News alert: UC Berkeley has announced its next university librarian
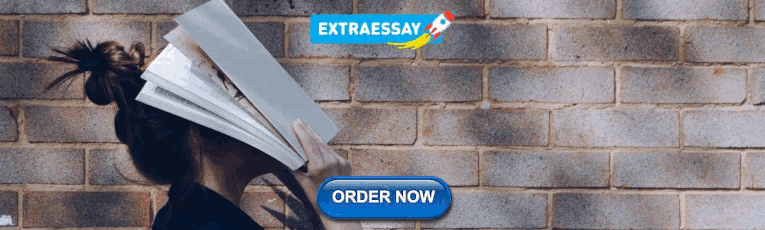
Secondary menu
- Log in to your Library account
- Hours and Maps
- Connect from Off Campus
- UC Berkeley Home
Search form
Research methods--quantitative, qualitative, and more: overview.
- Quantitative Research
- Qualitative Research
- Data Science Methods (Machine Learning, AI, Big Data)
- Text Mining and Computational Text Analysis
- Evidence Synthesis/Systematic Reviews
- Get Data, Get Help!
About Research Methods
This guide provides an overview of research methods, how to choose and use them, and supports and resources at UC Berkeley.
As Patten and Newhart note in the book Understanding Research Methods , "Research methods are the building blocks of the scientific enterprise. They are the "how" for building systematic knowledge. The accumulation of knowledge through research is by its nature a collective endeavor. Each well-designed study provides evidence that may support, amend, refute, or deepen the understanding of existing knowledge...Decisions are important throughout the practice of research and are designed to help researchers collect evidence that includes the full spectrum of the phenomenon under study, to maintain logical rules, and to mitigate or account for possible sources of bias. In many ways, learning research methods is learning how to see and make these decisions."
The choice of methods varies by discipline, by the kind of phenomenon being studied and the data being used to study it, by the technology available, and more. This guide is an introduction, but if you don't see what you need here, always contact your subject librarian, and/or take a look to see if there's a library research guide that will answer your question.
Suggestions for changes and additions to this guide are welcome!
START HERE: SAGE Research Methods
Without question, the most comprehensive resource available from the library is SAGE Research Methods. HERE IS THE ONLINE GUIDE to this one-stop shopping collection, and some helpful links are below:
- SAGE Research Methods
- Little Green Books (Quantitative Methods)
- Little Blue Books (Qualitative Methods)
- Dictionaries and Encyclopedias
- Case studies of real research projects
- Sample datasets for hands-on practice
- Streaming video--see methods come to life
- Methodspace- -a community for researchers
- SAGE Research Methods Course Mapping
Library Data Services at UC Berkeley
Library Data Services Program and Digital Scholarship Services
The LDSP offers a variety of services and tools ! From this link, check out pages for each of the following topics: discovering data, managing data, collecting data, GIS data, text data mining, publishing data, digital scholarship, open science, and the Research Data Management Program.
Be sure also to check out the visual guide to where to seek assistance on campus with any research question you may have!
Library GIS Services
Other Data Services at Berkeley
D-Lab Supports Berkeley faculty, staff, and graduate students with research in data intensive social science, including a wide range of training and workshop offerings Dryad Dryad is a simple self-service tool for researchers to use in publishing their datasets. It provides tools for the effective publication of and access to research data. Geospatial Innovation Facility (GIF) Provides leadership and training across a broad array of integrated mapping technologies on campu Research Data Management A UC Berkeley guide and consulting service for research data management issues
General Research Methods Resources
Here are some general resources for assistance:
- Assistance from ICPSR (must create an account to access): Getting Help with Data , and Resources for Students
- Wiley Stats Ref for background information on statistics topics
- Survey Documentation and Analysis (SDA) . Program for easy web-based analysis of survey data.
Consultants
- D-Lab/Data Science Discovery Consultants Request help with your research project from peer consultants.
- Research data (RDM) consulting Meet with RDM consultants before designing the data security, storage, and sharing aspects of your qualitative project.
- Statistics Department Consulting Services A service in which advanced graduate students, under faculty supervision, are available to consult during specified hours in the Fall and Spring semesters.
Related Resourcex
- IRB / CPHS Qualitative research projects with human subjects often require that you go through an ethics review.
- OURS (Office of Undergraduate Research and Scholarships) OURS supports undergraduates who want to embark on research projects and assistantships. In particular, check out their "Getting Started in Research" workshops
- Sponsored Projects Sponsored projects works with researchers applying for major external grants.
- Next: Quantitative Research >>
- Last Updated: Apr 3, 2023 3:14 PM
- URL: https://guides.lib.berkeley.edu/researchmethods

An official website of the United States government
Here’s how you know
Official websites use .gov A .gov website belongs to an official government organization in the United States.
Secure .gov websites use HTTPS A lock ( Lock A locked padlock ) or https:// means you’ve safely connected to the .gov website. Share sensitive information only on official, secure websites.

In Spring 2021, the National Library of Medicine (NLM) PubMed® Special Query on this page will no longer be curated by NLM. If you have questions, please contact NLM Customer Support at https://support.nlm.nih.gov/
This chart lists the major biomedical research reporting guidelines that provide advice for reporting research methods and findings. They usually "specify a minimum set of items required for a clear and transparent account of what was done and what was found in a research study, reflecting, in particular, issues that might introduce bias into the research" (Adapted from the EQUATOR Network Resource Centre ). The chart also includes editorial style guides for writing research reports or other publications.
See the details of the search strategy. More research reporting guidelines are at the EQUATOR Network Resource Centre .
Last Reviewed: April 14, 2023
When you choose to publish with PLOS, your research makes an impact. Make your work accessible to all, without restrictions, and accelerate scientific discovery with options like preprints and published peer review that make your work more Open.
- PLOS Biology
- PLOS Climate
- PLOS Complex Systems
- PLOS Computational Biology
- PLOS Digital Health
- PLOS Genetics
- PLOS Global Public Health
- PLOS Medicine
- PLOS Mental Health
- PLOS Neglected Tropical Diseases
- PLOS Pathogens
- PLOS Sustainability and Transformation
- PLOS Collections
- Collections Home
- About Collections
- Browse Collections
- Calls for Papers
Reporting Guidelines
Reporting guidelines are statements intended to advise authors reporting research methods and findings. They can be presented as a checklist, flow diagram or text, and describe what is required to give a clear and transparent account of a study's research and results. These guidelines are prepared through consideration of specific issues that may introduce bias, and are supported by the latest evidence in the field. The Reporting Guidelines Collection highlights articles published across PLOS journals and includes guidelines and guidance, commentary, and related research on guidelines. This collection features some of the many resources available to facilitate the rigorous reporting of scientific studies, and to improve the presentation and evaluation of published studies.
Image Credit: CCAC North Library, Flickr
- From the Blogs
- Observational & Epidemiological Research
- Randomized Controlled Trials
- Systematic Reviews & Meta-Analyses
- Diagnostic & Prognostic Research
- Animal & Cell Models
- General Guidance
- Image credit CCAC North Library, Flickr.com Speaking of Medicine Maximizing the Impact of Research: New Reporting Guidelines Collection from PLOS – Speaking of Medicine September 3, 2013 Amy Ross, Laureen Connell
- Image credit 10.1371/journal.pmed.1001885 PLOS Medicine The REporting of studies Conducted using Observational Routinely-collected health Data (RECORD) Statement October 6, 2015 Eric I. Benchimol, Liam Smeeth, Astrid Guttmann, Katie Harron, David Moher, Irene Petersen, Henrik T. Sørensen, Erik von Elm, Sinéad M. Langan, RECORD Working Committee
- Image credit 10.1371/journal.pmed.0040297 PLOS Medicine Strengthening the Reporting of Observational Studies in Epidemiology (STROBE): Explanation and Elaboration October 16, 2007 Jan P Vandenbroucke, Erik von Elm, Douglas G Altman, Peter C Gøtzsche, Cynthia D Mulrow, Stuart J Pocock, Charles Poole, James J Schlesselman, Matthias Egger, for the STROBE Initiative
- Image credit 10.1371/journal.pmed.0040296 PLOS Medicine The Strengthening the Reporting of Observational Studies in Epidemiology (STROBE) Statement: Guidelines for Reporting Observational Studies October 16, 2007 Erik von Elm, Douglas G Altman, Matthias Egger, Stuart J Pocock, Peter C Gøtzsche, Jan P Vandenbroucke, for the STROBE Initiative
- Image credit PLOS PLOS Medicine Reporting Guidelines for Survey Research: An Analysis of Published Guidance and Reporting Practices August 2, 2011 Carol Bennett, Sara Khangura, Jamie Brehaut, Ian Graham, David Moher, Beth Potter, Jeremy Grimshaw
- Image credit 10.1371/journal.pone.0064733 PLOS ONE Impact of STROBE Statement Publication on Quality of Observational Study Reporting: Interrupted Time Series versus Before-After Analysis August 26, 2013 Sylvie Bastuji-Garin, Emilie Sbidian, Caroline Gaudy-Marqueste, Emilie Ferrat, Jean-Claude Roujeau, Marie-Aleth Richard, Florence Canoui-Poitrine
- Image credit 10.1371/journal.pone.0094412 PLOS ONE The Reporting of Observational Clinical Functional Magnetic Resonance Imaging Studies: A Systematic Review April 22, 2014 Qing Guo, Melissa Parlar, Wanda Truong, Geoffrey Hall, Lehana Thabane, Margaret McKinnon, Ron Goeree, Eleanor Pullenayegum
- Image credit 10.1371/journal.pone.0101176 PLOS ONE A Review of Published Analyses of Case-Cohort Studies and Recommendations for Future Reporting June 27, 2014 Stephen Sharp, Manon Poulaliou, Simon Thompson, Ian White, Angela Wood
- Image credit 10.1371/journal.pone.0103360 PLOS ONE Outlier Removal and the Relation with Reporting Errors and Quality of Psychological Research July 29, 2014 Marjan Bakker, Jelte Wicherts
- Image credit 10.1371/journal.pmed.1000022 PLOS Medicine STrengthening the REporting of Genetic Association Studies (STREGA)— An Extension of the STROBE Statement February 3, 2009 Julian Little, Julian P.T Higgins, John P.A Ioannidis, David Moher, France Gagnon, Erik von Elm, Muin J Khoury, Barbara Cohen, George Davey-Smith, Jeremy Grimshaw, Paul Scheet, Marta Gwinn, Robin E Williamson, Guang Yong Zou, Kim Hutchings, Candice Y Johnson, Valerie Tait, Miriam Wiens, Jean Golding, Cornelia van Duijn, John McLaughlin, Andrew Paterson, George Wells, Isabel Fortier, Matthew Freedman, Maja Zecevic, Richard King, Claire Infante-Rivard, Alex Stewart, Nick Birkett
- Image credit 10.1371/journal.pmed.1001117 PLOS Medicine STrengthening the Reporting of OBservational studies in Epidemiology – Molecular Epidemiology (STROBE-ME): An Extension of the STROBE Statement October 25, 2011 Valentina Gallo, Matthias Egger, Valerie McCormack, Peter Farmer, John Ioannidis, Micheline Kirsch-Volders, Giuseppe Matullo, David Phillips, Bernadette Schoket, Ulf Strömberg, Roel Vermeulen, Christopher Wild, Miquel Porta, Paolo Vineis
- Image credit PLOS PLOS Medicine Observational Studies: Getting Clear about Transparency August 26, 2014 The PLoS Medicine Editors
- Image credit 10.1371/journal.pmed.0050020 PLOS Medicine CONSORT for Reporting Randomized Controlled Trials in Journal and Conference Abstracts: Explanation and Elaboration January 22, 2008 Sally Hopewell, Mike Clarke, David Moher, Elizabeth Wager, Philippa Middleton, Douglas G Altman, Kenneth F Schulz, and the CONSORT Group
- Image credit PLOS PLOS ONE Endorsement of the CONSORT Statement by High-Impact Medical Journals in China: A Survey of Instructions for Authors and Published Papers February 13, 2012 Xiao-qian Li, Kun-ming Tao, Qinghui Zhou, David Moher, Hong-yun Chen, Fu-zhe Wang, Chang-quan Ling
- Image credit PLOS PLOS ONE Assessing the Quality of Reports about Randomized Controlled Trials of Acupuncture Treatment on Diabetic Peripheral Neuropathy July 2, 2012 Chen Bo, Zhao Xue, Guo Yi, Chen Zelin, Bai Yang, Wang Zixu, Wang Yajun
- Image credit 10.1371/journal.pone.0065442 PLOS ONE Reporting Quality of Social and Psychological Intervention Trials: A Systematic Review of Reporting Guidelines and Trial Publications May 29, 2013 Sean Grant, Evan Mayo-Wilson, G. J. Melendez-Torres, Paul Montgomery
- Image credit 10.1371/journal.pone.0084779 PLOS ONE Are Reports of Randomized Controlled Trials Improving over Time? A Systematic Review of 284 Articles Published in High-Impact General and Specialized Medical Journals December 31, 2013 Matthew To, Jennifer Jones, Mohamed Emara, Alejandro Jadad
- Image credit 10.1371/journal.pone.0086360 PLOS ONE Assessment of the Reporting Quality of Randomized Controlled Trials on Treatment of Coronary Heart Disease with Traditional Chinese Medicine from the Chinese Journal of Integrated Traditional and Western Medicine: A Systematic Review January 28, 2014 Fan Fang, Xu Qin, Sun Qi, Zhao Jun, Wang Ping, Guo Rui
- Image credit 10.1371/journal.pmed.1001666 PLOS Medicine Evidence for the Selective Reporting of Analyses and Discrepancies in Clinical Trials: A Systematic Review of Cohort Studies of Clinical Trials June 24, 2014 Kerry Dwan, Douglas Altman, Mike Clarke, Carrol Gamble, Julian Higgins, Jonathan Sterne, Paula Williamson, Jamie Kirkham
- Image credit 10.1371/journal.pone.0110229 PLOS ONE Systematic Evaluation of the Patient-Reported Outcome (PRO) Content of Clinical Trial Protocols October 15, 2014 Derek Kyte, Helen Duffy, Benjamin Fletcher, Adrian Gheorghe, Rebecca Mercieca-Bebber, Madeleine King, Heather Draper, Jonathan Ives, Michael Brundage, Jane Blazeby, Melanie Calvert
- Image credit 10.1371/journal.pone.0110216 PLOS ONE Patient-Reported Outcome (PRO) Assessment in Clinical Trials: A Systematic Review of Guidance for Trial Protocol Writers October 15, 2014 Melanie Calvert, Derek Kyte, Helen Duffy, Adrian Gheorghe, Rebecca Mercieca-Bebber, Jonathan Ives, Heather Draper, Michael Brundage, Jane Blazeby, Madeleine King
- Image credit 10.1371/journal.pmed.1000261 PLOS Medicine Revised STandards for Reporting Interventions in Clinical Trials of Acupuncture (STRICTA): Extending the CONSORT Statement June 8, 2010 Hugh MacPherson, Douglas G. Altman, Richard Hammerschlag, Li Youping, Wu Taixiang, Adrian White, David Moher, on behalf of the STRICTA Revision Group
- Image credit PLOS PLOS Medicine Comparative Effectiveness Research: Challenges for Medical Journals April 27, 2010 Harold C. Sox, Mark Helfand, Jeremy Grimshaw, Kay Dickersin, the PLoS Medicine Editors , David Tovey, J. André Knottnerus, Peter Tugwell
- Image credit PLOS PLOS Medicine Reporting of Systematic Reviews: The Challenge of Genetic Association Studies June 26, 2007 Muin J Khoury, Julian Little, Julian Higgins, John P. A Ioannidis, Marta Gwinn
- Image credit 10.1371/journal.pmed.0040078 PLOS Medicine Epidemiology and Reporting Characteristics of Systematic Reviews March 27, 2007 David Moher, Jennifer Tetzlaff, Andrea C Tricco, Margaret Sampson, Douglas G Altman
- Image credit 10.1371/journal.pone.0027611 PLOS ONE From QUOROM to PRISMA: A Survey of High-Impact Medical Journals’ Instructions to Authors and a Review of Systematic Reviews in Anesthesia Literature November 16, 2011 Kun-ming Tao, Xiao-qian Li, Qing-hui Zhou, David Moher, Chang-quan Ling, weifeng yu
- Image credit 10.1371/journal.pone.0075122 PLOS ONE Testing the PRISMA-Equity 2012 Reporting Guideline: the Perspectives of Systematic Review Authors October 10, 2013 Belinda Burford, Vivian Welch, Elizabeth Waters, Peter Tugwell, David Moher, Jennifer O'Neill, Tracey Koehlmoos, Mark Petticrew
- Image credit 10.1371/journal.pone.0092508 PLOS ONE The Quality of Reporting Methods and Results in Network Meta-Analyses: An Overview of Reviews and Suggestions for Improvement March 26, 2014 Brian Hutton, Georgia Salanti, Anna Chaimani, Deborah Caldwell, Chris Schmid, Kristian Thorlund, Edward Mills, Lucy Turner, Ferran Catala-Lopez, Doug Altman, David Moher
- Image credit 10.1371/journal.pone.0096407 PLOS ONE Blinded by PRISMA: Are Systematic Reviewers Focusing on PRISMA and Ignoring Other Guidelines? May 1, 2014 Padhraig Fleming, Despina Koletsi, Nikolaos Pandis
- Image credit 10.1371/journal.pmed.1000097 PLOS Medicine Preferred Reporting Items for Systematic Reviews and Meta-Analyses: The PRISMA Statement July 21, 2009 David Moher, Alessandro Liberati, Jennifer Tetzlaff, Douglas G. Altman, The PRISMA Group
- Image credit 10.1371/journal.pmed.1001333 PLOS Medicine PRISMA-Equity 2012 Extension: Reporting Guidelines for Systematic Reviews with a Focus on Health Equity October 30, 2012 Vivian Welch, Mark Petticrew, Peter Tugwell, David Moher, Jennifer O'Neill, Elizabeth Waters, Howard White
- Image credit 10.1371/journal.pmed.1001419 PLOS Medicine PRISMA for Abstracts: Reporting Systematic Reviews in Journal and Conference Abstracts April 9, 2013 Elaine Beller, Paul Glasziou, Douglas Altman, Sally Hopewell, Hilda Bastian, Iain Chalmers, Peter Gotzsche, Toby Lasserson, David Tovey
- Image credit PLOS PLOS Medicine Many Reviews Are Systematic but Some Are More Transparent and Completely Reported than Others March 27, 2007 The PLoS Medicine Editors
- Image credit 10.1371/journal.pmed.1000100 PLOS Medicine The PRISMA Statement for Reporting Systematic Reviews and Meta-Analyses of Studies That Evaluate Health Care Interventions: Explanation and Elaboration July 21, 2009 Alessandro Liberati, Douglas G. Altman, Jennifer Tetzlaff, Cynthia Mulrow, Peter C. Gøtzsche, John P. A. Ioannidis, Mike Clarke, P. J. Devereaux, Jos Kleijnen, David Moher
- Image credit 10.1371/journal.pone.0007753 PLOS ONE Quality and Reporting of Diagnostic Accuracy Studies in TB, HIV and Malaria: Evaluation Using QUADAS and STARD Standards November 13, 2009 Patricia Scolari Fontela, Nitika Pant Pai, Ian Schiller, Nandini Dendukuri, Andrew Ramsay, Madhukar Pai
- Image credit 10.1371/journal.pmed.1001531 PLOS Medicine Use of Expert Panels to Define the Reference Standard in Diagnostic Research: A Systematic Review of Published Methods and Reporting October 15, 2013 Loes Bertens, Berna Broekhuizen, Christiana Naaktgeboren, Frans Rutten, Arno Hoes, Yvonne van Mourik, Karel Moons, Johannes Reitsma
- Image credit 10.1371/journal.pone.0085908 PLOS ONE The Assessment of the Quality of Reporting of Systematic Reviews/Meta-Analyses in Diagnostic Tests Published by Authors in China January 21, 2014 Long Ge, Jian-cheng Wang, Jin-long Li, Li Liang, Ni An, Xin-tong Shi, Yin-chun Liu, JH Tian
- Image credit 10.1371/journal.pmed.1000420 PLOS Medicine Strengthening the Reporting of Genetic Risk Prediction Studies: The GRIPS Statement March 15, 2011 A. Cecile J. W. Janssens, John P. A. Ioannidis, Cornelia M. van Duijn, Julian Little, Muin J. Khoury, for the GRIPS Group
- Image credit 10.1371/journal.pmed.1001216 PLOS Medicine Reporting Recommendations for Tumor Marker Prognostic Studies (REMARK): Explanation and Elaboration May 29, 2012 Doug Altman, Lisa McShane, Willi Sauerbrei, Sheila Taube
- Image credit 10.1371/journal.pmed.1001381 PLOS Medicine Prognosis Research Strategy (PROGRESS) 3: Prognostic Model Research February 5, 2013 Ewout Steyerberg, Karel Moons, Danielle van der Windt, Jill Hayden, Pablo Perel, Sara Schroter, Richard Riley, Harry Hemingway, Douglas Altman
- Image credit 10.1371/journal.pmed.1001380 PLOS Medicine Prognosis Research Strategy (PROGRESS) 2: Prognostic Factor Research February 5, 2013 Richard Riley, Jill Hayden, Ewout Steyerberg, Karel Moons, Keith Abrams, Panayiotis Kyzas, Nuria Malats, Andrew Briggs, Sara Schroter, Douglas Altman, Harry Hemingway
- Image credit 10.1371/journal.pmed.1001671 PLOS Medicine Improving the Transparency of Prognosis Research: The Role of Reporting, Data Sharing, Registration, and Protocols July 8, 2014 George Peat, Richard Riley, Peter Croft, Katherine Morley, Panayiotis Kyzas, Karel Moons, Pablo Perel, Ewout Steyerberg, Sara Schroter, Douglas Altman, Harry Hemingway
- Image credit 10.1371/journal.pone.0007824 PLOS ONE Survey of the Quality of Experimental Design, Statistical Analysis and Reporting of Research Using Animals November 30, 2009 Carol Kilkenny, Nick Parsons, Ed Kadyszewski, Michael F. W. Festing, Innes C. Cuthill, Derek Fry, Jane Hutton, Douglas G. Altman
- Image credit 10.1371/journal.pmed.1001489 PLOS Medicine Threats to Validity in the Design and Conduct of Preclinical Efficacy Studies: A Systematic Review of Guidelines for In Vivo Animal Experiments July 23, 2013 Valerie Henderson, Jonathan Kimmelman, Dean Fergusson, Jeremy Grimshaw, Dan Hackam
- Image credit 10.1371/journal.pone.0088266 PLOS ONE Five Years MIQE Guidelines: The Case of the Arabian Countries February 4, 2014 Afif Abdel Nour, Esam Azhar, Ghazi Damanhouri, Stephen Bustin
- Image credit 10.1371/journal.pone.0101131 PLOS ONE The Quality of Methods Reporting in Parasitology Experiments July 30, 2014 Oscar Flórez-Vargas, Michael Bramhall, Harry Noyes, Sheena Cruickshank, Robert Stevens, Andy Brass
- Image credit 10.1371/journal.pbio.1000412 PLOS Biology Improving Bioscience Research Reporting: The ARRIVE Guidelines for Reporting Animal Research June 29, 2010 Carol Kilkenny, William J. Browne, Innes C. Cuthill, Michael Emerson, Douglas G. Altman
- Image credit 10.1371/journal.pbio.1001481 PLOS Biology Whole Animal Experiments Should Be More Like Human Randomized Controlled Trials February 12, 2013 Beverly Muhlhausler, Frank Bloomfield, Matthew Gillman
- Image credit 10.1371/journal.pbio.1001756 PLOS Biology Two Years Later: Journals Are Not Yet Enforcing the ARRIVE Guidelines on Reporting Standards for Pre-Clinical Animal Studies January 7, 2014 David Baker, Katie Lidster, Ana Sottomayor, Sandra Amor
- Image credit PLOS PLOS Biology Reporting Animal Studies: Good Science and a Duty of Care June 29, 2010 Catriona J. MacCallum
- Image credit PLOS PLOS Biology Open Science and Reporting Animal Studies: Who’s Accountable? January 7, 2014 Catriona MacCallum, Jonathan Eisen, Emma Ganley
- Image credit PLOS PLOS Computational Biology Minimum Information About a Simulation Experiment (MIASE) April 28, 2011 Dagmar Waltemath, Richard Adams, Daniel A. Beard, Frank T. Bergmann, Upinder S. Bhalla, Randall Britten, Vijayalakshmi Chelliah, Michael T. Cooling, Jonathan Cooper, Edmund J. Crampin, Alan Garny, Stefan Hoops, Michael Hucka, Peter Hunter, Edda Klipp, Camille Laibe, Andrew K. Miller, Ion Moraru, David Nickerson, Poul Nielsen, Macha Nikolski, Sven Sahle, Herbert M. Sauro, Henning Schmidt, Jacky L. Snoep, Dominic Tolle, Olaf Wolkenhauer, Nicolas Le Novère
- Image credit 10.1371/journal.pmed.0050139 PLOS Medicine Guidelines for Reporting Health Research: The EQUATOR Network’s Survey of Guideline Authors June 24, 2008 Iveta Simera, Douglas G Altman, David Moher, Kenneth F Schulz, John Hoey
- Image credit 10.1371/journal.pmed.1000217 PLOS Medicine Guidance for Developers of Health Research Reporting Guidelines February 16, 2010 David Moher, Kenneth F. Schulz, Iveta Simera, Douglas G. Altman
- Image credit 10.1371/journal.pone.0035621 PLOS ONE Are Peer Reviewers Encouraged to Use Reporting Guidelines? A Survey of 116 Health Research Journals April 27, 2012 Allison Hirst, Douglas Altman
- Image credit PLOS PLOS Neglected Tropical Diseases Research Ethics and Reporting Standards at PLoS Neglected Tropical Diseases October 31, 2007 Gavin Yamey
- Image credit PLOS PLOS Medicine Better Reporting, Better Research: Guidelines and Guidance in PLoS Medicine April 29, 2008 The PLoS Medicine Editors
- Image credit CCAC North Library, Flickr.com PLOS Medicine Better Reporting of Scientific Studies: Why It Matters August 27, 2013 The PLoS Medicine Editors
- Image credit 10.1371/journal.pone.0097492 PLOS ONE Do Editorial Policies Support Ethical Research? A Thematic Text Analysis of Author Instructions in Psychiatry Journals June 5, 2014 Daniel Strech, Courtney Metz, Hannes Knüppel
Research methods & reporting
Quantifying possible bias in clinical and epidemiological studies with quantitative bias analysis: common approaches and limitations, assessing robustness to worst case publication bias using a simple subset meta-analysis, regression discontinuity design studies: a guide for health researchers, process guide for inferential studies using healthcare data from routine clinical practice to evaluate causal effects of drugs, updated recommendations for the cochrane rapid review methods guidance for rapid reviews of effectiveness, avoiding conflicts of interest and reputational risks associated with population research on food and nutrition, the estimands framework: a primer on the ich e9(r1) addendum, evaluation of clinical prediction models (part 3): calculating the sample size required for an external validation study, evaluation of clinical prediction models (part 2): how to undertake an external validation study, evaluation of clinical prediction models (part 1): from development to external validation, emulation of a target trial using electronic health records and a nested case-control design, rob-me: a tool for assessing risk of bias due to missing evidence in systematic reviews with meta-analysis, enhancing reporting quality and impact of early phase dose-finding clinical trials: consort dose-finding extension (consort-define) guidance, enhancing quality and impact of early phase dose-finding clinical trial protocols: spirit dose-finding extension (spirit-define) guidance, understanding how health interventions or exposures produce their effects using mediation analysis, a guide and pragmatic considerations for applying grade to network meta-analysis, a framework for assessing selection and misclassification bias in mendelian randomisation studies: an illustrative example between bmi and covid-19, practical thematic analysis: a guide for multidisciplinary health services research teams engaging in qualitative analysis, selection bias due to conditioning on a collider, the imprinting effect of covid-19 vaccines: an expected selection bias in observational studies, a step-by-step approach for selecting an optimal minimal important difference, recommendations for the development, implementation, and reporting of control interventions in trials of self-management therapies, methods for deriving risk difference (absolute risk reduction) from a meta-analysis, transparent reporting of multivariable prediction models for individual prognosis or diagnosis: checklist for systematic reviews and meta-analyses, consort harms 2022 statement, explanation, and elaboration: updated guideline for the reporting of harms in randomised trials, transparent reporting of multivariable prediction models: : explanation and elaboration, transparent reporting of multivariable prediction models: tripod-cluster checklist, bias by censoring for competing events in survival analysis, code-ehr best practice framework for the use of structured electronic healthcare records in clinical research, validation of prediction models in the presence of competing risks, reporting guideline for the early stage clinical evaluation of decision support systems driven by artificial intelligence, searching clinical trials registers: guide for systematic reviewers, how to design high quality acupuncture trials—a consensus informed by evidence, early phase clinical trials extension to guidelines for the content of statistical analysis plans, incorporating dose effects in network meta-analysis, consolidated health economic evaluation reporting standards 2022 statement, strengthening the reporting of observational studies in epidemiology using mendelian randomisation (strobe-mr): explanation and elaboration, a new framework for developing and evaluating complex interventions, adapting interventions to new contexts—the adapt guidance, recommendations for including or reviewing patient reported outcome endpoints in grant applications, consort extension for the reporting of randomised controlled trials conducted using cohorts and routinely collected data (consort-routine): checklist with explanation and elaboration, consort extension for the reporting of randomised controlled trials conducted using cohorts and routinely collected data, guidance for the design and reporting of studies evaluating the clinical performance of tests for present or past sars-cov-2 infection, the prisma 2020 statement: an updated guideline for reporting systematic reviews, prisma 2020 explanation and elaboration: updated guidance and exemplars for reporting systematic reviews, preferred reporting items for journal and conference abstracts of systematic reviews and meta-analyses of diagnostic test accuracy studies (prisma-dta for abstracts): checklist, explanation, and elaboration, designing and undertaking randomised implementation trials: guide for researchers, start-rwe: structured template for planning and reporting on the implementation of real world evidence studies, methodological standards for qualitative and mixed methods patient centered outcomes research, grade approach to drawing conclusions from a network meta-analysis using a minimally contextualised framework, grade approach to drawing conclusions from a network meta-analysis using a partially contextualised framework, use of multiple period, cluster randomised, crossover trial designs for comparative effectiveness research, when to replicate systematic reviews of interventions: consensus checklist, reporting guidelines for clinical trial reports for interventions involving artificial intelligence: the consort-ai extension, guidelines for clinical trial protocols for interventions involving artificial intelligence: the spirit-ai extension, preferred reporting items for systematic review and meta-analysis of diagnostic test accuracy studies (prisma-dta): explanation, elaboration, and checklist, non-adherence in non-inferiority trials: pitfalls and recommendations, the adaptive designs consort extension (ace) statement: a checklist with explanation and elaboration guideline for reporting randomised trials that use an adaptive design, machine learning and artificial intelligence research for patient benefit: 20 critical questions on transparency, replicability, ethics, and effectiveness, calculating the sample size required for developing a clinical prediction model, spirit extension and elaboration for n-of-1 trials: spent 2019 checklist, synthesis without meta-analysis (swim) in systematic reviews: reporting guideline, alternative approaches for confounding adjustment in observational studies using weighting based on the propensity score: a primer for practitioners, a guide to prospective meta-analysis, rob 2: a revised tool for assessing risk of bias in randomised trials, consort 2010 statement: extension to randomised crossover trials, when and how to use data from randomised trials to develop or validate prognostic models, guide to presenting clinical prediction models for use in clinical settings, a guide to systematic review and meta-analysis of prognostic factor studies, when continuous outcomes are measured using different scales: guide for meta-analysis and interpretation, the reporting of studies conducted using observational routinely collected health data statement for pharmacoepidemiology (record-pe), reporting of stepped wedge cluster randomised trials: extension of the consort 2010 statement with explanation and elaboration, delta,2, guidance on choosing the target difference and undertaking and reporting the sample size calculation for a randomised controlled trial, outcome reporting bias in trials: a methodological approach for assessment and adjustment in systematic reviews, reading mendelian randomisation studies: a guide, glossary, and checklist for clinicians, how to use fda drug approval documents for evidence syntheses, how to avoid common problems when using clinicaltrials.gov in research: 10 issues to consider, tidier-php: a reporting guideline for population health and policy interventions, analysis of cluster randomised trials with an assessment of outcome at baseline, key design considerations for adaptive clinical trials: a primer for clinicians, population attributable fraction, how to estimate the effect of treatment duration on survival outcomes using observational data, concerns about composite reference standards in diagnostic research, statistical methods to compare functional outcomes in randomized controlled trials with high mortality, consort-equity 2017 extension and elaboration for better reporting of health equity in randomised trials, handling time varying confounding in observational research, four study design principles for genetic investigations using next generation sequencing, amstar 2: a critical appraisal tool for systematic reviews that include randomised or non-randomised studies of healthcare interventions, or both, multivariate and network meta-analysis of multiple outcomes and multiple treatments: rationale, concepts, and examples, stard for abstracts: essential items for reporting diagnostic accuracy studies in journal or conference abstracts, statistics notes: percentage differences, symmetry, and natural logarithms, statistics notes: what is a percentage difference, gripp2 reporting checklists: tools to improve reporting of patient and public involvement in research, enhancing the usability of systematic reviews by improving the consideration and description of interventions, how to design efficient cluster randomised trials, consort 2010 statement: extension checklist for reporting within person randomised trials, life expectancy difference and life expectancy ratio: two measures of treatment effects in randomised trials with non-proportional hazards, standards for reporting implementation studies (stari) statement, meta-analytical methods to identify who benefits most from treatments: daft, deluded, or deft approach, follow us on, content links.
