Urban Education: Challenges and Possibilities
- First Online: 15 September 2020
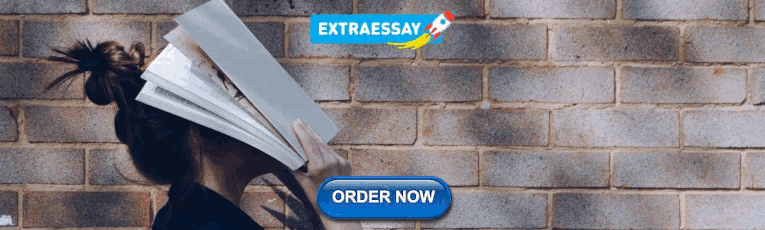
Cite this chapter
- Carlo Raffo 4 ,
- Kirstin Kerr 4 &
- Alan Dyson 4
Part of the book series: Education, Equity, Economy ((EEEC,volume 8))
342 Accesses
Urban education needs a set of conceptual tools that go beyond the simple description of particular urban phenomena, give due acknowledgement to macro-level forces, and also explore local variations in urban contexts that have the potential to expose local possibilities for action. This chapter articulates a newly synthesised discursive conceptual argument about what urban education might mean and how such an argument should become a central way of understanding some of the similar and yet distinct dynamics of education in urban contexts. In essence the broad argument developed in this chapter details a theory of the urban that appreciates the global dynamics of urban processes but does so through a historically and locally understood and articulated sense of place. Then such thinking is embedded in our critique of much of the urban education research literature and is exemplified through an exploration of our thinking with regard to a recent empirical study of young people’s educational aspirations in two urban contexts in Wales, a constituent country of the UK. Building on our exemplified theory of urban education developed in this chapter, we then explore in brief and schematic ways how such thinking might contribute to the challenges and possibilities of education in Central Asia documented in this book. We do so by focusing on educational pathways to labour market transitions in the city of Bishkek, Kyrgyzstan, as a particular case in point. In summary, the chapter argues for the importance of foundational urban theorising, appropriately contextualised, as a way of understanding the social, economic, and cultural foundations upon which young people and urban schooling operate.
This is a preview of subscription content, log in via an institution to check access.
Access this chapter
- Available as EPUB and PDF
- Read on any device
- Instant download
- Own it forever
- Compact, lightweight edition
- Dispatched in 3 to 5 business days
- Free shipping worldwide - see info
- Durable hardcover edition
Tax calculation will be finalised at checkout
Purchases are for personal use only
Institutional subscriptions
Similar content being viewed by others
The ‘Urban’ and Education in India: Section Editor’s Introduction
Common Points Between Urban Development and Education
On the margins of ‘opportunity’: urbanisation and education in delhi’s metropolitan fringe.
The authors of this chapter work in the Disadvantage and Poverty Research Group at the Manchester Institute of Education, a group that is also affiliated to the Manchester Urban Institute’s Spatial Inequalities and Poverty signature theme.
The argument about differently constituted experiences of urban place, identity, and diversity is well documented by Allen and Hollingworth ( 2013 ). In many respects, such research, and our own thinking, critique Beck’s argument (2011) that we need to move wholesale conceptually and methodologically from analytical notions of essential grouped identities linked to multiculturalism to methodological cosmopolitanism. What Beck perhaps fails to recognise is how urban theory can account for analytical differences in diversity generated by the differences in urban processes.
Ainscow, M., & West, M. (Eds.). (2006). Improving urban schools: Leadership and collaboration . Maidenhead: Open University Press.
Google Scholar
Allen, K., & Hollingworth, S. (2013). ‘Sticky subjects’ or ‘cosmopolitan creatives’? Social class, place and urban young people’s aspirations for work in the knowledge economy. Urban Studies, 50 (3), 499–517.
Article Google Scholar
Archer, L., Hollingworth, S., & Mendrick, H. (2010). Urban youth and schooling . Maidenhead: Open University Press.
Archer, L., Dawson, E., DeWitt, J., Godec, S., King, H., Mau, A., Nomikou, E., & Seakins, A. (2018). Using Bourdieu in practice? Urban secondary teachers’ and students’ experiences of a Bourdieusian-inspired pedagogical approach. British Journal of Sociology of Education, 39 (3), 283–298.
Ball, S. J. (2003). Class strategies and the educational market: The middle classes and social advantage . London: Routledge Falmer.
Baumann, A., Jansova, E., Saar, E. (2013). Transition from school to work in Kyrgyzstan: Results of the 2011/12 transition survey , European training foundation report. https://www.etf.europa.eu/sites/default/files/m/F468B191CAD95B8BC1257BBE005530DF_School-to-work%20transition_Kyrgyzstan.pdf . Accessed 9 Sept 2018.
Beck, U. (2011). Multiculturalism or cosmopolitanism: How can we describe and understand the diversity of the world? Social Sciences in China, 32 (4), 52–58.
Bourdieu, P., & Passeron, J. C. (1977). Reproduction in education (Society and culture). London: Sage.
Brenner, N., & Schmid, C. (2015). Towards a new epistemology of the urban. City, 19 , 151–182.
Brighouse, T., & Fullick, L. (Eds.). (2007). Education in a global city. Essays from London . London: Institute of Education.
Bright, G. (2011). ‘Non-servile virtuosi’ in insubordinate spaces: School disaffection, refusal and resistance in a former English coalfield. European Educational Research Journal . https://doi.org/10.2304/eerj.2011.10.4.502 .
Bryk, A. S., Sebring, P. B., Allensworth, E., Luppescu, S., & Easton, J. Q. (2010). Organizing schools for improvement: Lessons from Chicago . Chicago: University of Chicago Press.
Campbell, C., & Whitty, G. (2007). Urban education in the United Kingdom: Section editors introduction. In G. Noblit & W. Pink (Eds.), International handbook of urban education (Springer international handbooks of education) (Vol. 19, pp. 929–942). Dordrecht: Springer.
Castells, M. (1972). La question urbaine . Paris: Maspero.
Cochrane, A. (2006). Understanding urban policy: A critical introduction . Oxford: Blackwell.
Cremin, T., Mottram, M., Collins, F., & Drury, R. (2015). Researching literacy lives: Building communities between home and school . Oxford: Routledge.
DeLanda, M. (2002). Intensive science and virtual philosophy . London: Continuum.
Dillabough, J., & Kennelly, J. (2010). Lost youth in the global city: Class, culture and the urban imaginary . London: Routledge.
Donati, P., & Archer, M. (2015). The relational subject . Cambridge: Cambridge University Press.
Dreier, O. (2008). Learning in structures of social practice. In S. Brinkmann, C. Elmholdt, G. Kraft, P. Musaeus, K. Nielsen, & L. Tanggaard (Eds.), A qualitative stance: Essays in honor of Steinar Kvale (pp. 85–96). Aarhus: Aarhus University Press.
Dreier, O. (2011). Personality and the conduct of everyday life. Nordic Psychology, 63 (2), 4–23.
Duranton, G., & Puga, D. (2004). Micro-foundations of urban agglomeration economies. In J. V. Henderson & J.-F. Thisse (Eds.), Handbook of regional and urban economics . Amsterdam: Elsevier.
Evans, C. (2016). Framing young people’s educational transitions: The role of local and contemporary economic contexts. British Journal of Sociology of Education . https://doi.org/10.1080/01425692.2016.1150154 .
Gonzalez, N., Moll, L., & Amanti, C. (2005). Funds of knowledge: Theorising practices in households, communities and classroom . London: Lawrence Erlbaum.
Government Statistical Service. (2016). Guide to applying the 2011 rural urban classification to data . London: DEFRA.
Grace, G. (Ed.). (1984). Education and the city . Abingdon: Routledge and Kegan Paul.
Grace, G. (2006). Urban education: Confronting the contradictions: An analysis with special reference to London. London Review of Education, 4 (2), 115–131.
Grace, G. (2007). Urban education theory revisited: From the urban question to the end of the millennium. In G. Noblit & W. Pink (Eds.), International handbook of urban education (Springer international handbooks of education) (Vol. 19, pp. 959–978). Dordrecht: Springer.
Gulson, K., & Symes, C. (2007). Knowing one's place: Space, theory, education. Critical Studies in Education, 48 (1), 97–110.
Harvey, D. (1973). Social justice and the city . London: Edward Arnold.
Harvey, D. (2007). A brief history of neoliberalism . Oxford: Oxford University Press.
Harvey, D. (2012). Rebel cities: From the right to the city to the urban revolution . London: Verso.
Hodgson, A., & Spours, K. (2013). An ecological analysis of the dynamics of localities: A 14+ low opportunity progression equilibrium in action. Journal of Education and Work . https://doi.org/10.1080/13639080.2013.80518 .
Hubbard, P., Kitchin, R., & Valentine, G. (2004). Editors’ introduction. In P. Hubbard, R. Kitchin, & G. Valentine (Eds.), Key thinkers on space and place (pp. 1–15). London: Sage.
Jackson, P. (1989). Geography, race and racism. In R. Peet & N. Thrift (Eds.), New models in geography (Vol. 2). London: Unwin Hymen.
Kerr, K., Dyson, A., & Raffo, C. (2014). Education, disadvantage and place: Making the local matter . Bristol: Policy Press.
Latour, B. (2005). Reassembling the social: An introduction to actor-network theory . Oxford: Oxford University Press.
Lefebvre, H. (1970). La révolution urbaine . Paris: Gallimard.
Levin, B. (2008). How to change 5000 schools: A practical and positive approach for leading change at every level . Cambridge: Harvard Education Press.
Lipman, P. (2004). High stakes education . London: Routledge Falmer.
Lipman, P. (2011). The new political economy of urban education: Neoliberalism, race, and the right to the City . New York: Routledge.
Lipman, P. (2013). Economic crisis, accountability, and the state’s coercive assault on public education in the USA. Journal of Education Policy, 28 (5), 557–573. https://doi.org/10.1080/02680939.2012.758816 .
Lupton, R. (2016). Re-thinking values and schooling in white working class neighbourhoods. In C. Timmerman, N. Clycq, M. McAndrew, B. Alhassane, L. Braeckmans, & S. Mels (Eds.), Youth in education: The necessity of valuing ethnocultural diversity (pp. 233–248). New York: Routledge.
Maguire, M., Wooldridge, T., & Pratt-Adams, S. (2006). The urban primary school . Maidenhead: Open University Press.
Massey, D. (1991). Flexible sexism. Environment and Planning D: Society and Space, 9 (1), 31–57.
McFarlane, C. (2011). Assemblage and critical urbanism. City, 15 (2), 204–224.
Mould, O. (2015). A limitless urban theory? A response to Scott and Storper’s ‘the nature of cities: The scope and limits of urban theory’. International Journal of Urban and Regional Research . https://doi.org/10.1111/1468-2427.12288 .
Noblit, G., & Pink, W. (2007). Introduction: Urban education in the globalizing world. In G. W. Noblit & W. T. Pink (Eds.), International handbook of urban education (Vol. 19, pp. xv–xxxvi). Dordrecht: Springer.
Ofsted (2013). Unseen children: Educational access and achievement 20 years on: Evidence report. . https://www.gov.uk/government/publications/unseen-children-access-and-achievement-20-years-on . Accessed 18 Oct 2018.
Park, R. E., Burgess, E. W., & McKenzie, R. D. (1925). The city . Chicago: University of Chicago Press.
Phillips, D. (2005). Policy borrowing in education: Frameworks for analysis. In J. Zajda (Ed.), International handbook on globalisation, education and policy research (pp. 23–34). Dordrecht: Springer.
Pink, W. T., & Noblit, G. W. (2017). Globalised urban education: Tracking change. In W. T. Pink & G. W. Noblit (Eds.), Second international handbook of urban education (pp. li–lxvi). Dordrecht: Springer.
Pinoncely, V. (2014). Poverty, place and inequality: Why place-based approaches are key to tackling poverty and inequality. Policy paper RTBI, Scotland. http://www.rtpi.org.uk/media/1811222/poverty_place_and_inequality.pdf . Accessed 18 Oct 2018.
Raffo, C. (2011). Educational equity in poor urban contexts: Exploring issues of place/space and young People's identity and agency. British Journal of Educational Studies, 59 (1), 1–19.
Raffo, C. (2014). Improving educational equity in urban contexts . Abingdon: Routledge.
Raffo, C., & Dyson, A. (2007). Full service extended schools and educational inequality in urban contexts--new opportunities for progress? Journal of Education Policy, 22 (5), 263–282.
Raffo, C., Dyson, A., Kerr, K. (2014). Positive discrimination in European education and training systems--lessons from the implementation of area-based initiatives for policy and practice (an independent report authored for the European Commission by the NESET network of experts). http://www.nesetweb.eu/news/neset-report-lessons-area-based-initiatives-education-and-training . Accessed 18 Oct 2018.
Reay, D., Crozier, G., & James, D. (2011). White middle-lass identities and urban schooling . Basingstoke: Palgrame Macmillan.
Rees, G., Power, S., & Taylor, C. (2007). The governance of educational inequalities: The limits of area-based initiatives. Journal of Comparative Policy Analysis, 9 (3), 261–274.
Roberts, K., Kamruzzaman, P., & Tholen, J. (2009). Young people’s education to work transitions and inter-generational social mobility in post-soviet Central Asia. Nordic Journal of Youth Research, 17 (1), 59–80.
Roth, W.-M. (2016). Concrete human psychology . New York: Routledge.
Rubery, J., Johnson, M., Lupton, R., Zapata Roman, G. (2017). Human development report for Greater Manchester. http://www.research.mbs.ac.uk/ewerc/Portals/0/Documents/human-development-report.pdf . Accessed 18 Oct 2018.
Said, E. W. (1978). Orientalism . New York: Pantheon.
Sampson, R. J., Raudenbush, S. W., & Earls, F. (1997). Neighborhoods and violent crime: A multilevel study of collective efficacy. Science, 277 , 918–924.
Sassen, S. (1991). The global city: New York, London, Tokyo . Princeton: Princeton University Press.
Schröder, P. (2016). Urban spaces and lifestyles in Central Asia and beyond: An introduction. Central Asian Survey, 35 (2), 145–156. https://doi.org/10.1080/02634937.2016.1155862 .
Scott, A. J., & Storper, M. (2015). The nature of cities: The scope and limits of urban theory. International Journal of Urban and Regional Research, 39 (1), 1–15.
Spicker, P. (2001). Poor areas and the 'ecological fallacy'. Radical Statistics, 76 (Winter). http://www.radstats.org.uk/no076/spicker.htm . Accessed 18 Oct 2018.
Spivak, G. C. (2008). Can the subaltern speak? In C. Nelson & L. Grossberg (Eds.), Marxism and the interpretation of culture (pp. 271–313). Macmillan: Basingstoke.
Storper, M., & Scott, A. J. (2016). Current debates in urban theory: A critical assessment. Urban Studies, 53 (6), 1114–1136.
Taylor, I., Evans, K., & Fraser, P. (1996). A tale of two cities: Global change, local feeling and everyday life in the North of England. A Study in Manchester and Sheffield . London: Routledge.
Thomson, P. (2002). Schooling the rustbelt kids: Making the difference in changing times . Stoke-on-Trent: Trentham Books.
Thrupp, M., & Lupton, R. (2006). Taking school contexts more seriously: The social justice challenge. British Journal of Educational Studies, 54 (3), 308–328.
Townsend, T. (Ed.). (2007). International handbook of school effectiveness and improvement: Review, reflection and reframing . Dordrecht: Springer.
Waldinger, R., & Bozorgmehr, M. (Eds.). (1996). Ethnic Los Angeles . New York: Russell Sage Foundation.
Wessendorf, S. (2016). Settling in a super-diverse context: Recent migrants’ experiences of conviviality. Journal of Intercultural Studies, 37 (5), 449–463.
Williams, R. (1977). Marxism and literature . Oxford: Oxford University Press.
Williams, S., Dodd, L. J., Steele, C., & Randall, R. (2015). A systematic review of current understandings of employability. Journal of Education and Work . https://doi.org/10.1080/13639080.2015.1102210 .
Wirth, L. (1938). Urbanism as a way of life. American Journal of Sociology, 44 (1), 1–24.
Download references
Acknowledgements
Our gratitude goes to Ruth Lupton, Helen Gunter, Wolff-Michael Roth, Kevin Ward, Philipp Schröder, and Denise Egéa for their perceptive comments on earlier drafts of this chapter.
Author information
Authors and affiliations.
Manchester Institute of Education, University of Manchester, Manchester, UK
Carlo Raffo, Kirstin Kerr & Alan Dyson
You can also search for this author in PubMed Google Scholar
Corresponding author
Correspondence to Carlo Raffo .
Editor information
Editors and affiliations.
Louisiana State University, Baton Rouge, LA, USA, Nazarbayev University, Nur-Sultan, Kazakhstan
Denise Egéa
Rights and permissions
Reprints and permissions
Copyright information
© 2020 The Editor(s) (if applicable) and The Author(s), under exclusive license to Springer Nature Switzerland AG
About this chapter
Raffo, C., Kerr, K., Dyson, A. (2020). Urban Education: Challenges and Possibilities. In: Egéa, D. (eds) Education in Central Asia. Education, Equity, Economy, vol 8. Springer, Cham. https://doi.org/10.1007/978-3-030-50127-3_11
Download citation
DOI : https://doi.org/10.1007/978-3-030-50127-3_11
Published : 15 September 2020
Publisher Name : Springer, Cham
Print ISBN : 978-3-030-50126-6
Online ISBN : 978-3-030-50127-3
eBook Packages : Education Education (R0)
Share this chapter
Anyone you share the following link with will be able to read this content:
Sorry, a shareable link is not currently available for this article.
Provided by the Springer Nature SharedIt content-sharing initiative
- Publish with us
Policies and ethics
- Find a journal
- Track your research
VUE is an open-access journal published twice annually that endeavors to serve as a “roundtable-in-print” by bringing together diverse education stakeholders with a wide range of viewpoints, including leading education writers and thinkers and essential but frequently underrepresented voices in educational scholarship, such as students, parents, teachers, activists, and community members.
Each issue of VUE is organized around a theme and strives to provide cutting-edge analysis of a vital issue in urban public education—formats include visual arts, articles, interviews, video and written documentaries, poetry, and autoethnographies.
Our newly structured editorial board currently runs VUE. It consists of our lead editorial team, composed of faculty members in the roles of the editor-in-chief and the deputy editor. The Editorial Board also includes doctoral fellows who step into Senior Fellows, Junior Fellows, and Content Editor Fellows positions. These doctoral students join the board from various higher education institutions and bring a breadth of expertise to the board.
DOI: https://doi.org/10.35240/vue.collection.6
Introduction
The Enduring Fight for Justice
Matt Gonzales, Barbara Gross and Fabienne Doucet
2023-11-15 Volume 52 • Issue 1 • 2023 • Chasing Equity: Fighting for Justice in New York City's Schools • 1-2
Commentaries in Urban Education
Depolicing Schools
Norman Fruchter
2023-11-15 Volume 52 • Issue 1 • 2023 • Chasing Equity: Fighting for Justice in New York City's Schools • 3-4
An Alternative High School and a Police Chief
2023-11-15 Volume 52 • Issue 1 • 2023 • Chasing Equity: Fighting for Justice in New York City's Schools • 5-7
Refusing Schooling in the U.K. and the U.S.
Norman Fruchter and Christina Mokhtar-Ross
2023-11-15 Volume 52 • Issue 1 • 2023 • Chasing Equity: Fighting for Justice in New York City's Schools • 8-11
I Can't Read
2023-11-15 Volume 52 • Issue 1 • 2023 • Chasing Equity: Fighting for Justice in New York City's Schools • 12-14
“I Felt Like I Never Fit in”: Increasing College Access and Persistence
2023-11-15 Volume 52 • Issue 1 • 2023 • Chasing Equity: Fighting for Justice in New York City's Schools • 15–18
Recuperation and Innovation: New York City's Transfer High Schools
2023-11-15 Volume 52 • Issue 1 • 2023 • Chasing Equity: Fighting for Justice in New York City's Schools • 19-22
New York City's Small Schools
2023-11-15 Volume 52 • Issue 1 • 2023 • Chasing Equity: Fighting for Justice in New York City's Schools • 23-25
New York City's Affinity School District
2023-11-15 Volume 52 • Issue 1 • 2023 • Chasing Equity: Fighting for Justice in New York City's Schools • 26-31
Towards Career Apprenticeships
2023-11-15 Volume 52 • Issue 1 • 2023 • Chasing Equity: Fighting for Justice in New York City's Schools • 32-34
COVID 19, the 1918 Spanish Flu, and the City's Schools
2023-11-15 Volume 52 • Issue 1 • 2023 • Chasing Equity: Fighting for Justice in New York City's Schools • 35-37
Vulnerable Schools and COVID-19
2023-11-15 Volume 52 • Issue 1 • 2023 • Chasing Equity: Fighting for Justice in New York City's Schools • 38-40
School Districts’ COVID Decisions
2023-11-15 Volume 52 • Issue 1 • 2023 • Chasing Equity: Fighting for Justice in New York City's Schools • 41-43
The RECS–A Successful Pandemic Learning Experiment
2023-11-15 Volume 52 • Issue 1 • 2023 • Chasing Equity: Fighting for Justice in New York City's Schools • 44-46
Reducing New York City's Wage-Earner Inequity through Universal Pre-K
2023-11-15 Volume 52 • Issue 1 • 2023 • Chasing Equity: Fighting for Justice in New York City's Schools • 47–48
Rebuilding our City's Safety Net–Early Childcare
2023-11-15 Volume 52 • Issue 1 • 2023 • Chasing Equity: Fighting for Justice in New York City's Schools • 49–51
Universal Free Meals in NYC Schools
2023-11-15 Volume 52 • Issue 1 • 2023 • Chasing Equity: Fighting for Justice in New York City's Schools • 52–53
Redefining Infrastructure: Schools in a Care Economy
Norman Fruchter and Robert Gottlieb
2023-11-15 Volume 52 • Issue 1 • 2023 • Chasing Equity: Fighting for Justice in New York City's Schools • 54–55
A Successful College Access Experiment Reaches Citywide Scale
2023-11-15 Volume 52 • Issue 1 • 2023 • Chasing Equity: Fighting for Justice in New York City's Schools • 56–58
Equity Audits: Stakeholders Making Education Policy
2023-11-15 Volume 52 • Issue 1 • 2023 • Chasing Equity: Fighting for Justice in New York City's Schools • 59–61
Mayoral Control and the Panel for Educational Policy (PEP)
2023-11-15 Volume 52 • Issue 1 • 2023 • Chasing Equity: Fighting for Justice in New York City's Schools • 62–64
Funding Problems for City Schools
2023-11-15 Volume 52 • Issue 1 • 2023 • Chasing Equity: Fighting for Justice in New York City's Schools • 65–67
Revisiting the DOE’s Fair Student Funding Formula
2023-11-15 Volume 52 • Issue 1 • 2023 • Chasing Equity: Fighting for Justice in New York City's Schools • 68–71
Historic Steps towards Funding Equity for NYC's students
2023-11-15 Volume 52 • Issue 1 • 2023 • Chasing Equity: Fighting for Justice in New York City's Schools • 72–75
Opportunity to Learn
2023-11-15 Volume 52 • Issue 1 • 2023 • Chasing Equity: Fighting for Justice in New York City's Schools • 76–77
Misplaced: Mistreating Developmental Difference
2023-11-15 Volume 52 • Issue 1 • 2023 • Chasing Equity: Fighting for Justice in New York City's Schools • 78–81
Restructuring Special Education: The School-Based Model
Norman Fruchter, Christina Mokhtar-Ross and Dorothy Siegel
2023-11-15 Volume 52 • Issue 1 • 2023 • Chasing Equity: Fighting for Justice in New York City's Schools • 82–84
Black Political Statements Across Time
2023-11-15 Volume 52 • Issue 1 • 2023 • Chasing Equity: Fighting for Justice in New York City's Schools • 85–89
Scaling Reform in New York City Schools
2023-11-15 Volume 52 • Issue 1 • 2023 • Chasing Equity: Fighting for Justice in New York City's Schools • 90–92
Reducing Segregation: What Could the Mayor Do?
2023-11-15 Volume 52 • Issue 1 • 2023 • Chasing Equity: Fighting for Justice in New York City's Schools • 93–100
Structural Racism Begins in Kindergarten
2023-11-15 Volume 52 • Issue 1 • 2023 • Chasing Equity: Fighting for Justice in New York City's Schools • 101–105
The Spoils of Whiteness: New York City’s Gifted and Talented Programs
2023-11-15 Volume 52 • Issue 1 • 2023 • Chasing Equity: Fighting for Justice in New York City's Schools • 106–110
Monopolizing Whiteness
2023-11-15 Volume 52 • Issue 1 • 2023 • Chasing Equity: Fighting for Justice in New York City's Schools • 111–113
“Unlovely Student Distributions”: A Rebuttal to Chester Finn’s “Even More Social Engineering in New York Schools"
2023-11-15 Volume 52 • Issue 1 • 2023 • Chasing Equity: Fighting for Justice in New York City's Schools • 114–117
Posted by Pharoah Cranston on 2023-11-20
NYU Metro Center is overjoyed to present the latest issue of Voices in Urban Education (VUE). Each issue of VUE endeavors to serve as a roundtable-in-print by bringing together diverse education stakeholders with a wide range of viewpoints, including leading education writers and thinkers, as well as essential but frequently underrepresented voices in educational scholarship, such as students, [...]
Posted by Pharoah Cranston on 2023-07-24
Newly minted laws force families with trans children to seek gender reaffirming care in states other than where they reside. Twenty different states across the country “... have enacted laws restricting or banning gender-affirming care for trans minors …”(Alfonseca, 2023). This contemporary moment finds that queer and trans youth, “... and what they do with their own bodies and lives, are [...]
Posted by Paloma Garcia on 2023-04-19
Voices in Urban Education, VUE, is NYU Metro Center’s open-access journal. Each issue of VUE is organized around a theme and strives to provide cutting-edge analysis of a vital issue in urban public education. This “roundtable-in-print” endeavors to bring together diverse education stakeholders with a wide range of viewpoints, including leading education writers and thinkers, as well as essential [...]
An Economic Perspective on Urban Education
Subscribe to the economic studies bulletin, janet rothenberg pack , jrp janet rothenberg pack former brookings expert meghan mcnally , and mm meghan mcnally william g. gale william g. gale the arjay and frances fearing miller chair in federal economic policy, senior fellow - economic studies , co-director - urban-brookings tax policy center @williamgale2.
June 1, 2003
- 11 min read
Participants in the annual symposium on The Brookings-Wharton Papers on Urban Affairs —convened by Brookings and the University of Pennsylvania’s Wharton School—present research on issues unique to urban areas as well as on broader economic and policy topics that can apply to urban settings. This year’s participants focused on urban education and presented findings on the results of an experiment designed to detect cheating on standardized tests, the impact of school reform in an urban setting, the effect of school quality on housing values, and the determinants of improved academic performance. Two other studies addressed other urban economic issues: the increase in economic inequality across and within geographic regions, and local variation in land-use regulations. This year’s Brookings-Wharton symposium took place at Brookings in October 2002. The resulting bound volume is due out this month from the Brookings Institution Press.
Symposium on Urban Education
Education is one of the most important services provided by urban and local governments. But low test scores, high dropout rates, high teacher turnover rates, and other problems show that large urban school districts in the United States serve their students poorly. These deficiencies have led affluent families to leave cities for the suburbs or to move their children to private schools. When these families move, urban tax bases and economic activity are reduced. When good students move to private schools, the average academic quality of the remaining public school students declines, which can reduce the quality of the education received in the public schools through its influence on peer group effects and expenditure on schools. For all of these reasons, education reform has emerged as a key issue in urban areas.
Catching Cheating Teachers
One approach to improving urban schools involves increased emphasis on high-stakes testing, under which schools are held accountable, through a variety of sanctions, for their students? failure to exceed the thresholds on standardized tests. Supporters claim that testing provides accountability and raises test scores. Critics note that test score gains have been shown to be test-specific and thus may be ephemeral. Another concern is that the emphasis on high-stakes testing may increase the temptation for students, teachers, and administrators to cheat on standardized tests.
Brian A. Jacob of Harvard University and Steven D. Levitt of the University of Chicago examine cheating by teachers using a methodology they developed in previous work. Roughly 100,000 Chicago public school students take the Iowa Test of Basic Skills each spring, with retesting in 117 classrooms three to four weeks later. Jacob and Levitt analyze these results, controlling for past, current, and retest scores, demographics, and socioeconomic characteristics as explanatory variables to identify suspicious answer strings (see figure 1). The authors look at cheating in three types of classrooms:
- Classrooms with unusually large test score gains and highly suspicious patterns of answer strings
- Classrooms with suspicious answer strings but without unusually large test score gains
- Classrooms with anonymous allegations of cheating
The authors compare the retest performance of these classrooms to two control groups that are not suspected of cheating: one group with large test score gains but no suspicious answer strings, and one group chosen at random.
The main result is that classrooms that were suspected of cheating saw dramatic declines in test results in the retest relative to the original test, while the control classrooms saw little change in test results. Out of the approximately seventy suspicious classrooms that were retested, nearly all experienced test score declines that were significant. In addition, after the retest, school administrators in two schools were suspected of complicity in cheating because of the large number of classes in their schools identified as having cheated. Consequently, both the teachers and administrators suspected of cheating were subject to further investigation.
Public School Finance and Urban School Policy
Much of the analysis of school quality determinants focuses narrowly on the effects of financial resources on school performance. In contrast, Thomas Nechyba of Duke University explores how financing alters school quality in a framework that explicitly incorporates the fact that financing changes may alter the characteristics of neighborhoods, private school attendance rates, and political voting outcomes. He develops these insights in a simulation model calibrated to data from New Jersey. By examining the various policies in a single, consistent model, he is able to isolate the interlocking roles of different factors in determining the impact of changes in school finance. Nechyba examines the effects of centralizing school finance, changing state aid formulas, and issuing state-funded vouchers. A major result of Nechyba’s analysis is that the indirect effects of policy changes on school quality—for example, those that arise from households moving or students changing from public to private schools—often have a greater impact on school performance than the direct effects of funding levels and the availability of resources.
Among the specific results: centralizing school finance raises housing prices, reduces private school attendance, reduces spending per pupil in public schools, and narrows school quality differences across districts. Funding formulas that provide state aid not targeted at poor school districts result in school improvement in wealthy districts and also cause larger inequalities across districts. However, targeted state aid administered only to poor school districts achieves greater increases in school quality for all schools. State-funded vouchers reduce quality at poor-performing public schools as students choose to attend better public and private schools. At the same time, families in wealthier neighborhoods move to areas with more affordable housing and send their children to private schools using vouchers. As a result, overall school quality in poor districts rises slightly because of the increased quality of the new private schools. Vouchers have a negative impact on the public schools in wealthier districts as affluent families move out, causing a decline in quality and support of the public schools.
School Accountability Ratings and Housing Values
Previous research has found that there is a strong connection between student test scores at neighborhood schools and housing values at a point in time, but there is less evidence on how those variables evolve over time. This issue, however, will become more important in the future, because the No Child Left Behind Act of 2001 requires states to test all students in grades three through eight and publicly report the results.
Thomas J. Kane of UCLA, Douglas O. Staiger of Dartmouth College, and Gavin Samms of Harvard University evaluate the effects on housing values of test scores and composite school ratings. Using data on the sales prices of homes in Mecklenburg County, North Carolina, they find that a school’s long-term average test scores affect housing values in the immediate neighborhood, but that year-to-year fluctuations in scores do not. This suggests that homeowners care about school quality, but are aware that test scores are an imperfect indicator of neighborhood quality.
The authors also find that test scores may be related to unmeasured housing and neighborhood characteristics. This suggests that people tend to buy houses where they do because of their peers. Housing values tend to be higher closer to a neighborhood school, particularly in poor neighborhoods, and are more responsive to the test scores of white students than of black students. The study also shows that, when composite school ratings were introduced, local house prices did not decline in response to state ratings of “low-performing” for local schools. This may imply that residents already knew that these schools were low-performing.
Lessons from Chicago
After instituting high-stakes testing in 1996, the Chicago Public Schools realized a significant increase in test scores. Little is understood, however, about the channels through which high-stakes testing affects performance. Supporters of the program claim that testing makes teachers and students work harder and helps schools become more efficient. Critics claim the rules lead to “teaching to the test” and to reallocations of resources away from non-tested subjects like physical education, art, music, and social studies.
Brian Jacob examines the effects of the accountability policies instituted by the Chicago Public Schools (CPS) using school budget information for 456 elementary schools in 1995 and 2000. He distinguishes between changes in educational inputs—including student effort, parent involvement, and financial resources—and changes in school technology, such as instructional practices and school organization.
Jacob finds that most of the improvement in CPS scores comes from non-financial inputs like student effort and parental involvement rather than technological improvements. Schools made few changes to financial allocations or school organization. For example, low-achieving schools shifted only a small amount of resources away from non-tested areas, like art and music, and the changes in spending are not related to changes in student achievement. Most of the increase in spending was to hire more teachers or raise teacher salaries rather than to create new positions. Changes in educational efforts by students—including participation in after-school programs to help them with homework—had a greater effect on student achievement than changes in instructional practices or school organization. The findings will prove relevant as the federal government begins to implement the No Child Left Behind legislation that was enacted in 2001.
Other Research
In addition to the papers on education reform, the Brookings-Wharton symposium addressed two other urban economic issues:
The Geography of Inequality
Although trends in income inequality in the United States have received a substantial amount of attention over the past two decades, trends in the geographic distribution of income have been left relatively unexamined. As the affluent and poor become increasingly isolated from one another, the social and economic worlds and interests of the two groups will naturally diverge, with important implications for public finance and the provision of public services.
Using data available from 1950 to 2000, Douglas S. Massey and Mary J. Fischer of the University of Pennsylvania measure trends in class segregation and income concentration at the regional, state, and metropolitan levels, and on the neighborhood level for sixty metropolitan areas. The authors find that income and class segregation declined over the last half century as the rich and poor have become more evenly distributed throughout the country. They also show that the degree of spatial separation between affluent and poor families declined at the regional, state, and metropolitan levels.
The concentration of affluence and poverty, however, has increased in neighborhoods, leading to significant class segregation within metropolitan areas.
Income segregation measures the degree of social and class segregation between poor and affluent families. Income segregation is lowest on the regional level, having declined by more than half between 1950 and 2000. At the state level, income segregation decreased by nearly 40 percent, while in metropolitan areas, it decreased by one-third. However, census tract-level income segregation in sixty metropolitan areas increased dramatically between 1970 and 2000, indicating significant growth in class segregation within neighborhoods.
Massey and Fischer also examined income segregation by race and found that in the regions, states, and metropolitan areas, both blacks and whites experienced a comparable decrease in income segregation over the period. Neighborhood data tell a different story: income segregation within both races has increased since 1970 and moderated only slightly during the 1990s.
Poverty Concentration
Falling income inequality, declining poverty rates, and class segregation from 1950 to 2000 also produced declines in the geographic concentration of poverty at the regional, state, and metropolitan levels. Overall, the concentration of poverty fell sharply and hit a fifty-year low in 2000. Poverty isolation is greater for African Americans, since far more blacks live in the south and thus are distributed more unevenly than whites. The concentration of poverty in neighborhoods of metropolitan areas, in contrast to the decrease in overall concentration, increased by 80 percent between 1970 and 2000. Moreover, the concentration of poverty within neighborhoods for black families exceeded that for white families in each decade from 1970 through 2000.
According to Massey and Fischer, the concentration of affluence in regions, states and metropolitan areas increased between 1950 and 2000. Since class segregation and income inequality declined, the growth in the concentration of affluence is attributed to the increasing proportion of affluent families. This is also true on the neighborhood level. By 2000, the concentration of affluence in metropolitan neighborhoods was greater than the concentration of poverty. However, the authors find that the concentration of affluence does not differ by race in regions, states, or metropolitan areas within neighborhoods. On the other hand, the concentration of affluence was greater for whites than blacks within neighborhoods.
Massey and Fischer show that rich and poor families came to inhabit the same regions, states, and metropolitan areas while simultaneously moving into different neighborhoods segregated by class. Class segregation grew during the 1970s and the concentration of poverty increased through the 1980s. But the trends leveled off through the 1990s, indicating that because of that decade?s great prosperity, the situation has not deteriorated significantly. However, the trends also have not been reversed. Growing concentrations of poverty and affluence within neighborhoods indicates an increasingly divided society where rich people live in safe communities interacting with affluent peers and the poor live in high-risk, unstable neighborhoods with few prospects for economic development or social mobility.
Land-Use Regulations
The causes and effects of local land use regulation are long-standing topics of concern to researchers and policymakers, but to date little evidence has been brought to bear on this issue. Bengte Evenson of Illinois State University and William C. Wheaton of MIT use a unique dataset created for the state of Massachusetts to study local land-use regulations. They analyzed patterns of residential, commercial, industrial, open, and other land-use allocations.
The authors found that current zoning regulations of a town are a strong indicator of future zoning and development. Wealthy towns tend to protect more land and allow for less housing and commercial/industrial development while poorer towns tend to permit higher density commercial/industrial development. However, a town?s current residential or commercial density is a much better indicator of expected future density than income. In addition, Evenson and Wheaton find that towns further from Boston zone less open land for commercial/industrial uses, protect more open land, and put tighter constraints on density.
The authors’ findings support the current theoretical land-use literature and conventional wisdom. In the future, the Massachusetts data can be used to further test land-use theory and examine patterns of development.
Economic Studies
Vanessa Williamson
April 29, 2024
Richard V. Reeves, Ember Smith
April 26, 2024
Phillip Levine
April 12, 2024
- Search Menu
- Browse content in A - General Economics and Teaching
- Browse content in A1 - General Economics
- A12 - Relation of Economics to Other Disciplines
- A13 - Relation of Economics to Social Values
- A14 - Sociology of Economics
- Browse content in B - History of Economic Thought, Methodology, and Heterodox Approaches
- Browse content in B2 - History of Economic Thought since 1925
- B25 - Historical; Institutional; Evolutionary; Austrian
- B26 - Financial Economics
- Browse content in B4 - Economic Methodology
- B40 - General
- B41 - Economic Methodology
- Browse content in B5 - Current Heterodox Approaches
- B52 - Institutional; Evolutionary
- Browse content in C - Mathematical and Quantitative Methods
- Browse content in C0 - General
- C01 - Econometrics
- Browse content in C1 - Econometric and Statistical Methods and Methodology: General
- C11 - Bayesian Analysis: General
- C12 - Hypothesis Testing: General
- C14 - Semiparametric and Nonparametric Methods: General
- C15 - Statistical Simulation Methods: General
- Browse content in C2 - Single Equation Models; Single Variables
- C21 - Cross-Sectional Models; Spatial Models; Treatment Effect Models; Quantile Regressions
- C23 - Panel Data Models; Spatio-temporal Models
- C25 - Discrete Regression and Qualitative Choice Models; Discrete Regressors; Proportions; Probabilities
- C26 - Instrumental Variables (IV) Estimation
- Browse content in C3 - Multiple or Simultaneous Equation Models; Multiple Variables
- C31 - Cross-Sectional Models; Spatial Models; Treatment Effect Models; Quantile Regressions; Social Interaction Models
- C33 - Panel Data Models; Spatio-temporal Models
- C38 - Classification Methods; Cluster Analysis; Principal Components; Factor Models
- Browse content in C4 - Econometric and Statistical Methods: Special Topics
- C40 - General
- C41 - Duration Analysis; Optimal Timing Strategies
- C49 - Other
- Browse content in C6 - Mathematical Methods; Programming Models; Mathematical and Simulation Modeling
- C60 - General
- C61 - Optimization Techniques; Programming Models; Dynamic Analysis
- C62 - Existence and Stability Conditions of Equilibrium
- Browse content in C9 - Design of Experiments
- C90 - General
- C99 - Other
- Browse content in D - Microeconomics
- Browse content in D0 - General
- D00 - General
- D01 - Microeconomic Behavior: Underlying Principles
- D02 - Institutions: Design, Formation, Operations, and Impact
- D03 - Behavioral Microeconomics: Underlying Principles
- Browse content in D1 - Household Behavior and Family Economics
- D13 - Household Production and Intrahousehold Allocation
- D14 - Household Saving; Personal Finance
- D19 - Other
- Browse content in D2 - Production and Organizations
- D21 - Firm Behavior: Theory
- D22 - Firm Behavior: Empirical Analysis
- D23 - Organizational Behavior; Transaction Costs; Property Rights
- D24 - Production; Cost; Capital; Capital, Total Factor, and Multifactor Productivity; Capacity
- Browse content in D3 - Distribution
- D31 - Personal Income, Wealth, and Their Distributions
- Browse content in D4 - Market Structure, Pricing, and Design
- D41 - Perfect Competition
- D43 - Oligopoly and Other Forms of Market Imperfection
- Browse content in D5 - General Equilibrium and Disequilibrium
- D53 - Financial Markets
- Browse content in D6 - Welfare Economics
- D61 - Allocative Efficiency; Cost-Benefit Analysis
- D62 - Externalities
- D63 - Equity, Justice, Inequality, and Other Normative Criteria and Measurement
- D64 - Altruism; Philanthropy
- Browse content in D7 - Analysis of Collective Decision-Making
- D72 - Political Processes: Rent-seeking, Lobbying, Elections, Legislatures, and Voting Behavior
- D74 - Conflict; Conflict Resolution; Alliances; Revolutions
- D78 - Positive Analysis of Policy Formulation and Implementation
- Browse content in D8 - Information, Knowledge, and Uncertainty
- D80 - General
- D81 - Criteria for Decision-Making under Risk and Uncertainty
- D82 - Asymmetric and Private Information; Mechanism Design
- D83 - Search; Learning; Information and Knowledge; Communication; Belief; Unawareness
- D85 - Network Formation and Analysis: Theory
- Browse content in D9 - Micro-Based Behavioral Economics
- D92 - Intertemporal Firm Choice, Investment, Capacity, and Financing
- Browse content in E - Macroeconomics and Monetary Economics
- Browse content in E2 - Consumption, Saving, Production, Investment, Labor Markets, and Informal Economy
- E21 - Consumption; Saving; Wealth
- E22 - Investment; Capital; Intangible Capital; Capacity
- E24 - Employment; Unemployment; Wages; Intergenerational Income Distribution; Aggregate Human Capital; Aggregate Labor Productivity
- Browse content in E3 - Prices, Business Fluctuations, and Cycles
- E32 - Business Fluctuations; Cycles
- Browse content in E4 - Money and Interest Rates
- E40 - General
- Browse content in E5 - Monetary Policy, Central Banking, and the Supply of Money and Credit
- E58 - Central Banks and Their Policies
- Browse content in F - International Economics
- Browse content in F0 - General
- F00 - General
- F02 - International Economic Order and Integration
- Browse content in F1 - Trade
- F10 - General
- F11 - Neoclassical Models of Trade
- F12 - Models of Trade with Imperfect Competition and Scale Economies; Fragmentation
- F13 - Trade Policy; International Trade Organizations
- F14 - Empirical Studies of Trade
- F15 - Economic Integration
- F16 - Trade and Labor Market Interactions
- F18 - Trade and Environment
- Browse content in F2 - International Factor Movements and International Business
- F20 - General
- F21 - International Investment; Long-Term Capital Movements
- F22 - International Migration
- F23 - Multinational Firms; International Business
- Browse content in F3 - International Finance
- F30 - General
- F31 - Foreign Exchange
- F32 - Current Account Adjustment; Short-Term Capital Movements
- F33 - International Monetary Arrangements and Institutions
- F34 - International Lending and Debt Problems
- F36 - Financial Aspects of Economic Integration
- Browse content in F4 - Macroeconomic Aspects of International Trade and Finance
- F40 - General
- F41 - Open Economy Macroeconomics
- F43 - Economic Growth of Open Economies
- F44 - International Business Cycles
- Browse content in F5 - International Relations, National Security, and International Political Economy
- F50 - General
- F52 - National Security; Economic Nationalism
- F55 - International Institutional Arrangements
- Browse content in F6 - Economic Impacts of Globalization
- F60 - General
- F61 - Microeconomic Impacts
- F63 - Economic Development
- F65 - Finance
- F66 - Labor
- F68 - Policy
- Browse content in G - Financial Economics
- Browse content in G0 - General
- G00 - General
- G01 - Financial Crises
- G02 - Behavioral Finance: Underlying Principles
- Browse content in G1 - General Financial Markets
- G10 - General
- G11 - Portfolio Choice; Investment Decisions
- G12 - Asset Pricing; Trading volume; Bond Interest Rates
- G14 - Information and Market Efficiency; Event Studies; Insider Trading
- G15 - International Financial Markets
- G18 - Government Policy and Regulation
- Browse content in G2 - Financial Institutions and Services
- G20 - General
- G21 - Banks; Depository Institutions; Micro Finance Institutions; Mortgages
- G22 - Insurance; Insurance Companies; Actuarial Studies
- G23 - Non-bank Financial Institutions; Financial Instruments; Institutional Investors
- G24 - Investment Banking; Venture Capital; Brokerage; Ratings and Ratings Agencies
- G28 - Government Policy and Regulation
- Browse content in G3 - Corporate Finance and Governance
- G30 - General
- G31 - Capital Budgeting; Fixed Investment and Inventory Studies; Capacity
- G32 - Financing Policy; Financial Risk and Risk Management; Capital and Ownership Structure; Value of Firms; Goodwill
- G34 - Mergers; Acquisitions; Restructuring; Corporate Governance
- G38 - Government Policy and Regulation
- Browse content in H - Public Economics
- H0 - General
- Browse content in H1 - Structure and Scope of Government
- H11 - Structure, Scope, and Performance of Government
- H13 - Economics of Eminent Domain; Expropriation; Nationalization
- Browse content in H2 - Taxation, Subsidies, and Revenue
- H20 - General
- H21 - Efficiency; Optimal Taxation
- H23 - Externalities; Redistributive Effects; Environmental Taxes and Subsidies
- H25 - Business Taxes and Subsidies
- H26 - Tax Evasion and Avoidance
- H27 - Other Sources of Revenue
- Browse content in H3 - Fiscal Policies and Behavior of Economic Agents
- H31 - Household
- Browse content in H4 - Publicly Provided Goods
- H41 - Public Goods
- H43 - Project Evaluation; Social Discount Rate
- H44 - Publicly Provided Goods: Mixed Markets
- Browse content in H5 - National Government Expenditures and Related Policies
- H52 - Government Expenditures and Education
- H53 - Government Expenditures and Welfare Programs
- H54 - Infrastructures; Other Public Investment and Capital Stock
- H55 - Social Security and Public Pensions
- H56 - National Security and War
- H57 - Procurement
- Browse content in H7 - State and Local Government; Intergovernmental Relations
- H70 - General
- H71 - State and Local Taxation, Subsidies, and Revenue
- H72 - State and Local Budget and Expenditures
- H73 - Interjurisdictional Differentials and Their Effects
- H74 - State and Local Borrowing
- H75 - State and Local Government: Health; Education; Welfare; Public Pensions
- H76 - State and Local Government: Other Expenditure Categories
- H77 - Intergovernmental Relations; Federalism; Secession
- Browse content in H8 - Miscellaneous Issues
- H81 - Governmental Loans; Loan Guarantees; Credits; Grants; Bailouts
- H89 - Other
- Browse content in I - Health, Education, and Welfare
- I0 - General
- Browse content in I1 - Health
- I14 - Health and Inequality
- I18 - Government Policy; Regulation; Public Health
- Browse content in I2 - Education and Research Institutions
- I20 - General
- I21 - Analysis of Education
- I22 - Educational Finance; Financial Aid
- I23 - Higher Education; Research Institutions
- I24 - Education and Inequality
- I25 - Education and Economic Development
- I28 - Government Policy
- I29 - Other
- Browse content in I3 - Welfare, Well-Being, and Poverty
- I30 - General
- I31 - General Welfare
- I32 - Measurement and Analysis of Poverty
- I38 - Government Policy; Provision and Effects of Welfare Programs
- Browse content in J - Labor and Demographic Economics
- Browse content in J0 - General
- J01 - Labor Economics: General
- Browse content in J1 - Demographic Economics
- J10 - General
- J11 - Demographic Trends, Macroeconomic Effects, and Forecasts
- J12 - Marriage; Marital Dissolution; Family Structure; Domestic Abuse
- J13 - Fertility; Family Planning; Child Care; Children; Youth
- J15 - Economics of Minorities, Races, Indigenous Peoples, and Immigrants; Non-labor Discrimination
- J16 - Economics of Gender; Non-labor Discrimination
- J18 - Public Policy
- J19 - Other
- Browse content in J2 - Demand and Supply of Labor
- J21 - Labor Force and Employment, Size, and Structure
- J22 - Time Allocation and Labor Supply
- J23 - Labor Demand
- J24 - Human Capital; Skills; Occupational Choice; Labor Productivity
- Browse content in J3 - Wages, Compensation, and Labor Costs
- J30 - General
- J31 - Wage Level and Structure; Wage Differentials
- J32 - Nonwage Labor Costs and Benefits; Retirement Plans; Private Pensions
- J38 - Public Policy
- Browse content in J4 - Particular Labor Markets
- J40 - General
- J41 - Labor Contracts
- J42 - Monopsony; Segmented Labor Markets
- J44 - Professional Labor Markets; Occupational Licensing
- J45 - Public Sector Labor Markets
- Browse content in J5 - Labor-Management Relations, Trade Unions, and Collective Bargaining
- J50 - General
- J51 - Trade Unions: Objectives, Structure, and Effects
- J53 - Labor-Management Relations; Industrial Jurisprudence
- Browse content in J6 - Mobility, Unemployment, Vacancies, and Immigrant Workers
- J60 - General
- J61 - Geographic Labor Mobility; Immigrant Workers
- J62 - Job, Occupational, and Intergenerational Mobility
- J63 - Turnover; Vacancies; Layoffs
- J64 - Unemployment: Models, Duration, Incidence, and Job Search
- J65 - Unemployment Insurance; Severance Pay; Plant Closings
- Browse content in J7 - Labor Discrimination
- J71 - Discrimination
- Browse content in J8 - Labor Standards: National and International
- J80 - General
- J81 - Working Conditions
- J83 - Workers' Rights
- Browse content in K - Law and Economics
- Browse content in K0 - General
- K00 - General
- Browse content in K2 - Regulation and Business Law
- K23 - Regulated Industries and Administrative Law
- Browse content in K3 - Other Substantive Areas of Law
- K35 - Personal Bankruptcy Law
- K37 - Immigration Law
- Browse content in K4 - Legal Procedure, the Legal System, and Illegal Behavior
- K41 - Litigation Process
- K42 - Illegal Behavior and the Enforcement of Law
- K49 - Other
- Browse content in L - Industrial Organization
- Browse content in L0 - General
- L00 - General
- Browse content in L1 - Market Structure, Firm Strategy, and Market Performance
- L10 - General
- L11 - Production, Pricing, and Market Structure; Size Distribution of Firms
- L13 - Oligopoly and Other Imperfect Markets
- L14 - Transactional Relationships; Contracts and Reputation; Networks
- L16 - Industrial Organization and Macroeconomics: Industrial Structure and Structural Change; Industrial Price Indices
- Browse content in L2 - Firm Objectives, Organization, and Behavior
- L20 - General
- L21 - Business Objectives of the Firm
- L22 - Firm Organization and Market Structure
- L23 - Organization of Production
- L25 - Firm Performance: Size, Diversification, and Scope
- L26 - Entrepreneurship
- Browse content in L3 - Nonprofit Organizations and Public Enterprise
- L30 - General
- L31 - Nonprofit Institutions; NGOs; Social Entrepreneurship
- L38 - Public Policy
- Browse content in L5 - Regulation and Industrial Policy
- L51 - Economics of Regulation
- L52 - Industrial Policy; Sectoral Planning Methods
- L53 - Enterprise Policy
- Browse content in L6 - Industry Studies: Manufacturing
- L60 - General
- L62 - Automobiles; Other Transportation Equipment; Related Parts and Equipment
- L63 - Microelectronics; Computers; Communications Equipment
- L64 - Other Machinery; Business Equipment; Armaments
- L65 - Chemicals; Rubber; Drugs; Biotechnology
- L66 - Food; Beverages; Cosmetics; Tobacco; Wine and Spirits
- L67 - Other Consumer Nondurables: Clothing, Textiles, Shoes, and Leather Goods; Household Goods; Sports Equipment
- Browse content in L7 - Industry Studies: Primary Products and Construction
- L71 - Mining, Extraction, and Refining: Hydrocarbon Fuels
- L74 - Construction
- Browse content in L8 - Industry Studies: Services
- L80 - General
- L81 - Retail and Wholesale Trade; e-Commerce
- L82 - Entertainment; Media
- L83 - Sports; Gambling; Recreation; Tourism
- L84 - Personal, Professional, and Business Services
- L85 - Real Estate Services
- L86 - Information and Internet Services; Computer Software
- Browse content in L9 - Industry Studies: Transportation and Utilities
- L90 - General
- L91 - Transportation: General
- L92 - Railroads and Other Surface Transportation
- L93 - Air Transportation
- Browse content in M - Business Administration and Business Economics; Marketing; Accounting; Personnel Economics
- Browse content in M1 - Business Administration
- M10 - General
- M13 - New Firms; Startups
- M16 - International Business Administration
- Browse content in M2 - Business Economics
- M21 - Business Economics
- Browse content in M3 - Marketing and Advertising
- M30 - General
- M31 - Marketing
- M39 - Other
- Browse content in M5 - Personnel Economics
- M51 - Firm Employment Decisions; Promotions
- M52 - Compensation and Compensation Methods and Their Effects
- Browse content in N - Economic History
- Browse content in N1 - Macroeconomics and Monetary Economics; Industrial Structure; Growth; Fluctuations
- N10 - General, International, or Comparative
- N13 - Europe: Pre-1913
- N16 - Latin America; Caribbean
- Browse content in N2 - Financial Markets and Institutions
- N20 - General, International, or Comparative
- N25 - Asia including Middle East
- Browse content in N3 - Labor and Consumers, Demography, Education, Health, Welfare, Income, Wealth, Religion, and Philanthropy
- N30 - General, International, or Comparative
- N33 - Europe: Pre-1913
- N34 - Europe: 1913-
- N37 - Africa; Oceania
- Browse content in N4 - Government, War, Law, International Relations, and Regulation
- N44 - Europe: 1913-
- Browse content in N5 - Agriculture, Natural Resources, Environment, and Extractive Industries
- N50 - General, International, or Comparative
- N51 - U.S.; Canada: Pre-1913
- N57 - Africa; Oceania
- Browse content in N6 - Manufacturing and Construction
- N61 - U.S.; Canada: Pre-1913
- N64 - Europe: 1913-
- Browse content in N7 - Transport, Trade, Energy, Technology, and Other Services
- N70 - General, International, or Comparative
- N71 - U.S.; Canada: Pre-1913
- N72 - U.S.; Canada: 1913-
- N73 - Europe: Pre-1913
- N74 - Europe: 1913-
- N77 - Africa; Oceania
- Browse content in N9 - Regional and Urban History
- N90 - General, International, or Comparative
- N92 - U.S.; Canada: 1913-
- N93 - Europe: Pre-1913
- N94 - Europe: 1913-
- N95 - Asia including Middle East
- N97 - Africa; Oceania
- Browse content in O - Economic Development, Innovation, Technological Change, and Growth
- Browse content in O1 - Economic Development
- O10 - General
- O11 - Macroeconomic Analyses of Economic Development
- O12 - Microeconomic Analyses of Economic Development
- O13 - Agriculture; Natural Resources; Energy; Environment; Other Primary Products
- O14 - Industrialization; Manufacturing and Service Industries; Choice of Technology
- O15 - Human Resources; Human Development; Income Distribution; Migration
- O16 - Financial Markets; Saving and Capital Investment; Corporate Finance and Governance
- O17 - Formal and Informal Sectors; Shadow Economy; Institutional Arrangements
- O18 - Urban, Rural, Regional, and Transportation Analysis; Housing; Infrastructure
- O19 - International Linkages to Development; Role of International Organizations
- Browse content in O2 - Development Planning and Policy
- O20 - General
- O21 - Planning Models; Planning Policy
- O24 - Trade Policy; Factor Movement Policy; Foreign Exchange Policy
- O25 - Industrial Policy
- Browse content in O3 - Innovation; Research and Development; Technological Change; Intellectual Property Rights
- O30 - General
- O31 - Innovation and Invention: Processes and Incentives
- O32 - Management of Technological Innovation and R&D
- O33 - Technological Change: Choices and Consequences; Diffusion Processes
- O34 - Intellectual Property and Intellectual Capital
- O38 - Government Policy
- Browse content in O4 - Economic Growth and Aggregate Productivity
- O40 - General
- O41 - One, Two, and Multisector Growth Models
- O43 - Institutions and Growth
- O47 - Empirical Studies of Economic Growth; Aggregate Productivity; Cross-Country Output Convergence
- Browse content in O5 - Economywide Country Studies
- O51 - U.S.; Canada
- O52 - Europe
- O53 - Asia including Middle East
- O54 - Latin America; Caribbean
- O55 - Africa
- O57 - Comparative Studies of Countries
- Browse content in P - Economic Systems
- Browse content in P1 - Capitalist Systems
- P12 - Capitalist Enterprises
- P13 - Cooperative Enterprises
- P16 - Political Economy
- P18 - Energy: Environment
- Browse content in P2 - Socialist Systems and Transitional Economies
- P20 - General
- P25 - Urban, Rural, and Regional Economics
- P26 - Political Economy; Property Rights
- Browse content in P3 - Socialist Institutions and Their Transitions
- P30 - General
- P31 - Socialist Enterprises and Their Transitions
- Browse content in P4 - Other Economic Systems
- P48 - Political Economy; Legal Institutions; Property Rights; Natural Resources; Energy; Environment; Regional Studies
- Browse content in P5 - Comparative Economic Systems
- P51 - Comparative Analysis of Economic Systems
- Browse content in Q - Agricultural and Natural Resource Economics; Environmental and Ecological Economics
- Browse content in Q0 - General
- Q00 - General
- Q01 - Sustainable Development
- Q02 - Commodity Markets
- Browse content in Q1 - Agriculture
- Q10 - General
- Q12 - Micro Analysis of Farm Firms, Farm Households, and Farm Input Markets
- Q15 - Land Ownership and Tenure; Land Reform; Land Use; Irrigation; Agriculture and Environment
- Q16 - R&D; Agricultural Technology; Biofuels; Agricultural Extension Services
- Q17 - Agriculture in International Trade
- Browse content in Q2 - Renewable Resources and Conservation
- Q20 - General
- Q28 - Government Policy
- Browse content in Q3 - Nonrenewable Resources and Conservation
- Q32 - Exhaustible Resources and Economic Development
- Q34 - Natural Resources and Domestic and International Conflicts
- Q38 - Government Policy
- Browse content in Q4 - Energy
- Q40 - General
- Q42 - Alternative Energy Sources
- Q48 - Government Policy
- Q49 - Other
- Browse content in Q5 - Environmental Economics
- Q50 - General
- Q51 - Valuation of Environmental Effects
- Q52 - Pollution Control Adoption Costs; Distributional Effects; Employment Effects
- Q53 - Air Pollution; Water Pollution; Noise; Hazardous Waste; Solid Waste; Recycling
- Q54 - Climate; Natural Disasters; Global Warming
- Q55 - Technological Innovation
- Q56 - Environment and Development; Environment and Trade; Sustainability; Environmental Accounts and Accounting; Environmental Equity; Population Growth
- Browse content in R - Urban, Rural, Regional, Real Estate, and Transportation Economics
- Browse content in R0 - General
- R00 - General
- Browse content in R1 - General Regional Economics
- R10 - General
- R11 - Regional Economic Activity: Growth, Development, Environmental Issues, and Changes
- R12 - Size and Spatial Distributions of Regional Economic Activity
- R13 - General Equilibrium and Welfare Economic Analysis of Regional Economies
- R14 - Land Use Patterns
- R15 - Econometric and Input-Output Models; Other Models
- R19 - Other
- Browse content in R2 - Household Analysis
- R20 - General
- R21 - Housing Demand
- R23 - Regional Migration; Regional Labor Markets; Population; Neighborhood Characteristics
- R28 - Government Policy
- R29 - Other
- Browse content in R3 - Real Estate Markets, Spatial Production Analysis, and Firm Location
- R30 - General
- R31 - Housing Supply and Markets
- R32 - Other Spatial Production and Pricing Analysis
- R33 - Nonagricultural and Nonresidential Real Estate Markets
- R34 - Input Demand Analysis
- R38 - Government Policy
- R39 - Other
- Browse content in R4 - Transportation Economics
- R40 - General
- R41 - Transportation: Demand, Supply, and Congestion; Travel Time; Safety and Accidents; Transportation Noise
- R42 - Government and Private Investment Analysis; Road Maintenance; Transportation Planning
- R48 - Government Pricing and Policy
- R49 - Other
- Browse content in R5 - Regional Government Analysis
- R50 - General
- R51 - Finance in Urban and Rural Economies
- R52 - Land Use and Other Regulations
- R53 - Public Facility Location Analysis; Public Investment and Capital Stock
- R58 - Regional Development Planning and Policy
- R59 - Other
- Browse content in Y - Miscellaneous Categories
- Browse content in Y8 - Related Disciplines
- Y80 - Related Disciplines
- Browse content in Z - Other Special Topics
- Browse content in Z1 - Cultural Economics; Economic Sociology; Economic Anthropology
- Z10 - General
- Z11 - Economics of the Arts and Literature
- Z12 - Religion
- Z13 - Economic Sociology; Economic Anthropology; Social and Economic Stratification
- Z19 - Other
- Advance articles
- Author Guidelines
- Submission Site
- Open Access
- Why Publish
- About Journal of Economic Geography
- Editorial Board
- Advertising and Corporate Services
- Journals Career Network
- Self-Archiving Policy
- Dispatch Dates
- Terms and Conditions
- Journals on Oxford Academic
- Books on Oxford Academic

