
- Advanced Search
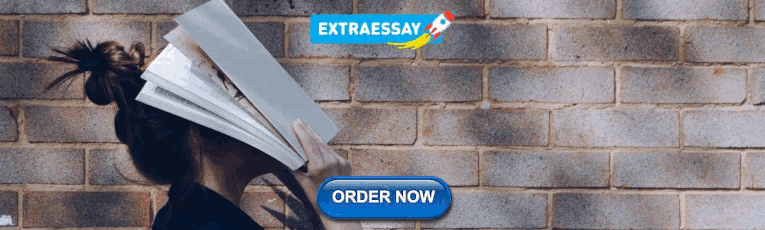
A systematic literature review of speech emotion recognition approaches
Computer Science Engineering Department, School of Computer Science Engineering and Technology, Bennett University, Greater Noida, UP-201310, India
Department of Computer Science and Information Technology, KIET Group of Institutions, Delhi-NCR, Ghaziabad, UP-201206, India
New Citation Alert added!
This alert has been successfully added and will be sent to:
You will be notified whenever a record that you have chosen has been cited.
To manage your alert preferences, click on the button below.
New Citation Alert!
Please log in to your account
- Publisher Site
Neurocomputing

Nowadays emotion recognition from speech (SER) is a demanding research area for researchers because of its wide real-life applications. There are many challenges for SER systems such as the availability of suitable emotional databases, identification of the relevant feature vector, and suitable classifiers. This paper critically analysed the literature on SER in terms of speech databases, speech features, traditional machine learning (ML) classifiers and DL approaches along with the areas for future directions. In recent years, there is a growing interest of researchers to use deep learning (DL) approaches for SER and get improvement in recognition rate. The focus of this review is on DL approaches for SER. A total of 152 papers have been reviewed from years 2000–2021. We have identified frequently used speech databases and related accuracies achieved using DL approaches. The motivations and limitations of DL approaches for SER are also summarized.
Index Terms
Computing methodologies
Artificial intelligence
Natural language processing
Speech recognition
Machine learning
Communication hardware, interfaces and storage
Signal processing systems
Recommendations
Speech emotion recognition: emotional models, databases, features, preprocessing methods, supporting modalities, and classifiers.
- Current methodologies in Speech Emotion Recognition (SER) technologies are reviewed.
Speech is the most natural way of expressing ourselves as humans. It is only natural then to extend this communication medium to computer applications. We define speech emotion recognition (SER) systems as a collection of methodologies ...
Speech Emotion Recognition Based on Improved MFCC
Speech1 Emotion Recognition SER uses the Berlin EMO-DB database, seven emotions. Traditional emotional features and their statistics are used in SER. Two improved Mel Frequency Cepstrum Coefficients MFCC features are added to this experiment, which ...
Speech emotion recognition approaches: A systematic review
The speech emotion recognition (SER) field has been active since it became a crucial feature in advanced Human–Computer Interaction (HCI), and wide real-life applications use it. In recent years, numerous SER systems have been covered by ...
- The speech-emotion recognition (SER) field became crucial in advanced Human-computer interaction (HCI).
- Numerous SER systems have been proposed by researchers using Machine Learning (ML) and Deep Learning (DL).
- This survey aims to ...
Login options
Check if you have access through your login credentials or your institution to get full access on this article.
Full Access
- Information
- Contributors
Published in
Elsevier B.V.
In-Cooperation
Elsevier Science Publishers B. V.
Netherlands
Publication History
- Published: 1 July 2022
Author Tags
- Deep learning approaches
- Speech database
- Speech emotion recognition
- Speech features
- Systematic review
Funding Sources
Other metrics.
- Bibliometrics
- Citations 6
Article Metrics
- 6 Total Citations View Citations
- 0 Total Downloads
- Downloads (Last 12 months) 0
- Downloads (Last 6 weeks) 0
Digital Edition
View this article in digital edition.
Share this Publication link
https://dlnext.acm.org/doi/10.1016/j.neucom.2022.04.028
Share on Social Media
- 0 References
Export Citations
- Please download or close your previous search result export first before starting a new bulk export. Preview is not available. By clicking download, a status dialog will open to start the export process. The process may take a few minutes but once it finishes a file will be downloadable from your browser. You may continue to browse the DL while the export process is in progress. Download
- Download citation
- Copy citation
We are preparing your search results for download ...
We will inform you here when the file is ready.
Your file of search results citations is now ready.
Your search export query has expired. Please try again.
Trends in speech emotion recognition: a comprehensive survey
- Published: 22 February 2023
- Volume 82 , pages 29307–29351, ( 2023 )
Cite this article
- Kamaldeep Kaur ORCID: orcid.org/0000-0002-3542-1214 1 , 2 &
- Parminder Singh 2
966 Accesses
2 Citations
Explore all metrics
Among the other modes of communication, such as text, body language, facial expressions, and so on, human beings employ speech as the most common. It contains a great deal of information, including the speaker’s feelings. Detecting the speaker’s emotions from his or her speech has shown to be quite useful in a variety of real-world applications. The dataset development, feature extraction, feature selection/dimensionality reduction, and classification are the four primary processes in the Speech Emotion Recognition process. In this context, more than 70 studies are thoroughly examined in terms of their databases, emotions, features extracted, and classifiers employed. The databases, characteristics, extraction and classification methods, as well as the results, are all thoroughly examined. The study also includes a comparative analysis of these research papers.
This is a preview of subscription content, log in via an institution to check access.
Access this article
Price includes VAT (Russian Federation)
Instant access to the full article PDF.
Rent this article via DeepDyve
Institutional subscriptions
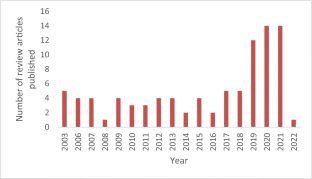
Similar content being viewed by others
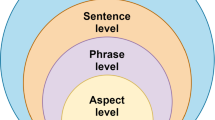
A survey on sentiment analysis methods, applications, and challenges
Mayur Wankhade, Annavarapu Chandra Sekhara Rao & Chaitanya Kulkarni
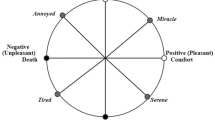
A review on sentiment analysis and emotion detection from text
Pansy Nandwani & Rupali Verma
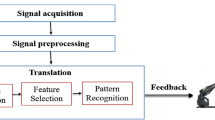
Human emotion recognition from EEG-based brain–computer interface using machine learning: a comprehensive review
Essam H. Houssein, Asmaa Hammad & Abdelmgeid A. Ali
Abdel-Hamid L (2020) Egyptian Arabic speech emotion recognition using prosodic, spectral and wavelet features. Speech Commun 122(May):19–30. https://doi.org/10.1016/j.specom.2020.04.005
Article Google Scholar
Abdelwahab M, Busso C (2019) “Active Learning for Speech Emotion Recognition Using Deep Neural Network,” 2019 8th International Conference on Affective Computing and Intelligent Interaction, ACII 2019, pp. 441–447, https://doi.org/10.1109/ACII.2019.8925524 .
Abdi H, Williams LJ (2010) Principal component analysis. WIREs Comput Stat 2(4):433–459. https://doi.org/10.1002/wics.101
Agrawal SS (2011) “Emotions in Hindi speech- Analysis, perception and recognition,” 2011 International Conference on Speech Database and Assessments, Oriental COCOSDA 2011 - Proceedings, pp. 7–13, https://doi.org/10.1109/ICSDA.2011.6085972 .
Akçay MB, Oğuz K (2020) Speech emotion recognition: emotional models, databases, features, preprocessing methods, supporting modalities, and classifiers. Speech Comm 116:56–76. https://doi.org/10.1016/j.specom.2019.12.001
Albawi S, Abed Mohammed T, Alzawi S (2017) Understanding of a convolutional neural network. In: 2017 IEEE International Conference on Engineering and Technology (ICET). https://doi.org/10.1109/ICEngTechnol.2017.8308186
Albornoz EM, Milone DH (2017) Emotion recognition in never-seen languages using a novel ensemble method with emotion profiles. IEEE Trans Affect Comput 8(1):43–53. https://doi.org/10.1109/TAFFC.2015.2503757
Albornoz EM, Milone DH, Rufiner HL (2011) Spoken emotion recognition using hierarchical classifiers. Comput Speech Lang 25(3):556–570. https://doi.org/10.1016/j.csl.2010.10.001
Anagnostopoulos CN, Iliou T, Giannoukos I (2012) Features and classifiers for emotion recognition from speech: a survey from 2000 to 2011. Artif Intell Rev 43(2):155–177. https://doi.org/10.1007/s10462-012-9368-5
Balakrishnama S, Ganapathiraju A (1998) “Linear Discriminant Analysis—A Brief Tutorial,” accessed on 10.09.2021
Bansal S, Dev A (2013) “Emotional hindi speech database,” 2013 International Conference Oriental COCOSDA Held Jointly with 2013 Conference on Asian Spoken Language Research and Evaluation, O-COCOSDA/CASLRE 2013, pp. 1–4, https://doi.org/10.1109/ICSDA.2013.6709867 .
Bansal S, Dev A (2015) Emotional Hindi speech: Feature extraction and classification. 2015 2nd International Conference on Computing for Sustainable Global Development (INDIACom) 03:1865–1868
Google Scholar
Beaufays F (1995) Transform-domain adaptive filters: an analytical approach. IEEE Trans Signal Process 43(2):422–431. https://doi.org/10.1109/78.348125
Bhattacharyya S et al (2018) Speech Background Noise Removal Using Different Linear Filtering Techniques. Lect Notes Electr Eng 475:297–307. https://doi.org/10.1007/978-981-10-8240-5
Boersma P, Weenink D (2001) PRAAT, a system for doing phonetics by computer. Glot Int 5:341–345
Boggs K, Liam (2017) Performance measures for machine learning, accessed on 11.08.2021
Bou-Ghazale SE, Hansen JHL (Jul. 2000) A comparative study of traditional and newly proposed features for recognition of speech under stress. IEEE Transact Speech Aud Process 8(4):429–442. https://doi.org/10.1109/89.848224
Brookes M (1997) Voicebox: Speech processing toolbox for matlab. Imperial College, London. http://www.ee.ic.ac.uk/hp/staff/dmb/voicebox/voicebox.html . Accessed 06.09.2021
Burges CJC (1998) A tutorial on support vector machines for pattern recognition. Data Min Knowl Disc 2(2):121–167. https://doi.org/10.1023/A:1009715923555
Burkhardt F, Paeschke A, Rolfes M, Sendlmeier W, Weiss B (2005) “A database of German emotional speech,” in 9th European Conference on Speech Communication and Technology, , vol. 5, pp. 1517–1520
Busso C, Bulut M, Lee CC, Kazemzadeh A, Mower E, Kim S, Chang JN, Lee S, Narayanan SS (2008) IEMOCAP: interactive emotional dyadic motion capture database. Lang Resour Eval 42:335–359. https://doi.org/10.1007/s10579-008-9076-6
Busso C, Metallinou A, Narayanan SS (2011) “Iterative feature normalization for emotional speech detection,” in 2011 IEEE International Conference on Acoustics, Speech and Signal Processing (ICASSP), pp. 5692–5695, https://doi.org/10.1109/ICASSP.2011.5947652 .
C. academic of science Institute of automation (2005) CASIA-Chinese emotional speech corpus, Chin Linguist Data Consortium (CLDC). http://shachi.org/resources/27 . Accessed 17 Oct 2021
Cao H, Verma R, Nenkova A (2015) Speaker-sensitive emotion recognition via ranking: studies on acted and spontaneous speech. Comput Speech Lang 29(1):186–202. https://doi.org/10.1016/j.csl.2014.01.003
Chakroborty S, Saha G (2010) Feature selection using singular value decomposition and QR factorization with column pivoting for text-independent speaker identification. Speech Commun 52(9):693–709. https://doi.org/10.1016/j.specom.2010.04.002
Chandrasekar P, Chapaneri S, Jayaswal D (2014) “Automatic speech emotion recognition: A survey,” in 2014 International Conference on Circuits, Systems, Communication and Information Technology Applications, CSCITA 2014, pp. 341–346, https://doi.org/10.1109/CSCITA.2014.6839284 .
Chen X, Jeong JC (2007) “Enhanced recursive feature elimination,” in Sixth International Conference on Machine Learning and Applications (ICMLA 2007), pp. 429–435, https://doi.org/10.1109/ICMLA.2007.35 .
Chen Y, Xie J (2012) “Emotional speech recognition based on SVM with GMM supervector,” Journal of Electronics (China), vol. 29, https://doi.org/10.1007/s11767-012-0871-2 .
Chen C, You M, Song M, Bu J, Liu J (2006) “An Enhanced Speech Emotion Recognition System Based on Discourse Information BT - Computational Science – ICCS 2006,” in ICCS, pp. 449–456
Chen B, Yin Q, Guo P (2014) “A study of deep belief network based Chinese speech emotion recognition,” Proceedings - 2014 10th International Conference on Computational Intelligence and Security, CIS 2014, pp. 180–184, https://doi.org/10.1109/CIS.2014.148 .
Chen M, He X, Yang J, Zhang H (2018) 3-D convolutional recurrent neural networks with attention model for speech emotion recognition. IEEE Signal Process Lett 25(10):1440–1444. https://doi.org/10.1109/LSP.2018.2860246
Chen Z, Jiang F, Cheng Y, Gu X, Liu W, Peng J (2018) “XGBoost Classifier for DDoS Attack Detection and Analysis in SDN-Based Cloud,” in 2018 IEEE international conference on big data and smart computing (BigComp), pp. 251–256, https://doi.org/10.1109/BigComp.2018.00044 .
Chenchen Huang DF, Gong W, Wenlong F (2014) A research of speech emotion recognition based on deep belief network and SVM. Math Problems Eng, Article ID 749604. https://doi.org/10.1155/2014/749604
Chiu S, Tavella D (2008) Introduction to data mining. Data Min Market Intel Optimal Market Returns:137–192. https://doi.org/10.1016/b978-0-7506-8234-3.00007-1
Choudhury AR, Ghosh A, Pandey R, Barman S (2018) “Emotion recognition from speech signals using excitation source and spectral features,” Proceedings of 2018 IEEE Applied Signal Processing Conference, ASPCON 2018, pp. 257–261, https://doi.org/10.1109/ASPCON.2018.8748626 .
Clavel C, Vasilescu I, Devillers L, Richard G, Ehrette T (2008) Fear-type emotion recognition for future audio-based surveillance systems. Speech Commun 50(6):487–503. https://doi.org/10.1016/j.specom.2008.03.012
Darekar RV, Dhande AP (2018) Emotion recognition from Marathi speech database using adaptive artificial neural network. Biol Inspired Cogn Architect 23(January):35–42. https://doi.org/10.1016/j.bica.2018.01.002
Dellaert F, Polzin T, Waibel A (1996) “Recognizing emotion in speech,” in Proceeding of Fourth International Conference on Spoken Language Processing. ICSLP ‘96, vol. 3, pp. 1970–1973, https://doi.org/10.1109/ICSLP.1996.608022 .
Devillers L, Vidrascu L (2007) Real-Life Emotion Recognition in Speech BT - Speaker Classification II: Selected Projects, C. Müller, Ed. Springer Berlin Heidelberg, Berlin, Heidelberg, pp 34–42
Dey A, Chattopadhyay S, Singh PK, Ahmadian A, Ferrara M, Sarkar R (2020) A hybrid Meta-heuristic feature selection method using Golden ratio and equilibrium optimization algorithms for speech emotion recognition. IEEE Access 8:200953–200970. https://doi.org/10.1109/ACCESS.2020.3035531
Dhall A, Goecke R, Gedeon T (2011) Acted facial expressions in the wild database. Tech Rep, no, [Online]. Available: http://cs.anu.edu.au/techreports/ . Accessed 27 Oct 2021
Duda PEHRO, Hart PE, Duda RO (1973) Pattern classification and scene analysis. Leonardo 19(4):462–463
MATH Google Scholar
Dupuis K, Pichora-Fuller M (2011) Recognition of emotional speech for younger and older talkers: Behavioural findings from the Toronto emotional speech set. Can Acoust Acoustique Canadienne 39:182–183
Ekman P (1992) An argument for basic emotions. Cognit Emot 6(3):169–200
El Ayadi M, Kamel MS, Karray F (2011) Survey on speech emotion recognition: features, classification schemes, and databases. Pattern Recogn 44(3):572–587. https://doi.org/10.1016/j.patcog.2010.09.020
Article MATH Google Scholar
Engberg IS, Hansen AV, Andersen O, Dalsgaard P (1997) “Design, recording and verification of a danish emotional speech database,” in 5th European Conference on Speech Communication and Technology, Rhodes, Greece, pp. 1–4
Er MB (2020) “A Novel Approach for Classification of Speech Emotions Based on Deep and Acoustic Features,” IEEE Access, vol. 8, https://doi.org/10.1109/ACCESS.2020.3043201 .