- Collections
- Health in South Asia
- Women’s, children’s & adolescents’ health
- News and views
- BMJ Opinion
- Rapid responses
- Editorial staff
- BMJ in the USA
- BMJ in South Asia
- Submit your paper
- BMA members
- Subscribers
- Advertisers and sponsors
Explore BMJ
- Our company
- BMJ Careers
- BMJ Learning
- BMJ Masterclasses
- BMJ Journals
- BMJ Student
- Academic edition of The BMJ
- BMJ Best Practice
- The BMJ Awards
- Email alerts
- Activate subscription
Information
Thank you for visiting nature.com. You are using a browser version with limited support for CSS. To obtain the best experience, we recommend you use a more up to date browser (or turn off compatibility mode in Internet Explorer). In the meantime, to ensure continued support, we are displaying the site without styles and JavaScript.
- View all journals
Collection 18 December 2023
Best Practices in Method Reporting
For many years, SpringerNature has raised awareness of the challenges in replicating scientific experiments, and promoted the publication of detailed methods and protocols. Following a workshop organised by European Commission's Joint Research Centre, we contributed to a document drafting recommendations to improve methodological clarity in life sciences publications which has inspired this Collection highlighting content across a spectrum of different journals and subject areas.
Other collections of similar articles can be found in the “Related Collections” tab. The “Resources” tab includes a list of journals dedicated to the publication of methods or protocols as well as links to suitable preprint servers related to method and data sharing, and recorded webinars.
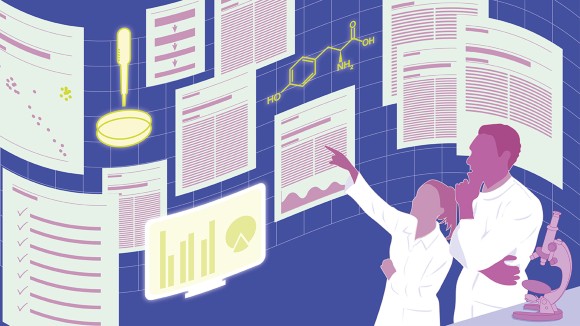
Bronwen Dekker
Nature Protocols
- Collection content
- Participating journals
- Related Collections
Editorials and Featues
Ensuring accurate resource identification.
Nature Protocols is pleased to be a part of the Resource Identification Initiative, a project aimed at improving the reproducibility of research by clearly identifying key biological resources. Stable unique digital identifiers, called Research Resource Identifiers (RRIDs), are assigned to individual resources, allowing users to accurately identify and source them, track their history, identify known problems (such as cell line contamination and misidentification) and find relevant research papers. Following a successful 6-month trial, we will now require authors to provide RRIDs for all antibodies and cell lines used in their protocols. We will also be encouraging them to add RRIDs for other tools (such as plasmids and organisms) where they think this is helpful.
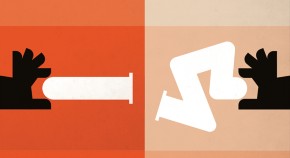
Five keys to writing a reproducible lab protocol
Effective sharing of experimental methods is crucial to ensuring that others can repeat results. An abundance of tools is available to help.
- Monya Baker
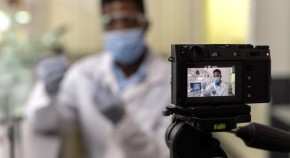
Share methods through visual and digital protocols
Documenting experimental methods ensures reproducibility and accountability, and there are innovative ways to disseminate and update them.
Registered Reports at Nature Methods
Nature Methods is introducing a new article format: Registered Reports. We encourage all authors interested in submitting comparative analyses of the performance of established, related tools or methods to familiarize themselves with this alternative approach to peer review.
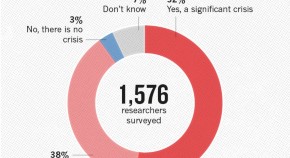
1,500 scientists lift the lid on reproducibility
Survey sheds light on the ‘crisis’ rocking research.
Consensus Statements
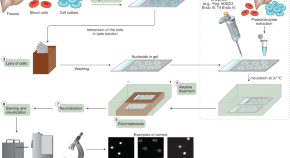
Minimum Information for Reporting on the Comet Assay (MIRCA): recommendations for describing comet assay procedures and results
Here, members of the hCOMET COST Action program provide a consensus statement on the Minimum Information for Reporting Comet Assays (MIRCA).
- Peter Møller
- Amaya Azqueta
- Sabine A. S. Langie
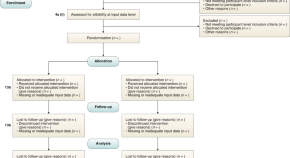
Reporting guidelines for clinical trial reports for interventions involving artificial intelligence: the CONSORT-AI extension
The CONSORT-AI and SPIRIT-AI extensions improve the transparency of clinical trial design and trial protocol reporting for artificial intelligence interventions.
- Xiaoxuan Liu
- Samantha Cruz Rivera
- The SPIRIT-AI and CONSORT-AI Working Group
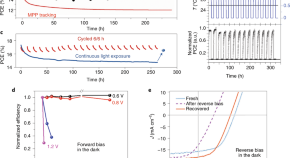
Consensus statement for stability assessment and reporting for perovskite photovoltaics based on ISOS procedures
Reliability of stability data for perovskite solar cells is undermined by a lack of consistency in the test conditions and reporting. This Consensus Statement outlines practices for testing and reporting stability tailoring ISOS protocols for perovskite devices.
- Mark V. Khenkin
- Eugene A. Katz
- Monica Lira-Cantu
Reviews and Perspectives
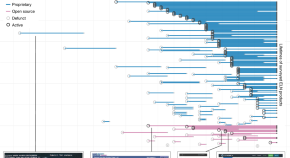
Considerations for implementing electronic laboratory notebooks in an academic research environment
This review explores factors to consider when introducing electronic laboratory notebooks, including discussion of integration with research data management and the functionalities to compare when evaluating specific software packages.
- Stuart G. Higgins
- Akemi A. Nogiwa-Valdez
- Molly M. Stevens
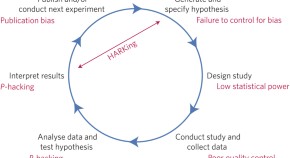
A manifesto for reproducible science
Leading voices in the reproducibility landscape call for the adoption of measures to optimize key elements of the scientific process.
- Marcus R. Munafò
- Brian A. Nosek
- John P. A. Ioannidis
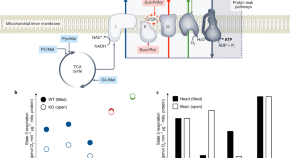
A practical guide for the analysis, standardization and interpretation of oxygen consumption measurements
Measurements of oxygen consumption rates have been central to the resurgent interest in studying cellular metabolism. To enhance the overall reproducibility and reliability of these measurements, Divakaruni and Jastroch provide a guide advising on the selection of experimental models and instrumentation as well as the analysis and interpretation of data.
- Ajit S. Divakaruni
- Martin Jastroch
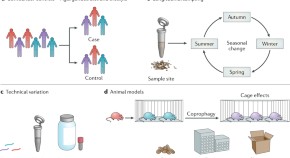
Best practices for analysing microbiomes
Complex microbial communities shape the dynamics of various environments. In this Review, Knight and colleagues discuss the best practices for performing a microbiome study, including experimental design, choice of molecular analysis technology, methods for data analysis and the integration of multiple omics data sets.
- Alison Vrbanac
- Pieter C. Dorrestein
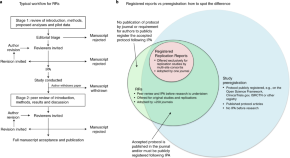
The past, present and future of Registered Reports
Registered Reports were introduced a decade ago as a means for improving the rigour and credibility of confirmatory research. Chambers and Tzavella overview the format’s past, its current status and future developments.
- Christopher D. Chambers
- Loukia Tzavella
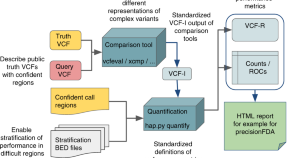
Best practices for benchmarking germline small-variant calls in human genomes
A new standard allows the accuracy of variant calls to be assessed and compared across different technologies, variant types and genomic regions.
- Peter Krusche
- the Global Alliance for Genomics and Health Benchmarking Team
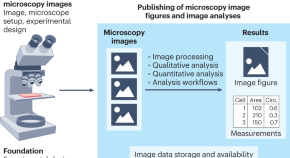
Community-developed checklists for publishing images and image analyses
Community-developed checklists offer best-practice guidance for biologists preparing light microscopy images and describing image analyses for publications.
- Christopher Schmied
- Michael S. Nelson
- Helena Klara Jambor
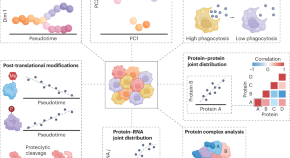
Initial recommendations for performing, benchmarking and reporting single-cell proteomics experiments
A community of researchers working in the emerging field of single-cell proteomics propose best-practice experimental and computational recommendations and reporting guidelines for studies analyzing proteins from single cells by mass spectrometry.
- Laurent Gatto
- Ruedi Aebersold
- Nikolai Slavov
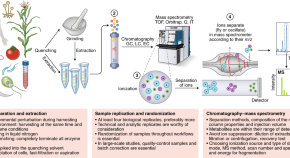
Mass spectrometry-based metabolomics: a guide for annotation, quantification and best reporting practices
This Perspective, from a large group of metabolomics experts, provides best practices and simplified reporting guidelines for practitioners of liquid chromatography– and gas chromatography–mass spectrometry-based metabolomics.
- Saleh Alseekh
- Asaph Aharoni
- Alisdair R. Fernie
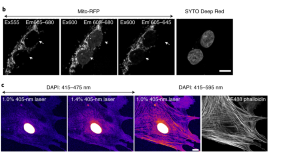
Best practices and tools for reporting reproducible fluorescence microscopy methods
Comprehensive guidelines and resources to enable accurate reporting for the most common fluorescence light microscopy modalities are reported with the goal of improving microscopy reporting, rigor and reproducibility.
- Paula Montero Llopis
- Rebecca A. Senft
- Michelle S. Itano
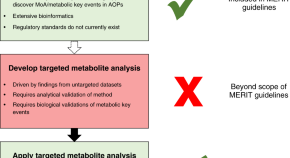
Use cases, best practice and reporting standards for metabolomics in regulatory toxicology
Lack of best practice guidelines currently limits the application of metabolomics in the regulatory sciences. Here, the MEtabolomics standaRds Initiative in Toxicology (MERIT) proposes methods and reporting standards for several important applications of metabolomics in regulatory toxicology.
- Mark R. Viant
- Timothy M. D. Ebbels
- Ralf J. M. Weber
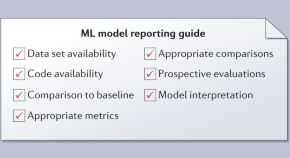
Evaluation guidelines for machine learning tools in the chemical sciences
Studies employing machine-learning (ML) tools in the chemical sciences often report their evaluations in a heterogeneous way. The evaluation guidelines provided in this Perspective should enable more rigorous ML reporting.
- Andreas Bender
- Nadine Schneider
- Tiago Rodrigues
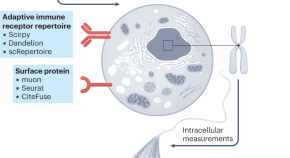
Best practices for single-cell analysis across modalities
Practitioners in the field of single-cell omics are now faced with diverse options for analytical tools to process and integrate data from various molecular modalities. In an Expert Recommendation article, the authors provide guidance on robust single-cell data analysis, including choices of best-performing tools from benchmarking studies.
- Lukas Heumos
- Anna C. Schaar
- Fabian J. Theis
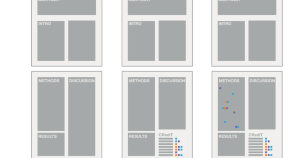
Method Reporting with Initials for Transparency (MeRIT) promotes more granularity and accountability for author contributions
Lack of information on authors’ contribution to specific aspects of a study hampers reproducibility and replicability. Here, the authors propose a new, easily implemented reporting system to clarify contributor roles in the Methods section of an article.
- Shinichi Nakagawa
- Edward R. Ivimey-Cook
- Malgorzata Lagisz
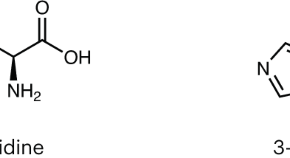
Towards a Rosetta stone for metabolomics: recommendations to overcome inconsistent metabolite nomenclature
The metabolomics literature suffers from ambiguity in the nomenclature for individual metabolites, which introduces a disconnect between publications and leads to misinterpretations. This Comment proposes recommendations for metabolite annotations to engage the scientific community and publishers to adopt a more consistent approach to metabolite nomenclature.
- Ville Koistinen
- Olli Kärkkäinen
- Kati Hanhineva
An update to SPIRIT and CONSORT reporting guidelines to enhance transparency in randomized trials
Results from clinical trials can be deemed trustworthy only if they are properly conducted and their methods are fully reported. The SPIRIT and CONSORT checklists, which have improved clinical trial design, conduct and reporting, are being updated to reflect recent advances and improve the assessment of healthcare interventions.
- Sally Hopewell
- Isabelle Boutron
- David Moher
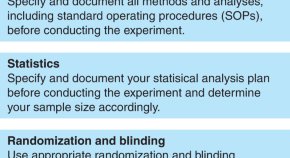
The EQIPD framework for rigor in the design, conduct, analysis and documentation of animal experiments
The EQIPD framework for rigor in animal experiments aims to unify current recommendations based on evidence behind their rationale and was prospectively tested for feasibility in multicenter animal experiments.
- Jan Vollert
- Malcolm Macleod
- Andrew S. C. Rice
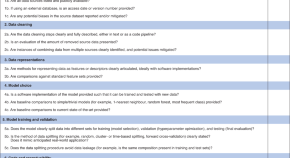
Best practices in machine learning for chemistry
Statistical tools based on machine learning are becoming integrated into chemistry research workflows. We discuss the elements necessary to train reliable, repeatable and reproducible models, and recommend a set of guidelines for machine learning reports.
- Nongnuch Artrith
- Keith T. Butler
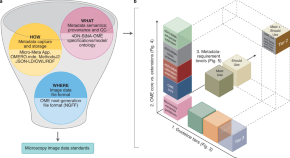
Towards community-driven metadata standards for light microscopy: tiered specifications extending the OME model
Rigorous record-keeping and quality control are required to ensure the quality, reproducibility and value of imaging data. The 4DN Initiative and BINA here propose light Microscopy Metadata Specifications that extend the OME Data Model, scale with experimental intent and complexity, and make it possible for scientists to create comprehensive records of imaging experiments.
- Mathias Hammer
- Maximiliaan Huisman
- Caterina Strambio-De-Castillia
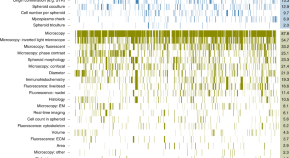
MISpheroID: a knowledgebase and transparency tool for minimum information in spheroid identity
A knowledgebase developed for increased the transparency of reporting in spheroid research.
- Arne Peirsman
- Eva Blondeel
- Olivier De Wever
Reproducibility standards for machine learning in the life sciences
To make machine-learning analyses in the life sciences more computationally reproducible, we propose standards based on data, model and code publication, programming best practices and workflow automation. By meeting these standards, the community of researchers applying machine-learning methods in the life sciences can ensure that their analyses are worthy of trust.
- Benjamin J. Heil
- Michael M. Hoffman
- Stephanie C. Hicks
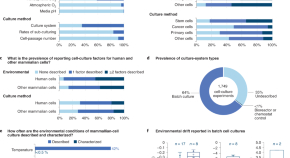
A prevalent neglect of environmental control in mammalian cell culture calls for best practices
In biomedical studies, the environmental conditions used in mammalian cell culture are often underreported, and are seldom monitored or controlled. Best-practice standards are urgently needed.
- Shannon G. Klein
- Samhan M. Alsolami
- Carlos M. Duarte
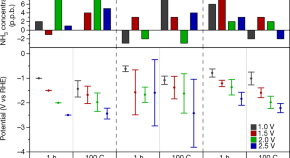
A rigorous electrochemical ammonia synthesis protocol with quantitative isotope measurements
A protocol for the electrochemical reduction of nitrogen to ammonia enables isotope-sensitive quantification of the ammonia produced and the identification and removal of contaminants.
- Suzanne Z. Andersen
- Viktor Čolić
- Ib Chorkendorff
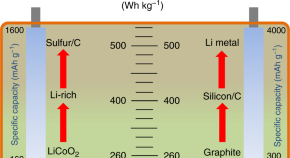
Aligning academia and industry for unified battery performance metrics
- Tiefeng Liu
- Chengdu Liang
Best practices in data analysis and sharing in neuroimaging using MRI
Responding to widespread concerns about reproducibility, the Organization for Human Brain Mapping created a working group to identify best practices in data analysis, results reporting and data sharing to promote open and reproducible research in neuroimaging. We describe the challenges of open research and the barriers the field faces.
- Thomas E Nichols
- B T Thomas Yeo
Reproducibility: changing the policies and culture of cell line authentication
Quality control of cell lines used in biomedical research is essential to ensure reproducibility. Although cell line authentication has been widely recommended for many years, misidentification, including cross-contamination, remains a serious problem. We outline a multi-stakeholder, incremental approach and policy-related recommendations to facilitate change in the culture of cell line authentication.
- Leonard P Freedman
- Mark C Gibson
- Yvonne A Reid
Improved reproducibility by assuring confidence in measurements in biomedical research
'Irreproducibility' is symptomatic of a broader challenge in measurement in biomedical research. From the US National Institute of Standards and Technology (NIST) perspective of rigorous metrology, reproducibility is only one aspect of establishing confidence in measurements. Appropriate controls, reference materials, statistics and informatics are required for a robust measurement process. Research is required to establish these tools for biological measurements, which will lead to greater confidence in research results.
- Anne L Plant
- Laurie E Locascio
- Patrick D Gallagher
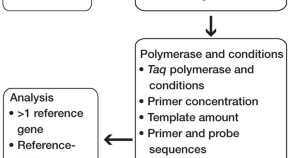
The need for transparency and good practices in the qPCR literature
Two surveys of over 1,700 publications whose authors use quantitative real-time PCR (qPCR) reveal a lack of transparent and comprehensive reporting of essential technical information. Reporting standards are significantly improved in publications that cite the Minimum Information for Publication of Quantitative Real-Time PCR Experiments (MIQE) guidelines, although such publications are still vastly outnumbered by those that do not.
- Stephen A Bustin
- Vladimir Benes
- Jo Vandesompele
Ethical reproducibility: towards transparent reporting in biomedical research
Optimism about biomedicine is challenged by the increasingly complex ethical, legal and social issues it raises. Reporting of scientific methods is no longer sufficient to address the complex relationship between science and society. To promote 'ethical reproducibility', we call for transparent reporting of research ethics methods used in biomedical research.
- James A Anderson
- Marleen Eijkholt
Quick links
- Explore articles by subject
- Guide to authors
- Editorial policies

- Privacy Policy
Buy Me a Coffee

Home » Research Methodology – Types, Examples and writing Guide
Research Methodology – Types, Examples and writing Guide
Table of Contents

Research Methodology
Definition:
Research Methodology refers to the systematic and scientific approach used to conduct research, investigate problems, and gather data and information for a specific purpose. It involves the techniques and procedures used to identify, collect , analyze , and interpret data to answer research questions or solve research problems . Moreover, They are philosophical and theoretical frameworks that guide the research process.
Structure of Research Methodology
Research methodology formats can vary depending on the specific requirements of the research project, but the following is a basic example of a structure for a research methodology section:
I. Introduction
- Provide an overview of the research problem and the need for a research methodology section
- Outline the main research questions and objectives
II. Research Design
- Explain the research design chosen and why it is appropriate for the research question(s) and objectives
- Discuss any alternative research designs considered and why they were not chosen
- Describe the research setting and participants (if applicable)
III. Data Collection Methods
- Describe the methods used to collect data (e.g., surveys, interviews, observations)
- Explain how the data collection methods were chosen and why they are appropriate for the research question(s) and objectives
- Detail any procedures or instruments used for data collection
IV. Data Analysis Methods
- Describe the methods used to analyze the data (e.g., statistical analysis, content analysis )
- Explain how the data analysis methods were chosen and why they are appropriate for the research question(s) and objectives
- Detail any procedures or software used for data analysis
V. Ethical Considerations
- Discuss any ethical issues that may arise from the research and how they were addressed
- Explain how informed consent was obtained (if applicable)
- Detail any measures taken to ensure confidentiality and anonymity
VI. Limitations
- Identify any potential limitations of the research methodology and how they may impact the results and conclusions
VII. Conclusion
- Summarize the key aspects of the research methodology section
- Explain how the research methodology addresses the research question(s) and objectives
Research Methodology Types
Types of Research Methodology are as follows:
Quantitative Research Methodology
This is a research methodology that involves the collection and analysis of numerical data using statistical methods. This type of research is often used to study cause-and-effect relationships and to make predictions.
Qualitative Research Methodology
This is a research methodology that involves the collection and analysis of non-numerical data such as words, images, and observations. This type of research is often used to explore complex phenomena, to gain an in-depth understanding of a particular topic, and to generate hypotheses.
Mixed-Methods Research Methodology
This is a research methodology that combines elements of both quantitative and qualitative research. This approach can be particularly useful for studies that aim to explore complex phenomena and to provide a more comprehensive understanding of a particular topic.
Case Study Research Methodology
This is a research methodology that involves in-depth examination of a single case or a small number of cases. Case studies are often used in psychology, sociology, and anthropology to gain a detailed understanding of a particular individual or group.
Action Research Methodology
This is a research methodology that involves a collaborative process between researchers and practitioners to identify and solve real-world problems. Action research is often used in education, healthcare, and social work.
Experimental Research Methodology
This is a research methodology that involves the manipulation of one or more independent variables to observe their effects on a dependent variable. Experimental research is often used to study cause-and-effect relationships and to make predictions.
Survey Research Methodology
This is a research methodology that involves the collection of data from a sample of individuals using questionnaires or interviews. Survey research is often used to study attitudes, opinions, and behaviors.
Grounded Theory Research Methodology
This is a research methodology that involves the development of theories based on the data collected during the research process. Grounded theory is often used in sociology and anthropology to generate theories about social phenomena.
Research Methodology Example
An Example of Research Methodology could be the following:
Research Methodology for Investigating the Effectiveness of Cognitive Behavioral Therapy in Reducing Symptoms of Depression in Adults
Introduction:
The aim of this research is to investigate the effectiveness of cognitive-behavioral therapy (CBT) in reducing symptoms of depression in adults. To achieve this objective, a randomized controlled trial (RCT) will be conducted using a mixed-methods approach.
Research Design:
The study will follow a pre-test and post-test design with two groups: an experimental group receiving CBT and a control group receiving no intervention. The study will also include a qualitative component, in which semi-structured interviews will be conducted with a subset of participants to explore their experiences of receiving CBT.
Participants:
Participants will be recruited from community mental health clinics in the local area. The sample will consist of 100 adults aged 18-65 years old who meet the diagnostic criteria for major depressive disorder. Participants will be randomly assigned to either the experimental group or the control group.
Intervention :
The experimental group will receive 12 weekly sessions of CBT, each lasting 60 minutes. The intervention will be delivered by licensed mental health professionals who have been trained in CBT. The control group will receive no intervention during the study period.
Data Collection:
Quantitative data will be collected through the use of standardized measures such as the Beck Depression Inventory-II (BDI-II) and the Generalized Anxiety Disorder-7 (GAD-7). Data will be collected at baseline, immediately after the intervention, and at a 3-month follow-up. Qualitative data will be collected through semi-structured interviews with a subset of participants from the experimental group. The interviews will be conducted at the end of the intervention period, and will explore participants’ experiences of receiving CBT.
Data Analysis:
Quantitative data will be analyzed using descriptive statistics, t-tests, and mixed-model analyses of variance (ANOVA) to assess the effectiveness of the intervention. Qualitative data will be analyzed using thematic analysis to identify common themes and patterns in participants’ experiences of receiving CBT.
Ethical Considerations:
This study will comply with ethical guidelines for research involving human subjects. Participants will provide informed consent before participating in the study, and their privacy and confidentiality will be protected throughout the study. Any adverse events or reactions will be reported and managed appropriately.
Data Management:
All data collected will be kept confidential and stored securely using password-protected databases. Identifying information will be removed from qualitative data transcripts to ensure participants’ anonymity.