Article Contents
1. introduction, 2. related literature, 3. context and data, 4. empirical approach, 6. discussion and conclusion, acknowledgments.
- < Previous
The urban–rural education gap: do cities indeed make us smarter? *
- Article contents
- Figures & tables
- Supplementary Data
Raoul van Maarseveen, The urban–rural education gap: do cities indeed make us smarter?, Journal of Economic Geography , Volume 21, Issue 5, September 2021, Pages 683–714, https://doi.org/10.1093/jeg/lbaa033
- Permissions Icon Permissions
Despite the large urban–rural education gap observed in most countries, little attention has been paid to whether cities actually enjoy a comparative advantage in the production of human capital. Using Dutch administrative data, this paper finds that children growing in urban regions consistently attain higher levels of human capital compared with children in rural regions, conditional on observed cognitive ability and various family characteristics. The elasticity of university attendance with respect to population density is 0.07, which is robust across a variety of specifications. Hence, the paper offers a different explanation to explain the recent success of cities.
The resurgence of the city has received widespread attention from both academics and policymakers in recent decades. Explanations typically highlight the success of cities at attracting well educated and skilled individuals from elsewhere, both by offering higher wages (see Combes et al., 2008 ) and superior consumption amenities (see Glaeser et al., 2001 ). Furthermore, cities are thought to have a comparative advantage in employing human capital in the production of goods and services, due to the sharing, matching and learning mechanisms described in Duranton and Puga (2004) and Rosenthal and Strange (2004) .
A small literature has analyzed the role of cities in the production of human capital itself. Both Glaeser and Mare (2001) and De La Roca and Puga (2017) find evidence that workers in larger cities accumulate human capital at a faster rate. However, little attention has been paid to the role of cities in human capital accumulation in the period prior to labor market entry. This is surprising, since agglomeration economies are likely to influence educational investment decisions through various channels. In this paper, I investigate whether exposure to urban environments during childhood affects the educational attainment of children and young adults.
Cross-sectional evidence reveals a sizeable rural–urban gap in educational outcomes across a wide range of countries. 1 It is unclear whether these differences are fully explained by the spatial sorting of households or whether they partially reflect an advantage of cities in educating their population. To answer this question, I make use of the particular institutional setting in one country, the Netherlands. The Dutch educational system requires students to make conscious decisions about their desired level of human capital investment both at the end of primary school and at the end of secondary school. Just before making the decisions, students participate in national tests of academic ability. These test scores are highly predictive of future academic outcomes and thus provide an excellent measure of the students’ cognitive and academic ability precisely at the moment when they make educational investment decisions. Furthermore, I observe a wide range of household characteristics, including detailed measures of parental education and household income.
The analyses reveal substantial differences in the human capital choices between children growing up in urban areas and rural areas. Conditional on family background and observed cognitive ability, a one log-point increase in population density is associated with a 1.68 percentage point increase in the likelihood that a child enrolls in an academic secondary school, from a base of 23%. Similarly, among high school students who have obtained all prerequisites to enroll for a university degree, a one log-point increase in population density is associated with a 0.8 percentage point increase in the likelihood that a child attends university, from a base of 84%. Children who grow up in more urban environments are thus significantly more likely to select into the schooling tracks that provide higher levels of human capital accumulation. Taken together, a one log-point increase in population density is associated with a 1.4 percentage point increase in the probability that a child attends university, which implies an elasticity of university attendance with respect to population density of 0.07. 2 Expressed differently, growing up in the center of Amsterdam rather than a place at the 25th percentile of the density distribution would increase the probability of attending university by 3 percentage points. Similar to Combes et al. (2008) in the case of the urban wage premium, I find an elasticity about twice as large when individual and family characteristics are not controlled for.
To assess whether differences in unobserved characteristics between urban and rural students might be responsible for differences in human capital investment decisions, I employ the methodology of Altonji et al. (2005) and Oster (2019) , which uses the selection on observed variables as guide to the selection on unobserved variables. Under the assumption of Altonji et al. (2005) and Oster (2019) that selection on unobserved characteristics is weakly less than selection on observed characteristics, omitted variable bias cannot account for the results obtained in this paper. In addition, the coefficients are similar across subgroups, not driven by functional form assumptions and robust to the inclusion of regional fixed effects. Using the historical densities of 1840 as instrument for modern densities to account for the potential endogeneity of urban settlements also leaves the results unaffected.
The findings of this paper contribute to three strands of literature. First, the paper contributes to the urban economics literature by showing that density affects human capital decisions of children. Earlier literature in this field has typically focused on the effect of density at a single decision moment ( Frenette, 2006 ; Newbold and Brown, 2015 ) or on a single outcome variable ( Gibbons and Silva, 2008 ), whereas this paper estimates the effect of population density on educational outcomes throughout the entire schooling career. In addition, the institutional setting and rich administrative data allow me to condition on a wide range of family characteristics and detailed measures of cognitive ability, which has typically remained unobserved in earlier studies. The closest related work is by Gibbons and Silva (2008) , who show that density affects high school test scores in the UK. This paper in comparison takes test scores as given, and focuses on students’ educational choices conditional on the primary and secondary school test scores. The resulting elasticity is around three times larger than the elasticity reported by Gibbons and Silva (2008) , reflecting that a substantial part of the effect of density on educational attainment operates through differences in educational choices. The finding that children in cities accumulate more human capital also well complements the work by Glaeser and Mare (2001) and De La Roca and Puga (2017) , who find that workers in cities accumulate human capital at a faster rate, and the recent work by Eckert et al. (2020) , who use a natural experiment to show that refugees who are placed in cities are more likely to start working in skill-intensive occupations.
Second, the results have implications for the long-term economic growth of regions and the persistence of regional differences. It is well known that the stocks of human capital are typically lower in rural regions. The findings of this study suggest that rural regions are in addition at a disadvantage in the expansion of their human capital stock, since children in rural areas invest less in human capital accumulation throughout their childhood, even when they have the same cognitive ability and family background as children in urban areas. While the lower human capital investment in rural regions might be efficient from the perspective of the individual, it does have significant implications for the long-term inequality between urban and rural regions. This is particularly important due to the fact that human capital is the most important predictor of economic growth in regions ( Gennaioli et al., 2013 ), 3 and hence provides a mechanism that could explain the slow observed convergence in incomes between regions within countries as noted by Gennaioli et al (2014) and others.
Finally, the paper contributes to a rapidly growing literature on the spatial (in)-equality of opportunity, following Chetty et al. (2014a ) and Chetty and Hendren (2018) in the USA, Alesina et al. (2019) in Africa and Deutscher (2020) in Australia. In this paper, I focus on one specific aspect of places, namely population density, and examine the effects on educational attainment. The results indicate that children who grow up in rural communities do not appear to enjoy or take advantage of the same educational opportunities as children in urban communities, even when they appear to have the same cognitive ability and family background as their urban peers. Hence, the findings of this paper highlight that the urban–rural differences in educational outcomes may not just be a result of spatial sorting of households, but may also reflect a lack of opportunities or awareness of opportunities for children residing in rural regions.
Finally, a limitation of this study is that it cannot attribute the overall effect of density on educational attainment to individual mechanisms. Based on the existing literature, there are three main channels through which density is likely to affect educational decisions. First, the returns to education are higher in cities as agglomeration forces mainly complement the productivity of high-skilled workers ( Baum-Snow et al., 2018 ; Autor, 2019 ), which raises the incentive to invest in human capital in urban areas. Second, the costs of obtaining education are lower in cities, both due to the smaller distance to educational institutes ( Frenette, 2006 ), as well as the possibility that the more diverse school choice in cities improves the match between students their needs and interests and the educational institutes. Third, it might be costlier for youth to acquire information about future educational possibilities in rural areas, due to the absence of university outreach programs as well as the absence of strong network linkages to higher educational institutes in rural communities ( Hoxby and Avery, 2012 ). However, the high correlations between the measures of the individual mechanisms make it difficult to attribute the overall effect of density to the individual mechanisms within the scope of this paper, and a detailed investigation along the lines of De La Roca and Puga (2017) and Dauth et al. (2018) in the case of the urban wage premium is thus left for future research.
The remainder of the paper proceeds as follows. Section 2 reviews the existing theoretical and empirical literature linking density to educational outcomes. Section 3 provides an overview of the context and data. Section 4 discusses the methodology and identification strategy. Section 5 presents the results and the various robustness analyses. Section 6 discusses the implications and concludes.
There are good reasons why children with similar capabilities might make different educational choices depending on whether they live in urban or rural communities. This section provides an overview of the theoretical and empirical support for three potential mechanisms, as well as review the empirical literature which links educational outcomes to urbanization.
2.1. Higher returns to education
A key determinant of educational choice in any model of educational investment is the expected returns to schooling. Most models of educational investment, such as the seminal Ben-Porath model, assume that students invest in education until the point where the discounted increase in future wages is equal to the opportunity costs and direct costs of obtaining education, thus providing a tight link between perceived returns to education and human capital investments. Theoretically one would expect highly skilled and educated workers to benefit most from agglomeration economies (see Berry and Glaeser, 2005 ; Behrens et al., 2014 ; Davis and Dingel, 2019 ), which matches the empirical findings that the returns to education are higher in urban environments ( Gould, 2007 ; Combes and Gobillon, 2015 ; Baum-Snow et al., 2018 ). This difference has become more pronounced over the last twenty years, during which the urban wage premium for non-college educated workers has essentially disappeared in the US ( Autor, 2019 ). Hence, in the absence of perfect mobility, one would expect the higher returns to education in cities to lead to higher educational investment by children growing up in urban places. However, a key question is whether children and young adults correctly infer the returns to education from their environment and if they respond accordingly. The way in which people form beliefs with regards to the returns to education is still largely unknown, but the experiment by Jensen (2010) suggests that these beliefs are not necessarily deeply held, and that beliefs are updated when new information is presented. Recent work by Adukia et al. (2020) provides evidence for this in the context of India. Rural villages which were connected to cities by roads experienced significant increases in educational attainment, with the effect increasing with the returns to education in newly connected cities.
2.2. Costs of schooling
A second channel through which population density can affect educational outcomes is through a reduction in the perceived costs of attending education. At the level of primary and secondary school, the higher density of schools in urban areas means that children on average will spend less time and financial resources on commuting to a school of a given level and quality. In the case of tertiary education, commuting to college or university may become simply unfeasible for children in rural areas, hence necessitating a costly move to attend further education. The empirical literature finds some supporting evidence for the importance of commuting costs on educational decisions, particularly in the case of tertiary education. Card (1993) uses distance to college as an instrument for educational attainment and finds a significant effect in the first stage. Frenette (2006) finds in Canada that children whose families live more than 80 km from the nearest university have a 40% lower probability to enroll at university compared with individuals who grow up within 40 km of a university. However, both studies include only a very limited set of control variables, and as such, it is not clear to what degree the results are driven by the spatial selection of households.
Furthermore, the higher density of educational institutions in cities may also allow for better matching between schools and students, as long as students and schools are heterogeneous in some dimensions. Burgess et al. (2019) show that the majority of students do not list the nearest secondary school as their first preference in the UK, indicating that students do not perceive schools as a homogenous good. Such school-child match specific component can be based on academic preferences of the child, such as the level of instruction (see for instance Bau, 2019 ) and the focus on certain specialization tracks, or can be based on more personal preferences of the child, such as the religious orientation of a school ( Cohen-Zada and Sander, 2008 ). Models of school choice typically assume that an outside option is available with zero utility, which depending on the context might consist of staying home, working or choosing a different level of schooling. The smaller the number of schools available, the more likely that unfavorable draws of the school-student match quality will result in the student choosing the outside option, which may reduce human capital investment in rural areas. Gibbons and Silva (2008) argue that greater school choice and competition in cites may explain the better performance of urban schools in the UK.
2.3. Information and network effects
Third, density may affect human capital decisions through the availability of information on future schooling prospects or due to network effects. Hoxby and Avery (2012) find in the USA that high-performing students who apply to non-selective colleges are disproportionally located in rural areas, as the lower density of high-performing students makes it less profitable for colleges to engage in outreach campaigns. In addition, Hoxby and Avery (2012) suggest that the lower Social-Economic Status (SES) composition of households in rural areas limits the exposure of children to alumni of various educational institutes, as well as reduce the expertise of study counselors on how to advise high-performing students. Hence, the limited information about future educational possibilities in rural areas provides a third potential channel through which density may affect educational investment.
2.4. Empirical evidence
Despite theoretical reasons to expect children and young adults in urban areas to invest more in their human capital, the empirical evidence on this subject is limited. Knight and Shi (1996) show large differences in years of educational attainment in China between urban and rural regions, although it remains unclear to what degree this is driven by household heterogeneity. Katz and Goldin (2008 , 222) instead report a negative relationship between town size and high school graduation rates in the early 20th century in the USA, which they suggest might be the result of the higher opportunity costs of education in cities. More recently, Newbold and Brown (2015) show that the likelihood that Canadian youth attend university is increasing in city size.
A key challenge in this literature has been dealing with the spatial sorting by households and unobserved heterogeneity between children. Previous work has established that households sort into cities based on parental education ( De la Roca, 2017 ; Ahlin et al., 2018 ; Bosquet and Overman, 2019 ) and cognitive skills ( Bacolod et al., 2010 ; Bütikofer and Peri, 2020 ). Separating spatial selection from area effects is particularly difficult in the case of education, since panel data on educational outcomes is typically unavailable on the individual level. As such, the methods developed to estimate the urban wage premium, which rely heavily on the inclusion of individual fixed effects, cannot easily be applied in the case of educational outcomes.
As a way to overcome the spatial sorting of households, some studies have relied on quasi-experimental variation to quantify the effect of exposure to neighborhood on educational outcomes. Chetty et al. (2016) use the Moving-to-Opportunity program to study neighborhood effects, which provided a randomly selected group of families the opportunity to move to a better neighborhood with less poverty and higher incomes. They find that children who moved to better neighborhoods before the age of 13 years had significantly higher schooling outcomes compared with those who did not receive the opportunity to move. However, such experiments are typically difficult to scale beyond the individual city. In a more general approach, Chetty and Hendren (2018) use the difference in the age of children when they move between regions to identify neighborhood effects. They find a negative relationship between population density and upward mobility as measured by the income at age 26 years, although it remains unclear to what extent differences in human capital accumulation play a role.
In this paper, I utilize the Dutch context to investigate the relationship between population density and educational outcomes, which is particularly well suited for this question. Tracking in the Dutch educational system starts at the age of 11–12 years, which means that meaningful decisions on human capital investment are taken from an early age onwards. Furthermore, students participate in high-stakes tests of academic ability shortly before making their key educational decisions. Hence, I observe detailed measures of cognitive ability precisely when students make educational decisions, which remains typically unobserved in most other settings. One concern with the Netherlands is that it is relatively urbanized by international standards. However, earlier studies in the Netherlands on the urban wage premium found results in line with other countries ( Groot et al., 2014 ; Verstraten et al., 2019 ). 4
3.1. Context
Figure 1 provides an overview of the Dutch school system, including the flows between school types. 5 Compulsory education starts at the age 6 years, when all students enroll in primary school. The primary school education lasts for 6 years, at the end of which students participate in a national test measuring their ability in reading comprehension, mathematics and vocabulary, as well as their reasoning and studying ability. 6 Tracking begins at the end of primary school when students can enroll in three different levels of secondary school: upper, middle and lower secondary school. The three levels differ both in length of study, the difficulty of the material and the access to tertiary education which a degree grants. Students are free to apply to any of the three levels of secondary school after finishing primary school, but secondary schools can choose whether to accept students. During the admission decision, secondary schools primarily rely on the scores on the standardized test at the end of the primary school, as well as the recommendation of the primary school teacher. In the years 2003–2015, the score on the national standardized test was considered the leading admission criteria for secondary schools. 7

