Have a language expert improve your writing
Run a free plagiarism check in 10 minutes, generate accurate citations for free.
- Knowledge Base
Methodology
- What Is Quantitative Research? | Definition, Uses & Methods
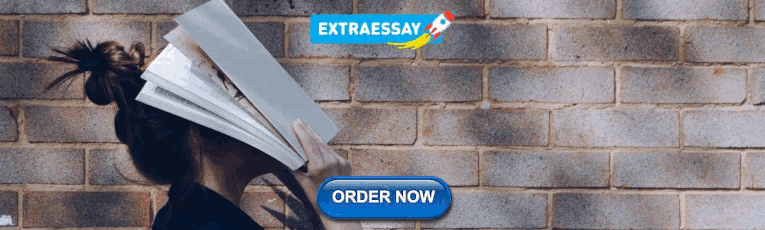
What Is Quantitative Research? | Definition, Uses & Methods
Published on June 12, 2020 by Pritha Bhandari . Revised on June 22, 2023.
Quantitative research is the process of collecting and analyzing numerical data. It can be used to find patterns and averages, make predictions, test causal relationships, and generalize results to wider populations.
Quantitative research is the opposite of qualitative research , which involves collecting and analyzing non-numerical data (e.g., text, video, or audio).
Quantitative research is widely used in the natural and social sciences: biology, chemistry, psychology, economics, sociology, marketing, etc.
- What is the demographic makeup of Singapore in 2020?
- How has the average temperature changed globally over the last century?
- Does environmental pollution affect the prevalence of honey bees?
- Does working from home increase productivity for people with long commutes?
Table of contents
Quantitative research methods, quantitative data analysis, advantages of quantitative research, disadvantages of quantitative research, other interesting articles, frequently asked questions about quantitative research.
You can use quantitative research methods for descriptive, correlational or experimental research.
- In descriptive research , you simply seek an overall summary of your study variables.
- In correlational research , you investigate relationships between your study variables.
- In experimental research , you systematically examine whether there is a cause-and-effect relationship between variables.
Correlational and experimental research can both be used to formally test hypotheses , or predictions, using statistics. The results may be generalized to broader populations based on the sampling method used.
To collect quantitative data, you will often need to use operational definitions that translate abstract concepts (e.g., mood) into observable and quantifiable measures (e.g., self-ratings of feelings and energy levels).
Note that quantitative research is at risk for certain research biases , including information bias , omitted variable bias , sampling bias , or selection bias . Be sure that you’re aware of potential biases as you collect and analyze your data to prevent them from impacting your work too much.
Here's why students love Scribbr's proofreading services
Discover proofreading & editing
Once data is collected, you may need to process it before it can be analyzed. For example, survey and test data may need to be transformed from words to numbers. Then, you can use statistical analysis to answer your research questions .
Descriptive statistics will give you a summary of your data and include measures of averages and variability. You can also use graphs, scatter plots and frequency tables to visualize your data and check for any trends or outliers.
Using inferential statistics , you can make predictions or generalizations based on your data. You can test your hypothesis or use your sample data to estimate the population parameter .
First, you use descriptive statistics to get a summary of the data. You find the mean (average) and the mode (most frequent rating) of procrastination of the two groups, and plot the data to see if there are any outliers.
You can also assess the reliability and validity of your data collection methods to indicate how consistently and accurately your methods actually measured what you wanted them to.
Quantitative research is often used to standardize data collection and generalize findings . Strengths of this approach include:
- Replication
Repeating the study is possible because of standardized data collection protocols and tangible definitions of abstract concepts.
- Direct comparisons of results
The study can be reproduced in other cultural settings, times or with different groups of participants. Results can be compared statistically.
- Large samples
Data from large samples can be processed and analyzed using reliable and consistent procedures through quantitative data analysis.
- Hypothesis testing
Using formalized and established hypothesis testing procedures means that you have to carefully consider and report your research variables, predictions, data collection and testing methods before coming to a conclusion.
Despite the benefits of quantitative research, it is sometimes inadequate in explaining complex research topics. Its limitations include:
- Superficiality
Using precise and restrictive operational definitions may inadequately represent complex concepts. For example, the concept of mood may be represented with just a number in quantitative research, but explained with elaboration in qualitative research.
- Narrow focus
Predetermined variables and measurement procedures can mean that you ignore other relevant observations.
- Structural bias
Despite standardized procedures, structural biases can still affect quantitative research. Missing data , imprecise measurements or inappropriate sampling methods are biases that can lead to the wrong conclusions.
- Lack of context
Quantitative research often uses unnatural settings like laboratories or fails to consider historical and cultural contexts that may affect data collection and results.
Receive feedback on language, structure, and formatting
Professional editors proofread and edit your paper by focusing on:
- Academic style
- Vague sentences
- Style consistency
See an example
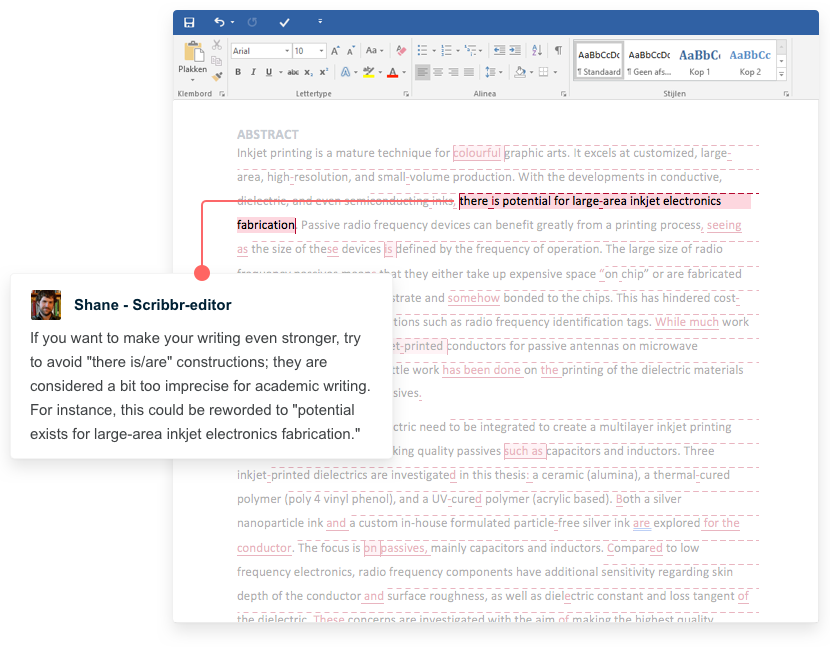
If you want to know more about statistics , methodology , or research bias , make sure to check out some of our other articles with explanations and examples.
- Chi square goodness of fit test
- Degrees of freedom
- Null hypothesis
- Discourse analysis
- Control groups
- Mixed methods research
- Non-probability sampling
- Inclusion and exclusion criteria
Research bias
- Rosenthal effect
- Implicit bias
- Cognitive bias
- Selection bias
- Negativity bias
- Status quo bias
Quantitative research deals with numbers and statistics, while qualitative research deals with words and meanings.
Quantitative methods allow you to systematically measure variables and test hypotheses . Qualitative methods allow you to explore concepts and experiences in more detail.
In mixed methods research , you use both qualitative and quantitative data collection and analysis methods to answer your research question .
Data collection is the systematic process by which observations or measurements are gathered in research. It is used in many different contexts by academics, governments, businesses, and other organizations.
Operationalization means turning abstract conceptual ideas into measurable observations.
For example, the concept of social anxiety isn’t directly observable, but it can be operationally defined in terms of self-rating scores, behavioral avoidance of crowded places, or physical anxiety symptoms in social situations.
Before collecting data , it’s important to consider how you will operationalize the variables that you want to measure.
Reliability and validity are both about how well a method measures something:
- Reliability refers to the consistency of a measure (whether the results can be reproduced under the same conditions).
- Validity refers to the accuracy of a measure (whether the results really do represent what they are supposed to measure).
If you are doing experimental research, you also have to consider the internal and external validity of your experiment.
Hypothesis testing is a formal procedure for investigating our ideas about the world using statistics. It is used by scientists to test specific predictions, called hypotheses , by calculating how likely it is that a pattern or relationship between variables could have arisen by chance.
Cite this Scribbr article
If you want to cite this source, you can copy and paste the citation or click the “Cite this Scribbr article” button to automatically add the citation to our free Citation Generator.
Bhandari, P. (2023, June 22). What Is Quantitative Research? | Definition, Uses & Methods. Scribbr. Retrieved April 5, 2024, from https://www.scribbr.com/methodology/quantitative-research/
Is this article helpful?
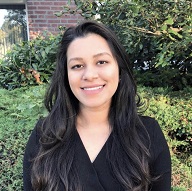
Pritha Bhandari
Other students also liked, descriptive statistics | definitions, types, examples, inferential statistics | an easy introduction & examples, what is your plagiarism score.
Thank you for visiting nature.com. You are using a browser version with limited support for CSS. To obtain the best experience, we recommend you use a more up to date browser (or turn off compatibility mode in Internet Explorer). In the meantime, to ensure continued support, we are displaying the site without styles and JavaScript.
- View all journals
- Explore content
- About the journal
- Publish with us
- Sign up for alerts
- Review Article
- Published: 01 June 2023
Data, measurement and empirical methods in the science of science
- Lu Liu 1 , 2 , 3 , 4 ,
- Benjamin F. Jones ORCID: orcid.org/0000-0001-9697-9388 1 , 2 , 3 , 5 , 6 ,
- Brian Uzzi ORCID: orcid.org/0000-0001-6855-2854 1 , 2 , 3 &
- Dashun Wang ORCID: orcid.org/0000-0002-7054-2206 1 , 2 , 3 , 7
Nature Human Behaviour volume 7 , pages 1046–1058 ( 2023 ) Cite this article
16k Accesses
8 Citations
120 Altmetric
Metrics details
- Scientific community
The advent of large-scale datasets that trace the workings of science has encouraged researchers from many different disciplinary backgrounds to turn scientific methods into science itself, cultivating a rapidly expanding ‘science of science’. This Review considers this growing, multidisciplinary literature through the lens of data, measurement and empirical methods. We discuss the purposes, strengths and limitations of major empirical approaches, seeking to increase understanding of the field’s diverse methodologies and expand researchers’ toolkits. Overall, new empirical developments provide enormous capacity to test traditional beliefs and conceptual frameworks about science, discover factors associated with scientific productivity, predict scientific outcomes and design policies that facilitate scientific progress.
Similar content being viewed by others
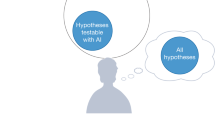
Artificial intelligence and illusions of understanding in scientific research
Lisa Messeri & M. J. Crockett
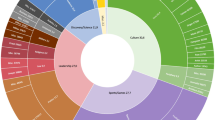
A cross-verified database of notable people, 3500BC-2018AD
Morgane Laouenan, Palaash Bhargava, … Etienne Wasmer
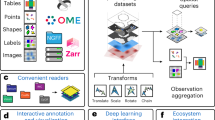
SpatialData: an open and universal data framework for spatial omics
Luca Marconato, Giovanni Palla, … Oliver Stegle
Scientific advances are a key input to rising standards of living, health and the capacity of society to confront grand challenges, from climate change to the COVID-19 pandemic 1 , 2 , 3 . A deeper understanding of how science works and where innovation occurs can help us to more effectively design science policy and science institutions, better inform scientists’ own research choices, and create and capture enormous value for science and humanity. Building on these key premises, recent years have witnessed substantial development in the ‘science of science’ 4 , 5 , 6 , 7 , 8 , 9 , which uses large-scale datasets and diverse computational toolkits to unearth fundamental patterns behind scientific production and use.
The idea of turning scientific methods into science itself is long-standing. Since the mid-20th century, researchers from different disciplines have asked central questions about the nature of scientific progress and the practice, organization and impact of scientific research. Building on these rich historical roots, the field of the science of science draws upon many disciplines, ranging from information science to the social, physical and biological sciences to computer science, engineering and design. The science of science closely relates to several strands and communities of research, including metascience, scientometrics, the economics of science, research on research, science and technology studies, the sociology of science, metaknowledge and quantitative science studies 5 . There are noticeable differences between some of these communities, mostly around their historical origins and the initial disciplinary composition of researchers forming these communities. For example, metascience has its origins in the clinical sciences and psychology, and focuses on rigour, transparency, reproducibility and other open science-related practices and topics. The scientometrics community, born in library and information sciences, places a particular emphasis on developing robust and responsible measures and indicators for science. Science and technology studies engage the history of science and technology, the philosophy of science, and the interplay between science, technology and society. The science of science, which has its origins in physics, computer science and sociology, takes a data-driven approach and emphasizes questions on how science works. Each of these communities has made fundamental contributions to understanding science. While they differ in their origins, these differences pale in comparison to the overarching, common interest in understanding the practice of science and its societal impact.
Three major developments have encouraged rapid advances in the science of science. The first is in data 9 : modern databases include millions of research articles, grant proposals, patents and more. This windfall of data traces scientific activity in remarkable detail and at scale. The second development is in measurement: scholars have used data to develop many new measures of scientific activities and examine theories that have long been viewed as important but difficult to quantify. The third development is in empirical methods: thanks to parallel advances in data science, network science, artificial intelligence and econometrics, researchers can study relationships, make predictions and assess science policy in powerful new ways. Together, new data, measurements and methods have revealed fundamental new insights about the inner workings of science and scientific progress itself.
With multiple approaches, however, comes a key challenge. As researchers adhere to norms respected within their disciplines, their methods vary, with results often published in venues with non-overlapping readership, fragmenting research along disciplinary boundaries. This fragmentation challenges researchers’ ability to appreciate and understand the value of work outside of their own discipline, much less to build directly on it for further investigations.
Recognizing these challenges and the rapidly developing nature of the field, this paper reviews the empirical approaches that are prevalent in this literature. We aim to provide readers with an up-to-date understanding of the available datasets, measurement constructs and empirical methodologies, as well as the value and limitations of each. Owing to space constraints, this Review does not cover the full technical details of each method, referring readers to related guides to learn more. Instead, we will emphasize why a researcher might favour one method over another, depending on the research question.
Beyond a positive understanding of science, a key goal of the science of science is to inform science policy. While this Review mainly focuses on empirical approaches, with its core audience being researchers in the field, the studies reviewed are also germane to key policy questions. For example, what is the appropriate scale of scientific investment, in what directions and through what institutions 10 , 11 ? Are public investments in science aligned with public interests 12 ? What conditions produce novel or high-impact science 13 , 14 , 15 , 16 , 17 , 18 , 19 , 20 ? How do the reward systems of science influence the rate and direction of progress 13 , 21 , 22 , 23 , 24 , and what governs scientific reproducibility 25 , 26 , 27 ? How do contributions evolve over a scientific career 28 , 29 , 30 , 31 , 32 , and how may diversity among scientists advance scientific progress 33 , 34 , 35 , among other questions relevant to science policy 36 , 37 .
Overall, this review aims to facilitate entry to science of science research, expand researcher toolkits and illustrate how diverse research approaches contribute to our collective understanding of science. Section 2 reviews datasets and data linkages. Section 3 reviews major measurement constructs in the science of science. Section 4 considers a range of empirical methods, focusing on one study to illustrate each method and briefly summarizing related examples and applications. Section 5 concludes with an outlook for the science of science.
Historically, data on scientific activities were difficult to collect and were available in limited quantities. Gathering data could involve manually tallying statistics from publications 38 , 39 , interviewing scientists 16 , 40 , or assembling historical anecdotes and biographies 13 , 41 . Analyses were typically limited to a specific domain or group of scientists. Today, massive datasets on scientific production and use are at researchers’ fingertips 42 , 43 , 44 . Armed with big data and advanced algorithms, researchers can now probe questions previously not amenable to quantification and with enormous increases in scope and scale, as detailed below.
Publication datasets cover papers from nearly all scientific disciplines, enabling analyses of both general and domain-specific patterns. Commonly used datasets include the Web of Science (WoS), PubMed, CrossRef, ORCID, OpenCitations, Dimensions and OpenAlex. Datasets incorporating papers’ text (CORE) 45 , 46 , 47 , data entities (DataCite) 48 , 49 and peer review reports (Publons) 33 , 50 , 51 have also become available. These datasets further enable novel measurement, for example, representations of a paper’s content 52 , 53 , novelty 15 , 54 and interdisciplinarity 55 .
Notably, databases today capture more diverse aspects of science beyond publications, offering a richer and more encompassing view of research contexts and of researchers themselves (Fig. 1 ). For example, some datasets trace research funding to the specific publications these investments support 56 , 57 , allowing high-scale studies of the impact of funding on productivity and the return on public investment. Datasets incorporating job placements 58 , 59 , curriculum vitae 21 , 59 and scientific prizes 23 offer rich quantitative evidence on the social structure of science. Combining publication profiles with mentorship genealogies 60 , 61 , dissertations 34 and course syllabi 62 , 63 provides insights on mentoring and cultivating talent.
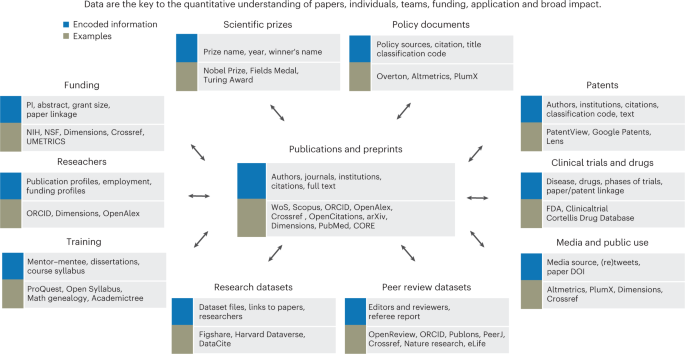
This figure presents commonly used data types in science of science research, information contained in each data type and examples of data sources. Datasets in the science of science research have not only grown in scale but have also expanded beyond publications to integrate upstream funding investments and downstream applications that extend beyond science itself.
Finally, today’s scope of data extends beyond science to broader aspects of society. Altmetrics 64 captures news media and social media mentions of scientific articles. Other databases incorporate marketplace uses of science, including through patents 10 , pharmaceutical clinical trials and drug approvals 65 , 66 . Policy documents 67 , 68 help us to understand the role of science in the halls of government 69 and policy making 12 , 68 .
While datasets of the modern scientific enterprise have grown exponentially, they are not without limitations. As is often the case for data-driven research, drawing conclusions from specific data sources requires scrutiny and care. Datasets are typically based on published work, which may favour easy-to-publish topics over important ones (the streetlight effect) 70 , 71 . The publication of negative results is also rare (the file drawer problem) 72 , 73 . Meanwhile, English language publications account for over 90% of articles in major data sources, with limited coverage of non-English journals 74 . Publication datasets may also reflect biases in data collection across research institutions or demographic groups. Despite the open science movement, many datasets require paid subscriptions, which can create inequality in data access. Creating more open datasets for the science of science, such as OpenAlex, may not only improve the robustness and replicability of empirical claims but also increase entry to the field.
As today’s datasets become larger in scale and continue to integrate new dimensions, they offer opportunities to unveil the inner workings and external impacts of science in new ways. They can enable researchers to reach beyond previous limitations while conducting original studies of new and long-standing questions about the sciences.
Measurement
Here we discuss prominent measurement approaches in the science of science, including their purposes and limitations.
Modern publication databases typically include data on which articles and authors cite other papers and scientists. These citation linkages have been used to engage core conceptual ideas in scientific research. Here we consider two common measures based on citation information: citation counts and knowledge flows.
First, citation counts are commonly used indicators of impact. The term ‘indicator’ implies that it only approximates the concept of interest. A citation count is defined as how many times a document is cited by subsequent documents and can proxy for the importance of research papers 75 , 76 as well as patented inventions 77 , 78 , 79 . Rather than treating each citation equally, measures may further weight the importance of each citation, for example by using the citation network structure to produce centrality 80 , PageRank 81 , 82 or Eigenfactor indicators 83 , 84 .
Citation-based indicators have also faced criticism 84 , 85 . Citation indicators necessarily oversimplify the construct of impact, often ignoring heterogeneity in the meaning and use of a particular reference, the variations in citation practices across fields and institutional contexts, and the potential for reputation and power structures in science to influence citation behaviour 86 , 87 . Researchers have started to understand more nuanced citation behaviours ranging from negative citations 86 to citation context 47 , 88 , 89 . Understanding what a citation actually measures matters in interpreting and applying many research findings in the science of science. Evaluations relying on citation-based indicators rather than expert judgements raise questions regarding misuse 90 , 91 , 92 . Given the importance of developing indicators that can reliably quantify and evaluate science, the scientometrics community has been working to provide guidance for responsible citation practices and assessment 85 .