Limitations:
One potential limitation of this study is that it only focuses on one type of psychotherapy, CBT, and may not generalize to other types of therapy or interventions. Another limitation is that the study will only include participants from community mental health clinics, which may not be representative of the general population.
Conclusion:
This research aims to investigate the effectiveness of CBT in reducing symptoms of depression in adults. By using a randomized controlled trial and a mixed-methods approach, the study will provide valuable insights into the mechanisms underlying the relationship between CBT and depression. The results of this study will have important implications for the development of effective treatments for depression in clinical settings.
How to Write Research Methodology
Writing a research methodology involves explaining the methods and techniques you used to conduct research, collect data, and analyze results. It’s an essential section of any research paper or thesis, as it helps readers understand the validity and reliability of your findings. Here are the steps to write a research methodology:
- Start by explaining your research question: Begin the methodology section by restating your research question and explaining why it’s important. This helps readers understand the purpose of your research and the rationale behind your methods.
- Describe your research design: Explain the overall approach you used to conduct research. This could be a qualitative or quantitative research design, experimental or non-experimental, case study or survey, etc. Discuss the advantages and limitations of the chosen design.
- Discuss your sample: Describe the participants or subjects you included in your study. Include details such as their demographics, sampling method, sample size, and any exclusion criteria used.
- Describe your data collection methods : Explain how you collected data from your participants. This could include surveys, interviews, observations, questionnaires, or experiments. Include details on how you obtained informed consent, how you administered the tools, and how you minimized the risk of bias.
- Explain your data analysis techniques: Describe the methods you used to analyze the data you collected. This could include statistical analysis, content analysis, thematic analysis, or discourse analysis. Explain how you dealt with missing data, outliers, and any other issues that arose during the analysis.
- Discuss the validity and reliability of your research : Explain how you ensured the validity and reliability of your study. This could include measures such as triangulation, member checking, peer review, or inter-coder reliability.
- Acknowledge any limitations of your research: Discuss any limitations of your study, including any potential threats to validity or generalizability. This helps readers understand the scope of your findings and how they might apply to other contexts.
- Provide a summary: End the methodology section by summarizing the methods and techniques you used to conduct your research. This provides a clear overview of your research methodology and helps readers understand the process you followed to arrive at your findings.
When to Write Research Methodology
Research methodology is typically written after the research proposal has been approved and before the actual research is conducted. It should be written prior to data collection and analysis, as it provides a clear roadmap for the research project.
The research methodology is an important section of any research paper or thesis, as it describes the methods and procedures that will be used to conduct the research. It should include details about the research design, data collection methods, data analysis techniques, and any ethical considerations.
The methodology should be written in a clear and concise manner, and it should be based on established research practices and standards. It is important to provide enough detail so that the reader can understand how the research was conducted and evaluate the validity of the results.
Applications of Research Methodology
Here are some of the applications of research methodology:
- To identify the research problem: Research methodology is used to identify the research problem, which is the first step in conducting any research.
- To design the research: Research methodology helps in designing the research by selecting the appropriate research method, research design, and sampling technique.
- To collect data: Research methodology provides a systematic approach to collect data from primary and secondary sources.
- To analyze data: Research methodology helps in analyzing the collected data using various statistical and non-statistical techniques.
- To test hypotheses: Research methodology provides a framework for testing hypotheses and drawing conclusions based on the analysis of data.
- To generalize findings: Research methodology helps in generalizing the findings of the research to the target population.
- To develop theories : Research methodology is used to develop new theories and modify existing theories based on the findings of the research.
- To evaluate programs and policies : Research methodology is used to evaluate the effectiveness of programs and policies by collecting data and analyzing it.
- To improve decision-making: Research methodology helps in making informed decisions by providing reliable and valid data.
Purpose of Research Methodology
Research methodology serves several important purposes, including:
- To guide the research process: Research methodology provides a systematic framework for conducting research. It helps researchers to plan their research, define their research questions, and select appropriate methods and techniques for collecting and analyzing data.
- To ensure research quality: Research methodology helps researchers to ensure that their research is rigorous, reliable, and valid. It provides guidelines for minimizing bias and error in data collection and analysis, and for ensuring that research findings are accurate and trustworthy.
- To replicate research: Research methodology provides a clear and detailed account of the research process, making it possible for other researchers to replicate the study and verify its findings.
- To advance knowledge: Research methodology enables researchers to generate new knowledge and to contribute to the body of knowledge in their field. It provides a means for testing hypotheses, exploring new ideas, and discovering new insights.
- To inform decision-making: Research methodology provides evidence-based information that can inform policy and decision-making in a variety of fields, including medicine, public health, education, and business.
Advantages of Research Methodology
Research methodology has several advantages that make it a valuable tool for conducting research in various fields. Here are some of the key advantages of research methodology:
- Systematic and structured approach : Research methodology provides a systematic and structured approach to conducting research, which ensures that the research is conducted in a rigorous and comprehensive manner.
- Objectivity : Research methodology aims to ensure objectivity in the research process, which means that the research findings are based on evidence and not influenced by personal bias or subjective opinions.
- Replicability : Research methodology ensures that research can be replicated by other researchers, which is essential for validating research findings and ensuring their accuracy.
- Reliability : Research methodology aims to ensure that the research findings are reliable, which means that they are consistent and can be depended upon.
- Validity : Research methodology ensures that the research findings are valid, which means that they accurately reflect the research question or hypothesis being tested.
- Efficiency : Research methodology provides a structured and efficient way of conducting research, which helps to save time and resources.
- Flexibility : Research methodology allows researchers to choose the most appropriate research methods and techniques based on the research question, data availability, and other relevant factors.
- Scope for innovation: Research methodology provides scope for innovation and creativity in designing research studies and developing new research techniques.
Research Methodology Vs Research Methods
About the author.
Muhammad Hassan
Researcher, Academic Writer, Web developer
You may also like

How to Cite Research Paper – All Formats and...

Data Collection – Methods Types and Examples

Delimitations in Research – Types, Examples and...

Research Paper Format – Types, Examples and...

Research Process – Steps, Examples and Tips

Research Design – Types, Methods and Examples
Research report guide: Definition, types, and tips
Last updated
5 March 2024
Reviewed by
From successful product launches or software releases to planning major business decisions, research reports serve many vital functions. They can summarize evidence and deliver insights and recommendations to save companies time and resources. They can reveal the most value-adding actions a company should take.
However, poorly constructed reports can have the opposite effect! Taking the time to learn established research-reporting rules and approaches will equip you with in-demand skills. You’ll be able to capture and communicate information applicable to numerous situations and industries, adding another string to your resume bow.
- What are research reports?
A research report is a collection of contextual data, gathered through organized research, that provides new insights into a particular challenge (which, for this article, is business-related). Research reports are a time-tested method for distilling large amounts of data into a narrow band of focus.
Their effectiveness often hinges on whether the report provides:
Strong, well-researched evidence
Comprehensive analysis
Well-considered conclusions and recommendations
Though the topic possibilities are endless, an effective research report keeps a laser-like focus on the specific questions or objectives the researcher believes are key to achieving success. Many research reports begin as research proposals, which usually include the need for a report to capture the findings of the study and recommend a course of action.
A description of the research method used, e.g., qualitative, quantitative, or other
Statistical analysis
Causal (or explanatory) research (i.e., research identifying relationships between two variables)
Inductive research, also known as ‘theory-building’
Deductive research, such as that used to test theories
Action research, where the research is actively used to drive change
- Importance of a research report
Research reports can unify and direct a company's focus toward the most appropriate strategic action. Of course, spending resources on a report takes up some of the company's human and financial resources. Choosing when a report is called for is a matter of judgment and experience.
Some development models used heavily in the engineering world, such as Waterfall development, are notorious for over-relying on research reports. With Waterfall development, there is a linear progression through each step of a project, and each stage is precisely documented and reported on before moving to the next.
The pace of the business world is faster than the speed at which your authors can produce and disseminate reports. So how do companies strike the right balance between creating and acting on research reports?
The answer lies, again, in the report's defined objectives. By paring down your most pressing interests and those of your stakeholders, your research and reporting skills will be the lenses that keep your company's priorities in constant focus.
Honing your company's primary objectives can save significant amounts of time and align research and reporting efforts with ever-greater precision.
Some examples of well-designed research objectives are:
Proving whether or not a product or service meets customer expectations
Demonstrating the value of a service, product, or business process to your stakeholders and investors
Improving business decision-making when faced with a lack of time or other constraints
Clarifying the relationship between a critical cause and effect for problematic business processes
Prioritizing the development of a backlog of products or product features
Comparing business or production strategies
Evaluating past decisions and predicting future outcomes
- Features of a research report
Research reports generally require a research design phase, where the report author(s) determine the most important elements the report must contain.
Just as there are various kinds of research, there are many types of reports.
Here are the standard elements of almost any research-reporting format:
Report summary. A broad but comprehensive overview of what readers will learn in the full report. Summaries are usually no more than one or two paragraphs and address all key elements of the report. Think of the key takeaways your primary stakeholders will want to know if they don’t have time to read the full document.
Introduction. Include a brief background of the topic, the type of research, and the research sample. Consider the primary goal of the report, who is most affected, and how far along the company is in meeting its objectives.
Methods. A description of how the researcher carried out data collection, analysis, and final interpretations of the data. Include the reasons for choosing a particular method. The methods section should strike a balance between clearly presenting the approach taken to gather data and discussing how it is designed to achieve the report's objectives.
Data analysis. This section contains interpretations that lead readers through the results relevant to the report's thesis. If there were unexpected results, include here a discussion on why that might be. Charts, calculations, statistics, and other supporting information also belong here (or, if lengthy, as an appendix). This should be the most detailed section of the research report, with references for further study. Present the information in a logical order, whether chronologically or in order of importance to the report's objectives.
Conclusion. This should be written with sound reasoning, often containing useful recommendations. The conclusion must be backed by a continuous thread of logic throughout the report.
- How to write a research paper
With a clear outline and robust pool of research, a research paper can start to write itself, but what's a good way to start a research report?
Research report examples are often the quickest way to gain inspiration for your report. Look for the types of research reports most relevant to your industry and consider which makes the most sense for your data and goals.
The research report outline will help you organize the elements of your report. One of the most time-tested report outlines is the IMRaD structure:
Introduction
...and Discussion
Pay close attention to the most well-established research reporting format in your industry, and consider your tone and language from your audience's perspective. Learn the key terms inside and out; incorrect jargon could easily harm the perceived authority of your research paper.
Along with a foundation in high-quality research and razor-sharp analysis, the most effective research reports will also demonstrate well-developed:
Internal logic
Narrative flow
Conclusions and recommendations
Readability, striking a balance between simple phrasing and technical insight
How to gather research data for your report
The validity of research data is critical. Because the research phase usually occurs well before the writing phase, you normally have plenty of time to vet your data.
However, research reports could involve ongoing research, where report authors (sometimes the researchers themselves) write portions of the report alongside ongoing research.
One such research-report example would be an R&D department that knows its primary stakeholders are eager to learn about a lengthy work in progress and any potentially important outcomes.
However you choose to manage the research and reporting, your data must meet robust quality standards before you can rely on it. Vet any research with the following questions in mind:
Does it use statistically valid analysis methods?
Do the researchers clearly explain their research, analysis, and sampling methods?
Did the researchers provide any caveats or advice on how to interpret their data?
Have you gathered the data yourself or were you in close contact with those who did?
Is the source biased?
Usually, flawed research methods become more apparent the further you get through a research report.
It's perfectly natural for good research to raise new questions, but the reader should have no uncertainty about what the data represents. There should be no doubt about matters such as:
Whether the sampling or analysis methods were based on sound and consistent logic
What the research samples are and where they came from
The accuracy of any statistical functions or equations
Validation of testing and measuring processes
When does a report require design validation?
A robust design validation process is often a gold standard in highly technical research reports. Design validation ensures the objects of a study are measured accurately, which lends more weight to your report and makes it valuable to more specialized industries.
Product development and engineering projects are the most common research-report examples that typically involve a design validation process. Depending on the scope and complexity of your research, you might face additional steps to validate your data and research procedures.
If you’re including design validation in the report (or report proposal), explain and justify your data-collection processes. Good design validation builds greater trust in a research report and lends more weight to its conclusions.
Choosing the right analysis method
Just as the quality of your report depends on properly validated research, a useful conclusion requires the most contextually relevant analysis method. This means comparing different statistical methods and choosing the one that makes the most sense for your research.
Most broadly, research analysis comes down to quantitative or qualitative methods (respectively: measurable by a number vs subjectively qualified values). There are also mixed research methods, which bridge the need for merging hard data with qualified assessments and still reach a cohesive set of conclusions.
Some of the most common analysis methods in research reports include:
Significance testing (aka hypothesis analysis), which compares test and control groups to determine how likely the data was the result of random chance.
Regression analysis , to establish relationships between variables, control for extraneous variables , and support correlation analysis.
Correlation analysis (aka bivariate testing), a method to identify and determine the strength of linear relationships between variables. It’s effective for detecting patterns from complex data, but care must be exercised to not confuse correlation with causation.
With any analysis method, it's important to justify which method you chose in the report. You should also provide estimates of the statistical accuracy (e.g., the p-value or confidence level of quantifiable data) of any data analysis.
This requires a commitment to the report's primary aim. For instance, this may be achieving a certain level of customer satisfaction by analyzing the cause and effect of changes to how service is delivered. Even better, use statistical analysis to calculate which change is most positively correlated with improved levels of customer satisfaction.
- Tips for writing research reports
There's endless good advice for writing effective research reports, and it almost all depends on the subjective aims of the people behind the report. Due to the wide variety of research reports, the best tips will be unique to each author's purpose.
Consider the following research report tips in any order, and take note of the ones most relevant to you:
No matter how in depth or detailed your report might be, provide a well-considered, succinct summary. At the very least, give your readers a quick and effective way to get up to speed.
Pare down your target audience (e.g., other researchers, employees, laypersons, etc.), and adjust your voice for their background knowledge and interest levels
For all but the most open-ended research, clarify your objectives, both for yourself and within the report.
Leverage your team members’ talents to fill in any knowledge gaps you might have. Your team is only as good as the sum of its parts.
Justify why your research proposal’s topic will endure long enough to derive value from the finished report.
Consolidate all research and analysis functions onto a single user-friendly platform. There's no reason to settle for less than developer-grade tools suitable for non-developers.
What's the format of a research report?
The research-reporting format is how the report is structured—a framework the authors use to organize their data, conclusions, arguments, and recommendations. The format heavily determines how the report's outline develops, because the format dictates the overall structure and order of information (based on the report's goals and research objectives).
What's the purpose of a research-report outline?
A good report outline gives form and substance to the report's objectives, presenting the results in a readable, engaging way. For any research-report format, the outline should create momentum along a chain of logic that builds up to a conclusion or interpretation.
What's the difference between a research essay and a research report?
There are several key differences between research reports and essays:
Research report:
Ordered into separate sections
More commercial in nature
Often includes infographics
Heavily descriptive
More self-referential
Usually provides recommendations
Research essay
Does not rely on research report formatting
More academically minded
Normally text-only
Less detailed
Omits discussion of methods
Usually non-prescriptive
Get started today
Go from raw data to valuable insights with a flexible research platform
Editor’s picks
Last updated: 21 December 2023
Last updated: 16 December 2023
Last updated: 6 October 2023
Last updated: 5 March 2024
Last updated: 25 November 2023
Last updated: 15 February 2024
Last updated: 11 March 2024
Last updated: 12 December 2023
Last updated: 6 March 2024
Last updated: 10 April 2023
Last updated: 20 December 2023
Latest articles
Related topics, log in or sign up.
Get started for free
Research Methods In Psychology
Saul Mcleod, PhD
Editor-in-Chief for Simply Psychology
BSc (Hons) Psychology, MRes, PhD, University of Manchester
Saul Mcleod, PhD., is a qualified psychology teacher with over 18 years of experience in further and higher education. He has been published in peer-reviewed journals, including the Journal of Clinical Psychology.
Learn about our Editorial Process
Olivia Guy-Evans, MSc
Associate Editor for Simply Psychology
BSc (Hons) Psychology, MSc Psychology of Education
Olivia Guy-Evans is a writer and associate editor for Simply Psychology. She has previously worked in healthcare and educational sectors.
Research methods in psychology are systematic procedures used to observe, describe, predict, and explain behavior and mental processes. They include experiments, surveys, case studies, and naturalistic observations, ensuring data collection is objective and reliable to understand and explain psychological phenomena.
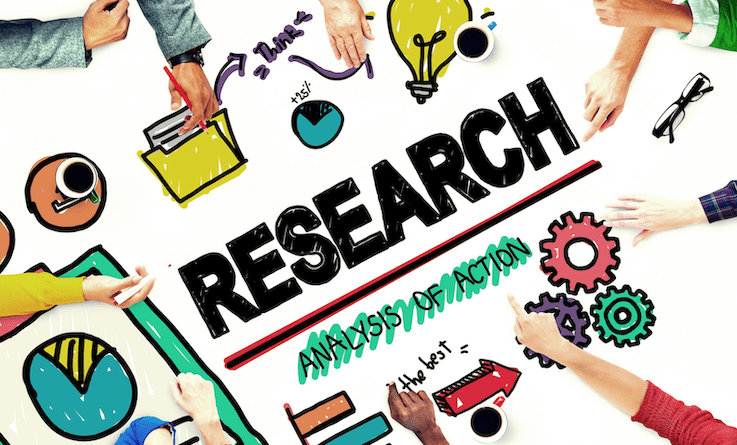
Hypotheses are statements about the prediction of the results, that can be verified or disproved by some investigation.
There are four types of hypotheses :
- Null Hypotheses (H0 ) – these predict that no difference will be found in the results between the conditions. Typically these are written ‘There will be no difference…’
- Alternative Hypotheses (Ha or H1) – these predict that there will be a significant difference in the results between the two conditions. This is also known as the experimental hypothesis.
- One-tailed (directional) hypotheses – these state the specific direction the researcher expects the results to move in, e.g. higher, lower, more, less. In a correlation study, the predicted direction of the correlation can be either positive or negative.
- Two-tailed (non-directional) hypotheses – these state that a difference will be found between the conditions of the independent variable but does not state the direction of a difference or relationship. Typically these are always written ‘There will be a difference ….’
All research has an alternative hypothesis (either a one-tailed or two-tailed) and a corresponding null hypothesis.
Once the research is conducted and results are found, psychologists must accept one hypothesis and reject the other.
So, if a difference is found, the Psychologist would accept the alternative hypothesis and reject the null. The opposite applies if no difference is found.
Sampling techniques
Sampling is the process of selecting a representative group from the population under study.
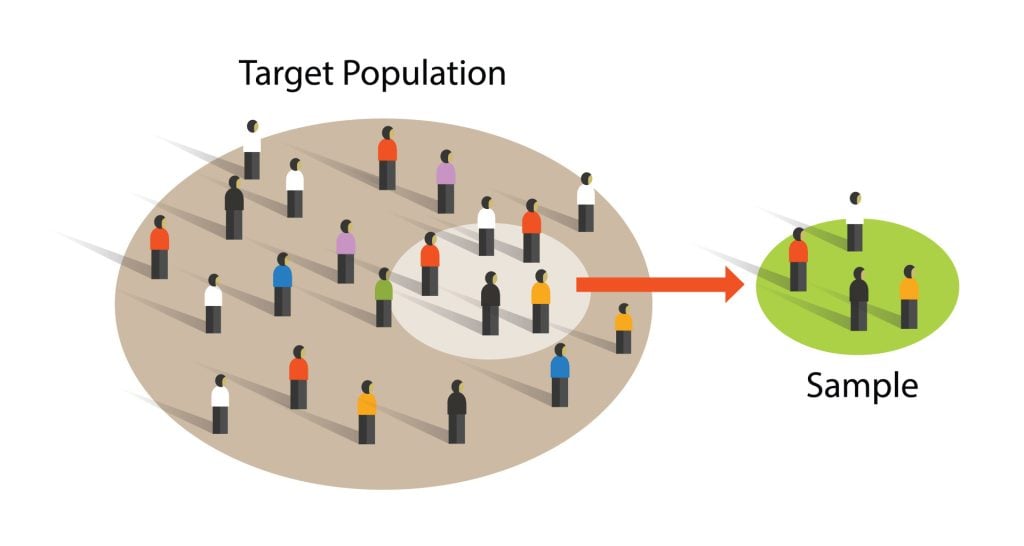
A sample is the participants you select from a target population (the group you are interested in) to make generalizations about.
Representative means the extent to which a sample mirrors a researcher’s target population and reflects its characteristics.
Generalisability means the extent to which their findings can be applied to the larger population of which their sample was a part.
- Volunteer sample : where participants pick themselves through newspaper adverts, noticeboards or online.
- Opportunity sampling : also known as convenience sampling , uses people who are available at the time the study is carried out and willing to take part. It is based on convenience.
- Random sampling : when every person in the target population has an equal chance of being selected. An example of random sampling would be picking names out of a hat.
- Systematic sampling : when a system is used to select participants. Picking every Nth person from all possible participants. N = the number of people in the research population / the number of people needed for the sample.
- Stratified sampling : when you identify the subgroups and select participants in proportion to their occurrences.
- Snowball sampling : when researchers find a few participants, and then ask them to find participants themselves and so on.
- Quota sampling : when researchers will be told to ensure the sample fits certain quotas, for example they might be told to find 90 participants, with 30 of them being unemployed.
Experiments always have an independent and dependent variable .
- The independent variable is the one the experimenter manipulates (the thing that changes between the conditions the participants are placed into). It is assumed to have a direct effect on the dependent variable.
- The dependent variable is the thing being measured, or the results of the experiment.
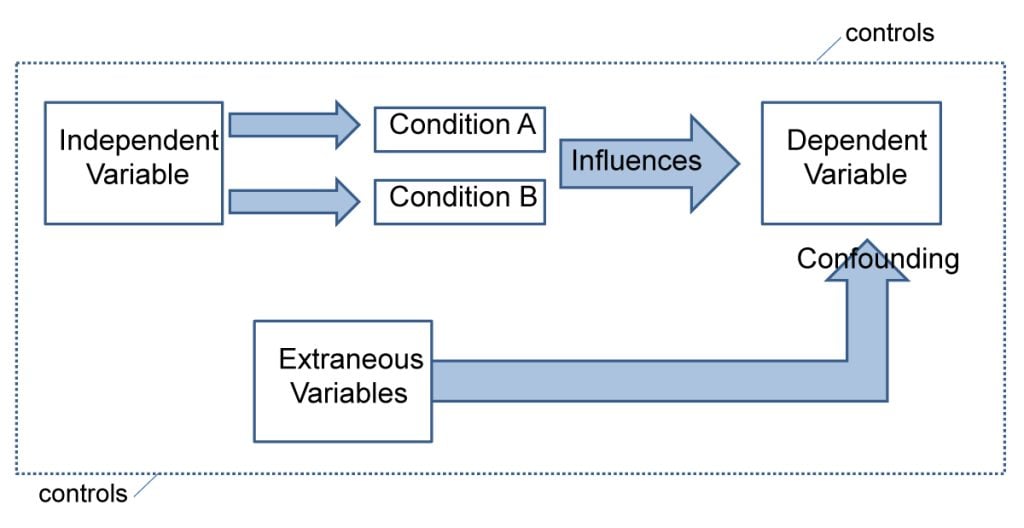
Operationalization of variables means making them measurable/quantifiable. We must use operationalization to ensure that variables are in a form that can be easily tested.
For instance, we can’t really measure ‘happiness’, but we can measure how many times a person smiles within a two-hour period.
By operationalizing variables, we make it easy for someone else to replicate our research. Remember, this is important because we can check if our findings are reliable.
Extraneous variables are all variables which are not independent variable but could affect the results of the experiment.
It can be a natural characteristic of the participant, such as intelligence levels, gender, or age for example, or it could be a situational feature of the environment such as lighting or noise.
Demand characteristics are a type of extraneous variable that occurs if the participants work out the aims of the research study, they may begin to behave in a certain way.
For example, in Milgram’s research , critics argued that participants worked out that the shocks were not real and they administered them as they thought this was what was required of them.
Extraneous variables must be controlled so that they do not affect (confound) the results.
Randomly allocating participants to their conditions or using a matched pairs experimental design can help to reduce participant variables.
Situational variables are controlled by using standardized procedures, ensuring every participant in a given condition is treated in the same way
Experimental Design
Experimental design refers to how participants are allocated to each condition of the independent variable, such as a control or experimental group.
- Independent design ( between-groups design ): each participant is selected for only one group. With the independent design, the most common way of deciding which participants go into which group is by means of randomization.
- Matched participants design : each participant is selected for only one group, but the participants in the two groups are matched for some relevant factor or factors (e.g. ability; sex; age).
- Repeated measures design ( within groups) : each participant appears in both groups, so that there are exactly the same participants in each group.
- The main problem with the repeated measures design is that there may well be order effects. Their experiences during the experiment may change the participants in various ways.
- They may perform better when they appear in the second group because they have gained useful information about the experiment or about the task. On the other hand, they may perform less well on the second occasion because of tiredness or boredom.
- Counterbalancing is the best way of preventing order effects from disrupting the findings of an experiment, and involves ensuring that each condition is equally likely to be used first and second by the participants.
If we wish to compare two groups with respect to a given independent variable, it is essential to make sure that the two groups do not differ in any other important way.
Experimental Methods
All experimental methods involve an iv (independent variable) and dv (dependent variable)..
- Field experiments are conducted in the everyday (natural) environment of the participants. The experimenter still manipulates the IV, but in a real-life setting. It may be possible to control extraneous variables, though such control is more difficult than in a lab experiment.
- Natural experiments are when a naturally occurring IV is investigated that isn’t deliberately manipulated, it exists anyway. Participants are not randomly allocated, and the natural event may only occur rarely.
Case studies are in-depth investigations of a person, group, event, or community. It uses information from a range of sources, such as from the person concerned and also from their family and friends.
Many techniques may be used such as interviews, psychological tests, observations and experiments. Case studies are generally longitudinal: in other words, they follow the individual or group over an extended period of time.
Case studies are widely used in psychology and among the best-known ones carried out were by Sigmund Freud . He conducted very detailed investigations into the private lives of his patients in an attempt to both understand and help them overcome their illnesses.
Case studies provide rich qualitative data and have high levels of ecological validity. However, it is difficult to generalize from individual cases as each one has unique characteristics.
Correlational Studies
Correlation means association; it is a measure of the extent to which two variables are related. One of the variables can be regarded as the predictor variable with the other one as the outcome variable.
Correlational studies typically involve obtaining two different measures from a group of participants, and then assessing the degree of association between the measures.
The predictor variable can be seen as occurring before the outcome variable in some sense. It is called the predictor variable, because it forms the basis for predicting the value of the outcome variable.
Relationships between variables can be displayed on a graph or as a numerical score called a correlation coefficient.
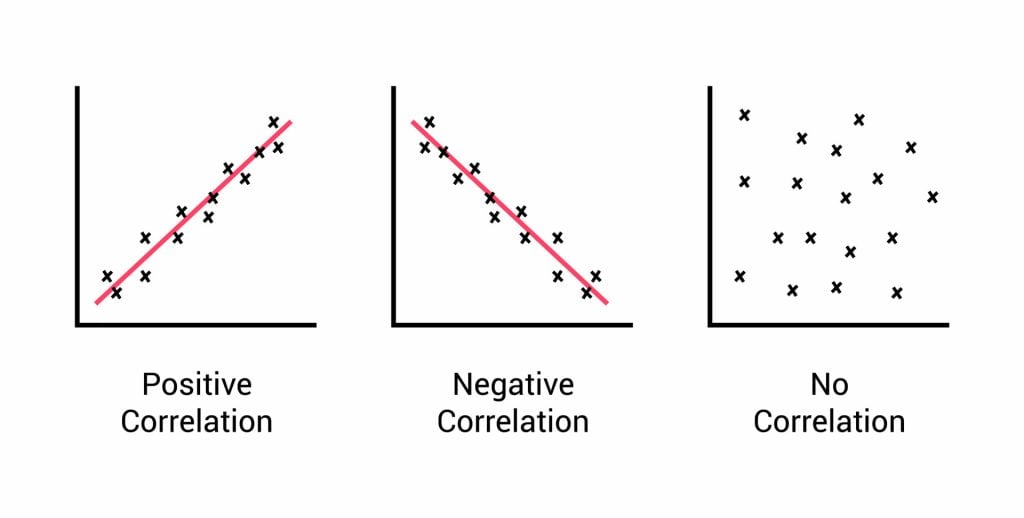
- If an increase in one variable tends to be associated with an increase in the other, then this is known as a positive correlation .
- If an increase in one variable tends to be associated with a decrease in the other, then this is known as a negative correlation .
- A zero correlation occurs when there is no relationship between variables.
After looking at the scattergraph, if we want to be sure that a significant relationship does exist between the two variables, a statistical test of correlation can be conducted, such as Spearman’s rho.
The test will give us a score, called a correlation coefficient . This is a value between 0 and 1, and the closer to 1 the score is, the stronger the relationship between the variables. This value can be both positive e.g. 0.63, or negative -0.63.
