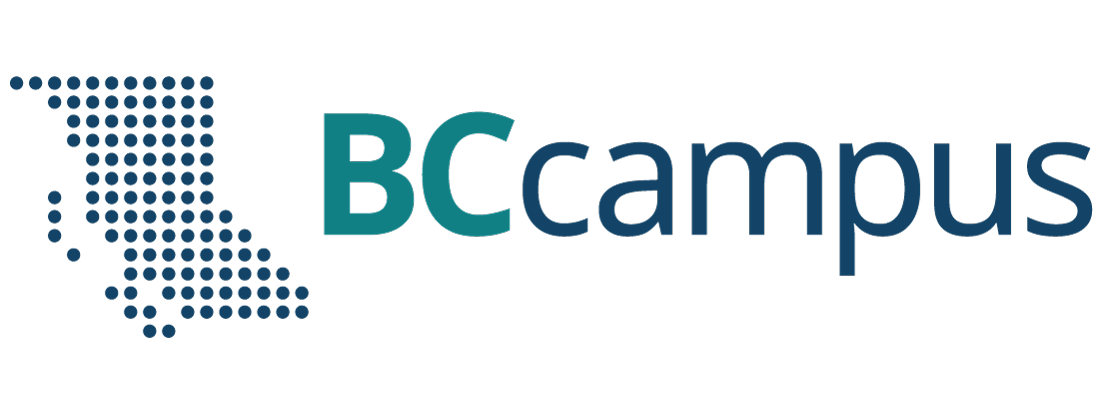
Want to create or adapt books like this? Learn more about how Pressbooks supports open publishing practices.
Chapter 10: Single-Subject Research
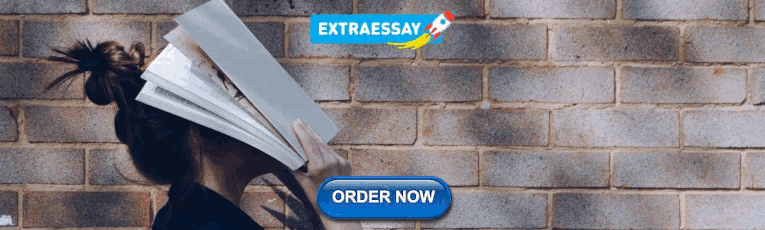
Single-Subject Research Designs
Learning Objectives
- Describe the basic elements of a single-subject research design.
- Design simple single-subject studies using reversal and multiple-baseline designs.
- Explain how single-subject research designs address the issue of internal validity.
- Interpret the results of simple single-subject studies based on the visual inspection of graphed data.
General Features of Single-Subject Designs
Before looking at any specific single-subject research designs, it will be helpful to consider some features that are common to most of them. Many of these features are illustrated in Figure 10.2, which shows the results of a generic single-subject study. First, the dependent variable (represented on the y -axis of the graph) is measured repeatedly over time (represented by the x -axis) at regular intervals. Second, the study is divided into distinct phases, and the participant is tested under one condition per phase. The conditions are often designated by capital letters: A, B, C, and so on. Thus Figure 10.2 represents a design in which the participant was tested first in one condition (A), then tested in another condition (B), and finally retested in the original condition (A). (This is called a reversal design and will be discussed in more detail shortly.)
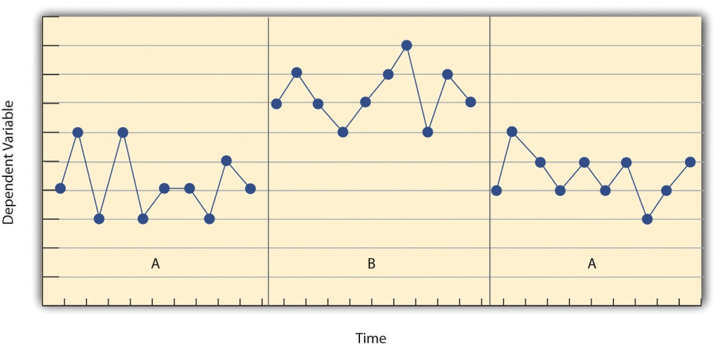
Another important aspect of single-subject research is that the change from one condition to the next does not usually occur after a fixed amount of time or number of observations. Instead, it depends on the participant’s behaviour. Specifically, the researcher waits until the participant’s behaviour in one condition becomes fairly consistent from observation to observation before changing conditions. This is sometimes referred to as the steady state strategy (Sidman, 1960) [1] . The idea is that when the dependent variable has reached a steady state, then any change across conditions will be relatively easy to detect. Recall that we encountered this same principle when discussing experimental research more generally. The effect of an independent variable is easier to detect when the “noise” in the data is minimized.
Reversal Designs
The most basic single-subject research design is the reversal design , also called the ABA design . During the first phase, A, a baseline is established for the dependent variable. This is the level of responding before any treatment is introduced, and therefore the baseline phase is a kind of control condition. When steady state responding is reached, phase B begins as the researcher introduces the treatment. There may be a period of adjustment to the treatment during which the behaviour of interest becomes more variable and begins to increase or decrease. Again, the researcher waits until that dependent variable reaches a steady state so that it is clear whether and how much it has changed. Finally, the researcher removes the treatment and again waits until the dependent variable reaches a steady state. This basic reversal design can also be extended with the reintroduction of the treatment (ABAB), another return to baseline (ABABA), and so on.
The study by Hall and his colleagues was an ABAB reversal design. Figure 10.3 approximates the data for Robbie. The percentage of time he spent studying (the dependent variable) was low during the first baseline phase, increased during the first treatment phase until it leveled off, decreased during the second baseline phase, and again increased during the second treatment phase.
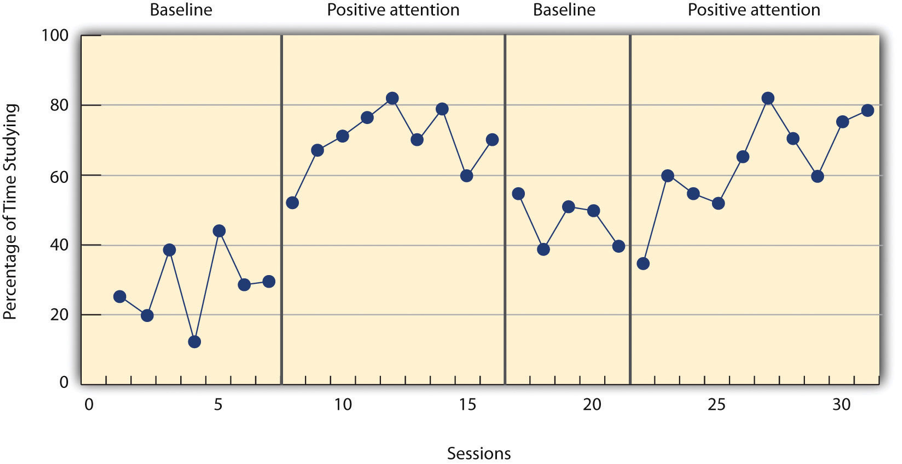
Why is the reversal—the removal of the treatment—considered to be necessary in this type of design? Why use an ABA design, for example, rather than a simpler AB design? Notice that an AB design is essentially an interrupted time-series design applied to an individual participant. Recall that one problem with that design is that if the dependent variable changes after the treatment is introduced, it is not always clear that the treatment was responsible for the change. It is possible that something else changed at around the same time and that this extraneous variable is responsible for the change in the dependent variable. But if the dependent variable changes with the introduction of the treatment and then changes back with the removal of the treatment (assuming that the treatment does not create a permanent effect), it is much clearer that the treatment (and removal of the treatment) is the cause. In other words, the reversal greatly increases the internal validity of the study.
There are close relatives of the basic reversal design that allow for the evaluation of more than one treatment. In a multiple-treatment reversal design , a baseline phase is followed by separate phases in which different treatments are introduced. For example, a researcher might establish a baseline of studying behaviour for a disruptive student (A), then introduce a treatment involving positive attention from the teacher (B), and then switch to a treatment involving mild punishment for not studying (C). The participant could then be returned to a baseline phase before reintroducing each treatment—perhaps in the reverse order as a way of controlling for carryover effects. This particular multiple-treatment reversal design could also be referred to as an ABCACB design.
In an alternating treatments design , two or more treatments are alternated relatively quickly on a regular schedule. For example, positive attention for studying could be used one day and mild punishment for not studying the next, and so on. Or one treatment could be implemented in the morning and another in the afternoon. The alternating treatments design can be a quick and effective way of comparing treatments, but only when the treatments are fast acting.
Multiple-Baseline Designs
There are two potential problems with the reversal design—both of which have to do with the removal of the treatment. One is that if a treatment is working, it may be unethical to remove it. For example, if a treatment seemed to reduce the incidence of self-injury in a developmentally disabled child, it would be unethical to remove that treatment just to show that the incidence of self-injury increases. The second problem is that the dependent variable may not return to baseline when the treatment is removed. For example, when positive attention for studying is removed, a student might continue to study at an increased rate. This could mean that the positive attention had a lasting effect on the student’s studying, which of course would be good. But it could also mean that the positive attention was not really the cause of the increased studying in the first place. Perhaps something else happened at about the same time as the treatment—for example, the student’s parents might have started rewarding him for good grades.
One solution to these problems is to use a multiple-baseline design , which is represented in Figure 10.4. In one version of the design, a baseline is established for each of several participants, and the treatment is then introduced for each one. In essence, each participant is tested in an AB design. The key to this design is that the treatment is introduced at a different time for each participant. The idea is that if the dependent variable changes when the treatment is introduced for one participant, it might be a coincidence. But if the dependent variable changes when the treatment is introduced for multiple participants—especially when the treatment is introduced at different times for the different participants—then it is extremely unlikely to be a coincidence.
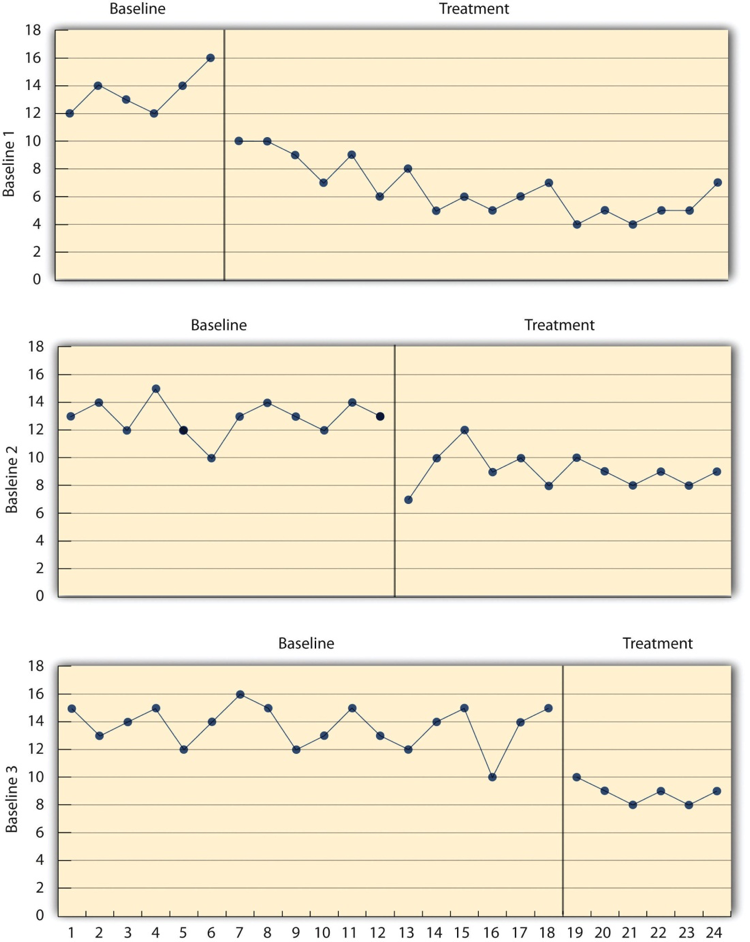
As an example, consider a study by Scott Ross and Robert Horner (Ross & Horner, 2009) [2] . They were interested in how a school-wide bullying prevention program affected the bullying behaviour of particular problem students. At each of three different schools, the researchers studied two students who had regularly engaged in bullying. During the baseline phase, they observed the students for 10-minute periods each day during lunch recess and counted the number of aggressive behaviours they exhibited toward their peers. (The researchers used handheld computers to help record the data.) After 2 weeks, they implemented the program at one school. After 2 more weeks, they implemented it at the second school. And after 2 more weeks, they implemented it at the third school. They found that the number of aggressive behaviours exhibited by each student dropped shortly after the program was implemented at his or her school. Notice that if the researchers had only studied one school or if they had introduced the treatment at the same time at all three schools, then it would be unclear whether the reduction in aggressive behaviours was due to the bullying program or something else that happened at about the same time it was introduced (e.g., a holiday, a television program, a change in the weather). But with their multiple-baseline design, this kind of coincidence would have to happen three separate times—a very unlikely occurrence—to explain their results.