Essentia Toolkit (n.d.) https://essentia.upf.edu . Accessed 16 Nov 2021
Eyben F (n.d.) Eight emotional speech databases. https://mediatum.ub.tum.de/ . Accessed 18 Nov 2021
Eyben F, Schuller B (2015) OpenSMILE: the Munich open-source large-scale multimedia feature extractor. SIG Multimed Rec 6(4):4–13. https://doi.org/10.1145/2729095.2729097
Eyben F, Wöllmer M, Schuller B (2009) “OpenEAR — Introducing the munich open-source emotion and affect recognition toolkit,” in 2009 3rd International Conference on Affective Computing and Intelligent Interaction and Workshops, pp. 1–6, https://doi.org/10.1109/ACII.2009.5349350 .
Eyben F, Wöllmer M, Schuller B (2010) “Opensmile: The Munich Versatile and Fast Open-Source Audio Feature Extractor,” in Proceedings of the 18th ACM International Conference on Multimedia, pp. 1459–1462, https://doi.org/10.1145/1873951.1874246 .
Eyben F, Scherer KR, Schuller BW, Sundberg J, Andre E, Busso C, Devillers LY, Epps J, Laukka P, Narayanan SS, Truong KP (2016) The Geneva minimalistic acoustic parameter set (GeMAPS) for voice research and affective computing. IEEE Trans Affect Comput 7(2):190–202. https://doi.org/10.1109/TAFFC.2015.2457417
Ezz-Eldin M, Khalaf AAM, Hamed HFA, Hussein AI (2021) Efficient feature-aware hybrid model of deep learning architectures for speech emotion recognition. IEEE Access 9:1–1. https://doi.org/10.1109/access.2021.3054345
Faramarzi A, Heidarinejad M, Stephens B, Mirjalili S (2020) Equilibrium optimizer: A novel optimization algorithm. Knowl-Based Syst 191:105190. https://doi.org/10.1016/j.knosys.2019.105190
Farhoudi Z, Setayeshi S, Rabiee A (2017) Using learning automata in brain emotional learning for speech emotion recognition. Int J Speech Technol 20(3):553–562. https://doi.org/10.1007/s10772-017-9426-0
Fayek HM, Lech M, Cavedon L (2017) Evaluating deep learning architectures for speech emotion recognition. Neural Netw 92:60–68. https://doi.org/10.1016/j.neunet.2017.02.013
Ferdib-Al-Islam, L. Akter, and M. M. Islam (2021) “Hepatocellular Carcinoma Patient’s Survival Prediction Using Oversampling and Machine Learning Techniques,” Int Conf Robot Electr Signal Process Tech, pp. 445–450, https://doi.org/10.1109/ICREST51555.2021.9331108 .
Fernandez R, Picard RW (2003) Modeling drivers’ speech under stress. Speech Commun 40(1):145–159. https://doi.org/10.1016/S0167-6393(02)00080-8
Fischer A, Igel C (2014) Training restricted Boltzmann machines: An introduction. Pattern Recognit 47(1):25–39. https://doi.org/10.1016/j.patcog.2013.05.025
Fonti V (2017) Feature selection using LASSO. VU Amsterdam:1–26
Fukunaga K, Mantock JM (1983) Nonparametric discriminant analysis. IEEE Trans Pattern Anal Mach Intell 5(6):671–678. https://doi.org/10.1109/tpami.1983.4767461
Giannakopoulos T (2015) PyAudioAnalysis: An open-source python library for audio signal analysis. PLoS One 10(12):1–17. https://doi.org/10.1371/journal.pone.0144610
Giannakopoulos T, Pikrakis A, Theodoridis S (2009) “A dimensional approach to emotion recognition of speech from movies,” in 2009 IEEE International Conference on Acoustics, Speech and Signal Processing, pp. 65–68, https://doi.org/10.1109/ICASSP.2009.4959521 .
Gomes J, El-Sharkawy M (2015) “i-Vector Algorithm with Gaussian Mixture Model for Efficient Speech Emotion Recognition,” 2015 International Conference on Computational Science and Computational Intelligence (CSCI), pp. 476–480, https://doi.org/10.1109/CSCI.2015.17 .
Grimm M, Kroschel K, Narayanan S (2007) “Support Vector Regression for Automatic Recognition of Spontaneous Emotions in Speech,” in 2007 IEEE International Conference on Acoustics, Speech and Signal Processing - ICASSP ‘07, vol. 4, pp. IV-1085-IV–1088, https://doi.org/10.1109/ICASSP.2007.367262 .
Hansen JHL, Bou-Ghazale SE (1997) Getting started with SUSAS: a speech under simulated and actual stress database. https://catalog.ldc.upenn.edu/LDC99S78 . Accessed 28 Nov 2021
Hifny Y, Ali A (2019) “Efficient Arabic Emotion Recognition Using Deep Neural Networks,” ICASSP, IEEE International Conference on Acoustics, Speech and Signal Processing - Proceedings, vol. 2019-May, pp. 6710–6714, https://doi.org/10.1109/ICASSP.2019.8683632 .
Hochreiter S, Schmidhuber J (1997) Long short-term memory. Neural Comput 9(8):1735–1780. https://doi.org/10.1162/neco.1997.9.8.1735
Hossain MS, Muhammad G (2019) Emotion recognition using deep learning approach from audio–visual emotional big data. Inf Fus 49:69–78. https://doi.org/10.1016/j.inffus.2018.09.008
Hossin M, Sulaiman MN (2015) A review on evaluation metrics for data classification evaluations. Int J Data Min Knowl Manag Process 5(2):01–11. https://doi.org/10.5121/ijdkp.2015.5201
Hozjan V, Kacic Z, Moreno A, Bonafonte A, Nogueiras A (2002) Interface databases: design and collection of a multilingual emotional speech database. http://www.lrec-conf.org/proceedings/lrec2002
Huang R, Ma C (2006) “Toward A Speaker-Independent Real-Time Affect Detection System,” in 18th International Conference on Pattern Recognition (ICPR’06), vol. 1, pp. 1204–1207, https://doi.org/10.1109/ICPR.2006.1127 .
Inger AVH, Engberg S (1996) Documentation of the danish emotional speech database DES. Aalborg. https://vbn.aau.dk/en . Accessed 14 Aug 2021
Islam MM, Islam MR, Islam MS (2020) “An Efficient Human Computer Interaction through Hand Gesture Using Deep Convolutional Neural Network,” SN Comput Sci, vol. 1, no. 4, https://doi.org/10.1007/s42979-020-00223-x .
Islam MM, Islam MZ, Asraf A, Ding W (2020) “Diagnosis of COVID-19 from X-rays using combined CNN-RNN architecture with transfer learning,” medRxiv, https://doi.org/10.1101/2020.08.24.20181339 .
Islam MR, Moni MA, Islam MM, Rashed-al-Mahfuz M, Islam MS, Hasan MK, Hossain MS, Ahmad M, Uddin S, Azad A, Alyami SA, Ahad MAR, Lio P (2021) Emotion recognition from EEG signal focusing on deep learning and shallow learning techniques. IEEE Access 9:94601–94624. https://doi.org/10.1109/ACCESS.2021.3091487
Islam MR et al (2021) EEG Channel Correlation Based Model for Emotion Recognition. Comput Biol Med 136(May):104757. https://doi.org/10.1016/j.compbiomed.2021.104757
Issa D, Fatih Demirci M, Yazici A (2020) Speech emotion recognition with deep convolutional neural networks. Biomed Signal Process Control 59:1–11. https://doi.org/10.1016/j.bspc.2020.101894
Jackson P, Haq S (2011) Surrey Audio-Visual Expressed Emotion (SAVEE) database. http://kahlan.eps.surrey.ac.uk/savee . Accessed 17 Sept 2021
Jaiswal JK, Samikannu R (2017) “Application of Random Forest Algorithm on Feature Subset Selection and Classification and Regression,” in 2017 World congress on computing and communication technologies (WCCCT), pp. 65–68, https://doi.org/10.1109/WCCCT.2016.25 .
Jaratrotkamjorn A, Choksuriwong A (2019) “Bimodal Emotion Recognition using Deep Belief Network,” ICSEC 2019 - 23rd International Computer Science and Engineering Conference, pp. 103–109, https://doi.org/10.1109/ICSEC47112.2019.8974707 .
Jiang P, Fu H, Tao H, Lei P, Zhao L (2019) Parallelized convolutional recurrent neural network with spectral features for speech emotion recognition. IEEE Access 7:90368–90377. https://doi.org/10.1109/ACCESS.2019.2927384
Jin Y, Zha C, Zhao L, Song P (2015) Speech emotion recognition method based on hidden factor analysis. Electron Lett 51(1):112–114. https://doi.org/10.1049/el.2014.3339
Jing S, Mao X, Chen L (2018) Prominence features: effective emotional features for speech emotion recognition. Digit Signal Process A Rev J 72:216–231. https://doi.org/10.1016/j.dsp.2017.10.016
Kamble VV, Gaikwad BP, Rana DM (2014) “Spontaneous emotion recognition for Marathi Spoken Words,” International Conference on Communication and Signal Processing, ICCSP 2014 - Proceedings, pp. 1984–1990, https://doi.org/10.1109/ICCSP.2014.6950191 .
Kandali AB, Routray A, Basu TK (2008) “Emotion recognition from Assamese speeches using MFCC features and GMM classifier,” IEEE Region 10 Annual International Conference, Proceedings/TENCON, https://doi.org/10.1109/TENCON.2008.4766487 .
Kate Dupuis MKP-F (2010) Toronto emotional speech set (TESS). University of Toronto , Psychology Department. https://tspace.library.utoronto.ca/handle/1807/24487 . Accessed 08.10.2021
Kattubadi IB, Garimella RM (2019) “Emotion Classification: Novel Deep Learning Architectures,” 2019 5th International Conference on Advanced Computing and Communication Systems, ICACCS 2019, pp. 285–290, https://doi.org/10.1109/ICACCS.2019.8728519 .
Khalil RA, Jones E, Babar MI, Jan T, Zafar MH, Alhussain T (2019) Speech emotion recognition using deep learning techniques: a review. IEEE Access 7:117327–117345. https://doi.org/10.1109/access.2019.2936124
Khan A, Islam M (2016) Deep belief networks. In: Proceedings of Introduction to Deep Neural Networks At: PIEAS, Islamabad, Pakistan. https://doi.org/10.13140/RG.2.2.17217.15200
King DE (2009) Dlib-ml: a machine learning toolkit. J Mach Learn Res 10:1755–1758
Koolagudi SG, Rao KS (2012) Emotion recognition from speech: a review. Int J Speech Technol 15(2):99–117. https://doi.org/10.1007/s10772-011-9125-1
Koolagudi SG, Rao KS (2012) Emotion recognition from speech using source, system, and prosodic features. Int J Speech Technol 15(2):265–289. https://doi.org/10.1007/s10772-012-9139-3
Koolagudi SG, Maity S, Kumar VA, Chakrabarti S, Rao KS (2009) “IITKGP-SESC: Speech Database for Emotion Analysis,” in Contemporary Computing, pp. 485–492
Koolagudi SG, Reddy R, Yadav J, Rao KS (2011) “IITKGP-SEHSC : Hindi speech corpus for emotion analysis,” 2011 International Conference on Devices and Communications, ICDeCom 2011 - Proceedings, https://doi.org/10.1109/ICDECOM.2011.5738540 .
Koolagudi SG, Murthy YVS, Bhaskar SP (2018) Choice of a classifier, based on properties of a dataset: case study-speech emotion recognition. Int J Speech Technol 21(1):167–183. https://doi.org/10.1007/s10772-018-9495-8
Krothapalli SR, Koolagudi SG (2013) Characterization and recognition of emotions from speech using excitation source information. Int J Speech Technol 16(2):181–201. https://doi.org/10.1007/s10772-012-9175-z
Kubat M (1999) Neural networks: a comprehensive foundation. Knowl Eng Rev 13(4):409–412. https://doi.org/10.1017/S0269888998214044
Kuchibhotla S, Vankayalapati HD, Vaddi RS, Anne KR (2014) A comparative analysis of classifiers in emotion recognition through acoustic features. Int J Speech Technol 17(4):401–408. https://doi.org/10.1007/s10772-014-9239-3
Kuchibhotla S, Deepthi H, Koteswara V, Anne R (2016) An optimal two stage feature selection for speech emotion recognition using acoustic features. Int J Speech Technol 19(4):657–667. https://doi.org/10.1007/s10772-016-9358-0
Kwon O-W, Chan K, Hao J, Lee T-W (2003) Emotion recognition by speech signals. In: 8th European Conference on Speech Communication and Technology. https://doi.org/10.21437/eurospeech.2003-80
Lalitha S, Mudupu A, Nandyala BV, Munagala R (2015) “Speech emotion recognition using DWT,” 2015 IEEE international conference on computational intelligence and computing research, ICCIC, 2016, https://doi.org/10.1109/ICCIC.2015.7435630 .
Lalitha S, Tripathi S, Gupta D (2019) Enhanced speech emotion detection using deep neural networks. Int J Speech Technol 22(3):497–510. https://doi.org/10.1007/s10772-018-09572-8
Langley P, Iba W, Thompson K (1998) “An Analysis of Bayesian Classifiers,” Proceedings of the Tenth National Conference on Artificial Intelligence, vol. 90
Lee C-Y, Chen B-S (2018) Mutually-exclusive-and-collectively-exhaustive feature selection scheme. Appl Soft Comput 68:961–971. https://doi.org/10.1016/j.asoc.2017.04.055
Lee C, Narayanan SS, Pieraccini R (2002) Classifying emotions in human-machine spoken dialogs. Proceed IEEE Int Conf Multimed Expo 1:737–740
Lee KH, Kyun Choi H, Jang BT, Kim DH (2019) “A Study on Speech Emotion Recognition Using a Deep Neural Network,” ICTC 2019 - 10th International Conference on ICT Convergence: ICT Convergence Leading the Autonomous Future, pp. 1162–1165, https://doi.org/10.1109/ICTC46691.2019.8939830 .
Li X (2007) SPEech Feature Toolbox (SPEFT) design and emotional speech feature extraction. https://epublications.marquette.edu/theses/1315 . Accessed 25 Aug 2021
Li J, Fu X, Shao Z, Shang Y (2019) “Improvement on Speech Depression Recognition Based on Deep Networks,” Proceedings 2018 Chinese Automation Congress, CAC 2018, pp. 2705–2709, https://doi.org/10.1109/CAC.2018.8623055 .
Li Y, Baidoo C, Cai T, Kusi GA (2019) “Speech Emotion Recognition Using 1D CNN with No Attention,” ICSEC 2019 - 23rd International Computer Science and Engineering Conference, pp. 351–356, https://doi.org/10.1109/ICSEC47112.2019.8974716 .
Li Z, Li J, Ma S, Ren H (2019) “Speech emotion recognition based on residual neural network with different classifiers,” Proceedings - 18th IEEE/ACIS International Conference on Computer and Information Science, ICIS 2019, pp. 186–190, https://doi.org/10.1109/ICIS46139.2019.8940308 .
Li D, Liu J, Yang Z, Sun L, Wang Z (2021) Speech Emotion Recognition Using Recurrent Neural Networks with Directional Self-Attention. Exp Syst Appl 173:11468. https://doi.org/10.1016/j.eswa.2021.114683
Liberman M (2002) Emotional prosody speech and transcripts. University of Pennsylvania. https://catalog.ldc.upenn.edu/LDC2002S28 . Accessed 14 Oct 2021
Lim W, Jang D, Lee T (2016) “Speech emotion recognition using convolutional and Recurrent Neural Networks,” 2016 Asia-Pacific signal and information processing association annual summit and conference , APSIPA, 2017, https://doi.org/10.1109/APSIPA.2016.7820699 .
