- - Google Chrome
Intended for healthcare professionals
- Access provided by Google Indexer
- My email alerts
- BMA member login
- Username * Password * Forgot your log in details? Need to activate BMA Member Log In Log in via OpenAthens Log in via your institution

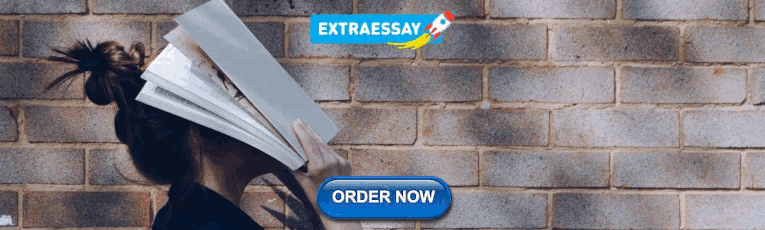
Search form
- Advanced search
- Search responses
- Search blogs
- Clinical problem...
Clinical problem solving and diagnostic decision making: selective review of the cognitive literature
- Related content
- Peer review
This article has a correction. Please see:
- Clinical problem solving and diagnostic decision making: selective review of the cognitive literature - November 02, 2006
- Arthur S Elstein , professor ( aelstein{at}uic.edu ) ,
- Alan Schwarz , assistant professor of clinical decision making.
- Department of Medical Education, University of Illinois College of Medicine, Chicago, IL 60612-7309, USA
- Correspondence to: A S Elstein
This is the fourth in a series of five articles
This article reviews our current understanding of the cognitive processes involved in diagnostic reasoning in clinical medicine. It describes and analyses the psychological processes employed in identifying and solving diagnostic problems and reviews errors and pitfalls in diagnostic reasoning in the light of two particularly influential approaches: problem solving 1 , 2 , 3 and decision making. 4 , 5 , 6 , 7 , 8 Problem solving research was initially aimed at describing reasoning by expert physicians, to improve instruction of medical students and house officers. Psychological decision research has been influenced from the start by statistical models of reasoning under uncertainty, and has concentrated on identifying departures from these standards.
Summary points
Problem solving and decision making are two paradigms for psychological research on clinical reasoning, each with its own assumptions and methods
The choice of strategy for diagnostic problem solving depends on the perceived difficulty of the case and on knowledge of content as well as strategy
Final conclusions should depend both on prior belief and strength of the evidence
Conclusions reached by Bayes's theorem and clinical intuition may conflict
Because of cognitive limitations, systematic biases and errors result from employing simpler rather than more complex cognitive strategies
Evidence based medicine applies decision theory to clinical diagnosis
Problem solving
Diagnosis as selecting a hypothesis.
The earliest psychological formulation viewed diagnostic reasoning as a process of testing hypotheses. Solutions to difficult diagnostic problems were found by generating a limited number of hypotheses early in the diagnostic process and using them to guide subsequent collection of data. 1 Each hypothesis can be used to predict what additional findings ought to be present if it were true, and the diagnostic process is a guided search for these findings. Experienced physicians form hypotheses and their diagnostic plan rapidly, and the quality of their hypotheses is higher than that of novices. Novices struggle to develop a plan and some have difficulty moving beyond collection of data to considering possibilities.
It is possible to collect data thoroughly but nevertheless to ignore, to misunderstand, or to misinterpret some findings, but also possible for a clinician to be too economical in collecting data and yet to interpret accurately what is available. Accuracy and thoroughness are analytically separable.
Pattern recognition or categorisation
Expertise in problem solving varies greatly between individual clinicians and is highly dependent on the clinician's mastery of the particular domain. 9 This finding challenges the hypothetico-deductive model of clinical reasoning, since both successful and unsuccessful diagnosticians use hypothesis testing. It appears that diagnostic accuracy does not depend as much on strategy as on mastery of content. Further, the clinical reasoning of experts in familiar situations frequently does not involve explicit testing of hypotheses. 3 10 , 11 , 12 Their speed, efficiency, and accuracy suggest that they may not even use the same reasoning processes as novices. 11 It is likely that experienced physicians use a hypothetico-deductive strategy only with difficult cases and that clinical reasoning is more a matter of pattern recognition or direct automatic retrieval. What are the patterns? What is retrieved? These questions signal a shift from the study of judgment to the study of the organisation and retrieval of memories.
Problem solving strategies
Hypothesis testing
Pattern recognition (categorisation)
By specific instances
By general prototypes
Viewing the process of diagnosis assigning a case to a category brings some other issues into clearer view. How is a new case categorised? Two competing answers to this question have been put forward and research evidence supports both. Category assignment can be based on matching the case to a specific instance (“instance based” or “exemplar based” recognition) or to a more abstract prototype. In the former, a new case is categorised by its resemblance to memories of instances previously seen. 3 11 This model is supported by the fact that clinical diagnosis is strongly affected by context—for example, the location of a skin rash on the body—even when the context ought to be irrelevant. 12
The prototype model holds that clinical experience facilitates the construction of mental models, abstractions, or prototypes. 2 13 Several characteristics of experts support this view—for instance, they can better identify the additional findings needed to complete a clinical picture and relate the findings to an overall concept of the case. These features suggest that better diagnosticians have constructed more diversified and abstract sets of semantic relations, a network of links between clinical features and diagnostic categories. 14
The controversy about the methods used in diagnostic reasoning can be resolved by recognising that clinicians approach problems flexibly; the method they select depends upon the perceived characteristics of the problem. Easy cases can be solved by pattern recognition: difficult cases need systematic generation and testing of hypotheses. Whether a diagnostic problem is easy or difficult is a function of the knowledge and experience of the clinician.
The strategies reviewed are neither proof against error nor always consistent with statistical rules of inference. Errors that can occur in difficult cases in internal medicine include failure to generate the correct hypothesis; misperception or misreading the evidence, especially visual cues; and misinterpretations of the evidence. 15 16 Many diagnostic problems are so complex that the correct solution is not contained in the initial set of hypotheses. Restructuring and reformulating should occur as data are obtained and the clinical picture evolves. However, a clinician may quickly become psychologically committed to a particular hypothesis, making it more difficult to restructure the problem.
Decision making
Diagnosis as opinion revision.
From the point of view of decision theory, reaching a diagnosis means updating opinion with imperfect information (the clinical evidence). 8 17 The standard rule for this task is Bayes's theorem. The pretest probability is either the known prevalence of the disease or the clinician's subjective impression of the probability of disease before new information is acquired. The post-test probability, the probability of disease given new information, is a function of two variables, pretest probability and the strength of the evidence, measured by a “likelihood ratio.”
Bayes's theorem tells us how we should reason, but it does not claim to describe how opinions are revised. In our experience, clinicians trained in methods of evidence based medicine are more likely than untrained clinicians to use a Bayesian approach to interpreting findings. 18 Nevertheless, probably only a minority of clinicians use it in daily practice and informal methods of opinion revision still predominate. Bayes's theorem directs attention to two major classes of errors in clinical reasoning: in the assessment of either pretest probability or the strength of the evidence. The psychological study of diagnostic reasoning from this viewpoint has focused on errors in both components, and on the simplifying rules or heuristics that replace more complex procedures. Consequently, this approach has become widely known as “heuristics and biases.” 4 19
Errors in estimation of probability
Availability —People are apt to overestimate the frequency of vivid or easily recalled events and to underestimate the frequency of events that are either very ordinary or difficult to recall. Diseases or injuries that receive considerable media attention are often thought of as occurring more commonly than they actually do. This psychological principle is exemplified clinically in the overemphasis of rare conditions, because unusual cases are more memorable than routine problems.
