Have a language expert improve your writing
Run a free plagiarism check in 10 minutes, automatically generate references for free.
- Knowledge Base
- Dissertation
- What Is a Research Methodology? | Steps & Tips
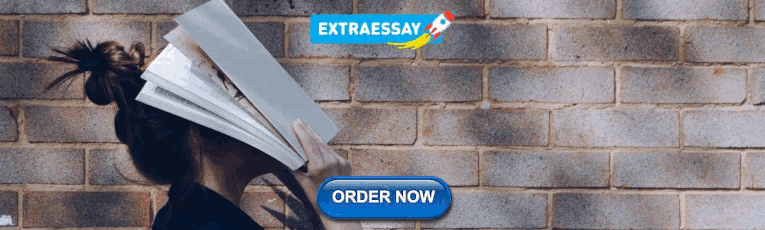
What Is a Research Methodology? | Steps & Tips
Published on 25 February 2019 by Shona McCombes . Revised on 10 October 2022.
Your research methodology discusses and explains the data collection and analysis methods you used in your research. A key part of your thesis, dissertation, or research paper, the methodology chapter explains what you did and how you did it, allowing readers to evaluate the reliability and validity of your research.
It should include:
- The type of research you conducted
- How you collected and analysed your data
- Any tools or materials you used in the research
- Why you chose these methods
- Your methodology section should generally be written in the past tense .
- Academic style guides in your field may provide detailed guidelines on what to include for different types of studies.
- Your citation style might provide guidelines for your methodology section (e.g., an APA Style methods section ).
Instantly correct all language mistakes in your text
Be assured that you'll submit flawless writing. Upload your document to correct all your mistakes.
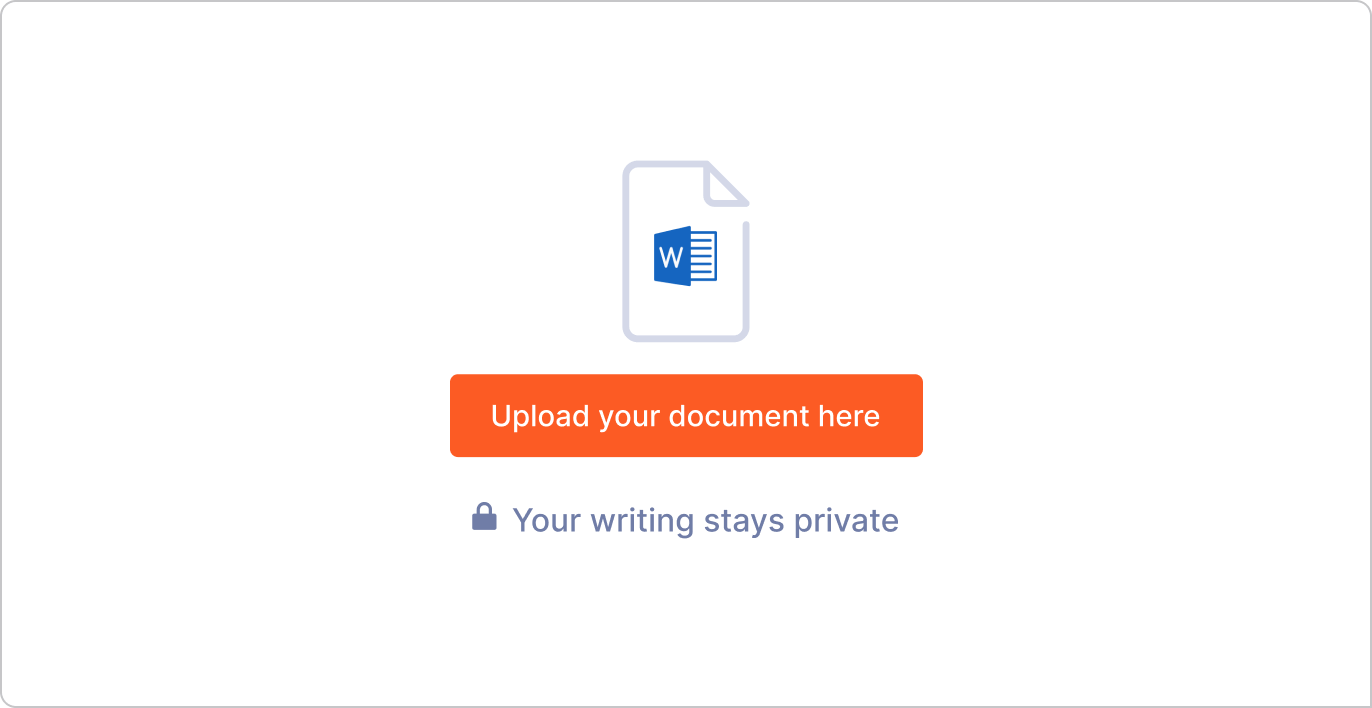
Table of contents
How to write a research methodology, why is a methods section important, step 1: explain your methodological approach, step 2: describe your data collection methods, step 3: describe your analysis method, step 4: evaluate and justify the methodological choices you made, tips for writing a strong methodology chapter, frequently asked questions about methodology.
Prevent plagiarism, run a free check.
Your methods section is your opportunity to share how you conducted your research and why you chose the methods you chose. It’s also the place to show that your research was rigorously conducted and can be replicated .
It gives your research legitimacy and situates it within your field, and also gives your readers a place to refer to if they have any questions or critiques in other sections.
You can start by introducing your overall approach to your research. You have two options here.
Option 1: Start with your “what”
What research problem or question did you investigate?
- Aim to describe the characteristics of something?
- Explore an under-researched topic?
- Establish a causal relationship?
And what type of data did you need to achieve this aim?
- Quantitative data , qualitative data , or a mix of both?
- Primary data collected yourself, or secondary data collected by someone else?
- Experimental data gathered by controlling and manipulating variables, or descriptive data gathered via observations?
Option 2: Start with your “why”
Depending on your discipline, you can also start with a discussion of the rationale and assumptions underpinning your methodology. In other words, why did you choose these methods for your study?
- Why is this the best way to answer your research question?
- Is this a standard methodology in your field, or does it require justification?
- Were there any ethical considerations involved in your choices?
- What are the criteria for validity and reliability in this type of research ?
Once you have introduced your reader to your methodological approach, you should share full details about your data collection methods .
Quantitative methods
In order to be considered generalisable, you should describe quantitative research methods in enough detail for another researcher to replicate your study.
Here, explain how you operationalised your concepts and measured your variables. Discuss your sampling method or inclusion/exclusion criteria, as well as any tools, procedures, and materials you used to gather your data.
Surveys Describe where, when, and how the survey was conducted.
- How did you design the questionnaire?
- What form did your questions take (e.g., multiple choice, Likert scale )?
- Were your surveys conducted in-person or virtually?
- What sampling method did you use to select participants?
- What was your sample size and response rate?
Experiments Share full details of the tools, techniques, and procedures you used to conduct your experiment.
- How did you design the experiment ?
- How did you recruit participants?
- How did you manipulate and measure the variables ?
- What tools did you use?
Existing data Explain how you gathered and selected the material (such as datasets or archival data) that you used in your analysis.
- Where did you source the material?
- How was the data originally produced?
- What criteria did you use to select material (e.g., date range)?
The survey consisted of 5 multiple-choice questions and 10 questions measured on a 7-point Likert scale.
The goal was to collect survey responses from 350 customers visiting the fitness apparel company’s brick-and-mortar location in Boston on 4–8 July 2022, between 11:00 and 15:00.
Here, a customer was defined as a person who had purchased a product from the company on the day they took the survey. Participants were given 5 minutes to fill in the survey anonymously. In total, 408 customers responded, but not all surveys were fully completed. Due to this, 371 survey results were included in the analysis.
Qualitative methods
In qualitative research , methods are often more flexible and subjective. For this reason, it’s crucial to robustly explain the methodology choices you made.
Be sure to discuss the criteria you used to select your data, the context in which your research was conducted, and the role you played in collecting your data (e.g., were you an active participant, or a passive observer?)
Interviews or focus groups Describe where, when, and how the interviews were conducted.
- How did you find and select participants?
- How many participants took part?
- What form did the interviews take ( structured , semi-structured , or unstructured )?
- How long were the interviews?
- How were they recorded?
Participant observation Describe where, when, and how you conducted the observation or ethnography .
- What group or community did you observe? How long did you spend there?
- How did you gain access to this group? What role did you play in the community?
- How long did you spend conducting the research? Where was it located?
- How did you record your data (e.g., audiovisual recordings, note-taking)?
Existing data Explain how you selected case study materials for your analysis.
- What type of materials did you analyse?
- How did you select them?
In order to gain better insight into possibilities for future improvement of the fitness shop’s product range, semi-structured interviews were conducted with 8 returning customers.
Here, a returning customer was defined as someone who usually bought products at least twice a week from the store.
Surveys were used to select participants. Interviews were conducted in a small office next to the cash register and lasted approximately 20 minutes each. Answers were recorded by note-taking, and seven interviews were also filmed with consent. One interviewee preferred not to be filmed.
Mixed methods
Mixed methods research combines quantitative and qualitative approaches. If a standalone quantitative or qualitative study is insufficient to answer your research question, mixed methods may be a good fit for you.
Mixed methods are less common than standalone analyses, largely because they require a great deal of effort to pull off successfully. If you choose to pursue mixed methods, it’s especially important to robustly justify your methods here.
Next, you should indicate how you processed and analysed your data. Avoid going into too much detail: you should not start introducing or discussing any of your results at this stage.
In quantitative research , your analysis will be based on numbers. In your methods section, you can include:
- How you prepared the data before analysing it (e.g., checking for missing data , removing outliers , transforming variables)
- Which software you used (e.g., SPSS, Stata or R)
- Which statistical tests you used (e.g., two-tailed t test , simple linear regression )
In qualitative research, your analysis will be based on language, images, and observations (often involving some form of textual analysis ).
Specific methods might include:
- Content analysis : Categorising and discussing the meaning of words, phrases and sentences
- Thematic analysis : Coding and closely examining the data to identify broad themes and patterns
- Discourse analysis : Studying communication and meaning in relation to their social context
Mixed methods combine the above two research methods, integrating both qualitative and quantitative approaches into one coherent analytical process.
Above all, your methodology section should clearly make the case for why you chose the methods you did. This is especially true if you did not take the most standard approach to your topic. In this case, discuss why other methods were not suitable for your objectives, and show how this approach contributes new knowledge or understanding.
In any case, it should be overwhelmingly clear to your reader that you set yourself up for success in terms of your methodology’s design. Show how your methods should lead to results that are valid and reliable, while leaving the analysis of the meaning, importance, and relevance of your results for your discussion section .
- Quantitative: Lab-based experiments cannot always accurately simulate real-life situations and behaviours, but they are effective for testing causal relationships between variables .
- Qualitative: Unstructured interviews usually produce results that cannot be generalised beyond the sample group , but they provide a more in-depth understanding of participants’ perceptions, motivations, and emotions.
- Mixed methods: Despite issues systematically comparing differing types of data, a solely quantitative study would not sufficiently incorporate the lived experience of each participant, while a solely qualitative study would be insufficiently generalisable.
Remember that your aim is not just to describe your methods, but to show how and why you applied them. Again, it’s critical to demonstrate that your research was rigorously conducted and can be replicated.
1. Focus on your objectives and research questions
The methodology section should clearly show why your methods suit your objectives and convince the reader that you chose the best possible approach to answering your problem statement and research questions .
2. Cite relevant sources
Your methodology can be strengthened by referencing existing research in your field. This can help you to:
- Show that you followed established practice for your type of research
- Discuss how you decided on your approach by evaluating existing research
- Present a novel methodological approach to address a gap in the literature
3. Write for your audience
Consider how much information you need to give, and avoid getting too lengthy. If you are using methods that are standard for your discipline, you probably don’t need to give a lot of background or justification.
Regardless, your methodology should be a clear, well-structured text that makes an argument for your approach, not just a list of technical details and procedures.
Methodology refers to the overarching strategy and rationale of your research. Developing your methodology involves studying the research methods used in your field and the theories or principles that underpin them, in order to choose the approach that best matches your objectives.
Methods are the specific tools and procedures you use to collect and analyse data (e.g. interviews, experiments , surveys , statistical tests ).
In a dissertation or scientific paper, the methodology chapter or methods section comes after the introduction and before the results , discussion and conclusion .
Depending on the length and type of document, you might also include a literature review or theoretical framework before the methodology.
Quantitative research deals with numbers and statistics, while qualitative research deals with words and meanings.
Quantitative methods allow you to test a hypothesis by systematically collecting and analysing data, while qualitative methods allow you to explore ideas and experiences in depth.
A sample is a subset of individuals from a larger population. Sampling means selecting the group that you will actually collect data from in your research.
For example, if you are researching the opinions of students in your university, you could survey a sample of 100 students.
Statistical sampling allows you to test a hypothesis about the characteristics of a population. There are various sampling methods you can use to ensure that your sample is representative of the population as a whole.
Cite this Scribbr article
If you want to cite this source, you can copy and paste the citation or click the ‘Cite this Scribbr article’ button to automatically add the citation to our free Reference Generator.
McCombes, S. (2022, October 10). What Is a Research Methodology? | Steps & Tips. Scribbr. Retrieved 6 May 2024, from https://www.scribbr.co.uk/thesis-dissertation/methodology/
Is this article helpful?
Shona McCombes
Other students also liked, how to write a dissertation proposal | a step-by-step guide, what is a literature review | guide, template, & examples, what is a theoretical framework | a step-by-step guide.
- Cookies & Privacy
- GETTING STARTED
- Introduction
- FUNDAMENTALS

Getting to the main article
Choosing your route
Setting research questions/ hypotheses
Assessment point
Building the theoretical case
Setting your research strategy
Data collection
Data analysis
Data analysis techniques
In STAGE NINE: Data analysis , we discuss the data you will have collected during STAGE EIGHT: Data collection . However, before you collect your data, having followed the research strategy you set out in this STAGE SIX , it is useful to think about the data analysis techniques you may apply to your data when it is collected.
The statistical tests that are appropriate for your dissertation will depend on (a) the research questions/hypotheses you have set, (b) the research design you are using, and (c) the nature of your data. You should already been clear about your research questions/hypotheses from STAGE THREE: Setting research questions and/or hypotheses , as well as knowing the goal of your research design from STEP TWO: Research design in this STAGE SIX: Setting your research strategy . These two pieces of information - your research questions/hypotheses and research design - will let you know, in principle , the statistical tests that may be appropriate to run on your data in order to answer your research questions.
We highlight the words in principle and may because the most appropriate statistical test to run on your data not only depend on your research questions/hypotheses and research design, but also the nature of your data . As you should have identified in STEP THREE: Research methods , and in the article, Types of variables , in the Fundamentals part of Lærd Dissertation, (a) not all data is the same, and (b) not all variables are measured in the same way (i.e., variables can be dichotomous, ordinal or continuous). In addition, not all data is normal , nor is the data when comparing groups necessarily equal , terms we explain in the Data Analysis section in the Fundamentals part of Lærd Dissertation. As a result, you might think that running a particular statistical test is correct at this point of setting your research strategy (e.g., a statistical test called a dependent t-test ), based on the research questions/hypotheses you have set, but when you collect your data (i.e., during STAGE EIGHT: Data collection ), the data may fail certain assumptions that are important to such a statistical test (i.e., normality and homogeneity of variance ). As a result, you have to run another statistical test (e.g., a Wilcoxon signed-rank test instead of a dependent t-test ).
At this stage in the dissertation process, it is important, or at the very least, useful to think about the data analysis techniques you may apply to your data when it is collected. We suggest that you do this for two reasons:
REASON A Supervisors sometimes expect you to know what statistical analysis you will perform at this stage of the dissertation process
This is not always the case, but if you have had to write a Dissertation Proposal or Ethics Proposal , there is sometimes an expectation that you explain the type of data analysis that you plan to carry out. An understanding of the data analysis that you will carry out on your data can also be an expected component of the Research Strategy chapter of your dissertation write-up (i.e., usually Chapter Three: Research Strategy ). Therefore, it is a good time to think about the data analysis process if you plan to start writing up this chapter at this stage.
REASON B It takes time to get your head around data analysis
When you come to analyse your data in STAGE NINE: Data analysis , you will need to think about (a) selecting the correct statistical tests to perform on your data, (b) running these tests on your data using a statistics package such as SPSS, and (c) learning how to interpret the output from such statistical tests so that you can answer your research questions or hypotheses. Whilst we show you how to do this for a wide range of scenarios in the in the Data Analysis section in the Fundamentals part of Lærd Dissertation, it can be a time consuming process. Unless you took an advanced statistics module/option as part of your degree (i.e., not just an introductory course to statistics, which are often taught in undergraduate and master?s degrees), it can take time to get your head around data analysis. Starting this process at this stage (i.e., STAGE SIX: Research strategy ), rather than waiting until you finish collecting your data (i.e., STAGE EIGHT: Data collection ) is a sensible approach.
Final thoughts...
Setting the research strategy for your dissertation required you to describe, explain and justify the research paradigm, quantitative research design, research method(s), sampling strategy, and approach towards research ethics and data analysis that you plan to follow, as well as determine how you will ensure the research quality of your findings so that you can effectively answer your research questions/hypotheses. However, from a practical perspective, just remember that the main goal of STAGE SIX: Research strategy is to have a clear research strategy that you can implement (i.e., operationalize ). After all, if you are unable to clearly follow your plan and carry out your research in the field, you will struggle to answer your research questions/hypotheses. Once you are sure that you have a clear plan, it is a good idea to take a step back, speak with your supervisor, and assess where you are before moving on to collect data. Therefore, when you are ready, proceed to STAGE SEVEN: Assessment point .

