Accessibility Links
- Skip to content
- Skip to search IOPscience
- Skip to Journals list
- Accessibility help
- Accessibility Help
Click here to close this panel.
Purpose-led Publishing is a coalition of three not-for-profit publishers in the field of physical sciences: AIP Publishing, the American Physical Society and IOP Publishing.
Together, as publishers that will always put purpose above profit, we have defined a set of industry standards that underpin high-quality, ethical scholarly communications.
We are proudly declaring that science is our only shareholder.
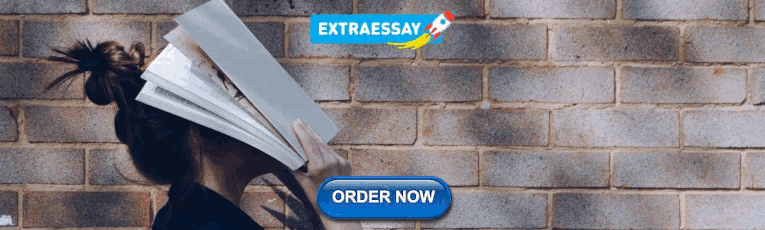
ABC Analysis: A Qualitative Case Study on Inventory Management in Giant Superstore Taman Connaught, An Outlet Of GCH Retail (Malaysia) SDN. BHD.
Shamani Jayakumaran 1 , Wong Zi Shan 1 and Dazmin Daud 1
Published under licence by IOP Publishing Ltd IOP Conference Series: Materials Science and Engineering , Volume 780 , 2019 2nd International Symposium on Traffic Transportation and Civil Architecture (ISTTCA 2019) 13-15 December 2019, Chengdu, China Citation Shamani Jayakumaran et al 2020 IOP Conf. Ser.: Mater. Sci. Eng. 780 072016 DOI 10.1088/1757-899X/780/7/072016
Article metrics
2921 Total downloads
Share this article
Author e-mails.
Author affiliations
1 Faculty of Business and Information Science, UCSI University, Jalan Menara Gading, 56000 Cheras, Kuala Lumpur, Malaysia
Buy this article in print
This study aimed to understand the inventory management of Giant Superstore Taman Connaught. The study was qualitative study that explored the ABC analysis efficiency of inventory management in Giant Superstore, Taman Connaught an outlet of GCH Retail (Malaysia) SDN. BHD. ABC analysis was one method to manage the inventory management who good position was arranged according to the category such as A category product was high in value but low in quantity and C category product was low in value and high in quantity. The elements that were selected as significance included the product consumption rate, carrying cost and replenishment product lead time which contributed toward ABC analysis of inventory management efficiency in Giant Superstore. The study indicated dissimilarities in controlling the inventory in Giant superstore. The study found Giant had used POM.net in their inventory management since this software from ABC tool. HR manager needs to recruit quality skilled workers that have proper qualifications and acceptable for particular job duties.
Export citation and abstract BibTeX RIS
Content from this work may be used under the terms of the Creative Commons Attribution 3.0 licence . Any further distribution of this work must maintain attribution to the author(s) and the title of the work, journal citation and DOI.
Inventory Management of Construction Project Through ABC Analysis: A Case Study
- Conference paper
- First Online: 31 July 2023
- Cite this conference paper
- P. Murthi 11 ,
- K. Poongodi 12 &
- M. Geetha 13
Part of the book series: Lecture Notes in Civil Engineering ((LNCE,volume 346))
Included in the following conference series:
- National conference on Advances in Construction Materials and Management
214 Accesses
An effective inventory management is the prime parameter for success of any construction projects. The issues related to inventory management in construction particularly medium budget projects are not monitored properly and lead to increase in construction cost. However every project have a detailed project schedule and reviewed periodically with technical persons. The insufficient review process affecting the inventory management and also cost effectiveness of construction projects. The improper material management extends the project completion duration which directly affects the finance management. A volume of such kind of issues are prevailing in the developing areas particularly the proximity of Metros. In order to evaluate the problem, the proposed analysis was identified private construction projects and examined the status at completion stage. The questionnaire based survey was identified five completed projects with the project cost of more than 100 Lakhs. The net profit after completion of the project was considered as the benchmark factor and identified a project which was produced 5.14% loss when compared to the estimated cost. The impact of inventory management for the poor performance in the selected project was predicted through ABC analysis and the suggestions were given for the sustainability of construction.
This is a preview of subscription content, log in via an institution to check access.
Access this chapter
- Available as PDF
- Read on any device
- Instant download
- Own it forever
- Available as EPUB and PDF
- Durable hardcover edition
- Dispatched in 3 to 5 business days
- Free shipping worldwide - see info
Tax calculation will be finalised at checkout
Purchases are for personal use only
Institutional subscriptions
Kar, S., & Neeraj Jha, K. (2020). Examining the effect of material management issues on the schedule and cost performance of construction projects based on a structural equation model: Survey of Indian experiences. Journal of Construction Engineering and Management , 146 (9). https://doi.org/10.1061/(ASCE)CO.1943-7862.0001906 .
Said, H., & EI Rayes, K. (2011). Optimizing material procurement and storage on construction sites. Journal of Construction Engineering and Management , 137 (6). https://doi.org/10.1061/(ASCE)CO.1943-7862.0000307 .
Jun, D.H., & EI Rayes, K. (2011). Fast and Accurate risk evaluation for scheduling large-scale construction projects. Journal of Construction Engineering and Management , 25 (5). https://doi.org/10.1061/(ASCE)CP.1943-5487.0000106 .
Hegazy, T., & Menesi, W. (2008). Delay Analysis under multiple baseline updates. Journal of Construction Engineering and Management , 134 (8). https://doi.org/10.1061/(ASCE)0733-9364(2008)134:8(575) .
Ibbs, W., Nguyen, L.D., & Lee, S. (2007). Quantified impacts of project change. J. Profess. Iss. Eng. Educ. Pract. 133 (1). https://doi.org/10.1061/(ASCE)1052-3928(2007)133:1(45) .
Randolph Thomas, H., Riley, D.R., & Messner, J.I. (2005). Fundamental principles of site material management”, Journal of Construction Engineering and Management , 131(7). https://doi.org/10.1061/(ASCE)0733-9364(2005)131:7(808 ).
Gurmu, A.T. (2019). Tools for measuring construction materials management practices and predicting labor productivity in multistory building projects”, Journal of Construction Engineering and Management , 145 (2). https://doi.org/10.1061/(ASCE)CO.1943-7862.0001611 .
Venkat Reddy, P., Siva Krishna, A., & Ravi Kumar, T. (2017). Study on concept of smart city and its structural components. International Journal of Civil Engineering and Technology , 8 (8), 101–112.
Google Scholar
Baba, S. K. V., & Haripriya, S. (2017). Performance analysis of black cotton soil treated with granite dust and lime. International Journal of Civil Engineering and Technology, 8 (10), 1341–1350.
Singh, S.P., Satheesh Raju, G., & Shravan, M.: Waste management in Construction - A study with reference to India. International Journal of Civil Engineering and Technology , 9 (9), 533–538.
Shravan, M., Satheesh Raju, G., Singh, S.P., Yamsuani, N., & Mahesh, D. (2018). Construction materials management on construction sites. International Journal of Civil Engineering and Technology , 9 (3), 809–818.
Download references
Author information
Authors and affiliations.
Centre for Construction Methods and Materials, SR University, Warangal, India
Department of Civil Engineering, SR University, Warangal, India
K. Poongodi
School of Business, SR University, Warangal, India
You can also search for this author in PubMed Google Scholar
Corresponding author
Correspondence to P. Murthi .
Editor information
Editors and affiliations.
Department of Civil Engineering, National Institute of Technology Warangal, Warangal, Telangana, India
Aneetha Vilventhan
Department of Civil Engineering, Birla Institute of Technology and Science Pilani, Pilani, Rajasthan, India
Shamsher Bahadur Singh
Department of Civil Engineering, IIT Bombay, Mumbai, Maharashtra, India
Venkata Santosh Kumar Delhi
Rights and permissions
Reprints and permissions
Copyright information
© 2023 The Author(s), under exclusive license to Springer Nature Singapore Pte Ltd.
About this paper
Cite this paper.
Murthi, P., Poongodi, K., Geetha, M. (2023). Inventory Management of Construction Project Through ABC Analysis: A Case Study. In: Vilventhan, A., Singh, S.B., Delhi, V.S.K. (eds) Advances in Construction Materials and Management. ACMM 2022. Lecture Notes in Civil Engineering, vol 346. Springer, Singapore. https://doi.org/10.1007/978-981-99-2552-0_8
Download citation
DOI : https://doi.org/10.1007/978-981-99-2552-0_8
Published : 31 July 2023
Publisher Name : Springer, Singapore
Print ISBN : 978-981-99-2551-3
Online ISBN : 978-981-99-2552-0
eBook Packages : Engineering Engineering (R0)
Share this paper
Anyone you share the following link with will be able to read this content:
Sorry, a shareable link is not currently available for this article.
Provided by the Springer Nature SharedIt content-sharing initiative
- Publish with us
Policies and ethics
- Find a journal
- Track your research
- Case Studies
- Choosing a Software
- Implementation
- Manufacturing Tips
- Production planning
- Supply Chain
ABC Analysis (80/20 Rule) in Inventory Management
ABC analysis helps businesses get insight into their supply chain, reduce inventory, and improve inventory management efficiency. And properly applying it does not require a data analyst or a substantial amount of resources.
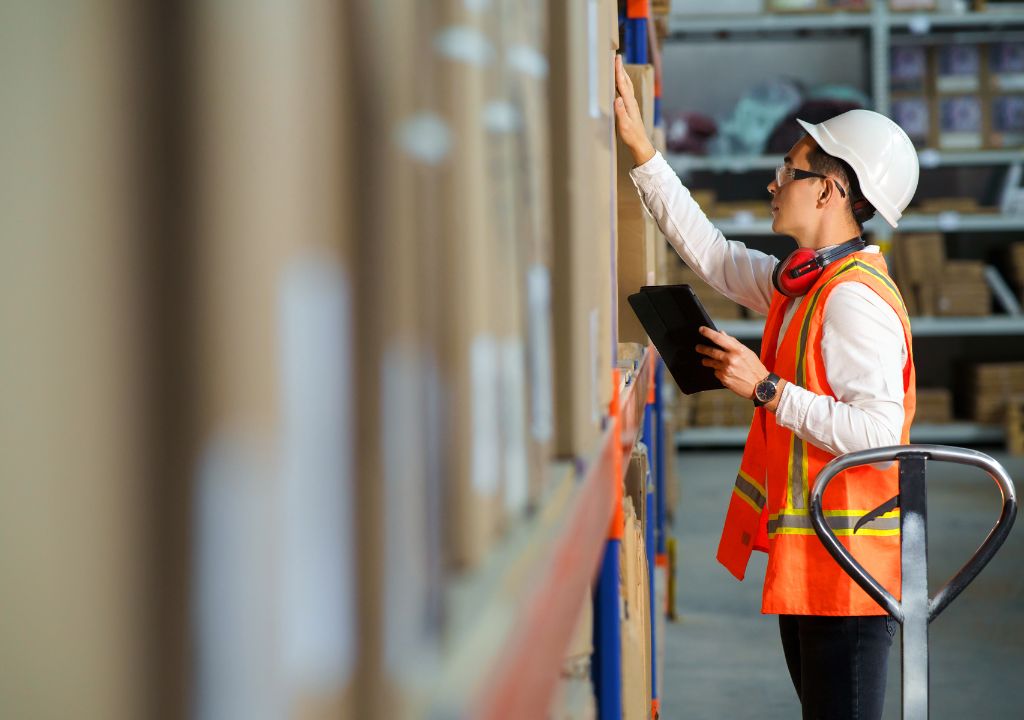
Table of Contents
What is ABC analysis?
ABC analysis is a popular categorization technique used in inventory optimization. It involves classifying stock-keeping units according to a certain criterion, most often by their importance or consumption value, sometimes also by their utilization frequency.
The technique is based on the Pareto principle (or 80/20 rule), which states that roughly 80% of the effects within any system originate from 20% of the causes, meaning that the relationship between inputs and outputs is unequal.
By applying this principle to inventory, we find that a small variety (20%) of inventory items comprise most (80%) of the total inventory value, be it consumption value, sales volume, consumption frequency, or any other criterion.
Knowing which SKUs make up most of your business allows you to set priorities for the items before negotiating prices with suppliers, determining service levels, safety stocks, and reorder points, assigning workers to tasks like inventory review, and physically organizing your stockroom.
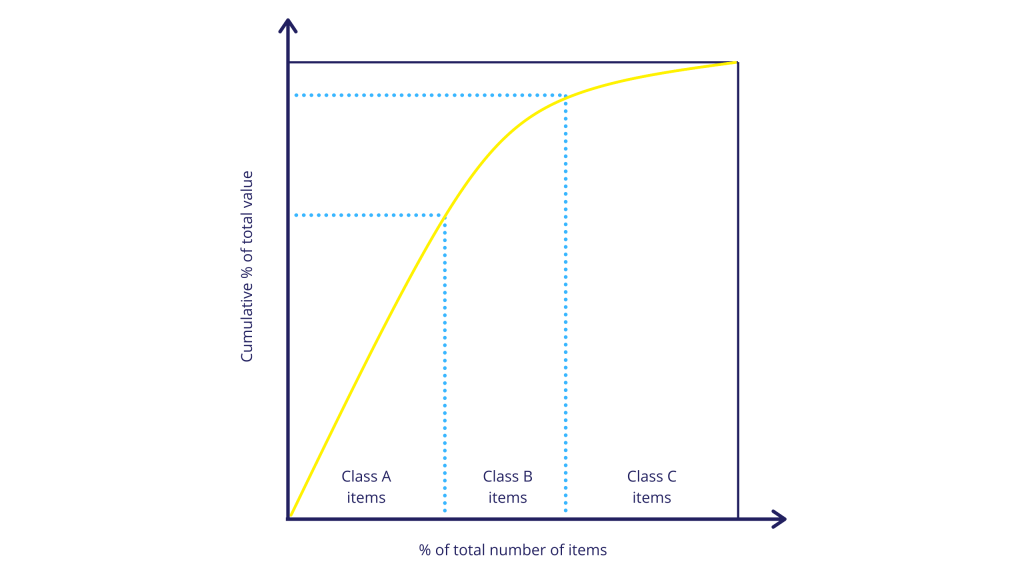
Applying ABC analysis in manufacturing and inventory management
ABC analysis has many applications in the realm of manufacturing and inventory management:
- Setting management priorities. ABC inventory analysis helps set management priorities by identifying which items require more management attention. Resources can be allocated more effectively, with A-items receiving the highest level of scrutiny and control, ensuring that investments in inventory management yield the greatest return.
- Applying reordering strategies. By categorizing items, ABC analysis allows companies to apply different reordering strategies for each category. A-items, due to their high value and impact on the business, require more attention and a more frequent replenishment schedule. This ensures that these important items are always available to meet customer demand, thereby optimizing stock levels and reducing the risk of stockouts.
- Determining stocktake frequency. ABC classification significantly influences stocktake frequency. High-value A-items may require more frequent counts to ensure accuracy, given their substantial impact on financials and operations. In contrast, C-items, being less critical, can have less frequent stocktakes, enabling you to save time and resources while still maintaining adequate inventory control.
- Physical optimization of the warehouse. The physical arrangement of the stockroom can also be optimized based on ABC analysis. In this case, SKUs should be categorized based on their movement frequency, i.e. fast-selling or frequently consumed A-items can be positioned for easy access and handling, reducing time and labor in inventory handling. This warehouse organization not only streamlines operations but also minimizes errors and enhances overall efficiency in inventory management.
In conclusion, applying ABC analysis in manufacturing and inventory management provides a structured approach to managing inventory. It enables businesses to focus on the most critical items, streamline their processes, and optimize the use of resources.
Benefits of ABC analysis
As evident from the numerous applications of ABC analysis in manufacturing and inventory management, implementing the technique comes with an array of advantages. These include:
- Prioritizing important items. ABC analysis leads to more efficient management processes by enabling you to focus on high-value items and ensure sensible resource allocation.
- Improved inventory accuracy. Determining your A-category items allows you to cycle count these goods more often than other category items. This improves inventory accuracy for those items deemed most important for the business.
- Reduced risk of stockouts and excess inventory. Employing more stringent supply chain management practices for your A-items helps avoid shortages of goods most valuable for the business. On the other hand, ABC inventory enables you to reduce the inventory levels of C-category items.
- Lower inventory costs. ABC inventory management helps companies identify C-items that are typically low-value but high in quantity. Reducing this type of inventory directly helps reduce carrying costs, free up capital, improve cash flow, and bolster the bottom line.
- Targeted forecasting. The ABC classification allows businesses to focus on A-items, i.e. the goods that significantly impact customer demand. This targeting helps companies make sure that high-value items are readily available, aligning inventory levels with market needs.
Proper ABC inventory analysis, therefore, could have a great effect on your overall inventory efficiency. By focusing on optimizing stock levels and replenishment strategies for high-priority items, businesses can improve inventory turnover, ensuring efficient use and management of their inventory.
How to conduct an ABC analysis
While ABC inventory analysis may seem a daunting task at first, using this step-by-step guide will help you succeed.
ABC analysis divides your SKUs into three categories:
- Category A represents a small percentage (around 20%) of the total number of SKUs but has the highest value (ca. 80% altogether).
- Category B represents a moderate amount of the total inventory with a moderate criterion value.
- Category C represents SKUs that constitute a large part of the total number of items (about 50%) but have a low criterion value (ca. 5% altogether).
It is strongly recommended to use consumption value or revenue generated by an SKU as the criterion, as quantity or volume is often not a great indicator of business value. Use an SKU’s consumption volume only when conducting ABC analysis for physically organizing your inventory.
An item’s consumption value can be calculated by taking the item’s cost per unit and multiplying it by its quantity used during a set period, preferably a year.
You can also easily find all your items’ yearly consumption values in your MRP software .
To not over-complicate the example, we are using a very small number of products and consumption for calculations.
You can, however, use ABC analysis for inventory management in a business of any scale.
Find the percentage by dividing the item’s yearly consumption value by the total yearly inventory consumption value and multiplying the result by 100.
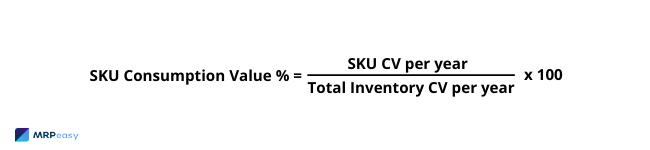
Now add up the percentages starting from the largest.
When you reach the cumulative 80%, set a cap. The items making up the 80% belong to the A-category.
Then start again with the next items to get the 15% for the B-category, and then 5% for the C-category.
Approximations are absolutely fine if you cannot reach a perfectly round cumulative percentage.
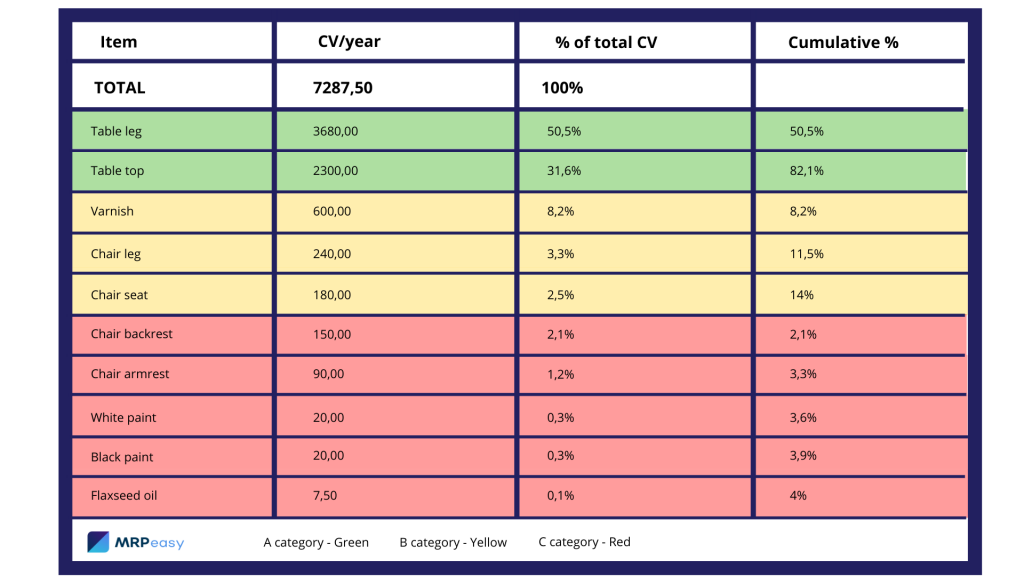
How to use ABC analysis
Now, as your inventory is categorized by the value of the SKUs, it is time to put it into use.
Take a look at how you have managed your inventory so far. Do you keep the same amount of stock regardless of the article? Do you apply the same purchasing policies to all items? Do you spend an equal amount of time reviewing all SKUs?
If any of your answers to these questions was “yes”, you can put the analysis to good use right away.
Start by rethinking your purchasing and inventory policies according to the priorities set, giving more attention to A- and B-category SKUs, and less to C-items.
A-category items should get:
- Higher service levels and mathematically defined reorder points and safety stocks;
- More review time per item;
- More attention when negotiating prices and lead times with the suppliers;
- More resources allocated to them in general.
B-category items should receive less attention than A-class items, but should not be overlooked.
C-category items should receive the least amount of attention. In fact, C-items can most often be purchased in bulk or by using a just-in-time approach .
You can also assign separate key metrics and reporting practices to different class items to get better insight into their performance.
For example, A-items could be assigned a monthly review while C-products would be reviewed once every six months.