Litman DJ, Forbes-Riley K (2004) “Predicting Student Emotions in Computer-Human Tutoring Dialogues,” in Proceedings of the 42nd Annual Meeting on Association for Computational Linguistics, pp. 351–358, https://doi.org/10.3115/1218955.1219000 .
Liu Y, Zhou Y, Wen S, Tang C (2014) A strategy on selecting performance metrics for classifier evaluation. Int J Mob Comput Multimed Commun 6(4):20–35. https://doi.org/10.4018/IJMCMC.2014100102
Liu B, Zhou Y, Xia Z, Liu P, Yan Q, Xu H (2018) Spectral regression based marginal Fisher analysis dimensionality reduction algorithm. Neurocomputing 277:101–107. https://doi.org/10.1016/j.neucom.2017.05.097
Liu ZT, Xie Q, Wu M, Cao WH, Mei Y, Mao JW (2018) Speech emotion recognition based on an improved brain emotion learning model. Neurocomputing 309:145–156. https://doi.org/10.1016/j.neucom.2018.05.005
Liu ZT, Wu M, Cao WH, Mao JW, Xu JP, Tan GZ (2018) Speech emotion recognition based on feature selection and extreme learning machine decision tree. Neurocomputing 273:271–280. https://doi.org/10.1016/j.neucom.2017.07.050
Livingstone S, Russo F (2018) The Ryerson audio-visual database of emotional speech and Song (RAVDESS): a dynamic, multimodal set of facial and vocal expressions in north American English. PLoS One 13:e0196391. https://doi.org/10.1371/journal.pone.0196391
Loizou PC (1998) COLEA: A MATLAB software tool for speech analysis. https://ecs.utdallas.edu/loizou/speech/colea.htm . Accessed 20 Oct 2021
Lotfian R, Busso C (2019) Building naturalistic emotionally balanced speech Corpus by retrieving emotional speech from existing podcast recordings. IEEE Trans Affect Comput 10(4):471–483. https://doi.org/10.1109/TAFFC.2017.2736999
Luengo I, Navas E, Hernáez I (2010) Feature analysis and evaluation for automatic emotion identification in speech. IEEE Trans Multimed 12(6):490–501. https://doi.org/10.1109/TMM.2010.2051872
Majkowski A, Kołodziej M, Rak RJ, Korczynski R (2016) “Classification of emotions from speech signal,” Signal Processing - Algorithms, Architectures, Arrangements, and Applications Conference Proceedings, SPA, pp. 276–281, https://doi.org/10.1109/SPA.2016.7763627 .
Manjunath R (2013) Dimensionality reduction and classification of color features data using svm and knn. Int J Image Process Vis Commun 1:16–21
Mannepalli K, Sastry PN, Suman M (2016) FDBN: design and development of fractional deep belief networks for speaker emotion recognition. Int J Speech Technol 19(4):779–790. https://doi.org/10.1007/s10772-016-9368-y
Mao KZ (2004) Orthogonal forward selection and backward elimination algorithms for feature subset selection. IEEE Trans Syst Man, Cybern Part B (Cybernetics) 34(1):629–634. https://doi.org/10.1109/TSMCB.2002.804363
Mao X, Chen L (2010) Speech emotion recognition based on parametric filter and fractal dimension. IEICE Trans Inf Syst E93-D(8):2324–2326. https://doi.org/10.1587/transinf.E93.D.2324
Mao Q, Dong M, Huang Z, Zhan Y (2014) Learning salient features for speech emotion recognition using convolutional neural networks. IEEE Trans Multimed 16(8):2203–2213. https://doi.org/10.1109/TMM.2014.2360798
Marill T, Green D (1963) On the effectiveness of receptors in recognition systems. IEEE Trans Inf Theory 9(1):11–17. https://doi.org/10.1109/TIT.1963.1057810
Martin O, Kotsia I, Macq B, Pitas I (2006) “The eNTERFACE’ 05 audio-visual emotion database,” https://doi.org/10.1109/ICDEW.2006.145 .
Martinez AM, Kak AC (Feb. 2001) PCA versus LDA. IEEE Trans Pattern Anal Mach Intell 23(2):228–233. https://doi.org/10.1109/34.908974
McFee B et al. (2015) “librosa: Audio and Music Signal Analysis in Python,” Proceedings of the 14th Python in Science Conference, no. Scipy, pp. 18–24, https://doi.org/10.25080/majora-7b98e3ed-003 .
Meftah A, Alotaibi Y, Selouani SA (2014) Designing, building, and analyzing an Arabic speech emotional Corpus. In: Ninth International Conference on Language Resources and Evaluation at: Reykjavik, Iceland
Meftah A, Alotaibi Y, Selouani S-A (2016) “Emotional Speech Recognition: A Multilingual Perspective,” 2016 International Conference on Bio-Engineering for Smart Technologies(Biosmart)
Milton A, Tamil Selvi S (2014) Class-specific multiple classifiers scheme to recognize emotions from speech signals. Comput Speech Lang 28(3):727–742. https://doi.org/10.1016/j.csl.2013.08.004
Mohanta A, Sharma U (2016) “Bengali Speech Emotion Recognition,” in 2016 3rd International Conference on Computing for Sustainable Global Development (INDIACom), pp. 2812–2814
Montenegro CS, Maravillas EA (2015) “Acoustic-prosodic recognition of emotion in speech,” 8th International Conference on Humanoid, Nanotechnology, Information Technology, Communication and Control, Environment and Management, HNICEM, 2016, https://doi.org/10.1109/HNICEM.2015.7393229 .
Morin D (2004) “Beads-on-A-string,” Encyclopedic Dictionary of Genetics, Genomics and Proteomics, pp. 1–22, https://doi.org/10.1002/0471684228.egp01270 .
Mustafa MB, Yusoof MAM, Don ZM, Malekzadeh M (2018) Speech emotion recognition research: an analysis of research focus. Int J Speech Technol 21(1):137–156. https://doi.org/10.1007/s10772-018-9493-x
Nanavare VV, Jagtap SK (2015) Recognition of human emotions from speech processing. Proced Comput Sci 49(1):24–32. https://doi.org/10.1016/j.procs.2015.04.223
Nematollahi AF, Rahiminejad A, Vahidi B (2020) A novel meta-heuristic optimization method based on golden ratio in nature. Soft Comput 24(2):1117–1151. https://doi.org/10.1007/s00500-019-03949-w
Nicholson J, Takahashi K, Nakatsu R (2000) Emotion recognition in speech using neural networks. Neural Comput Applic 9(4):290–296. https://doi.org/10.1007/s005210070006
Nooteboom S (1997) The prosody of speech: Melody and rhythm. Handbook Phon Sci, vol 5
O’Shea K, Nash R (2015) An introduction to convolutional neural networks. ArXiv e-prints. https://doi.org/10.48550/arXiv.1511.08458
Ortony A, Clore GL, Collins A (1988) The cognitive structure of emotions. Cambridge University Press, Cambridge
Book Google Scholar
Özseven T, Düğenci M (2018) SPeech ACoustic (SPAC): A novel tool for speech feature extraction and classification. Appl Acoust 136(February):1–8. https://doi.org/10.1016/j.apacoust.2018.02.009
Palo HK, Sagar S (2018) Comparison of Neural Network Models for Speech Emotion Recognition. Proceed 2nd Int Conf Data Sci Bus Anal ICDSBA 20:127–131. https://doi.org/10.1109/ICDSBA.2018.00030
Palo HK, Mohanty MN, Chandra M (2016) Efficient feature combination techniques for emotional speech classification. Int J Speech Technol 19(1):135–150. https://doi.org/10.1007/s10772-016-9333-9
Pandey SK, Shekhawat HS, Prasanna SRM (2019) “Deep Learning Techniques for Speech Emotion Recognition: A Review,” in 2019 29th international conference RADIOELEKTRONIKA (RADIOELEKTRONIKA), pp. 1–6, https://doi.org/10.1109/RADIOELEK.2019.8733432 .
Partila P, Tovarek J, Voznak M, Rozhon J, Sevcik L, Baran R (2018) “Multi-Classifier Speech Emotion Recognition System,” 2018 26th Telecommunications Forum, TELFOR 2018 - Proceedings, pp. 1–4, https://doi.org/10.1109/TELFOR.2018.8612050 .
Pathak BV, Patil DR, More SD, Mhetre NR (2019) “Comparison between five classification techniques for classifying emotions in human speech,” 2019 International Conference on Intelligent Computing and Control Systems, ICCS 2019, pp. 201–207, https://doi.org/10.1109/ICCS45141.2019.9065620 .
Pedregosa F et al. (2012) “Scikit-learn: Machine Learning in Python,” J Mach Learn Res, vol. 12
Petrushin V (2000) “Emotion in speech: recognition and application to call centers,” Proceedings of Artificial Neural Networks in Engineering
Picard RW (1997) Affective computing. MIT Press. https://direct.mit.edu/books/book/4296/Affective-Computing . Accessed 24 Jun 2021
Pratiwi O, Rahardjo B, Supangkat S (2015) “Attribute Selection Based on Information Gain for Automatic Grouping Student System,” in Communications in Computer and Information Science, vol. 516, pp. 205–211, https://doi.org/10.1007/978-3-662-46742-8_19 .
Prinz J (2004) Which emotions are basic? Oxford University Press. https://doi.org/10.1093/acprof:oso/9780198528975.003.0004
Pudil S, Pavel, N, Jana, Bláha (1991) “Statistical approach to pattern recognition: Theory and practical solution by means of PREDITAS system,” Kybernetika 27, vol. 1, no. 76
Pyrczak F, Oh DM, Pyrczak F, Oh DM (2019) “Introduction to the t test,” https://doi.org/10.4324/9781315179803-28 .
Qayyum CSABA, Arefeen A (2019) “Convolutional Neural Network ( CNN ) Based Speech Recognition,” in 2019IEEE International Conference onSignal Processing, Information, Communication & Systems(SPICSCON, pp. 122–125
Rabiner LR (1989) A tutorial on hidden Markov models and selected applications in speech recognition. Proc IEEE 77(2):257–286. https://doi.org/10.1109/5.18626
Rahman MM, Islam MM, Manik MMH, Islam MR, Al-Rakhami MS (2021) “Machine Learning Approaches for Tackling Novel Coronavirus (COVID-19) Pandemic,” SN Comput Sci, vol. 2, no. 5, https://doi.org/10.1007/s42979-021-00774-7 .
Rajisha TM, Sunija AP, Riyas KS (2016) Performance analysis of Malayalam language speech emotion recognition system using ANN/SVM. Proced Technol 24:1097–1104. https://doi.org/10.1016/j.protcy.2016.05.242
Rajoo R, Aun CC (2016) “Influences of languages in speech emotion recognition: A comparative study using Malay, English and Mandarin languages,” ISCAIE 2016–2016 IEEE Symposium on Computer Applications and Industrial Electronics, pp. 35–39, https://doi.org/10.1109/ISCAIE.2016.7575033 .
Ram CS, Ponnusamy R (2014) “An effective automatic speech emotion recognition for Tamil language using Support Vector Machine,” in 2014 International Conference on Issues and Challenges in Intelligent Computing Techniques (ICICT), pp. 19–23, https://doi.org/10.1109/ICICICT.2014.6781245 .
Rao KS, Koolagudi SG, Vempada RR (2013) Emotion recognition from speech using global and local prosodic features. Int J Speech Technol 16(2):143–160. https://doi.org/10.1007/s10772-012-9172-2
Ren M, Nie W, Liu A, Su Y (2019) Multi-modal correlated network for emotion recognition in speech. Vis Inf 3(3):150–155. https://doi.org/10.1016/j.visinf.2019.10.003
Revathi A, Jeyalakshmi C (2019) Emotions recognition: different sets of features and models. Int J Speech Technol 22(3):473–482. https://doi.org/10.1007/s10772-018-9533-6
Roccetti M, Delnevo G, Casini L, Mirri S (2021) An alternative approach to dimension reduction for pareto distributed data: a case study. J Big Data 8(1):39. https://doi.org/10.1186/s40537-021-00428-8
Rong J, Li G, Chen YPP (2009) Acoustic feature selection for automatic emotion recognition from speech. Inf Process Manag 45(3):315–328. https://doi.org/10.1016/j.ipm.2008.09.003
Roubos H, Setnes M, Abonyi J (2000) Learning fuzzy classification rules from data, vol 150. In: Proceedings Developments in Soft Computing, pp 108–115. https://doi.org/10.1007/978-3-7908-1829-1_13
Ryerson RU (2017) Multimedia research lab. RML Emotion Database. http://shachi.org/resources/4965 . Accessed 30 Oct 2021
Sadeghyan S (2018) A new robust feature selection method using variance-based sensitivity analysis. arXiv. https://doi.org/10.48550/arXiv.1804.05092
Sari H, Cochet PY (1996) “Transform-Domain Signal Processing in Digital Communications,” in Signal Processing in Telecommunications, pp. 374–384
Sarikaya R, Hinton GE, Deoras A (2014) Application of deep belief networks for natural language understanding. IEEE Trans Audio Speech Lang Process 22(4):778–784. https://doi.org/10.1109/TASLP.2014.2303296
Savargiv M, Bastanfard A (2013) “Text material design for fuzzy emotional speech corpus based on persian semantic and structure,” in 2013 International Conference on Fuzzy Theory and Its Applications (iFUZZY), pp. 380–384, https://doi.org/10.1109/iFuzzy.2013.6825469 .
Savargiv M, Bastanfard A (2015) “Persian speech emotion recognition,” in 2015 7th Conference on Information and Knowledge Technology (IKT), pp. 1–5, https://doi.org/10.1109/IKT.2015.7288756 .
Schlosberg H (1954) Three dimensions of emotion. Psychol Rev 61(2):81–88
Schmidhuber J (2015) Deep learning in neural networks: An overview. Neural Netw 61:85–117. https://doi.org/10.1016/j.neunet.2014.09.003
Schubiger M (1958) English intonation:its form and function. M. Niemeyer Verlag, Tübingen
Schuller B, Batliner A, Steidl S, Seppi D (2011) Recognising realistic emotions and affect in speech: state of the art and lessons learnt from the first challenge. Speech Comm 53(9–10):1062–1087. https://doi.org/10.1016/j.specom.2011.01.011
Schuller B et al (2013) The INTERSPEECH 2013 computational paralinguistics challenge: social signals, conflict, emotion, autism. In: Proceedings 14th Annual Conference of the International Speech Communication Association. https://doi.org/10.21437/Interspeech.2013-56
Shah Fahad M, Ranjan A, Yadav J, Deepak A (2021) A survey of speech emotion recognition in natural environment. Digit Signal Process A Rev J 110:10295. https://doi.org/10.1016/j.dsp.2020.102951
Shahin I, Nassif AB, Hamsa S (2019) Emotion recognition using hybrid Gaussian mixture model and deep neural network. IEEE Access 7:26777–26787. https://doi.org/10.1109/ACCESS.2019.2901352
Sheikhan M, Bejani M, Gharavian D (2013) Modular neural-SVM scheme for speech emotion recognition using ANOVA feature selection method. Neural Comput & Applic 23(1):215–227. https://doi.org/10.1007/s00521-012-0814-8
Spolaôr N, Cherman EA, Monard MC, Lee HD (2013) “ReliefF for multi-label feature selection,” Proceedings - 2013 Brazilian Conference on Intelligent Systems, BRACIS 2013, pp. 6–11, https://doi.org/10.1109/BRACIS.2013.10 .
Steidl S (2009) Automatic classification of emotion related user states in spontaneous children’s speech. Logos Verlag. http://www5.informatik.uni-erlangen.de . Accessed 03.06.2021
Suganya S, Charles E (2019) “Speech emotion recognition using deep learning on audio recordings,” https://doi.org/10.1109/ICTer48817.2019.9023737 .
Sun Y, Wong AKC, Kamel MS (2009) Classification of imbalanced data: a review. Int J Pattern Recognit Artif Intell 23(4):687–719. https://doi.org/10.1142/S0218001409007326
Sun L, Zou B, Fu S, Chen J, Wang F (2019) “Speech emotion recognition based on DNN-decision tree SVM model,” Speech Commun, https://doi.org/10.1016/j.specom.2019.10.004 .