Library Guides
Dissertations 4: methodology: methods.
- Introduction & Philosophy
- Methodology
Primary & Secondary Sources, Primary & Secondary Data
When describing your research methods, you can start by stating what kind of secondary and, if applicable, primary sources you used in your research. Explain why you chose such sources, how well they served your research, and identify possible issues encountered using these sources.
Definitions
There is some confusion on the use of the terms primary and secondary sources, and primary and secondary data. The confusion is also due to disciplinary differences (Lombard 2010). Whilst you are advised to consult the research methods literature in your field, we can generalise as follows:
Secondary sources
Secondary sources normally include the literature (books and articles) with the experts' findings, analysis and discussions on a certain topic (Cottrell, 2014, p123). Secondary sources often interpret primary sources.
Primary sources
Primary sources are "first-hand" information such as raw data, statistics, interviews, surveys, law statutes and law cases. Even literary texts, pictures and films can be primary sources if they are the object of research (rather than, for example, documentaries reporting on something else, in which case they would be secondary sources). The distinction between primary and secondary sources sometimes lies on the use you make of them (Cottrell, 2014, p123).
Primary data
Primary data are data (primary sources) you directly obtained through your empirical work (Saunders, Lewis and Thornhill 2015, p316).
Secondary data
Secondary data are data (primary sources) that were originally collected by someone else (Saunders, Lewis and Thornhill 2015, p316).
Comparison between primary and secondary data
Use
Virtually all research will use secondary sources, at least as background information.
Often, especially at the postgraduate level, it will also use primary sources - secondary and/or primary data. The engagement with primary sources is generally appreciated, as less reliant on others' interpretations, and closer to 'facts'.
The use of primary data, as opposed to secondary data, demonstrates the researcher's effort to do empirical work and find evidence to answer her specific research question and fulfill her specific research objectives. Thus, primary data contribute to the originality of the research.
Ultimately, you should state in this section of the methodology:
What sources and data you are using and why (how are they going to help you answer the research question and/or test the hypothesis.
If using primary data, why you employed certain strategies to collect them.
What the advantages and disadvantages of your strategies to collect the data (also refer to the research in you field and research methods literature).
Quantitative, Qualitative & Mixed Methods
The methodology chapter should reference your use of quantitative research, qualitative research and/or mixed methods. The following is a description of each along with their advantages and disadvantages.
Quantitative research
Quantitative research uses numerical data (quantities) deriving, for example, from experiments, closed questions in surveys, questionnaires, structured interviews or published data sets (Cottrell, 2014, p93). It normally processes and analyses this data using quantitative analysis techniques like tables, graphs and statistics to explore, present and examine relationships and trends within the data (Saunders, Lewis and Thornhill, 2015, p496).
Qualitative research
Qualitative research is generally undertaken to study human behaviour and psyche. It uses methods like in-depth case studies, open-ended survey questions, unstructured interviews, focus groups, or unstructured observations (Cottrell, 2014, p93). The nature of the data is subjective, and also the analysis of the researcher involves a degree of subjective interpretation. Subjectivity can be controlled for in the research design, or has to be acknowledged as a feature of the research. Subject-specific books on (qualitative) research methods offer guidance on such research designs.
Mixed methods
Mixed-method approaches combine both qualitative and quantitative methods, and therefore combine the strengths of both types of research. Mixed methods have gained popularity in recent years.
When undertaking mixed-methods research you can collect the qualitative and quantitative data either concurrently or sequentially. If sequentially, you can for example, start with a few semi-structured interviews, providing qualitative insights, and then design a questionnaire to obtain quantitative evidence that your qualitative findings can also apply to a wider population (Specht, 2019, p138).
Ultimately, your methodology chapter should state:
Whether you used quantitative research, qualitative research or mixed methods.
Why you chose such methods (and refer to research method sources).
Why you rejected other methods.
How well the method served your research.
The problems or limitations you encountered.
Doug Specht, Senior Lecturer at the Westminster School of Media and Communication, explains mixed methods research in the following video:
LinkedIn Learning Video on Academic Research Foundations: Quantitative
The video covers the characteristics of quantitative research, and explains how to approach different parts of the research process, such as creating a solid research question and developing a literature review. He goes over the elements of a study, explains how to collect and analyze data, and shows how to present your data in written and numeric form.
Link to quantitative research video
Some Types of Methods
There are several methods you can use to get primary data. To reiterate, the choice of the methods should depend on your research question/hypothesis.
Whatever methods you will use, you will need to consider:
why did you choose one technique over another? What were the advantages and disadvantages of the technique you chose?
what was the size of your sample? Who made up your sample? How did you select your sample population? Why did you choose that particular sampling strategy?)
ethical considerations (see also tab...)
safety considerations
validity
feasibility
recording
procedure of the research (see box procedural method...).
Check Stella Cottrell's book Dissertations and Project Reports: A Step by Step Guide for some succinct yet comprehensive information on most methods (the following account draws mostly on her work). Check a research methods book in your discipline for more specific guidance.
Experiments
Experiments are useful to investigate cause and effect, when the variables can be tightly controlled. They can test a theory or hypothesis in controlled conditions. Experiments do not prove or disprove an hypothesis, instead they support or not support an hypothesis. When using the empirical and inductive method it is not possible to achieve conclusive results. The results may only be valid until falsified by other experiments and observations.
For more information on Scientific Method, click here .
Observations
Observational methods are useful for in-depth analyses of behaviours in people, animals, organisations, events or phenomena. They can test a theory or products in real life or simulated settings. They generally a qualitative research method.
Questionnaires and surveys
Questionnaires and surveys are useful to gain opinions, attitudes, preferences, understandings on certain matters. They can provide quantitative data that can be collated systematically; qualitative data, if they include opportunities for open-ended responses; or both qualitative and quantitative elements.
Interviews
Interviews are useful to gain rich, qualitative information about individuals' experiences, attitudes or perspectives. With interviews you can follow up immediately on responses for clarification or further details. There are three main types of interviews: structured (following a strict pattern of questions, which expect short answers), semi-structured (following a list of questions, with the opportunity to follow up the answers with improvised questions), and unstructured (following a short list of broad questions, where the respondent can lead more the conversation) (Specht, 2019, p142).
This short video on qualitative interviews discusses best practices and covers qualitative interview design, preparation and data collection methods.
Focus groups
In this case, a group of people (normally, 4-12) is gathered for an interview where the interviewer asks questions to such group of participants. Group interactions and discussions can be highly productive, but the researcher has to beware of the group effect, whereby certain participants and views dominate the interview (Saunders, Lewis and Thornhill 2015, p419). The researcher can try to minimise this by encouraging involvement of all participants and promoting a multiplicity of views.
This video focuses on strategies for conducting research using focus groups.
Check out the guidance on online focus groups by Aliaksandr Herasimenka, which is attached at the bottom of this text box.
Case study
Case studies are often a convenient way to narrow the focus of your research by studying how a theory or literature fares with regard to a specific person, group, organisation, event or other type of entity or phenomenon you identify. Case studies can be researched using other methods, including those described in this section. Case studies give in-depth insights on the particular reality that has been examined, but may not be representative of what happens in general, they may not be generalisable, and may not be relevant to other contexts. These limitations have to be acknowledged by the researcher.
Content analysis
Content analysis consists in the study of words or images within a text. In its broad definition, texts include books, articles, essays, historical documents, speeches, conversations, advertising, interviews, social media posts, films, theatre, paintings or other visuals. Content analysis can be quantitative (e.g. word frequency) or qualitative (e.g. analysing intention and implications of the communication). It can detect propaganda, identify intentions of writers, and can see differences in types of communication (Specht, 2019, p146). Check this page on collecting, cleaning and visualising Twitter data.
Extra links and resources:
Research Methods
A clear and comprehensive overview of research methods by Emerald Publishing. It includes: crowdsourcing as a research tool; mixed methods research; case study; discourse analysis; ground theory; repertory grid; ethnographic method and participant observation; interviews; focus group; action research; analysis of qualitative data; survey design; questionnaires; statistics; experiments; empirical research; literature review; secondary data and archival materials; data collection.
Doing your dissertation during the COVID-19 pandemic
Resources providing guidance on doing dissertation research during the pandemic: Online research methods; Secondary data sources; Webinars, conferences and podcasts;
- Virtual Focus Groups Guidance on managing virtual focus groups
5 Minute Methods Videos
The following are a series of useful videos that introduce research methods in five minutes. These resources have been produced by lecturers and students with the University of Westminster's School of Media and Communication.
Case Study Research
Research Ethics
Quantitative Content Analysis
Sequential Analysis
Qualitative Content Analysis
Thematic Analysis
Social Media Research
Mixed Method Research
Procedural Method
In this part, provide an accurate, detailed account of the methods and procedures that were used in the study or the experiment (if applicable!).
Include specifics about participants, sample, materials, design and methods.
If the research involves human subjects, then include a detailed description of who and how many participated along with how the participants were selected.
Describe all materials used for the study, including equipment, written materials and testing instruments.
Identify the study's design and any variables or controls employed.
Write out the steps in the order that they were completed.
Indicate what participants were asked to do, how measurements were taken and any calculations made to raw data collected.
Specify statistical techniques applied to the data to reach your conclusions.
Provide evidence that you incorporated rigor into your research. This is the quality of being thorough and accurate and considers the logic behind your research design.
Highlight any drawbacks that may have limited your ability to conduct your research thoroughly.
You have to provide details to allow others to replicate the experiment and/or verify the data, to test the validity of the research.
Bibliography
Cottrell, S. (2014). Dissertations and project reports: a step by step guide. Hampshire, England: Palgrave Macmillan.
Lombard, E. (2010). Primary and secondary sources. The Journal of Academic Librarianship , 36(3), 250-253
Saunders, M.N.K., Lewis, P. and Thornhill, A. (2015). Research Methods for Business Students. New York: Pearson Education.
Specht, D. (2019). The Media And Communications Study Skills Student Guide . London: University of Westminster Press.
- << Previous: Introduction & Philosophy
- Next: Ethics >>
- Last Updated: Sep 14, 2022 12:58 PM
- URL: https://libguides.westminster.ac.uk/methodology-for-dissertations
CONNECT WITH US
- +44 7897 053596
- [email protected]
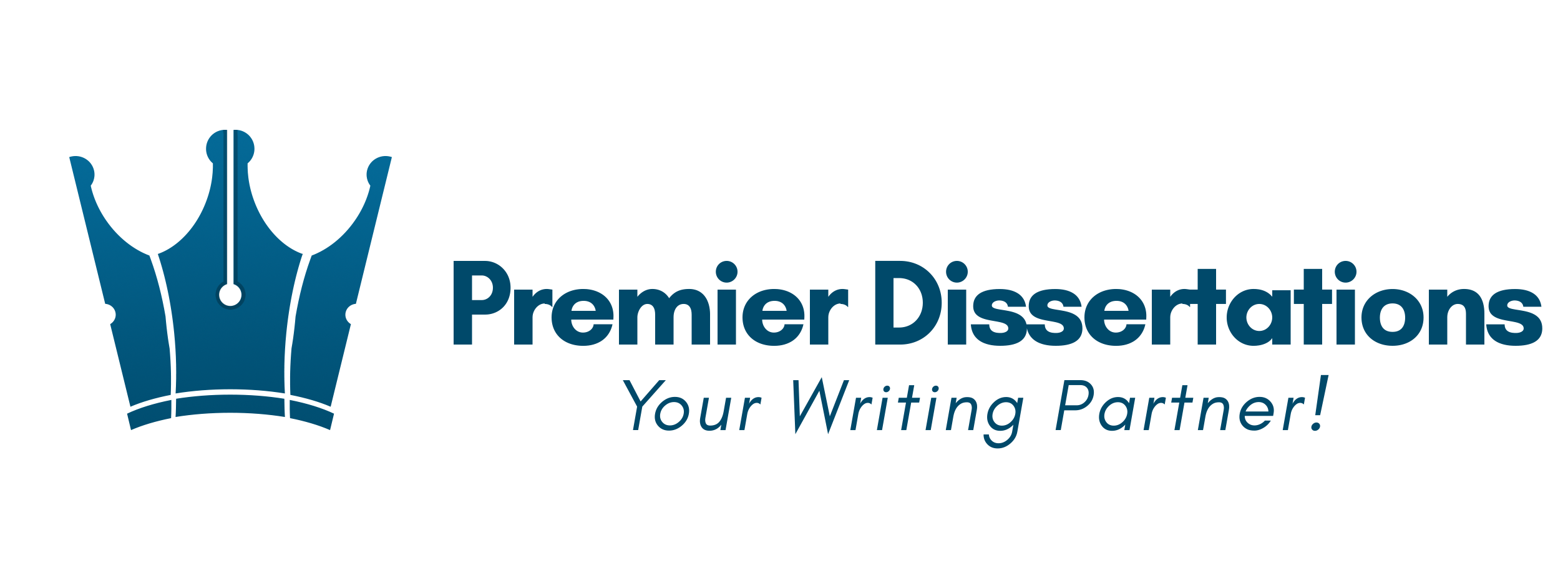
Get an experienced writer start working
Review our examples before placing an order, learn how to draft academic papers, a step-by-step guide to dissertation data analysis.
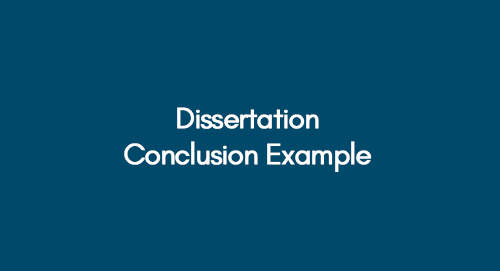
How to Write a Dissertation Conclusion? | Tips & Examples
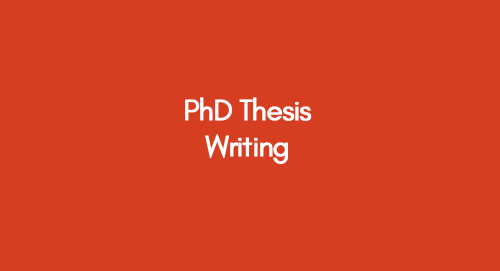
What is PhD Thesis Writing? | Beginner’s Guide
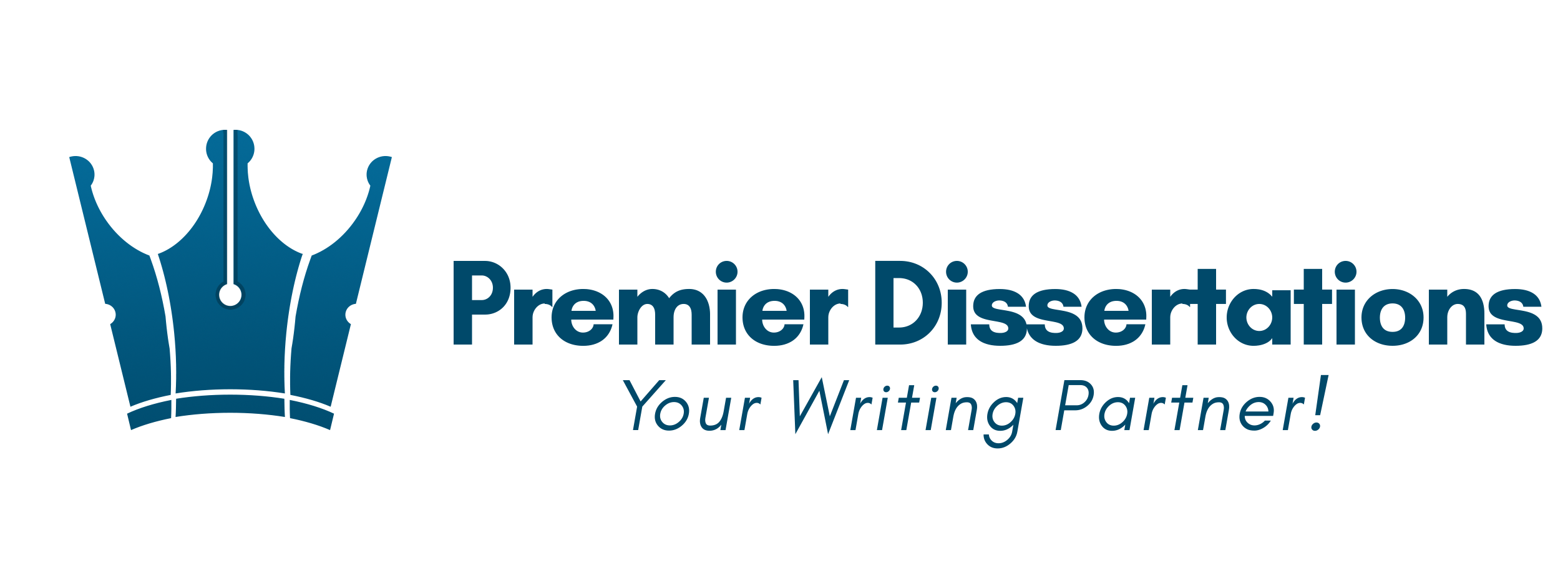
A data analysis dissertation is a complex and challenging project requiring significant time, effort, and expertise. Fortunately, it is possible to successfully complete a data analysis dissertation with careful planning and execution.
As a student, you must know how important it is to have a strong and well-written dissertation, especially regarding data analysis. Proper data analysis is crucial to the success of your research and can often make or break your dissertation.
To get a better understanding, you may review the data analysis dissertation examples listed below;
- Impact of Leadership Style on the Job Satisfaction of Nurses
- Effect of Brand Love on Consumer Buying Behaviour in Dietary Supplement Sector
- An Insight Into Alternative Dispute Resolution
- An Investigation of Cyberbullying and its Impact on Adolescent Mental Health in UK
3-Step Dissertation Process!

