- Physical Chemistry
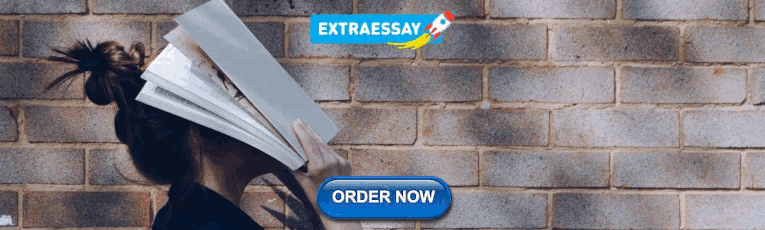
Enthalpy change of solution of hydrated magnesium sulphate
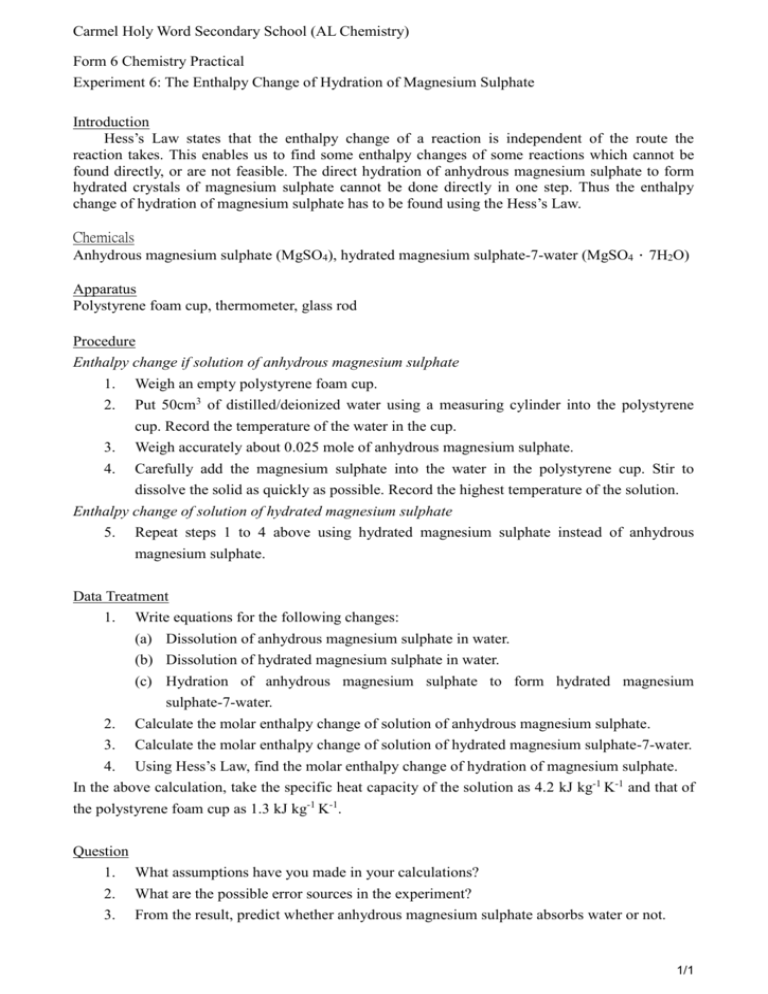
Related documents
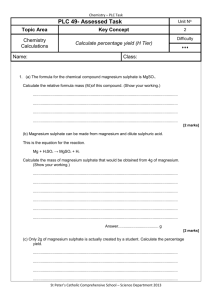
Add this document to collection(s)
You can add this document to your study collection(s)
Add this document to saved
You can add this document to your saved list
Suggest us how to improve StudyLib
(For complaints, use another form )
Input it if you want to receive answer

Solubility of Magnesium Sulphate: Unraveling the Science Behind It
The solubility of magnesium sulphate refers to the ability of this compound to dissolve in a solvent, typically water . Magnesium sulphate, also known as Epsom salt, is a white crystalline substance that is commonly used in various applications, including agriculture, medicine, and personal care products . Its solubility in water is an important factor to consider when using it for different purposes . Understanding the solubility of magnesium sulphate can help determine the appropriate concentrations for specific applications .
Key Takeaways
Understanding solubility.
Solubility refers to the ability of a substance , known as the solute , to dissolve in another substance , known as the solvent. It is an essential concept in chemistry that helps us understand how different substances interact with each other. In this section , we will explore the definition of solubility and the factors that affect it.
Definition of Solubility
Solubility is defined as the maximum amount of solute that can dissolve in a given amount of solvent at a specific temperature and pressure. It is usually expressed in terms of grams of solute per 100 grams of solvent, or in terms of moles of solute per liter of solvent.
When we talk about solubility, we often encounter terms like “magnesium sulphate in water” or “solubility rate .” Let’s take a closer look at these terms and understand their significance .
Magnesium Sulphate in Water
Magnesium sulphate, also known as Epsom salt, is a common example used to explain solubility. When we add magnesium sulphate crystals to water, they dissolve and form a clear, colorless solution . The solubility of magnesium sulphate in water is influenced by various factors, which we will discuss later.
Solubility Rate
The solubility rate refers to how quickly a solute dissolves in a solvent. Some substances dissolve rapidly, while others take longer. The rate of dissolution depends on factors such as the surface area of the solute , stirring or agitation, and the temperature of the solvent.
Factors Affecting Solubility
Several factors can influence the solubility of a solute in a solvent. Let’s explore some of the key factors that affect solubility:
Effect of Temperature on Solubility : In general, an increase in temperature leads to an increase in solubility for most solid solutes in liquid solvents . However, the effect of temperature on solubility can vary depending on the specific solute-solvent system . For example, the solubility of magnesium sulphate in water increases with temperature.
Nature of the Solute and Solvent : The nature of the solute and solvent plays a crucial role in determining solubility. Ionic compounds, such as magnesium sulphate, tend to have higher solubilities in polar solvents like water due to the attraction between the oppositely charged ions . On the other hand, nonpolar solvents like ethanol may have limited solubility for ionic compounds.
Solubility Curve and Solubility Product Constant : A solubility curve is a graphical representation of the solubility of a solute at different temperatures. It helps us understand the relationship between temperature and solubility. The solubility product constant (Ksp) is a measure of the extent to which a solute dissolves in a solvent and reaches a state of chemical equilibrium.
Saturated Solution : When the maximum amount of solute has dissolved in a solvent at a given temperature, the solution is said to be saturated. Any additional solute added will not dissolve and will form a precipitate . The solubility of magnesium sulphate in water can be determined by observing the point at which the solution becomes saturated.
Solubility Rules : Solubility rules are guidelines that help predict the solubility of salts in water. These rules are based on the general trends observed in the solubility of different ionic compounds . For example, most nitrate salts and alkali metal salts are soluble in water.
Solubility in Different Solvents : The solubility of magnesium sulphate can vary depending on the solvent used. While it is highly soluble in water, its solubility in other solvents like ethanol may be limited or even negligible.
Understanding solubility and the factors that influence it is crucial in various fields, including pharmaceuticals, environmental science , and chemical engineering . By studying solubility, scientists can predict the behavior of substances in different solutions and design processes that rely on the dissolution or precipitation of specific compounds .
Now that we have a better understanding of solubility and its definition , let’s delve deeper into the factors that affect solubility and explore their significance .
Magnesium Sulphate: An Overview
Magnesium sulphate, also known as Epsom salt, is a chemical compound with the formula MgSO4. It is a white crystalline solid that is commonly found in nature as a mineral . Magnesium sulphate has various applications in industries such as agriculture, medicine, and cosmetics due to its unique chemical and physical properties .
Chemical Properties of Magnesium Sulphate
Magnesium sulphate is an ionic compound composed of magnesium cations (Mg2+) and sulphate anions (SO42-). When dissolved in water, it dissociates into these ions, forming an aqueous solution . The solubility of magnesium sulphate in water is influenced by temperature. As the temperature increases , the solubility rate also increases. This relationship can be observed in the solubility curve of magnesium sulphate.
The dissolution process of magnesium sulphate involves the breaking of ionic bonds between magnesium and sulphate ions in the crystal lattice. This allows the ions to separate and disperse in the solvent, forming a homogeneous solution . The solubility product constant (Ksp) is a measure of the extent to which magnesium sulphate dissolves in water at a given temperature. It represents the equilibrium between the dissolved ions and the undissolved solid.
Physical Properties of Magnesium Sulphate
Magnesium sulphate exists in different forms , including anhydrous and hydrated crystals . The most common hydrated form is magnesium sulphate heptahydrate (MgSO4·7H2O), which has seven water molecules attached to each magnesium sulphate unit . These water molecules are called water of crystallization.
The presence of water molecules affects the physical properties of magnesium sulphate. Hydrated crystals are usually more soluble in water compared to anhydrous forms . The molar solubility of magnesium sulphate heptahydrate is higher than that of the anhydrous form due to the additional water molecules acting as solvents.
Magnesium sulphate exhibits a white crystalline appearance and is odorless. It has a bitter taste and is commonly used in bath salts and foot soaks for its therapeutic effects . The solubility of magnesium sulphate in ethanol is relatively low compared to its solubility in water.
Solubility of Magnesium Sulphate in Water
Why is magnesium sulphate soluble in water.
Magnesium sulphate, also known as Epsom salt, is a compound that exhibits solubility in water. This means that when magnesium sulphate is added to water, it dissolves and forms a homogeneous mixture . The solubility of magnesium sulphate in water is due to the nature of its chemical structure and the interaction s between its ions and water molecules.
Ionic compounds like magnesium sulphate are composed of positively charged ions (cations) and negatively charged ions (anions). In the case of magnesium sulphate, it consists of magnesium ions (Mg2+) and sulphate ions (SO42-). When these ions come into contact with water, the water molecules surround and separate the ions, allowing them to disperse evenly throughout the solution.
The dissolution process of magnesium sulphate in water involves the breaking of the ionic bonds between the magnesium and sulphate ions . As water molecules are polar, with a partial positive charge on the hydrogen atoms and a partial negative charge on the oxygen atom , they are able to attract and surround the ions. The positive hydrogen atoms of water molecules are attracted to the negatively charged sulphate ions , while the negative oxygen atoms are attracted to the positively charged magnesium ions .
Solubility of Magnesium Sulphate in Hot Water
The solubility of magnesium sulphate in water is influenced by temperature. Generally, an increase in temperature leads to an increase in the solubility rate of most solid solutes, including magnesium sulphate. This means that more magnesium sulphate can dissolve in hot water compared to cold water.
To illustrate the effect of temperature on the solubility of magnesium sulphate, we can refer to a solubility curve. A solubility curve is a graphical representation of the relationship between temperature and the maximum amount of solute that can dissolve in a solvent to form a saturated solution. For magnesium sulphate, the solubility curve shows that as temperature increases , the solubility of magnesium sulphate in water also increases.
It is important to note that the solubility of magnesium sulphate in water is not unlimited. There is a point at which no more magnesium sulphate can dissolve in the water , resulting in a saturated solution. At this point , the rate of dissolution is equal to the rate of precipitation, establishing a chemical equilibrium .
In addition to water, magnesium sulphate can also exhibit solubility in other solvents such as ethanol. However, the solubility of magnesium sulphate in ethanol is generally lower compared to its solubility in water.
Solubility of Magnesium Sulphate in Different Solvents
Solubility of magnesium sulphate in acetone.
When it comes to the solubility of magnesium sulphate in different solvents, acetone is an interesting one to explore. Acetone is a commonly used organic solvent that is highly miscible with water. However, when it comes to magnesium sulphate, its solubility in acetone is quite low. This means that only a small amount of magnesium sulphate can dissolve in acetone. The solubility rate of magnesium sulphate in acetone is significantly lower compared to its solubility in water.
Solubility of Magnesium Sulphate in Ether
Ether is another solvent that is often used in laboratories for various purposes . When it comes to the solubility of magnesium sulphate, it is important to note that ether is not a suitable solvent for dissolving this compound. Magnesium sulphate has a very low solubility in ether, which means that only a small amount of it can dissolve in this solvent . The solubility rate of magnesium sulphate in ether is much lower compared to its solubility in water.
Solubility of Magnesium Sulphate in Organic Solvents
Apart from acetone and ether, there are various other organic solvents that can be used to dissolve magnesium sulphate. However, it is important to consider the solubility of magnesium sulphate in these solvents . Organic solvents , such as ethanol, methanol, and chloroform, have different solubility properties compared to water. In the case of magnesium sulphate, its solubility in organic solvents is generally lower than in water. This means that only a limited amount of magnesium sulphate can dissolve in these solvents .
It is worth noting that the solubility of magnesium sulphate in different solvents can be influenced by various factors, such as temperature and the presence of other solutes . The solubility of ionic compounds, like magnesium sulphate, is generally affected by the dissolution process , which involves the separation of the compound’s ions in the solvent. The solubility curve of magnesium sulphate can be determined by measuring the amount of the compound that can dissolve at different temperatures. The solubility product constant can also be used to calculate the solubility of magnesium sulphate in a given solvent at a specific temperature.
In chemical equilibrium, a saturated solution is formed when the rate of dissolution of magnesium sulphate crystals is equal to the rate of precipitation. This means that the concentration of magnesium sulphate in the solution remains constant over time. The solubility of magnesium sulphate heptahydrate, which is the most common form of the compound, can be expressed in terms of its molar solubility .
Overall, the solubility of magnesium sulphate in different solvents is an important aspect to consider in various applications, such as in the preparation of aqueous solutions and in precipitation reactions. Understanding the solubility of salts, like magnesium sulphate, in different solvents is crucial for conducting experiments and ensuring accurate results .