Tracking through the Dutch education system for the 1996 cohort. The figure is based on the cohort born in 1996. The secondary school enrollment percentages reflects enrollment 4 years after completing primary school. The tertiary education percentages reflect enrollment within 3 years after finishing the highest secondary school degree. There are some streams within the secondary schooling system (for instance, students dropping from upper secondary school to middle secondary school), which are not displayed here. In addition, some students continue with applied university after obtaining a vocational education degree or with university after obtaining an applied university degree. These streams are not displayed here either, as this usually happens outside of the 3-year window.
Figure 2 shows the distribution of the end of primary school test score for the cohort born in 1996 (panel a) as well as the probability that students with a given test-score enroll in an upper secondary school (panel b). The test score is highly predictive of whether a student enrolls in upper secondary school. Students who score less than 535 (which is about the median test score) are highly unlikely to be enrolled in an upper secondary school 4 years later, whereas among the group of students who obtain the maximum score of 550, 8 95% enrolls at an upper secondary school. Panel c in Figure 2 shows how these probabilities differ between urban and rural regions, providing a first indication of the differences in educational decisions between urban and rural regions.

Frequency and probability of enrolling in upper secondary school by end-of-primary school test score. ( a ) Displays the frequency for each of the 50 potential scores (501–550) that students could receive at the end-of-primary school test for the cohort born in 1996. ( b ) Displays the percentage of students that are enrolled at an upper secondary school 4 years after taking the test by the end-of-primary school test score. ( c ) Splits the sample between those who grew up in a place with less than the median density (rural) and those who grew up in a place with above-median density (urban). The green line shows the difference in upper secondary school enrollment between urban and rural places in percentage points.
Once students enroll in a secondary school, they continue for another 4–6 years depending on the selected type of secondary school. At the end of secondary school, all students take a national examination which determines if they are granted a degree. This end of secondary school test is specific for their level of secondary school. 9 Once students obtain a secondary school degree, they continue to tertiary education. 10 The tracking system is designed such that certain levels of secondary school feed into a specific level of tertiary education. As can be seen from Figure 1 , 84% of the students who obtain a degree from an upper secondary school are registered at a university 3 years after graduation. Similarly, 88% of the students who obtain a degree from a middle secondary school and 98% of the students completing the lower secondary school are, respectively, registered at an applied university 11 and a vocational education 3 years after graduation. 12 Hence, the choice that students make at the end of primary school matters greatly for their future educational prospects.
Tertiary education in the Netherlands differs from many other European countries in the sense that few university degrees have a binding constraint on the number of students they accept. 13 Students who have obtained the relevant qualifications can typically register for their study of choice at the university of their choice, without additional entry conditions. Tuition fees are around 1800 euros per year for universities and applied universities and around 1000 for vocational education, with governmental student loans available if needed. Primary schools and secondary schools are free of tuition. Educational institutes at all levels are financed directly by the national government, with the financing based on the number and type of students enrolled.
The data used in the analysis are primarily for the cohorts born between 1994 and 1998. 14 I restrict the sample to individuals born in the Netherlands for whom both parents can be identified. For these individuals, I observe the place of residence between the ages 1 and 20 years, the enrollment status in all types of secondary and tertiary education and the results on the national tests at the end of primary school 15 and secondary school. In addition, a large number of family characteristics are available, including parental income, education, country of birth and year of birth. The summary statistics for all variables are provided in Table 1 .
Summary statistics for individuals born in the Netherlands between the years 1994 and 1998
Note : The table shows the summary statistics for the individuals born between 1994 and 1998 in the Netherlands. Due to the privacy-sensitive nature of the microdata, it is not possible to report minima or maxima, hence the 1st and 99th percentiles values are displayed instead. As explained in the main text, education is not available for all parents. Secondary school enrollment is measured 4 years after completing primary school. The GPA and specialization track of the upper secondary school degree are only observed for students who graduated from upper secondary school. Tertiary education enrollment is based on cohorts born in 1994–1996, as for the cohorts born in 1997/1998 not enough time has passed for all students to measure. Tertiary education enrollment is defined as the highest enrollment within 3 years of high-school graduation. The graduation rate of middle secondary school is higher than enrollment due to the fact that about 10% of the children enrolled in an upper secondary school drop down to a middle secondary school during their final three years in secondary school.
Parental income is defined similarly as in Chetty and Hendren (2018) and consists of the sum of income for both parents when the child is between ages 14 and 18 years, divided by the number of parent-years with non-missing income. Some parents have a negative income, top-coded income or have missing income for more than 5 parent-years. For the parents with negative income and parents with fewer than 5 parent-year income observations, income is set to zero and a dummy is included for both groups in each regression. A dummy is also included for top-coded incomes. The robustness checks reveal that the results are not sensitive to exclusion of these (small) groups, which together contain about 1.5% of the observations. As the coverage of the income tax data may have improved slightly over the years, I allow the coefficient of parental income as well as the coefficients of the three dummy variables to vary across cohorts.
For each parent, I observe 13 possible levels of education. 16 I do not observe education for all parents, particularly for older parents who completed schooling before some of the national education registers started. Discussions with Statistics Netherlands reveal that parents with missing education are more likely to be low educated, since highly educated parents are more likely to be captured by the various educational registers. In the baseline result, missing education is included as a 14th education type. However, the results are robust to the exclusion of this group. To opt for an as flexible approach as possible when controlling for parental education, I include dummies for each of the 196 possible parental education combinations. Similarly, parental country of birth is grouped into ‘The Netherlands’, ‘Europe’ and ‘Non-European’ for each parent, and nine dummies are included to account for each possible parental combination. Finally, the age of the oldest parent at the time of the birth is added as an additional control variable.
The next step is to construct an index of urbanization. For each individual, the zip code in which he or she resided between the years 1995 and 2018 is observed. The size of the zip codes is relatively small at 8 km 2 . I define urbanization as the log of the number of people living within 10 km of the centroid of the zip code in which an individual resides (see Appendix B for the details of the procedure). 17 The threshold of 10 km is selected based on the fact that the majority of Dutch children travel to school by bicycle, where 10 km seems a reasonable upper bound for their reach. Furthermore, the choice of a 10-km radius is in line with recent studies of agglomeration economies on wages ( De La Roca and Puga, 2017 ; Verstraten et al., 2019 ). Nonetheless, the number of individuals that live within, respectively, 5, 10 and 20 km is highly correlated, and as such, the exact distance cut-off has little influence on the results. 18 Densities are the highest in the urbanized Western part of the Netherlands and are relatively low in the North and East, as shown in Figure 3 .

Urbanization measure per zip code. The map displays the log of number of individuals within 10 km, based on the 1995 population distribution. For graphical purposes, areas with an urbanization grade less than 9 are gray (containing 230 out of the 631.890 observations in the baseline sample). Appendix B provides details on the construction of the urbanization measure.
Under the assumption that the covariance between ε i t and the urbanization measure is zero, Equations (1) and (2) will correctly identify the effect of growing up in an urban environment on educational outcomes. One concern is that parents move to places best fitted to realize the potential outcomes of their children, in which case the estimates of α 4 and β 4 would be biased. However, I find no evidence of this in the Dutch setting. For the cohorts born between 1994 and 1998, only 5.4% of the families with children between the ages 6 and 17 years make a substantial move (more than 20 km), thus limiting the degree to which families respond to the realized potential of their children. In addition, within the group of children who move, there is no correlation between the change in density and the observed academic ability of the children. 21 Nonetheless, I show that the results of Equations (1) and (2) are robust to estimating it on either the full sample or on the group of children who do not move across municipalities between the ages 6 and 17 years. 22
A second concern is that parental characteristics may vary between urban and rural places in ways that are not fully captured by the control variables. For instance, it might be that parents in urban areas are more ambitious than parents in rural areas and that this is not fully captured by the differences in wages. Separating spatial sorting from area effects has been a key challenge in the urban literature and solutions to this have typically relied heavily on individual-fixed effects ( Combes et al., 2008 ; De La Roca and Puga, 2017 ). However, such approaches are typically not possible in the case of educational outcomes due to the lack of consistent national panel data on the educational outcomes of children. 23 Section 5.2 explores this potential threat to identification, using the methodology of Altonji et al. (2005) and Oster (2019) to assess the potential importance of sorting on unobserved characteristics.
Finally, conditioning on the test scores in Equation (1) and (2) might risk overcontrolling for the effect of density on educational attainment, as test scores themselves may also be affected by density. Gibbons and Silva (2008) find that students in urban schools in the UK show more rapid improvements in test scores compared with rural students. Hence, to the degree that urban–rural differences in test scores reflect better learning outcomes in urban areas rather than heterogeneity in cognitive ability between students, the reported coefficients may somewhat underestimate the full effect of population density on educational outcomes.
5.1. Baseline results
Table 2 presents the results of Equation (1) , analyzing how the decision of children to enroll in an upper secondary school depends on population density. Column (1) shows the regression when only the urbanization measure is included, whereas column (2) adds child and family characteristics and column (3) adds dummies for the end of primary school test scores. The coefficient of urbanization is statistically significant in all three specifications at the 1% level. In economic terms, the baseline result of column (3) indicates that an increase in density by one log-point (which coincidentally is close to one standard deviation, see Table 1 ) is associated with a 1.68 percentage point increase in the probability that a child attends upper secondary school. Given that the mean percentage of children attending the upper secondary school is about 23%, this increase is substantial. When comparing the individual columns, the coefficient drops somewhat when family characteristics are added, which is largely driven by the differences in parental education between urban and rural regions. The coefficient declines further when the test scores are added, which might reflect a lower initial academic ability of children in rural regions, or alternatively might be the effect of overcontrolling to the degree that growing up in an urban environment may also affect end-of-primary school test scores. 24
Effect of urbanization on probability of enrolling in upper secondary school
Note : All results apart from column (5) are based on individuals born between 1994 and 1998. Dependent variable is whether a child is enrolled in an upper secondary school 4 years after completing primary school. Individual controls include a gender dummy and cohort dummies. Family controls include dummies for parental education combinations (168 dummies), parental nationality combinations (9 dummies), year-of-birth of oldest parent (40 dummies), family income (interacted with cohort-fixed effects), low-income dummy (interacted with cohort-fixed effects), top-coded income dummy (interacted with cohort-fixed effects) and dummy for insufficient income data (interacted with cohort-fixed effects). For a detailed explanation of the construction of the urbanization measure or the family controls, see Section 3. Column 4 excludes all children who moved municipalities during school-going age. Column 5 instead estimates the model on the cohorts born between the years 1999 and 2002. Column 6 removes all parents for whom uncertainty exists about the education level of one or both parents. Column 7 adds municipality-fixed effects for the municipality in which children live at age 11 years (430 dummies), whereas column (8) adds province-fixed effect for the province in which children live at age 11 years (12 dummies). Column (9) instruments for modern densities (based on 1995 population distribution, see Appendix B) with historical densities. All standard errors are clustered on the municipality level. Columns (1–8) are estimated by OLS, column (9) by 2SLS. First stage results of column (9) are reported in Appendix C3 (first stage F -statistic: 2349.94).
Columns 4–6 test the robustness of the results by excluding various subgroups. Column (4) excludes children who moved between municipalities during their school-going age, as their families may have sorted themselves into places based on the potential outcomes of their children. Column (5) changes the sample and instead estimates the effect on the cohorts born between 1999 and 2002, for whom information is also available. Finally, column (6) reduces the sample to the individuals for whom the exact education level of both parents is known. The coefficient is very stable across the various subgroups, suggesting that these groups are not driving the results.
One concern might be that children in urban environments enroll in classes that are too difficult for their level of ability, resulting in higher drop-out rates in urban areas. 25 Around 21% of the students who are enrolled in an upper secondary school four years after finishing primary school eventually do not obtain an upper secondary school degree, which is far from negligible. 26 To control for the possibility that differences in dropout rates between urban and rural areas are driving the results, Table 3 instead analyzes the probability that a student obtains a degree from an upper secondary school. The results are very similar to Table 2 , indicating that a misallocation of students at the end of primary school in urban communities is unlikely to drive the results. Furthermore, Table C1 shows the results when directly analyzing drop-out rates, by estimating the probability that a student obtains a degree from an upper secondary school, conditional on being enrolled in an upper secondary school 4 years after finishing primary school. The results indicate that children in urban areas are actually slightly less likely to drop out from an upper secondary school, again showing that differential dropout rates between urban and rural areas are not driving the results of Table 2 .
Effect of urbanization on probability of obtaining a degree in upper secondary school
Note : All results are based on individuals born between 1994 and 1998. Dependent variable is whether a child graduates from an upper secondary school. Individual controls include a gender-dummy and cohort-dummies. Family controls include dummies for parental education combinations (168 dummies), parental nationality combinations (nine dummies), year-of-birth of oldest parent (40 dummies), family income (interacted with cohort fixed effects), low-income dummy (interacted with cohort-fixed effects), top-coded income dummy (interacted with cohort-fixed effects) and dummy for insufficient income data (interacted with cohort-fixed effects). For a detailed explanation of the construction of the urbanization measure or the family controls, see Section 3. Column (4) removes all parents for whom uncertainty exists about the education level of one or both parents. Column (5) adds municipality-fixed effects for the municipality in which children live at age 11 years (430 dummies), whereas column (6) adds province-fixed effect for the province in which children live at age 11 years (12 dummies). Column (7) instruments for modern densities (based on 1995 population distribution, see Appendix B) with historical densities. All standard errors are clustered on the municipality level. Columns (1–7) are estimated by OLS, column (8) by 2SLS. First stage results of column (8) are reported in Appendix C3 (first stage F -statistic: 2349.94).
The second key educational decision moment is after children obtain an upper secondary school degree, when they have to decide on the level of tertiary education. Table 4 shows the estimates for Equation (2) , analyzing the effect of urbanization on the probability that a student enrolls at a university within 3 years of obtaining an upper secondary school degree, which provides access to the large majority of university–subject combinations. Column (1) only includes the urbanization measure, whereas column (2) adds student and family characteristics and column (3) adds controls for the specialization track and the Grade Point Average (GPA) on the national examination at the end of upper secondary school. 27 Since I condition on the national end of high school examination scores (instead of end of primary school test scores), it is possible to use a larger sample and to also include the cohorts born between 1989 and 1993. 28
Effect of urbanization on probability of enrolling at university/applied university, conditional on having an upper secondary school degree
Note : All results apart from column (5) are based on individuals born between 1989 and 1998. Dependent variable is whether a child attends university within 3 years of graduating from upper secondary school. Individual controls include a gender dummy and cohort dummies. Family controls include dummies for parental education combinations (168 dummies), parental nationality combinations (nine dummies), year-of-birth of oldest parent (40 dummies), family income (interacted with cohort-fixed effects), low-income dummy (interacted with cohort-fixed effects), top-coded income dummy (interacted with cohort-fixed effects) and dummy for insufficient income data (interacted with cohort-fixed effects). For a detailed explanation of the construction of the urbanization measure or the family controls, see Section 3. Column (4) excludes all children who moved municipalities during school-going age. Column (5) limits the sample to the cohorts born between 1994 and 1998, in line with the baseline sample of Table II . Column (6) removes all parents for whom uncertainty exists about the education level of one or both parents. Column (7) adds municipality-fixed effects for the municipality in which children live at age 11 years (430 dummies), whereas column (8) adds province-fixed effect for the province in which children live at age 11 years (12 dummies). Column (9) instruments for modern densities (based on 1995 population distribution, see Appendix B) with historical densities. All standard errors are clustered on the municipality level. Columns (1–8) are estimated by OLS and column (9) by 2SLS. First stage results of column (9) are reported in Appendix C3 (first stage F -statistic: 1028.11).
The results in Table 4 indicate that children who grow up in urban areas are more likely to enroll at a university, conditional on their academic ability and family characteristics. The preferred specification in column (3) indicates that children who grow up in an area where log density is one-point higher (about one standard deviation) are 0.8 percentage points more likely to enroll in university, from a base of 84%. The coefficient remains very similar when excluding children who moved between municipalities during their childhood (column 4), limiting the sample to the cohorts born between 1994 and 1998 (column 5) or limiting the sample to the children for whom no uncertainty exists over parental education (column 6).
The next question that arises is which alternatives these children select when they decide not to attend university. One alternative is to enroll at the lower rated applied universities. As Figure 1 shows, about 16% of the students who obtained an upper secondary school degree decide to enroll at an applied university, despite having the necessary qualifications to start at a university. Table C2 shows the effect of density on the probability that a student enrolls at an applied university, conditional having obtained a degree from an upper secondary school. The coefficient is significant and negative, indicating that students growing up in rural communities are more likely to instead enroll at an applied university. The coefficients are nearly identical to the coefficients in Table 4 , suggesting that diversion of rural students into the lower rated applied universities fully explains the lower enrollment rates at universities found in Table 4 .
5.2. Robustness
The results in Section 5.1 indicate that population density may play a role in the educational decisions of children. Naturally, some concerns arise over the interpretation and the robustness of this result. This section will discuss four potential threats to identification: the log-linear functional form assumption, the influence of general regional differences, the endogeneity of population density and spatial sorting on unobserved characteristics.
5.2.1. Functional form assumption
All estimations have been based on the assumption of a log-linear relationship between population density and educational outcomes, which may or may not accurately represent the true functional form. If the functional form is misspecified, it might bias the coefficients. To test this possibility, Figure 4 plots the residuals of the baseline estimations (columns 3 in Tables 2 and 4 ) without the urbanization measure against the urbanization measure itself. The residuals are averaged over 0.2 log point intervals. As Figure 4 shows, the log-linear functional form assumption seems to fit the data quite well and hence is unlikely to bias the results. In addition, the residuals reveal that the results do not depend on any specific part of the density distribution: the log-linear relationship seems to describe the actual relationship well throughout the observed density distribution.