Get 3+ Topics

Dissertation Proposal

Get Final Dissertation
Types of data analysis for dissertation.
The various types of data Analysis in a Dissertation are as follows;
1. Qualitative Data Analysis
Qualitative data analysis is a type of data analysis that involves analyzing data that cannot be measured numerically. This data type includes interviews, focus groups, and open-ended surveys. Qualitative data analysis can be used to identify patterns and themes in the data.
2. Quantitative Data Analysis
Quantitative data analysis is a type of data analysis that involves analyzing data that can be measured numerically. This data type includes test scores, income levels, and crime rates. Quantitative data analysis can be used to test hypotheses and to look for relationships between variables.
3. Descriptive Data Analysis
Descriptive data analysis is a type of data analysis that involves describing the characteristics of a dataset. This type of data analysis summarizes the main features of a dataset.
4. Inferential Data Analysis
Inferential data analysis is a type of data analysis that involves making predictions based on a dataset. This type of data analysis can be used to test hypotheses and make predictions about future events.
5. Exploratory Data Analysis
Exploratory data analysis is a type of data analysis that involves exploring a data set to understand it better. This type of data analysis can identify patterns and relationships in the data.
Time Period to Plan and Complete a Data Analysis Dissertation?
When planning dissertation data analysis, it is important to consider the dissertation methodology structure and time series analysis as they will give you an understanding of how long each stage will take. For example, using a qualitative research method, your data analysis will involve coding and categorizing your data.
This can be time-consuming, so allowing enough time in your schedule is important. Once you have coded and categorized your data, you will need to write up your findings. Again, this can take some time, so factor this into your schedule.
Finally, you will need to proofread and edit your dissertation before submitting it. All told, a data analysis dissertation can take anywhere from several weeks to several months to complete, depending on the project’s complexity. Therefore, starting planning early and allowing enough time in your schedule to complete the task is important.
Essential Strategies for Data Analysis Dissertation
A. Planning
The first step in any dissertation is planning. You must decide what you want to write about and how you want to structure your argument. This planning will involve deciding what data you want to analyze and what methods you will use for a data analysis dissertation.
B. Prototyping
Once you have a plan for your dissertation, it’s time to start writing. However, creating a prototype is important before diving head-first into writing your dissertation. A prototype is a rough draft of your argument that allows you to get feedback from your advisor and committee members. This feedback will help you fine-tune your argument before you start writing the final version of your dissertation.
C. Executing
After you have created a plan and prototype for your data analysis dissertation, it’s time to start writing the final version. This process will involve collecting and analyzing data and writing up your results. You will also need to create a conclusion section that ties everything together.
D. Presenting
The final step in acing your data analysis dissertation is presenting it to your committee. This presentation should be well-organized and professionally presented. During the presentation, you’ll also need to be ready to respond to questions concerning your dissertation.
Data Analysis Tools
Numerous suggestive tools are employed to assess the data and deduce pertinent findings for the discussion section. The tools used to analyze data and get a scientific conclusion are as follows:
a. Excel
Excel is a spreadsheet program part of the Microsoft Office productivity software suite. Excel is a powerful tool that can be used for various data analysis tasks, such as creating charts and graphs, performing mathematical calculations, and sorting and filtering data.
b. Google Sheets
Google Sheets is a free online spreadsheet application that is part of the Google Drive suite of productivity software. Google Sheets is similar to Excel in terms of functionality, but it also has some unique features, such as the ability to collaborate with other users in real-time.
c. SPSS
SPSS is a statistical analysis software program commonly used in the social sciences. SPSS can be used for various data analysis tasks, such as hypothesis testing, factor analysis, and regression analysis.
d. STATA
STATA is a statistical analysis software program commonly used in the sciences and economics. STATA can be used for data management, statistical modelling, descriptive statistics analysis, and data visualization tasks.
SAS is a commercial statistical analysis software program used by businesses and organizations worldwide. SAS can be used for predictive modelling, market research, and fraud detection.
R is a free, open-source statistical programming language popular among statisticians and data scientists. R can be used for tasks such as data wrangling, machine learning, and creating complex visualizations.
g. Python
A variety of applications may be used using the distinctive programming language Python, including web development, scientific computing, and artificial intelligence. Python also has a number of modules and libraries that can be used for data analysis tasks, such as numerical computing, statistical modelling, and data visualization.
Testimonials
Very satisfied students
This is our reason for working. We want to make all students happy, every day. Review us on Sitejabber
Tips to Compose a Successful Data Analysis Dissertation
a. Choose a Topic You’re Passionate About
The first step to writing a successful data analysis dissertation is to choose a topic you’re passionate about. Not only will this make the research and writing process more enjoyable, but it will also ensure that you produce a high-quality paper.
Choose a topic that is particular enough to be covered in your paper’s scope but not so specific that it will be challenging to obtain enough evidence to substantiate your arguments.
b. Do Your Research
data analysis in research is an important part of academic writing. Once you’ve selected a topic, it’s time to begin your research. Be sure to consult with your advisor or supervisor frequently during this stage to ensure that you are on the right track. In addition to secondary sources such as books, journal articles, and reports, you should also consider conducting primary research through surveys or interviews. This will give you first-hand insights into your topic that can be invaluable when writing your paper.
c. Develop a Strong Thesis Statement
After you’ve done your research, it’s time to start developing your thesis statement. It is arguably the most crucial part of your entire paper, so take care to craft a clear and concise statement that encapsulates the main argument of your paper.
Remember that your thesis statement should be arguable—that is, it should be capable of being disputed by someone who disagrees with your point of view. If your thesis statement is not arguable, it will be difficult to write a convincing paper.
d. Write a Detailed Outline
Once you have developed a strong thesis statement, the next step is to write a detailed outline of your paper. This will offer you a direction to write in and guarantee that your paper makes sense from beginning to end.
Your outline should include an introduction, in which you state your thesis statement; several body paragraphs, each devoted to a different aspect of your argument; and a conclusion, in which you restate your thesis and summarize the main points of your paper.
e. Write Your First Draft
With your outline in hand, it’s finally time to start writing your first draft. At this stage, don’t worry about perfecting your grammar or making sure every sentence is exactly right—focus on getting all of your ideas down on paper (or onto the screen). Once you have completed your first draft, you can revise it for style and clarity.
And there you have it! Following these simple tips can increase your chances of success when writing your data analysis dissertation. Just remember to start early, give yourself plenty of time to research and revise, and consult with your supervisor frequently throughout the process.
How Does It Work ?
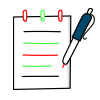
Fill the Form
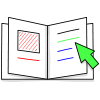
Writer Starts Working
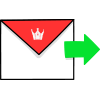
3+ Topics Emailed!
Studying the above examples gives you valuable insight into the structure and content that should be included in your own data analysis dissertation. You can also learn how to effectively analyze and present your data and make a lasting impact on your readers.
In addition to being a useful resource for completing your dissertation, these examples can also serve as a valuable reference for future academic writing projects. By following these examples and understanding their principles, you can improve your data analysis skills and increase your chances of success in your academic career.
You may also contact Premier Dissertations to develop your data analysis dissertation.
For further assistance, some other resources in the dissertation writing section are shared below;
How Do You Select the Right Data Analysis
How to Write Data Analysis For A Dissertation?
How to Develop a Conceptual Framework in Dissertation?
What is a Hypothesis in a Dissertation?
Get an Immediate Response
Discuss your requirments with our writers
WhatsApp Us Email Us Chat with Us
Get 3+ Free Dissertation Topics within 24 hours?
Your Number
Academic Level Select Academic Level Undergraduate Masters PhD
Area of Research
admin farhan
Related posts.
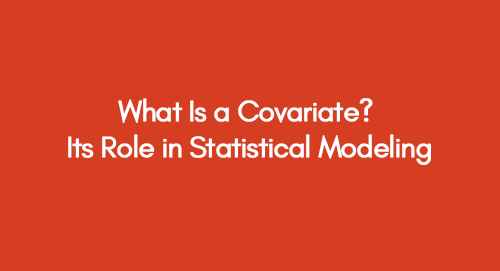
What Is a Covariate? Its Role in Statistical Modeling
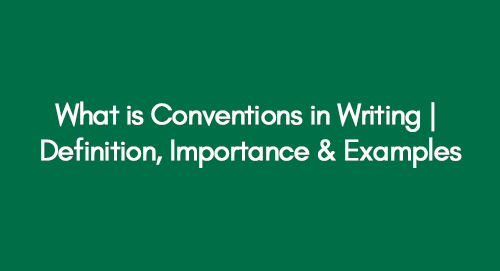
What is Conventions in Writing | Definition, Importance & Examples
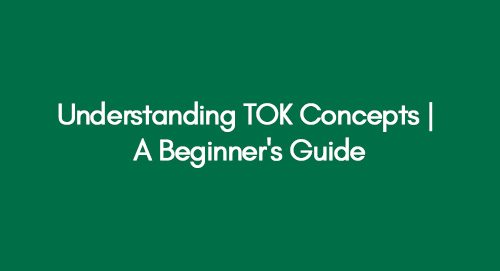
Understanding TOK Concepts: A Beginner’s Guide
Comments are closed.
ON YOUR 1ST ORDER
Mastering Dissertation Data Analysis: A Comprehensive Guide
By Laura Brown on 29th December 2023
To craft an effective dissertation data analysis chapter, you need to follow some simple steps:
- Start by planning the structure and objectives of the chapter.
- Clearly set the stage by providing a concise overview of your research design and methodology.
- Proceed to thorough data preparation, ensuring accuracy and organisation.
- Justify your methods and present the results using visual aids for clarity.
- Discuss the findings within the context of your research questions.
- Finally, review and edit your chapter to ensure coherence.
This approach will ensure a well-crafted and impactful analysis section.
Before delving into details on how you can come up with an engaging data analysis show in your dissertation, we first need to understand what it is and why it is required.
What Is Data Analysis In A Dissertation?
The data analysis chapter is a crucial section of a research dissertation that involves the examination, interpretation, and synthesis of collected data. In this chapter, researchers employ statistical techniques, qualitative methods, or a combination of both to make sense of the data gathered during the research process.
Why Is The Data Analysis Chapter So Important?
The primary objectives of the data analysis chapter are to identify patterns, trends, relationships, and insights within the data set. Researchers use various tools and software to conduct a thorough analysis, ensuring that the results are both accurate and relevant to the research questions or hypotheses. Ultimately, the findings derived from this chapter contribute to the overall conclusions of the dissertation, providing a basis for drawing meaningful and well-supported insights.
Steps Required To Craft Data Analysis Chapter To Perfection
Now that we have an idea of what a dissertation analysis chapter is and why it is necessary to put it in the dissertation, let’s move towards how we can create one that has a significant impact. Our guide will move around the bulleted points that have been discussed initially in the beginning. So, it’s time to begin.
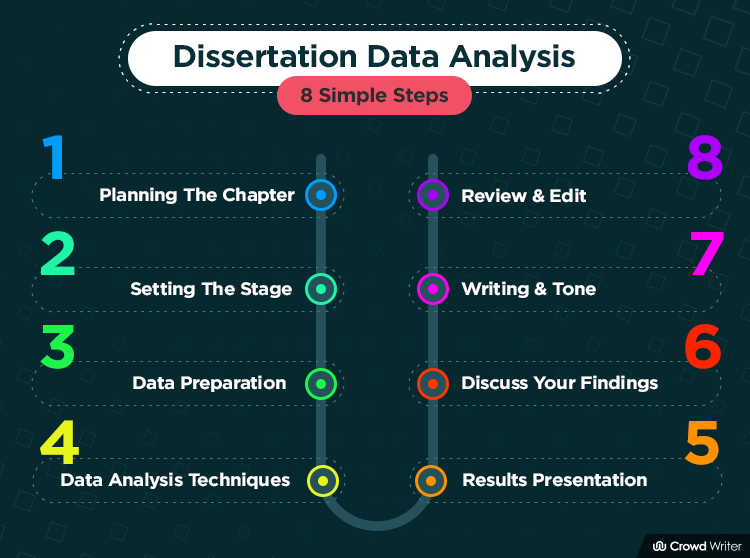
Step 1: Planning Your Data Analysis Chapter
Planning your data analysis chapter is a critical precursor to its successful execution.
- Begin by outlining the chapter structure to provide a roadmap for your analysis.
- Start with an introduction that succinctly introduces the purpose and significance of the data analysis in the context of your research.
- Following this, delineate the chapter into sections such as Data Preparation, where you detail the steps taken to organise and clean your data.
- Plan on to clearly define the Data Analysis Techniques employed, justifying their relevance to your research objectives.
- As you progress, plan for the Results Presentation, incorporating visual aids for clarity. Lastly, earmark a section for the Discussion of Findings, where you will interpret results within the broader context of your research questions.
This structured approach ensures a comprehensive and cohesive data analysis chapter, setting the stage for a compelling narrative that contributes significantly to your dissertation. You can always seek our dissertation data analysis help to plan your chapter.
Step 2: Setting The Stage – Introduction to Data Analysis
Your primary objective is to establish a solid foundation for the analytical journey. You need to skillfully link your data analysis to your research questions, elucidating the direct relevance and purpose of the upcoming analysis.
Simultaneously, define key concepts to provide clarity and ensure a shared understanding of the terms integral to your study. Following this, offer a concise overview of your data set characteristics, outlining its source, nature, and any noteworthy features.
This meticulous groundwork alongside our help with dissertation data analysis lays the base for a coherent and purposeful chapter, guiding readers seamlessly into the subsequent stages of your dissertation.
Step 3: Data Preparation
Now this is another pivotal phase in the data analysis process, ensuring the integrity and reliability of your findings. You should start with an insightful overview of the data cleaning and preprocessing procedures, highlighting the steps taken to refine and organise your dataset. Then, discuss any challenges encountered during the process and the strategies employed to address them.
Moving forward, delve into the specifics of data transformation procedures, elucidating any alterations made to the raw data for analysis. Clearly describe the methods employed for normalisation, scaling, or any other transformations deemed necessary. It will not only enhance the quality of your analysis but also foster transparency in your research methodology, reinforcing the robustness of your data-driven insights.
Step 4: Data Analysis Techniques
The data analysis section of a dissertation is akin to choosing the right tools for an artistic masterpiece. Carefully weigh the quantitative and qualitative approaches, ensuring a tailored fit for the nature of your data.
Quantitative Analysis
- Descriptive Statistics: Paint a vivid picture of your data through measures like mean, median, and mode. It’s like capturing the essence of your data’s personality.
- Inferential Statistics:Take a leap into the unknown, making educated guesses and inferences about your larger population based on a sample. It’s statistical magic in action.
Qualitative Analysis
- Thematic Analysis: Imagine your data as a novel, and thematic analysis as the tool to uncover its hidden chapters. Dissect the narrative, revealing recurring themes and patterns.
- Content Analysis: Scrutinise your data’s content like detectives, identifying key elements and meanings. It’s a deep dive into the substance of your qualitative data.
Providing Rationale for Chosen Methods
You should also articulate the why behind the chosen methods. It’s not just about numbers or themes; it’s about the story you want your data to tell. Through transparent rationale, you should ensure that your chosen techniques align seamlessly with your research goals, adding depth and credibility to the analysis.
Step 5: Presentation Of Your Results
You can simply break this process into two parts.
a. Creating Clear and Concise Visualisations
Effectively communicate your findings through meticulously crafted visualisations. Use tables that offer a structured presentation, summarising key data points for quick comprehension. Graphs, on the other hand, visually depict trends and patterns, enhancing overall clarity. Thoughtfully design these visual aids to align with the nature of your data, ensuring they serve as impactful tools for conveying information.
b. Interpreting and Explaining Results
Go beyond mere presentation by providing insightful interpretation by taking data analysis services for dissertation. Show the significance of your findings within the broader research context. Moreover, articulates the implications of observed patterns or relationships. By weaving a narrative around your results, you guide readers through the relevance and impact of your data analysis, enriching the overall understanding of your dissertation’s key contributions.
Step 6: Discussion of Findings
While discussing your findings and dissertation discussion chapter , it’s like putting together puzzle pieces to understand what your data is saying. You can always take dissertation data analysis help to explain what it all means, connecting back to why you started in the first place.
Be honest about any limitations or possible biases in your study; it’s like showing your cards to make your research more trustworthy. Comparing your results to what other smart people have found before you adds to the conversation, showing where your work fits in.
Looking ahead, you suggest ideas for what future researchers could explore, keeping the conversation going. So, it’s not just about what you found, but also about what comes next and how it all fits into the big picture of what we know.
Step 7: Writing Style and Tone
In order to perfectly come up with this chapter, follow the below points in your writing and adjust the tone accordingly,
- Use clear and concise language to ensure your audience easily understands complex concepts.
- Avoid unnecessary jargon in data analysis for thesis, and if specialised terms are necessary, provide brief explanations.
- Keep your writing style formal and objective, maintaining an academic tone throughout.
- Avoid overly casual language or slang, as the data analysis chapter is a serious academic document.
- Clearly define terms and concepts, providing specific details about your data preparation and analysis procedures.
- Use precise language to convey your ideas, minimising ambiguity.
- Follow a consistent formatting style for headings, subheadings, and citations to enhance readability.
- Ensure that tables, graphs, and visual aids are labelled and formatted uniformly for a polished presentation.
- Connect your analysis to the broader context of your research by explaining the relevance of your chosen methods and the importance of your findings.
- Offer a balance between detail and context, helping readers understand the significance of your data analysis within the larger study.
- Present enough detail to support your findings but avoid overwhelming readers with excessive information.
- Use a balance of text and visual aids to convey information efficiently.
- Maintain reader engagement by incorporating transitions between sections and effectively linking concepts.
- Use a mix of sentence structures to add variety and keep the writing engaging.
- Eliminate grammatical errors, typos, and inconsistencies through thorough proofreading.
- Consider seeking feedback from peers or mentors to ensure the clarity and coherence of your writing.
You can seek a data analysis dissertation example or sample from CrowdWriter to better understand how we write it while following the above-mentioned points.
Step 8: Reviewing and Editing
Reviewing and editing your data analysis chapter is crucial for ensuring its effectiveness and impact. By revising your work, you refine the clarity and coherence of your analysis, enhancing its overall quality.
Seeking feedback from peers, advisors or dissertation data analysis services provides valuable perspectives, helping identify blind spots and areas for improvement. Addressing common writing pitfalls, such as grammatical errors or unclear expressions, ensures your chapter is polished and professional.
Taking the time to review and edit not only strengthens the academic integrity of your work but also contributes to a final product that is clear, compelling, and ready for scholarly scrutiny.
Concluding On This Data Analysis Help
Be it master thesis data analysis, an undergraduate one or for PhD scholars, the steps remain almost the same as we have discussed in this guide. The primary focus is to be connected with your research questions and objectives while writing your data analysis chapter.
Do not lose your focus and choose the right analysis methods and design. Make sure to present your data through various visuals to better explain your data and engage the reader as well. At last, give it a detailed read and seek assistance from experts and your supervisor for further improvement.
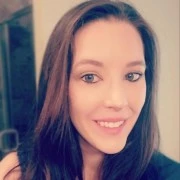
Laura Brown, a senior content writer who writes actionable blogs at Crowd Writer.
How to Analyse Secondary Data for a Dissertation
Secondary data refers to data that has already been collected by another researcher. For researchers (and students!) with limited time and resources, secondary data, whether qualitative or quantitative can be a highly viable source of data. In addition, with the advances in technology and access to peer reviewed journals and studies provided by the internet, it is increasingly popular as a form of data collection. The question that frequently arises amongst students however, is: how is secondary data best analysed?
The process of data analysis in secondary research
Secondary analysis (i.e., the use of existing data) is a systematic methodological approach that has some clear steps that need to be followed for the process to be effective. In simple terms there are three steps:
- Step One: Development of Research Questions
- Step Two: Identification of dataset
- Step Three: Evaluation of the dataset.
Let’s look at each of these in more detail:
Step One: Development of research questions
Using secondary data means you need to apply theoretical knowledge and conceptual skills to be able to use the dataset to answer research questions. Clearly therefore, the first step is thus to clearly define and develop your research questions so that you know the areas of interest that you need to explore for location of the most appropriate secondary data.
Step Two: Identification of Dataset
This stage should start with identification, through investigation, of what is currently known in the subject area and where there are gaps, and thus what data is available to address these gaps. Sources can be academic from prior studies that have used quantitative or qualitative data, and which can then be gathered together and collated to produce a new secondary dataset. In addition, other more informal or “grey” literature can also be incorporated, including consumer report, commercial studies or similar. One of the values of using secondary research is that original survey works often do not use all the data collected which means this unused information can be applied to different settings or perspectives.
Key point: Effective use of secondary data means identifying how the data can be used to deliver meaningful and relevant answers to the research questions. In other words that the data used is a good fit for the study and research questions.
Step Three: Evaluation of the dataset for effectiveness/fit
A good tip is to use a reflective approach for data evaluation. In other words, for each piece of secondary data to be utilised, it is sensible to identify the purpose of the work, the credentials of the authors (i.e., credibility, what data is provided in the original work and how long ago it was collected). In addition, the methods used and the level of consistency that exists compared to other works. This is important because understanding the primary method of data collection will impact on the overall evaluation and analysis when it is used as secondary source. In essence, if there is no understanding of the coding used in qualitative data analysis to identify key themes then there will be a mismatch with interpretations when the data is used for secondary purposes. Furthermore, having multiple sources which draw similar conclusions ensures a higher level of validity than relying on only one or two secondary sources.
A useful framework provides a flow chart of decision making, as shown in the figure below.
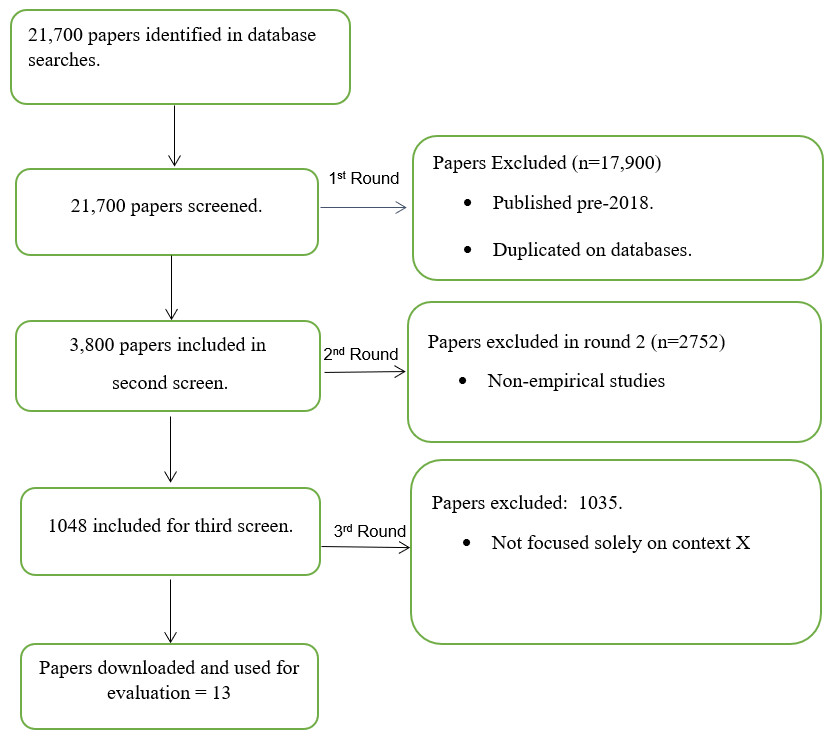
Following this process ensures that only those that are most appropriate for your research questions are included in the final dataset, but also demonstrates to your readers that you have been thorough in identifying the right works to use.
Writing up the Analysis
Once you have your dataset, writing up the analysis will depend on the process used. If the data is qualitative in nature, then you should follow the following process.
Pre-Planning
- Read and re-read all sources, identifying initial observations, correlations, and relationships between themes and how they apply to your research questions.
- Once initial themes are identified, it is sensible to explore further and identify sub-themes which lead on from the core themes and correlations in the dataset, which encourages identification of new insights and contributes to the originality of your own work.
Structure of the Analysis Presentation
Introduction.
The introduction should commence with an overview of all your sources. It is good practice to present these in a table, listed chronologically so that your work has an orderly and consistent flow. The introduction should also incorporate a brief (2-3 sentences) overview of the key outcomes and results identified.
The body text for secondary data, irrespective of whether quantitative or qualitative data is used, should be broken up into sub-sections for each argument or theme presented. In the case of qualitative data, depending on whether content, narrative or discourse analysis is used, this means presenting the key papers in the area, their conclusions and how these answer, or not, your research questions. Each source should be clearly cited and referenced at the end of the work. In the case of qualitative data, any figures or tables should be reproduced with the correct citations to their original source. In both cases, it is good practice to give a main heading of a key theme, with sub-headings for each of the sub themes identified in the analysis.
Do not use direct quotes from secondary data unless they are:
- properly referenced, and
- are key to underlining a point or conclusion that you have drawn from the data.
All results sections, regardless of whether primary or secondary data has been used should refer back to the research questions and prior works. This is because, regardless of whether the results back up or contradict previous research, including previous works shows a wider level of reading and understanding of the topic being researched and gives a greater depth to your own work.
Summary of results
The summary of the results section of a secondary data dissertation should deliver a summing up of key findings, and if appropriate a conceptual framework that clearly illustrates the findings of the work. This shows that you have understood your secondary data, how it has answered your research questions, and furthermore that your interpretation has led to some firm outcomes.
You may also like
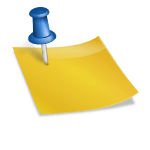
- Privacy Policy

Home » Dissertation – Format, Example and Template
Dissertation – Format, Example and Template
Table of Contents