Second, scientists use citations to trace knowledge flows. Each citation in a paper is a link to specific previous work from which we can proxy how new discoveries draw upon existing ideas 76 , 93 and how knowledge flows between fields of science 94 , 95 , research institutions 96 , regions and nations 97 , 98 , 99 , and individuals 81 . Combinations of citation linkages can also approximate novelty 15 , disruptiveness 17 , 100 and interdisciplinarity 55 , 95 , 101 , 102 . A rapidly expanding body of work further examines citations to scientific articles from other domains (for example, patents, clinical drug trials and policy documents) to understand the applied value of science 10 , 12 , 65 , 66 , 103 , 104 , 105 .
Individuals
Analysing individual careers allows researchers to answer questions such as: How do we quantify individual scientific productivity? What is a typical career lifecycle? How are resources and credits allocated across individuals and careers? A scholar’s career can be examined through the papers they publish 30 , 31 , 106 , 107 , 108 , with attention to career progression and mobility, publication counts and citation impact, as well as grant funding 24 , 109 , 110 and prizes 111 , 112 , 113 ,
Studies of individual impact focus on output, typically approximated by the number of papers a researcher publishes and citation indicators. A popular measure for individual impact is the h -index 114 , which takes both volume and per-paper impact into consideration. Specifically, a scientist is assigned the largest value h such that they have h papers that were each cited at least h times. Later studies build on the idea of the h -index and propose variants to address limitations 115 , these variants ranging from emphasizing highly cited papers in a career 116 , to field differences 117 and normalizations 118 , to the relative contribution of an individual in collaborative works 119 .
To study dynamics in output over the lifecycle, individuals can be studied according to age, career age or the sequence of publications. A long-standing literature has investigated the relationship between age and the likelihood of outstanding achievement 28 , 106 , 111 , 120 , 121 . Recent studies further decouple the relationship between age, publication volume and per-paper citation, and measure the likelihood of producing highly cited papers in the sequence of works one produces 30 , 31 .
As simple as it sounds, representing careers using publication records is difficult. Collecting the full publication list of a researcher is the foundation to study individuals yet remains a key challenge, requiring name disambiguation techniques to match specific works to specific researchers. Although algorithms are increasingly capable at identifying millions of career profiles 122 , they vary in accuracy and robustness. ORCID can help to alleviate the problem by offering researchers the opportunity to create, maintain and update individual profiles themselves, and it goes beyond publications to collect broader outputs and activities 123 . A second challenge is survivorship bias. Empirical studies tend to focus on careers that are long enough to afford statistical analyses, which limits the applicability of the findings to scientific careers as a whole. A third challenge is the breadth of scientists’ activities, where focusing on publications ignores other important contributions such as mentorship and teaching, service (for example, refereeing papers, reviewing grant proposals and editing journals) or leadership within their organizations. Although researchers have begun exploring these dimensions by linking individual publication profiles with genealogical databases 61 , 124 , dissertations 34 , grants 109 , curriculum vitae 21 and acknowledgements 125 , scientific careers beyond publication records remain under-studied 126 , 127 . Lastly, citation-based indicators only serve as an approximation of individual performance with similar limitations as discussed above. The scientific community has called for more appropriate practices 85 , 128 , ranging from incorporating expert assessment of research contributions to broadening the measures of impact beyond publications.
Over many decades, science has exhibited a substantial and steady shift away from solo authorship towards coauthorship, especially among highly cited works 18 , 129 , 130 . In light of this shift, a research field, the science of team science 131 , 132 , has emerged to study the mechanisms that facilitate or hinder the effectiveness of teams. Team size can be proxied by the number of coauthors on a paper, which has been shown to predict distinctive types of advance: whereas larger teams tend to develop ideas, smaller teams tend to disrupt current ways of thinking 17 . Team characteristics can be inferred from coauthors’ backgrounds 133 , 134 , 135 , allowing quantification of a team’s diversity in terms of field, age, gender or ethnicity. Collaboration networks based on coauthorship 130 , 136 , 137 , 138 , 139 offer nuanced network-based indicators to understand individual and institutional collaborations.
However, there are limitations to using coauthorship alone to study teams 132 . First, coauthorship can obscure individual roles 140 , 141 , 142 , which has prompted institutional responses to help to allocate credit, including authorship order and individual contribution statements 56 , 143 . Second, coauthorship does not reflect the complex dynamics and interactions between team members that are often instrumental for team success 53 , 144 . Third, collaborative contributions can extend beyond coauthorship in publications to include members of a research laboratory 145 or co-principal investigators (co-PIs) on a grant 146 . Initiatives such as CRediT may help to address some of these issues by recording detailed roles for each contributor 147 .
Institutions
Research institutions, such as departments, universities, national laboratories and firms, encompass wider groups of researchers and their corresponding outputs. Institutional membership can be inferred from affiliations listed on publications or patents 148 , 149 , and the output of an institution can be aggregated over all its affiliated researchers 150 . Institutional research information systems (CRIS) contain more comprehensive research outputs and activities from employees.
Some research questions consider the institution as a whole, investigating the returns to research and development investment 104 , inequality of resource allocation 22 and the flow of scientists 21 , 148 , 149 . Other questions focus on institutional structures as sources of research productivity by looking into the role of peer effects 125 , 151 , 152 , 153 , how institutional policies impact research outcomes 154 , 155 and whether interdisciplinary efforts foster innovation 55 . Institution-oriented measurement faces similar limitations as with analyses of individuals and teams, including name disambiguation for a given institution and the limited capacity of formal publication records to characterize the full range of relevant institutional outcomes. It is also unclear how to allocate credit among multiple institutions associated with a paper. Moreover, relevant institutional employees extend beyond publishing researchers: interns, technicians and administrators all contribute to research endeavours 130 .
In sum, measurements allow researchers to quantify scientific production and use across numerous dimensions, but they also raise questions of construct validity: Does the proposed metric really reflect what we want to measure? Testing the construct’s validity is important, as is understanding a construct’s limits. Where possible, using alternative measurement approaches, or qualitative methods such as interviews and surveys, can improve measurement accuracy and the robustness of findings.
Empirical methods
In this section, we review two broad categories of empirical approaches (Table 1 ), each with distinctive goals: (1) to discover, estimate and predict empirical regularities; and (2) to identify causal mechanisms. For each method, we give a concrete example to help to explain how the method works, summarize related work for interested readers, and discuss contributions and limitations.
Descriptive and predictive approaches
Empirical regularities and generalizable facts.
The discovery of empirical regularities in science has had a key role in driving conceptual developments and the directions of future research. By observing empirical patterns at scale, researchers unveil central facts that shape science and present core features that theories of scientific progress and practice must explain. For example, consider citation distributions. de Solla Price first proposed that citation distributions are fat-tailed 39 , indicating that a few papers have extremely high citations while most papers have relatively few or even no citations at all. de Solla Price proposed that citation distribution was a power law, while researchers have since refined this view to show that the distribution appears log-normal, a nearly universal regularity across time and fields 156 , 157 . The fat-tailed nature of citation distributions and its universality across the sciences has in turn sparked substantial theoretical work that seeks to explain this key empirical regularity 20 , 156 , 158 , 159 .
Empirical regularities are often surprising and can contest previous beliefs of how science works. For example, it has been shown that the age distribution of great achievements peaks in middle age across a wide range of fields 107 , 121 , 160 , rejecting the common belief that young scientists typically drive breakthroughs in science. A closer look at the individual careers also indicates that productivity patterns vary widely across individuals 29 . Further, a scholar’s highest-impact papers come at a remarkably constant rate across the sequence of their work 30 , 31 .
The discovery of empirical regularities has had important roles in shaping beliefs about the nature of science 10 , 45 , 161 , 162 , sources of breakthrough ideas 15 , 163 , 164 , 165 , scientific careers 21 , 29 , 126 , 127 , the network structure of ideas and scientists 23 , 98 , 136 , 137 , 138 , 139 , 166 , gender inequality 57 , 108 , 126 , 135 , 143 , 167 , 168 , and many other areas of interest to scientists and science institutions 22 , 47 , 86 , 97 , 102 , 105 , 134 , 169 , 170 , 171 . At the same time, care must be taken to ensure that findings are not merely artefacts due to data selection or inherent bias. To differentiate meaningful patterns from spurious ones, it is important to stress test the findings through different selection criteria or across non-overlapping data sources.
Regression analysis
When investigating correlations among variables, a classic method is regression, which estimates how one set of variables explains variation in an outcome of interest. Regression can be used to test explicit hypotheses or predict outcomes. For example, researchers have investigated whether a paper’s novelty predicts its citation impact 172 . Adding additional control variables to the regression, one can further examine the robustness of the focal relationship.
Although regression analysis is useful for hypothesis testing, it bears substantial limitations. If the question one wishes to ask concerns a ‘causal’ rather than a correlational relationship, regression is poorly suited to the task as it is impossible to control for all the confounding factors. Failing to account for such ‘omitted variables’ can bias the regression coefficient estimates and lead to spurious interpretations. Further, regression models often have low goodness of fit (small R 2 ), indicating that the variables considered explain little of the outcome variation. As regressions typically focus on a specific relationship in simple functional forms, regressions tend to emphasize interpretability rather than overall predictability. The advent of predictive approaches powered by large-scale datasets and novel computational techniques offers new opportunities for modelling complex relationships with stronger predictive power.
Mechanistic models
Mechanistic modelling is an important approach to explaining empirical regularities, drawing from methods primarily used in physics. Such models predict macro-level regularities of a system by modelling micro-level interactions among basic elements with interpretable and modifiable formulars. While theoretical by nature, mechanistic models in the science of science are often empirically grounded, and this approach has developed together with the advent of large-scale, high-resolution data.
Simplicity is the core value of a mechanistic model. Consider for example, why citations follow a fat-tailed distribution. de Solla Price modelled the citing behaviour as a cumulative advantage process on a growing citation network 159 and found that if the probability a paper is cited grows linearly with its existing citations, the resulting distribution would follow a power law, broadly aligned with empirical observations. The model is intentionally simplified, ignoring myriad factors. Yet the simple cumulative advantage process is by itself sufficient in explaining a power law distribution of citations. In this way, mechanistic models can help to reveal key mechanisms that can explain observed patterns.
Moreover, mechanistic models can be refined as empirical evidence evolves. For example, later investigations showed that citation distributions are better characterized as log-normal 156 , 173 , prompting researchers to introduce a fitness parameter to encapsulate the inherent differences in papers’ ability to attract citations 174 , 175 . Further, older papers are less likely to be cited than expected 176 , 177 , 178 , motivating more recent models 20 to introduce an additional aging effect 179 . By combining the cumulative advantage, fitness and aging effects, one can already achieve substantial predictive power not just for the overall properties of the system but also the citation dynamics of individual papers 20 .
In addition to citations, mechanistic models have been developed to understand the formation of collaborations 136 , 180 , 181 , 182 , 183 , knowledge discovery and diffusion 184 , 185 , topic selection 186 , 187 , career dynamics 30 , 31 , 188 , 189 , the growth of scientific fields 190 and the dynamics of failure in science and other domains 178 .
At the same time, some observers have argued that mechanistic models are too simplistic to capture the essence of complex real-world problems 191 . While it has been a cornerstone for the natural sciences, representing social phenomena in a limited set of mathematical equations may miss complexities and heterogeneities that make social phenomena interesting in the first place. Such concerns are not unique to the science of science, as they represent a broader theme in computational social sciences 192 , 193 , ranging from social networks 194 , 195 to human mobility 196 , 197 to epidemics 198 , 199 . Other observers have questioned the practical utility of mechanistic models and whether they can be used to guide decisions and devise actionable policies. Nevertheless, despite these limitations, several complex phenomena in the science of science are well captured by simple mechanistic models, showing a high degree of regularity beneath complex interacting systems and providing powerful insights about the nature of science. Mixing such modelling with other methods could be particularly fruitful in future investigations.
Machine learning
The science of science seeks in part to forecast promising directions for scientific research 7 , 44 . In recent years, machine learning methods have substantially advanced predictive capabilities 200 , 201 and are playing increasingly important parts in the science of science. In contrast to the previous methods, machine learning does not emphasize hypotheses or theories. Rather, it leverages complex relationships in data and optimizes goodness of fit to make predictions and categorizations.
Traditional machine learning models include supervised, semi-supervised and unsupervised learning. The model choice depends on data availability and the research question, ranging from supervised models for citation prediction 202 , 203 to unsupervised models for community detection 204 . Take for example mappings of scientific knowledge 94 , 205 , 206 . The unsupervised method applies network clustering algorithms to map the structures of science. Related visualization tools make sense of clusters from the underlying network, allowing observers to see the organization, interactions and evolution of scientific knowledge. More recently, supervised learning, and deep neural networks in particular, have witnessed especially rapid developments 207 . Neural networks can generate high-dimensional representations of unstructured data such as images and texts, which encode complex properties difficult for human experts to perceive.
Take text analysis as an example. A recent study 52 utilizes 3.3 million paper abstracts in materials science to predict the thermoelectric properties of materials. The intuition is that the words currently used to describe a material may predict its hitherto undiscovered properties (Fig. 2 ). Compared with a random material, the materials predicted by the model are eight times more likely to be reported as thermoelectric in the next 5 years, suggesting that machine learning has the potential to substantially speed up knowledge discovery, especially as data continue to grow in scale and scope. Indeed, predicting the direction of new discoveries represents one of the most promising avenues for machine learning models, with neural networks being applied widely to biology 208 , physics 209 , 210 , mathematics 211 , chemistry 212 , medicine 213 and clinical applications 214 . Neural networks also offer a quantitative framework to probe the characteristics of creative products ranging from scientific papers 53 , journals 215 , organizations 148 , to paintings and movies 32 . Neural networks can also help to predict the reproducibility of papers from a variety of disciplines at scale 53 , 216 .
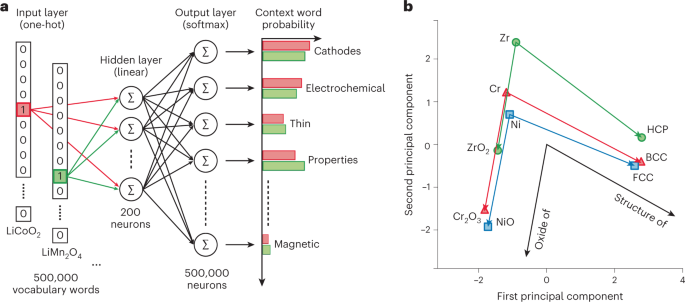
This figure illustrates the word2vec skip-gram methods 52 , where the goal is to predict useful properties of materials using previous scientific literature. a , The architecture and training process of the word2vec skip-gram model, where the 3-layer, fully connected neural network learns the 200-dimensional representation (hidden layer) from the sparse vector for each word and its context in the literature (input layer). b , The top two principal components of the word embedding. Materials with similar features are close in the 2D space, allowing prediction of a material’s properties. Different targeted words are shown in different colours. Reproduced with permission from ref. 52 , Springer Nature Ltd.
While machine learning can offer high predictive accuracy, successful applications to the science of science face challenges, particularly regarding interpretability. Researchers may value transparent and interpretable findings for how a given feature influences an outcome, rather than a black-box model. The lack of interpretability also raises concerns about bias and fairness. In predicting reproducible patterns from data, machine learning models inevitably include and reproduce biases embedded in these data, often in non-transparent ways. The fairness of machine learning 217 is heavily debated in applications ranging from the criminal justice system to hiring processes. Effective and responsible use of machine learning in the science of science therefore requires thoughtful partnership between humans and machines 53 to build a reliable system accessible to scrutiny and modification.
Causal approaches
The preceding methods can reveal core facts about the workings of science and develop predictive capacity. Yet, they fail to capture causal relationships, which are particularly useful in assessing policy interventions. For example, how can we test whether a science policy boosts or hinders the performance of individuals, teams or institutions? The overarching idea of causal approaches is to construct some counterfactual world where two groups are identical to each other except that one group experiences a treatment that the other group does not.
Towards causation
Before engaging in causal approaches, it is useful to first consider the interpretative challenges of observational data. As observational data emerge from mechanisms that are not fully known or measured, an observed correlation may be driven by underlying forces that were not accounted for in the analysis. This challenge makes causal inference fundamentally difficult in observational data. An awareness of this issue is the first step in confronting it. It further motivates intermediate empirical approaches, including the use of matching strategies and fixed effects, that can help to confront (although not fully eliminate) the inference challenge. We first consider these approaches before turning to more fully causal methods.
Matching. Matching utilizes rich information to construct a control group that is similar to the treatment group on as many observable characteristics as possible before the treatment group is exposed to the treatment. Inferences can then be made by comparing the treatment and the matched control groups. Exact matching applies to categorical values, such as country, gender, discipline or affiliation 35 , 218 . Coarsened exact matching considers percentile bins of continuous variables and matches observations in the same bin 133 . Propensity score matching estimates the probability of receiving the ‘treatment’ on the basis of the controlled variables and uses the estimates to match treatment and control groups, which reduces the matching task from comparing the values of multiple covariates to comparing a single value 24 , 219 . Dynamic matching is useful for longitudinally matching variables that change over time 220 , 221 .
Fixed effects. Fixed effects are a powerful and now standard tool in controlling for confounders. A key requirement for using fixed effects is that there are multiple observations on the same subject or entity (person, field, institution and so on) 222 , 223 , 224 . The fixed effect works as a dummy variable that accounts for the role of any fixed characteristic of that entity. Consider the finding where gender-diverse teams produce higher-impact papers than same-gender teams do 225 . A confounder may be that individuals who tend to write high-impact papers may also be more likely to work in gender-diverse teams. By including individual fixed effects, one accounts for any fixed characteristics of individuals (such as IQ, cultural background or previous education) that might drive the relationship of interest.
In sum, matching and fixed effects methods reduce potential sources of bias in interpreting relationships between variables. Yet, confounders may persist in these studies. For instance, fixed effects do not control for unobserved factors that change with time within the given entity (for example, access to funding or new skills). Identifying casual effects convincingly will then typically require distinct research methods that we turn to next.
Quasi-experiments
Researchers in economics and other fields have developed a range of quasi-experimental methods to construct treatment and control groups. The key idea here is exploiting randomness from external events that differentially expose subjects to a particular treatment. Here we review three quasi-experimental methods: difference-in-differences, instrumental variables and regression discontinuity (Fig. 3 ).