Residuals of baseline estimates plotted against urbanization measure. Average residuals of columns (3) of Tables 2 (left) and 4 (right) without the urbanization measure plotted against the urbanization measure. Each dot corresponds to the average residual in a 0.2 log-point bin. Bins containing fewer than 500 observations are not depicted.
5.2.2. Influence of broad regional differences
Second, the map of the urbanization measure displayed in Figure 3 shows that urbanization is highest in the West and relatively low in the North and East of the country. As a result, the urbanization measure may at least partially reflect broader economic or cultural regional differences within the country, rather than urbanization per se . To test this possibility, columns (7) and (8) in Tables 2 and 4 add provincial and municipality fixed effects to the model. The coefficient of the urbanization measure in this case is identified on the variation in population density within provinces and municipalities. However, the coefficients change relatively little and remain statistically significant in all cases, even though the standard errors increase substantially as most of the variation in the urbanization measure is discarded. Hence, the results are not driven by broader differences between the various regions. 29
5.2.3. Endogeneity of Urbanization
Third, one might be concerned that population density itself is endogenous. Factors that attract population to certain areas and hence contribute to city formation may also directly affect educational outcomes. Furthermore, reverse causality can play a role if individuals migrate to areas with good schools or favorable schooling policies. Combes et al. (2008) highlight the potential of such contemporary factors to bias estimations in the case of the urban wage premium and use historical densities as an instrumental variable (IV). To alleviate concerns that the results in this paper are driven by the endogeneity of the urbanization measure, I follow Combes et al. (2008) and instrument current population densities with the population density based on the 1840 Dutch census. 30 , 31 The details of this procedure and a map of the population densities of 1840 are contained in Appendix C3. Even though it is not a perfect historical instrument, as 4 of the 13 Dutch universities predate 1840, it should substantially reduce any bias arising from the endogeneity of the urbanization measure. The first stage is significant as shown in Appendix C3, which indicates that the instrument is relevant. The results of the 2SLS estimations are provided in column (9) in Tables 2 and 4 . In all three cases, the coefficients hardly change when using the 2SLS estimator. Hence, the endogeneity of urbanization is not driving the results.
5.2.4. Sorting on unobserved variables
Finally, a key concern for many urban and regional studies is the spatial sorting of households on unobserved characteristics. Despite observing some of the key factors of importance for educational decisions, such as parental education and cognitive ability of the child, some other factors such as ambition and non-cognitive skills remain unobserved. One method to assess the importance of such sorting on unobserved variables is provided by Altonji et al. (2005) and Oster (2019) . Their procedure relies on the assumption that the sorting on observed variables is informative of sorting on unobserved variables, and in particular that the sorting on unobserved characteristics is weakly less than the sorting on observed characteristics. As such, the degree to which the coefficient of interest and the R 2 change when control variables are added can provide an indication of the potential importance of omitted variable bias.
Altonji et al. (2005) and Oster (2019) argue that sorting on unobserved variables in most cases is less severe than sorting on observable characteristics for two reasons. First of all, they argue that in the most extreme case, researchers observe a random set of variables since they typically have no direct influence over the data collection in surveys and administrative data. In such cases, the observed variables are a random subset, and as such, sorting on observed and unobserved variables should be equally important in generating bias. However, Altonji et al. (2005) argue that researchers typically direct their effort toward obtaining and including the control variables which are seen as being most likely to generate omitted variable bias. Hence, the included control variables in all likelihood contribute more to the omitted variable bias compared with the unobserved variables. For this reason, both Altonji et al. (2005) and Oster (2019) argue that a reasonable upper bound for the degree of selection on unobserved variables is the selection on observed variables. Furthermore, Altonji et al. (2005) argue that when there is a lag between observing the explanatory variables and the outcome measures, any idiosyncratic shocks that occur between observing the explanatory variables and the outcome measure cannot bias the effect of the predetermined explanatory variable. Hence, to the extent that (unobserved) idiosyncratic shocks are present, they provide an additional reason why sorting on unobserved variables may be less important than sorting on observed variables in generating omitted variable bias.
Importance of selection on unobserved variables
Note : Adjustment for unobserved variables based on Oster (2019) . The R 2 based on sibling regression comes from a regression of the model in Table 2 , column (3) on the subset of siblings, with the secondary educational choice of the sibling added as control variable. To provide a conservative inference, the standard errors of Table 2 / 4 have been used to calculate significance.
The results in this paper show evidence that population density affects the educational investment decisions of children in the context of the Netherlands. Conditional on observed ability and parental background, children who grow up in urban areas consistently choose to invest more in their education compared with children who grow up in more rural environments. This result is robust across various specifications, subgroups and spatial scope, and cannot be accounted for by the endogeneity of the urbanization measure or sorting on unobserved variables under the assumptions of Oster (2019) . Taken together, the results imply that conditional on family characteristics and academic ability, a one log-point increase in density is associated with a 1.4 percentage point increase in the probability that a child attends university. Given the mean university attendance rate of 20%, this implies an elasticity of university attendance with respect to density of 0.07. 33 Expressed differently, moving from a place at the 25th percentile of the density distribution to Amsterdam increases the probability that a child attends university by 3 percentage points.
It is surprising that the potential for agglomeration economies to affect human capital decisions of children and young adults has not received more attention in the literature, particularly given the implications for long-term regional and national growth. Children in rural communities do not seem to enjoy or take the same educational opportunities as children who grow up in urban communities, even in a country such as the Netherlands where the rural areas are relatively accessible from an international perspective. Hence, the findings suggest that differences in educational attainment between urban and rural communities observed in a wide range of countries may reflect more than just the spatial sorting of households.
Finally, one question that remains is which mechanisms drive the increased human capital accumulation of children in urban environments. Sections 2.1 and 2.2 indicated that based on existing literature, one would expect the agglomeration mechanisms to play a role in the early-life human capital decisions, either through an increase in the returns to education in cities or by increasing the availability of schooling and by reducing the commuting or moving costs to attend further education. Furthermore, there might also be a role for network effects as highlighted in Section 2.3. However, a detailed exposition of specific mechanisms in the spirit of De La Roca and Puga (2017) or Dauth et al. (2018) in the case of the urban wage premium is beyond the scope of this paper and will be an interesting avenue for future research.
The author would like to express his gratitude to the editor and two anonymous referees, as well as Niklas Bengtsson, Cristina Bratu, Matz Dahlberg, Peter Fredriksson, Edward Glaeser, Matthew Lindquist, Björn Öckert, Albert Saiz, Anna Tompsett, Santiago Truffa, Dinand Webbink and Peter Zwaneveld, and the participants to the 2019 Urban Economics Association conference and VU Amsterdam/Uppsala University seminar series for their excellent comments and suggestions. Furthermore, he would like to thank CPB Netherlands Bureau for Economic Policy Analysis for making the data available for this research, as well as their excellent suggestions. All results in this paper are based on calculations by the author using non-public microdata from Statistics Netherlands. The code used to generate the results is available upon request.
See Appendix A for the urban–rural education gap based on a wide variety of countries.
A total of 1.23 pp of the increase is due to the higher probability that a child enrolls in an upper secondary school, taking into account high school dropout rates and that not all students with an academic secondary school degree pursue university studies. A further 0.16 pp is due to the 0.8% increase in university attendance among the children who complete upper secondary school, which contains about 20% of the population. Given that a one-log point increase in density raises university enrollment by 1.4 pp and the mean university enrollment rate of 0.2, an elasticity of 0.07 is obtained.
Although Gennaioli et al. (2013) may overestimate the returns to entrepreneurial education relative to worker education due to selection into entrepreneurial activities, as pointed out by Behrens et al. (2014) .
Verstraten et al. (2019) find an elasticity of wages with respect to employment density of 0.02, whereas Groot et al. (2014) find an elasticity of 0.048 without including individual fixed effects. Both are very similar to the estimates cited by Combes and Gobillon (2015) for models with and without person fixed effects in other countries.
All data on school enrollment en educational attainment is collected by the Ministry of Education and is made available for research via Statistics Netherlands. The school enrollment data for all levels is taken from the “Onderwijsdeelnemerstab” register, the end of primary school test from the “Citotab” registry and the secondary school final examination grades from the “Examvotab” registry.
This test consists of 200 multiple choice questions (100 on reading comprehension and vocabulary, 60 on Mathematics and 40 on studying and reasoning ability). The tests are centrally graded by the Citigroup agency, which also develops the annual test.
At the end of 2015, the system was reformed to make the primary school teacher’s recommendation binding and secondary schools were no longer allowed to use the end-of-primary school test score. Politicians and teachers felt that the test had become the only selection criteria on which secondary schools evaluated children, which they argued put undue pressure on the children to perform at one specific moment in time.
The maximum score of 550 is obtained by 5.7% of the students in the 1996 cohort.
All students have a basic set of subjects (such as Dutch, English and Math A), as well as a set of subjects related to their chosen subject specialization (health, humanities, natural sciences or social sciences) in which they complete the national examination. The material covered in the examination as well as the difficulty varies depending on the type of secondary school.
Upon obtaining a middle or lower secondary school degree, students with excellent grades have the opportunity to take the final two years of the next secondary school level. When discussing degree completion or conditioning on degree, these students are always classified according to the highest degree they obtained.
The applied university is similar to the Fachhochschule in the German system. Applied university is more focused on practical skills compared with universities and part of the degree requirement typically involves various long-term internships at companies. For illustrational purposes, individuals with a university/applied university/vocational education on average had an annual income of respectively 50.00/36.000/25.000 euro between 2007 and 2009 (Statistics Netherlands, 2011) .
These figures only count students who remained in the Netherlands after completing their secondary school. Around 2% of Dutch students register for a bachelor- or master degree abroad at some point during their education (Department of Education, 2016). As robustness check in the main analysis, I exclude all students from the analysis who are not registered at any educational institution at least 2 out of the 3 years between the ages 19 and 21 years.
The most important exceptions are medicine, veterinary medicine and dentistry, as these studies typically are comparatively expensive to offer.
Some analyses can be carried out using a larger sample. For consistency, results in the main text are based on the cohorts 1994–1998 unless specified otherwise. The main tables also report results for a larger sample as robustness test whenever possible.
A small number of students is exempted from making the test due to disabilities and not all schools have agreed to make the test scores available to Statistics Netherlands. Nonetheless, I observe the test score for the large majority of the population (70%). The coefficients on the urbanization measure of columns (1) and (2) of Tables 2 and 3 are virtually unchanged (within 10% in all cases) when estimating it either on the full sample of individuals born between 1994 and 1998 or the 70% sample for whom I observe the test score. Hence, different selection between rural and urban regions into which schools agreed to make the test scores available to Statistics Netherlands is not driving the results.
These groups are kindergarten, primary school, some secondary education (low, middle or high), secondary education degree (low, middle or high), some university or applied university, applied university bachelor, university bachelor, university master or applied university master, and doctoral degree. Vocational education is not listed separately, but included in the three levels of secondary education.
To avoid potential reverse causality problems, I calculate the density based on the spatial distribution of the population in 1995. As the robustness analysis reveals, the results remain virtually unchanged when I use the spatial distribution of 1840 as instrumental variable.
For instance, the correlation between density within 10 km and the density within 5 km and the density within 20 km is 0.89 in both cases. Alternatively one could also define density based on the number of schools within a given distance as in Gibbons and Silva (2008) or only use the population density of the zip code itself. The results are similar and the conclusions of this paper do not depend on the selected measure of density.
In the empirical framework, I treat the decision for secondary school and tertiary school as two separate decisions. It would also be possible to estimate the two decisions in a joint framework, in which the decision for secondary school and tertiary education are made jointly. While a joint framework might be a better representation for the decision process of certain students, it would significantly complicate the exposition and the interpretation of the coefficients. Furthermore, the most important effect of a joint framework would be a change in the timing when a student does or not does decide to attend university, which should leave the joint effect of density on university attendance across the two decision moments unaffected.
The reason for allowing a 3-year lag is that some students opt for a gap year between completing secondary school and starting tertiary education.
Result not separately included in the paper but available from the author upon request.
A different approach would be to use the Chetty and Hendren (2018) identification strategy and use children who move between regions at different ages to generate urban exposure effects. However, within the group of movers there is a substantial decline in the average end-of-primary school test score by the age of moving, even after the age of 12 years when the test score is essentially predetermined. This differential selection into migration by the age of children hence makes it difficult to apply the Chetty and Hendren (2018) identification strategy within this context.
Such approach is difficult to implement for educational outcomes, as most countries lack repeated outcomes for children on a national level, which would allow fixed-effect estimations similar to Combes et al. (2008) with grades instead of wages. Individual-fixed effect approaches have been successfully applied within schools in the economics of education literature to identify effects of school level variables, such as teacher quality (see Chetty et al., 2014b ).
Children in urban environments indeed have higher average test scores on the end-of-primary school tests, even when conditioning on household and child characteristics. A one-log point increase in population density raises the average end-of-primary school test score by 0.2 points and increases the probability that a child has at least a score of 545 by about 1 percentage point (mean 15 pp).
Alternatively, one may also worry that children in rural areas obtain a degree from a middle secondary school, then continue with some years of applied university before switching to university. In this case, children from rural areas would simply follow a different track to end up at university. I do find some evidence for this, as children who obtain a middle secondary school degree are slightly more likely to enroll in university in the seven years after graduating from middle secondary school if they grow up in a rural area rather than an urban area. However, the increased usage of this alternative route in rural areas is far too small to compensate for the negative effects described in Tables 2 and 4. Overall, a one-log point increase in density reduces the probability that a student enrolls for university by 0.6% among the group of middle secondary school graduates (25% of the sample, see Table 1). Hence, taking this path to university into account reduces the overall effect of density on university enrollment by 0.25 * 0.6 = 0.15%. Hence, this alternative route can compensate for about 10% of the total effect of density on university enrollment of 1.4%.
The majority (62%) of these students instead obtains a degree from a middle secondary school. The drop-out figure of 21% is in line with the statistics reported for this period by Statistics Netherlands (2019) .
The GPA is based on the national examinations at the end of high school. In the final 2 years of secondary school, students can enroll in one of the possible four tracks (humanities, social sciences, biology or natural sciences). The track determines the subjects in which the students take their final examinations.
For the students born in 1994–1998, it is possible to include both the secondary school test score as well as end of primary school test score. However, adding the end of primary test score results offers little explanatory power over the high school GPA and leaves the coefficient virtually unchanged. Hence, the loss of this variable due is more than outweigh by the higher precision obtained due to the larger sample.
Kelly (2019 , 2020 ) shows that spatial autocorrelation in the dependent and independent variables means that the standard errors might be too small in many spatial regressions. Using the standard error correction proposed by Kelly (2020) increases the standard errors of column 3 in Table 2 by a factor of 1.8, which thus remains significant at the 0.1% level. The correction for Table 4 standard errors is somewhat more complicated due to the low number of observations in many zip codes, which results in very noisy estimates of the zip code fixed effect. If we assume the same spatial autocorrelation in Table 2 as in Table 4 then the estimates in Table 4 remain significant at the 5% level with a t -statistic of 2.6.
I would like to express my gratitude to Paul Verstraten (CPB Netherlands Bureau for Economics Policy Analysis) for providing the shape files containing the boundaries of the 1840 municipalities as well as the digitalized 1840 census.
A small number of zip codes cannot be matched to the densities of 1840, as they are located on land that has been reclaimed from the sea after 1840. Nonetheless, the instrument is available for 99.6% of the observations.
The reported coefficients in Table 5 are based on the general estimator as reported in Oster (2019 , Section 3.2), which allows for a more general covariance structure of the unobserved variable with the observed explanatory and outcomes variables. However, the adjusted coefficients are virtually identical when using the simplified and general estimator in this study.
1.23% of this increase is due to the increase 1.46% in probability that a child graduates from upper secondary school as seen in Table 3, taking into account that on average 84% continues with university. A further 0.15% of the increase is due to the 0.8% increase in university attendance among the children who complete upper secondary school, which contains about 20% of the population (see Table 1).
This means that I implicitly assume that the population is spread equally across zip codes. However, as zip codes are fairly small (average of 8 km 2 ) compared to the 10-km radius (314 km 2 ) radius, this assumption has little effect on the relative differences in urbanization between regions.
For some very isolated zip codes (for instance, on the islands in the North), there are no other zip codes within 10 km, and hence the population with 10 km is simply the zip codes own population.
The author wishes to acknowledge the statistical offices that provided the underlying data making this research possible: National Institute of Statistics and Censuses, Argentina; National Statistical Service, Armenia; Bangladesh Bureau of Statistics, Bangladesh; Ministry of Statistics and Analysis, Belarus; National Institute for Statistics and Economic Analysis, Benin; National Institute of Statistics, Bolivia; Central Statistics Office, Botswana; Institute of Geography and Statistics, Brazil; National Institute of Statistics and Demography, Burkina Faso; National Institute of Statistics, Cambodia; Central Bureau of Census and Population Studies, Cameroon; Statistics Canada, Canada; National Bureau of Statistics, China; National Administrative Department of Statistics, Colombia; National Institute of Statistics and Censuses, Costa Rica; National Statistics Office, Dominican Republic; National Institute of Statistics and Censuses, Ecuador; Central Agency for Public Mobilization and Statistics, Egypt; Bureau of Statistics, Fiji; National Institute of Statistics and Economic Studies, France; Federal Statistical Office, Germany; National Institute of Statistics, Guatemala; Institute of Statistics and Informatics, Haiti; Central Statistical Office, Hungary; BPS Statistics Indonesia, Indonesia; Central Statistics Office, Ireland; Central Bureau of Statistics, Israel; National Institute of Statistics, Italy; Statistical Institute, Jamaica; National Bureau of Statistics, Kenya; National Statistical Committee, Kyrgyz Republic; Statistics Bureau, Laos; Bureau of Statistics, Lesotho; Institute of Statistics and Geo-Information Systems, Liberia; National Statistical Office, Malawi; Department of Statistics, Malaysia; National Directorate of Statistics and Informatics, Mali; National Institute of Statistics, Geography, and Informatics, Mexico; National Institute of Statistics, Mozambique; Central Bureau of Statistics, Nepal; National Institute of Information Development, Nicaragua; Statistics Division, Pakistan; Central Bureau of Statistics, Palestine; Census and Statistics Directorate, Panama; National Statistical Office, Papua New Guinea; General Directorate of Statistics, Surveys, and Censuses, Paraguay; National Institute of Statistics and Informatics, Peru; Central Statistics Office, Poland; National Institute of Statistics, Portugal; National Institute of Statistics, Romania; Federal State Statistics Service, Russia; National Institute of Statistics, Rwanda; Government Statistics Department, Saint Lucia; Statistics Sierra Leone, Sierra Leone; Statistical Office of the Republic of Slovenia, Slovenia; Statistics South Africa, South Africa; National Bureau of Statistics, South Sudan; Central Bureau of Statistics, Sudan; Bureau of Statistics, Tanzania; National Statistical Office, Thailand; National Institute of Statistics, Togo; Bureau of Statistics, Uganda; State Committee of Statistics, Ukraine; Bureau of the Census, United States; National Institute of Statistics, Venezuela; General Statistics Office, Vietnam; Central Statistics Office, Zambia and National Statistics Agency, Zimbabwe.
Adukia A. , Asher S. , Novosad P. ( 2020 ) Educational investment responses to economic opportunity: evidence from Indian road construction . American Economic Journal: Applied Economics , 12 : 348 – 376 .
Google Scholar
Ahlin L. , Andersson M. , Thulin P. ( 2018 ) Human capital sorting: the “when” and “who” of the sorting of educated workers to urban regions . Journal of Regional Science , 58 : 581 – 610 .
Alesina A. , Hohmann S. , Michalopoulos S. , Papaioannou E. ( 2019 ) Intergenerational Mobility in Africa (No. w25534). National Bureau of Economic Research.
Altonji J. G. , Elder T. E. , Taber C. R. ( 2005 ) Selection on observed and unobserved variables: assessing the effectiveness of Catholic schools . Journal of Political Economy , 113 : 151 – 184 .
Autor D. ( 2019 ) Work of the past. Work of the future. American Economic Association: Papers and Proceeding.
Bacolod M. , Blum B. S. , Strange W. C. ( 2010 ) Elements of skill: traits, intelligence, education, and agglomeration . Journal of Regional Science , 50 : 245 – 280 .
Bau N. ( 2019 ) Estimating an Equilibrium Model of Horizontal Competition in Education . London: Centre for Economic Policy Research.
Baum-Snow N. , Freedman M. , Pavan R. ( 2018 ) Why has urban inequality increased? American Economic Journal: Applied Economics , 10 : 1 – 42 .
Behrens K. , Duranton G. , Robert-Nicoud F. ( 2014 ) Productive cities: sorting, selection, and agglomeration . Journal of Political Economy 122 : 507 – 53 .
Berry C. R. , Glaeser E. L. ( 2005 ) The divergence of human capital levels across cities . Papers in Regional Science , 84 : 407 – 444 .
Bosquet C. , Overman H. G. ( 2019 ) Why does birthplace matter so much? Journal of Urban Economics , 110 : 26 – 34 .
Burgess S. , Greaves E. , Vignoles A. ( 2019 ) School choice in England: evidence from national administrative data . Oxford Review of Education , 1 – 21 .
Bütikofer A Peri G. How cognitive ability and personality traits affect geographic mobility . Journal of Labor Economics, forthcoming .
Card D. ( 1993 ) Using geographic variation in college proximity to estimate the return to schooling (No. w4483). National Bureau of Economic Research.
Chetty R. , Hendren N. , Kline P. , Saez E. ( 2014 a) Where is the land of opportunity? The geography of intergenerational mobility in the United States . The Quarterly Journal of Economics , 129 : 1553 – 1623 .
Chetty R. , Friedman J. N. , Rockoff J. E. ( 2014 b) Measuring the impacts of teachers I: evaluating bias in teacher value-added estimates . American Economic Review , 104 : 2593 – 2632 .
Chetty R. , Hendren N. ( 2018 ) The impacts of neighborhoods on intergenerational mobility I: childhood exposure effects . The Quarterly Journal of Economics , 133 : 1107 – 1162 .
Chetty R. , Hendren N. , Katz L. F. ( 2016 ) The effects of exposure to better neighborhoods on children: new evidence from the Moving to Opportunity experiment . American Economic Review , 106 : 855 – 902 .
Cohen-Zada D. , Sander W. ( 2008 ) Religion, religiosity and private school choice: implications for estimating the effectiveness of private schools . Journal of Urban Economics , 64 : 85 – 100 .
Combes P. P. , Duranton G. , Gobillon L. ( 2008 ) Spatial wage disparities: sorting matters ! Journal of Urban Economics , 63 : 723 – 742 .
Combes P. P. , Gobillon L. ( 2015 ) The empirics of agglomeration economies. In Duranton G. , Henderson J. V. , Strange W. C. (eds) Handbook of Regional and Urban Economics , Vol. 5 , pp. 247 – 348 . Amsterdam : Elsevier .
Google Preview
Dauth W. , Findeisen S. , Moretti E. , Suedekum J. ( 2018 ). Matching in cities (No. w25227). National Bureau of Economic Research.
Davis D. R. , Dingel J. I. ( 2019 ). A spatial knowledge economy . American Economic Review , 109 : 153 – 70 .
Deutscher N. ( 2020 ) Place, peers, and the teenage years: long-run neighborhood effects in Australia . American Economic Journal: Applied Economics , 12 : 220 – 249 .
Duranton G. , Puga D. ( 2004 ) Micro-foundations of urban agglomeration economies. In Duranton G. , Henderson J. V. , Strange W. C. (eds) Handbook of Regional and Urban Economics , Vol. 4 , pp. 2063 – 2117 . Amsterdam : Elsevier .
Eckert F. , Hejlesen M. , Walsh C. ( 2020 ) The Return to Big City Experience: Evidence from Refugees in Denmark . NBER Working Paper.
Frenette M. ( 2006 ) Too far to go on? Distance to school and university participation . Education Economics , 14 : 31 – 58 .
Gennaioli N. , La Porta R. , Lopez-de-Silanes F. , Shleifer A. ( 2013 ) Human capital and regional development . The Quarterly Journal of Economics , 128 : 105 – 164 .
Gennaioli N. , La Porta R. , De Silanes F. L. , Shleifer A. ( 2014 ) Growth in regions . Journal of Economic Growth , 19 : 259 – 309 .
Gibbons S. , Silva O. ( 2008 ) Urban density and pupil attainment . Journal of Urban Economics , 63 : 631 – 650 .
Glaeser E. L. , Kolko J. , Saiz A. ( 2001 ) Consumer city . Journal of Economic Geography , 1 , 27 – 50 .
Glaeser E. L. , Mare D. C. ( 2001 ). Cities and skills . Journal of Labor Economics , 19 : 316 – 342 .
Gould E. D. ( 2007 ) Cities, workers, and wages: a structural analysis of the urban wage premium . The Review of Economic Studies , 74 : 477 – 506 .
Groot S. P. , de Groot H. L. , Smit M. J. ( 2014 ) Regional wage differences in the Netherlands: micro evidence on agglomeration externalities . Journal of Regional Science , 54 : 503 – 523 .
Hoxby C. M. , Avery C. ( 2012 ) The missing “one-offs”: the hidden supply of high-achieving, low income students (No. w18586). National Bureau of Economic Research.
Jensen R. ( 2010 ). The (perceived) returns to education and the demand for schooling . The Quarterly Journal of Economics , 125 : 515 – 548 .
Katz L. F. , Goldin C. D. ( 2008 ) The Race between Education and Technology . Cambridge : Harvard University Press .
Kelly M. ( 2019 ) The Standard Errors of Persistence . London : CEPR
Kelly M. ( 2020 ) Understanding Persistence . London : CEPR
Knight J. , Shi L. ( 1996 ) Educational attainment and the rural–urban divide in China . Oxford Bulletin of Economics and Statistics , 58 : 83 – 117 .
Newbold K. B. , Brown W. M. ( 2015 ) The urban–rural gap in university attendance: determinants of university participation among Canadian youth . Journal of Regional Science , 55 : 585 – 608 .
Minnesota Population Center. ( 2019 ). Integrated Public Use Microdata Series , International: Version 7.2 [dataset]. Minneapolis (MN): IPUMS ( https://doi.org/10.18128/D020.V7.2).
Oster E. ( 2019 ) Unobservable selection and coefficient stability: theory and evidence . Journal of Business & Economic Statistics , 37 : 187 – 204 .
De La Roca J. , Puga D. ( 2017 ) Learning by working in big cities . The Review of Economic Studies , 84 : 106 – 142 .
De la Roca J. ( 2017 ). Selection in initial and return migration: evidence from moves across Spanish cities . Journal of Urban Economics , 100 , 33 – 53 .
Rosenthal S. S. , Strange W. C. ( 2004 ) Evidence on the nature and sources of agglomeration economies. In Duranton G. , Henderson J. V. , Strange W. C. (eds) Handbook of Regional and Urban Economics , Vol. 4 , pp. 2119 – 2171 . Amsterdam : Elsevier .
Statistics Netherlands. ( 2011 ) Studeren loont. Inkomens van afgestudeerden in het mbo, hbo en wetenschappelijk onderwijs [Online publication].
Statistics Netherlands. ( 2019 ). Statline. https://opendata.cbs.nl/statline/#/CBS/en/
Verstraten P. , Verweij G. , Zwaneveld P. J. ( 2019 ) Complexities in the spatial scope of agglomeration economies . Journal of Regional Science , 59 : 29 – 55 .
A. The Urban–Rural Educational Gap

Urban–rural education gap based on 25-year-old males in DHS data. The figure is based on 57 countries included in the Demographic and Health Survey (DHS). Reported are the average years of schooling in urban and rural areas for 25-year-old males. The urban–rural definition follows the definition of DHS, which is based on the urban–rural definition of the country in question. Countries with fewer than 100 18-year old males for either the rural or urban region of the country have been excluded from the original sample of 75 countries.