Dissertation
Definition:
Dissertation is a lengthy and detailed academic document that presents the results of original research on a specific topic or question. It is usually required as a final project for a doctoral degree or a master’s degree.
Dissertation Meaning in Research
In Research , a dissertation refers to a substantial research project that students undertake in order to obtain an advanced degree such as a Ph.D. or a Master’s degree.
Dissertation typically involves the exploration of a particular research question or topic in-depth, and it requires students to conduct original research, analyze data, and present their findings in a scholarly manner. It is often the culmination of years of study and represents a significant contribution to the academic field.
Types of Dissertation
Types of Dissertation are as follows:
Empirical Dissertation
An empirical dissertation is a research study that uses primary data collected through surveys, experiments, or observations. It typically follows a quantitative research approach and uses statistical methods to analyze the data.
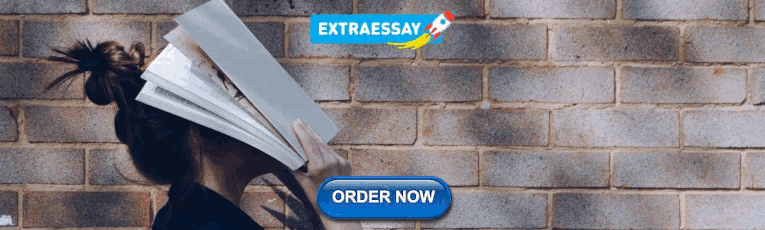
Non-Empirical Dissertation
A non-empirical dissertation is based on secondary sources, such as books, articles, and online resources. It typically follows a qualitative research approach and uses methods such as content analysis or discourse analysis.
Narrative Dissertation
A narrative dissertation is a personal account of the researcher’s experience or journey. It typically follows a qualitative research approach and uses methods such as interviews, focus groups, or ethnography.
Systematic Literature Review
A systematic literature review is a comprehensive analysis of existing research on a specific topic. It typically follows a qualitative research approach and uses methods such as meta-analysis or thematic analysis.
Case Study Dissertation
A case study dissertation is an in-depth analysis of a specific individual, group, or organization. It typically follows a qualitative research approach and uses methods such as interviews, observations, or document analysis.
Mixed-Methods Dissertation
A mixed-methods dissertation combines both quantitative and qualitative research approaches to gather and analyze data. It typically uses methods such as surveys, interviews, and focus groups, as well as statistical analysis.
How to Write a Dissertation
Here are some general steps to help guide you through the process of writing a dissertation:
- Choose a topic : Select a topic that you are passionate about and that is relevant to your field of study. It should be specific enough to allow for in-depth research but broad enough to be interesting and engaging.
- Conduct research : Conduct thorough research on your chosen topic, utilizing a variety of sources, including books, academic journals, and online databases. Take detailed notes and organize your information in a way that makes sense to you.
- Create an outline : Develop an outline that will serve as a roadmap for your dissertation. The outline should include the introduction, literature review, methodology, results, discussion, and conclusion.
- Write the introduction: The introduction should provide a brief overview of your topic, the research questions, and the significance of the study. It should also include a clear thesis statement that states your main argument.
- Write the literature review: The literature review should provide a comprehensive analysis of existing research on your topic. It should identify gaps in the research and explain how your study will fill those gaps.
- Write the methodology: The methodology section should explain the research methods you used to collect and analyze data. It should also include a discussion of any limitations or weaknesses in your approach.
- Write the results: The results section should present the findings of your research in a clear and organized manner. Use charts, graphs, and tables to help illustrate your data.
- Write the discussion: The discussion section should interpret your results and explain their significance. It should also address any limitations of the study and suggest areas for future research.
- Write the conclusion: The conclusion should summarize your main findings and restate your thesis statement. It should also provide recommendations for future research.
- Edit and revise: Once you have completed a draft of your dissertation, review it carefully to ensure that it is well-organized, clear, and free of errors. Make any necessary revisions and edits before submitting it to your advisor for review.
Dissertation Format
The format of a dissertation may vary depending on the institution and field of study, but generally, it follows a similar structure:
- Title Page: This includes the title of the dissertation, the author’s name, and the date of submission.
- Abstract : A brief summary of the dissertation’s purpose, methods, and findings.
- Table of Contents: A list of the main sections and subsections of the dissertation, along with their page numbers.
- Introduction : A statement of the problem or research question, a brief overview of the literature, and an explanation of the significance of the study.
- Literature Review : A comprehensive review of the literature relevant to the research question or problem.
- Methodology : A description of the methods used to conduct the research, including data collection and analysis procedures.
- Results : A presentation of the findings of the research, including tables, charts, and graphs.
- Discussion : A discussion of the implications of the findings, their significance in the context of the literature, and limitations of the study.
- Conclusion : A summary of the main points of the study and their implications for future research.
- References : A list of all sources cited in the dissertation.
- Appendices : Additional materials that support the research, such as data tables, charts, or transcripts.
Dissertation Outline
Dissertation Outline is as follows:
Title Page:
- Title of dissertation
- Author name
- Institutional affiliation
- Date of submission
- Brief summary of the dissertation’s research problem, objectives, methods, findings, and implications
- Usually around 250-300 words
Table of Contents:
- List of chapters and sections in the dissertation, with page numbers for each
I. Introduction
- Background and context of the research
- Research problem and objectives
- Significance of the research
II. Literature Review
- Overview of existing literature on the research topic
- Identification of gaps in the literature
- Theoretical framework and concepts
III. Methodology
- Research design and methods used
- Data collection and analysis techniques
- Ethical considerations
IV. Results
- Presentation and analysis of data collected
- Findings and outcomes of the research
- Interpretation of the results
V. Discussion
- Discussion of the results in relation to the research problem and objectives
- Evaluation of the research outcomes and implications
- Suggestions for future research
VI. Conclusion
- Summary of the research findings and outcomes
- Implications for the research topic and field
- Limitations and recommendations for future research
VII. References
- List of sources cited in the dissertation
VIII. Appendices
- Additional materials that support the research, such as tables, figures, or questionnaires.
Example of Dissertation
Here is an example Dissertation for students:
Title : Exploring the Effects of Mindfulness Meditation on Academic Achievement and Well-being among College Students
This dissertation aims to investigate the impact of mindfulness meditation on the academic achievement and well-being of college students. Mindfulness meditation has gained popularity as a technique for reducing stress and enhancing mental health, but its effects on academic performance have not been extensively studied. Using a randomized controlled trial design, the study will compare the academic performance and well-being of college students who practice mindfulness meditation with those who do not. The study will also examine the moderating role of personality traits and demographic factors on the effects of mindfulness meditation.
Chapter Outline:
Chapter 1: Introduction
- Background and rationale for the study
- Research questions and objectives
- Significance of the study
- Overview of the dissertation structure
Chapter 2: Literature Review
- Definition and conceptualization of mindfulness meditation
- Theoretical framework of mindfulness meditation
- Empirical research on mindfulness meditation and academic achievement
- Empirical research on mindfulness meditation and well-being
- The role of personality and demographic factors in the effects of mindfulness meditation
Chapter 3: Methodology
- Research design and hypothesis
- Participants and sampling method
- Intervention and procedure
- Measures and instruments
- Data analysis method
Chapter 4: Results
- Descriptive statistics and data screening
- Analysis of main effects
- Analysis of moderating effects
- Post-hoc analyses and sensitivity tests
Chapter 5: Discussion
- Summary of findings
- Implications for theory and practice
- Limitations and directions for future research
- Conclusion and contribution to the literature
Chapter 6: Conclusion
- Recap of the research questions and objectives
- Summary of the key findings
- Contribution to the literature and practice
- Implications for policy and practice
- Final thoughts and recommendations.
References :
List of all the sources cited in the dissertation
Appendices :
Additional materials such as the survey questionnaire, interview guide, and consent forms.
Note : This is just an example and the structure of a dissertation may vary depending on the specific requirements and guidelines provided by the institution or the supervisor.
How Long is a Dissertation
The length of a dissertation can vary depending on the field of study, the level of degree being pursued, and the specific requirements of the institution. Generally, a dissertation for a doctoral degree can range from 80,000 to 100,000 words, while a dissertation for a master’s degree may be shorter, typically ranging from 20,000 to 50,000 words. However, it is important to note that these are general guidelines and the actual length of a dissertation can vary widely depending on the specific requirements of the program and the research topic being studied. It is always best to consult with your academic advisor or the guidelines provided by your institution for more specific information on dissertation length.
Applications of Dissertation
Here are some applications of a dissertation:
- Advancing the Field: Dissertations often include new research or a new perspective on existing research, which can help to advance the field. The results of a dissertation can be used by other researchers to build upon or challenge existing knowledge, leading to further advancements in the field.
- Career Advancement: Completing a dissertation demonstrates a high level of expertise in a particular field, which can lead to career advancement opportunities. For example, having a PhD can open doors to higher-paying jobs in academia, research institutions, or the private sector.
- Publishing Opportunities: Dissertations can be published as books or journal articles, which can help to increase the visibility and credibility of the author’s research.
- Personal Growth: The process of writing a dissertation involves a significant amount of research, analysis, and critical thinking. This can help students to develop important skills, such as time management, problem-solving, and communication, which can be valuable in both their personal and professional lives.
- Policy Implications: The findings of a dissertation can have policy implications, particularly in fields such as public health, education, and social sciences. Policymakers can use the research to inform decision-making and improve outcomes for the population.
When to Write a Dissertation
Here are some situations where writing a dissertation may be necessary:
- Pursuing a Doctoral Degree: Writing a dissertation is usually a requirement for earning a doctoral degree, so if you are interested in pursuing a doctorate, you will likely need to write a dissertation.
- Conducting Original Research : Dissertations require students to conduct original research on a specific topic. If you are interested in conducting original research on a topic, writing a dissertation may be the best way to do so.
- Advancing Your Career: Some professions, such as academia and research, may require individuals to have a doctoral degree. Writing a dissertation can help you advance your career by demonstrating your expertise in a particular area.
- Contributing to Knowledge: Dissertations are often based on original research that can contribute to the knowledge base of a field. If you are passionate about advancing knowledge in a particular area, writing a dissertation can help you achieve that goal.
- Meeting Academic Requirements : If you are a graduate student, writing a dissertation may be a requirement for completing your program. Be sure to check with your academic advisor to determine if this is the case for you.
Purpose of Dissertation
some common purposes of a dissertation include:
- To contribute to the knowledge in a particular field : A dissertation is often the culmination of years of research and study, and it should make a significant contribution to the existing body of knowledge in a particular field.
- To demonstrate mastery of a subject: A dissertation requires extensive research, analysis, and writing, and completing one demonstrates a student’s mastery of their subject area.
- To develop critical thinking and research skills : A dissertation requires students to think critically about their research question, analyze data, and draw conclusions based on evidence. These skills are valuable not only in academia but also in many professional fields.
- To demonstrate academic integrity: A dissertation must be conducted and written in accordance with rigorous academic standards, including ethical considerations such as obtaining informed consent, protecting the privacy of participants, and avoiding plagiarism.
- To prepare for an academic career: Completing a dissertation is often a requirement for obtaining a PhD and pursuing a career in academia. It can demonstrate to potential employers that the student has the necessary skills and experience to conduct original research and make meaningful contributions to their field.
- To develop writing and communication skills: A dissertation requires a significant amount of writing and communication skills to convey complex ideas and research findings in a clear and concise manner. This skill set can be valuable in various professional fields.
- To demonstrate independence and initiative: A dissertation requires students to work independently and take initiative in developing their research question, designing their study, collecting and analyzing data, and drawing conclusions. This demonstrates to potential employers or academic institutions that the student is capable of independent research and taking initiative in their work.
- To contribute to policy or practice: Some dissertations may have a practical application, such as informing policy decisions or improving practices in a particular field. These dissertations can have a significant impact on society, and their findings may be used to improve the lives of individuals or communities.
- To pursue personal interests: Some students may choose to pursue a dissertation topic that aligns with their personal interests or passions, providing them with the opportunity to delve deeper into a topic that they find personally meaningful.
Advantage of Dissertation
Some advantages of writing a dissertation include:
- Developing research and analytical skills: The process of writing a dissertation involves conducting extensive research, analyzing data, and presenting findings in a clear and coherent manner. This process can help students develop important research and analytical skills that can be useful in their future careers.
- Demonstrating expertise in a subject: Writing a dissertation allows students to demonstrate their expertise in a particular subject area. It can help establish their credibility as a knowledgeable and competent professional in their field.
- Contributing to the academic community: A well-written dissertation can contribute new knowledge to the academic community and potentially inform future research in the field.
- Improving writing and communication skills : Writing a dissertation requires students to write and present their research in a clear and concise manner. This can help improve their writing and communication skills, which are essential for success in many professions.
- Increasing job opportunities: Completing a dissertation can increase job opportunities in certain fields, particularly in academia and research-based positions.
About the author
Muhammad Hassan
Researcher, Academic Writer, Web developer
You may also like

Data Collection – Methods Types and Examples

Delimitations in Research – Types, Examples and...

Research Process – Steps, Examples and Tips

Research Design – Types, Methods and Examples

Institutional Review Board – Application Sample...

Evaluating Research – Process, Examples and...

How To Write The Results/Findings Chapter
For qualitative studies (dissertations & theses).
By: Jenna Crossley (PhD). Expert Reviewed By: Dr. Eunice Rautenbach | August 2021
So, you’ve collected and analysed your qualitative data, and it’s time to write up your results chapter. But where do you start? In this post, we’ll guide you through the qualitative results chapter (also called the findings chapter), step by step.
Overview: Qualitative Results Chapter
- What (exactly) the qualitative results chapter is
- What to include in your results chapter
- How to write up your results chapter
- A few tips and tricks to help you along the way
- Free results chapter template
What exactly is the results chapter?
The results chapter in a dissertation or thesis (or any formal academic research piece) is where you objectively and neutrally present the findings of your qualitative analysis (or analyses if you used multiple qualitative analysis methods ). This chapter can sometimes be combined with the discussion chapter (where you interpret the data and discuss its meaning), depending on your university’s preference. We’ll treat the two chapters as separate, as that’s the most common approach.
In contrast to a quantitative results chapter that presents numbers and statistics, a qualitative results chapter presents data primarily in the form of words . But this doesn’t mean that a qualitative study can’t have quantitative elements – you could, for example, present the number of times a theme or topic pops up in your data, depending on the analysis method(s) you adopt.
Adding a quantitative element to your study can add some rigour, which strengthens your results by providing more evidence for your claims. This is particularly common when using qualitative content analysis. Keep in mind though that qualitative research aims to achieve depth, richness and identify nuances , so don’t get tunnel vision by focusing on the numbers. They’re just cream on top in a qualitative analysis.
So, to recap, the results chapter is where you objectively present the findings of your analysis, without interpreting them (you’ll save that for the discussion chapter). With that out the way, let’s take a look at what you should include in your results chapter.
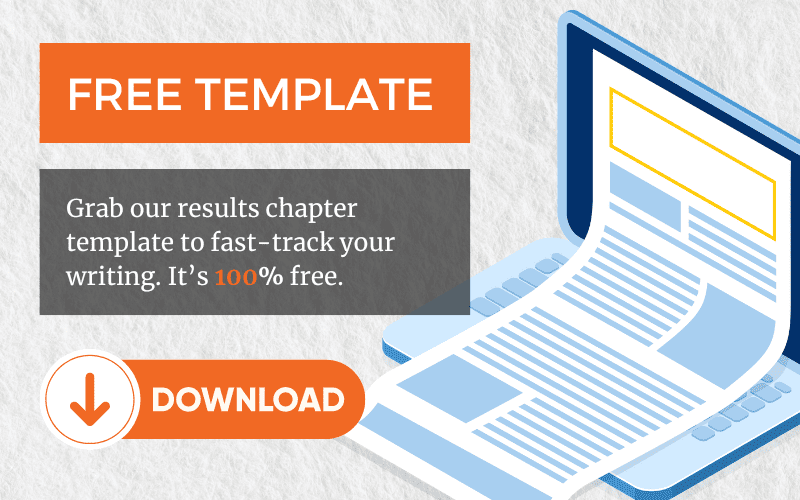
What should you include in the results chapter?
As we’ve mentioned, your qualitative results chapter should purely present and describe your results , not interpret them in relation to the existing literature or your research questions . Any speculations or discussion about the implications of your findings should be reserved for your discussion chapter.
In your results chapter, you’ll want to talk about your analysis findings and whether or not they support your hypotheses (if you have any). Naturally, the exact contents of your results chapter will depend on which qualitative analysis method (or methods) you use. For example, if you were to use thematic analysis, you’d detail the themes identified in your analysis, using extracts from the transcripts or text to support your claims.
While you do need to present your analysis findings in some detail, you should avoid dumping large amounts of raw data in this chapter. Instead, focus on presenting the key findings and using a handful of select quotes or text extracts to support each finding . The reams of data and analysis can be relegated to your appendices.
While it’s tempting to include every last detail you found in your qualitative analysis, it is important to make sure that you report only that which is relevant to your research aims, objectives and research questions . Always keep these three components, as well as your hypotheses (if you have any) front of mind when writing the chapter and use them as a filter to decide what’s relevant and what’s not.
Need a helping hand?
How do I write the results chapter?
Now that we’ve covered the basics, it’s time to look at how to structure your chapter. Broadly speaking, the results chapter needs to contain three core components – the introduction, the body and the concluding summary. Let’s take a look at each of these.
Section 1: Introduction
The first step is to craft a brief introduction to the chapter. This intro is vital as it provides some context for your findings. In your introduction, you should begin by reiterating your problem statement and research questions and highlight the purpose of your research . Make sure that you spell this out for the reader so that the rest of your chapter is well contextualised.
The next step is to briefly outline the structure of your results chapter. In other words, explain what’s included in the chapter and what the reader can expect. In the results chapter, you want to tell a story that is coherent, flows logically, and is easy to follow , so make sure that you plan your structure out well and convey that structure (at a high level), so that your reader is well oriented.
The introduction section shouldn’t be lengthy. Two or three short paragraphs should be more than adequate. It is merely an introduction and overview, not a summary of the chapter.
Pro Tip – To help you structure your chapter, it can be useful to set up an initial draft with (sub)section headings so that you’re able to easily (re)arrange parts of your chapter. This will also help your reader to follow your results and give your chapter some coherence. Be sure to use level-based heading styles (e.g. Heading 1, 2, 3 styles) to help the reader differentiate between levels visually. You can find these options in Word (example below).