Representativeness —Representativeness refers to estimating the probability of disease by judging how similar a case is to a diagnostic category or prototype. It can lead to overestimation of probability either by causing confusion of post-test probability with test sensitivity or by leading to neglect of base rates and implicitly considering all hypotheses equally likely. This is an error, because if a case resembles disease A and disease B equally, and A is much more common than B, then the case is more likely to be an instance of A. Representativeness is associated with the “conjunction fallacy”—incorrectly concluding that the probability of a joint event (such as the combination of findings to form a typical clinical picture) is greater than the probability of any one of these events alone.
Heuristics and biases
Availability
Representativeness
Probability transformations
Effect of description detail
Conservatism
Anchoring and adjustment
Order effects
Decision theory assumes that in psychological processing of probabilities, they are not transformed from the ordinary probability scale. Prospect theory was formulated as a descriptive account of choices involving gambling on two outcomes, 20 and cumulative prospect theory extends the theory to cases with multiple outcomes. 21 Both prospect theory and cumulative prospect theory propose that, in decision making, small probabilities are overweighted and large probabilities underweighted, contrary to the assumption of standard decision theory. This “compression” of the probability scale explains why the difference between 99% and 100% is psychologically much greater than the difference between, say, 60% and 61%. 22
Support theory
Support theory proposes that the subjective probability of an event is inappropriately influenced by how detailed the description is. More explicit descriptions yield higher probability estimates than compact, condensed descriptions, even when the two refer to exactly the same events. Clinically, support theory predicts that a longer, more detailed case description will be assigned a higher subjective probability of the index disease than a brief abstract of the same case, even if they contain the same information about that disease. Thus, subjective assessments of events, while often necessary in clinical practice, can be affected by factors unrelated to true prevalence. 23
Errors in revision of probability
In clinical case discussions, data are presented sequentially, and diagnostic probabilities are not revised as much as is implied by Bayes's theorem 8 ; this phenomenon is called conservatism. One explanation is that diagnostic opinions are revised up or down from an initial anchor, which is either given in the problem or subjectively formed. Final opinions are sensitive to the starting point (the “anchor”), and the shift (“adjustment”) from it is typically insufficient. 4 Both biases will lead to collecting more information than is necessary to reach a desired level of diagnostic certainty.
It is difficult for everyday judgment to keep separate accounts of the probability of a disease and the benefits that accrue from detecting it. Probability revision errors that are systematically linked to the perceived cost of mistakes show the difficulties experienced in separating assessments of probability from values, as required by standard decision theory. There is a tendency to overestimate the probability of more serious but treatable diseases, because a clinician would hate to miss one. 24
Bayes's theorem implies that clinicians given identical information should reach the same diagnostic opinion, regardless of the order in which information is presented. However, final opinions are also affected by the order of presentation of information. Information presented later in a case is given more weight than information presented earlier. 25
Other errors identified in data interpretation include simplifying a diagnostic problem by interpreting findings as consistent with a single hypothesis, forgetting facts inconsistent with a favoured hypothesis, overemphasising positive findings, and discounting negative findings. From a Bayesian standpoint, these are all errors in assessing the diagnostic value of clinical evidence—that is, errors in implicit likelihood ratios.
Featured Clinical Reviews
- Screening for Atrial Fibrillation: US Preventive Services Task Force Recommendation Statement JAMA Recommendation Statement January 25, 2022
- Evaluating the Patient With a Pulmonary Nodule: A Review JAMA Review January 18, 2022
- Download PDF
- Share X Facebook Email LinkedIn
- Permissions
Problem Solving in Clinical Medicine: From Data to Diagnosis
New York University School of Medicine New York
This article is only available in the PDF format. Download the PDF to view the article, as well as its associated figures and tables.
This volume is specifically designed for the medical student who is at or just beyond the junction of preclinical and clinical experiences. There are 31 contributing physicians, 25 of whom are at the University of Texas Health Science Centers at San Antonio.
The book is written and guided by an obviously experienced senior physician, whose goal is to enable students "to enter the physician's mind during the gathering of data and to learn why he pursues certain pathways in his quest for solutions." The volume is obviously more than that, but, to this reviewer, that is its most notable message.
The first half is effectively conceptual and methodological, reminding medical students that they can, in fact, utilize their broad knowledge in the care of actual patients. The second half provides specific examples of "problem solving in action." Students might enjoy looking at specific case studies in this section to determine
Wessler S. Problem Solving in Clinical Medicine: From Data to Diagnosis. JAMA. 1985;254(21):3109. doi:10.1001/jama.1985.03360210125049
Manage citations:
© 2024
Artificial Intelligence Resource Center
Cardiology in JAMA : Read the Latest
Browse and subscribe to JAMA Network podcasts!
Others Also Liked
Select your interests.
Customize your JAMA Network experience by selecting one or more topics from the list below.
- Academic Medicine
- Acid Base, Electrolytes, Fluids
- Allergy and Clinical Immunology
- American Indian or Alaska Natives
- Anesthesiology
- Anticoagulation
- Art and Images in Psychiatry
- Artificial Intelligence
- Assisted Reproduction
- Bleeding and Transfusion
- Caring for the Critically Ill Patient
- Challenges in Clinical Electrocardiography
- Climate and Health
- Climate Change
- Clinical Challenge
- Clinical Decision Support
- Clinical Implications of Basic Neuroscience
- Clinical Pharmacy and Pharmacology
- Complementary and Alternative Medicine
- Consensus Statements
- Coronavirus (COVID-19)
- Critical Care Medicine
- Cultural Competency
- Dental Medicine
- Dermatology
- Diabetes and Endocrinology
- Diagnostic Test Interpretation
- Drug Development
- Electronic Health Records
- Emergency Medicine
- End of Life, Hospice, Palliative Care
- Environmental Health
- Equity, Diversity, and Inclusion
- Facial Plastic Surgery
- Gastroenterology and Hepatology
- Genetics and Genomics
- Genomics and Precision Health
- Global Health
- Guide to Statistics and Methods
- Hair Disorders
- Health Care Delivery Models
- Health Care Economics, Insurance, Payment
- Health Care Quality
- Health Care Reform
- Health Care Safety
- Health Care Workforce
- Health Disparities
- Health Inequities
- Health Policy
- Health Systems Science
- History of Medicine
- Hypertension
- Images in Neurology
- Implementation Science
- Infectious Diseases
- Innovations in Health Care Delivery
- JAMA Infographic
- Law and Medicine
- Leading Change
- Less is More
- LGBTQIA Medicine
- Lifestyle Behaviors
- Medical Coding
- Medical Devices and Equipment
- Medical Education
- Medical Education and Training
- Medical Journals and Publishing
- Mobile Health and Telemedicine
- Narrative Medicine
- Neuroscience and Psychiatry
- Notable Notes
- Nutrition, Obesity, Exercise
- Obstetrics and Gynecology
- Occupational Health
- Ophthalmology
- Orthopedics
- Otolaryngology
- Pain Medicine
- Palliative Care
- Pathology and Laboratory Medicine
- Patient Care
- Patient Information
- Performance Improvement
- Performance Measures
- Perioperative Care and Consultation
- Pharmacoeconomics
- Pharmacoepidemiology
- Pharmacogenetics
- Pharmacy and Clinical Pharmacology
- Physical Medicine and Rehabilitation
- Physical Therapy
- Physician Leadership
- Population Health
- Primary Care
- Professional Well-being
- Professionalism
- Psychiatry and Behavioral Health
- Public Health
- Pulmonary Medicine
- Regulatory Agencies
- Reproductive Health
- Research, Methods, Statistics
- Resuscitation
- Rheumatology
- Risk Management
- Scientific Discovery and the Future of Medicine
- Shared Decision Making and Communication
- Sleep Medicine
- Sports Medicine
- Stem Cell Transplantation
- Substance Use and Addiction Medicine
- Surgical Innovation
- Surgical Pearls
- Teachable Moment
- Technology and Finance
- The Art of JAMA
- The Arts and Medicine
- The Rational Clinical Examination
- Tobacco and e-Cigarettes
- Translational Medicine
- Trauma and Injury
- Treatment Adherence
- Ultrasonography
- Users' Guide to the Medical Literature
- Vaccination
- Venous Thromboembolism
- Veterans Health
- Women's Health
- Workflow and Process
- Wound Care, Infection, Healing
- Register for email alerts with links to free full-text articles
- Access PDFs of free articles
- Manage your interests
- Save searches and receive search alerts
We will keep fighting for all libraries - stand with us!