In another version of the multiple-baseline design, multiple baselines are established for the same participant but for different dependent variables, and the treatment is introduced at a different time for each dependent variable. Imagine, for example, a study on the effect of setting clear goals on the productivity of an office worker who has two primary tasks: making sales calls and writing reports. Baselines for both tasks could be established. For example, the researcher could measure the number of sales calls made and reports written by the worker each week for several weeks. Then the goal-setting treatment could be introduced for one of these tasks, and at a later time the same treatment could be introduced for the other task. The logic is the same as before. If productivity increases on one task after the treatment is introduced, it is unclear whether the treatment caused the increase. But if productivity increases on both tasks after the treatment is introduced—especially when the treatment is introduced at two different times—then it seems much clearer that the treatment was responsible.
In yet a third version of the multiple-baseline design, multiple baselines are established for the same participant but in different settings. For example, a baseline might be established for the amount of time a child spends reading during his free time at school and during his free time at home. Then a treatment such as positive attention might be introduced first at school and later at home. Again, if the dependent variable changes after the treatment is introduced in each setting, then this gives the researcher confidence that the treatment is, in fact, responsible for the change.
Data Analysis in Single-Subject Research
In addition to its focus on individual participants, single-subject research differs from group research in the way the data are typically analyzed. As we have seen throughout the book, group research involves combining data across participants. Group data are described using statistics such as means, standard deviations, Pearson’s r , and so on to detect general patterns. Finally, inferential statistics are used to help decide whether the result for the sample is likely to generalize to the population. Single-subject research, by contrast, relies heavily on a very different approach called visual inspection . This means plotting individual participants’ data as shown throughout this chapter, looking carefully at those data, and making judgments about whether and to what extent the independent variable had an effect on the dependent variable. Inferential statistics are typically not used.
In visually inspecting their data, single-subject researchers take several factors into account. One of them is changes in the level of the dependent variable from condition to condition. If the dependent variable is much higher or much lower in one condition than another, this suggests that the treatment had an effect. A second factor is trend , which refers to gradual increases or decreases in the dependent variable across observations. If the dependent variable begins increasing or decreasing with a change in conditions, then again this suggests that the treatment had an effect. It can be especially telling when a trend changes directions—for example, when an unwanted behaviour is increasing during baseline but then begins to decrease with the introduction of the treatment. A third factor is latency , which is the time it takes for the dependent variable to begin changing after a change in conditions. In general, if a change in the dependent variable begins shortly after a change in conditions, this suggests that the treatment was responsible.
In the top panel of Figure 10.5, there are fairly obvious changes in the level and trend of the dependent variable from condition to condition. Furthermore, the latencies of these changes are short; the change happens immediately. This pattern of results strongly suggests that the treatment was responsible for the changes in the dependent variable. In the bottom panel of Figure 10.5, however, the changes in level are fairly small. And although there appears to be an increasing trend in the treatment condition, it looks as though it might be a continuation of a trend that had already begun during baseline. This pattern of results strongly suggests that the treatment was not responsible for any changes in the dependent variable—at least not to the extent that single-subject researchers typically hope to see.
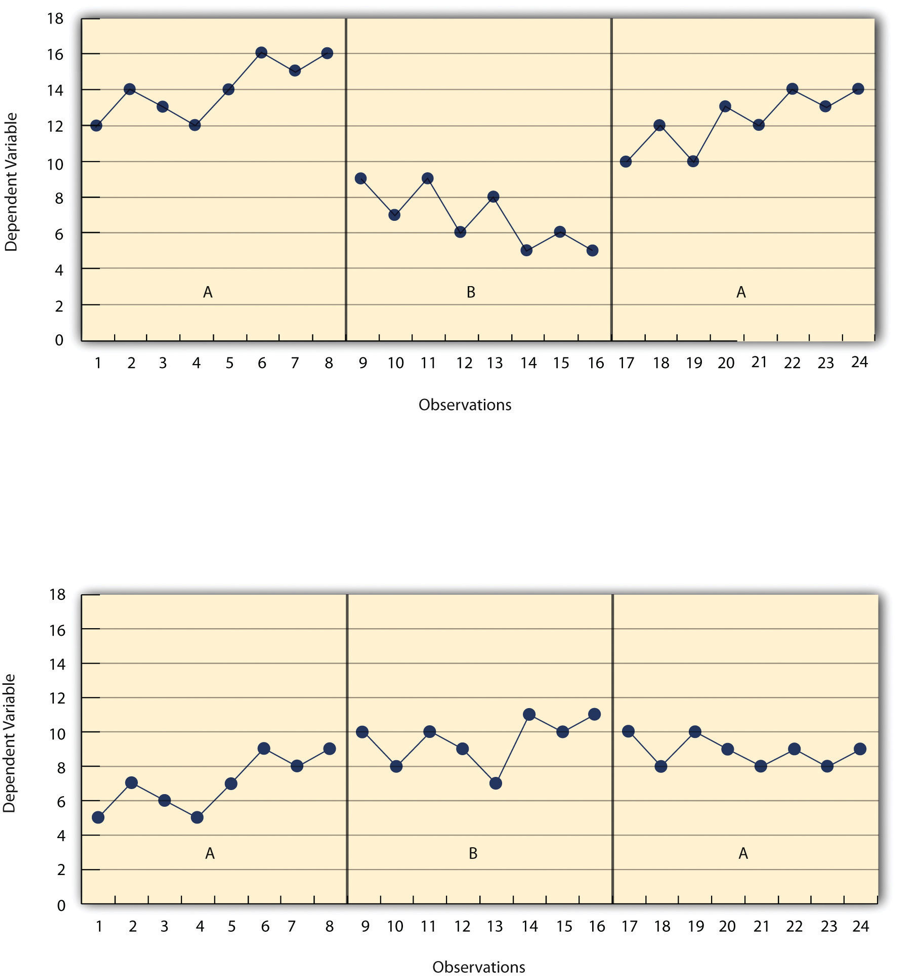
The results of single-subject research can also be analyzed using statistical procedures—and this is becoming more common. There are many different approaches, and single-subject researchers continue to debate which are the most useful. One approach parallels what is typically done in group research. The mean and standard deviation of each participant’s responses under each condition are computed and compared, and inferential statistical tests such as the t test or analysis of variance are applied (Fisch, 2001) [3] . (Note that averaging across participants is less common.) Another approach is to compute the percentage of nonoverlapping data (PND) for each participant (Scruggs & Mastropieri, 2001) [4] . This is the percentage of responses in the treatment condition that are more extreme than the most extreme response in a relevant control condition. In the study of Hall and his colleagues, for example, all measures of Robbie’s study time in the first treatment condition were greater than the highest measure in the first baseline, for a PND of 100%. The greater the percentage of nonoverlapping data, the stronger the treatment effect. Still, formal statistical approaches to data analysis in single-subject research are generally considered a supplement to visual inspection, not a replacement for it.
Key Takeaways
- Single-subject research designs typically involve measuring the dependent variable repeatedly over time and changing conditions (e.g., from baseline to treatment) when the dependent variable has reached a steady state. This approach allows the researcher to see whether changes in the independent variable are causing changes in the dependent variable.
- In a reversal design, the participant is tested in a baseline condition, then tested in a treatment condition, and then returned to baseline. If the dependent variable changes with the introduction of the treatment and then changes back with the return to baseline, this provides strong evidence of a treatment effect.
- In a multiple-baseline design, baselines are established for different participants, different dependent variables, or different settings—and the treatment is introduced at a different time on each baseline. If the introduction of the treatment is followed by a change in the dependent variable on each baseline, this provides strong evidence of a treatment effect.
- Single-subject researchers typically analyze their data by graphing them and making judgments about whether the independent variable is affecting the dependent variable based on level, trend, and latency.
- Does positive attention from a parent increase a child’s toothbrushing behaviour?
- Does self-testing while studying improve a student’s performance on weekly spelling tests?
- Does regular exercise help relieve depression?
- Practice: Create a graph that displays the hypothetical results for the study you designed in Exercise 1. Write a paragraph in which you describe what the results show. Be sure to comment on level, trend, and latency.
Long Descriptions
Figure 10.3 long description: Line graph showing the results of a study with an ABAB reversal design. The dependent variable was low during first baseline phase; increased during the first treatment; decreased during the second baseline, but was still higher than during the first baseline; and was highest during the second treatment phase. [Return to Figure 10.3]
Figure 10.4 long description: Three line graphs showing the results of a generic multiple-baseline study, in which different baselines are established and treatment is introduced to participants at different times.
For Baseline 1, treatment is introduced one-quarter of the way into the study. The dependent variable ranges between 12 and 16 units during the baseline, but drops down to 10 units with treatment and mostly decreases until the end of the study, ranging between 4 and 10 units.
For Baseline 2, treatment is introduced halfway through the study. The dependent variable ranges between 10 and 15 units during the baseline, then has a sharp decrease to 7 units when treatment is introduced. However, the dependent variable increases to 12 units soon after the drop and ranges between 8 and 10 units until the end of the study.
For Baseline 3, treatment is introduced three-quarters of the way into the study. The dependent variable ranges between 12 and 16 units for the most part during the baseline, with one drop down to 10 units. When treatment is introduced, the dependent variable drops down to 10 units and then ranges between 8 and 9 units until the end of the study. [Return to Figure 10.4]
Figure 10.5 long description: Two graphs showing the results of a generic single-subject study with an ABA design. In the first graph, under condition A, level is high and the trend is increasing. Under condition B, level is much lower than under condition A and the trend is decreasing. Under condition A again, level is about as high as the first time and the trend is increasing. For each change, latency is short, suggesting that the treatment is the reason for the change.
In the second graph, under condition A, level is relatively low and the trend is increasing. Under condition B, level is a little higher than during condition A and the trend is increasing slightly. Under condition A again, level is a little lower than during condition B and the trend is decreasing slightly. It is difficult to determine the latency of these changes, since each change is rather minute, which suggests that the treatment is ineffective. [Return to Figure 10.5]
- Sidman, M. (1960). Tactics of scientific research: Evaluating experimental data in psychology . Boston, MA: Authors Cooperative. ↵
- Ross, S. W., & Horner, R. H. (2009). Bully prevention in positive behaviour support. Journal of Applied Behaviour Analysis, 42 , 747–759. ↵
- Fisch, G. S. (2001). Evaluating data from behavioural analysis: Visual inspection or statistical models. Behavioural Processes, 54 , 137–154. ↵
- Scruggs, T. E., & Mastropieri, M. A. (2001). How to summarize single-participant research: Ideas and applications. Exceptionality, 9 , 227–244. ↵
The researcher waits until the participant’s behaviour in one condition becomes fairly consistent from observation to observation before changing conditions. This way, any change across conditions will be easy to detect.
A study method in which the researcher gathers data on a baseline state, introduces the treatment and continues observation until a steady state is reached, and finally removes the treatment and observes the participant until they return to a steady state.