Swain M, Routray A, Kabisatpathy P, Kundu JN (2017) “Study of prosodic feature extraction for multidialectal Odia speech emotion recognition,” IEEE Region 10 Annual International Conference, Proceedings/TENCON, pp 1644–1649, https://doi.org/10.1109/TENCON.2016.7848296 .
Swain M, Routray A, Kabisatpathy P (2018) Databases, features and classifiers for speech emotion recognition: a review. Int J Speech Technol 21(1):93–120. https://doi.org/10.1007/s10772-018-9491-z
Tacconi D et al. (2008) “Activity and emotion recognition to support early diagnosis of psychiatric diseases,” in 2008 Second International Conference on Pervasive Computing Technologies for Healthcare, pp. 100–102, https://doi.org/10.1109/PCTHEALTH.2008.4571041 .
Taha M, Adeel A, Hussain A (2018) A survey on techniques for enhancing speech. Int J Comput Appl 179(17):1–14. https://doi.org/10.5120/ijca2018916290
Trigeorgis G, Nicolaou MA, Schuller W (2017) End-to-end multimodal emotion recognition using deep neural networks. IEEE J Select Top Signal Process 11(8):1301–1309
Uhrin D, Partila P, Voznak M, Chmelikova Z, Hlozak M, Orcik L (2014) “Design and implementation of Czech database of speech emotions,” 2014 22nd Telecommunications Forum, TELFOR 2014 - Proceedings of Papers, no. November, pp. 529–532, https://doi.org/10.1109/TELFOR.2014.7034463 .
Urbanowicz RJ, Meeker M, La Cava W, Olson RS, Moore JH (2018) Relief-based feature selection: Introduction and review. J Biomed Inf 85:189–203. https://doi.org/10.1016/j.jbi.2018.07.014
Valstar M et al. (2014) “AVEC 2014 - 3D dimensional affect and depression recognition challenge,” AVEC 2014 - Proceedings of the 4th International Workshop on Audio/Visual Emotion Challenge, Workshop of MM 2014, no. January 2021, pp. 3–10, https://doi.org/10.1145/2661806.2661807 .
Van Der Maaten LJP, Postma EO, Van Den Herik HJ (2009) Dimensionality reduction: a comparative review. J Mach Learn Res 10:1–41. https://doi.org/10.1080/13506280444000102
Van Lierde K, Moerman M, Vermeersch H, Van Cauwenberge P (1996) An introduction to computerised speech lab. Acta Otorhinolaryngol Belg 50(4):309–314
Ververidis D, Kotropoulos C (2006) Emotional speech recognition: resources, features, and methods. Speech Comm 48(9):1162–1181. https://doi.org/10.1016/j.specom.2006.04.003
Ververidis D, Kotropoulos C (2006) “Emotional speech recognition: resources, features, and methods,” Speech Commun, https://doi.org/10.1016/j.specom.2006.04.003 .
Vihari S, Murthy AS, Soni P, Naik DC (2016) Comparison of speech enhancement algorithms. Proced Comput Sci 89:666–676. https://doi.org/10.1016/j.procs.2016.06.032
Vlasenko B, Wendemuth A (2007) Tuning hidden Markov model for speech emotion recognition. DAGA 1:1
Vrebcevic N, Mijic I, Petrinovic D (2019) “Emotion classification based on convolutional neural network using speech data,” 2019 42nd International Convention on Information and Communication Technology, Electronics and Microelectronics, MIPRO 2019 - Proceedings, pp. 1007–1012, https://doi.org/10.23919/MIPRO.2019.8756867 .
Wang X, Paliwal KK (2003) Feature extraction and dimensionality reduction algorithms and their applications in vowel recognition. Pattern Recognit 36(10):2429–2439. https://doi.org/10.1016/S0031-3203(03)00044-X
Wang K, An N, Li BN, Zhang Y, Li L (2015) “Speech emotion recognition using Fourier parameters,” IEEE Trans Affect Comput
Wei H, Shi X, Yang J, Pu Y (2010) “Speech Independent Component Analysis,” in 2010 International Conference on Measuring Technology and Mechatronics Automation, vol. 3, pp. 445–448, https://doi.org/10.1109/ICMTMA.2010.604 .
Whitney AW (1971) A Direct Method of Nonparametric Measurement Selection. IEEE Trans Comput C–20(9):1100–1103. https://doi.org/10.1109/T-C.1971.223410
Williams CE, Stevens KN (1981) “Vocal correlates of emotional states,” in Speech Eval Psychiatry
Wu J (2017) Introduction to convolutional neural networks. Introduct Convolutional Neural Netw, pp 1–31. https://cs.nju.edu.cn/wujx/paper/CNN.pdf . Accessed 14 Nov 2021
Wu G, Li F (2021) A randomized exponential canonical correlation analysis method for data analysis and dimensionality reduction. Appl Numer Math 164:101–124. https://doi.org/10.1016/j.apnum.2020.09.013
Article MathSciNet MATH Google Scholar
Yao Z, Wang Z, Liu W, Liu Y, Pan J (2020) Speech emotion recognition using fusion of three multi-task learning-based classifiers: HSF-DNN, MS-CNN and LLD-RNN. Speech Commun 120(April 2019):11–19. https://doi.org/10.1016/j.specom.2020.03.005
Ye C, Liu J, Chen C, Song M, Bu J (2008) “Speech Emotion Classification on a Riemannian Manifold,” in Advances in Multimedia Information Processing - PCM 2008, pp. 61–69
Yegnanarayana B (2009) Artificial neural networks. PHI Learning Pvt. Ltd
Yildirim S, Narayanan S, Potamianos A (2011) Detecting emotional state of a child in a conversational computer game. Comput Speech Lang 25(1):29–44. https://doi.org/10.1016/j.csl.2009.12.004
Yu C, Aoki P, Woodruff A (2004) Detecting user engagement in everyday conversations. ArXiv. https://doi.org/10.48550/arXiv.cs/0410027
Zang Q, Wang SLK (2013) A database of elderly emotional speech. In: 2013 International symposium on signal processing, biomedical engineering and informatics
Zeynep Inanoglu RC (2005) Emotive alert: hmm-based emotion detection in voicemail messages. https://vismod.media.mit.edu/tech-reports/TR-585.pdf
Zhalehpour S, Onder O, Akhtar Z, Erdem C (2016) BAUM-1: A Spontaneous Audio-Visual Face Database of Affective and Mental States. IEEE Trans Affect Comput PP:1. https://doi.org/10.1109/TAFFC.2016.2553038
Zhang Z, Coutinho E, Deng J, Schuller B (2015) Cooperative learning and its application to emotion recognition from speech. IEEE/ACM Trans Audio Speech Lang Process 23(1):115–126. https://doi.org/10.1109/TASLP.2014.2375558
Zhang S, Zhang S, Huang T, Gao W (2018) Speech emotion recognition using deep convolutional neural network and discriminant temporal pyramid matching. IEEE Trans Multimed 20(6):1576–1590. https://doi.org/10.1109/TMM.2017.2766843
Zhang X, Wu G, Ren F (2018) “Searching Audio-Visual Clips for Dual-mode Chinese Emotional Speech Database,” 2018 1st Asian Conference on Affective Computing and Intelligent Interaction, ACII Asia 2018, pp. 1–6, https://doi.org/10.1109/ACIIAsia.2018.8470387 .
Zhang S, Chen A, Guo W, Cui Y, Zhao X, Liu L (2020) Learning deep binaural representations with deep convolutional neural networks for spontaneous speech emotion recognition. IEEE Access 8:23496–23505. https://doi.org/10.1109/ACCESS.2020.2969032
Zhang H, Huang H, Han H (2021) Attention-based convolution skip bidirectional long short-term memory network for speech emotion recognition. IEEE Access 9:5332–5342. https://doi.org/10.1109/ACCESS.2020.3047395
Zhang C, Liu Y, Fu H (n.d.) “AE 2 -Nets : Autoencoder in Autoencoder Networks,” in 2019 IEEE/CVF Conference on Computer Vision and Pattern Recognition (CVPR), pp. 2577–2585
Zhao J, Mao X, Chen L (2018) Learning deep features to recognise speech emotion using merged deep CNN. IET Signal Process 12(6):713–721. https://doi.org/10.1049/iet-spr.2017.0320
Zhao Z, Bao Z, Zhao Y, Zhang Z, Cummins N, Ren Z, Schuller B (2019) Exploring deep Spectrum representations via attention-based recurrent and convolutional neural networks for speech emotion recognition. IEEE Access 7:97515–97525. https://doi.org/10.1109/ACCESS.2019.2928625
Download references
Acknowledgements
We would like to thank IKG Punjab Technical University, Kapurthala, Punjab (India) for providing the opportunity to carry out the research work.
Author information
Authors and affiliations.
IKG Punjab Technical University, Kapurthala, Punjab, India
Kamaldeep Kaur
Department of Computer Science & Engineering, Guru Nanak Dev Engineering College, Ludhiana, Punjab, India
Kamaldeep Kaur & Parminder Singh
You can also search for this author in PubMed Google Scholar
Corresponding author
Correspondence to Kamaldeep Kaur .
Ethics declarations
Conflicts of interests.
The authors declare that they have no conflict of interest.
Additional information
Publisher’s note.
Springer Nature remains neutral with regard to jurisdictional claims in published maps and institutional affiliations.
Rights and permissions
Springer Nature or its licensor (e.g. a society or other partner) holds exclusive rights to this article under a publishing agreement with the author(s) or other rightsholder(s); author self-archiving of the accepted manuscript version of this article is solely governed by the terms of such publishing agreement and applicable law.
Reprints and permissions
About this article
Kaur, K., Singh, P. Trends in speech emotion recognition: a comprehensive survey. Multimed Tools Appl 82 , 29307–29351 (2023). https://doi.org/10.1007/s11042-023-14656-y
Download citation
Received : 15 December 2021
Revised : 06 April 2022
Accepted : 03 February 2023
Published : 22 February 2023
Issue Date : August 2023
DOI : https://doi.org/10.1007/s11042-023-14656-y
Share this article
Anyone you share the following link with will be able to read this content:
Sorry, a shareable link is not currently available for this article.
Provided by the Springer Nature SharedIt content-sharing initiative
- Classifiers
- Find a journal
- Publish with us
- Track your research
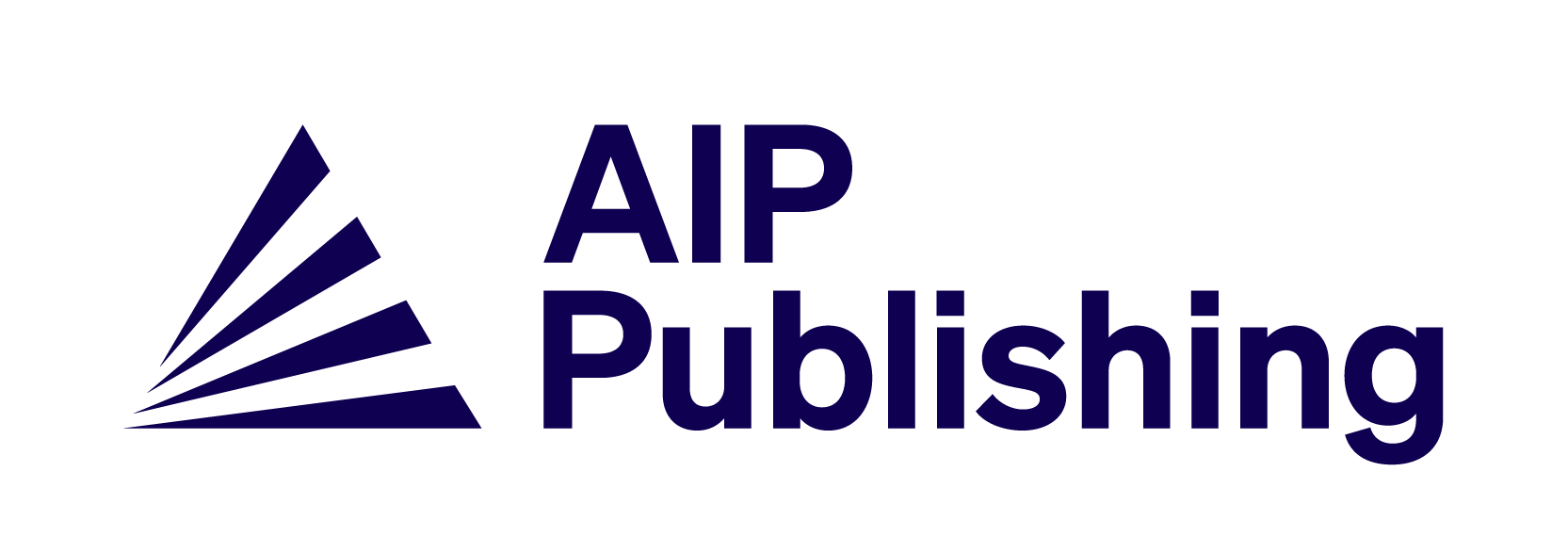
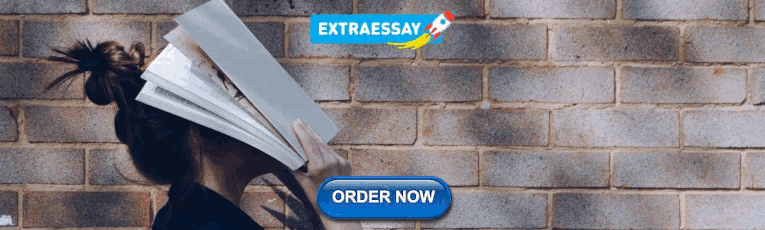
Speech emotion recognition methods: A literature review
- Split-Screen
- Article contents
- Figures & tables
- Supplementary Data
- Peer Review
- Open the PDF for in another window
- Reprints and Permissions
- Cite Icon Cite
- Search Site
Babak Basharirad , Mohammadreza Moradhaseli; Speech emotion recognition methods: A literature review. AIP Conf. Proc. 3 October 2017; 1891 (1): 020105. https://doi.org/10.1063/1.5005438
Download citation file:
- Ris (Zotero)
- Reference Manager
Recently, attention of the emotional speech signals research has been boosted in human machine interfaces due to availability of high computation capability. There are many systems proposed in the literature to identify the emotional state through speech. Selection of suitable feature sets, design of a proper classifications methods and prepare an appropriate dataset are the main key issues of speech emotion recognition systems. This paper critically analyzed the current available approaches of speech emotion recognition methods based on the three evaluating parameters (feature set, classification of features, accurately usage). In addition, this paper also evaluates the performance and limitations of available methods. Furthermore, it highlights the current promising direction for improvement of speech emotion recognition systems.
Citing articles via
Publish with us - request a quote.
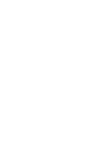
Sign up for alerts
- Online ISSN 1551-7616
- Print ISSN 0094-243X
- For Researchers
- For Librarians
- For Advertisers
- Our Publishing Partners
- Physics Today
- Conference Proceedings
- Special Topics
pubs.aip.org
- Privacy Policy
- Terms of Use
Connect with AIP Publishing
This feature is available to subscribers only.
Sign In or Create an Account
Thank you for visiting nature.com. You are using a browser version with limited support for CSS. To obtain the best experience, we recommend you use a more up to date browser (or turn off compatibility mode in Internet Explorer). In the meantime, to ensure continued support, we are displaying the site without styles and JavaScript.
- View all journals
- My Account Login
- Explore content
- About the journal
- Publish with us
- Sign up for alerts
- Open access
- Published: 25 July 2023
Speech emotion classification using attention based network and regularized feature selection
- Samson Akinpelu 1 na1 &
- Serestina Viriri 1 na1
Scientific Reports volume 13 , Article number: 11990 ( 2023 ) Cite this article
2694 Accesses
1 Citations
1 Altmetric
Metrics details
- Computational science
- Computer science
- Mathematics and computing
Speech emotion classification (SEC) has gained the utmost height and occupied a conspicuous position within the research community in recent times. Its vital role in Human–Computer Interaction (HCI) and affective computing cannot be overemphasized. Many primitive algorithmic solutions and deep neural network (DNN) models have been proposed for efficient recognition of emotion from speech however, the suitability of these methods to accurately classify emotion from speech with multi-lingual background and other factors that impede efficient classification of emotion is still demanding critical consideration. This study proposed an attention-based network with a pre-trained convolutional neural network and regularized neighbourhood component analysis (RNCA) feature selection techniques for improved classification of speech emotion. The attention model has proven to be successful in many sequence-based and time-series tasks. An extensive experiment was carried out using three major classifiers (SVM, MLP and Random Forest) on a publicly available TESS (Toronto English Speech Sentence) dataset. The result of our proposed model (Attention-based DCNN+RNCA+RF) achieved 97.8% classification accuracy and yielded a 3.27% improved performance, which outperforms state-of-the-art SEC approaches. Our model evaluation revealed the consistency of attention mechanism and feature selection with human behavioural patterns in classifying emotion from auditory speech.
