the operational definitions of the six application areas (i.e., clinical decision support, healthcare administration, privacy and fraud detection, mental health, public health, and pharmacovigilance) identified in this review. Figure 10 shows the percentage of articles in each area. Among different classes in healthcare analytics, data mining application is mostly applied in clinical decision support (42%) and administrative purposes (32%). This section discusses the application of data mining in these areas and identifies the main aims of these studies, performance gaps, and key features.

Percentage of papers utilized healthcare analytics by application area (92 articles out of 117).
4.1. Clinical Decision Support
Clinical decision support consists of descriptive and/or predictive analysis mostly related to cardiovascular disease (CVD), cancer, diabetes, and emergency/critical care unit patients. Some studies developed novel data mining algorithms which we review. Table 5 describes the topics investigated and data sources used by papers using clinical decision-making, organized by major diseases category.
Topics and data sources of papers using clinical decision-making, organized by major disease category.
4.1.1. Cardiovascular Disease (CVD)
CVD is one of the most common causes of death globally [ 45 , 77 ]. Its public health relevance is reflected in the literature—it was addressed by seven articles (18% of articles in clinical decision support).
Risk factors related to Coronary Heart Disease (CHD) were distilled into a decision tree based classification system by researchers [ 40 ]. The authors investigated three events: Coronary Artery Bypass Graft Surgery (CABG), Percutaneous Coronary Intervention (PCI), and Myocardial Infarction (MI). They developed three models: CABG vs. non-CABG, PCI vs. non-PCI, and MI VS non-MI. The risk factors for each event were divided into four groups in two stages. The risk factors were separated into before and after the event at the 1st stage and modifiable (e.g., smoking habit or blood pressure) and non-modifiable (e.g., age or sex) at the 2nd stage for each group. After classification, the most important risk factors were identified by extracting the classification rules. The Framingham equation [ 78 ]—which is widely used to calculate global risk for CHD was used to calculate the risk for each event. The most important risk factors identified were age, smoking habit, history of hypertension, family history, and history of diabetes. Other studies on CHD show similar results [ 79 , 80 , 81 ]. This study had implications for healthcare providers and patients by identifying risk factors to specifically target, identify and in the case of modifiable factors, reduce CHD risk [ 40 ].
Data mining has also been applied to diagnose Coronary Artery Disease (CAD) [ 41 ]. Researchers showed that in lieu of existing diagnostic methods (i.e., Coronary Angiography (CA))—which are costly and require high technical skill—data mining using existing data like demographics, medical history, simple physical examination, blood tests, and noninvasive simple investigations (e.g., heart rate, glucose level, body mass index, creatinine level, cholesterol level, arterial stiffness) is simple, less costly, and can be used to achieve a similar level of accuracy. Researchers used a four-step classification process: (1) Decision tree was used to classify the data; (2) Crisp classification rules were generated; (3) A fuzzy model was created by fuzzifying the crisp classifier rules; and (4) Fuzzy model parameters were optimized and the final classification was made. The proposed optimized fuzzy model achieved 73% of prediction accuracy and improved upon an existing Artificial Neural Network (ANN) by providing better interpretability.
Traditional data mining and machine learning algorithms (e.g., probabilistic neural networks and SVM) may not be advanced enough to handle the data used for CVD diagnosis, which is often uncertain and highly dimensional in nature. To tackle this issue, researchers [ 42 ] proposed a Fuzzy standard additive model (SAM) for classification. They used adaptive vector quantization clustering to generate unsupervised fuzzy rules which were later optimized (minimized the number of rules) by Genetic Algorithm (GA). They then used the incremental form of a supervised technique, Gradient Descent, to fine tune the rules. Considering the highly time consuming process of the fuzzy system given large number of features in the data, the number of features was reduced with wavelet transformation. The proposed algorithm achieved better accuracy (78.78%) than the probabilistic neural network (73.80%), SVM (74.27%), fuzzy ARTMAP (63.46%), and adaptive neuro-fuzzy inference system (74.90%). Another common issue in cardiovascular event risk prediction is the censorship of data (i.e., the patient’s condition is not followed up after they leave hospital and until a new event occurs; the available data becomes right-censored). Elimination and exclusion of the censored data create bias in prediction results. To address the censorship of the data in their study on CVD event risk prediction after time, two studies [ 43 , 44 ] used Inverse Probability Censoring Weighting (IPCW). IPCW is a pre-processing step used to calculate the weights on data which are later classified using Bayesian Network. One of these studies [ 43 ] provided an IPCW based system which is compatible with any machine learning algorithm.
Electrocardiography (ECG)—non-invasive measurement of the electrical activity of the heartbeat—is the most commonly used medical studies in the assessment of CVD. Machine learning offers potential optimization of traditional ECG assessment which requires decompressing before making any diagnosis. This process takes time and large space in computers. In one study, researchers [ 45 ] developed a framework for real-time diagnosis of cardiovascular abnormalities based on compressed ECG. To reduce diagnosis time—which is critical for clinical decision-making regarding appropriate and timely treatment—they proposed and tested a mobile based framework and applied it to wireless monitoring of the patient. The ECG was sent to the hospital server where the ECG signals were divided into normal and abnormal clusters. The system detected cardiac abnormality with 97% accuracy. The cluster information was sent to patient’s mobile phone; and if any life-threatening abnormality was detected, the mobile phone alerted the hospital or the emergency personnel.
Data analytics have also been applied to more rare CVDs. One study [ 46 ] developed an intervention prediction model for Hypoplastic Left Heart Syndrome (HLHS). HLHS is a rare form of fatal heart disease in infants, which requires surgery. Post-surgical evaluation is critical as patient condition can shift very quickly. Indicators of wellness of the patients are not easily or directly measurable, but inferences can be made based on measurable physiological parameters including pulse, heart rhythm, systemic blood pressure, common atrial filling pressure, urine output, physical exam, and systemic and mixed venous oxygen saturations. A subtle physiological shift can cause death if not noticed and intervened upon. To help healthcare providers in decision-making, the researchers developed a prediction model by identifying the correlation between physiological parameters and interventions. They collected 19,134 records of 17 patients in Pediatric Intensive Care Units (PICU). Each record contained different physiological parameters measured by devices and noted by nurses. For each record, a wellness score was calculated by the domain experts. After classifying the data using a rough set algorithm, decision rules were extracted for each wellness score to aid in making intervention plans. A new measure for feature selection—Combined Classification Quality (CCQ)—was developed by considering the effect of variations in a feature values and distinct outcome each feature value leads to. Authors showed that higher value of CCQ leads to higher classification accuracy which is not always true for commonly used measure classification quality (CQ). For example, two features with CQ value of 1 leads to very different classification accuracy—35.5% and 75%. Same two features had CCQ value 0.25 and 0.40, features with 0.40 CCQ produced 75% classification accuracy. By using CCQ instead of CQ, researchers can avoid such inconsistency.
4.1.2. Diabetes
The disease burden related to diabetes is high and rising in every country. According to the World Health Organization’s (WHO) prediction, it will become the seventh leading cause of death by 2030 [ 82 ]. Data mining has been applied to identify rare forms of diabetes, identify the important factors to control diabetes, and explore patient history to extract knowledge. We reviewed 7 studies that applied healthcare analytics to diabetes.
Researchers extracted knowledge about diabetes treatment pathways and identified rare forms and complications of diabetes using a three level clustering framework from examination history of diabetic patients [ 48 ]. In this three-level clustering framework, the first level clustered patients who went through regular tests for monitoring purposes (e.g., checkup visit, glucose level, urine test) or to diagnose diabetes-related complications (e.g., eye tests for diabetic retinopathy). The second level explored patients who went through diagnosis for specific or different diabetic complications only (e.g., cardiovascular, eye, liver, and kidney related complications). These two level produced 2939 outliers out of 6380 patients. At the third level, authors clustered these outlier patients to gain insight about rare form of diabetes or rare complications. A density based clustering algorithm, DBSCAN, was used for clustering as it doesn’t require to specify the number of clusters apriori and is less sensitive to noise and outliers. This framework for grouping patients by treatment pathway can be utilized to evaluate treatment plans and costs. Another group of researchers [ 49 ] investigated the important factors related to type 2 diabetes control. They used feature selection via supervised model construction (FSSMC) to select the important factors with rank/order. They applied naïve bayes, IB1 and C4.5 algorithm with FSSMC technique to classify patients having poor or good diabetes control and evaluate the classification efficiency for different subsets of features. Experiments performed with physiological and laboratory information collected from 3857 patients showed that the classifier algorithms performed best (1–3% increase in accuracy) with the features selected by FSSMC. Age, diagnosis duration, and Insulin treatment were the top three important factors.
Data analytics have also been applied to identify patients with type 2 diabetes. In one study [ 52 ], using fragmented data from two different healthcare centers, researchers evaluated the effect of data fragmentation on a high throughput clinical phenotyping (HTCP) algorithm to identify patients at risk of developing type 2 diabetes. When a patient visits multiple healthcare centers during a study period, his/her data is stored in different EMRs and is called fragmented. In such cases, using HTPC algorithm can lead to improper classification. An experiment performed in a rural setting showed that using data from two healthcare centers instead of one decreased the false negative rate from 32.9% to 0%. In another study, researchers [ 51 ] utilized sparse logistic regression to predict type 2 diabetes risk from insurance claims data. They developed a model that outperformed the traditional risk prediction methods for large data sets and data sets with missing value cases by increasing the AUC value from 0.75 to 0.80. The dataset contained more than 500 features including demography, specific medical conditions, and comorbidity. And in another study, researchers [ 53 ] developed prediction and risk diagnosis model using a hybrid system with SVM. Using features like blood pressure, fasting blood sugar, two-hour post-glucose tolerance, cholesterol level along with other demographic and anthropometric features, the SVM algorithm was able to predict diabetes risk with 97% accuracy. One reason for achieving high accuracy compared to the study using insurance claims data [ 51 ] is the structured nature of the data which came from a cross-sectional survey on diabetes.