The level of responding before any treatment is introduced and therefore acts as a kind of control condition.
A baseline phase is followed by separate phases in which different treatments are introduced.
Two or more treatments are alternated relatively quickly on a regular schedule.
A baseline is established for several participants and the treatment is then introduced to each participant at a different time.
The plotting of individual participants’ data, examining the data, and making judgements about whether and to what extent the independent variable had an effect on the dependent variable.
Whether the data is higher or lower based on a visual inspection of the data; a change in the level implies the treatment introduced had an effect.
The gradual increases or decreases in the dependent variable across observations.
The time it takes for the dependent variable to begin changing after a change in conditions.
The percentage of responses in the treatment condition that are more extreme than the most extreme response in a relevant control condition.
Research Methods in Psychology - 2nd Canadian Edition Copyright © 2015 by Paul C. Price, Rajiv Jhangiani, & I-Chant A. Chiang is licensed under a Creative Commons Attribution-NonCommercial-ShareAlike 4.0 International License , except where otherwise noted.
Share This Book

Using Single Subject Experimental Designs

What are the Characteristics of Single Subject Experimental Designs?
Single-subject designs are the staple of applied behavior analysis research. Those preparing for the BCBA exam or the BCaBA exam must know single subject terms and definitions. When choosing a single-subject experimental design, ABA researchers are looking for certain characteristics that fit their study. First, individuals serve as their own control in single subject research. In other words, the results of each condition are compared to the participant’s own data. If 3 people participate in the study, each will act as their own control. Second, researchers are trying to predict, verify, and replicate the outcomes of their intervention. Prediction, replication, and verification are essential to single-subject design research and help prove experimental control. Prediction: the hypothesis related to what the outcome will be when measured Verification : showing that baseline data would remain consistent if the independent variable was not manipulated Replication: repeating the independent variable manipulation to show similar results across multiple phases Some experimental designs like withdrawal designs are better suited for demonstrating experimental control than others, but each design has its place. We will now look at the different types of single subject experimental designs and the core features of each.
Reversal Design/Withdrawal Design/A-B-A
Arguably the simplest single subject design, the reversal/withdrawal design is excellent at identifying experimental control. First, baseline data is recorded. Then, an intervention is introduced and the effects are recorded. Finally, the intervention is withdrawn and the experiment returns to baseline. The researcher or researchers then visually analyze the changes from baseline to intervention and determine whether or not experimental control was established. Prediction, verification, and replication are also clearly demonstrated in the withdrawal design. Below is a simple example of this A-B-A design.
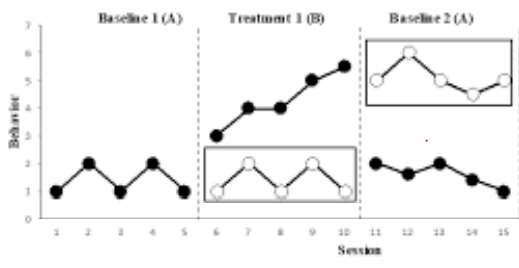
Advantages: Demonstrate experimental control Disadvantages: Ethical concerns, some behaviors cannot be reversed, not great for high-risk or dangerous behaviors
Multiple Baseline Design/Multiple Probe Design
Multiple baseline designs are used when researchers need to measure across participants, behaviors, or settings. For instance, if you wanted to examine the effects of an independent variable in a classroom, in a home setting, and in a clinical setting, you might use a multiple baseline across settings design. Multiple baseline designs typically involve 3-5 subjects, settings, or behaviors. An intervention is introduced into each segment one at a time while baseline continues in the other conditions. Below is a rough example of what a multiple baseline design typically looks like:

Multiple probe designs are identical to multiple baseline designs except baseline is not continuous. Instead, data is taken only sporadically during the baseline condition. You may use this if time and resources are limited, or you do not anticipate baseline changing. Advantages: No withdrawal needed, examine multiple dependent variables at a time Disadvantages : Sometimes difficult to demonstrate experimental control
Alternating Treatment Design
The alternating treatment design involves rapid/semirandom alternating conditions taking place all in the same phase. There are equal opportunities for conditions to be present during measurement. Conditions are alternated rapidly and randomly to test multiple conditions at once.

Advantages: No withdrawal, multiple independent variables can be tried rapidly Disadvantages : The multiple treatment effect can impact measurement
Changing Criterion Design
The changing criterion design is great for reducing or increasing behaviors. The behavior should already be in the subject’s repertoire when using changing criterion designs. Reducing smoking or increasing exercise are two common examples of the changing criterion design. With the changing criterion design, treatment is delivered in a series of ascending or descending phases. The criterion that the subject is expected to meet is changed for each phase. You can reverse a phase of a changing criterion design in an attempt to demonstrate experimental control.
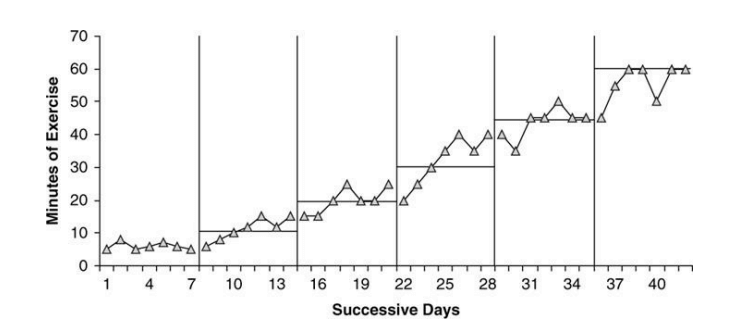
Summary of Single Subject Experimental Designs
Single subject designs are popular in both social sciences and in applied behavior analysis. As always, your research question and purpose should dictate your design choice. You will need to know experimental design and the details behind single subject design for the BCBA exam and the BCaBA exam. For BCBA exam study materials check out our BCBA exam prep. For a full breakdown of the BCBA fifth edition task list, check out our YouTube :
A Meta-Analysis of Single-Case Research on Applied Behavior Analytic Interventions for People With Down Syndrome
Affiliation.
- 1 Nicole Neil, Ashley Amicarelli, Brianna M. Anderson, and Kailee Liesemer, Western University, Canada.
- PMID: 33651891
- DOI: 10.1352/1944-7558-126.2.114
This systematic review evaluates single-case research design studies investigating applied behavior analytic (ABA) interventions for people with Down syndrome (DS). One hundred twenty-five studies examining the efficacy of ABA interventions on increasing skills and/or decreasing challenging behaviors met inclusion criteria. The What Works Clearinghouse standards and Risk of Bias in N-of-1 Trials scale were used to analyze methodological characteristics, and Tau-U effect sizes were calculated. Results suggest the use of ABA-based interventions are promising for behavior change in people with DS. Thirty-six high-quality studies were identified and demonstrated a medium overall effect. A range of outcomes was targeted, primarily involving communication and challenging behavior. These outcomes will guide future research on ABA interventions and DS.
Keywords: Down syndrome; Tau-U; applied behavior analysis; single-case research.
Publication types
- Meta-Analysis
- Systematic Review
- Behavior Therapy
- Communication
- Down Syndrome* / therapy
- Writing Center
- Brightspace
- Campus Directory
- My Library Items

Applied Behavior Analysis: Single Subject Research Design
- Find Articles
- Formatting the APA 7th Paper
- Using crossref.org
- Single Subject Research Design
Terms to Use for Articles
"reversal design" OR "withdrawal design" OR "ABAB design" OR "A-B-A-B design" OR "ABC design" OR "A-B-C design" OR "ABA design" OR "A-B-A design" OR "multiple baseline" OR "alternating treatments design" OR "multi-element design" OR "multielement design" OR "changing criterion design" OR "single case design" OR "single subject design" OR “single case series" or "single subject" or "single case"
Go To Databases
- ProQuest Education Database This link opens in a new window ProQuest Education Database indexes, abstracts, and provides full-text to leading scholarly and trade publications as well as reports in the field of education. Content includes primary, secondary, higher education, special education, home schooling, adult education, and more.
- PsycINFO This link opens in a new window PsycINFO, from the American Psychological Association's (APA), is a resource for abstracts of scholarly journal articles, book chapters, books, and dissertations across a range of disciplines in psychology. PsycINFO is indexed using APA's Thesaurus of Psychological Index Terms. Subscription ends 6/30/24.
Research Hints
Stimming – or self-stimulatory behaviour – is repetitive or unusual body movement or noises . Stimming might include:
- hand and finger mannerisms – for example, finger-flicking and hand-flapping
- unusual body movements – for example, rocking back and forth while sitting or standing
- posturing – for example, holding hands or fingers out at an angle or arching the back while sitting
- visual stimulation – for example, looking at something sideways, watching an object spin or fluttering fingers near the eyes
- repetitive behaviour – for example, opening and closing doors or flicking switches
- chewing or mouthing objects
- listening to the same song or noise over and over.
How to Search for a Specific Research Methodology in JABA
Single Case Design (Research Articles)
- Single Case Design (APA Dictionary of Psychology) an approach to the empirical study of a process that tracks a single unit (e.g., person, family, class, school, company) in depth over time. Specific types include the alternating treatments design, the multiple baseline design, the reversal design, and the withdrawal design. In other words, it is a within-subjects design with just one unit of analysis. For example, a researcher may use a single-case design for a small group of patients with a tic. After observing the patients and establishing the number of tics per hour, the researcher would then conduct an intervention and watch what happens over time, thus revealing the richness of any change. Such studies are useful for generating ideas for broader studies and for focusing on the microlevel concerns associated with a particular unit. However, data from these studies need to be evaluated carefully given the many potential threats to internal validity; there are also issues relating to the sampling of both the one unit and the process it undergoes. Also called N-of-1 design; N=1 design; single-participant design; single-subject (case) design.
- Anatomy of a Primary Research Article Document that goes through a research artile highlighting evaluative criteria for every section. Document from Mohawk Valley Community College. Permission to use sought and given
- Single Case Design (Explanation) Single case design (SCD), often referred to as single subject design, is an evaluation method that can be used to rigorously test the success of an intervention or treatment on a particular case (i.e., a person, school, community) and to also provide evidence about the general effectiveness of an intervention using a relatively small sample size. The material presented in this document is intended to provide introductory information about SCD in relation to home visiting programs and is not a comprehensive review of the application of SCD to other types of interventions.
- Single-Case Design, Analysis, and Quality Assessment for Intervention Research The purpose of this article is to describe single-case studies, and contrast them with case studies and randomized clinical trials Lobo, M. A., Moeyaert, M., Baraldi Cunha, A., & Babik, I. (2017). Single-case design, analysis, and quality assessment for intervention research. Journal of neurologic physical therapy : JNPT, 41(3), 187–197. https://doi.org/10.1097/NPT.0000000000000187
- The difference between a case study and single case designs There is a big difference between case studies and single case designs, despite them superficially sounding similar. (This is from a Blog posting)
- Single Case Design (Amanda N. Kelly, PhD, BCBA-D, LBA-aka Behaviorbabe) Despite the aka Behaviorbabe, Dr. Amanda N. Kelly, PhD, BCBA-D, LBA] provides a tutorial and explanation of single case design in simple terms.
- Lobo (2018). Single-Case Design, Analysis, and Quality Assessment for Intervention Research Lobo, M. A., Moeyaert, M., Cunha, A. B., & Babik, I. (2017). Single-case design, analysis, and quality assessment for intervention research. Journal of neurologic physical therapy: JNPT, 41(3), 187.. https://doi.org/10.1097/NPT.0000000000000187
- << Previous: Using crossref.org
- Next: Feedback >>
- Last Updated: Apr 6, 2024 2:39 PM
- URL: https://mville.libguides.com/appliedbehavioranalysis
Applied Behavior Analysis
- Find Articles on a Topic
Two Ways to Find Single Subject Research Design (SSRD) Articles
Finding ssrd articles via the browsing method, finding ssrd articles via the searching method.