Internet Archive Audio
- This Just In
- Grateful Dead
- Old Time Radio
- 78 RPMs and Cylinder Recordings
- Audio Books & Poetry
- Computers, Technology and Science
- Music, Arts & Culture
- News & Public Affairs
- Spirituality & Religion
- Radio News Archive
- Flickr Commons
- Occupy Wall Street Flickr
- NASA Images
- Solar System Collection
- Ames Research Center
- All Software
- Old School Emulation
- MS-DOS Games
- Historical Software
- Classic PC Games
- Software Library
- Kodi Archive and Support File
- Vintage Software
- CD-ROM Software
- CD-ROM Software Library
- Software Sites
- Tucows Software Library
- Shareware CD-ROMs
- Software Capsules Compilation
- CD-ROM Images
- ZX Spectrum
- DOOM Level CD

- Smithsonian Libraries
- FEDLINK (US)
- Lincoln Collection
- American Libraries
- Canadian Libraries
- Universal Library
- Project Gutenberg
- Children's Library
- Biodiversity Heritage Library
- Books by Language
- Additional Collections
- Prelinger Archives
- Democracy Now!
- Occupy Wall Street
- TV NSA Clip Library
- Animation & Cartoons
- Arts & Music
- Computers & Technology
- Cultural & Academic Films
- Ephemeral Films
- Sports Videos
- Videogame Videos
- Youth Media
Search the history of over 866 billion web pages on the Internet.
Mobile Apps
- Wayback Machine (iOS)
- Wayback Machine (Android)
Browser Extensions
Archive-it subscription.
- Explore the Collections
- Build Collections
Save Page Now
Capture a web page as it appears now for use as a trusted citation in the future.
Please enter a valid web address
- Donate Donate icon An illustration of a heart shape
Problem solving in clinical medicine : from data to diagnosis
Bookreader item preview, share or embed this item, flag this item for.
- Graphic Violence
- Explicit Sexual Content
- Hate Speech
- Misinformation/Disinformation
- Marketing/Phishing/Advertising
- Misleading/Inaccurate/Missing Metadata
![[WorldCat (this item)] [WorldCat (this item)]](https://archive.org/images/worldcat-small.png)
plus-circle Add Review comment Reviews
235 Previews
Better World Books
DOWNLOAD OPTIONS
No suitable files to display here.
EPUB and PDF access not available for this item.
IN COLLECTIONS
Uploaded by Alethea Bowser on December 8, 2011
SIMILAR ITEMS (based on metadata)

- Medical Books
Buy new: .savingPriceOverride { color:#CC0C39!important; font-weight: 300!important; } .reinventMobileHeaderPrice { font-weight: 400; } #apex_offerDisplay_mobile_feature_div .reinventPriceSavingsPercentageMargin, #apex_offerDisplay_mobile_feature_div .reinventPricePriceToPayMargin { margin-right: 4px; } $41.99 $ 41 . 99 FREE delivery May 8 - 10 Ships from: The Art of Savings Sold by: The Art of Savings
Save with used - good .savingpriceoverride { color:#cc0c39important; font-weight: 300important; } .reinventmobileheaderprice { font-weight: 400; } #apex_offerdisplay_mobile_feature_div .reinventpricesavingspercentagemargin, #apex_offerdisplay_mobile_feature_div .reinventpricepricetopaymargin { margin-right: 4px; } $34.67 $ 34 . 67 free delivery wednesday, may 8 on orders shipped by amazon over $35 ships from: amazon sold by: azbook73, return this item for free.
Free returns are available for the shipping address you chose. You can return the item for any reason in new and unused condition: no shipping charges
- Go to your orders and start the return
- Select the return method

Download the free Kindle app and start reading Kindle books instantly on your smartphone, tablet, or computer - no Kindle device required .
Read instantly on your browser with Kindle for Web.
Using your mobile phone camera - scan the code below and download the Kindle app.

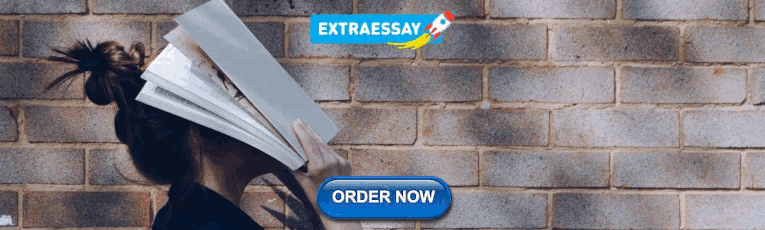
Image Unavailable

- To view this video download Flash Player
Follow the author

Problem Solving in Clinical Medicine: From Data to Diagnosis Subsequent Edition
There is a newer edition of this item:, purchase options and add-ons.
- ISBN-10 0683022520
- ISBN-13 978-0683022520
- Edition Subsequent
- Publisher Williams & Wilkins
- Publication date January 1, 1985
- Language English
- Dimensions 7.25 x 1.5 x 1.5 inches
- Print length 636 pages
- See all details

Customers who bought this item also bought

Product details
- Publisher : Williams & Wilkins; Subsequent edition (January 1, 1985)
- Language : English
- Hardcover : 636 pages
- ISBN-10 : 0683022520
- ISBN-13 : 978-0683022520
- Item Weight : 2.72 pounds
- Dimensions : 7.25 x 1.5 x 1.5 inches
- #410 in Medical Diagnosis (Books)
- #241,538 in Unknown
About the author
Paul cutler.
Discover more of the author’s books, see similar authors, read author blogs and more
Customer reviews
Customer Reviews, including Product Star Ratings help customers to learn more about the product and decide whether it is the right product for them.
To calculate the overall star rating and percentage breakdown by star, we don’t use a simple average. Instead, our system considers things like how recent a review is and if the reviewer bought the item on Amazon. It also analyzed reviews to verify trustworthiness.
- Sort reviews by Top reviews Most recent Top reviews
Top reviews from the United States
There was a problem filtering reviews right now. please try again later..

Top reviews from other countries

- Amazon Newsletter
- About Amazon
- Accessibility
- Sustainability
- Press Center
- Investor Relations
- Amazon Devices
- Amazon Science
- Sell on Amazon
- Sell apps on Amazon
- Supply to Amazon
- Protect & Build Your Brand
- Become an Affiliate
- Become a Delivery Driver
- Start a Package Delivery Business
- Advertise Your Products
- Self-Publish with Us
- Become an Amazon Hub Partner
- › See More Ways to Make Money
- Amazon Visa
- Amazon Store Card
- Amazon Secured Card
- Amazon Business Card
- Shop with Points
- Credit Card Marketplace
- Reload Your Balance
- Amazon Currency Converter
- Your Account
- Your Orders
- Shipping Rates & Policies
- Amazon Prime
- Returns & Replacements
- Manage Your Content and Devices
- Recalls and Product Safety Alerts
- Conditions of Use
- Privacy Notice
- Consumer Health Data Privacy Disclosure
- Your Ads Privacy Choices
Thank you for visiting nature.com. You are using a browser version with limited support for CSS. To obtain the best experience, we recommend you use a more up to date browser (or turn off compatibility mode in Internet Explorer). In the meantime, to ensure continued support, we are displaying the site without styles and JavaScript.