Apparent Solubility of Magnesium Sulphate
Magnesium sulphate, also known as Epsom salt, is an ionic compound that readily dissolves in water. The apparent solubility of magnesium sulphate refers to the maximum amount of the compound that can dissolve in a given amount of solvent under specific conditions . Understanding the factors affecting the apparent solubility of magnesium sulphate can provide insights into its dissolution process and its behavior in different solvents.
Factors Affecting the Apparent Solubility of Magnesium Sulphate
Several factors influence the apparent solubility of magnesium sulphate. Let’s take a closer look at each of these factors :
Effect of Temperature : The solubility rate of magnesium sulphate in water is temperature-dependent. Generally, an increase in temperature leads to an increase in solubility. This is because higher temperatures provide more energy for the dissolution process , allowing more magnesium sulphate crystals to break down and mix with the solvent.
Solubility Curve : The solubility of magnesium sulphate can be represented by a solubility curve, which shows the relationship between temperature and the maximum amount of the compound that can dissolve in water. The curve typically exhibits an upward trend , indicating an increase in solubility with temperature.
Solubility Product Constant : The solubility product constant (Ksp) is a measure of the equilibrium between the dissolved ions and the undissolved solid in a saturated solution. For magnesium sulphate, the Ksp value determines the maximum concentration of magnesium and sulphate ions that can exist in a saturated solution at a given temperature.
Chemical Equilibrium : The apparent solubility of magnesium sulphate is influenced by the chemical equilibrium between the dissolved ions and the undissolved solid. When the dissolution process reaches equilibrium, the rate of dissolution equals the rate of precipitation, resulting in a saturated solution.
Solute and Solvent : The solubility of magnesium sulphate is primarily determined by the interaction between the solute (magnesium sulphate) and the solvent (water). The polar nature of water molecules allows them to effectively dissolve the ionic compound by surrounding the magnesium and sulphate ions , breaking their crystal lattice structure .
Solubility of Salts : Magnesium sulphate is a salt , and its solubility follows the general solubility rules for salts. These rules state that most salts are more soluble in hot water than in cold water. However, there are exceptions, and the solubility of magnesium sulphate in different solvents, such as ethanol, may vary.
Differences between Actual and Apparent Solubility
The apparent solubility of magnesium sulphate refers to the maximum amount of the compound that can dissolve in a given amount of solvent under specific conditions . However, it is important to note that the actual solubility may differ from the apparent solubility due to various factors.
One such factor is the presence of impurities or other dissolved substances in the solvent. These impurities can affect the solubility of magnesium sulphate by either increasing or decreasing its apparent solubility . Additionally, the presence of other ions in the solution can lead to the formation of insoluble compounds through precipitation reactions, further affecting the solubility of magnesium sulphate.
It is also worth mentioning that the apparent solubility of magnesium sulphate may change over time. This is because the dissolution process is dynamic, with ions continuously moving between the dissolved and undissolved states . As a result , the concentration of dissolved magnesium and sulphate ions in the solution may fluctuate, leading to changes in the apparent solubility.
Solubility Curve of Magnesium Sulphate
Understanding the solubility curve.
The solubility curve of magnesium sulphate in water provides valuable insights into the solubility rate of this ionic compound at different temperatures. Solubility refers to the ability of a solute, in this case , magnesium sulphate, to dissolve in a solvent, which is water in this context . The solubility curve graphically represents the relationship between temperature and the maximum amount of solute that can dissolve in a given amount of solvent.
When magnesium sulphate crystals are added to water, they undergo a dissolution process where the ionic compound dissociates into its constituent ions , magnesium (Mg2+) and sulphate (SO42-). The solubility curve illustrates the solubility product constant , which is a measure of the concentration of these ions in a saturated solution at a specific temperature. At equilibrium, the rate of dissolution of magnesium sulphate crystals is equal to the rate of precipitation, resulting in a saturated solution.
Factors Influencing the Solubility Curve of Magnesium Sulphate
Several factors influence the solubility curve of magnesium sulphate. Let’s explore some of the key factors :
Effect of Temperature: The solubility of most salts, including magnesium sulphate, generally increases with an increase in temperature. As the temperature rises, the kinetic energy of the particles increases, leading to more collisions between the solute and solvent particles. This increased collision rate enhances the dissolution of magnesium sulphate crystals, resulting in a higher solubility .
Solubility Rules : The solubility of salts, including magnesium sulphate, can also be influenced by solubility rules . These rules provide guidelines for predicting the solubility of various compounds based on their chemical properties . For example, magnesium sulphate is soluble in water, but insoluble in ethanol.
Solvent Selection: The choice of solvent can significantly impact the solubility of magnesium sulphate. Different solvents have varying polarities and interactions with the solute particles. For instance, magnesium sulphate exhibits different solubility in water compared to other solvents like ethanol. This variation in solubility is due to the difference s in the intermolecular forces between the solute and solvent molecules.
Precipitation Reactions : The solubility curve of magnesium sulphate is also influenced by precipitation reactions. When two solutions containing ions that can form an insoluble compound , such as magnesium sulphate, are mixed, a precipitation reaction occurs. This reaction reduces the solubility of magnesium sulphate and leads to the formation of a solid precipitate .
Understanding the solubility curve of magnesium sulphate and the factors influencing it is crucial in various scientific and industrial applications . By studying the solubility behavior of this compound, scientists and researchers can make informed decisions regarding its use in different processes and formulations.
Solubility of Anhydrous Magnesium Sulphate
What is anhydrous magnesium sulphate.
Anhydrous Magnesium Sulphate is a chemical compound with the formula MgSO4. It is an ionic compound composed of magnesium cations (Mg2+) and sulphate anions (SO4^2-). The term “anhydrous” indicates that it does not contain any water molecules in its crystal structure . Anhydrous Magnesium Sulphate is a white, crystalline solid that is commonly used in various industrial and laboratory applications .
Solubility Characteristics of Anhydrous Magnesium Sulphate
The solubility of Anhydrous Magnesium Sulphate in water is an important property to understand its behavior in different conditions . The solubility rate of this compound is influenced by several factors , including temperature and the presence of other solutes .
Effect of Temperature on Solubility
The solubility of Anhydrous Magnesium Sulphate in water increases with an increase in temperature. As the temperature rises, more energy is available to break the ionic bonds holding the compound together, allowing it to dissolve more readily. This relationship between temperature and solubility can be observed by constructing a solubility curve, which shows the maximum amount of solute that can dissolve at different temperatures.
Dissolution Process
When Anhydrous Magnesium Sulphate is added to water, the dissolution process begins. The water molecules surround the magnesium and sulphate ions , pulling them away from the crystal lattice and into the solution. This process continues until the solution reaches a state of equilibrium, where the rate of dissolution is equal to the rate of precipitation.
Solubility Product Constant
The solubility of Anhydrous Magnesium Sulphate can be quantified using the solubility product constant (Ksp). This constant represents the equilibrium expression for the dissolution of the compound in water. The value of Ksp depends on the temperature and provides information about the extent to which the compound can dissolve in water.
Saturated Solution
A saturated solution of Anhydrous Magnesium Sulphate is a solution in which no more solute can be dissolved at a given temperature. At this point , the solution is in a state of dynamic equilibrium , with the dissolved ions continuously being formed and precipitated. The concentration of the solute in a saturated solution is known as the molar solubility .
Solubility in Different Solvents
Apart from water, Anhydrous Magnesium Sulphate has different solubility characteristics in various solvents . For example, it has limited solubility in ethanol, meaning it does not dissolve as readily in ethanol as it does in water. The solubility of magnesium sulphate in different solvents is influenced by the polarity and interactions between the solute and solvent molecules.
Understanding the solubility of Anhydrous Magnesium Sulphate is essential for various applications, such as in pharmaceutical formulations , agriculture, and chemical reactions . By considering factors like temperature and solvent choice , scientists and researchers can manipulate the solubility of this compound to achieve desired outcomes in their experiments and processes.
What is the effect of magnesium sulfate on the solubility of methanol?
In order to understand the intersection between the solubility of magnesium sulfate and methanol, it is important to investigate the effect of the former on the latter. “Methanol’s Solubility: A Comprehensive Study” provides a comprehensive analysis of the solubility of methanol. This study examines various factors that can influence the solubility of methanol, including the presence of other substances such as magnesium sulfate. To explore this further, please refer to “Methanol’s Solubility: A Comprehensive Study” .
Frequently Asked Questions
Why is mgso4 soluble in water.
Magnesium sulphate (MgSO4) is soluble in water because it is an ionic compound. When it is added to water, the polar water molecules are attracted to the ions of MgSO4, causing it to dissociate into Mg2+ and SO4 2- ions. This process is known as dissolution.
What is the solubility rate of magnesium sulphate?
The solubility rate of magnesium sulphate depends on various factors such as temperature and the presence of other substances . In general, the solubility of magnesium sulphate in water at 20°C is approximately 26.9 grams per 100 grams of water.
How does temperature affect the solubility of magnesium sulphate?
The solubility of magnesium sulphate increases with temperature. This is because the energy provided by the heat increases the kinetic energy of the water molecules, thereby increasing the rate of dissolution.
What is the solubility of magnesium sulphate in acetone?
The solubility of magnesium sulphate in acetone is considerably less than in water due to the difference in polarity between the two solvents . Acetone’s polarity is less than water’s, which makes it a less effective solvent for ionic compounds like magnesium sulphate.
What is the solubility curve of magnesium sulphate?
A solubility curve is a graphical representation of the solubility of a solute as a function of temperature. For magnesium sulphate, the curve typically shows that solubility increases with temperature.
What is the solubility of magnesium sulphate in ether?
Magnesium sulphate is not very soluble in ether. This is because ether is a nonpolar solvent , and ionic compounds like magnesium sulphate dissolve best in polar solvents.
How does the solubility of anhydrous magnesium sulphate differ from that of magnesium sulphate heptahydrate?
Anhydrous magnesium sulphate has a lower solubility than magnesium sulphate heptahydrate. This is because the water molecules in the heptahydrate form are already in the crystal lattice, making it easier for the compound to dissolve in water.
Is magnesium sulphate soluble in organic solvents?
Magnesium sulphate has limited solubility in organic solvents . Its solubility is generally higher in polar solvents, like water, due to its ionic nature .
What is the molar solubility of magnesium sulphate?
The molar solubility of magnesium sulphate can be determined using the solubility product constant (Ksp). It refers to the maximum amount of MgSO4 that can dissolve in a liter of water to form a saturated solution.
How does the dissolution of magnesium sulphate affect chemical equilibrium?
The dissolution of magnesium sulphate in water results in the formation of a dynamic equilibrium between the dissolved ions and the undissolved solid. The position of this equilibrium can be described by the solubility product constant (Ksp), which remains constant at a given temperature.
- Ascorbic acid solubility
- Potassium nitrate solubility
- Solubility of butanol
- Fecl3 solubility
- Magnesium oxide solubility
- Koh solubility
- Solubility of calcium hydroxide
- Solubility of mgcl2
- Potassium chloride solubility
- Solubility of caso4 with temperature

The TechieScience Core SME Team is a group of experienced subject matter experts from diverse scientific and technical fields including Physics, Chemistry, Technology,Electronics & Electrical Engineering, Automotive, Mechanical Engineering. Our team collaborates to create high-quality, well-researched articles on a wide range of science and technology topics for the TechieScience.com website.
All Our Senior SME are having more than 7 Years of experience in the respective fields . They are either Working Industry Professionals or assocaited With different Universities. Refer Our Authors Page to get to know About our Core SMEs.
Laboratory Notes
Preparation of 1M Magnesium Sulfate (MgSO4) Stock Solution
Magnesium sulfate (MgSO4) is soluble in water. Magnesium sulfate is available in anhydrous as well as hydrated forms. Since anhydrous Magnesium sulfate is highly hygroscopic (quickly absorbs water from the air), it is difficult to weigh it accurately, therefore, is not used for preparing solution. Magnesium sulfate heptahydrate (MgSO4·7H2O, Mol Wt: 246.47) is quite stable and often used for the preparation of 1M Magnesium sulfate solution.
A 1M stock solution can be prepared by dissolving 24.6.47 g of Magnesium sulfate heptahydrate (MgSO4.7H2O, Mol Wt: 246.47) in water to a final volume of 1000 ml.
REQUIREMENTS
Reagents and solutions Magnesium sulfate (MgSO4.7H2O) Deionized / Distilled water
Equipment and disposables Measuring cylinder Conical flask / Beaker
Composition 1 M Magnesium sulfate
Objective Preparation of 100 ml 1 M Magnesium sulfate
Step 1: To prepare 100 ml of 1 M Magnesium sulfate, weigh out 24.647 g Magnesium sulfate heptahydrate (MgSO4·7H2O, Mol Wt: 246.47) in a 250 ml conical flask/beaker. Add 800 ml deionized/Milli-Q water and mix until dissolved completely.
Tip: One can use manual shaking using a glass pipette to mix the ingredients. Magnetic stirrer makes the dissolving process easy and convenient.
Precaution: Do not dissolve in 100 ml of deionized / Milli-Q water. In most cases, solution volume increases when a large amount of solute is dissolved in a solvent.
Step 2: Adjust the volume to 100 ml with deionized / Milli-Q water. Mix it again.
Step 3: Sterilize the solution by autoclaving (20 minutes at 15 lb/sq.in. (psi) from 121-124°C on liquid cycle).
Note: The solution can also be sterilized by filtering it through a filter unit containing a membrane with a pore size of 0.22µm or less under sterile conditions.
Storage The solution can be stored in the cold room.
Was this post helpful?
2 thoughts on “ Preparation of 1M Magnesium Sulfate (MgSO4) Stock Solution ”
In the table: 0.1 M 0.246 g 2.46 g 30.80 g (it should be 12.3)
Leave a Reply Cancel reply
Your email address will not be published. Required fields are marked *
Save my name, email, and website in this browser for the next time I comment.