Different statistical and machine learning algorithms are available for classification purposes. Researchers [ 50 ] compared the performance of two statistical method (LR and Fisher linear discriminant analysis) and four machine learning algorithms (SVM (using radial basis function kernel), ANN, Random Forest, and Fuzzy C-mean) for predicting diabetes diagnosis. Ten features (age, gender, BMI, waist circumference, smoking, job, hypertension, residential region (rural/urban), physical activity, and family history of diabetes) were used to test the classification performance (diabetes or no diabetes). Parameters for ANN and SVM were optimized through Greedy search. SVM showed best performance in all performance measures. SVM was at least 5% more accurate than other classification techniques. Statistical methods performed similar to the other machine learning algorithms. This study was limited by a low prevalence of diabetes in the dataset, however, which can cause poor classification performance. Researchers [ 47 ] also proposed a novel pattern recognition algorithm by using convolutional nonnegative matrix factorization. They considered a patient as an entity and each of patients’ visit to the doctor, prescriptions, test result, and diagnosis are considered as an event over time. Finding such patterns can be helpful to group similar patients, identify their treatment pathway as well as patient management. Though they did not compare the pattern recognition accuracy with existing methods like single value decomposition (SVD), the matrix-like representation makes it intuitive.
4.1.3. Cancer
Cancer is another major threat to public health [ 83 ]. Machine learning has been applied to cancer patients to predict survival, and diagnosis. We reviewed five studies that applied healthcare analytics to cancer.
Despite many advances in treatment, accurate prediction of survival in patients with cancer remains challenging considering the heterogeneity of cancer complexity, treatment options, and patient population. Survival of prostate cancer patients has been predicted using a classification model [ 54 ]. The model used a public database-SEER (Surveillance, Epidemiology, and End Result) and applied a stratified ten-fold sampling approach. Survival prediction among prostate cancer patients was made using DT, ANN and SVM algorithm. SVM outperformed other algorithms with 92.85% classification accuracy wherein DT and ANN achieved 90% and 91.07% accuracy respectively. This same database has been used to predict survival of lung cancer patients [ 56 ]. After preprocessing the 11 features available in the data set, authors identified two features (1. removed and examined regional lymph node count and 2. malignant/in-situ tumor count) which had the strongest predictive power. They used several supervised classification methods on the preprocessed data; ensemble voting of five decision tree based classifiers and meta-classifiers (J48 DT, RF, LogitBoost, Random Subspace, and Alternating DT) provided the best performance—74% for 6 months, 75% for 9 months, 77% for 1 year, 86% for 2 years, and 92% for 5 years survival. Using this technique, they developed an online lung cancer outcome calculator to estimate the risk of mortality after 6 months, 9 months, 1 year, 2 years and 5 years of diagnosis.
In addition to predicting survival, machine learning techniques have also been used to identify patients with cancer. Among patients with breast cancer, researchers [ 38 ] have proposed a new hybrid algorithm to classify breast cancer patient from patients who do not have breast cancer. They used correlation and regression to select the significant features at the first stage. Then, at the second stage, they used discrete Particle Swarm Optimization (PSO) to classify the data. This hybrid algorithm was applied to Wisconsin Breast Cancer Data set available at UCI machine learning repository. It achieved better accuracy (98.71%) compared to a genetic algorithm (GA) (96.14%) [ 84 ] and another PSO-based algorithm (93.4%) [ 85 ].
Machine learning has also been used to identify the nature of cancer (benign or malignant) and to understand demographics related to cancer. Among patients with breast cancer, researchers [ 42 ] applied the Fuzzy standard additive model (SAM) with GA (discussed earlier in relation to CVD)-predicting the nature of breast cancer (benign or malignant). They used a UCI machine learning repository which was capable of classifying uncertain and high dimensional data with greater accuracy (by 1–2%). Researchers have also used big data [ 55 ] to create a visualization tool to provide a dynamic view of cancer statistics (e.g., trend, association with other diseases), and how they are associated with different demographic variables (e.g., age, sex) and other diseases (e.g., diabetes, kidney infection). Use of data mining provided a better understanding of cancer patients both at demographic and outcome level which in terms provides an opportunity of early identification and intervention.
4.1.4. Emergency Care
The Emergency department (ED) is the primary route to hospital admission [ 58 ]. In 2011, 20% of US population had at least one or more visits to the ED [ 86 ]. EDs are experiencing significant financial pressure to increase efficiency and throughput of patients. Discrete event simulation (i.e., modeling system operations with sequence of isolated events) is a useful tool to understand and improve ED operations by simulating the behavior and performance of EDs. Certain features of the ED (e.g., different types of patients, treatments, urgency, and uncertainty) can complicate simulation. One way to handle the complexity is to group the patients according to required treatment. Previously, the “casemix” principle, which was developed by expert clinicians to groups of similar patients in case-specific settings (e.g., telemetry or nephrology units), was used, but it has limitations in the ED setting [ 58 ]. Researchers applied [ 58 ] data mining (clustering) to the ED setting to group the patients based on treatment pattern (e.g., full ward test, head injury observation, ECG, blood glucose, CT scan, X-ray). The clustering model was verified and validated by ED clinicians. These grouping data were then used in discrete event simulation to understand and improve ED operations (mainly length of stay) and process flows for each group.
Chest pain admissions to the ED have also been examined using decision-making framework. Researchers [ 57 ] proposed a three stage decision-making framework for classifying severity of chest pain as: AMI, angina pectoris, or other. At the first stage, lab tests and diagnoses were collected and the association between them were extracted. In the second stage, experts developed association rules between lab tests diagnosis to help physicians make quick diagnostic decisions based diagnostic tests and avoid further unnecessary lab tests. In the third stage, authors developed a classification tree to classify the chest pain diagnosis based on selected lab test, diagnosis and medical record. This hybrid model was applied to the emergency department at one hospital. They developed the classification system using 327 association rules to selected lab tests using C5.0, Neural Network (NN) and SVM. C5.0 algorithm achieved 94.18% accuracy whereas NN and SVM achieved 88.89% and 85.19% accuracy respectively.
4.1.5. Intensive Care
Intensive care units cater to patients with severe and life-threatening illness and injury which require constant, close monitoring and support to ensure normal bodily function. Death is a much more common event in an ICU compared to a general medical unit—one study showed that 22.4% of total death in hospitals occurred in the ICU [ 87 ]. Survival predictions and identification of important factors related to mortality can help healthcare providers plan care. We identified two papers [ 59 , 60 ] that developed prediction models for ICU mortality rate prediction. Using a large amount of ICU patient data (specifically from the first 24 h of the stay) collected from University of Kentucky Hospital from 1998 to 2007 (38,474 admissions), one group of researchers identified 15 out of 40 significant features using Pearson’s Chi-square test (for categorical variables) and Student-t test (for continuous variable) [ 59 ]. The mortality rate was predicted by DT, ANN, SVM and APACHE III, a logistic regression based approach. Compared to the other methods applied, DT’s AUC value was higher by 0.02. The study was limited, however, by only considering the first 24 h of admission to the ICU, which may not be enough to make prediction on mortality rate. Another team of researchers [ 60 ] applied a similarity metric to predict 30-day mortality prediction in 17,152 ICU admissions data extracted from MIMIC-II database [ 88 ]. Their analysis concluded that a large group of similar patient data (e.g., vital sign, laboratory test result) instead of all patient data would lead to slightly better prediction accuracy. The logistic regression model for mortality prediction achieved 0.83 AUC value when 5000 similar patients were used for training but, its performance declined to 0.81 AUC when all the available patient data were used.
4.1.6. Other Applications
In addition to CVD, diabetes, cancer, emergency care, and ICU care, data mining has been applied to various clinical decision-making problems like pressure ulcer risk prediction, general problem lists, and personalized medical care. To predict pressure ulcer formation (localized skin and tissue damage because of shear, friction, pressure or any combination of these factors), researchers [ 62 ] developed two classification-based predictive models. One included all 14 features (including age, sex, course, Anesthesia, body position during operation, and skin status) and another, reduced model, including significant features only (5 in DT model, 7 in SVM, LR and Mahalanobis Taguchi System model). Mahalanobis Taguchi System (MTS), SVM, DT, and LR were used for both classification and feature selection (in the second model only) purposes. LR and SVM performed slightly better when all the features were included, but MTS achieved better sensitivity and specificity in the reduced model (+10% to +15%). These machine learning techniques can provide better assistance in pressure ulcer risk prediction than the traditional Norton and Braden medical scale [ 62 ]. Though the study provides the advantages of using data mining algorithms, the data set used here was imbalanced as it only had 8 cases of pressure ulcer in 168 patients. Also among patients with pressure ulcers, another team of researchers [ 63 ] recommended a data mining based alternative to the Braden scale for prediction. They applied data mining algorithms to four years of longitudinal patient data to identify the most important factors related to pressure ulcer prediction (i.e., days of stay in the hospital, serum albumin, and age). In terms of C-statistics, RF (0.83) provided highest predictive accuracy over DT (0.63), LR (0.82), and multivariate adaptive regression splines (0.78).