- View all journals
- Explore content
- About the journal
- Publish with us
- Sign up for alerts
- Perspective
- Published: 19 April 2024
Causal machine learning for predicting treatment outcomes
- Stefan Feuerriegel ORCID: orcid.org/0000-0001-7856-8729 1 , 2 ,
- Dennis Frauen 1 , 2 ,
- Valentyn Melnychuk 1 , 2 ,
- Jonas Schweisthal ORCID: orcid.org/0000-0003-3725-3821 1 , 2 ,
- Konstantin Hess ORCID: orcid.org/0009-0003-8552-6588 1 , 2 ,
- Alicia Curth 3 ,
- Stefan Bauer ORCID: orcid.org/0000-0003-1712-060X 4 , 5 ,
- Niki Kilbertus ORCID: orcid.org/0000-0001-8718-4305 2 , 4 , 5 ,
- Isaac S. Kohane 6 &
- Mihaela van der Schaar 7 , 8
Nature Medicine volume 30 , pages 958–968 ( 2024 ) Cite this article
8695 Accesses
141 Altmetric
Metrics details
- Medical research
- Predictive markers
- Clinical trial design
- Therapeutics
Causal machine learning (ML) offers flexible, data-driven methods for predicting treatment outcomes including efficacy and toxicity, thereby supporting the assessment and safety of drugs. A key benefit of causal ML is that it allows for estimating individualized treatment effects, so that clinical decision-making can be personalized to individual patient profiles. Causal ML can be used in combination with both clinical trial data and real-world data, such as clinical registries and electronic health records, but caution is needed to avoid biased or incorrect predictions. In this Perspective, we discuss the benefits of causal ML (relative to traditional statistical or ML approaches) and outline the key components and steps. Finally, we provide recommendations for the reliable use of causal ML and effective translation into the clinic.
This is a preview of subscription content, access via your institution
Access options
Access Nature and 54 other Nature Portfolio journals
Get Nature+, our best-value online-access subscription
24,99 € / 30 days
cancel any time
Subscribe to this journal
Receive 12 print issues and online access
195,33 € per year
only 16,28 € per issue
Buy this article
- Purchase on Springer Link
- Instant access to full article PDF
Prices may be subject to local taxes which are calculated during checkout
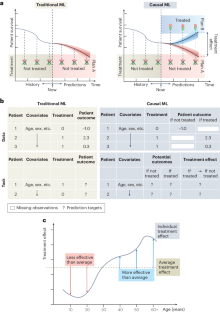
Similar content being viewed by others
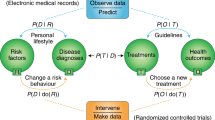
Causal inference and counterfactual prediction in machine learning for actionable healthcare
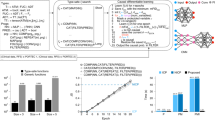
Automated causal inference in application to randomized controlled clinical trials
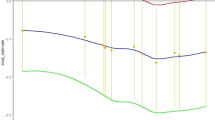
Prediction of treatment outcome in clinical trials under a personalized medicine perspective
Kaddour, J., Lynch, A., Liu, Q., Kusner, M. J. & Silva, R. Causal machine learning: a survey and open problems. Preprint at arXiv https://doi.org/10.48550/arXiv.2206.15475 (2022).
Yoon, J., Jordon, J. & van der Schaar, M. GANITE: estimation of individualized treatment effects using generative adversarial nets. In Proc. 6th International Conference on Learning Representations (ICLR, 2018).
Evans, W. E. & Relling, M. V. Pharmacogenomics: translating functional genomics into rational therapeutics. Science 286 , 487–491 (1999).
Article CAS PubMed Google Scholar
Esteva, A. et al. A guide to deep learning in healthcare. Nat. Med. 25 , 24–29 (2019).
Kopitar, L., Kocbek, P., Cilar, L., Sheikh, A. & Stiglic, G. Early detection of type 2 diabetes mellitus using machine learning-based prediction models. Sci. Rep. 10 , 11981 (2020).
Article CAS PubMed PubMed Central Google Scholar
Alaa, A. M., Bolton, T., Di Angelantonio, E., Rudd, J. H. & van der Schaar, M. Cardiovascular disease risk prediction using automated machine learning: a prospective study of 423,604 UK Biobank participants. PLoS ONE 14 , e0213653 (2019).
Cahn, A. et al. Prediction of progression from pre-diabetes to diabetes: development and validation of a machine learning model. Diabetes/Metab. Res. Rev. 36 , e3252 (2020).
Article PubMed Google Scholar
Zueger, T. et al. Machine learning for predicting the risk of transition from prediabetes to diabetes. Diabetes Technol. Ther. 24 , 842–847 (2022).
Krittanawong, C. et al. Machine learning prediction in cardiovascular diseases: a metaanalysis. Sci. Rep. 10 , 16057 (2020).
Xie, Y. et al. Comparative effectiveness of SGLT2 inhibitors, GLP-1 receptor agonists, DPP-4 inhibitors, and sulfonylureas on risk of major adverse cardiovascular events: Emulation of a randomised target trial using electronic health records. Lancet Diabetes Endocrinol. 11 , 644–656 (2023).
Deng, Y. et al. Comparative effectiveness of second line glucose lowering drug treatments using real world data: emulation of a target trial. BMJ Med. 2 , e000419 (2023).
Article PubMed PubMed Central Google Scholar
Kalia, S. et al. Emulating a target trial using primary-care electronic health records: sodium glucose cotransporter 2 inhibitor medications and hemoglobin A1c. Am. J. Epidemiol. 192 , 782–789 (2023).
Petito, L. C. et al. Estimates of overall survival in patients with cancer receiving different treatment regimens: emulating hypothetical target trials in the Surveillance, Epidemiology, and End Results (SEER)–Medicare linked database. JAMA Netw. Open 3 , e200452 (2020).
Rubin, D. B. Estimating causal effects of treatments in randomized and nonrandomized studies. J. Educ. Psychol. 66 , 688–701 (1974).
Article Google Scholar
Rubin, D. B. Causal inference using potential outcomes: design, modeling, decisions. J. Am. Stat. Assoc. 100 , 322–331 (2005).
Article CAS Google Scholar
Robins, J. M. Correcting for non-compliance in randomized trials using structural nested mean models. Commun. Stat. 23 , 2379–2412 (1994).
Robins, J. M. Robust estimation in sequentially ignorable missing data and causal inference models. In 1999 Proceedings of the American Statistical Association on Bayesian Statistical Science 6–10 (2000).
Holland, P. W. Statistics and causal inference. J. Am. Stat. Assoc. 81 , 945–960 (1986).
Pearl, J. Causality: Models , Reasoning , and Inference (Cambridge University Press, 2009).
Hemkens, L. G. et al. Interpretation of epidemiologic studies very often lacked adequate consideration of confounding. J. Clin. Epidemiol. 93 , 94–102 (2018).
Dang, L. E. et al. A causal roadmap for generating high-quality real-world evidence. J. Clin. Transl. Sci. 7 , e212 (2023).
Petersen, M. L. & van der Laan, M. J. Causal models and learning from data: integrating causal modeling and statistical estimation. Epidemiology 25 , 418–426 (2014).
van der Laan, M. J. & Rubin, D. Targeted maximum likelihood learning. Int. J. Biostatistics 2 , 11 (2006).
Hirano, K. & Imbens, G. W. in Applied Bayesian Modeling and Causal Inference from Incomplete-Data Perspectives: An Essential Journey with Donald Rubin’s Statistical Family (eds Gelman, A. & Meng, X.-L.) Ch. 7 (John Wiley & Sons, 2004).