Section 2: Body
Before we get started on what to include in the body of your chapter, it’s vital to remember that a results section should be completely objective and descriptive, not interpretive . So, be careful not to use words such as, “suggests” or “implies”, as these usually accompany some form of interpretation – that’s reserved for your discussion chapter.
The structure of your body section is very important , so make sure that you plan it out well. When planning out your qualitative results chapter, create sections and subsections so that you can maintain the flow of the story you’re trying to tell. Be sure to systematically and consistently describe each portion of results. Try to adopt a standardised structure for each portion so that you achieve a high level of consistency throughout the chapter.
For qualitative studies, results chapters tend to be structured according to themes , which makes it easier for readers to follow. However, keep in mind that not all results chapters have to be structured in this manner. For example, if you’re conducting a longitudinal study, you may want to structure your chapter chronologically. Similarly, you might structure this chapter based on your theoretical framework . The exact structure of your chapter will depend on the nature of your study , especially your research questions.
As you work through the body of your chapter, make sure that you use quotes to substantiate every one of your claims . You can present these quotes in italics to differentiate them from your own words. A general rule of thumb is to use at least two pieces of evidence per claim, and these should be linked directly to your data. Also, remember that you need to include all relevant results , not just the ones that support your assumptions or initial leanings.
In addition to including quotes, you can also link your claims to the data by using appendices , which you should reference throughout your text. When you reference, make sure that you include both the name/number of the appendix , as well as the line(s) from which you drew your data.
As referencing styles can vary greatly, be sure to look up the appendix referencing conventions of your university’s prescribed style (e.g. APA , Harvard, etc) and keep this consistent throughout your chapter.
Section 3: Concluding summary
The concluding summary is very important because it summarises your key findings and lays the foundation for the discussion chapter . Keep in mind that some readers may skip directly to this section (from the introduction section), so make sure that it can be read and understood well in isolation.
In this section, you need to remind the reader of the key findings. That is, the results that directly relate to your research questions and that you will build upon in your discussion chapter. Remember, your reader has digested a lot of information in this chapter, so you need to use this section to remind them of the most important takeaways.
Importantly, the concluding summary should not present any new information and should only describe what you’ve already presented in your chapter. Keep it concise – you’re not summarising the whole chapter, just the essentials.
Tips for writing an A-grade results chapter
Now that you’ve got a clear picture of what the qualitative results chapter is all about, here are some quick tips and reminders to help you craft a high-quality chapter:
- Your results chapter should be written in the past tense . You’ve done the work already, so you want to tell the reader what you found , not what you are currently finding .
- Make sure that you review your work multiple times and check that every claim is adequately backed up by evidence . Aim for at least two examples per claim, and make use of an appendix to reference these.
- When writing up your results, make sure that you stick to only what is relevant . Don’t waste time on data that are not relevant to your research objectives and research questions.
- Use headings and subheadings to create an intuitive, easy to follow piece of writing. Make use of Microsoft Word’s “heading styles” and be sure to use them consistently.
- When referring to numerical data, tables and figures can provide a useful visual aid. When using these, make sure that they can be read and understood independent of your body text (i.e. that they can stand-alone). To this end, use clear, concise labels for each of your tables or figures and make use of colours to code indicate differences or hierarchy.
- Similarly, when you’re writing up your chapter, it can be useful to highlight topics and themes in different colours . This can help you to differentiate between your data if you get a bit overwhelmed and will also help you to ensure that your results flow logically and coherently.
If you have any questions, leave a comment below and we’ll do our best to help. If you’d like 1-on-1 help with your results chapter (or any chapter of your dissertation or thesis), check out our private dissertation coaching service here or book a free initial consultation to discuss how we can help you.
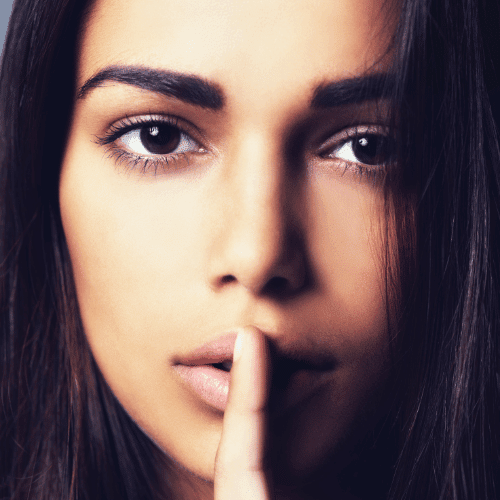
Psst... there’s more!
This post was based on one of our popular Research Bootcamps . If you're working on a research project, you'll definitely want to check this out ...
You Might Also Like:
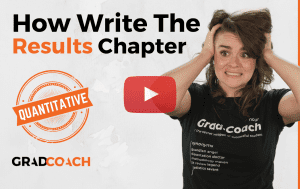
20 Comments
This was extremely helpful. Thanks a lot guys
Hi, thanks for the great research support platform created by the gradcoach team!
I wanted to ask- While “suggests” or “implies” are interpretive terms, what terms could we use for the results chapter? Could you share some examples of descriptive terms?
I think that instead of saying, ‘The data suggested, or The data implied,’ you can say, ‘The Data showed or revealed, or illustrated or outlined’…If interview data, you may say Jane Doe illuminated or elaborated, or Jane Doe described… or Jane Doe expressed or stated.
I found this article very useful. Thank you very much for the outstanding work you are doing.
What if i have 3 different interviewees answering the same interview questions? Should i then present the results in form of the table with the division on the 3 perspectives or rather give a results in form of the text and highlight who said what?
I think this tabular representation of results is a great idea. I am doing it too along with the text. Thanks
That was helpful was struggling to separate the discussion from the findings
this was very useful, Thank you.
Very helpful, I am confident to write my results chapter now.
It is so helpful! It is a good job. Thank you very much!
Very useful, well explained. Many thanks.
Hello, I appreciate the way you provided a supportive comments about qualitative results presenting tips
I loved this! It explains everything needed, and it has helped me better organize my thoughts. What words should I not use while writing my results section, other than subjective ones.
Thanks a lot, it is really helpful
Thank you so much dear, i really appropriate your nice explanations about this.
Thank you so much for this! I was wondering if anyone could help with how to prproperly integrate quotations (Excerpts) from interviews in the finding chapter in a qualitative research. Please GradCoach, address this issue and provide examples.
what if I’m not doing any interviews myself and all the information is coming from case studies that have already done the research.
Very helpful thank you.
This was very helpful as I was wondering how to structure this part of my dissertation, to include the quotes… Thanks for this explanation
This is very helpful, thanks! I am required to write up my results chapters with the discussion in each of them – any tips and tricks for this strategy?
Submit a Comment Cancel reply
Your email address will not be published. Required fields are marked *
Save my name, email, and website in this browser for the next time I comment.
- Print Friendly
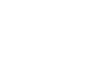
Types of Analytical Skills Used Throughout Dissertation Writing
The prime objective of critical skill is to increase the ability of the students so that they can analyse critically the specific information to solve a problem or a case study. It is directly connected with critical thinking skills and problem-solving skills. Analytical skills are much required throughout the academic and professional life of the students. This is one of the reasons that I recommend students to work on their assignments so that they can effectively develop these skills.
If you want to develop analytical skills throughout the academic period, then it is important that you pay attention to the claims and arguments you provide throughout the assignments. Analytical skills are developed among students when they work on academic writing activities. There are many academic activities that demand analytical skills as a basic requirement. Analytical skills are not an option but a necessity for every academic writing activity.
Dissertation writing is one of the activities which is entirely dependent on analytical skills. For dissertation writing, students have to select a topic for the dissertation based on the specialisation selected at the beginning of the program. It is important for the students to select the relevant topic so that we can effectively complete the process. The dissertation is descriptive writing and demands are a huge amount of words to complete the research.
The most difficult thing for the students is to understand the requirements and demands of professional dissertation writing. However, I always recommend students to get professional UK dissertation writing services if they find any kind of difficulties throughout the process of research. It is good to ask for the help of the experts instead of following the wrong track throughout the process of research. This will also help you to meet the standards and the requirements of dissertation writing effectively and efficiently.
Before talking about anything on analytical skills, let’s see the importance of analytical skills throughout dissertation writing.
Significance of Analytical Skills
There are no doubts that analytical skills are one of the most significant skills that students can have throughout their academic life. They are considered significant because it allows many of the students to make the right decisions or to find the best possible solution for a specific problem. Analytical skills are a requirement for all types of academics regardless of any nature or level.
Analytical skills of the basic requirement for research. It is more effective and required when you are conducting quantitative research. It is because quantitative research requires numerical data with some statistical reasons. Students have to analyse the data so that they can provide the claim while supporting their names by providing logical figures along with facts and reasoning.
The quantitative research method is mostly used for mathematics and other numerical subjects. However, if we talk about qualitative research, the entire data is collected through questionnaires and other modes of collection. Qualitative research also demands a good grip on analytical skills to get the desired results.
The main thing is the collection of the data. A question comes in the mind of the students, that where to get the data from? Well, there are many sources of information available online for the students that they can get all types of informational data to be used throughout its addition writing. However, not everything provided on the internet is reliable and relevant. I always advise students to select the most relevant, reliable, and authentic source of information to gather the required information data.
What Are the Types of Analytical Skills?
There are many types of analytical skills used throughout different types of situations depending on the nature of the problem. Today we are going to discuss some of the most important and significant types of analytical skills that are must be developed for professional dissertation writing.
Communication Skills
Communication skills are mostly misunderstood by students. It is not only how you communicate with your professional social circle, but it is highly for academic dissertation writing. It doesn’t matter how good you have conducted the research, if you do not have the right to words to explain your results and findings to the readers, then your entire research is of no use.
The prime objective of academic research is to provide the right and deep understanding of the results and findings to the readers so that they can understand your claims and accept them at the same time. This is one of the reasons that students are always advised to work on their communication skills if they want to get top quality results for dissertation writing.
Creative Thinking
Creative thinking is also a basic factor of analytical skills. The prime objective of analytical skills is to create things for innovative thoughts and ideas to solve a certain issue. There are different types of scenarios that you have to face throughout academic life, and for that, you need to think out of the box to get the best possible solution to the problem. When you think creatively, you automatically get the best possible solution to the problem.
Critical Thinking
Critical thinking is one of the most important factors of analytical skills. If you want to develop and maintain your analytical skills, then I would advise you to work on your critical thinking skills. Critical thinking always enables you to analyse and issue and to provide the right solution through analysis. The objective of critical thinking skill is to link the provided ideas along with the logical evidence throughout the dissertation writing.
Data Analysis
It doesn’t matter if you are conducting quantitative or qualitative research, for both of them you need to analyse the data in the best possible way so that you can get the desired results. Dissertation writing required to identify the patterns and to analyse huge data.
Conclusion:
There are a certain basic set of skills that are required for dissertation writing. Analytical skills are a basic requirement for writing a dissertation according to the standards and the requirements of the university. The above-mentioned types and importance of analytical skills would allow you to be effective throughout the process of professional dissertation writing.
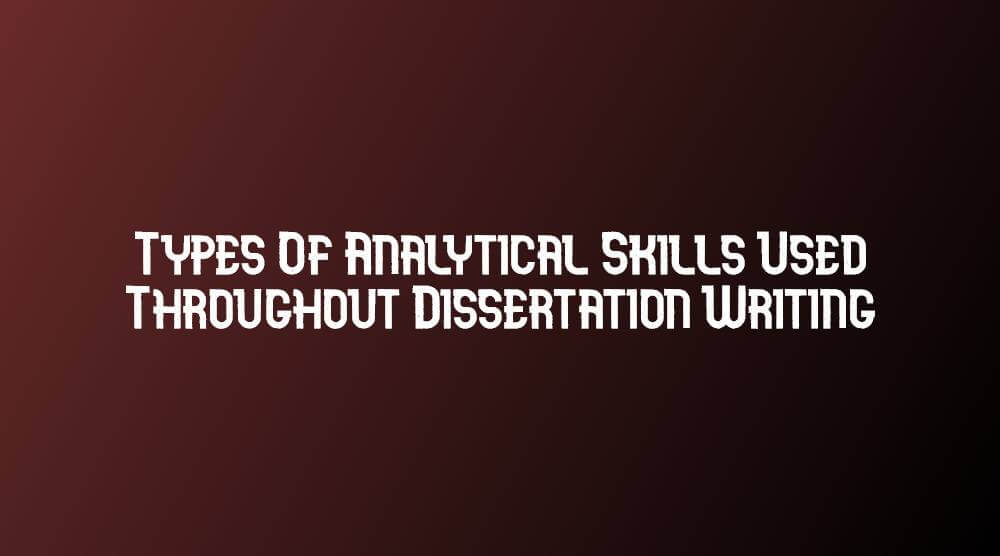
Dibble’s Reduction Thesis: Implications for Global Lithic Analysis
- Open access
- Published: 07 May 2024
- Volume 7 , article number 12 , ( 2024 )
Cite this article
You have full access to this open access article
- Michael J. Shott ORCID: orcid.org/0000-0002-6592-4904 1
289 Accesses
Explore all metrics
Harold Dibble demonstrated the systematic effects of reduction by retouch upon the size and shape of Middle Paleolithic tools. The result was the reduction thesis, with its far-reaching implications for the understanding of Middle Paleolithic assemblage variation that even now are incompletely assimilated. But Dibble’s influence extended beyond the European Paleolithic. Others identified additional reduction methods and measures that complement Dibble’s reduction thesis, and applied analytical concepts and methods consistent with it to industries and assemblages around the world. These developments facilitated comprehensive reduction analysis of archaeological tools and assemblages and their comparison in the abstract despite the great diversity of their time–space contexts. Dibble argued that many assemblages are time-averaged accumulations. In cases from New Zealand to North America, methods he pioneered and that others extended reveal the complex processes by which behavior, tool use, curation, and time interacted to yield those accumulations. We are coming to understand that the record is no mere collection of ethnographic vignettes, instead a body of data that requires macroarchaeological approaches. Archaeology’s pending conceptual revolution in part is a legacy of Dibble’s thought.
Similar content being viewed by others
Urie Bronfenbrenner’s Bioecological Theory: Its Development, Core Concepts, and Critical Issues
Heritage as a Focus of Research: Past, Present and New Directions
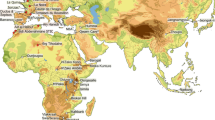
What function(s) for Palaeolithic polyhedrons, spheroids and bolas? Cases from France and North Africa
Avoid common mistakes on your manuscript.
Around 1980, Harold Dibble began a career that examined sources of variation in Middle Paleolithic industries, mostly in France and southwest Asia. His untimely death in 2018 could not diminish the scale and impact of Dibble’s contributions to Paleolithic archaeology. Other contributors can testify to his stature in that field. As an archaeologist who cannot tell a Quina scraper from a chapeau de gendarme platform, my task is different: to sketch some of Dibble’s broader contributions to lithic analysis beyond Paleolithic studies, and especially to emphasize several current lines of thought and practice that at once derive in part from Dibble’s work but extend beyond it. This essay does not pretend to be comprehensive evaluation of Dibble’s oeuvre, merely to trace the extent of some of its parts, on the logic that a scholar’s work can be gauged partly by surveying how others use and expand it.
Experimental Controls and Key Variables in Fracture Mechanics
In a series of highly controlled experiments over several decades, Dibble and students demonstrated systematic effects of the fracture mechanics of reduction upon the size and shape of flakes struck from cores. Results synthesized by Li et al. ( 2023 ), this experimental program identified variables, mostly continuous, that were independent (e.g., platform dimensions) and dependent (e.g. length, mass or volume) in the fracture mechanics of flake production. The program established a framework for study of variation in flake size and shape. Experiments’ designs showed the limited effects of unobservable variables like angle and force of blow, suggesting that observable independent variables could predict original size of flake tools.
Results may seem narrow but these experiments had very broad implications indeed. By itself, inferring flake size and shape has modest value; for the great mass of unretouched flakes, it has none at all, because these dimensions can be measured directly and require no inference. But the results have great value in the study of flake tools that underwent resharpening between first use at larger size and discard at smaller size. In that context, Dibble’s experimental program identified independent or causal variables, again mostly continuous, like platform area and mathematically expressed their effects upon dependent continuous variables of size and shape. This insight made it possible to predict original flake size from properties like platform area that are retained on many retouched flakes. To the extent that flake tools were smaller at discard than experimental controls predicted, reduction from resharpening or other reasons is implicated. To the further extent that shape changed as size declined, variation in flake-tool shape may be a by-product of reduction, not a reflection of intended original form. Enter the reduction thesis.
The Reduction Thesis
With some ethnographic support (see citations in Dibble et al., 2017 :823), Dibble’s work showed that many—not all—stone tools varied substantially and systematically between first use and discard. Trivially, they only could become smaller, not larger, but tools and types varied greatly in degree and pattern of reduction experienced and the range of intermediate forms they took between first use and discard. Size and shape at discard are observable directly, but Dibble’s contribution was to demonstrate that, for many retouched tools, remnant unchanged segments of the original detached flake (e.g., platform variables) furnished estimates of original size. Thus, arose the reduction thesis (Shott, 2005 ; Iovita’s, 2009 :1448 “reversed ontogenies”).
Lithic analysts readily appreciate the importance of inferring original size of retouched and therefore reduced archaeological specimens. Again, by itself the knowledge is modest. But it looms larger in the context of Middle Paleolithic studies, where tool types were regarded as Platonic essences based on particular configurations of their form, and placement and extent of retouch qua reduction. Alternatively, as Dibble ( 1987 ) suggested, the pattern and degree of reduction by retouch allowed large flake tools to transit from what seemed one essential Middle Paleolithic type, often one or another variety of scraper, through a second, possibly to a third, and so on. For example, Middle Paleolithic backed knives experienced “transformations from one morphological Keilmesser form to another” (Jöris, 2009 :295) as a result of resharpening to maintain functional edges. If so, tool form at discard reflects not original design, least of all size, but “the last stages of a series of metamorphoses” (Jelinek, 1976 :27) of original form, as Dibble’s mentor put it. In this perspective, the ontological validity of essentialist Middle Paleolithic tool types is in doubt, and to some typology has passed from analytical to descriptive enterprise. Assuming that the form in which a tool was discarded was its intended, unchanging form is Davidson and Noble’s ( 1989 ) “finished-artefact fallacy,” rephrased by Dibble et al. as “the fallacy of the ‘desired end product’” (2017:814). In North American practice, it also has been called the “Frison effect” (Frison, 1968 ).
Dibble’s insight later was expanded in three respects:
The reduction thesis applied to cores as well as tools, for instance, in Dibble’s ( 1995 ) analysis of the Biache St.-Vaast Level IIA reduction sequence that demonstrated how core form and scar-patterning varied with their degree of working. (Throughout this essay, “reduction sequence” indicates the patterned ways that cobbles were reduced in the process of shaping them into tools or detaching flakes from them for use as tools, and subsequent reduction by retouch of core and flake tools, usage consistent with Dibble [e.g., 1995 :101]. This is not the place to address the contested issue of how or whether the reduction-sequence concept, originating over a century ago in North America, differs from the more recent and, in Paleolithic studies, more popular “ chaîne opératoire ”; interested readers may consult Shott [ 2003a ].)
It explained variation in Paleolithic flake-tool types besides scrapers, e.g., notched flakes (e.g., Bustos Pérez, 2020 ; Holdaway et al., 1996 ; Roebroeks et al., 1997 ). It also was applied to extensively or completely retouched, quasi-formal tools like Acheulean handaxes (McPherron, 1994 ) in Africa and Europe, European Middle Paleolithic bifaces (Serwatka, 2015 ) and Upper Paleolithic endscrapers (Morales, 2016 ), late Paleolithic core tools in Southeast Asia (Nguyen & Clarkson, 2016 ), Middle Stone Age Aterian points (Iovita, 2011 ) and Still Bay points (Archer et al., 2015 ) in Africa and unifacial and bifacial points in northern (Hiscock, 2009 :83) and western Australia (Maloney et al., 2017 ). Such analyses linked in the same tool-use and -reduction sequences what initially were defined as distinct types (e.g., Middle Paleolithic Keilmesser handaxes and leaf points [Serwatka, 2015 :19] and late Paleolithic core tools such that “various tool types are viewed as points or stages along a trajectory of continued reduction, rather than as discrete or separate types as in a segmented and discontinuous scheme” [Nguyen & Clarkson, 2016 :38]).
Largely implicit in Dibble’s work, reduction is or at least can be understood as a continuous process.
Expansion of the reduction thesis itself is significant in two further respects. First, it suggested the argument’s universal scope, the recognition that stone tools of all times and places can be subject to systematic transformations during use. What began, then, as an effort to understand variation in Middle Paleolithic flake tools might apply to stone-tool variation of any age, any industrial character, anywhere. In this perspective, the thesis can “put the analysis of tool’s live [sic] histories in a global and standardized framework to interpret the organization of past societies” (Morales, 2016 :243). Second, and starting from studies of Acheulean handaxes (McPherron, 1994 ), the reduction thesis engaged the concept of allometry to explain variation in stone tools. (Crompton and Gowlett introduced allometric analysis to Paleolithic research, defining allometry somewhat broadly, as “size-related variability” [1993:178]. No doubt suitable for their purposes, allometry is best understood as a biological concept—change in shape with change in size—and process that unfolds during growth to maturity. In lithic studies, obviously, the direction of size change is reversed; there, the allometric process unfolds during reduction. In biology where the concept originated and in lithic studies more generally, allometry measures the degree and strength of shape’s dependence upon size variation. Although Crompton and Gowlett found allometric variation at Kilombe, they explained it in functional, i.e., design, terms, not as the product of reduction.) Allometry is an inherently continuous process that requires measurement in continuous terms. Allometric variation certainly describes some aspects of the morphological transformations of Middle Paleolithic flake tools wrought by the reduction process. But it is especially pertinent to the analysis of extensively retouched tools, Paleolithic or otherwise, whose distinctive forms and time–space distributions make them markers of industries or cultures. Expansion of the reduction thesis, therefore, is particularly relevant in archaeological contexts that abound in such tools, not least the Americas.
Besides pertaining to many Paleolithic and other defined tool types and besides its invocation of allometry, the reduction thesis bears upon other theoretical and methodological matters. It engages the concept of tool curation and encompasses the methodology of tool failure or survivorship analysis. It has implications for long-term accumulations that help to disentangle the complexities of the formation of stone-tool assemblages. It begs—and can help answer—a deceptively complicated question about stone-tool quantification. Finally, it can contribute to the intellectual transformation that archaeology desperately needs, a “macroarchaeology” (Perreault, 2019 ) that eschews ethnographic dependency, studies archaeological units in their own terms with their own long durations and applies uniquely archaeological theory to explain their time–space variation. All of this from experiments on the fracture mechanics of flakes and their implications for Middle Paleolithic flake tools. The following sections untangle and address some threads of the reduction thesis.
The reduction thesis has far-reaching implications, one of course that concerns the integrity of French Middle Paleolithic scraper typology. Tool types from scrapers to notches may not be the Platonic essences sometimes assumed (e.g., like Dibble [ 1987 ], Bustos Pérez’s [ 2020 :Table 43] and Roebroeks et al.’s [ 1997 :148 and Figs. 17–18] observations that varieties of Middle Paleolithic scraper types transit via reduction to varieties of notch and denticulate types). If so, the reduction thesis reveals Middle Paleolithic Mousterian assemblage variation not as a chronicle of the “acrobatic manoeuvering of…typological tribes” (Clarke, 1973 :10) signaling their self-conscious identity by fixed tool form and assemblage composition as they alternate between rockshelters. Instead, variation might be a record of adaptive behavior, when freed of the constraint of subjectively defined “technocomplexes” (Monnier & Missal, 2014 ; cf. Faivre et al., 2017 ).
Significantly, Dibble’s thesis applies, as above, to more formal Paleolithic tools types and equally to other areas and research contexts. As examples of the reduction thesis’s even broader scope, Dibble’s argument echoes in the variation exhibited by Hoabinhian and other Southeast Asian industries (Marwick, 2008 ), in a comprehensive revision of the causes and meaning of variation in Australian flake tools (Hiscock & Attenbrow, 2005 ), in Hoffman’s ( 1986 ) concern that a range of Holocene North American point “types” defined using traditional approaches (what Maloney et al., 2017 :43 called “ad hoc” classification) capture merely various degrees of reduction of a single original type (reading Fig. 1 from Stages B to E; see Hamsa [ 2013 ] for a similar conclusion from a different sample in a different North American region), in New World Paleoindian points (Suárez & Cardillo, 2019 ; Thulman et al., 2023 ), and in the need to identify original sizes and shapes of distinct Holocene Patagonian point types whose forms converge in reduction (Charlin & Cardillo, 2018 ).
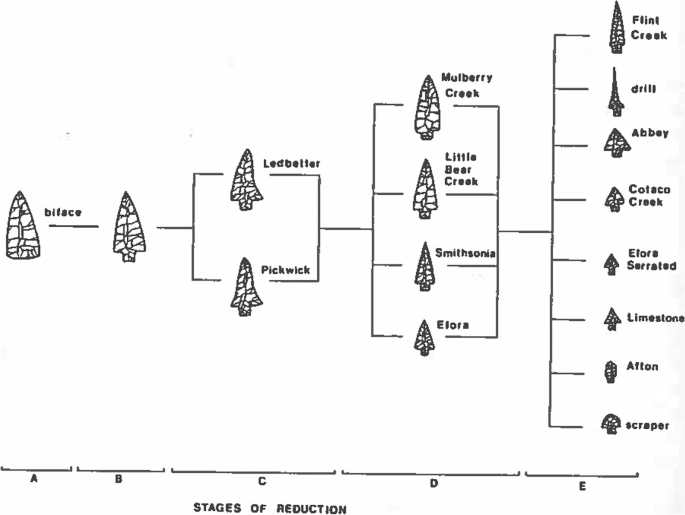
(Source: Hoffman, 1986 :Fig. 5)
Reduction’s effect upon typology. A single original bifacial tool type (far left) retouched in varying pattern and degree ( x -axis) yields several apparent “types” (far right)
Reduction as Continuum
If tools undergo continuous reduction then, ipso facto , reduction is a continuum. Increasingly, reduction’s continuous nature is assimilated to European Paleolithic practice, with productive results applied to flake tools (e.g., Iovita, 2009 ; Morales, 2016 :236; Serwatka, 2015 ). What goes for tools goes for debitage; since the reduction thesis arose, lithic analysts have modeled reduction’s entire span in continuous terms. Dibble’s work on these lines parallels, not presages, research elsewhere, particularly in North America. As there, he questioned typological approaches to flake analysis that involved subjective judgments of selected products and favored detailed measurements of full ranges of flake classes (e.g. Dibble, 1988 ). (See Shott, 2021 :57–70 on the comparative merits of typological and attribute methods in flake analysis.) By engaging the full range of materials in the Biache St.-Vaast Level IIA assemblage and recording dimensions and other continuous measures, for instance, Dibble ( 1995 ) showed that cores themselves exhibited systematic, size-related variation according to degree of reduction, and that resulting flakes also patterned by size regardless of the supposedly distinct types to which some of each were assigned. In this way, “Dibble was able to show that relying solely on scar pattern analysis of cores and some Levallois products was not suitable for studying the dynamics of a reduction strategy” (Wojtczak, 2014 :26). The continuous nature of this reduction process largely remained implicit in Dibble’s treatment, yet is apparent upon close reading.
Reduction sequences, that is, are continuous because the size, shape, and technological properties of cores and even unretouched flakes vary continuously along the reduction continuum from the first to last flake detached from a cobble. Some still question a continuous view of reduction, arguing for instance that “the dichotomy between ‘discrete’ vs. ‘continuous’ is difficult to place on neutral grounds – lithic scholars rarely come up with convincing means to evaluate the alternative to their preferred view” (Hussain, 2019 :243). This view relates stances—reduction as continuum or successive, discrete stages—to distinct ontological first principles incapable of comparative evaluation. Indeed, to some “Stage-like descriptions of technological choices are the hallmark of” traditional French systematics (Anghelinu et al., 2020 :35). If so, the question of reduction as continuous or staged becomes a matter of a priori predilection rather than reasoned inference, metaphysic more than logic.
Yet precisely such evaluations of competing alternatives have been made, testing a priori stances rather than merely choosing between them. Dibble ( 1988 ) tested a stage-based thesis of “predetermination” in Levallois reduction. He showed instead that a wide range of reduction products varied continuously among and between themselves, a result inconsistent with stage views. Analyzing experimental flake assemblages, Bradbury and Carr ( 1999 ) found no evidence for reduction “stages,” and expressed relative order of flake detachment (from 0 to 100% of core reduction) as a joint, continuous, function of faceting measures and flake size. A later study systematically tested key implications of both “stage” and “continuum” views in experimental data, again finding no support for the validity of stages and extensive support for the continuous alternative (Shott, 2017 ). A complementary approach supplements attribute recording with mass analysis and involves flake-size distributions that, in the same experimental assemblage, vary predictably between successive segments defined arbitrarily or, for instance, by change in hammer. When such assemblage segments hypothetically are “mixed” in various combinations, they model the mixing that characterizes empirical flake assemblages accumulating over long periods. Using suitable methods—in this case, constrained least-squares regression—the approach offers the prospect of disentangling—unmixing—empirical assemblage accumulations. Applied to two large North American Great Basin quarry assemblages, it identified mostly early but also intermediate segments of reduction that varied continuously across contexts and between assemblages (Shott, 2021 :98–103), complex mixing and variation that rigid “stage” approaches could neither detect nor characterize. Thus, individual reduction sequences and their products can be understood in continuous terms, as can the complex mixing of many reduction sequences in archaeological accumulations. Again, the continuous nature of the reduction process mostly was implicit in Dibble’s work, but clearly his approach paralleled those taken elsewhere and led to similar conclusions.
Allometry and Modularity
Typically, continuous flake-tool reduction produces allometry; some segments—usually distal and/or lateral edges—are reduced while others—usually butts or platforms–remain unchanged. Shape changes as size declines, i.e., allometry. Shape changes because various distinct segments— modules —of flakes are retouched to varying degrees or not at all. Hence, the reduction thesis views even humble flakes as composites of modular parts. Because it draws an analytical distinction between segments qua modules of flakes, the thesis encompasses allometry and modularity as latent properties, made explicit in recent applications of landmark-based geometric morphometrics (GM) to flake assemblages (Knell, 2022 ).
Allometry can be analyzed using tool dimensions like length and thickness (e.g., Crompton & Gowlett, 1993 ). Yet GM methods are particularly suited to analysis of allometry. GM itself is an innovative way to characterize and measure stone tools. GM methods are not “size-free” (cf. Caruana & Herries, 2021 :92) in the sense of separating all variation in size from all variation in shape. Rather, they separate shape variation that is independent of size from shape variation that is size-dependent (Shott & Otárola-Castillo, 2022 :95). As a result, GM methods can be instrumental to allometric analysis, not obstacles to it.
GM facilitates allometric analysis only by defining modules, segments of larger wholes whose landmarks vary more internally than they do with other modules of the same points. The modularity concept originated in biology, modules there comprising distinct anatomical segments like wings or limbs. As above, though, Dibble’s experiments and the reduction thesis arguably preadapted lithic analysis to receive it. Among Paleolithic flake tools, one salient modular distinction is between platforms, which may change little during use and retouch, and distal segments, which may be extensively retouched. Other modules can be defined and their correlations studied depending upon the research focus. In Western Hemisphere bifacial points, an equally salient distinction is between stems and blades as separate modules (e.g., Shott & Otárola-Castillo, 2022 ; Thulman et al., 2023 ), again not the only conceivable modular subdivisions. (For instance, Patagonian Bird Type IV-V points support a tip-versus-rest-of-point modularity [González-José & Charlin, 2012 ], and point margins also can function as modules.) In this perspective, allometry occurs by changing size proportions among modules as functions of overall specimen size. Archaeological GM analysis transforms stone tools from integral wholes to things of complementary parts—modular constructions—in complex interaction. In the process, it invokes a concept of modules implicit in Dibble’s experiments.
Curation and Its Distributions
Pattern and especially degree of reduction reflect the practice of curation. Originating with Binford ( 1973 ), the curation concept was (Hayden, 1976 ; Nash, 1996 ; Odell, 1996 )—still is, in some quarters—fraught with ambiguity. Yet a consensus has emerged that views curation as a continuous property of individual tools, not a categorical trait of entire assemblages or industries (e.g., Morales et al., 2015b :302). It expresses the ratio between realized and maximum utility (Shott, 1996 ), calculated in subjects like retouched stone tools as the difference between size at first use and at discard, usually on a 0–1 scale. Thus, curation of retouched flake tools scales as the difference between each tool’s size at detachment (or modification in preparation for first use) and at discard. As above, size at discard is a simple matter of observation but Dibble and colleagues’ experiments permitted inference to size at detachment. Hence, Dibble’s experimental results are key to the measurement of curation.
Again tools, not assemblages or industries, are curated (Shott, 1996 ), and specimens of a single type can be curated to varying degrees. Of course their original size, shape, and production technology are important properties of tools and their types. But the reduction thesis underscores the equal importance of the characteristic patterns and degrees of reduction that tools of any type experienced. Reduction is inherent in stone-tool curation, so must be measured. Analysts have devised a range of measures, mostly geometric or allometric (e.g., Morale et al., 2015a ; Shott, 2005 ). So many reduction measures demand criteria for their evaluation (Hiscock & Tabrett, 2010 ) and, considering their diversity and varying statistical properties, may even reward synthesis as pooled or “multifactorial” measures derived from ordination methods (e.g. Shott & Seeman, 2017 ).