a – c , This figure presents illustrations of ( a ) differences-in-differences, ( b ) instrumental variables and ( c ) regression discontinuity methods. The solid line in b represents causal links and the dashed line represents the relationships that are not allowed, if the IV method is to produce causal inference.
Difference-in-differences. Difference-in-difference regression (DiD) investigates the effect of an unexpected event, comparing the affected group (the treated group) with an unaffected group (the control group). The control group is intended to provide the counterfactual path—what would have happened were it not for the unexpected event. Ideally, the treated and control groups are on virtually identical paths before the treatment event, but DiD can also work if the groups are on parallel paths (Fig. 3a ). For example, one study 226 examines how the premature death of superstar scientists affects the productivity of their previous collaborators. The control group are collaborators of superstars who did not die in the time frame. The two groups do not show significant differences in publications before a death event, yet upon the death of a star scientist, the treated collaborators on average experience a 5–8% decline in their quality-adjusted publication rates compared with the control group. DiD has wide applicability in the science of science, having been used to analyse the causal effects of grant design 24 , access costs to previous research 155 , 227 , university technology transfer policies 154 , intellectual property 228 , citation practices 229 , evolution of fields 221 and the impacts of paper retractions 230 , 231 , 232 . The DiD literature has grown especially rapidly in the field of economics, with substantial recent refinements 233 , 234 .
Instrumental variables. Another quasi-experimental approach utilizes ‘instrumental variables’ (IV). The goal is to determine the causal influence of some feature X on some outcome Y by using a third, instrumental variable. This instrumental variable is a quasi-random event that induces variation in X and, except for its impact through X , has no other effect on the outcome Y (Fig. 3b ). For example, consider a study of astronomy that seeks to understand how telescope time affects career advancement 235 . Here, one cannot simply look at the correlation between telescope time and career outcomes because many confounds (such as talent or grit) may influence both telescope time and career opportunities. Now consider the weather as an instrumental variable. Cloudy weather will, at random, reduce an astronomer’s observational time. Yet, the weather on particular nights is unlikely to correlate with a scientist’s innate qualities. The weather can then provide an instrumental variable to reveal a causal relationship between telescope time and career outcomes. Instrumental variables have been used to study local peer effects in research 151 , the impact of gender composition in scientific committees 236 , patents on future innovation 237 and taxes on inventor mobility 238 .
Regression discontinuity. In regression discontinuity, policies with an arbitrary threshold for receiving some benefit can be used to construct treatment and control groups (Fig. 3c ). Take the funding paylines for grant proposals as an example. Proposals with scores increasingly close to the payline are increasingly similar in their both observable and unobservable characteristics, yet only those projects with scores above the payline receive the funding. For example, a study 110 examines the effect of winning an early-career grant on the probability of winning a later, mid-career grant. The probability has a discontinuous jump across the initial grant’s payline, providing the treatment and control groups needed to estimate the causal effect of receiving a grant. This example utilizes the ‘sharp’ regression discontinuity that assumes treatment status to be fully determined by the cut-off. If we assume treatment status is only partly determined by the cut-off, we can use ‘fuzzy’ regression discontinuity designs. Here the probability of receiving a grant is used to estimate the future outcome 11 , 110 , 239 , 240 , 241 .
Although quasi-experiments are powerful tools, they face their own limitations. First, these approaches identify causal effects within a specific context and often engage small numbers of observations. How representative the samples are for broader populations or contexts is typically left as an open question. Second, the validity of the causal design is typically not ironclad. Researchers usually conduct different robustness checks to verify whether observable confounders have significant differences between the treated and control groups, before treatment. However, unobservable features may still differ between treatment and control groups. The quality of instrumental variables and the specific claim that they have no effect on the outcome except through the variable of interest, is also difficult to assess. Ultimately, researchers must rely partly on judgement to tell whether appropriate conditions are met for causal inference.
This section emphasized popular econometric approaches to causal inference. Other empirical approaches, such as graphical causal modelling 242 , 243 , also represent an important stream of work on assessing causal relationships. Such approaches usually represent causation as a directed acyclic graph, with nodes as variables and arrows between them as suspected causal relationships. In the science of science, the directed acyclic graph approach has been applied to quantify the causal effect of journal impact factor 244 and gender or racial bias 245 on citations. Graphical causal modelling has also triggered discussions on strengths and weaknesses compared to the econometrics methods 246 , 247 .
Experiments
In contrast to quasi-experimental approaches, laboratory and field experiments conduct direct randomization in assigning treatment and control groups. These methods engage explicitly in the data generation process, manipulating interventions to observe counterfactuals. These experiments are crafted to study mechanisms of specific interest and, by designing the experiment and formally randomizing, can produce especially rigorous causal inference.
Laboratory experiments. Laboratory experiments build counterfactual worlds in well-controlled laboratory environments. Researchers randomly assign participants to the treatment or control group and then manipulate the laboratory conditions to observe different outcomes in the two groups. For example, consider laboratory experiments on team performance and gender composition 144 , 248 . The researchers randomly assign participants into groups to perform tasks such as solving puzzles or brainstorming. Teams with a higher proportion of women are found to perform better on average, offering evidence that gender diversity is causally linked to team performance. Laboratory experiments can allow researchers to test forces that are otherwise hard to observe, such as how competition influences creativity 249 . Laboratory experiments have also been used to evaluate how journal impact factors shape scientists’ perceptions of rewards 250 and gender bias in hiring 251 .
Laboratory experiments allow for precise control of settings and procedures to isolate causal effects of interest. However, participants may behave differently in synthetic environments than in real-world settings, raising questions about the generalizability and replicability of the results 252 , 253 , 254 . To assess causal effects in real-world settings, researcher use randomized controlled trials.
Randomized controlled trials. A randomized controlled trial (RCT), or field experiment, is a staple for causal inference across a wide range of disciplines. RCTs randomly assign participants into the treatment and control conditions 255 and can be used not only to assess mechanisms but also to test real-world interventions such as policy change. The science of science has witnessed growing use of RCTs. For instance, a field experiment 146 investigated whether lower search costs for collaborators increased collaboration in grant applications. The authors randomly allocated principal investigators to face-to-face sessions in a medical school, and then measured participants’ chance of writing a grant proposal together. RCTs have also offered rich causal insights on peer review 256 , 257 , 258 , 259 , 260 and gender bias in science 261 , 262 , 263 .
While powerful, RCTs are difficult to conduct in the science of science, mainly for two reasons. The first concerns potential risks in a policy intervention. For instance, while randomizing funding across individuals could generate crucial causal insights for funders, it may also inadvertently harm participants’ careers 264 . Second, key questions in the science of science often require a long-time horizon to trace outcomes, which makes RCTs costly. It also raises the difficulty of replicating findings. A relative advantage of the quasi-experimental methods discussed earlier is that one can identify causal effects over potentially long periods of time in the historical record. On the other hand, quasi-experiments must be found as opposed to designed, and they often are not available for many questions of interest. While the best approaches are context dependent, a growing community of researchers is building platforms to facilitate RCTs for the science of science, aiming to lower their costs and increase their scale. Performing RCTs in partnership with science institutions can also contribute to timely, policy-relevant research that may substantially improve science decision-making and investments.
Research in the science of science has been empowered by the growth of high-scale data, new measurement approaches and an expanding range of empirical methods. These tools provide enormous capacity to test conceptual frameworks about science, discover factors impacting scientific productivity, predict key scientific outcomes and design policies that better facilitate future scientific progress. A careful appreciation of empirical techniques can help researchers to choose effective tools for questions of interest and propel the field. A better and broader understanding of these methodologies may also build bridges across diverse research communities, facilitating communication and collaboration, and better leveraging the value of diverse perspectives. The science of science is about turning scientific methods on the nature of science itself. The fruits of this work, with time, can guide researchers and research institutions to greater progress in discovery and understanding across the landscape of scientific inquiry.
Bush, V . S cience–the Endless Frontier: A Report to the President on a Program for Postwar Scientific Research (National Science Foundation, 1990).
Mokyr, J. The Gifts of Athena (Princeton Univ. Press, 2011).
Jones, B. F. in Rebuilding the Post-Pandemic Economy (eds Kearney, M. S. & Ganz, A.) 272–310 (Aspen Institute Press, 2021).
Wang, D. & Barabási, A.-L. The Science of Science (Cambridge Univ. Press, 2021).
Fortunato, S. et al. Science of science. Science 359 , eaao0185 (2018).
Article PubMed PubMed Central Google Scholar
Azoulay, P. et al. Toward a more scientific science. Science 361 , 1194–1197 (2018).
Article PubMed Google Scholar
Clauset, A., Larremore, D. B. & Sinatra, R. Data-driven predictions in the science of science. Science 355 , 477–480 (2017).
Article CAS PubMed Google Scholar
Zeng, A. et al. The science of science: from the perspective of complex systems. Phys. Rep. 714 , 1–73 (2017).
Article Google Scholar
Lin, Z., Yin. Y., Liu, L. & Wang, D. SciSciNet: a large-scale open data lake for the science of science research. Sci. Data, https://doi.org/10.1038/s41597-023-02198-9 (2023).
Ahmadpoor, M. & Jones, B. F. The dual frontier: patented inventions and prior scientific advance. Science 357 , 583–587 (2017).
Azoulay, P., Graff Zivin, J. S., Li, D. & Sampat, B. N. Public R&D investments and private-sector patenting: evidence from NIH funding rules. Rev. Econ. Stud. 86 , 117–152 (2019).
Yin, Y., Dong, Y., Wang, K., Wang, D. & Jones, B. F. Public use and public funding of science. Nat. Hum. Behav. 6 , 1344–1350 (2022).
Merton, R. K. The Sociology of Science: Theoretical and Empirical Investigations (Univ. Chicago Press, 1973).
Kuhn, T. The Structure of Scientific Revolutions (Princeton Univ. Press, 2021).
Uzzi, B., Mukherjee, S., Stringer, M. & Jones, B. Atypical combinations and scientific impact. Science 342 , 468–472 (2013).
Zuckerman, H. Scientific Elite: Nobel Laureates in the United States (Transaction Publishers, 1977).
Wu, L., Wang, D. & Evans, J. A. Large teams develop and small teams disrupt science and technology. Nature 566 , 378–382 (2019).
Wuchty, S., Jones, B. F. & Uzzi, B. The increasing dominance of teams in production of knowledge. Science 316 , 1036–1039 (2007).
Foster, J. G., Rzhetsky, A. & Evans, J. A. Tradition and innovation in scientists’ research strategies. Am. Sociol. Rev. 80 , 875–908 (2015).
Wang, D., Song, C. & Barabási, A.-L. Quantifying long-term scientific impact. Science 342 , 127–132 (2013).
Clauset, A., Arbesman, S. & Larremore, D. B. Systematic inequality and hierarchy in faculty hiring networks. Sci. Adv. 1 , e1400005 (2015).
Ma, A., Mondragón, R. J. & Latora, V. Anatomy of funded research in science. Proc. Natl Acad. Sci. USA 112 , 14760–14765 (2015).
Article CAS PubMed PubMed Central Google Scholar
Ma, Y. & Uzzi, B. Scientific prize network predicts who pushes the boundaries of science. Proc. Natl Acad. Sci. USA 115 , 12608–12615 (2018).
Azoulay, P., Graff Zivin, J. S. & Manso, G. Incentives and creativity: evidence from the academic life sciences. RAND J. Econ. 42 , 527–554 (2011).
Schor, S. & Karten, I. Statistical evaluation of medical journal manuscripts. JAMA 195 , 1123–1128 (1966).
Platt, J. R. Strong inference: certain systematic methods of scientific thinking may produce much more rapid progress than others. Science 146 , 347–353 (1964).
Ioannidis, J. P. Why most published research findings are false. PLoS Med. 2 , e124 (2005).
Simonton, D. K. Career landmarks in science: individual differences and interdisciplinary contrasts. Dev. Psychol. 27 , 119 (1991).
Way, S. F., Morgan, A. C., Clauset, A. & Larremore, D. B. The misleading narrative of the canonical faculty productivity trajectory. Proc. Natl Acad. Sci. USA 114 , E9216–E9223 (2017).
Sinatra, R., Wang, D., Deville, P., Song, C. & Barabási, A.-L. Quantifying the evolution of individual scientific impact. Science 354 , aaf5239 (2016).
Liu, L. et al. Hot streaks in artistic, cultural, and scientific careers. Nature 559 , 396–399 (2018).
Liu, L., Dehmamy, N., Chown, J., Giles, C. L. & Wang, D. Understanding the onset of hot streaks across artistic, cultural, and scientific careers. Nat. Commun. 12 , 5392 (2021).
Squazzoni, F. et al. Peer review and gender bias: a study on 145 scholarly journals. Sci. Adv. 7 , eabd0299 (2021).
Hofstra, B. et al. The diversity–innovation paradox in science. Proc. Natl Acad. Sci. USA 117 , 9284–9291 (2020).
Huang, J., Gates, A. J., Sinatra, R. & Barabási, A.-L. Historical comparison of gender inequality in scientific careers across countries and disciplines. Proc. Natl Acad. Sci. USA 117 , 4609–4616 (2020).
Gläser, J. & Laudel, G. Governing science: how science policy shapes research content. Eur. J. Sociol. 57 , 117–168 (2016).
Stephan, P. E. How Economics Shapes Science (Harvard Univ. Press, 2012).
Garfield, E. & Sher, I. H. New factors in the evaluation of scientific literature through citation indexing. Am. Doc. 14 , 195–201 (1963).
Article CAS Google Scholar
de Solla Price, D. J. Networks of scientific papers. Science 149 , 510–515 (1965).
Etzkowitz, H., Kemelgor, C. & Uzzi, B. Athena Unbound: The Advancement of Women in Science and Technology (Cambridge Univ. Press, 2000).
Simonton, D. K. Scientific Genius: A Psychology of Science (Cambridge Univ. Press, 1988).
Khabsa, M. & Giles, C. L. The number of scholarly documents on the public web. PLoS ONE 9 , e93949 (2014).
Xia, F., Wang, W., Bekele, T. M. & Liu, H. Big scholarly data: a survey. IEEE Trans. Big Data 3 , 18–35 (2017).
Evans, J. A. & Foster, J. G. Metaknowledge. Science 331 , 721–725 (2011).
Milojević, S. Quantifying the cognitive extent of science. J. Informetr. 9 , 962–973 (2015).
Rzhetsky, A., Foster, J. G., Foster, I. T. & Evans, J. A. Choosing experiments to accelerate collective discovery. Proc. Natl Acad. Sci. USA 112 , 14569–14574 (2015).