- Search by Article Citation in OneSearch
- Find Reading Lists (AKA 'Course Reserves')
- Get Articles We Don't Have through Interlibrary Loan
- Browse ABA Journals
- APA citation style
- Install LibKey Nomad
Types of Single Subject Research Design
Types of SSRDs to look for as you skim abstracts:
- reversal design
- withdrawal design
- ABAB design
- A-B-A-B design
- A-B-C design
- A-B-A design
- multiple baseline
- alternating treatments design
- multi-element design
- changing criterion design
- single case design
- single subject design
- single case series
Behavior analysts recognize the advantages of single-subject design for establishing intervention efficacy. Much of the research performed by behavior analysts will use SSRD methods.
When you need to find SSRD articles, there are two methods you can use:

- Click on a title from the list of ABA Journal Titles .
- Scroll down on the resulting page to the View Online section.
- Choose a link which includes the date range you're interested in.
- Click on a link to an issue (date) you want to explore.
- From the resulting Table of Contents, explore titles of interest, reading the abstract carefully for signs that the research was carried out using a SSRD. (To help, look for the box on this page with a list of SSRD types.)
- APA PsycInfo This link opens in a new window When you search in APA PsycInfo, you are searching through abstracts and descriptions of articles published in these ABA Journals in addition to thousands of other psychology-related journals. more... less... Description: PsycInfo is a key database in the field of psychology. Includes information of use to psychologists, students, and professionals in related fields such as psychiatry, management, business, and education, social science, neuroscience, law, medicine, and social work. Time Period: 1887 to present Sources: Indexes more than 2,500 journals. Subject Headings: Education, Mobile, Psychology, Social Sciences (Psychology) Scholarly or Popular: Scholarly Primary Materials: Journal Articles Information Included: Abstracts, Citations, Linked Full Text FindIt@BALL STATE: Yes Print Equivalent: None Publisher: American Psychological Association Updates: Monthly Number of Simultaneous Users: Unlimited

First , go to APA PsycInfo.
Second , copy and paste this set of terms describing different types of SSRDs into an APA PsycInfo search box, and choose "Abstract" in the drop-down menu.

Third , copy and paste this list of ABA journals into another search box in APA PsycInfo, and choose "SO Publication Name" in the drop-down menu.

Fourth , type in some keywords in another APA PsycInfo search box (or two) describing what you're researching. Use OR and add synonyms or related words for the best results.
Hit SEARCH, and see what kind of results you get!
Here's an example of a search for SSRDs in ABA journals on the topic of fitness:

Note that the long list of terms in the top two boxes gets cut off in the screenshot - - but they're all there!
The reason this works:
- To find SSRD articles, we can't just search on the phrase "single subject research" because many studies which use SSRD do not include that phrase anywhere in the text of the article; instead such articles typically specify in the abstract (and "Methods" section) what type of SSRD method was used (ex. withdrawal design, multiple baseline, or ABA design). That's why we string together all the possible descriptions of SSRD types with the word OR in between -- it enables us to search for any sort of SSRD, regardless of how it's described. Choosing "Abstract" in the drop-down menu ensures that we're focusing on these terms being used in the abstract field (not just popping up in discussion in the full-text).
- To search specifically for studies carried out in the field of Applied Behavior Analysis, we enter in the titles of the ABA journals, strung together, with OR in between. The quotation marks ensure each title is searched as a phrase. Choosing "SO Publication Name" in the drop-down menu ensures that results will be from articles published in those journals (not just references to those journals).
- To limit the search to a topic we're interested in, we type in some keywords in another search box. The more synonyms you can think of, the better; that ensures you'll have a decent pool of records to look through, including authors who may have described your topic differently.
Search ideas:
To limit your search to just the top ABA journals, you can use this shorter list in place of the long one above:
"Behavior Analysis in Practice" OR "Journal of Applied Behavior Analysis" OR "Journal of Behavioral Education" OR "Journal of Developmental and Physical Disabilities" OR "Journal of the Experimental Analysis of Behavior"
To get more specific, topic-wise, add another search box with another term (or set of terms), like in this example:

To search more broadly and include other psychology studies outside of ABA journals, simply remove the list of journal titles from the search, as shown here:

- << Previous: Find Articles on a Topic
- Next: Search by Article Citation in OneSearch >>
- Last Updated: Mar 1, 2024 3:30 PM
- URL: https://bsu.libguides.com/appliedbehavioranalysis

- Previous Article
- Next Article
A Meta-Analysis of Single-Case Research on Applied Behavior Analytic Interventions for People With Down Syndrome
- Article contents
- Figures & tables
- Supplementary Data
- Peer Review
- Get Permissions
- Cite Icon Cite
- Search Site
Nicole Neil , Ashley Amicarelli , Brianna M. Anderson , Kailee Liesemer; A Meta-Analysis of Single-Case Research on Applied Behavior Analytic Interventions for People With Down Syndrome. Am J Intellect Dev Disabil 1 March 2021; 126 (2): 114–141. doi: https://doi.org/10.1352/1944-7558-126.2.114
Download citation file:
- Ris (Zotero)
- Reference Manager
This systematic review evaluates single-case research design studies investigating applied behavior analytic (ABA) interventions for people with Down syndrome (DS). One hundred twenty-five studies examining the efficacy of ABA interventions on increasing skills and/or decreasing challenging behaviors met inclusion criteria. The What Works Clearinghouse standards and Risk of Bias in N-of-1 Trials scale were used to analyze methodological characteristics, and Tau-U effect sizes were calculated. Results suggest the use of ABA-based interventions are promising for behavior change in people with DS. Thirty-six high-quality studies were identified and demonstrated a medium overall effect. A range of outcomes was targeted, primarily involving communication and challenging behavior. These outcomes will guide future research on ABA interventions and DS.
Client Account
Sign in via your institution.
AJIDD Call for Papers
The American Journal on Intellectual and Developmental Disabilities is looking for high-quality research articles on bio-psycho-social processes and their role in the healthy development and behavioral outcomes for people with IDD across the lifespan. For more information, contact the AJIDD editor, Frank Symons ( [email protected] ) or go to the AJIDD author instructions at https://meridian.allenpress.com/ajidd/pages/Info-for-Authors .
AAIDD Members
To access the journals, use your member log in credentials on the AAIDD website and return here to gain access. Your member credentials do not work with the login widgets on these pages. Always log in on the AAIDD website .
Citing articles via
Get email alerts.
- American Association on Intellectual and Developmental Disabilities
- eISSN 1944-7558
- ISSN 1944-7515
- Privacy Policy
- Get Adobe Acrobat Reader
This Feature Is Available To Subscribers Only
Sign In or Create an Account

An official website of the United States government
The .gov means it’s official. Federal government websites often end in .gov or .mil. Before sharing sensitive information, make sure you’re on a federal government site.
The site is secure. The https:// ensures that you are connecting to the official website and that any information you provide is encrypted and transmitted securely.
- Publications
- Account settings
Preview improvements coming to the PMC website in October 2024. Learn More or Try it out now .
- Advanced Search
- Journal List
- v.37(1); 2014 May
The Evidence-Based Practice of Applied Behavior Analysis
Timothy a. slocum.
Utah State University, Logan, UT USA
Ronnie Detrich
Wing Institute, Oakland, CA USA
Susan M. Wilczynski
Ball State University, Muncie, IN USA
Trina D. Spencer
Northern Arizona University, Flagstaff, AZ USA
Oregon State University, Corvallis, OR USA
Katie Wolfe
University of South Carolina, Columbia, SC USA
Evidence-based practice (EBP) is a model of professional decision-making in which practitioners integrate the best available evidence with client values/context and clinical expertise in order to provide services for their clients. This framework provides behavior analysts with a structure for pervasive use of the best available evidence in the complex settings in which they work. This structure recognizes the need for clear and explicit understanding of the strength of evidence supporting intervention options, the important contextual factors including client values that contribute to decision making, and the key role of clinical expertise in the conceptualization, intervention, and evaluation of cases. Opening the discussion of EBP in this journal, Smith ( The Behavior Analyst, 36 , 7–33, 2013 ) raised several key issues related to EBP and applied behavior analysis (ABA). The purpose of this paper is to respond to Smith’s arguments and extend the discussion of the relevant issues. Although we support many of Smith’s ( The Behavior Analyst, 36 , 7–33, 2013 ) points, we contend that Smith’s definition of EBP is significantly narrower than definitions that are used in professions with long histories of EBP and that this narrowness conflicts with the principles that drive applied behavior analytic practice. We offer a definition and framework for EBP that aligns with the foundations of ABA and is consistent with well-established definitions of EBP in medicine, psychology, and other professions. In addition to supporting the systematic use of research evidence in behavior analytic decision making, this definition can promote clear communication about treatment decisions across disciplines and with important outside institutions such as insurance companies and granting agencies.
Almost 45 years ago, Baer et al. ( 1968 ) described a new discipline—applied behavior analysis (ABA). This discipline was distinguished from the experimental analysis of behavior by its focus on social impact (i.e., solving socially important problems in socially important settings). ABA has produced remarkably powerful interventions in fields such as education, developmental disabilities and autism, clinical psychology, behavioral medicine, organizational behavior management, and a host of other fields and populations. Behavior analysts have long recognized that developing interventions capable of improving client behavior solves only one part of the problem. The problem of broad social impact must be solved by having interventions implemented effectively in socially important settings and at scales of social importance (Baer et al. 1987 ; Horner et al. 2005b ; McIntosh et al. 2010 ). This latter set of challenges has proved to be more difficult. In many cases, demonstrations of effectiveness are not sufficient to produce broad adoption and careful implementation of these procedures. Key decision makers may be more influenced by variables other than the increases and decreases in the behaviors of our clients. In addition, even when client behavior is a very powerful factor in decision making, it does not guarantee that empirical data will be the basis for treatment selection; anecdotes, appeals to philosophy, or marketing have been given priority over evidence of outcomes (Carnine 1992 ; Polsgrove 2003 ).
Across settings in which behavior analysts work, there has been a persistent gap between what is known from research and what is actually implemented in practice. Behavior analysts have been concerned with the failed adoption of research-based practices for years (Baer et al. 1987 ). Even in the fields in which behavior analysts have produced powerful interventions, the vast majority of current practice fails to take advantage of them.
Behavior analysts have not been alone in recognizing serious problems with the quality of interventions used employed in practice settings. In the 1960s, many within the medical field recognized a serious research-to-practice gap. Studies suggested that a relatively small percentage (estimates range from 10 to 25 %) of medical treatment decisions were based on high-quality evidence (Goodman 2003 ). This raised the troubling question of what basis was used for the remaining decisions if it was not high-quality evidence. These concerns led to the development of evidence-based practice (EBP) of medicine (Goodman 2003 ; Sackett et al. 1996 ).
The research-to-practice gap appears to be universal across professions. For example, Kazdin ( 2000 ) has reported that less than 10 % of the child and adolescent mental health treatments reported in the professional literature have been systematically evaluated and found to be effective and those that have not been evaluated are more likely to be adopted in practice settings. In recognition of their own research-to-practice gaps, numerous professions have adopted an EBP framework. Nursing and other areas of health care, social work, clinical and educational psychology, speech and language pathology, and many others have adopted this framework and adapted it to the specific needs of their discipline to help guide decision-making. Not only have EBP frameworks been helping to structure professional practice, but they have also been used to guide federal policy. With the passage of No Child Left Behind ( 2002 ) and the reauthorization of the Individuals with Disabilities Education Improvement Act ( 2005 ), the federal department of education has aligned itself with the EBP movement. A recent memorandum from the federal Office of Management and Budget instructed agencies to consider evidence of effectiveness when awarding funds, increase the use of evidence in competitions, and to encourage widespread program evaluation (Zients 2012 ). The memo, which used the term evidence-based practice extensively, stated: “Where evidence is strong, we should act on it. Where evidence is suggestive, we should consider it. Where evidence is weak, we should build the knowledge to support better decisions in the future” (Zients 2012 , p. 1).