Specht, L. et al. Modern radiation therapy for Hodgkin lymphoma: field and dose guidelines from the international lymphoma radiation oncology group (ILROG). Int. J. Radiat. Oncol. Biol. Phys. 89 , 854–862 (2014).
van Geloven, N. et al. Prediction meets causal inference: the role of treatment in clinical prediction models. Eur. J. Epidemiol. 35 , 619–630 (2020).
Kennedy, E. H. Towards optimal doubly robust estimation of heterogeneous causal effects. Electron. J. Stat. 17 , 3008–3049 (2023).
Imbens, G. W. & Rubin, D. B. Causal Inference in Statistics, Social, and Biomedical Sciences (Cambridge University Press, 2015).
Book Google Scholar
Chen, J., Vargas-Bustamante, A., Mortensen, K. & Ortega, A. N. Racial and ethnic disparities in health care access and utilization under the Affordable Care Act. Med. Care 54 , 140–146 (2016).
Cinelli, C., Forney, A. & Pearl, J. A crash course in good and bad controls. Sociol. Methods Res. https://doi.org/10.1177/00491241221099552 (2022).
Laffers, L. & Mellace, G. Identification of the average treatment effect when SUTVA is violated. Department of Economics SDU. Discussion Papers on Business and Economics No. 3 (University of Southern Denmark, 2020).
Huber, M. & Steinmayr, A. A framework for separating individual-level treatment effects from spillover effects. J. Bus. Econ. Stat. 39 , 422–436 (2021).
Syrgkanis, V. et al. Machine learning estimation of heterogeneous treatment effects with instruments. In Proc. 33rd International Conference on Neural Information Processing Systems (eds Wallach, H. M. & Larochelle, H.) 15193–15202 (NeurIPS, 2019).
Frauen, D. & Feuerriegel, S. Estimating individual treatment effects under unobserved confounding using binary instruments. In Proc. 11th International Conference on Learning Representations (ICLR, 2023).
Lim, B. Forecasting treatment responses over time using recurrent marginal structural networks. In Proc. Advances in Neural Information Processing Systems 31 (eds Bengio, H. et al.) (NeurIPS, 2018).
Liu, R., Yin, C. & Zhang, P. Estimating individual treatment effects with time-varying confounders. In Proc. IEEE International Conference on Data Mining (ICDM) 382–391 (IEEE, 2020).
Li, R. et al. G-Net: a deep learning approach to G-computation for counterfactual outcome prediction under dynamic treatment regimes. In Proc. Machine Learning for Health (eds Roy, S. et al.) 282–299 (PMLR, 2021).
Bica, I., Alaa, A. M., Jordon, J. & van der Schaar, M. Estimating counterfactual treatment outcomes over time through adversarially balanced representations. In Proc. 8th International Conference on Learning Representations 11790–11817 (ICLR, 2020).
Liu, R., Hunold, K. M., Caterino, J. M. & Zhang, P. Estimating treatment effects for time-to-treatment antibiotic stewardship in sepsis. Nat. Mach. Intell. 5 , 421–431 (2023).
Melnychuk, V., Frauen, D. & Feuerriegel, S. Causal transformer for estimating counterfactual outcomes. In Proc. 39th International Conference on Machine Learning (eds Chaudhuri, K. et al.) 15293–15329 (PMLR, 2022).
Schulam, P. & Saria, S. Reliable decision support using counterfactual models. In Proc. 31st International Conference on Neural Information Processing Systems (eds von Luxburg, U. et al.) 1696–1706 (NeurIPS, 2017).
Vanderschueren, T., Curth, A., Verbeke, W. & van der Schaar, M. Accounting for informative sampling when learning to forecast treatment outcomes over time. In Proc. 40th International Conference on Machine Learning (eds Krause, A. et al.) 34855–34874 (PMLR, 2023).
Seedat, N., Imrie, F., Bellot, A., Qian, Z. & van der Schaar, M. Continuous-time modeling of counterfactual outcomes using neural controlled differential equations. In Proc. 39th International Conference on Machine Learning (eds Chaudhuri, K. et al.) 19497–19521 (PMLR, 2022).
Hess, K., Melnychuk, V., Frauen, D. & Feuerriegel, S. Bayesian neural controlled differential equations for treatment effect estimation. In Proc. 12th International Conference on Learning Representations (ICLR, 2024).
Hatt, T., Berrevoets, J., Curth, A., Feuerriegel, S. & van der Schaar, M. Combining observational and randomized data for estimating heterogeneous treatment effects. Preprint at arXiv https://doi.org/10.48550/arXiv.2202.12891 (2022).
Colnet, B. et al. Causal inference methods for combining randomized trials and observational studies: a review. Stat. Sci. 39 , 165–191 (2024).
Kallus, N., Puli, A. M. & Shalit, U. Removing hidden confounding by experimental grounding. In Proc. 32nd Conference on Neural Information Processing Systems (eds Bengio, S. et al.) 10888–10897 (NeurIPS, 2018).
van der Laan, M. J., Polley, E. C. & Hubbard, A. E. Super learner. Stat. Appl. Genet. Mol. Biol. 6 , 25 (2007).
Google Scholar
van der Laan, M. J. & Rose, S. Targeted Learning: Causal Inference for Observational and Experimental Data 1st edn (Springer, 2011).
Zheng, W. & van der Laan, M. J. in Targeted Learning: Causal Inference for Observational and Experimental Data 1st edn, 459–474 (Springer, 2011).
Díaz, I. & van der Laan, M. J. Targeted data adaptive estimation of the causal dose–response curve. J. Causal Inference 1 , 171–192 (2013).
Luedtke, A. R. & van der Laan, M. J. Super-learning of an optimal dynamic treatment rule. Int. J. Biostatistics 12 , 305–332 (2016).
Künzel, S. R., Sekhon, J. S., Bickel, P. J. & Yu, B. Metalearners for estimating heterogeneous treatment effects using machine learning. Proc. Natl Acad. Sci. USA 116 , 4156–4165 (2019).
Curth, A. & van der Schaar, M. Nonparametric estimation of heterogeneous treatment effects: From theory to learning algorithms. In Proc. 24th International Conference on Artificial Intelligence and Statistics (eds Banerjee, A. & Fukumizu, K.) 1810–1818 (PMLR, 2021).
Athey, S. & Imbens, G. Recursive partitioning for heterogeneous causal effects. Proc. Natl Acad. Sci. USA 113 , 7353–7360 (2016).
Wager, S. & Athey, S. Estimation and inference of heterogeneous treatment effects using random forests. J. Am. Stat. Assoc. 113 , 1228–1242 (2018).
Athey, S., Tibshirani, J. & Wager, S. Generalized random forests. Ann. Stat. 47 , 1148–1178 (2019).
Shalit, U., Johansson, F. D. & Sontag, D. Estimating individual treatment effect: generalization bounds and algorithms. In Proc. 34th International Conference on Machine Learning (eds Precup, D. & Teh, Y. W.) 3076–3085 (PMLR, 2017).
Shi, C., Blei, D. & Veitch, V. Adapting neural networks for the estimation of treatment effects. In Proc. 33rd International Conference on Neural Information Processing Systems (eds Wallach, H. M. et al.) 2496–2506 (NeurIPS, 2019).
Bach, P., Chernozhukov, V., Kurz, M. S. & Spindler, M. DoubleML: an object-oriented implementation of double machine learning in Python. J. Mach. Learn. Res. 23 , 2469–2474 (2022).
Foster, D. J. & Syrgkanis, V. Orthogonal statistical learning. Ann. Stat. 51 , 879–908 (2023).
Kennedy, E. H., Ma, Z., McHugh, M. D. & Small, D. S. Nonparametric methods for doubly robust estimation of continuous treatment effects. J. R. Stat. Soc. Series B Stat. Methodol. 79 , 1229–1245 (2017).