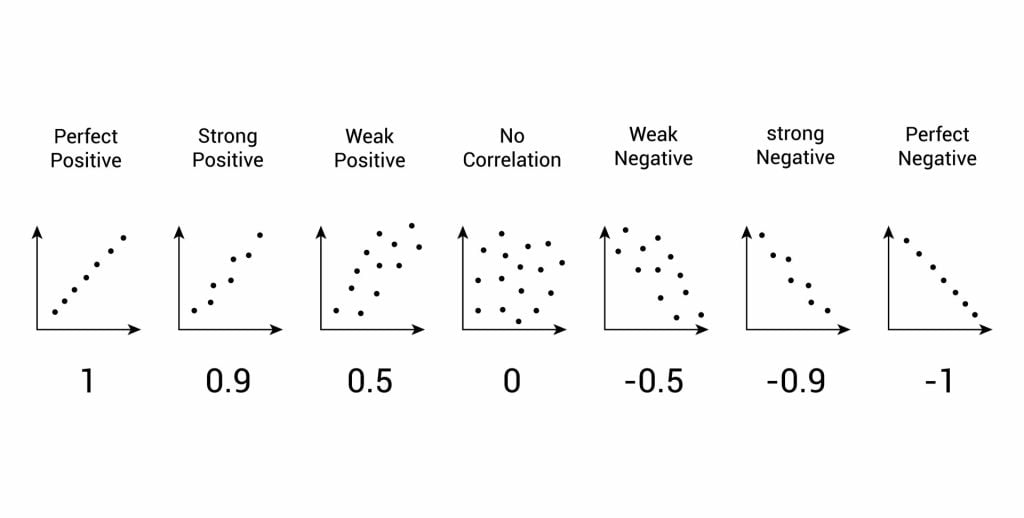
A correlation between variables, however, does not automatically mean that the change in one variable is the cause of the change in the values of the other variable. A correlation only shows if there is a relationship between variables.
Correlation does not always prove causation, as a third variable may be involved.
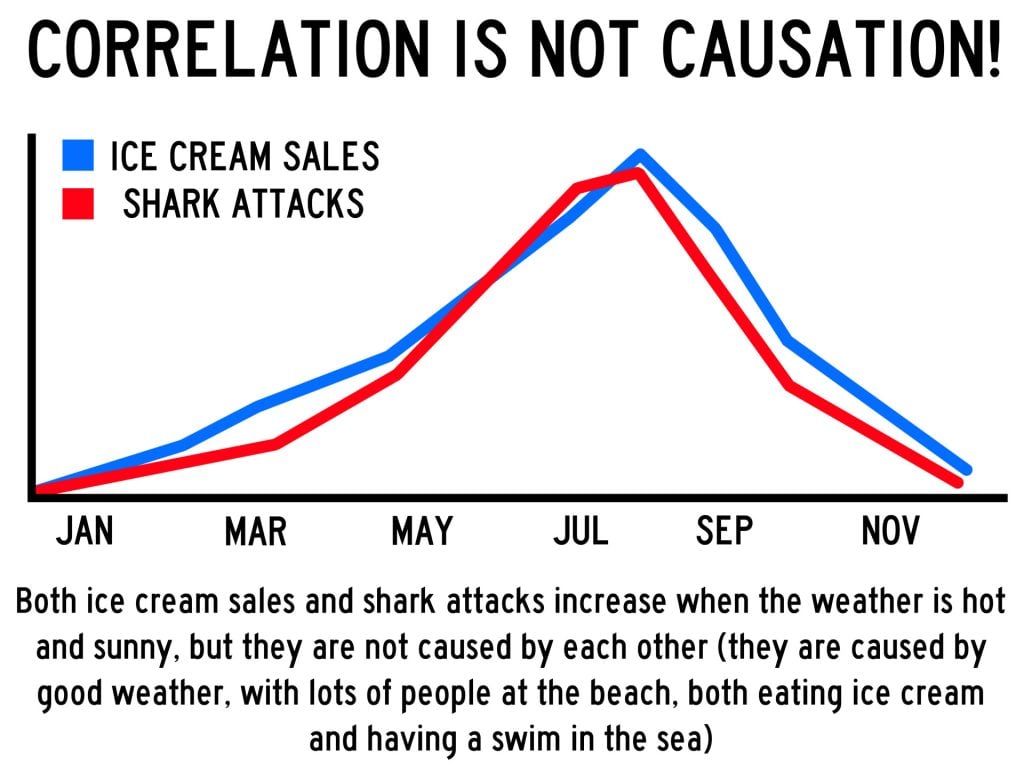
Interview Methods
Interviews are commonly divided into two types: structured and unstructured.
A fixed, predetermined set of questions is put to every participant in the same order and in the same way.
Responses are recorded on a questionnaire, and the researcher presets the order and wording of questions, and sometimes the range of alternative answers.
The interviewer stays within their role and maintains social distance from the interviewee.
There are no set questions, and the participant can raise whatever topics he/she feels are relevant and ask them in their own way. Questions are posed about participants’ answers to the subject
Unstructured interviews are most useful in qualitative research to analyze attitudes and values.
Though they rarely provide a valid basis for generalization, their main advantage is that they enable the researcher to probe social actors’ subjective point of view.
Questionnaire Method
Questionnaires can be thought of as a kind of written interview. They can be carried out face to face, by telephone, or post.
The choice of questions is important because of the need to avoid bias or ambiguity in the questions, ‘leading’ the respondent or causing offense.
- Open questions are designed to encourage a full, meaningful answer using the subject’s own knowledge and feelings. They provide insights into feelings, opinions, and understanding. Example: “How do you feel about that situation?”
- Closed questions can be answered with a simple “yes” or “no” or specific information, limiting the depth of response. They are useful for gathering specific facts or confirming details. Example: “Do you feel anxious in crowds?”
Its other practical advantages are that it is cheaper than face-to-face interviews and can be used to contact many respondents scattered over a wide area relatively quickly.
Observations
There are different types of observation methods :
- Covert observation is where the researcher doesn’t tell the participants they are being observed until after the study is complete. There could be ethical problems or deception and consent with this particular observation method.
- Overt observation is where a researcher tells the participants they are being observed and what they are being observed for.
- Controlled : behavior is observed under controlled laboratory conditions (e.g., Bandura’s Bobo doll study).
- Natural : Here, spontaneous behavior is recorded in a natural setting.
- Participant : Here, the observer has direct contact with the group of people they are observing. The researcher becomes a member of the group they are researching.
- Non-participant (aka “fly on the wall): The researcher does not have direct contact with the people being observed. The observation of participants’ behavior is from a distance
Pilot Study
A pilot study is a small scale preliminary study conducted in order to evaluate the feasibility of the key s teps in a future, full-scale project.
A pilot study is an initial run-through of the procedures to be used in an investigation; it involves selecting a few people and trying out the study on them. It is possible to save time, and in some cases, money, by identifying any flaws in the procedures designed by the researcher.
A pilot study can help the researcher spot any ambiguities (i.e. unusual things) or confusion in the information given to participants or problems with the task devised.
Sometimes the task is too hard, and the researcher may get a floor effect, because none of the participants can score at all or can complete the task – all performances are low.
The opposite effect is a ceiling effect, when the task is so easy that all achieve virtually full marks or top performances and are “hitting the ceiling”.
Research Design
In cross-sectional research , a researcher compares multiple segments of the population at the same time
Sometimes, we want to see how people change over time, as in studies of human development and lifespan. Longitudinal research is a research design in which data-gathering is administered repeatedly over an extended period of time.
In cohort studies , the participants must share a common factor or characteristic such as age, demographic, or occupation. A cohort study is a type of longitudinal study in which researchers monitor and observe a chosen population over an extended period.
Triangulation means using more than one research method to improve the study’s validity.
Reliability
Reliability is a measure of consistency, if a particular measurement is repeated and the same result is obtained then it is described as being reliable.
- Test-retest reliability : assessing the same person on two different occasions which shows the extent to which the test produces the same answers.
- Inter-observer reliability : the extent to which there is an agreement between two or more observers.
Meta-Analysis
A meta-analysis is a systematic review that involves identifying an aim and then searching for research studies that have addressed similar aims/hypotheses.
This is done by looking through various databases, and then decisions are made about what studies are to be included/excluded.
Strengths: Increases the conclusions’ validity as they’re based on a wider range.
Weaknesses: Research designs in studies can vary, so they are not truly comparable.
Peer Review
A researcher submits an article to a journal. The choice of the journal may be determined by the journal’s audience or prestige.
The journal selects two or more appropriate experts (psychologists working in a similar field) to peer review the article without payment. The peer reviewers assess: the methods and designs used, originality of the findings, the validity of the original research findings and its content, structure and language.
Feedback from the reviewer determines whether the article is accepted. The article may be: Accepted as it is, accepted with revisions, sent back to the author to revise and re-submit or rejected without the possibility of submission.
The editor makes the final decision whether to accept or reject the research report based on the reviewers comments/ recommendations.
Peer review is important because it prevent faulty data from entering the public domain, it provides a way of checking the validity of findings and the quality of the methodology and is used to assess the research rating of university departments.
Peer reviews may be an ideal, whereas in practice there are lots of problems. For example, it slows publication down and may prevent unusual, new work being published. Some reviewers might use it as an opportunity to prevent competing researchers from publishing work.
Some people doubt whether peer review can really prevent the publication of fraudulent research.
The advent of the internet means that a lot of research and academic comment is being published without official peer reviews than before, though systems are evolving on the internet where everyone really has a chance to offer their opinions and police the quality of research.
Types of Data
- Quantitative data is numerical data e.g. reaction time or number of mistakes. It represents how much or how long, how many there are of something. A tally of behavioral categories and closed questions in a questionnaire collect quantitative data.
- Qualitative data is virtually any type of information that can be observed and recorded that is not numerical in nature and can be in the form of written or verbal communication. Open questions in questionnaires and accounts from observational studies collect qualitative data.
- Primary data is first-hand data collected for the purpose of the investigation.
- Secondary data is information that has been collected by someone other than the person who is conducting the research e.g. taken from journals, books or articles.
Validity means how well a piece of research actually measures what it sets out to, or how well it reflects the reality it claims to represent.
Validity is whether the observed effect is genuine and represents what is actually out there in the world.
- Concurrent validity is the extent to which a psychological measure relates to an existing similar measure and obtains close results. For example, a new intelligence test compared to an established test.
- Face validity : does the test measure what it’s supposed to measure ‘on the face of it’. This is done by ‘eyeballing’ the measuring or by passing it to an expert to check.
- Ecological validit y is the extent to which findings from a research study can be generalized to other settings / real life.
- Temporal validity is the extent to which findings from a research study can be generalized to other historical times.
Features of Science
- Paradigm – A set of shared assumptions and agreed methods within a scientific discipline.
- Paradigm shift – The result of the scientific revolution: a significant change in the dominant unifying theory within a scientific discipline.
- Objectivity – When all sources of personal bias are minimised so not to distort or influence the research process.
- Empirical method – Scientific approaches that are based on the gathering of evidence through direct observation and experience.
- Replicability – The extent to which scientific procedures and findings can be repeated by other researchers.
- Falsifiability – The principle that a theory cannot be considered scientific unless it admits the possibility of being proved untrue.
Statistical Testing
A significant result is one where there is a low probability that chance factors were responsible for any observed difference, correlation, or association in the variables tested.
If our test is significant, we can reject our null hypothesis and accept our alternative hypothesis.
If our test is not significant, we can accept our null hypothesis and reject our alternative hypothesis. A null hypothesis is a statement of no effect.
In Psychology, we use p < 0.05 (as it strikes a balance between making a type I and II error) but p < 0.01 is used in tests that could cause harm like introducing a new drug.
A type I error is when the null hypothesis is rejected when it should have been accepted (happens when a lenient significance level is used, an error of optimism).
A type II error is when the null hypothesis is accepted when it should have been rejected (happens when a stringent significance level is used, an error of pessimism).
Ethical Issues
- Informed consent is when participants are able to make an informed judgment about whether to take part. It causes them to guess the aims of the study and change their behavior.
- To deal with it, we can gain presumptive consent or ask them to formally indicate their agreement to participate but it may invalidate the purpose of the study and it is not guaranteed that the participants would understand.
- Deception should only be used when it is approved by an ethics committee, as it involves deliberately misleading or withholding information. Participants should be fully debriefed after the study but debriefing can’t turn the clock back.
- All participants should be informed at the beginning that they have the right to withdraw if they ever feel distressed or uncomfortable.
- It causes bias as the ones that stayed are obedient and some may not withdraw as they may have been given incentives or feel like they’re spoiling the study. Researchers can offer the right to withdraw data after participation.
- Participants should all have protection from harm . The researcher should avoid risks greater than those experienced in everyday life and they should stop the study if any harm is suspected. However, the harm may not be apparent at the time of the study.
- Confidentiality concerns the communication of personal information. The researchers should not record any names but use numbers or false names though it may not be possible as it is sometimes possible to work out who the researchers were.


An official website of the United States government
The .gov means it’s official. Federal government websites often end in .gov or .mil. Before sharing sensitive information, make sure you’re on a federal government site.
The site is secure. The https:// ensures that you are connecting to the official website and that any information you provide is encrypted and transmitted securely.
- Publications
- Account settings
Preview improvements coming to the PMC website in October 2024. Learn More or Try it out now .
- Advanced Search
- Journal List
- HHS Author Manuscripts

How to Conduct Responsible Research: A Guide for Graduate Students
Alison l. antes.
1 Department of Medicine, Division of General Medical Sciences, Washington University School of Medicine, St. Louis, Missouri, 314-362-6006
Leonard B. Maggi, Jr.
2 Department of Medicine, Division of Molecular Oncology, Siteman Cancer Center, Washington University School of Medicine, St. Louis, Missouri, 314-362-4102
Researchers must conduct research responsibly for it to have an impact and to safeguard trust in science. Essential responsibilities of researchers include using rigorous, reproducible research methods, reporting findings in a trustworthy manner, and giving the researchers who contributed appropriate authorship credit. This “how-to” guide covers strategies and practices for doing reproducible research and being a responsible author. The article also covers how to utilize decision-making strategies when uncertain about the best way to proceed in a challenging situation. The advice focuses especially on graduate students but is appropriate for undergraduates and experienced researchers. The article begins with an overview of the responsible conduct of research, research misconduct, and ethical behavior in the scientific workplace. The takeaway message is that responsible conduct of research requires a thoughtful approach to doing research to ensure trustworthy results and conclusions and that researchers receive fair credit.
INTRODUCTION
Doing research is stimulating and fulfilling work. Scientists make discoveries to build knowledge and solve problems, and they work with other dedicated researchers. Research is a highly complex activity, so it takes years for beginning researchers to learn everything they need to know to do science well. Part of this large body of knowledge is learning how to do research responsibly. Our purpose in this article is to provide graduate students a guide for how to perform responsible research. Our advice is also relevant to undergraduate researchers and for principal investigators (PIs), postdocs, or other researchers who mentor beginning researchers and wish to share our advice.
We begin by introducing some fundamentals about the responsible conduct of research (RCR), research misconduct, and ethical behavior. We focus on how to do reproducible science and be a responsible author. We provide practical advice for these topics and present scenarios to practice thinking through challenges in research. Our article concludes with decision-making strategies for addressing complex problems.
What is the responsible conduct of research?
To be committed to RCR means upholding the highest standards of honesty, accuracy, efficiency, and objectivity ( Steneck, 2007 ). Each day, RCR requires engaging in research in a conscientious, intentional fashion that yields the best science possible ( “Research Integrity is Much More Than Misconduct,” 2019 ). We adopt a practical, “how-to” approach, discussing the behaviors and habits that yield responsible research. However, some background knowledge about RCR is helpful to frame our discussion.
The scientific community uses many terms to refer to ethical and responsible behavior in research: responsible conduct of research, research integrity, scientific integrity, and research ethics ( National Academies of Science, 2009 ; National Academies of Sciences Engineering and Medicine, 2017 ; Steneck, 2007 ). A helpful way to think about these concepts is “doing good science in a good manner” ( DuBois & Antes, 2018 ). This means that the way researchers do their work, from experimental procedures to data analysis and interpretation, research reporting, and so on, leads to trustworthy research findings and conclusions. It also includes respectful interactions among researchers both within research teams (e.g., between peers, mentors and trainees, and collaborators) and with researchers external to the team (e.g., peer reviewers). We expand on trainee-mentor relationships and interpersonal dynamics with labmates in a companion article ( Antes & Maggi, 2021 ). When research involves human or animal research subjects, RCR includes protecting the well-being of research subjects.
We do not cover all potential RCR topics but focus on what we consider fundamentals for graduate students. Common topics covered in texts and courses on RCR include the following: authorship and publication; collaboration; conflicts of interest; data management, sharing, and ownership; intellectual property; mentor and trainee responsibilities; peer review; protecting human subjects; protecting animal subjects; research misconduct; the role of researchers in society; and laboratory safety. A number of topics prominently discussed among the scientific community in recent years are also relevant to RCR. These include the reproducibility of research ( Baker, 2016 ; Barba, 2016 ; Winchester, 2018 ), diversity and inclusion in science ( Asplund & Welle, 2018 ; Hofstra et al., 2020 ; Meyers, Brown, Moneta-Koehler, & Chalkley, 2018 ; National Academies of Sciences Engineering and Medicine, 2018a ; Roper, 2019 ), harassment and bullying ( Else, 2018 ; National Academies of Sciences Engineering and Medicine, 2018b ; “ No Place for Bullies in Science,” 2018 ), healthy research work environments ( Norris, Dirnagl, Zigmond, Thompson-Peer, & Chow, 2018 ; “ Research Institutions Must Put the Health of Labs First,” 2018 ), and the mental health of graduate students ( Evans, Bira, Gastelum, Weiss, & Vanderford, 2018 ).
The National Institutes of Health (NIH) ( National Institutes of Health, 2009 ) and the National Science Foundation ( National Science Foundation, 2017 ) have formal policies indicating research trainees must receive education in RCR. Researchers are accountable to these funding agencies and the public which supports research through billions in tax dollars annually. The public stands to benefit from, or be harmed by, research. For example, the public may be harmed if medical treatments or social policies are based on untrustworthy research findings. Funding for research, participation in research, and utilization of the fruits of research all rely on public trust ( Resnik, 2011 ). Trustworthy findings are also essential for good stewardship of scarce resources ( Emanuel, Wendler, & Grady, 2000 ). Researchers are further accountable to their peers, colleagues, and scientists more broadly. Trust in the work of other researchers is essential for science to advance. Finally, researchers are accountable for complying with the rules and policies of their universities or research institutions, such as rules about laboratory safety, bullying and harassment, and the treatment of animal research subjects.
What is research misconduct?
When researchers intentionally misrepresent or manipulate their results, these cases of scientific fraud often make the news headlines ( Chappell, 2019 ; O’Connor, 2018 ; Park, 2012 ), and they can seriously undermine public trust in research. These cases also harm trust within the scientific community.
The U.S. defines research misconduct as fabrication, falsification, and plagiarism (FFP) ( Department of Health and Human Services, 2005 ). FFP violate the fundamental ethical principle of honesty. Fabrication is making up data, and falsification is manipulating or changing data or results so they are no longer truthful. Plagiarism is a form of dishonesty because it includes using someone’s words or ideas and portraying them as your own. When brought to light, misconduct involves lengthy investigations and serious consequences, such as ineligibility to receive federal research funding, loss of employment, paper retractions, and, for students, withdrawal of graduate degrees.
One aspect of responsible behavior includes addressing misconduct if you observe it. We suggest a guide titled “Responding to Research Wrongdoing: A User-Friendly Guide” that provides advice for thinking about your options if you think you have observed misconduct ( Keith-Spiegel, Sieber, & Koocher, 2010 ). Your university will have written policies and procedures for investigating allegations of misconduct. Making an allegation is very serious. As Keith-Spiegel et al.’s guide indicates, it is important to know the evidence that supports your claim, and what to expect in the process. We encourage, if possible, talking to the persons involved first. For example, one of us knew of a graduate student who reported to a journal editor their suspicion of falsified data in a manuscript. It turned out that the student was incorrect. Going above the PI directly to the editor ultimately led to the PI leaving the university, and the student had a difficult time finding a new lab to complete their degree. If the student had first spoken to the PI and lab members, they could have learned that their assumptions about the data in the paper were wrong. In turn, they could have avoided accusing the PI of a serious form of scientific misconduct—making up data—and harming everyone’s scientific career.
What shapes ethical behavior in the scientific workplace?
Responsible conduct of research and research misconduct are two sides of a continuum of behavior—RCR upholds the ideals of research and research misconduct violates them. Problematic practices that fall in the middle but are not defined formally as research misconduct have been labeled as detrimental research practices ( National Academies of Sciences Engineering and Medicine, 2017 ). Researchers conducting misleading statistical analyses or PIs providing inadequate supervision are examples of the latter. Research suggests that characteristics of individual researchers and research environments explain (un)ethical behavior in the scientific workplace ( Antes et al., 2007 ; Antes, English, Baldwin, & DuBois, 2018 ; Davis, Riske-Morris, & Diaz, 2007 ; DuBois et al., 2013 ).
These two influences on ethical behavior are helpful to keep in mind when thinking about your behavior. When people think about their ethical behavior, they think about their personal values and integrity and tend to overlook the influence of their environment. While “being a good person” and having the right intentions are essential to ethical behavior, the environment also has an influence. In addition, knowledge of standards for ethical research is important for ethical behavior, and graduate students new to research do not yet know everything they need to. They also have not fully refined their ethical decision-making skills for solving professional problems. We discuss strategies for ethical decision-making in the final section of this article ( McIntosh, Antes, & DuBois, 2020 ).
The research environment influences ethical behavior in a number of ways. For example, if a research group explicitly discusses high standards for research, people will be more likely to prioritize these ideals in their behavior ( Plemmons et al., 2020 ). A mentor who sets a good example is another important factor ( Anderson et al., 2007 ). Research labs must also provide individuals with adequate training, supervision and feedback, opportunities to discuss data, and the psychological safety to feel comfortable communicating about problems, including mistakes ( Antes, Kuykendall, & DuBois, 2019a , 2019b ). On the other hand, unfair research environments, inadequate supervision, poor communication, and severe stress and anxiety may undermine ethical decision-making and behavior; particularly when many of these factors exist together. Thus, (un)ethical behavior is a complex interplay of individual factors (e.g., personality, stress, decision-making skills) and the environment.
For graduate students, it is important to attend to what you are learning and how the environment around you might influence your behavior. You do not know what you do not know, and you necessarily rely on others to teach you responsible practices. So, it is important to be aware. Ultimately, you are accountable for your behavior. You cannot just say “I didn’t know.” Rather, just like you are curious about your scientific questions, maintain a curiosity about responsible behavior as a researcher. If you feel uncomfortable with something, pay attention to that feeling, speak to someone you trust, and seek out information about how to handle the situation. In what follows, we cover key tips for responsible behavior in the areas of reproducibility and authorship that we hope will help you as you begin.
HOW TO DO REPRODUCIBLE SCIENCE
The foremost responsibility of scientists is to ensure they conduct research in such a manner that the findings are trustworthy. Reproducibility is the ability to duplicate results ( Goodman, Fanelli, & Ioannidis, 2016 ). The scientific community has called for greater openness, transparency, and rigor as key remedies for lack of reproducibility ( Munafò et al., 2017 ). As a graduate student, essential to fostering reproducibility is the rigor of your approach to doing experiments and handling data. We discuss how to utilize research protocols, document experiments in a lab notebook, and handle data responsibly.
Utilize research protocols
1. learn and utilize the lab’s protocols.
Research protocols describe the step-by-step procedures for doing an experiment. They are critical for the quality and reproducibility of experiments. Lab members must learn and follow the lab’s protocols with the understanding that they may need to make adjustments based on the requirements of a specific experiment.
Also, it is important to distinguish between the experiment you are performing and analyzing the data from that experiment. For example, the experiment you want to perform might be to determine if loss of a gene blocks cell growth. Several protocols, each with pros and cons, will allow you to examine “cell growth.” Using the wrong experimental protocol can produce data that leads to muddled conclusions. In this example, the gene does block cell growth, but the experiment used to produce the data that you analyze to understand cell growth is wrong, thus giving a result that is a false negative.
When first joining a lab, it is essential to commit to learning the protocols necessary for your assigned research project. Researchers must ensure they are proficient in executing a protocol and can perform their experiments reliably. If you do not feel confident with a protocol, you should do practice runs if possible. Repetition is the best way to work through difficulties with protocols. Often it takes several attempts to work through the steps of a protocol before you will be comfortable performing it. Asking to watch another lab member perform the protocol is also helpful. Be sure to watch closely how steps are performed, as often there are minor steps taken that are not written down. Also, experienced lab members may do things as second nature and not think to explicitly mention them when working through the protocol. Ask questions of other lab members so that you can improve your knowledge and gain confidence with a protocol. It is better to ask a question than potentially ruin a valuable or hard-to-get sample.
Be cautious of differences in the standing protocols in the lab and how you actually perform the experiment. Even the most minor deviations can seriously impact the results and reproducibility of an experiment. As mentioned above, often there are minor things that are done that might not be listed in the protocol. Paying attention and asking questions are the best ways to learn, in addition to adding notes to the protocol if you find minor details are missing.
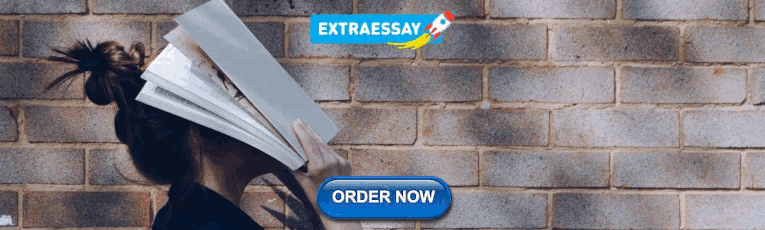
2. Develop your own protocols
Often you will find that a project requires a protocol that has not been performed in the lab. If performing a new experiment in the lab and no protocol exists, find a protocol and try it. Protocols can be obtained from many different sources. A great source is other labs on campus, as you can speak directly to the person who performs the experiment. There are many journal sources as well, such as Current Protocols, Nature Protocols, Nature Methods, and Cell STAR Methods . These methods journals provide the most detailed protocols for experiments often with troubleshooting tips. Scientific papers are the most common source of protocols. However, keep in mind that due to the common brevity of methods sections, they often omit crucial details or reference other papers that may not contain a complete description of the protocol.
3. Handle mistakes or problems promptly
At some point, everyone encounters problems with a protocol, or realizes they made a mistake. You should be prepared to handle this situation by being able to detail exactly how you performed the experiment. Did you skip a step? Shorten or lengthen a time point? Did you have to make a new buffer or borrow a labmate’s buffer? There are too many ways an experiment can go wrong to list here but being able to recount all the steps you performed in detail will help you work through the problem. Keep in mind that often the best way to understand how to perform an experiment is learning from when something goes wrong. This situation requires you to critically think through what was done and understand the steps taken. When everything works perfectly, it is easy to pay less attention to the details, which can lead to problems down the line.
It is up to you to be attentive and meticulous in the lab. Paying attention to the details may feel like a pain at first, or even seem overwhelming. Practice and repetition will help this focus on details become a natural part of your lab work. Ultimately, this skill will be essential to being a responsible scientist.
Document experiments in a lab notebook
1. recognize the importance of a lab notebook.
Maintaining detailed documentation in a lab notebook allows researchers to keep track of their experiments and generation of data. This detailed documentation helps you communicate about your research with others in the lab, and serves as a basis for preparing publications. It also provides a lasting record for the lab that exists beyond your time in the lab. After graduate students leave the lab, sometimes it is necessary to go back to the results of older experiments. A complete and detailed notebook is essential, or all of the time, effort, and resources are lost.
2. Learn the note-keeping practices in your lab
When you enter a new lab, it is important to understand how the lab keeps notebooks and the expectations for documentation. Being conscientious about documentation will make you a better scientist. In some labs, the PI might routinely examine your notebook, while in other labs you may be expected to maintain a notebook, but it may not be regularly viewed by others. It is tempting to become relaxed in documentation if you think your notebook may not be reviewed. Avoid this temptation; documentation of your ideas and process will improve your ability to think critically about research. Further, even if the PI or lab members do not physically view your notebook, you will need to communicate with them about your experiments. This documentation is necessary to communicate effectively about your work.
3. Organize your lab notebook
Different labs use different formats; some use electronic notebooks while others handwritten notebooks. The contents of a good notebook include the purpose of the experiment, the details of the experimental procedure, the data, and thoughts about the results. To effectively document your experiment, there are 5 critical questions that the information you record should be able to answer.
- Why I am doing this experiment? (purpose)
- What did I do to perform the experiment? (protocol)
- What are the results of what I did? (data, graphs)
- What do I think about the results?
- What do I think are the next steps?
We also recommend a table of contents. It will make the information more useful to you and the lab in the future. The table of contents should list the title of the experiment, the date(s) it was performed, and the page numbers on which it is recorded. Also, make sure that you write clearly and provide a legend or explanation of any shorthand or non-standard abbreviation you use. Often labs will have a combination of written lab notebooks and electronic data. It is important to reference where electronic data are located that go with each experiment. The idea is to make it as easy as possible to understand what you did and where to find all the data (electronic and hard copy) that accompanies your experiment.