EBP is more broadly an effort to improve decision-making in applied settings by explicitly articulating the central role of evidence in these decisions and thereby improving outcomes. It addresses one of the long-standing challenges for ABA; the need to effectively support and disseminate interventions in the larger social systems in which our work is embedded. In particular, EBP addresses the fact that many decision-makers are not sufficiently influenced by the best evidence that is relevant to important decisions. EBP is an explicit statement of one of ABA’s core tenets—a commitment to evidence-based decision-making. Given that the EBP framework is well established in many disciplines closely related to ABA and in the larger institutional contexts in which we operate (e.g., federal policy and funding agencies), aligning ABA with EBP offers an opportunity for behavior analysts to work more effectively within broader social systems.
Discussion of issues related to EBP in ABA has taken place across several years. Researchers have extensively discussed methods for identifying well-supported treatments (e.g., Horner et al. 2005a ; Kratochwill et al. 2010 ), and systematically reviewed the evidence to identify these treatments (e.g., Maggin et al. 2011 ; National Autism Center 2009 ). However, until recently, discussion of an explicit definition of EBP in ABA has been limited to conference papers (e.g., Detrich 2009 ). Smith ( 2013 ) opened a discussion of the definition and critical features of EBP of ABA in the pages of The Behavior Analyst . In his thought-provoking article, Smith raised many important points that deserve serious discussion as the field moves toward a clear vision of EBP of ABA. Most importantly, Smith ( 2013 ) argued that behavior analysts must carefully consider how EBP is to be defined and understood by researchers and practitioners of behavior analysis.
Definitions Matter
We find much to agree with in Smith’s paper, and we will describe these points of agreement below. However, we have a core disagreement with Smith concerning the vision of what EBP is and how it might enhance and expand the effective practice of ABA. As behavior analysts know, definitions matter. A well-conceived definition can promote conceptual understanding and set the context for effective action. Conversely, a poor definition or confusion about definitions hinders clear understanding, communication, and action.
In providing a basis for his definition of EBP, Smith refers to definitions in professions that have well-developed conceptions of EBP. He quotes the American Psychological Association (APA) ( 2005 ) definition (which we quote here more extensively than he did):
Evidence-based practice in psychology (EBPP) is the integration of the best available research with clinical expertise in the context of patient characteristics, culture, and preferences. This definition of EBPP closely parallels the definition of evidence-based practice adopted by the Institute of Medicine ( 2001 , p. 147) as adapted from Sackett et al. ( 2000 ): “Evidence-based practice is the integration of best research evidence with clinical expertise and patient values.” The purpose of EBPP is to promote effective psychological practice and enhance public health by applying empirically supported principles of psychological assessment, case formulation, therapeutic relationship, and intervention.
The key to understanding this definition is to note how APA and the Institute of Medicine use the word practice . Clearly, practice does not refer to an intervention; instead, it references one’s professional behavior. This is the sense in which one might speak of the professional practice of behavior analysis. American Psychological Association Presidential Task Force of Evidence-Based Practice ( 2006 ) further elaborates this point:
It is important to clarify the relation between EBPP and empirically supported treatments (ESTs)…. ESTs are specific psychological treatments that have been shown to be efficacious in controlled clinical trials, whereas EBPP encompasses a broader range of clinical activities (e.g., psychological assessment, case formulation, therapy relationships). As such, EBPP articulates a decision-making process for integrating multiple streams of research evidence—including but not limited to RCTs—into the intervention process. (p. 273)
In contrast, Smith defined EBP not as a decision-making process but as a set of interventions that have been shown to be efficacious through rigorous research. He stated:
An evidence-based practice is a service that helps solve a consumer’s problem. Thus it is likely to be an integrated package of procedures, operationalized in a manual, and validated in studies of socially meaningful outcomes, usually with group designs. (p. 27).
Smith’s EBP is what APA has clearly labeled an empirically supported treatment . This is a common misconception found in conversation and in published articles (e.g., Cook and Cook 2013 ) but at odds with formal definitions provided by many professional organizations; definitions which result from extensive consideration and debate by representative leaders of each professional field (e.g., APA 2005 ; American Occupational Therapy Association 2008 ; American Speech-Language Hearing Association 2005 ; Institute of Medicine 2001 ).
Before entering into the discussion of a useful definition of EBP of ABA, we should clarify the functions that we believe a useful definition of EBP should perform. First, a useful definition should align with the philosophical tenets of ABA, support the most effective current practice of ABA, and contribute to further improvement of ABA practice. A definition that is in conflict with the foundations of ABA or detracts from effective practice clearly would be counterproductive. Second, a useful definition of EBP of ABA should enhance social support for ABA practice by describing its empirical basis and decision-making processes in a way that is understandable to professions that already have well-established definitions of EBP. A definition that corresponds with the fundamental components of EBP in other fields would promote ABA practice by improving communication with external audiences. This improved communication is critical in the interdisciplinary contexts in which behavior analysts often practice and for legitimacy among those familiar with EBP who often control local contingencies (e.g., policy makers and funding agencies).
Based on these functions, we propose the following definition: Evidence-based practice of applied behavior analysis is a decision-making process that integrates (a) the best available evidence with (b) clinical expertise and (c) client values and context. This definition positions EBP as a pervasive feature of all professional decision-making by a behavior analyst with respect to client services; it is not limited to a narrowly restricted set of situations or decisions. The definition asserts that the best available evidence should be a primary influence on all decision-making related to services for clients (e.g., intervention selection, progress monitoring, etc.). It also recognizes that evidence cannot be the sole basis for a decision; effective decision-making in a discipline as complex as ABA requires clinical expertise in identifying, defining, and analyzing problems, determining what evidence is relevant, and deciding how it should be applied. In the absence of this decision-making framework, practitioners of ABA would be conceptualized as behavioral technicians rather than analysts. Further, the definition of EBP of ABA includes client values and context. Decision-making is necessarily based on a set of values that determine the goals that are to be pursued and the means that are appropriate to achieve them. Context is included in recognition of the fact that the effectiveness of an intervention is highly dependent upon the context in which it is implemented. The definition asserts that effective decision-making must be informed by important contextual factors. We elaborate on each component of the definition below, but first we contrast our definition with that offered by Smith ( 2013 ).
Although Smith ( 2013 ) made brief reference to the other critical components of EBP, he framed EBP as a list of multicomponent interventions that can claim a sufficient level of research support. We agree with his argument that such lists are valuable resources for practitioners and therefore developing them should be a goal of researchers. However, such lists are not, by themselves , a powerful means of improving the effectiveness of behavior analytic practice. The vast majority of decisions faced in the practice of behavior analysis cannot be made by implementing the kind of manualized, multicomponent treatment packages described by Smith.
There are a number of reasons a list of interventions is not an adequate basis for EBP of ABA. First, there are few interventions that qualify as “practices” under Smith’s definition. For example, when discussing the importance of manuals for operationalizing treatments, Smith stated that the requirement that a “practice” be based on a manual, “sharply reduces the number of ABA approaches that can be regarded as evidence based. Of the 11 interventions for ASD identified in the NAC ( 2009 ) report, only the three that have been standardized in manuals might be considered to be practices, and even these may be incomplete” (p. 18). Thus, although the example referenced the autism treatment literature, it seems apparent that even a loose interpretation of this particular criterion would leave all practitioners with a highly restricted number of intervention options.
Second, even if more “practices” were developed and validated, many consumers cannot be well served with existing multicomponent packages. In order to meet their clients’ needs, behavior analysts must be able to selectively implement focused interventions alone or in combination. This flexibility is necessary to meet the diverse needs of their clients and to minimize the response demands on direct care providers or staff, who are less likely to implement a complicated intervention with fidelity (Riley-Tillman and Chafouleas 2003 ).
Third, the strategy of assembling a list of treatments and describing these as “practices” severely limits the ways in which research findings are used by practitioners. With the list approach to defining EBP, research only impacts practice by placing an intervention on a list when a specific criteria has been met. Thus, any research on an intervention that is not sufficiently broad or manualized to qualify as a “practice” has no influence on EBP. Similarly, a research study that shows clear results but is not part of a sufficient body of support for an intervention would also have no influence. A study that provides suggestive results but is not methodologically strong enough to be definitive would have no influence, even if it were the only study that is relevant to a given problem.
The primary problem with a list approach is that it does not provide a strong framework that directs practitioners to include the best available evidence in all of their professional decision-making. Too often, practitioners who consult such lists find that no interventions relevant to their specific case have been validated as “evidence-based” and therefore EBP is irrelevant. In contrast, definitions of EBP as a decision-making process can provide a robust framework for including research evidence along with clinical expertise and client values and context in the practice of behavior analysis. In the next sections, we explore the components of this definition in more detail.
Best Available Evidence
The term “best available evidence” occupies a critical and central place in the definition and concept of EBP; this aligns with the fundamental reliance on scientific research that is one of the core tenets of ABA. The Behavior Analyst Certification Board ( 2010 ) Guidelines for Responsible Conduct for Behavior Analysts repeatedly affirm ways in which behavior analysts should base their professional conduct on the best available evidence. For example:
Behavior analysts rely on scientifically and professionally derived knowledge when making scientific or professional judgments in human service provision, or when engaging in scholarly or professional endeavors.
- The behavior analyst always has the responsibility to recommend scientifically supported most effective treatment procedures. Effective treatment procedures have been validated as having both long-term and short-term benefits to clients and society.
- Clients have a right to effective treatment (i.e., based on the research literature and adapted to the individual client).
A Continuum of Evidence Quality
The term best implies that evidence can be of varying quality, and that better quality evidence is preferred over lower quality evidence. Quality of evidence for informing a specific practical question involves two dimensions: (a) relevance of the evidence and (b) certainty of the evidence.
The dimension of relevance recognizes that some evidence is more germane to a particular decision than is other evidence. This idea is similar to the concept of external validity. External validity refers to the degree to which research results apply to a range of applied situations whereas relevance refers to the degree to which research results apply to a specific applied situation. In general, evidence is more relevant when it matches the particular situation in terms of (a) important characteristics of the clients, (b) specific treatments or interventions under consideration, (c) outcomes or target behaviors including their functions, and (d) contextual variables such as the physical and social environment, staff skills, and the capacity of the organization. Unless all conditions match perfectly, behavior analysts are necessarily required to use their expertise to determine the applicability of the scientific evidence to each unique clinical situation. Evidence based on functionally similar situations is preferred over evidence based on situations that share fewer important characteristics with the specific practice situation. However, functional similarity between a study or set of studies and a particular applied problem is not always obvious.
The dimension of certainty of evidence recognizes that some evidence provides stronger support for claims that a particular intervention produced a specific result. Any instance of evidence can be evaluated for its methodological rigor or internal validity (i.e., the degree to which it provides strong support for the claim of effectiveness and rules out alternative explanations). Anecdotes are clearly weaker than more systematic observations, and well-controlled experiments provide the strongest evidence. Methodological rigor extends to the quality of the dependent measure, treatment fidelity, and other variables of interest (e.g., maintenance of skill acquisition), all of which influence the certainty of evidence. But the internal validity of any particular study is not the only variable influencing the certainty of evidence; the quantity of evidence supporting a claim is also critical to its certainty. Both systematic and direct replication are vital for strengthening claims of effectiveness (Johnston and Pennypacker 1993 ; Sidman 1960 ). Certainty of evidence is based on both the rigor of each bit of evidence and the degree to which the findings have been consistently replicated. Although these issues are simple in principle, operationalizing and measuring rigor of research is extremely complex. Numerous quality appraisal systems for both group and single-subject research have been proposed and used in systematic reviews (see below for more detail).