Nie, L., Ye, M., Liu, Q. & Nicolae, D. VCNet and functional targeted regularization for learning causal effects of continuous treatments. In Proc. 9th International Conference on Learning Representations (ICLR, 2021).
Bica, I., Jordon, J. & van der Schaar, M. Estimating the effects of continuous-valued interventions using generative adversarial networks. In Proc. 34th Annual Conference on Neural Information Processing Systems (eds Larochelle, H. et al.) (NeurIPS, 2020).
Hill, J. L. Bayesian nonparametric modeling for causal inference. J. Computational Graph. Stat. 20 , 217–240 (2011).
Schwab, P., Linhardt, L., Bauer, S., Buhmann, J. M. & Karlen, W. Learning counterfactual representations for estimating individual dose-response curves. In Proc. 34th AAAI Conference on Artificial Intelligence 5612–5619 (AAAI, 2020).
Schweisthal, J., Frauen, D., Melnychuk, V. & Feuerriegel, S. Reliable off-policy learning for dosage combinations. In Proc. 37th Annual Conference on Neural Information Processing Systems (NeurIPS, 2023).
Melnychuk, V., Frauen, D. & Feuerriegel, S. Normalizing flows for interventional density estimation. In Proc. 40th International Conference on Machine Learning (eds Krause, A. et al.) 24361–24397 (PMLR, 2023).
Banerji, C. R., Chakraborti, T., Harbron, C. & MacArthur, B. D. Clinical AI tools must convey predictive uncertainty for each individual patient. Nat. Med. 29 , 2996–2998 (2023).
Alaa, A. M. & van der Schaar, M. Bayesian inference of individualized treatment effects using multi-task Gaussian processes. In Proc. 31st Annual Conference on Neural Information Processing Systems (eds von Luxburg, U. et al.) 3425–3433 (NeurIPS, 2017).
Alaa, A., Ahmad, Z. & van der Laan, M. Conformal meta-learners for predictive inference of individual treatment effects. In Proc. 37th Annual Conference on Neural Information Processing Systems (eds Oh, A. et al.) (NeurIPS, 2023).
Curth, A., Svensson, D., Weatherall, J. & van der Schaar, M. Really doing great at estimating CATE? A critical look at ML benchmarking practices in treatment effect estimation. In Proc. 35th Conference on Neural Information Processing Systems Datasets and Benchmarks Track (eds Vanschoren, J. & Yeung, S.-K.) (NeurIPS, 2021).
Boyer, C. B., Dahabreh, I. J. & Steingrimsson, J. A. Assessing model performance for counterfactual predictions. Preprint at arXiv https://doi.org/10.48550/arXiv.2308.13026 (2023).
Keogh, R. H. & van Geloven, N. Prediction under interventions: evaluation of counterfactual performance using longitudinal observational data. Preprint at arXiv https://doi.org/10.48550/arXiv.2304.10005 (2023).
Curth, A. & van der Schaar, M. In search of insights, not magic bullets: towards demystification of the model selection dilemma in heterogeneous treatment effect estimation. In Proc. 40th International Conference on Machine Learning (eds Krause, A. et al.) 6623–6642 (PMLR, 2023).
Sharma, A., Syrgkanis, V., Zhang, C. & Kıcıman, E. DoWhy: addressing challenges in expressing and validating causal assumptions. Preprint at arXiv https://doi.org/10.48550/arXiv.2108.13518 (2021).
Vokinger, K. N., Feuerriegel, S. & Kesselheim, A. S. Mitigating bias in machine learning for medicine. Commun. Med. 1 , 25 (2021).
Petersen, M. L., Porter, K. E., Gruber, S., Wang, Y. & van der Laan, M. J. Diagnosing and responding to violations in the positivity assumption. Stat. Methods Med. Res. 21 , 31–54 (2012).
Jesson, A., Mindermann, S., Shalit, U. & Gal, Y. Identifying causal-effect inference failure with uncertainty-aware models. In Proc. 34th Conference on Neural Information Processing Systems (eds Larochelle, H. et al.) 11637–11649 (NeurIPS, 2020).
Rudolph, K. E. et al. When effects cannot be estimated: redefining estimands to understand the effects of naloxone access laws. Epidemiology 33 , 689–698 (2022).
Cornfield, J. et al. Smoking and lung cancer: recent evidence and a discussion of some questions. J. Natl Cancer Inst. 22 , 173–203 (1959).
CAS PubMed Google Scholar
Frauen, D., Melnychuk, V. & Feuerriegel, S. Sharp bounds for generalized causal sensitivity analysis. In Proc. 37th Annual Conference on Neural Information Processing Systems (eds Oh, A. et al.) (NeurIPS, 2023).
Kallus, N., Mao, X. & Zhou, A. Interval estimation of individual-level causal effects under unobserved confounding. In Proc. 22nd International Conference on Artificial Intelligence and Statistics (eds Chaudhuri, K. & Sugiyama, M.) 2281–2290 (PMLR, 2019).
Jin, Y., Ren, Z. & Candès, E. J. Sensitivity analysis of individual treatment effects: a robust conformal inference approach. Proc. Natl Acad. Sci. USA 120 , e2214889120 (2023).
Dorn, J. & Guo, K. Sharp sensitivity analysis for inverse propensity weighting via quantile balancing. J. Am. Stat. Assoc. 118 , 2645–2657 (2023).
Oprescu, M. et al. B-learner: quasi-oracle bounds on heterogeneous causal effects under hidden confounding. In Proc. 40th International Conference on Machine Learning (eds Krause, A. et al.) 26599–26618 (PMLR, 2023).
Hernán, M. A. & Robins, J. M. Using big data to emulate a target trial when a randomized trial is not available. Am. J. Epidemiol. 183 , 758–764 (2016).
Xu, J. et al. Protocol for the development of a reporting guideline for causal and counterfactual prediction models in biomedicine. BMJ Open 12 , e059715 (2022).
Fournier, J. C. et al. Antidepressant drug effects and depression severity: a patient-level meta-analysis. JAMA 303 , 47–53 (2010).
Booth, C. M., Karim, S. & Mackillop, W. J. Real-world data: towards achieving the achievable in cancer care. Nat. Rev. Clin. Oncol. 16 , 312–325 (2019).
Chien, I. et al. Multi-disciplinary fairness considerations in machine learning for clinical trials. In Proc. 2022 ACM Conference on Fairness , Accountability , and Transparency (FACCT '22) 906–924 (ACM, 2022).
Ross, E. L. et al. Estimated average treatment effect of psychiatric hospitalization in patients with suicidal behaviors: a precision treatment analysis. JAMA Psychiatry 81 , 135–143 (2023).
Cole, S. R. & Stuart, E. A. Generalizing evidence from randomized clinical trials to target populations: the ACTG 320 trial. Am. J. Epidemiol. 172 , 107–115 (2010).
Hatt, T., Tschernutter, D. & Feuerriegel, S. Generalizing off-policy learning under sample selection bias. In Proc. 38th Conference on Uncertainty in Artificial Intelligence (eds Cussens, J. & Zhang, K.) 769–779 (PMLR, 2022).
Sherman, R. E. et al. Real-world evidence—what is it and what can it tell us. N. Engl. J. Med. 375 , 2293–2297 (2016).
Norgeot, B. et al. Minimum information about clinical artificial intelligence modeling: the MI-CLAIM checklist. Nat. Med. 26 , 1320–1324 (2020).
Von Elm, E. et al. The strengthening the reporting of observational studies in epidemiology (STROBE) statement: Guidelines for reporting observational studies. Lancet 370 , 1453–1457 (2007).
Nie, X. & Wager, S. Quasi-oracle estimation of heterogeneous treatment effects. Biometrika 108 , 299–319 (2021).
Chernozhukov, V. et al. Double/debiased machine learning for treatment and structural parameters. Econom. J. 21 , C1–C68 (2018).