Recent Posts
Copyright © 2024 Laboratory Notes
Design by ThemesDNA.com

- Periodic table of the elements
- Chemistry calculators
- Image gallery
PREPARATION OF SOLUTIONS
- List of abbreviations and acronyms
- List of chemical compounds
What you want?
Desired solution:
Final volume:
Desired concentration:
What you have?
Molar mass = 246.47496 g/mol
Purity of solid compound:
Source concentration:
You need ...
Mass of solid compound:
Volume of stock solution:
- Labeling of chemical containers
- Laboratory report with calculations
= Short Lab Report =
September 6, 2016
- Transfer the prepared solution to a clean, dry storage bottle and label it. Never store solutions in a volumetric flask.
- All chemicals that you are unfamiliar with should be treated with extreme care and assumed to be highly flammable and toxic.
- We provide the calculator as a public service only and you use it at your own risk. If you don't understand the results, DON'T use them.
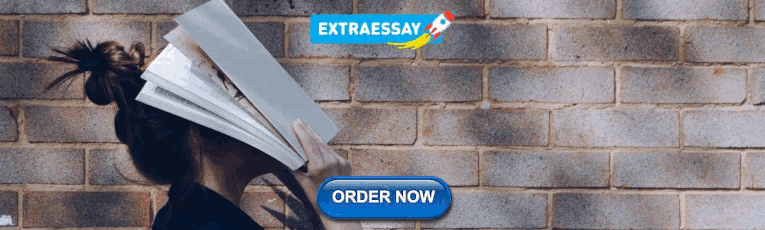
Bibliography:
- J. Meija et al., Atomic Weights of the Elements 2013 . Pure Appl. Chem. 88 , 265-291 (2016)
- D.W. Green, R.H. Perry, "Densities of Aqueous Inorganic Solutions", Perry's Chemical Engineers' Handbook , McGraw-Hill, (2) 99-118, 2008.
- "GESTIS Substance Database." IFA . 23 Sep. 2015. < http://www.dguv.de/ifa/gestis/gestis-stoffdatenbank/index-2.jsp >.
- "Regulation (EC) No 1272/2008 Classification, packaging and labelling of chemicals and their mixtures." 16 Dec. 2008. European Parliament, Council of the European Union . 28 Nov. 2015. < http://eur-lex.europa.eu/legal-content/EN/TXT/?uri=CELEX:32008R1272 >.
- Information for Authors . JOC. ACS Publications. 21 Sep. 2012. < http://pubs.acs.org/page/joceah/submission/authors.html >.

Back to top ↑
Citing this page:
Generalic, Eni. "Preparation of Solutions." EniG. Periodic Table of the Elements . KTF-Split, 18 Jan. 2024. Web. {Date of access} . <https://www.periodni.com/preparation_of_solutions.php>.

- Online calculators
- Scientific calculator
- Preparation of solutions
- Oxidation numbers calculator
- Balancing redox reactions
- Memory game
- Articles and tables
- Chemistry dictionary
- Printable periodic table
- Portable applications
- Chemistry images gallery
- Short form of the periodic table
- Long form of the periodic table
- History of the Periodic table of elements
- Electronic configurations of the elements
- Alphabetical list of chemical elements
- Naming of elements of atomic numbers greater than 100
- ASCII Periodic table
- Scientific calculator for chemists
- Gas laws calculator
- Molar mass calculator
- Angle converter
- Roman numerals converter
- Number systems converter
- Oxidation number change method
- Ion-electron method
- Gauss elimination method
- Find the pairs
- Crystal systems and Bravais lattices
- GHS - Hazard pictograms
- NFPA 704 Hazard Diamond
- Fundamental physical constants
- Solubility product constants
- SI - International System of Units
- Composition of mixtures and solutions
- Stoichiometric calculations
- Chlorinity and salinity of seawater
- Rare earth elements (REE)
- Climate change
- Global warming and mankind
- Story of ozone and ozone holes
- World War 3: Battle for Earth
- The ozone layer is not a shield
- Hexadecimal color codes
- Writing equations on the Web
- Writing chemical equations on the Web
- Character entity references in HTML
- Unicode UTF-8 encoding
- Download PDF Documents
- Download Software
- Download Images
- Periodic table

- Français
- Español
- Search on periodni.com

- school Campus Bookshelves
- menu_book Bookshelves
- perm_media Learning Objects
- login Login
- how_to_reg Request Instructor Account
- hub Instructor Commons
Margin Size
- Download Page (PDF)
- Download Full Book (PDF)
- Periodic Table
- Physics Constants
- Scientific Calculator
- Reference & Cite
- Tools expand_more
- Readability
selected template will load here
This action is not available.
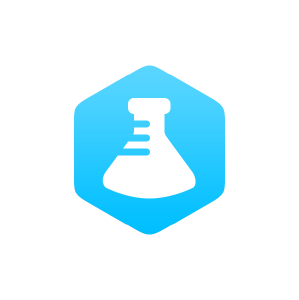
1.8: Experiment 7 - Precipitation
- Last updated
- Save as PDF
- Page ID 255065
\( \newcommand{\vecs}[1]{\overset { \scriptstyle \rightharpoonup} {\mathbf{#1}} } \)
\( \newcommand{\vecd}[1]{\overset{-\!-\!\rightharpoonup}{\vphantom{a}\smash {#1}}} \)
\( \newcommand{\id}{\mathrm{id}}\) \( \newcommand{\Span}{\mathrm{span}}\)
( \newcommand{\kernel}{\mathrm{null}\,}\) \( \newcommand{\range}{\mathrm{range}\,}\)
\( \newcommand{\RealPart}{\mathrm{Re}}\) \( \newcommand{\ImaginaryPart}{\mathrm{Im}}\)
\( \newcommand{\Argument}{\mathrm{Arg}}\) \( \newcommand{\norm}[1]{\| #1 \|}\)
\( \newcommand{\inner}[2]{\langle #1, #2 \rangle}\)
\( \newcommand{\Span}{\mathrm{span}}\)
\( \newcommand{\id}{\mathrm{id}}\)
\( \newcommand{\kernel}{\mathrm{null}\,}\)
\( \newcommand{\range}{\mathrm{range}\,}\)
\( \newcommand{\RealPart}{\mathrm{Re}}\)
\( \newcommand{\ImaginaryPart}{\mathrm{Im}}\)
\( \newcommand{\Argument}{\mathrm{Arg}}\)
\( \newcommand{\norm}[1]{\| #1 \|}\)
\( \newcommand{\Span}{\mathrm{span}}\) \( \newcommand{\AA}{\unicode[.8,0]{x212B}}\)
\( \newcommand{\vectorA}[1]{\vec{#1}} % arrow\)
\( \newcommand{\vectorAt}[1]{\vec{\text{#1}}} % arrow\)
\( \newcommand{\vectorB}[1]{\overset { \scriptstyle \rightharpoonup} {\mathbf{#1}} } \)
\( \newcommand{\vectorC}[1]{\textbf{#1}} \)
\( \newcommand{\vectorD}[1]{\overrightarrow{#1}} \)
\( \newcommand{\vectorDt}[1]{\overrightarrow{\text{#1}}} \)
\( \newcommand{\vectE}[1]{\overset{-\!-\!\rightharpoonup}{\vphantom{a}\smash{\mathbf {#1}}}} \)
Learning Objectives
By the end of this lab, students should be able to:
- Describe precipitation reactions from the molecular perspective
- Record detailed observations for a reaction.
- Predict if a precipitate will form when combining two solutions.
- Predict when a chemical reaction will result in the formation of a gas.
- Write molecular, ionic, and net ionic equations for various reactions.
- Design an experiment to determine the concentration of a known solution of an ionic species utilizing solubility rules and stoichiometry.
- Calculate the concentration of a solution based on experimental (gravimetric) data.
Prior Knowledge:
- 3.1: Introduction to Chemical Equations
- 3.2: Types of Chemical Reactions
- 3.3: Balancing Chemical Equations
- 3.4: Aqueous Reactions
Aqueous solution is any solution where water is present as a solvent. Rain, vinegar, orange juice are all examples of aqueous solutions that you come across in your everyday life. In chemistry aqueous solution indicated by adding "(aq)" to the reactant formula. For example, NaCl(aq) present as individual ions Na + and Cl - dissolved in water. You might have heard that water is the universal solvent, however, water only dissolves substances that are hydrophilic (from the Greek "hydros" - water and "philia" - bonding or friendship). Compounds that do not dissolve in water remain a solid and indicated by "(s)". For example, AgCl(s).
- Magnesium sulfate
- Calcium chloride
- Copper (II) Sulfate
- Sodium bicarbonate (baking soda)
- Vinegar (~5% acidity)
- Scale
- Clean and dry test tubes
- Cell phone with camera
- Laptop or computer with camera, speakers and microphone hooked up to internet
Solubility rules could be useful in our everyday life, but they are also extremely important in medicine. Sometimes doctors prescribe more than one solution to be administered by the intravenous (IV) route. Mixing two solutions that form a precipitate can lead to very serious consequences. For example, magnesium sulfate is used as an electrolyte replenisher or anticonvulsant, calcium chloride is indicated in the immediate treatment of hypocalcemic tetany (abnormally low levels of calcium in the body that cause muscle spasm) and intravenous sodium bicarbonate is a medication primarily used to treat severe metabolic acidosis. But what will happen if you mix them?
Safety if you were to complete this lab in person:
- Obtain and wear goggles and gloves!!!
- Do non ingest any chemicals or inhale the vapors.
- Clean up all spills immediately! If contact with skin rinse with water for 15 minutes.
- Before proceeding with this or any other experiment students must sign the chemical lab safety form.
For each reaction in Part A and Part B record your observations, molecular equation , total ionic equation and net ionic equation . Make sure to write any evidence of a chemical reaction with sufficient detail to help you distinguish between similar precipitation reactions. Don't write “became cloudy” or “white solid”. Indicate if a gel is produced or crystals form, if the solid was powdery, etc. Keep in mind that some reactions will not occur and you should write NR (for No Reaction). You will know that a reaction occur if a precipitate, a gas, or color change occurred. Heat (whether it was consumed or evolved) can also be an indicator that reaction occurred, but you may not be able to tell in these videos.
Make sure to write any evidence of any evidence of a chemical reaction with sufficient detail to help you distinguish between similar precipitation reactions.
Reactions Worksheet
Part A. Precipitation Reaction Simulation
Using the simulation below, answer the questions on your worksheet.
Part B. Predictions using the solubility table
Using this solubility table predict what happens in the reactions in part B.
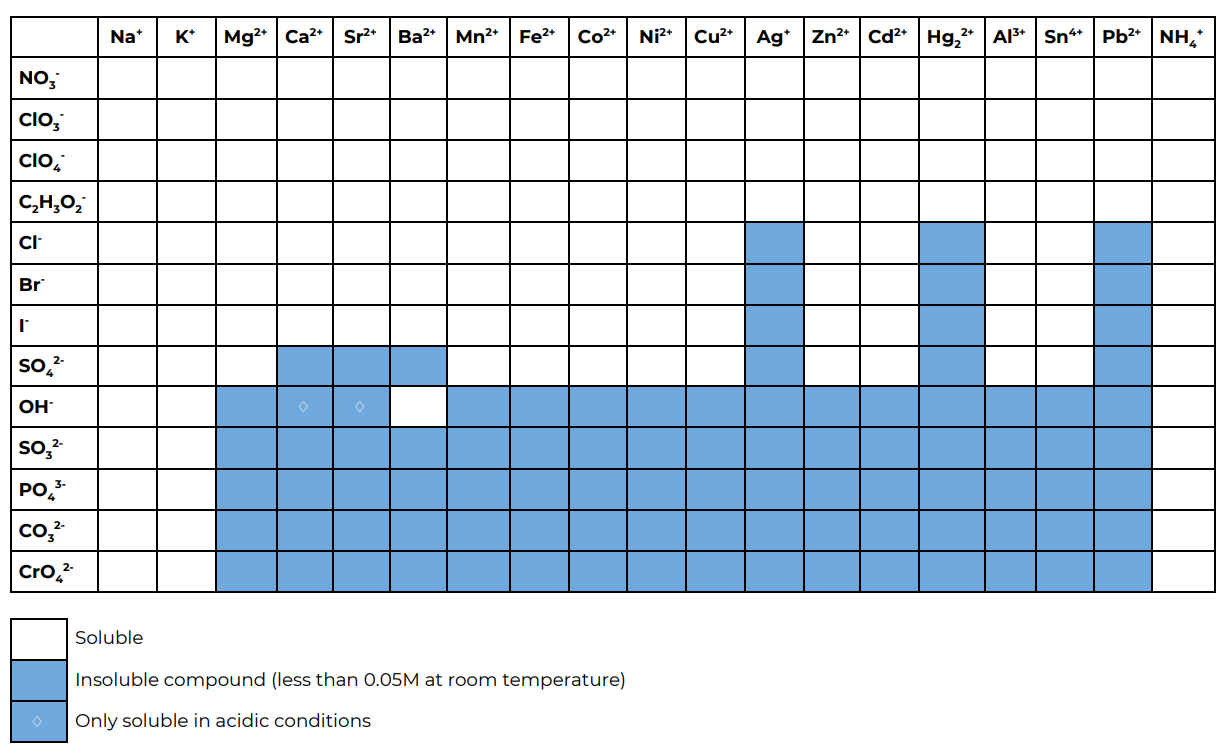
Interactive Element
Group Worksheet
Before watching each video predict the outcome of the reaction (formation of gas (CO 2 or H 2 ) or precipitate, or no reaction). Then watch the video and on your worksheet write down 1) your observations, 2) molecular equation, 3) ionic equation and 4) net ionic equation for each reaction before moving to the next video.