By doing all that, you could substantially cut down on inventory, reduce holding costs, prevent stock-outs and overstocking, develop a more reliable order processing time, and keep customers happy.
It is recommended to re-analyze your inventory at least once a year, as regular re-analysis can help you track changes in SKU performance and act accordingly by reassigning them to another category.
Expertly manage your inventory with MRPeasy
Limitations of ABC analysis
Although ABC analysis is seemingly very easy to conduct and provides plenty of benefits, it has its limitations.
- Spreadsheets could quickly become unreliable . Excel spreadsheets require manual updating and are prone to human error, making them less reliable for dynamic inventory classification, particularly in growing operations.
- Its stability depends on the stability of your items’ performance. Be prepared for a portion of your SKUs to be reclassified each time you review the analysis.
- To use it, you are going to need reliable historical cost and demand data by item. That means in case new items enter your inventory, you can reliably categorize them only after a while.
- It is static, which means it does not account for changing consumption patterns, e.g. seasonality. ABC analysis often categorizes inventory based on historical data. This static approach can lead to inaccuracies in a rapidly changing market, especially in e-commerce, where consumer preferences and demand patterns shift quickly.
- It does not account for essential items that are used infrequently. Especially in manufacturing, inventory items may have interdependencies that are crucial for building final products. ABC analysis might leave SKUs that are rarely consumed yet are still essential to your business without the necessary attention.
- Managing large inventories can be very complicated. In businesses with extensive and diverse inventory, the simplicity of ABC analysis can be a drawback. The process can become cumbersome and less effective when dealing with a vast array of products.
In conclusion, while ABC analysis is a useful framework for inventory management, its effectiveness can be limited by several factors that businesses need to account for.
XYZ analysis
One of the greatest weaknesses of ABC analysis is that it employs only one parameter – mostly consumption value – and does not account for fluctuations in consumption.
For example, if you have items that are consumed in huge quantities at a time but very irregularly, then by using the ABC method, you could classify them as A-class articles. However, due to them being used periodically, having a large amount of them in inventory at all times would be unfeasible.
More so, it would be detrimental to business due to elevated holding costs, and possible damaging or perishing of the goods .
On the other hand, having an item with low but constant demand would be classified as a C-article. Stable demand, however, requires constant attention. That means we are going to need to account for the various levels of uncertainty in consumption. This is where XYZ analysis comes into the equation.
XYZ analysis is a great addition to your ABC analysis if the demand for some of your items fluctuates.
While ABC analysis classifies items by value, XYZ analysis categorizes them by uncertainty of demand.
Using ABC analysis together with XYZ gives you a much better overview of which articles in your inventory should get more attention. It helps avoid shortages and overstocking by providing the basis to accurately determine stock and safety stock levels in case the demand for your SKUs is not always stable.
How to conduct an XYZ analysis
Similarly to the ABC analysis, the XYZ approach divides your stock into three categories:
- X marks items with stable consumption.
- Y marks items with some fluctuation in consumption.
- Z marks items with heavy fluctuation in consumption.
You can categorize your inventory with the XYZ analysis by looking at your items’ historical data and seeing by what margin their weekly or monthly consumption fluctuates.
You can also get a simple visual overview of each item’s fluctuations in stock from your MRP system at Stock -> Items -> Item Reports.
Assigning your items both into the ABC and XYZ categories will give you a classification matrix with 9 categories:
- AX items have high consumption value and stable demand
- AY items have high consumption value and fluctuating demand
- AZ items have high consumption value and greatly fluctuating demand
- BX items have average consumption value and stable demand
- BY items have average consumption value and fluctuating demand
- BZ items have average consumption value and greatly fluctuating demand
- CX items have low volumes and stable demand
- CY items have low volumes and fluctuating demand
- CZ items have low volumes and greatly fluctuating demand
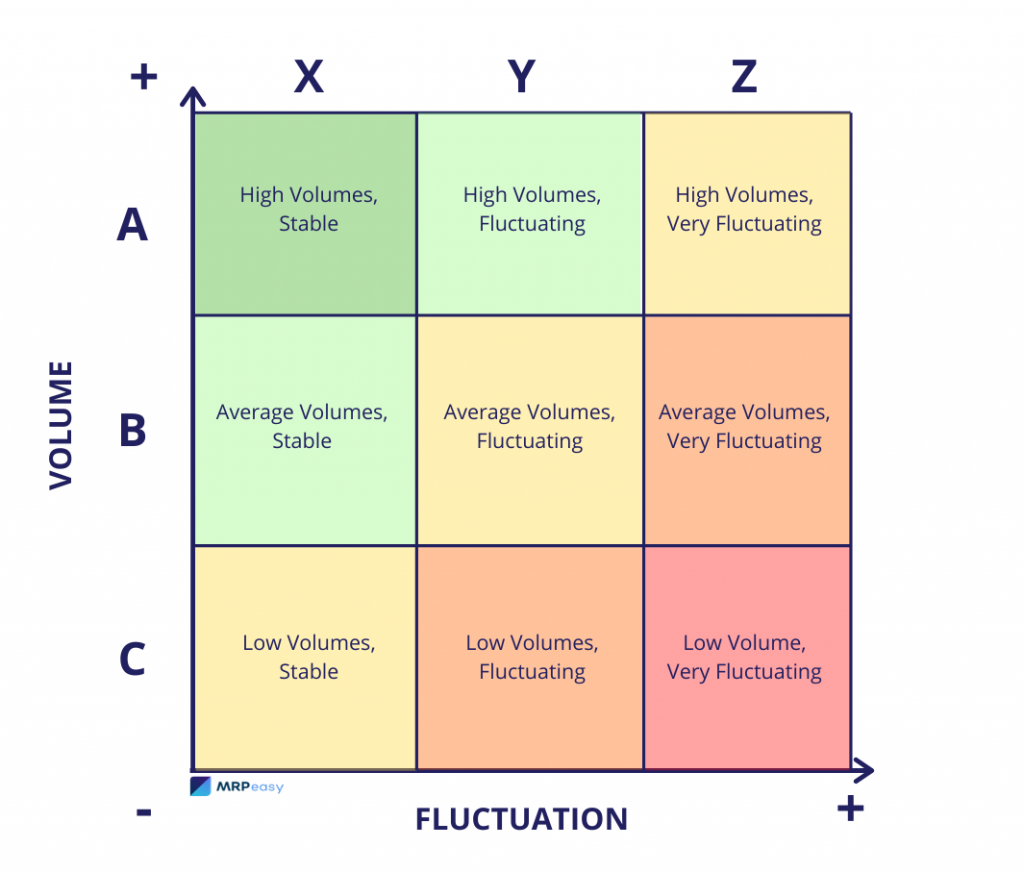
How to use XYZ analysis
You can use the ABC XYZ analysis to better determine the items’ safety stock.
- AX or BX items are stable , so, counter-intuitively, they do not need a lot of buffer for a high service level.
- AY or BY items have noticeable fluctuations so they require more inventory coverage.
- AZ or BZ items have very uncertain demand so they would need a larger amount of safety stock for a high service level. So, it may be reasonable to define a lower service level for these to free up cash and reduce standing inventory.
For C-class items with low consumption value, you do not need a lot of safety stock by definition.
- Large fluctuations of CX items rarely occur , so they have a very low risk of stock-outs or overstocking.
- CY items would require more safety stock due to fluctuations in demand to prevent stock-outs, but that may not be so important to your core business.
- CZ products can make up a large portion of your inventory and have a high risk of overstocking. For those items, you could have a minimum amount of safety stock available, or none at all.
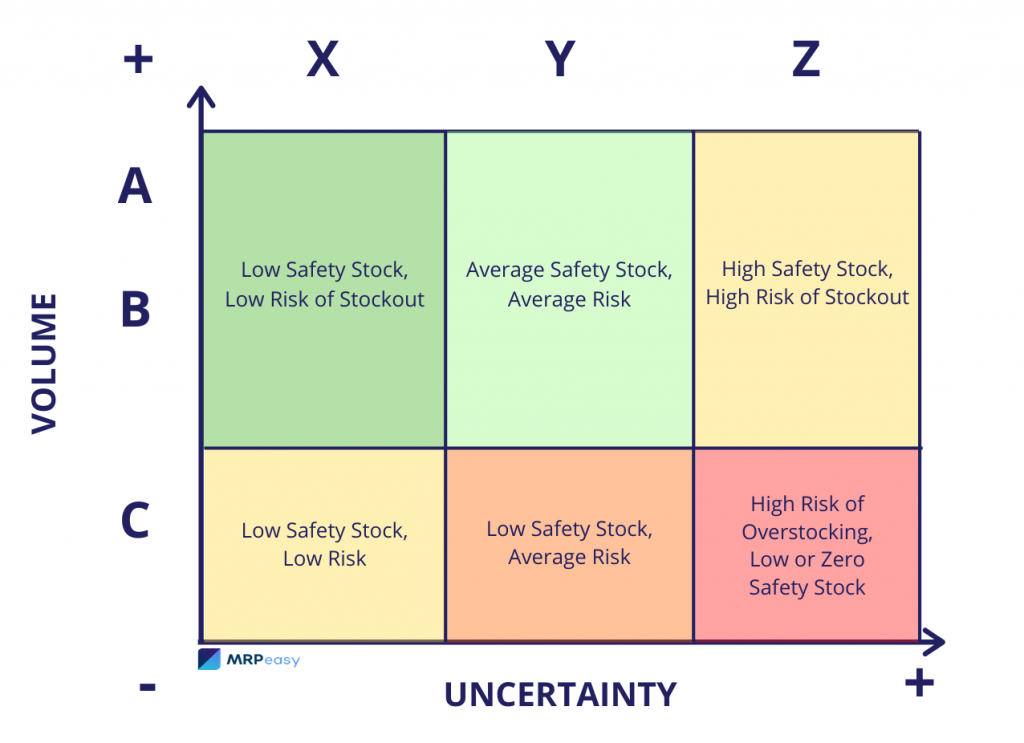
Using ERP software for inventory optimization
Utilizing an inventory management system such as manufacturing ERP software can significantly aid in conducting ABC analysis and optimizing inventory.
ERP systems consolidate and analyze vast amounts of data related to sales, procurement, production, and inventory levels, which is crucial for categorizing items into A, B, and C groups based on their value and turnover. By providing detailed insights into inventory usage patterns, costs, and demand trends, an ERP system helps in identifying high-value items (Category A) that require close monitoring and frequent replenishment, moderate-value items (Category B) needing less frequent attention, and low-value but essential items (Category C) that might benefit from bulk purchasing or just-in-time ordering.
In terms of overall inventory optimization , an ERP system offers real-time tracking and automated reporting features, enabling more accurate forecasting and demand planning. This results in better stock level management, reduced overstock or stockouts, and improved cash flow. The integration capabilities of ERP software also mean that information from various departments is centralized, allowing for a more coordinated approach to inventory management, where purchasing, production, and sales data are aligned to ensure inventory efficiency across the entire supply chain. This holistic view provided by an ERP system is essential for effective inventory optimization, ensuring that resources are allocated effectively and inventory investment is aligned with business goals.
Key takeaways
- ABC analysis is a practical inventory control technique that enhances supply chain insight, reduces sitting inventory, and improves inventory efficiency.
- It categorizes stock-keeping units based on importance or consumption value, applying the Pareto principle to identify a small percentage of items constituting the majority of inventory value.
- This analysis aids in setting management priorities, with high-value A-items requiring more attention and control, thereby optimizing resource allocation and inventory management.
- ABC analysis influences stocktake frequency and guides the physical arrangement of stockrooms, ensuring high-demand items are easily accessible, thereby streamlining operations and minimizing errors.
- The technique has broad applications in manufacturing and inventory management, leading to improved inventory accuracy, reduced stockouts and excess inventory, and lower inventory costs.
- While effective, ABC analysis has limitations, including reliance on static historical data, which may not accurately reflect changing market dynamics or inter-item dependencies, especially in large, diverse inventories.
- Supplementing ABC analysis with XYZ analysis, which categorizes items by demand uncertainty, provides a more comprehensive approach, helping to avoid shortages and overstocking, and optimize safety stock levels.
Frequently asked questions
In a retail store, ABC analysis might categorize high-cost electronics like laptops as ‘A’ items, moderately priced appliances like blenders as ‘B’ items, and low-cost accessories like phone cases as ‘C’ items, based on their value and sales volume.
Analyzing ABC analysis involves categorizing inventory items based on criteria such as sales volume, consumption value, or frequency of use, and then using this categorization to prioritize inventory management efforts and resources.
ABC market analysis is a strategic approach where a market or customer base is segmented into three categories – A, B, and C – based on factors like revenue generation or purchasing behavior, to prioritize marketing and sales efforts towards the most profitable segments.
XYZ analysis is an inventory categorization technique that classifies items based on the variability of their demand. ‘X’ items have stable and predictable demand, ‘Y’ items have moderately fluctuating demand, and ‘Z’ items experience high levels of demand variability. This analysis helps in optimizing inventory management by aligning stocking strategies with the predictability of demand.
You may also like: 11 Most Important Inventory Management KPIs
Get manufacturing know-how delivered to your inbox!

Madis Kuuse
Madis is an experienced content writer and translator with a deep interest in manufacturing and inventory management. Combining scientific literature with his easily digestible writing style, he shares his industry-findings by creating educational articles for manufacturing novices and experts alike. Collaborating with manufacturers to write process improvement case studies, Madis keeps himself up to date with all the latest developments and challenges that the industry faces in their everyday operations.
Related articles
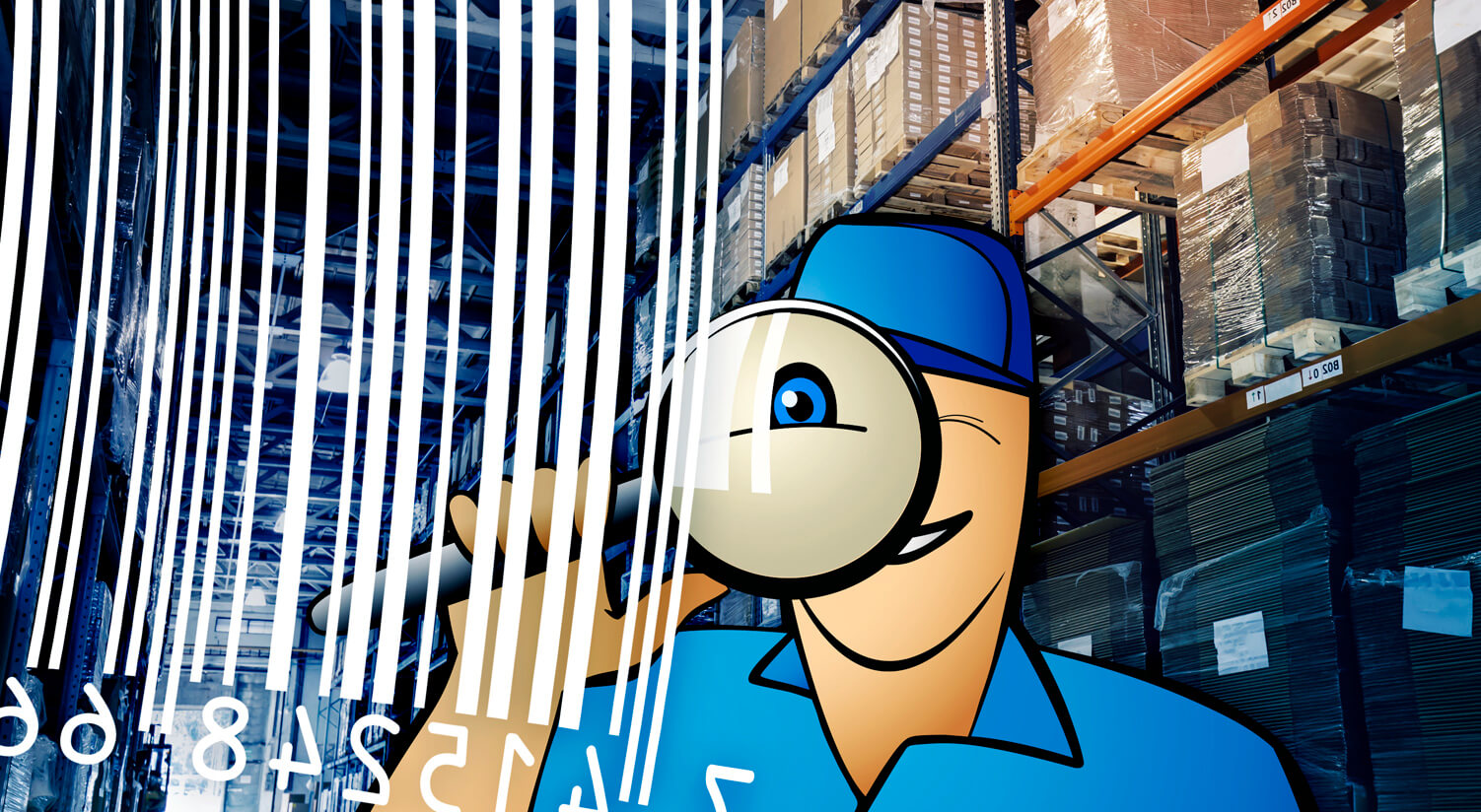
Privacy Policy Update
You can read our full privacy policy and terms of service .
These cookies help us track site metrics to improve our sites and provide a better user experience.
These cookies used to serve advertisements aligned with your interests.
These cookies are required to provide basic functions like page navigation and access to secure areas of the website.
Don't Leave Yet!
Click through the PLOS taxonomy to find articles in your field.
For more information about PLOS Subject Areas, click here .
Loading metrics
Open Access
Peer-reviewed
Research Article
Computed ABC Analysis for Rational Selection of Most Informative Variables in Multivariate Data
Affiliation DataBionics Research Group, University of Marburg, Hans–Meerwein—Straße, 35032, Marburg, Germany
* E-mail: [email protected]
Affiliations Institute of Clinical Pharmacology, Goethe—University, Theodor—Stern—Kai 7, 60590, Frankfurt am Main, Germany, Fraunhofer Institute for Molecular Biology and Applied Ecology IME, Project Group Translational Medicine and Pharmacology TMP, Theodor–Stern—Kai 7, 60596, Frankfurt am Main, Germany
- Alfred Ultsch,
- Jörn Lötsch
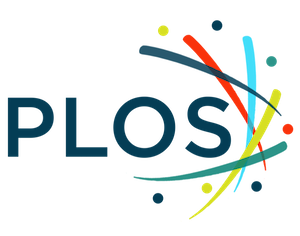
- Published: June 10, 2015
- https://doi.org/10.1371/journal.pone.0129767
- Reader Comments
Multivariate data sets often differ in several factors or derived statistical parameters, which have to be selected for a valid interpretation. Basing this selection on traditional statistical limits leads occasionally to the perception of losing information from a data set. This paper proposes a novel method for calculating precise limits for the selection of parameter sets.
The algorithm is based on an ABC analysis and calculates these limits on the basis of the mathematical properties of the distribution of the analyzed items. The limits im-plement the aim of any ABC analysis, i.e., comparing the increase in yield to the required additional effort. In particular, the limit for set A, the “important few”, is optimized in a way that both, the effort and the yield for the other sets (B and C), are minimized and the additional gain is optimized.
As a typical example from biomedical research, the feasibility of the ABC analysis as an objective replacement for classical subjective limits to select highly relevant variance components of pain thresholds is presented. The proposed method improved the biological inter-pretation of the results and increased the fraction of valid information that was obtained from the experimental data.
Conclusions
The method is applicable to many further biomedical problems in-cluding the creation of diagnostic complex biomarkers or short screening tests from comprehensive test batteries. Thus, the ABC analysis can be proposed as a mathematically valid replacement for traditional limits to maximize the information obtained from multivariate research data.
Citation: Ultsch A, Lötsch J (2015) Computed ABC Analysis for Rational Selection of Most Informative Variables in Multivariate Data. PLoS ONE 10(6): e0129767. https://doi.org/10.1371/journal.pone.0129767
Academic Editor: Yongtang Shi, Nankai University, CHINA
Received: February 19, 2015; Accepted: May 13, 2015; Published: June 10, 2015
Copyright: © 2015 Ultsch, Lötsch. This is an open access article distributed under the terms of the Creative Commons Attribution License , which permits unrestricted use, distribution, and reproduction in any medium, provided the original author and source are credited
Data Availability: This is a method paper. The example used for demonstration purposes is taken from the authors' previous work. All data is available in Table 2 of the paper, originally from the published reference [ 3 ] (Neddermeyer TJ, Flühr K, Lötsch J (2008) Principal components analysis of pain thresholds to thermal, electrical, and mechanical stimuli suggests a predominant common source of variance. Pain 138: 286-291) In addition, the related R library is freely available at http://cran.r-project.org/web/packages/ABCanalysis/index.html .
Funding: The research received funding, in particular the necessary computation equipment, from the European Union Seventh Framework Programme (FP7/2007 – 2013) under grant agreement no. 602919 (JL). The funders had no role in method design, data selection and analysis, decision to publish, or preparation of the manuscript.
Competing interests: The authors have declared that no competing interests exist.
Introduction
A recurring problem in biomedical research is the high dimensionality of data sets and the complexity of derived results. Multivariate data sets often differ in several factors or derived statistical parameters, which have to be selected for a valid interpretation. This selection is usually based on contextual and mainly traditional statistical limits. This leads occasionally to the perception of losing information from a data set; however, crossing the accepted statistical limits will be rejected almost certainly by a scientific audience. Dealing with the problem of statistical limits is an active research topic; however, the correct statistical approach at a rational selection of the most informative set of variables derived from multivariate analyses is not obvious. Scientists are therefore often inclined to use conservative statistical selection criteria to avoid α error. This is widely accepted but has a tendency toward occasionally disregard of valid information from experimental data.