Morzywołek, P., Decruyenaere, J. & Vansteelandt, S. On a general class of orthogonal learners for the estimation of heterogeneous treatment effects. Preprint at arXiv https://doi.org/10.48550/arXiv.2303.12687 (2023).
Download references
Acknowledgements
S.F. acknowledges funding via Swiss National Science Foundation Grant 186932.
Author information
Authors and affiliations.
LMU Munich, Munich, Germany
Stefan Feuerriegel, Dennis Frauen, Valentyn Melnychuk, Jonas Schweisthal & Konstantin Hess
Munich Center for Machine Learning, Munich, Germany
Stefan Feuerriegel, Dennis Frauen, Valentyn Melnychuk, Jonas Schweisthal, Konstantin Hess & Niki Kilbertus
Department of Applied Mathematics & Theoretical Physics, University of Cambridge, Cambridge, UK
Alicia Curth
School of Computation, Information and Technology, TU Munich, Munich, Germany
Stefan Bauer & Niki Kilbertus
Helmholtz Munich, Munich, Germany
Department of Biomedical Informatics, Harvard Medical School, Boston, MA, USA
Isaac S. Kohane
Cambridge Centre for AI in Medicine, University of Cambridge, Cambridge, UK
Mihaela van der Schaar
The Alan Turing Institute, London, UK
You can also search for this author in PubMed Google Scholar
Contributions
All authors contributed to conceptualization, manuscript writing and approval of the manuscript.
Corresponding author
Correspondence to Stefan Feuerriegel .
Ethics declarations
Competing interests.
The authors declare no competing interests.
Peer review
Peer review information.
Nature Medicine thanks Matthew Sperrin and the other, anonymous, reviewer(s) for their contribution to the peer review of this work. Primary Handling Editor: Karen O’Leary, in collaboration with the Nature Medicine team.
Additional information
Publisher’s note Springer Nature remains neutral with regard to jurisdictional claims in published maps and institutional affiliations.
Rights and permissions
Springer Nature or its licensor (e.g. a society or other partner) holds exclusive rights to this article under a publishing agreement with the author(s) or other rightsholder(s); author self-archiving of the accepted manuscript version of this article is solely governed by the terms of such publishing agreement and applicable law.
Reprints and permissions
About this article
Cite this article.
Feuerriegel, S., Frauen, D., Melnychuk, V. et al. Causal machine learning for predicting treatment outcomes. Nat Med 30 , 958–968 (2024). https://doi.org/10.1038/s41591-024-02902-1
Download citation
Received : 03 January 2024
Accepted : 04 March 2024
Published : 19 April 2024
Issue Date : April 2024
DOI : https://doi.org/10.1038/s41591-024-02902-1
Share this article
Anyone you share the following link with will be able to read this content:
Sorry, a shareable link is not currently available for this article.
Provided by the Springer Nature SharedIt content-sharing initiative
Quick links
- Explore articles by subject
- Guide to authors
- Editorial policies
Sign up for the Nature Briefing newsletter — what matters in science, free to your inbox daily.

- Contributors
- Sign Up Here!
Diagnostic frameworks
- Illness scripts
- Residency Match
- Publications

All Frameworks
Abdominal Distension
Abdominal Pain Overview
Abdominal Pain – Image Negative
Abdominal Pain – Image Negative – Action Steps
Abdominal Pain Thought Train
Acute Mesenteric Ischemia
Acute Pancreatitis
AKI – overview
AKI and cancer
Aldosterone – Inappropriate
Altered mental status 1.0
Altered Mental Status 2.0
Altered Mental Status – MIST Negative
Anemia Thought Train
Antibiotic Failure
Aortitis: Paths to the Problem
Bilateral Lower Extremity Weakness
Bone lesions
Bony Back Pain – Overview
Brain Abscess
Bronchiectasis
Bowel Obstruction
Bowel Perforation
Bowel Wall Thickening
Cancer and Hypercalcemia
Cervical Lymphadenopathy
CHF – Hyperacute Heart Failure
Chronic Cough
Chronic Diarrhea Overview
Chronic Diarrhea: 4 quarters approach
Chronic Kidney Disease
Chylothorax
Cirrhosis and Dyspnea
Coagulopathy – Elevated INR and PTT
Community Acquired Pneumonia
Community Acquired Pneumonia – Ddx
Congestive Heart Failure – HFrEF
Constipation
CSF Lymphocytic Pleocytosis
Diabetes – Causes
Diabetes Insipidus
Diarrhea Thought Train
Diffuse Alveolar Hemorrhage – DAH
Diffuse Lymphadenopathy Overview
Diffuse Lymphadenopathy: A Guide
Diverticulitis Complications
Doxycycline Deficiency State
Dyspnea and Cancer
Dyspnea Pyramid
Eosinophilia
Extra-Hepatic Biliary Obstruction
Fever Overview
Fever and Back Pain
Fever and rash
Fever in a returning traveler
Fever – Path to Inflammation
Focal Splenic Lesion
Gastric Mass
Glomerulonephritis
Gram Positive Organisms
Granulomatous Infection – by exposure
HCV – Extrahepatic Manifestations
HCV – Skin Manifestations
Headache (Secondary)
Headache (Thunderclap)
Heart Failure and Weight Loss
Hemolysis: Autoimmune Hemolytic Anemia (AIHA)
Hemolysis: Chronic Hemolysis Complications
Hemolysis: Hemolytic Anemia
Hemolysis: Microangiopathic Hemolytic Anemia (MAHA)
Hemoptysis: The Arc
Hemoptysis: Overview
Hemoptysis: Massive
HIV overview
HIV & Chronic Diarrhea
HIV & Infection
HIV: Non-infectious complications
Hyperammonemia
Hypercalcemia
Hyperkalemia
Hypernatremia
Hypertension Overview
Hypertension – Secondary
Hypoalbuminemia
Hypocalcemia
Hypoglycemia
Hypokalemia – Overview
Hypokalemia – Practical Approach
Hyponatremia
Hypophosphatemia- Overview
Hypophosphatemia – Severe
Hypothermia
Hypothyroidism
Hypoxemia Thought Train
IgM Disorders
Indirect Hyperbilirubinemia
Infection in the inpatient 1.0
Infection in the Inpatient – Secondary Evaluation
Infectious Aortitis: Clues
Inflammation overview
Inflammation Thought Train
Inflammatory Abdominal Pain
Inguinal Lymphadenopathy
Intrarenal AKI
Iron deficiency anemia
Jaundice – Overview
Jaundice – Intrahepatic Cholestasis
Jaundice Thought Train
Kidney Stone Overview
Lactic Acidosis
Lactate Dehydrogenase – LDH
Low Back Pain – Overview
Lung Cancer
Lung cavity
Lung Nodules
Mediastinal Mass
Macrocytic Anemia
Metabolic Acidosis Overview
Metabolic Acidosis: AGMA
Metabolic Acidosis: NAGMA
Metabolic Alkalosis
Monoarticular Arthritis
Nausea and Vomiting Thought Train
Neurologic Complications of Systemic Cancer
Nonstress Fracture
Optic Disc Swelling
Pancreatic Mass
Pancreatic Mass: Simple Approach
Pancytopenia
Parkinson’s Dz – The Time Course
Parkinsonism
Peptic Ulcer Disease
Peripheral Edema
Peripheral Neuropathy
Peritonitis
Petechial Rash
Pharyngitis
Photophobia
Pituitary Adenoma
Pleural effusion
Pleuritic Chest Pain
Pleural Nodularity
Pneumonia Complications
Pneumothorax
Polycythemia
Portal Vein Thrombosis
Postprandial Abdominal Pain
Post-renal AKI
Primary Respiratory Alkalosis
Pulmonary disease with eosinophilia
Pulmonary Function Tests – PFTs
Pulmonary Hypertension
Pulmonary Renal Syndromes
Raynaud’s Syndrome
Severe Acute Liver Injury
Splenic Infarct
Splenic Infarct vs Abscess
Splenomegaly
Specific Back Pain Overview
Subacute Dyspnea on Exertion
Sudden Symptoms
Supraventricular tachycardia – SVT
Thorax and Inflammation
Thrombocytopenia
Thrombocytosis
Thrombosis- Overview
Thrombosis – Bland
Thrombosis on Anticoagulation
Thrombosis – Ultrahypercoagulable
Thyroid Function Test in the Evaluation of Hyperthyroidism
Thyrotoxicosis
Tick borne infection
Transverse Myelitis
Upper GI Bleed
Urinalysis interpretation
Urinary Retention
Urinary Tract Infection
Vaginal Bleeding
Vasculitis Mimics
Vasculopathy – Systemic
Vasculopathy – Skin
Vasculopathy – CNS
Visceral Air
Visual Field Defects
Weakness Thought Train
Weight loss
Wide complex tachycardia
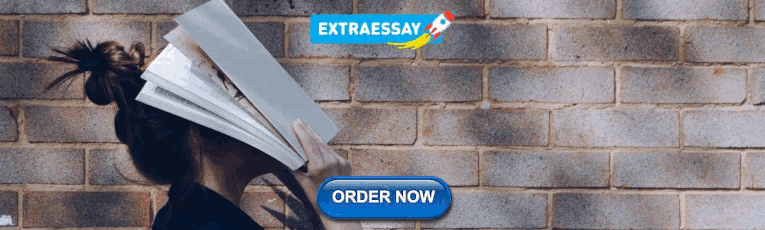
IMAGES
VIDEO
COMMENTS
Flipping the Switch. M.K. Finta and OthersN Engl J Med 2024;390:456-462. A 43-year-old woman presented with a 1-week history of dysuria and lower abdominal pressure but no fevers, hematuria, or ...