1. Copper(II)* sulfate and sodium phosphate
* The video says Cu 2 SO 4 , but the reaction shown in this video is between copper (II) sulfate and sodium phosphate.
2. Cadmium (II) chloride and sodium sulfide
3. Nickel (II) chloride and sodium carbonate
4. Lead (II) nitrate** and sodium sulfide
** The video says Pb 2 NO 3 , but the reaction shown is between lead (II) nitrate and sodium sulfide
5. Magnesium and hydrochloric acid
6. Nickel(II) chloride and sodium phosphate
7. Silver nitrate and sodium carbonate
Virtual Lab
Use the Gravimetric Analysis Virtual Lab for this assignment. Read Part I, then work in groups to complete Part II. Part III is your individual assignment and every group member will have their own unknown. You should receive an email from the TA with the unknown solution you need to use for the individual section of this assignment.
NOTE: If virtual lab says "Default Lab Setup" refresh your page
it should say: "Gravimetric Analysis of Unknown Lead Solutions"
Virtual Lab \(\PageIndex{1}\): ChemCollective Virtual Lab developed by Dave Yaron of Carnegie Mellon University, ( http://www.chemcollective.org/ ). NOTE: if the lab loads as "Default Lab Setup" (with a bunch of acids, bases and indicators), refresh the page . You want it to load the "Gravimetric Analysis of Unknown Leas Solutions", which contains sodium chloride, potassium chromate and six unknowns.
Contributors and Attributions
Robert E. Belford (University of Arkansas Little Rock; Department of Chemistry) led the creation of this page for a 5 week summer course.
Elena Lisitsyna contributed to the creation and implementation of this page, including the generation of the HP5 question modules.
- Mark Baillie coordinated the modifications of this activity for implementation in a 15 week fall course, with the help of Elena Lisitsyna and Karie Sanford.
- Chemical Compound Formulas
Magnesium sulfate - MgSO 4
What is magnesium sulfate (mgso 4 ).
MgSO 4 is an inorganic salt with a chemical name Magnesium sulfate. It is also known as Sulfuric acid magnesium salt or Magnesium sulfate anhydrous. It is commonly referred to as Epsom salt. Magnesium sulfate is a crystalline solid that has a white appearance and is odourless. It is majorly used as a bath soak to get rid of sore muscles, to ease the pain of sprains and bruises, etc. It also helps in the removal of splinters and is quite effective.
A magnesium salt with sulphate is known as magnesium sulphate. It functions as a calcium channel blocker, anaesthetic, tocolytic, anti-arrhythmia, analgesic, fertiliser, anticonvulsant, cardiovascular medication, analgesic, and all of the above. It consists of a metal sulphate, an organic magnesium salt, and a magnesium salt.
Table of Contents
- Properties of Magnesium sulfate-MgSO4
- Structure of MgSO4 Molecule
Uses of Magnesium Sulfate
Health hazards associated with magnesium sulfate.
- Frequently Asked Questions – FAQs
Properties of Magnesium sulfate – MgSO 4
It is important to note that anhydrous magnesium sulfate undergoes decomposition at temperatures above 1124 degrees Celsius. Since MgSO 4 is an ionic salt, it exhibits high solubility in water. It can also be noted that the solubility of magnesium sulfate in water increases when the temperature is increased. For example, the solubility of anhydrous MgSO 4 in water at a temperature of O o C is 269 grams per litre. When the temperature is increased to 100 o C, the solubility of this ionic salt in water almost doubles to 502 grams per litre.
Magnesium sulfate is known to be a component of many double salts (ionic salts containing more than one cation or more than one anion). Commonly known double salts containing MgSO 4 include potassium magnesium sulfates and sodium magnesium sulfates. Under standard conditions for temperature and pressure (STP), MgSO 4 exists as a white crystalline solid which does not have any characteristic odour.
Structure of MgSO 4 Molecules
The structure of a magnesium sulfate molecule is illustrated below. Note that MgSO 4 molecules contain one Mg 2+ cation (the magnesium ion) and one SO 4 2- anion (the sulfate anion).
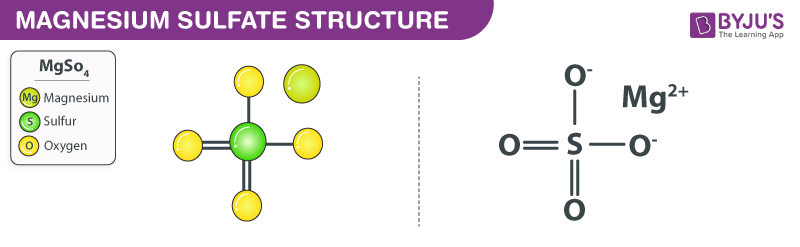
An ionic bond is formed between the magnesium cation and the sulfate anion in magnesium sulfate. In the sulfate anion, there exist two sulphur-oxygen double bonds and two sulphur-oxygen single bonds. The oxygen atoms that are singly bonded to the sulphur atom each hold a negative charge of magnitude -1. Therefore, the total charge on the sulphate anion is -2. The charge on the magnesium cation has a magnitude of +2. Therefore, the positive and negative charges in this ionic compound cancel each other out, resulting in a neutrally charged magnesium sulphate molecule. However, when this compound is dissolved in water and other polar solvents, the magnesium and the sulphate ions dissociate.
- It is used in agriculture to increase the magnesium and sulphur content in the soil.
- It is used in making beer as a brewing salt.
- It is used as a coagulant to make tofu.
- It is used in organic synthesis as a desiccant.
- Consumption of magnesium sulfate orally as osmotic purgative or saline laxative.
- Medically it is used in IV (intravenously) to control seizures in pregnant women.
One of the most important applications of magnesium sulfate is in the preparation of intravenous magnesium, a vital medication for the treatment of eclampsia. It is also used in replacement therapies for the treatment of magnesium deficiency. It can also be noted that magnesium sulfate can be employed as a coagulating agent for the preparation of certain food items like tofu.
This compound is dangerous when used in a higher dose than that is recommended by your doctor or instructed on the package. A high dosage of this compound causes serious side effects and can also be life-threatening. Abnormally high concentrations of magnesium in plasma can result in a medical condition known as hypermagnesemia.
Frequently Asked Questions
Is magnesium sulfate a compound or mixture.
Magnesium sulfate, MgSO4, is a colourless crystalline substance formed by the sulphur dioxide and air reaction of magnesium hydroxide.
Is magnesium sulfate soluble in alcohol?
Magnesium sulfate heptahydrate is a white, crystalline, or brilliant, usually needle-like crystals. It is readily soluble in water, in boiling water more easily soluble, and in alcohol virtually insoluble.
What is the hydrating formula for MgSO 4
To obtain the mole ratio, divide the moles of water by moles of anhydrate. 5 H2O/1 mole MgSO 4 moles = 5:1. To write the equation, use the mole ratio. Because for every 1 mole of MgSO 4 there are 5 moles of H2O, the formula is 5H 2 O MgSO 4 .
What is magnesium sulfate heptahydrate used for?
One of the main applications of magnesium sulfate is to be used as a fertilizer in farming and gardening. For bath salts, magnesium sulfate is used.
How moles are calculated?
Use the molecular formula to calculate the molar mass; divide the weight of the compound by the compound’s molar mass measured in grams to obtain the number of moles.
To explore more about the structure, physical and chemical properties of Magnesium sulfate (MgSO 4 ) from the experts, register with BYJU’S now!
Other related links:
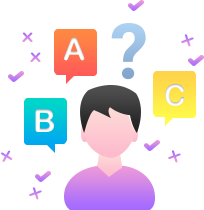
Put your understanding of this concept to test by answering a few MCQs. Click ‘Start Quiz’ to begin!
Select the correct answer and click on the “Finish” button Check your score and answers at the end of the quiz
Visit BYJU’S for all Chemistry related queries and study materials
Your result is as below
Request OTP on Voice Call
Leave a Comment Cancel reply
Your Mobile number and Email id will not be published. Required fields are marked *
Post My Comment

- Share Share
Register with BYJU'S & Download Free PDFs
Register with byju's & watch live videos.

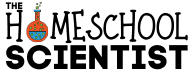
Endothermic Chemical Reaction Science Activity
Sharing is caring!
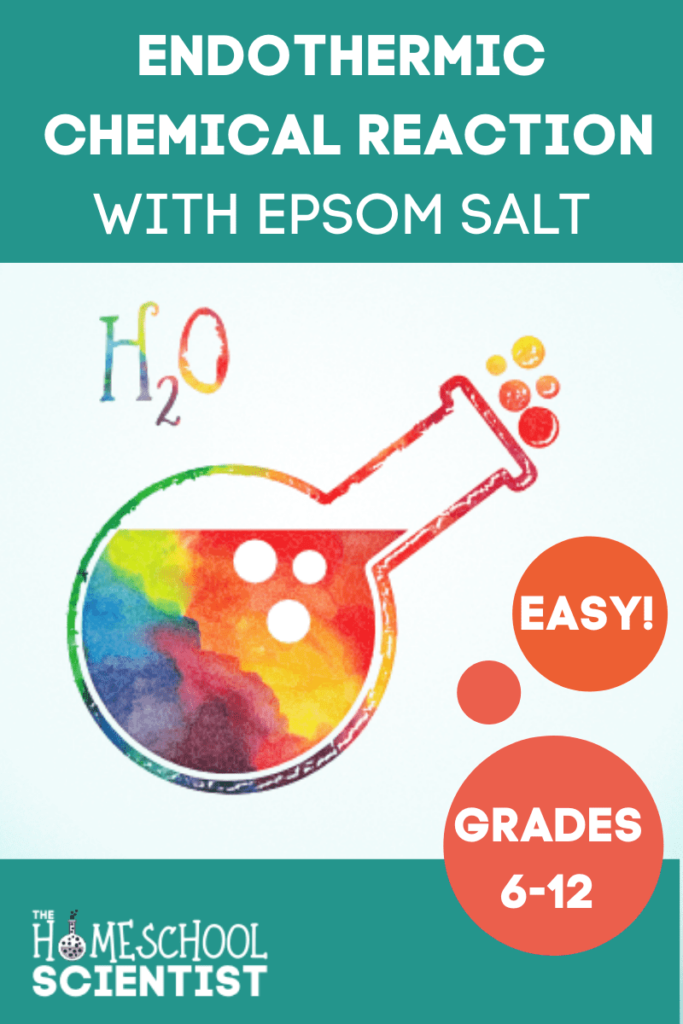
Endothermic Chemical Reaction Science Activity Using Epsom Salt
You may have used Epsom salt and water in a garden or as a foot soak. Epsom salt and water create an interesting endothermic chemical reaction.
In this simple chemistry activity, your student will be able to feel the results of the chemical reaction, as well as to measure what happened. This is what makes it a fun demonstration and effective teaching activity.
Before we get started, let’s go over some vocabulary and concepts.
Helpful Definitions and Concepts
- Epsom salt is magnesium sulfate, so we will use that label going forward. We will be mixing magnesium sulfate with water.
- When the water and magnesium sulfate are mixed together it forms a solution. A solution is a mixture that has a solute and a solvent. The solute is what is being dissolved. The solvent is what the solute is dissolved into.
- Chemical reactions and chemical changes always involve energy changes. During these reactions and changes, bonds are being made and/or being broken; these processes involve energy.
- Both of these words have Greek origins. Exo means out, Endo means within, and thermic is from the Greek word therme , which means heat.
- In an endothermic chemical reaction more energy is released into the surrounding area than is absorbed.
- In an exothermic chemical reaction, more energy is released into the surrounding area than is absorbed
Let’s get started!
What You’ll Need for this Endothermic Chemical Reaction Activity
Endothermic chemical reaction activity with epsom salt and water.
- 1 liquid measuring cup
- 1 dry ingredient measuring cup
- 1 1/4 cups Room temperature water
- 1 bowl
- 1 paper cup
- Thermometer
Instructions
- Set 1 ¼ cups of water out so it comes to room temperature
- Pour ¼ cup of water into the paper cup and 1 cup of water into the bowl.
- Measure and record the temperature of the water in the cup and in the bowl.
- Add ½ cup of Epsom salt to the paper cup only and STIR, STIR, STIR.
- Let sit for 30-45 seconds.
- Take the temperature of the water in the bowl, then the temperature of the water in the cup.
- Continue to stir a little and take the temperature of the water in the cup. What’s the temperature after 1 minute, 5 minutes, 15 minutes?
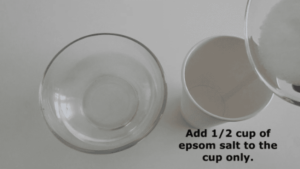
What happened?
The temperature of the water in the cup went down. Have your student review the information listed at the beginning of the post.
Why do you think the temperature of the water dropped?
What do you call the magnesium sulfate & water mixture? (a solution)
Which is the solute? (magnesium sulfate) which is the solvent? (water)
When the magnesium sulfate and water mix, two processes are happening. First, when the water dissolves the magnesium sulfate, the water’s heat energy is used to split apart the molecules into magnesium ions and sulfate ions.
In the second process, the water molecules attach to the individual magnesium and sulfate ions, which releases energy .
In this chemical reaction, first energy is used, then energy is released. However, more energy is used when the water molecules break up the magnesium sulfate into ions than is released in the second “step” when the water molecules attach to the magnesium and sulfate ions.
This is called an endothermic chemical reaction because it takes in more energy than it produces, which leaves the surrounding area cooler than it was.
Turn This Activity Into An Experiment
To turn this into an endothermic chemical reaction experiment with a dependent and independent variable.