Keeping a lab notebook becomes easier with practice. It can be thought of almost like journaling about your experiment. Sometimes people think of it as just a place to paste their protocol and a graph or data. We strongly encourage you to include your thoughts about why you made the decisions you made when conducting the experiment and to document your thoughts about next steps.
4. Commit to doing it the right way
A common reason to become lax in documentation is feeling rushed for time. Although documentation takes time, it saves time in the long-run and fosters good science. Without good notes, you will waste time trying to recall precisely what you did, reproduce your findings, and remember what you thought would be important next steps. The lab notebook helps you think about your research critically and keep your thoughts together. It can also save you time later when writing up results for publication. Further, well-documented data will help you draft a cogent and rigorous dissertation.
Handle data responsibly
1. keep all data.
Data are the product of research. Data include raw data, processed data, analyzed data, figures, and tables. Many data today are electronic, but not all. Generating data requires a lot of time and resources and researchers must treat data with care. The first essential tip is to keep all data. Do not discard data just because the experiment did not turn out as expected. A lot of experiments do not turn out to yield publishable data, but the results are still important for informing next steps.
Always keep the original, raw data. That is, as you process and analyze data, always maintain an unprocessed version of the original data.
Universities and funding agencies have data retention policies. These policies specify the number of years beyond a grant that data must be kept. Some policies also indicate researchers need to retain original data that served as the basis for a publication for a certain number of years. Therefore, your data will be important well beyond your time in graduate school. Most labs require you to keep samples for reanalysis until a paper is published, then the analyzed data are enough. If you leave a lab before a paper is accepted for publication, you are responsible for ensuring your data and original samples are well documented for others to find and use.
2. Document all data
In addition to keeping all data, data must be well-organized and documented. This means that no matter the way you keep your data (e.g., electronic or in written lab notebooks), there is a clear guide—in your lab notebook, a binder, or on a lab hard drive—to finding the data for a particular experiment. For example, it must be clear which data produced a particular graph. Version control of data is also critical. Your documentation should include “metadata” (data about your data) that tracks versions of the data. For example, as you edit data for a table, you should save separate versions of the tables, name the files sequentially, and note the changes that were made to each version.
3. Backup your data
You should backup electronic data regularly. Ideally, your lab has a shared server or cloud storage to backup data. If you are supposed to put your data there, make sure you do it! When you leave the lab, it must be possible to find your data.
4. Perform data analysis honestly and competently
Inappropriate use of statistics is a major concern in the scientific community, as the results and conclusions will be misleading if done incorrectly ( DeMets, 1999 ). Some practices are clearly an abuse of statistics, while other inappropriate practices stem from lack of knowledge. For example, a practice called “p-hacking” describes when researchers “collect or select data or statistical analyses until nonsignificant results become significant” ( Head, Holman, Lanfear, Kahn, & Jennions, 2015 ). In addition to avoiding such misbehavior, it is essential to be proficient with statistics to ensure you do statistical procedures appropriately. Learning statistical procedures and analyzing data takes many years of practice, and your statistics courses may only cover the basics. You will need to know when to consult others for help. In addition to consulting members in your lab or your PI, your university may have statistical experts who can provide consultations.
5. Master pressure to obtain favored results
When you conduct an experiment, the results are the results. As a beginning researcher, it is important to be prepared to manage the frustration of experiments not turning out as expected. It is also important to manage the real or perceived pressure to produce favored results. Investigators can become wedded to a hypothesis, and they can have a difficult time accepting the results. Sometimes you may feel this pressure coming from yourself; for example, if you want to please your PI, or if you want to get results for a certain publication. It is important to always follow the data no matter where it leads.
If you do feel pressure, this situation can be uncomfortable and stressful. If you have been meticulous and followed the above recommendations, this can be one great safeguard. You will be better able to confidently communicate your results to the PI because of your detailed documentation, and you will be more confident in your procedures if the possibility of error is suggested. Typically, with enough evidence that the unexpected results are real, the PI will concede. We recommend seeking the support of friends or colleagues to vent and cope with stress. In the rare case that the PI does not relent, you could turn to an advisor outside the lab if you need advice about how to proceed. They can help you look at the data objectively and also help you think about the interpersonal aspects of navigating this situation.
6. Communicate about your data in the lab
A critical element of reproducible research is communication in the lab. Ideally, there are weekly or bi-weekly meetings to discuss data. You need to develop your communication skills for writing and speaking about data. Often you and your labmates will discuss experimental issues and results informally during the course of daily work. This is an excellent way to hone critical thinking and communication skills about data.
Scenario 1 – The Protocol is Not Working
At the beginning of a rotation during their first year, a graduate student is handed a lab notebook and a pen and is told to keep track of their work. There does not appear to be a specific format to follow. There are standard lab protocols that everyone follows, but minor tweaks to the protocols do not seem to be tracked from experiment to experiment in the standard lab protocol nor in other lab notebooks. After two weeks of trying to follow one of the standard lab protocols, the student still cannot get the experiment to work. The student has included the appropriate positive and negative controls which are failing, making the experiment uninterpretable. After asking others in the lab for help, the graduate student learns that no one currently in the lab has performed this particular experiment. The former lab member who had performed the experiment only lists the standard protocol in their lab notebook.
How should the graduate student start to solve the problem?
Speaking to the PI would be the next logical step. As a first-year student in a lab rotation, the PI should expect this type of situation and provide additional troubleshooting guidance. It is possible that the PI may want to see how the new graduate student thinks critically and handles adversity in the lab. Rather than giving an answer, the PI might ask the student to work through the problem. The PI should give guidance, but it may not be an immediate fix for the problem. If the PI’s suggestions fail to correct the problem, asking a labmate or the PI for the contact information of the former lab member who most recently performed the experiment would be a reasonable next step. The graduate student’s conversations with the PI and labmates in this situation will help them learn a lot about how the people in the lab interact.
Most of the answers for these types of problems will require you as a graduate student to take the initiative to answer. They will require your effort and ingenuity to talk to other lab members, other labs at the university, and even scour the literature for alternatives. While labs have standard protocols, there are multiple ways to do many experiments, and working out an alternative will teach you more than when everything works. Having to troubleshoot problems will result in better standard protocols in the lab and better science.
HOW TO BE A RESPONSIBLE AUTHOR
Researchers communicate their findings via peer-reviewed publications, and publications are important for advancing in a research career. Many graduate students will first author or co-author publications in graduate school. For good advice on how to write a research manuscript, consult the Current Protocols article “How to write a research manuscript” ( Frank, 2018 ). We focus on the issues of assigning authors and reporting your findings responsibly. First, we describe some important basics: journal impact factors, predatory journals, and peer review.
What are journal impact factors?
It is helpful to understand journal impact factors. There is criticism about an overemphasis on impact factors for evaluating the quality or importance of researchers’ work ( DePellegrin & Johnston, 2015 ), but they remain common for this purpose. Journal impact factors reflect the average number of times articles in a journal were cited in the last two years. Higher impact factors place journals at a higher rank. Approximately 2% of journals have an impact factor of 10 or higher. For example, Cell, Science, and Nature have impact factors of approximately 39, 42, and 43, respectively. Journals can be great journals but have lower impact factors; often this is because they focus on a smaller specialty field. For example, Journal of Immunology and Oncogene are respected journals, but their impact factors are about 4 and 7, respectively.
Research trainees often want to publish in journals with the highest possible impact factor because they expect this to be viewed favorably when applying to future positions. We encourage you to bear in mind that many different journals publish excellent science and focus on publishing where your work will reach the desired audience. Also, keep in mind that while a high impact factor can direct you to respectable, high-impact science, it does not guarantee that the science in the paper is good or even correct. You must critically evaluate all papers you read no matter the impact factor.
What are predatory journals?
Predatory journals have flourished over the past few years as publishing science has moved online. An international panel defined predatory journals as follows ( Grudniewicz et al., 2019 ):
Predatory journals and publishers are entities that prioritize self-interest at the expense of scholarship and are characterized by false or misleading information, deviation from best editorial and publication practices, a lack of transparency, and/or the use of aggressive and indiscriminate solicitation practices. (p. 211)
Often young researchers receive emails soliciting them to submit their work to a journal. There are typically small fees (around $99 US) requested but these fees will be much lower than open access fees of reputable journals (often around $2000 US). A warning sign of a predatory journal is outlandish promises, such as 24-hour peer review or immediate publication. You can find a list of predatory journals created by a postdoc in Europe at BeallsList.net ( “Beall’s List of Potential Predatory Journals and Publishers,” 2020 ).
What is peer review?
Peer reviewers are other scientists who have the expertise to evaluate a manuscript. Typically 2 or 3 reviewers evaluate a manuscript. First, an editor performs an initial screen of the manuscript to ensure its appropriateness for the journal and that it meets basic quality standards. At this stage, an editor can decide to reject the manuscript and not send it to review. Not sending a paper for peer review is common in the highest impact journals that receive more submissions per year than can be reviewed and published. For average-impact journals and specialty journals, typically your paper will be sent for peer review.
In general, peer review focuses on three aspects of a manuscript: research design and methods, validity of the data and conclusions, and significance. Peer reviewers assess the merit and rigor of the research design and methodology, and they evaluate the overall validity of the results, interpretations, and conclusions. Essentially, reviewers want to ensure that the data support the claims. Additionally, reviewers evaluate the overall significance, or contribution, of the findings, which involves the novelty of the research and the likelihood that the findings will advance the field. Significance standards vary between journals. Some journals are open to publishing findings that are incremental advancements in a field, while others want to publish only what they deem as major advancements. This feature can distinguish the highest impact journals which seek the most significant advancements and other journals that tend to consider a broader range of work as long as it is scientifically sound. It is important to keep in mind that determining at the stage of review and publication whether a paper is “high impact” is quite subjective. In reality, this can only really be determined in retrospect.
The key ethical issues in peer review are fairness, objectivity, and confidentiality ( Shamoo & Resnik, 2015 ). Peer reviewers are to evaluate the manuscript on its merits and not based on biases related to the authors or the science itself. If reviewers have a conflict of interest, this should be disclosed to the editor. Confidentiality of peer review means that the reviewers should keep private the information; they should not share the information with others or use it to their benefit. Reviewers can ultimately recommend that the manuscript is rejected, revised, and resubmitted (major or minor revisions), or accepted. The editor evaluates the reviewers’ feedback and makes a judgment about rejecting, accepting, or requesting a revision. Sometimes PIs will ask experienced graduate students to assist with peer reviewing a manuscript. This is a good learning opportunity. The PI should disclose to the editor that they included a trainee in preparing the review.
Assign authorship fairly
Authorship gives credit to the people who contributed to the research. This includes thinking of the ideas, designing and performing experiments, interpreting the results, and writing the paper. Two key questions regarding authorship include: 1 - Who will be an author? 2 - What will be the order in which authors are listed? These seem simple on the surface but can get quite complex.
1. Know authorship guidelines
Authorship guidelines published by journals, professional societies, and universities communicate key principles of authorship and standards for earning authorship. The core ethical principle of assigning authorship is fairness in who receives credit for the work. The people who contributed to the work should get credit for it. This seems simply enough, but determining authorship can (and often does) create conflict.
Many universities have authorship guidelines, and you should know the policies at your university. The International Committee of Medical Journal Editors (ICMJE) provides four criteria for determining who should be an author ( International Committee of Medical Journal Editors, 2020 ). These criteria indicate that an author should do all of the following: 1) make “substantial contributions” to the development of the idea or research design, or to acquiring, analyzing, or interpreting the data, 2) write the manuscript or revise it a substantive way, 3) give approval of the final manuscript (i.e., before it is submitted for review, and after it is revised, if necessary), and 4) agree to be responsible for any questions about the accuracy or integrity of the research.
Several types of authorship violate these guidelines and should be avoided. Guest authorship is when respected researchers are added out of appreciation, or to have the manuscript be perceived more favorably to get it published or increase its impact. Gift authorship is giving authorship to reward an individual, or as a favor. Ghost authorship is when someone made significant contributions to the paper but is not listed as an author. To increase transparency, some journals require authors to indicate how each individual contributed to the research and manuscript.
2. Apply the guidelines
Conflicts often arise from disagreements about how much people contributed to the research and whether those contributions merit authorship. The best approach is an open, honest, and ongoing discussion about authorship, which we discuss in #3 below. To have effective, informed conversations about authorship, you must understand how to apply the guidelines to your specific situation. The following is a simple rule of thumb that indicates there are three components of authorship. We do not list giving final approval of the manuscript and agreeing to be accountable, but we do consider these essentials of authorship.
- Thinking – this means contributing to the ideas leading to the hypothesis of the work, designing experiments to address the hypothesis, and/or analyzing the results in the larger context of the literature in the field.
- Doing – this means performing and analyzing the experiments.
- Writing – this means editing a draft, or writing the entire paper. The first author often writes the entire first draft.
In our experience, a first author would typically do all three. They also usually coordinate the writing and editing process. Co-authors are typically very involved in at least two of the three, and are somewhat involved in the other. The PI, who oversees and contributes to all three, is often the last, or “senior author.” The “senior author” is typically the “corresponding author”—the person listed as the individual to contact about the paper. The other co-authors are listed between the first and senior author either alphabetically, or more commonly, in order from the largest to smallest contribution.
Problems in assigning authorship typically arise due to people’s interpretations of #1 (thinking) and #2 (doing)—what and how much each individual contributed to a project’s design, execution, and analysis. Different fields or PIs may have their own slight variations on these guidelines. The potential conflicts associated with assigning authorship lead to the most common recommendation for responsibly assigning authorship: discuss authorship expectations early and revisit them during the project.
3. Discuss authorship with your collaborators
Publications are important for career advancement, so you can see why people might be worried about fairness in assigning authorship. If the problem arises from a lack of a shared understanding about contributions to the research, the only way to clarify this is an open discussion. This discussion should ideally take place very early at the beginning of a project, and should be ongoing. Hopefully you work in a laboratory that makes these discussions a natural part of the research process; this makes it much easier to understand the expectations upfront.
We encourage you to speak up about your interest in making a contribution that would merit authorship, especially if you want to earn first authorship. Sometimes norms about authoring papers in a lab make it clear you are expected to first and co-author publications, but it is best to communicate your interest in earning authorship. If the project is not yours, but you wish to collaborate, you can inquire what you may be able to contribute that would merit authorship.
If it is not a norm in your lab to discuss authorship throughout the life of projects, then as a graduate student you may feel reluctant to speak up. You could initiate a conversation with a more senior graduate student, a postdoc, or your PI, depending on the dynamics in the group. You could ask generally about how the lab approaches assignment of authorship, but discussing a specific project and paper may be best. It may feel awkward to ask, but asking early is less uncomfortable than waiting until the end of the project. If the group is already drafting a manuscript and you are told that your contribution is insufficient for authorship, this situation is much more discouraging than if you had asked earlier about what is expected to earn authorship.
How to report findings responsibly
The most significant responsibility of authors is to present their research accurately and honestly. Deliberately presenting misleading information is clearly unethical, but there are significant judgment calls about how to present your research findings. For example, an author can mislead by overstating the conclusions given what the data support.
1. Commit to presenting your findings honestly
Any good scientific manuscript writer will tell you that you need to “tell a good story.” This means that your paper is organized and framed to draw the reader into the research and convince them of the importance of the findings. But, this story must be sound and justified by the data. Other authors are presenting their findings in the best, most “publishable” light, so it is a balancing act to be persuasive but also responsible in presenting your findings in a trustworthy manner. To present your findings honestly, you must be conscious of how you interpret your data and present your conclusions so that they are accurate and not overstated.
One misbehavior known as “HARKing,” Hypothesis After the Results are Known, occurs when hypotheses are created after seeing the results of an experiment, but they are presented as if they were defined prior to collecting the data ( Munafò et al., 2017 ). This practice should be avoided. HARKing may be driven, in part, by a concern in scientific publishing known as publication bias. This bias is a preference that reviewers, editors, and researchers have for papers describing positive findings instead of negative findings ( Carroll, Toumpakari, Johnson, & Betts, 2017 ). This preference can lead to manipulating one’s practices, such as by HARKing, so that positive findings can be reported.
It is important to note that in addition to avoiding misbehaviors such as HARKing, all researchers are susceptible to a number of more subtle traps in judgment. Even the most well-intentioned researcher may jump to conclusions, discount alternative explanations, or accept results that seem correct without further scrutiny ( Nuzzo, 2015 ). Therefore, researchers must not only commit to presenting their findings honestly but consider how they can counteract such traps by slowing down and increasing their skepticism towards their findings.
2. Provide an appropriate amount of detail
Providing enough detail in a manuscript can be a challenge with the word limits imposed by most journals. Therefore, you will need to determine what details to include and which to exclude, or potentially include in the supplemental materials. Methods sections can be long and are often the first to be shortened, but complete methods are important for others to evaluate the research and to repeat the methods in other studies. Even more significant is making decisions about what experimental data to include and potentially exclude from the manuscript. Researchers must determine what data is required to create a complete scientific story that supports the central hypothesis of the paper. On the other hand, it is not necessary or helpful to include so much data in the manuscript, or in supplemental material, that the central point of the paper is difficult to discern. It is a tricky balance.
3. Follow proper citation practices
Of course, responsible authorship requires avoiding plagiarism. Many researchers think that plagiarism is not a concern for them because they assume it is always done intentionally by “copying and pasting” someone else’s words and claiming them as your own. Sometimes poor writing practices, such as taking notes from references without distinguishing between direct quotes and paraphrased material, can lead to including material that is not quoted properly. More broadly, proper citation practices include accurately and completely referencing prior studies to provide appropriate context for your manuscript.
4. Attend to the other important details
The journal will require several pieces of additional information, such as disclosure of sources of funding and potential conflicts of interest. Typically, graduate students do not have relationships that constitute conflicts of interest, but a PI who is a co-author may. In submitting a manuscript, also make sure to acknowledge individuals not listed as authors but who contributed to the work.
5. Share data and promote transparency
Data sharing is a key facet of promoting transparency in science ( Nosek et al., 2015 ). It will be important to know the expectations of the journals in which you wish to publish. Many top journals now require data sharing; for example, sharing your data files in an online repository so others have access to the data for secondary use. Funding agencies like NIH also increasingly require data sharing. To further foster transparency and public trust in research, researchers must deposit their final peer-reviewed manuscripts that report on research funded by NIH to PubMed Central. PubMed makes biomedical and life science research publicly accessible in a free, online database.
Scenario 2 – Authors In Conflict
To prepare a manuscript for publication, a postdoc’s data is added to a graduate student’s thesis project. After working together to combine the data and write the paper, the postdoc requests co-first authorship on the paper. The graduate student balks at this request on the basis that it is their thesis project. In a weekly meeting with the lab’s PI to discuss the status of the paper, the graduate student states that they should divide the data between the authors as a way to prove that the graduate student should be the sole first author. The PI agrees to this attempt to quantify how much data each person contributed to the manuscript. All parties agree the writing and thinking were equally shared between them. After this assessment, the graduate student sees that the postdoc actually contributed more than half of the data presented in the paper. The graduate student and a second graduate student contributed the remaining data; this means the graduate student contributed much less than half of the data in the paper. However, the graduate student is still adamant that they must be the sole first author of the paper because it is their thesis project.
Is the graduate student correct in insisting that it is their project, so they are entitled to be the sole first author?
Co-first authorship became popular about 10 years ago as a way to acknowledge shared contributions to a paper in which authors worked together and contributed equally. If the postdoc contributed half of the data and worked with the graduate student to combine their interpretations and write the first draft of the paper, then the postdoc did make a substantial contribution. If the graduate student wrote much of the first draft of the paper, contributed significantly to the second half of data, and played a major role in the thesis concept and design, this is also a major contribution. We summarized authorship requirements as contributing to thinking, doing, and writing, and we noted that a first author usually contributes to all of these. The graduate student has met all 3 elements to claim first authorship. However, it appears that the postdoc has also met these 3 requirements. Thus, it is at least reasonable for the postdoc to ask about co-first authorship.
The best way to move forward is to discuss their perspectives openly. Both the graduate student and postdoc want first authorship on papers to advance their careers. The postdoc feels they contributed more to the overall concept and design than the graduate student is recognizing, and the postdoc did contribute half of the data. This is likely frustrating and upsetting for the postdoc. On the other hand, perhaps the postdoc is forgetting how much a thesis becomes like “your baby,” so to speak. The work is the graduate student’s thesis, so it is easy to see why the graduate student would feel a sense of ownership of it. Given this fact, it may be hard for the graduate student to accept the idea that they would share first-author recognition for the work. Yet, the graduate student should consider that the manuscript would not be possible without the postdoc’s contribution. Further, if the postdoc was truly being unreasonable, then the postdoc could make the case for sole first authorship based on contributing the most data to the paper, in addition to contributing ideas and writing the paper. The graduate student should consider that the postdoc may be suggesting co-first authorship in good faith.
As with any interpersonal conflict, clear communication is key. While it might be temporarily uncomfortable to voice their views and address this disagreement, it is critical to avoiding permanent damage to their working relationship. The pair should consider each other’s perspectives and potential alternatives. For example, if the graduate student is first author and the postdoc second, at a minimum they could include an author note in the manuscript that describes the contribution of each author. This would make it clear the scope of the postdoc’s contribution, if they decided not to go with co-first authorship. Also, the graduate student should consider their assumptions about co-first authorship. Maybe they assume it makes it appear they contributed less, but instead, perhaps co-first authorship highlights their collaborative approach to science. Collaboration is a desirable quality many (although arguably not all) research organizations look for when they are hiring.
They will also need to speak with others for advice. The pair should definitely speak with the PI who could provide input about how these cases have been handled in the past. Ultimately, if they cannot reach an agreement, the PI, who is likely to be the last or “senior” author, may make the final decision. They should also speak to the other graduate student who is an author.
If either individual is upset with the situation, they will want to discuss it when they have had time to cool down. This might mean taking a day before discussing, or speaking with someone outside of the lab for support. Ideally, all authors on this paper would have initiated this conversation earlier, and the standards in the lab for first authorship would be discussed routinely. Clear communication may have avoided the conflict.
HOW TO USE DECISION-MAKING STRATEGIES TO NAVIGATE CHALLENGES
We have provided advice on some specific challenges you might encounter in research. This final section covers our overarching recommendation that you adopt a set of ethical decision-making strategies. These strategies help researchers address challenges by helping them think through a problem and possible alternatives ( McIntosh et al., 2020 ). The strategies encourage you to gather information, examine possible outcomes, consider your assumptions, and address emotional reactions before acting. They are especially helpful when you are uncertain how to proceed, face a new problem, or when the consequences of a decision could negatively impact you or others. The strategies also help people be honest with themselves, such as when they are discounting important factors or have competing goals, by encouraging them to identify outside perspectives and test their motivations. You can remember the strategies using the acronym SMART .
1. S eek Help
Obtain input from others who can be objective and that you trust. They can assist you with assessing the situation, predicting possible outcomes, and identifying potential options. They can also provide you with support. Individuals to consult may be peers, other faculty, or people in your personal life. It is important that you trust the people you talk with, but it is also good when they challenge your perspective, or encourage you to think in a new way about a problem. Keep in mind that people such as program directors and university ombudsmen are often available for confidential, objective advice.
2. M anage Emotions
Consider your emotional reaction to the situation and how it might influence your assessment of the situation, and your potential decisions and actions. In particular, identify negative emotions, like frustration, anxiety, fear, and anger, as they particularly tend to diminish decision-making and the quality of interactions with others. Take time to address these emotions before acting, for example, by exercising, listening to music, or simply taking a day before responding.
3. A nticipate Consequences
Think about how the situation could turn out. This includes for you, for the research team, and anyone else involved. Consider the short, middle-term, and longer-term impacts of the problem and your potential approach to addressing the situation. Ideally, it is possible to identify win-win outcomes. Often, however, in tough professional situations, you may need to select the best option from among several that are not ideal.
4. R ecognize Rules and Context
Determine if any ethical principles, professional policies, or rules apply that might help guide your choices. For instance, if the problem involves an authorship dispute, consider the authorship guidelines that apply. Recognizing the context means considering the situational factors that could impact your options and how you proceed. For example, factors such as the reality that ultimately the PI may have the final decision about authorship.
5. T est Assumptions and Motives
Examine your beliefs about the situation and whether any of your thoughts may not be justified. This includes critically examining the personal motivations and goals that are driving your interpretation of the problem and thoughts about how to resolve it.
These strategies do not have to be engaged in order, and they are interrelated. For example, seeking help can help you manage emotions, test assumptions, and anticipate consequences. Go back to the scenarios and our advice throughout this article, and you will see many of our suggestions align with these strategies. Practice applying SMART strategies when you encounter a problem and they will become more natural.
Learning practices for responsible research will be the foundation for your success in graduate school and your career. We encourage you to be reflective and intentional as you learn and hope that our advice helps you along the way.
ACKNOWLEDGEMENTS
This work was supported by the National Human Genome Research Institute (Antes, K01HG008990) and the National Center for Advancing Translational Sciences (UL1 TR002345).