Under ideal circumstances, consistently high-quality evidence that closely matches the specifics of the practice situation is available; unfortunately, this is not always the case, and evidence-based practitioners of ABA must proceed despite an imperfect evidence base. The mandate to use the best available evidence specifies that the practitioner make decisions based on the best evidence that is available. Although this statement may seem rather obvious, the point is worth underscoring because the implications are highly relevant to behavior analysts. In an area with considerable high-quality relevant research, the standards for evidence should be quite high. But in an area with more limited research, the practitioner should take advantage of the best evidence that is available. This may require tentative reliance on research that is somewhat weaker or is only indirectly relevant to the specific situation at hand. For example, ideally, evidence-based practitioners of ABA would rely on well-controlled experimental results that have been replicated with the precise population with whom they are working. However, if this kind of evidence is not available, they might have to make decisions based on a single study that involves a similar but not identical population.
This idea of using the best of the available evidence is very different from one of using only extremely high-quality evidence (i.e., empirically supported treatments). If we limit EBP to considering only the highest quality evidence, we leave the practitioner with no guidance in the numerous situations in which high-quality and directly relevant evidence (i.e., precise matching of setting, function, behavior, motivating operations and precise procedures) simply does not exist. This approach would lead to a form of EBP that is irrelevant to the majority of decisions that a behavior analyst must make on a daily basis. Instead, our proposed definition of EBP asserts that the practitioner should be informed by the best evidence that is available.
Expanding Research on Utility of Treatments
Smith ( 2013 ) argued that the research methods used by behavior analysts to evaluate these treatments should be expanded to more comprehensively describe the utility of interventions. He suggested that too much ABA research is conducted in settings that do not approximate typical service settings, optimizing experimental control at the expense of external validity. Along this same line of reasoning, he noted that it is important to test the generality of effects across clients and identify variables that predict differential effectiveness. He suggested systematically reporting results from all research participants (e.g., the intent-to-treat model), and purposive selection of participants would provide a more complete account of the situations in which treatments are successful and those in which they are unsuccessful. Smith argued that researchers should include more distal and socially important outcomes because with a narrow target “behavior may change, but remain a problem for the individual or may be only a small component of a much larger cluster of problems such as addiction or delinquency.” He pointed out that in order to best support effective practice, research must demonstrate that an intervention produces or contributes to producing the socially important outcomes that would cause a consumer to say that the problem is solved.
Further, Smith argues that many of the questions most relevant to EBP—questions about the likely outcomes of a treatment when applied in a particular type of situation—are well suited to group research designs. He argued that RCTs are likely to be necessary within a program of research because:
most problems pose important actuarial questions (e.g., determining whether an intervention package is more effective than community treatment as usual; deciding whether to invest in one intervention package or another, both, or neither; and determining whether the long-term benefits justify the resources devoted to the intervention)…. A particularly important actuarial issue centers on the identification of the conditions under which the intervention is most likely to be effective. (p. 23)
We agree that selection of research methods should be driven by the kinds of questions being asked and that group research designs are the methods of choice for some types of questions that are central to EBP. Therefore, we support Smith’s call for increased use of group research designs within ABA. If practice decisions are to be informed by the best available evidence, we must take advantage of both group and single-subject designs. However, we disagree with Smith’s statement that EBP should be limited to treatments that are validated “usually with group designs” (Smith, p. 27). Practitioners should be supported by reviews of research that draw from all of the available evidence and provide the best recommendations possible given the state of knowledge on the particular question. In most areas of behavior analytic practice, single-subject research makes up a large portion of the best available evidence. The Institute for Education Science (IES) has recognized the contribution single case designs can make toward identifying effective practices and has recently established standards for evaluating the quality of single case design studies (Institute of Educational Sciences, n.d. ; Kratochwill et al. 2013 ).
Classes of Evidence
Identifying the best available evidence to inform specific practice decisions is extremely complex, and no single currently available source of evidence can adequately inform all aspects of practice. Therefore, we outline a number of strategies for identifying and summarizing evidence in ways that can support the EBP of ABA. We do not intend to cover all sources of evidence comprehensively, but merely outline some of the options available to behavior analysts.
Empirically Supported Treatment Reviews
Empirically supported treatments (EST) are identified through a particular form of systematic literature review. Systematic reviews bring a rigorous methodology to the process of reviewing research. The development and use of these methods are, in part, a response to the recognition that the process of reviewing the literature is subject to threats to validity. The systematic review process is characterized by explicitly stated and replicable methods for (a) searching for studies, (b) screening studies for relevance to the review question, (c) appraising the methodological quality of studies, (d) describing outcomes from each study, and (e) determining the degree to which the treatment (or treatments) is supported by the research. When the evidence in support of a treatment is plentiful and of high quality, the treatment generally earns the status of an EST. Many systematic reviews, however, find that no intervention for a particular problem has sufficient evidence to qualify as an EST.
Well-known organizations in medicine (e.g., Cochrane Collaboration), education (e.g., What Works Clearinghouse), and mental health (e.g., National Registry of Evidence-based Programs and Practices) conduct EST reviews. Until recently, systematic reviews have focused nearly exclusively on group research; however, systematic reviews of single-subject research are quickly becoming more common and more sophisticated (e.g., Carr 2009 ; NAC 2009 ; Maggin et al. 2012 ).
Systematic reviews for EST status is one important way to summarize the best available evidence because it can give a relatively objective evaluation of the strength of the research literature supporting a particular intervention. But systematic reviews are not infallible; as with all other research and evaluation methods, they require skillful application and are subject to threats to validity. The results of reviews can change dramatically based on seemingly minor changes in operational definitions and procedures for locating articles, screening for relevance, describing treatments, appraising methodological quality, describing outcomes, summarizing outcomes for the body of research as a whole, and rating the degree to which an intervention is sufficiently supported (Slocum et al. 2012a ; Wilczynski 2012 ). Systematic reviews and claims based upon them must be examined critically with full recognition of their limitations just as one examines primary research reports.
Behavior analysts encounter many situations in which no ESTs have been established for the particular combination of client characteristics, target behaviors, functions, contexts, and other parameters for decision-making. This dearth may exist because no systematic review has addressed the particular problem or because a systematic review has been conducted but failed to find any well-supported treatments for the particular problem. For example, in a recent review of all of the recommendations in the empirically supported practice guides published by the IES, 45 % of the recommendations had minimal support (Slocum et al. 2012b ). As Smith noted ( 2013 ), only 3 of the 11 interventions that the NAC identified as meeting quality standards might be considered practices in the sense that they are manualized. In these common situations, a behavior analyst cannot respond by simply selecting an intervention from a list of ESTs. A comprehensive EBP of ABA requires additional strategies for reviewing research evidence and drawing practice recommendations from existing evidence—strategies that can glean the best available evidence from an imperfect research base and formulate practice recommendations that are most likely to lead to favorable outcomes under conditions of uncertainty.
Other Methods for Reviewing Research Literature
The three strategies outlined below may complement systematic reviews in guiding behavior analysts toward effective decision-making.
Narrative Reviews of the Literature
There has been a long tradition across disciplines of relying on narrative reviews to summarize what is known with respect to treatments for a class of problems (e.g., aggression) or what is known about a particular treatment (e.g., token economy). The author of the review, presumably an expert, selects the theme and synthesizes the research literature that he or she considers most relevant. Narrative reviews allow the author to consider a wide range of research including studies that are indirectly relevant (e.g., those studying a given problem with a different population or demonstrating general principles) and studies that may not qualify for systematic reviews because of methodological limitations but which illustrate important points nonetheless. Narrative reviews can consider a broader array of evidence and have greater interpretive flexibility than most systematic reviews.
As with all sources of evidence, there are difficulties with narrative reviews. The selection of the literature is left up to the author’s discretion; there are no methodological guidelines and little transparency about how the author decided which literature to include and which to exclude. There is always the risk of confirmation bias that the author emphasized literature that is consistent with her preconceived opinions. Even with a peer-review process, it is always possible that the author neglected or misinterpreted research relevant to the discussion. These concerns not withstanding, narrative reviews may provide the best available evidence when no systematic reviews exist or when substantial generalizations from the systematic review to the practice context are needed. Many textbooks (e.g., Cooper et al. 2007 ) and handbooks (e.g., Fisher et al. 2011 ; Madden et al. 2013 ) provide excellent examples of narrative reviews that can provide important guidance for evidence-based practitioners of ABA.
Best Practice Guides
Best practice guides are another source of evidence that can inform decisions in the absence of available and relevant systematic reviews. Best practice guides provide recommendations that reflect the collective wisdom of an expert panel. It is presumed that the recommendations reflect what is known from the research literature, but the validity of recommendations is largely derived from the panel’s expertise rather than from the rigor of their methodology. Recommendations from best practice panels are usually much broader than the recommendations from systematic reviews. The recommendations from these guides can provide important information about how to implement a treatment, how to adapt the treatment for specific circumstances, and what is necessary for broad scale or system-wide implementation.
The limitations to best practice guides are similar to those for narrative reviews; specifically, potential bias and lack of transparency are significant concerns. Panel members are typically not selected using a specific set of operationalized criteria. Bias is possible if the panel is drawn too narrowly. If the panel is drawn too broadly; however, the panel may have difficulty reaching a consensus (Wilczynski 2012 ).
Empirically Supported Practice Guides
Empirically supported practice guides, a more recently developed strategy, integrate the strengths of systematic reviews and best practice panels. In this type of review, an expert panel is charged with developing recommendations on a topic. As part of the process, a systematic review of the literature is conducted. Following the systematic review, the panel generates a set of recommendations and objectively determines the strength of evidence for the recommendation and assigns an evidence rating. When there is little empirical evidence directly related to a specific issue, the panel’s recommendations may have weak research support but nonetheless may be based on the best evidence that is available. The obvious advantage of empirically supported practice guides is that there is greater transparency about the review process and certainty of recommendations. Practice recommendations are usually broader than those derived from systematic reviews and address issues related to implementation and acceptable variations to enhance the treatment’s contextual fit (Shanahan et al. 2010 ; Slocum et al. 2012b ). Although empirically supported practice guides offer the objectivity of a systematic review and the flexibility of best practice guidelines, they also face potential sources of error from both methods. Systematic and explicit criteria are used to review the research and rate the level of evidence for each recommendation; however, it is the panel that formulates recommendations. Thus, results of these reviews are influenced by the selection of panel members. When research evidence is incomplete or equivocal, panelists must exercise judgment in interpreting the evidence and drawing conclusions (Shanahan et al. 2010 ).
Other Units of Analysis
Smith ( 2013 ) weighed in on the critical issue of the unit of analysis when describing and evaluating treatments (Slocum and Wilczynski 2008 ). The unit of analysis refers to whether EBP should focus on (a) principles, such as reinforcement; (b) tactics, such as backward chaining; (c) multicomponent packages, such as Functional Communication Training; or (d) even more comprehensive systems, such as Early Intensive Behavioral Intervention. After reviewing the ongoing debate between those favoring a smaller unit of analysis that focuses on specific procedures and those favoring a larger unit of analysis that evaluates the effects of multicomponent packages, Smith made a case that the multicomponent treatment package is the key unit in EBP. Smith noted that practitioners rarely solve a client’s problem with a single procedure; instead, solutions typically involve combinations of procedures. He argued that the unit should be “a service aimed at solving people’s problems” and procedures that are merely components of such services are not sufficiently complete to be the proper unit of analysis for EBP. He further stated that these treatment packages should include strategies for implementation in typical service settings and an intervention manual.
We concur that the multicomponent treatment package is a particularly significant and strategic unit of treatment because it specifies a suite of procedures and exactly how they are to be used together to solve a problem. Validated treatment packages are far more than the sum of their parts. A well-developed treatment package can be revised and optimized over many iterations in a way that would be difficult or impossible for a practitioner to accomplish independently. In addition, research outcomes from implementation of treatment packages reflect the interaction of the components, and these interactions may not be evident in the research literature on the individual components. Further, research on the outcomes from multicomponent packages can evaluate broader and more socially important outcomes than is generally possible when evaluating more narrowly defined treatments. For example, in the case of teaching a child with autism to communicate, research on a focused procedure such as time delay may indicate that its use leads to more independent communicative responses; however, research on a comprehensive Early Intensive Behavioral Intervention can evaluate the impact of the program on children’s global development or intellectual functioning.