Poncela-Casasnovas, J., Gerlach, M., Aguirre, N. & Amaral, L. A. Large-scale analysis of micro-level citation patterns reveals nuanced selection criteria. Nat. Hum. Behav. 3 , 568–575 (2019).
Hardwicke, T. E. et al. Data availability, reusability, and analytic reproducibility: evaluating the impact of a mandatory open data policy at the journal Cognition. R. Soc. Open Sci. 5 , 180448 (2018).
Nagaraj, A., Shears, E. & de Vaan, M. Improving data access democratizes and diversifies science. Proc. Natl Acad. Sci. USA 117 , 23490–23498 (2020).
Bravo, G., Grimaldo, F., López-Iñesta, E., Mehmani, B. & Squazzoni, F. The effect of publishing peer review reports on referee behavior in five scholarly journals. Nat. Commun. 10 , 322 (2019).
Tran, D. et al. An open review of open review: a critical analysis of the machine learning conference review process. Preprint at https://doi.org/10.48550/arXiv.2010.05137 (2020).
Tshitoyan, V. et al. Unsupervised word embeddings capture latent knowledge from materials science literature. Nature 571 , 95–98 (2019).
Yang, Y., Wu, Y. & Uzzi, B. Estimating the deep replicability of scientific findings using human and artificial intelligence. Proc. Natl Acad. Sci. USA 117 , 10762–10768 (2020).
Mukherjee, S., Uzzi, B., Jones, B. & Stringer, M. A new method for identifying recombinations of existing knowledge associated with high‐impact innovation. J. Prod. Innov. Manage. 33 , 224–236 (2016).
Leahey, E., Beckman, C. M. & Stanko, T. L. Prominent but less productive: the impact of interdisciplinarity on scientists’ research. Adm. Sci. Q. 62 , 105–139 (2017).
Sauermann, H. & Haeussler, C. Authorship and contribution disclosures. Sci. Adv. 3 , e1700404 (2017).
Oliveira, D. F. M., Ma, Y., Woodruff, T. K. & Uzzi, B. Comparison of National Institutes of Health grant amounts to first-time male and female principal investigators. JAMA 321 , 898–900 (2019).
Yang, Y., Chawla, N. V. & Uzzi, B. A network’s gender composition and communication pattern predict women’s leadership success. Proc. Natl Acad. Sci. USA 116 , 2033–2038 (2019).
Way, S. F., Larremore, D. B. & Clauset, A. Gender, productivity, and prestige in computer science faculty hiring networks. In Proc. 25th International Conference on World Wide Web 1169–1179. (ACM 2016)
Malmgren, R. D., Ottino, J. M. & Amaral, L. A. N. The role of mentorship in protege performance. Nature 465 , 622–626 (2010).
Ma, Y., Mukherjee, S. & Uzzi, B. Mentorship and protégé success in STEM fields. Proc. Natl Acad. Sci. USA 117 , 14077–14083 (2020).
Börner, K. et al. Skill discrepancies between research, education, and jobs reveal the critical need to supply soft skills for the data economy. Proc. Natl Acad. Sci. USA 115 , 12630–12637 (2018).
Biasi, B. & Ma, S. The Education-Innovation Gap (National Bureau of Economic Research Working papers, 2020).
Bornmann, L. Do altmetrics point to the broader impact of research? An overview of benefits and disadvantages of altmetrics. J. Informetr. 8 , 895–903 (2014).
Cleary, E. G., Beierlein, J. M., Khanuja, N. S., McNamee, L. M. & Ledley, F. D. Contribution of NIH funding to new drug approvals 2010–2016. Proc. Natl Acad. Sci. USA 115 , 2329–2334 (2018).
Spector, J. M., Harrison, R. S. & Fishman, M. C. Fundamental science behind today’s important medicines. Sci. Transl. Med. 10 , eaaq1787 (2018).
Haunschild, R. & Bornmann, L. How many scientific papers are mentioned in policy-related documents? An empirical investigation using Web of Science and Altmetric data. Scientometrics 110 , 1209–1216 (2017).
Yin, Y., Gao, J., Jones, B. F. & Wang, D. Coevolution of policy and science during the pandemic. Science 371 , 128–130 (2021).
Sugimoto, C. R., Work, S., Larivière, V. & Haustein, S. Scholarly use of social media and altmetrics: a review of the literature. J. Assoc. Inf. Sci. Technol. 68 , 2037–2062 (2017).
Dunham, I. Human genes: time to follow the roads less traveled? PLoS Biol. 16 , e3000034 (2018).
Kustatscher, G. et al. Understudied proteins: opportunities and challenges for functional proteomics. Nat. Methods 19 , 774–779 (2022).
Rosenthal, R. The file drawer problem and tolerance for null results. Psychol. Bull. 86 , 638 (1979).
Franco, A., Malhotra, N. & Simonovits, G. Publication bias in the social sciences: unlocking the file drawer. Science 345 , 1502–1505 (2014).
Vera-Baceta, M.-A., Thelwall, M. & Kousha, K. Web of Science and Scopus language coverage. Scientometrics 121 , 1803–1813 (2019).
Waltman, L. A review of the literature on citation impact indicators. J. Informetr. 10 , 365–391 (2016).
Garfield, E. & Merton, R. K. Citation Indexing: Its Theory and Application in Science, Technology, and Humanities (Wiley, 1979).
Kelly, B., Papanikolaou, D., Seru, A. & Taddy, M. Measuring Technological Innovation Over the Long Run Report No. 0898-2937 (National Bureau of Economic Research, 2018).
Kogan, L., Papanikolaou, D., Seru, A. & Stoffman, N. Technological innovation, resource allocation, and growth. Q. J. Econ. 132 , 665–712 (2017).
Hall, B. H., Jaffe, A. & Trajtenberg, M. Market value and patent citations. RAND J. Econ. 36 , 16–38 (2005).
Google Scholar
Yan, E. & Ding, Y. Applying centrality measures to impact analysis: a coauthorship network analysis. J. Am. Soc. Inf. Sci. Technol. 60 , 2107–2118 (2009).
Radicchi, F., Fortunato, S., Markines, B. & Vespignani, A. Diffusion of scientific credits and the ranking of scientists. Phys. Rev. E 80 , 056103 (2009).
Bollen, J., Rodriquez, M. A. & Van de Sompel, H. Journal status. Scientometrics 69 , 669–687 (2006).
Bergstrom, C. T., West, J. D. & Wiseman, M. A. The eigenfactor™ metrics. J. Neurosci. 28 , 11433–11434 (2008).
Cronin, B. & Sugimoto, C. R. Beyond Bibliometrics: Harnessing Multidimensional Indicators of Scholarly Impact (MIT Press, 2014).
Hicks, D., Wouters, P., Waltman, L., De Rijcke, S. & Rafols, I. Bibliometrics: the Leiden Manifesto for research metrics. Nature 520 , 429–431 (2015).
Catalini, C., Lacetera, N. & Oettl, A. The incidence and role of negative citations in science. Proc. Natl Acad. Sci. USA 112 , 13823–13826 (2015).
Alcacer, J. & Gittelman, M. Patent citations as a measure of knowledge flows: the influence of examiner citations. Rev. Econ. Stat. 88 , 774–779 (2006).
Ding, Y. et al. Content‐based citation analysis: the next generation of citation analysis. J. Assoc. Inf. Sci. Technol. 65 , 1820–1833 (2014).
Teufel, S., Siddharthan, A. & Tidhar, D. Automatic classification of citation function. In Proc. 2006 Conference on Empirical Methods in Natural Language Processing, 103–110 (Association for Computational Linguistics 2006)
Seeber, M., Cattaneo, M., Meoli, M. & Malighetti, P. Self-citations as strategic response to the use of metrics for career decisions. Res. Policy 48 , 478–491 (2019).
Pendlebury, D. A. The use and misuse of journal metrics and other citation indicators. Arch. Immunol. Ther. Exp. 57 , 1–11 (2009).
Biagioli, M. Watch out for cheats in citation game. Nature 535 , 201 (2016).
Jo, W. S., Liu, L. & Wang, D. See further upon the giants: quantifying intellectual lineage in science. Quant. Sci. Stud. 3 , 319–330 (2022).
Boyack, K. W., Klavans, R. & Börner, K. Mapping the backbone of science. Scientometrics 64 , 351–374 (2005).
Gates, A. J., Ke, Q., Varol, O. & Barabási, A.-L. Nature’s reach: narrow work has broad impact. Nature 575 , 32–34 (2019).
Börner, K., Penumarthy, S., Meiss, M. & Ke, W. Mapping the diffusion of scholarly knowledge among major US research institutions. Scientometrics 68 , 415–426 (2006).
King, D. A. The scientific impact of nations. Nature 430 , 311–316 (2004).
Pan, R. K., Kaski, K. & Fortunato, S. World citation and collaboration networks: uncovering the role of geography in science. Sci. Rep. 2 , 902 (2012).
Jaffe, A. B., Trajtenberg, M. & Henderson, R. Geographic localization of knowledge spillovers as evidenced by patent citations. Q. J. Econ. 108 , 577–598 (1993).
Funk, R. J. & Owen-Smith, J. A dynamic network measure of technological change. Manage. Sci. 63 , 791–817 (2017).
Yegros-Yegros, A., Rafols, I. & D’este, P. Does interdisciplinary research lead to higher citation impact? The different effect of proximal and distal interdisciplinarity. PLoS ONE 10 , e0135095 (2015).
Larivière, V., Haustein, S. & Börner, K. Long-distance interdisciplinarity leads to higher scientific impact. PLoS ONE 10 , e0122565 (2015).
Fleming, L., Greene, H., Li, G., Marx, M. & Yao, D. Government-funded research increasingly fuels innovation. Science 364 , 1139–1141 (2019).
Bowen, A. & Casadevall, A. Increasing disparities between resource inputs and outcomes, as measured by certain health deliverables, in biomedical research. Proc. Natl Acad. Sci. USA 112 , 11335–11340 (2015).
Li, D., Azoulay, P. & Sampat, B. N. The applied value of public investments in biomedical research. Science 356 , 78–81 (2017).
Lehman, H. C. Age and Achievement (Princeton Univ. Press, 2017).
Simonton, D. K. Creative productivity: a predictive and explanatory model of career trajectories and landmarks. Psychol. Rev. 104 , 66 (1997).
Duch, J. et al. The possible role of resource requirements and academic career-choice risk on gender differences in publication rate and impact. PLoS ONE 7 , e51332 (2012).
Wang, Y., Jones, B. F. & Wang, D. Early-career setback and future career impact. Nat. Commun. 10 , 4331 (2019).
Bol, T., de Vaan, M. & van de Rijt, A. The Matthew effect in science funding. Proc. Natl Acad. Sci. USA 115 , 4887–4890 (2018).
Jones, B. F. Age and great invention. Rev. Econ. Stat. 92 , 1–14 (2010).
Newman, M. Networks (Oxford Univ. Press, 2018).
Mazloumian, A., Eom, Y.-H., Helbing, D., Lozano, S. & Fortunato, S. How citation boosts promote scientific paradigm shifts and nobel prizes. PLoS ONE 6 , e18975 (2011).
Hirsch, J. E. An index to quantify an individual’s scientific research output. Proc. Natl Acad. Sci. USA 102 , 16569–16572 (2005).
Alonso, S., Cabrerizo, F. J., Herrera-Viedma, E. & Herrera, F. h-index: a review focused in its variants, computation and standardization for different scientific fields. J. Informetr. 3 , 273–289 (2009).
Egghe, L. An improvement of the h-index: the g-index. ISSI Newsl. 2 , 8–9 (2006).
Kaur, J., Radicchi, F. & Menczer, F. Universality of scholarly impact metrics. J. Informetr. 7 , 924–932 (2013).
Majeti, D. et al. Scholar plot: design and evaluation of an information interface for faculty research performance. Front. Res. Metr. Anal. 4 , 6 (2020).
Sidiropoulos, A., Katsaros, D. & Manolopoulos, Y. Generalized Hirsch h-index for disclosing latent facts in citation networks. Scientometrics 72 , 253–280 (2007).
Jones, B. F. & Weinberg, B. A. Age dynamics in scientific creativity. Proc. Natl Acad. Sci. USA 108 , 18910–18914 (2011).
Dennis, W. Age and productivity among scientists. Science 123 , 724–725 (1956).
Sanyal, D. K., Bhowmick, P. K. & Das, P. P. A review of author name disambiguation techniques for the PubMed bibliographic database. J. Inf. Sci. 47 , 227–254 (2021).
Haak, L. L., Fenner, M., Paglione, L., Pentz, E. & Ratner, H. ORCID: a system to uniquely identify researchers. Learn. Publ. 25 , 259–264 (2012).
Malmgren, R. D., Ottino, J. M. & Amaral, L. A. N. The role of mentorship in protégé performance. Nature 465 , 662–667 (2010).
Oettl, A. Reconceptualizing stars: scientist helpfulness and peer performance. Manage. Sci. 58 , 1122–1140 (2012).
Morgan, A. C. et al. The unequal impact of parenthood in academia. Sci. Adv. 7 , eabd1996 (2021).
Morgan, A. C. et al. Socioeconomic roots of academic faculty. Nat. Hum. Behav. 6 , 1625–1633 (2022).
San Francisco Declaration on Research Assessment (DORA) (American Society for Cell Biology, 2012).
Falk‐Krzesinski, H. J. et al. Advancing the science of team science. Clin. Transl. Sci. 3 , 263–266 (2010).
Cooke, N. J. et al. Enhancing the Effectiveness of Team Science (National Academies Press, 2015).
Börner, K. et al. A multi-level systems perspective for the science of team science. Sci. Transl. Med. 2 , 49cm24 (2010).
Leahey, E. From sole investigator to team scientist: trends in the practice and study of research collaboration. Annu. Rev. Sociol. 42 , 81–100 (2016).
AlShebli, B. K., Rahwan, T. & Woon, W. L. The preeminence of ethnic diversity in scientific collaboration. Nat. Commun. 9 , 5163 (2018).
Hsiehchen, D., Espinoza, M. & Hsieh, A. Multinational teams and diseconomies of scale in collaborative research. Sci. Adv. 1 , e1500211 (2015).
Koning, R., Samila, S. & Ferguson, J.-P. Who do we invent for? Patents by women focus more on women’s health, but few women get to invent. Science 372 , 1345–1348 (2021).
Barabâsi, A.-L. et al. Evolution of the social network of scientific collaborations. Physica A 311 , 590–614 (2002).
Newman, M. E. Scientific collaboration networks. I. Network construction and fundamental results. Phys. Rev. E 64 , 016131 (2001).
Newman, M. E. Scientific collaboration networks. II. Shortest paths, weighted networks, and centrality. Phys. Rev. E 64 , 016132 (2001).
Palla, G., Barabási, A.-L. & Vicsek, T. Quantifying social group evolution. Nature 446 , 664–667 (2007).
Ross, M. B. et al. Women are credited less in science than men. Nature 608 , 135–145 (2022).
Shen, H.-W. & Barabási, A.-L. Collective credit allocation in science. Proc. Natl Acad. Sci. USA 111 , 12325–12330 (2014).
Merton, R. K. Matthew effect in science. Science 159 , 56–63 (1968).
Ni, C., Smith, E., Yuan, H., Larivière, V. & Sugimoto, C. R. The gendered nature of authorship. Sci. Adv. 7 , eabe4639 (2021).
Woolley, A. W., Chabris, C. F., Pentland, A., Hashmi, N. & Malone, T. W. Evidence for a collective intelligence factor in the performance of human groups. Science 330 , 686–688 (2010).
Feldon, D. F. et al. Postdocs’ lab engagement predicts trajectories of PhD students’ skill development. Proc. Natl Acad. Sci. USA 116 , 20910–20916 (2019).
Boudreau, K. J. et al. A field experiment on search costs and the formation of scientific collaborations. Rev. Econ. Stat. 99 , 565–576 (2017).
Holcombe, A. O. Contributorship, not authorship: use CRediT to indicate who did what. Publications 7 , 48 (2019).
Murray, D. et al. Unsupervised embedding of trajectories captures the latent structure of mobility. Preprint at https://doi.org/10.48550/arXiv.2012.02785 (2020).
Deville, P. et al. Career on the move: geography, stratification, and scientific impact. Sci. Rep. 4 , 4770 (2014).
Edmunds, L. D. et al. Why do women choose or reject careers in academic medicine? A narrative review of empirical evidence. Lancet 388 , 2948–2958 (2016).
Waldinger, F. Peer effects in science: evidence from the dismissal of scientists in Nazi Germany. Rev. Econ. Stud. 79 , 838–861 (2012).
Agrawal, A., McHale, J. & Oettl, A. How stars matter: recruiting and peer effects in evolutionary biology. Res. Policy 46 , 853–867 (2017).
Fiore, S. M. Interdisciplinarity as teamwork: how the science of teams can inform team science. Small Group Res. 39 , 251–277 (2008).
Hvide, H. K. & Jones, B. F. University innovation and the professor’s privilege. Am. Econ. Rev. 108 , 1860–1898 (2018).
Murray, F., Aghion, P., Dewatripont, M., Kolev, J. & Stern, S. Of mice and academics: examining the effect of openness on innovation. Am. Econ. J. Econ. Policy 8 , 212–252 (2016).
Radicchi, F., Fortunato, S. & Castellano, C. Universality of citation distributions: toward an objective measure of scientific impact. Proc. Natl Acad. Sci. USA 105 , 17268–17272 (2008).
Waltman, L., van Eck, N. J. & van Raan, A. F. Universality of citation distributions revisited. J. Am. Soc. Inf. Sci. Technol. 63 , 72–77 (2012).
Barabási, A.-L. & Albert, R. Emergence of scaling in random networks. Science 286 , 509–512 (1999).
de Solla Price, D. A general theory of bibliometric and other cumulative advantage processes. J. Am. Soc. Inf. Sci. 27 , 292–306 (1976).
Cole, S. Age and scientific performance. Am. J. Sociol. 84 , 958–977 (1979).
Ke, Q., Ferrara, E., Radicchi, F. & Flammini, A. Defining and identifying sleeping beauties in science. Proc. Natl Acad. Sci. USA 112 , 7426–7431 (2015).
Bornmann, L., de Moya Anegón, F. & Leydesdorff, L. Do scientific advancements lean on the shoulders of giants? A bibliometric investigation of the Ortega hypothesis. PLoS ONE 5 , e13327 (2010).
Mukherjee, S., Romero, D. M., Jones, B. & Uzzi, B. The nearly universal link between the age of past knowledge and tomorrow’s breakthroughs in science and technology: the hotspot. Sci. Adv. 3 , e1601315 (2017).
Packalen, M. & Bhattacharya, J. NIH funding and the pursuit of edge science. Proc. Natl Acad. Sci. USA 117 , 12011–12016 (2020).