For data mining algorithms, which often show poor performance with imbalanced (i.e., low occurrence of one class compared to other classes) data, researchers [ 70 ] developed a sub-sampling technique. They designed two experiments, one considered sub-sampling technique and another one did not. For a highly imbalanced data set, Random Forest (RF), SVM, and Bagging and Boosting achieved better classification accuracy with this sub-sampling technique in classifying eight diseases (male genital disease, testis cancer, encephalitis, aneurysm, breast cancer, peripheral atherosclerosis, and diabetes mellitus) that had less than 5% occurrences in the National Inpatient Sample (NIS) data of Healthcare Cost and Utilization Project (HCUP). Surprisingly, possibly due to balancing the dataset through sub-sampling, RF slightly outperformed (+0.01 AUC) the other two methods.
The patient problem list is a vital component of clinical medicine. It enables decision support and quality measurement. But, it is often incomplete. Researchers have [ 64 ] suggested that a complete list of problems leads to better quality treatment in terms of final outcome [ 64 ]. Complete problem lists enable clinicians to get a better understanding of the issue and influence diagnostic reasoning. One group of researchers proposed a data mining model to find an association between patient problems and prescribed medications and laboratory tests which can act as a support to clinical decision-making [ 64 ]. Currently, domain experts spend a large amount of time for this purpose but, association rule mining can save both time and other resources. Additionally, consideration of unstructured data like doctor’s and/or nurse’s written comments and notes can provide additional information. These association rules can aid clinicians in preventing errors in diagnosis and reduce treatment complexity. For example, a set of problems and medications can co-occur frequently. If a clinician has knowledge about this relation, he/she can prescribe similar medications when faced with a similar set of problems. One group of researchers [ 61 ] developed an approach which achieved 90% accuracy in finding association between medications and problems, and 55% accuracy between laboratory tests and problems. Among outpatients diagnosed with respiratory infection, 92.79% were treated with drugs. Physicians could choose any of the 100,013 drugs available in the inventory. Moreover, in an attempt to examine the treatment plan patterns, they identified the 78 most commonly used drugs which could be prescribed, regardless of patient’s complaints and demography. The classification model used to identify the most common drugs achieved 74.73% accuracy and most importantly found variables like age, race, gender, and complaints of patients were insignificant.
Personalized medicine—tailored treatment based on a patient’s predicted response or risk of disease—is another venue for data mining algorithms. One group of researchers [ 66 ] used a big data framework to create personalized care system. One patient’s medical history is compared with other available patient data. Based on that comparison, possibility of a disease of an individual was calculated. All the possible diseases were ranked from high risk to low risk diseases. This approach is very similar to how online giants Netflix and Amazon suggest movies and books to the customer [ 66 ]. Another group of researchers [ 67 ] used the Electronic Patient Records (EPR), which contains structured data (e.g., disease code) and unstructured data (e.g., notes and comments made by doctors and nurses at different stages of treatment) to develop personalized care. From the unstructured text data, the researchers extracted clinical terms and mapped them to an ontology. Using this mapped codes and existing structured data (disease code), they created a phenotypic profile for each patient. The patients were divided into different clusters (with 87.78% precision) based on the similarity of their phenotypic profile. Correlation of diseases were captured by counting the occurrences of two or more diseases in patient phenotype. Then, the protein/gene structure associated with the diseases was identified and a protein network was created. From the sharing of specific protein structure by the diseases, correlation was identified.
Among patients with asthma, researchers [ 65 ] used environmental and patient physiological data to develop a prediction model for asthma attack to give doctors and patients a chance for prevention. They used data from a home-care institute where patients input their physical condition online; and environmental data (air pollutant and weather data). Their data mining model involved feature selection through sequential pattern mining and risk prediction using DT and association rule mining. This model can make asthma attack risk prediction with 86.89% accuracy. Real implementation showed that patients found risk prediction helpful to avoid severe asthma attacks.
Among patients with Parkinson’s disease, researchers [ 73 ] introduced a comprehensive end-to-end protocol for complex and heterogeneous data characterization, manipulation, processing, cleaning, analysis and validation. Specifically, the researchers used a Synthetic Minority Over-sampling Technique (SMOTE) to rebalance the data set. Rebalancing the dataset using SMOTE improved SVM’s classification accuracy from 76% to 96% and AdaBoost’s classification accuracy from 96% to 99%. Moreover, the study found that traditional statistical classification approaches (e.g., generalized linear model) failed to generate reliable predictions but machine learning-based classification methods performed very well in terms of predictive precision and reliability.
Among patients with kidney disease, researchers [ 71 ] developed a prediction model to forecast survival. Data collected from four facilities of University of Iowa Hospital and Clinics contains 188 patients with over 707 visits and features like blood pressure measures, demographic variables, and dialysis solution contents. Data was transformed using functional relation (i.e., the similarity between two or more features when two features have same values for a set of patients, they are combined to form a single feature) between the features. The data set was randomly divided into eight sub-sets. Sixteen classification rules were generated for the eight sub-sets using two classification algorithms—Rough Set (RS) and DT. Classes represented survival beyond three years, less than three years and undetermined. To make predictions, each classification rule (out of 16) had one vote and the majority vote decided the final predictive class. Transformed data increased predictive accuracy by 11% than raw data and DT (67% accuracy) performed better than RS (56% accuracy). The researchers suggested that this type of predictive analysis can be helpful in personalized treatment selection, resource allocation for patients, and designing clinical study. Among patients on kidney dialysis, another group of researchers [ 74 ] applied temporal pattern mining to predict hospitalization using biochemical data. Their result showed that amount of albumin—a type of protein float in blood—is the most important predictor of hospitalization due to kidney disease.
Among patients over 50 years of age, researchers [ 75 ] developed a data mining model to predict five years mortality using the EHR of 7463 patients. They used Ensemble Rotating Forest algorithm with alternating decision tree to classify the patients into two classes of life expectancy: (1) less than five years and (2) equal or greater than five years. Age, comorbidity count, previous record of hospitalization record, and blood urea nitrogen were a few of the significant features selected by correlation feature selection along with greedy stepwise search method. Accuracy achieved by this approach (AUC 0.86) was greater than the standard modified Charlson Index (AUC 0.81) and modified Walter Index (AUC 0.78). Their study showed that age, hospitalization prior the visit, and highest blood urea nitrogen were the most important factors for predicting five years morbidity. This five-year morbidity prediction model can be very helpful to optimally use resources like cancer screening for those patients who are more likely to be benefit from the resources.
Another group of researchers [ 76 ] addressed the limitations of existing software technology for disease diagnosis and prognosis, such as inability to handle data stream (DT), impractical for complex and large systems (Bayesian Network), exhaustive training process (NN). To overcome these restriction, authors proposed a decision tree based algorithm called “Very Fast Decision Tree (VFDT)”. Comparison with a similar system developed by IBM showed that VFDT utilizes lesser amount of system resources and it can perform real time classification.
Researchers have also used data mining to optimize the glaucoma diagnosis process [ 68 ]. Traditional approaches including Optical Coherence Tomography, Scanning Laser Polarimetry (SLP), and Heidelberg Retina Tomography (HRT) scanning methods are costly. This group used Fundus image data which is less costly and classified patient as either normal or glaucoma patient using SVM classifier. Before classification, authors selected significant features by using Higher Order Spectra (HOS) and Discrete Wavelet Transform (DWT) method combined and separately. Several kernel functions for SVM—all delivering similar levels of accuracy—were applied. Their approach produced 95% accuracy in glaucoma prediction. For diagnostic evaluation of chest imaging for suspicion for malignancy, researchers [ 69 ] designed trigger criteria to identify potential follow-up delays. The developed trigger predicted the patients who didn’t require follow-up evaluation. The analysis of the experiment result indicated that the algorithm to identify patients’ delays in follow-up of abnormal imaging is effective with 99% sensitivity and 38% specificity.
Data mining has also been applied to [ 72 ] compare three metrics to identify health care associated infections—Catheter Associated Bloodstream Infections, Catheter Associated Urinary Tract Infections and Ventilator Associated Pneumonia. Researchers compared traditional surveillance using National Healthcare Safety Network methodology to data mining using MedMined Data Mining Surveillance (CareFusion Corporation, San Diego, CA, USA), and administrative coding using ICD-9-CM. Traditional surveillance proved to be superior than data mining in terms of sensitivity, positive predictive value and rate estimation.
Data mining has been used in 38 studies of clinical decision-making CVD (7 articles), diabetes (seven articles), cancer (five articles), emergency care (two articles), intensive care (two articles), and other applications (16 articles). Most of the studies developed predictive models to facilitate decision-making and some developed decision support system or tools. Authors often tested their models with multiple algorithms; SVM was at the top of that list and often outperformed other algorithms. However, 15 [ 38 , 40 , 42 , 45 , 47 , 51 , 54 , 56 , 58 , 60 , 61 , 66 , 73 , 74 , 76 ] of the studies did not incorporate expert opinion from doctors, clinician, or appropriate healthcare personals in building models and interpreting results (see the study characteristics in Supplementary Materials Table S3 ). We also noted that there is an absence of follow-up studies on the predictive models, and specifically, how the models performed in dynamic decision-making situations, if doctors and healthcare professionals comfortable in using these predictive models, and what are the challenges in implementing the models if any exist? Existing literature does not focus on these salient issues.
4.2. Healthcare Administration
Data mining was applied to administrative purposes in healthcare in 32% (29 articles) of the articles reviewed. Researchers have applied data mining to: data warehousing and cloud computing; quality improvement; cost reduction; resource utilization; patient management; and other areas. Table 6 provides a list of these articles with major focus areas, problems analyzed and the data source.
Problem analyzed and data sources in healthcare administration.