Having recognized our agreement with Smith ( 2013 ) on the special importance of multicomponent treatment packages for EBP, we hasten to add that this type of intervention is not enough to support a broad and robust EBP of ABA. EBP must also provide guidance to the practitioner in the frequently encountered situations in which well-established treatment packages are not available. In these situations, problems may be best addressed by building an intervention from a set of elemental components. These components, referred to as practice elements (Chorpita et al. 2005 , 2007 ) or kernels (Embry 2004 ; Embry and Biglan 2008 ), may be validated either directly or indirectly. The practitioner assembles a particular combination of components to solve a specific problem. Because this newly constructed package has not been evaluated as a whole, there is additional uncertainty about the effectiveness of the package, and the quality of evidence may be considered lower than a well-supported treatment package (Slocum et al. 2012b ; Smith 2013 ; however, see Chorpita ( 2003 ) for a differing view). Nonetheless, treatment components that are supported by strong evidence provide the practitioner with tools to solve practical problems when EST packages are not relevant.
In some cases, behavior analysts are presented with problems that cannot be addressed even by assembling established components. In these cases, the ABA practitioner must apply principles of behavior to construct an intervention and must depend on these principles to guide sensible modifications of interventions in response to client needs and to support sensible implementation of interventions. Principles of behavior are broadly generalized statements describing behavioral relations. Their empirical base is extremely large and diverse including both human and nonhuman participants across numerous contexts, behaviors, and consequences. Although principles of behavior are based on an extremely broad research literature, they are also stated at a broad level. As a result, the behavior analyst must use a great deal of judgment in applying principles to particular problems and a particular attempt to apply a principle to solve a problem may not be successful. Thus, although behavioral principles are supported by evidence, newly constructed interventions based on these principles have not yet been evaluated. These interventions must be considered less certain or validated than treatment packages or elements that have been demonstrated to be effective for specific problems, populations, and context (Slocum et al. 2012b ).
Evidence-based practitioners of ABA recognize that the process of selecting and implementing treatments always includes some level of uncertainty (Detrich et al. 2013 ). One of the fundamental tenets of ABA shared with many other professions is that the best evidence regarding the effectiveness of an intervention does not come from systematic literature reviews, best practice guides, or principles of behavior, but from close continual contact with the relevant outcomes (Bushell and Baer 1994 ). The BACB guidelines ( 2010 ) state that, “behavior analysts recognize limits to the certainty with which judgments or predictions can be made about individuals” (item 3.0 [c]). As a result, “the behavior analyst collects data…needed to assess progress within the program” (item 4.07) and “modifies the program on the basis of data” (item 4.08). Thus, an important feature of the EBP of ABA is that professional decision-making does not end with the selection of an initial intervention. The process continues with ongoing progress monitoring and adjustments to the treatment plan as needed to achieve the targeted outcomes. Progress monitoring and data-based decision-making are the ultimate hedge against the inherent uncertainties of imperfect knowledge derived from research. As the quality of the best available evidence decreases, the importance of frequent direct measurement of client progress increases.
Practice decisions are always accompanied by some degree of uncertainty; however, better decisions are likely when multiple of sources of evidence are integrated. For example, a multicomponent treatment package may be an EST for clients who differ slightly from those the practitioner currently serves. Confidence in the use of this treatment may be increased if there is evidence showing the central components are effective with clients belonging to the population of interest. The principles of behavior might further inform sensible variations appropriate for the specific context of practice. When considered together, numerous sources of evidence increase the confidence the behavior analyst can have in the intervention. And when the plan is implemented, progress monitoring may reveal the need for additional adjustments. Each of these different classes of evidence provides answers to different questions for the practitioner, resulting in a more fine-grained analysis of the clinical problem and solutions to it (Detrich et al. 2013 ).
Client Values and Context
In order to be compatible with the underlying tenets of ABA, parallel with other professions, and to promote effective practice, a definition of EBP of ABA must include client values and context among the primary contributors to professional decision-making. Baer et al. ( 1968 ) suggested that the word applied refers to an immediate and important change in behavior that has practical value and that this value is determined “by the interest which society shows in the problems” (p. 92)—that is, by social values. Wolf ( 1978 ) went on to specify that behavior analytic practice can only be termed successful if it addresses goals that are meaningful to our clients, uses procedures that are judged appropriate by our clients, and produces effects that are valued by our clients. These foundational tenets of ABA correspond with the centrality of client values in classic definitions of EBP (e.g., Institute of Medicine 2001 ). Like medical professionals and those in the many other fields that have adopted similar conceptualizations of EBP, behavior analysts have long recognized that client values are critical contributors to responsible decision-making.
Behavior analysts have defined the client as the individual who is the focus of the behavior change, other individuals who are critical to the behavior change process (Baer et al. 1968 ; Heward et al. 2005 ), as well as outside individuals or groups who may have a stake in the target behavior or improved outcomes (Baer et al. 1987 ; Wolf 1978 ). Wolf ( 1978 ) argued that only our clients can judge the social validity of our work and suggested that behavior analysts address three levels of social validity: (a) the social significance of the goals, (b) the social desirability of the procedures, and (c) the social importance of the outcomes. With respect to selection of interventions, Wolf noted, “not only is it important to determine the acceptability of treatment procedures to participants for ethical reasons, it may also be that the acceptability of the program is related to effectiveness, as well as to the likelihood that the program will be adopted and supported by others” (p. 210). He further maintained that clients are the ultimate arbiters of whether or not the effects of a program are sufficiently helpful to be termed successful.
The concept of social validity directs our attention to some of the important aspects of the context of intervention. Intervention always occurs in some context and features of that context can directly influence the fidelity with which the intervention is implemented and its effectiveness. Albin et al. ( 1996 ) expanded further on the contextual variables that might be critical for designing and implementing effective interventions. They described the concept of contextual fit or the congruence of a behavioral support plan and the context and indicate that this fit will determine its implementation, effectiveness, and maintenance.
Contextual fit includes the issues of social validity, but also explicitly encompasses issues associated with the individuals who implement treatments and manage other aspects of the environments within which treatments are implemented. Behavioral intervention plans prescribe the behavior of implementers. These implementers may include professionals, such as therapists and teachers, as well as nonprofessionals, such as family and community members. It is important to consider characteristics of these implementers when developing plans because the success of a plan may hinge on how it corresponds with the values, skills, goals, and stressors of the implementers. Effective plans must be within the skill repertoire of the implementers, or training to fidelity must occur to introduce the plan components into that repertoire. Values, goals, and stressors refer to motivating operations that determine the reinforcing or punishing value of implementing the plan. Plans that provide little reinforcement and substantial punishment in the process of implementation or outcomes are unlikely to be implemented with fidelity or maintained over time. The effectiveness of behavioral interventions is also influenced by their compatibility with other aspects of their context. Plans that are compatible with ongoing routines are more likely to be implemented than those that conflict (Riley-Tillman and Chafouleas 2003 ). Interventions require various kinds of resources to be implemented and sustained. For example, financial resources may be necessary to purchase curricula, equipment, or other goods. Interventions may require human resources such as direct service staff, training, supervision, administration, and consultation. Fixsen et al. ( 2005 ) have completed an extensive review of contextual variables that can potentially influence the quality of intervention implementation. Behavior analytic practice is unlikely to be effective if it does not consider the context in which interventions will be implemented.
Extensive behavior analytic research has documented the importance of social validity and other contextual factors in producing behavioral changes with practical value. This research tradition is as old as our field (e.g., Jones and Azrin 1969 ) and continues through the present day. For example, Strain et al. ( 2012 ) provided multiple examples of the impact of social validity considerations on relevant outcomes. They reported that integrating client values, preferences, and characteristics in the selection and implementation of an intervention can successfully inform decisions regarding (a) how to design service delivery systems, (b) how to support implementers with complex strategies, (c) when to fade support, (e) how to identify important and unanticipated effects, and (f) how to focus on future research efforts.
Benazzi et al. ( 2006 ) examined the effect of stakeholder participation in intervention planning on the acceptability and usability of behavior intervention plans (BIP) based on descriptive functional behavior assessments (FBA). Plans developed by behavior experts were rated as high in technical adequacy, but low in acceptability. Conversely, plans developed by key stakeholders were highly acceptable, but lacked technical adequacy. However, when the process included both behavior experts and key stakeholders, BIPs were considered both acceptable and technically adequate. Thus, the BIPs developed by behavior analysts may be marginalized and implementation may be less likely to occur in the absence of key stakeholder input. Thus, a practical commitment to effective interventions that are implemented and maintained with integrity over time requires that behavior analysts consider motivational variables such as the alignment of interventions with the values, reinforcers, and punishers of relevant stakeholders.
Clinical Expertise
All of the key components for expert behavior analytic practice (i.e., identification of important behavioral problems, recognition of underlying behavioral processes, weighing of evidence supporting various treatment options, selecting and implementing treatments in complex social contexts, engaging in ongoing data-based decision making, and being responsive to client values and context) require clinical expertise. Clinical expertise refers to the competence attained by practitioners through education, training, and experience that results in effective practice (American Psychological Association Presidential Task Force of Evidence-Based Practice 2006 ). Clinical expertise is the means by which the best available evidence is applied to individual cases in all their complexity. Based on the work of Goodheart ( 2006 ), we suggest that clinical expertise in EBP of ABA includes (a) knowledge of the research literature and its applicability to particular clients, (b) incorporation of the conceptual system of ABA, (c) breadth and depth of clinical and interpersonal skills, (d) integration of client values and context, (e) recognition of the need for outside consultation, (f) data-based decision making, and (g) ongoing professional development. In the sections that follow, we describe each component of clinical expertise in ABA.
Knowledge and Application of the Research Literature
ABA practitioners must be skilled in applying the best available evidence to unique cases in specific contexts. The role of the best available evidence in EBP of ABA was discussed above. Practitioners need to be knowledgeable about the scientific literature and able to appropriately apply the literature to behaviors, clients, and contexts that are rarely a perfect match to the behaviors, clients, and contexts in any particular study. This confluence of knowledge and skillful application requires that the behavior analyst respond to the functionally important features of cases. A great deal of training is necessary to build the expertise required to discriminate critical functional features from those that are incidental. These discriminations must be made with respect to the presenting problem (i.e., the behavioral patterns that have been identified as problematic, their antecedent stimuli, motivating operations, and consequences); client variables such as histories, skills, and preferences; and contextual variables that may impact the effectiveness of various treatment options as applied to the particular case. These skills are reflected in BACB Guidelines 1.01 and 2.10 cited above.
Incorporation of the Conceptual System
The critical features of a case must be identified and mapped onto the conceptual system of ABA. It is not enough to recognize that a particular feature of the environment is important; it must also be understood in terms of its likely behavioral function. This initial conceptualization is necessary in order to generate reasonable hypotheses that may be tested in more thorough analyses. Developing the skill of describing cases in terms of likely behavioral functions typically requires a great deal of formal and informal training as well as ongoing learning from experience. These repertoires are usually acquired through extensive training, supervised practice, and the ongoing feedback of client outcomes. This is recognized in BACB Guidelines; for example, 4.0 states that “the behavior analyst designs programs that are based on behavior analytic principles” (BACB 2010 ).
Breadth and Depth of Clinical and Interpersonal Skills
Evidence-based practitioners of behavior analysis must be able to implement various assessment and intervention procedures with fidelity, and often to train and supervise others to implement such procedures with fidelity. Further, clinical expertise in ABA requires that the practitioner have effective interpersonal skills. For example, he must be able to explain the behavioral philosophy and approach, in nonbehavioral terms, to various audiences who may have different theoretical orientations. BCBA Guidelines 1.05 specifies that behavior analysts “use language that is fully understandable to the recipient of those services” (BACB 2010 ).