At any time, each person has a single value for age, trivially. The populations they comprise do not have discrete ages. But they can be characterized by their age distributions, the number or proportion of individuals at each age or pooled intervals of age from birth to greatest age. Similarly, each retouched tool has a single, individual, curation value. But when numbers of tools of any type are analyzed (types necessarily being defined before compiling curation distributions to avoid the mistake of conflating ranges of reduction and curation [e.g., the limited curation of “single scrapers” versus the more extensive curation of “double scrapers”] with distinct types), the resulting range and relative frequency of reduction values are population properties of the type. Ranging from unretouched to extensively reduced specimens, tools’ reduction values form curation distributions for the types. Such distributions plot the fate of any number x of specimens of a type similar or identical in original size and shape as they undergo varying patterns and degrees of reduction. Fractions of x experience discard at progressive intervals along the range of curation from larger original to smaller discarded size and shape. Across a range of specimens of the type, degree of reduction (ascending on the x-axis in Fig. 2 ) leaves fewer survivals (cumulative survivorship descending on the y-axis there). Figure 2 shows distributions for two variants of reduction indices computed from the same set of North American Paleoindian unifacial scrapers (LT1NP, LT2NP which, for illustration only, are treated here as separate distributions) and one for reduction of a replicate scraper (LTMorrow). (See Sahle & Negash, 2016 :Fig. 5 for similar distributions characterizing Ethiopian ethnographic scrapers.) Reduction distributions may indicate high (LT1NP) or comparatively low (LTMorrow) curation. Empirical distributions can reveal differences that certainly are continuous and sometimes are subtle.
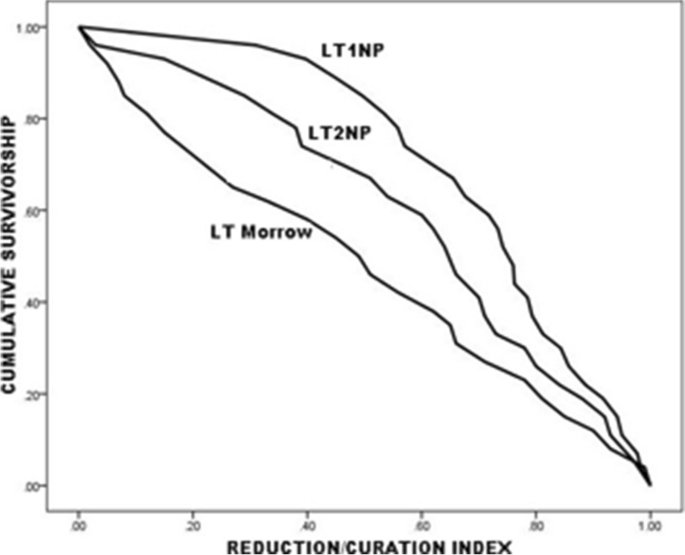
(Source: Shott & Seeman, 2015 : Fig. 5)
Reduction distributions plotting cumulative survivorship (descending on y -axis) against degree of curation (ascending on x -axis). Upper, convex distribution (LT1NP) indicates high curation, most specimens surviving until they experience extensive reduction. Lower, less convex distribution (LT2NP) indicates lower curation by continuous degree, more specimens discarded at low to modest degree of reduction. Distribution of experimental replica (LTMorrow) indicates lowest curation by comparison
Whatever their form, curation distributions are properties of tool types no less integral than their original design (Iovita, 2009 ). The variation they exhibit itself has analytical value. For instance, reduction distributions correlate degree of utility extracted to varying hunting return rates, making curation a behavioral variable that tracks long-term adaptations (Miller, 2018 :55–63). They can be fitted to failure models like Weibull that gauge their scales and shapes and identify causes of discard in experimental assemblages (Lin et al., 2016 ), and among Upper Paleolithic Iberian endscrapers (Morales, 2016 ; Morales et al., 2015b :302–303) and late Pleistocene North American scrapers (Shott & Seeman, 2017 ). Differences between distributions beg explanation, perhaps by industrial variation in Paleolithic assemblages or by changing access to toolstones, varying land-use scales or technological organization, changing population density or sociopolitical organization in assemblages anywhere. In this way, the reduction thesis creates variables by which to explain prehistoric behavior.
Assemblage Formation
Curation rate itself arguably measures relative use-life of tools (Shott, 1996 ). In turn, use-life is a key quantity in assemblage-formation models, along with tool-using activity rates and “mapping relations” (how types “map onto” functions or uses) (Ammerman & Feldman, 1974 ). Tool-use rates and “mapping relations” establish the functional or activity correlates of tool use. They contribute to assemblage variation, but are irrelevant in the following discussion that holds them constant in order to illustrate how curation and use-life alone can generate assemblage variation. Curation, which can be estimated in stone tools from the reduction thesis, and use-life thereby extend the reduction thesis’s scope beyond individual tools to the size and composition of entire assemblages as time-averaged accumulations.
Use-life is measured in time, and assemblages accumulate in time, a truism but one with important implications. Assemblage size increases, ceteris paribus , with time, therefore with accumulation span. But assemblage composition also changes as size increases, even holding tool-use activity rate and mapping relations constant, if tool types vary among themselves in use-life. How and why this occurs is explained elsewhere (e.g., Schiffer, 1975 ; Shott, 2010 ). Relevant here is that the composition of assemblages—presence or absence of and, if present, proportions of, various tool types—can vary strictly as a function of time and the accumulation of discarded specimens; assemblage size and composition are not always, possibly not often, independent quantities, composition instead changing with size up to an equilibrium point determined by the relationship between accumulation span and tool-type use-lives. When assemblage composition (as richness—number of types present—or other measures like heterogeneity) is plotted against assemblage size, either between assemblages or in bootstrap sampling within an assemblage, a positive linear relationship can result, up to the equilibrium point beyond which composition changes little. Before that point, assemblage composition has not stabilized for use-life and assemblage-size effects; beyond it, composition is stabilized with respect to those effects.
The reduction thesis bears directly upon assemblage formation only in helping to reveal types’ relative use-lives. But because the thesis demonstrates that some Paleolithic “types” like single scrapers are not types at all but merely modestly reduced versions of the legitimate type “flake tool,” indirectly it also helps explain some patterns of assemblage variation. For instance, assemblage size-composition correlations are documented in contexts as diverse as the French Middle Paleolithic (Shott, 2003b ), the North American Paleoindian (Shott, 2010 ) and late prehistoric New Zealand (Phillipps et al., 2022 ). As one example, Olduvai Paleolithic flake-tool “types” can, like Middle Paleolithic ones, be linked as segments of cobble reduction sequences (Potts, 1991 ); they are not legitimate types. Bootstrapped plots of richness, a composition measure, against number or size distinguish assemblages there whose size-composition relationships had stabilized (Fig. 3 a, FLK1-2) from those that had not (Fig. 3 b, HWK-1)(see Shott, 2003b :142–143 for similar treatment of French Middle Paleolithic assemblages).
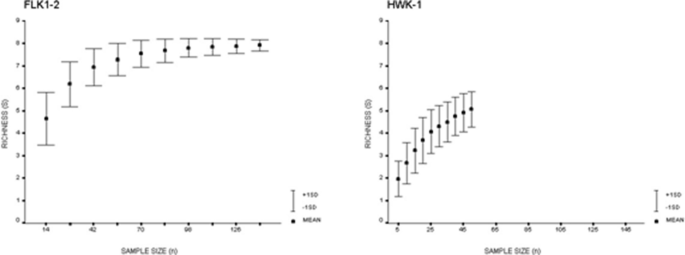
Examples of bootstrap gauging of richness adequacy and standard deviation in Oldowan assemblages. a FLK1-2, adequate because empirical size is sufficient to stabilize richness and narrow standard deviation; b HWK-1, inadequate because richness fails to stabilize and standard deviation to narrow before reaching empirical size (Data source: Leakey, 1971 )
Similarly, Dibble argued that a Middle Paleolithic scraper’s “type” registers not its Platonic essence—single, double and convergent scrapers are not legitimate, distinct types but merely segments of the reduction continuum of the legitimate type “flake tool”–but its curation rate and, ceteris paribus , relative use-life (Lin, 2018 :1791). Dibble and Rolland ( 1992 :11) defined “intensity of occupations” in part as the ratio of Bordean scrapers to notches. In size-stabilized assemblages, Bordean single scrapers correlated inversely with the intensity ratio, double scrapers positively at high slope or rate, convergent ones positively at lower rate. The reduction thesis explains this size-composition pattern; single scrapers first must become double scrapers before they might become convergent ones. Both double and convergent scrapers can be transformed single scrapers, but double scrapers are transformed sooner because they form directly from single scrapers. As a joint probability of transformation-by-reduction first to double scraper and only later, possibly, to convergent scraper, a lower proportion of convergent scrapers is a highly probable arithmetic consequence of the reduction thesis. Scraper “types” considered as successive segments of a reduction continuum of a single flake-tool type increase proportionally in size-stabilized assemblages as measured by Dibble and Rolland’s scraper:notch ratio of occupational intensity because the ratio measures increasing scraper use and discard (Shott, 2003b :145 and Fig. 11.9). Recognition of such size-composition correlations also contributed to one of Dibble’s and colleagues’ later arguments (e.g., Dibble et al., 2017 ) that surface assemblages may be time-averaged palimpsests revisited as.
Quantification
Assimilating several components of the reduction thesis—its prevalence, resulting allometric variation, curation rates and their connection to use-life, and assemblage size-composition correlations—begs a question that appears trivial at first glance: how much is a tool? In limited respects, this question was broached years ago (e.g., Hiscock, 2002 ; Shott, 2000 ), chiefly to improve and standardize assemblage characterization for comparative analysis. Applied to a Syrian Middle Paleolithic assemblage, for instance, several measures of original number of specimens yielded generally concordant results, best among them considered total length of all intact and broken specimens combined divided by mean length of intact tools at discard (“TLV 1”) (Wojtczak, 2014 :63–72).
We regard tools as integral wholes not only for purposes of typological assignment and various analytical approaches, but also for counting. Leaving aside the fragmentation that further complicates quantification, for counting purposes one Quina scraper or one Early Side-notched (ESN) point, to use a North American example, is as much as another, no more or less: it’s one. But recognizing that many tools are subject to reduction of varying degree and pattern, whether or not they transit between types in any taxonomic system, we might change our perspective. A newly minted, large ESN point (Fig. 4 a) is, trivially an ESN point. But is it as much of an ESN point as a heavily resharpened stub (Fig. 4 e)? More? Less? Is the large, new point “one,” the reduced stub much less than one? Alternatively, is the latter, owing to its extensive use, more than one mint-condition ESN point? Questions so abstruse may seem unworthy of consideration. Yet if assemblages reflect, at least in part, patterns and frequencies of past activities, then not all ESN points register the same amount, or necessarily kind, of activity. For the study of original design, the specimen shown in Fig. 4 a is more than that shown in Fig. 4 e; as registers of use, Fig. 4 e is much more of a tool than is Fig. 4 a. The reduction thesis is essential to the calibration of tool occurrence to past design and behavior, in part by linking amount of use to degree or pattern of reduction.
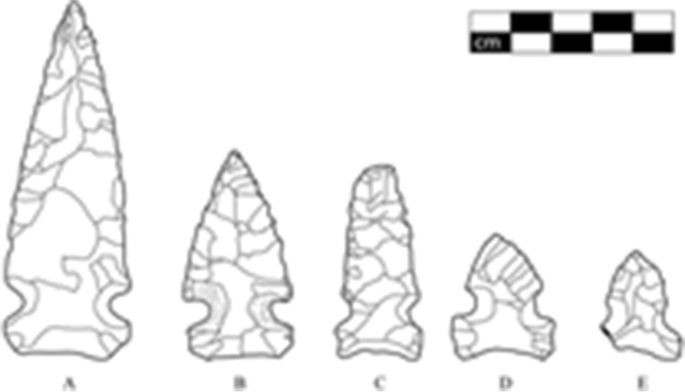
(Source: Randall, 2002 :Fig. 4.2)
Reduction sequence of North American Early Side-notched points, a–e representing progressive intervals of reduction
- Macroarchaeology
Fitfully, archaeology is evolving as a scholarly discipline. In the mid-twentieth century, essentially it was culture history. Later, American archaeology became a functional or ecological anthropology, later still a postmodern critique of whatever postmodernists disliked, latterly a forum for identity construction and defense. Archaeology can be all of those things; it also can be a science of the human past, a possibility that encompasses at least part of all such approaches save postmodernism.
Dibble practiced a scientific archaeology, although not exactly as conceived by Perreault’s ( 2019 ) “macroarchaeology” that extensively revises the field’s ontology. Yet despite macroarchaeology’s breadth, even the limited domain of the reduction thesis and the study of stone tools bear upon it. For instance, objects like stone tools and their attributes are directly observable. But so trivial a statement obscures important implications. In macroarchaeological perspective, objects and the attributes they possess are “primary historical events” or units (Kitts, 1992 :136), of a time–space scale commensurate with individual observation and experience. Anyone can observe an object in production or use today, and lithic analysts can directly examine a prehistoric stone tool. The theory required to explain objects and their attributes, be it technological, functional, symbolic or social, and how they serve their broader cultural context, be it material (e.g., behavioral ecology), symbolic, structural, or social (e.g., agency, Marxism), is suitable to primary historical units, i.e., of a time–space scale commensurate with individual experience. Such theory explains the actions of individuals or social groups at moments or short intervals in time; it is historical (e.g., the movement of populations, the rise or decline of complex societies), material (e.g., environmental change, adaptation), or ethnographic. Little or none is unique to archaeology or its customary time–space scales.
Tool types are defined by repetitive patterning in attributes across specimens. Industries or assemblages of specimens of various types are defined by joint patterns of use and deposition. Types and industries or assemblages, and the cultures constructed from them, are bounded empirically by their time–space distributions. Types may occur over broad areas and persist for generations or longer, and their distribution at any moment surpasses the scale of individual experience. Pompeiis excepted, industries or assemblages are time-averaged over at least years, usually much longer. Neither types, assemblages, and cultures, or their time–space boundaries, are primary historical units. Types persist, and assemblages and industries accumulate, at time scales orders of magnitude greater than ethnographic or historical contexts. They are secondary historical events or units that “have no counterpart in the present…[and] are composed of primary events related in a spatial and temporal nexus” (Kitts, 1992 :137). As secondary units, types and assemblages possess properties that are emergent at the lower level of primary events—not deducible from the properties of units at that level—and that require “explanatory principles emergent with respect to” (Kitts, 1992 :142) them. Secondary units’ salient properties must be constructed from the material record. Units’ origins—how and why types or other secondary units arise, according to what causes–and behavior—their duration, changing incidence or distribution over that span, how and why they end, either by termination, transformation or branching—can be explained only by theory that pertains to their nature and time–space scales as secondary historical units. No other discipline has or needs such theory; archaeology has yet to develop it for its own purposes. Here lies its greatest challenge: conceiving the method and theory to define and explain the character and behavior of secondary historical units.
Perreault argued that the time–space scale that defined secondary historical units compromise the application of explanatory theory based on primary units, that the archaeological record was underdetermined by such theory (2019:29–32). Then he posed questions that limn the macroarchaeological challenge, some pertinent to lithic studies and the reduction thesis (2019:169–173). Merely as examples relevant in this context, macroarchaeological questions include the following examples. Do tool types or the industries they form and the reduction sequences that produced them trend in complexity over archaeological time? If so, why? Are types’ or industries’ rates of change related to that complexity, to population size, even to curation rate if, like biological taxa whose evolutionary rates are proportional to individual lifespan, higher curation implies fewer instances of replication? What explains why and how tool types, industries or other constructs originate and, crucially, why and how they end? No current theory–from behavioral ecology to evolutionary archaeology to any prehistoric equivalent of Annales to archaeology of the long term–approximates the macroarchaeological approach that Perreault advocates.
Of course macroarchaeology far surpasses the scope of the reduction thesis, which nevertheless has relevant implications for its development. The thesis promotes typological hygiene and thereby the definition of valid types qua secondary units. It distinguishes resharpening allometry and the modularity on which allometry rests from typological variation. Degree and pattern of allometry measure curation rate; the latter then becomes, as above, a continuous attribute of types as secondary units. Through its effect upon assemblage formation and accumulation (e.g., the size-composition effects noted above), the thesis links the composition of assemblages or industries as secondary units to the composition of tool inventories at the level of primary units.
Even if most of Dibble’s work did not attempt the shift in scale and focus that Perreault’s macroarchaeology entails—no one has, to this point—he helped establish knowable, replicable—positivist—foundations for scientific inference from the material record. And Dibble et al.’s ( 2017 ) accumulations view takes a limited macroarchaeological perspective on the formation and transformation of assemblages. Until macroarchaeology prevails, we will continue to define the wrong units at the wrong scales whose nature and behavior we try to explain using the wrong theory. The reduction thesis has a role, admittedly modest, in this necessary transformation.
Reception of the Reduction Thesis
The reduction thesis rejects the view of Paleolithic tool types as Platonic essences. Being a powerful explanation for considerable variation in lithic industries and assemblages, as sketched above, it has earned broad if uneven acceptance, particularly in New World and Australian archaeology. Ironically, that reception is conspicuously uneven in European Paleolithic archaeology, where the thesis originated. If to some there the reduction thesis is “reasonably demonstrated” (Anghelinu et al., 2020 :37), others dismiss or ignore it. Despite noteworthy exceptions, my outsider’s impression is that many, possibly most, European Paleolithic scholars remain unpersuaded by, or indifferent to, the reduction thesis and its far-reaching implications for our understanding of the past.
No doubt the number of such scholars and the breadth of their practice surpass any simplistic opposition between views of Paleolithic tool types as Platonic essences or mere domains of nominal variation (Marwick, 2008 :109), of French versus American paradigms (Clark, 2002 ), of Bordes’s facies qua cultures versus Binford’s toolkits. Nor can an outsider like me command the relevant literature or be attuned to possibly subtle changes in approach or ontology in Paleolithic studies. But even recent efforts to reconcile or synthesize approaches betray a strong predisposition toward essentialism (e.g., Hussain, 2019 ; Reynolds, 2020 :193; cf. Anghelinu et al., 2020 , whose attempt at synthesis deserves close study). Even if, then, Dibble’s reduction thesis is a figurative prophet with highly uneven honor in its field of origin, it has transformed the analysis of stone tools in other contexts.
One Recent Example of Dibble’s Influence
Many Dibble students and colleagues are recognizable by the nature and quality of their work, itself one of his greatest legacies; you know who you are, Holdaway, Iovita, Li, Lin, McPherron, Monnier, Olszewski, Rezek and others. But Dibble influenced many more.
As one example among many, my current collaborative project involves GM allometric analysis of a fairly large sample—over 5000—midcontinental North American points catalogued from private collections that form a time sequence that spans more than 10,000 years of prehistory (Nolan et al., 2022 ). The reduction thesis and its implications, sketched above, are integral to our analytical approach. We can chart time trends in curation rates, allometric trajectories and degrees of modularity and integration in our dataset (e.g., Shott et al., 2023 ), and relate these properties of secondary historical types to environmental, demographic, or sociopolitical trends at suitable time–space scales. Certainly in its current form, this project would be inconceivable without Dibble’s work. In prehistoric archaeology, Dibble’s influence extends well beyond the Old World Paleolithic. In theoretical terms, it extends well beyond the fracture mechanics of brittle solids.
This essay began with flakes and ended at some of the greatest ontological challenges confronting archaeology today. In the process, it discussed other archaeologists’ practice as much as Dibble’s. That is at once deliberate and meant as praise. Dibble’s own interests lay in important details of fracture mechanics and in Middle Paleolithic archaeology, as well as field-recording and database management. Yet implications of his work were explored and elaborated in time–space contexts that far surpass the Middle Paleolithic. Today, we can devise reduction measures suitable to a range of tool types and practice typological hygiene by distinguishing continuous or categorical variation between types from continuous allometric reduction variation within them. We can gauge that allometric variation in the context of varying integration of modular segments of stone tools. We can derive curation distributions, measure their properties in detail and compare variation among types or periods. We can begin to probe the complexities of assemblage formation, the persistent correlation between assemblage size and composition. We can pose and begin to address deceptively profound questions like “How much is a tool?”. We even can contemplate needed, macroarchaeological, revisions to the field’s ontology. We can do these things and more in part because of Dibble’s work with his students and colleagues. Not a bad legacy, that.
Data Availability
Not applicable.
Ammerman, A., & Feldman, M. (1974). On the ‘making’ of an assemblage of stone tools. American Antiquity, 39 , 610–616.
Article Google Scholar
Anghelinu, M., Nită, L., & Cordoş, C. (2020). Contrasting approaches to lithic assemblages: A view from no man’s land. Cercetări Arheologice, 27 , 33–44.
Archer, W., Gunz, P., van Niekerk, K., Henshilwood, C., & McPherron, S. (2015). Diachronic change within the Still Bay at Blombos Cave. South Africa. Plos One, 10 , e0132428. https://doi.org/10.1371/journal.pone.0132428
Binford, L. R. (1973). Interassemblage variability: The Mousterian and the ‘functional’ argument. In C. Renfrew (Ed.), The explanation of culture change: Models in prehistory (pp. 227–254). Duckworth.
Google Scholar
Bradbury, A. P., & Carr, P. J. (1999). Examining stage and continuum models of flake debris analysis. Journal of Archaeological Science, 26 , 105–116.
Bustos Pérez, G. (2020). Procesos de Reducción en la Industria Lítica: Cambio Diacrónico y Patrones de Ocupación en el Paleolítico Medio de la Península Ibérica. Unpublished PhD dissertation, Depto. de Prehistoria y Arqueología, Universidad Autónoma de Madrid.
Caruana, M., & Herries, A. (2021). An Acheulian Balancing Act: A multivariate examination of size and shape in handaxes from Amanzi Springs, Eastern Cape, South Africa. In J. Cole, J. McNabb, M. Grove, & R. Hosfield (Eds.), Landscapes of Human Evolution: Contributions in Honour of John Gowlett (pp. 91–115). Oxford: Archaeopress.
Charlin, J., & Cardillo, M. (2018). Reduction constraints and shape convergence along tool ontogenetic trajectories: An example from Late Holocene projectile points of Southern Patagonia. In B. Buchanan, M. Eren, & M. O’Brien (Eds.), Convergent evolution and stone-tool technology (pp. 109–129). Cambridge: MIT Press.
Chapter Google Scholar
Clark, G.A. (2002). Observations on paradigmatic bias in French and American Paleolithic archaeology. In L.Strauss (Ed.), The role of American archaeologists in the study of the European Upper Paleolithic , (pp. 19–26). British Archaeological Reports International Series 1048.
Clarke, D. (1973). Archaeology: The loss of innocence. Antiquity, 47 , 6–18.
Crompton, R. H., & Gowlett, J. A. (1993). Allometry and multidimensional form in Acheulean bifaces from Kilombe, Kenya. Journal of Human Evolution, 25 , 175–199.
Davidson, I., & Noble, W. (1989). The archaeology of perception: Traces of depiction and language. Current Anthropology, 30 , 125–155.
Dibble, H. L. (1987). The interpretation of Middle Paleolithic scraper morphology. American Antiquity, 52 , 109–117.
Dibble, H. L. (1988). Typological aspects of reduction and intensity of utilization of lithic resources in the French Mousterian. In H. L. Dibble & A. Montet-White (Eds.), Upper Pleistocene prehistory of Western Eurasia (pp. 181–197). University Museum.
Dibble, H. L. (1995). Biache Saint-Vaast, Level IIa: A comparison of analytical approaches. In H. L. Dibble & O. Bar-Yosef (Eds.), The definition and interpretation of Levallois variability (pp. 96–113). Prehistory Press.
Dibble, H. L., Holdaway, S. J., Lin, S. C., Braun, D. R., Douglass, M. J., Iovita, R., McPherron, S. P., Olszewski, D. I., & Sandgathe, D. (2017). Major fallacies surrounding stone artifacts and assemblages. Journal of Archeological Method and Theory, 24 , 813–851. https://doi.org/10.1007/s10816-016-9297-8
Dibble, H.L., & Rolland, N. (1992). On assemblage variability in the Middle Paleolithic of Western Europe. In H.L. Dibble & P. Mellars (Eds.), The Middle Paleolithic: adaptations, behaviour and variability (pp. 1–28). University Museum of Philadelphia Museum Monograph 78.
Faivre, G.-P., Gravina, B., Bourguignon, L., Discamps, E., & Turq, A. (2017). Late Middle Palaeolithic lithic technocomplexes (MIS 5–3) in the Northeastern Aquitaine Basin: Advances and challenges. Quaternary International, 433 , 116–131. https://doi.org/10.1016/j.quaint.2016.02.060
Frison, G. (1968). A functional analysis of certain chipped stone tools. American Antiquity, 33 , 149–155.
González-José, R., & Charlin, J. (2012). Relative importance of modularity and other morphological attributes on different types of lithic point weapons: Assessing functional variations. PLoS ONE, 7 (10), e48009. https://doi.org/10.1371/journal.pone.0048009
Hamsa, A. (2013). Cultural differences or archaeological constructs: An assessment of projectile variability from Late Middle prehistoric sites on the Northwest Great Plains . Lethbridge, ALB: Unpublished MA Thesis, Dept. of Geography, University of Lethbridge.
Hayden, B. (1976). Curation: Old and New. In J. S. Raymond, B. Loveseth, C. Arnold, & G. Reardon (Eds.), Primitive art and technology (pp. 47–59). Archaeological Association.
Hiscock, P. (2002). Quantifying the size of artefact assemblages. Journal of Archaeological Science, 29 , 251–258.
Hiscock, P. (2009). Reduction, recycling, and raw material procurement in Western Arnhem Land, Australia. In B. Adams & B. Blades (Eds.), Lithic materials and Paleolithic societies (pp. 78–93). Blackwell.
Hiscock, P., & Tabrett, A. (2010). Generalization, inference and the quantification of lithic reduction. World Archaeology, 42 , 545–561. https://doi.org/10.1080/00438243.2010.517669
Hiscock, P., & Attenbrow, V. (2005). Australia’s eastern regional sequence revisited: Technology and change at Capertee 3 . BAR International Series 1397.
Hoffman, C. M. (1986). Projectile point maintenance and typology: Assessment with factor analysis and canonical correlation. In C. Carr (Ed.), For concordance in archaeological analysis: Bridging data structure, quantitative technique, and theory (pp. 566–612). Westport Publishing.
Holdaway, S. J., McPherron, S. P., & Roth, B. (1996). Notched tool reuse and raw material availability in French Middle Paleolithic sites. American Antiquity, 61 , 377–387.
Hussain, S.T. (2019). The French-Anglophone divide in lithic research A plea for pluralism in Palaeolithic archaeology. Unpublished PhD dissertation, University of Leiden. http://hdl.handle.net/1887/69812 .
Iovita, R. (2009). Ontogenetic scaling and lithic systematics: Method and application. Journal of Archaeological Science, 36 , 1447–1457. https://doi.org/10.1016/j.jas.2009.02.008
Iovita, R. (2011). Shape variation in Aterian tanged tools and the origins of projectile technology: A Morphometric Perspective on Stone Tool Function. PLoS ONE, 6 (12), e29029.
Jelinek, A. J. (1976). Form, function, and style in lithic analysis. In C. E. Cleland (Ed.), For the director: Essays in cultural continuity and change in honor of James B. Griffin (pp. 19–33). New York: Academic.
Jöris, O. (2009). Bifacially backed knives ( Keilmesser ) in the Central European Middle Palaeolithic. In N. Goren-Inbar & G. Sharon (Eds.), Axe age: Acheulian tool-making from quarry to discard (pp. 287–310). Equinox.
Kitts, D. B. (1992). The conditions for a nomothetic paleontology. In M. Nitecki & D. Nitecki (Eds.), History and evolution (pp. 131–145). State University of New York.
Knell, E.J. (2022). Allometry of unifacial flake tools from Mojave Desert Terminal Pleistocene/Early Holocene sites: Implications for landscape knowledge, tool design, and land use. Journal of Archaeological Science: Reports 41 https://doi.org/10.1016/j.jasrep.2021.103314
Leakey, M.D. (1971). Olduvai Gorge. Volume III: Excavations in Beds I and II, 1960–1963 . Cambridge University Press.
Li, L., Lin, S. C., McPherron, S. P., Abdolahzadeh, A., Chan, A., Dogandžić, T., Iovita, R., Leader, G. M., Magnani, M., Rezek, Z., Dibble, H. L. A., synthesis of the Dibble, et al. (2023). controlled experiments into the mechanics of lithic production. Journal of Archaeological Method and Theory, 30 , 1284–1325. https://doi.org/10.1007/s10816-022-09586-2
Lin, S. C. (2018). Flake selection and scraper retouch probability: An alternative model for explaining Middle Paleolithic assemblage retouch variability. Archaeological and Anthropological Sciences, 10 , 1791–1806. https://doi.org/10.1007/s12520-017-0496-3
Lin, S. C., Pop, C. M., Dibble, H. L., Archer, W., Desta, D., Weiss, M., & McPherron, S. P. (2016). A core reduction experiment finds no effect of original stone size and reduction intensity on flake debris size distribution. American Antiquity, 81 , 562–575. https://doi.org/10.7183/0002-7316.81.3.5
Maloney, T. R., O’Connor, S., & Balme, J. (2017). The effect of retouch intensity on Mid to Late Holocene unifacial and bifacial points from the Kimberley. Australian Archaeology, 83 , 42–55. https://doi.org/10.1080/03122417.2017.1350345
Marwick, B. (2008). Beyond typologies: The reduction thesis and its implications for lithic assemblages in Southeast Asia. Indo-Pacific Prehistory Association Bulletin, 28 , 108–116.
McPherron, S.P. (1994). A reduction model for variability in Acheulian biface morphology. Unpublished PhD dissertation, Department of Anthropology, University of Pennsylvania.
Miller, D. S. (2018). From colonization to domestication: Population, environment, and the origins of agriculture in Eastern North America . University of Utah Press.
Book Google Scholar
Monnier, G. F., & Missal, K. (2014). Another Mousterian debate? Bordian Facies, Chaîne Opèratoire technocomplexes, and patterns of lithic variability in the Western European Middle and Upper Pleistocene. Quaternary International, 350 , 59–83. https://doi.org/10.1016/j.quaint.2014.06.053
Morales, J. I. (2016). Distribution patterns of stone-tool reduction: Establishing frames of reference to approximate occupational features and formation processes in Paleolithic societies. Journal of Anthropological Archaeology, 41 , 231–245. https://doi.org/10.1016/j.jaa.2016.01.0040278-4165
Morales, J. I., Lorenzo, C., & Vergès, J. M. (2015a). Measuring retouch intensity in lithic tools: A new proposal using 3D scan data. Journal of Archaeological Method and Theory, 22 , 543–558. https://doi.org/10.1007/s10816-013-9189-0
Morales, J. I., Soto, M., Lorenzo, C., & Vergès, J. M. (2015b). The evolution and stability of stone tools: The effects of different mobility scenarios in tool reduction and shape features. Journal of Archaeological Science: Reports, 3 , 295–305. https://doi.org/10.1016/j.jasrep.2015.06.019
Nash, S. E. (1996). Is curation a useful heuristic? In G. H. Odell (Ed.), Stone tools: Theoretical insights into human prehistory (pp. 81–99). Plenum.
Nguyen, D., & Clarkson, C. (2016). Typological transformations among Late Paleolithic flaked core tools in Vietnam: An examination of the Pa Muoi assemblage. Journal of Indo-Pacific Archaeology, 40 , 32–41.
Nolan, K. C., Shott, M. J., & Olson, E. (2022). The Central Ohio Archaeological Digitization Survey: A demonstration of amplified public good from collaboration with private collectors. Advances in Archaeological Practice, 10 , 83–90. https://doi.org/10.1017/aap.2021.33
Odell, G. H. (1996). Economizing behavior and the concept of ‘curation.’ In G. H. Odell (Ed.), Stone tools: Theoretical insights into human prehistory (pp. 81–99). Plenum.
Perreault, C. (2019). The quality of the archaeological record . University of Chicago Press.
Phillipps, R., Holdaway, S. J., Barrett, M., & Emmitt, J. (2022). Archaeological site types, and assemblage size and diversity in Aotearoa New Zealand. Archaeology in Oceania, 57 , 111–126. https://doi.org/10.1002/arco.5259
Potts, R. (1991). Why the Oldowan? Plio-Pleistocene Toolmaking and the Transport of Resources. Journal of Anthropological Research, 47 , 153–176.
Randall, A.R. (2002). Technological variation in Early Side-notched hafted bifaces: A view from the Middle Tennessee River Valley in Northwest Alabama. Unpublished MA thesis, Department of Anthropology, University of Florida.
Reynolds, N. (2020). Threading the weft, testing the warp: Population concepts and the European Upper Paleolithic Chronocultural Framework. In H. Groucutt (Ed.), Culture History and Convergent Evolution (pp. 187–212). Springer.
Roebroeks, W., Kolen, J., van Poecke, M., & Van Gijn, A. (1997). “Site J”: An Early Weichselian (Middle Palaeolithic) flint scatter at Maastricht-Belvedere, The Netherlands. Paleo, 9 , 143–172.
Sahle, Y. and Negash, A. (2016). An ethnographic experiment of endscraper curation rate among Hadiya Hideworkers, Ethiopia. Lithic Technology DOI: https://doi.org/10.1179/2051618515Y.0000000022 .
Schiffer, M. B. (1975). The effects of occupation span on site content. In M. B. Schiffer & J. H. House (Eds.), The Cache River Archeological Project: An experiment in contract archeology (pp. 265–269). Fayetteville: Arkansas Archeological Survey, Research Series no. 8.
Serwatka, K. (2015). Bifaces in plain sight: Testing elliptical Fourier analysis in identifying reduction effects on Late Middle Palaeolithic Bifacial Tools. Litikum, 3 , 13–25.
Shott, M. J. (1996). An exegesis of the curation concept. Journal of Anthropological Research, 52 , 259–280.
Shott, M. J. (2000). The quantification problem in stone tool assemblages. American Antiquity, 65 , 725–738.
Shott, M. (2003a). Reduction sequence and Chaîne Opèratoire . Lithic Technology, 28 , 95–105.
Shott, M. J. (2003b). Size as a factor in assemblage variation: The European Middle Palaeolithic viewed from a North American perspective. In N. Moloney & M. Shott (Eds.), Lithic Analysis at the Millennium (pp. 137–149). Archtype.
Shott, M. J. (2010). Size dependence in assemblage measures: Essentialism, materialism, and “SHE” analysis in archaeology. American Antiquity, 75 , 886–906. https://doi.org/10.7183/0002-7316.75.4.886
Shott, M. J. (2017). Stage and continuum approaches in Prehistoric biface production: A North American perspective. PLoS One, 12 (3), e0170947.
Shott, M. J. (2021). Prehistoric quarries and terranes: The Modena and Tempiute Obsidian sources of the American Great Basin . University of Utah Press.
Shott, M. J., & Otárola-Castillo, E. (2022). Parts and wholes: Reduction allometry and modularity in experimental Folsom points. American Antiquity, 87 , 80–99. https://doi.org/10.1017/aaq.2021.62
Shott, M. J., & Seeman, M. F. (2015). Curation and recycling: Estimating Paleoindian endscraper curation rates at Nobles Pond, Ohio, USA. Quaternary International, 361 , 319–331. https://doi.org/10.1016/j.quaint.2014.06.023
Shott, M. J., & Seeman, M. F. (2017). Use and multifactorial reconciliation of uniface reduction measures: A pilot study at the Nobles Pond Paleoindian Site. American Antiquity, 82 , 723–741. https://doi.org/10.1017/aaq.2017.40
Shott, M. J., Nolan, K. C., & Olson, E. (2023). Original design and allometric variation in kirk points of the Central Ohio Archaeological Digitization Survey. Journal of Archaeological Method and Theory . https://doi.org/10.1007/s10816-023-09612-x
Shott, M.J. (2005). The reduction thesis and its discontents: Review of Australian approaches. In C.Clarkson and L.Lamb (Eds.), Lithics ‘DownUnder’: Australian perspectives on lithic reduction, use and classification (pp. 109–125). British Archaeological Reports International Series 1408.
Suárez, R., & Cardillo, M. (2019). Life history or stylistic variation? A geometric morphometric method for evaluation of fishtail point variability. Journal of Archaeological Science: Reports, 27 , 101997.
Thulman, D., Shott, M. J., Williams, J., & Slade, A. (2023). Clovis point allometry, modularity, and integration: Exploring shape variation due to tool use with landmark-based geometric morphometrics. PLoS ONE, 18 (8), e0289489. https://doi.org/10.1371/journal.pone.0289489
Wojtczak, D. (2014). The Early Middle Palaeolithic blade industry from Hummal, Central Syria. Unpublished PhD dissertation, Natural Sciences Faculty, University of Basel.
Download references
Acknowledgements
My thanks to Gilliane Monnier and Shannon McPherron for the kind invitation to participate in the Society for American Archaeology symposium from which this essay derived. The editor and three anonymous reviewers helped clarify important points. A. Randall kindly permitted use of Figure 4 . Of course the essay is dedicated to Harold Dibble, for his many contributions to lithic analysis.
Author information
Authors and affiliations.
Department of Anthropology, University of Akron, Akron, OH, 44325, USA
Michael J. Shott
You can also search for this author in PubMed Google Scholar
Contributions
M.S. wrote the manuscript text, prepared Figures 1-4, and reviewed the ms.
Corresponding author
Correspondence to Michael J. Shott .
Ethics declarations
Ethics approval, competing interests.
The author declares no competing interests.
Additional information
Publisher's note.
Springer Nature remains neutral with regard to jurisdictional claims in published maps and institutional affiliations.
Rights and permissions
Open Access This article is licensed under a Creative Commons Attribution 4.0 International License, which permits use, sharing, adaptation, distribution and reproduction in any medium or format, as long as you give appropriate credit to the original author(s) and the source, provide a link to the Creative Commons licence, and indicate if changes were made. The images or other third party material in this article are included in the article's Creative Commons licence, unless indicated otherwise in a credit line to the material. If material is not included in the article's Creative Commons licence and your intended use is not permitted by statutory regulation or exceeds the permitted use, you will need to obtain permission directly from the copyright holder. To view a copy of this licence, visit http://creativecommons.org/licenses/by/4.0/ .
Reprints and permissions
About this article
Shott, M.J. Dibble’s Reduction Thesis: Implications for Global Lithic Analysis. J Paleo Arch 7 , 12 (2024). https://doi.org/10.1007/s41982-024-00178-y
Download citation
Accepted : 06 April 2024
Published : 07 May 2024
DOI : https://doi.org/10.1007/s41982-024-00178-y
Share this article
Anyone you share the following link with will be able to read this content:
Sorry, a shareable link is not currently available for this article.
Provided by the Springer Nature SharedIt content-sharing initiative
- Reduction thesis
Advertisement
- Find a journal
- Publish with us
- Track your research