Here are some ideas: repeat the steps, but substitute NoSalt brand salt alternative for the Epsom salt. Or repeat the steps above substituting an ice cube for the Epsom salt.
Before starting the experiment, form your hypothesis. What do you predict will happen when the Epsom salt, No Salt, and ice are each added to the water? How will you test and measure your hypothesis? What will be the dependent variable and independent variable?
You could also test if the starting water temperature has an effect on the temperature change when water is added to the Epsom salt.
What other variable could you change, measure, and test?
Other Chemistry Resources and Activities
- Testing the properties of Water
- Water Purifying Experiment
- Why Leaves Change Color In The Fall (includes 20-page free printable)
- Does your family like to garden? Incorporate a magnesium sulfate experiment along with your garden for some interesting soil science! The University of Montana Extension Service has some good chemistry information about the use of magnesium sulfate in the garden.
I hold a master’s degree in child development and early education and am working on a post-baccalaureate in biology. I spent 15 years working for a biotechnology company developing IT systems in DNA testing laboratories across the US. I taught K4 in a private school, homeschooled my children, and have taught on the mission field in southern Asia. For 4 years, I served on our state’s FIRST Lego League tournament Board and served as the Judging Director. I own thehomeschoolscientist and also write a regular science column for Homeschooling Today Magazine. You’ll also find my writings on the CTCMath blog. Through this site, I have authored over 50 math and science resources.
Thank you for visiting nature.com. You are using a browser version with limited support for CSS. To obtain the best experience, we recommend you use a more up to date browser (or turn off compatibility mode in Internet Explorer). In the meantime, to ensure continued support, we are displaying the site without styles and JavaScript.
- View all journals
- My Account Login
- Explore content
- About the journal
- Publish with us
- Sign up for alerts
- Open access
- Published: 12 September 2023
Searching the chemical space for effective magnesium dissolution modulators: a deep learning approach using sparse features
- Elisabeth J. Schiessler ORCID: orcid.org/0000-0001-5520-8325 1 ,
- Tim Würger ORCID: orcid.org/0000-0003-0346-0371 2 , 3 ,
- Bahram Vaghefinazari 2 ,
- Sviatlana V. Lamaka 2 ,
- Robert H. Meißner ORCID: orcid.org/0000-0003-1926-114X 2 , 3 ,
- Christian J. Cyron 1 , 4 ,
- Mikhail L. Zheludkevich 2 , 5 , 6 ,
- Christian Feiler ORCID: orcid.org/0000-0003-4312-7629 2 , 6 &
- Roland C. Aydin ORCID: orcid.org/0000-0002-9542-9146 1 , 4
npj Materials Degradation volume 7 , Article number: 74 ( 2023 ) Cite this article
746 Accesses
1 Altmetric
Metrics details
- Computational methods
- Mathematics and computing
- Metals and alloys
Small organic molecules can alter the degradation rates of the magnesium alloy ZE41. However, identifying suitable candidate compounds from the vast chemical space requires sophisticated tools. The information contained in only a few molecular descriptors derived from recursive feature elimination was previously shown to hold the potential for determining such candidates using deep neural networks. We evaluate the capability of these networks to generalise by blind testing them on 15 randomly selected, completely unseen compounds. We find that their generalisation ability is still somewhat limited, most likely due to the relatively small amount of available training data. However, we demonstrate that our approach is scalable; meaning deficiencies caused by data limitations can presumably be overcome as the data availability increases. Finally, we illustrate the influence and importance of well-chosen descriptors towards the predictive power of deep neural networks.
Similar content being viewed by others
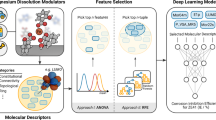
Predicting the inhibition efficiencies of magnesium dissolution modulators using sparse machine learning models
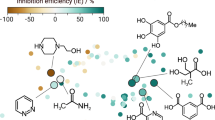
Exploring structure-property relationships in magnesium dissolution modulators
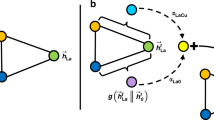
Predicting materials properties without crystal structure: deep representation learning from stoichiometry
Introduction.
Magnesium (Mg) and its alloys have distinct properties that render them promising materials for various applications, ranging from aerospace and automotive to biomedical and energy storage. However, it is essential to control the surface reactivity characteristics of Mg to unlock its full potential in each particular application field. For example, preventing corrosion is crucial for transport applications (e.g., aerospace and automotive), while medical applications (e.g., temporary biodegradable implants) require tailored degradation rates. For batteries with a Mg anode, the dissolution rate has to be adapted to maintain a constant output voltage and to protect the utilisation efficiency, e.g., from the occurring chunk effect 1 , 2 , 3 . Small organic molecules exhibit great potential in controlling corrosion in these applications, for which they are—depending on the target application— typically incorporated into a complex coating system in transportation applications or become a dissolved component of the electrolyte in Mg-air batteries.
The chemical space of compounds with potentially useful properties is practically infinite 4 , rendering purely experimental approaches insufficient despite impressive progress in the field of high-throughput testing. Data-driven computational methods have emerged as powerful tools for the prediction and identification of useful corrosion inhibitors and can thus enable a more efficient design of experiments. Exploring large areas of chemical space can become orders of magnitude faster, allowing the pre-selection of promising candidates for in-depth experimental testing. At the same time, further insights into the underlying chemical mechanisms of corrosion and its inhibition can be obtained, which in turn provide additional input features for predictive quantitative structure-property relationships (QSPRs).
To develop accurate and robust predictive models, a sufficiently large, reliable, and chemically diverse database is required, reflecting the complexity of the relevant chemical environment. Cheminformatics software packages, such as RDKit and alvaDesc, enable the structural encoding of the numerous different functional entities and molecular features included in such databases. Aside from that, advances in computing power and simulation algorithms have enabled simulations (e.g., relying on density functional theory or (semi empirical) force field calculations) that can provide a wide range of potentially useful molecular descriptors 5 . By selecting only the most suitable descriptors and using them as input for a QSPR model, a more thorough and nuanced analysis of the potential effectiveness of a given compound can be provided. As additional data becomes available, the model can be continually refined and improved, ensuring that the most effective dissolution modulators are identified.
The predictive performance of the trained QSPR model depends significantly on the selected molecular features, as high correlation between input features or low correlation with the target property can compromise the model. In recent years, machine learning models have become increasingly popular in corrosion modelling 6 , 7 , 8 , 9 . In Schiessler et al. 10 , we compared the capabilities of statistical methods, such as the analysis of variance (ANOVA 11 , 12 , 13 , 14 ), with recursive feature elimination (RFE 15 ) based on random forests 16 , 17 , 18 in selecting suitable input features of 60 compounds for a deep neural network to predict the corrosion inhibition efficiencies of chemical compounds for the magnesium alloy ZE41. Descriptors derived from density functional theory calculations could be identified as highly significant for predicting the experimental performance of corrosion inhibitors, when joined with input features derived from the molecular structure. Combining the sparse feature selection strategies with deep learning forms a predictive QSPR framework that can be used for the identification of promising corrosion inhibitors. However, when working with small datasets there exists a risk of overfitting on the training data, which will lead to results that do not generalise well and may not be able to give useful insights beyond the training domain 19 , 20 .
In this study, we predict and test the corrosion inhibition efficiencies of 15 previously unseen compounds that were selected using the ExChem 21 routine to evaluate the limitations of the models presented in Schiessler et al. 10 . The fundamental concept of ExChem is based on molecular similarities calculated from the Smooth Overlap of Atomic Positions (SOAP) 22 , 23 approach. The molecules in the dataset that was used to train the underlying supervised machine learning model are represented in form of a 2D map following a dimension reduction approach, thereby visualising the relationships between molecular structure and corrosion inhibition performance via the formation of similarity clusters. Moreover, ExChem facilitates the projection of a database of commercially available compounds onto the landscape of known chemical space and thus enables a rational selection of compounds for subsequent experimental evaluation based on structural similarities between the two databases and by providing estimates for the corrosion inhibition performance of the untested small organic molecules. After confirming the robustness of the feature selection process, the predictive performance of the neural networks is evaluated. Identified outliers are discussed with respect to their chemical features to explain deviations occurring between experimental and predicted corrosion inhibition properties. Furthermore we assess the effect of integrating more data into the training set and confirm the scalability of our approach.
Results and discussion
Similarity-based compound selection.
Under the overarching goal to find promising magnesium dissolution modulators for the magnesium alloy ZE41 in the vast chemical space, we tested the limits of the machine learning models as presented in our previous study 10 with respect to prediction performance and scalability. Therefore, we selected blind test candidates using the ExChem routine from a database of over 7000 commercially available chemicals, as provided by Thermo Fisher Scientific 21 . A database of 60 magnesium dissolution modulators for ZE41, originally used to train the machine learning models, served as foundation for the approach 10 , 24 . Molecular similarities of the original training data and the database of commercially available compounds were calculated using the SOAP kernel with a cutoff radius r c = 2.0 Å, a Gaussian width ξ = 0.3 Å and ζ = 2 (cf. Methods) 22 , 23 . We reduced the resulting high-dimensional similarity matrix to two dimensions using kernel principal component analysis. Correlating the two-dimensional data with experimentally measured corrosion inhibition efficiencies for the respective compounds resulted in a structure-property landscape, as shown in Fig. 1 .
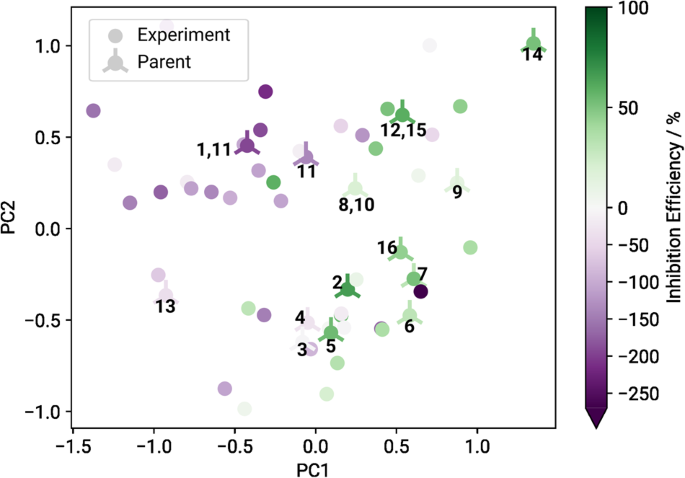
The axes represent the two principal components (PC) resulting from the kernel principal component analysis. Based on this map, untested compounds of interest were selected for further investigation using the ExChem routine. Twenty of the original 60 structures were randomly chosen as `parents' (crossed circles), for which highly similar compounds were determined out of a pool of commercially available chemicals. The numbers indicate which of the selected test candidates as defined in Table 1 correspond to which parents.
A clear relationship between molecular structure and corrosion inhibition efficiency becomes evident, where compounds yielding corrosion inhibiting effects are located predominantly on the right side of the landscape (green circles) and compounds accelerating corrosion are located mainly on the left side (purple circles). The ExChem routine was used to identify potential test candidates in the commercial database that exhibit high similarity to certain compounds that were already experimentally validated. Initially, 20 compounds of interest were randomly selected from the experimental database. Each compound served as reference (‘parent’) to identify five highly similar structures (‘children’) in the commercial database based on the underlying SOAP similarities. Out of the resulting 100 structures, 20 were randomly chosen for experimental blind testing. Since four of these 20 were not soluble in water, they were removed from the pool of blind test candidates. The remaining 16 selected compounds are listed in Table 1 along with their respective indices, names and experimentally measured inhibition efficiencies. The associated parent structures are marked with crossed circles in Fig. 1 along with the indices of the selected children, i.e., the chosen blind test candidates. Compound 2 was excluded during the evaluation phase as the required materials could not be delivered. In the following, we evaluate the robustness of the feature selection process given the availability of this additional dataset, as well as the performance of the predictive models against the presented blind test data, which have been withheld from the model training process.
Feature selection robustness
We investigated the quality of selected features that were presented in our previous study 10 by exploring how susceptible the feature selection results are to changes in input data. The original 60 sample dataset 10 , 24 was augmented by the 15 blind testing samples given in Table 1 , forming a combined dataset of 75 compounds. This gave us a number of dataset compositions that we use throughout this manuscript:
original dataset (60 compounds): DS 60
blind testing dataset (15 compounds): DS 15
combined dataset (75 compounds): DS 75
On each composition, we performed grouped feature selection using RFE based on random forests. Data were split into 10 cross-validation folds (which differ per dataset composition), and on each fold the process was repeated 100 times using varying random seeds. From the resulting 1,000 top five groups per dataset composition we report the ones that got selected most often, cf. Table 2 .
As we can see in Table 2 , the top five feature sets FS 60 and FS 75 found for the original (DS 60 ) and combined (DS 75 ) dataset compositions overlap in three out of five components. The remaining two from each set (CATS3D_02_AP and Mor04m for FS 60 , HOMO and E2s for FS 75 ) do in fact come up in the other dataset composition’s respective best feature sets list, just not in first place. FS 60 and FS 75 were chosen in 38% and 30% of cases respectively. The winning feature set FS 15 for the blind testing dataset composition DS 15 on the other hand was chosen in only 12% of all runs, with a greater variation in included candidates. This comes as no surprise, as 15 data points is quite few in most machine learning contexts. The best features for the original dataset, FS 60 , have no overlap with the blind testing set winners FS 15 . From this we surmise a somewhat limited ability of FS 60 to accurately capture the specific properties of the blind testing dataset, as well as a reduced capacity to generalise. The winning feature set FS 75 determined from the combined dataset composition includes descriptors from both FS 60 and FS 15 . It is noteworthy that HOMO, a DFT-derived descriptor denoting the highest occupied molecular orbital energy level, was present in the second best feature set for DS 60 , and came up in the shared first place for best feature set in our original study 10 . This descriptor is included in both FS 75 and FS 15 and seems to play a crucial role in capturing properties of the presented corrosion inhibition dataset.