LITERATURE CITED
- Anderson MS, Horn AS, Risbey KR, Ronning EA, De Vries R, & Martinson BC (2007). What Do Mentoring and Training in the Responsible Conduct of Research Have To Do with Scientists’ Misbehavior? Findings from a National Survey of NIH-Funded Scientists . Academic Medicine , 82 ( 9 ), 853–860. doi: 10.1097/ACM.0b013e31812f764c [ PubMed ] [ CrossRef ] [ Google Scholar ]
- Antes AL, Brown RP, Murphy ST, Waples EP, Mumford MD, Connelly S, & Devenport LD (2007). Personality and Ethical Decision-Making in Research: The Role of Perceptions of Self and Others . Journal of Empirical Research on Human Research Ethics , 2 ( 4 ), 15–34. doi: 10.1525/jer.2007.2.4.15 [ PubMed ] [ CrossRef ] [ Google Scholar ]
- Antes AL, English T, Baldwin KA, & DuBois JM (2018). The Role of Culture and Acculturation in Researchers’ Perceptions of Rules in Science . Science and Engineering Ethics , 24 ( 2 ), 361–391. doi: 10.1007/s11948-017-9876-4 [ PMC free article ] [ PubMed ] [ CrossRef ] [ Google Scholar ]
- Antes AL, Kuykendall A, & DuBois JM (2019a). The Lab Management Practices of “Research Exemplars” that Foster Research Rigor and Regulatory Compliance: A Qualitative Study of Successful Principal Investigators . PloS One , 14 ( 4 ), e0214595. doi: 10.1371/journal.pone.0214595 [ PMC free article ] [ PubMed ] [ CrossRef ] [ Google Scholar ]
- Antes AL, Kuykendall A, & DuBois JM (2019b). Leading for Research Excellence and Integrity: A Qualitative Investigation of the Relationship-Building Practices of Exemplary Principal Investigators . Accountability in Research , 26 ( 3 ), 198–226. doi: 10.1080/08989621.2019.1611429 [ PMC free article ] [ PubMed ] [ CrossRef ] [ Google Scholar ]
- Antes AL, & Maggi LB Jr. (2021). How to Navigate Trainee-Mentor Relationships and Interpersonal Dynamics in the Lab . Current Protocols Essential Laboratory Techniques. [ PMC free article ] [ PubMed ] [ Google Scholar ]
- Asplund M, & Welle CG (2018). Advancing Science: How Bias Holds Us Back . Neuron , 99 ( 4 ), 635–639. doi: 10.1016/j.neuron.2018.07.045 [ PubMed ] [ CrossRef ] [ Google Scholar ]
- Baker M (2016). Is There a Reproducibility Crisis? Nature , 533 , 452–454. doi: 10.1038/533452a [ PubMed ] [ CrossRef ] [ Google Scholar ]
- Barba LA (2016). The Hard Road to Reproducibility . Science , 354 ( 6308 ), 142. doi: 10.1126/science.354.6308.142 [ PubMed ] [ CrossRef ] [ Google Scholar ]
- Beall’s List of Potential Predatory Journals and Publishers . (2020). Retrieved from https://beallslist.net/#update [ Google Scholar ]
- Carroll HA, Toumpakari Z, Johnson L, & Betts JA (2017). The Perceived Feasibility of Methods to Reduce Publication Bias . PloS One , 12 ( 10 ), e0186472–e0186472. doi: 10.1371/journal.pone.0186472 [ PMC free article ] [ PubMed ] [ CrossRef ] [ Google Scholar ]
- Chappell B (2019). Duke Whistleblower Gets More Than $33 Million in Research Fraud Settlement . NPR. Retrieved from https://www.npr.org/2019/03/25/706604033/duke-whistleblower-gets-more-than-33-million-in-research-fraud-settlement [ Google Scholar ]
- Davis MS, Riske-Morris M, & Diaz SR (2007). Causal Factors Implicated in Research Misconduct: Evidence from ORI Case Files . Science and Engineering Ethics , 13 ( 4 ), 395–414. doi: 10.1007/s11948-007-9045-2 [ PubMed ] [ CrossRef ] [ Google Scholar ]
- DeMets DL (1999). Statistics and Ethics in Medical Research . Science and Engineering Ethics , 5 ( 1 ), 97–117. doi: 10.1007/s11948-999-0059-9 [ PubMed ] [ CrossRef ] [ Google Scholar ]
- Department of Health and Human Services. (2005). 42 CFR Parts 50 and 93 Public Health Service Policies on Research Misconduct; Final Rule. Retrieved from https://ori.hhs.gov/sites/default/files/42_cfr_parts_50_and_93_2005.pdf [ Google Scholar ]
- DePellegrin TA, & Johnston M (2015). An Arbitrary Line in the Sand: Rising Scientists Confront the Impact Factor . Genetics , 201 ( 3 ), 811–813. [ PMC free article ] [ PubMed ] [ Google Scholar ]
- DuBois JM, Anderson EE, Chibnall J, Carroll K, Gibb T, Ogbuka C, & Rubbelke T (2013). Understanding Research Misconduct: A Comparative Analysis of 120 Cases of Professional Wrongdoing . Account Res , 20 ( 5–6 ), 320–338. doi: 10.1080/08989621.2013.822248 [ PMC free article ] [ PubMed ] [ CrossRef ] [ Google Scholar ]
- DuBois JM, & Antes AL (2018). Five Dimensions of Research Ethics: A Stakeholder Framework for Creating a Climate of Research Integrity . Academic Medicine , 93 ( 4 ), 550–555. doi: 10.1097/ACM.0000000000001966 [ PMC free article ] [ PubMed ] [ CrossRef ] [ Google Scholar ]
- Else H (2018). Does Science have a Bullying Problem? Nature , 563 , 616–618. doi: 10.1038/d41586-018-07532-5 [ PubMed ] [ CrossRef ] [ Google Scholar ]
- Emanuel EJ, Wendler D, & Grady C (2000). What Makes Clinical Research Ethical ? Journal of the American Medical Association , 283 ( 20 ), 2701–2711. doi:jsc90374 [pii] [ PubMed ] [ Google Scholar ]
- Evans TM, Bira L, Gastelum JB, Weiss LT, & Vanderford NL (2018). Evidence for a Mental Health Crisis in Graduate Education . Nature Biotechnology , 36 ( 3 ), 282–284. doi: 10.1038/nbt.4089 [ PubMed ] [ CrossRef ] [ Google Scholar ]
- Frank DJ (2018). How to Write a Research Manuscript . Current Protocols Essential Laboratory Techniques , 16 ( 1 ), e20. doi: 10.1002/cpet.20 [ CrossRef ] [ Google Scholar ]
- Goodman SN, Fanelli D, & Ioannidis JPA (2016). What Does Research Reproducibility Mean? Science Translational Medicine , 8 ( 341 ), 341ps312. doi: 10.1126/scitranslmed.aaf5027 [ PubMed ] [ CrossRef ] [ Google Scholar ]
- Grudniewicz A, Moher D, Cobey KD, Bryson GL, Cukier S, Allen K, … Lalu MM (2019). Predatory journals: no definition, no defence . Nature , 576 ( 7786 ), 210–212. doi: 10.1038/d41586-019-03759-y [ PubMed ] [ CrossRef ] [ Google Scholar ]
- Head ML, Holman L, Lanfear R, Kahn AT, & Jennions MD (2015). The Extent and Consequences of P-Hacking in Science . PLoS Biology , 13 ( 3 ), e1002106. doi: 10.1371/journal.pbio.1002106 [ PMC free article ] [ PubMed ] [ CrossRef ] [ Google Scholar ]
- Hofstra B, Kulkarni VV, Munoz-Najar Galvez S, He B, Jurafsky D, & McFarland DA (2020). The Diversity–Innovation Paradox in Science . Proceedings of the National Academy of Sciences , 117 ( 17 ), 9284. doi: 10.1073/pnas.1915378117 [ PMC free article ] [ PubMed ] [ CrossRef ] [ Google Scholar ]
- International Committee of Medical Journal Editors. (2020). Defining the Role of Authors and Contributors . Retrieved from http://www.icmje.org/recommendations/browse/roles-and-responsibilities/defining-the-role-of-authors-and-contributors.html
- Keith-Spiegel P, Sieber J, & Koocher GP (2010). Responding to Research Wrongdoing: A User-Friendly Guide . Retrieved from http://users.neo.registeredsite.com/1/4/0/20883041/assets/RRW_11-10.pdf
- McIntosh T, Antes AL, & DuBois JM (2020). Navigating Complex, Ethical Problems in Professional Life: A Guide to Teaching SMART Strategies for Decision-Making . Journal of Academic Ethics . doi: 10.1007/s10805-020-09369-y [ PMC free article ] [ PubMed ] [ CrossRef ] [ Google Scholar ]
- Meyers LC, Brown AM, Moneta-Koehler L, & Chalkley R (2018). Survey of Checkpoints along the Pathway to Diverse Biomedical Research Faculty . PloS One , 13 ( 1 ), e0190606–e0190606. doi: 10.1371/journal.pone.0190606 [ PMC free article ] [ PubMed ] [ CrossRef ] [ Google Scholar ]
- Munafò MR, Nosek BA, Bishop DVM, Button KS, Chambers CD, Percie du Sert N, … Ioannidis JPA (2017). A manifesto for reproducible science . Nature Human Behaviour , 1 ( 1 ), 0021. doi: 10.1038/s41562-016-0021 [ PMC free article ] [ PubMed ] [ CrossRef ] [ Google Scholar ]
- National Academies of Science. (2009). On Being a Scientist: A Guide to Responsible Conduct in Research . Washington DC: National Academics Press. [ PubMed ] [ Google Scholar ]
- National Academies of Sciences Engineering and Medicine. (2017). Fostering Integrity in Research . Washington, DC: The National Academies Press [ PubMed ] [ Google Scholar ]
- National Academies of Sciences Engineering and Medicine. (2018a). An American Crisis: The Growing Absence of Black Men in Medicine and Science: Proceedings of a Joint Workshop . Washington, DC: The National Academies Press. [ PubMed ] [ Google Scholar ]
- National Academies of Sciences Engineering and Medicine. (2018b). Sexual harassment of women: climate, culture, and consequences in academic sciences, engineering, and medicine : National Academies Press. [ PubMed ] [ Google Scholar ]
- National Institutes of Health. (2009). Update on the Requirement for Instruction in the Responsible Conduct of Research . NOT-OD-10-019 . Retrieved from https://grants.nih.gov/grants/guide/notice-files/NOT-OD-10-019.html
- National Science Foundation. (2017). Important Notice No. 140 Training in Responsible Conduct of Research – A Reminder of the NSF Requirement . Retrieved from https://www.nsf.gov/pubs/issuances/in140.jsp
- No Place for Bullies in Science. (2018). Nature , 559 ( 7713 ), 151. doi: 10.1038/d41586-018-05683-z [ PubMed ] [ CrossRef ] [ Google Scholar ]
- Norris D, Dirnagl U, Zigmond MJ, Thompson-Peer K, & Chow TT (2018). Health Tips for Research Groups . Nature , 557 , 302–304. doi: 10.1038/d41586-018-05146-5 [ PubMed ] [ CrossRef ] [ Google Scholar ]
- Nosek BA, Alter G, Banks GC, Borsboom D, Bowman SD, Breckler SJ, … Yarkoni T (2015). Scientific Standards . Promoting an Open Research Culture. Science , 348 ( 6242 ), 1422–1425. doi: 10.1126/science.aab2374 [ PMC free article ] [ PubMed ] [ CrossRef ] [ Google Scholar ]
- Nuzzo R (2015). How Scientists Fool Themselves - and How They Can Stop . Nature , 526 , 182–185. [ PubMed ] [ Google Scholar ]
- O’Connor A (2018). More Evidence that Nutrition Studies Don’t Always Add Up . The New York Times. Retrieved from https://www.nytimes.com/2018/09/29/sunday-review/cornell-food-scientist-wansink-misconduct.html [ Google Scholar ]
- Park A (2012). Great Science Frauds . Time. Retrieved from https://healthland.time.com/2012/01/13/great-science-frauds/slide/the-baltimore-case/ [ Google Scholar ]
- Plemmons DK, Baranski EN, Harp K, Lo DD, Soderberg CK, Errington TM, … Esterling KM (2020). A Randomized Trial of a Lab-embedded Discourse Intervention to Improve Research Ethics . Proceedings of the National Academy of Sciences , 117 ( 3 ), 1389. doi: 10.1073/pnas.1917848117 [ PMC free article ] [ PubMed ] [ CrossRef ] [ Google Scholar ]
- Research Institutions Must Put the Health of Labs First. (2018). Nature , 557 ( 7705 ), 279–280. doi: 10.1038/d41586-018-05159-0 [ PubMed ] [ CrossRef ] [ Google Scholar ]
- Research Integrity is Much More Than Misconduct . (2019). ( 570 ). doi: 10.1038/d41586-019-01727-0 [ PubMed ] [ CrossRef ] [ Google Scholar ]
- Resnik DB (2011). Scientific Research and the Public Trust . Science and Engineering Ethics , 17 ( 3 ), 399–409. doi: 10.1007/s11948-010-9210-x [ PMC free article ] [ PubMed ] [ CrossRef ] [ Google Scholar ]
- Roper RL (2019). Does Gender Bias Still Affect Women in Science? Microbiology and Molecular Biology Reviews , 83 ( 3 ), e00018–00019. doi: 10.1128/MMBR.00018-19 [ PMC free article ] [ PubMed ] [ CrossRef ] [ Google Scholar ]
- Shamoo AE, & Resnik DB (2015). Responsible Conduct of Research (3rd ed.). New York: Oxford University Press. [ Google Scholar ]
- Steneck NH (2007). ORI Introduction to the Responsible Conduct of Research (Updated ed.). Washington, D.C.: U.S. Government Printing Office. [ Google Scholar ]
- Winchester C (2018). Give Every Paper a Read for Reproducibility . Nature , 557 , 281. doi: 10.1038/d41586-018-05140-x [ PubMed ] [ CrossRef ] [ Google Scholar ]
How to Conduct Responsible Research: A Guide for Graduate Students
Affiliations.
- 1 Department of Medicine, Division of General Medical Sciences, Washington University School of Medicine, St. Louis, Missouri.
- 2 Department of Medicine, Division of Molecular Oncology, Siteman Cancer Center, Washington University School of Medicine, St. Louis, Missouri.
- PMID: 33740292
- PMCID: PMC8277481
- DOI: 10.1002/cpz1.87
Researchers must conduct research responsibly for it to have an impact and to safeguard trust in science. Essential responsibilities of researchers include using rigorous, reproducible research methods, reporting findings in a trustworthy manner, and giving the researchers who contributed appropriate authorship credit. This "how-to" guide covers strategies and practices for doing reproducible research and being a responsible author. The article also covers how to utilize decision-making strategies when uncertain about the best way to proceed in a challenging situation. The advice focuses especially on graduate students, but is appropriate for undergraduates and experienced researchers. It begins with an overview of responsible conduct of research, research misconduct, and ethical behavior in the scientific workplace. The takeaway message is that responsible conduct of research requires a thoughtful approach to doing research in order to ensure trustworthy results and conclusions, and that researchers receive fair credit. © 2021 Wiley Periodicals LLC.
Keywords: authorship; graduate students; publication; reproducibility; research ethics; research integrity; responsible conduct of research; rigor; scientific integrity.
© 2021 Wiley Periodicals LLC.
- Authorship*
- Research Personnel
- Scientific Misconduct*
Grants and funding
- K01 HG008990/HG/NHGRI NIH HHS/United States
- UL1 TR002345/TR/NCATS NIH HHS/United States
- K01HG008990/HG/NHGRI NIH HHS/United States
- TR002345/TR/NCATS NIH HHS/United States
Skip navigation
- Log in to UX Certification
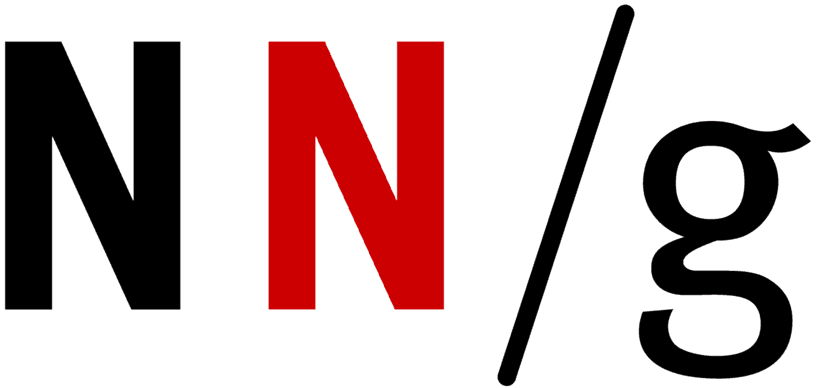
World Leaders in Research-Based User Experience
Planning research with generative ai.
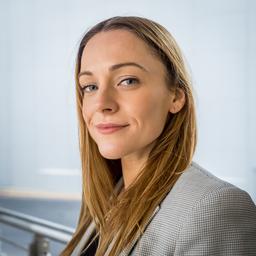
April 5, 2024 2024-04-05
- Email article
- Share on LinkedIn
- Share on Twitter
AI chatbots (like ChatGPT, Google’s Gemini, and Microsoft Copilot) can support UX researchers of all experience levels in planning their research.
In This Article:
What is a research plan, using ai chatbots to write a research plan.
Good research always starts with a plan.
A research plan is a document that outlines the research objectives and how the research will be executed.
Research plans should include:
- The research goals or questions that the research is hoping to achieve or answer
- The method to be used and a description of how it will be carried out
- The tasks or questions that will be given to study participants
- The profile of the target participants
- The screener questionnaire used to recruit participants
Creating a research plan can be time-consuming. Even with a good template, a researcher must generate research questions, select the appropriate method(s), decide how to run sessions, and often create study collateral (like screeners and tasks) from scratch. The good news is that AI can help with many, if not all, of these tasks!
It can be tempting to just ask an AI tool to give you a research plan for a project. Don’t do that.
❌ Bad Prompt : Generate a research plan for a usability test of a food-delivery app.
This kind of request results in a generic, template-like response, partly because AI lacks the context to propose a complete research plan and partly because of how the model has been trained.
To construct a useful research plan, deconstruct the plan into parts and have the AI chatbot tackle each part individually. You can assemble responses in a final research plan.
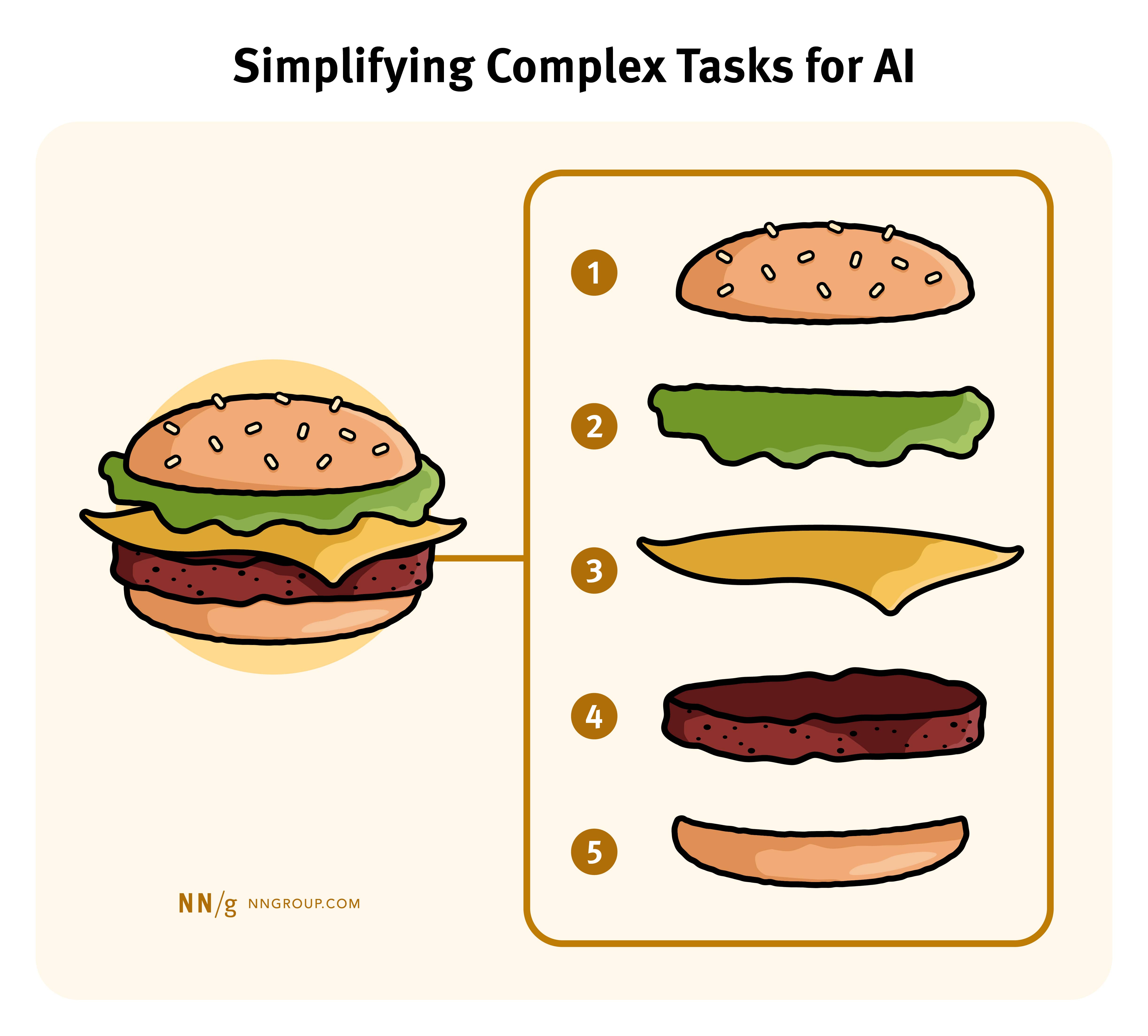
Don’t expect the AI to ask you the right questions in order to get a comprehensive outcome. View the AI tool as a UX assistant , not as a UX mentor. You need to feed into the AI all the steps and details you want it to consider.
Follow the steps below for the best results.
Step 1: Provide Context
Imagine asking an experienced colleague for recommendations on what research study to run without giving them any context on what project you’re working on and what you’re trying to achieve. They would probably say, "It depends on what you’re trying to learn.” Conversing with AI presents no exception.
Start your conversation with enough context , especially if the AI tool does not already have this information stored about you or your current project. ( ChatGPT 4 allows users to store context for each new conversation.)
The contextual information you share should include who you work for, the scope of your project, and what you’re looking to achieve. Remember that some AI tools may use your conversations to train their models, so share only information you would be comfortable with being in the open domain.
Prompt Template: I work for [type or organization] . My company provides [what kind of product, service, or experience] . I need to run a user-research study to learn [something about users or a problem] .
Step 2: Generate Research Questions
After providing the AI tool with context, ask it to suggest specific research questions for the study. Here’s an example from a recent study on research repositories that I conducted for NN/g.
Prompt Example: I will run a research study and report on how UX practitioners across different organizations set up and use research repositories. Please generate 10 research questions that would be suitable for this sort of study.
It’s a good idea to ask generative AI tools for more options or ideas than you think you’ll need, because some will not be a good fit.
Microsoft Copilot responded to the prompt above with the 10 research questions below.
10 Research questions generated by Microsoft Copilot
Who are the primary users of the research repository within different organizations? What motivates users to engage with the research repository? How do different teams (design, product, sales, marketing) access and benefit from the research repository? What challenges do practitioners face when contributing to or retrieving information from the repository? What types of research artifacts (reports, raw data, templates) are most commonly stored in the repository? How do organizations structure their research repositories? What tools and templates are commonly used to facilitate research repository management? How do practitioners ensure the quality and accuracy of information in the repository? What impact does the research repository have on decision-making and project outcomes? What strategies can organizations implement to encourage widespread adoption and active participation in the research repository?
To broaden your pool of research questions, ask for further research questions or provide the same prompt in a new session. Repeat this process as many times as you need to generate a list that feels exhaustive of what you’d like to learn.
Some of the research questions Copilot generated for me were appropriate, and others were not. As a result, I had AI generate more research questions to choose from.
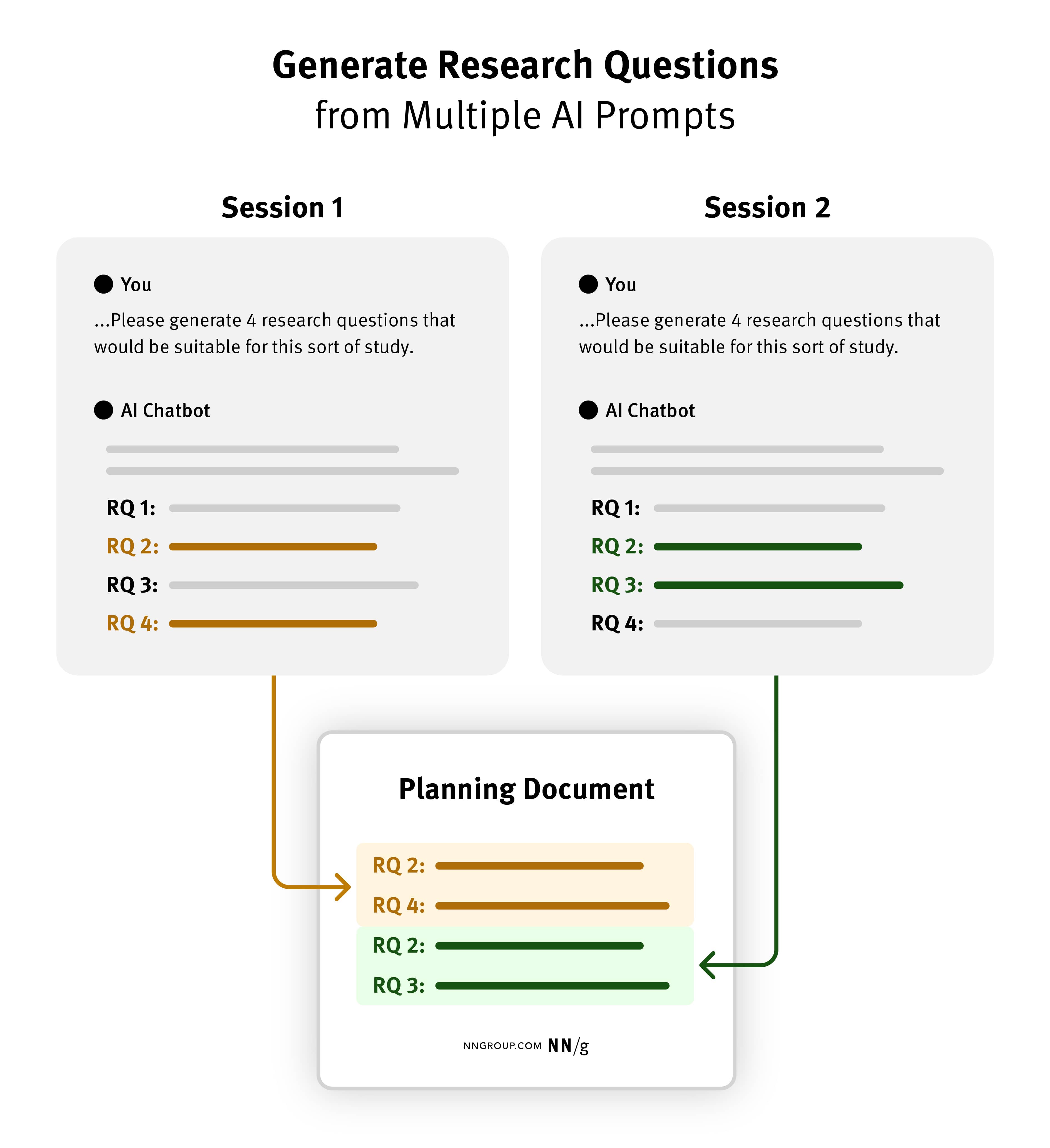
Unfortunately, most AI chatbots don’t offer an easy way to dismiss suggestions or combine specific responses and work from only these (a behavior called apple-picking ).
Pulling in generated research questions into an offline document (like a FigJam or Google Doc) allows you to easily group items, remove duplicates, or reword suggested research questions.
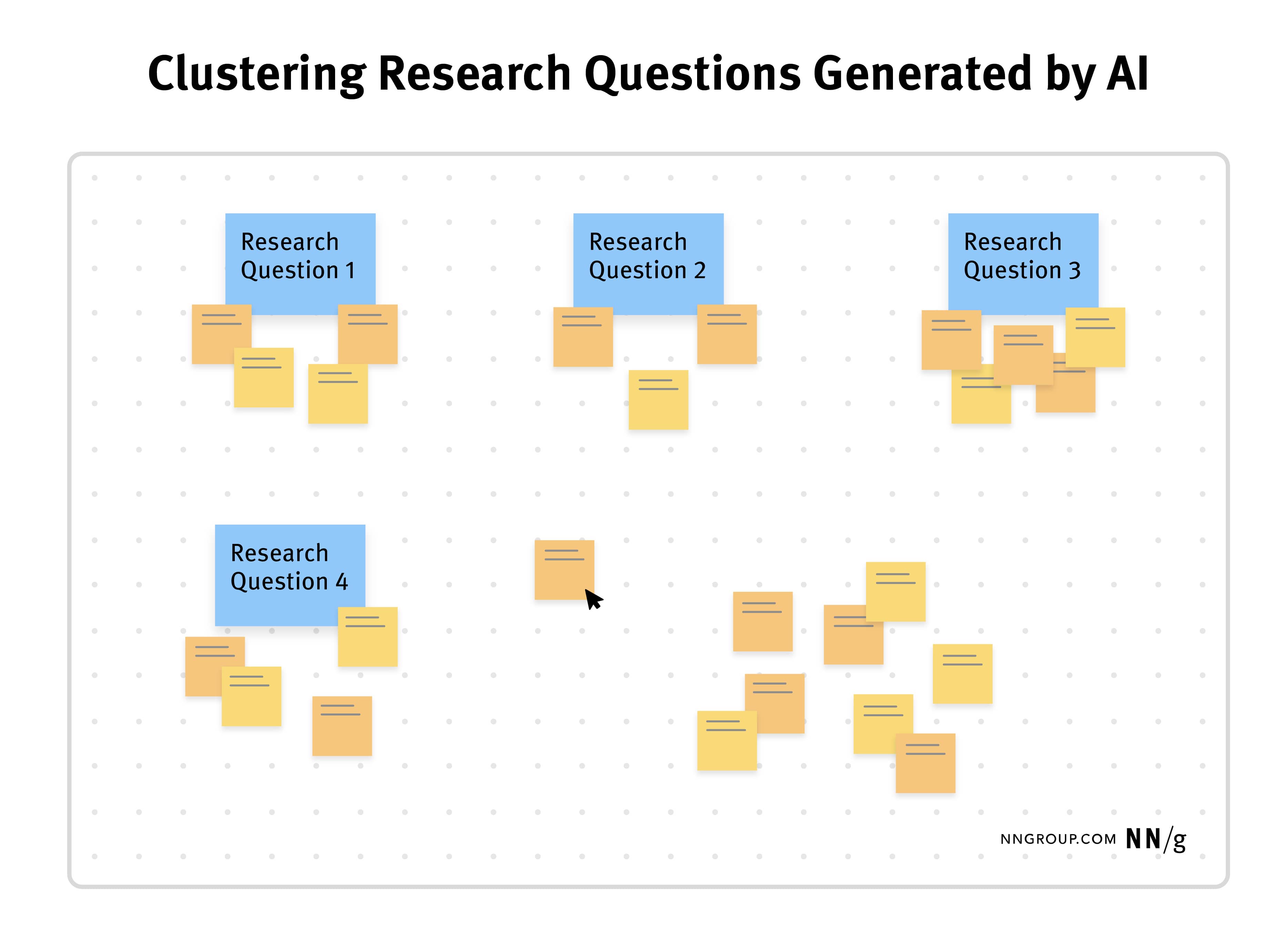
Begin a new chat session with your selected and refined set of research questions, so that the unwanted research questions are removed from the chat history.
Step 3: Request Methods
After sharing the context and your chosen research questions, ask the AI tool to identify suitable research methods.
Example Prompt : What study would you suggest to answer these research questions? Please be specific; cite which research questions would be answered by which research method if you suggest multiple methods.
Generative-AI advice is not always good advice. Often, these tools will suggest various methods and suggest you triangulate data from multiple sources. This approach is not always needed. Also, not all methods will be practical or the best fit for your study. Additionally, AI may suggest interviews and focus groups even for research questions better suited to a behavioral research method .
Ask AI chatbots to tell you which research methods would be suited to which research question and why. We also recommend doing some further reading on your own about any methods that are unfamiliar to you.
In response to the prompt above (and given my chosen research questions), ChatGPT recommended a survey, interviews with select UX practitioners, and case studies. These were all my chosen methods, so AI had done well here!
Step 4: Request Inclusion Criteria
AI can create inclusion criteria — a necessary component of your research plan. Do this step only after generating research questions and methods since these will inform who should participate in the research study.
Inclusion criteria (or recruitment criteria) are specific characteristics of the target population that need to be represented in your sample.
Start with inclusion criteria before asking the AI to help you write a screening questionnaire ; AI can only craft an appropriate screener after it “knows” who you’re looking to recruit.