Therefore, a theoretical basis for the selection of parameter sets that are interpretable in multivariate data is highly desirable to identify the optimum information that can be validly retrieved from biomedical data. The present report proposes a novel method that uses concepts developed in economical sciences. In particular concepts are used in the search for a minimum possible effort that gives the maximum yield. In many circumstances it has been observed that this converges toward the effect that with 20% of the effort 80% of all yield can be obtained, which is commonly called the “Pareto 80/20 rule” [ 1 , 2 ]. A more general approach is the so-called “ABC analysis”, which divides the data set into the three disjoint sets A, B and C, in such way that set “A” should contain the “important few”while set “C” contains the “trivial many” [ 2 ].
The determination of the set limits for an ABC analysis has so far been left to subjective considerations. In this paper, a calculation method is presented that allows calculating these limits on the basis of the mathematical properties of the distribution of the analyzed items. The utility of the proposed method will be illustrated by an example from own previous research [ 3 ] where this method improves the biological interpretation of the results and increased the fraction of valid information that can be obtained from experimental data. Further biomedical applications, such as deriving screening tests from complex test batteries, will be discussed.
Properties of ABC curves
- PPT PowerPoint slide
- PNG larger image
- TIFF original image
In addition, APC plots of the identity distribution, x i = constant (magenta line), and of the uniform distribution in the data range U[min(xi) , max(xi)] (green line) are shown. The second main diagonal (dashed line) delivers the points where the yield Y equals the remaining effort described as Y = 1—E . The BreakEven point, i.e., the point on the ABC curve where the profit gain dABC equals 1 which means that the slope of the ABC curve at this point equals a value of 1, is marked a as green star. The limits of sets A, B and C resulting from the present ABC analysis are drawn as red lines.
https://doi.org/10.1371/journal.pone.0129767.g001
ABC curves are always non-decreasing concave functions in the unit square space. They are scale-invariant in the values of x . That is, a multiplicative modification of the x-values does not modify the ABC curve, i.e., ABC(x) = ABC(x∙c) for any c ≠ 0. However they are not invariant to the location of x . That is, an additive modification of the values of x does modify the ABC curve, i.e., ABC(p) ≠ ABC(p+c) for any c ≠ 0. For x i ’ = x i + c with c > 0, the ABC curve ABC(x’) will flatten and approach the identity distribution (green line in Fig 1 ). A special case is observed when x i approaches a value of 0. Then, the curve approaches a “winner-takes-it all” distribution, i.e., tends to take a path through the points (0,0)—(0,1)—(1,1). Thus, the localization of ABC curves of a given data set relatively to (i) the identity distribution, i.e., all x i have the same value x i = c , and (ii) the uniform distribution, i.e., all values that x i can take are equally likely in the interval from min(x i ) to max(x i ) , can be used to investigate the inequality of a distribution ( Fig 1 ). Specifically, for all more right-skewed data distributions than the uniform distribution, the ABC curves will be located more toward the upper left corner of the plot.
ABC curves are related to Lorenz curves as follows: Let L(p) be a Lorenz curve for a probability distribution. The corresponding ABC curve ABC(p) can be derived as ABC(p) = 1—L(1—p) and vice versa L(p) = 1—ABC(1—p) . Following this interrelations, theoretical properties of ABC curves can be derived from the corresponding Lorenz curves. Moreover, analytical derivations of well-known distributions available for Lorenz curves can also be used for ABC curves ( Table 1 ).
https://doi.org/10.1371/journal.pone.0129767.t001
Calculation of precise limits for ABC analysis
An ABC analysis aims at identifying the minimum possible effort that gives the maximum yield. It divides the values x 1 , …, x n into three disjoined sets A, B, and C [ 8 ]. Set A should contain the “critical few”, i.e., those elements that allow obtaining a maximum of yield with a minimal effort [ 1 , 2 ]. Set B comprises those elements where an increase in effort is proportional to the increase in yield. In contrast, set C contains the “trivial many”, i.e., those elements with which the yield can only be achieved with an over-proportionally large additional effort. The determination of these sets has been so far left to subjective judgments [ 8 , 9 ].
The derivation of statistically justified set limits regards the increase in “yield”( Y ) versus the increase in “effort” ( E ). Formally, this is the first derivative (slope) of the ABC curve ( dY/dE = dABC ), in the following called “profit gain”. Set A should contain profit gains > 1 ( COND1 ), set B should contain profit gains around a value of 1 ( COND2 ), while the profit gain in set C should be substantially less than 1 ( COND3 ). During ABC analysis the yield Y should be maximized while the necessary effort E minimized. Thus, to obtain the limit between sets A and B two variables need to be optimized. Moreover, as maximizing Y can be achieved via maximizing the unrealized yield UY = 1 –E , the optimization problem can be reduced to concomitantly minimizing both, E and UY .
Derivation of the limit between sets A and B.
The fraction of the first i elements to n (i/n) represents costs or efforts ( E i = i/n ), the fraction of the cumulative sum of the first xi values with regard to the total sum of the x i is called the yield, Y i , of the set x 1 , …x i . The ABC curve (blue line) is a plot of Y i versus E i . Intermediate points are interpolated by means of quadratic splines [ 7 ]. The ABC curve is independent of m. The red star marks the so called Pareto point A(A x , A y ) , i.e., the point at the smallest distance (left oblique black line) to the ideal point at, xy, E = 0 and Y = 1 . The green star marks the point on the ABC curve where its slope, dY/dE , equals 1. At this point, the profit gain dABC equals 1, therefore it is called the BreakEven point B(Bx , By) . The blue star marks the point C(C x , C y ) that has the smallest distance to the ideal situation where all gain has been achieved, i.e., E = B x and Y = 1 (right oblique black line).
https://doi.org/10.1371/journal.pone.0129767.g002
The point on the ABC curve which has the smallest (Euclidean) distance to ABCideal is called the Pareto point A(A x , A y ) . Its x-value, A x , provides a precise limit for data points in the set A. The point on the ABC curve where the profit gain dABC equals a value of 1 is called the “ BreakEven” point B(B x , B y ) . Usually A x ≤ B x holds in practice. However, this cannot be guaranteed for all possible distributions. In the case of A x > B x the points A and B exchange their role in ABC analysis. This procedure assures COND1 for all distributions, i.e., for x i ≤ A x the “profit gain” is ≥ 1.
From this derivations the set limit between sets A and B, t AB , is given as min(A x , B x ) . Set A is defined as A = {x i | x i ≤ icdf(X , t AB *100)} where icdf(X , p) for X = { x 1 , …, x n . } is the x i corresponding to the p th percentile. Set A contains the largest values of x i down to a point where the ABC curve is closest to the ideal situation of zero effort and complete yield, as long as the ProfitGain is larger than 1. For the Uniform distribution set A contains the largest 41% of all values ( Fig 2 , red star). A geometric interpretation of the set limit between A and B is the point on the ABC curve that has the smallest distance from the ideal point (0,1; black line to the red star in Fig 2 ).
Derivation of the limit between sets B and C.
According to the characteristics of an ABC analysis, the profit gain in set C should be substantially less than 1 ( COND3 ). At an ideal point B y = 1 , called the BreakEven point, all yield would be gained. The point on the ABC curve at a minimum distance from this ideal point ( B x , 1 ) is called SubMarginal point ( C x ,C y ). For all points to the right of C x , i.e., x i ≥ C x , the profit gain is substantially less than 1 as required by COND3 . From this derivations the set limit between sets B and C is given by t BC = C x and set C is C = {x i | x i > icdf(X , t BC *100))} . For the Uniform distribution, set C contains the smallest 38% of the values ( Fig 2 blue star). The remaining values of x i , neither associated to set A nor to set C, have to belong to set B. This set contains the values “around” a profit gain of 1 ( COND2 ). For the uniform distribution these profit gains are in the range of 0.78 to 1.18. A geometric interpretation of the set limit between B and C is determined by the point at the smallest distance to the ideal point ( B x , 1 ; black line to the blue star in Fig 2 ).
The programs used to calculate the following ABC curves, which also perform the described precise ABC analysis and draw ABC plots, are part of the R package “ABCanalysis” (M. Thrun, Marburg, Germany) published on CRAN at http://cran.r-project.org/web/packages/ABCanalysis/index.html .
ABC analyses of known distributions
This probability distribution is a typical example of a right skewed inequality distribution. The magenta lines indicate the location of the median (0.47) of the distribution (see also Fig 1 for comparison with less skewed distributions).
https://doi.org/10.1371/journal.pone.0129767.g003
https://doi.org/10.1371/journal.pone.0129767.g004
ABC analysis of biomedical sample data
The following example from biomedical research shows the utility of the present analysis for providing a statistically valid rationale selection of components for principal components analysis. Specifically, empirical data often consist of a high dimensional set of observed variables. For example, we have previously analyzed the sources of variance of pain thresholds to six different nociceptive stimuli, i.e., thermal (heat or cold), electrical or mechanical (blunt or punctate pressure) pain stimuli [ 3 ]. Some of these variables showed a (linear) correlation with others. For dimensionality reduction without losing too much information and a conversion of the possibly correlated variables into a set of values of linearly uncorrelated variables, a principal component analysis (PCA) was used. This resulted in eight variance components ( Table 2 ). Setting the limit of the number of principal components, PCs, ( Fig 5 ) at the traditionally advised Kaiser-Guttman criterion of an eigenvalue > 1 of the covariance matrix [ 11 , 12 ] resulted in three major sources of variance that could be used to interpret the most important sources of variance of human pain thresholds. A similar selection of PCs also results when applying the “elbow criterion” in a so-called scree plot of the absolute values of the eigenvalues sorted for decreasing size ( Fig 5 top left, red curve). The elbow criterion is estimated as the point where the steep slope to the left of the scree plot levels to a flat slope [ 13 ]. The PCs thus identified by these classical criteria as results of this analysis carried high loadings from all pain stimuli (PC #1), from electrical, blunt pressure and thermal pain stimuli (PC #2) or from punctate pressure pain stimuli (PC #3). However, these PCs failed to translate the distinction between thresholds despite the involvement of different receptors in their perception [ 14 ].
Left panel: sample data showing the original results of a principal component analysis (PCA) of the covariance matrix among six different measurements of pain thresholds [ 3 ]. For classical selection of the set of relevant (largest) eigenvalues, either the Kaiser-Guttman criterion (eigenvalue > 1, left top panel , magenta line) [ 11 , 12 ] or the “elbow criterion” [ 13 ] in a so-called scree plot of the absolute values of eigenvalues sorted by descending size (left top panel, red curve) can be used. According to the PCA results, the sources of variance of pain thresholds to different nociceptive stimuli comprise eight variance components (see Table 2 in [ 3 ]). The left bottom panel shows the cumulative fraction of the variance explained by the principal components, with indication of the variance explained by the components that could be selected based on the classical criteria. It can also be seen that the inclusion of a further principal component would have provided a better coverage of the total variance. Right panel: ABC plot of the same data. The ABC curve (blue line) shows from bottom to top the increasing faction of the total sum of all eigenvalues and from left to right the increasing fraction of the total number of variance components which contribute these eigenvalues. The goal of the analysis is to identify the number of variance components (abscissa) that is associated with a satisfactorily high coverage (ordinate) of the total sum of available eigenvalues. Set A contains the largest data points, corresponding to the largest proportion of yield. For this particular distribution the set limit for A is obtained by the point with slope of the ABC curve of 1 (green star) resulting in the two largest eigenvalues. The set B consist of the next two eigenvalues including the Pareto point, i.e., the point closest to (0,1) (red star). In the present example the cumulative variance of component #1, 2and 3 contributed 75% to the total variance. The inclusion of component #4 results in a cumulative explained variance of 84%. On the other end, set C contains 50% of the eigenvalues, which share only 16% of the variance and are obviously of minor importance.
https://doi.org/10.1371/journal.pone.0129767.g005
https://doi.org/10.1371/journal.pone.0129767.t002
The present ABC analysis can provide a better alternative to the rather subjective Kaiser-Guttman or Elbow criteria. Specifically, following calculation of precise limits for the obtained eigenvalues, set A contained the largest eigenvalue while set B contained three further eigenvalues ( Fig 5 ). Thus, when disregarding set C with the four smallest eigenvalues, the present analysis provides support to take four eigenvalues into account, instead of three eigenvalues when applying classical limits. The attritional PC, with an eigenvalue of 0.74 that had to be dropped from the results in the classical analyses, carried loadings from Heat + capsaicin pain threshold (see Table 2 in [ 3 ]). This better reflects the different molecular biology involved in the perception of heat pain, mediated via ion channels such as TRPV1 and TRPV4 [ 15 ], from the perception of pain evoked by other stimuli. Thus, the present ABC analysis substantially improved the identification of the important few among the variance components of pain thresholds. The curvatures of the ABC curve for the eigenvalues correspond to a multimodal probability density function of the data [ 16 ]. Moreover, the modified results were not only biologically more meaningful; they also included a larger part of the information contained in the pain thresholds data set. That is, while the Kaiser-Guttman criterion [ 11 , 12 ], requesting an eigenvalue > 1 for a PC to be considered, explained only 75% of the total variance in the pain thresholds, the ABC analysis resulting in n = 4 PCs provided 84% of the total variance explained, to which set A of n = 1 PCs contributed 48% ( Fig 5 bottom left).
In the analysis of multivariate biomedical data the usually peremptory application of traditional statistical limits is sometimes perceived as leading to a loss of information that could have been validly drawn from a data set. Without a theoretical basis, however, crossing classical limits cannot be advised. We therefore suggest a method to identify the “important few” from sets of items that show a clear inequality in their distribution and provide a calculation of precise set limits based on mathematical properties of the distribution of the analyze items. The present method is based on a calculated ABC analysis, replacing the traditional subjective estimations of ABC set limits by algorithmically determined optimal limits. The innovation of the present method consists of using minimization of the effort and of the unrealized yield, together with optimization of the slope of the ABC curve to precisely calculate these limits as a basis for a valid selection criterion for items from a set of data or parameters.
ABC analyses have their roots in economic thinking. That is, the success of a business depends on efficiency in the sense that returns are always regarded with respect to the efforts or costs required to obtain them. Therefore a large application domain of ABC analysis is business administration or material management. However, its application into the biomedical domain relates to effect sizes, which are ubiquitously addressed in this field. For example, the modest predictive value of common genetic variants in human traits can be attributed, despite statistically significant effects, to the mostly small effect sizes conferred by these variants [ 17 ]. The present ABC approach directly addresses this issue by selecting the “important few”, i.e., those items that confer the relatively largest effect sizes. In this respect, it completely fits with contemporary statistical data analysis approaches and is meant to be used there as the example of pain threshold variance components emphasizes.
Indeed, when exemplary joining genetics and pain thresholds, common functional variants exert small effect sizes [ 18 ] but when combined, they are able to predict particular pain phenotypes at an accuracy of 80% [ 19 ]. When applying ABC analysis to that data, the variants that have previously been included in the predictive combined genotype were identified as those lying in ABC set A (details not shown). A further example of the utility of the present method in biomedicine is the applicability of the effort versus gain problem to common medical screening test problems. A common desire of physicians in practice is the availability of short and easily applicable tests. This has led to various efforts to create abbreviated tests from comprehensive test batteries, such as a three-item test for olfactory diagnosis derived from a comprehensive 48-item test [ 20 ]. The development of this test is, retrospectively, a candidate for an ABC analysis, which could provide the important few olfactory tests items on a statistically valid level rather than the intuitive selection that had been applied when developing the test.
An advantage of the present method is its applicability to small data sets such as the present example of pain threshold data containing only eight data points (the eight eigenvalues obtained by means of PCA). For small numbers of points the ABC analysis relied on the quadratic spline interpolation of the ABC curve. This interpolation has been established as optimal for generating valid Lorenz curves [ 7 ], therefore, via the above-explained relation of ABC curves to Lorenz cures this is also valid for ABC curves. Importantly, data preprocessing, typically consisting of adjustments of the data range and or variance, must take into account that ABC curves are invariant to scaling, i.e., multiplication by some constant but not to location, i.e., the addition/subtraction of a constant to the data. In particular ABC curves are only defined for non-negative data points x i ≥ 0. So a standardization of the data should be restricted to a mapping of the data to unit variance.
However, the method is neither restricted to biomedical data nor to small data sets. Another example where it can be applied is taken from demographic analyses. The “SwissInhabitants” data set was obtained from an official statistics source [ 21 ] and consists of the number of inhabitants in the 2896 villages and cities in Switzerland in the year 1900. Such data can be explored by the present method to describe the population structure of a country. Applying the present ABC analysis to this data set showed that 69% of the Swiss population lived in only 639 places (22%) in 1900( Fig 6 ). Nearly 80% of the villages were populated the remaining approximately 30% of the population. A reanalysis of the distribution for the year 1970 shows a concentration effect such that set A now contains only 19% of the cities in which 76% of the Swiss population lives. This somehow resembles the 80/20 rule. The “SwissInhabitants” data set was analyzed in detail in elsewhere [ 22 ] and is this freely available data set is also included as an example data set within the above-mentioned “ABCanalysis” R package published on CRAN at http://cran.r-project.org/web/packages/ABCanalysis/index.html .
The data consists of the number of inhabitants in the 2896 villages and cities in Switzerland in the year 1900. The analysis shows that 69% of the population lives in 22% of the locations. The ABC curve (blue line) is a plot of Y i , here the cumulative fraction of the Swiss population in 1900, versus E i , here the number of locations (villages or towns in Switzerland). The distribution shows higher inequality than the uniform (green line) and the identity (magenta line) distributions. The red star marks the so called Pareto point A(A x , A y ) , i.e., the point at the smallest distance (left oblique black line) to the ideal point at, xy, E = 0 and Y = 1 . The green star marks the point on the ABC curve where its slope, dY/dE , equals 1. At this point, the profit gain dABC equals 1, therefore it is called the BreakEven point B(Bx , By) . The freely available data set on which this analysis was done is also included as an example data set within the above-mentioned “ABCanalysis” R package published on CRAN at http://cran.r-project.org/web/packages/ABCanalysis/index.html .
https://doi.org/10.1371/journal.pone.0129767.g006
Finally, the relation of ABC curves to the so-called “Pareto 80/20 Rule”, mentioned above because of its broad recognition, needs clarification. There is no such thing as a “Pareto 80/20-Rule”. Juran has clarified [ 2 ] that he mistakenly attributed the 80/20 rule of “roughly 80% of the yield comes from 20% of the effects” to Vilfredo Pareto (1848–1923), who, however, has never published an “80/20-rule”. It should rather be called “Juran 80/20 Rule”. A family of probability distributions, which depend on a parameter α, are called Pareto distributions [ 26 ]. For a special value of α = 1.16 the ABC curve of this particular Pareto distribution passes through the point P = (0.2, 0.8). The, so called “80/20 rule” is just the observation that the ABC curve passes in many empirical situations close the point P. If there is a physical law, that systems tend to show ABC curves with the 80/20 rule, it is still unknown [ 27 ].
In this work mathematically defined unique and precise limits for an ABC analysis have been derived. The limits implement the aim of any ABC analysis, i.e., comparing the increase in yield to the required additional effort. In particular, the limit for set A, the “important few”, is optimized in a way that both, the effort and the yield for the other sets (B and C), are minimized. As a typical example from biomedical research, the feasibility of the ABC analysis as an objective replacement for classical subjective limits to select highly relevant variance components of pain thresholds is presented. The method is applicable to many further biomedical problems including the creation of diagnostic complex biomarkers or short screening tests from comprehensive test batteries. Thus, the ABC analysis can be proposed as a mathematically valid replacement for traditional limits to maximize the information obtained from multivariate research data.
Acknowledgments
We thank Michael Thrun for the implementation of the R package and his critical discussions.
Author Contributions
Conceived and designed the experiments: AU. Analyzed the data: AU JL. Wrote the paper: JL AU.
- 1. Pareto V (1909) Manuale di economia politica, Milan: Società editrice libraria, revised and translated into French as Manuel d’économie politique. Paris: Giard et Briére.
- View Article
- Google Scholar
- PubMed/NCBI
- 9. Wild T (2002) Best practice in inventory management. Oxford: Butterworth-Heinemann (imprint of Elsevier).
- 10. Krause EF (1988) Taxicab geometry: Adventure in non-euclidean geometry. Mineoly, NY: Dover Publications.
- 13. Krzanowski WJ (2000) Principles of Multivariate Analysis. A User’s Perspective. New York: Oxford University Press.
- 20. Hummel T, Pfetzing U, Lötsch J (2010) A short olfactory test based on the identification of three odors. J Neurol.
- 21. Schuler M, Ullmann D, Haug W (2002) Eidgenössische Volkszählung 2000—Bevölkerungsentwicklung der Gemeinden 1850–2000. In: Statistik Bf, editor. Neuchâtel: Bundesamt für Statistik. pmid:11186393
- 22. Behnisch M, Ultsch A (2010) Clustering of Population Patterns in Switzerland 1850–2000; Gaul W, Geyer-Schulz A, Schmidt-Thieme L, Kunze J, editors. Heidelberg: Springer.
- 23. Knoblauch J, Kurz J (2009) Die besten Mitarbeiter finden und halten. Die ABC-Strategie nutzen. Frankfurt, New Yoek: Campus Verlag.
- 24. Farrington B, Lysons K (2012) Purchasing and supply chain management. Harlow: Peason.
- 25. Färber I, Günnemann S, Kriegel H-P, Kröger P, Müller E, Schubert E, et al. On using class-labels in evaluation of clusterings. In: Fern XZ, Davidson I, Dy J, editors; 2010; Washington, DC.
- 26. Arnold BC (1983) Pareto distributions. Fairland, Maryland: International Cooperative Publishing House
Academia.edu no longer supports Internet Explorer.
To browse Academia.edu and the wider internet faster and more securely, please take a few seconds to upgrade your browser .
Enter the email address you signed up with and we'll email you a reset link.
- We're Hiring!