4.2.1. Data Warehousing and Cloud Computing
Data warehousing [ 90 ] and cloud computing are used to securely and cost-effectively store the growing volume of electronic patient data [ 1 ] and to improve hospital outcomes including readmissions. To identify cause of readmission, researchers [ 89 ] developed an open source software—Analytic Information Warehouse (AIW). Users can design a virtual data model (VDM) using this software. Required data to test the model can be extracted in terms of a temporal ontology from the data warehouse and analysis can be performed using any standard analyzing tool. Another group of researchers took a similar approach to develop a Clinical Data Warehouse (CDW) for traditional Chinese medicine (TCM). The warehouse contains clinical information (e.g., symptoms, disease, and treatment) for 20,000 inpatients and 20,000 outpatients. Data was collected in a structured way using pre-specified ontology in electronic form. CDW provides an interface for online data mining, online analytical processing (OLAP) and network analysis to discover knowledge and provide clinical decision support. Using these tools, classification, association and network analysis between symptoms, diseases and medications (i.e., herbs) can be performed.
Apart from clinical purposes, data warehouses can be used for research, training, education, and quality control purposes. Such a data repository was created using the basic idea of Google search engine [ 92 ]. Users can pull the radiology report files by searching keywords like a simple google search following the predefined patient privacy protocol. Another data repository was created as a part of collaborative study between IBM and University of Virginia and its partner, Virginia Commonwealth University Health System was created [ 93 ]. The repository contains 667,000 patient record with 208 attributes. HealthMiner—a data mining package for healthcare created by IBM—was used to perform unsupervised analysis like finding associations, pattern and knowledge discovery. This study also showed the research benefits of this type of large data repository. Researchers [ 91 ] proposed a framework based on cloud computing and big data to unify data collected from different sources like public databases and personal health devices. The architecture was divided into 3 layers. The first layer unified heterogeneous data from different sources, the second layer provided storage support and facilitated data processing and analytics access, and the third layer provided result of analysis and platform for professionals to develop analytical tools. Some researchers [ 94 ] used mobile devices to collect personal health data. Users took part in a survey on their mobile devices and got a diagnosis report based on their health parameters input in the survey. Each survey data were saved in a cloud-based interface for effective storage and management. From user input stored in cloud, interactive geo-spatial maps were developed to provide effective data visualization facility.
4.2.2. Healthcare Cost, Quality and Resource Utilization
Ten articles applied data mining to cost reduction, quality improvement and resource utilization issues. One group of researchers predicted healthcare costs using an algorithmic approach [ 96 ]. They used medical claim data of 800,000 people collected by an insurance company over the period of 2004–2007. The data included diagnoses, procedures, and drugs. They used classification and clustering algorithms and found that these data mining algorithms improve the absolute prediction error more than 16%. Two prediction models were developed, one using both cost and medical information and the other used only cost information. Both models had similar accuracy on predicting healthcare costs but performed better than traditional regression methods. The study also showed that including medical information does not improve cost prediction accuracy. Risk-adjusted health care cost predictions, with diagnostic groups and demographic variables as inputs, have also been assessed using regression tree boosting [ 100 ]. Boosted regression tree and main effects linear models were used and fitted to predict current (2001) and prospective (2002) total health care costs per patient. The authors concluded that the combination of regression tree boosting and a diagnostic grouping scheme are a competitive alternative to commonly used risk-adjustment systems.
A sizable amount ($37.6 billion) of healthcare costs is attributable to medical errors, 45% of which stems from preventable errors [ 95 ]. To aid in physician decision-making and reduce medical errors, researchers [ 95 ] proposed a data mining-based framework-Sequential Clustering Algorithm. They identified patterns of treatment plans, tests, medication types and dosages prescribed for specific diseases, and other services provided to treat a patient throughout his/her stay in the hospital. The proposed framework was based on cloud computing so that the knowledge extracted from the data could be shared among hospitals without sharing the actual record. They proposed to share models using Virtual Machine (VM) images to facilitate collaboration among international institutions and prevent the threat of data leakage. This model was implemented in two hospitals, one in Taiwan and another in Mongolia. To identify best practices for specific diseases and prevent medical errors, another group of researchers [ 101 ] proposed a decision support system using information extraction from online documents through text and data mining. They focused on evidence based management, quality control, and best practice recommendations for medical prescriptions.
Length of Stay (LOS) is another important indicator of cost and quality of care. Accurate prediction of LOS can lead to efficient management of hospital beds and resources. To predict LOS for CAD patients, researchers [ 98 ] compared multiple models—SVM, ANN, DT and an ensemble algorithm, combing SVM, C5.0, and ANN. Ensemble algorithm and SVM produced highest accuracy, 95.9% and 96.4% respectively. In contrast, ANN was least accurate with 53.9% accuracy wherein DT achieved 83.5% accuracy. Anticoagulant drugs, nitrate drugs, and diagnosis were the top three predictors along with diastolic blood pressure, marital status, sex, presence of comorbidity, and insurance status.
To predict healthcare quality, researchers [ 104 ] used sentiment analysis (computationally categorizing opinions into categories like positive, negative and neutral) on patients’ online comments about their experience. They found above 80% agreement between sentiment analysis from online forums and traditional paper based surveys on quality prediction (e.g., cleanliness, good behavior, recommendation). Proposed approach can be an inexpensive alternative to traditional surveys and reports to measure healthcare quality.
Identification of influential factors in insurance coverage using data mining can aid insurance providers and regulators to design targeted service, additional service or proper allocation of resources to increase coverage rates. To develop a classification model to identify health insurance coverage, researchers [ 103 ] used data mining techniques. Based on 23 socio-economic, lifestyle and demographic factors, they developed a classification model with two classes, Insured and uninsured. The model was solved by ANN and DT. ANN provided 4% more accuracy than DT in predicting health insurance coverage. Among the factors, income, employment status, education, and marital status were the most important predictive factors of insurance coverage.
Among patients with lung cancer, researchers [ 97 ] investigated healthcare resource utilization (i.e., the number of visits to the medical oncologists) characteristics. They used DT, ANN and LR separately and an ensemble algorithm combining DT and ANN which resulted in the greatest accuracy (60% predictive accuracy). DT was employed to identify the important predictive features (among demographics, diagnosis, and other medical information) and ANN for classification. Data mining revealed that the utilization of healthcare resources by lung cancer patients is “supply-sensitive and patient sensitive” where supply represents availability of resources in certain region and patient represents patient preference and comorbidity. A resource allocation monitoring model for better management of primary healthcare network has also been developed [ 99 ]. Researchers considered the primary-care network as a collection of hierarchically connected modules given that patients could visit multiple physicians and physicians could have multiple care location, which is an indication of imbalanced resource distribution (e.g., number of physicians, care locations). The first level of the hierarchy consisted of three modules: health activities, population, and health resources. The second level monitored the healthcare provider availability and dispersion. The third level considered the actual visits, physicians and their availability, accessibility, and unlisted (i.e., without any assigned physician) patients. The top level of this network conducted an overall assessment of the network and made allocation accordingly. This hierarchical model was developed for a specific region in Slovenia, however, it could be easily adapted for any other region.
Overuse of screening and tests by physicians also contributes to inefficiencies and excess costs [ 102 ]. Current practice in pathology diagnosis is limited by disease focus. As an alternative to disease based system, researchers [ 102 ] used data mining in cooperation with case-based reasoning to develop an evidence based decision support system to decrease the use of unnecessary tests and reduce costs.
4.2.3. Patient Management
Patient management involves activities related to efficient scheduling and providing care to patients during their stay in a healthcare institute. Researchers [ 105 ] developed an efficient scheduling system for a rural free clinic in the United States. They proposed a hybrid system where data mining was used to classify the patients and association rule mining was used to assign a “no-show” probability. Results obtained from data mining were used to simulate and evaluate different scheduling techniques. On the other hand, these schedules could be divided into visits with administrative purposes and medical purposes. Researchers [ 108 ] suggested that patients who visit the health center for administrative purposes take less time than the patients with medical reasons. They proposed a predictive model to forecast the number of visits for administrative purposes. Their model improved the scheduling system with time saving of 21.73% (660,538 min). In contrast to administrative information/task seeking patients, some patients come for medical care very frequently and consume a large percentage of clinical workload [ 107 ]. Identifying the risk factors for frequent visit to health centers can help in reducing cost and resource utilization. A study among 85 working age “frequent attenders” identified the primary risk factors using Bayesian classification technique. The risk factors are, “high body mass index, alcohol abstinence, irritable bowel syndrome, low patient satisfaction, and fear of death” [ 107 ].
Improving publicly reported patient safety outcomes is also critical to healthcare institutions. Falls are one such outcome and are the most common and costly source of injury during hospitalization [ 110 ]. Researchers [ 109 ] analyzed the important factors related to patient falls during hospitalization. First, the authors selected significant features by Chi-square test (10 features out of 72 fall related variables were selected) and then applied ANN to develop a predictive model which achieves 0.77 AUC value. Stepwise logistic regression achieved 0.42 AUC value with 3 important variables. Both models showed that the fall assessment by nurses and use of anti-psychotic medication are associated with a lower risk of falls, and the use of diuretics is associated with an increased risk of falls. Another group of researchers [ 110 ] used fall related injury data to validate the structured information in EMR from clinical notes with the help of text mining. A group of nurses manually reviewed the electronic records to separate the correct documents from the erroneous ones which was considered as the basis of comparison. Authors employed both supervised (using a portion of manually labeled files as training set) and unsupervised technique (without considering the file labels) to classify and cluster the records. The unsupervised technique failed to separate the fare documents from the erroneous ones, wherein supervised technique performed better with 86% of fare documents in one cluster. This method can be applicable to semi-automate the EMR entry system.