Zeng, A., Fan, Y., Di, Z., Wang, Y. & Havlin, S. Fresh teams are associated with original and multidisciplinary research. Nat. Hum. Behav. 5 , 1314–1322 (2021).
Newman, M. E. The structure of scientific collaboration networks. Proc. Natl Acad. Sci. USA 98 , 404–409 (2001).
Larivière, V., Ni, C., Gingras, Y., Cronin, B. & Sugimoto, C. R. Bibliometrics: global gender disparities in science. Nature 504 , 211–213 (2013).
West, J. D., Jacquet, J., King, M. M., Correll, S. J. & Bergstrom, C. T. The role of gender in scholarly authorship. PLoS ONE 8 , e66212 (2013).
Gao, J., Yin, Y., Myers, K. R., Lakhani, K. R. & Wang, D. Potentially long-lasting effects of the pandemic on scientists. Nat. Commun. 12 , 6188 (2021).
Jones, B. F., Wuchty, S. & Uzzi, B. Multi-university research teams: shifting impact, geography, and stratification in science. Science 322 , 1259–1262 (2008).
Chu, J. S. & Evans, J. A. Slowed canonical progress in large fields of science. Proc. Natl Acad. Sci. USA 118 , e2021636118 (2021).
Wang, J., Veugelers, R. & Stephan, P. Bias against novelty in science: a cautionary tale for users of bibliometric indicators. Res. Policy 46 , 1416–1436 (2017).
Stringer, M. J., Sales-Pardo, M. & Amaral, L. A. Statistical validation of a global model for the distribution of the ultimate number of citations accrued by papers published in a scientific journal. J. Assoc. Inf. Sci. Technol. 61 , 1377–1385 (2010).
Bianconi, G. & Barabási, A.-L. Bose-Einstein condensation in complex networks. Phys. Rev. Lett. 86 , 5632 (2001).
Bianconi, G. & Barabási, A.-L. Competition and multiscaling in evolving networks. Europhys. Lett. 54 , 436 (2001).
Yin, Y. & Wang, D. The time dimension of science: connecting the past to the future. J. Informetr. 11 , 608–621 (2017).
Pan, R. K., Petersen, A. M., Pammolli, F. & Fortunato, S. The memory of science: Inflation, myopia, and the knowledge network. J. Informetr. 12 , 656–678 (2018).
Yin, Y., Wang, Y., Evans, J. A. & Wang, D. Quantifying the dynamics of failure across science, startups and security. Nature 575 , 190–194 (2019).
Candia, C. & Uzzi, B. Quantifying the selective forgetting and integration of ideas in science and technology. Am. Psychol. 76 , 1067 (2021).
Milojević, S. Principles of scientific research team formation and evolution. Proc. Natl Acad. Sci. USA 111 , 3984–3989 (2014).
Guimera, R., Uzzi, B., Spiro, J. & Amaral, L. A. N. Team assembly mechanisms determine collaboration network structure and team performance. Science 308 , 697–702 (2005).
Newman, M. E. Coauthorship networks and patterns of scientific collaboration. Proc. Natl Acad. Sci. USA 101 , 5200–5205 (2004).
Newman, M. E. Clustering and preferential attachment in growing networks. Phys. Rev. E 64 , 025102 (2001).
Iacopini, I., Milojević, S. & Latora, V. Network dynamics of innovation processes. Phys. Rev. Lett. 120 , 048301 (2018).
Kuhn, T., Perc, M. & Helbing, D. Inheritance patterns in citation networks reveal scientific memes. Phys. Rev. 4 , 041036 (2014).
Jia, T., Wang, D. & Szymanski, B. K. Quantifying patterns of research-interest evolution. Nat. Hum. Behav. 1 , 0078 (2017).
Zeng, A. et al. Increasing trend of scientists to switch between topics. Nat. Commun. https://doi.org/10.1038/s41467-019-11401-8 (2019).
Siudem, G., Żogała-Siudem, B., Cena, A. & Gagolewski, M. Three dimensions of scientific impact. Proc. Natl Acad. Sci. USA 117 , 13896–13900 (2020).
Petersen, A. M. et al. Reputation and impact in academic careers. Proc. Natl Acad. Sci. USA 111 , 15316–15321 (2014).
Jin, C., Song, C., Bjelland, J., Canright, G. & Wang, D. Emergence of scaling in complex substitutive systems. Nat. Hum. Behav. 3 , 837–846 (2019).
Hofman, J. M. et al. Integrating explanation and prediction in computational social science. Nature 595 , 181–188 (2021).
Lazer, D. et al. Computational social science. Science 323 , 721–723 (2009).
Lazer, D. M. et al. Computational social science: obstacles and opportunities. Science 369 , 1060–1062 (2020).
Albert, R. & Barabási, A.-L. Statistical mechanics of complex networks. Rev. Mod. Phys. 74 , 47 (2002).
Newman, M. E. The structure and function of complex networks. SIAM Rev. 45 , 167–256 (2003).
Song, C., Qu, Z., Blumm, N. & Barabási, A.-L. Limits of predictability in human mobility. Science 327 , 1018–1021 (2010).
Alessandretti, L., Aslak, U. & Lehmann, S. The scales of human mobility. Nature 587 , 402–407 (2020).
Pastor-Satorras, R. & Vespignani, A. Epidemic spreading in scale-free networks. Phys. Rev. Lett. 86 , 3200 (2001).
Pastor-Satorras, R., Castellano, C., Van Mieghem, P. & Vespignani, A. Epidemic processes in complex networks. Rev. Mod. Phys. 87 , 925 (2015).
Goodfellow, I., Bengio, Y. & Courville, A. Deep Learning (MIT Press, 2016).
Bishop, C. M. Pattern Recognition and Machine Learning (Springer, 2006).
Dong, Y., Johnson, R. A. & Chawla, N. V. Will this paper increase your h-index? Scientific impact prediction. In Proc. 8th ACM International Conference on Web Search and Data Mining, 149–158 (ACM 2015)
Xiao, S. et al. On modeling and predicting individual paper citation count over time. In IJCAI, 2676–2682 (IJCAI, 2016)
Fortunato, S. Community detection in graphs. Phys. Rep. 486 , 75–174 (2010).
Chen, C. Science mapping: a systematic review of the literature. J. Data Inf. Sci. 2 , 1–40 (2017).
CAS Google Scholar
Van Eck, N. J. & Waltman, L. Citation-based clustering of publications using CitNetExplorer and VOSviewer. Scientometrics 111 , 1053–1070 (2017).
LeCun, Y., Bengio, Y. & Hinton, G. Deep learning. Nature 521 , 436–444 (2015).
Senior, A. W. et al. Improved protein structure prediction using potentials from deep learning. Nature 577 , 706–710 (2020).
Krenn, M. & Zeilinger, A. Predicting research trends with semantic and neural networks with an application in quantum physics. Proc. Natl Acad. Sci. USA 117 , 1910–1916 (2020).
Iten, R., Metger, T., Wilming, H., Del Rio, L. & Renner, R. Discovering physical concepts with neural networks. Phys. Rev. Lett. 124 , 010508 (2020).
Guimerà, R. et al. A Bayesian machine scientist to aid in the solution of challenging scientific problems. Sci. Adv. 6 , eaav6971 (2020).
Segler, M. H., Preuss, M. & Waller, M. P. Planning chemical syntheses with deep neural networks and symbolic AI. Nature 555 , 604–610 (2018).
Ryu, J. Y., Kim, H. U. & Lee, S. Y. Deep learning improves prediction of drug–drug and drug–food interactions. Proc. Natl Acad. Sci. USA 115 , E4304–E4311 (2018).
Kermany, D. S. et al. Identifying medical diagnoses and treatable diseases by image-based deep learning. Cell 172 , 1122–1131.e9 (2018).
Peng, H., Ke, Q., Budak, C., Romero, D. M. & Ahn, Y.-Y. Neural embeddings of scholarly periodicals reveal complex disciplinary organizations. Sci. Adv. 7 , eabb9004 (2021).
Youyou, W., Yang, Y. & Uzzi, B. A discipline-wide investigation of the replicability of psychology papers over the past two decades. Proc. Natl Acad. Sci. USA 120 , e2208863120 (2023).
Mehrabi, N., Morstatter, F., Saxena, N., Lerman, K. & Galstyan, A. A survey on bias and fairness in machine learning. ACM Computing Surveys (CSUR) 54 , 1–35 (2021).
Way, S. F., Morgan, A. C., Larremore, D. B. & Clauset, A. Productivity, prominence, and the effects of academic environment. Proc. Natl Acad. Sci. USA 116 , 10729–10733 (2019).
Li, W., Aste, T., Caccioli, F. & Livan, G. Early coauthorship with top scientists predicts success in academic careers. Nat. Commun. 10 , 5170 (2019).
Hendry, D. F., Pagan, A. R. & Sargan, J. D. Dynamic specification. Handb. Econ. 2 , 1023–1100 (1984).
Jin, C., Ma, Y. & Uzzi, B. Scientific prizes and the extraordinary growth of scientific topics. Nat. Commun. 12 , 5619 (2021).
Azoulay, P., Ganguli, I. & Zivin, J. G. The mobility of elite life scientists: professional and personal determinants. Res. Policy 46 , 573–590 (2017).
Slavova, K., Fosfuri, A. & De Castro, J. O. Learning by hiring: the effects of scientists’ inbound mobility on research performance in academia. Organ. Sci. 27 , 72–89 (2016).
Sarsons, H. Recognition for group work: gender differences in academia. Am. Econ. Rev. 107 , 141–145 (2017).
Campbell, L. G., Mehtani, S., Dozier, M. E. & Rinehart, J. Gender-heterogeneous working groups produce higher quality science. PLoS ONE 8 , e79147 (2013).
Azoulay, P., Graff Zivin, J. S. & Wang, J. Superstar extinction. Q. J. Econ. 125 , 549–589 (2010).
Furman, J. L. & Stern, S. Climbing atop the shoulders of giants: the impact of institutions on cumulative research. Am. Econ. Rev. 101 , 1933–1963 (2011).
Williams, H. L. Intellectual property rights and innovation: evidence from the human genome. J. Polit. Econ. 121 , 1–27 (2013).
Rubin, A. & Rubin, E. Systematic Bias in the Progress of Research. J. Polit. Econ. 129 , 2666–2719 (2021).
Lu, S. F., Jin, G. Z., Uzzi, B. & Jones, B. The retraction penalty: evidence from the Web of Science. Sci. Rep. 3 , 3146 (2013).
Jin, G. Z., Jones, B., Lu, S. F. & Uzzi, B. The reverse Matthew effect: consequences of retraction in scientific teams. Rev. Econ. Stat. 101 , 492–506 (2019).
Azoulay, P., Bonatti, A. & Krieger, J. L. The career effects of scandal: evidence from scientific retractions. Res. Policy 46 , 1552–1569 (2017).
Goodman-Bacon, A. Difference-in-differences with variation in treatment timing. J. Econ. 225 , 254–277 (2021).
Callaway, B. & Sant’Anna, P. H. Difference-in-differences with multiple time periods. J. Econ. 225 , 200–230 (2021).
Hill, R. Searching for Superstars: Research Risk and Talent Discovery in Astronomy Working Paper (Massachusetts Institute of Technology, 2019).
Bagues, M., Sylos-Labini, M. & Zinovyeva, N. Does the gender composition of scientific committees matter? Am. Econ. Rev. 107 , 1207–1238 (2017).
Sampat, B. & Williams, H. L. How do patents affect follow-on innovation? Evidence from the human genome. Am. Econ. Rev. 109 , 203–236 (2019).
Moretti, E. & Wilson, D. J. The effect of state taxes on the geographical location of top earners: evidence from star scientists. Am. Econ. Rev. 107 , 1858–1903 (2017).
Jacob, B. A. & Lefgren, L. The impact of research grant funding on scientific productivity. J. Public Econ. 95 , 1168–1177 (2011).
Li, D. Expertise versus bias in evaluation: evidence from the NIH. Am. Econ. J. Appl. Econ. 9 , 60–92 (2017).
Pearl, J. Causal diagrams for empirical research. Biometrika 82 , 669–688 (1995).
Pearl, J. & Mackenzie, D. The Book of Why: The New Science of Cause and Effect (Basic Books, 2018).
Traag, V. A. Inferring the causal effect of journals on citations. Quant. Sci. Stud. 2 , 496–504 (2021).
Traag, V. & Waltman, L. Causal foundations of bias, disparity and fairness. Preprint at https://doi.org/10.48550/arXiv.2207.13665 (2022).
Imbens, G. W. Potential outcome and directed acyclic graph approaches to causality: relevance for empirical practice in economics. J. Econ. Lit. 58 , 1129–1179 (2020).
Heckman, J. J. & Pinto, R. Causality and Econometrics (National Bureau of Economic Research, 2022).
Aggarwal, I., Woolley, A. W., Chabris, C. F. & Malone, T. W. The impact of cognitive style diversity on implicit learning in teams. Front. Psychol. 10 , 112 (2019).
Balietti, S., Goldstone, R. L. & Helbing, D. Peer review and competition in the Art Exhibition Game. Proc. Natl Acad. Sci. USA 113 , 8414–8419 (2016).
Paulus, F. M., Rademacher, L., Schäfer, T. A. J., Müller-Pinzler, L. & Krach, S. Journal impact factor shapes scientists’ reward signal in the prospect of publication. PLoS ONE 10 , e0142537 (2015).
Williams, W. M. & Ceci, S. J. National hiring experiments reveal 2:1 faculty preference for women on STEM tenure track. Proc. Natl Acad. Sci. USA 112 , 5360–5365 (2015).
Collaboration, O. S. Estimating the reproducibility of psychological science. Science 349 , aac4716 (2015).
Camerer, C. F. et al. Evaluating replicability of laboratory experiments in economics. Science 351 , 1433–1436 (2016).
Camerer, C. F. et al. Evaluating the replicability of social science experiments in Nature and Science between 2010 and 2015. Nat. Hum. Behav. 2 , 637–644 (2018).
Duflo, E. & Banerjee, A. Handbook of Field Experiments (Elsevier, 2017).
Tomkins, A., Zhang, M. & Heavlin, W. D. Reviewer bias in single versus double-blind peer review. Proc. Natl Acad. Sci. USA 114 , 12708–12713 (2017).
Blank, R. M. The effects of double-blind versus single-blind reviewing: experimental evidence from the American Economic Review. Am. Econ. Rev. 81 , 1041–1067 (1991).
Boudreau, K. J., Guinan, E. C., Lakhani, K. R. & Riedl, C. Looking across and looking beyond the knowledge frontier: intellectual distance, novelty, and resource allocation in science. Manage. Sci. 62 , 2765–2783 (2016).
Lane, J. et al. When Do Experts Listen to Other Experts? The Role of Negative Information in Expert Evaluations for Novel Projects Working Paper #21-007 (Harvard Business School, 2020).
Teplitskiy, M. et al. Do Experts Listen to Other Experts? Field Experimental Evidence from Scientific Peer Review (Harvard Business School, 2019).
Moss-Racusin, C. A., Dovidio, J. F., Brescoll, V. L., Graham, M. J. & Handelsman, J. Science faculty’s subtle gender biases favor male students. Proc. Natl Acad. Sci. USA 109 , 16474–16479 (2012).
Forscher, P. S., Cox, W. T., Brauer, M. & Devine, P. G. Little race or gender bias in an experiment of initial review of NIH R01 grant proposals. Nat. Hum. Behav. 3 , 257–264 (2019).
Dennehy, T. C. & Dasgupta, N. Female peer mentors early in college increase women’s positive academic experiences and retention in engineering. Proc. Natl Acad. Sci. USA 114 , 5964–5969 (2017).
Azoulay, P. Turn the scientific method on ourselves. Nature 484 , 31–32 (2012).
Download references
Acknowledgements
The authors thank all members of the Center for Science of Science and Innovation (CSSI) for invaluable comments. This work was supported by the Air Force Office of Scientific Research under award number FA9550-19-1-0354, National Science Foundation grant SBE 1829344, and the Alfred P. Sloan Foundation G-2019-12485.
Author information
Authors and affiliations.
Center for Science of Science and Innovation, Northwestern University, Evanston, IL, USA
Lu Liu, Benjamin F. Jones, Brian Uzzi & Dashun Wang
Northwestern Institute on Complex Systems, Northwestern University, Evanston, IL, USA
Kellogg School of Management, Northwestern University, Evanston, IL, USA
College of Information Sciences and Technology, Pennsylvania State University, University Park, PA, USA
National Bureau of Economic Research, Cambridge, MA, USA
Benjamin F. Jones
Brookings Institution, Washington, DC, USA
McCormick School of Engineering, Northwestern University, Evanston, IL, USA
- Dashun Wang
You can also search for this author in PubMed Google Scholar
Corresponding author
Correspondence to Dashun Wang .
Ethics declarations
Competing interests.
The authors declare no competing interests.
Peer review
Peer review information.
Nature Human Behaviour thanks Ludo Waltman, Erin Leahey and Sarah Bratt for their contribution to the peer review of this work.
Additional information
Publisher’s note Springer Nature remains neutral with regard to jurisdictional claims in published maps and institutional affiliations.
Rights and permissions
Springer Nature or its licensor (e.g. a society or other partner) holds exclusive rights to this article under a publishing agreement with the author(s) or other rightsholder(s); author self-archiving of the accepted manuscript version of this article is solely governed by the terms of such publishing agreement and applicable law.
Reprints and permissions
About this article
Cite this article.
Liu, L., Jones, B.F., Uzzi, B. et al. Data, measurement and empirical methods in the science of science. Nat Hum Behav 7 , 1046–1058 (2023). https://doi.org/10.1038/s41562-023-01562-4
Download citation
Received : 30 June 2022
Accepted : 17 February 2023
Published : 01 June 2023
Issue Date : July 2023
DOI : https://doi.org/10.1038/s41562-023-01562-4
Share this article
Anyone you share the following link with will be able to read this content:
Sorry, a shareable link is not currently available for this article.
Provided by the Springer Nature SharedIt content-sharing initiative
This article is cited by
Rescaling the disruption index reveals the universality of disruption distributions in science.
- Alex J. Yang
- Hongcun Gong
- Sanhong Deng
Scientometrics (2024)
SciSciNet: A large-scale open data lake for the science of science research
Scientific Data (2023)
Quick links
- Explore articles by subject
- Guide to authors
- Editorial policies
Sign up for the Nature Briefing newsletter — what matters in science, free to your inbox daily.