- Help Center
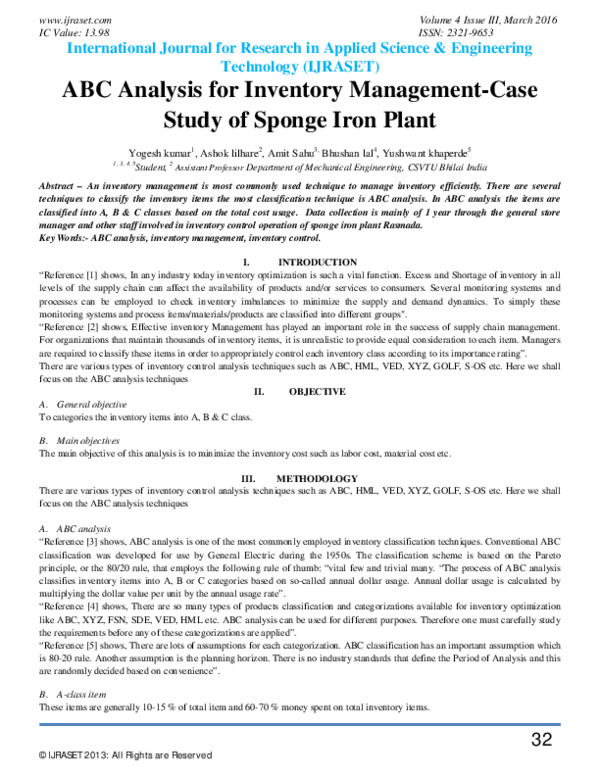
ABC Analysis for Inventory Management-Case Study of Sponge Iron Plant

An inventory management is most commonly used technique to manage inventory efficiently. There are several techniques to classify the inventory items the most classification technique is ABC analysis. In ABC analysis the items are classified into A, B & C classes based on the total cost usage. Data collection is mainly of 1 year through the general store manager and other staff involved in inventory control operation of sponge iron plant Rasmada.
Related Papers
Abbas Toloie Eshlaghy
Today, the effective inventory management plays an im-portant role in the success of the organizations in the new business environment. It is not clearly possible for the organizations that store hundreds of inventory items to economically design an inventory management policy for each inventory item separately. Moreover, various inven-tory items may play quite different roles in the business of the organization. Hence, the managers need to classify these items in order to control each inventory category properly based on its importance rating. This research is composed of the following sections: In the first section, the criteria affecting the evaluation of the inventory control system of the studied factory and the priority of each one of them will be identified, in the second section, the prior-ity of each criterion in each inventory category (A, B, C) is calculated using the analytical hierarchy process, in the third section, a mathematical model will be presented to evaluate th...
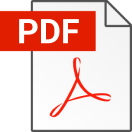
Industrial Engineering and Management
Ephrem Gidey
shashank soni
IOSR Journal of Business and Management
Dr. Dinesh Dhoka
International Journal of Research in Engineering, Science and Management
Maheshwar jaybhaye
This article is a contextual analysis of ABC and HML examination of inventory control methods for an effective Inventory administration framework in the assembling business of India. The organization needs to control its inventory expense, so it utilized various kinds of inventory methods to control this. There are various strategies, for example, ABC, HML, VED, XYZ and S-O-S. In the ABC analysis, things are classifications in classifications A, B, C as per the utilization of the all-out expense. In the HML analysis, things are classifications in H, M, L classification as per the unit cost. Information assortment is chiefly a year through the head supervisor of the store and other work force associated with the Inventory control activity of the assembling business.
Computers & Industrial Engineering
ching-wu chu
Lecture Notes in Management and Industrial Engineering
In this study, inventory and production control of a group of products from a major manufacturer of domestic and industrial gas meters is examined. ABC and XYZ analyses are carried out for the inventory items to determine the production strategy of each item class and the Economic Order Quantity (EOQ). After this examination, one of the end products of the company is chosen to develop the Materials Requirement Plan (MRP) for. The Bill of Materials (BOM) for the chosen product is created and MRP is developed according to the BOM levels. The monthly demand data for the final product is obtained based on the annual demand and the required quantities for all sub materials of the final product are calculated with MRP. Finally, after the ABC/XYZ analysis, BOM structuring, and MRP calculations, a user interface is developed in Excel using Visual Basic for Applications (VBA) to access, edit, and add the desired information easily.
Ankit Sappal
Inherent uncertainties in demands and supply make it difficult for supply chains to achieve optimum inventory replenishment, resulting in loss of sales or keeping excessive inventories. An unkempt inventory can take up to one-third of an organization's annual investment. Therefore, in order to compete with invariably erratic demands, it is not only challenging to develop an intelligent system to maintain and control an optimum level of inventory but has also become mandatory. Here we have tried to study the supply chain logistic management in manufacturing industry using XYZ analysis method. Supply chain: Supply chain management (SCM) is the oversight of materials, information, and finances as they move in a process from supplier to manufacturer to wholesaler to retailer to consumer. Supply chain management involves coordinating and integrating these flows both within and among companies Logistic: Logistics management is that part of the supply chain that plans, implements, and ...
rahmat nurcahyo
Journal of Advanced Management Science
makram ben jeddou
RELATED PAPERS
N. Pöllath et alii (eds.), Animals and Humans through Time and Space: Investigating Diverse Relationships. Essays in Honour of Joris Peters. Documenta Archaeobiologiae 16
Carola Metzner-Nebelsick
in Tesori dell’Iraq. Le Missioni Archeologiche Italiana nella Terra tra i due Fiumi, Treccani, Roma 2023, pp. 184-199
Luca Peyronel
George Wenzel
MATEC Web of Conferences
Pavel Prosuntsov
LILY BORELLI
Revista de Biologia Neotropical
VERA LUCIA GOMES KLEIN
InfoDesign - Revista Brasileira de Design da Informação
Ruth Nogueira
Journal of medical virology
Mahmoud Said Eid
Revista Austral de Ciencias Sociales
marcelo godoy
Bulletin of the American Physical Society
Dalila Robledo
Agustina Yañez
Harold Greeney
Bárbara Brum
Nephrology Dialysis Transplantation
Arthur Doyle
Carbon Management
Khajanchi Lal
METALLOFIZIKA I NOVEISHIE TEKHNOLOGII
布鲁克大学毕业证书 购买加拿大学历BU文凭学位证书成绩单
NWU毕业证书 西北大学学位证
Antimicrobial Agents and Chemotherapy
Amina Jindani
Systematic Reviews in Pharmacy
Hani Plumeriastuti
Cirujano General
Rolando García-matus
Aquaculture
Francisco Vargas-Albores
- We're Hiring!
- Help Center
- Find new research papers in:
- Health Sciences
- Earth Sciences
- Cognitive Science
- Mathematics
- Computer Science
- Academia ©2024
Thank you for visiting nature.com. You are using a browser version with limited support for CSS. To obtain the best experience, we recommend you use a more up to date browser (or turn off compatibility mode in Internet Explorer). In the meantime, to ensure continued support, we are displaying the site without styles and JavaScript.
- View all journals
- My Account Login
- Explore content
- About the journal
- Publish with us
- Sign up for alerts
- Open access
- Published: 17 October 2023
The impact of founder personalities on startup success
- Paul X. McCarthy 1 , 2 ,
- Xian Gong 3 ,
- Fabian Braesemann 4 , 5 ,
- Fabian Stephany 4 , 5 ,
- Marian-Andrei Rizoiu 3 &
- Margaret L. Kern 6
Scientific Reports volume 13 , Article number: 17200 ( 2023 ) Cite this article
60k Accesses
2 Citations
305 Altmetric
Metrics details
- Human behaviour
- Information technology
An Author Correction to this article was published on 07 May 2024
This article has been updated
Startup companies solve many of today’s most challenging problems, such as the decarbonisation of the economy or the development of novel life-saving vaccines. Startups are a vital source of innovation, yet the most innovative are also the least likely to survive. The probability of success of startups has been shown to relate to several firm-level factors such as industry, location and the economy of the day. Still, attention has increasingly considered internal factors relating to the firm’s founding team, including their previous experiences and failures, their centrality in a global network of other founders and investors, as well as the team’s size. The effects of founders’ personalities on the success of new ventures are, however, mainly unknown. Here, we show that founder personality traits are a significant feature of a firm’s ultimate success. We draw upon detailed data about the success of a large-scale global sample of startups (n = 21,187). We find that the Big Five personality traits of startup founders across 30 dimensions significantly differ from that of the population at large. Key personality facets that distinguish successful entrepreneurs include a preference for variety, novelty and starting new things (openness to adventure), like being the centre of attention (lower levels of modesty) and being exuberant (higher activity levels). We do not find one ’Founder-type’ personality; instead, six different personality types appear. Our results also demonstrate the benefits of larger, personality-diverse teams in startups, which show an increased likelihood of success. The findings emphasise the role of the diversity of personality types as a novel dimension of team diversity that influences performance and success.
Similar content being viewed by others
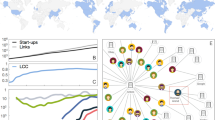
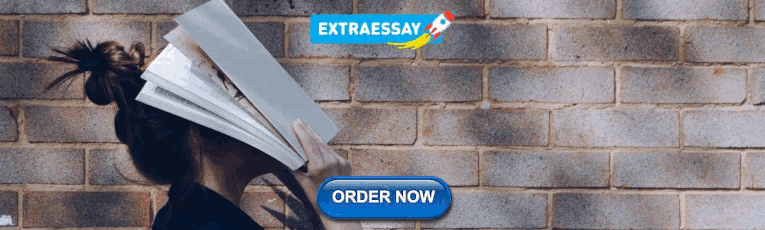
Predicting success in the worldwide start-up network
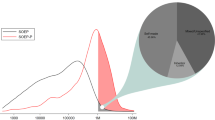
The personality traits of self-made and inherited millionaires
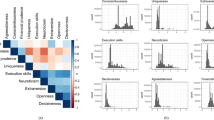
The nexus of top executives’ attributes, firm strategies, and outcomes: Large firms versus SMEs
Introduction.
The success of startups is vital to economic growth and renewal, with a small number of young, high-growth firms creating a disproportionately large share of all new jobs 1 , 2 . Startups create jobs and drive economic growth, and they are also an essential vehicle for solving some of society’s most pressing challenges.
As a poignant example, six centuries ago, the German city of Mainz was abuzz as the birthplace of the world’s first moveable-type press created by Johannes Gutenberg. However, in the early part of this century, it faced several economic challenges, including rising unemployment and a significant and growing municipal debt. Then in 2008, two Turkish immigrants formed the company BioNTech in Mainz with another university research colleague. Together they pioneered new mRNA-based technologies. In 2020, BioNTech partnered with US pharmaceutical giant Pfizer to create one of only a handful of vaccines worldwide for Covid-19, saving an estimated six million lives 3 . The economic benefit to Europe and, in particular, the German city where the vaccine was developed has been significant, with windfall tax receipts to the government clearing Mainz’s €1.3bn debt and enabling tax rates to be reduced, attracting other businesses to the region as well as inspiring a whole new generation of startups 4 .
While stories such as the success of BioNTech are often retold and remembered, their success is the exception rather than the rule. The overwhelming majority of startups ultimately fail. One study of 775 startups in Canada that successfully attracted external investment found only 35% were still operating seven years later 5 .
But what determines the success of these ‘lucky few’? When assessing the success factors of startups, especially in the early-stage unproven phase, venture capitalists and other investors offer valuable insights. Three different schools of thought characterise their perspectives: first, supply-side or product investors : those who prioritise investing in firms they consider to have novel and superior products and services, investing in companies with intellectual property such as patents and trademarks. Secondly, demand-side or market-based investors : those who prioritise investing in areas of highest market interest, such as in hot areas of technology like quantum computing or recurrent or emerging large-scale social and economic challenges such as the decarbonisation of the economy. Thirdly, talent investors : those who prioritise the foundation team above the startup’s initial products or what industry or problem it is looking to address.
Investors who adopt the third perspective and prioritise talent often recognise that a good team can overcome many challenges in the lead-up to product-market fit. And while the initial products of a startup may or may not work a successful and well-functioning team has the potential to pivot to new markets and new products, even if the initial ones prove untenable. Not surprisingly, an industry ‘autopsy’ into 101 tech startup failures found 23% were due to not having the right team—the number three cause of failure ahead of running out of cash or not having a product that meets the market need 6 .
Accordingly, early entrepreneurship research was focused on the personality of founders, but the focus shifted away in the mid-1980s onwards towards more environmental factors such as venture capital financing 7 , 8 , 9 , networks 10 , location 11 and due to a range of issues and challenges identified with the early entrepreneurship personality research 12 , 13 . At the turn of the 21st century, some scholars began exploring ways to combine context and personality and reconcile entrepreneurs’ individual traits with features of their environment. In her influential work ’The Sociology of Entrepreneurship’, Patricia H. Thornton 14 discusses two perspectives on entrepreneurship: the supply-side perspective (personality theory) and the demand-side perspective (environmental approach). The supply-side perspective focuses on the individual traits of entrepreneurs. In contrast, the demand-side perspective focuses on the context in which entrepreneurship occurs, with factors such as finance, industry and geography each playing their part. In the past two decades, there has been a revival of interest and research that explores how entrepreneurs’ personality relates to the success of their ventures. This new and growing body of research includes several reviews and meta-studies, which show that personality traits play an important role in both career success and entrepreneurship 15 , 16 , 17 , 18 , 19 , that there is heterogeneity in definitions and samples used in research on entrepreneurship 16 , 18 , and that founder personality plays an important role in overall startup outcomes 17 , 19 .
Motivated by the pivotal role of the personality of founders on startup success outlined in these recent contributions, we investigate two main research questions:
Which personality features characterise founders?
Do their personalities, particularly the diversity of personality types in founder teams, play a role in startup success?
We aim to understand whether certain founder personalities and their combinations relate to startup success, defined as whether their company has been acquired, acquired another company or listed on a public stock exchange. For the quantitative analysis, we draw on a previously published methodology 20 , which matches people to their ‘ideal’ jobs based on social media-inferred personality traits.
We find that personality traits matter for startup success. In addition to firm-level factors of location, industry and company age, we show that founders’ specific Big Five personality traits, such as adventurousness and openness, are significantly more widespread among successful startups. As we find that companies with multi-founder teams are more likely to succeed, we cluster founders in six different and distinct personality groups to underline the relevance of the complementarity in personality traits among founder teams. Startups with diverse and specific combinations of founder types (e. g., an adventurous ‘Leader’, a conscientious ‘Accomplisher’, and an extroverted ‘Developer’) have significantly higher odds of success.
We organise the rest of this paper as follows. In the Section " Results ", we introduce the data used and the methods applied to relate founders’ psychological traits with their startups’ success. We introduce the natural language processing method to derive individual and team personality characteristics and the clustering technique to identify personality groups. Then, we present the result for multi-variate regression analysis that allows us to relate firm success with external and personality features. Subsequently, the Section " Discussion " mentions limitations and opportunities for future research in this domain. In the Section " Methods ", we describe the data, the variables in use, and the clustering in greater detail. Robustness checks and additional analyses can be found in the Supplementary Information.
Our analysis relies on two datasets. We infer individual personality facets via a previously published methodology 20 from Twitter user profiles. Here, we restrict our analysis to founders with a Crunchbase profile. Crunchbase is the world’s largest directory on startups. It provides information about more than one million companies, primarily focused on funding and investors. A company’s public Crunchbase profile can be considered a digital business card of an early-stage venture. As such, the founding teams tend to provide information about themselves, including their educational background or a link to their Twitter account.
We infer the personality profiles of the founding teams of early-stage ventures from their publicly available Twitter profiles, using the methodology described by Kern et al. 20 . Then, we correlate this information to data from Crunchbase to determine whether particular combinations of personality traits correspond to the success of early-stage ventures. The final dataset used in the success prediction model contains n = 21,187 startup companies (for more details on the data see the Methods section and SI section A.5 ).
Revisions of Crunchbase as a data source for investigations on a firm and industry level confirm the platform to be a useful and valuable source of data for startups research, as comparisons with other sources at micro-level, e.g., VentureXpert or PwC, also suggest that the platform’s coverage is very comprehensive, especially for start-ups located in the United States 21 . Moreover, aggregate statistics on funding rounds by country and year are quite similar to those produced with other established sources, going to validate the use of Crunchbase as a reliable source in terms of coverage of funded ventures. For instance, Crunchbase covers about the same number of investment rounds in the analogous sectors as collected by the National Venture Capital Association 22 . However, we acknowledge that the data source might suffer from registration latency (a certain delay between the foundation of the company and its actual registration on Crunchbase) and success bias in company status (the likeliness that failed companies decide to delete their profile from the database).
The definition of startup success
The success of startups is uncertain, dependent on many factors and can be measured in various ways. Due to the likelihood of failure in startups, some large-scale studies have looked at which features predict startup survival rates 23 , and others focus on fundraising from external investors at various stages 24 . Success for startups can be measured in multiple ways, such as the amount of external investment attracted, the number of new products shipped or the annual growth in revenue. But sometimes external investments are misguided, revenue growth can be short-lived, and new products may fail to find traction.
Success in a startup is typically staged and can appear in different forms and times. For example, a startup may be seen to be successful when it finds a clear solution to a widely recognised problem, such as developing a successful vaccine. On the other hand, it could be achieving some measure of commercial success, such as rapidly accelerating sales or becoming profitable or at least cash positive. Or it could be reaching an exit for foundation investors via a trade sale, acquisition or listing of its shares for sale on a public stock exchange via an Initial Public Offering (IPO).
For our study, we focused on the startup’s extrinsic success rather than the founders’ intrinsic success per se, as its more visible, objective and measurable. A frequently considered measure of success is the attraction of external investment by venture capitalists 25 . However, this is not in and of itself a good measure of clear, incontrovertible success, particularly for early-stage ventures. This is because it reflects investors’ expectations of a startup’s success potential rather than actual business success. Similarly, we considered other measures like revenue growth 26 , liquidity events 27 , 28 , 29 , profitability 30 and social impact 31 , all of which have benefits as they capture incremental success, but each also comes with operational measurement challenges.
Therefore, we apply the success definition initially introduced by Bonaventura et al. 32 , namely that a startup is acquired, acquires another company or has an initial public offering (IPO). We consider any of these major capital liquidation events as a clear threshold signal that the company has matured from an early-stage venture to becoming or is on its way to becoming a mature company with clear and often significant business growth prospects. Together these three major liquidity events capture the primary forms of exit for external investors (an acquisition or trade sale and an IPO). For companies with a longer autonomous growth runway, acquiring another company marks a similar milestone of scale, maturity and capability.
Using multifactor analysis and a binary classification prediction model of startup success, we looked at many variables together and their relative influence on the probability of the success of startups. We looked at seven categories of factors through three lenses of firm-level factors: (1) location, (2) industry, (3) age of the startup; founder-level factors: (4) number of founders, (5) gender of founders, (6) personality characteristics of founders and; lastly team-level factors: (7) founder-team personality combinations. The model performance and relative impacts on the probability of startup success of each of these categories of founders are illustrated in more detail in section A.6 of the Supplementary Information (in particular Extended Data Fig. 19 and Extended Data Fig. 20 ). In total, we considered over three hundred variables (n = 323) and their relative significant associations with success.
The personality of founders
Besides product-market, industry, and firm-level factors (see SI section A.1 ), research suggests that the personalities of founders play a crucial role in startup success 19 . Therefore, we examine the personality characteristics of individual startup founders and teams of founders in relationship to their firm’s success by applying the success definition used by Bonaventura et al. 32 .
Employing established methods 33 , 34 , 35 , we inferred the personality traits across 30 dimensions (Big Five facets) of a large global sample of startup founders. The startup founders cohort was created from a subset of founders from the global startup industry directory Crunchbase, who are also active on the social media platform Twitter.
To measure the personality of the founders, we used the Big Five, a popular model of personality which includes five core traits: Openness to Experience, Conscientiousness, Extraversion, Agreeableness, and Emotional stability. Each of these traits can be further broken down into thirty distinct facets. Studies have found that the Big Five predict meaningful life outcomes, such as physical and mental health, longevity, social relationships, health-related behaviours, antisocial behaviour, and social contribution, at levels on par with intelligence and socioeconomic status 36 Using machine learning to infer personality traits by analysing the use of language and activity on social media has been shown to be more accurate than predictions of coworkers, friends and family and similar in accuracy to the judgement of spouses 37 . Further, as other research has shown, we assume that personality traits remain stable in adulthood even through significant life events 38 , 39 , 40 . Personality traits have been shown to emerge continuously from those already evident in adolescence 41 and are not significantly influenced by external life events such as becoming divorced or unemployed 42 . This suggests that the direction of any measurable effect goes from founder personalities to startup success and not vice versa.
As a first investigation to what extent personality traits might relate to entrepreneurship, we use the personality characteristics of individuals to predict whether they were an entrepreneur or an employee. We trained and tested a machine-learning random forest classifier to distinguish and classify entrepreneurs from employees and vice-versa using inferred personality vectors alone. As a result, we found we could correctly predict entrepreneurs with 77% accuracy and employees with 88% accuracy (Fig. 1 A). Thus, based on personality information alone, we correctly predict all unseen new samples with 82.5% accuracy (See SI section A.2 for more details on this analysis, the classification modelling and prediction accuracy).
We explored in greater detail which personality features are most prominent among entrepreneurs. We found that the subdomain or facet of Adventurousness within the Big Five Domain of Openness was significant and had the largest effect size. The facet of Modesty within the Big Five Domain of Agreeableness and Activity Level within the Big Five Domain of Extraversion was the subsequent most considerable effect (Fig. 1 B). Adventurousness in the Big Five framework is defined as the preference for variety, novelty and starting new things—which are consistent with the role of a startup founder whose role, especially in the early life of the company, is to explore things that do not scale easily 43 and is about developing and testing new products, services and business models with the market.