4.2.4. Other Applications
Data mining has beed applied [ 111 ] to investigate the relationship between physician’s training at specific schools, procedures performed, and costs of the procedure. Researchers explored this relationship at three level: (1) they explored the distribution of procedures performed; (2) the relationship between procedures performed by physician and their alma mater—the institute that a doctor attended or got his/her degree from; and (3) geographic distribution of amount billed and payment received. This study suggested that medical school training does relate to practice in terms of procedures performed and bill charged. Patients can also provide useful information about physicians and their performance. Another group of researchers [ 112 ] used topic modeling algorithm—Latent Dirichlet Allocation (LDA)—to understand patients’ review of physicians and their concerns.
Data mining has also been applied [ 115 ] to analyze the information seeking behavior of health care professionals, and to assess the feasibility of measuring drug safety alert response from the usage logs of online medical information resources. Researchers analyzed two years of user log-in data in UpToDate website to measure the volume of searches associated with medical conditions and the seasonal distribution of those searches. In addition, they used a large collection of online media articles and web log posts as they characterized food and drug alert through the changes in UpToDate search activity compared to the general media activity. Some researchers [ 113 ] examined changes of key performance indicators (KPIs) and clinical workload indicators in Greek National Health System (NHS) hospitals with the help of data mining. They found significant changes in KPIs when necessary adjustments (e.g., workload) were made according to the diagnostic related group. The results remained for general hospitals like cancer hospitals, cardiac surgery as well as small health centers and regional hospitals. Their findings suggested that the assessment methodology of Greek NHS hospitals should be re-evaluated in order to identify the weaknesses in the system, and improve overall performance. And in home healthcare, another group of researchers [ 116 ] reviewed why traditional statistical analysis fails to evaluate the performance of home healthcare agencies. The authors proposed to use data mining to identify the drivers of home healthcare service among patients with heart failure, hip replacement, and chronic obstructive pulmonary disease using length of stay and discharge destination.
The relationship between epidemiological and genetic evidence and post market medical device performance has been evaluated using HCUPNet data [ 114 ]. This feasibility study explored the potential of using publicly accessible data for identifying genetic evidence (e.g., comorbidity of genetic factors like race, sex, body structure, and pneumothorax or fibrosis) related to devices. It focused on the ventilation-associated iatrogenic pneumothorax outcome in discharge of mechanical ventilation and continuous positive airway pressure (CPAP). The results demonstrated that genetic evidence-based epidemiologic analysis could lead to both cost and time efficient identification of predictive features. The literature of data mining applications in healthcare administration encompasses efficient patient management, healthcare cost reduction, quality of care, and data warehousing to facilitate analytics. We identified four studies that used cloud-based computing and analytical platforms. Most of the research proposed promising ideas, however, they do not provide the results and/or challenges during and after implementation. An ideal example of implementation could be the study of efficient appointment scheduling of patients [ 108 ].
4.3. Healthcare Privacy and Fraud Detection
Health data privacy and medical fraud are issues of prominent importance [ 118 ]. We reviewed four articles—displayed and described in Table 7 —that discussed healthcare privacy and fraud detection.
List of papers in healthcare privacy and fraud detection.
The challenges of privacy protection have been addressed by a group of researchers [ 122 ] who proposed a new anonymization algorithm for both distributed and centralized anonymization. Their proposed model performed better than K-anonymization model in terms of retaining data utility without losing much data privacy (for K = 20, the discernibility ratio—a normalized measure of data quality—of the proposed approach and traditional K-anonymization method were 0.1 and 0.4 respectively). Moreover, their proposed algorithm could handle large scale, high dimensional datasets. To address the limitations of today’s healthcare information systems—EHR data systems limited by lack of inter-operability, data size, and security—a mobile cloud computing-based big data framework has been proposed [ 119 ]. This novel cloud-based framework proposed storing EHR data from different healthcare providers in an Internet provider’s facility, offering providers and patients different levels of access and authority. Security would be ensured by using encryption algorithms, one-time passwords, or 2-factor authentication. Big data analytics would be handled using Google big query or MapReduce software. This framework could reduce cost, increase efficiency, and ensure security compared to the traditional technique which uses de-identification or anonymization technique. This traditional technique leaves healthcare data vulnerable to re-identification. In a case study, researchers demonstrated that hackers can make association between small pieces of information and can identify patients [ 120 ]. The case study made use of personal information provided in two Medicare social networking sites, MedHelp and Mp and Th1 to identify an individual.
Detection of fraud and abuse (i.e., suspicious care activity, intentional misrepresentation of information, and unnecessary repetitive visits) uses big data analytics. Using gynecological hospital data, researchers [ 121 ] developed a framework from two domain experts manually identifying features of fraudulent cases from a data pool of treatment plans doctors frequently follow. They applied this framework to Bureau of National Health Insurance (BNHI) data from Taiwan; their proposed framework detected 69% of the fraudulent cases, which improved the existing model that detected 63% of the fraudulent cases.
In summary, patient data privacy and fraud detection are of major concern given increasing use of social media and people’s tendency to put personal information on social media. Existing data anonymization or de-identification techniques can become less effective if they are not designed considering the fact that a large portion of our personal information is now available on social media.
4.4. Mental Health
Mental illness is a global and national concern [ 123 ]. According to the National Survey on Drug Use and Health (NSDUH) data from 2010 to 2012, 52.2% of U.S. population had either mental illness, or substance abuse/dependence [ 124 ]. Additionally, nearly 30 million people in the U.S. suffer from anxiety disorders [ 125 ]. Table 8 summarizes the four articles we reviewed that apply data mining in analyzing, diagnosing, and treating mental health issues.
List of data mining application in mental health with data sources.
To classify developmental delays of children based on illness, researchers [ 126 ] examined the association between illness diagnosis and delays by building a decision tree and finding association between cognitive, language, motor, and social emotional developmental delays. This study has implications for healthcare professionals to identify and intervene on delays at an early stage. To assist physicians in monitoring anxiety disorder, another group of researchers [ 125 ] developed a data mining based personalized treatment. The researchers used Context Awareness Information including static (personal information like, age, sex, family status etc.) and dynamic (stress, environmental, and symptoms context) information to build static and dynamic user models. The static model contained personal information and the dynamic model contained four treatment-supportive services (i.e., lifestyle and habits pattern detection service, context and stress level pattern detection service, symptoms and stress level pattern detection service, and stress level prediction service). Relations between different dynamic parameters were identified in first three services and the last service was used for stress level prediction under different scenarios. The model was validated using data from 27 volunteers who were selected by anxiety measuring test.
To predict early diagnosis for mental disorders (e.g., insomnia, dementia), researchers developed a model detecting abnormal physical activity recorded by a wearable device [ 127 ]. They performed two experiments to compare the development of a reference model using historical user physical movement data. In the first experiment, users wore the watch for one day and based on that day, a reference behavior model was developed. After 22 days, the same user used it again for a day and abnormality was detected if the user’s activities were significantly different from the reference model. In the second experiment, users used the watch regularly for one month. Abnormality was detected with a fuzzy valuation function and validated with user’s reported activity level. In both experiments, users manually reported their activity level, which was used as a validating point, only two out of 26 abnormal events were undetected. Through these two experiments, the researchers claimed that their model could be useful for both online and offline abnormal behavior detection as the model was able to detect 92% of the unusual events.
To classify schizophrenia, another study [ 128 ] used free speech (transcribed text) written or verbalized by psychiatric patients. In a pool of patients with schizophrenia and control subjects, using supervised algorithms (SVM and DT), they discriminated between patients with schizophrenia and normal control patients. SVM achieved 77% classification accuracy whereas DT achieved 78% accuracy. When they added patients with mania to the pool, they were unable to differentiate patients with schizophrenia.
Use of data analytics in diagnosing, analyzing, or treating mental health patients is quite different than applying analytics to predict cancer or diabetes. Context of data (static, dynamic, or unobservable environment) seemed more important than volume in this case [ 125 ], however, this is not always adopted in literature. A model without situational awareness (a context independent model) may lose predictive accuracy due to the confounding effect of surrounding environment [ 129 ].
4.5. Public Health
Seven articles addressed issues that were not limited to any specific disease or a demographic group, which we classified as public health problems. Table 9 contains the list of papers considering public health problems with data sources.
List of data mining application in public health with data sources.
To make data mining accessible to non-expert users, specifically public health decision makers who manage public cancer treatment programs in Brazil, researchers [ 134 ] developed a framework for an automated data mining system. This system performed a descriptive analysis (i.e., identifying relationships between demography, expenditure, and tumor or cancer type) for public decision makers with little or no technical knowledge. The automation process was done by creating pre-processed database, ontology, analytical platform and user interface.
Analysis of disease outbreaks has also applied data analytics. [ 131 , 133 ] Influenza, a highly contagious disease, is associated with seasonal outbreaks. The ability to predict peak outbreaks in advance would allow for anticipatory public health planning and interventions to lessen the effect of the outbreaks. To predict peak influenza visits to U.S. military health centers, researchers [ 131 ] developed a method to create models using environmental and epidemiological data. They compared six classification algorithms—One-Classifier 1, One-Classifier 2 [ 137 ], a fusion of the One-Classifiers, DT, RF, and SVM. Among them, One-Classifier 1 was the most efficient with F-score 0.672 and SVM was second best with F-score 0.652. To examine the factors that drive public and professional search patterns for infectious disease outbreaks another group of researchers [ 133 ] used online behavior records and media coverage. They identified distinct factors that drive professional and layperson search patterns with implications for tailored messaging during outbreaks and emergencies for public health agencies.