Quantitative research
Affiliation.
- 1 Faculty of Health and Social Care, University of Hull, Hull, England.
- PMID: 25828021
- DOI: 10.7748/ns.29.31.44.e8681
This article describes the basic tenets of quantitative research. The concepts of dependent and independent variables are addressed and the concept of measurement and its associated issues, such as error, reliability and validity, are explored. Experiments and surveys – the principal research designs in quantitative research – are described and key features explained. The importance of the double-blind randomised controlled trial is emphasised, alongside the importance of longitudinal surveys, as opposed to cross-sectional surveys. Essential features of data storage are covered, with an emphasis on safe, anonymous storage. Finally, the article explores the analysis of quantitative data, considering what may be analysed and the main uses of statistics in analysis.
Keywords: Experiments; measurement; nursing research; quantitative research; reliability; surveys; validity.
- Biomedical Research / methods*
- Double-Blind Method
- Evaluation Studies as Topic
- Longitudinal Studies
- Randomized Controlled Trials as Topic
- United Kingdom

An official website of the United States government
The .gov means it’s official. Federal government websites often end in .gov or .mil. Before sharing sensitive information, make sure you’re on a federal government site.
The site is secure. The https:// ensures that you are connecting to the official website and that any information you provide is encrypted and transmitted securely.
- Publications
- Account settings
Preview improvements coming to the PMC website in October 2024. Learn More or Try it out now .
- Advanced Search
- Journal List
- Can J Infect Dis
- v.8(2); Mar-Apr 1997
Broadening horizons: Integrating quantitative and qualitative research
Health research usually employs quantitative, often experimental, methods to study clinical conditions and outcomes. The use of qualitative methods in this type of research is much less common. However, we contend that qualitative research, in combination with quantitative research, can play an important role generating an improved understanding of disease, health and health care.
Data collected in qualitative research are usually in narrative rather than numerical form, such as the transcript of an unstructured, in-depth interview. Analysis of qualitative data organizes, summarizes and interprets these nonnumerical observations. The goal of qualitative research is the development of concepts that help clarify phenomena in natural, rather than experimental, settings, giving due emphasis to the meanings, experiences and views of all the participants being studied. For example, to understand why some members of ethnic minorities have refused tuberculosis treatment, qualitative, culturally sensitive interviews may be much more informative than standardized quantitative interviews.
Both quantitative and qualitative research have weaknesses that to some degree are compensated for by the strengths of the other. Quantitative research is very well suited to establishing cause-and-effect relationships, to testing hypotheses and to determining the opinions, attitudes and practices of a large population, whereas qualitative research lends itself very well to developing hypotheses and theories and to describing processes such as decision making or communication processes. Quantitative research generates factual, reliable outcome data that are usually generalizable to some larger populations, and qualitative research produces rich, detailed and valid process data based on the participant’s, rather than the investigator’s, perspectives and interpretations ( 1 ).
Quantitative research is usually deductive, relying on experimental and survey methods to test specific hypotheses based on general principles. It is strong in inductive reasoning, building and expanding theories concerning relationships among phenomena. In the actual practice of scientific research, theory and research interact through a never-ending cycle of deduction, induction, deduction, induction and so forth ( 2 ). By combining quantitative and qualitative methods, a degree of comprehensiveness may be achieved that neither approach, if used alone, can achieve. For example, to target populations of children who are not being immunized for common childhood infectious diseases, it is critical to quantify the existence of a low rate of immunization. However, to intervene to rectify the identified problem, it is important to explore why parents are not having their children vaccinated. Qualitative interviews are most appropriate for this purpose.
The nature of inquiry is similar in both quantitative and qualitative research, it is couched in the human desire to understand and explain behaviour and events, their components, antecedents, corollaries and consequences. If differences among researchers exist, it is not because they aspire to different ends, but because they have operationalized their methods for reaching those ends differently ( 3 ).
Even though both approaches are different from one another, one is not necessarily inferior to the other. However, qualitative research is often considered to be lacking scientific rigour. Unfortunately, the standard strategies used to enhance validity, reliability and objectivity in quantitative research are not always relevant to qualitative research. Fortunately, more attention is being paid to strategies to enhance the quality of the data and interpretations collected through qualitative research ( 4 , 5 ). An example of a commonly used approach is triangulation, which refers to data collection in which evidence is deliberately sought from a wide range of different, independent sources and often by different means (for instance, comparing oral testimony with written records) ( 4 ).
The rigid demarcation between the two types of research has not encouraged interaction between the two camps. However, there are some good reasons for combining qualitative and quantitative research. First, a researcher may wish to explore an issue to understand what the relevant variables are or to develop hypotheses that can then be studied or tested in quantitative research. This way of combining the two approaches is also used in the development of scales or questionnaires. For example, qualitative techniques, such as observation, in-depth interviews or focus groups, can provide a description and understanding of a situation or behaviour. At their most basic, these techniques can be used simply to discover the most comprehensive terms or words to use in a subsequent survey questionnaire ( 6 ). Second, qualitative research also may follow quantitative research with the aim of explaining the quantitative results. For example, designing and evaluating an effective health campaign promoting influenza vaccinations faces multimethod challenges. To determine whether the campaign works so that the strategy can be effectively used again, it is not only important to identify how many people received shots, but also why and how they decided to get vaccinated (ie, linking process to outcome). Third, qualitative and quantitative research can be combined to enhance the validity of the results, much the same as in triangulation, but now using both quantitative and qualitative approaches, for their combined strength, rather than using one method to validate the result of the other. For example, overall validity would be enhanced through the use of a multimethods approach in instances where measuring the technical accuracy of a diagnostic or treatment intervention was important alongside an understanding of patient response to or acceptance of such a diagnostic test or treatment protocol ( 7 ).
Several barriers may prevent the further development of integrated research, such as lack of expertise, time and funding, mutual prejudices, and publication bias. If methodological integration is going to progress, a number of changes in the current environment are needed. These include acceptance and refinement of the underlying paradigms of qualitative and quantitative research, recognition by funding agencies of the need for both perspectives and a willingness to allocate sufficient resources, awareness by editorial boards of the importance of publishing multimethods research, training of researchers in both paradigms, encouragement of teamwork and promotion of mutual acceptance and respect by the adherents of each approach.
Given the multiple challenges facing health researchers today, broadening the horizons of health research to embrace the use and benefits of both quantitative and qualitative analysis is a methodological advance that must be supported and nurtured.
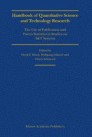
- © 2005
Handbook of Quantitative Science and Technology Research
The Use of Publication and Patent Statistics in Studies of S&T Systems
- Henk F. Moed 0 ,
- Wolfgang Glänzel 1 ,
- Ulrich Schmoch 2
Centre for Science and Technology Studies, University of Leiden, The Netherlands
You can also search for this editor in PubMed Google Scholar
Steunpunt O&O Statistieken, K.U. Leuven, Belgium
Fraunhofer Institute for Systems and Innovation Research, Karlsruhe, Germany
- Focuses on the development and application of indicators derived from data on scientific publications and patents
148k Accesses
786 Citations
45 Altmetric
- Table of contents
About this book
Editors and affiliations, bibliographic information.
- Publish with us
Buying options
- Available as PDF
- Read on any device
- Instant download
- Own it forever
- Compact, lightweight edition
- Dispatched in 3 to 5 business days
- Free shipping worldwide - see info
- Durable hardcover edition
Tax calculation will be finalised at checkout
Other ways to access
This is a preview of subscription content, log in via an institution to check for access.
Table of contents (35 chapters)
Front matter, editors’ introduction.
- Henk F. Moed, Wolfgang Glänzel, Ulrich Schmoch
Disciplinary Approaches
Measuring science.
- Anthony F.J. van Raan
Econometric Approaches to the Analysis of Productivity of R&D Systems
- Andrea Bonaccorsi, Cinzia Daraio
Indicators for National Science and Technology Policy
- Hariolf Grupp, Mary Ellen Mogee
Keeping the Gates of Science Journals
- Tibor Braun
S&T Indicators for Policy Making in a Changing Science-Society Relationship
Paradigms and trajectories of technological opportunities 1890–1990.
- Birgitte Andersen
Science on the Periphery: Bridging the Information Divide
- Subbiah Arunachalam
General Methodology
Data mining and text mining for science & technology research.
- Edda Leopold, Michael May, Gerhard Paaß
Opening the Black Box
- Sybille Hinze, Ulrich Schmoch
Science Maps Within a Science Policy Context
- C. M. Noyons
Analysing Scientific Networks Through Co-Authorship
- Wolfgang Glänzel, András Schubert
Patent Citations and the Economic Value of Patents
- Bhaven N. Sampat, Arvids A. Ziedonis
Scientific and Technological Performance by Gender
- Fulvio Naldi, Daniela Luzi, Adriana Valente, Ilaria Vannini Parenti
The Use of Input Data in the Performance Analysis of R&D Systems
Methodological issues of webometric studies.
- Peter Ingwersen, Lennart Björneborn
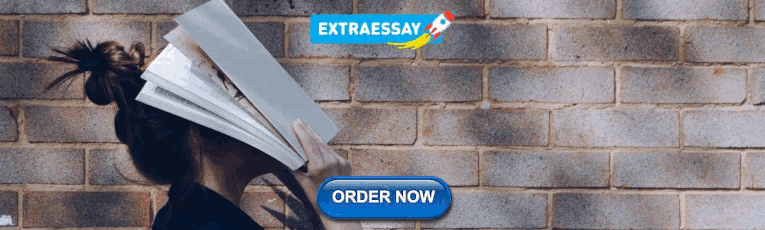
The Science System
Descriptive versus evaluative bibliometrics.
- Thed van Leeuwen
What Happens When Funding is Linked to Publication Counts?
- Linda Butler
Internationalisation in Science in the Prism of Bibliometric Indicators
- Michel Zitt, Elise Bassecoulard
- Forschung & Entwicklung
- R programming language
- berckelaercompscience
- data mining
- performance
- science and technology
- technology policy
Henk F. Moed
Steunpunt O&O Statistieken, K.U. Leuven, Belgium
Wolfgang Glänzel
Ulrich Schmoch
Book Title : Handbook of Quantitative Science and Technology Research
Book Subtitle : The Use of Publication and Patent Statistics in Studies of S&T Systems
Editors : Henk F. Moed, Wolfgang Glänzel, Ulrich Schmoch
DOI : https://doi.org/10.1007/1-4020-2755-9
Publisher : Springer Dordrecht
eBook Packages : Humanities, Social Sciences and Law , History (R0)
Copyright Information : Springer Science+Business Media B.V. 2005
Hardcover ISBN : 978-1-4020-2702-4 Published: 10 September 2004
Softcover ISBN : 978-90-481-6709-8 Published: 27 January 2011
eBook ISBN : 978-1-4020-2755-0 Published: 23 February 2006
Edition Number : 1
Number of Pages : X, 800
Topics : Science, Humanities and Social Sciences, multidisciplinary , Information Storage and Retrieval , Economic Growth , R & D/Technology Policy , Social Policy , Innovation/Technology Management
Policies and ethics
- Find a journal
- Track your research
- Privacy Policy
Buy Me a Coffee