Once we derived and tested the Big Five personality features for each entrepreneur in our data set, we examined whether there is evidence indicating that startup founders naturally cluster according to their personality features using a Hopkins test (see Extended Data Figure 6 ). We discovered clear clustering tendencies in the data compared with other renowned reference data sets known to have clusters. Then, once we established the founder data clusters, we used agglomerative hierarchical clustering. This ‘bottom-up’ clustering technique initially treats each observation as an individual cluster. Then it merges them to create a hierarchy of possible cluster schemes with differing numbers of groups (See Extended Data Fig. 7 ). And lastly, we identified the optimum number of clusters based on the outcome of four different clustering performance measurements: Davies-Bouldin Index, Silhouette coefficients, Calinski-Harabas Index and Dunn Index (see Extended Data Figure 8 ). We find that the optimum number of clusters of startup founders based on their personality features is six (labelled #0 through to #5), as shown in Fig. 1 C.
To better understand the context of different founder types, we positioned each of the six types of founders within an occupation-personality matrix established from previous research 44 . This research showed that ‘each job has its own personality’ using a substantial sample of employees across various jobs. Utilising the methodology employed in this study, we assigned labels to the cluster names #0 to #5, which correspond to the identified occupation tribes that best describe the personality facets represented by the clusters (see Extended Data Fig. 9 for an overview of these tribes, as identified by McCarthy et al. 44 ).
Utilising this approach, we identify three ’purebred’ clusters: #0, #2 and #5, whose members are dominated by a single tribe (larger than 60% of all individuals in each cluster are characterised by one tribe). Thus, these clusters represent and share personality attributes of these previously identified occupation-personality tribes 44 , which have the following known distinctive personality attributes (see also Table 1 ):
Accomplishers (#0) —Organised & outgoing. confident, down-to-earth, content, accommodating, mild-tempered & self-assured.
Leaders (#2) —Adventurous, persistent, dispassionate, assertive, self-controlled, calm under pressure, philosophical, excitement-seeking & confident.
Fighters (#5) —Spontaneous and impulsive, tough, sceptical, and uncompromising.
We labelled these clusters with the tribe names, acknowledging that labels are somewhat arbitrary, based on our best interpretation of the data (See SI section A.3 for more details).
For the remaining three clusters #1, #3 and #4, we can see they are ‘hybrids’, meaning that the founders within them come from a mix of different tribes, with no one tribe representing more than 50% of the members of that cluster. However, the tribes with the largest share were noted as #1 Experts/Engineers, #3 Fighters, and #4 Operators.
To label these three hybrid clusters, we examined the closest occupations to the median personality features of each cluster. We selected a name that reflected the common themes of these occupations, namely:
Experts/Engineers (#1) as the closest roles included Materials Engineers and Chemical Engineers. This is consistent with this cluster’s personality footprint, which is highest in openness in the facets of imagination and intellect.
Developers (#3) as the closest roles include Application Developers and related technology roles such as Business Systems Analysts and Product Managers.
Operators (#4) as the closest roles include service, maintenance and operations functions, including Bicycle Mechanic, Mechanic and Service Manager. This is also consistent with one of the key personality traits of high conscientiousness in the facet of orderliness and high agreeableness in the facet of humility for founders in this cluster.
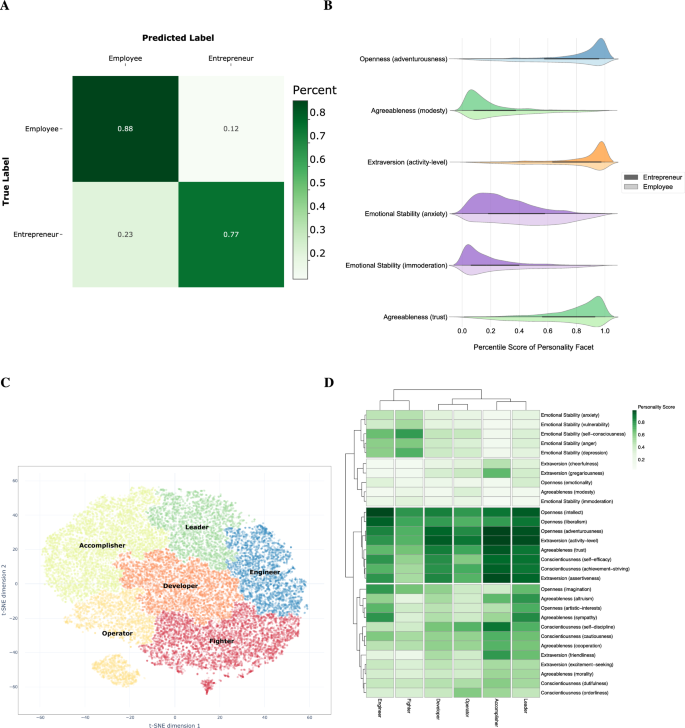
Founder-Level Factors of Startup Success. ( A ), Successful entrepreneurs differ from successful employees. They can be accurately distinguished using a classifier with personality information alone. ( B ), Successful entrepreneurs have different Big Five facet distributions, especially on adventurousness, modesty and activity level. ( C ), Founders come in six different types: Fighters, Operators, Accomplishers, Leaders, Engineers and Developers (FOALED) ( D ), Each founder Personality-Type has its distinct facet.
Together, these six different types of startup founders (Fig. 1 C) represent a framework we call the FOALED model of founder types—an acronym of Fighters, Operators, Accomplishers, Leaders, Engineers and D evelopers.
Each founder’s personality type has its distinct facet footprint (for more details, see Extended Data Figure 10 in SI section A.3 ). Also, we observe a central core of correlated features that are high for all types of entrepreneurs, including intellect, adventurousness and activity level (Fig. 1 D).To test the robustness of the clustering of the personality facets, we compare the mean scores of the individual facets per cluster with a 20-fold resampling of the data and find that the clusters are, overall, largely robust against resampling (see Extended Data Figure 11 in SI section A.3 for more details).
We also find that the clusters accord with the distribution of founders’ roles in their startups. For example, Accomplishers are often Chief Executive Officers, Chief Financial Officers, or Chief Operating Officers, while Fighters tend to be Chief Technical Officers, Chief Product Officers, or Chief Commercial Officers (see Extended Data Fig. 12 in SI section A.4 for more details).
The ensemble theory of success
While founders’ individual personality traits, such as Adventurousness or Openness, show to be related to their firms’ success, we also hypothesise that the combination, or ensemble, of personality characteristics of a founding team impacts the chances of success. The logic behind this reasoning is complementarity, which is proposed by contemporary research on the functional roles of founder teams. Examples of these clear functional roles have evolved in established industries such as film and television, construction, and advertising 45 . When we subsequently explored the combinations of personality types among founders and their relationship to the probability of startup success, adjusted for a range of other factors in a multi-factorial analysis, we found significantly increased chances of success for mixed foundation teams:
Initially, we find that firms with multiple founders are more likely to succeed, as illustrated in Fig. 2 A, which shows firms with three or more founders are more than twice as likely to succeed than solo-founded startups. This finding is consistent with investors’ advice to founders and previous studies 46 . We also noted that some personality types of founders increase the probability of success more than others, as shown in SI section A.6 (Extended Data Figures 16 and 17 ). Also, we note that gender differences play out in the distribution of personality facets: successful female founders and successful male founders show facet scores that are more similar to each other than are non-successful female founders to non-successful male founders (see Extended Data Figure 18 ).
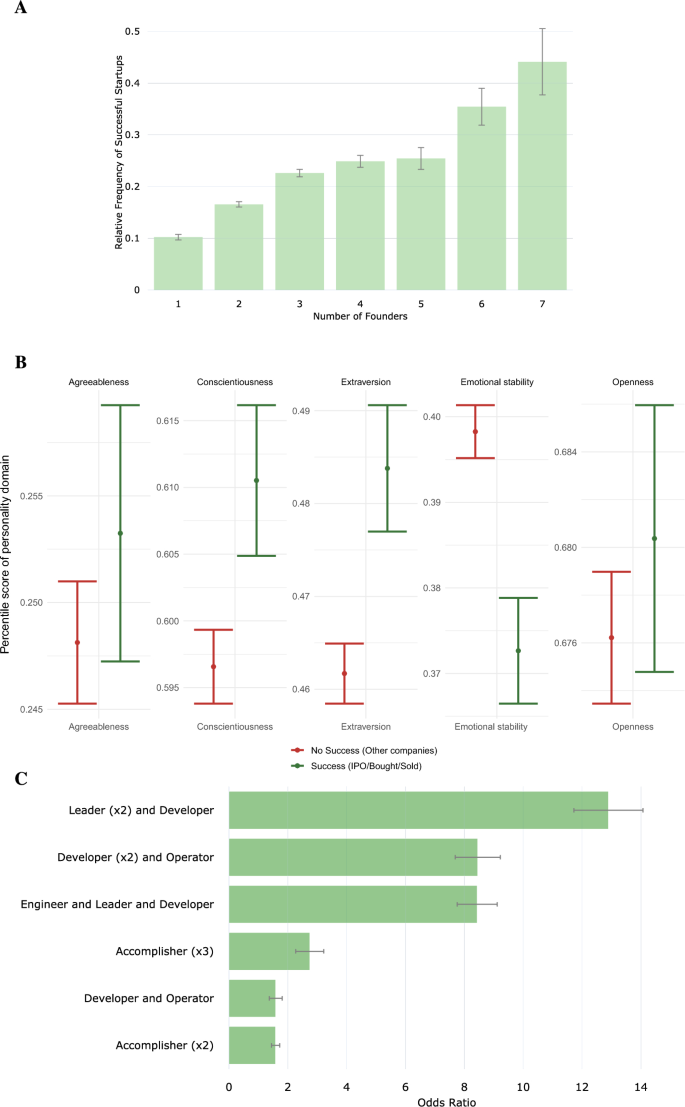
The Ensemble Theory of Team-Level Factors of Startup Success. ( A ) Having a larger founder team elevates the chances of success. This can be due to multiple reasons, e.g., a more extensive network or knowledge base but also personality diversity. ( B ) We show that joint personality combinations of founders are significantly related to higher chances of success. This is because it takes more than one founder to cover all beneficial personality traits that ‘breed’ success. ( C ) In our multifactor model, we show that firms with diverse and specific combinations of types of founders have significantly higher odds of success.
Access to more extensive networks and capital could explain the benefits of having more founders. Still, as we find here, it also offers a greater diversity of combined personalities, naturally providing a broader range of maximum traits. So, for example, one founder may be more open and adventurous, and another could be highly agreeable and trustworthy, thus, potentially complementing each other’s particular strengths associated with startup success.
The benefits of larger and more personality-diverse foundation teams can be seen in the apparent differences between successful and unsuccessful firms based on their combined Big Five personality team footprints, as illustrated in Fig. 2 B. Here, maximum values for each Big Five trait of a startup’s co-founders are mapped; stratified by successful and non-successful companies. Founder teams of successful startups tend to score higher on Openness, Conscientiousness, Extraversion, and Agreeableness.
When examining the combinations of founders with different personality types, we find that some ensembles of personalities were significantly correlated with greater chances of startup success—while controlling for other variables in the model—as shown in Fig. 2 C (for more details on the modelling, the predictive performance and the coefficient estimates of the final model, see Extended Data Figures 19 , 20 , and 21 in SI section A.6 ).
Three combinations of trio-founder companies were more than twice as likely to succeed than other combinations, namely teams with (1) a Leader and two Developers , (2) an Operator and two Developers , and (3) an Expert/Engineer , Leader and Developer . To illustrate the potential mechanisms on how personality traits might influence the success of startups, we provide some examples of well-known, successful startup founders and their characteristic personality traits in Extended Data Figure 22 .
Startups are one of the key mechanisms for brilliant ideas to become solutions to some of the world’s most challenging economic and social problems. Examples include the Google search algorithm, disability technology startup Fingerwork’s touchscreen technology that became the basis of the Apple iPhone, or the Biontech mRNA technology that powered Pfizer’s COVID-19 vaccine.
We have shown that founders’ personalities and the combination of personalities in the founding team of a startup have a material and significant impact on its likelihood of success. We have also shown that successful startup founders’ personality traits are significantly different from those of successful employees—so much so that a simple predictor can be trained to distinguish between employees and entrepreneurs with more than 80% accuracy using personality trait data alone.
Just as occupation-personality maps derived from data can provide career guidance tools, so too can data on successful entrepreneurs’ personality traits help people decide whether becoming a founder may be a good choice for them.
We have learnt through this research that there is not one type of ideal ’entrepreneurial’ personality but six different types. Many successful startups have multiple co-founders with a combination of these different personality types.
To a large extent, founding a startup is a team sport; therefore, diversity and complementarity of personalities matter in the foundation team. It has an outsized impact on the company’s likelihood of success. While all startups are high risk, the risk becomes lower with more founders, particularly if they have distinct personality traits.
Our work demonstrates the benefits of personality diversity among the founding team of startups. Greater awareness of this novel form of diversity may help create more resilient startups capable of more significant innovation and impact.
The data-driven research approach presented here comes with certain methodological limitations. The principal data sources of this study—Crunchbase and Twitter—are extensive and comprehensive, but there are characterised by some known and likely sample biases.
Crunchbase is the principal public chronicle of venture capital funding. So, there is some likely sample bias toward: (1) Startup companies that are funded externally: self-funded or bootstrapped companies are less likely to be represented in Crunchbase; (2) technology companies, as that is Crunchbase’s roots; (3) multi-founder companies; (4) male founders: while the representation of female founders is now double that of the mid-2000s, women still represent less than 25% of the sample; (5) companies that succeed: companies that fail, especially those that fail early, are likely to be less represented in the data.
Samples were also limited to those founders who are active on Twitter, which adds additional selection biases. For example, Twitter users typically are younger, more educated and have a higher median income 47 . Another limitation of our approach is the potentially biased presentation of a person’s digital identity on social media, which is the basis for identifying personality traits. For example, recent research suggests that the language and emotional tone used by entrepreneurs in social media can be affected by events such as business failure 48 , which might complicate the personality trait inference.
In addition to sampling biases within the data, there are also significant historical biases in startup culture. For many aspects of the entrepreneurship ecosystem, women, for example, are at a disadvantage 49 . Male-founded companies have historically dominated most startup ecosystems worldwide, representing the majority of founders and the overwhelming majority of venture capital investors. As a result, startups with women have historically attracted significantly fewer funds 50 , in part due to the male bias among venture investors, although this is now changing, albeit slowly 51 .
The research presented here provides quantitative evidence for the relevance of personality types and the diversity of personalities in startups. At the same time, it brings up other questions on how personality traits are related to other factors associated with success, such as:
Will the recent growing focus on promoting and investing in female founders change the nature, composition and dynamics of startups and their personalities leading to a more diverse personality landscape in startups?
Will the growth of startups outside of the United States change what success looks like to investors and hence the role of different personality traits and their association to diverse success metrics?
Many of today’s most renowned entrepreneurs are either Baby Boomers (such as Gates, Branson, Bloomberg) or Generation Xers (such as Benioff, Cannon-Brookes, Musk). However, as we can see, personality is both a predictor and driver of success in entrepreneurship. Will generation-wide differences in personality and outlook affect startups and their success?
Moreover, the findings shown here have natural extensions and applications beyond startups, such as for new projects within large established companies. While not technically startups, many large enterprises and industries such as construction, engineering and the film industry rely on forming new project-based, cross-functional teams that are often new ventures and share many characteristics of startups.
There is also potential for extending this research in other settings in government, NGOs, and within the research community. In scientific research, for example, team diversity in terms of age, ethnicity and gender has been shown to be predictive of impact, and personality diversity may be another critical dimension 52 .
Another extension of the study could investigate the development of the language used by startup founders on social media over time. Such an extension could investigate whether the language (and inferred psychological characteristics) change as the entrepreneurs’ ventures go through major business events such as foundation, funding, or exit.
Overall, this study demonstrates, first, that startup founders have significantly different personalities than employees. Secondly, besides firm-level factors, which are known to influence firm success, we show that a range of founder-level factors, notably the character traits of its founders, significantly impact a startup’s likelihood of success. Lastly, we looked at team-level factors. We discovered in a multifactor analysis that personality-diverse teams have the most considerable impact on the probability of a startup’s success, underlining the importance of personality diversity as a relevant factor of team performance and success.
Data sources
Entrepreneurs dataset.
Data about the founders of startups were collected from Crunchbase (Table 2 ), an open reference platform for business information about private and public companies, primarily early-stage startups. It is one of the largest and most comprehensive data sets of its kind and has been used in over 100 peer-reviewed research articles about economic and managerial research.
Crunchbase contains data on over two million companies - mainly startup companies and the companies who partner with them, acquire them and invest in them, as well as profiles on well over one million individuals active in the entrepreneurial ecosystem worldwide from over 200 countries and spans. Crunchbase started in the technology startup space, and it now covers all sectors, specifically focusing on entrepreneurship, investment and high-growth companies.
While Crunchbase contains data on over one million individuals in the entrepreneurial ecosystem, some are not entrepreneurs or startup founders but play other roles, such as investors, lawyers or executives at companies that acquire startups. To create a subset of only entrepreneurs, we selected a subset of 32,732 who self-identify as founders and co-founders (by job title) and who are also publicly active on the social media platform Twitter. We also removed those who also are venture capitalists to distinguish between investors and founders.
We selected founders active on Twitter to be able to use natural language processing to infer their Big Five personality features using an open-vocabulary approach shown to be accurate in the previous research by analysing users’ unstructured text, such as Twitter posts in our case. For this project, as with previous research 20 , we employed a commercial service, IBM Watson Personality Insight, to infer personality facets. This service provides raw scores and percentile scores of Big Five Domains (Openness, Conscientiousness, Extraversion, Agreeableness and Emotional Stability) and the corresponding 30 subdomains or facets. In addition, the public content of Twitter posts was collected, and there are 32,732 profiles that each had enough Twitter posts (more than 150 words) to get relatively accurate personality scores (less than 12.7% Average Mean Absolute Error).
The entrepreneurs’ dataset is analysed in combination with other data about the companies they founded to explore questions about the nature and patterns of personality traits of entrepreneurs and the relationships between these patterns and company success.
For the multifactor analysis, we further filtered the data in several preparatory steps for the success prediction modelling (for more details, see SI section A.5 ). In particular, we removed data points with missing values (Extended Data Fig. 13 ) and kept only companies in the data that were founded from 1990 onward to ensure consistency with previous research 32 (see Extended Data Fig. 14 ). After cleaning, filtering and pre-processing the data, we ended up with data from 25,214 founders who founded 21,187 startup companies to be used in the multifactor analysis. Of those, 3442 startups in the data were successful, 2362 in the first seven years after they were founded (see Extended Data Figure 15 for more details).
Entrepreneurs and employees dataset
To investigate whether startup founders show personality traits that are similar or different from the population at large (i. e. the entrepreneurs vs employees sub-analysis shown in Fig. 1 A and B), we filtered the entrepreneurs’ data further: we reduced the sample to those founders of companies, which attracted more than US$100k in investment to create a reference set of successful entrepreneurs (n \(=\) 4400).
To create a control group of employees who are not also entrepreneurs or very unlikely to be of have been entrepreneurs, we leveraged the fact that while some occupational titles like CEO, CTO and Public Speaker are commonly shared by founders and co-founders, some others such as Cashier , Zoologist and Detective very rarely co-occur seem to be founders or co-founders. To illustrate, many company founders also adopt regular occupation titles such as CEO or CTO. Many founders will be Founder and CEO or Co-founder and CTO. While founders are often CEOs or CTOs, the reverse is not necessarily true, as many CEOs are professional executives that were not involved in the establishment or ownership of the firm.
Using data from LinkedIn, we created an Entrepreneurial Occupation Index (EOI) based on the ratio of entrepreneurs for each of the 624 occupations used in a previous study of occupation-personality fit 44 . It was calculated based on the percentage of all people working in the occupation from LinkedIn compared to those who shared the title Founder or Co-founder (See SI section A.2 for more details). A reference set of employees (n=6685) was then selected across the 112 different occupations with the lowest propensity for entrepreneurship (less than 0.5% EOI) from a large corpus of Twitter users with known occupations, which is also drawn from the previous occupational-personality fit study 44 .
These two data sets were used to test whether it may be possible to distinguish successful entrepreneurs from successful employees based on the different patterns of personality traits alone.
Hierarchical clustering
We applied several clustering techniques and tests to the personality vectors of the entrepreneurs’ data set to determine if there are natural clusters and, if so, how many are the optimum number.
Firstly, to determine if there is a natural typology to founder personalities, we applied the Hopkins statistic—a statistical test we used to answer whether the entrepreneurs’ dataset contains inherent clusters. It measures the clustering tendency based on the ratio of the sum of distances of real points within a sample of the entrepreneurs’ dataset to their nearest neighbours and the sum of distances of randomly selected artificial points from a simulated uniform distribution to their nearest neighbours in the real entrepreneurs’ dataset. The ratio measures the difference between the entrepreneurs’ data distribution and the simulated uniform distribution, which tests the randomness of the data. The range of Hopkins statistics is from 0 to 1. The scores are close to 0, 0.5 and 1, respectively, indicating whether the dataset is uniformly distributed, randomly distributed or highly clustered.
To cluster the founders by personality facets, we used Agglomerative Hierarchical Clustering (AHC)—a bottom-up approach that treats an individual data point as a singleton cluster and then iteratively merges pairs of clusters until all data points are included in the single big collection. Ward’s linkage method is used to choose the pair of groups for minimising the increase in the within-cluster variance after combining. AHC was widely applied to clustering analysis since a tree hierarchy output is more informative and interpretable than K-means. Dendrograms were used to visualise the hierarchy to provide the perspective of the optimal number of clusters. The heights of the dendrogram represent the distance between groups, with lower heights representing more similar groups of observations. A horizontal line through the dendrogram was drawn to distinguish the number of significantly different clusters with higher heights. However, as it is not possible to determine the optimum number of clusters from the dendrogram, we applied other clustering performance metrics to analyse the optimal number of groups.