To store and integrate multidimensional and heterogeneous data (e.g., diabetes, food, nutrients) applied to diabetes management, but generalizable to other diseases researchers [ 130 ] proposed an intelligent information management framework. Their proposed methodology is a robust back-end application for web-based patient-doctor consultation and e-Health care management systems with implications for cost savings.
A real-time medical emergency response system using the Internet of Things (networking of devices to facilitate data flow) based body area networks (BANs)—a wireless network of wearable computing devices was proposed by researchers [ 136 ]. The system consists of “Intelligent Building”—a data analysis model which processes the data collected from the sensors for analysis and decision. Though the author claims that the proposed system had the capability of efficiently processing wireless BAN data from millions of users to provide real-time response for emergencies, they did not provide any comparison with the state-of-the-art methods.
Decision support tools for regional health institutes in Slovenia [ 135 ] have been developed using descriptive data mining methods and visualization techniques. These visualization methods could analyze resource availability, utilization and aid to assist in future planning of public health service.
To build better customer relations management at an Iranian hospital, researchers [ 132 ] applied data mining techniques on demographic and transactions information. The authors extended the traditional Recency, Frequency, and Monetary (RFM) model by adapting a new parameter “Length” to estimate the customer life time value (CLV) of each patient. Patients were separated into classes according to estimated CLV with a combination of clustering and classification algorithms. Both DT and ANN performed similarly in classification with approximately 90% accuracy. This type of stratification of patient groups with CLV values would help hospitals to introduce new marketing strategies to attract new customers and retain existing ones.
The application of data mining to public health decision-making has become increasingly common. Researchers utilized data mining to design healthcare programs and emergency response, to identify resource utilization, patient satisfaction as well as to develop automated analytics tool for non-expert users. Continuation of this effort could lead to a patient-centered, robust healthcare system.
4.6. Pharmacovigilance
Pharmacovigilance involves post-marketing monitoring and detection of adverse drug reactions (ADRs) to ensure patient safety [ 138 ]. The estimated annual social cost of ADR events exceeds one billion dollars, making it an important part of healthcare system [ 139 ]. Characteristics of the nine papers addressing pharmacovigilance are displayed in Table 10 .
List of data mining application in pharmacovigilance with data sources.
Researchers considered muscular and renal AEs caused by pravastatin, simvastatin, atorvastatin, and rosuvastatin by applying data mining techniques to the FDA’s Adverse Event Reporting System (FAERS) database reports from 2004 to 2009 [ 143 ]. They found that all statins except simvastatin were associated with muscular AE; rosuvastatin had the strongest association. All statins, besides atorvastatin, were associated with acute renal failure. The criteria used to identify significant association were: proportional reporting ratio (PRR), reporting odds ratio (ROR), information component (IC), and empirical Bayes geometric mean (EBGM). In another study of AEs related to statin family, researchers used a Korean claims database [ 145 ] and showed that a relative risk-based data-mining approach successfully detected signals for rosuvastatin.
Three more studies used the FDA’s AERS report database. In an examination of ADR “hypersensitivity” to six anticancer agents [ 142 ] data mining results showed that Paclitaxel is associated with mild to lethal reaction wherein Docetaxel is associated to lethal reaction, and the other four drugs were not associated to hypersensitivity [ 142 ]. Another researcher [ 139 ] argued that AEs can be caused not only by a single drug, but also by a combination of drugs [ 140 ]. They showed that that 84% of the AERs reports contain an association between at least one drug and two AEs or two drugs and one AE. Another group [ 138 ] increased precision in detecting ADRs by considering multiple data sources together. They achieved 31% (on average) improvement in identification by using publicly available EHRs in combination with the FDA’s AERS reports.
Furthermore, dose-dependent ADRs have been identified by researchers using models developed from structured and unstructured EHR data [ 141 ]. Among the top five drugs associated with ADRs, four were found to be related to dose [ 141 ]. Pharmacovigilance activity has also been prioritized using unstructured text data in EHRs [ 144 ]. In traditional pharmacovigilance, ADRs are unknown. While looking for association between a drug and any possible ADR, it is possible to get false signals. Such false signals can be avoided if a list of possible ADRs is already known. Researchers [ 144 ] developed an ordered list of 23 ADRs which can be very helpful for future pharmacovigilance activities. To detect unexpected and rare ADRs in real-world healthcare administrative databases, another group of researchers [ 146 ] designed an algorithm—Unexpected Temporal Association Rules (UTARs)—that performs more effectively than existing techniques.
We identified one study that used data outside of adverse event reports or HER data. For early detection of ADR, one group of researchers used online forums [ 140 ]. They identified the side effect of a specific drug called “Erlotinib” used for lung cancer. Sentiment analysis—a technique of categorizing opinions—on data collected from different cancer discussion forums showed that 70% of users had a positive experience after using this drug. Users most frequently reported were acne and rash. Apart from pharmacovigilance, this type of analysis can be very helpful for the pharmaceutical companies to analyze customer feedback. Researchers can take advantage of the popularity of social media and online forums for identifying adverse events. These sources can provide signals of AEs quicker than FDA database as it takes time to update the database. By the time AE reports are available in the FDA database, there could already be significant damage to patient and society. Moreover, it can help to avoid the limitations of FDA AERS database like biased reporting and underreporting [ 141 ].
5. Theoretical Study
Twenty-five of the articles we reviewed focus on the theoretical aspects of the application of data mining in healthcare including designing the database framework, data collection, and management to algorithmic development. These intellectual contributions extend beyond the analytical perspective of data—descriptive, predictive or prescriptive analytics—to the sectors and problems highlighted in Table 11 .
Problem analyzed in theoretical studies.
The existing theoretical literature on disease control highlighted the current state of epidemics, cancer and mental health. To help physicians make real-time decisions about patient care, one group of researchers [ 147 ] proposed a real-time EMR data mining based clinical decision support system. They emphasized the need to have an anonymized EMR database which can be explored by using a search engine similar to web search engine. In addition, they focused on designing a framework for next generation EMR-based database that can facilitate the clinical decision-making process, and is also capable of updating a central population database once patients’ recent (new) clinical records are available. Another researcher [ 148 ] forecasted future challenges in infection control that entails the importance of having timely surveillance system and prevention programs in place. To that end, they necessitate the formation, control and utilization of fully computerized patient record and data-mining-derived epidemiology. Finally, they recommended performance feedback to caregivers, wide accessibility of infection prevention tools, and access to documents like lessons learned and evidence-based best practices to strengthen the infection control, surveillance, and prevention scheme. Authors in [ 150 ] addressed the activities executed by national Institute of Mental Health (NIMH) in collaboration with other state organizations (e.g., Substance Abuse and Mental Health Service Administration (SAMSHSA), Center for Mental Health Service (CMHS) to promote optimal collection, pooling/aggregation, and use of big data to support ongoing and future researches of mental health practices. The outcome summary showcased that effective pooling/aggregation of state-level data from different sources can be used as a dashboard to set priorities to improve service qualities, measure system performance and to gain specific context-based insights that are generalizable and scalable across other systems, leading to a successful learning-based mental health care system. Another group of researchers [ 150 ] outlined the barriers and potential benefits of using big data from CancerLinQ (a quality and measurement reporting system as an initiative of the American Society of Clinical Oncology (ASCO) that collects information from EHRs of cancer patients for oncologists to improve the outcome and quality of care they provide to their patients). However, the authors also mentioned that these benefits are contingent upon the confidence of the patients, encouraging them to share their data out of the belief that their health records would be used appropriately as a knowledge base to improve the quality of the health care of others, as it is for themselves. This motivated ASCO to ensure that proper policies and procedures are in place to deal with the data quality, data security and data access, and adopt a comprehensive regulatory framework to ensure patients’ data privacy and security.
Another group of researchers [ 151 ] data quality and database management to quantify, and consequentially understand the inherent uncertainty originating from radiology reporting system. They discussed the necessity of having a structured reporting system and emphasized the use of standardize language, leading to Natural Language Processing (NLP). Furthermore, they also indicated the need for creating a Knowledge Discovery Database (KDD) which will be consistent to facilitate the data-driven and automated decision support technologies to help improving the care provided to patients based on enhanced diagnosis quality and clinical outcome. A group of authors in [ 152 ] pointed that the success derived from the current trend of big-data analytics largely depends on how better the quality of the data collected from variety of sources are ensured. Their findings imply that the data quality should be assessed across the entire lifecycle of health data by considering the errors and inaccuracies stemmed from multiple of sources, and should also quantify the impact that data collection purpose on the knowledge and insights derived from the big data analytics. For that to ensure, they recommend that enterprises who deal with healthcare big data should develop a systematic framework including custom software or data quality rule engines, leading to an effective management of specific data-quality related problems. Researchers in [ 155 ] uncovered the lack of connection between phenomenological and mechanistic models in computational biomedicines. They emphasized the importance of big data which, when successfully extracted and analyzed, followed by the combination with Virtual Physiological Human (VPH)—an initiative to encourage personalized healthcare—can afford with effective and robust medicine solutions. In order for that to happen, they mentioned some challenges (e.g., confidentiality, volume and complexity of big data; integration of bioinformatics, systems biology and phenomics data; efficient storage of partial or complete data within organization to maximize the performance of overall predictive analytics) and concluded that these need to be addressed for successful development of big data technologies in computational medicines, enabling their adoption in clinical settings. Even though big data can generate significant value in modern healthcare system, researchers in [ 154 ] stated that without a set of proper IT infrastructures, analytical and visualization tools, and interactive interfaces to represent the work flows, the insights generated from big data will not be able to reach its full potential. To overcome this, they recommended that health care organizations engaging in data sharing devise new policies to protect patients’ data against potential data breaches.