Urban–rural school attendance gap based on 18-year-old males in the IPUMS International database (Minnesota Population Center, 2019) . The figure is based on the latest census for 55 countries included in the IPUMS International data which include an urban/rural definition in their census (which excludes most developed countries). The y -axis shows the percentage of children that indicate that they were attending school at the time of the census in urban and rural areas for 18-year-old males. The urban–rural definition is based on the definition of the statistical office of the country in question.
B. Construction of Urbanization Measure
The administrative data include information on place of residence from January 1995 onward. Hence, I start by calculating the number of individuals registered per zip code on the 5th January 1995. As the next step, I determine the centroid of each zip code using GIS software. The map of the Netherlands with all the centroids is displayed in Figure A1 . For each centroid, I determine which other zip codes lie within a 10-km radius of the centroids and add up the population of these zip codes. For zip codes that lie partially in the 10-km radius, I multiply the share of the zip code area covered by the 10-km radius with the population of that particular zip code. 34 , 35 The average zip code has 43 other zip-codes within a 10-km radius and even the first percentile of zip codes has 8 other zip-codes within a 10-km radius. The correlation between the number of individuals living within 5, 10 and 20 km is fairly high at 0.89. Finally, I take the log of the number of individuals within the 10-km radius as urbanization measure. Figure 3 in the main text shows the resulting map of the urbanization measure. In addition, Figure B2 shows a histogram of the urbanization measure.

Map of Dutch zip codes and centroids.

Histogram of urbanization measure at the age 11 years. The histogram of urbanization measure for the cohorts born between 1994 and 1998. Areas with an urbanization score below 9 (containing 230 out of the 631.815 observations in the baseline sample) not displayed here.
C. Additional Robustness Analyses
C.1. probability of upper secondary school graduation, conditional on being registered at an upper secondary school.
Effect of urbanization on probability of obtaining a degree in upper secondary school, conditional on enrollment in upper secondary school
All results are based on individuals born between 1994 and 1998. Dependent variable is whether a child graduates from an upper secondary school, conditional on being enrolled at the third grade of upper secondary school. Individual controls include a gender dummy and cohort dummies. Family controls include dummies for parental education combinations (168 dummies), parental nationality combinations (nine dummies), year-of-birth of oldest parent (40 dummies), family income (interacted with cohort-fixed effects), low-income dummy (interacted with cohort-fixed effects), top-coded income dummy (interacted with cohort-fixed effects) and dummy for insufficient income data (interacted with cohort-fixed effects). For a detailed explanation of the construction of the urbanization measure or the family controls, see Section 3. Column (4) excludes all children who moved municipalities during school-going age. Column (5) removes all parents for whom uncertainty exists about the education level of one or both parents. Column (6) adds municipality-fixed effects for the municipality in which children live at age 11 years (430 dummies), whereas column (7) adds province-fixed effect for the province in which children live at age 11 years (12 dummies). Column (8) instruments for modern densities (based on 1995 population distribution, see Appendix B) with historical densities. All standard errors are clustered on the municipality level. Columns (1–7) are estimated by OLS and column 8 by 2SLS. First stage results of column (8) are reported in Appendix C3 (first stage F -statistic: 596.93).
C.2. Applied university enrollment
Effect of urbanization on applied university attendance
Note : All results apart from column (5) are based on individuals born between 1989 and 1998. Dependent variable is whether a child attends applied university as highest education within 3 years of graduating from upper secondary school. Individual controls include a gender dummy and cohort dummies. Family controls include dummies for parental education combinations (168 dummies), parental nationality combinations (nine dummies), year-of-birth of oldest parent (40 dummies), family income (interacted with cohort-fixed effects), low-income dummy (interacted with cohort-fixed effects), top-coded income dummy (interacted with cohort-fixed effects) and dummy for insufficient income data (interacted with cohort-fixed effects). For a detailed explanation of the construction of the urbanization measure or the family controls, see Section 3. Column (4) excludes all children who moved municipalities during school-going age. Column (5) limits the sample to the cohorts born between 1994 and 1998, in line with the baseline sample of Table 2 . Column (6) removes all parents for whom uncertainty exists about the education level of one or both parents. Column (7) adds municipality-fixed effects for the municipality in which children live at age 11 years (430 dummies), whereas column (8) adds province-fixed effect for the province in which children live at age 11 years (12 dummies). Column (9) instruments for modern densities (based on 1995 population distribution, see Appendix B) with historical densities. All standard errors are clustered on the municipality level. Columns (1–8) are estimated by OLS and column (9) by 2SLS. First stage results of column (9) are reported in Appendix C3 (first stage F -statistic: 1028.11).
C.3. IV first stage
Figure C3.1 displays the population densities of Dutch municipalities in 1840. The number of municipalities (1340) is relatively large compared the modern day number of municipalities (380). The map below is used as input to construct the IV measure, namely the number of individuals in 1840 living within 10 km of all current zip codes, using the procedure outlined in Appendix B. Notice that I make the implicit assumption that the population in 1840 was spread homogenously within municipalities, as the 1840 population statistics are only available at the municipality level. Figure C3.2 shows the resulting IV-density measure for the zip codes.

Population density per municipality in 1840. When calculating the densities based on the 1840 population map, I implicitly assumed that population is spread homogenously within municipalities. Notice that the Netherlands contained a substantially larger amount of inland water compared with the contemporary Netherlands, due to the land reclamation programs in the 19th and 20th centuries.

Urbanization measure based on 1840 population distribution. The map displays the urbanization measure for each zip code (log of number of people living within 10 km), based the 1840 population distribution. See Appendix B for the construction of the measure and Figure C3.1 for the 1840 population distribution. The gray areas have an urbanization measure below 8. The gray mass in the center of the Netherlands consists of land that has been reclaimed since 1840, and hence had very few to no individuals living with 10 km in 1840, as can be also seen in Figure C3.1 .
First stage of regressing urbanization based on the 1840 densities on contemporary urbanization measure (based on 1995 densities)
Note : First stage of 2SLS regression of baseline urbanization measure (based on the 1995 population distribution) on the historical urbanization measure (based on the 1840 population distribution). The first stage of Tables 4 and C2 are virtually identical and not separately reported here, with a slightly smaller F -statistics (1028.11) due to the smaller number of observations.
Email alerts
Companion article.
- Erratum to: The urban–rural education gap: do cities indeed make us smarter?
Citing articles via
- Recommend to your Library
Affiliations
- Online ISSN 1468-2710
- Print ISSN 1468-2702
- Copyright © 2024 Oxford University Press
- About Oxford Academic
- Publish journals with us
- University press partners
- What we publish
- New features
- Open access
- Institutional account management
- Rights and permissions
- Get help with access
- Accessibility
- Advertising
- Media enquiries
- Oxford University Press
- Oxford Languages
- University of Oxford
Oxford University Press is a department of the University of Oxford. It furthers the University's objective of excellence in research, scholarship, and education by publishing worldwide
- Copyright © 2024 Oxford University Press
- Cookie settings
- Cookie policy
- Privacy policy
- Legal notice
This Feature Is Available To Subscribers Only
Sign In or Create an Account
This PDF is available to Subscribers Only
For full access to this pdf, sign in to an existing account, or purchase an annual subscription.
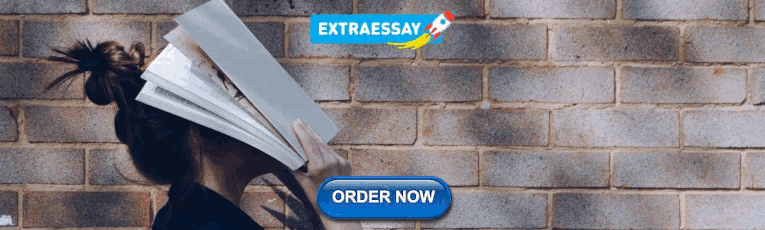
The New Problem Facing Urban School Districts

- Share article
Many of our great urban school districts have been in crisis for decades, struggling to deliver on the promise of a high-quality education for all students. That is especially true for Rust Belt cities that have seen years of declining public school enrollment, eroding the operational foundations of districts and thus making it even more difficult to set a steady course for improvement.
This long-standing problem in cities like Detroit and Memphis, Tenn., that have had overall declining populations is now a problem for school systems in cities like Austin, Texas; Denver; and Nashville, Tenn. These cities are thriving economically yet because of declining birthrates and rising housing costs, now have school districts with decreasing student populations.
And all these changes are happening in the context of the COVID-19 pandemic, which has exacerbated many preexisting problems, including academic learning that falls significantly short of state standards, especially for students from poor families and those of color. It has also created a mental health crisis for millions of students.
Our big-cit y school systems are on the front lines of these challenges and have historically had the greatest difficulty adjusting to change because of their politics, size, cultures, and management structures. They were designed a century ago when student populations seemed to be ever-growing and middle-income-generating factory jobs required just a basic education—a far cry from the present.
And now, in most American cities, housing costs are increasing, pricing out younger families who then move elsewhere. At the same time, birthrates are dropping. The two trends together leave many populations with smaller proportions of school-age children. That often impacts urban-district enrollment to a greater extent than the defection of families to other kinds of schooling—private, nondistrict charter, or home schooling, even with pandemic-related increases.
Many demographers have focused on the long-term risk to Social Security or Medicare posed by the lower birthrates, but K-12 education is actually the first institution to be dramatically affected. Shrinking is hard. But it does not have to be catastrophic and if done thoughtfully can even be an opportunity to restart or build higher-quality schools.
Many demographers have focused on the long-term risk to Social Security or Medicare posed by the lower birthrates, but K-12 education is actually the first institution to be dramatically affected.
We know. We’ve both had considerable experience with district downsizing, Brian from the inside as the director of planning for the Denver public schools from 2014 to 2018 and Van from the outside supporting a civic organization interested in the proces s over the past two decades. We have also observed districts do this work poorly, though some like Denver, nearby Mapleton, and Indianapolis have so far managed these difficult changes with far more wins than losses for students.
In general, school districts are good at adjusting to growth but not its opposite. Ribbon cuttings create far fewer protesters than a school closure. Few district actions are as unpopular as consolidating schools, given how deeply people care about them and how much they view them, rightly, as the center of their communities
Nonetheless, the budget constraints of operating an aging set of underutilized facilities can create political, operational, and educational challenges. Certainly pandemic-related staffing shortages among classroom teachers, nurses, mental health professionals, bus drivers, and more, exacerbated by too many schools for the number of children, deprive students of the education they deserve. Further, given tight budgets, the fewer dollars that are spent on the educational “envelope” (including utilities and maintenance), the more dollars that can flow to the classroom.
School closings and consolidations become inevitable, and the sooner districts start grappling with that reality, the better for students, their families, and their neighborhoods. We’ve found that a steady focus on preparing students for the future by delivering as high-quality education as possible creates the best path forward.

Here are three actions we would encourage districts and their partner organizations to pursue:
- Create a fact base that incorporates demographic trends like birthrates, housing prices, and U.S. Census Bureau data with district, charter, and private school enrollment data to forecast enrollments and build a common understanding among key stakeholders about the underlying dynamics and the magnitude of the challenges.
- Design stakeholder engagement plans to capture the voice of city, district, school, and community members in designing solutions that respect these voices while addressing the challenges. Often, processes have greater buy-in from the community when the school district works in collaboration with existing community organizations rather than working alone without trusted community partners.
- Ensure impacted students have dramatically stronger educational opportunities as a result of the tough decisions districts will need to make. If school closure must occur, prioritize the entry of the students most affected into higher-performing existing schools, providing transportation for the students and additional resources to the schools so their effectiveness is not compromised. Or instead of using existing schools, especially if that means merging several low-performing ones, consider implementing the practices of high-performing schools and launching new school models that reflect the wants and needs of the impacted community.
The district must also create financial and other incentives so that the most effective teachers and leaders are serving in the schools with the students most affected by downsizing. It is critical that the entire system shift to focusing on these students and their communities. All these efforts, if done with rather than to communities, can lead to wins for those affected.
Declining school district enrollment is inevitable in most cities, and system leaders should be prepared to educate their communities and make short- and long-range plans to meet the challenges. Right-sizing doesn’t have to be a torturous process aimed just at reducing financial strain on a district. If done well, it can be an opportunity to reset district organizational structures and finances to drive student achievement upward.
A version of this article appeared in the March 09, 2022 edition of Education Week as The New Problem Facing Urban School Districts
Sign Up for The Savvy Principal
Edweek top school jobs.

Sign Up & Sign In

- NAEYC Login
- Member Profile
- Hello Community
- Accreditation Portal
- Online Learning
- Online Store
Popular Searches: DAP ; Coping with COVID-19 ; E-books ; Anti-Bias Education ; Online Store
Urban Education
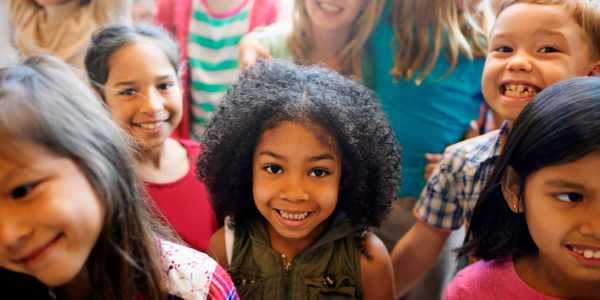
You are here
Most recent.
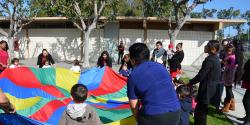
Preschool Without Walls: Empowering Families Through Outdoor Community Classrooms
Authored by.
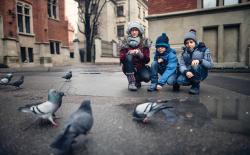
From Puddles to Pigeons: Learning about Nature in Cities
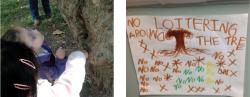
“There’s a Hole in the Tree!” Kindergartners Learning in an Urban Park
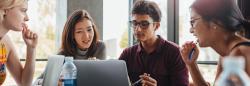
Seven Ideas for Early Childhood Educators Teaching in Cities
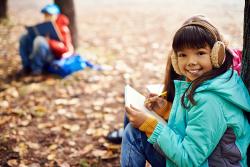
Community-Based Engineering STEM Experiences From a Second Grade Urban Classroom
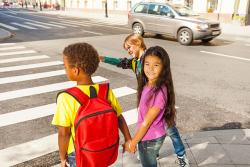
Extreme Diversity in Cities: Challenges and Solutions for Programs Serving Young Children and Their Families
Experiencias de stem en ingeniería basada en la comunidad en un salón de clases de segundo grado en una escuela urbana.
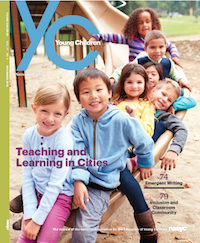
November 2016
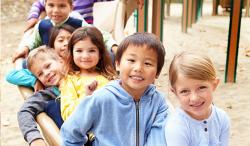
Read the Full Issue (November 2016)
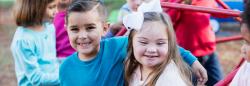
Our Proud Heritage—Playground: A Historical Context