Analytical Methods
A one-pot crispr-rca strategy for ultrasensitive and specific detection of circrna †.

* Corresponding authors
a Children's Hospital, Zhejiang University School of Medicine, National Clinical Research Center for Child Health, Hangzhou, China E-mail: [email protected]
b School of Basic Medical Sciences & Forensic Medicine, Hangzhou Medical College, Hangzhou 310000, China
c School of Materials Science and Engineering, University of Jinan, Jinan 250022, China
d Department of Obstetrics, Shanghai First Maternity and Infant Hospital, Obstetrics and Gynecology Hospital, School of Medicine, Tongji University, Shanghai 201204, China
Accurate and precise detection of circular RNA (circRNA) is imperative for its clinical use. However, the inherent challenges in circRNA detection, arising from its low abundance and potential interference from linear isomers, necessitate innovative solutions. In this study, we introduce, for the first time, the application of the CRISPR/Cas12a system to establish a one-pot, rapid (30 minutes to 2 hours), specific and ultrasensitive circRNA detection strategy, termed RETA-CRISPR (reverse transcription-rolling circle amplification (RT-RCA) with the CRISPR/Cas12a). This method comprises two steps: (1) the RT-RCA process of circRNA amplification, generating repeat units containing the back-splicing junction (BSJ) sequences; and (2) leveraging the protospacer adjacent motif (PAM)-independent Cas12a/crRNA complex to precisely recognize target sequences with BSJ, thereby initiating the collateral cleavage activity of Cas12a to generate a robust fluorescence signal. Remarkably, this approach exhibits the capability to detect circRNAs at a concentration as low as 300 aM. The sensor has been successfully employed for accurate detection of a potential hepatocellular carcinoma biomarker hsa_circ_0001445 (circRNA1445) in various cell lines. In conclusion, RETA-CRISPR seamlessly integrates the advantages of exponential amplification reaction and the robust collateral cleavage activity of Cas12a, positioning it as a compelling tool for practical CRISPR-based diagnostics.
Supplementary files
- Supplementary information PDF (518K)
Article information
Download citation, permissions.