A range of Clustering performance metrics were used to help determine the optimal number of clusters in the dataset after an apparent clustering tendency was confirmed. The following metrics were implemented to evaluate the differences between within-cluster and between-cluster distances comprehensively: Dunn Index, Calinski-Harabasz Index, Davies-Bouldin Index and Silhouette Index. The Dunn Index measures the ratio of the minimum inter-cluster separation and the maximum intra-cluster diameter. At the same time, the Calinski-Harabasz Index improves the measurement of the Dunn Index by calculating the ratio of the average sum of squared dispersion of inter-cluster and intra-cluster. The Davies-Bouldin Index simplifies the process by treating each cluster individually. It compares the sum of the average distance among intra-cluster data points to the cluster centre of two separate groups with the distance between their centre points. Finally, the Silhouette Index is the overall average of the silhouette coefficients for each sample. The coefficient measures the similarity of the data point to its cluster compared with the other groups. Higher scores of the Dunn, Calinski-Harabasz and Silhouette Index and a lower score of the Davies-Bouldin Index indicate better clustering configuration.
Classification modelling
Classification algorithms.
To obtain a comprehensive and robust conclusion in the analysis predicting whether a given set of personality traits corresponds to an entrepreneur or an employee, we explored the following classifiers: Naïve Bayes, Elastic Net regularisation, Support Vector Machine, Random Forest, Gradient Boosting and Stacked Ensemble. The Naïve Bayes classifier is a probabilistic algorithm based on Bayes’ theorem with assumptions of independent features and equiprobable classes. Compared with other more complex classifiers, it saves computing time for large datasets and performs better if the assumptions hold. However, in the real world, those assumptions are generally violated. Elastic Net regularisation combines the penalties of Lasso and Ridge to regularise the Logistic classifier. It eliminates the limitation of multicollinearity in the Lasso method and improves the limitation of feature selection in the Ridge method. Even though Elastic Net is as simple as the Naïve Bayes classifier, it is more time-consuming. The Support Vector Machine (SVM) aims to find the ideal line or hyperplane to separate successful entrepreneurs and employees in this study. The dividing line can be non-linear based on a non-linear kernel, such as the Radial Basis Function Kernel. Therefore, it performs well on high-dimensional data while the ’right’ kernel selection needs to be tuned. Random Forest (RF) and Gradient Boosting Trees (GBT) are ensembles of decision trees. All trees are trained independently and simultaneously in RF, while a new tree is trained each time and corrected by previously trained trees in GBT. RF is a more robust and straightforward model since it does not have many hyperparameters to tune. GBT optimises the objective function and learns a more accurate model since there is a successive learning and correction process. Stacked Ensemble combines all existing classifiers through a Logistic Regression. Better than bagging with only variance reduction and boosting with only bias reduction, the ensemble leverages the benefit of model diversity with both lower variance and bias. All the above classification algorithms distinguish successful entrepreneurs and employees based on the personality matrix.
Evaluation metrics
A range of evaluation metrics comprehensively explains the performance of a classification prediction. The most straightforward metric is accuracy, which measures the overall portion of correct predictions. It will mislead the performance of an imbalanced dataset. The F1 score is better than accuracy by combining precision and recall and considering the False Negatives and False Positives. Specificity measures the proportion of detecting the true negative rate that correctly identifies employees, while Positive Predictive Value (PPV) calculates the probability of accurately predicting successful entrepreneurs. Area Under the Receiver Operating Characteristic Curve (AUROC) determines the capability of the algorithm to distinguish between successful entrepreneurs and employees. A higher value means the classifier performs better on separating the classes.
Feature importance
To further understand and interpret the classifier, it is critical to identify variables with significant predictive power on the target. Feature importance of tree-based models measures Gini importance scores for all predictors, which evaluate the overall impact of the model after cutting off the specific feature. The measurements consider all interactions among features. However, it does not provide insights into the directions of impacts since the importance only indicates the ability to distinguish different classes.
Statistical analysis
T-test, Cohen’s D and two-sample Kolmogorov-Smirnov test are introduced to explore how the mean values and distributions of personality facets between entrepreneurs and employees differ. The T-test is applied to determine whether the mean of personality facets of two group samples are significantly different from one another or not. The facets with significant differences detected by the hypothesis testing are critical to separate the two groups. Cohen’s d is to measure the effect size of the results of the previous t-test, which is the ratio of the mean difference to the pooled standard deviation. A larger Cohen’s d score indicates that the mean difference is greater than the variability of the whole sample. Moreover, it is interesting to check whether the two groups’ personality facets’ probability distributions are from the same distribution through the two-sample Kolmogorov-Smirnov test. There is no assumption about the distributions, but the test is sensitive to deviations near the centre rather than the tail.
Privacy and ethics
The focus of this research is to provide high-level insights about groups of startups, founders and types of founder teams rather than on specific individuals or companies. While we used unit record data from the publicly available data of company profiles from Crunchbase , we removed all identifiers from the underlying data on individual companies and founders and generated aggregate results, which formed the basis for our analysis and conclusions.
Data availability
A dataset which includes only aggregated statistics about the success of startups and the factors that influence is released as part of this research. Underlying data for all figures and the code to reproduce them are available on GitHub: https://github.com/Braesemann/FounderPersonalities . Please contact Fabian Braesemann ( [email protected] ) in case you have any further questions.
Change history
07 may 2024.
A Correction to this paper has been published: https://doi.org/10.1038/s41598-024-61082-7
Henrekson, M. & Johansson, D. Gazelles as job creators: A survey and interpretation of the evidence. Small Bus. Econ. 35 , 227–244 (2010).
Article Google Scholar
Davila, A., Foster, G., He, X. & Shimizu, C. The rise and fall of startups: Creation and destruction of revenue and jobs by young companies. Aust. J. Manag. 40 , 6–35 (2015).
Which vaccine saved the most lives in 2021?: Covid-19. The Economist (Online) (2022). noteName - AstraZeneca; Pfizer Inc; BioNTech SE; Copyright - Copyright The Economist Newspaper NA, Inc. Jul 14, 2022; Last updated - 2022-11-29.
Oltermann, P. Pfizer/biontech tax windfall brings mainz an early christmas present (2021). noteName - Pfizer Inc; BioNTech SE; Copyright - Copyright Guardian News & Media Limited Dec 27, 2021; Last updated - 2021-12-28.
Grant, K. A., Croteau, M. & Aziz, O. The survival rate of startups funded by angel investors. I-INC WHITE PAPER SER.: MAR 2019 , 1–21 (2019).
Google Scholar
Top 20 reasons start-ups fail - cb insights version (2019). noteCopyright - Copyright Newstex Oct 21, 2019; Last updated - 2022-10-25.
Hochberg, Y. V., Ljungqvist, A. & Lu, Y. Whom you know matters: Venture capital networks and investment performance. J. Financ. 62 , 251–301 (2007).
Fracassi, C., Garmaise, M. J., Kogan, S. & Natividad, G. Business microloans for us subprime borrowers. J. Financ. Quantitative Ana. 51 , 55–83 (2016).
Davila, A., Foster, G. & Gupta, M. Venture capital financing and the growth of startup firms. J. Bus. Ventur. 18 , 689–708 (2003).
Nann, S. et al. Comparing the structure of virtual entrepreneur networks with business effectiveness. Proc. Soc. Behav. Sci. 2 , 6483–6496 (2010).
Guzman, J. & Stern, S. Where is silicon valley?. Science 347 , 606–609 (2015).
Article ADS CAS PubMed Google Scholar
Aldrich, H. E. & Wiedenmayer, G. From traits to rates: An ecological perspective on organizational foundings. 61–97 (2019).
Gartner, W. B. Who is an entrepreneur? is the wrong question. Am. J. Small Bus. 12 , 11–32 (1988).
Thornton, P. H. The sociology of entrepreneurship. Ann. Rev. Sociol. 25 , 19–46 (1999).
Eikelboom, M. E., Gelderman, C. & Semeijn, J. Sustainable innovation in public procurement: The decisive role of the individual. J. Public Procure. 18 , 190–201 (2018).
Kerr, S. P. et al. Personality traits of entrepreneurs: A review of recent literature. Found. Trends Entrep. 14 , 279–356 (2018).
Hamilton, B. H., Papageorge, N. W. & Pande, N. The right stuff? Personality and entrepreneurship. Quant. Econ. 10 , 643–691 (2019).
Salmony, F. U. & Kanbach, D. K. Personality trait differences across types of entrepreneurs: A systematic literature review. RMS 16 , 713–749 (2022).
Freiberg, B. & Matz, S. C. Founder personality and entrepreneurial outcomes: A large-scale field study of technology startups. Proc. Natl. Acad. Sci. 120 , e2215829120 (2023).
Article CAS PubMed PubMed Central Google Scholar
Kern, M. L., McCarthy, P. X., Chakrabarty, D. & Rizoiu, M.-A. Social media-predicted personality traits and values can help match people to their ideal jobs. Proc. Natl. Acad. Sci. 116 , 26459–26464 (2019).
Article ADS CAS PubMed PubMed Central Google Scholar
Dalle, J.-M., Den Besten, M. & Menon, C. Using crunchbase for economic and managerial research. (2017).
Block, J. & Sandner, P. What is the effect of the financial crisis on venture capital financing? Empirical evidence from us internet start-ups. Ventur. Cap. 11 , 295–309 (2009).
Antretter, T., Blohm, I. & Grichnik, D. Predicting startup survival from digital traces: Towards a procedure for early stage investors (2018).
Dworak, D. Analysis of founder background as a predictor for start-up success in achieving successive fundraising rounds. (2022).
Hsu, D. H. Venture capitalists and cooperative start-up commercialization strategy. Manage. Sci. 52 , 204–219 (2006).
Blank, S. Why the lean start-up changes everything (2018).
Kaplan, S. N. & Lerner, J. It ain’t broke: The past, present, and future of venture capital. J. Appl. Corp. Financ. 22 , 36–47 (2010).
Hallen, B. L. & Eisenhardt, K. M. Catalyzing strategies and efficient tie formation: How entrepreneurial firms obtain investment ties. Acad. Manag. J. 55 , 35–70 (2012).
Gompers, P. A. & Lerner, J. The Venture Capital Cycle (MIT Press, 2004).
Shane, S. & Venkataraman, S. The promise of entrepreneurship as a field of research. Acad. Manag. Rev. 25 , 217–226 (2000).
Zahra, S. A. & Wright, M. Understanding the social role of entrepreneurship. J. Manage. Stud. 53 , 610–629 (2016).
Bonaventura, M. et al. Predicting success in the worldwide start-up network. Sci. Rep. 10 , 1–6 (2020).
Schwartz, H. A. et al. Personality, gender, and age in the language of social media: The open-vocabulary approach. PLoS ONE 8 , e73791 (2013).
Plank, B. & Hovy, D. Personality traits on twitter-or-how to get 1,500 personality tests in a week. In Proceedings of the 6th workshop on computational approaches to subjectivity, sentiment and social media analysis , pp 92–98 (2015).
Arnoux, P.-H. et al. 25 tweets to know you: A new model to predict personality with social media. In booktitleEleventh international AAAI conference on web and social media (2017).
Roberts, B. W., Kuncel, N. R., Shiner, R., Caspi, A. & Goldberg, L. R. The power of personality: The comparative validity of personality traits, socioeconomic status, and cognitive ability for predicting important life outcomes. Perspect. Psychol. Sci. 2 , 313–345 (2007).
Article PubMed PubMed Central Google Scholar
Youyou, W., Kosinski, M. & Stillwell, D. Computer-based personality judgments are more accurate than those made by humans. Proc. Natl. Acad. Sci. 112 , 1036–1040 (2015).
Soldz, S. & Vaillant, G. E. The big five personality traits and the life course: A 45-year longitudinal study. J. Res. Pers. 33 , 208–232 (1999).
Damian, R. I., Spengler, M., Sutu, A. & Roberts, B. W. Sixteen going on sixty-six: A longitudinal study of personality stability and change across 50 years. J. Pers. Soc. Psychol. 117 , 674 (2019).
Article PubMed Google Scholar
Rantanen, J., Metsäpelto, R.-L., Feldt, T., Pulkkinen, L. & Kokko, K. Long-term stability in the big five personality traits in adulthood. Scand. J. Psychol. 48 , 511–518 (2007).
Roberts, B. W., Caspi, A. & Moffitt, T. E. The kids are alright: Growth and stability in personality development from adolescence to adulthood. J. Pers. Soc. Psychol. 81 , 670 (2001).
Article CAS PubMed Google Scholar
Cobb-Clark, D. A. & Schurer, S. The stability of big-five personality traits. Econ. Lett. 115 , 11–15 (2012).
Graham, P. Do Things that Don’t Scale (Paul Graham, 2013).
McCarthy, P. X., Kern, M. L., Gong, X., Parker, M. & Rizoiu, M.-A. Occupation-personality fit is associated with higher employee engagement and happiness. (2022).
Pratt, A. C. Advertising and creativity, a governance approach: A case study of creative agencies in London. Environ. Plan A 38 , 1883–1899 (2006).
Klotz, A. C., Hmieleski, K. M., Bradley, B. H. & Busenitz, L. W. New venture teams: A review of the literature and roadmap for future research. J. Manag. 40 , 226–255 (2014).
Duggan, M., Ellison, N. B., Lampe, C., Lenhart, A. & Madden, M. Demographics of key social networking platforms. Pew Res. Center 9 (2015).
Fisch, C. & Block, J. H. How does entrepreneurial failure change an entrepreneur’s digital identity? Evidence from twitter data. J. Bus. Ventur. 36 , 106015 (2021).
Brush, C., Edelman, L. F., Manolova, T. & Welter, F. A gendered look at entrepreneurship ecosystems. Small Bus. Econ. 53 , 393–408 (2019).
Kanze, D., Huang, L., Conley, M. A. & Higgins, E. T. We ask men to win and women not to lose: Closing the gender gap in startup funding. Acad. Manag. J. 61 , 586–614 (2018).
Fan, J. S. Startup biases. UC Davis Law Review (2022).
AlShebli, B. K., Rahwan, T. & Woon, W. L. The preeminence of ethnic diversity in scientific collaboration. Nat. Commun. 9 , 1–10 (2018).
Article CAS Google Scholar
Żbikowski, K. & Antosiuk, P. A machine learning, bias-free approach for predicting business success using crunchbase data. Inf. Process. Manag. 58 , 102555 (2021).
Corea, F., Bertinetti, G. & Cervellati, E. M. Hacking the venture industry: An early-stage startups investment framework for data-driven investors. Mach. Learn. Appl. 5 , 100062 (2021).
Chapman, G. & Hottenrott, H. Founder personality and start-up subsidies. Founder Personality and Start-up Subsidies (2021).
Antoncic, B., Bratkovicregar, T., Singh, G. & DeNoble, A. F. The big five personality-entrepreneurship relationship: Evidence from slovenia. J. Small Bus. Manage. 53 , 819–841 (2015).
Download references
Acknowledgements
We thank Gary Brewer from BuiltWith ; Leni Mayo from Influx , Rachel Slattery from TeamSlatts and Daniel Petre from AirTree Ventures for their ongoing generosity and insights about startups, founders and venture investments. We also thank Tim Li from Crunchbase for advice and liaison regarding data on startups and Richard Slatter for advice and referrals in Twitter .
Author information
Authors and affiliations.
The Data Science Institute, University of Technology Sydney, Sydney, NSW, Australia
Paul X. McCarthy
School of Computer Science and Engineering, UNSW Sydney, Sydney, NSW, Australia
Faculty of Engineering and Information Technology, University of Technology Sydney, Sydney, Australia
Xian Gong & Marian-Andrei Rizoiu
Oxford Internet Institute, University of Oxford, Oxford, UK
Fabian Braesemann & Fabian Stephany
DWG Datenwissenschaftliche Gesellschaft Berlin, Berlin, Germany
Melbourne Graduate School of Education, The University of Melbourne, Parkville, VIC, Australia
Margaret L. Kern
You can also search for this author in PubMed Google Scholar
Contributions
All authors designed research; All authors analysed data and undertook investigation; F.B. and F.S. led multi-factor analysis; P.M., X.G. and M.A.R. led the founder/employee prediction; M.L.K. led personality insights; X.G. collected and tabulated the data; X.G., F.B., and F.S. created figures; X.G. created final art, and all authors wrote the paper.
Corresponding author
Correspondence to Fabian Braesemann .
Ethics declarations
Competing interests.
The authors declare no competing interests.
Additional information
Publisher's note.
Springer Nature remains neutral with regard to jurisdictional claims in published maps and institutional affiliations.
The original online version of this Article was revised: The Data Availability section in the original version of this Article was incomplete, the link to the GitHub repository was omitted. Full information regarding the corrections made can be found in the correction for this Article.
Supplementary Information
Supplementary information., rights and permissions.
Open Access This article is licensed under a Creative Commons Attribution 4.0 International License, which permits use, sharing, adaptation, distribution and reproduction in any medium or format, as long as you give appropriate credit to the original author(s) and the source, provide a link to the Creative Commons licence, and indicate if changes were made. The images or other third party material in this article are included in the article's Creative Commons licence, unless indicated otherwise in a credit line to the material. If material is not included in the article's Creative Commons licence and your intended use is not permitted by statutory regulation or exceeds the permitted use, you will need to obtain permission directly from the copyright holder. To view a copy of this licence, visit http://creativecommons.org/licenses/by/4.0/ .
Reprints and permissions
About this article
Cite this article.
McCarthy, P.X., Gong, X., Braesemann, F. et al. The impact of founder personalities on startup success. Sci Rep 13 , 17200 (2023). https://doi.org/10.1038/s41598-023-41980-y
Download citation
Received : 15 February 2023
Accepted : 04 September 2023
Published : 17 October 2023
DOI : https://doi.org/10.1038/s41598-023-41980-y
Share this article
Anyone you share the following link with will be able to read this content:
Sorry, a shareable link is not currently available for this article.
Provided by the Springer Nature SharedIt content-sharing initiative
By submitting a comment you agree to abide by our Terms and Community Guidelines . If you find something abusive or that does not comply with our terms or guidelines please flag it as inappropriate.
Quick links
- Explore articles by subject
- Guide to authors
- Editorial policies
Sign up for the Nature Briefing: AI and Robotics newsletter — what matters in AI and robotics research, free to your inbox weekly.

Among opposition leaders, Peter Dutton is a miracle survival story. But is he about to nuke himself with women voters?
Analysis Among opposition leaders, Peter Dutton is a miracle survival story. But is he about to nuke himself with women voters?
Peter Dutton is a freak of nature. Politically, that is.
Think about it: this is a man who has just completed his second year as Liberal leader, after a shocking election loss in which the House of Menzies was burgled for its richest electoral jewels.
Under normal circumstances, a new Liberal leader by this stage of the cycle is in Dante's ninth circle of hell, pleading for mercy while factional enemies armed with petrol-soaked rags on sticks conduct live press conferences chanting his personal failings.
Two years in, Dutton is not only still in office, but nobody inside his own party — or even in the National Party — is trying to blow him out of it. It is a truly extraordinary achievement.
His public popularity remains firmly in negative territory, according to Newspoll. So why isn't this translating into the customary seasonal orgy of backstabbing?
Two reasons.
The first is that there really isn't, ahem, any alternative.
The 2022 election's principal damage to the Liberal Party was the wiping-out of its leadership hatcheries. Wentworth, Kooyong, Warringah and Higgins, "safe" Liberal seats which over the years have incubated the Turnbulls and the Menzies and the Abbotts and the Hewsons and the Costellos and so forth, are now flushed of Liberal fingerlings and occupied by female independents who don't – in the main – harbour ambitions to be PM. Leader-in-waiting Josh Frydenberg is gone.
The closest thing to a challenge Dutton has now is from shadow treasurer Angus Taylor, and even he's not up to anything, and that's mainly for the second reason.
Which is: Peter Dutton is broadly thought inside his party to be doing a good job. Not because everyone in the party shares all of his views. It's more because he tends to listen to colleagues, doesn't get angry or paranoid, or bolt out on TV when silence is a more prudent option.
"I've never seen him lose his shit," says one colleague.
The times have suited Peter Dutton of late. He's been able to campaign on some of his favourite patches – crime and immigration – and has even been able to knock them together thanks to the High Court-sanctioned release of detained immigrants who have gone on to commit crimes.
Last year, Dutton resisted internal pressure to make an early call opposing the Voice to Parliament like the National Party did. His decision to remain uncommitted for months, then send send Jacinta Nampijinpa Price out to take the lead in opposition to the referendum, is now cited with admiration by colleagues.
Being thought a tactical genius by colleagues is a nice feeling. As is being broadly electorally competitive just two years after a terrible defeat.
All of the above makes Dutton much a much more dangerous opponent for Anthony Albanese than is commonly assumed.
But there is one risk associated with this unseasonably warm bath of internal approbation. The risk is that you start drinking your own bathwater. And in one area, one wonders whether Dutton isn't taking a discreet sip.
Of the policies the opposition leader has announced so far, most are designed to respond to already-existing anxieties within the population. Cutting the immigration rate, for instance, while explicitly linking high immigration to housing shortages.
But his decision to pursue nuclear energy as a principal policy decision is a much more high-risk call, as a new piece of research — supplied to the ABC — makes clear.
When it comes to nuclear, public opinion divides along gender lines
Over recent weeks, the RedBridge Group conducted a survey of around 2,000 Australian voters, seeking their views on various issues including nuclear energy. Respondents were asked whether they would support or oppose an Australian government lifting the ban on nuclear power so private investors could build nuclear power plants here.