Feature selection robustness was furthermore investigated under change of target metric (using inhibition power/dB instead of inhibition efficiency/%, 25 ) and exhibited qualitatively comparable behaviour to the case we presented here. Since subsequent predictive models trained on the thereby identified feature sets did not lead to relevant performance increase, we elected to only present inhibition efficiency/% results which are directly comparable to our previous study 10 . Additional information regarding this metric as well as results from the related feature selection process can be found in the Supplementary Notes as well as Supplementary Table 1 .
Generalisation ability of predictive models
One very important concern is the question of how well predictive models trained on the original data are able to generalise and capture the properties of completely unseen (i.e., blind testing) data. To this end, we repeatedly fitted a deep neural network on DS 60 , using only inputs based on the associated winning feature set FS 60 . The training data were split into the same 10 cross-validation folds that we used during the feature selection process, and on each fold the network was trained 100 times using varying random seeds. The blind testing dataset DS 15 served as a completely unseen test set. Figure 2 shows the distribution of predicted inhibition efficiency values per compound in the blind testing set, aggregated over all cross-validation folds and random seeds. The detailed prediction means and standard deviations are provided in Supplementary Table 2 .
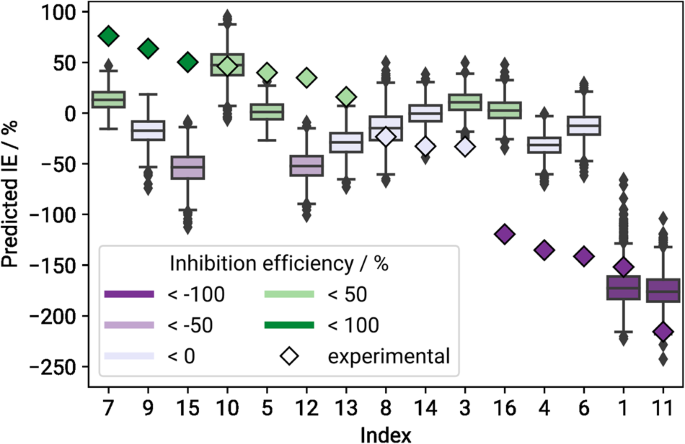
Boxes are coloured according to the compound’s mean predicted IE values in %. Compounds are sorted by descending mean experimental IE values, which are depicted as coloured diamonds.
Only about half of the compounds in DS 15 get predicted correctly or within reasonable margins of error. The resulting root mean squared error (RMSE) for the blind testing set is fairly high at 73 percentage points (pp), cf. Table 3 for more statistics. We can see that the models have a tendency to underestimate inhibitors (i.e., compounds with IE > 0), but overestimate accelerators, as can be seen also in previous studies 21 , 26 . It is also notable that all but two prediction means lie within approximately ± 50 IE, which is where the majority of both the original as well as blind testing target values are situated. It is a common problem in machine learning that simply predicting the mean value of the target variable distribution might lead to a lower training loss than trying to find more complex dependencies. This behaviour can be indicative of overfitting or a suboptimal network architecture 27 . Figure 3 shows the average predicted over experimental IE, with the solid blue line representing the resulting linear regression curve, and the orange dashed line marking the perfect fit.
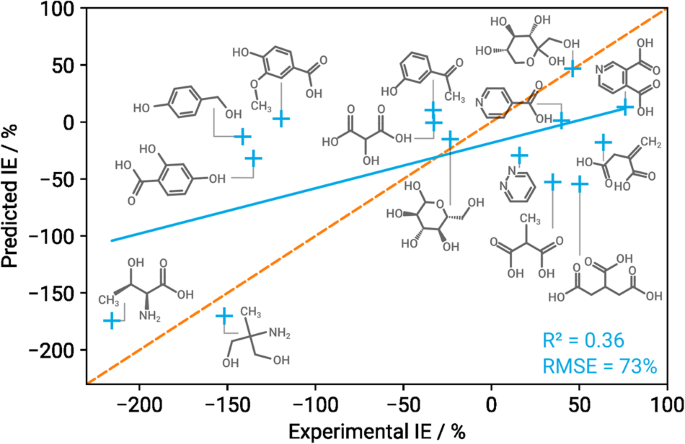
The solid blue line marks the resulting linear regression curve, the dashed orange line represents perfect fit.
Overall we can conclude that the model trained on the original dataset, with features selected only for those data (denoted FS 60 /DS 60 ), is able to predict the behaviour of completely unseen components only moderately well. This does not come as a huge surprise for two main reasons: Firstly, there is no overlap between FS 60 and FS 15 . This need not necessarily mean that FS 60 is entirely unable to adequately capture the properties of compounds from DS 15 , but it is an early indicator for results of reduced quality. Secondly, with only 60 samples in the original dataset we have to expect overfitting both for the feature selection process and especially the training of deep neural networks. The network architectures in Schiessler et al. 10 where chosen to vary as little as possible across a range of input feature counts, leading to overparameterised networks especially when working with very few features. With more fine-tuning of the network architecture and training hyperparameters, improved results might well be possible even on the blind dataset. However we can also make use of existing outliers to both gain important insights into the predictive domain of our models, as well as better understand the involved corrosion processes, or even identify yet unknown aspects of corrosion. In the following section we therefore include an extensive discussion of several components that obtained particularly conspicuous results.
In Fig. 2 there are six compounds which are particularly salient, and which we consider to be strong outliers from the perspective of our deep learning models, cf. Fig. 4 . These are compounds 9, 12, and 15, which are moderate to strong inhibitors but get qualitatively mispredicted as mild to strong accelerators, as well as compounds 4, 6 and 16, which are very strong accelerators but get predicted as only mild to moderate accelerators.
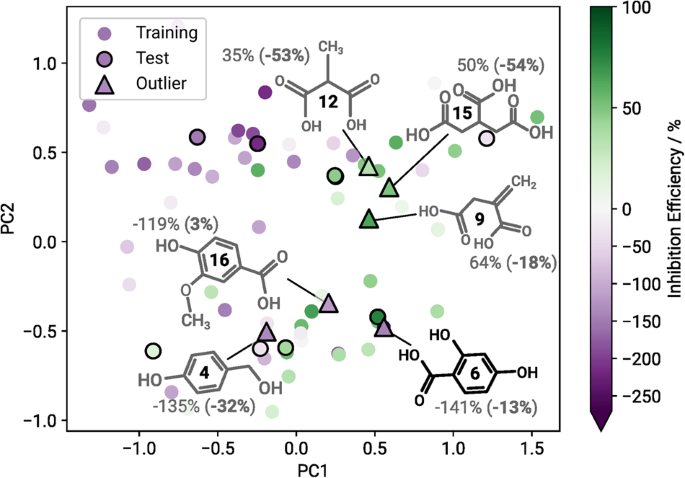
Compounds identified as extreme outliers are marked accordingly ( △ ) and illustrated along with their measured ( predicted ) inhibition efficiency. Predictions from FS 60 / DS 60 experiments.
To better understand potential reasons why these compounds appear as outliers for the prediction models, deeper insights into their molecular structure shall be given. Analogously to Fig. 1 , a structure-property landscape was generated for the total dataset of 75 compounds, where the compounds we consider to be outliers are marked accordingly (see Fig. 4) . Analysing the resulting map, regions where compounds exhibit a similar corrosion inhibition efficiency indicate a structure-property relationship. Generally, it appears that corrosion accelerators are predominantly on the left side of the map and corrosion inhibitors on the right. Additionally, the structures are split into aliphatics (top side of the map) and aromatics (bottom side of the map).
2,4-Dihydroxybenzoic acid (compound 6) is located in a cluster predominantly populated by corrosion inhibitors, although experimentally it turns out to be a strong corrosion accelerator. It was still qualitatively correctly predicted as an accelerator. Compounds 6 is projected directly on top of 3,4-Dihydroxybenzoic acid, the strongest corrosion accelerator (-270% IE) of the original dataset. However the strongest corrosion inhibitor present in the blind testing set, 3,4-Pyridinedicarboxylic acid (compound 7), is located in the direct proximity as well. Apparently both corrosion inhibitors as well as accelerators contain mutual features in this region, rendering them similar in structure, even though they show different behaviours in the experiment. The trained models recognised a corrosion accelerator based on the selected features, but did not capture the subtle features that distinguish a strong from a weak accelerator, which is why the IE was overestimated. The overestimated IEs of 4-Hydroxybenzylalcohol (compound 4) and vanillic acid (compound 16) are situated in the same area of the map and can be explained accordingly. The structure-property relationship is not obvious in this region, as the compounds projected onto this area of the map exhibit structural features that are connected to varying corrosion inhibition efficiencies. Additionally, the experimental values of the three compounds 4, 6 and 16 lie at the lower edge of the target data distribution, further complicating accurate predictions. Adding more data points to this region, i.e., experimentally testing more compounds that exhibit similar structural features, is likely to improve the prediction performance for this domain.
Analysis of compounds 9, 12 and 15 shows that they were projected close to a region populated by weak corrosion inhibitors and accelerators. All of these compounds yield a moderate IE in the experiment and are mapped close to each other onto the structure-property landscape. The significant underestimations of the IEs probably stem from the absence of comparable corrosion inhibitors in this region. Furthermore, the selected features do not seem to capture the occurring structure-property relationship here accurately. However, future predictions for this region of the structure-property landscape are expected to improve with additional data.
Generalisation ability of the winning feature sets
In order to guarantee comparability to Schiessler et al. 10 , we abstained from adjusting network architecture and training details in this work. Instead we examined the influence of using “better” feature sets towards improving the predictive quality and ability to generalise of our neural networks. In particular, we investigated whether predictive models that were trained on the original dataset DS 60 could be improved if selected features were more suitable for the blind testing data, i.e., when training occurred in combination with FS 15 or FS 75 .
Clearly this approach is not applicable in practice without already having experimental values available for any data we wish to investigate, as those values are already needed during the feature selection process. Therefore the following results should not be seen as claims to the predictive capabilities of our already existing models. We can rather consider them as a lower bar on how well we are able to do given feature sets that really generalise well (recall that we still did not use the blind testing data during training of these neural networks).
We repeated the training process for the neural networks, using DS 60 along with the same cross-validation folds as before as our training data, and again aggregating predictions on the blind testing set across all runs afterwards. The only difference was that FS 15 and FS 75 features were used as input instead. With this approach we hoped to improved predictive quality on the blind testing compounds, as their most relevant properties now played a direct role in adjusting the deep learning weights. Distributions of predictions for the blind testing data generated by FS 15 / DS 60 and FS 75 / DS 60 models can be found in Fig. 5 . Detailed prediction means are provided in Supplementary Table 2 .
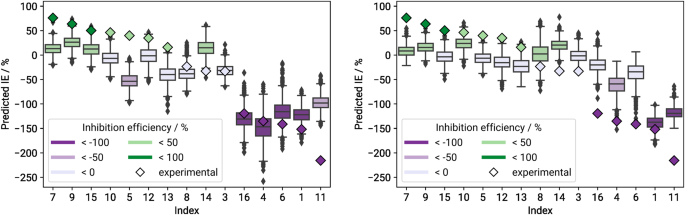
In fact, in both cases we saw a drastic increase in accuracy with much fewer outliers and reduced RMSE of 52 percentage points (pp) and 62pp for the models using FS 15 and FS 75 respectively compared to 73pp for the FS 60 models, cf. Table 3 . Especially in the case of using features devised from only the blind testing data, this RMSE is on par with what was presented in Schiessler et al. 10 , but without ever seeing these data during the training process.
The hidden downside, however, is that FS 15 / DS 60 models capture the qualities of the original dataset much more inaccurately. The overall RMSE for predictions on both the blind testing set and validation splits for this case is the highest of all three at 80pp, opposed to 67pp for both the FS 60 / DS 60 and FS 75 / DS 60 models.
Scalability
In order to further validate our approach we repeated the training process with cross-validation splits drawn from the combined dataset DS 75 (the same that were used to determine FS 75 ). In this setup, there are no more blind testing data as they were incorporated into the combined dataset, thus we only report results aggregated from the respective validation sets per fold. At 64pp, the RMSE of the FS 75 / DS 75 models is on par with the mean RMSE of 63pp reported in Schiessler et al. 10 , demonstrating that previous results can be replicated with different training sets and were not a consequence of for example overfitting.
From a machine learning perspective, a 25% increase of the dataset is not huge, and most likely the properties of the original data will still dominate overall results. From an experimental point of view, however, a great amount of time and effort went into performing the required analyses and already slight improvements in predicting the inhibition efficiency of organic compounds go a long way. At any rate we were able to increase the domain of applicability of our predictive models by virtue of the combined dataset, confirming the scalability of our method.
In this work we investigated how well the predictive model that performed best in our previous study 10 holds up under blind testing. To this end, 15 previously unused compounds were randomly selected using the ExChem Routine 21 and their inhibition efficiencies w.r.t. the magnesium alloy ZE41 were experimentally determined using the setup presented by Lamaka et al. 24 , forming the blind testing dataset DS 15 .
Feature selection based on RFE suggested that the five features determined via the original dataset DS 60 might not be able to generalise very well as there was no overlap between the winning feature sets for DS 60 and DS 15 . However, when regarding both the original and blind testing data in the form of a combined dataset DS 75 , winning features were a 3:2 mixture from the winners of both individual sets, indicating that the feature selection process is indeed robust and scalable when further information is added. It is notable that the DFT-derived descriptor HOMO came up in the runner-up second best feature set for the original data, and was included in winning sets for both the blind testing and combined dataset compositions, and in general seems to contain important information w.r.t. the inhibition efficiency properties of magnesium dissolution modulators.