Similar content being viewed by others
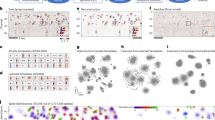
Spike sorting with Kilosort4
Marius Pachitariu, Shashwat Sridhar, … Carsen Stringer
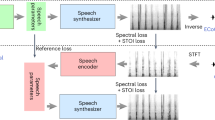
A neural speech decoding framework leveraging deep learning and speech synthesis
Xupeng Chen, Ran Wang, … Adeen Flinker
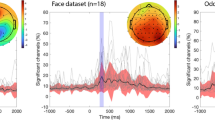
EEG is better left alone
Arnaud Delorme
Introduction
Human has various ways of exhibiting their emotion, which has placed them at the highest level of civilization among other creatures. These expressions can take the form of speech, facial, gestures, and other physiological modes. However, interaction and relationships among individuals are best sustained through communication from human speech. Human speech carries huge para-linguistic 1 content that can reveal the state of emotion, both in direct and indirect communication. Therefore, speech emotion classification has been occupying a key position in advancing affective computing and speech research domain. Besides, unlike other methods of recognizing emotion, speech emotion can be said to reveal 90% of the intent of the speaker without pretence, hence, the reason why it is sporadically attracting researchers within the last decade.
In SEC, the cultural and racial background may have a significant impact, but the ground truth remains that emotion is universal. Because of peculiarities associated with the speech emotion domain, efforts have been made by professionals to generate a standardized synthetic dataset (emotional corpus) that had been useful for conducting research on emotion classification 2 . Among these corpora are IEMOCAP (Interactive and Diadic Motion Capture), TESS (Toronto English Speech Set), RAVDESS (Rayson Visual Emotion Speech Set), EMOVO, etc and their performances concerning speech emotion classification has been yielding appreciable result, even when sometimes compared with real world dataset. These datasets came in different languages (English, Spanish, German, Chinese) 3 . Speech emotion classification has its application in customer support management, self-driving cars, psycho-medicine, e-learning, etc. Its importance in human-computer interaction cannot be overemphasized. Gordon 4 opined that affective behaviour may serve as a precursor to the emergence of mental health conditions like depression and cognitive decline and may aid in the development of therapeutic tools for automatically identifying and tracking the progress of diseases.
Classical techniques of classifying emotion in the past follows the extraction of primitives, acoustic features and low-level detectors (LLD), from raw speech 5 . These features (pitch, energy, etc) represents frame-level features and speech analysis on it, do generate another level of features (Utterance-level). Thereafter, the concatenation of these feature in vector form will be fed into a machine learning algorithm also referred to as classifiers in this context, for actual classification of emotion. Support Vector Machine (SVM), Gaussian Mixture Model (GMM), Hidden Makov Model (HMM) and K-Nearest Neighbour (KNN) are popular classifiers 6 , 7 , 8 . Figure 1 shows a classical structure of emotion recognition.
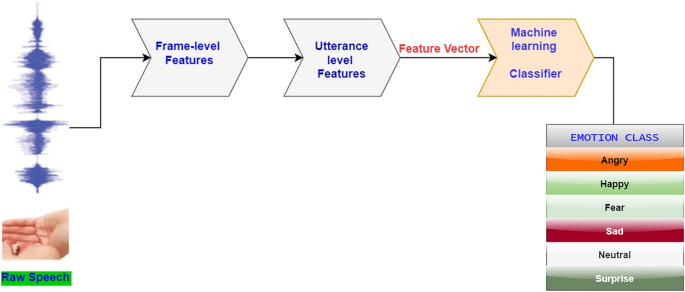
Conventional speech emotion classification system.
Though these approaches have proven to be efficient in their capacity, however, they are bewildered with salient challenges that rendered them unsuitable in achieving state-of-the-art result for SEC.
The focus of this study is to enhance and improve performance of speech emotion classification through attention-based network and feature selection techniques. To the best of our knowledge, this is the first-time feature selection is to be fused with attention layer of high dimensional features extracted from deep convolutional neural network, for accurate emotion classification (Attention based DCNN+RNCA+RF). We utilized TESS dataset in this study as a standard speech emotion corpus which captures seven classes of emotions express by human. The main contributions of this study are:
To experiment the efficiency of attention mechanism and regularized feature selection (Regularized Neighbourhood Component Analysis) techniques for speech emotion classification. A pretrained transfer learning network is set up as the based model. The feature selection neutralizes additional parameter weight added by attention layer and thereby minimize complexity.
To propose an Attention-based DCNN+RNCA+RF. After exploratory and thorough experiment with three different classifiers, our model achieved 97.8% accuracy on TESS dataset.
The remainder of the article is arranged as follows. An overview of related works is presented in Section “ Review of related works ”. The proposed technique and methods are described in Sect. “Methods and techniques ”. Results and discussions are given in Sect. " Experimental results and discussion ", while Sect. " Conclusion " is the conclusion and future recommendation for further study.
Review of related works
The classification of emotion has its history traced to psychological submission 9 , 10 where human emotion are grouped into six main classes (Sadness, Happiness, Anger, Disgust, Surprise and Fear). However, affective computing cannot be based on this primitive divisions, as computers are not perceiving moods, but they are interpreting them as a set of sequence of technical parameters, that are captured from the audio decoding process. Therefore, speech emotion classification requires efficient learning of paralinguistic information that can mitigates misclassification of emotion. The machine learning classifiers were first explored for SEC before the application of convolutional neural network models. The shortcomings of conventional classification approaches have paved ways for Convolutional Neural Networks (CNNs) 11 , 12 and LSTM networks 13 . Occasionally, these two combined to form a robust model 14 which have been widely employed in sequence modelling and its associated domain. A feature selection-based CNN was utilized by Farooq et al. 15 , for combating the artificial design influence which hampered accurate description of speakers’ emotional condition. Hajarolasvadi & Demirel 16 proposed 3D-CNN for speech emotion classification based on overlapping frames segregation and MFCC features extraction. A 10-fold cross-validation parameter was used in their evaluation on three publicly available speech corpora, which were Ryerson Multimedia Laboratory (RML), Survey Audio-Visual Expressed Emotion (SAVEE) and eNTERFACE’05. The convolutional model achieved 81.05% accuracy on six emotions classes. Deep Belief Network (DBN) and SVM was proposed in Zhu et al. 17 for extracting acoustics features, MFCC and zero-crossing rate were employed before emotion classification. Wang et al. 18 combined Deep Neural Network and Extreme Learning machine (ELM) for speech emotion classification through the encoding of speech features (pitch and formants) and segmentation of audio feature vectors.
However, conventional CNN performs woefully in high-dimensional speech features extraction. This, and many more shortcomings, paved the way for the introduction of recurrent neural network (RNN) model. It was a great milestone improvement over CNN in speech emotion classification, because it addresses the failure of CNN in time-series data extraction. RNN has a hidden layer in its structure that updates the output value with respect to time on constant basis 19 . Kerkeni et al. 20 proposed a RNN for speech emotion classification through analysis of speech signal using Teager-Kaiser Energy Operator (TKEO) combine with empirical mode decomposition (EMD). After extraction of speech cepstral features, SVM classifier was utilized for multi-classification of emotion. They achieved 91.16% on Berlin and Spanish based dataset. Nevertheless, RNN also suffers from dependency (long-term) and gradient descent problems. In some studies, CNN and RNN were combined to form a hybrid CRNN (Convolution Recurrent Neural Network) model to enhance speech emotion classification 21 .
As RNN is not isolated from its own limitations and by way of proffering quick fix to the issues peculiar to it, Long-Short-Term-Memory (LSTM) was proposed by Hochreiter & Schmidhuber 22 and its combination with convolutional neural network has yielded a notable improvement. LSTM is a variant of RNN consisting of feedback connections for dependency learning in sequence prediction. A 1D and 2D CNN was combined with LSTM for SEC, which resulted in an appreciable accuracy of 82.4% with EMO-DB speech corpus by Zhao et al. 23 . Puri et al. 24 , proposed a hybridized LSTM, CNN and DNN approach for speech emotion classification. MFCC and mel-spectrogram were fed into eight contiguous 2D convolutional sequential neural network layers of their model. RAVDESS dataset was used, but there was no accuracy of emotion recognition reported. Besides, their technique is expensive to train because of the huge convolutional layers adopted. LSTM has a key component called forget gate and research has proven that it has high probability of forgetting emotional feature, while it focuses on the most recent ones and this hampered its efficiency within SEC domain.
Recent advancement in deep learning coupled with incessant search for a way of improvement and addressing the age long challenges in SEC made Bahdanau et al. 25 , to introduce attention network which is able to sieve out irrelevant information peculiar to speech data and concentrate on emotional rich information. Attention mechanism has been successfully adapted to other object recognition discipline with a notable improvement in models’ performance. An attention-based network was adopted in the work of Qamhan et al. 26 , where an accuracy of over 60% was achieved on IEMOCAP dataset. Attention models emulate the human way of focusing on important features for the recognition of an object.
Three-dimensional attention-based CRNN was used by Chen et al. 27 to choose discriminative features for speech emotion classification. Their proposed model’s input layer accepted a Mel-spectrogram with delta-deltas. The employed delta-deltas reduced the intrusion of unimportant elements that can result in subpar classification performance, while keeping vital emotional data. Finally, a mechanism for attention that could take salient aspects into account was adopted. With an accuracy report of 82.82% on EMO-DB and 64.74% on the IEMOCAP speech dataset, their experiment’s outcome was supported the efficacy of attention technique for emotion classification.
Zhao et al. 28 . utilized attention-based model comprises Bidirectional LSTM, a Fully Connected Networks (FCN) for learning spatio-temporal emotional features and machine learning classifier for speech emotion classification. In the same vain, the author in Du et al. 29 . utilized attention-based model and 1Dimensional CNN for SEC. Softmax activation function was used at the top layer of their model after feature extraction. A cross-modal SEC was carried out in Seo and Kim 30 using Visual Attention Convolutional Neural Network (VACNN) in partitioning the spectral feature from dataset. Combining speech dataset with text and video requires special techniques in extracting features for efficient prediction of emotion. In Zhang et al. 31 , the author applied 5 attention heads mechanism for multimodal speech emotion classification. Their novel model achieved 75.6% on IEMOCAP dataset.
Zhang et al. 32 applied Deep convolutional Neural Network and attention-based network for emotion classification. In their method, a pre-trained DCNN was used as a based model in extracting segment-level features, before the introduction of Bidirectional LSTM for higher-level emotional features. Thereafter, an attention layer was introduced at the top layer of their model, with the utmost focus on features that are relevant to emotion recognition. Their model evaluation achieved UAR of 87.86% and 68.50% respectively on EMODB and IEMOCAP dataset. However, their experiment did not reflect the influence of speech enhancement carried out on raw speech. They augmented the speech corpus used through speed adjustment at varying time-step before the extraction of spectral features was fed into DCNN. Chen et al. 33 proposed self and global attention mechanism in determining the impact of the attention model on speech emotion classification. Their state-of-the-art approach achieved an accuracy of 85.43% on EMO-DB speech corpus. Their model was built using a sequential network, which requires more computing resources to train. In this paper, two pre-trainned DCNN model are used with attention model and regularized feature selection for SEC. More often than not, many researchers focused on the efficiency of attention mechanism as weight calculator in sequence representation Zhao et al. 34 , however, our proposed model has revealed that the performance of attention-based network is increased when co-join with regularized feature selection for SEC. Nevertheless, this paper concludes with an opportunity for future research in the use of attention mechanism and feature selection to improve the accuracy of classification (Fig. 2 ).
Methods and techniques
A general description of the model proposed is given in this section. As a classification problem, speech emotion is categorized rather than dimensional representations 35 . It can be defined as follows, \(D = {(X, z)}\) , where X are input from the acoustic features and z is dimensional output equivalent to the emotion classification. Also, a function \(D = {f : X \xrightarrow {} z}\) representing emotional features is to be found before its classification.
This study proposed a unique framework for speech emotion classification using attention-based mechanism on pretrained DCNN with regularized feature selection (RNCA) algorithm, as shown in Fig. 3 . There are four main phases in our model for speech emotion classification which includes, efficient pre-processing (pre-emphasis) of raw speech from TESS speech corpus, feature learning and extraction, feature selection and emotion classification. As noted in the literature 36 that the performance of any SEC model rests heavily on dataset pre-processing carried out. In this work, we extracted log mel-spectrogram with three channels (weight, height and input channel) from original speech database containing WAV files. Three channel mel-spectrogram usually comprises of the number of mel-filter banks (in terms of frequency dimension), frame number and the number of channel. The number of channels used for this paper is 3. Three different colours are used to indicate the magnitudes of the Short-Term Fourier Transform (STFT) in a three-channel mel-spectrogram. The low (below 500 Hz-blue), mid (between 500 Hz - 2 kHz-yellow), and high (above 2 kHz-red) frequency ranges of the audio signal are typically represented by the channels, which can offer a more intuitive visual form of the spectral content of the audio signal. The latter is used in this paper. Mel-spectrogram has been widely used 37 , 38 in speech-related task, and the reason is not far-fetched from the fact that it’s representation involves time and frequency of speech signals.signal.
At the pre-emphasis stage, the amplification of speech signals ( x ) to high frequency 39 is performed through a pre-emphasis filter using Eq. ( 1 ), where s ( t ) represent the speech audio signal before pre-emphasis. We utilized 64 mel-filter banks with 64 frames content window. To obtain the standard frame segment length, we processed \(655 ms (10 ms \times 63 + 25)\) fragments, however, a frame segment over 250 ms has been confirmed 40 to possess enough paralinguistic information rich enough for emotion classification. The speech signal framing adopted ensures the breaking down of the speech signal into segments of fixed-length. Because the length of human speech varies, framing is required to maintain the size of the voice. The hamming window function of 25 ms length and 10 ms shift was applied to frames as computed in Eq. ( 2 ), where S represents the size of the window w ( n ). This is illustrated in Fig. 2 .
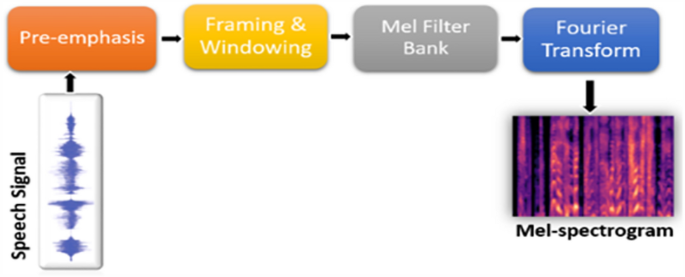
Structure of mel-spectrogram extraction.
The FFT (Fast Fourier Transform) is applied to produce a three channel mel-spectrogram suited as input to our model from raw speech signal with a sample frequency rate of 16 kHz . This mel-spectrogram can be represented as \(M, M \in R^{K \times L \times C}\) where the total number of the filter bank is denoted 32 by K in terms of dimension of the frequency, L denotes the length of the segment and the number of channels is C .
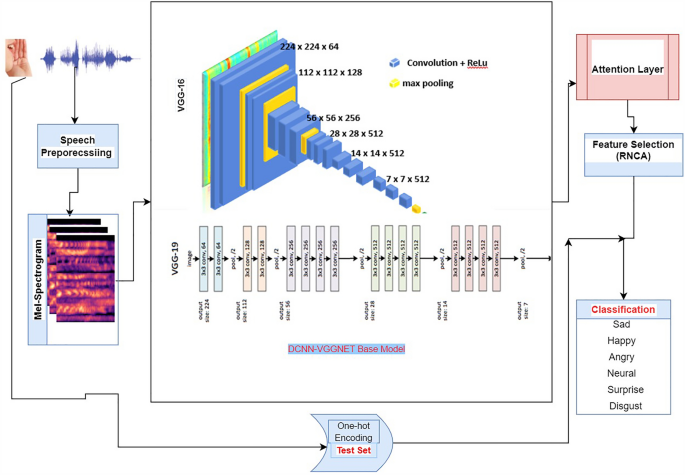
Proposed model architecture.