Home » Quantitative Research – Methods, Types and Analysis
Quantitative Research – Methods, Types and Analysis
Table of Contents

Quantitative Research
Quantitative research is a type of research that collects and analyzes numerical data to test hypotheses and answer research questions . This research typically involves a large sample size and uses statistical analysis to make inferences about a population based on the data collected. It often involves the use of surveys, experiments, or other structured data collection methods to gather quantitative data.
Quantitative Research Methods

Quantitative Research Methods are as follows:
Descriptive Research Design
Descriptive research design is used to describe the characteristics of a population or phenomenon being studied. This research method is used to answer the questions of what, where, when, and how. Descriptive research designs use a variety of methods such as observation, case studies, and surveys to collect data. The data is then analyzed using statistical tools to identify patterns and relationships.
Correlational Research Design
Correlational research design is used to investigate the relationship between two or more variables. Researchers use correlational research to determine whether a relationship exists between variables and to what extent they are related. This research method involves collecting data from a sample and analyzing it using statistical tools such as correlation coefficients.
Quasi-experimental Research Design
Quasi-experimental research design is used to investigate cause-and-effect relationships between variables. This research method is similar to experimental research design, but it lacks full control over the independent variable. Researchers use quasi-experimental research designs when it is not feasible or ethical to manipulate the independent variable.
Experimental Research Design
Experimental research design is used to investigate cause-and-effect relationships between variables. This research method involves manipulating the independent variable and observing the effects on the dependent variable. Researchers use experimental research designs to test hypotheses and establish cause-and-effect relationships.
Survey Research
Survey research involves collecting data from a sample of individuals using a standardized questionnaire. This research method is used to gather information on attitudes, beliefs, and behaviors of individuals. Researchers use survey research to collect data quickly and efficiently from a large sample size. Survey research can be conducted through various methods such as online, phone, mail, or in-person interviews.
Quantitative Research Analysis Methods
Here are some commonly used quantitative research analysis methods:
Statistical Analysis
Statistical analysis is the most common quantitative research analysis method. It involves using statistical tools and techniques to analyze the numerical data collected during the research process. Statistical analysis can be used to identify patterns, trends, and relationships between variables, and to test hypotheses and theories.
Regression Analysis
Regression analysis is a statistical technique used to analyze the relationship between one dependent variable and one or more independent variables. Researchers use regression analysis to identify and quantify the impact of independent variables on the dependent variable.
Factor Analysis
Factor analysis is a statistical technique used to identify underlying factors that explain the correlations among a set of variables. Researchers use factor analysis to reduce a large number of variables to a smaller set of factors that capture the most important information.
Structural Equation Modeling
Structural equation modeling is a statistical technique used to test complex relationships between variables. It involves specifying a model that includes both observed and unobserved variables, and then using statistical methods to test the fit of the model to the data.
Time Series Analysis
Time series analysis is a statistical technique used to analyze data that is collected over time. It involves identifying patterns and trends in the data, as well as any seasonal or cyclical variations.
Multilevel Modeling
Multilevel modeling is a statistical technique used to analyze data that is nested within multiple levels. For example, researchers might use multilevel modeling to analyze data that is collected from individuals who are nested within groups, such as students nested within schools.
Applications of Quantitative Research
Quantitative research has many applications across a wide range of fields. Here are some common examples:
- Market Research : Quantitative research is used extensively in market research to understand consumer behavior, preferences, and trends. Researchers use surveys, experiments, and other quantitative methods to collect data that can inform marketing strategies, product development, and pricing decisions.
- Health Research: Quantitative research is used in health research to study the effectiveness of medical treatments, identify risk factors for diseases, and track health outcomes over time. Researchers use statistical methods to analyze data from clinical trials, surveys, and other sources to inform medical practice and policy.
- Social Science Research: Quantitative research is used in social science research to study human behavior, attitudes, and social structures. Researchers use surveys, experiments, and other quantitative methods to collect data that can inform social policies, educational programs, and community interventions.
- Education Research: Quantitative research is used in education research to study the effectiveness of teaching methods, assess student learning outcomes, and identify factors that influence student success. Researchers use experimental and quasi-experimental designs, as well as surveys and other quantitative methods, to collect and analyze data.
- Environmental Research: Quantitative research is used in environmental research to study the impact of human activities on the environment, assess the effectiveness of conservation strategies, and identify ways to reduce environmental risks. Researchers use statistical methods to analyze data from field studies, experiments, and other sources.
Characteristics of Quantitative Research
Here are some key characteristics of quantitative research:
- Numerical data : Quantitative research involves collecting numerical data through standardized methods such as surveys, experiments, and observational studies. This data is analyzed using statistical methods to identify patterns and relationships.
- Large sample size: Quantitative research often involves collecting data from a large sample of individuals or groups in order to increase the reliability and generalizability of the findings.
- Objective approach: Quantitative research aims to be objective and impartial in its approach, focusing on the collection and analysis of data rather than personal beliefs, opinions, or experiences.
- Control over variables: Quantitative research often involves manipulating variables to test hypotheses and establish cause-and-effect relationships. Researchers aim to control for extraneous variables that may impact the results.
- Replicable : Quantitative research aims to be replicable, meaning that other researchers should be able to conduct similar studies and obtain similar results using the same methods.
- Statistical analysis: Quantitative research involves using statistical tools and techniques to analyze the numerical data collected during the research process. Statistical analysis allows researchers to identify patterns, trends, and relationships between variables, and to test hypotheses and theories.
- Generalizability: Quantitative research aims to produce findings that can be generalized to larger populations beyond the specific sample studied. This is achieved through the use of random sampling methods and statistical inference.
Examples of Quantitative Research
Here are some examples of quantitative research in different fields:
- Market Research: A company conducts a survey of 1000 consumers to determine their brand awareness and preferences. The data is analyzed using statistical methods to identify trends and patterns that can inform marketing strategies.
- Health Research : A researcher conducts a randomized controlled trial to test the effectiveness of a new drug for treating a particular medical condition. The study involves collecting data from a large sample of patients and analyzing the results using statistical methods.
- Social Science Research : A sociologist conducts a survey of 500 people to study attitudes toward immigration in a particular country. The data is analyzed using statistical methods to identify factors that influence these attitudes.
- Education Research: A researcher conducts an experiment to compare the effectiveness of two different teaching methods for improving student learning outcomes. The study involves randomly assigning students to different groups and collecting data on their performance on standardized tests.
- Environmental Research : A team of researchers conduct a study to investigate the impact of climate change on the distribution and abundance of a particular species of plant or animal. The study involves collecting data on environmental factors and population sizes over time and analyzing the results using statistical methods.
- Psychology : A researcher conducts a survey of 500 college students to investigate the relationship between social media use and mental health. The data is analyzed using statistical methods to identify correlations and potential causal relationships.
- Political Science: A team of researchers conducts a study to investigate voter behavior during an election. They use survey methods to collect data on voting patterns, demographics, and political attitudes, and analyze the results using statistical methods.
How to Conduct Quantitative Research
Here is a general overview of how to conduct quantitative research:
- Develop a research question: The first step in conducting quantitative research is to develop a clear and specific research question. This question should be based on a gap in existing knowledge, and should be answerable using quantitative methods.
- Develop a research design: Once you have a research question, you will need to develop a research design. This involves deciding on the appropriate methods to collect data, such as surveys, experiments, or observational studies. You will also need to determine the appropriate sample size, data collection instruments, and data analysis techniques.
- Collect data: The next step is to collect data. This may involve administering surveys or questionnaires, conducting experiments, or gathering data from existing sources. It is important to use standardized methods to ensure that the data is reliable and valid.
- Analyze data : Once the data has been collected, it is time to analyze it. This involves using statistical methods to identify patterns, trends, and relationships between variables. Common statistical techniques include correlation analysis, regression analysis, and hypothesis testing.
- Interpret results: After analyzing the data, you will need to interpret the results. This involves identifying the key findings, determining their significance, and drawing conclusions based on the data.
- Communicate findings: Finally, you will need to communicate your findings. This may involve writing a research report, presenting at a conference, or publishing in a peer-reviewed journal. It is important to clearly communicate the research question, methods, results, and conclusions to ensure that others can understand and replicate your research.
When to use Quantitative Research
Here are some situations when quantitative research can be appropriate:
- To test a hypothesis: Quantitative research is often used to test a hypothesis or a theory. It involves collecting numerical data and using statistical analysis to determine if the data supports or refutes the hypothesis.
- To generalize findings: If you want to generalize the findings of your study to a larger population, quantitative research can be useful. This is because it allows you to collect numerical data from a representative sample of the population and use statistical analysis to make inferences about the population as a whole.
- To measure relationships between variables: If you want to measure the relationship between two or more variables, such as the relationship between age and income, or between education level and job satisfaction, quantitative research can be useful. It allows you to collect numerical data on both variables and use statistical analysis to determine the strength and direction of the relationship.
- To identify patterns or trends: Quantitative research can be useful for identifying patterns or trends in data. For example, you can use quantitative research to identify trends in consumer behavior or to identify patterns in stock market data.
- To quantify attitudes or opinions : If you want to measure attitudes or opinions on a particular topic, quantitative research can be useful. It allows you to collect numerical data using surveys or questionnaires and analyze the data using statistical methods to determine the prevalence of certain attitudes or opinions.
Purpose of Quantitative Research
The purpose of quantitative research is to systematically investigate and measure the relationships between variables or phenomena using numerical data and statistical analysis. The main objectives of quantitative research include:
- Description : To provide a detailed and accurate description of a particular phenomenon or population.
- Explanation : To explain the reasons for the occurrence of a particular phenomenon, such as identifying the factors that influence a behavior or attitude.
- Prediction : To predict future trends or behaviors based on past patterns and relationships between variables.
- Control : To identify the best strategies for controlling or influencing a particular outcome or behavior.
Quantitative research is used in many different fields, including social sciences, business, engineering, and health sciences. It can be used to investigate a wide range of phenomena, from human behavior and attitudes to physical and biological processes. The purpose of quantitative research is to provide reliable and valid data that can be used to inform decision-making and improve understanding of the world around us.
Advantages of Quantitative Research
There are several advantages of quantitative research, including:
- Objectivity : Quantitative research is based on objective data and statistical analysis, which reduces the potential for bias or subjectivity in the research process.
- Reproducibility : Because quantitative research involves standardized methods and measurements, it is more likely to be reproducible and reliable.
- Generalizability : Quantitative research allows for generalizations to be made about a population based on a representative sample, which can inform decision-making and policy development.
- Precision : Quantitative research allows for precise measurement and analysis of data, which can provide a more accurate understanding of phenomena and relationships between variables.
- Efficiency : Quantitative research can be conducted relatively quickly and efficiently, especially when compared to qualitative research, which may involve lengthy data collection and analysis.
- Large sample sizes : Quantitative research can accommodate large sample sizes, which can increase the representativeness and generalizability of the results.
Limitations of Quantitative Research
There are several limitations of quantitative research, including:
- Limited understanding of context: Quantitative research typically focuses on numerical data and statistical analysis, which may not provide a comprehensive understanding of the context or underlying factors that influence a phenomenon.
- Simplification of complex phenomena: Quantitative research often involves simplifying complex phenomena into measurable variables, which may not capture the full complexity of the phenomenon being studied.
- Potential for researcher bias: Although quantitative research aims to be objective, there is still the potential for researcher bias in areas such as sampling, data collection, and data analysis.
- Limited ability to explore new ideas: Quantitative research is often based on pre-determined research questions and hypotheses, which may limit the ability to explore new ideas or unexpected findings.
- Limited ability to capture subjective experiences : Quantitative research is typically focused on objective data and may not capture the subjective experiences of individuals or groups being studied.
- Ethical concerns : Quantitative research may raise ethical concerns, such as invasion of privacy or the potential for harm to participants.
About the author
Muhammad Hassan
Researcher, Academic Writer, Web developer
You may also like

Questionnaire – Definition, Types, and Examples

Case Study – Methods, Examples and Guide

Observational Research – Methods and Guide

Qualitative Research Methods

Explanatory Research – Types, Methods, Guide

Survey Research – Types, Methods, Examples
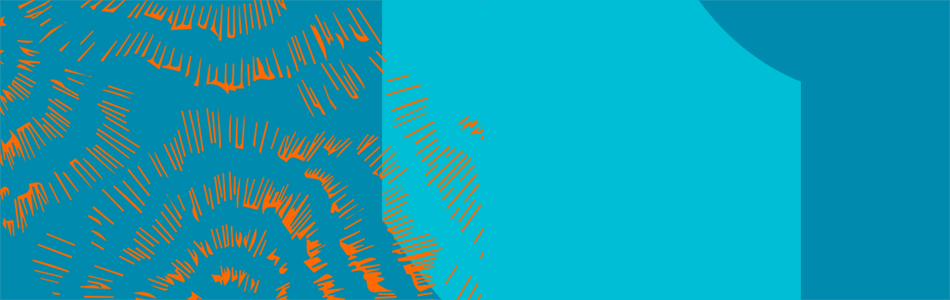
What is social science?
Quantitative research.
Quantitative research can measure and describe whole societies, or institutions, organisations or groups of individuals that are part of them. The strength of quantitative methods is that they can provide vital information about a society or community, through surveys, examinations, records or censuses, that no individual could obtain by observation.
Quantitative methodologies
Some of the most common quantitative research methodologies are described here. These methodologies are widely used in research funded by the Economic and Social Research Council (ESRC).
Cross-sectional studies
Cross-sectional studies are surveys undertaken at one point in time, rather like a photo taken by a camera. If the same or similar survey is repeated, we can get good measures of how society is changing.
Longitudinal studies
Longitudinal studies follow the same respondents over an extended period of time. They can employ both qualitative and quantitative research methods, and they follow the same group of people over time. For example, the Millennium Cohort Study has been keeping track of more than 11,000 children born between 2000 and 2002. Some of its many findings have shown how childhood factors such as poverty and birth weight can affect health and success at school as children get older.
Opinion polls
An opinion poll is a form of survey designed to measure the opinions of a target population about an issue, such as support for political parties and views about crime and justice, the economy or the environment.
Questionnaires
Questionnaires collect data in a standardised way, so that useful summaries can be made about large groups of respondents, such as the proportion of all young people of a given age who are bullied. Usually most questions are ‘closed response’, where respondents are given a range of options to choose from. Researchers have to be careful that the questions are not ‘leading’, that the options are comprehensive (they cover every possible answer) and are mutually exclusive, so that only one answer is correct for any respondent.
Social attitude surveys
Social attitude surveys ask more general questions about beliefs and behaviour, for example, how often people go to church, how much trust they have in the police force, whether they think children need a strict upbringing, how content they are with their life, how often they see other family members, and whether they are in employment.
Surveys and censuses
A census is a survey of everyone in the population. Because of the vast number of respondents, they are very expensive to organise. Governments now depend much more on sample surveys and administrative records, for example those created by a stay in hospital or tax returns. Surveys use a questionnaire to investigate respondents in a sample. Samples are chosen in such a way that they can represent a much larger population. A precise calculation can be made of how accurate the information from any sample is likely to be.
Further information
See our social science for schools resource page on the UK Government Web Archive for more information on social science methodologies.
Last updated: 31 March 2022
This is the website for UKRI: our seven research councils, Research England and Innovate UK. Let us know if you have feedback or would like to help improve our online products and services .

- Current Students
- Faculty / Staff
- Paying for College
- Alumni Services
- Partnerships
- Program Finder
- Affordable, Flexible, Accessible
- Distance Education
- All Online Courses & Degrees
- Baccalaureate Online
- Graduate Online
- Start Dates
- Admissions, Costs & Aid
- Faculty and Contacts
- Academic and Career Support
- Student Testimonials
- Distance Education Advantage
- About Hybrid Learning
- Hybrid Learning Degrees
- Admissions Requirements
- Top Destination Courses
- Student Life
- Academic Support
- Academic Calendar
- Faculty & Contacts
- Technical Institute for Environmental Professions
- Term Calendar
- Sustainable Ventures
- Careers & Outcomes
- About Unity
- Office of the President
- Announcing Our Evolution
- Sustainable Achievements & Initiatives
- Reinventing College
- Extended Reality (XR)
- Commencement
- Give to Unity Environmental University
- Institutional Communications
- Unity Environmental University News
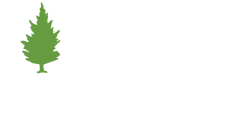
The Importance of Quantitative Methods in Environmental Science and Sustainability Measurement
March 23, 2017
Home / News / The Importance of Quantitative Methods in Environmental Science and Sustainability Measurement
Environmental science is a continuously evolving academic field that seeks to help us gain a progressively better understanding of our natural world and develop effective solutions for important sustainability issues. Challenging the status quo to address environmental problems requires solid evidence to persuade decision makers of the necessity of change. This makes quantitative literacy essential for sustainability professionals to interpret scientific data and implement management procedures.
With our world facing increasingly complex environmental issues, quantitative techniques reduce the numerous uncertainties by providing a reliable representation of reality, enabling us to proceed toward potential solutions with greater confidence. A wide range of statistical tools and approaches are now available for sustainability scientists to measure environmental indicators and inform responsible policy-making.
How Quantitative Methods Provide Context for Environmental Science and Sustainability
Environmental science brings a transdisciplinary systems approach to analyzing sustainability concerns. As the intrinsic concept of sustainability can be interpreted according to diverse values and definitions, quantitative methods based on rigorous scientific research are crucial for establishing an evidence-based consensus on pertinent issues that provide a foundation for meaningful policy implementation.
Statistical evidence is often necessary to defend conservation conclusions
Descriptive and inferential statistical evidence provides a strong foundation for defending conclusions to various audiences. Applying an appropriate range of data sources and quantitative models can produce logical inferences to estimate the probability of future results while quantifying the extent of uncertainty, limits, and future research needs. Given the urgency of environmental issues like climate change and the prevalence of skeptics, effectively summarizing and communicating irrefutable results of complex statistical analyses can make the difference in developing successful courses of action.
How an M.S. in Sustainability Integrates Quantitative Methods
In an M.S. in Sustainability , such as natural resource management, students acquire the fundamental quantitative literacy to correctly evaluate and interpret ecological literature. They learn how to design effective studies, integrate quantitative models, and apply advanced statistical approaches.
For example, Bayesian methods are used to enable scientists to systematically factor in various forms of prior evidence while observing how conclusions change with the new information. This allows a quicker reaction to emerging conditions. Bayesian statistical inference has successfully been applied in conservation biology, addressing many of the problems inherent in standard hypothesis testing while including important factors causing uncertainty. It provides an alternate framework for decision-making that permits more options and better conclusions.
Statistical Models Mitigate Environmental Science and Sustainability Uncertainty
The principles of statistics and probability, multivariate analysis, and spatial analysis methods provide a common ground for scientists, engineers, and other environmental professionals to communicate with each other. Despite the sophistication of the latest mathematical models, the enormous complexity of interactions between environmental systems introduces some level of uncertainty into all predictions.
The quantitative methods acquired in a Sustainability Master’s online combine information from various sources to create more informed predictions, while importantly providing the scientific reasoning to accurately describe what is known and what is not. This quantification of uncertainty makes it impossible to dismiss climate and conservation models, therefore providing a clearer impetus for change.
Interested in pursuing a rewarding career in environmental science and sustainability ?
Contact Unity College to learn about our online Master’s Professional Science programs.
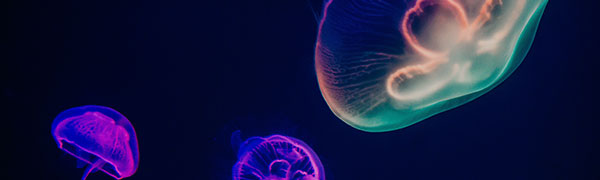
Start Your Journey
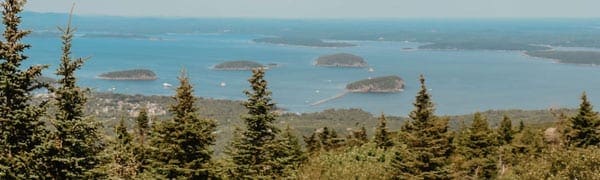
Looking for Answers
Get More Info
© Unity Environmental University 2024. “America’s Environmental University.™”
Privacy Overview
Navigation and service