Predictive modelling using deep neural networks trained on the original dataset and feature set confirmed that the originally selected descriptors showed only moderate success in correctly identifying the IE of the blind testing compounds. Training the networks on the newly identified feature sets managed to drastically improve the predictive quality even though the blind testing data themselves were only used during the feature selection step but never included in the training process. In summary we conclude that the identified feature sets are not yet able to thoroughly cover large parts of chemical space of potential additive components and need to be updated on a regular basis as more and more experimental data become available. Yet, even when given knowledge only about a very limited amount of data, our method already has a demonstrated predictive power in estimating the inhibition efficiency of magnesium dissolution modulators. Scalability of the method was confirmed via training the neural networks on the combined dataset composition.
In general, the architecture of the neural networks appears to be overparameterised given that we only used a total of five input features for training. This occurred in order to ensure comparability to the original setup presented in our previous study 10 . We aim to address this in future works using automated neural architecture search such as developed by Schiessler et al. 28 which can be helpful in choosing a better suited network topology while limiting the risk of overfitting on the training data. One issue with regression type machine learning is that there is less punishment during the learning process when the model qualitatively mispredicts target values (e.g., a positive target value is predicted to be negative and vice versa). This can be mitigated using classification type models, however, once higher levels of granularity are desired (e.g., for discerning between moderate and strong accelerators or inhibitors), custom loss functions are required that take into account ordered classes.
Another goal for future extensions is to further explore outlier detection using other related approaches such as autoencoders which are restricted to the features used in the machine learning models, as was briefly touched upon in Schiessler et al. 10 .
Corrosion experiments
Since the dataset used to train the initial deep neural network in this study was extracted from the work of Lamaka et al. 24 , the model validation by blind testing was carried out with the same experimental setup and under the same conditions. The inhibition efficiency (IE) of the compounds selected by the ExChem routine was calculated based on hydrogen evolution tests, in which the amount of evolved hydrogen due to the corrosion of magnesium is measured during immersion in a NaCl solution. 0.5 g of ZE41 Mg chips with the surface area of 490 ± 15 cm 2 g −1 from the same batch used in Lamaka et al. 24 were immersed in 0.5 wt.% NaCl solution without (reference solution) and with the untested compounds, respectively. The chemical composition of the ZE41 chips used for our experiments was identical to the work of Lamaka et al. 24 and is provided in Supplementary Table 3 . The concentration of compounds was 0.05 M and the pH of solutions was adjusted to 7.0 ± 0.1 by adding NaOH. Compound 3 (3-Hydroxyacetophenone) was used at its saturation, which was measured as 0.03 M. Since compound 1 (2-Amino-2-methyl-1,3-propanediol) has alkaline properties, 0.05 M of this chemical was first dissolved in an HCl solution with a Cl − concentration equivalent to that of a 0.5 % NaCl reference solution. This solution’s pH was then adjusted to 7.0 ± 0.1 with NaOH, similar to the other solutions.
The hydrogen evolution measurements were repeated three times for each solution and the mean of the calculated IEs was used for the corresponding blind test data point. IE is defined as follows
where \({V}_{{{{{\rm{H}}}}}_{2}}^{0}\) and \({V}_{{{{{\rm{H}}}}}_{2}}^{{{{\rm{Inh}}}}}\) are the volumes of H 2 evolved after 20 h of immersion in the reference NaCl solution and the NaCl solution containing the investigated chemical compound, respectively. More details on the hydrogen evolution tests are available in the original publication by Lamaka et al. 24 .
Molecular similarity
We selected suitable blind test candidates by using the ExChem routine 21 . ExChem exploits molecular similarities to find structurally similar chemical structures in a given database with respect to a selected chemical compound of interest. We calculated the underlying molecular similarities using the Smooth Overlap of Atomic Positions (SOAP) kernel that represents a high-dimensional similarity representation for the considered molecular compounds 22 , 23 . For each given compound, a local environment is first defined in a spherical region of radius r c around each atom and then built by a superposition of Gaussian functions with width ξ . The structural information around an atom that flows into the similarity measure is directly dependent on the size of r c . Calculating the translationally and rotationally invariant overlap between two local environments results in the SOAP kernel. The kernel can be further raised to a power ζ for improved discrimination between small or large similarities. Averaging over all local atomic environments enables the calculation of a global similarity measure that contains the molecular similarities between all chemical structures in a given dataset.
Interpretation of the molecular similarities in high-dimensional space was facilitated by projection to a two-dimensional latent space and correlation with experimental data. Distant (dissimilar) or close (similar) structures in the high-dimensional space maintain their relationships in the low-dimensional space. By evaluating the relative positions of compounds with respect to the formation of clusters in the two-dimensional similarity landscape, we can reveal existing structure-property relationships.
Feature generation
First, the geometries of the 15 blind test molecules were optimized using the quantum chemical software package Turbomole 7.4. 29 at the TPSSh/def2SVP 30 , 31 level of density functional theory. The optimized structures were subsequently used as input for the cheminformatics software package alvaDesc 1.0 32 and combined with six properties (HOMO, LUMO, HOMO-LUMO gap (Δ E HL ) as well as C p , C v , μ calculated at 293 K) that are directly derived from the output of the performed DFT calculations to generate the same pool of 1260 molecular descriptors that have been used in our previous work 10 .
Feature selection
In Schiessler et al. 10 , features (i.e. molecular descriptors) were selected using both ANOVA 11 , 12 , 13 , 14 and recursive feature elimination (RFE 15 ) with a random forest regressor 16 , 17 , 18 as the underlying selector, and the corrosion inhibition efficiency as the target variable. RFE is a feature selection method that fits a specified regression (or classification) model given the available training data, and then determines a number of features that least influence the predictive result. These features are excluded from the available pool, and the whole process is repeated until only the desired number or features remain.
Both methods were used to identify the group of top three, five, as well as 63 (i.e. top 5%) features. In all cases, the experiments were performed 100 times with a fixed train-test split of the available dataset, and then the group was determined that got selected most often (i.e., the selection mode). Subsequent predictive models trained on the various feature groups identified the set of five features as determined by RFE to be the most relevant w.r.t. predictions of inhibition efficiency of the available dataset. A full 10-fold cross-validation analysis confirmed both the composition of the top performing group as well as its status as most relevant set of features for predictive modelling.
In this work, we investigated the robustness of previous feature selection results under expansion of the training data. The 15 compounds listed in Table 1 were added to the original dataset used in Schiessler et al. 10 , resulting in a combined dataset of 75 compounds. The resulting dataset compositions were denoted by DS 60 , DS 15 and DS 75 , respectively.
Since in Schiessler et al. 10 features selected by ANOVA and groups of three features found by RFE produced significantly worse results when used in predictive modelling, and the set of 63 features showed signs of having a high noise-to-signal ratio, we focused our robustness analysis on grouped selection using RFE for groups of five features only.
For each dataset composition, we repeated the steps described in Schiessler et al. 10 , running RFE 100 times using various random seeds per cross-validation fold, in order to select the grouped top five features per setting. Cross-validating experiments, such as we are doing, means splitting available datasets into n equal parts, called the folds 33 . The same experiment is then run n times, where a different portion of the data is withheld each time and serves as validation set for this fold. In the end, predictive results on the validation sets are averaged across all folds. This method is especially relevant when working with small datasets, to reduce overfitting and to reduce the influence of potential outliers that may be contained withing the data 19 , 20 .
On DS 60 , the cross-validation folds reported in our previous study 10 we re-used. On the other dataset variations, separate folds were drawn. Note that DS 15 on its own, consisting only of 15 samples, is too small to expect consistent results under cross-validation. The winning feature sets where the ones that got selected most often per cross-validation fold and random seed. We named these FS 60 , FS 75 and FS 15 , respectively.
Predictive modelling
As before in Schiessler et al. 10 , we used deep learning to evaluate the relevance of identified feature sets for predicting inhibition efficiency of magnesium modulators. Since we restricted the feature selection process to sets of five features, only the architecture for what were called ‘small’ networks in Schiessler et al. 10 was reused. Our deep learning networks thus consist of the following layers:
An input layer accepting inputs from the selected five descriptors
A Gaussian noise layer with hyperparameters μ = 0 and σ = 0.1
Three fully connected layers with 50, 20, and 10 units, respectively, all using relu activation
An output layer with one unit and no activation
The Gaussian noise layer adds some randomness to each input during training, drawn from a normal distribution with mean μ and standard deviation σ , which helps to counter the risk of overfitting on the training data. This layer is only active during the training phase. The networks were trained for 25 epochs using an Adam optimiser with learning rate 0.01, and mean squared error (MSE) as the loss function.
As a preprocessing step, all data that get passed through the networks were scaled using min-max-scaling, with the target variable being scaled into the range [0, 1], and the input variables into the range [ − 1, 1].
We applied the same cross-validation folds that were used during the feature selection process. On each fold and setting, the same architecture was trained 100 times using different random seeds. Detailed software specifications are included in the Supplementary Notes .
For statistical analyses such as calculating the root mean squared error (RMSE) of the models, predictions for each compound were first averaged across all cross-validation folds and random seeds. Note that for the scalability analysis presented in Section Scalability, the blind testing data were included in the cross-validation folds. Analyses in this section were therefore not performed specifically on the blind testing data, but on the validation set results from each cross-validation fold.
Data availability
The data used for this study is available at Zenodo via https://doi.org/10.5281/zenodo.7780743 .
Code availability
The code used for this study is available at Zenodo via https://doi.org/10.5281/zenodo.7780743 .
Feng, Y., Xiong, W., Zhang, J., Wang, R. & Wang, N. Electrochemical discharge performance of the Mg-Al-Pb-Ce-Y alloy as the anode for Mg-air batteries. J. Mater. Chem. A 4 , 8658–8668 (2016).
Article CAS Google Scholar
Vaghefinazari, B., Höche, D., Lamaka, S. V., Snihirova, D. & Zheludkevich, M. L. Tailoring the Mg-air primary battery performance using strong complexing agents as electrolyte additives. J. Power Sources 453 , 227880 (2020).
Deng, M. et al. High-energy and durable aqueous magnesium batteries: recent advances and perspectives. Energy Stor. Mater. 43 , 238–247 (2021).
Google Scholar
Erlanson, D. A., Fesik, S. W., Hubbard, R. E., Jahnke, W. & Jhoti, H. Twenty years on: the impact of fragments on drug discovery. Nat. Rev. Drug. Discov. 15 , 605–619 (2016).
Fockaert, L. I. et al. ATR-FTIR in Kretschmann configuration integrated with electrochemical cell as in situ interfacial sensitive tool to study corrosion inhibitors for magnesium substrates. Electrochim. Acta 345 , 136166 (2020).
Wang, Y. et al. High-throughput calculations combining machine learning to investigate the corrosion properties of binary Mg alloys. J. Magnesium Alloys https://doi.org/10.1016/j.jma.2021.12.007 (2022).
Lu, Z. et al. Prediction of Mg alloy corrosion based on machine learning models. Adv. Mater. Sci. Eng. 2022 , 9597155 (2022).
Article Google Scholar
Hughes, A. E. et al. Corrosion inhibition, inhibitor environments, and the role of machine learning. Corros. Mater. Degrad. 3 , 672–693 (2022).
Sutojo, T. et al. A machine learning approach for corrosion small datasets. npj Mater. Degrad. 7 , 18 (2023).
Schiessler, E. J. et al. Predicting the inhibition efficiencies of magnesium dissolution modulators using sparse machine learning models. npj Comput. Mater. 7 , 193 (2021).
Johnson, K. J. & Synovec, R. E. Pattern recognition of jet fuels: comprehensive GC × GC with ANOVA-based feature selection and principal component analysis. Chemometr. Intell. Lab. Syst. 60 , 225–237 (2002).
Kim, T. K. Understanding one-way ANOVA using conceptual figures. Korean J. Anesthesiol. 70 , 22–26 (2017).
Burgard, D. R. Chemometrics: Chemical and Sensory Data (CRC Press, 2018).
van der Vaart, A., Jonker, M. & Bijma, F. An Introduction to Mathematical Statistics (Amsterdam University Press, 2017).
Guyon, I., Weston, J., Barnhill, S. & Vapnik, V. Gene selection for cancer classification using support vector machines. Mach. Learn. 46 , 389–422 (2002).
Ho, T. K. Random decision forests. In Proceedings of 3rd International Conference on Document Analysis and Recognition Vol. 1, 278–282 (IEEE, 1995).
Genuer, R., Poggi, J.-M. & Tuleau-Malot, C. Variable selection using random forests. Pattern Recognit. Lett. 31 , 2225–2236 (2010).
Chavent, M., Genuer, R. & Saracco, J. Combining clustering of variables and feature selection using random forests. Commun. Stat. B: Simul. Comput. 50 , 426–445 (2021).
Arlot, S. & Celisse, A. A survey of cross-validation procedures for model selection. Stat. Surv. 4 , 40–79 (2010).
Cawley, G. C. & Talbot, N. L. C. On over-fitting in model selection and subsequent selection bias in performance evaluation. J. Mach. Learn. Res. 11 , 2079–2107 (2010).
Würger, T. et al. Exploring structure-property relationships in magnesium dissolution modulators. npj Mater. Degrad. 5 , 2 (2021).
Bartók, A. P., Kondor, R. & Csányi, G. On representing chemical environments. Phys. Rev. B 87 , 184115 (2013).
De, S., Bartók, A. P., Csányi, G. & Ceriotti, M. Comparing molecules and solids across structural and alchemical space. Phys. Chem. Chem. Phys. 18 , 13754–13769 (2016).