Three papers [ 155 , 156 , 157 ] considered health care policies and ethical and legal issues. One [ 155 ] outlined a national action plan to incorporate sharable and comparable nursing data beyond documentation of care into quality reporting and translational research. The plan advocates for standardized nursing terminologies, common data models, and information structures within EHRs. Another paper [ 157 ] analyzed the major policy, ethical, and legal challenges of performing predictive analytics on health care big data. Their proposed recommendations for overcoming challenges raised in the four-phase life cycle of a predictive analytics model (i.e., data acquisition, model formulation and validation, testing in real-world setting and implementation and use in broader scale) included developing a governance structure at the earliest phase of model development to guide patients and participating stakeholders across the process (from data acquisition to model implementation). They also recommended that model developers strictly comply with the federal laws and regulations in concert with human subject research and patients information privacy when using patients’ data. And another paper [ 156 ] explored four central questions regarding: (i) aspects of big-data most relevant to health care, (ii) policy implications, (iii) potential obstacles in achieving policy objectives, and (iv) availability of policy levers, particularly for policy makers to consider when developing public policy for using big data in healthcare. They discussed barriers (including ensuring transparency among patients and health care providers during data collection) to achieve policy objectives based on a recent UK policy experiment, and argued for providing real-life examples of ways in which data sharing can improve healthcare.
Three papers [ 158 , 159 , 160 ] offered examples of realistic ways such as establishing policy leadership and risk management framework combining commercial and health care entities to recognize existing privacy related problem and devise pragmatic and actionable strategies of maintaining patient privacy in big data analytics. One paper [ 158 ] provided a policy overview of health care and data analytics, outlined the utility of health care data from a policy perspective, reviewed a variety of methods for data collection from public and private sources, mobile devices and social media, examined laws and regulations that protect data and patients’ privacy, and discussed a dynamic interplay among three aspects of today’s big data driven personal health care—policy goals to tackle both cost, population health problem and eliminate disparity in patient care while maintaining their privacy. Another study [ 159 ] proposed a Secure and Privacy Preserving Opportunistic Computing (SPOC) framework to be used in healthcare emergencies focused on collecting intensive personal health information (through mobile devices like smart phone or wireless sensors) with minimal privacy disclosure. The premise of this framework is that when a user of this system (called medical user) faces any emergency, other users in the vicinity with similar disease or symptom (if available) can come to help that user before professional help arrives. It is assumed that two persons with similar disease are skilled enough to help each other and the threshold of similarity is controlled by the user. And in physician prescribing—another paper [ 160 ] identified strategies for data mining from physicians’ prescriptions while maintaining patient privacy.
Theoretical research on personalized-health care services—treatment plans designed for someone based on the susceptibility of his/her genomic structure to a disease—also emerged from the literature review. One study [ 161 ] highlighted the potential of powerful analytical tools to open an avenue for predictive, preventive, participatory, and personalized (P4) medicine. They suggested a more nuanced understanding of the human systems to design an accurate computational model for P4 medicine. Reviewing the research paradgims of current person-centered approaches and traditions, another study [ 162 ] advocated a transdisciplinary and complex systems approach to improve the field. They synthesized the emerging aproaches and methodologies and highlighted the gaps between academic research and accessibility of evaluation, informatics, and big data from health information systems. Another paper [ 163 ] reviewed the availability of big data and the role of biomedical informatics in personalized medicine, emphasizing the ethical concerns related to personalized medicines and health equity. Personalized medicine has a potential to reduce healthcare cost, however, the researchers think it can create race, income, and educational disparity. Certain socioeconomic and demographic groups currently have less or no access to healthcare and data driven personalized medicine will exclude those groups, increasing disparities. They also highlighted the impact of EHRs and CDWs on the field of personalized medicine through acclerated research and decreased the delivery time of new technologies.
A myriad of extant theoretical points has also been identified in the literature. These topics range from exploiting big data to: study the paradigm shift in healthcare policy and management from prioritizing volume to value [ 164 , 167 ]; aid medical device consumers in their decision-making [ 166 ]; improve emergency departments [ 169 ]; perform command surveillance and policy analysis for Army leadership [ 170 ]; to comparing different simulation methods (i.e., systems dynamics, discrete event simulation and agent based modeling) for specific health care system problems like resource allocation, length of stay [ 165 ]; to the ethical challenges of security, management, and ownership [ 170 ]. Another researcher outlined the challenges the E.U. is facing in data mining given numerous historical, technical, legal, and political barriers [ 168 ].
6. Future Research and Challenges
Data mining has been applied in many fields including finance, marketing, and manufacturing [ 172 ]. Its application in healthcare is becoming increasingly popular [ 173 ]. A growing literature addresses the challenges of data mining including noisy data, heterogeneity, high dimensionality, dynamic nature, computational time. In this section, we focus on future research applications including personalized care, information loss in preprocessing, collecting healthcare data for research purposes, automation for non-experts, interdisciplinarity of study and domain expert knowledge, integration into the healthcare system, and prediction-specific to data mining application and integration in healthcare.
- Personalized care
The EMR is increasingly used to document demographic and clinician patient information [ 1 ]. EMR data can be utilized to develop personalized care plans, enhancing patient experience [ 162 ] and improving care quality.
- Loss of information in pre-processing
Pre-processing of data, including handling missing data, is the most time-consuming and costly part of data mining. The most common method used in the papers reviewed was deletion or elimination of missing data. In one study, approximately 46.5% of the data and 363 of 410 features were eliminated due to missing values [ 49 ]. In another, researchers [ 98 ] were only able to use 2064 of 4948 observations (42%) [ 98 ]. By eliminating missing value cases and outliers, we are losing a significant amount of information. Future research should focus on finding a better method of missing value estimation than elimination. Moreover, data collection techniques should be developed or modified to avoid this issue.
Similar to missing data, deletion or elimination is a common way to handle outliers [ 174 ]. However, as illustrated in one of the studies we reviewed [ 48 ], outliers can be used to gain information about rare forms of diseases. Instead of neglecting the outliers, future research should analyze them to gain insight.
- Collecting healthcare data for research purpose
Traditionally, the primary objective of data collection in healthcare is documentation of patient condition and care planning [ 109 ]. Including research objectives in the data collection process through structured fields could yield more structured data with fewer cases of error and missing values [ 64 ]. A successful example of data collection for research purpose is the Study of Health in Pomerania (SHIP) [ 175 ]. The objective of SHIP was to identify common diseases, population level risk factors, and overall health of people living in the north-east region of Germany. This study only suffered from one “mistake” for every 1000 data entries [ 175 ] which ensures a structured form of data with high reliability, less noise and fewer missing values. We can take advantage of current documentation processes (EMR or EHR) by modifying them to collect more reliable and structured data. Long-term vision and planning is required to introduce research purpose in healthcare data collection.
- Automation of data mining process for non-expert users
The end users of data mining in healthcare are doctors, nurses, and healthcare professionals with limited training in analytics. One solution for this problem is to develop an automated (i.e., without human supervision) system for the end users [ 134 ]. A cloud-based automated structure to prevent medical errors could also be developed [ 95 ]; but the task would be challenging as it involves different application areas and one algorithm will not have similar accuracy for all applications [ 134 ].
- Interdisciplinary nature of study and domain expert knowledge
Healthcare analytics is an interdisciplinary research field [ 134 ]. As a form of analytics, data mining should be used in combination with expert opinion from specific domains—healthcare and problem specific (i.e., oncologist for cancer study, cardiologist for CVD) [ 106 ]. Approximately 32% of the articles in analytics did not utilize expert opinion in any form. Future research should include members from different disciplines including healthcare.
- Integration in healthcare system
Very few articles reviewed made an effort to integrate the data mining process into the actual decision-making framework. The impact of knowledge discovery through data mining on healthcare professional’s workload and time is unclear. Future studies should consider the integration of the developed system and explore the effect on work environments.
- Prediction error and “The Black Swan” effect
In healthcare, it is better not to predict than making an erroneous prediction [ 46 ]. A little under half of the literature we identified in analytics is dedicated to prediction but, none of the articles discussed the consequence of a prediction error. High prediction accuracy for cancer or any other disease does not ensure an accurate application to decision-making.
Moreover, prediction models may be better at predicting commonplace events than rare ones [ 176 ]. Researchers should develop more sophisticated models to address the unpredictable, “The Black Swan” [ 176 ]. One study [ 101 ] addressed a similar issue in evidence based recommendations for medical prescriptions. Their concern was, how much evidence should be sufficient to make a recommendation. Many of the studies in this review do not address these salient issues. Future research should address the implementation challenges of predictive models, especially how the decision-making process should adapt in case of errors and unpredictable incidents.
7. Conclusions
The development of an informed decision-making framework stems from the growing concern of ensuring a high value and patient-focused health care system. Concurrently, the availability of big data has created a promising research avenue for academicians and practitioners. As highlighted in our review, the increased number of publications in recent years corroborates the importance of health care analytics to build improved health care systems world-wide. The ultimate goal is to facilitate coordinated and well-informed health care systems capable of ensuring maximum patient satisfaction.
This paper adds to the literature on healthcare and data mining ( Table 1 ) as it is the first, to our knowledge, to take a comprehensive review approach and offer a holistic picture of health care analytics and data mining. The comprehensive and methodologically rigorous approach we took covers the application and theoretical perspective of analytics and data mining in healthcare. Our systematic approach starting with the review process and categorizing the output as analytics or theoretical provides readers with a more widespread review with reference to specific fields.