Quantitative research on the science sector
The science sector has been acquiring great significance for more and more areas of our society. This makes it all the more important for the science system to be structured and funded as advantageously as possible. To an increasing degree, quality and productivity issues in science and research are being studied with the help of indicators that yield information about quality, the factors that define it and the factors that bring it about. One aim of the funding line "Quantitative research on the science sector" is to make even better use of such indicators' usefulness for research on the science system – and, in the process, to develop new indicators where appropriate. Quantitative research in this context is designed to improve our understanding of the science system's workings, structural dependencies and dynamics. It also considers the impacts of data-based evaluation of research.
More content
Finished projects.
Breaking, breaking or breaking? Factors influencing scientific careers in the natural and social sciences Otto Friedrich University of Bamberg; Bergische University of Wuppertal; University of Stuttgart
Quantification of research efficiency in the German higher education sector Technical University Braunschweig; Research Centre Jülich
A quantitative study of the efficiency, dynamics and structures of the German life sciences Technical University of Munich (TUM); Hamburg Institute for Social Research (HIS)
Communication patterns in the humanities and social sciences Technical University of Berlin
Research rankings, output measurement, recruitment of young researchers, choice of topics and incentives for care University of Kassel; Technical University of Dresden
IMPRO – An integrative approach, combining bibliometrics and text mining, for completely automatic content analysis of citation contexts RWTH Aachen University
Interdisciplinarity of science institutions, structures and effects Fraunhofer Institute for Systems and Innovation Research (ISI); Max Planck Society for the Advancement of Science (MPG) - Max Planck Digital Library (MPDL)
LEISTUNGSMESSUNG
Capturing scientific productivity, its preconditions and consequences Philipps University Marburg
Publications, and the efficient use of resources, in university knowledge production Dresden University of Technology
Motives to publish – structures, strategies and motives of/for scientific publication by companies Centre for European Economic Research (ZEW), Mannheim; Fraunhofer-Society for the Promotion of Applied Research registered association
Quantitative analysis of the dynamics of the scientific, economic and societal impacts of research activities and networks ZBW – Leibniz Information Centre for Economics; Kiel University; ZB MED – Information Centre for Life Sciences
Quantitative analysis of publication bias, impact factors and project tools University of Konstanz; LMU Munich
A mapping of the origin and success of cooperation relationships in regional research networks and innovation clusters L3S Research Center/Kassel; University of Kassel/INCHER; Julius-Maximilians-Universität Würzburg; HU Berlin
Reference implementation for open scientometric indicators German National Library of Science and Technology (TIB)
Sectoral and institutional nowcasts of bibliometric reception indicators German Centre for Higher Education and Science Research GmbH
Registration, Adherence and Data Availability of Clinical Trials in Germany - Temporal Trends and Structural Influencing Factors University of Applied Sciences Bielefeld
Bibliometric micro-macro links for science research Berlin University of Technology
In which journals shall we trust? – An analysis of the interrelationship between the impact factors of medical journals and the correctness of the study findings published in the journals University of Lübeck
Quality measures for the evaluation of medical research German Centre for Higher Education and Scientific Research GmbH; Georg-August-Universität Göttingen; German National Library of Medicine (ZB MED); ZBW - Leibniz Information Centre for Economics
Function, reception and performativity of review literature in science, in the context of the bibliometrics of driven incentive structures German Centre for Higher Education Research and Science Studies (DZHW), Berlin
"Open Access Effects" - Structural and author-specific factors influencing the impact of OA publications in various disciplines GESIS - Leibniz Institute for the Social Sciences; ZBW – Leibniz Information Centre for Economics
Open-access publication at universities in Germany: Development and influencing factors Göttingen State and University Library; University of Bielefeld
Social Sciences Research: Qualitative vs. Quantitative Research
- What is Social Science?
Qualitative vs. Quantitative Research
- Scholarly vs. Popular Sources This link opens in a new window
- Evaluating Sources This link opens in a new window
- Social Research Organizations
- Anatomy of an APA Article
- Social Sciences Databases
- Sociology This link opens in a new window
Focus Groups

Social science research, or social research as it is sometimes called, stems from the natural sciences, and similar to its precursory field, it uses empirical, measurable outcomes to arrive at a conclusion. While natural scientists use the scientific method, social scientists often use quantitative research to go about their method of discovery.
Quantitative research "is the systematic examination of social phenomena, using statistical models and mathematical theories to develop, accumulate, and refine the scientific knowledge base" (" Quantitative Research," 2008 ). Quantitative research also provides "generalizable" findings, and according to Marlow (1993), is "characterized by hypothesis testing, using large samples, standardized measures, a deductive approach, and rigorously structured data collection instruments" (cited in "Quantitative Research").
As an alternative to quantitative research, qualitative research is also employed in social science research and is contrasted with quantitative research as such:
- Insider rather than outsider
- Person-centered rather than variable-centered
- Holistic rather than particularistic
- Depth rather than breadth
(" Qualitative Research, " 2008)
Trochim (2006), however, warns that researchers should not become so caught up in the polarizing differences between qualitative and quantiative research. He writes, "All quantitative data is based upon qualitative judgments; and all qualitative data can be described and manipulated numerically" (para. 3).

- << Previous: What is Social Science?
- Next: Scholarly vs. Popular Sources >>
- Last Updated: Jan 2, 2024 11:55 AM
- URL: https://spcollege.libguides.com/ssresearch
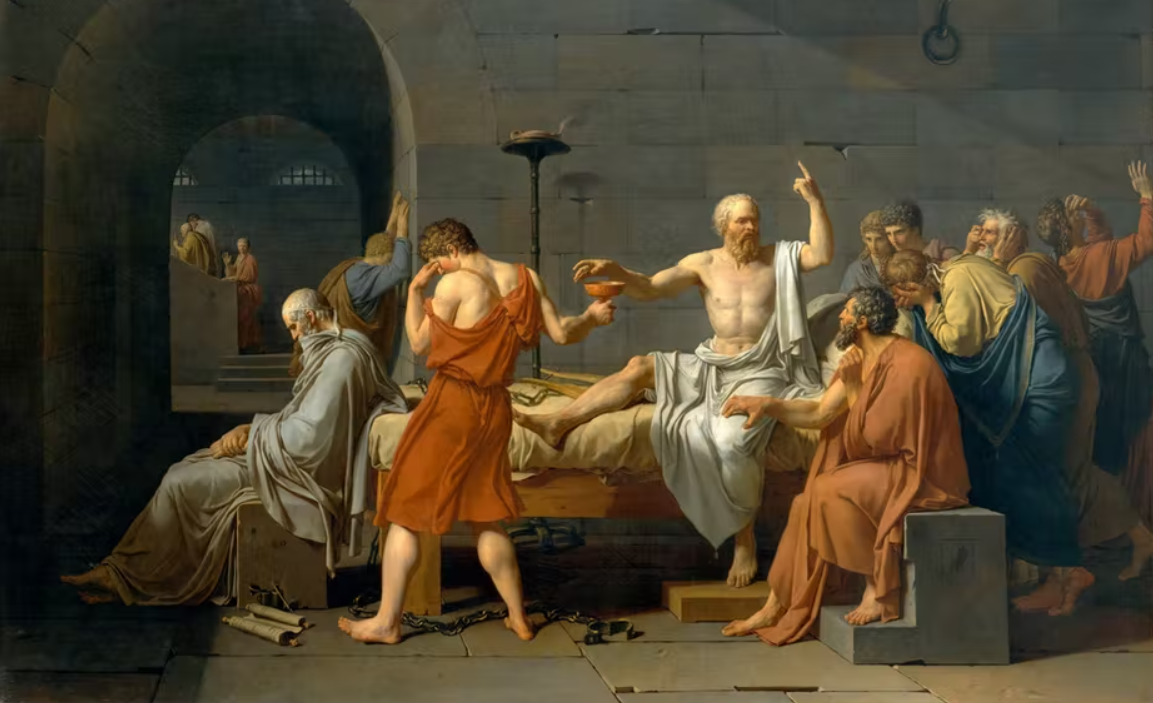
PHILO-notes
Free Online Learning Materials
Importance of Quantitative Research Across Fields
First of all, research is necessary and valuable in society because, among other things, 1) it is an important tool for building knowledge and facilitating learning; 2) it serves as a means in understanding social and political issues and in increasing public awareness; 3) it helps people succeed in business; 4) it enables us to disprove lies and support truths; and 5) it serves as a means to find, gauge, and seize opportunities, as well as helps in finding solutions to social and health problems (in fact, the discovery of COVID-19 vaccines is a product of research).
Now, quantitative research, as a type of research that explains phenomena according to numerical data which are analyzed by means of mathematically based methods, especially statistics, is very important because it relies on hard facts and numerical data to gain as objective a picture of people’s opinion as possible or an objective understanding of reality. Hence, quantitative research enables us to map out and understand the world in which we live.
In addition, quantitative research is important because it enables us to conduct research on a large scale; it can reveal insights about broader groups of people or the population as a whole; it enables researchers to compare different groups to understand similarities and differences; and it helps businesses understand the size of a new opportunity. As we can see, quantitative research is important across fields and disciplines.
Let me now briefly discuss the importance of quantitative research across fields and disciplines. But for brevity’ sake, the discussion that follows will only focus on the importance of quantitative research in psychology, economics, education, environmental science and sustainability, and business.
First, on the importance of quantitative research in psychology .
We know for a fact that one of the major goals of psychology is to understand all the elements that propel human (as well as animal) behavior. Here, one of the most frequent tasks of psychologists is to represent a series of observations or measurements by a concise and suitable formula. Such a formula may either express a physical hypothesis, or on the other hand be merely empirical, that is, it may enable researchers in the field of psychology to represent by a few well selected constants a wide range of experimental or observational data. In the latter case it serves not only for purposes of interpolation, but frequently suggests new physical concepts or statistical constants. Indeed, quantitative research is very important for this purpose.
It is also important to note that in psychology research, researchers would normally discern cause-effect relationships, such as the study that determines the effect of drugs on teenagers. But cause-effect relationships cannot be elucidated without hard statistical data gathered through observations and empirical research. Hence, again, quantitative research is very important in the field of psychology because it allows researchers to accumulate facts and eventually create theories that allow researchers in psychology to understand human condition and perhaps diminish suffering and allow human race to flourish.
Second, on the importance of quantitative research in economics .
In general perspective, the economists have long used quantitative methods to provide us with theories and explanations on why certain things happen in the market. Through quantitative research too, economists were able to explain why a given economic system behaves the way it does. It is also important to note that the application of quantitative methods, models and the corresponding algorithms helps to make more accurate and efficient research of complex economic phenomena and issues, as well as their interdependence with the aim of making decisions and forecasting future trends of economic aspects and processes.
Third, on the importance of quantitative research in education .
Again, quantitative research deals with the collection of numerical data for some type of analysis. Whether a teacher is trying to assess the average scores on a classroom test, determine a teaching standard that was most commonly missed on the classroom assessment, or if a principal wants to assess the ways the attendance rates correlate with students’ performance on government assessments, quantitative research is more useful and appropriate.
In many cases too, school districts use quantitative data to evaluate teacher effectiveness from a number of measures, including stakeholder perception surveys, students’ performance and growth on standardized government assessments, and percentages on their levels of professionalism. Quantitative research is also good for informing instructional decisions, measuring the effectiveness of the school climate based on survey data issued to teachers and school personnel, and discovering students’ learning preferences.
Fourth, on the importance of quantitative research in Environmental Science and Sustainability.
Addressing environmental problems requires solid evidence to persuade decision makers of the necessity of change. This makes quantitative literacy essential for sustainability professionals to interpret scientific data and implement management procedures. Indeed, with our world facing increasingly complex environmental issues, quantitative techniques reduce the numerous uncertainties by providing a reliable representation of reality, enabling policy makers to proceed toward potential solutions with greater confidence. For this purpose, a wide range of statistical tools and approaches are now available for sustainability scientists to measure environmental indicators and inform responsible policymaking. As we can see, quantitative research is very important in environmental science and sustainability.
But how does quantitative research provide the context for environmental science and sustainability?
Environmental science brings a transdisciplinary systems approach to analyzing sustainability concerns. As the intrinsic concept of sustainability can be interpreted according to diverse values and definitions, quantitative methods based on rigorous scientific research are crucial for establishing an evidence-based consensus on pertinent issues that provide a foundation for meaningful policy implementation.
And fifth, on the importance of quantitative research in business .
As is well known, market research plays a key role in determining the factors that lead to business success. Whether one wants to estimate the size of a potential market or understand the competition for a particular product, it is very important to apply methods that will yield measurable results in conducting a market research assignment. Quantitative research can make this happen by employing data capture methods and statistical analysis. Quantitative market research is used for estimating consumer attitudes and behaviors, market sizing, segmentation and identifying drivers for brand recall and product purchase decisions.
Indeed, quantitative data open a lot of doors for businesses. Regression analysis, simulations, and hypothesis testing are examples of tools that might reveal trends that business leaders might not have noticed otherwise. Business leaders can use this data to identify areas where their company could improve its performance.
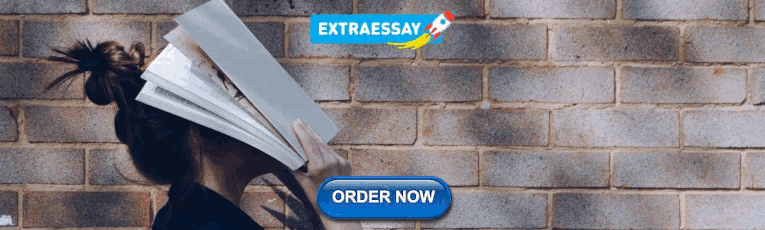
IMAGES
VIDEO
COMMENTS
Revised on June 22, 2023. Quantitative research is the process of collecting and analyzing numerical data. It can be used to find patterns and averages, make predictions, test causal relationships, and generalize results to wider populations. Quantitative research is the opposite of qualitative research, which involves collecting and analyzing ...
INTRODUCTION. Scientific research is usually initiated by posing evidenced-based research questions which are then explicitly restated as hypotheses.1,2 The hypotheses provide directions to guide the study, solutions, explanations, and expected results.3,4 Both research questions and hypotheses are essentially formulated based on conventional theories and real-world processes, which allow the ...
Advantages of Quantitative Research. Quantitative researchers aim to create a general understanding of behavior and other phenomena across different settings and populations. Quantitative studies are often fast, focused, scientific and relatable. 4. The speed and efficiency of the quantitative method are attractive to many researchers.
Quantitative research is a research strategy that focuses on quantifying the collection and analysis of data. ... In the field of climate science, researchers compile and compare statistics such as temperature or atmospheric concentrations of carbon dioxide. ... it has been argued that measurement often plays a more important role in ...
The science of science closely relates to several strands and communities of research, including metascience, scientometrics, the economics of science, research on research, science and technology ...
Abstract. Quantitative methods and approaches have been playing an increasingly important role in cell biology in recent years. They involve making accurate measurements to test a predefined hypothesis in order to compare experimental data with predictions generated by theoretical models, an approach that has benefited physicists for decades.
Introduction. Quantitative research, in contrast to qualitative research, deals with data that are numerical or that can be converted into numbers. The basic methods used to investigate numerical data are called 'statistics'. Statistical techniques are concerned with the organisation, analysis, interpretation and presentation of numerical data.
Abstract. This article describes the basic tenets of quantitative research. The concepts of dependent and independent variables are addressed and the concept of measurement and its associated issues, such as error, reliability and validity, are explored. Experiments and surveys - the principal research designs in quantitative research - are ...
1.2. Foundations of quantitative research methods 1.2.1. Realism, subjectivism and the 'paradigm wars' Now we have defined quantitative research, it is a good idea to compare it with qualitative research, to which it is usually put in opposition. While quantitative research is based on numerical data analysed statistically, qual-
Quantitative research methods are concerned with the planning, design, and implementation of strategies to collect and analyze data. Descartes, the seventeenth-century philosopher, suggested that how the results are achieved is often more important than the results themselves, as the journey taken along the research path is a journey of discovery. . High-quality quantitative research is ...
Quantitative research generates factual, reliable outcome data that are usually generalizable to some larger populations, and qualitative research produces rich, detailed and valid process data based on the participant's, rather than the investigator's, perspectives and interpretations ( 1 ). Quantitative research is usually deductive ...
This handbook offers a state-of-the-art overview of quantitative science and technology research. It focuses on the development and application of indicators derived from data on scientific or scholarly publications and patents. It comprises 34 chapters written by leading specialists in the various sub-domains. These chapters deal with ...
Social Science Research: Quantitative research is used in social science research to study human behavior, attitudes, and social structures. Researchers use surveys, experiments, and other quantitative methods to collect data that can inform social policies, educational programs, and community interventions. ... It is important to clearly ...
Quantitative research explains phenomena by collecting numerical unchanging d etailed data t hat. are analyzed using mathematically based methods, in particular statistics that pose questions of ...
Quantitative research can measure and describe whole societies, or institutions, organisations or groups of individuals that are part of them. The strength of quantitative methods is that they can provide vital information about a society or community, through surveys, examinations, records or censuses, that no individual could obtain by observation.
Translational Issues in Psychological Science is an ideal setting for continuing our momentum toward advances in quantitative methods. Its collaborative and multidisciplinary nature provides a perfect backdrop for increasing the awareness of statistical developments across subdisciplines.
As the intrinsic concept of sustainability can be interpreted according to diverse values and definitions, quantitative methods based on rigorous scientific research are crucial for establishing an evidence-based consensus on pertinent issues that provide a foundation for meaningful policy implementation.
Quantitative research on the science sector. The science sector has been acquiring great significance for more and more areas of our society. This makes it all the more important for the science system to be structured and funded as advantageously as possible. To an increasing degree, quality and productivity issues in science and research are ...
Abstract. "'Quantitative Research for the Behavioral Sciences" introduces students to a broad range of research methods used by behavioral scientists. It attempts to provide an understanding of how quantitative research is conducted, analyzed, and used in such a way that it will remain with students until they themselves have to deal with the ...
This paper discusses the quantitative research approach and how it applies to Library and Information Science (LIS). Although many different research methodologies are used in the diverse subject ...
Qualitative vs. Quantitative Research. Social science research, or social research as it is sometimes called, stems from the natural sciences, and similar to its precursory field, it uses empirical, measurable outcomes to arrive at a conclusion. While natural scientists use the scientific method, social scientists often use quantitative ...
Quantitative research is also good for informing instructional decisions, measuring the effectiveness of the school climate based on survey data issued to teachers and school personnel, and discovering students' learning preferences. Fourth, on the importance of quantitative research in Environmental Science and Sustainability.
a. Section 1 introduces the central challenges of quantitative empirical analysis in political science, and offers some overviews of one promising approach to tackling these challenges. b. Section 2 starts this thusly-organized tour of quantitative empirical-research methodology from the starting point of all empirical analysis: measurement.