The responses, across all voters, were kind of evenly divided. Strong supporters constituted 17 per cent, another 17 said they were supportive, 19 per cent were "neither", 15 per cent were opposed, 20 per cent strongly opposed, and 12 per cent were unsure. This shakes out to an extremely slender net negative of -1.
But the truly fascinating detail in the survey comes when you dig down into who especially loves the idea of nuclear, and who hates it.
And the biggest difference of opinion on nuclear, it turns out, breaks along gender lines.
Women disapproved of nuclear power strongly – just 7 per cent strongly agreed a ban should be lifted, compared with 24 per cent of male respondents. That's a net negative of -29 for women, and net positive of 26 for men.
The only demographics showing real enthusiasm for nuclear power were Coalition voters, those aged over 65, those who earn more than $3,000 a week, and those who own their own home. In each of these instances, every other group was majority opposed.
In other words, every other party's voters apart from the Coalition's registered a net negative, as well as every other age bracket apart from the most elderly, and all other income brackets apart from the top one.
Renters and mortgage holders alike disapproved on the whole. Among those who described themselves as under "a great deal of financial stress", the feeling on nuclear ran at negative 15. Among those under "no stress at all", however, the reception was much warmer – positive 19.
Tony Barry, a former Liberal staffer and strategist and now principal at Redbridge, has conducted multiple focus groups exploring attitudes to nuclear power and says that the headline one-third broad support for and openness to nuclear "is very soft".
"People know that nuclear power is used in other parts of the world, maybe they've been to Europe and seen power stations … but when you start talking about doing it in their state … [support] just evaporates,” he says.
"The Simpsons is an incredibly powerful cultural reference. Every group, someone will say something about three-eyed fish, or that they don’t want some Homer Simpson-type in charge of the plant."
Electoral popularity isn't an argument in itself, of course, and Dutton and his shadow energy minister Ted O'Brien are both genuinely convinced that nuclear should be on the table, regardless of its front-line saleability.
Surely we want political candidates to back their own judgment, and stake their own fortunes on proposals they think are the right ones.
The RedBridge numbers, though, make it incredibly clear where the risk lies: the most likely people to vote enthusiastically for the Coalition's nuclear future are the people who were going to vote for them anyway.
And in the two years that have elapsed since female voters demonstrated their annoyance at being ignored and talked down to, the Liberal Party has failed to do anything about its structural under-representation of women in parliament, even relegating its most senior woman in South Australia — shadow health minister Anne Ruston — to an unwinnable spot on the Senate ticket , replacing her with backbencher Alex Antic. A merit appointment, presumably.
On this Saturday just gone, NSW Liberal preselectors similarly relegated Hollie Hughes , a busy frontbencher and working mum known for her work on the NDIS. Senator Hughes has taken this week off Parliament, suggesting that perhaps someone else can take on her full rota of committee commitments for the week, and who could blame her?
There always seems to be an abundance of reasons to get rid of women; almost as many reasons as traditionally abound for holding on to and even promoting male duds. Women do notice this stuff.
And "Never mind ladies, have a nuclear power plant" may not be a very compelling change of subject.
- X (formerly Twitter)
Related Stories
Dutton's reply was everything we should expect: a calculated, ideological butchery of the most vulnerable budget bits.
- Open access
- Published: 30 May 2024
Challenges and advantages of electronic prescribing system: a survey study and thematic analysis
- Hamid Bouraghi 1 ,
- Behzad Imani 2 ,
- Abolfazl Saeedi 3 ,
- Ali Mohammadpour 1 ,
- Soheila Saeedi 1 na1 ,
- Taleb Khodaveisi 1 na1 &
- Tooba Mehrabi 4
BMC Health Services Research volume 24 , Article number: 689 ( 2024 ) Cite this article
Metrics details
Introduction
Electronic prescribing (e-prescribing) systems can bring many advantages and challenges. This system has been launched in Iran for more than two years. This study aimed to investigate the challenges and advantages of the e-prescribing system from the point of view of physicians.
In this survey study and thematic analysis, which was conducted in 2023, a researcher-made questionnaire was created based on the literature review and opinions of the research team members and provided to the physician. Quantitative data were analyzed using SPSS software, and qualitative data were analyzed using ATLAS.ti software. Rank and point biserial, Kendall’s tau b, and Phi were used to investigate the correlation between variables.
Eighty-four physicians participated in this study, and 71.4% preferred to use paper-based prescribing. According to the results, 53.6%, 38.1%, and 8.3% of physicians had low, medium, and high overall satisfaction with this system, respectively. There was a statistically significant correlation between the sex and overall satisfaction with the e-prescribing system ( p -value = 0.009) and the computer skill level and the prescribing methods ( P -value = 0.042). Physicians face many challenges with this system, which can be divided into five main categories: technical, patient-related, healthcare providers-related, human resources, and architectural and design issues. Also, the main advantages of the e-prescribing system were process improvement, economic efficiency, and enhanced prescribing accuracy.
The custodian and service provider organizations should upgrade the necessary information technology infrastructures, including hardware, software, and network infrastructures. Furthermore, it would be beneficial to incorporate the perspectives of end users in the system design process.
Peer Review reports
Medicine, a crucial commodity in healthcare due to its economic and strategic value, is a fundamental pillar in primary disease treatment. It constitutes significant health expenditures and budgets worldwide [ 1 ]. The prudent management of this valuable resource, through its appropriate prescription and usage, is essential. This is a key factor in ensuring the health security of communities [ 2 ]. Numerous studies indicate that errors in drug administration are prevalent. Although a significant proportion of these errors are preventable, they can leave serious complications for patients and even fatalities [ 3 ]. As the complexity of the drug prescribing process increases, resultant injuries and complications will likely escalate. Therefore, medication prescription is one of the main concerns and priorities of policymakers and trustees in the healthcare domain. In this regard, relentless endeavors are undertaken to enhance and optimize this process, and new supplementary solutions will be used as required. Employing electronic prescription (e-prescribing) systems as an alternative to manual prescription is a practical solution that can enhance and streamline this critical process [ 4 ].
In the traditional paper-based prescribing system, numerous issues arise, including illegible prescriptions, ambiguous orders, omissions, prescription forgery, and misidentification of patients. Studies indicate that these problems compromise patient safety and negatively impact the outcomes of drug treatments [ 5 , 6 ]. E-prescribing emerges as an effective and definitive solution to the inefficiencies, susceptibility to fraud, and administrative burdens associated with paper-based prescribing systems [ 7 ]. E-prescribing extends beyond merely utilizing a computer for prescription writing and storage. This technology encompasses all stages of the prescription process, including patient identification, prescription registration, prescription modification, duplication and renewal of prescriptions, and the transfer of prescriptions among stakeholders, all facilitated through specialized software and internet platforms [ 8 , 9 , 10 ].
As an information system, the e-prescribing system can integrate with other organizational systems, such as electronic health records and pharmacy information systems, within healthcare centers like hospitals [ 11 ]. Through the implementation and utilization of such a system, it is possible to overcome the problems and constraints of the traditional prescribing system due to the complexity of medical care and the increase in the number of drugs, thereby benefiting from its potential advantages. Some of the benefits of an e-prescribing system include reducing healthcare costs for stakeholders (patients, healthcare providers, insurers, and policymakers), reducing common prescribing errors, improving medication outcomes, increasing patient safety, increasing the readability and accuracy of prescriptions, enhancing coordination among stakeholders involved in the drug therapy process, and supporting clinical decision-making at the time of drug administration [ 12 , 13 , 14 ].
Despite the potential benefits of e-prescribing systems in the healthcare industry and significant investments and efforts by stakeholders to support such systems, their usage and adoption remain low, resulting in the failure of numerous implemented projects [ 11 , 12 ]. Given that e-prescribing systems are designed according to the specific needs and internal standards of each country, numerous studies have been conducted worldwide to investigate the benefits, challenges, the reasons for the failure and lack of acceptance of such systems [ 15 , 16 ].
E-prescribing systems in countries like Denmark, the United States, Finland, Sweden, and the United Kingdom are commonly tested and implemented at state, local, or regional levels. These systems cover the entire or a significant portion of the prescribing process. Variations in healthcare and insurance systems across different countries lead to diverse approaches regarding e-prescribing and its evolution. Consequently, these countries exhibit distinct starting points, implementation procedures, and technical strategies. Moreover, e-prescribing systems and models vary not only across different countries but also within the same country [ 17 ]. While meticulously developed and successfully implemented in the United States of America, England, and Germany, this system has reached significant maturity and yielded substantial advantages for the health systems of these countries. However, in other nations, especially developing countries, e-prescribing still encounters significant challenges on its path to widespread acceptance and goal achievement [ 18 , 19 , 20 , 21 ].
Recognizing that the implementation of e-prescribing is a priority for the Iran Ministry of Health and Medical Education (MOH), the Iran Food and Drug Administration (IFDA) established a multi-stakeholder working group in 2015. This group, composed of medical informatics experts, aimed to develop recommendations for effective e-prescribing implementation [ 22 ]. In Iran, adopting e-prescribing in governmental and university hospitals has been proposed as a legal requirement since 2020. The Social Security Organization, a pioneering institution in this domain, has aligned with the implementation policies of this plan and has ceased issuing treatment booklets since early 2021 [ 23 ]. The Health Insurance Organization, as another government institution, independently developed and deployed its e-prescription system across all medical education centers affiliated with universities of medical sciences in Iran. Consequently, the two primary organizations (Social Security Organization and Health Insurance Organization) have successfully implemented the e-prescribing system. Their goals include efficient management of healthcare resources, reduction of common manual prescribing errors, and enhancement of patient safety [ 24 ].
In general, medical centers in Iran employ three distinct electronic prescription systems. “Electronic Prescription (EP)” and “Dinad” serve outpatients covered by the Social Security and Health Insurance Organization, while “Shafa” caters to all inpatients. For individuals without coverage from these insurances, physicians resort to paper prescriptions [ 25 ]. Electronic prescribing was not implemented simultaneously in all provinces of Iran. It was first used on a trial basis in a few provinces and then implemented throughout the country. Although these systems have provided significant benefits to their users in Iran, they have also encountered numerous challenges. Consequently, this comprehensive study was undertaken to explore both the advantages and obstacles associated with e-prescribing systems in Iran.
This survey study and thematic analysis was conducted to examine the challenges and advantages of the e-prescribing system in Iran in 2023. This study was conducted in three main steps: literature review and questionnaire design, data collection, and data analysis.
Literature review and questionnaire design
In the first step of this research, a questionnaire was designed based on the review of similar studies and the opinions of the research team members. To design the questionnaire, various databases, including PubMed, Google Scholar, and Scopus, were searched with related terms such as “electronic prescribing,” “electronic prescribing challenges,” and “electronic prescribing advantages.” Then, the most relevant articles retrieved from these databases were examined, and relevant data were extracted from these articles. Then, focus group sessions were held with the research team. The data extracted from the articles were presented in the sessions, and based on these data and the opinions of the research team, the questionnaire was finalized. This questionnaire had three sections: (1) demographic data (2), questions related to the advantages and challenges of e-prescribing, and (3) open-ended questions related to the challenges and advantages of the e-prescribing system. A five-point Likert scale from completely agree to completely disagree was used for the questions of the second part of the questionnaire. The face and content validity of the questionnaire was checked and confirmed with the cooperation of five experts in health information management, medical informatics, and information technology who were thoroughly familiar with prescribing systems. The content validity of the questionnaire was measured using the Content Validity Index (CVI) and Content Validity Ratio (CVR). To determine CVR, the experts were asked to classify each of the questions based on the three-point Likert scale as follows:
The question is necessary
The question is useful but not necessary
The question is not necessary
Then, the following formula was used to calculate CVR:
CVR = (Ne − N/2)/ (N/2), (N: total number of experts, Ne: the number of experts who have chosen the “necessary” option.).
Based on the Lawshe table for minimum values of CVR, items with CVR equal to or greater than 0.99 were kept. To calculate the CVI, the experts determined the degree of relevance of each question on a 4-point Likert scale from not relevant to completely relevant. The following formula was used to decide about the acceptance of each question:
CVI: The number of experts who chose options 3 and 4 / the total number of experts. It was decided to reject or accept each question as follows: < 0.7 = rejected, 0.7–0.79 = revised, > 0.79 = accepted. The reliability of the questionnaire was calculated using Cronbach’s alpha and Guttman coefficient. Values greater than 0.7, 0.5–0.7, and less than 0.5 indicate high, acceptable, and low reliability of the questionnaire, respectively.
The third part of the questionnaire included open-ended questions. Two following questions were placed at the end of the questionnaire and were asked to the physicians:
In your opinion, what other advantages does this electronic prescribing system have?
In your opinion, what other challenges does this electronic prescribing system have?
Data collection
After the questionnaire was finalized, it was prepared in both paper and electronic formats. The electronic version of the questionnaire was prepared on the Porsline platform. For the survey, first, a list of physicians working in the teaching hospitals was prepared, and then we tried to get the contact numbers of the physicians as well. The questionnaire link was sent to physicians through the local social networks whose contact numbers were available, and physicians whose contact numbers were not available were referred to them in person. Many physicians refused to receive the questionnaire and answers due to lack of time. Two reminder messages were also sent to the doctors who had received the questionnaire link through social networks. In the face-to-face group, the doctors who did not have enough time to complete the questionnaire at that moment, the researcher provided the questionnaire to the physicians and coordinated with them to receive it at a later time. A total of 122 physicians agreed to participate in the study. It should be noted that to avoid missing data, it was mandatory to answer all the questions in the electronic questionnaire, and in the paper-based questionnaire, the researchers checked the questionnaire immediately, and if any fields were not completed, they asked the physicians to complete the incomplete items of the questionnaire again.
Data analysis
Descriptive statistics including mean, standard deviation, frequency median, interquartile range and percentage were used for data analysis.
The relationship of “sex,” “specialty,” “physician’s computer skills,” “age,” and “duration” with “satisfaction” was investigated. Since “satisfaction” is a qualitative ordinal variable, the Rank-biserial index was used to examine the relationship between this variable and two-level nominal variables such as “gender” and “specialty.” Kendall’s tau b index was also used to examine the relationship between “satisfaction” (ordinal variable) with rank variables such as “physician’s computer skills” and continuous quantitative variables such as “age” and “duration.” To investigate the relationship between “willingness to use paper-based or e-prescribing” with “sex,” “specialty,” “physician’s computer skills,” “age,” and “duration,” Phi, Rank-biserial, and Point-biserial were used respectively. The p -values obtained from the chi-square test were also reported to check the presence or absence of a relationship between two variables. The type I error in this study was considered 5%. Data analysis was carried out using SPSS version 26.
The answers given by 84 physicians to two open-ended questions were typed in Word.
Thematic analysis was used to analyze the open-ended questions and identify themes within qualitative data. For thematic analysis, first, the answers typed in the Word were imported into the ATLAS.ti software, and then the pattern extraction process was carried out according to the following steps:
The imported text was read several times to get familiar with the data
After familiarizing with the data, initial coding was done
After coding, the extracted codes were checked and revised many times
Similar codes were merged and grouped, and subthemes were created
Finally, the sub-themes were reviewed and linked, and the main themes were created
The designed questionnaire was given to 122 physicians, of which 84 physicians completed the questionnaires (response rate: 68.85%). Demographic characteristics of physicians are given in Table 1 . Most of the participants were general practitioners (56%) and women (53.6%). 91.7% of the physicians believed that they have medium and high computer skills and the average duration of using the e-prescribing system was 15.50 ± 8.798 months.
The results showed that the questionnaire had acceptable reliability (Cronbach’s alpha = 0.605, Guttman’s coefficient = 0.718). The mean (std. deviation), median and interquartile range of each question in the questionnaire are given in Table 2 . The questions were categorized into two sections: advantages and challenges of the e-prescribing system. The total mean score of advantages for the e-prescribing system was 2.15 and this value for challenges of this system was 2.75. Out of the advantages of this technology, the highest mean score (2.79) was related to the “E-prescribing system has reduced the possibility of wrong drug delivery due to illegible prescriptions” and the lowest (1.24) was related to the “The e-prescribing system has led to improved physician performance”. The most important challenge that physicians had with the e-prescribing system was the insufficient bandwidth with an average of 3.49. Two other challenges mentioned by physicians about this system and received a high mean score (3.43) were the challenges related to lengthening the duration of each visit and increasing the waiting time of patients.
The results of investigating the correlation between the duration of e-prescribing system use, age, sex, specialty, and the physician’s computer skills with the overall satisfaction with the e-prescribing system are reported in Table 3 . According to the results, 45 (53.6%), 32 (38.1%), and 7 (8.3%) physicians had low, medium and high overall satisfaction with this system, respectively. There was a statistically significant correlation between the sex and overall satisfaction with the e-prescribing system ( p -value = 0.009).
The results of the correlation between duration, age, sex, specialty, and the physician’s computer skills with the willingness to use paper-based prescribing or the e-prescribing system are reported in Table 4 . According to the results, 60 (71.4%) and 24 (28.6%) physicians preferred to use paper-based and e-prescribing respectively. There was a statistically significant correlation between the computer skill level and the prescribing methods ( P -value = 0.042).
The themes and sub-themes extracted from the question related to the advantages of the e-prescribing system are shown in Fig. 1 . The main themes of the e-prescribing system’s advantages were the following:
Process improvement
Economic efficiency
Enhance the accuracy of prescribing
These three themes included a total of 10 sub-themes.
Among the advantages noted for electronic prescribing, the possibility of editing prescriptions, providing different dosages of drugs, and the impossibility of manipulating prescriptions by patients or other people were mentioned more than other advantages. Also another mentioned advantage was the possibility of providing pre-prepared prescriptions for common diseases, which led to the acceleration of prescribing for these diseases.
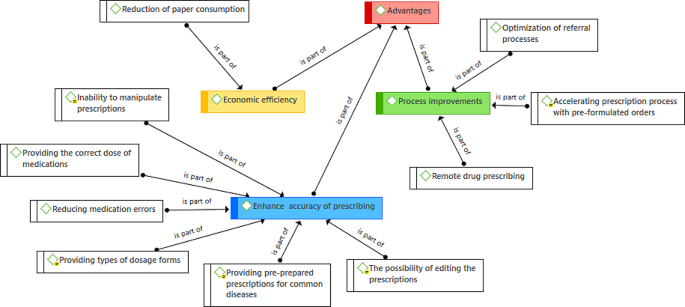
Thematic map of concepts extracted from qualitative data related to the advantages of the e-prescribing system
Concepts related to the challenges of the e-prescribing system were categorized into five main themes as follows (Fig. 2 ):
Technical issues
Patient-related issues
Healthcare providers-related issues
Human resources challenges
Architectural and design issues
These five themes included more than 30 sub-themes.
Many challenges for electronic prescribing were mentioned in the form of given themes. One of the most important challenges mentioned by many physicians was various technical problems including network disconnection. Also, another big challenge that caused the dissatisfaction of the patients was the lack of skill of many physicians in working with computer systems, which led to the low speed of typing the drugs in the system and as a result, increased the duration of the patients’ visits. Also, many physicians did not have computer systems in their clinics, which led to the lack of electronic prescriptions and, as a result, the lack of use of insurance services for patients. Also, considering that many physicians are used to the paper prescription method, they were not willing to accept the changes and resisted these changes, as a result, they needed personnel to register the prescriptions.
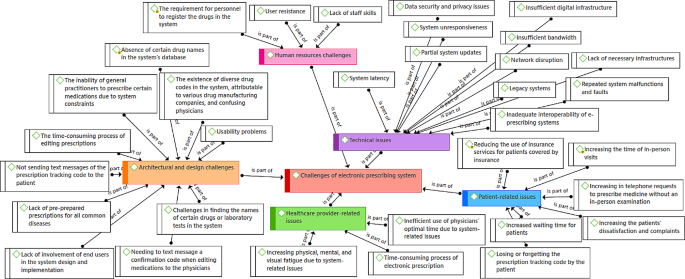
Thematic map of concepts related to the challenges of the e-prescribing system
E-prescribing systems have many advantages, but they also pose certain challenges. These systems can enhance medication safety by reducing prescription errors caused by illegible handwriting or oral miscommunication. They can also improve efficiency by streamlining the prescription process, reducing the time spent on phone calls and faxes between healthcare providers and pharmacies. Furthermore, e-prescribing can provide clinicians with up-to-date information about patients’ medications and allergies, thereby improving patient care.
Although e-prescribing systems have many advantages, their implementation is not without any challenges. These include the costs associated with system implementation and maintenance, issues related to system interoperability, and the necessity for user training and technical support. Moreover, while these systems can mitigate traditional medication errors, they may also introduce new types of errors, such as those caused by user interface design or software glitches. Maximizing the benefits and minimizing the challenges associated with e-prescribing systems requires meticulous system design, comprehensive user training, and continuous system evaluation.
As demonstrated in the results section, the e-prescribing system’s mean overall benefit score was 2.15. This score suggests a moderate level of perceived benefits. It implies that while certain advantages are acknowledged, the system still needs to be improved to enhance user satisfaction and the perception of benefits. In this context, among the factors associated with the system’s benefits from the users’ perspective, the statements “Improved workflow has resulted from e-prescribing” and “The e-prescribing system has led to improved physician performance” received average scores of 1.48 and 1.24, respectively. These relatively low scores suggest that respondents of the survey or study largely disagree that the electronic system has enhanced their workflow or improved their performance. Several studies [ 11 , 12 , 26 , 27 ] have demonstrated that users do not concur that the use of prescribing systems leads to workflow improvement or performance enhancement. There are multiple possible reasons for this, including:
Usability issues: The e-prescribing system might not be user-friendly or intuitive, leading to difficulties in adoption among healthcare professionals.
Training and support: There might be a lack of adequate training and support for the users, making it challenging for them to adapt to the new system.