Feature extraction
In this research study, two pre-trained DCNN model serve as our based model (VGG16 and VGG19). We experimented with both pre-trained network on our attention mechanism to establish which one yields better classification performance accuracy after feature selection. We leverage on the weight of these two networks being already trained on ImageNet. Therefore, the convolutional layers comprised of our based model are frozen from training. The input to our model is reshaped from the original \(64\times 64 \times 3\) to \(224 \times 224\times 3\) , as the required input size to the base model of VGGNet.This is achieved using a built-in python library called OpenCV and a bilinear interpolation approach. The base model comprises five convolutional layers with ReLu (Reactivation Linear Unit) activation function for extracting segment-level features from the input mel-spectrogram. A drop-out layer is utilized to prevent overfitting. The output from based model feature extraction is also reshaped to make it suited for the attention layer in extracting high-level emotional features before it is fed into RNCA for eventual feature selection. This is carried out by The block diagram in Fig. 4 depicts the structure of DCNN phase of our model. The pooling layer adopted is max-pooling. This layer performs the function of aggregating the feature sample from the several convolutions of 2D convolutional layers and produces a unified output for the next layer. No fully connected layer was used in the base model.

Convolutional layers block diagram.
Attention layer
Attention mechanism application in computer vision has contributed immensely to the task of image recognition 41 . It mimics the human mode of paying a closer look at what are relevant information that may contribute to their opinion or conclusion on what they see and hear.
In the speech emotion task, the role of the attention network cannot be overlooked, as it carefully concentrates the focus of the model on the frame segment with much emotional content. The attention mechanism lowers the training time 42 and ensures concentration on features with much emotional information, which can increase model performance. Silent and semi-silent frames are eliminated at the attention layer, as this has a tendency of impairing and distorting the model accuracy. In other words, attention gives insight into the behavioural performance of the deep learning model as it calculates weight from feature representation from the previous layer. The Eq. ( 3 ) and ( 4 ) indicate how the attention mechanism utilized in this work is computed. Given \(X= (x_{1}, x_{2}...x_{n} )\) as the output of features from a convolutional layer.
where alpha \(\alpha _{i}\) represents the weight of the attention network, \(\mu\) and X are the output of feature representation from the attention layer. At first, the weight of the attention \(\alpha _{i}\) is calculated, and it is obtained from Eq. ( 3 ) (softmax function) through the training process. Y is got from the weighted sum of X , as deeper features at the utterance level. The attention mechanism has proven to be of tremendous help in generating more distinctive features for SEC. The attention layer is responsible for dynamically highlighting and weighting various input feature components according to their applicability to the emotion recognition task. The power of the model to successfully learn and represent the attention weights depends on the number of neurons in the attention layer. We used 128 neurons, increasing our model’s capacity for capturing fine-grained feature importance while minimizing complexity.
Regularized neighbourhood component analysis (RNCA) feature selection
The RNCA feature selection mechanism is a specific class of feature weighting approach that carries out its operation by learning feature weight and maximizing the leave-one-out (LOO) accuracy of classification over sample data 43 . The LOO provides an unbiased estimate of a deep learning model performance. RNCA works by assessing the vector weight w that corresponds to the feature vector \(x_i\) through the optimization of a classifier that is based on the nearest neighbour scheme. It has a mechanism for controlling complexity and preventing overfitting on the density estimation. RNCA adopts a framework of selecting a certain reference sample called \(x_j\) for the sample \(x_i\) from all emotion feature samples randomly. However, the probability of the selected feature \((P_{ij})\) to \(x_j\) rest heavily on the distance \(D_w\) that exists between two samples. This distance can be computed 44 as in Eq. ( 5 ) below:
Where mth the feature’s weight is denoted by \(w_m\) . A kernel function k established the relation \(P_{ij}\) and Dw on the condition that the smaller the Dw the larger the values of k. The likelihood \(P_{ij}\) and kernel function k can be computed for Eqs. 6 and 7 respectfully as below
where the kernel width is represented by \(\sigma\) that influences the likelihood that a reference point selected will be \(x_j\) sample. Therefore, the likelihood of correctly classifying \(x_i\) can be computed from Eq. ( 8 ).
Where \(y_{ij}\) can only indicate one if both \(y_i\) and \(y_j\) are equal to each other. The average LOO accuracy of classification is the sum of all \(P_i\) of all the samples divided by the total number of samples, as indicated in Eq. ( 9 ). This equation can be termed as the objective function that required maximization. Nevertheless, the objective function defined above is not insulated from overfitting, which calls for the introduction of a parameter \(\lambda\) termed regularizer to prevent overfitting. The modified objective function that represents RNCA can be defined as
The RNCA algorithm adopted in this work operates on the output from the attention layer of our model to aid feature selection, therefore, it is essential to evaluate generalization error (Eq. 10 ) to properly fine-tune the regularization parameter \(\lambda\) to obtain a minimized classification loss.
where the predicted label is represented by \(k_i\) and \(t_i\) denotes the real label of the feature sample. The RNCA feature selection technique is diagrammatically shown in Fig. 5 .
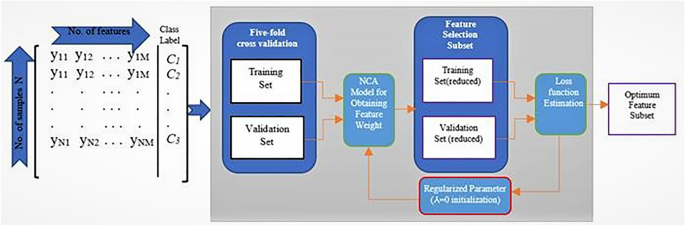
RNCA framework.
Emotion classification
In this study, three primitive classifiers were utilized in carrying out the classification of emotion. The classifiers take their simplified input from the output of the feature selection layer of our model after feature extraction. The essence of employing three different classifiers is to ensure the robustness of the entire model and aid the analysis of the result. Multi-layer perceptron (MLP) classifier is first introduced. As a feedforward network-based classifier 6 , a set of suitable outputs are mapped from a set of input datasets by this feedforward artificial neural network model. An MLP is made up of several layers, each of which is completely connected to the one before it. Except for the nodes in the input layer, the nodes of the layers represent neurons with nonlinear activation functions.
Secondly, we also utilized a support vector machine (SVM). An SVM operates as a discriminative classifier, well-defined by dividing hyperplane. It fits into supervised and unsupervised machine-learning tasks. For instance, given a set of selected features (or data), the algorithm outputs an optimal hyperplane that classifies new samples. In two-dimensional space, this hyperplane is a line dividing a plane into two parts, wherein each class lay on either side 45 . Besides, SVM can effectively handle multiclass problems as it is obtainable with emotion classification. One distinguishable function of SVM is that it selects a hyper-plane with a large margin, reducing the likelihood of miss-classification and its low sensitivity to outliers.
Lastly, Random Forest (RF) was also employed as the third classifier. RF is a meta-estimator that employs averaging to increase classification accuracy and reduce overfitting after fitting several decision tree classifiers to different emotional feature subsamples. Random forest possesses an inbuilt mechanism for managing class imbalance, and this has given it an edge over other classifiers.
Experimental results and discussion
In this study, we benchmarked our experiment on one of the publicly available datasets named Toronto English Speech Set (TESS). In 2010, at Northwestern University’s Auditory Laboratory, TESS speech samples were recorded 46 . During the spontaneous event, two actresses were asked to recite a handful of the 200 words, and their voices were recorded, resulting in a complete collection of 2800 speech utterances. Seven different emotions comprise happy, angry, fear, disgust, pleasant, surprise, sad and neutral were observed in the scene.
Experimental configuration
In this study, the experiment was carried out using a 64-bit operating system, an Intel Core i7 processor, 8 GB of RAM, and a Python 3.9 environment. Deep learning software and additional third-party libraries (including Tensorflow, Numpy, and audio processing) were also utilized. The audio sample first needed to be pre-processed because the input layer for our model has to be in 224 x 224 x 3. To meet the requirements of the model, the voice signal has to be scaled and transformed into a log-mel spectrogram. The FFT technique was used to separate the mel-spectrogram feature from the original audio data. The dataset is then sectionalized into a training set and a testing set (80%:20%). Both the exam and practice sets’ data were normalized to pixels.
Implementation parameters
In implementing our model and compilation of the network, we utilized the Adams optimizer with a learning rate set to 5e-5 notation. One-hot encoding technique was used in vectorizing the label. It ensures that the data point is binarized. We adopted sparse categorical cross entropy for the loss function. To actualize the objective of increasing accuracy, we initialize our model set up with 100 epochs and 16 batch size, however the result of our training after 25 epochs yielded optimum accuracy. We utilized a custom-early stopping mechanism to monitor (checkpoint) the loss and accuracy value to prevent overfitting, and the corresponding curve was obtained as well.
Experimental Results
The result of our experiment using attention-based networks and regularized feature selection with three classifiers are presented in this section. For the first experiment where the Vgg16 pre-trained network was utilized, the confusion matrix of emotion classification is shown in Figs. 6 , 7 , 8 , 9 . We observed that the attention network of our model achieved the highest accuracy (97.8%) of recognition with the RF classifier compared to the other classifiers (SVM:97.4% and MLP: 97.6%). From the figures, the emotional class of angry, disgust, fear and sad accuracy reach 100% with the attention-based network and RF, SVM and MLP. The Neural emotion class got 98% the highest accuracy of recognition with the RF classifier, while 94% best accuracy was obtained on surprise emotion from Figs. 6 and 7 respectively (Figs. 10 and 11 ). The performance evaluation chart in Fig. 12 shows other evaluation metrics (specificity, sensitivity, F1-score and unweighted average recall) used to establish the robustness of our model. The two experiments are captured on the chart.
In our second experiment, the pre-trained model used was Vgg19 before the attention layer was added. The result generated is shown in Figs. 9 , 10 , 11 . Disgust emotion carries the highest classification accuracy of 100% from the three classifiers, while surprise emotion has the least classification accuracy of 93%. Neutral emotion differs in accuracy from the three classifiers, its optimum accuracy is at 99% with the SVM classifier. The overall model classification accuracy obtained from the second experiment is 97.5%. This is low compared to the previous experiment where vgg16 was used as the convolutional layer, however, the impact of the attention network for the extraction of emotionally related features combined with regularized feature selection has improved the classification accuracy of speech emotion.
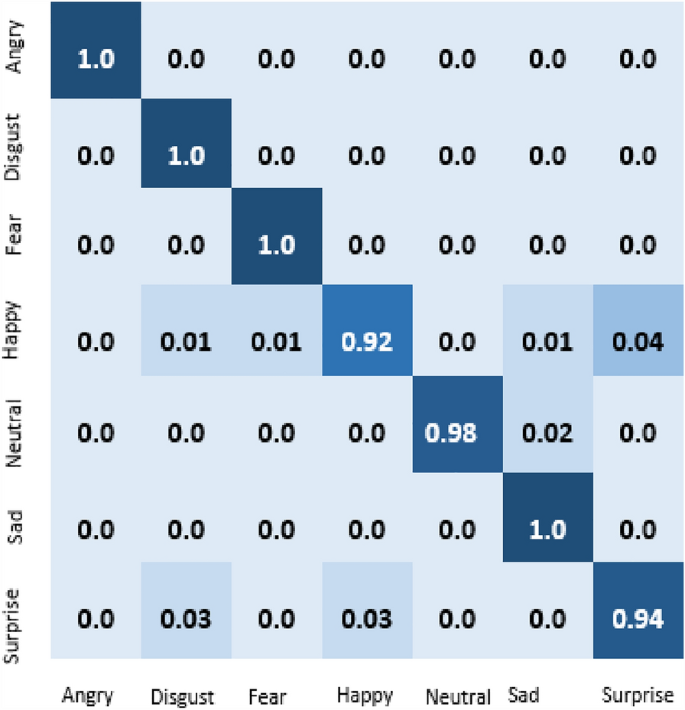
Attention-based Vgg16+RNCA+RF.
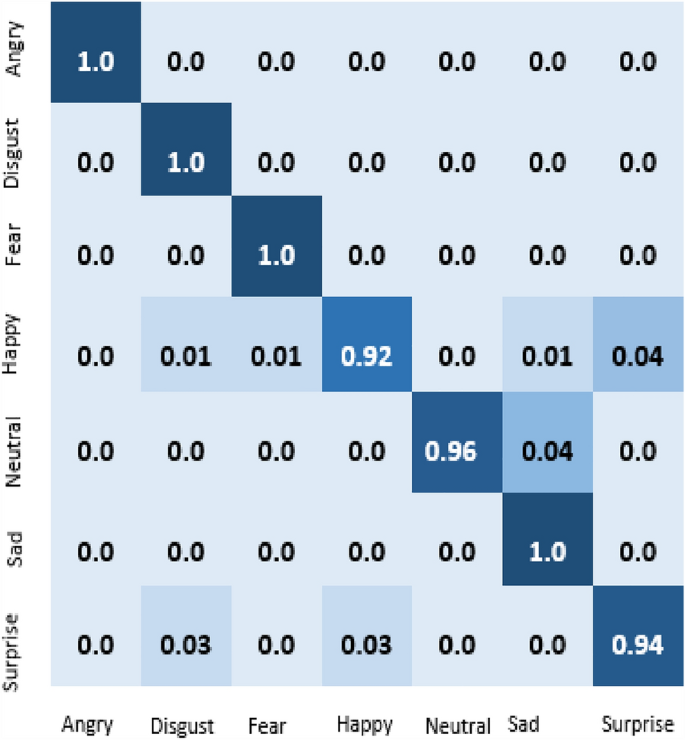
Attention-based Vgg16+RNCA+MLP.
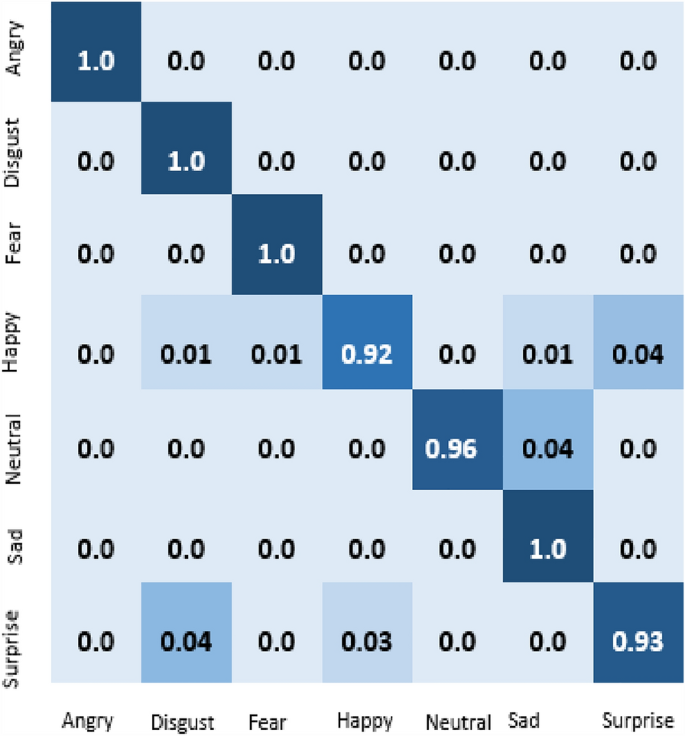
Attention-based Vgg16+RNCA+SVM.
Besides the accuracy obtained through the confusion matrix, the model loss and ROC (Return of Characteristics) curves in Figs. 13 and 14 further testify to the performance of our model in this paper. The loss value from the curve is relatively low, indicating that our model has prevented overfitting. The loss curve decreases over time as our model improved. Also, the loss curve shows a smooth convergence which is a further indication that our model prediction is accurate to an acceptable level. The low initial loss value with respect to the convergence point as shown confirmed the reduction in model complexity and training time. The ROC curve shows the seven categories of emotion as indicated in Table 1 below with the area under the curve (AUC) which demonstrates the performance average across all potential emotion classification thresholds. The diagonal dotted line is the threshold. The closeness of the curve to the top left-hand corner for the seven emotional classes indicates a high True Positive Rate (TPR) and low False Positive Rate (FPR). The least AUC score recorded is 0.98, an evidence of the good performance of our model on emotion classification.