Example Prompt: So that I recruit the right people for my interviews, help me create some inclusion criteria. What characteristics or behaviors should I recruit for?
Step 5: Request Help with Screeners, Interview Questions, and Tasks
Finally, ask the AI to put together:
- Interview questions or an interview guide (if conducting interviews)
- Tasks for a usability test
- Diary-study prompts (if relevant)
- Recruitment confirmation emails or other communication messages.
Unfortunately, there are a lot of bad examples of the above on the web. Conversational AI has been trained on all this data. Therefore, don’t be surprised if it produces poor study collateral on its first attempt! This is a major risk area for new researchers.
One way to mitigate this danger is to give the AI tool advice when crafting any of these outputs . Think of AI as a new research assistant who can learn extremely quickly.
Common mistakes that AI tools make include:
- Using words that appear in the interface in task instructions (priming)
- Creating task instructions that ask users to imagine they are someone that they are not
- Not including a goal or a call to action in the task instruction
- Not including distractor options in screening questionnaires
- Using overenthusiastic marketing language in recruitment materials
It’s not surprising that AI makes these mistakes since UX practitioners also make them!
To improve outputs, feed the AI essential tips, such as:
- When crafting tasks: Do not use the name of words or link labels in the task instruction. Find a natural-language equivalent to explain what the participant should do . (You can ask AI to “read” a website or an image.)
- When crafting recruitment materials: Use neutral and concise language in the recruitment email. Avoid using overly enthusiastic marketing language.
- When crafting a screener: Include multiple-choice questions and answer options that might disguise what the study is about and who I am looking to recruit.
Additionally, when possible, feed the AI with good examples of screener questionnaires, tasks, or interview questions, so it can follow their format or style.
Even with this advice, AI can still make mistakes. If you’re doubting its answers, check primary sources or speak with an experienced researcher for old-fashioned human guidance.
If you have ChatGPT’s Plus Plan, you can use our GPT for planning your research.
With the proper context, examples, and advice, AI tools, like ChatGPT and Microsoft Copilot, can craft helpful research questions, tasks, interview questions, and other study collateral far more quickly than you could if you started from scratch.
Research leads and ResearchOps personnel can support junior researchers and PWDRs (People Who Do Research) by providing examples and advice that can be fed to AI agents. Experienced researchers can benefit from using AI to speed up their research-planning process and obtain further inspiration.
Free Downloads
Related courses, practical ai for ux professionals.
Leverage artificial intelligence tools to enhance your UX work and save valuable time
Interaction
ResearchOps: Scaling User Research
Orchestrate and optimize research to amplify its impact
Analytics and User Experience
Study your users’ real-life behaviors and make data-informed design decisions
Related Topics
- Artificial Intelligence Artificial Intelligence
- Research Methods
Learn More:
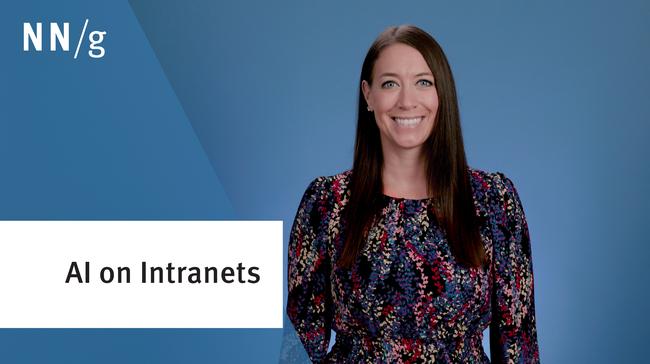
AI on Intranets: 5 Valuable Features
Anna Kaley · 3 min
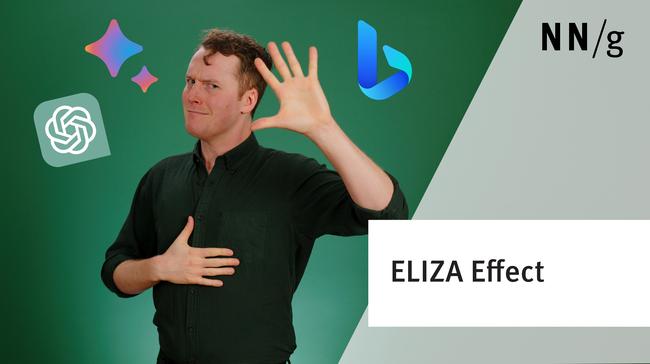
ELIZA Effect: Why We Fall in Love With AI
Caleb Sponheim · 3 min
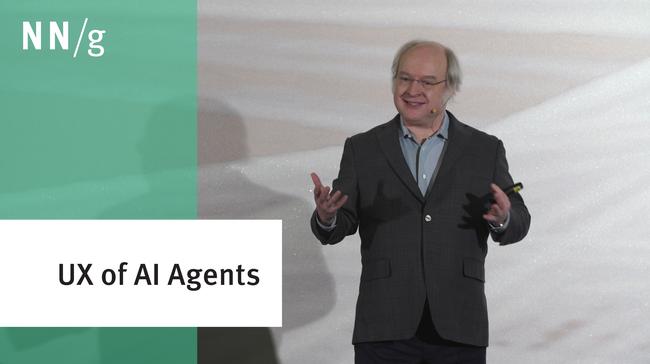
Is Aggressive Marketing Influencing the UX of AI Agents?
Jakob Nielsen · 2 min
Related Articles:
Generative UI and Outcome-Oriented Design
Kate Moran and Sarah Gibbons · 6 min
AI Chat Is Not (Always) the Answer
Sarah Gibbons and Kate Moran · 5 min
The UX of AI: Lessons from Perplexity
Kate Moran · 5 min
Response Outlining with Generative-AI Chatbots
Tarun Mugunthan · 3 min
Sycophancy in Generative-AI Chatbots
Caleb Sponheim · 4 min
Prompt Structure in Conversations with Generative AI
Raluca Budiu, Feifei Liu, Amy Zhang, and Emma Cionca · 16 min
Have a language expert improve your writing
Run a free plagiarism check in 10 minutes, generate accurate citations for free.
- Knowledge Base
Methodology
- What Is Qualitative Research? | Methods & Examples
What Is Qualitative Research? | Methods & Examples
Published on June 19, 2020 by Pritha Bhandari . Revised on June 22, 2023.
Qualitative research involves collecting and analyzing non-numerical data (e.g., text, video, or audio) to understand concepts, opinions, or experiences. It can be used to gather in-depth insights into a problem or generate new ideas for research.
Qualitative research is the opposite of quantitative research , which involves collecting and analyzing numerical data for statistical analysis.
Qualitative research is commonly used in the humanities and social sciences, in subjects such as anthropology, sociology, education, health sciences, history, etc.
- How does social media shape body image in teenagers?
- How do children and adults interpret healthy eating in the UK?
- What factors influence employee retention in a large organization?
- How is anxiety experienced around the world?
- How can teachers integrate social issues into science curriculums?
Table of contents
Approaches to qualitative research, qualitative research methods, qualitative data analysis, advantages of qualitative research, disadvantages of qualitative research, other interesting articles, frequently asked questions about qualitative research.
Qualitative research is used to understand how people experience the world. While there are many approaches to qualitative research, they tend to be flexible and focus on retaining rich meaning when interpreting data.
Common approaches include grounded theory, ethnography , action research , phenomenological research, and narrative research. They share some similarities, but emphasize different aims and perspectives.
Note that qualitative research is at risk for certain research biases including the Hawthorne effect , observer bias , recall bias , and social desirability bias . While not always totally avoidable, awareness of potential biases as you collect and analyze your data can prevent them from impacting your work too much.
Receive feedback on language, structure, and formatting
Professional editors proofread and edit your paper by focusing on:
- Academic style
- Vague sentences
- Style consistency
See an example
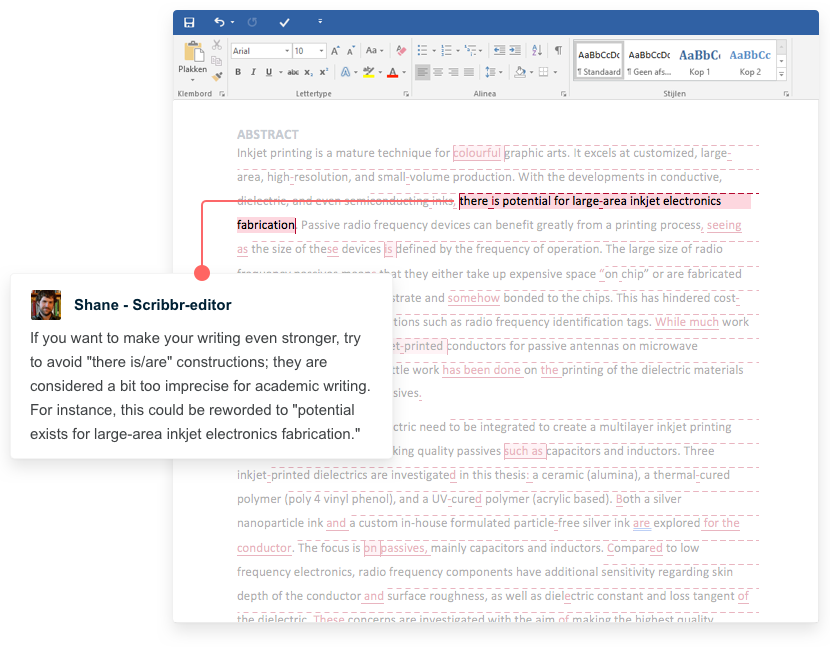
Each of the research approaches involve using one or more data collection methods . These are some of the most common qualitative methods:
- Observations: recording what you have seen, heard, or encountered in detailed field notes.
- Interviews: personally asking people questions in one-on-one conversations.
- Focus groups: asking questions and generating discussion among a group of people.
- Surveys : distributing questionnaires with open-ended questions.
- Secondary research: collecting existing data in the form of texts, images, audio or video recordings, etc.
- You take field notes with observations and reflect on your own experiences of the company culture.
- You distribute open-ended surveys to employees across all the company’s offices by email to find out if the culture varies across locations.
- You conduct in-depth interviews with employees in your office to learn about their experiences and perspectives in greater detail.
Qualitative researchers often consider themselves “instruments” in research because all observations, interpretations and analyses are filtered through their own personal lens.
For this reason, when writing up your methodology for qualitative research, it’s important to reflect on your approach and to thoroughly explain the choices you made in collecting and analyzing the data.
Qualitative data can take the form of texts, photos, videos and audio. For example, you might be working with interview transcripts, survey responses, fieldnotes, or recordings from natural settings.
Most types of qualitative data analysis share the same five steps:
- Prepare and organize your data. This may mean transcribing interviews or typing up fieldnotes.
- Review and explore your data. Examine the data for patterns or repeated ideas that emerge.
- Develop a data coding system. Based on your initial ideas, establish a set of codes that you can apply to categorize your data.
- Assign codes to the data. For example, in qualitative survey analysis, this may mean going through each participant’s responses and tagging them with codes in a spreadsheet. As you go through your data, you can create new codes to add to your system if necessary.
- Identify recurring themes. Link codes together into cohesive, overarching themes.
There are several specific approaches to analyzing qualitative data. Although these methods share similar processes, they emphasize different concepts.
Qualitative research often tries to preserve the voice and perspective of participants and can be adjusted as new research questions arise. Qualitative research is good for:
- Flexibility
The data collection and analysis process can be adapted as new ideas or patterns emerge. They are not rigidly decided beforehand.
- Natural settings
Data collection occurs in real-world contexts or in naturalistic ways.
- Meaningful insights
Detailed descriptions of people’s experiences, feelings and perceptions can be used in designing, testing or improving systems or products.
- Generation of new ideas
Open-ended responses mean that researchers can uncover novel problems or opportunities that they wouldn’t have thought of otherwise.
Prevent plagiarism. Run a free check.
Researchers must consider practical and theoretical limitations in analyzing and interpreting their data. Qualitative research suffers from:
- Unreliability
The real-world setting often makes qualitative research unreliable because of uncontrolled factors that affect the data.
- Subjectivity
Due to the researcher’s primary role in analyzing and interpreting data, qualitative research cannot be replicated . The researcher decides what is important and what is irrelevant in data analysis, so interpretations of the same data can vary greatly.
- Limited generalizability
Small samples are often used to gather detailed data about specific contexts. Despite rigorous analysis procedures, it is difficult to draw generalizable conclusions because the data may be biased and unrepresentative of the wider population .
- Labor-intensive
Although software can be used to manage and record large amounts of text, data analysis often has to be checked or performed manually.
If you want to know more about statistics , methodology , or research bias , make sure to check out some of our other articles with explanations and examples.
- Chi square goodness of fit test
- Degrees of freedom
- Null hypothesis
- Discourse analysis
- Control groups
- Mixed methods research
- Non-probability sampling
- Quantitative research
- Inclusion and exclusion criteria
Research bias
- Rosenthal effect
- Implicit bias
- Cognitive bias
- Selection bias
- Negativity bias
- Status quo bias
Quantitative research deals with numbers and statistics, while qualitative research deals with words and meanings.
Quantitative methods allow you to systematically measure variables and test hypotheses . Qualitative methods allow you to explore concepts and experiences in more detail.
There are five common approaches to qualitative research :
- Grounded theory involves collecting data in order to develop new theories.
- Ethnography involves immersing yourself in a group or organization to understand its culture.
- Narrative research involves interpreting stories to understand how people make sense of their experiences and perceptions.
- Phenomenological research involves investigating phenomena through people’s lived experiences.
- Action research links theory and practice in several cycles to drive innovative changes.
Data collection is the systematic process by which observations or measurements are gathered in research. It is used in many different contexts by academics, governments, businesses, and other organizations.
There are various approaches to qualitative data analysis , but they all share five steps in common:
- Prepare and organize your data.
- Review and explore your data.
- Develop a data coding system.
- Assign codes to the data.
- Identify recurring themes.
The specifics of each step depend on the focus of the analysis. Some common approaches include textual analysis , thematic analysis , and discourse analysis .
Cite this Scribbr article
If you want to cite this source, you can copy and paste the citation or click the “Cite this Scribbr article” button to automatically add the citation to our free Citation Generator.
Bhandari, P. (2023, June 22). What Is Qualitative Research? | Methods & Examples. Scribbr. Retrieved April 9, 2024, from https://www.scribbr.com/methodology/qualitative-research/
Is this article helpful?
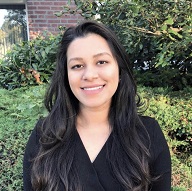
Pritha Bhandari
Other students also liked, qualitative vs. quantitative research | differences, examples & methods, how to do thematic analysis | step-by-step guide & examples, "i thought ai proofreading was useless but..".
I've been using Scribbr for years now and I know it's a service that won't disappoint. It does a good job spotting mistakes”
Featured Clinical Reviews
- Screening for Atrial Fibrillation: US Preventive Services Task Force Recommendation Statement JAMA Recommendation Statement January 25, 2022
- Evaluating the Patient With a Pulmonary Nodule: A Review JAMA Review January 18, 2022
- Download PDF
- Share X Facebook Email LinkedIn
- Permissions
Strengthening the FDA’s Enforcement of ClinicalTrials.gov Reporting Requirements
- 1 National Clinician Scholars Program, Yale School of Medicine, Department of Internal Medicine, New Haven, Connecticut
- 2 Veterans Affairs Connecticut Healthcare System and Yale University, West Haven, Connecticut
- 3 Columbia Law School, New York, New York
- 4 Global Health Justice Partnership, Yale Law School, New Haven, Connecticut
- 5 Section of General Internal Medicine, Yale School of Medicine, New Haven, Connecticut
- Research Letter Characterizing Gender Descriptions for Trials Registered on ClinicalTrials.gov Amanda Burton, PhD; Swapna Mohan, PhD; Yvonne Puplampu-Dove, PhD; Tony Tse, PhD JAMA
- Research Letter Results Reporting for Trials With the Same Sponsor, Drug, and Condition Kevin M. Fain, JD, MPH, DrPH; Thiyagu Rajakannan, MPharm, PhD; Tony Tse, PhD; Rebecca J. Williams, PharmD, MPH; Deborah A. Zarin, MD JAMA Internal Medicine
- Original Investigation Trends in ClinicalTrials.gov Registration Data by Sponsor Type, 2000-2019 Gillian Gresham, PhD; Jill L. Meinert; Arthur G. Gresham, BASc; Curtis L. Meinert, PhD JAMA Network Open
- Research Letter Characteristics and Strength of Evidence of COVID-19 Studies on ClinicalTrials.gov Krishna Pundi, MD; Alexander C. Perino, MD; Robert A. Harrington, MD; Harlan M. Krumholz, MD, SM; Mintu P. Turakhia, MD, MAS JAMA Internal Medicine
On April 28, 2021, the US Food and Drug Administration (FDA) announced that it “issued its first Notice of Noncompliance to Acceleron Pharma, Inc. (Acceleron) for failing to submit required summary results information to ClinicalTrials.gov.” 1 These results were based on a phase 2 trial that reached its primary completion date in June 2017. The trial examined the safety and efficacy of the candidate drug dalantercept in combination with an FDA-approved drug, axitinib, in patients with advanced renal cell carcinoma. Acceleron not only missed its 2018 deadline for submitting results to ClinicalTrials.gov, but also ignored an initial warning (Pre-Notice of Noncompliance) that the FDA sent the company in July 2020. 1 The FDA’s first Notice of Noncompliance was recognized as an important, if long overdue, step by the agency toward fulfilling its responsibility to enforce the federal law that requires clinical trial sponsors to disclose trial results to the public via ClinicalTrials.gov. But, the Notice of Noncompliance also prompts a key question: what comes next for noncompliant trial sponsors and the FDA?
Read More About
Ramachandran R , Morten CJ , Ross JS. Strengthening the FDA’s Enforcement of ClinicalTrials.gov Reporting Requirements. JAMA. 2021;326(21):2131–2132. doi:10.1001/jama.2021.19773
Manage citations:
© 2024
Artificial Intelligence Resource Center
Cardiology in JAMA : Read the Latest
Browse and subscribe to JAMA Network podcasts!
Others Also Liked
Select your interests.
Customize your JAMA Network experience by selecting one or more topics from the list below.
- Academic Medicine
- Acid Base, Electrolytes, Fluids
- Allergy and Clinical Immunology
- American Indian or Alaska Natives
- Anesthesiology
- Anticoagulation
- Art and Images in Psychiatry
- Artificial Intelligence
- Assisted Reproduction
- Bleeding and Transfusion
- Caring for the Critically Ill Patient
- Challenges in Clinical Electrocardiography
- Climate and Health
- Climate Change
- Clinical Challenge
- Clinical Decision Support
- Clinical Implications of Basic Neuroscience
- Clinical Pharmacy and Pharmacology
- Complementary and Alternative Medicine
- Consensus Statements
- Coronavirus (COVID-19)
- Critical Care Medicine
- Cultural Competency
- Dental Medicine
- Dermatology
- Diabetes and Endocrinology
- Diagnostic Test Interpretation
- Drug Development
- Electronic Health Records
- Emergency Medicine
- End of Life, Hospice, Palliative Care
- Environmental Health
- Equity, Diversity, and Inclusion
- Facial Plastic Surgery
- Gastroenterology and Hepatology
- Genetics and Genomics
- Genomics and Precision Health
- Global Health
- Guide to Statistics and Methods
- Hair Disorders
- Health Care Delivery Models
- Health Care Economics, Insurance, Payment
- Health Care Quality
- Health Care Reform
- Health Care Safety
- Health Care Workforce
- Health Disparities
- Health Inequities
- Health Policy
- Health Systems Science
- History of Medicine
- Hypertension
- Images in Neurology
- Implementation Science
- Infectious Diseases
- Innovations in Health Care Delivery
- JAMA Infographic
- Law and Medicine
- Leading Change
- Less is More
- LGBTQIA Medicine
- Lifestyle Behaviors
- Medical Coding
- Medical Devices and Equipment
- Medical Education
- Medical Education and Training
- Medical Journals and Publishing
- Mobile Health and Telemedicine
- Narrative Medicine
- Neuroscience and Psychiatry
- Notable Notes
- Nutrition, Obesity, Exercise
- Obstetrics and Gynecology
- Occupational Health
- Ophthalmology
- Orthopedics
- Otolaryngology
- Pain Medicine
- Palliative Care
- Pathology and Laboratory Medicine
- Patient Care
- Patient Information
- Performance Improvement
- Performance Measures
- Perioperative Care and Consultation
- Pharmacoeconomics
- Pharmacoepidemiology
- Pharmacogenetics
- Pharmacy and Clinical Pharmacology
- Physical Medicine and Rehabilitation
- Physical Therapy
- Physician Leadership
- Population Health
- Primary Care
- Professional Well-being
- Professionalism
- Psychiatry and Behavioral Health
- Public Health
- Pulmonary Medicine
- Regulatory Agencies
- Reproductive Health
- Research, Methods, Statistics
- Resuscitation
- Rheumatology
- Risk Management
- Scientific Discovery and the Future of Medicine
- Shared Decision Making and Communication
- Sleep Medicine
- Sports Medicine
- Stem Cell Transplantation
- Substance Use and Addiction Medicine
- Surgical Innovation
- Surgical Pearls
- Teachable Moment
- Technology and Finance
- The Art of JAMA
- The Arts and Medicine
- The Rational Clinical Examination
- Tobacco and e-Cigarettes
- Translational Medicine
- Trauma and Injury
- Treatment Adherence
- Ultrasonography
- Users' Guide to the Medical Literature
- Vaccination
- Venous Thromboembolism
- Veterans Health
- Women's Health
- Workflow and Process
- Wound Care, Infection, Healing
- Register for email alerts with links to free full-text articles
- Access PDFs of free articles
- Manage your interests
- Save searches and receive search alerts
- Victor Yocco
- Apr 9, 2024
Connecting With Users: Applying Principles Of Communication To UX Research
- 30 min read
- UX , User Research , Communication
- Share on Twitter , LinkedIn
About The Author
Victor is a Philadelphia based researcher, author, and speaker. His book Design for the Mind , is available from Manning Publications. Victor frequently writes … More about Victor ↬
Email Newsletter
Weekly tips on front-end & UX . Trusted by 200,000+ folks.
Communication is in everything we do. We communicate with users through our research, our design, and, ultimately, the products and services we offer. UX practitioners and those working on digital product teams benefit from understanding principles of communication and their application to our craft. Treating our UX processes as a mode of communication between users and the digital environment can help unveil in-depth, actionable insights.
In this article, I’ll focus on UX research. Communication is a core component of UX research , as it serves to bridge the gap between research insights, design strategy, and business outcomes. UX researchers, designers, and those working with UX researchers can apply key aspects of communication theory to help gather valuable insights, enhance user experiences, and create more successful products.
Fundamentals of Communication Theory
Communications as an academic field encompasses various models and principles that highlight the dynamics of communication between individuals and groups. Communication theory examines the transfer of information from one person or group to another. It explores how messages are transmitted, encoded, and decoded, acknowledges the potential for interference (or ‘noise’), and accounts for feedback mechanisms in enhancing the communication process.
In this article, I will focus on the Transactional Model of Communication . There are many other models and theories in the academic literature on communication. I have included references at the end of the article for those interested in learning more.
The Transactional Model of Communication (Figure 1) is a two-way process that emphasizes the simultaneous sending and receiving of messages and feedback . Importantly, it recognizes that communication is shaped by context and is an ongoing, evolving process. I’ll use this model and understanding when applying principles from the model to UX research. You’ll find that much of what is covered in the Transactional Model would also fall under general best practices for UX research, suggesting even if we aren’t communications experts, much of what we should be doing is supported by research in this field.
Understanding the Transactional Model
Let’s take a deeper dive into the six key factors and their applications within the realm of UX research:
- Sender: In UX research, the sender is typically the researcher who conducts interviews, facilitates usability tests, or designs surveys. For example, if you’re administering a user interview, you are the sender who initiates the communication process by asking questions.
- Receiver: The receiver is the individual who decodes and interprets the messages sent by the sender. In our context, this could be the user you interview or the person taking a survey you have created. They receive and process your questions, providing responses based on their understanding and experiences.
- Message: This is the content being communicated from the sender to the receiver. In UX research, the message can take various forms, like a set of survey questions, interview prompts, or tasks in a usability test.
- Channel: This is the medium through which the communication flows. For instance, face-to-face interviews, phone interviews, email surveys administered online, and usability tests conducted via screen sharing are all different communication channels. You might use multiple channels simultaneously, for example, communicating over voice while also using a screen share to show design concepts.
- Noise: Any factor that may interfere with the communication is regarded as ‘noise.’ In UX research, this could be complex jargon that confuses respondents in a survey, technical issues during a remote usability test, or environmental distractions during an in-person interview.
- Feedback: The communication received by the receiver, who then provides an output, is called feedback. For example, the responses given by a user during an interview or the data collected from a completed survey are types of feedback or the physical reaction of a usability testing participant while completing a task.
Applying the Transactional Model of Communication to Preparing for UX Research
We can become complacent or feel rushed to create our research protocols. I think this is natural in the pace of many workplaces and our need to deliver results quickly. You can apply the lens of the Transactional Model of Communication to your research preparation without adding much time. Applying the Transactional Model of Communication to your preparation should:
- Improve Clarity The model provides a clear representation of communication, empowering the researcher to plan and conduct studies more effectively.
- Minimize misunderstanding By highlighting potential noise sources, user confusion or misunderstandings can be better anticipated and mitigated.
- Enhance research participant participation With your attentive eye on feedback, participants are likely to feel valued, thus increasing active involvement and quality of input.
You can address the specific elements of the Transactional Model through the following steps while preparing for research:
Defining the Sender and Receiver
In UX research, the sender can often be the UX researcher conducting the study, while the receiver is usually the research participant. Understanding this dynamic can help researchers craft questions or tasks more empathetically and efficiently. You should try to collect some information on your participant in advance to prepare yourself for building a rapport.
For example, if you are conducting contextual inquiry with the field technicians of an HVAC company, you’ll want to dress appropriately to reflect your understanding of the context in which your participants (receivers) will be conducting their work. Showing up dressed in formal attire might be off-putting and create a negative dynamic between sender and receiver.
Message Creation
The message in UX research typically is the questions asked or tasks assigned during the study. Careful consideration of tenor, terminology, and clarity can aid data accuracy and participant engagement. Whether you are interviewing or creating a survey, you need to double-check that your audience will understand your questions and provide meaningful answers. You can pilot-test your protocol or questionnaire with a few representative individuals to identify areas that might cause confusion.
Using the HVAC example again, you might find that field technicians use certain terminology in a different way than you expect, such as asking them about what “tools” they use to complete their tasks yields you an answer that doesn’t reflect digital tools you’d find on a computer or smartphone, but physical tools like a pipe and wrench.
Choosing the Right Channel
The channel selection depends on the method of research. For instance, face-to-face methods might use physical verbal communication, while remote methods might rely on emails, video calls, or instant messaging. The choice of the medium should consider factors like tech accessibility, ease of communication, reliability, and participant familiarity with the channel. For example, you introduce an additional challenge (noise) if you ask someone who has never used an iPhone to test an app on an iPhone.
Minimizing Noise
Noise in UX research comes in many forms, from unclear questions inducing participant confusion to technical issues in remote interviews that cause interruptions. The key is to foresee potential issues and have preemptive solutions ready.
Facilitating Feedback
You should be prepared for how you might collect and act on participant feedback during the research. Encouraging regular feedback from the user during UX research ensures their understanding and that they feel heard. This could range from asking them to ‘think aloud’ as they perform tasks or encouraging them to email queries or concerns after the session. You should document any noise that might impact your findings and account for that in your analysis and reporting.
Track Your Alignment to the Framework
You can track what you do to align your processes with the Transactional Model prior to and during research using a spreadsheet. I’ll provide an example of a spreadsheet I’ve used in the later case study section of this article. You should create your spreadsheet during the process of preparing for research, as some of what you do to prepare should align with the factors of the model.
You can use these tips for preparation regardless of the specific research method you are undertaking. Let’s now look closer at a few common methods and get specific on how you can align your actions with the Transactional Model.
Applying the Transactional Model to Common UX Research Methods
UX research relies on interaction with users. We can easily incorporate aspects of the Transactional Model of Communication into our most common methods. Utilizing the Transactional Model in conducting interviews, surveys, and usability testing can help provide structure to your process and increase the quality of insights gathered.
Interviews are a common method used in qualitative UX research. They provide the perfect method for applying principles from the Transactional Model. In line with the Transactional Model, the researcher (sender) sends questions (messages) in-person or over the phone/computer medium (channel) to the participant (receiver), who provides answers (feedback) while contending with potential distraction or misunderstanding (noise). Reflecting on communication as transactional can help remind us we need to respect the dynamic between ourselves and the person we are interviewing. Rather than approaching an interview as a unidirectional interrogation, researchers need to view it as a conversation.
Applying the Transactional Model to conducting interviews means we should account for a number of facts to allow for high-quality communication. Note how the following overlap with what we typically call best practices.
Asking Open-ended Questions
To truly harness a two-way flow of communication, open-ended questions, rather than close-ended ones, are crucial. For instance, rather than asking, “Do you use our mobile application?” ask, “Can you describe your use of our mobile app?”. This encourages the participant to share more expansive and descriptive insights, furthering the dialogue.