This chapter introduces the concept of clinical reasoning. It attempts to define what clinical reasoning is and what its features are. Solving clinical problems involves the ability to reason about causality of pathological processes, requiring knowledge of anatomy and the working and pathology of organ systems, and it requires the ability to compare patient problems as patterns with instances ...
This is the fourth in a series of five articles This article reviews our current understanding of the cognitive processes involved in diagnostic reasoning in clinical medicine. It describes and analyses the psychological processes employed in identifying and solving diagnostic problems and reviews errors and pitfalls in diagnostic reasoning in the light of two particularly influential ...
Problem Solving in Clinical Medicine. : Paul Cutler. Lippincott Williams & Wilkins, 1998 - Medical - 530 pages. The clinical reasoning process is explained in terms of formation of an initial concept, formation of hypotheses, the further expansion of inquiry tactics, and application of appropriate clinical skills.
Problem Solving in Clinical Medicine: From Data to Diagnosis. Stanford Wessler, MD. Author Affiliations. New York University School of Medicine New York ... utilize their broad knowledge in the care of actual patients. The second half provides specific examples of "problem solving in action." Students might enjoy looking at specific case ...
The descriptive perspective has its roots in cognitive psychology and began as a special case of general problem-solving studies. It focuses on clinical reasoning as a domain in which the problems are complex and there is a clear role for expertise. The critical role of knowledge distinguishes medicine from many other domains of problem-solving ...
This article reviews our current understanding of the cognitive processes involved in diagnostic reasoning in clinical medicine. It describes and analyses the psychological processes employed in identifying and solving diagnostic problems and reviews errors and pitfalls in diagnostic reasoning in the light of two particularly influential approaches: problem solving 1 - 3 and decision making ...
Abstract. IN this issue of the Journal we begin a new series, "Clinical Problem-Solving," which focuses on the difficult diagnostic, therapeutic, and ethical challenges that physicians face every ...
Problem solving in clinical medicine : from data to diagnosis by Cutler, Paul, 1920-Publication date 1985 Topics Diagnosis -- Problems, exercises, etc, Medical logic -- Problems, exercises, etc, Problem solving, Clinical medicine -- Problems, exercises, etc, Diagnosis, Internal Medicine, Problem Solving
In this article, we present a comprehensive approach to solving complex clinical problems based on the work by Weed, 12,13,14 Eddy and Clanton, 15 and Evans and Gadd 16 and elaborated at the Medical Faculty at the University of Nijmegen, Nijmegen, The Netherlands. This approach, called clinical problem analysis (CPA), has three major goals:
Amazon.com: Problem Solving in Clinical Medicine: From Data to Diagnosis: 9780683303773: Cutler, Paul: Books
This item: Problem Solving in Clinical Medicine: From Data to Diagnosis . $20.96 $ 20. 96. Get it Nov 28 - 30. Only 1 left in stock - order soon. Ships from and sold by b4cause. + Introduction to Clinical Informatics (Health Informatics) $109.99 $ 109. 99. Usually ships within 2 to 3 days.
Innovations in Medicine and Surgery; In the Limelight; Letters; My Treatment Approach; Residents' Clinics; Clinical Topics Clinical Practice Guidelines; Coronavirus (COVID-19) Gerontology and Aging; ... Clinical Problem-Solving. William C. Mundell, MD . William C. Mundell. Affiliations.
Problem Solving in Clinical Medicine: From Data to Diagnosis $29.95 Only 1 left in stock - order soon. BlackBerry OS 4.1 or Higher / iPhone/iPod Touch 2.0 or Higher /Palm OS 3.5 or higher / Palm Pre Classic / Symbian S60, 3rd edition (Nokia) / Windows Mobile℠Pocket PC (all versions) / Windows Mobile Smartphone / Windows 98SE/2000/ME/XP ...
We are confronted by a growing body of information, much of it invalid or irrelevant to clinical practice. This article discusses evidence based medicine, a process of turning clinical problems into questions and then systematically locating, appraising, and using contemporaneous research findings as the basis for clinical decisions.
The clinical problem solving/diagnostic reasoning session is designed to provide a window into the master clinician's thought process and reasoning strategies. The session serves as a valuable tool to learn and teach the process of hypothesis generation (eliciting the right question), problem representation (problem list), prioritized ...
Problem Solving in Clinical Medicine: From Data to Diagnosis, Paul Cutter Baltimore, MD: Williams & Wilkins, 1985, 400 pp, $28.00 (paper). - Volume 4 Issue 3
Clinical reasoning cases. Overview of resources. NEJM Clinical Problem Solving. Clinical Care Conundrums - Journal of Hospital Medicine. Exercises in Clinical Reasoning - Journal of General Internal Medicine. WebsitesCLOSLERExercises in Clinical ReasoningGrepMedHuman Diagnosis ProjectMedical Rants - Dr. Bob CentorNEJM interactive ...
The Clinical Problem Solvers. Democratizing clinical reasoning education. Podcast. Morning Report. Frameworks - Scripts.
Causal machine learning (ML) offers flexible, data-driven methods for predicting treatment outcomes including efficacy and toxicity, thereby supporting the assessment and safety of drugs. A key ...
Problem Solving in Clinical Medicine From data to diagnosis - PMC. Advanced Search. User Guide. Journal List. J Can Chiropr Assoc. v.31 (1); 1987 Mar. PMC2484400. As a library, NLM provides access to scientific literature. Inclusion in an NLM database does not imply endorsement of, or agreement with, the contents by NLM or the National ...
Visceral Air. Visual Field Defects. Weakness Thought Train. Weight loss. Wide complex tachycardia. Frameworks alphabetically Cardiac Neuro Lung GI Renal Infectious Liver Rheum Blood Electrolytes Endocrine Miscellaneous Icons made by Kara Lau All Frameworks Abdominal DistensionAbdominal Pain OverviewAbdominal Pain - Image NegativeAbdominal Pain ...