Urban Education: The State of Urban Schooling at the Start of the 21st Century
Monday, November 1, 2004 Martin Haberman Distinguished Professor University of Wisconsin Milwaukee
These objective size/density definitions however do not convey the range of meanings intended or received when the term is most commonly used. Perceptions of urban areas differ widely. Rooted in the early history of this country and illustrated in the writings of Alexander Hamilton is a vision of urban as fostering freedoms. This perception defines cities as places of refuge and opportunity and is a vision widely accepted in most other countries. (Meyer, 1957) Also rooted in America’s history and illustrated in the writings of Thomas Jefferson is the opposing perception of urban as dysfunctional and the cause of many societal problems. (Malone, 1948) In American parlance “God’s country” is used to refer to rural areas or nature preserves not cities.
During the first half of the 20th century urban areas were viewed by many as economically dynamic, attracting and employing migrant populations from small towns, rural areas and abroad. During the second half of the 20th century however the term urban became a pejorative code word for the problems caused by the large numbers of poor and minorities who live in cities. Such negative perceptions of urban profoundly affect education and shape the nature of urban schooling.
URBAN EDUCATION: STUDENTS AND STRUCTURE
Unlike most other countries where education is a federal or national function schooling in America is a decentralized one. States are the legally responsible entities but local districts are generally perceived as the accountable units of administration. There were approximately 53 million American children entering public and private schools in the fall of the year 2000. Thirty five percent are members of minority groups. One in five comes from immigrant households. Nearly one-fifth live in poverty. (Education Week, Sept.27, 2000) Eleven states account for more than half of the children in poverty: California, Texas, New York, Florida, New Jersey, Pennsylvania, Ohio, Illinois, Michigan, North Carolina and Georgia. All these students are overseen by more than 15,000 local districts with almost 90,000 schools. (Cuban, 2001) The 120 largest school districts, generally defined as the urban ones, serve 11 million students most of whom are of color or in poverty. (Education Commission of the States, 1997).
Since 1962 the achievement gap between disadvantaged populations and more affluent ones has widened. At one extreme urban school districts graduate half or fewer of their students. (Arbanas, 2001) At the other extreme 11% of American students are now among the top 10 percent of world achievers. “If you’re in the top economic quarter of the population, your children have a 76% chance of getting through college and graduating by age 24…If you’re in the bottom quarter, however, the figure is 4 %.” (Loeb, 1999) White students’ achievement in reading, math and science ranks 2nd, 7th and 4th when compared with students worldwide. Black and Hispanic students however rank 26, 27th and 27th on these basic skills. (Bracey, 2002) Such data describe but do not explain the causes of such wide disparities among educational outcomes. The following section describes some of the challenges which, taken together, help to explain the failure of urban school districts. A final section describes many of the characteristics of successful urban schools.
URBAN EDUCATION: SPECIAL CHALLENGES
1.Highly Politicized School Boards. Board politics in major urban school districts often impede judicious decision-making (Ortiz, 1991) Two practices are particularly dysfunctional. First, in an effort to better represent diverse constituencies, citywide board seats have given way to narrowly drawn district seats. Board members elected from such districts may find it difficult to support policies and budgets aimed at the good of the total district when doing so is viewed negatively by parents, citizens and educators in their own neighborhood schools. Second, board members too frequently try to micromanage large, complicated school organizations thereby abrogating the leadership and accountability of their own superintendent. Finally, it is not unusual for narrow majorities on boards to change after a board election and for a superintendent to find his/her initiatives no longer supported and even have his/her contract bought out.
2.Superintendent Turnover. The average years of service for an urban superintendent are 2 and 1/3yrs. (Urban Indicator, 2000). As a result a new superintendent may function more as a temporary employee of a school board than as the educational leader of the district and the community. Administrators and teachers are reluctant to throw themselves into new initiatives that are not likely to remain in place long enough to show any results. Constituencies (governments, businesses, church groups, foundations, universities, etc.) with whom the superintendent must interact may take a wait and see attitude rather than become active partners in the new superintendent’s initiatives.
3.Principals as Managers and Leaders. The size and complexity of most urban schools inevitably lead to a focus on the principal as the manager or CEO of a major business enterprise. This emphasis has led to a transformation of the traditional principal role as an instructional leader. (Haberman, 2001) Few urban districts dismiss principals because of low student achievement unless the achievement falls low enough for the school to be taken over by the state or district and be reconstituted. In practice the typical urban principal who is transferred or coaxed into retirement is one that has “lost control of the building.” The district’s stated system of accountability may place student learning as the highest priority but the real basis for defining urban principals as “failing” is not because they have been unable to demonstrate increasing student achievement but because they have been unable to maintain a custodial institution. The fact that most urban principals spend the preponderance of their time and energy on management issues demonstrates that they fully understand this reality. (Kimball & Sirotnik, 2000)
4.Government Oversight. Local and state government officials involve themselves more and more in educational policies that impact urban districts. This politicization of education produces an endless stream of regulations and funding mechanisms, which encourage or penalize the efforts of local urban districts. Like an overmedicated patient the treatments frequently counteract one another or have unintended negative consequences.
5.Central Office Bureaucracies. In rural, small town and suburban districts, classroom teachers comprise 80% or more of the school district’s employees. In the 120 largest urban districts the number of employees other than teachers is approaching a ratio of almost 2:1; that is, for every classroom teacher there are almost two others employed in the district ostensibly to perform services which would help these teachers. (Knott & Miller, 1987) The effect of this distortion is frequently a proliferation of procedures, regulations, interruptions and paperwork that impedes rather than facilitates student learning. Many teachers leaving urban districts cite paperwork and bureaucratic over regulation as among the most debilitating conditions they face.
The self-serving nature of the district bureaucracy frequently impedes initiatives, which would decentralize decision making and transfer power to individual school staffs. (McClafferty, 2000) Historically centralized systems are reluctant to change. Prodded by parents, community and business leaders, urban districts are gradually allowing more decentralized decision making at the school level. In response to bureaucratic rigidities choices are proliferating within public systems. Examples include open enrollment plans, magnet and specialty schools, schools-within-schools, alternative schools, and public choice and charter schools. Urban parents also have increased options outside the public systems through private school voucher programs but these efforts account for less than one percent of enrollment in urban districts. (Hill, 1999)
6.School Staff Accountability. As public school options increase so do calls for accountability. The most frequently tried accountability efforts in the last century have been attempts at merit pay for teachers based on student achievement test scores. Many of these trials have been funded by private foundations and several have been supported initially by local teachers unions. Thus far, however, there have been no successful models for holding either principals or teachers accountable based on achievement scores. (Ross, 1994) In some cases superintendents have clauses in their contracts stating that their tenure or salaries are dependent on improvements in student achievement. In some districts school principals’ annual evaluations and contract extensions are now tied to improving student achievement. Currently, many states have adopted systems for declaring particular schools (or districts) as failing if a given number of the school’s students are below a minimum level of achievement. In these cases the state may mandate that a failing school be reconstituted and may grant the local district the authority to re-staff the school with a new principal and teaching staff. (Crosby, 1999) The staff of a failing school is typically permitted to transfer to other schools in the district. This means that while an urban school district is being held accountable based on achievement data the individual staff members are not. Furthermore the concept of accountability is non-existent for curriculum specialists, hiring officials, or those who appoint principals, psychologists, safety aides or other school staff.
7.Teacher Shortages. The public clearly understand the importance of well-prepared teachers: 82% believe that the “recruitment and retention of better teachers is the most important measure for improving public schools, more effective than investing in computers or smaller class size.” (Education Commission of the States, 2000) In the next decade there may be as many as 1 million new teachers hired because of turnover, retirement and the fact that the typical teaching career has shortened to approximately 11 years. (Langdon & Vesper, 2000) If the school age population continues to increase, another million teachers may be needed. While all districts face occasional selected shortages of special education teachers, bilingual teachers, math or science teachers, the major impact of the current and continuing teacher shortage falls on the urban school districts. These are the teaching positions that many traditionally prepared teachers are unwilling to take. This problem is confounded by the fact that many urban districts must lay off teachers to make up for budget deficits in a given year while they are simultaneously recruiting teachers to remedy their chronic shortages. (Reid, 2002)
In the states that prepare a majority of the teachers in traditional university based programs more than half of those who graduate and are certified never take teaching positions. (Schug, 1997) “ One third of new teachers leave the profession within five years.” (Education Commission of the States, 2000) The typical teacher education graduate is a 22-year-old white female, monolingual with little work or life experience. She will teach within fifty miles of where she herself attended school. The profile of teachers who succeed and stay in urban school districts differs in important respects. (Sprinthall, 1996) While they are still predominantly women, they are over 30 years of age, attended urban schools themselves, completed a bachelor’s degree in college but not necessarily in education, worked at other full-time jobs and are parents themselves. This successful pool also contains a substantially higher number of individuals who are African American, Latino and male. Typically, the teacher educators who serve as faculty in traditional university-based teacher preparation programs have had little or no teaching experience in urban school districts while those mentoring teachers in alternative licensure programs typically come from long, successful careers as teachers in urban districts.
8.State Licensure Laws. While traditional teacher preparation programs seek to attract more young people into the teaching profession, past experience suggests that many of these graduates will not seek employment in large urban school districts where most of the new hires will be needed. (Schug 1997) To assist in meeting this urban district need, new kinds of recruiting and training programs are being established to attract older, more experienced and more diverse candidates into the teaching profession. States differ widely in their response to these new programs. “Conventional wisdom holds that the key to attracting better teachers is to regulate entry into the classroom ever more tightly…” while others argue that “the surest route to quality is to widen the entryway, deregulate the processes, and hold people accountable for their results…” (Thomas B. Fordham Foundation, 1999) Forty-three states have passed alternative licensure laws which permit the hiring of college graduates who were not trained in traditional programs of teacher preparation. But licensure requirements vary greatly across the states and implementation of new approaches is often controversial even though an increasing number of urban districts now “grow their own” teachers using alternative training programs. (Feistritzer, 1993)
9.Funding for Districts and Classrooms. Students in urban school districts often have substantially less annual per student support than they need. The level of support in urban districts, however, generally exceeds the per pupil expenditures in small towns and rural areas. Many argue, therefore, that in total there is no shortage of funds for urban schools especially when categorical aids and grants are considered. The overall problem of inadequate funding is often exacerbated after the urban school district receives its funds and distributes the monies from the central office levels to the individual schools. Too often too many funds are expended to maintain central office functions leaving too little to cover the direct costs of instruction and equipment in specific school buildings. In addition, many urban districts are characterized by buildings that are outmoded, even unsafe, creating conditions which make learning problematic. New York City, for example, has over 150 buildings still heated by coal.
10.Projectitis. New school board members and superintendents often believe they must set their personal stamps on the district through new initiatives. It is common for urban districts to claim they are aware of and experimenting with the latest curricula in reading, math, science, etc. (Schuttloffel, 2000). In addition, administrators are pressured to try out new programs against drugs, violence, gangs, smoking, sex, etc. This proliferation of programs and projects results in so many new initiatives being tried simultaneously it is not possible to know which initiative caused what results. Furthermore, not enough time is devoted to the program to give it sufficient time to demonstrate intended results. The problem is compounded by the fact that many of these new initiatives are not systematically or carefully evaluated. Veteran teachers, when confronted with the latest initiative from the school board or administration, typically become passive resistors. “This too shall pass.” The constant claims of experts, school boards and superintendents that their latest initiative will transform their schools is frequently stonewalled by the very people who must be the heart of the effort for it to succeed. (Van Dunk, 1999)
11.Narrowing Curriculum and Lowering Expectations. As presented in state and local district philosophy and mission statements the list of what the American people generally expect from their public schools is impressive. A typical list is likely to include the following goals for students: basic skills; motivated life long learners; positive self concept; humane, democratic values; active citizens; success in higher education and in the world of work; effective functioning in a culturally diverse society and a global economy; technological competence; development of individual talents; maintenance of physical and emotional health; appreciation and participation in the arts. In many suburban and small town schools the parents, community and professional school educators maintain a broad general vision about the goals that 13 years of full time schooling is supposed to accomplish. But in the urban districts serving culturally diverse students in poverty these broad missions are frequently narrowed down to “getting a job and staying out of jail.” (Russell, 1986)
Narrowing down the curriculum is particularly evident among the burgeoning populations of students labeled as special or exceptional. The urban districts have disproportionately large and wildly accelerating numbers of students labeled with some form of disability. In urban districts the numbers of special students currently range from 6% to 20% of the student body. This means that exceptional education may account for between 20 and 35% of a total urban district’s budget. Well intentioned but sometimes misapplied state and federal initiatives for special education students encourage the labeling of increasing numbers of students as learning disabled, cognitively disabled or having attention deficit hyperactivity disorder. (Breggin & Breggin, 1994) It is not uncommon for many urban teachers who do not have in depth knowledge of child development to perceive undesirable behavior as abnormal rather than as a temporary stage or as student responses to poor teaching. Thus it is common in urban middle schools to find many students doing well academically who have been labeled as disabled in primary grades and who will carry these labels throughout the remainder of their school careers. Teacher expectations are likely to be very modest for such children; testing may be waived. Some low-income parents may be enticed to agree to have their children labeled exceptional because of financial grants. Recent efforts at inclusion for exceptional students in regular classrooms are aimed at breaking the cycle of low expectations and isolation. In urban districts, however, inclusion mandates are most frequently followed in the primary grades but seldom at the high school level. (Cohen, 2001) The disproportionate number of children of color, particularly males, labeled exceptional further exacerbates this problem.
12.Achievement and Testing. There are four curricula operating in schools. The first is the broadest. It is the written mission of the school district. The second curriculum is what the teachers actually teach. The third operative curriculum is what the students actually learn which is considerably less than what the district claims or what the teachers teach. The fourth curriculum is what is tested for and this is the narrowest of the four. The tested for curriculum frequently supports the narrowing and lowering of expectations. (D’Amico, 1985) As total school and district programs are evaluated by norm referenced tests the accountability of teachers and principals is also narrowed and lowered to the kinds of learning that can be readily tested. Recognition of this problem has led to a new emphasis on standards-led testing or performance assessment closely linked to curriculum in place of the norm reference testing that compares student’s performance to that of others. Done carefully, such assessment measures the performance of successive cohorts of students against an annual rate of improvement (local or state) that is sufficient to achieve whatever curriculum goals have been set. (Education Commission of the States , 1997) For the most part, aligning the goals, curriculum, instruction and testing is yet to be accomplished.
After decades of ignoring low student scores in urban schools or explaining them away as predictable because of family income, national attention has shifted to the numerous and widespread examples of individual urban schools in which students’ scores are being raised and increasing numbers of low income children are reaching grade level achievement. Educators at all levels are being called upon to focus time, thought and resources on the poorest performing schools and the persistent cultural and racial gaps between high and low performing students.
13.Research on Urban School Practices. The research literature in teaching, learning and best practice is robust. We know how children learn, best practices for teachers and what makes specific urban schools successful. (Ascher & Flaxman, 1985) The problem is that schools, even failing schools in urban districts which we would expect would be more amenable to change, are resistant institutions shaped by history, culture and their economic support systems. Schools reflect not only general American norms and values but also their local cultures. In recent years the plethora of federal and state laws and local administrative mandates is testimony to the fact that education is also a flourishing political activity. It seems clear that schools reflect culture more than research, or even logic and theory. (Hunt Jr., 1996) Schools reflect and maintain a multiplicity of social norms contradicted by research based knowledge regarding best practice. It is ironic that those seeking to transform failed urban school districts are frequently expected to prove beforehand that their advocacies are research based while those who stonewall change rely on a rationale of laws, funding mechanisms, school organization and practices which reflect culture and tradition, unsupported by a research knowledge base.
One example lies in what has been described as the pedagogy of poverty (Haberman, 1991). Teaching in many urban schools consists of ritualized teacher acts, which seldom engage students in meaningful learning that is connected to their lives. Such teaching includes giving directions and information; making assignments; monitoring seatwork; testing and grading; settling disputes and punishing noncompliance. While such activities are part of teaching, the research literature is clear that more is needed if schools are to reach diverse groups of students with widely varied backgrounds, interests and experiences. (Smith, 1979) Allowing these limited teaching practices to become the typical ones in the urban districts serving diverse student populations of low income students not only dumbs down the content of the curriculum but also narrows the pedagogy by which it is offered. It is a process in which the student is treated in a disrespectful manner—as if she/he is incapable of appreciating or responding to the genuine teaching of important knowledge.
Taken together, these formidable urban challenges demand the best of educational practices if children are to succeed. While there are no fully successful urban districts every district has individual schools which are effective. Indeed there are examples of outstanding schools in some of the poorest performing urban districts. This anomaly of how individual schools can be successful in the midst of chaos and failure has been sufficiently documented to enable us to state with some certainty the characteristics that account for their effectiveness.
CHARACTERISTICS OF SUCCESSFUL URBAN PROGRAMS
The correlates of the effective school literature are as follows: a clearly stated mission; a safe climate for learning; high expectations for students, teachers and administrators; high student time on task; administrators who are instructional leaders; frequent monitoring of student progress; and positive home-school relations. (Taylor, 2002) These and other necessary conditions are demonstrated in urban schools in the following ways: First, such schools have outstanding principals who serve as leaders rather than building managers. These individuals are instructional leaders with a deep understanding of the teaching and learning process. They also know, appreciate and respect the cultures of the ethnic/racial groups the school serves. (Mitchell, 2000)
Second, there is a critical mass of star teachers or teachers on their way to becoming stars. These are individuals who believe the students and their families are the clients. They believe that student effort rather than ability accounts for success in school and their teaching reflects their ability to generate student effort. These teachers not only know the content of what they teach as well as best practice, but also have effective relationship skills that connect them with students. The ideology and behaviors of star teachers has been well documented. (Haberman, 1995) While there are numerous exceptions star urban teachers tend to be people who are more mature with more varied life experience than college youth. They are often people of color who have attended urban schools themselves. Many have experienced poverty first hand. It is also increasingly likely that they did not go through traditional teacher training.
Third, effective urban schools have a vision of the school’s mission commonly held by students, the entire staff, parents, caregivers and the community. There is a unity of purpose that grows out of everyone who is involved with the school believing, sharing and contributing to this common vision.
Fourth, there is a deep and growing knowledge of how computers and information systems can be used in classrooms and for all school activities. The students and staff are connected to the full resources of the Internet and to the latest instructional programs and not engaged in merely drill and kill activities using a computer.
Fifth, parents are involved in integral ways in the life of the school and not merely as homework tutors or disciplinarians. Parents have a strong voice in all aspects of the school’s decision-making processes. They are regarded as resources able to inform school policy and curriculum.
Sixth, the curriculum is aligned with achievement tests. There is also a closed loop so that the results of testing inform and guide curriculum revisions as well as what teachers teach everyday. Student evaluation includes more than norm referenced tests and places great emphasis on the systematic use of students’ work samples and work products. While achievement tests are important the teachers offer a broad curriculum and do not narrow or dumb it down to prepare for the tests. The acquisition of important knowledge for all students, including those with special needs, is maintained as the school priority.
Seventh, the curriculum is sensitive to issues of equity and social justice. What the teachers plan to teach on any given day can be set aside as students and teachers consider issues that arise in the school. “Problems” are not generally seen as intrusions on the curriculum but are dealt with as opportunities to make learning relevant. The students learn that school is not preparation for living later but rather learning to deal with issues and challenges now.
Eighth, there are frequent celebrations of student achievements. These take the form of student accomplishments in all areas which then culminate in exhibits, publications, performances and displays for other students, parents and the community. The climate and schedule of the building clearly manifest student learning and accomplishment.
Ninth, the faculty and staff are themselves a community of learners. Teachers and administrators design annual educational plans to develop further as people and as professionals. Such plans include team and cooperative activities to help teachers combat isolation. Professional development occurs during the workday as well as during non-school periods. It provides “opportunities to build meaningful partnerships with parents, businesses, educational and cultural institutions to create exciting new learning experiences.” (Renyi, 1996)
Tenth, the school provides a healthy, safe environment for learning. The staff is expert at deescalating rather than escalating student behavior problems. There are few suspensions and expulsions. Every effort is made to continue student learning during a suspension period. (Hyman & Snook, (2000)
Finally, successful urban schools frequently find ways to extend the time children spend with knowledgeable, caring adults through preschool, extended day, weekend and summer school programs, often working as partners with their communities.
At the beginning of the 21st century the greatest challenge to every major urban school system is to create and replicate these effective conditions, already practiced in specific school buildings, throughout the district as a whole.
Arbanas, David (2001) “Dropout Rates by States” Milwaukee Journal Sentinel. November 11, 2001 p.1.
Ascher, Carol and Flaxman, Erwin. (1985) “Toward Excellence: An Urban Response to the Recommendations of School Reform” ERIC/CUE Trends and Issues Series 2:7-14
Bracey, Gerald W. (2002) Put to the Test: An Educator’s and Consumer’s Guide to Standardized Tests. Phi Delta Kappan, Bloomington, IN
Breggin, Peter R. and Breggin, Ginger R. (1994) The War Against Children. St. Martin’s Press, New York: p 49
Cohen, Michael (2001) Transforming the American High School. The Aspen Institute, Washington, D.C.: p. 1-3
Council of the Great City Schools (2000) “Urban School Superintendents: Characteristics, Tenure and Salary. Second Biennial Survey” URBAN INDICATOR v52n2: p.39-51
Crosby, Emeral A. (1999) “Urban Schools: Forced to Fail.” Kappan v81n4: p. 298
Cuban, Larry (2001) “How Systemic Reform Harms Urban Schools.” Education Week. May 30, 2001 p. 48
D’Amico. Joseph J. and Corcoran, Thomas B. (1985) The Impact of Tests and Promotion Standards on Urban Schools and Students. Position Paper #6. Research for Better Schools, Inc. Philadelphia, PA
Education Commission of the States (2000) In Pursuit of Quality Teaching: Five Key Strategies for Policymakers. Denver, CO: p. 6
Education Commission of the States (1997) Redesigning the Urban School District.Denver, CO: p. 3
Feistritzer, Emily (1993) Report Card on American Education: A State by State Analysis. National Center on Education Information. Washington. D.C.: p. 34
Haberman, Martin (1991) “The Pedagogy of Poverty Versus good Teaching”
KAPPAN.73(4): p. 290-294
Haberman, Martin (1995) Star Teachers of Children in Poverty. Kappa Delta Pi. West Lafayette, IN
Haberman, Martin (2001) The Leadership Functions of Star Principals Serving Children in Poverty. The Haberman Educational Foundation, Houston, TX
Hill, Paul (1999) “Getting It Right the Eighth Time” in Marci Kanstoroom and Chester E. Finn Jr. (eds.) New Directions. Thomas B. Fordham Foundation, Washington, D.C.: p.132
Hunt Jr., James B. (1996) What Matters Most: Teachers for America’s Future. National Commission on Teaching and America’s Future, Woodbridge, VA: p.3
Hyman, Irwin A. and Snook, Pamela A. (2000) “Dangerous Schools and What you Can Do About Them” Kappan v81n7: p. 489
Kimball, Kathy & Sirotnik, Kenneth A. (2000) “The Urban School Principal: Take This Job and…” EDUCATION AND URBAN SOCIETY. v32n4: p. 535-543
Knott, Jack H. and Miller, Gary J. (1987) Reforming Bureaucracy: The Politics of Institutional Choice, Prentice Hall, Englewood Cliffs, NJ: p. 116
Langdon, Carol A. and Vesper, Nick (2000) “The Sixth Phi Delta Kappan Poll of Teachers’ Attitudes Toward the Public Schools.” Kappan v81n8: p. 607
Linn, Robert L. and Herman, Joan L (1997) A Policymaker’s Guide to Standards-Led Assessment, Education Commission of the States. Denver, CO: p. iv-v
Loeb, Paul R. (1999) Soul of a Citizen: Living with Conviction in a Cynical Time.
St. Martin’s Griffin. New York: p. 87-88
Malone, Dumas (1948) Jefferson and His Time Little, Brown and Co. Boston: p.104
McClafferty, Karen A., Torres, Carlos A. & Mitchell, Theodore R., (eds.) (2000)
CHALLENGES OF URBAN EDUCATION: SOCIOLOGICAL PERSPECTIVES FOR THE NEXT CENTURY. State University of New York Press. Albany, NY
Meyer, Adolphe E. (1957) An Educational History of the American People.
McGraw-Hill, New York: p. 245
Mitchell, Lourdes Z. (2000) “A Place Where Every Teacher Teaches and Every Student Learns” EDUCATION AND URBAN SOCIETY v32n4: p.506-518
Olson, Lynn (2000) “2000 & Beyond: The Changing Face of American Schools”
Education Week. September 27, 2000: p. 31-41
Ortiz, Flora I. (1991) Superintendent Leadership in Urban Schools. Paper presented at the Annual Meeting of the American Educational Research Association. Chicago, IL, April 3-7, 1991
Reid, Karen S. (2002) “City Schools Feel the Pain of Fiscal Bites” Education Week. January 23, 2002: p. 410
Renyi, Judith (1996) Teachers Take Charge of Their Learning, The National Foundation for the Improvement of Education, Washington, D.C.: p. 18
Ross, Randy (1994) Effective Teacher Development Through Salary Incentives, Rand Corporation. Santa Monica, CA: p.17
Russell, Avery (ed) (1986) “The Urban School Principal: The Rocky Road to Instructional Leadership” CARNEGIE QUARTERLY v31n1. Carnegie Corporation. New York: p.49-68
Schug, Mark and Western, Richard D. (1997) “Deregulating Teacher Training”
Wisconsin Policy Research Institute. Madison 10(4): p. 1-3
Schuttloffel, Merylann J. (2000) “Social Reconstruction of School Failure”
EDUCATION POLICY ANALYSIS ARCHIVES v8n45: p. 157-171
Sprinthall, Norman A., Rieman, A.J. and Tries-Sprinthall, Lois (1996) “Teacher Professional Development” in J. Sikula (ed.) Handbook for Research on Teacher Education. Rand McNally, New York: Chapter 29 p. 666
Smith, Bunny Othanial in collaboration with Stuart H. Silverman, Jean M. Borg and Betty V. Fry (1979) A Briefing for a College of Pedagogy, University of South Florida. Tampa, FL
Taylor, Barbara O. (2002) “Effective Schools Process: Alive and Well” KAPPAN v83n5. January, 2000: p. 377
Thomas B. Fordham Foundation (1999) The Teachers We Need and How to Get More of Them: A Manifesto p. 1
Tuneberg, J. (1996) The State’s Role in Implementing Legislative Mandates: The Urban School Superintendent’s Perspective. Paper presented at the Annual Meeting of the American Educational Research Association, New York, April 8-12, 1996
Van Dunk, Emily (1999) Encouraging Best Practices at MPS, The Public Policy Forum Milwaukee, WI: p.11
Haberman Educational Foundation https://habermanfoundation.org
What a Difference!
Sherri hart.
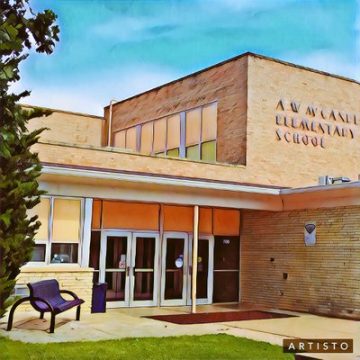
A Far Reaching Impact
Matthew capps.
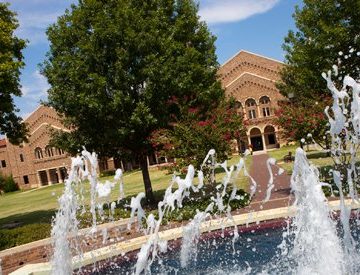
Loved the Information
Administrator.
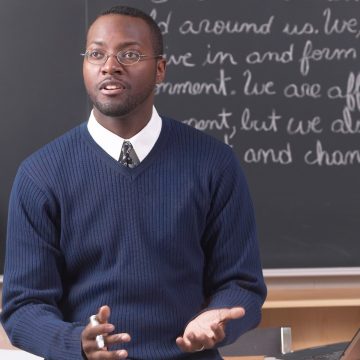
Students Deserve the Best
Dr. rodney watson.
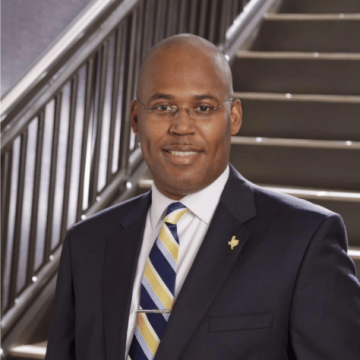
I Loved this Training!
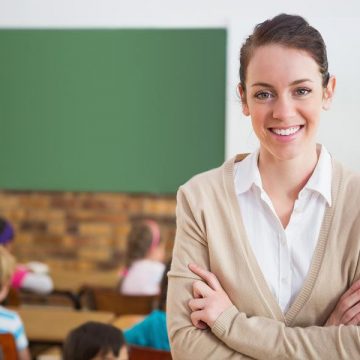
“Two years ago, our team decided to pass on an experienced applicant to instead hire a first-year teacher based on her Haberman score. Her first year however, she struggled mightily. And even though there were intense interventions put in place for her, in the spring, we discussed non-renewing her. Before we were to meet with her, our team went back and reviewed her Haberman score and answers and decided again, based on that resource, that we needed to renew her contract for a second year. What a difference! This year her class was effective and calm, and both the teacher and her students had a positive, rich experience. I am glad we trusted the Haberman interview tool – our decision to rely on it saved this teacher and helped our students and school.”
“Overall, I would rate my experience with Haberman with highest regards. I believe hiring teachers is a critical decision for schools, one that can have a far-reaching impact, both good and bad, depending on the quality of the screening process. The historical data and validity provided by Haberman provides for increased levels of confidence in making that decision.”
Loved the information and what these questions can tell me about my interview candidates.
The Haberman Foundation and theoretical framework that encompasses the work of Dr. Martin Haberman continues to be one of the most influential frameworks I believe to identify the core beliefs of teachers and administrators alike. As a previous school teacher, human resource chief and current school superintendent, I continue to rely on the Haberman Foundation to help select individuals to fill some of the most important positions in the school district. Students deserve the best possible teachers and administrators.
Where has this been? I loved this training. Presenters were friendly and very knowledgeable.
- Future Students
- Current Students
- Faculty/Staff

News and Media
- News & Media Home
- Research Stories
- School's In
- In the Media
You are here
70 years after brown v. board of education, new research shows rise in school segregation.

As the nation prepares to mark the 70th anniversary of the landmark U.S. Supreme Court ruling in Brown v. Board of Education , a new report from researchers at Stanford and USC shows that racial and economic segregation among schools has grown steadily in large school districts over the past three decades — an increase that appears to be driven in part by policies favoring school choice over integration.
Analyzing data from U.S. public schools going back to 1967, the researchers found that segregation between white and Black students has increased by 64 percent since 1988 in the 100 largest districts, and segregation by economic status has increased by about 50 percent since 1991.
The report also provides new evidence about the forces driving recent trends in school segregation, showing that the expansion of charter schools has played a major role.
The findings were released on May 6 with the launch of the Segregation Explorer , a new interactive website from the Educational Opportunity Project at Stanford University. The website provides searchable data on racial and economic school segregation in U.S. states, counties, metropolitan areas, and school districts from 1991 to 2022.
“School segregation levels are not at pre- Brown levels, but they are high and have been rising steadily since the late 1980s,” said Sean Reardon , the Professor of Poverty and Inequality in Education at Stanford Graduate School of Education and faculty director of the Educational Opportunity Project. “In most large districts, school segregation has increased while residential segregation and racial economic inequality have declined, and our findings indicate that policy choices – not demographic changes – are driving the increase.”
“There’s a tendency to attribute segregation in schools to segregation in neighborhoods,” said Ann Owens , a professor of sociology and public policy at USC. “But we’re finding that the story is more complicated than that.”
Assessing the rise
In the Brown v. Board decision issued on May 17, 1954, the U.S. Supreme Court ruled that racially segregated public schools violated the Equal Protection Clause of the Fourteenth Amendment and established that “separate but equal” schools were not only inherently unequal but unconstitutional. The ruling paved the way for future decisions that led to rapid school desegregation in many school districts in the late 1960s and early 1970s.
Though segregation in most school districts is much lower than it was 60 years ago, the researchers found that over the past three decades, both racial and economic segregation in large districts increased. Much of the increase in economic segregation since 1991, measured by segregation between students eligible and ineligible for free lunch, occurred in the last 15 years.
White-Hispanic and white-Asian segregation, while lower on average than white-Black segregation, have both more than doubled in large school districts since the 1980s.
Racial-economic segregation – specifically the difference in the proportion of free-lunch-eligible students between the average white and Black or Hispanic student’s schools – has increased by 70 percent since 1991.
School segregation is strongly associated with achievement gaps between racial and ethnic groups, especially the rate at which achievement gaps widen during school, the researchers said.
“Segregation appears to shape educational outcomes because it concentrates Black and Hispanic students in higher-poverty schools, which results in unequal learning opportunities,” said Reardon, who is also a senior fellow at the Stanford Institute for Economic Policy Research and a faculty affiliate of the Stanford Accelerator for Learning .
Policies shaping recent trends
The recent rise in school segregation appears to be the direct result of educational policy and legal decisions, the researchers said.
Both residential segregation and racial disparities in income declined between 1990 and 2020 in most large school districts. “Had nothing else changed, that trend would have led to lower school segregation,” said Owens.
But since 1991, roughly two-thirds of districts that were under court-ordered desegregation have been released from court oversight. Meanwhile, since 1998, the charter sector – a form of expanded school choice – has grown.
Expanding school choice could influence segregation levels in different ways: If families sought schools that were more diverse than the ones available in their neighborhood, it could reduce segregation. But the researchers found that in districts where the charter sector expanded most rapidly in the 2000s and 2010s, segregation grew the most.
The researchers’ analysis also quantified the extent to which the release from court orders accounted for the rise in school segregation. They found that, together, the release from court oversight and the expansion of choice accounted entirely for the rise in school segregation from 2000 to 2019.
The researchers noted enrollment policies that school districts can implement to mitigate segregation, such as voluntary integration programs, socioeconomic-based student assignment policies, and school choice policies that affirmatively promote integration.
“School segregation levels are high, troubling, and rising in large districts,” said Reardon. “These findings should sound an alarm for educators and policymakers.”
Additional collaborators on the project include Demetra Kalogrides, Thalia Tom, and Heewon Jang. This research, including the development of the Segregation Explorer data and website, was supported by the Russell Sage Foundation, the Robert Wood Johnson Foundation, and the Bill and Melinda Gates Foundation.
More Stories
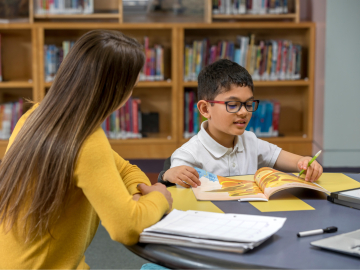
⟵ Go to all Research Stories
Get the Educator
Subscribe to our monthly newsletter.
Stanford Graduate School of Education
482 Galvez Mall Stanford, CA 94305-3096 Tel: (650) 723-2109
- Contact Admissions
- GSE Leadership
- Site Feedback
- Web Accessibility
- Career Resources
- Faculty Open Positions
- Explore Courses
- Academic Calendar
- Office of the Registrar
- Cubberley Library
- StanfordWho
- StanfordYou
Improving lives through learning

- Stanford Home
- Maps & Directions
- Search Stanford
- Emergency Info
- Terms of Use
- Non-Discrimination
- Accessibility
© Stanford University , Stanford , California 94305 .
Enrichment only for the rich? How school segregation continues to divide students by income
Seventy years after brown v. board, school funding policies continue to segregate students by wealth. it doesn't have to be that way..