We also shed light on some promising recommendations for future areas of research including integration of domain-expert knowledge, approaches to decrease prediction error, and integration of predictive models in actual work environments. Future research should recommend ways so that the analytic decision can effectively adapt with the predictive model subject to errors and unpredictable incidents. Regardless of these insightful outcomes, we are not constrained to mention some limitations of our proposed review approach. The sole consideration of academic journals and exclusion of conference papers, which may have some good coverage in this sector is the prime limitation of this review. In addition to this, the search span was narrowed to three databases for 12 years which may have ignored some prior works in this area, albeit the increasing trend since 2005 and less number of publications before 2008 can minimize this limitation. The omission of articles published in languages other than English can also restrict the scope of this review as related papers written in other languages might be evident in the literature. Moreover, we did not conduct forward (reviewing the papers which cited the selected paper) and backward (reviewing the references in the selected paper and authors’ prior works) search as suggested by Levy and Ellis [ 31 ].
Despite these limitations, the systematic methodology followed in this review can be used in the universe of healthcare areas.
Supplementary Materials
The following are available online at http://www.mdpi.com/2227-9032/6/2/54/s1 , Table S1: PRISMA checklist, Table S2: Modified checklists and comparison, Table S3: Study characteristics, Table S4: Classification of reviewed papers by analytics type, application area, data type, and data mining techniques.
Author Contributions
Contribution of the authors can be summarized in following manner. Conceptualization: M.S.I., M.N.-E.-A.; Formal analysis: M.S.I., M.M.H., X.W.; Investigation: M.S.I., M.M.H., X.W.; Methodology: M.S.I.; Project administration: M.S.I., M.N.-E.-A.; Supervision: M.N.-E.-A.; Visualization: M.S.I., X.W.; Writing—draft: M.S.I., M.M.H., H.D.G.; Writing—review and editing: M.S.I., M.M.H., H.D.G., M.N.-E.-A.
Germack is supported by CTSA Grant Number TL1 TR001864 from the National Center for Advancing Translational Science (NCATS), a component of the National Institutes of Health (NIH). The content is solely the responsibility of the authors and does not necessarily represent the official views of this organization.
Conflicts of Interest
The authors declare no conflict of interest.
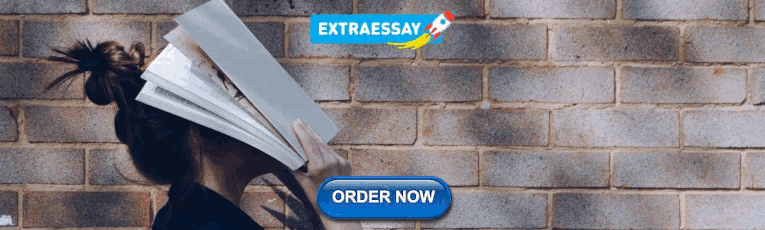
COMMENTS
Qualitative descriptive designs are common in nursing and healthcare research due to their inherent simplicity, flexibility and utility in diverse healthcare contexts. However, the application of descriptive research is sometimes critiqued in terms of scientific rigor. Inconsistency in decision making within the research process coupled with a ...
INTRODUCTION. In our previous article in this series, [ 1] we introduced the concept of "study designs"- as "the set of methods and procedures used to collect and analyze data on variables specified in a particular research question.". Study designs are primarily of two types - observational and interventional, with the former being ...
Two main elements constant with qualitative description studies in health care research are learning from the participants and their descriptions, and second, using this knowledge to ... Colorafi K. J., Evans B. (2016). Qualitative descriptive methods in health science research. HERD: Health Environments Research & Design Journal, 9(4), 16-25 ...
QD is useful for providing rich descriptions and insights into health issues and is particularly relevant where information is needed directly from those experiencing health-related phenomena and when research resources are constrained (Bradshaw et al., 2017). QD is instrumental in various aspects of health research.
Characteristics of Qualitative Descriptive Studies: A Systematic Review. ... is a term that is widely used to describe qualitative studies of health care and nursing-related phenomena. ... After searching and screening, data were extracted from the sample of 55 QD articles and examined to characterize research objectives, design justification ...
Descriptive research is designed to document the factors that describe characteristics, behaviors and conditions of individuals and groups. For example, researchers have used this approach to describe a sample of individuals with spinal cord injuries with respect to gender, age, and cause and severity of injury to see whether these properties were similar to those described in the past. 1 ...
A qualitative descriptive design is frequently used in nursing and healthcare research, as such an approach can provide broad insight into particular phenomena and can be used in a variety of ways ...
Objective: The purpose of this methodology paper is to describe an approach to qualitative design known as qualitative descriptive that is well suited to junior health sciences researchers because it can be used with a variety of theoretical approaches, sampling techniques, and data collection strategies. Background: It is often difficult for junior qualitative researchers to pull together the ...
The key attributes and value of qualitative description research in the health care professions will be highlighted with the aim of extending its usage. Keywords: qualitative description, philosophy, epistemology, ... Qualitative descriptive methods in health science research. HERD: Health Environments Research & Design Journal, 9 (4), 16-25.
Revised on June 22, 2023. Descriptive research aims to accurately and systematically describe a population, situation or phenomenon. It can answer what, where, when and how questions, but not why questions. A descriptive research design can use a wide variety of research methods to investigate one or more variables.
Qualitative descriptive designs are common in nursing and healthcare research due to their inherent simplicity, flexibility and utility in diverse healthcare contexts. However, the application of descriptive research is sometimes critiqued in terms of scientific rigor.
One of the first steps in planning a research study is the choice of study design. The available stu. Study designs: Part 2 - Descriptive studies : Perspectives in Clinical Research ... (health-related characteristic), whether exposure (e.g., a risk factor) or outcome (e.g., a disease) as they exist in a defined population at one particular ...
Descriptive research is a study of status and is widely used in education, nutrition, epidemiology, and the behavioral sciences. Its value is based on the premise that problems can be solved and practices improved through observation, analysis, and description. The most common descriptive research method is the survey, which includes ...
The purpose of this methodology paper is to describe an approach to qualitative design known as qualitative descriptive that is well suited to junior health sciences researchers because it can be used with a variety of theoretical approaches, sampling techniques, and data collection strategies.
Health research: Descriptive research design is useful in health research to describe the prevalence and distribution of a disease or health condition in a population. Social science research: Descriptive research design is used in social science research to describe social phenomena such as cultural norms, values, and beliefs.
Presidential Popularity. M.B. Grossman, in International Encyclopedia of the Social & Behavioral Sciences, 2001 2 The Fabric of Presidential Popularity. The second line of study involves descriptive research emphasizing the action and/or statements of a president and his entourage as they attempt to bolster his standing with the public. Using interviews, observations, and reviewing documents ...
Qualitative description (QD) is a label used in qualitative research for studies which are descriptive in nature, particularly for examining health care and nursing-related phenomena (Polit & Beck, 2009, 2014).QD is a widely cited research tradition and has been identified as important and appropriate for research questions focused on discovering the who, what, and where of events or ...
The growing adoption of continuous quality improvement (CQI) initiatives in healthcare has generated a surge in research interest to gain a deeper understanding of CQI. However, comprehensive evidence regarding the diverse facets of CQI in healthcare has been limited. Our review sought to comprehensively grasp the conceptualization and principles of CQI, explore existing models and tools ...
Decision support tool development. The interdisciplinary research team (MBF, VB, JH) and collaborators (a PCP, a RN and a podiatrist) developed a one-page decision tool to help FMGs' professionals manage DFUs based on a rapid review of current literature including practice guidelines and related evidence-based literature [] during the fall of 2019.
Background: Type 2 diabetes disproportionately affects South Asian subgroups. Lifestyle prevention programs help prevent and manage diabetes; however, there is a need to tailor these programs for mobile health (mHealth). Objective: This study examined technology access, current use, and preferences for health communication among South Asian immigrants diagnosed with or at risk for diabetes ...
Background In recent years, health systems have expanded the focus on health equity to include health-related social needs (HRSNs) screening. Community health workers (CHWs) are positioned to address HRSNs by serving as linkages between health systems, social services, and the community. This study describes a health system's 12-month experience integrating CHWs to navigate HRSNs among ...
Introduction. Qualitative descriptive approaches to nursing and healthcare research provide a broad insight into particular phenomena and can be used in a variety of ways including as a standalone research design, as a precursor to larger qualitative studies and commonly as the qualitative component in mixed-methods studies.
Exploratory research is the umbrella term used by Brink and Wood (2001) to describe all description qualitative research and suggest it "is a Level 1 research endeavor" (p. 85), and Savin-Baden and Howell Major (2013) refer to a pragmatic qualitative approach. This interchangeable use of terms creates ambiguity and confusion in relation to ...
The greatest strength of the qualitative research approach lies in the richness and depth of the healthcare exploration and description it makes. In health research, these methods are considered as the most humanistic and person-centered way of discovering and uncovering thoughts and actions of human beings. Table 1.
Understanding patients' experiences with health care utilization from the pilot SSK scheme is important for enhancing the quality of health care service delivery during the national-level scale-up of the scheme. ... satisfied, 4; neither satisfied nor dissatisfied, 3; dissatisfied, 2; very dissatisfied, 1). Descriptive statistics, statistical ...
Motivation and Scope. There is a large body of recently published review/conceptual studies on healthcare and data mining. We outline the characteristics of these studies—e.g., scope/healthcare sub-area, timeframe, and number of papers reviewed—in Table 1.For example, one study reviewed awareness effect in type 2 diabetes published between 2001 and 2005, identifying 18 papers [].