In this work, the Mel-spectrogram was used to extract the input feature, producing a feature vector with a dimensionality of 40 (mel frequency bins). These features record important details about the speech signal’s spectral composition and temporal dynamics. The feature space was high dimensional, so feature selection was used to lower the dimensionality and concentrate on the most useful features for the task. The feature selection algorithm assessed each feature’s relevance based on how it contributed to the performance of emotion recognition, taking into account measures like mutual information and feature importance scores, thereby increasing the model’s efficiency, lowering the amount of computing power required, and improving the interpretability of the learned representations. Our experiments’ findings showed that feature selection significantly enhanced the speech emotion recognition model’s performance, resulting in an increase in accuracy of 3.7%, underscoring the significance of feature selection in improving the model’s discriminative power for emotion recognition tasks.
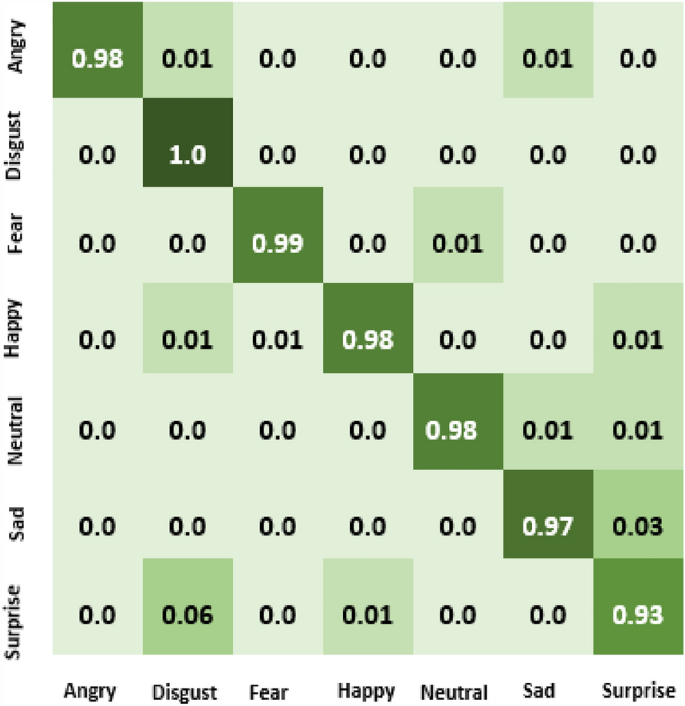
Attention-based Vgg19+RNCA+RF.
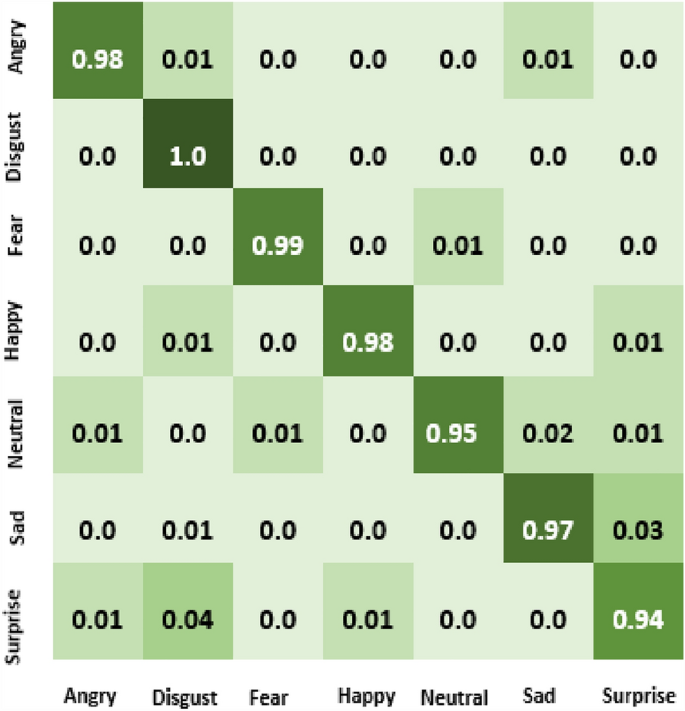
Attention-based Vgg19+RNCA+MLP.
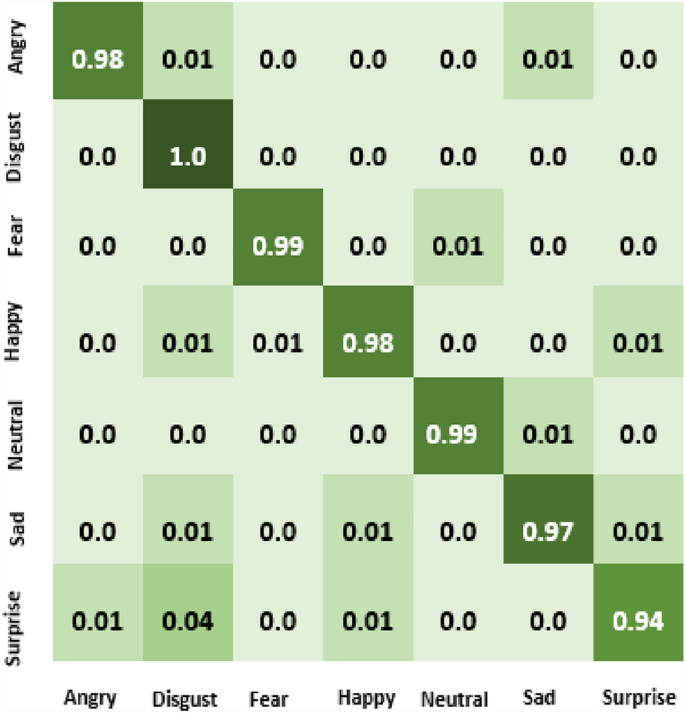
Attention-based Vgg19+RNCA+SVM.
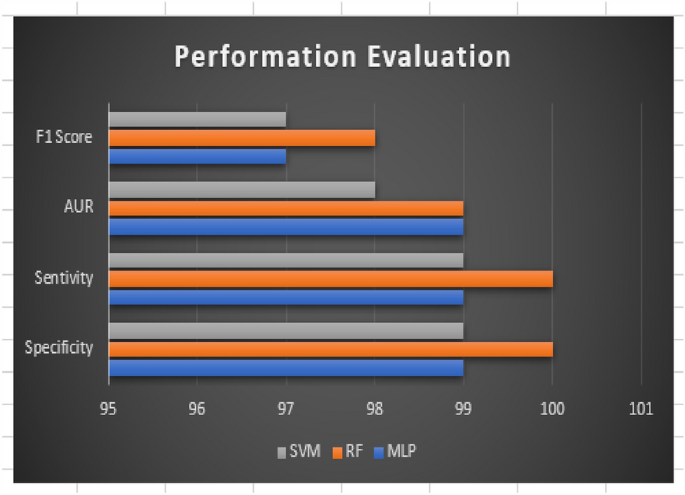
Performance chart with 4 metrics and 3 classifiers.
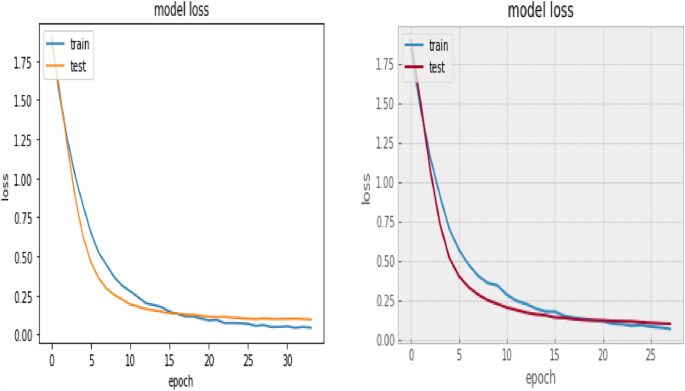
Model loss curve.
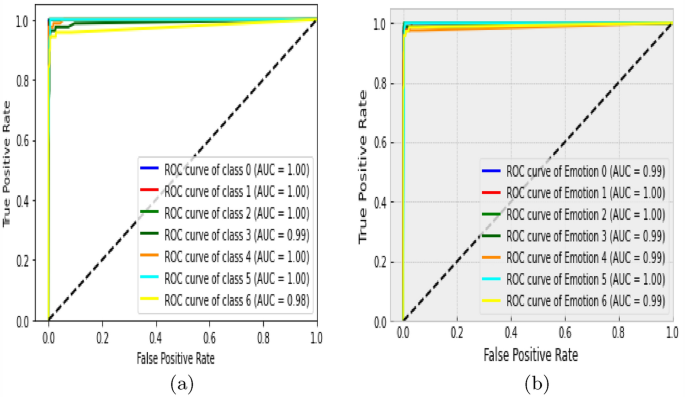
Performance Comparison
Additionally, our proposed model in this study was compared with other work carried out by others benchmarked on the same speech dataset, as indicated in Table 2 . We also carried out a comparative analysis of our proposed model without the attention layer, RNCA, and with the attention mechanism and RNCA feature selection as shown in Table 3 .
In terms of accuracy, reduction of complexity and prevention of overfitting, our method surpasses other methods 47 , 48 , 49 , 50 , 51 utilized for speech emotion classification or recognition.
In this study, we proposed a SEC system using an attention-based network and regularized feature selection. First and foremost, we extracted the mel-spectrogram from the TESS dataset used for this study. This was carried out, after extensive speech processing and analysis, to feed (input layer) our model with appropriate features for enhanced feature extraction in the subsequent layers. A pre-trained DCNN base model was adopted for our attention network to extract local features, while the attention layer deals with emotionally rich features (global features) which ultimately reduces misclassification to the barest minimum. The core principle of the attention network is to estimate feature weight. In our attempt to increase the efficiency of our model, a regularized feature selection is introduced after the attention layer to actualize optimum results. The feature selection aided the attention mechanism to focus more on salient features. Thereafter, three classifiers were fed with selected emotional features with RNCA, for the classification of emotion.
After a comparison of the result of our experiments, an attention-based DCNN+RNCA+RF model for speech emotion classification was proposed. The experimental result attained the optimum accuracy of 97.8% on the TESS dataset. Seven classes of emotions comprised of anger, sad, happy, fear, neutral, disgust and surprise that reflect human major emotions were accurately classified. Besides, by contrasting our proposed model in this study with other methods that have recently been put forward, obviously, our model outperforms many of them in speech emotion classification tasks.
Moreover, the computational cost peculiar to most deep learning tasks is prevented in this study, simply because our based model for the attention network requires no training and the total number of trainable parameters has been reduced to the barest minimum (101,480) out of the total parameters of 14,017,704. The number of floating-point operations per seconds(FLOPs), and the model’s (size of 98MB) memory requirement have been reduced to minimize complexity because the top layer of the VGGNet has been frozen. The average time taken for each emotional utterance to be classified by the proposed model is 0.12. However, though, the result obtained from this study has undoubtedly provided some insight for researchers on the application of attention mechanism with feature selection for SEC tasks, we recommend that future work can be carried out using a sequential network, more pre-trained based network, low-level features and introduction of other speech emotion dataset.
Data availability
Benchmarked publicly available dataset, Toronto English Speech Set (TESS) is used.
Costantini, G., Parada-Cabaleiro, E., Casali, D. & Cesarini, V. The emotion probe: On the universality of cross-linguistic and cross-gender speech emotion recognition via machine learning. Sensors https://doi.org/10.3390/s22072461 (2022).
Article PubMed PubMed Central Google Scholar
Chimthankar, P. P. Speech Emotion Recognition using Deep Learning. http://norma.ncirl.ie/5142/1/priyankaprashantchimthankar.pdf (2021)
Saad, H. F.and Mahmud, Shaheen, M., Hasan, M., Farastu, P. & Kabir, M. Is speech emotion recognition language-independent? Analysis of english and bangla languages using language-independent vocal features. arXiv:2111.10776 (2021)
Burghardt, G. M. A place for emotions in behavior systems research. Behavioural Process. https://doi.org/10.1016/j.beproc.2019.06.004 (2019).
Article Google Scholar
Mustaqeem, & Kwon, S. The emotion probe: On the universality of cross-linguistic and cross-gender speech emotion recognition via machine learning. Appl. Soft Comput. https://doi.org/10.1016/j.asoc.2021.107101 (2021).
Ba’abbad, I., Althubiti, T., Alharbi, A., Alfarsi, K. & Rasheed, S. A short review of classification algorithms accuracy for data prediction in data mining applications. J. Data Anal. Inform. Process. 09 , 162–174. https://doi.org/10.4236/jdaip.2021.93011 (2021).
Choudhary, G. R., Meena, G. & Mohbey, K. Speech emotion based sentiment recognition using deep neural networks. J. Phys. Conf. Ser. 2236 (1), 012003. https://doi.org/10.1088/1742-6596/2236/1/012003 (2022).
Wani, T., Gunawan, T., Qadri, S., Kartiwi, M. & Ambikairajah, E. A comprehensive review of speech emotion recognition systems. IEEE Access 9 , 47795–47814. https://doi.org/10.1109/ACCESS.2021.3068045 (2021).
Cowen, A. & Keltner, D. Self-report captures 27 distinct categories of emotion bridged by continuous gradients. Proc. Nat. Acad. Sci. U.S.A. 114 (38), 7900–7909. https://doi.org/10.1073/pnas.1702247114 (2017).
Article ADS CAS Google Scholar
Oaten, M., Stevenson, R. J. & Case, T. I. Disgust as a disease-avoidance mechanism. Psychol. Bull. 135 (2), 303–321. https://doi.org/10.1037/a0014823 (2009).
Article PubMed Google Scholar
Anvarjon, T., Mustaqeem, & Kwon, S. Deep-net: A lightweight CNN-based speech emotion recognition system using deep frequency features. Sensors (Switzerland) 20 (18), 1–16. https://doi.org/10.3390/s20185212 (2020).
Kwon, S. A CNN-assisted enhanced audio signal processing. Sensors https://doi.org/10.3390/s20185212 (2020).
Staudemeyer, R. & Morris, E. Understanding LSTM–a tutorial into Long Short-Term Memory Recurrent Neural Networks. arXiv:1909.09586 (2019)
Atila, O. & Şengür, A. Attention guided 3d CNN-LSTM model for accurate speech based emotion recognition. Appl. Acoustics https://doi.org/10.1016/j.apacoust.2021.108260 (2021).
Farooq, M., Hussain, F., Baloch, N., Raja, F. & Zikria, Y. Impact of feature selection algorithm on speech emotion recognition using deep convolutional neural network. Sensors (Switzerland) 20 (21), 1–18. https://doi.org/10.3390/s20185212 (2020).
Hajarolasvadi, N. & Demirel, H. 3d CNN-based speech emotion recognition using k-means clustering and spectrograms. Entropy https://doi.org/10.3390/e21050479 (2019).
Zhu, L., Chen, L., Zhao, D., Zhou, J. & Zhang, W. Emotion recognition from Chinese speech for smart affective services using a combination of SVM and DBN. Sensors (Switzerland) https://doi.org/10.3390/s17071694 (2017).
Article PubMed Central Google Scholar
Wang, Z. & Tashev, I. Learning utterance-level representations for speech emotion and age/gender recognition using deep neural networks. IEEE Int. Conf. Acoustics Speech Signal Process. 17 (7), 5150–5154. https://doi.org/10.1109/ICASSP.2017.7953138 (2017).
Pascanu, R., Gulcehre, C., Cho, K. & Bengio, Y. How to construct deep recurrent neural networks. In 2nd International Conference on Learning Representations, ICLR 2014 - Conference Track Proceedings , p. 1–13 (2014)
Kerkeni, L. et al. Automatic speech emotion recognition using an optimal combination of features based on EMD-TKEO. Speech Commun. 114 , 22–35. https://doi.org/10.1016/j.specom.2019.09.002 (2019).
Lieskovská, E., Jakubec, M., Jarina, R. & Chmulík, M. A review on speech emotion recognition using deep learning and attention mechanism. In Electronics (Switzerland) https://doi.org/10.3390/electronics10101163 (2021).