Kathy Giglio’s high school memories in Bridgeport, Connecticut, include regular lockdowns and the sound of gunfire in the lobby. Classrooms lacked basic supplies and teachers didn’t notice how often she skipped class . Desks tended to be broken and textbooks decades old.
“You could tell it was very poverty-driven, with the attitudes of the students and the attitudes of the staff,” said Giglio, 34. “It was a zoo in my high school – there wasn’t a lot of learning that you were able to do because life was in the way.” Her 10-year-old daughter now attends school in the same district, and conditions haven’t improved much.
Giglio, who is Latina, appreciates living in the community she grew up in, near her parents and among other people of color working hard to give their children as many opportunities as possible. Most Bridgeport residents are Black or Latino.
She has also seen how different learning is for others in the area through her job at an after-school program in Westport, which feels like a world apart even though it’s a short drive away. The students learn on electronic whiteboards and eat in cafeterias that spill into beautiful courtyards. Kids who are struggling get one-on-one tutoring.
This week marks 70 years since Brown v. Board of Education, the landmark U.S. Supreme Court case that determined school segregation violated the Constitution. Decades later, severe divides like those Giglio has seen persist in communities around the country. Although they have received a historic infusion of relief funding since COVID-19 capsized learning, many schools are struggling to help kids catch up – especially low-income ones. School funding has remained uneven, which means the disparities could worsen once relief dollars expire later this year.
Data shared exclusively with USA TODAY shows that even within the same metropolitan area, school districts in wealthy communities often get more dollars per pupil than lower-income districts. In many regions, state funding policies designed to offset these disparities don’t make much of a dent, according to the analysis by the think tank Bellwether. Bellwether's research found some of the greatest funding disparities in blue states like Connecticut. Some of the most equitably funded metro areas are in red states such as Texas.
The findings provide a window into the insidious forces that continue to segregate children – decades after the high court ruled it was unfair to deny a Black girl access to a better-resourced school blocks from her Kansas home. In 2024, students are still sorted into school systems that reinforce rather than level out inequities.
"It's been a long road since Brown v. Board," said Alex Spurrier, an associate partner with Bellwether who helped lead the research.
"A lot of progress has been made on many fronts," he said, "but looking at the way we resource our schools right now, it's very clear there's still a lot of progress to be made."
The communities are side by side. They have wildly different education outcomes – by design.
The reality after Brown v. Board: Same urban area, starkly different worlds
Bellwether, a research nonprofit that focuses on educational equity, examined 123 metro areas across 38 states. Low-income districts tend to get the most dollars per student in just a third of those metro areas. In 40% of the metro areas, wealthier districts get the most funding. A little more than half of the students in the study live in a metro area where the wealthiest communities get the most resources.
The inequities tend to happen in metro areas divided into tiny school districts that draw in vastly different tax revenue. Schools in affluent neighborhoods rake in so much money from taxes on expensive homes that it dwarfs the amount invested in poorer schools through progressive state funding formulas meant to balance the resources.
“If you have a metro area that only has a handful of school districts, that helps to smooth out those differences in the levels of wealth,” Spurrier said. “The more boundaries and borders you have within a metro area, the more there will be these inequities in overall funding.”
Nationally, local property taxes comprise about 37% of public schools' total revenue. That percentage is often higher in wealthy areas. While state funding formulas usually seek to address the gaps, “it’s just not enough to overcome these vast differences in local revenue,” Spurrier said.
For example, in the greater Columbus, Ohio, area, affluent districts raise $13,477 per pupil on average, compared with $9,129 per pupil in lower-income districts like Columbus proper. Even with state dollars, the suburbs maintain a $1,333 advantage.
In the San Francisco Bay Area, elite districts such as Piedmont raise more than twice as much as poorer ones – and despite state interventions, they still eke out a $2,379 per-pupil advantage. Similar phenomena are seen in Philadelphia and its surrounding districts.
See which wealth class in your metro area receives the most funding:
Inequities have become ‘normalized’.
Bridgeport is another metro area where funding differences result in vastly different educational experiences for poor and wealthy kids. The city’s local tax rate is more than four times higher than the rate in Greenwich, but the affluent suburb can still produce more per-pupil funding because of property values.
Wealthy districts in the Bridgeport area, such as Greenwich, raise an average of $24,922 per pupil in local revenue. That’s $18,325 more than Bridgeport and Danbury, another poor city nearby. The additional $6,763 these low-income districts receive from the state only goes so far: Even after that funding, the elite districts still generate $11,548 more in per-pupil dollars.
According to separate research by the think tank New America, Connecticut is home to many of the country’s most unequal sets of neighboring school districts. The report compared economic circumstances in districts that sit next to each other. In Connecticut, acute differences in wealth and funding between districts almost always correlate with acute differences in racial composition.
Steven Hernández, the executive director of a local advocacy organization called ConnCAN, said these inequities are rooted in Connecticut’s past: It is a provincial state whose borders were drawn based on centuries-old religious, economic, racial and ethnic divides. Some of those drastic divides are relics of former eras, but they reverberate for present-day students.
“Each town considers itself different from the others in meaningful ways,” he said. But “these days, what you see more than anything is the divide between the haves and have-nots.”
In conversations with USA TODAY, more than a dozen Bridgeport parents and students highlighted the lack of resources.
Chantal Almonte, a parent who immigrated from the Dominican Republic, explained in Spanish that while she’s generally happy with her children’s teachers, the school facilities are abysmal. During a heat wave last year, her son was sent home early several times with headaches because his school didn’t have air conditioning.
Francisca Gabriel García, a Bridgeport resident who grew up in Mexico, cares for her niece and two children. Visiting different campuses, she has seen how unequal the funding is. Before she was accepted into her daughters’ magnet program, Gabriel García’s niece often said she was afraid to attend her former school because she worried the building would collapse. The school routinely closed for days at a time because the classrooms couldn’t operate in the heat.
The magnet program also has issues. Every Monday, the school sends home a packet of homework and activities for the week. Almost always, Gabriel García said, it comes with a note requesting the students bring basic supplies – paper towels, crayons, disinfecting spray.
“I don’t understand – my question has always been, why? Why are towns like Fairfield, Trumbull reputed to have a much better system if they are also public schools, if we also pay taxes?” Gabriel García, who volunteers as a community advocate , said in Spanish. “I am afraid that in the future – when my daughters go to high school – they will not be competitive girls.”
Giglio has considered moving to another district so her daughter, who was recently diagnosed with ADHD, could get tutoring and other necessary support. But doing so has felt nearly impossible, she said. She tried to take advantage of a program that allows select students to attend schools in other districts, but her daughter didn’t get in.
“I don’t work in a field that pays well enough” to move, Giglio said, remarking on the high cost of homes. According to Realtor.com, the median sold home price in Westport, where Giglio works, is $2.3 million.
The not-having has become “normalized,” said Tracey Elizabeth Garcia, a 15-year-old freshman in Bridgeport. One of her earliest school memories is of a teacher cautioning her and her classmates to share and conserve their gluesticks. As a freshman in high school, the lack of resources is stymying her ability to prepare for college – her school doesn’t have enough counselors.
School district lines are ‘arbitrary’
The landmark Brown v. Board of Education ruling focused on the segregation of students by race – it didn’t tackle the more subtle dynamic of uneven school funding . However, recent studies show that funding disparities break down along racial lines. A 2018 report found that school districts with the greatest number of students of color received $1,800 – or 13% – less than those with the fewest.
To address these seemingly intractable disparities, Bellwether estimates that states would need to increase allocations to poorer districts in areas with gaps by more than 20% on average. But such vast funding increases aren’t realistic, Spurrier said. Instead, he and his colleagues propose limits on how much districts can pull from local tax revenue or policies that allow the revenue to be spread more evenly across a metro area. Another solution could be consolidating districts.
“School district lines are an arbitrary thing,” said Preston Green, a professor of educational leadership and law at the University of Connecticut who studies school funding and segregation. “Other than the politics, there’s nothing that prevents us from redrawing school district lines.”
The politics are a major barrier, parents said. As one mother, Elizabeth Robinson, put it when asked about district consolidation: “I think as a resident of Bridgeport, it’s is a great idea, but if I was a resident of Southport? Absolutely not.” The median sold home price in Southport is $1.2 million.
Connecticut is known as “the Land of Steady Habits,” said Hernández, from ConnCAN. And in an educational context, habits have become a curse. “There is this quiet complicity between the haves and have-nots about the way things are. … The people who live in poverty will always live in poverty.” Decision-makers might never say that explicitly, “but that’s how they budget.”
Mixed messages: Parents think their kids are doing well in school. More often than not, they're wrong.
This persistent inequity forces families that can do so to vote with their feet. Some Bridgeport families USA TODAY interviewed said they had been forced to attend or send their children to schools elsewhere to avoid the poor conditions of city campuses.
Jackson Thomas, who now attends a Trumbull private school thanks to tuition help, noted how much safer and higher-quality his education is now – he went from struggling with failing grades to earning A’s and B’s and playing on the football team. Arantza Victoria Gonzalez Cardenas, who goes to school in Milford, said her learning now feels more organized, relaxed and connected. Everything is different, she said.
More: Supreme Court allows Louisiana's congressional map with new, mostly Black district
Several mothers who have participated in a local parent leadership institute told USA TODAY they would invest in the Bridgeport system if the system invested in them.
“Being in Bridgeport, in order for your child to really succeed, you have to go into a private school setting,” said Arlene Harris-Webber, whose son was denied special-needs services in Bridgeport schools. She later discovered her son tested in the top percentile. “If his IQ is so high, why is he failing?”
Graphics: Javier Zarracina , USA TODAY
Contact Alia Wong at (202) 507-2256 or [email protected]. Follow her on X at @aliaemily.
- Open access
- Published: 14 May 2024
Developing a survey to measure nursing students’ knowledge, attitudes and beliefs, influences, and willingness to be involved in Medical Assistance in Dying (MAiD): a mixed method modified e-Delphi study
- Jocelyn Schroeder 1 ,
- Barbara Pesut 1 , 2 ,
- Lise Olsen 2 ,
- Nelly D. Oelke 2 &
- Helen Sharp 2
BMC Nursing volume 23 , Article number: 326 ( 2024 ) Cite this article
161 Accesses
Metrics details
Medical Assistance in Dying (MAiD) was legalized in Canada in 2016. Canada’s legislation is the first to permit Nurse Practitioners (NP) to serve as independent MAiD assessors and providers. Registered Nurses’ (RN) also have important roles in MAiD that include MAiD care coordination; client and family teaching and support, MAiD procedural quality; healthcare provider and public education; and bereavement care for family. Nurses have a right under the law to conscientious objection to participating in MAiD. Therefore, it is essential to prepare nurses in their entry-level education for the practice implications and moral complexities inherent in this practice. Knowing what nursing students think about MAiD is a critical first step. Therefore, the purpose of this study was to develop a survey to measure nursing students’ knowledge, attitudes and beliefs, influences, and willingness to be involved in MAiD in the Canadian context.
The design was a mixed-method, modified e-Delphi method that entailed item generation from the literature, item refinement through a 2 round survey of an expert faculty panel, and item validation through a cognitive focus group interview with nursing students. The settings were a University located in an urban area and a College located in a rural area in Western Canada.
During phase 1, a 56-item survey was developed from existing literature that included demographic items and items designed to measure experience with death and dying (including MAiD), education and preparation, attitudes and beliefs, influences on those beliefs, and anticipated future involvement. During phase 2, an expert faculty panel reviewed, modified, and prioritized the items yielding 51 items. During phase 3, a sample of nursing students further evaluated and modified the language in the survey to aid readability and comprehension. The final survey consists of 45 items including 4 case studies.
Systematic evaluation of knowledge-to-date coupled with stakeholder perspectives supports robust survey design. This study yielded a survey to assess nursing students’ attitudes toward MAiD in a Canadian context.
The survey is appropriate for use in education and research to measure knowledge and attitudes about MAiD among nurse trainees and can be a helpful step in preparing nursing students for entry-level practice.
Peer Review reports
Medical Assistance in Dying (MAiD) is permitted under an amendment to Canada’s Criminal Code which was passed in 2016 [ 1 ]. MAiD is defined in the legislation as both self-administered and clinician-administered medication for the purpose of causing death. In the 2016 Bill C-14 legislation one of the eligibility criteria was that an applicant for MAiD must have a reasonably foreseeable natural death although this term was not defined. It was left to the clinical judgement of MAiD assessors and providers to determine the time frame that constitutes reasonably foreseeable [ 2 ]. However, in 2021 under Bill C-7, the eligibility criteria for MAiD were changed to allow individuals with irreversible medical conditions, declining health, and suffering, but whose natural death was not reasonably foreseeable, to receive MAiD [ 3 ]. This population of MAiD applicants are referred to as Track 2 MAiD (those whose natural death is foreseeable are referred to as Track 1). Track 2 applicants are subject to additional safeguards under the 2021 C-7 legislation.
Three additional proposed changes to the legislation have been extensively studied by Canadian Expert Panels (Council of Canadian Academics [CCA]) [ 4 , 5 , 6 ] First, under the legislation that defines Track 2, individuals with mental disease as their sole underlying medical condition may apply for MAiD, but implementation of this practice is embargoed until March 2027 [ 4 ]. Second, there is consideration of allowing MAiD to be implemented through advanced consent. This would make it possible for persons living with dementia to receive MAID after they have lost the capacity to consent to the procedure [ 5 ]. Third, there is consideration of extending MAiD to mature minors. A mature minor is defined as “a person under the age of majority…and who has the capacity to understand and appreciate the nature and consequences of a decision” ([ 6 ] p. 5). In summary, since the legalization of MAiD in 2016 the eligibility criteria and safeguards have evolved significantly with consequent implications for nurses and nursing care. Further, the number of Canadians who access MAiD shows steady increases since 2016 [ 7 ] and it is expected that these increases will continue in the foreseeable future.
Nurses have been integral to MAiD care in the Canadian context. While other countries such as Belgium and the Netherlands also permit euthanasia, Canada is the first country to allow Nurse Practitioners (Registered Nurses with additional preparation typically achieved at the graduate level) to act independently as assessors and providers of MAiD [ 1 ]. Although the role of Registered Nurses (RNs) in MAiD is not defined in federal legislation, it has been addressed at the provincial/territorial-level with variability in scope of practice by region [ 8 , 9 ]. For example, there are differences with respect to the obligation of the nurse to provide information to patients about MAiD, and to the degree that nurses are expected to ensure that patient eligibility criteria and safeguards are met prior to their participation [ 10 ]. Studies conducted in the Canadian context indicate that RNs perform essential roles in MAiD care coordination; client and family teaching and support; MAiD procedural quality; healthcare provider and public education; and bereavement care for family [ 9 , 11 ]. Nurse practitioners and RNs are integral to a robust MAiD care system in Canada and hence need to be well-prepared for their role [ 12 ].
Previous studies have found that end of life care, and MAiD specifically, raise complex moral and ethical issues for nurses [ 13 , 14 , 15 , 16 ]. The knowledge, attitudes, and beliefs of nurses are important across practice settings because nurses have consistent, ongoing, and direct contact with patients who experience chronic or life-limiting health conditions. Canadian studies exploring nurses’ moral and ethical decision-making in relation to MAiD reveal that although some nurses are clear in their support for, or opposition to, MAiD, others are unclear on what they believe to be good and right [ 14 ]. Empirical findings suggest that nurses go through a period of moral sense-making that is often informed by their family, peers, and initial experiences with MAID [ 17 , 18 ]. Canadian legislation and policy specifies that nurses are not required to participate in MAiD and may recuse themselves as conscientious objectors with appropriate steps to ensure ongoing and safe care of patients [ 1 , 19 ]. However, with so many nurses having to reflect on and make sense of their moral position, it is essential that they are given adequate time and preparation to make an informed and thoughtful decision before they participate in a MAID death [ 20 , 21 ].
It is well established that nursing students receive inconsistent exposure to end of life care issues [ 22 ] and little or no training related to MAiD [ 23 ]. Without such education and reflection time in pre-entry nursing preparation, nurses are at significant risk for moral harm. An important first step in providing this preparation is to be able to assess the knowledge, values, and beliefs of nursing students regarding MAID and end of life care. As demand for MAiD increases along with the complexities of MAiD, it is critical to understand the knowledge, attitudes, and likelihood of engagement with MAiD among nursing students as a baseline upon which to build curriculum and as a means to track these variables over time.
Aim, design, and setting
The aim of this study was to develop a survey to measure nursing students’ knowledge, attitudes and beliefs, influences, and willingness to be involved in MAiD in the Canadian context. We sought to explore both their willingness to be involved in the registered nursing role and in the nurse practitioner role should they chose to prepare themselves to that level of education. The design was a mixed-method, modified e-Delphi method that entailed item generation, item refinement through an expert faculty panel [ 24 , 25 , 26 ], and initial item validation through a cognitive focus group interview with nursing students [ 27 ]. The settings were a University located in an urban area and a College located in a rural area in Western Canada.
Participants
A panel of 10 faculty from the two nursing education programs were recruited for Phase 2 of the e-Delphi. To be included, faculty were required to have a minimum of three years of experience in nurse education, be employed as nursing faculty, and self-identify as having experience with MAiD. A convenience sample of 5 fourth-year nursing students were recruited to participate in Phase 3. Students had to be in good standing in the nursing program and be willing to share their experiences of the survey in an online group interview format.
The modified e-Delphi was conducted in 3 phases: Phase 1 entailed item generation through literature and existing survey review. Phase 2 entailed item refinement through a faculty expert panel review with focus on content validity, prioritization, and revision of item wording [ 25 ]. Phase 3 entailed an assessment of face validity through focus group-based cognitive interview with nursing students.
Phase I. Item generation through literature review
The goal of phase 1 was to develop a bank of survey items that would represent the variables of interest and which could be provided to expert faculty in Phase 2. Initial survey items were generated through a literature review of similar surveys designed to assess knowledge and attitudes toward MAiD/euthanasia in healthcare providers; Canadian empirical studies on nurses’ roles and/or experiences with MAiD; and legislative and expert panel documents that outlined proposed changes to the legislative eligibility criteria and safeguards. The literature review was conducted in three online databases: CINAHL, PsycINFO, and Medline. Key words for the search included nurses , nursing students , medical students , NPs, MAiD , euthanasia , assisted death , and end-of-life care . Only articles written in English were reviewed. The legalization and legislation of MAiD is new in many countries; therefore, studies that were greater than twenty years old were excluded, no further exclusion criteria set for country.
Items from surveys designed to measure similar variables in other health care providers and geographic contexts were placed in a table and similar items were collated and revised into a single item. Then key variables were identified from the empirical literature on nurses and MAiD in Canada and checked against the items derived from the surveys to ensure that each of the key variables were represented. For example, conscientious objection has figured prominently in the Canadian literature, but there were few items that assessed knowledge of conscientious objection in other surveys and so items were added [ 15 , 21 , 28 , 29 ]. Finally, four case studies were added to the survey to address the anticipated changes to the Canadian legislation. The case studies were based upon the inclusion of mature minors, advanced consent, and mental disorder as the sole underlying medical condition. The intention was to assess nurses’ beliefs and comfort with these potential legislative changes.
Phase 2. Item refinement through expert panel review
The goal of phase 2 was to refine and prioritize the proposed survey items identified in phase 1 using a modified e-Delphi approach to achieve consensus among an expert panel [ 26 ]. Items from phase 1 were presented to an expert faculty panel using a Qualtrics (Provo, UT) online survey. Panel members were asked to review each item to determine if it should be: included, excluded or adapted for the survey. When adapted was selected faculty experts were asked to provide rationale and suggestions for adaptation through the use of an open text box. Items that reached a level of 75% consensus for either inclusion or adaptation were retained [ 25 , 26 ]. New items were categorized and added, and a revised survey was presented to the panel of experts in round 2. Panel members were again asked to review items, including new items, to determine if it should be: included, excluded, or adapted for the survey. Round 2 of the modified e-Delphi approach also included an item prioritization activity, where participants were then asked to rate the importance of each item, based on a 5-point Likert scale (low to high importance), which De Vaus [ 30 ] states is helpful for increasing the reliability of responses. Items that reached a 75% consensus on inclusion were then considered in relation to the importance it was given by the expert panel. Quantitative data were managed using SPSS (IBM Corp).
Phase 3. Face validity through cognitive interviews with nursing students
The goal of phase 3 was to obtain initial face validity of the proposed survey using a sample of nursing student informants. More specifically, student participants were asked to discuss how items were interpreted, to identify confusing wording or other problematic construction of items, and to provide feedback about the survey as a whole including readability and organization [ 31 , 32 , 33 ]. The focus group was held online and audio recorded. A semi-structured interview guide was developed for this study that focused on clarity, meaning, order and wording of questions; emotions evoked by the questions; and overall survey cohesion and length was used to obtain data (see Supplementary Material 2 for the interview guide). A prompt to “think aloud” was used to limit interviewer-imposed bias and encourage participants to describe their thoughts and response to a given item as they reviewed survey items [ 27 ]. Where needed, verbal probes such as “could you expand on that” were used to encourage participants to expand on their responses [ 27 ]. Student participants’ feedback was collated verbatim and presented to the research team where potential survey modifications were negotiated and finalized among team members. Conventional content analysis [ 34 ] of focus group data was conducted to identify key themes that emerged through discussion with students. Themes were derived from the data by grouping common responses and then using those common responses to modify survey items.
Ten nursing faculty participated in the expert panel. Eight of the 10 faculty self-identified as female. No faculty panel members reported conscientious objector status and ninety percent reported general agreement with MAiD with one respondent who indicated their view as “unsure.” Six of the 10 faculty experts had 16 years of experience or more working as a nurse educator.
Five nursing students participated in the cognitive interview focus group. The duration of the focus group was 2.5 h. All participants identified that they were born in Canada, self-identified as female (one preferred not to say) and reported having received some instruction about MAiD as part of their nursing curriculum. See Tables 1 and 2 for the demographic descriptors of the study sample. Study results will be reported in accordance with the study phases. See Fig. 1 for an overview of the results from each phase.