Actively Listening
As the success of an interview relies on the participant’s responses, active listening is a crucial skill for UX researchers. The researcher should encourage participants to express their thoughts and feelings freely. Reflective listening techniques , such as paraphrasing or summarizing what the participant has shared, can reinforce to the interviewee that their contributions are being acknowledged and valued. It also provides an opportunity to clarify potential noise or misunderstandings that may arise.
Being Responsive
Building on the simultaneous send-receive nature of the Transactional Model, researchers must remain responsive during interviews. Providing non-verbal cues (like nodding) and verbal affirmations (“I see,” “Interesting”) lets participants know their message is being received and understood, making them feel comfortable and more willing to share.
We should always attempt to account for noise in advance, as well as during our interview sessions. Noise, in the form of misinterpretations or distractions, can disrupt effective communication. Researchers can proactively reduce noise by conducting a dry run in advance of the scheduled interviews . This helps you become more fluent at going through the interview and also helps identify areas that might need improvement or be misunderstood by participants. You also reduce noise by creating a conducive interview environment, minimizing potential distractions, and asking clarifying questions during the interview whenever necessary.
For example, if a participant uses a term the researcher doesn’t understand, the researcher should politely ask for clarification rather than guessing its meaning and potentially misinterpreting the data.
Additional forms of noise can include participant confusion or distraction. You should let participants know to ask if they are unclear on anything you say or do. It’s a good idea to always ask participants to put their smartphones on mute. You should only provide information critical to the process when introducing the interview or tasks. For example, you don’t need to give a full background of the history of the product you are researching if that isn’t required for the participant to complete the interview. However, you should let them know the purpose of the research, gain their consent to participate, and inform them of how long you expect the session to last.
Strategizing the Flow
Researchers should build strategic thinking into their interviews to support the Transaction Model. Starting the interview with less intrusive questions can help establish rapport and make the participant more comfortable, while more challenging or sensitive questions can be left for later when the interviewee feels more at ease.
A well-planned interview encourages a fluid dialogue and exchange of ideas. This is another area where conducting a dry run can help to ensure high-quality research. You and your dry-run participants should recognize areas where questions aren’t flowing in the best order or don’t make sense in the context of the interview, allowing you to correct the flow in advance.
While much of what the Transactional Model informs for interviews already aligns with common best practices, the model would suggest we need to have a deeper consideration of factors that we can sometimes give less consideration when we become overly comfortable with interviewing or are unaware of the implications of forgetting to address the factors of context considerations, power dynamics, and post-interview actions.
Context Considerations
You need to account for both the context of the participant, e.g., their background, demographic, and psychographic information, as well as the context of the interview itself. You should make subtle yet meaningful modifications depending on the channel you are conducting an interview.
For example, you should utilize video and be aware of your facial and physical responses if you are conducting an interview using an online platform, whereas if it’s a phone interview, you will need to rely on verbal affirmations that you are listening and following along, while also being mindful not to interrupt the participant while they are speaking.
Power Dynamics
Researchers need to be aware of how your role, background, and identity might influence the power dynamics of the interview. You can attempt to address power dynamics by sharing research goals transparently and addressing any potential concerns about bias a participant shares.
We are responsible for creating a safe and inclusive space for our interviews. You do this through the use of inclusive language, listening actively without judgment, and being flexible to accommodate different ways of knowing and expressing experiences. You should also empower participants as collaborators whenever possible . You can offer opportunities for participants to share feedback on the interview process and analysis. Doing this validates participants’ experiences and knowledge and ensures their voices are heard and valued.
Post-Interview Actions
You have a number of options for actions that can close the loop of your interviews with participants in line with the “feedback” the model suggests is a critical part of communication. Some tactics you can consider following your interview include:
- Debriefing Dedicate a few minutes at the end to discuss the participant’s overall experience, impressions, and suggestions for future interviews.
- Short surveys Send a brief survey via email or an online platform to gather feedback on the interview experience.
- Follow-up calls Consider follow-up calls with specific participants to delve deeper into their feedback and gain additional insight if you find that is warranted.
- Thank you emails Include a “feedback” section in your thank you email, encouraging participants to share their thoughts on the interview.
You also need to do something with the feedback you receive. Researchers and product teams should make time for reflexivity and critical self-awareness.
As practitioners in a human-focused field, we are expected to continuously examine how our assumptions and biases might influence our interviews and findings. “
We shouldn’t practice our craft in a silo. Instead, seeking feedback from colleagues and mentors to maintain ethical research practices should be a standard practice for interviews and all UX research methods.
By considering interviews as an ongoing transaction and exchange of ideas rather than a unidirectional Q&A, UX researchers can create a more communicative and engaging environment. You can see how models of communication have informed best practices for interviews. With a better knowledge of the Transactional Model, you can go deeper and check your work against the framework of the model.
The Transactional Model of Communication reminds us to acknowledge the feedback loop even in seemingly one-way communication methods like surveys. Instead of merely sending out questions and collecting responses, we need to provide space for respondents to voice their thoughts and opinions freely. When we make participants feel heard, engagement with our surveys should increase, dropouts should decrease, and response quality should improve.
Like other methods, surveys involve the researcher(s) creating the instructions and questionnaire (sender), the survey, including any instructions, disclaimers, and consent forms (the message), how the survey is administered, e.g., online, in person, or pen and paper (the channel), the participant (receiver), potential misunderstandings or distractions (noise), and responses (feedback).
Designing the Survey
Understanding the Transactional Model will help researchers design more effective surveys. Researchers are encouraged to be aware of both their role as the sender and to anticipate the participant’s perspective as the receiver. Begin surveys with clear instructions, explaining why you’re conducting the survey and how long it’s estimated to take. This establishes a more communicative relationship with respondents right from the start. Test these instructions with multiple people prior to launching the survey.
Crafting Questions
The questions should be crafted to encourage feedback and not just a simple yes or no. You should consider asking scaled questions or items that have been statistically validated to measure certain attributes of users.
For example, if you were looking deeper at a mobile banking application, rather than asking, “Did you find our product easy to use?” you would want to break that out into multiple aspects of the experience and ask about each with a separate question such as “On a scale of 1–7, with 1 being extremely difficult and 7 being extremely easy, how would you rate your experience transferring money from one account to another?” .
Reducing ‘noise,’ or misunderstandings, is crucial for increasing the reliability of responses. Your first line of defense in reducing noise is to make sure you are sampling from the appropriate population you want to conduct the research with. You need to use a screener that will filter out non-viable participants prior to including them in the survey. You do this when you correctly identify the characteristics of the population you want to sample from and then exclude those falling outside of those parameters.
Additionally, you should focus on prioritizing finding participants through random sampling from the population of potential participants versus using a convenience sample, as this helps to ensure you are collecting reliable data.
When looking at the survey itself, there are a number of recommendations to reduce noise. You should ensure questions are easily understandable, avoid technical jargon, and sequence questions logically. A question bank should be reviewed and tested before being finalized for distribution.
For example, question statements like “Do you use and like this feature?” can confuse respondents because they are actually two separate questions: do you use the feature, and do you like the feature? You should separate out questions like this into more than one question.
You should use visual aids that are relevant whenever possible to enhance the clarity of the questions. For example, if you are asking questions about an application’s “Dashboard” screen, you might want to provide a screenshot of that page so survey takers have a clear understanding of what you are referencing. You should also avoid the use of jargon if you are surveying a non-technical population and explain any terminology that might be unclear to participants taking the survey.
The Transactional Model suggests active participation in communication is necessary for effective communication . Participants can become distracted or take a survey without intending to provide thoughtful answers. You should consider adding a question somewhere in the middle of the survey to check that participants are paying attention and responding appropriately, particularly for longer surveys.
This is often done using a simple math problem such as “What is the answer to 1+1?” Anyone not responding with the answer of “2” might not be adequately paying attention to the responses they are providing and you’d want to look closer at their responses, eliminating them from your analysis if deemed appropriate.
Encouraging Feedback
While descriptive feedback questions are one way of promoting dialogue, you can also include areas where respondents can express any additional thoughts or questions they have outside of the set question list. This is especially useful in online surveys, where researchers can’t immediately address participant’s questions or clarify doubts.
You should be mindful that too many open-ended questions can cause fatigue , so you should limit the number of open-ended questions. I recommend two to three open-ended questions depending on the length of your overall survey.
Post-Survey Actions
After collecting and analyzing the data, you can send follow-up communications to the respondents. Let them know the changes made based on their feedback, thank them for their participation, or even share a summary of the survey results. This fulfills the Transactional Model’s feedback loop and communicates to the respondent that their input was received, valued, and acted upon.
You can also meet this suggestion by providing an email address for participants to follow up if they desire more information post-survey. You are allowing them to complete the loop themselves if they desire.
Applying the transactional model to surveys can breathe new life into the way surveys are conducted in UX research. It encourages active participation from respondents, making the process more interactive and engaging while enhancing the quality of the data collected. You can experiment with applying some or all of the steps listed above. You will likely find you are already doing much of what’s mentioned, however being explicit can allow you to make sure you are thoughtfully applying these principles from the field communication.
Usability Testing
Usability testing is another clear example of a research method highlighting components of the Transactional Model. In the context of usability testing, the Transactional Model of Communication’s application opens a pathway for a richer understanding of the user experience by positioning both the user and the researcher as sender and receiver of communication simultaneously.
Here are some ways a researcher can use elements of the Transactional Model during usability testing:
Task Assignment as Message Sending
When a researcher assigns tasks to a user during usability testing, they act as the sender in the communication process. To ensure the user accurately receives the message, these tasks need to be clear and well-articulated. For example, a task like “Register a new account on the app” sends a clear message to the user about what they need to do.
You don’t need to tell them how to do the task, as usually, that’s what we are trying to determine from our testing, but if you are not clear on what you want them to do, your message will not resonate in the way it is intended. This is another area where a dry run in advance of the testing is an optimal solution for making sure tasks are worded clearly.
Observing and Listening as Message Receiving
As the participant interacts with the application, concept, or design, the researcher, as the receiver, picks up on verbal and nonverbal cues. For instance, if a user is clicking around aimlessly or murmuring in confusion, the researcher can take these as feedback about certain elements of the design that are unclear or hard to use. You can also ask the user to explain why they are giving these cues you note as a way to provide them with feedback on their communication.
Real-time Interaction
The transactional nature of the model recognizes the importance of real-time interaction. For example, if during testing, the user is unsure of what a task means or how to proceed, the researcher can provide clarification without offering solutions or influencing the user’s action. This interaction follows the communication flow prescribed by the transactional model. We lose the ability to do this during unmoderated testing; however, many design elements are forms of communication that can serve to direct users or clarify the purpose of an experience (to be covered more in article two).
In usability testing, noise could mean unclear tasks, users’ preconceived notions, or even issues like slow software response. Acknowledging noise can help researchers plan and conduct tests better. Again, carrying out a pilot test can help identify any noise in the main test scenarios, allowing for necessary tweaks before actual testing. Other forms of noise can be less obvious but equally intrusive. For example, if you are conducting a test using a Macbook laptop and your participant is used to a PC, there is noise you need to account for, given their unfamiliarity with the laptop you’ve provided.
The fidelity of the design artifact being tested might introduce another form of noise. I’ve always advocated testing at any level of fidelity, but you should note that if you are using “Lorem Ipsum” or black and white designs, this potentially adds noise.
One of my favorite examples of this was a time when I was testing a financial services application, and the designers had put different balances on the screen; however, the total for all balances had not been added up to the correct total. Virtually every person tested noted this discrepancy, although it had nothing to do with the tasks at hand. I had to acknowledge we’d introduced noise to the testing. As at least one participant noted, they wouldn’t trust a tool that wasn’t able to total balances correctly.
Under the Transactional Model’s guidance, feedback isn’t just final thoughts after testing; it should be facilitated at each step of the process. Encouraging ‘think aloud’ protocols , where the user verbalizes their thoughts, reactions, and feelings during testing, ensures a constant flow of useful feedback.
You are receiving feedback throughout the process of usability testing, and the model provides guidance on how you should use that feedback to create a shared meaning with the participants. You will ultimately summarize this meaning in your report. You’ll later end up uncovering if this shared meaning was correctly interpreted when you design or redesign the product based on your findings.
We’ve now covered how to apply the Transactional Model of Communication to three common UX Research methods. All research with humans involves communication. You can break down other UX methods using the Model’s factors to make sure you engage in high-quality research.
Analyzing and Reporting UX Research Data Through the Lens of the Transactional Model
The Transactional Model of Communication doesn’t only apply to the data collection phase (interviews, surveys, or usability testing) of UX research. Its principles can provide valuable insights during the data analysis process.
The Transactional Model instructs us to view any communication as an interactive, multi-layered dialogue — a concept that is particularly useful when unpacking user responses. Consider the ‘message’ components: In the context of data analysis, the messages are the users’ responses. As researchers, thinking critically about how respondents may have internally processed the survey questions, interview discussion, or usability tasks can yield richer insights into user motivations.
Understanding Context
Just as the Transactional Model emphasizes the simultaneous interchange of communication, UX researchers should consider the user’s context while interpreting data. Decoding the meaning behind a user’s words or actions involves understanding their background, experiences, and the situation when they provide responses.
Deciphering Noise
In the Transactional Model, noise presents a potential barrier to effective communication. Similarly, researchers must be aware of snowballing themes or frequently highlighted issues during analysis. Noise, in this context, could involve patterns of confusion, misunderstandings, or consistently highlighted problems by users. You need to account for this, e.g., the example I provided where participants constantly referred to the incorrect math on static wireframes.
Considering Sender-Receiver Dynamics
Remember that as a UX researcher, your interpretation of user responses will be influenced by your understandings, biases, or preconceptions, just as the responses were influenced by the user’s perceptions. By acknowledging this, researchers can strive to neutralize any subjective influence and ensure the analysis remains centered on the user’s perspective. You can ask other researchers to double-check your work to attempt to account for bias.
For example, if you come up with a clear theme that users need better guidance in the application you are testing, another researcher from outside of the project should come to a similar conclusion if they view the data; if not, you should have a conversation with them to determine what different perspectives you are each bringing to the data analysis.
Reporting Results
Understanding your audience is crucial for delivering a persuasive UX research presentation. Tailoring your communication to resonate with the specific concerns and interests of your stakeholders can significantly enhance the impact of your findings. Here are some more details:
- Identify Stakeholder Groups Identify the different groups of stakeholders who will be present in your audience. This could include designers, developers, product managers, and executives.
- Prioritize Information Prioritize the information based on what matters most to each stakeholder group. For example, designers might be more interested in usability issues, while executives may prioritize business impact.
- Adapt Communication Style Adjust your communication style to align with the communication preferences of each group. Provide technical details for developers and emphasize user experience benefits for executives.
Acknowledging Feedback
Respecting this Transactional Model’s feedback loop, remember to revisit user insights after implementing design changes. This ensures you stay user-focused, continuously validating or adjusting your interpretations based on users’ evolving feedback. You can do this in a number of ways. You can reconnect with users to show them updated designs and ask questions to see if the issues you attempted to resolve were resolved.
Another way to address this without having to reconnect with the users is to create a spreadsheet or other document to track all the recommendations that were made and reconcile the changes with what is then updated in the design. You should be able to map the changes users requested to updates or additions to the product roadmap for future updates. This acknowledges that users were heard and that an attempt to address their pain points will be documented.
Crucially, the Transactional Model teaches us that communication is rarely simple or one-dimensional. It encourages UX researchers to take a more nuanced, context-aware approach to data analysis, resulting in deeper user understanding and more accurate, user-validated results.
By maintaining an ongoing feedback loop with users and continually refining interpretations, researchers can ensure that their work remains grounded in real user experiences and needs. “
Tracking Your Application of the Transactional Model to Your Practice
You might find it useful to track how you align your research planning and execution to the framework of the Transactional Model. I’ve created a spreadsheet to outline key factors of the model and used this for some of my work. Demonstrated below is an example derived from a study conducted for a banking client that included interviews and usability testing. I completed this spreadsheet during the process of planning and conducting interviews. Anonymized data from our study has been furnished to show an example of how you might populate a similar spreadsheet with your information.
You can customize the spreadsheet structure to fit your specific research topic and interview approach. By documenting your application of the transactional model, you can gain valuable insights into the dynamic nature of communication and improve your interview skills for future research.
You can use the suggested columns from this table as you see fit, adding or subtracting as needed, particularly if you use a method other than interviews. I usually add the following additional Columns for logistical purposes:
- Date of Interview,
- Participant ID,
- Interview Format (e.g., in person, remote, video, phone).
By incorporating aspects of communication theory into UX research, UX researchers and those who work with UX researchers can enhance the effectiveness of their communication strategies, gather more accurate insights, and create better user experiences. Communication theory provides a framework for understanding the dynamics of communication, and its application to UX research enables researchers to tailor their approaches to specific audiences, employ effective interviewing techniques, design surveys and questionnaires, establish seamless communication channels during usability testing, and interpret data more effectively.
As the field of UX research continues to evolve, integrating communication theory into research practices will become increasingly essential for bridging the gap between users and design teams, ultimately leading to more successful products that resonate with target audiences.
As a UX professional, it is important to continually explore and integrate new theories and methodologies to enhance your practice . By leveraging communication theory principles, you can better understand user needs, improve the user experience, and drive successful outcomes for digital products and services.
Integrating communication theory into UX research is an ongoing journey of learning and implementing best practices. Embracing this approach empowers researchers to effectively communicate their findings to stakeholders and foster collaborative decision-making, ultimately driving positive user experiences and successful design outcomes.
References and Further Reading
- The Mathematical Theory of Communication (PDF), Shannon, C. E., & Weaver, W.
- From organizational effectiveness to relationship indicators: Antecedents of relationships, public relations strategies, and relationship outcomes , Grunig, J. E., & Huang, Y. H.
- Communication and persuasion: Psychological studies of opinion change, Hovland, C. I., Janis, I. L., & Kelley, H. H. (1953). Yale University Press
- Communication research as an autonomous discipline, Chaffee, S. H. (1986). Communication Yearbook, 10, 243-274
- Interpersonal Communication: Everyday Encounters (PDF), Wood, J. (2015)
- Theories of Human Communication , Littlejohn, S. W., & Foss, K. A. (2011)
- McQuail’s Mass Communication Theory (PDF), McQuail, D. (2010)
- Bridges Not Walls: A Book About Interpersonal Communication , Stewart, J. (2012)
Smashing Newsletter
Tips on front-end & UX, delivered weekly in your inbox. Just the things you can actually use.
Front-End & UX Workshops, Online
With practical takeaways, live sessions, video recordings and a friendly Q&A.
TypeScript in 50 Lessons
Everything TypeScript, with code walkthroughs and examples. And other printed books.
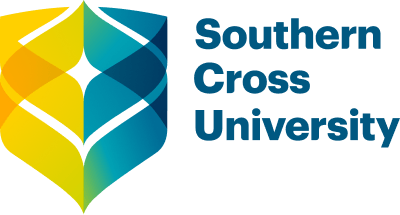
Main Navigation
/prod01/channel_8/media/scu-dep/current-students/images/Coffs-harbour_student-group_20220616_33.jpg)
- Accept offer and enrol
- Current Students
Personalise your experience
Did you mean..., diploma of arts and social sciences, art/science collaboration wins waterhouse natural science art prize, unit of study scin4003 scientific research context, perspective and methods 2 (2025).
Future students: T: 1800 626 481 E: Email your enquiry here
Current students: Contact: Faculty of Science and Engineering
Students studying at an education collaboration: Please contact your relevant institution
updated - DO NOT REMOVE THIS LINE 6:07 AM on Tue, 9 April
Show me unit information for year
Unit snapshot, credit points, faculty & college.
Faculty of Science and Engineering
Co-requisites
Students must have either completed, or enrol concurrently in, SCIN4002 - Scientific Research Context, Perspective and Methods 1
Unit description
Introduces science Honours students to the range of theoretical frameworks which may inform different types of scientific research and to the methods and methodologies which may be employed in the scientific research process. Encourages students to acquire the skills necessary to carry out, produce and report well designed and articulated research proposals and projects.
Unit content
Orientation: the nature of research; types of research.
The processes of developing a research project: planning and design; identifying the scope and range of a research project; the research design; research methods; formulating research questions; articulating research aims; the ethics of research.
Writing a research proposal: identification and articulation of theoretical frameworks and knowledge gaps relevant to your topic; literature reviews, identification and articulation of methodology; writing the text; citation and referencing; articulating expected outcomes; budget justification.
Introduction to research dissemination skills (scientific writing).
Availabilities
2025 unit offering information will be available in November 2024
Learning outcomes
Unit Learning Outcomes express learning achievement in terms of what a student should know, understand and be able to do on completion of a unit. These outcomes are aligned with the graduate attributes . The unit learning outcomes and graduate attributes are also the basis of evaluating prior learning.
On completion of this unit, students should be able to:
critically evaluate techniques and methods used in scientific research
demonstrate awareness of current scientific issues and methods
integrate scientific and management concepts and theories
develop a well designed and articulated research proposal including project summary, project description and budget.
Fee information
Commonwealth Supported courses For information regarding Student Contribution Amounts please visit the Student Contribution Amounts .
Fee paying courses For postgraduate or undergraduate full-fee paying courses please check Domestic Postgraduate Fees OR Domestic Undergraduate Fees .
International
Please check the international course and fee list to determine the relevant fees.
Courses that offer this unit
Bachelor of science with honours (2025), bachelor of science with honours (2024), any questions we'd love to help.
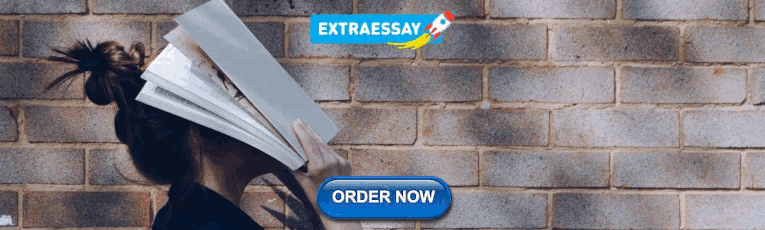
IMAGES
VIDEO
COMMENTS
Continue to all research methods & reporting articles. RMR articles discuss the nuts and bolts of doing and writing up research. For doctors interested in doing and interpreting clinical research. Also papers that present new or updated research reporting guidelines.
Research methods are specific procedures for collecting and analyzing data. Developing your research methods is an integral part of your research design. When planning your methods, there are two key decisions you will make. First, decide how you will collect data. Your methods depend on what type of data you need to answer your research question:
Step 1: Explain your methodological approach. Step 2: Describe your data collection methods. Step 3: Describe your analysis method. Step 4: Evaluate and justify the methodological choices you made. Tips for writing a strong methodology chapter. Other interesting articles.
Report all of the procedures applied for administering the study, processing the data, and for planned data analyses. Data collection methods and research design. Data collection methods refers to the general mode of the instruments: surveys, interviews, observations, focus groups, neuroimaging, cognitive tests, and so on. Summarize exactly how ...
About Research Methods. This guide provides an overview of research methods, how to choose and use them, and supports and resources at UC Berkeley. As Patten and Newhart note in the book Understanding Research Methods, "Research methods are the building blocks of the scientific enterprise. They are the "how" for building systematic knowledge.
The PRISMA 2020 statement replaces the 2009 statement and includes new reporting guidance that reflects advances in methods to identify, select, appraise, and synthesise studies. The structure and presentation of the items have been modified to facilitate implementation. In this article, we present the PRISMA 2020 27-item checklist, an expanded ...
research methods & reporting. objective of the review. Under a . Data sources. heading, they summarise sources that were searched, any lan-guage or publication type restrictions, and the start and end dates of searches. Study selection . statements then ideally describe who selected studies using what inclusion criteria. Data extraction methods ...
This chart lists the major biomedical research reporting guidelines that provide advice for reporting research methods and findings. They usually "specify a minimum set of items required for a clear and transparent account of what was done and what was found in a research study, reflecting, in particular, issues that might introduce bias into the research" (Adapted from the EQUATOR Network ...
Reporting guidelines are statements intended to advise authors reporting research methods and findings. They can be presented as a checklist, flow diagram or text, and describe what is required to give a clear and transparent account of a study's research and results. These guidelines are prepared through consideration of specific issues that may introduce bias, and are supported by the latest ...
Research methods & reporting Showing results 1-93. Sorted by: Most recent. Understanding how health interventions or exposures produce their effects using mediation analysis. July 19, 2023. A guide and pragmatic considerations for applying GRADE to network meta-analysis. June 27, 2023.
Thesis is a type of research report. A thesis is a long-form research document that presents the findings and conclusions of an original research study conducted by a student as part of a graduate or postgraduate program. It is typically written by a student pursuing a higher degree, such as a Master's or Doctoral degree, although it can also ...
A research design is a strategy for answering your research question using empirical data. Creating a research design means making decisions about: Your overall research objectives and approach. Whether you'll rely on primary research or secondary research. Your sampling methods or criteria for selecting subjects. Your data collection methods.
The PRISMA 2020 statement replaces the 2009 statement and includes new reporting guidance that reflects advances in methods to identify, select, appraise, and synthesise studies. The structure and presentation of the items have been modified to facilitate implementation. In this article, we present the PRISMA 2020 27-item checklist, an expanded ...
In this Review, Knight and colleagues discuss the best practices for performing a microbiome study, including experimental design, choice of molecular analysis technology, methods for data ...
The methods described within the papers matched the principles outlined in the guidance for the development of health research reporting guidelines . While some guideline developers utilised multiple components and others were more selective, we believe the overarching principles remained.
Quantitative research methods are used to collect and analyze numerical data. This type of research is useful when the objective is to test a hypothesis, determine cause-and-effect relationships, and measure the prevalence of certain phenomena. Quantitative research methods include surveys, experiments, and secondary data analysis.
bility to accommodate various paradigms, approaches, and methods. Method The authors identified guidelines, reporting standards, and critical appraisal criteria for qualitative research by searching PubMed, Web of Science, and Google through July 2013; reviewing the reference lists of retrieved sources; and contacting experts. Specifically, two authors reviewed a sample of sources to generate ...
The research methodology is an important section of any research paper or thesis, as it describes the methods and procedures that will be used to conduct the research. It should include details about the research design, data collection methods, data analysis techniques, and any ethical considerations.
A new section of the BMJ about how to do and write up research Nearly 15 years ago Doug Altman, the BMJ 's senior statistical adviser and professor of medical statistics, asked in this journal, "What should we think about researchers who use the wrong techniques (either wilfully or in ignorance), use the right techniques wrongly, misinterpret their results, report their results selectively ...
A research report is a collection of contextual data, gathered through organized research, that provides new insights into a particular challenge (which, for this article, is business-related). Research reports are a time-tested method for distilling large amounts of data into a narrow band of focus.
Olivia Guy-Evans, MSc. Research methods in psychology are systematic procedures used to observe, describe, predict, and explain behavior and mental processes. They include experiments, surveys, case studies, and naturalistic observations, ensuring data collection is objective and reliable to understand and explain psychological phenomena.
Abstract. Researchers must conduct research responsibly for it to have an impact and to safeguard trust in science. Essential responsibilities of researchers include using rigorous, reproducible research methods, reporting findings in a trustworthy manner, and giving the researchers who contributed appropriate authorship credit.
This course covers foundations of the research process for experimental Psychology: reviewing and evaluating published journal articles, refining new research questions, conducting pilot studies, creating stimuli, sequencing experiments for optimal control and data quality, analyzing data, and communicating scientific methods and results clearly, effectively, and professionally in APA style.
Abstract. Researchers must conduct research responsibly for it to have an impact and to safeguard trust in science. Essential responsibilities of researchers include using rigorous, reproducible research methods, reporting findings in a trustworthy manner, and giving the researchers who contributed appropriate authorship credit.
A research plan is a document that outlines the research objectives and how the research will be executed. Research plans should include: The research goals or questions that the research is hoping to achieve or answer; The method to be used and a description of how it will be carried out; The tasks or questions that will be given to study ...
Qualitative research involves collecting and analyzing non-numerical data (e.g., text, video, or audio) to understand concepts, opinions, or experiences. It can be used to gather in-depth insights into a problem or generate new ideas for research. Qualitative research is the opposite of quantitative research, which involves collecting and ...
On April 28, 2021, the US Food and Drug Administration (FDA) announced that it "issued its first Notice of Noncompliance to Acceleron Pharma, Inc. (Acceleron) for failing to submit required summary results information to ClinicalTrials.gov." 1 These results were based on a phase 2 trial that reached its primary completion date in June 2017. The trial examined the safety and efficacy of the ...
The channel selection depends on the method of research. For instance, face-to-face methods might use physical verbal communication, while remote methods might rely on emails, video calls, or instant messaging. ... Analyzing and Reporting UX Research Data Through the Lens of the Transactional Model. The Transactional Model of Communication ...
Introduces science Honours students to the range of theoretical frameworks which may inform different types of scientific research and to the methods and methodologies which may be employed in the scientific research process. Encourages students to acquire the skills necessary to carry out, produce and report well designed and articulated research proposals and projects.
research methods & reporting. Authors and presenters are responsible for how medi‑ cal research is interpreted and communicated. Often their work is the product of collaborations with other individuals (such as clinical investigators, biostatisticians, and professional medical writers) from around the world.