Hochreiter, S. & Schmidhuber, J. Long short-term memory. Neural Comput. 9 (8), 1735–1780. https://doi.org/10.1162/neco.1997.9.8.1735 (1997).
Article CAS PubMed Google Scholar
Zhao, Z. et al. Self-attention transfer networks for speech emotion recognition. Virtual Real. Intell. Hardw. 3 (1), 43–54. https://doi.org/10.1016/j.vrih.2020.12.002 (2021).
Puri, T., Soni, M., Dhiman, G., Khalaf, O. & Khan, I. Detection of emotion of speech for Ravdess audio using hybrid convolution neural network. Hindawi J. Healthc. Eng. https://doi.org/10.1155/2022/8472947 (2022).
Bahdanau, D., Cho, K. & Bengio, Y. Neural machine translation by jointly learning to align and translate. In 3rd International Conference on Learning Representations, ICLR 2015 - Conference Track Proceedings , 1–15 (2015)
Qamhan, M., Meftah, A., Selouani, S., Alotaibi, Y., Zakariah, M. & Seddiq, Y. Speech emotion recognition using convolutional recurrent neural networks with attention model. Canadian Conference on Electrical and Computer Engineering 2020-Augus(Cii), 341–350 (2020). https://doi.org/10.1109/CCECE47787.2020.9255752
Chen, M., He, X., Yang, J. & Zhang, H. 3-d convolutional recurrent neural networks with attention model for speech emotion recognition. IEEE Signal Process. Lett. 25 (10), 1440–1444. https://doi.org/10.1109/CCECE47787.2020.9255752 (2018).
Article ADS Google Scholar
Zhao, Z., Zheng, Y., Zhang, Z., Wang, H., Zhao, Y., Li, C.: Exploring spatio-temporal representations by integrating attention-based bidirectional-LSTM-RNNS and FCNS for speech emotion recognition. In Proceedings of the Annual Conference of the International Speech Communication Association, INTERSPEECH , 2018-Septe(September), 272–276 (2018). https://doi.org/10.21437/Interspeech.2018-1477
Du, Q., Gu, L., Zhang, W. & Huang, S. Poster abstract: Attention-based LSTM-CNNS for time-series classification. In SenSys 2018 - Proceedings of the 16th Conference on Embedded Networked Sensor Systems , 410–411 (2018). https://doi.org/10.1145/3274783.3275208
Seo, M. & Kim, M. Fusing visual attention CNN and bag of visual words for cross-corpus speech emotion recognition. Sensors 20 , 5559. https://doi.org/10.3390/s20195559 (2018).
Zhang, J., Xing, L., Tan, Z., Wang, H. & Wang, K. Multi-head attention fusion networks for multi-modal speech emotion recognition. Comput. Ind. Eng. 168 , 108078. https://doi.org/10.1016/j.cie.2022.108078 (2022).
Zhang, H. et al. Pre-trained deep convolution neural network model with attention for speech emotion recognition. Front. Physiol. https://doi.org/10.3389/fphys.2021.6432028 (2021).
Chen, S. et al. The impact of attention mechanisms on speech emotion recognition. Sensors https://doi.org/10.3390/s21227530 (2021).
Zhao, Z. et al. Self-attention transfer networks for speech emotion recognition. Virtual Real. Intell. Hardw. https://doi.org/10.1016/j.vrih.2020.12.002 (2021).
Zhou, S. & Beigi, H. A transfer learning method for speech emotion recognition from automatic speech recognition. arXiv:2008.02863 (2021)
Singh, Y. & Goel, S. A systematic literature review of speech emotion recognition approaches. Neurocomput. Elsevier https://doi.org/10.1016/j.neucom.2022.04.028 (2022).
Atsavasirilert, K., Theeramunkong, T., Usanavasin, S., Rugchatjaroen, A., Boonkla, S., Karnjana, J., Keerativittayanun, S. & Okumura, M. A light-weight deep convolutional neural network for speech emotion recognition using mel-spectrograms. In 2019 14th International Joint Symposium on Artificial Intelligence and Natural Language Processing (ISAI-NLP) (2019)
Zhou, Q. et al. Cough recognition based on MEL-spectrogram and convolutional neural network. Front. Robot. AI https://doi.org/10.3389/frobt.2021.580080 (2021).
Chen, Q. & Huang, G. A novel dual attention-based BLSTM with hybrid features in speech emotion recognition. Eng. Appl. Artif. Intell. https://doi.org/10.1016/j.engappai.2021.104277 (2021).
Bilal, M. Unsupervised adversarial domain adaptation for cross-lingual speech emotion recognition. arXiv:1907.06083v2 (2019)
Tursunov, A., Mustaqeem, Choeh, J. Y. & Kwon, S. Age and gender recognition using a convolutional neural network with a specially designed multi-attention module through speech spectrograms. Sensors https://doi.org/10.3390/s21175892 (2021).
Ho, N., Yang, H., Kim, S. & Lee, G. Multimodal approach of speech emotion recognition using multi-level multi-head fusion attention-based recurrent neural network. IEEE Access 2020 (8) (2020)
Zhou, A., Luktarhan, N. & Ai, Z. Research on webshell detection method based on regularized neighborhood component analysis (RNCA). Symmetry https://doi.org/10.3390/sym13071202 (2021).
Malan, N. & Sharma, S. Feature selection using regularized neighbourhood component analysis to enhance the classification performance of motor imagery signals. Comput. Biol. Med. https://doi.org/10.1016/j.compbiomed.2019.02.009 (2019).
Duville, M., Alonso-Valerdi, L. & Ibarra-Zarate, D. Mexican emotional speech database based on semantic, frequency, familiarity, concreteness, and cultural shaping of affective prosody. Data https://doi.org/10.3390/data6120130 (2021).
Dupuis, K. & Kathleen Pichora-Fuller, M. Recognition of emotional speech for younger and older talkers: Behavioural findings from the Toronto emotional speech set. Can. Acoust. https://doi.org/10.3389/fphys.2021.6432028 (2011).
Verma, D. M. Age driven automatic speech emotion recognition system. IEEE Int. Conf. Comput. Commun. Autom https://doi.org/10.1109/CCAA.2016.7813862 (2017).
Praseetha, V. & Vadivel, S. Deep learning models for speech emotion recognition. J. Comput. Sci. https://doi.org/10.3844/jcssp.2018.1577.1587 (2018).
Gao, Y. Speech-Based Emotion Recognition. https://libraetd.lib.virginia.edu/downloads/2f75r8498?filename=1_Gao_Ye_2019_MS.pdf (2019)
Krishnan, P., Joseph Raj, A. & Rajangam, V. Emotion classification from speech signal based on empirical mode decomposition and non-linear features. Complex Intell. Syst. https://doi.org/10.1007/s40747-021-00295-z (2021).
Akinpelu, S. & Viriri, S. Robust feature selection-based speech emotion classification using deep transfer learning. Appl. Sci. 12 , 8265. https://doi.org/10.3390/app12168265 (2022).
Article CAS Google Scholar
Download references
Author information
These authors contributed equally: Samson Akinpelu and Serestina Viriri.
Authors and Affiliations
School of Mathematics, Statistics and Computer Science, University of KwaZulu-Natal, Durban, 4000, South Africa
Samson Akinpelu & Serestina Viriri
You can also search for this author in PubMed Google Scholar
Corresponding author
Correspondence to Serestina Viriri .
Ethics declarations
Competing interests.
The authors declare no competing interests.
Additional information
Publisher's note.
Springer Nature remains neutral with regard to jurisdictional claims in published maps and institutional affiliations.
Rights and permissions
Open Access This article is licensed under a Creative Commons Attribution 4.0 International License, which permits use, sharing, adaptation, distribution and reproduction in any medium or format, as long as you give appropriate credit to the original author(s) and the source, provide a link to the Creative Commons licence, and indicate if changes were made. The images or other third party material in this article are included in the article’s Creative Commons licence, unless indicated otherwise in a credit line to the material. If material is not included in the article’s Creative Commons licence and your intended use is not permitted by statutory regulation or exceeds the permitted use, you will need to obtain permission directly from the copyright holder. To view a copy of this licence, visit http://creativecommons.org/licenses/by/4.0/ .
Reprints and permissions
About this article
Cite this article.
Akinpelu, S., Viriri, S. Speech emotion classification using attention based network and regularized feature selection. Sci Rep 13 , 11990 (2023). https://doi.org/10.1038/s41598-023-38868-2
Download citation
Received : 13 January 2023
Accepted : 16 July 2023
Published : 25 July 2023
DOI : https://doi.org/10.1038/s41598-023-38868-2
Share this article
Anyone you share the following link with will be able to read this content:
Sorry, a shareable link is not currently available for this article.
Provided by the Springer Nature SharedIt content-sharing initiative
By submitting a comment you agree to abide by our Terms and Community Guidelines . If you find something abusive or that does not comply with our terms or guidelines please flag it as inappropriate.
Quick links
- Explore articles by subject
- Guide to authors
- Editorial policies
Sign up for the Nature Briefing: AI and Robotics newsletter — what matters in AI and robotics research, free to your inbox weekly.

A Comprehensive Review of Speech Emotion Recognition Systems
Ieee account.
- Change Username/Password
- Update Address
Purchase Details
- Payment Options
- Order History
- View Purchased Documents
Profile Information
- Communications Preferences
- Profession and Education
- Technical Interests
- US & Canada: +1 800 678 4333
- Worldwide: +1 732 981 0060
- Contact & Support
- About IEEE Xplore
- Accessibility
- Terms of Use
- Nondiscrimination Policy
- Privacy & Opting Out of Cookies
A not-for-profit organization, IEEE is the world's largest technical professional organization dedicated to advancing technology for the benefit of humanity. © Copyright 2024 IEEE - All rights reserved. Use of this web site signifies your agreement to the terms and conditions.
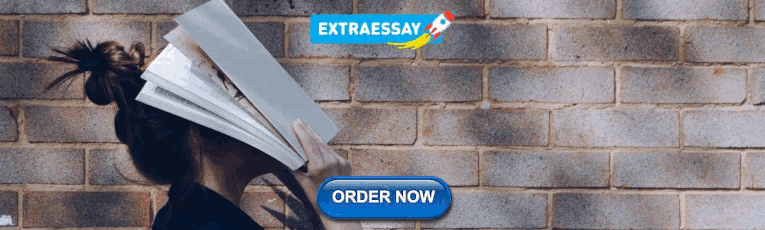
IMAGES
VIDEO
COMMENTS
Abstract. Nowadays emotion recognition from speech (SER) is a demanding research area for researchers because of its wide real-life applications. There are many challenges for SER systems such as the availability of suitable emotional databases, identification of the relevant feature vector, and suitable classifiers.
Abstract. The speech emotion recognition (SER) field has been active since it became a crucial feature in advanced Human-Computer Interaction (HCI), and wide real-life applications use it. In recent years, numerous SER systems have been covered by researchers, including the availability of appropriate emotional databases, selecting robustness ...
This review study aims to identify and examine articles related to SER regarding emotional speech databases, speech features, and classifiers of the traditional and new approaches. Based on that, the research questions were identified. Table 1 illustrates the research questions with the purpose. Table 1.
The focus of this review is on DL approaches for SER. A total of 152 papers have been reviewed from years 2000-2021. We have identified frequently used speech databases and related accuracies achieved using DL approaches. The motivations and limitations of DL approaches for SER are also summarized.
The speech emotion recognition (SER) field has been active since it became a crucial feature in advanced Human-Computer Interaction (HCI), and wide real-life applications use it. In recent years, numerous SER systems have been covered by researchers, including the availability of appropriate emotional databases, selecting robustness features, and applying suitable classifiers using Machine ...
Automatic Speech Emotion Recognition (ASER) has recently garnered attention across various fields including artificial intelligence, pattern recognition, and human-computer interaction. However, ASER encounters numerous challenges such as a shortage of diverse datasets, appropriate feature selection, and suitable intelligent recognition techniques. To address these challenges, a systematic ...
In their study, Singh and Goel conducted a literature review on the databases used in speech emotion recognition studies between 2000-2021 and the motivations and limitations of deep learning used ...
2021. TLDR. The results reveal that the proposed approaches for classifying emotions from speech by combining conventional mel-frequency cepstral coefficients (MFCCs) with image features extracted from spectrograms by a pretrained convolutional neural network (CNN) lead to an improvement in prediction accuracy. Expand.
Speech emotion recognition (SER) systems identify emotions from the human voice in the areas of smart healthcare, driving a vehicle, call centers, automatic translation systems, and human-machine ...
2022. TLDR. This paper proposes a systematical and robust approach to implement an emotion recognition system for low resource languages such as Persian using deep learning techniques and demonstrates that the proposed method achieves about 74% classification accuracy on ShEMO dataset. Expand.
Nowadays emotion recognition from speech (SER) is a demanding research area for researchers because of its wide real-life applications. There are many challenges for SER systems such as the availability of suitable emotional databases, identification of the relevant feature vector, and suitable classifiers.This paper critically analysed the literature on SER in terms of speech databases ...
The primary goal of this systematic literature review (SLR) is to determine the research approaches in the SER field based on different aspects. The methodology used in SLR is based on three stages: the planning stage, the conducting stage, and finally, the reporting stage. The planning stage is divided into five stages.
Furthermore, more than 100 papers were employed to conduct the systematic literature review, which were published throughout a 13-year period between 2008 and 2021. ... "A dimensional approach to emotion recognition of speech from movies," in 2009 IEEE International Conference on Acoustics, Speech and Signal Processing, pp. 65-68, https ...
This paper critically analyzed the current available approaches of speech emotion recognition methods based on the three evaluating parameters (feature set, classification of features, accurately usage). In addition, this paper also evaluates the performance and limitations of available methods.
A review on speech emotion recognition using deep learning and attention mechanism. ... A systematic literature review of speech emotion recognition approaches. ... Kim, S. & Lee, G. Multimodal ...
During the last decade, Speech Emotion Recognition (SER) has emerged as an integral component within Human-computer Interaction (HCI) and other high-end speech processing systems. Generally, an SER system targets the speaker's existence of varied emotions by extracting and classifying the prominent features from a preprocessed speech signal. However, the way humans and machines recognize and ...
A key source of emotional information is the spoken expression, which may be part of the interaction between the human and the machine. Speech emotion recognition (SER) is a very active area of research that involves the application of current machine learning and neural networks tools. This ongoing review covers recent and classical approaches ...
TLDR. A speech emotion recognition method based on gender classification using MLP to classify the original speech by gender, which shows that the proposed emotion recognition models have an advantage in terms of average recognition accuracy compared with gender-mixed recognition models. Expand. 5. [PDF]
Speech emotion recognition (SER) as a Machine Learning (ML) problem continues to garner a significant amount of research interest, especially in the affective computing domain. This is due to its increasing potential, algorithmic advancements, and applications in real-world scenarios. Human speech contains para-linguistic information that can ...
The aim of the paper is to detect the emotions which are elicited by the speaker while speaking by using different classification algorithms to recognize the emotions, Support Vector Machine, Multi layer perception, and the audio feature MFCC, MEL, chroma, Tonnetz were used. Expand. 20. PDF.
Speech emotion recognition technology is the process of extracting feature parameters related to emotional representation from speech signals and establishing a mapping model between these feature parameters and emotional categories. ... demonstrating the potential of multimodal approaches in emotion recognition and providing new perspectives ...
A key source of emotional information is the spoken expression, which may be part of the interaction between the human and the machine. Speech emotion recognition (SER) is a very active area of research that involves the application of current machine learning and neural networks tools. This ongoing review covers recent and classical approaches ...
Based on the literature findings, ... Arunnehru J. Synthesis approach for emotion recognition from cepstral and pitch coefficients using machine learning. Singapore: Springer; 2021; p. 515-528. ... Siang TG, et al. Particle swarm optimisation for emotion recognition systems: a decade review of the literature. Appl Sci. 2023;13, 7054. doi:10. ...
2021. TLDR. This is a survey paper that aims to give reviews about that finest architectures of machine learning, the use of algorithms and the applications of the system and speech and vision processes using the most used models of deep learning used in the vision and speech systems. Expand. 61.
Our review analysis also presented potential challenges in the existing literature and directions for future research. ... Singh and Goel [30] presented a systematic review of emotion recognition using speech signals following PRISMA guidelines. Their method covered the application of ML and DL techniques, but failed to cover research ...