A one-pot CRISPR-RCA strategy for ultrasensitive and specific detection of circRNA
X. Ke, A. Liang, C. Chen and T. Hu, Anal. Methods , 2024, Advance Article , DOI: 10.1039/D4AY00693C
To request permission to reproduce material from this article, please go to the Copyright Clearance Center request page .
If you are an author contributing to an RSC publication, you do not need to request permission provided correct acknowledgement is given.
If you are the author of this article, you do not need to request permission to reproduce figures and diagrams provided correct acknowledgement is given. If you want to reproduce the whole article in a third-party publication (excluding your thesis/dissertation for which permission is not required) please go to the Copyright Clearance Center request page .
Read more about how to correctly acknowledge RSC content .
Social activity
Search articles by author.
This article has not yet been cited.
Advertisements
Help | Advanced Search
Mathematics > Numerical Analysis
Title: a score-based particle method for homogeneous landau equation.
Abstract: We propose a novel score-based particle method for solving the Landau equation in plasmas, that seamlessly integrates learning with structure-preserving particle methods [ arXiv:1910.03080 ]. Building upon the Lagrangian viewpoint of the Landau equation, a central challenge stems from the nonlinear dependence of the velocity field on the density. Our primary innovation lies in recognizing that this nonlinearity is in the form of the score function, which can be approximated dynamically via techniques from score-matching. The resulting method inherits the conservation properties of the deterministic particle method while sidestepping the necessity for kernel density estimation in [ arXiv:1910.03080 ]. This streamlines computation and enhances scalability with dimensionality. Furthermore, we provide a theoretical estimate by demonstrating that the KL divergence between our approximation and the true solution can be effectively controlled by the score-matching loss. Additionally, by adopting the flow map viewpoint, we derive an update formula for exact density computation. Extensive examples have been provided to show the efficiency of the method, including a physically relevant case of Coulomb interaction.
Submission history
Access paper:.
- Other Formats
References & Citations
- Google Scholar
- Semantic Scholar
BibTeX formatted citation

Bibliographic and Citation Tools
Code, data and media associated with this article, recommenders and search tools.
- Institution
arXivLabs: experimental projects with community collaborators
arXivLabs is a framework that allows collaborators to develop and share new arXiv features directly on our website.
Both individuals and organizations that work with arXivLabs have embraced and accepted our values of openness, community, excellence, and user data privacy. arXiv is committed to these values and only works with partners that adhere to them.
Have an idea for a project that will add value for arXiv's community? Learn more about arXivLabs .
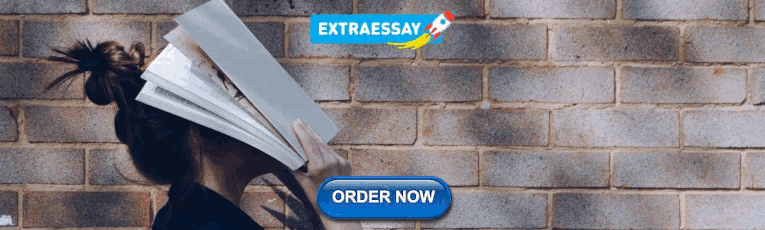
IMAGES
VIDEO
COMMENTS
Step 1: Explain your methodological approach. Step 2: Describe your data collection methods. Step 3: Describe your analysis method. Step 4: Evaluate and justify the methodological choices you made. Tips for writing a strong methodology chapter. Other interesting articles.
Revised on 10 October 2022. Your research methodology discusses and explains the data collection and analysis methods you used in your research. A key part of your thesis, dissertation, or research paper, the methodology chapter explains what you did and how you did it, allowing readers to evaluate the reliability and validity of your research.
As you should have identified in STEP THREE: Research methods, and in the article, Types of variables, in the Fundamentals part of Lærd Dissertation, (a) not all data is the same, and (b) not all variables are measured in the same way (i.e., variables can be dichotomous, ordinal or continuous). In addition, not all data is normal, nor is the ...
The results chapter (also referred to as the findings or analysis chapter) is one of the most important chapters of your dissertation or thesis because it shows the reader what you've found in terms of the quantitative data you've collected. It presents the data using a clear text narrative, supported by tables, graphs and charts.
In any research, the methodology chapter is one of the key components of your dissertation. It provides a detailed description of the methods you used to conduct your research and helps readers understand how you obtained your data and how you plan to analyze it. This section is crucial for replicating the study and validating its results.
Quantitative data analysis is one of those things that often strikes fear in students. It's totally understandable - quantitative analysis is a complex topic, full of daunting lingo, like medians, modes, correlation and regression.Suddenly we're all wishing we'd paid a little more attention in math class…. The good news is that while quantitative data analysis is a mammoth topic ...
Mixed-method approaches combine both qualitative and quantitative methods, and therefore combine the strengths of both types of research. Mixed methods have gained popularity in recent years. When undertaking mixed-methods research you can collect the qualitative and quantitative data either concurrently or sequentially.
A data analysis dissertation is a complex and challenging project requiring significant time, effort, and expertise. ... For example, using a qualitative research method, your data analysis will involve coding and categorizing your data. This can be time-consuming, so allowing enough time in your schedule is important. Once you have coded and ...
The results chapter of a thesis or dissertation presents your research results concisely and objectively. In quantitative research, for each question or hypothesis, state: The type of analysis used; Relevant results in the form of descriptive and inferential statistics; Whether or not the alternative hypothesis was supported
The method you choose will depend on your research objectives and questions. These are the most common qualitative data analysis methods to help you complete your dissertation: 2. Content analysis: This method is used to analyze documented information from texts, email, media and tangible items.
QDA Method #3: Discourse Analysis. Discourse is simply a fancy word for written or spoken language or debate. So, discourse analysis is all about analysing language within its social context. In other words, analysing language - such as a conversation, a speech, etc - within the culture and society it takes place.
Step 1: Planning Your Data Analysis Chapter. Planning your data analysis chapter is a critical precursor to its successful execution. Begin by outlining the chapter structure to provide a roadmap for your analysis. Start with an introduction that succinctly introduces the purpose and significance of the data analysis in the context of your ...
The process of data analysis in secondary research. Secondary analysis (i.e., the use of existing data) is a systematic methodological approach that has some clear steps that need to be followed for the process to be effective. In simple terms there are three steps: Step One: Development of Research Questions. Step Two: Identification of dataset.
Descriptive Analytics. Definition: Descriptive analytics focused on describing or summarizing raw data and making it interpretable. This type of analytics provides insight into what has happened in the past. It involves the analysis of historical data to identify patterns, trends, and insights. Descriptive analytics often uses visualization ...
Thematic analysis is a research method used to identify and interpret patterns or themes in a data set; it often leads to new insights and understanding (Boyatzis, 1998; Elliott, 2018; Thomas, 2006).However, it is critical that researchers avoid letting their own preconceptions interfere with the identification of key themes (Morse & Mitcham, 2002; Patton, 2015).
A non-empirical dissertation is based on secondary sources, such as books, articles, and online resources. It typically follows a qualitative research approach and uses methods such as content analysis or discourse analysis. Narrative Dissertation. A narrative dissertation is a personal account of the researcher's experience or journey.
The results chapter in a dissertation or thesis (or any formal academic research piece) is where you objectively and neutrally present the findings of your qualitative analysis (or analyses if you used multiple qualitative analysis methods ). This chapter can sometimes be combined with the discussion chapter (where you interpret the data and ...
The quantitative research method is mostly used for mathematics and other numerical subjects. However, if we talk about qualitative research, the entire data is collected through questionnaires and other modes of collection. ... There are a certain basic set of skills that are required for dissertation writing. Analytical skills are a basic ...
Analytical performance demonstrated precision and accuracy below 15% and a quantification limit of 1 ng mL-1, meeting validation requirements set by regulations worldwide. Thus, the DPX/LC-MS technique offers a faster and more cost-effective method for analyzing NAs in medicines compared to traditional approaches.
Harold Dibble demonstrated the systematic effects of reduction by retouch upon the size and shape of Middle Paleolithic tools. The result was the reduction thesis, with its far-reaching implications for the understanding of Middle Paleolithic assemblage variation that even now are incompletely assimilated. But Dibble's influence extended beyond the European Paleolithic. Others identified ...
Analytical Methods. ... strategy, termed RETA-CRISPR (reverse transcription-rolling circle amplification (RT-RCA) with the CRISPR/Cas12a). This method comprises two steps: (1) the RT-RCA process of circRNA amplification, generating repeat units containing the back-splicing junction (BSJ) sequences; and (2) leveraging the protospacer adjacent ...
We propose a novel score-based particle method for solving the Landau equation in plasmas, that seamlessly integrates learning with structure-preserving particle methods [arXiv:1910.03080]. Building upon the Lagrangian viewpoint of the Landau equation, a central challenge stems from the nonlinear dependence of the velocity field on the density. Our primary innovation lies in recognizing that ...