Lamaka, S. V. et al. Comprehensive screening of Mg corrosion inhibitors. Corros. Sci. 128 , 224–240 (2017).
Kokalj, A. et al. Simplistic correlations between molecular electronic properties and inhibition efficiencies: do they really exist? Corros. Sci. 179 , 108856 (2021).
Feiler, C. et al. In silico screening of modulators of magnesium dissolution. Corros. Sci. 163 , 108245 (2020).
Géron, A. Hands-On Machine Learning with Scikit-Learn, Keras and TensorFlow (O’Reilly Media, Inc., 2019).
Schiessler, E. J., Aydin, R. C., Linka, K. & Cyron, C. J. Neural network surgery: combining training with topology optimization. Neural Netw. 144 , 384–393 (2021).
Turbomole. V7.4. A Development of University of Karlsruhe and Forschungszentrum Karlsruhe GmbH, 1989–2019 Since 2007 . https://www.scirp.org/(S(i43dyn45teexjx455qlt3d2q))/reference/ReferencesPapers.aspx?ReferenceID=768588 (2019).
Staroverov, V. N., Scuseria, G. E., Tao, J. & Perdew, J. P. Comparative assessment of a new nonempirical density functional: molecules and hydrogen-bonded complexes. J. Chem. Phys. 119 , 12129–12137 (2003).
Eichkorn, K., Weigend, F., Treutler, O. & Ahlrichs, R. Auxiliary basis sets for main row atoms and transition metals and their use to approximate Coulomb potentials. Theor. Chem. Acc. 97 , 119–124 (1997).
Mauri, A. alvaDesc: A tool to calculate and analyze molecular descriptors and fingerprints. Methods Pharmacol. Toxicol. 64 , 801–820 (2020).
Stone, M. Cross-validatory choice and assessment of statistical predictions. J. R. Stat. Soc., B: Stat. 36 , 111–147 (1974).
Download references
Acknowledgements
Funding by the Helmholtz Association is gratefully acknowledged. TW, BV, SL and CF gratefully acknowledge financial support from the Helmholtz Artificial Intelligence Cooperation Unit via the A I 2 project (Projektnummer ZT-I-PF-5-102). The authors thank Thermo Fisher Scientific for providing a chemical database that was used to select additional compounds for model validation using the previously developed ExChem approach.
Open Access funding enabled and organized by Projekt DEAL.
Author information
Authors and affiliations.
Institute of Material Systems Modeling, Helmholtz-Zentrum Hereon, Geesthacht, Germany
Elisabeth J. Schiessler, Christian J. Cyron & Roland C. Aydin
Institute of Surface Science, Helmholtz-Zentrum Hereon, Geesthacht, Germany
Tim Würger, Bahram Vaghefinazari, Sviatlana V. Lamaka, Robert H. Meißner, Mikhail L. Zheludkevich & Christian Feiler
Institute of Polymers and Composites, Hamburg University of Technology, Hamburg, Germany
Tim Würger & Robert H. Meißner
Institute for Continuum and Material Mechanics, Hamburg University of Technology, Hamburg, Germany
Christian J. Cyron & Roland C. Aydin
Institute for Materials Science, Faculty of Engineering, Kiel University, Kiel, Germany
Mikhail L. Zheludkevich
Kiel Nano, Surface and Interface Science KiNSIS, Kiel University, Kiel, Germany
Mikhail L. Zheludkevich & Christian Feiler
You can also search for this author in PubMed Google Scholar
Contributions
E.J.S., T.W., B.V., S.V.L., R.H.M., C.J.C., M.L.Z., C.F. and R.C.A. contributed to the conception and design of the study. C.F. and T.W. generated the molecular descriptor database and selected the compounds for experimental validation of the model. B.V. and S.V.L. conducted the validation experiments. E.J.S. did the theoretical analyses and wrote the supporting code. E.J.S., T.W., R.C.A and C.F. evaluated the quality of the presented models. E.J.S. and T.W. created the figures. E.J.S., T.W., C.F. and R.C.A. wrote the first draft of the manuscript. All authors contributed to the manuscript revision, read, and approved the submitted version.
Corresponding authors
Correspondence to Christian Feiler or Roland C. Aydin .
Ethics declarations
Competing interests.
The authors declare no competing interest.
Additional information
Publisher’s note Springer Nature remains neutral with regard to jurisdictional claims in published maps and institutional affiliations.
Supplementary information
Supplemental material, rights and permissions.
Open Access This article is licensed under a Creative Commons Attribution 4.0 International License, which permits use, sharing, adaptation, distribution and reproduction in any medium or format, as long as you give appropriate credit to the original author(s) and the source, provide a link to the Creative Commons license, and indicate if changes were made. The images or other third party material in this article are included in the article’s Creative Commons license, unless indicated otherwise in a credit line to the material. If material is not included in the article’s Creative Commons license and your intended use is not permitted by statutory regulation or exceeds the permitted use, you will need to obtain permission directly from the copyright holder. To view a copy of this license, visit http://creativecommons.org/licenses/by/4.0/ .
Reprints and permissions
About this article
Cite this article.
Schiessler, E.J., Würger, T., Vaghefinazari, B. et al. Searching the chemical space for effective magnesium dissolution modulators: a deep learning approach using sparse features. npj Mater Degrad 7 , 74 (2023). https://doi.org/10.1038/s41529-023-00391-0
Download citation
Received : 18 April 2023
Accepted : 08 August 2023
Published : 12 September 2023
DOI : https://doi.org/10.1038/s41529-023-00391-0
Share this article
Anyone you share the following link with will be able to read this content:
Sorry, a shareable link is not currently available for this article.
Provided by the Springer Nature SharedIt content-sharing initiative
Quick links
- Explore articles by subject
- Guide to authors
- Editorial policies
Sign up for the Nature Briefing: AI and Robotics newsletter — what matters in AI and robotics research, free to your inbox weekly.

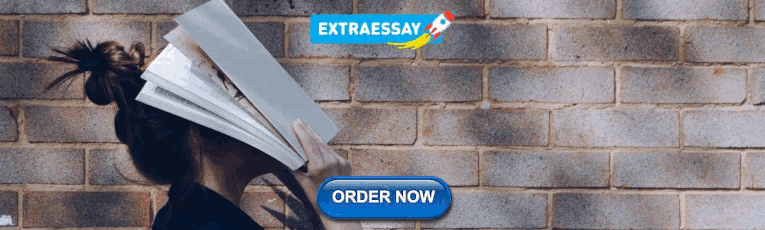
IMAGES
VIDEO
COMMENTS
Introduction. Epsom salt (MgSO 4 ·7H 2 O) is a heptahydrate of magnesium sulfate: within one mole of magnesium sulfate heptahydrate are seven moles of water. This water can be driven off by heat to form the anhydrous (dehydrated) ionic compound, magnesium sulfate. The mass of water evaporated is obtained by subtracting the mass of the anhydrous solid from the mass of the original hydrate ...
2.12: Hydrates. Epsom salt (MgSO 4 ·7H 2 O) is a heptahydrate of magnesium sulfate: within one mole of magnesium sulfate heptahydrate are seven moles of water. This water can be driven off by heat to form the anhydrous (dehydrated) ionic compound, magnesium sulfate. The mass of water evaporated is obtained by subtracting the mass of the ...
Even if magnesium sulfate appears as promising candidate with high theoretical energy density of 2.8 GJ/m −3, technological process is rather complicated. The main problem that thermodynamic and kinetic data are poorly understood to present. In these study salt hydrates equilibrium of magnesium sulfate was investigated by new approach.
Magnesium sulfate or magnesium sulphate is a chemical compound, a salt with the formula MgSO 4, consisting of magnesium cations Mg 2+ (20.19% by mass) and sulfate anions SO 2− 4.It is a white crystalline solid, soluble in water but not in ethanol.. Magnesium sulfate is usually encountered in the form of a hydrate MgSO 4 ·nH 2 O, for various values of n between 1 and 11.
Notice that the compounds are solids (s) ( s) that become ions when dissolved in water, producing an aqueous solution (aq) ( a q). Figure 9.4.5 9.4. 5: Calcium nitrate is a typical ionic compound. In an aqueous solution, it dissociates into calcium ions and nitrate ions. Nonelectrolytes do not dissociate when forming an aqueous solution.
Making magnesium sulfate . Magnesium sulfate is a complex fertiliser. This simple salt is a source of two nutrients - magnesium and sulfur. Magnesium sulfate occurs naturally as the mineral kieserite, MgSO. 4.H. 2. O. This is dissolved in water (usually hot) and the purified sulfate is obtained by crystallisation from solution. Industrially, the
The dissolution equation for this compound is. The dissolution stoichiometry shows a 1:1 relation between the molar amounts of compound and its two ions, and so both [Pb 2+] and are equal to the molar solubility of PbCrO 4: Ksp = [Pb 2+] = (1.4 10 -8 ) (1.4 10 -8) = 2.0 10 -16.
um sulfate (i.e. less than 6 mol H 2 O per mol MgSO 4) and the formation of an amorphous phase have been observed. The hypothesis proposed to explain this phenomenon is that the reorganization of the crystal structure is slow and inhomogeneous. Donkers has studied the water transport on 1-2 mm crystal of heptahydrate of magnesium sulfate by
Data Treatment 1. Write equations for the following changes: (a) Dissolution of anhydrous magnesium sulphate in water. (b) Dissolution of hydrated magnesium sulphate in water. (c) Hydration of anhydrous magnesium sulphate to form hydrated magnesium sulphate-7-water. 2. Calculate the molar enthalpy change of solution of anhydrous magnesium ...
Equation (9) depicts the dissolution of magnesium sulphate in water and Equation (10) and Equation (11) depicts the HSO 4 − formation. The sulphate reacts with water and H + , which results in the formation of the hydrogensulphate ion, responsible for acidifying the solution after crystallization.
We report new measurements of equilibrium relative humidities for stable and metastable hydration-dehydration equilibria involving several magnesium sulfates in the MgSO 4 ·nH 2 O series. We also report a comprehensive thermodynamic treatment of the system including solution properties and experimental data from the published literature, i.e. solubilities, heat capacities and additional ...
The solubility of magnesium sulphate refers to the ability of this compound to dissolve in a solvent, typically water.Magnesium sulphate, also known as Epsom salt, is a white crystalline substance that is commonly used in various applications, including agriculture, medicine, and personal care products.Its solubility in water is an important factor to consider when using it for different purposes.
Step 1: To prepare 100 ml of 1 M Magnesium sulfate, weigh out 24.647 g Magnesium sulfate heptahydrate (MgSO4·7H2O, Mol Wt: 246.47) in a 250 ml conical flask/beaker. Add 800 ml deionized/Milli-Q water and mix until dissolved completely. Tip:One can use manual shaking using a glass pipette to mix the ingredients.
magnesium sulfate. Both types of packs contain a water-filled bag along with the urea or magnesium sulfate powder inside the outer bag. Both types of packs can be purchased on www.amazon.com. • The hand warmer shown in the video in the Extend contains a super-saturated solution of sodium acetate with a small metal disk inside a clear plastic bag.
To prepare 1000 mL of a 0.1 mol/L solution of Magnesium sulfate we have to dissolve 24.6475 g of MgSO4×7H2O (100 % purity) in deionized or distilled water. After the solid is completely dissolved, dilute the solution to a final volume with deionized (distilled) water. we will need to dilute 13.72 mL of 73 % AlCl3×6H2O to a final volume with ...
Then watch the video and on your worksheet write down 1) your observations, 2) molecular equation, 3) ionic equation and 4) net ionic equation for each reaction before moving to the next video. 1. Copper(II)* sulfate and sodium phosphate * The video says Cu 2 SO 4, but the reaction shown in this video is between copper (II) sulfate and sodium ...
In this video we will describe the equation MgSO4 + H2O and write what happens when MgSO4 is dissolved in water.When MgSO4 is dissolved in H2O (water) it wil...
Question: Magnesium sulfate is a soluble salt. Suppose you prepare a 0.025 M solution of MgSO_4. Write the dissolution reaction that occurs when MgSO_4(s) dissolves in water In reality, the following ion pairing reaction also occurs in solutions of MgSO_4: Mg^2+-(aq)+ SO_4^2-(aq) MgSO_4(aq) The ion-pair formation constant K is the equilibrium constant for this
MgSO 4 is an inorganic salt with a chemical name Magnesium sulfate. It is also known as Sulfuric acid magnesium salt or Magnesium sulfate anhydrous. It is commonly referred to as Epsom salt. Magnesium sulfate is a crystalline solid that has a white appearance and is odourless. It is majorly used as a bath soak to get rid of sore muscles, to ...
previous magnesium sulfate crystallizations. A volume of 20 mL was used, and the solutions were stirred with small cylindrical stirrer bars at 250 rpm. It was not possible to control the temperature so closely as in crystallization vessels, and much faster cooling rates were employed, 10−60 °C per hour. The temperatures were recorded to half ...
Epsom salt is magnesium sulfate, so we will use that label going forward. We will be mixing magnesium sulfate with water. When the water and magnesium sulfate are mixed together it forms a solution. A solution is a mixture that has a solute and a solvent. The solute is what is being dissolved. The solvent is what the solute is dissolved into.
To investigate the effect of sulfate on the dissolution rate of magnesium hydrate, a certain amount of MgSO 4 was added into the solution, and the results are shown in Fig. 5. It is clear that sulfate can promote the dissolution process of magnesium hydrate. And the promotion effect seems more obvious with increasing sulfate concentration.
Similarity-based compound selection. Under the overarching goal to find promising magnesium dissolution modulators for the magnesium alloy ZE41 in the vast chemical space, we tested the limits of ...