Fig. 1 Overview of survey development findings
Phase 1: survey item generation
Review of the literature identified that no existing survey was available for use with nursing students in the Canadian context. However, an analysis of themes across qualitative and quantitative studies of physicians, medical students, nurses, and nursing students provided sufficient data to develop a preliminary set of items suitable for adaptation to a population of nursing students.
Four major themes and factors that influence knowledge, attitudes, and beliefs about MAiD were evident from the literature: (i) endogenous or individual factors such as age, gender, personally held values, religion, religiosity, and/or spirituality [ 35 , 36 , 37 , 38 , 39 , 40 , 41 , 42 ], (ii) experience with death and dying in personal and/or professional life [ 35 , 40 , 41 , 43 , 44 , 45 ], (iii) training including curricular instruction about clinical role, scope of practice, or the law [ 23 , 36 , 39 ], and (iv) exogenous or social factors such as the influence of key leaders, colleagues, friends and/or family, professional and licensure organizations, support within professional settings, and/or engagement in MAiD in an interdisciplinary team context [ 9 , 35 , 46 ].
Studies of nursing students also suggest overlap across these categories. For example, value for patient autonomy [ 23 ] and the moral complexity of decision-making [ 37 ] are important factors that contribute to attitudes about MAiD and may stem from a blend of personally held values coupled with curricular content, professional training and norms, and clinical exposure. For example, students report that participation in end of life care allows for personal growth, shifts in perception, and opportunities to build therapeutic relationships with their clients [ 44 , 47 , 48 ].
Preliminary items generated from the literature resulted in 56 questions from 11 published sources (See Table 3 ). These items were constructed across four main categories: (i) socio-demographic questions; (ii) end of life care questions; (iii) knowledge about MAiD; or (iv) comfort and willingness to participate in MAiD. Knowledge questions were refined to reflect current MAiD legislation, policies, and regulatory frameworks. Falconer [ 39 ] and Freeman [ 45 ] studies were foundational sources for item selection. Additionally, four case studies were written to reflect the most recent anticipated changes to MAiD legislation and all used the same open-ended core questions to address respondents’ perspectives about the patient’s right to make the decision, comfort in assisting a physician or NP to administer MAiD in that scenario, and hypothesized comfort about serving as a primary provider if qualified as an NP in future. Response options for the survey were also constructed during this stage and included: open text, categorical, yes/no , and Likert scales.
Phase 2: faculty expert panel review
Of the 56 items presented to the faculty panel, 54 questions reached 75% consensus. However, based upon the qualitative responses 9 items were removed largely because they were felt to be repetitive. Items that generated the most controversy were related to measuring religion and spirituality in the Canadian context, defining end of life care when there is no agreed upon time frames (e.g., last days, months, or years), and predicting willingness to be involved in a future events – thus predicting their future selves. Phase 2, round 1 resulted in an initial set of 47 items which were then presented back to the faculty panel in round 2.
Of the 47 initial questions presented to the panel in round 2, 45 reached a level of consensus of 75% or greater, and 34 of these questions reached a level of 100% consensus [ 27 ] of which all participants chose to include without any adaptations) For each question, level of importance was determined based on a 5-point Likert scale (1 = very unimportant, 2 = somewhat unimportant, 3 = neutral, 4 = somewhat important, and 5 = very important). Figure 2 provides an overview of the level of importance assigned to each item.
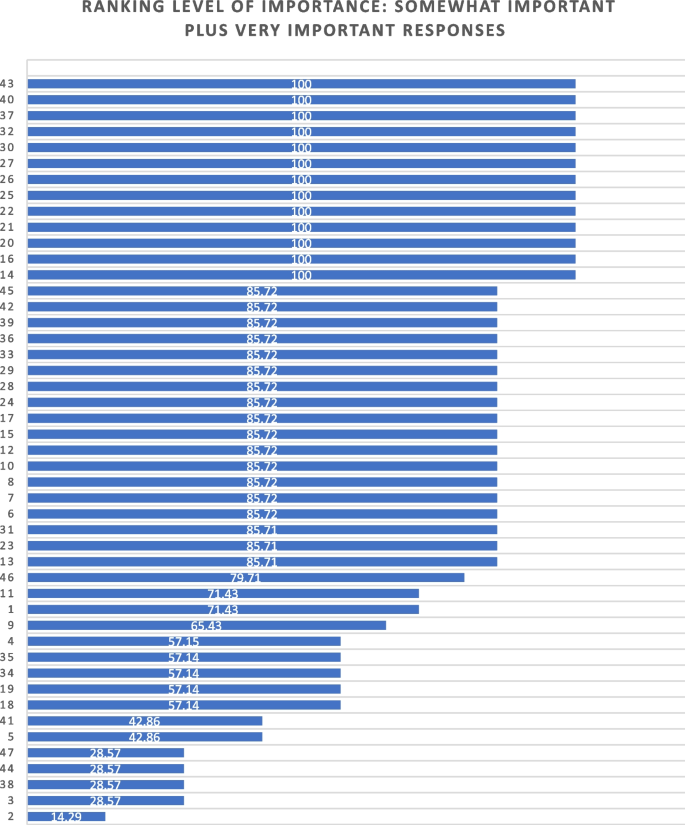
Ranking level of importance for survey items
After round 2, a careful analysis of participant comments and level of importance was completed by the research team. While the main method of survey item development came from participants’ response to the first round of Delphi consensus ratings, level of importance was used to assist in the decision of whether to keep or modify questions that created controversy, or that rated lower in the include/exclude/adapt portion of the Delphi. Survey items that rated low in level of importance included questions about future roles, sex and gender, and religion/spirituality. After deliberation by the research committee, these questions were retained in the survey based upon the importance of these variables in the scientific literature.
Of the 47 questions remaining from Phase 2, round 2, four were revised. In addition, the two questions that did not meet the 75% cut off level for consensus were reviewed by the research team. The first question reviewed was What is your comfort level with providing a MAiD death in the future if you were a qualified NP ? Based on a review of participant comments, it was decided to retain this question for the cognitive interviews with students in the final phase of testing. The second question asked about impacts on respondents’ views of MAiD and was changed from one item with 4 subcategories into 4 separate items, resulting in a final total of 51 items for phase 3. The revised survey was then brought forward to the cognitive interviews with student participants in Phase 3. (see Supplementary Material 1 for a complete description of item modification during round 2).
Phase 3. Outcomes of cognitive interview focus group
Of the 51 items reviewed by student participants, 29 were identified as clear with little or no discussion. Participant comments for the remaining 22 questions were noted and verified against the audio recording. Following content analysis of the comments, four key themes emerged through the student discussion: unclear or ambiguous wording; difficult to answer questions; need for additional response options; and emotional response evoked by questions. An example of unclear or ambiguous wording was a request for clarity in the use of the word “sufficient” in the context of assessing an item that read “My nursing education has provided sufficient content about the nursing role in MAiD.” “Sufficient” was viewed as subjective and “laden with…complexity that distracted me from the question.” The group recommended rewording the item to read “My nursing education has provided enough content for me to care for a patient considering or requesting MAiD.”
An example of having difficulty answering questions related to limited knowledge related to terms used in the legislation such as such as safeguards , mature minor , eligibility criteria , and conscientious objection. Students were unclear about what these words meant relative to the legislation and indicated that this lack of clarity would hamper appropriate responses to the survey. To ensure that respondents are able to answer relevant questions, student participants recommended that the final survey include explanation of key terms such as mature minor and conscientious objection and an overview of current legislation.
Response options were also a point of discussion. Participants noted a lack of distinction between response options of unsure and unable to say . Additionally, scaling of attitudes was noted as important since perspectives about MAiD are dynamic and not dichotomous “agree or disagree” responses. Although the faculty expert panel recommended the integration of the demographic variables of religious and/or spiritual remain as a single item, the student group stated a preference to have religion and spirituality appear as separate items. The student focus group also took issue with separate items for the variables of sex and gender, specifically that non-binary respondents might feel othered or “outed” particularly when asked to identify their sex. These variables had been created based upon best practices in health research but students did not feel they were appropriate in this context [ 49 ]. Finally, students agreed with the faculty expert panel in terms of the complexity of projecting their future involvement as a Nurse Practitioner. One participant stated: “I certainly had to like, whoa, whoa, whoa. Now let me finish this degree first, please.” Another stated, “I'm still imagining myself, my future career as an RN.”
Finally, student participants acknowledged the array of emotions that some of the items produced for them. For example, one student described positive feelings when interacting with the survey. “Brought me a little bit of feeling of joy. Like it reminded me that this is the last piece of independence that people grab on to.” Another participant, described the freedom that the idea of an advance request gave her. “The advance request gives the most comfort for me, just with early onset Alzheimer’s and knowing what it can do.” But other participants described less positive feelings. For example, the mature minor case study yielded a comment: “This whole scenario just made my heart hurt with the idea of a child requesting that.”
Based on the data gathered from the cognitive interview focus group of nursing students, revisions were made to 11 closed-ended questions (see Table 4 ) and 3 items were excluded. In the four case studies, the open-ended question related to a respondents’ hypothesized actions in a future role as NP were removed. The final survey consists of 45 items including 4 case studies (see Supplementary Material 3 ).
The aim of this study was to develop and validate a survey that can be used to track the growth of knowledge about MAiD among nursing students over time, inform training programs about curricular needs, and evaluate attitudes and willingness to participate in MAiD at time-points during training or across nursing programs over time.
The faculty expert panel and student participants in the cognitive interview focus group identified a need to establish core knowledge of the terminology and legislative rules related to MAiD. For example, within the cognitive interview group of student participants, several acknowledged lack of clear understanding of specific terms such as “conscientious objector” and “safeguards.” Participants acknowledged discomfort with the uncertainty of not knowing and their inclination to look up these terms to assist with answering the questions. This survey can be administered to nursing or pre-nursing students at any phase of their training within a program or across training programs. However, in doing so it is important to acknowledge that their baseline knowledge of MAiD will vary. A response option of “not sure” is important and provides a means for respondents to convey uncertainty. If this survey is used to inform curricular needs, respondents should be given explicit instructions not to conduct online searches to inform their responses, but rather to provide an honest appraisal of their current knowledge and these instructions are included in the survey (see Supplementary Material 3 ).
Some provincial regulatory bodies have established core competencies for entry-level nurses that include MAiD. For example, the BC College of Nurses and Midwives (BCCNM) requires “knowledge about ethical, legal, and regulatory implications of medical assistance in dying (MAiD) when providing nursing care.” (10 p. 6) However, across Canada curricular content and coverage related to end of life care and MAiD is variable [ 23 ]. Given the dynamic nature of the legislation that includes portions of the law that are embargoed until 2024, it is important to ensure that respondents are guided by current and accurate information. As the law changes, nursing curricula, and public attitudes continue to evolve, inclusion of core knowledge and content is essential and relevant for investigators to be able to interpret the portions of the survey focused on attitudes and beliefs about MAiD. Content knowledge portions of the survey may need to be modified over time as legislation and training change and to meet the specific purposes of the investigator.
Given the sensitive nature of the topic, it is strongly recommended that surveys be conducted anonymously and that students be provided with an opportunity to discuss their responses to the survey. A majority of feedback from both the expert panel of faculty and from student participants related to the wording and inclusion of demographic variables, in particular religion, religiosity, gender identity, and sex assigned at birth. These and other demographic variables have the potential to be highly identifying in small samples. In any instance in which the survey could be expected to yield demographic group sizes less than 5, users should eliminate the demographic variables from the survey. For example, the profession of nursing is highly dominated by females with over 90% of nurses who identify as female [ 50 ]. Thus, a survey within a single class of students or even across classes in a single institution is likely to yield a small number of male respondents and/or respondents who report a difference between sex assigned at birth and gender identity. When variables that serve to identify respondents are included, respondents are less likely to complete or submit the survey, to obscure their responses so as not to be identifiable, or to be influenced by social desirability bias in their responses rather than to convey their attitudes accurately [ 51 ]. Further, small samples do not allow for conclusive analyses or interpretation of apparent group differences. Although these variables are often included in surveys, such demographics should be included only when anonymity can be sustained. In small and/or known samples, highly identifying variables should be omitted.
There are several limitations associated with the development of this survey. The expert panel was comprised of faculty who teach nursing students and are knowledgeable about MAiD and curricular content, however none identified as a conscientious objector to MAiD. Ideally, our expert panel would have included one or more conscientious objectors to MAiD to provide a broader perspective. Review by practitioners who participate in MAiD, those who are neutral or undecided, and practitioners who are conscientious objectors would ensure broad applicability of the survey. This study included one student cognitive interview focus group with 5 self-selected participants. All student participants had held discussions about end of life care with at least one patient, 4 of 5 participants had worked with a patient who requested MAiD, and one had been present for a MAiD death. It is not clear that these participants are representative of nursing students demographically or by experience with end of life care. It is possible that the students who elected to participate hold perspectives and reflections on patient care and MAiD that differ from students with little or no exposure to end of life care and/or MAiD. However, previous studies find that most nursing students have been involved with end of life care including meaningful discussions about patients’ preferences and care needs during their education [ 40 , 44 , 47 , 48 , 52 ]. Data collection with additional student focus groups with students early in their training and drawn from other training contexts would contribute to further validation of survey items.
Future studies should incorporate pilot testing with small sample of nursing students followed by a larger cross-program sample to allow evaluation of the psychometric properties of specific items and further refinement of the survey tool. Consistent with literature about the importance of leadership in the context of MAiD [ 12 , 53 , 54 ], a study of faculty knowledge, beliefs, and attitudes toward MAiD would provide context for understanding student perspectives within and across programs. Additional research is also needed to understand the timing and content coverage of MAiD across Canadian nurse training programs’ curricula.
The implementation of MAiD is complex and requires understanding of the perspectives of multiple stakeholders. Within the field of nursing this includes clinical providers, educators, and students who will deliver clinical care. A survey to assess nursing students’ attitudes toward and willingness to participate in MAiD in the Canadian context is timely, due to the legislation enacted in 2016 and subsequent modifications to the law in 2021 with portions of the law to be enacted in 2027. Further development of this survey could be undertaken to allow for use in settings with practicing nurses or to allow longitudinal follow up with students as they enter practice. As the Canadian landscape changes, ongoing assessment of the perspectives and needs of health professionals and students in the health professions is needed to inform policy makers, leaders in practice, curricular needs, and to monitor changes in attitudes and practice patterns over time.
Availability of data and materials
The datasets used and/or analysed during the current study are not publicly available due to small sample sizes, but are available from the corresponding author on reasonable request.
Abbreviations
British Columbia College of Nurses and Midwives
Medical assistance in dying
Nurse practitioner
Registered nurse
University of British Columbia Okanagan
Nicol J, Tiedemann M. Legislative Summary: Bill C-14: An Act to amend the Criminal Code and to make related amendments to other Acts (medical assistance in dying). Available from: https://lop.parl.ca/staticfiles/PublicWebsite/Home/ResearchPublications/LegislativeSummaries/PDF/42-1/c14-e.pdf .
Downie J, Scallion K. Foreseeably unclear. The meaning of the “reasonably foreseeable” criterion for access to medical assistance in dying in Canada. Dalhousie Law J. 2018;41(1):23–57.
Nicol J, Tiedeman M. Legislative summary of Bill C-7: an act to amend the criminal code (medical assistance in dying). Ottawa: Government of Canada; 2021.
Google Scholar
Council of Canadian Academies. The state of knowledge on medical assistance in dying where a mental disorder is the sole underlying medical condition. Ottawa; 2018. Available from: https://cca-reports.ca/wp-content/uploads/2018/12/The-State-of-Knowledge-on-Medical-Assistance-in-Dying-Where-a-Mental-Disorder-is-the-Sole-Underlying-Medical-Condition.pdf .
Council of Canadian Academies. The state of knowledge on advance requests for medical assistance in dying. Ottawa; 2018. Available from: https://cca-reports.ca/wp-content/uploads/2019/02/The-State-of-Knowledge-on-Advance-Requests-for-Medical-Assistance-in-Dying.pdf .
Council of Canadian Academies. The state of knowledge on medical assistance in dying for mature minors. Ottawa; 2018. Available from: https://cca-reports.ca/wp-content/uploads/2018/12/The-State-of-Knowledge-on-Medical-Assistance-in-Dying-for-Mature-Minors.pdf .
Health Canada. Third annual report on medical assistance in dying in Canada 2021. Ottawa; 2022. [cited 2023 Oct 23]. Available from: https://www.canada.ca/en/health-canada/services/medical-assistance-dying/annual-report-2021.html .
Banner D, Schiller CJ, Freeman S. Medical assistance in dying: a political issue for nurses and nursing in Canada. Nurs Philos. 2019;20(4): e12281.
Article PubMed Google Scholar
Pesut B, Thorne S, Stager ML, Schiller CJ, Penney C, Hoffman C, et al. Medical assistance in dying: a review of Canadian nursing regulatory documents. Policy Polit Nurs Pract. 2019;20(3):113–30.
Article PubMed PubMed Central Google Scholar
College of Registered Nurses of British Columbia. Scope of practice for registered nurses [Internet]. Vancouver; 2018. Available from: https://www.bccnm.ca/Documents/standards_practice/rn/RN_ScopeofPractice.pdf .
Pesut B, Thorne S, Schiller C, Greig M, Roussel J, Tishelman C. Constructing good nursing practice for medical assistance in dying in Canada: an interpretive descriptive study. Global Qual Nurs Res. 2020;7:2333393620938686. https://doi.org/10.1177/2333393620938686 .
Article Google Scholar
Pesut B, Thorne S, Schiller CJ, Greig M, Roussel J. The rocks and hard places of MAiD: a qualitative study of nursing practice in the context of legislated assisted death. BMC Nurs. 2020;19:12. https://doi.org/10.1186/s12912-020-0404-5 .
Pesut B, Greig M, Thorne S, Burgess M, Storch JL, Tishelman C, et al. Nursing and euthanasia: a narrative review of the nursing ethics literature. Nurs Ethics. 2020;27(1):152–67.
Pesut B, Thorne S, Storch J, Chambaere K, Greig M, Burgess M. Riding an elephant: a qualitative study of nurses’ moral journeys in the context of Medical Assistance in Dying (MAiD). Journal Clin Nurs. 2020;29(19–20):3870–81.
Lamb C, Babenko-Mould Y, Evans M, Wong CA, Kirkwood KW. Conscientious objection and nurses: results of an interpretive phenomenological study. Nurs Ethics. 2018;26(5):1337–49.
Wright DK, Chan LS, Fishman JR, Macdonald ME. “Reflection and soul searching:” Negotiating nursing identity at the fault lines of palliative care and medical assistance in dying. Social Sci & Med. 2021;289: 114366.
Beuthin R, Bruce A, Scaia M. Medical assistance in dying (MAiD): Canadian nurses’ experiences. Nurs Forum. 2018;54(4):511–20.
Bruce A, Beuthin R. Medically assisted dying in Canada: "Beautiful Death" is transforming nurses' experiences of suffering. The Canadian J Nurs Res | Revue Canadienne de Recherche en Sci Infirmieres. 2020;52(4):268–77. https://doi.org/10.1177/0844562119856234 .
Canadian Nurses Association. Code of ethics for registered nurses. Ottawa; 2017. Available from: https://www.cna-aiic.ca/en/nursing/regulated-nursing-in-canada/nursing-ethics .
Canadian Nurses Association. National nursing framework on Medical Assistance in Dying in Canada. Ottawa: 2017. Available from: https://www.virtualhospice.ca/Assets/cna-national-nursing-framework-on-maidEng_20170216155827.pdf .
Pesut B, Thorne S, Greig M. Shades of gray: conscientious objection in medical assistance in dying. Nursing Inq. 2020;27(1): e12308.
Durojaiye A, Ryan R, Doody O. Student nurse education and preparation for palliative care: a scoping review. PLoS ONE. 2023. https://doi.org/10.1371/journal.pone.0286678 .
McMechan C, Bruce A, Beuthin R. Canadian nursing students’ experiences with medical assistance in dying | Les expériences d’étudiantes en sciences infirmières au regard de l’aide médicale à mourir. Qual Adv Nurs Educ - Avancées en Formation Infirmière. 2019;5(1). https://doi.org/10.17483/2368-6669.1179 .
Adler M, Ziglio E. Gazing into the oracle. The Delphi method and its application to social policy and public health. London: Jessica Kingsley Publishers; 1996
Keeney S, Hasson F, McKenna H. Consulting the oracle: ten lessons from using the Delphi technique in nursing research. J Adv Nurs. 2006;53(2):205–12.
Keeney S, Hasson F, McKenna H. The Delphi technique in nursing and health research. 1st ed. City: Wiley; 2011.
Willis GB. Cognitive interviewing: a tool for improving questionnaire design. 1st ed. Thousand Oaks, Calif: Sage; 2005. ISBN: 9780761928041
Lamb C, Evans M, Babenko-Mould Y, Wong CA, Kirkwood EW. Conscience, conscientious objection, and nursing: a concept analysis. Nurs Ethics. 2017;26(1):37–49.
Lamb C, Evans M, Babenko-Mould Y, Wong CA, Kirkwood K. Nurses’ use of conscientious objection and the implications of conscience. J Adv Nurs. 2018;75(3):594–602.
de Vaus D. Surveys in social research. 6th ed. Abingdon, Oxon: Routledge; 2014.
Boateng GO, Neilands TB, Frongillo EA, Melgar-Quiñonez HR, Young SL. Best practices for developing and validating scales for health, social, and behavioral research: A primer. Front Public Health. 2018;6:149. https://doi.org/10.3389/fpubh.2018.00149 .
Puchta C, Potter J. Focus group practice. 1st ed. London: Sage; 2004.
Book Google Scholar
Streiner DL, Norman GR, Cairney J. Health measurement scales: a practical guide to their development and use. 5th ed. Oxford: Oxford University Press; 2015.
Hsieh H-F, Shannon SE. Three approaches to qualitative content analysis. Qual Health Res. 2005;15(9):1277–88.
Adesina O, DeBellis A, Zannettino L. Third-year Australian nursing students’ attitudes, experiences, knowledge, and education concerning end-of-life care. Int J of Palliative Nurs. 2014;20(8):395–401.
Bator EX, Philpott B, Costa AP. This moral coil: a cross-sectional survey of Canadian medical student attitudes toward medical assistance in dying. BMC Med Ethics. 2017;18(1):58.
Beuthin R, Bruce A, Scaia M. Medical assistance in dying (MAiD): Canadian nurses’ experiences. Nurs Forum. 2018;53(4):511–20.
Brown J, Goodridge D, Thorpe L, Crizzle A. What is right for me, is not necessarily right for you: the endogenous factors influencing nonparticipation in medical assistance in dying. Qual Health Res. 2021;31(10):1786–1800.
Falconer J, Couture F, Demir KK, Lang M, Shefman Z, Woo M. Perceptions and intentions toward medical assistance in dying among Canadian medical students. BMC Med Ethics. 2019;20(1):22.
Green G, Reicher S, Herman M, Raspaolo A, Spero T, Blau A. Attitudes toward euthanasia—dual view: Nursing students and nurses. Death Stud. 2022;46(1):124–31.
Hosseinzadeh K, Rafiei H. Nursing student attitudes toward euthanasia: a cross-sectional study. Nurs Ethics. 2019;26(2):496–503.
Ozcelik H, Tekir O, Samancioglu S, Fadiloglu C, Ozkara E. Nursing students’ approaches toward euthanasia. Omega (Westport). 2014;69(1):93–103.
Canning SE, Drew C. Canadian nursing students’ understanding, and comfort levels related to medical assistance in dying. Qual Adv Nurs Educ - Avancées en Formation Infirmière. 2022;8(2). https://doi.org/10.17483/2368-6669.1326 .
Edo-Gual M, Tomás-Sábado J, Bardallo-Porras D, Monforte-Royo C. The impact of death and dying on nursing students: an explanatory model. J Clin Nurs. 2014;23(23–24):3501–12.
Freeman LA, Pfaff KA, Kopchek L, Liebman J. Investigating palliative care nurse attitudes towards medical assistance in dying: an exploratory cross-sectional study. J Adv Nurs. 2020;76(2):535–45.
Brown J, Goodridge D, Thorpe L, Crizzle A. “I am okay with it, but I am not going to do it:” the exogenous factors influencing non-participation in medical assistance in dying. Qual Health Res. 2021;31(12):2274–89.
Dimoula M, Kotronoulas G, Katsaragakis S, Christou M, Sgourou S, Patiraki E. Undergraduate nursing students’ knowledge about palliative care and attitudes towards end-of-life care: A three-cohort, cross-sectional survey. Nurs Educ Today. 2019;74:7–14.
Matchim Y, Raetong P. Thai nursing students’ experiences of caring for patients at the end of life: a phenomenological study. Int J Palliative Nurs. 2018;24(5):220–9.
Canadian Institute for Health Research. Sex and gender in health research [Internet]. Ottawa: CIHR; 2021 [cited 2023 Oct 23]. Available from: https://cihr-irsc.gc.ca/e/50833.html .
Canadian Nurses’ Association. Nursing statistics. Ottawa: CNA; 2023 [cited 2023 Oct 23]. Available from: https://www.cna-aiic.ca/en/nursing/regulated-nursing-in-canada/nursing-statistics .
Krumpal I. Determinants of social desirability bias in sensitive surveys: a literature review. Qual Quant. 2013;47(4):2025–47. https://doi.org/10.1007/s11135-011-9640-9 .
Ferri P, Di Lorenzo R, Stifani S, Morotti E, Vagnini M, Jiménez Herrera MF, et al. Nursing student attitudes toward dying patient care: a European multicenter cross-sectional study. Acta Bio Medica Atenei Parmensis. 2021;92(S2): e2021018.
PubMed PubMed Central Google Scholar
Beuthin R, Bruce A. Medical assistance in dying (MAiD): Ten things leaders need to know. Nurs Leadership. 2018;31(4):74–81.
Thiele T, Dunsford J. Nurse leaders’ role in medical assistance in dying: a relational ethics approach. Nurs Ethics. 2019;26(4):993–9.
Download references
Acknowledgements
We would like to acknowledge the faculty and students who generously contributed their time to this work.
JS received a student traineeship through the Principal Research Chairs program at the University of British Columbia Okanagan.
Author information
Authors and affiliations.
School of Health and Human Services, Selkirk College, Castlegar, BC, Canada
Jocelyn Schroeder & Barbara Pesut
School of Nursing, University of British Columbia Okanagan, Kelowna, BC, Canada
Barbara Pesut, Lise Olsen, Nelly D. Oelke & Helen Sharp
You can also search for this author in PubMed Google Scholar
Contributions
JS made substantial contributions to the conception of the work; data acquisition, analysis, and interpretation; and drafting and substantively revising the work. JS has approved the submitted version and agreed to be personally accountable for the author's own contributions and to ensure that questions related to the accuracy or integrity of any part of the work, even ones in which the author was not personally involved, are appropriately investigated, resolved, and the resolution documented in the literature. BP made substantial contributions to the conception of the work; data acquisition, analysis, and interpretation; and drafting and substantively revising the work. BP has approved the submitted version and agreed to be personally accountable for the author's own contributions and to ensure that questions related to the accuracy or integrity of any part of the work, even ones in which the author was not personally involved, are appropriately investigated, resolved, and the resolution documented in the literature. LO made substantial contributions to the conception of the work; data acquisition, analysis, and interpretation; and substantively revising the work. LO has approved the submitted version and agreed to be personally accountable for the author's own contributions and to ensure that questions related to the accuracy or integrity of any part of the work, even ones in which the author was not personally involved, are appropriately investigated, resolved, and the resolution documented in the literature. NDO made substantial contributions to the conception of the work; data acquisition, analysis, and interpretation; and substantively revising the work. NDO has approved the submitted version and agreed to be personally accountable for the author's own contributions and to ensure that questions related to the accuracy or integrity of any part of the work, even ones in which the author was not personally involved, are appropriately investigated, resolved, and the resolution documented in the literature. HS made substantial contributions to drafting and substantively revising the work. HS has approved the submitted version and agreed to be personally accountable for the author's own contributions and to ensure that questions related to the accuracy or integrity of any part of the work, even ones in which the author was not personally involved, are appropriately investigated, resolved, and the resolution documented in the literature.
Authors’ information
JS conducted this study as part of their graduate requirements in the School of Nursing, University of British Columbia Okanagan.
Corresponding author
Correspondence to Barbara Pesut .
Ethics declarations
Ethics approval and consent to participate.
The research was approved by the Selkirk College Research Ethics Board (REB) ID # 2021–011 and the University of British Columbia Behavioral Research Ethics Board ID # H21-01181.
All participants provided written and informed consent through approved consent processes. Research was conducted in accordance with the Declaration of Helsinki.
Consent for publication
Not applicable.
Competing interests
The authors declare they have no competing interests.
Additional information
Publisher’s note.
Springer Nature remains neutral with regard to jurisdictional claims in published maps and institutional affiliations.
Supplementary Information
Supplementary material 1., supplementary material 2., supplementary material 3., rights and permissions.
Open Access This article is licensed under a Creative Commons Attribution 4.0 International License, which permits use, sharing, adaptation, distribution and reproduction in any medium or format, as long as you give appropriate credit to the original author(s) and the source, provide a link to the Creative Commons licence, and indicate if changes were made. The images or other third party material in this article are included in the article's Creative Commons licence, unless indicated otherwise in a credit line to the material. If material is not included in the article's Creative Commons licence and your intended use is not permitted by statutory regulation or exceeds the permitted use, you will need to obtain permission directly from the copyright holder. To view a copy of this licence, visit http://creativecommons.org/licenses/by/4.0/ . The Creative Commons Public Domain Dedication waiver ( http://creativecommons.org/publicdomain/zero/1.0/ ) applies to the data made available in this article, unless otherwise stated in a credit line to the data.
Reprints and permissions
About this article
Cite this article.
Schroeder, J., Pesut, B., Olsen, L. et al. Developing a survey to measure nursing students’ knowledge, attitudes and beliefs, influences, and willingness to be involved in Medical Assistance in Dying (MAiD): a mixed method modified e-Delphi study. BMC Nurs 23 , 326 (2024). https://doi.org/10.1186/s12912-024-01984-z
Download citation
Received : 24 October 2023
Accepted : 28 April 2024
Published : 14 May 2024
DOI : https://doi.org/10.1186/s12912-024-01984-z
Share this article
Anyone you share the following link with will be able to read this content:
Sorry, a shareable link is not currently available for this article.
Provided by the Springer Nature SharedIt content-sharing initiative
- Medical assistance in dying (MAiD)
- End of life care
- Student nurses
- Nursing education
BMC Nursing
ISSN: 1472-6955
- General enquiries: [email protected]
- Membership Membership Overview Benefits of Membership Types of Membership Renew Membership Student Programs Member Recognition Volunteer Opportunities Join Now
- Credentials Credentials Overview Benefits of Credentials Types of Credentials Digital Badges Continuing Education Exam Information ISA Ethics and Integrity FAQ Apply Now
- Online Learning Online Learning Overview Online Courses Online Quizzes Podcasts More Resources
- Publications Publications Overview Arborist News Arboriculture & Urban Forestry ISA Today ISA Today Archives Advertising Opportunities CTLA 10th Edition Comment Period
- Research Research Overview ISA's Research Mission ISA's Research Network Arboriculture and Urban Forestry Funding Sources Research Resources Donate to Research
- Events Events Overview Annual Conference Climbing Competitions Scientific Symposia Events Calendar
- Who We Are Who We Are Overview Our Organization Our Leaders Our Services Our Network
- Careers Careers Overview Work at ISA Careers in Arboriculture Academic Programs Career Center
- For the Public
Email us Phone: +1.678.367.0981 Fax: +1.240.547.1795
Headquarters Address
International Society of Arboriculture 270 Peachtree Street NW Suite 1900 Atlanta, GA 30303 United States
Mailing Address
International Society of Arboriculture PO Box 191 Annapolis Junction, MD 20701 United States
Conference Rewind 2024 is Here With All New Presentations
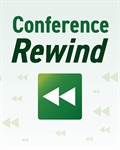
May 17, 2024 Categories: Industry News , ISA News
Learn How to Better Assess Tree Risk with this BMP
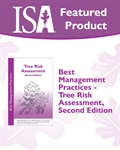
May 7, 2024 Categories: Industry News , ISA News
May 2024 Issue of Arboriculture & Urban Forestry Now Online!
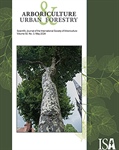
May 1, 2024 Categories: Industry News , ISA News
ISA Board of Directors Election Notice to Membership
1.png)
Apr 10, 2024 Categories: In the News
Earn CEUs with the New Collection of Articles
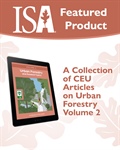
Apr 8, 2024 Categories: Industry News , ISA News
April Issue of Arborist News Online
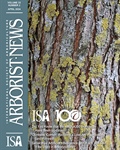
Apr 1, 2024 Categories: Industry News , ISA News
2024 Women in Arboriculture - International Women's Day
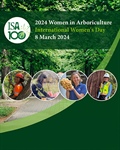
Mar 8, 2024 Categories: Industry News , ISA News
Learn More About the Underground System of Trees
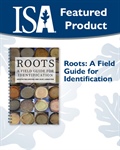
Mar 4, 2024 Categories: Industry News , ISA News
March 2024 Issue of Arboriculture & Urban Forestry Now Online!
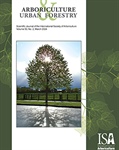
Mar 1, 2024 Categories: Industry News , ISA News
February Issue of Arborist News Online
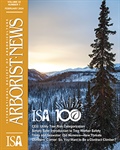
Feb 1, 2024 Categories: Industry News , ISA News
Theme picker
Image database.
ISA has a vast inventory of arboriculture- and urban forestry-related images that were compiled with the help of our members and volunteers.
Through a partnership with Forestry Images, ISA has thousands of photos and graphics available for use. ISA either owns the photos or has permission to permit their use.
Access the Image Database to browse the collection of images.
International Tree Climbing Championships
- Press Releases
- Public Service Announcements
- Industry News
- In the News
For inquiries about ISA news and media opportunities, contact our Communications Specialist .
In the tech world and beyond, new 5G applications are being discovered every day. From driverless cars to smarter cities, farms, and even shopping experiences, the latest standard in wireless networks is poised to transform the way we interact with information, devices and each other. What better time to take a closer look at how humans are putting 5G to use to transform their world.
What is 5G?
5G (fifth-generation mobile technology is the newest standard for cellular networks. Like its predecessors, 3G, 4G and 4G LTE, 5G technology uses radio waves for data transmission. However, due to significant improvements in latency, throughput and bandwidth, 5G is capable of faster download and upload speeds than previous networks.
Since its release in 2019, 5G broadband technology has been hailed as a breakthrough technology with significant implications for both consumers and businesses. Primarily, this is due to its ability to handle large volumes of data that is generated by complex devices that use its networks.
As mobile technology has expanded over the years, the number of data users generate every day has increased exponentially. Currently, other transformational technologies like artificial intelligence (AI), the Internet of Things (IoT ) and machine learning (ML) require faster speeds to function than 3G and 4G networks offer. Enter 5G, with its lightning-fast data transfer capabilities that allow newer technologies to function in the way they were designed to.
Here are some of the biggest differences between 5G and previous wireless networks.
- Physical footprint : The transmitters that are used in 5G technology are smaller than in predecessors’ networks, allowing for discrete placement in out-of-the-way places. Furthermore, “cells”—geographical areas that all wireless networks require for connectivity—in 5G networks are smaller and require less power to run than in previous generations.
- Error rates : 5G’s adaptive Modulation and Coding Scheme (MCS), a schematic that wifi devices use to transmit data, is more powerful than ones in 3G and 4G networks. This makes 5G’s Block Error Rate (BER)—a metric of error frequency—much lower.
- Bandwidth : By using a broader spectrum of radio frequencies than previous wireless networks, 5G networks can transmit on a wider range of bandwidths. This increases the number of devices that they can support at any given time.
- Lower latency : 5G’s low latency , a measurement of the time it takes data to travel from one location to another, is a significant upgrade over previous generations. This means that routine activities like downloading a file or working in the cloud is going to be faster with a 5G connection than a connection on a different network.
Like all wireless networks, 5G networks are separated into geographical areas that are known as cells. Within each cell, wireless devices—such as smartphones, PCs, and IoT devices—connect to the internet via radio waves that are transmitted between an antenna and a base station. The technology that underpins 5G is essentially the same as in 3G and 4G networks. But due to its lower latency, 5G networks are capable of delivering faster download speeds—in some cases as high as 10 gigabits per second (Gbps).
As more and more devices are built for 5G speeds, demand for 5G connectivity is growing. Today, many popular Internet Service Providers (ISPs), such as Verizon, Google and AT&T, offer 5G networks to homes and businesses. According to Statista, more than 200 million homes and businesses have already purchased it with that number expected to at least double by 2028 (link resides outside ibm.com).
Let’s take a look at three areas of technological improvement that have made 5G so unique.
New telecom specifications
The 5G NR (New Radio) standard for cellular networks defines a new radio access technology (RAT) specification for all 5G mobile networks. The 5G rollout began in 2018 with a global initiative known as the 3rd Generation Partnership Project (3FPP). The initiative defined a new set of standards to steer the design of devices and applications for use on 5G networks.
The initiative was a success, and 5G networks grew swiftly in the ensuing years. Today, 45% of networks worldwide are 5G compatible, with that number forecasted to rise to 85% by the end of the decade according to a recent report by Ericsson (link resides outside ibm.com).
Independent virtual networks (network slicing)
On 5G networks, network operators can offer multiple independent virtual networks (in addition to public ones) on the same infrastructure. Unlike previous wireless networks, this new capability allows users to do more things remotely with greater security than ever before. For example, on a 5G network, enterprises can create use cases or business models and assign them their own independent virtual network. This dramatically improves the user experience for their employees by adding greater customizability and security.
Private networks
In addition to network slicing, creating a 5G private network can also enhance personalization and security features over those available on previous generations of wireless networks. Global businesses seeking more control and mobility for their employees increasingly turn to private 5G network architectures rather than public networks they’ve used in the past.
Now that we better understand how 5G technology works, let’s take a closer look at some of the exciting applications it’s enabling.
Autonomous vehicles
From taxi cabs to drones and beyond, 5G technology underpins most of the next-generation capabilities in autonomous vehicles. Until the 5G cellular standard came along, fully autonomous vehicles were a bit of a pipe dream due to the data transmission limitations of 3G and 4G technology. Now, 5G’s lightning-fast connection speeds have made transport systems for cars, trains and more, faster than previous generations, transforming the way systems and devices connect, communicate and collaborate.
Smart factories
5G, along with AI and ML, is poised to help factories become not only smarter but more automated, efficient, and resilient. Today, many mundane but necessary tasks that are associated with equipment repair and optimization are being turned over to machines thanks to 5G connectivity paired with AI and ML capabilities. This is one area where 5G is expected to be highly disruptive, impacting everything from fuel economy to the design of equipment lifecycles and how goods arrive at our homes.
For example, on a busy factory floor, drones and cameras that are connected to smart devices that use the IoT can help locate and transport something more efficiently than in the past and prevent theft. Not only is this better for the environment and consumers, but it also frees up employees to dedicate their time and energy to tasks that are more suited to their skill sets.
Smart cities
The idea of a hyper-connected urban environment that uses 5G network speeds to spur innovation in areas like law enforcement, waste disposal and disaster mitigation is fast becoming a reality. Some cities already use 5G-enabled sensors to track traffic patterns in real time and adjust signals, helping guide the flow of traffic, minimize congestion, and improve air quality.
In another example, 5G power grids monitor supply and demand across heavily populated areas and deploy AI and ML applications to “learn” what times energy is in high or low demand. This process has been shown to significantly impact energy conservation and waste, potentially reducing carbon emissions and helping cities reach sustainability goals.
Smart healthcare
Hospitals, doctors, and the healthcare industry as a whole already benefit from the speed and reliability of 5G networks every day. One example is the area of remote surgery that uses robotics and a high-definition live stream that is connected to the internet via a 5G network. Another is the field of mobile health, where 5G gives medical workers in the field quick access to patient data and medical history. This enables them to make smarter decisions, faster, and potentially save lives.
Lastly, as we saw during the pandemic, contact tracing and the mapping of outbreaks are critical to keeping populations safe. 5G’s ability to deliver of volumes of data swiftly and securely allows experts to make more informed decisions that have ramifications for everyone.
5G paired with new technological capabilities won’t just result in the automation of employee tasks, it will dramatically improve them and the overall employee experience . Take virtual reality (VR) and augmented reality (AR), for example. VR (digital environments that shut out the real world) and AR (digital content that augments the real world) are already used by stockroom employees, transportation drivers and many others. These employees rely on wearables that are connected to a 5G network capable of high-speed data transfer rates that improve several key capabilities, including the following:
- Live views : 5G connectivity provides live, real-time views of equipment, events, and even people. One way in which this feature is being used in professional sports is to allow broadcasters to remotely call a sporting event from outside the stadium where the event is taking place.
- Digital overlays : IoT applications in a warehouse or industrial setting allow workers that are equipped with smart glasses (or even just a smartphone) to obtain real-time insights from an application. This includes repair instructions or the name and location of a spare part.
- Drone inspections : Right now, one of the leading causes of employee injury is inspection of equipment or project sites in remote and potentially dangerous areas. Drones, which are connected via 5G networks, can safely monitor equipment and project sites and even take readings from hard-to-reach gauges.
Edge computing , a computing framework that allows computations to be done closer to data sources, is fast becoming the standard for enterprises. According to this Gartner white paper (link resides outside ibm.com), by 2025, 75% of enterprise data will be processed at the edge (compared to only 10% today). This shift saves businesses time and money and enables better control over large volumes of data. It would be impossible without the new speed standards that are generated by 5G technology.
Ultra-reliable edge computing and 5G enable the enterprise to achieve faster transmission speeds, increased control and greater security over massive volumes of data. Together, these twin technologies will help reduce latency while increasing speed, reliability and bandwidth, resulting in faster, more comprehensive data analysis and insights for businesses everywhere.
5G solutions with IBM Cloud Satellite
5G presents significant opportunities for the enterprise, but first, you need a platform that can handle its speed. IBM Cloud Satellite® lets you deploy and run apps consistently across on-premises, edge computing and public cloud environments on a 5G network. And it’s all enabled by secure and auditable communications within the IBM Cloud®.
Get the latest tech insights and expert thought leadership in your inbox.
Get our newsletters and topic updates that deliver the latest thought leadership and insights on emerging trends.
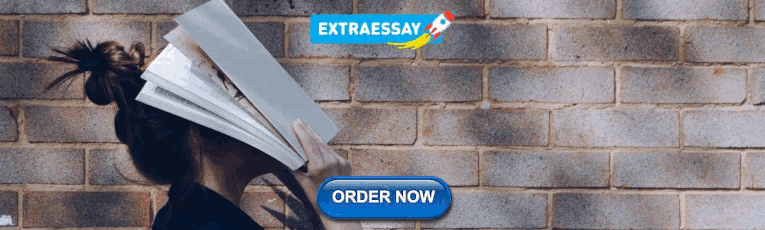
IMAGES
VIDEO
COMMENTS
Urban Education. Get hard-hitting, focused analyses of critical concerns facing inner-city schools in Urban Education (UE). This ground-breaking publication provides thought-provoking commentary … | View full journal description. This journal is a member of the Committee on Publication Ethics (COPE).
Urban education suffers from many problems, but worst among them is the spread of dense areas of poverty, where multiple social ills converge. The correlates of poverty—poor health, inadequate ...
Frequently, efforts to improve urban education are focused on interventions at the student, school, or district level. However, urban scholars recognize that many of these problems are embedded in urban poverty and related issues, including residential racial segregation, housing and food insecurity, and high levels of residential mobility and ...
As a field of study, urban education—at its most basic, education as it takes place in major conurbations, minor conurbations, or cities or towns, all with high population densities (Government Statistical Service 2016)—has attracted much interest in recent years and has usefully directed attention to the concentrations of poverty and poor educational outcomes that are characteristic of ...
implications for urban education and develop possible strategic options that could be implemented today by the coalition in an effort to "transform urban education in Ohio to become a high-demand, high-performing system in 2020." Implications for State-Level Policy In the remainder of this brief, we review the primary recommendations derived
Each issue of VUE is organized around a theme and strives to provide cutting-edge analysis of a vital issue in urban public education—formats include visual arts, articles, interviews, video and written documentaries, poetry, and autoethnographies. Our newly structured editorial board currently runs VUE. It consists of our lead editorial team ...
To paraphrase Cornel West (1992), race has mattered and does matter in urban school reform. Ethnicity has also proved to be an important factor in education in cities. As urban schools consolidated and grew into large bureaucracies from the late 1890s into the 1920s, immigrants entered cities in vast numbers.
Further, studies of both pre-service and recent graduates of urban teacher education programs and integrative reviews of educational research have found that teachers tend to view students and ...
Symposium on Urban Education. Education is one of the most important services provided by urban and local governments. But low test scores, high dropout rates, high teacher turnover rates, and ...
Education Week tracked COVID-19 protocols for some of America's largest school districts at the beginning of the 2021-22 school year. Tonya Harris , August 30, 2021 1 min read
This systematic literature review seeks to provide information on the limitations and opportunities faced by the urban poor in leveraging the potential benefits of education. The review covers the period 1995-2017 and includes 66 articles. The analysis addresses: a) the educational achievement of the urban poor and b) the conditions under ...
Despite the large urban-rural education gap observed in most countries, little attention has been paid to whether cities actually enjoy a comparative advantage in the production of human capital. Using Dutch administrative data, this paper finds that children growing in urban regions consistently attain higher levels of human capital compared ...
Urban Education School Funding Enrollment. A version of this article appeared in the March 09, 2022 edition of Education Week as The New Problem Facing Urban School Districts. Big city district ...
The Journal of Urban Learning, Teaching, & Research is published by the AERA ULTR SIG to promote new developments in urban education.
Extreme Diversity in Cities: Challenges and Solutions for Programs Serving Young Children and Their Families. While urban schools may face complex challenges in providing effective education for children who speak many languages, they also have access to resources and supports not found in suburban and rural areas. Authored by:
This means that exceptional education may account for between 20 and 35% of a total urban district's budget. Well intentioned but sometimes misapplied state and federal initiatives for special education students encourage the labeling of increasing numbers of students as learning disabled, cognitively disabled or having attention deficit ...
May 9, 2024 5:30 am ET. Text. LOS ANGELES—In a huge city awash with tiny schools, few are smaller than the Hilda L. Solis Learning Academy. The public high school, housed in a former hospital in ...
As the nation prepares to mark the 70th anniversary of the landmark U.S. Supreme Court ruling in Brown v.Board of Education, a new report from researchers at Stanford and USC shows that racial and economic segregation among schools has grown steadily in large school districts over the past three decades — an increase that appears to be driven in part by policies favoring school choice over ...
In 40% of the metro areas, wealthier districts get the most funding. A little more than half of the students in the study live in a metro area where the wealthiest communities get the most ...
The settings were a University located in an urban area and a College located in a rural area in Western Canada. During phase 1, a 56-item survey was developed from existing literature that included demographic items and items designed to measure experience with death and dying (including MAiD), education and preparation, attitudes and beliefs ...
May 2024 Issue of Arboriculture & Urban Forestry Now Online! May 1, 2024 Categories: Industry News, ISA News. Read More.
The idea of a hyper-connected urban environment that uses 5G network speeds to spur innovation in areas like law enforcement, waste disposal and disaster mitigation is fast becoming a reality. Some cities already use 5G-enabled sensors to track traffic patterns in real time and adjust signals, helping guide the flow of traffic, minimize ...