System limitations: The system might not be flexible enough to accommodate the diverse needs of different healthcare settings, leading to workflow inefficiencies.
Resistance to change: Healthcare professionals, like any other group, might resist changes to established routines. This resistance could affect their perception of the system’s benefits.
Among the challenges identified in the use of e-prescribing systems, the statement “Doctors have faced challenges with e-prescribing due to insufficient bandwidth” received the highest score of 3.49. According to this relatively high score, the survey or study respondents strongly agree that insufficient bandwidth has been a significant obstacle to the use of e-prescribing. This issue results in prolonged patient waiting times, leading to extended queues and a decrease in physician productivity. There are multiple factors that can cause insufficient bandwidth, such as:
Network Infrastructure: In areas with poor network infrastructure, insufficient bandwidth can significantly slow down the operation of e-prescribing systems, making it difficult for doctors to use them effectively.
System Requirements: To function optimally, e-prescribing systems may need a certain level of bandwidth. System lags or downtime could result if the available bandwidth is below this level.
Data Transfer: E-prescribing systems often need to transfer large amounts of data, including patient records, prescriptions, and other related information. Insufficient bandwidth can slow down this data transfer, affecting the system’s efficiency.
Real-time Updates: Many e-prescribing systems provide real-time updates to ensure that all users have the most current information. If there is not enough bandwidth, these updates can be delayed, resulting in potential errors or miscommunications.
Generally, as indicated by various studies [ 28 , 29 , 30 ], the implementation of e-prescribing systems requires robust hardware, sophisticated software, and a reliable network infrastructure. These elements are integral to the successful deployment and operation of such systems. According to this study, the hardware, software, and network infrastructure in Iran are not suitable for the implementation of e-prescribing systems. This inadequacy has caused increased challenges and dissatisfaction among users. Furthermore, our evaluation of physicians’ overall satisfaction with the e-prescribing system revealed that the majority, 45 (53.6%), had low satisfaction. Conversely, only a small proportion, 7 (8.3%), reported high satisfaction. Subsequently, the e-prescribing system is not widely accepted by users, with the majority (71.4%) favoring paper-based prescribing. Many other studies have indicated higher levels of user satisfaction and a greater willingness to accept and use e-prescribing systems, contrary to our study’s findings [ 31 , 32 , 33 , 34 ]. The low level of satisfaction and users’ reluctance to adopt the e-prescribing system can be attributed to various challenges and problems identified by them. Users have been greatly impacted by these issues, which range from technical difficulties to system design and architecture issues, resulting in dissatisfaction, diminished motivation, and resistance towards the system.
Although e-prescribing systems represent a novel and transformative approach in healthcare, they offer numerous benefits, including improved efficiency, reduced medication errors, and enhanced patient safety. However, our study highlights the presence of significant challenges, such as technical issues and problems related to system design and architecture, which result in low user satisfaction and hinder system adoption. The custodian and service provider organizations should upgrade the necessary information technology infrastructures, including hardware, software, and network infrastructures, to address the technical challenges. Furthermore, given that the design and architectural issues of the e-prescribing systems have resulted in user dissatisfaction and diminished motivation to use the system, identifying and addressing these problems and shortcomings in future updates is recommended. Moreover, it is important to take into account the end users’ perspectives during the system design process.
Data availability
All data generated or analyzed during this study are included within this article.
Babaie J, Elmi S. Drug prescribing in family physician program involved health care centers and hospital of Hashtrood (Iran) in 2017. Iran J Health Insurance. 2019;2(3):162–71.
Google Scholar
Borriharn S, Kaewvichit S, Pannavalee W, Thiankhanithikun K, Kanjanarat P. A systematic review: quality indicators for assessing Drug System Management. J Health Sci. 2014:934–42.
Pirmohamed M, James S, Meakin S, Green C, Scott AK, Walley TJ, et al. Adverse drug reactions as cause of admission to hospital: prospective analysis of 18 820 patients. Bmj. 2004;329(7456):15–9.
Cresswell K, Coleman J, Slee A, Williams R, Sheikh A, Team eP. Investigating and learning lessons from early experiences of implementing ePrescribing systems into NHS hospitals: a questionnaire study. PLoS ONE. 2013;8(1):e53369.
Article CAS PubMed PubMed Central Google Scholar
Elliott RA, Lee CY, Hussainy SY. Electronic prescribing and medication management at a residential aged care facility. Appl Clin Inf. 2016;7(01):116–27.
Article Google Scholar
Shawahna R, Rahman NU, Ahmad M, Debray M, Yliperttula M, Decleves X. Electronic prescribing reduces prescribing error in public hospitals. J Clin Nurs. 2011;20(21–22):3233–45.
Article PubMed Google Scholar
Ahmadi M, Samadbeik M, Sadoughi F. Modeling of outpatient prescribing process in Iran: a gateway toward electronic prescribing system. Iran J Pharm Res. 2014;13(2):725.
PubMed PubMed Central Google Scholar
Shi L-P, Liu C-H, Cao J-F, Lu Y, Xuan F-X, Jiang Y-T, et al. Development and application of a closed-loop medication administration system in University of Hongkong-Shenzhen Hospital. Front Nurs. 2018;5(2):105–9.
Grossman JM, Gerland A, Reed MC, Fahlman C. Physicians’ experiences using Commercial E-Prescribing systems: Physicians are optimistic about e-prescribing systems but face barriers to their adoption. Health Aff. 2007;26(Suppl2):393–404.
Bell DS, Cretin S, Marken RS, Landman AB. A conceptual framework for evaluating outpatient electronic prescribing systems based on their functional capabilities. J Am Med Inf. 2004;11(1):60–70.
Vejdani M, Varmaghani M, Meraji M, Jamali J, Hooshmand E, Vafaee-Najar A. Electronic prescription system requirements: a scoping review. BMC Med Inf Decis Mak. 2022;22(1):1–13.
Mohsin-Shaikh S, Furniss D, Blandford A, McLeod M, Ma T, Beykloo MY, et al. The impact of electronic prescribing systems on healthcare professionals’ working practices in the hospital setting: a systematic review and narrative synthesis. BMC Health Serv Res. 2019;19:1–8.
Gates PJ, Hardie R-A, Raban MZ, Li L, Westbrook JI. How effective are electronic medication systems in reducing medication error rates and associated harm among hospital inpatients? A systematic review and meta-analysis. J Am Med Inform Assoc. 2021;28(1):167–76.
Hailiye Teferi G, Wonde TE, Tadele MM, Assaye BT, Hordofa ZR, Ahmed MH, et al. Perception of physicians towards electronic prescription system and associated factors at resource limited setting 2021: Cross sectional study. PLoS ONE. 2022;17(3):e0262759.
Boonstra A. Interpretive perspectives on the acceptance of an electronic prescription system. J Inform Technol Case Application Res. 2003;5(2):27–50.
Boonstra A, Boddy D, Fischbacher M. The limited acceptance of an electronic prescription system by general practitioners: reasons and practical implications. New Technol Work Employ. 2004;19(2):128–44.
Samadbeik M, Ahmadi M, Sadoughi F, Garavand A. A copmarative review of electronic prescription systems: lessons learned from developed countries. J Res Pharm Pract. 2017;6(1):3–11.
Article PubMed PubMed Central Google Scholar
Chang H-Y, Kan HJ, Shermock KM, Alexander GC, Weiner JP, Kharrazi H. Integrating e-prescribing and pharmacy claims data for predictive modeling: comparing costs and utilization of health plan members who fill their initial medications with those who do not. J Managed Care Specialty Pharm. 2020;26(10):1282–90.
Cresswell KM, Lee L, Slee A, Coleman J, Bates DW, Sheikh A. Qualitative analysis of vendor discussions on the procurement of computerised physician order entry and clinical decision support systems in hospitals. BMJ open. 2015;5(10):e008313.
Fischer SH, Rudin RS, Shi Y, Shekelle P, Amill-Rosario A, Scanlon D, et al. Trends in the use of computerized physician order entry by health-system affiliated ambulatory clinics in the United States, 2014–2016. BMC Health Serv Res. 2020;20:1–6.
Gall W, Aly A-F, Sojer R, Spahni S, Ammenwerth E. The national e-medication approaches in Germany, Switzerland and Austria: a structured comparison. Int J Med Informatics. 2016;93:14–25.
Dehghan H, Eslami S, Ghasemi SH, Jahangiri M, Bahaadinbeigy K, Kimiafar K, et al. Development of a National Roadmap for electronic prescribing implementation. Stud Health Technol Inform. 2019;260:121–7.
PubMed Google Scholar
Jebraeily M, Rashidi A, Mohitmafi T, Muossazadeh R. Evaluation of Outpatient Electronic prescription system capabilities from the perspective of Physicians in Specialized Polyclinics of Urmia Social Security Organization. Payavard Salamat. 2021;14(6):557–68.
Raeesi A, Abbasi R, Khajouei R. Evaluating physicians’ perspectives on the efficiency and effectiveness of the electronic prescribing system. Int J Technol Assess Health Care. 2021;37(1):e42.
Hayavi-Haghighi MH, Davoodi S, Teshnizi SH, Jookar R. Usability evaluation of electronic prescribing systems from physicians’ perspective: a case study from southern Iran. Inf Med Unlocked. 2024;45:101460.
Williams J, Bates DW, Sheikh A. Optimising electronic prescribing in hospitals: a scoping review protocol. BMJ Health Care Inf. 2020;27(1).
Santiago BC, Bengoechea MM, Barrueta OI, Ibañez AS, Aramburu EA, Garcia EI, et al. OHP-005 advantages and disadvantages of an electronic prescribing system. Aspects to consider during pharmacist validation. Eur J Hosp Pharmacy: Sci Pract. 2013;20(Suppl 1):A137–A.
Tamblyn R, Huang A, Kawasumi Y, Bartlett G, Grad R, Jacques A, et al. The development and evaluation of an integrated electronic prescribing and drug management system for primary care. J Am Med Inform Assoc. 2006;13(2):148–59.
Elson B. Electronic prescribing in ambulatory care: a market primer and implications for managed care pharmacy. J Managed Care Pharm. 2001;7(2):115–20.
Farida S, Krisnamurti DGB, Hakim RW, Dwijayanti A, Purwaningsih EH. Implementation of electronic prescribing. eJournal Kedokteran Indonesia. 2017;5(3):16–211.
Abdel-Qader DH, Cantrill JA, Tully MP. Satisfaction predictors and attitudes towards electronic prescribing systems in three UK hospitals. Pharm World Sci. 2010;32:581–93.
Shams MEHES. Implementation of an e-prescribing service: users’ satisfaction and recommendations. Can Pharmacists J. 2011;144(4):186–91.
Bright HR, Peter J, Chandy S. Electronic prescribing system in a Teaching Hospital-user satisfaction and factors affecting successful implementation. Der Pharmacia Letter. 2019;11(2):10–24.
Jariwala KS, Holmes ER, Banahan DJ III. Adoption of and experience with e-prescribing by primary care physicians. Res Social Administrative Pharm. 2013;9(1):120–8.
Download references
Acknowledgements
This work was supported by a grant from Hamadan University of Medical Sciences Research Council (140206074578).
Author information
Soheila Saeedi and Taleb Khodaveisi contributed equally to this work.
Authors and Affiliations
Department of Health Information Technology, School of Allied Medical Sciences, Hamadan University of Medical Sciences, Shahid Fahmideh Blvd, Hamadan, Iran
Hamid Bouraghi, Ali Mohammadpour, Soheila Saeedi & Taleb Khodaveisi
Department of Operating Room, School of Paramedicine, Hamadan University of Medical Sciences, Hamadan, Iran
Behzad Imani
School of Medicine, Iran University of Medical Sciences, Tehran, Iran
Abolfazl Saeedi
Health Information Management Department, Besat Hospital, Hamadan University of Medical Sciences, Hamadan, Iran
Tooba Mehrabi
You can also search for this author in PubMed Google Scholar
Contributions
SS, TKH and HB developed the concept for the study. SS, TM, and AS collected data. SS and TKH carried out the analysis and interpretation under the supervision of HB and BI. Finally, SS, AM, AS, and HB drafted the manuscript. All authors reviewed the content and approved it.
Corresponding authors
Correspondence to Soheila Saeedi or Taleb Khodaveisi .
Ethics declarations
Ethics approval and consent to participate.
The study was conducted in accordance with the Declaration of Helsinki and approved by a local ethics committee in Iran, namely Ethics Committee of the Hamadan University of Medical Sciences (IR.UMSHA.REC.1402.408). Verbal informed consent obtained from all the participants included in the study and was approved by the Ethics Committee of the Hamadan University of Medical Sciences.
Consent for publication
Not applicable.
Competing interests
The authors declare no competing interests.
Additional information
Publisher’s note.
Springer Nature remains neutral with regard to jurisdictional claims in published maps and institutional affiliations.
Rights and permissions
Open Access This article is licensed under a Creative Commons Attribution 4.0 International License, which permits use, sharing, adaptation, distribution and reproduction in any medium or format, as long as you give appropriate credit to the original author(s) and the source, provide a link to the Creative Commons licence, and indicate if changes were made. The images or other third party material in this article are included in the article’s Creative Commons licence, unless indicated otherwise in a credit line to the material. If material is not included in the article’s Creative Commons licence and your intended use is not permitted by statutory regulation or exceeds the permitted use, you will need to obtain permission directly from the copyright holder. To view a copy of this licence, visit http://creativecommons.org/licenses/by/4.0/ . The Creative Commons Public Domain Dedication waiver ( http://creativecommons.org/publicdomain/zero/1.0/ ) applies to the data made available in this article, unless otherwise stated in a credit line to the data.
Reprints and permissions
About this article
Cite this article.
Bouraghi, H., Imani, B., Saeedi, A. et al. Challenges and advantages of electronic prescribing system: a survey study and thematic analysis. BMC Health Serv Res 24 , 689 (2024). https://doi.org/10.1186/s12913-024-11144-3
Download citation
Received : 11 December 2023
Accepted : 23 May 2024
Published : 30 May 2024
DOI : https://doi.org/10.1186/s12913-024-11144-3
Share this article
Anyone you share the following link with will be able to read this content:
Sorry, a shareable link is not currently available for this article.
Provided by the Springer Nature SharedIt content-sharing initiative
- Electronic prescribing system
- Thematic analysis
- Prescription
BMC Health Services Research
ISSN: 1472-6963
- General enquiries: [email protected]
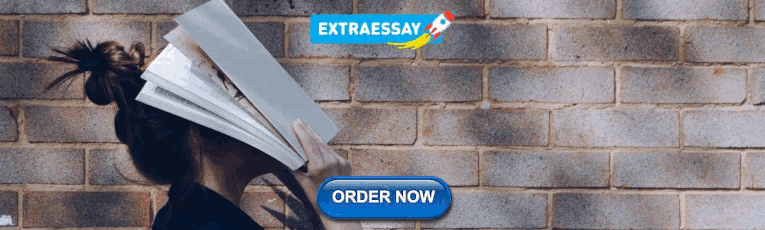
IMAGES
VIDEO
COMMENTS
ABC Analysis, an Inventory Management Technique at a. Manufacturing Company. Dr.S.Kavitha. A.Kandeepan. N.Narmadha. Abstract. The process of control and management of inventory is a very important ...
ABC Analysis For Inventory Management: Bridging The Gap Between Research And Classroom Handanhal Ravinder, Montclair State University, USA ... paper, it is argued that it is time to bring multi-criteria ABC analysis center-stage in the textbooks. Today's businesses and supply chains operate in a world where the ability to deliver the right ...
ABC analysis is a well-established categorization technique based on the Pareto Principle for determining which items should get priority in the management of a company's inventory. In ...
The ABC analysis is perhaps the most widely used method in inventory management aiming to classify items into three ordered classes: class A contains a relatively small number (5-10%) of the most important items, class B includes a larger number (20-30%) of items with moderate importance while the remaining (50-70%) items with relatively little importance belong to class C. Footnote 1 ...
2.2.4. ABC analysis. The ABC stock control technique relies on that the decision a little bundle of the things may usually address the weight of money estimation of the total stock. It is used as a part of the era method, while a tremendous number of things may happen from a little part of the money estimation of stores.
This study aimed to understand the inventory management of Giant Superstore Taman Connaught. The study was qualitative study that explored the ABC analysis efficiency of inventory management in Giant Superstore, Taman Connaught an outlet of GCH Retail (Malaysia) SDN. BHD. ABC analysis was one method to manage the inventory management who good position was arranged according to the category ...
ABC and XYZ analyses are carried out for the inventory items to determine the production strategy of each item class and the Economic Order Quantity (EOQ). After this examination, one of the end products of the company is chosen to develop the Materials Requirement Plan (MRP) for. The Bill of Materials (BOM) for the chosen product is created ...
The large body of research was summarized based on multiple criteria ABC analysis that has accumulated since the 1980s and the authors recommend that textbooks incorporate their key findings and methods into their discussions of this topic. ABC analysis is a well-established categorization technique based on the Pareto Principle for determining which items should get priority in the management ...
Academia.edu is a platform for academics to share research papers. ABC Analysis For Inventory Management: Bridging The Gap Between Research And Classroom ... 3. STATUS OF RESEARCH ON MULTI-CRITERIA ABC ANALYSIS Since Flores and Whybark (1987) first proposed looking at more than one criterion, this has been an area of active research. ...
The inventory management practices of six major companies/institutions in various industries were compared with recommendations for improvement by using the ABC analysis inventory management method. The ABC analysis tool was found to be beneficial to most companies discussed in this paper, with several companies already utilising the basic principles behind the method, either manually or with ...
20% of total annual consumption value. The Class 'C' items normally constitute 70% to 75 % of all inventory items. and account for 5% to 10% of total annual consu mption value. Steps for ...
The materials used for construction are listed based on ABC analysis as shown in Table 3. The ABC analysis is a tool for material administration which involves three groups such as A, B and C. A group consists of the most valuable items accounting 70% - 80% of the project cost and 20% of the total inventory.
Most of the distribution centers of chain supermarkets in China adopt the excessively simple activity-based classification (ABC) as the management method of classifying warehousing work, which often leads to an increase in operation and storage costs of the enterprise, a decrease in efficiency of the commodity circulation operation, and, eventually, a loss in enterprise growth because the ...
P. Zhou and L. Fan, A note on multi-criteria ABC inventory classification using weighted linear optimization, European Journal of Operational Research 182 (2007) 1488-1491. Crossref, Web of Science, Google Scholar; 7. W. L. Ng, A simple classifier for multiple criteria ABC analysis, European Journal of Operational Research 177 (2007) 344-353.
After more than 30 years of using Activity-Based Costing (ABC) to determine costs, just a few studies have analyzed its publications. This article aims to conduct a systematic, comprehensive literature review analyzing papers on ABC to overview what has been researched and give future research directions.
ABC Analysis as a Inventory Control Technique. PCT No. PCT/US98/05628 Sec. 371 Date Mar. 20, 1998 Sec. 102 (e) Date Mar. 20, 1998 PCT Filed Mar. 20, 1998 PCT Pub. No. WO99/09547 PCT Pub. Date Feb. 25, 1999A storage device for storing information includes a disc storage medium having a disc surface. An armature is positioned over the disc ...
ABC analysis is a popular categorization technique used in inventory optimization. It involves classifying stock-keeping units according to a certain criterion, most often by their importance or consumption value, sometimes also by their utilization frequency. The technique is based on the Pareto principle (or 80/20 rule), which states that ...
This paper proposes a novel method for calculating precise limits for the selection of parameter sets. ... Results As a typical example from biomedical research, the feasibility of the ABC analysis as an objective replacement for classical subjective limits to select highly relevant variance components of pain thresholds is presented. The ...
This paper is a thematic research review on the ABC System, its development, applications, challenges, and benefits. Several researchers claim that ABC is efficient in product pricing, cost-cutting strategy, and customer and profitability analysis. Meanwhile, Time-Driven ABC was introduced with the advantages of firm-wide application and lower ...
An inventory management is most commonly used technique to manage inventory efficiently. There are several techniques to classify the inventory items the most classification technique is ABC analysis. In ABC analysis the items are classified into A, B & C classes based on the total cost usage. Data collection is mainly of 1 year through the ...
In this thesis we consider a model of college hostel mess stores items (grocery and vegetables) for inventory management through ABC analysis. This research is composed of the following sections ...
The papers were published between 1980 and 2022 and were subjected to bibliometric analysis based on the most prolific contributors, references, countries, and keywords. CiteSpace software 6.1 R6 was applied to visualize information about seed orchard research.
Here, we show that founder personality traits are a significant feature of a firm's ultimate success. We draw upon detailed data about the success of a large-scale global sample of startups (n ...
Information about the Australian Broadcasting Corporation including history, management, corporate reports, plans and submissions and the latest news from our media centre.
John Wiley & Sons Inc is a publisher of academic journals. The company, better known as Wiley, is listed on the New York Stock Exchange (NYSE) and each year churns out more than 1,400 scientific ...
Peter Dutton's political survival as an opposition leader thus far is an impressive feat, but the issue of nuclear power could be one that damages his popularity with women, a new piece of ...
Electronic prescribing (e-prescribing) systems can bring many advantages and challenges. This system has been launched in Iran for more than two years. This study aimed to investigate the challenges and advantages of the e-prescribing system from the point of view of physicians. In this survey study and thematic analysis, which was conducted in 2023, a researcher-made questionnaire was created ...
In the process of addressing the challenge of meeting the criteria for completing the exploration of the Problem Statement and submitting a Research Paper for quantitative students to obtain their degree, one approach that can be adopted is the integration of a hybrid quantitative questionnaire alongside open-ended inquiries. Following the completion of the Pilot-Test, analyzing the open-ended ...
A development of the principles of activity based costing. (ABC) is activity based management (ABM). Operational ABM is defined as 'Actions, based on activity. driver analysis, that increase ...