Integration of Client Values and Context
In all aspects of their work, practitioners of evidence-based ABA must integrate the values and preferences of the client and other stakeholders as well as the features of the specific context that may impact the effectiveness of an intervention. These factors can be considered additional variables that the behavior analyst must attend to when planning and providing behavior-analytic services. For example, when assessment data suggest behavior serves a particular function, a range of intervention alternatives may be considered (see Geiger, Carr, and LeBlanc for an example of a model for selecting treatments for escape-maintained problem behavior). A caregiver’s statements might suggest that one type of intervention may not be viable due to limited resources while another treatment may be acceptable based on financial considerations, available resources, or other practical factors; the behavior analyst must have the training and expertise to evaluate and incorporate these factors into initial treatment selection and to re-evaluate these concerns as a part of progress monitoring for both treatment integrity and client improvement. BACB Guideline 4.0 states that the behavior analyst “involves the client … in the planning of … programs, [and] obtains the consent of the client” and 4.1 states that “if environmental conditions hamper implementation of the behavior analytic program, the behavior analyst seeks to eliminate the environmental constraints, or identifies in writing the obstacles to doing so” (BACB 2010 ).
Recognition of Need for Outside Consultation
Behavior analysts engaging in responsible evidence-based practice discriminate between behaviors and contexts that are within the scope of their training and those that are not, and respond differently based on this discrimination. For example, a behavior analyst who has been trained to provide assessment and intervention for severe problem behavior may not have the specific training to provide organizational behavior management services to a corporation; in this case, a behavior analyst with clinical expertise would make this discrimination and seek additional consultation or make appropriate referrals. This aspect of expertise is described in BACB ( 2010 ) Guidelines 1.02 and 2.02.
Data-Based Decision Making
Data-based decision making plays a central role in the practice of ABA and is an indispensable feature of clinical expertise. The process of data-based decision making includes identifying useful measurement pinpoints, constructing measurement systems, and graphing results, as well as identifying meaningful patterns in data, interpreting these patterns, and making appropriate responses to them (e.g., maintaining, modifying, replacing, or ending a program). The functional features of the case, the best available research evidence, and the new evidence obtained through progress monitoring must inform these judgments and are central to this model of EBP of ABA. BACB ( 2010 ) Guidelines 4.07 and 4.08 specify that behavior analysts collect data to assess progress and modify programs on the basis of data.
Ongoing Professional Development
Clinical expertise is not static; rather, it requires ongoing professional development. Clinical expertise in ABA requires ongoing contact with the research literature to ensure that practice reflects current knowledge about the most effective and efficient assessment and intervention procedures. The critical literature includes primary empirical research as well as reviews and syntheses such as those described in the section on “ Best Available Evidence ”. In addition, professional consensus on important topics for professional practice evolves over time. For example, in ABA, there has been increased emphasis recently on ethics and supervision competence. All of these dynamics point to the need for ongoing professional development. This is reflected in the requirement that certified behavior analysts “undertake ongoing efforts to maintain competence in the skills they use by reading the appropriate literature, attending conferences and conventions, participating in workshops, and/or obtaining Behavior Analyst Certification Board certification” (Guideline 1.03, BACB 2010 ).
Conclusions
We propose that EBP of ABA be understood as a professional decision-making framework that draws on the best available evidence, client values and context, and clinical expertise. We argue that this conception of EBP of ABA is more compatible with the basic tenets of ABA and more closely aligned with definitions of EBP in other fields than that provided by Smith ( 2013 ). It is noteworthy that this notion of EBP is not necessarily in conflict with many of the observations and arguments put forth by Smith ( 2013 ). His concerns were primarily about how to define and validate EST, which is an important way to inform practitioners about the best available evidence to integrate into their overall EBP.
Given the close alignment between the proposed framework of EBP of ABA and broadly accepted descriptions of behavior analytic practice, one might wonder whether EBP offers anything new. We believe that the EBP of ABA framework, offered here, has several important implications for our field. First, this framework draws together numerous elements of ABA practice into a single coherent system, which can help behavior analysts provide an explicit rationale for their decision-making to clients and other stakeholders. The EBP of ABA provides a decision-making framework that supports a cogent and transparent description of (a) the evidence considered, including direct and frequent measurement of the client’s behavior; (b) why this evidence was identified as the “best available” for the particular case; (c) how client values and contextual factors influenced the process; and (d) the ways in which clinical expertise was used to conceptualize the case and integrate the various considerations. This transparency and explicitness allows the behavior analyst to offer empirically based treatment recommendations while addressing the concerns raised by stakeholders. It also highlights the critical analysis required to be an effective behavior analyst. For example, if an EST is available and appropriate, the behavior analyst can describe the relevance and certainty of the evidence for this intervention. If no relevant EST is available, the behavior analyst can describe how the best available evidence supports the intervention and emphasize the importance of progress monitoring.
Second, the EBP framework prompts the behavior analyst to refer to the important client values that underlie the goals of intervention, the specific methods of intervention, and describe how the intervention is supported by features of the context. This requires the behavior analyst to explicitly recognize that the effectiveness of an intervention is always context dependent. By serving as a prompt, the EBP framework should increase behavior analysts’ adherence to this central tenet of ABA.
Third, by explicitly recognizing the role of clinical expertise, the framework gives the behavior analyst a way to talk about the complex skills required to make appropriate decisions about client needs. In addition, the fact that the proposed definition of EBP of ABA is so closely aligned with definitions in other professions such as medicine and psychology that it provides a common framework and language for communicating about a particular case that can enhance collaboration between behavior analysts and other professionals.
Fourth, this framework for EBP of ABA suggests further development of behavior analysis as well. Examination of the meaning of best available evidence encourages behavior analysts to continue to refine methods for systematically reviewing research literature and identifying ESTs. Further, behavior analysts could better support EBP if we developed methods for validating other units of intervention such as practice elements, kernels, and even the principles of behavior; when these are invoked to support interventions, they must be supported by a clearly specified research base.
Finally, the explicit recognition of the role of clinical expertise in the EBP of ABA has important implications for training behavior analysts. This framework suggests that decision-making is at the heart of EBP of ABA and could be an organizing theme for ABA training programs. Training programs could systematically teach students to articulate the chain of logic that is the basis for their treatment recommendations. The chain of logic would include statements about which research was considered and why, how the client’s values influenced decision-making, and how contextual factors influenced the selection and adaptation (if necessary) of the treatment. This type of training could be embedded in all instructional activities. Formally requiring students to articulate a rationale for the decisions and receiving feedback about their decisions would sharpen their clinical expertise.
In addition to influencing our behavior analytic practice, the EBP of ABA framework impacts our relationship with other members of the broader human service field as well as individuals and agencies that control contingencies relevant to practitioners and scientists. Methodologically rigorous reviews that identify ESTs and other treatments supported by the best available evidence are extremely important for working with organizations that control funding for behavior analytic research and practice. Federal funding for research and service provision is moving strongly towards EBP and ESTs. This trend is clear in education through the No Child Left Behind Act of 2001 , the Individuals with Disabilities Education Act of 2004 , the funding policies of IES, and the What Works Clearinghouse. The recent memorandum by the Director of the Office of Management and Budget (Zients 2012 ) makes it clear that the importance of EBP is not limited to a single discipline or to one political party. In addition, insurance companies are increasingly making reimbursement decisions based, in part, on whether or not credible scientific evidence supports the use of the treatment (Small 2004 ). The insurance companies have consistently adopted criteria for scientific evidence that are closely related to EST (Bogduk and Fraifeld 2010 ). As a result, reimbursement for ABA services may depend on the scientific credibility of EST reviews, a critical component of EBP. Methodologically rigorous reviews that identify ESTs within a broader framework of EBP appear to be critical for ABA to maintain and expand its access to federal funding and insurance reimbursement for services. Establishment of this literature base will require behavior analysts to develop appropriate methods for reviewing and summarizing research based on single-subject designs. IES has established such standards for reviewing studies, but to date, there are no accepted methods for calculating a measure of effect size as an objective basis for combining result across studies (Kratochwill et al. 2013 ). If behavior analysts develop such a measure, it would reflect a significant methodological advance as a field and it would increase the credibility of behavior analytic research with agencies that fund research and services.
EBP of ABA emphasizes the research-supported selection of treatments and data-driven decisions about treatment progress that have always been at the core of ABA. ABA’s long-standing recognition of the importance of social validity is reflected in the definition of EBP. This framework for EBP of ABA offers many positive professional consequences for scientists and practitioners while promoting the best of the behavior analytic tradition and making contact with developments in other disciplines and the larger context in which behavior analysts work.
- Albin RW, Lucyshyn JM, Horner RH, Flannery KB. Contextual fit for behavior support plans. In: Koegel LK, Koegel RL, Dunlap G, editors. Positive behavioral support: Including people with difficult behaviors in the community. Baltimore: Brookes; 1996. pp. 81–92. [ Google Scholar ]
- American Occupational Therapy Association Occupational therapy practice framework: domain and process (2nd ed.) American Journal of Occupational Therapy. 2008; 62 :625–683. doi: 10.5014/ajot.62.6.625. [ PubMed ] [ CrossRef ] [ Google Scholar ]
- American Psychological Association (2005). Policy statement on evidence-based practice in psychology. http://www.apa.org/practice/resources/evidence/evidence-based-statement.pdf .
- American Psychological Association Presidential Task Force of Evidence-Based Practice Evidence-based practice in psychology. American Psychologist. 2006; 61 :271–285. doi: 10.1037/0003-066X.61.4.271. [ PubMed ] [ CrossRef ] [ Google Scholar ]
- American Speech-Language-Hearing Association (2005). Evidence-based practice in communication disorders [position statement]. www.asha.org/policy .
- Baer DM, Wolf MM, Risley TR. Some current dimensions of applied behavior analysis. Journal of Applied Behavior Analysis. 1968; 1 :91–97. doi: 10.1901/jaba.1968.1-91. [ PMC free article ] [ PubMed ] [ CrossRef ] [ Google Scholar ]
- Baer DM, Wolf MM, Risley TR. Some still-current dimensions of applied behavior analysis. Journal of Applied Behavior Analysis. 1987; 20 :313–327. doi: 10.1901/jaba.1987.20-313. [ PMC free article ] [ PubMed ] [ CrossRef ] [ Google Scholar ]
- Behavior Analyst Certification Board (2010). Guidelines for responsible conduct for behavior analysts. http://www.bacb.com/index.php?page=57 .
- Benazzi L, Horner RH, Good RH. Effects of behavior support team composition on the technical adequacy and contextual-fit of behavior support plans. The Journal of Special Education. 2006; 40 (3):160–170. doi: 10.1177/00224669060400030401. [ CrossRef ] [ Google Scholar ]
- Bogduk N, Fraifeld EM. Proof or consequences: who shall pay for the evidence in pain medicine? Pain Medicine. 2010; 11 (1):1–2. doi: 10.1111/j.1526-4637.2009.00770.x. [ PubMed ] [ CrossRef ] [ Google Scholar ]
- Bushell D, Jr, Baer DM. Measurably superior instruction means close, continual contact with the relevant outcome data. Revolutionary! In: Gardner R III, Sainato DM, Cooper JO, Heron TE, Heward WL, Eshleman J, Grossi TA, editors. Behavior analysis in education: Focus on measurably superior instruction. Pacific Grove: Brooks; 1994. pp. 3–10. [ Google Scholar ]
- Carnine D. Expanding the notion of teachers’ rights: access to tools that work. Journal of Applied Behavior Analysis. 1992; 25 (1):13–19. doi: 10.1901/jaba.1992.25-13. [ PMC free article ] [ PubMed ] [ CrossRef ] [ Google Scholar ]
- Carr JE, Severtson JM, Lepper TL. Noncontingent reinforcement is an empirically supported treatment for problem behavior exhibited by individuals with developmental disabilities. Research in Developmental Disabilities. 2009; 30 :44–57. doi: 10.1016/j.ridd.2008.03.002. [ PubMed ] [ CrossRef ] [ Google Scholar ]
- Chorpita BF. The frontier of evidence-based practice. In: Kazdin AE, Weisz JR, editors. Evidence-based psychotherapies for children and adolescents. New York: Oxford; 2003. pp. 42–59. [ Google Scholar ]
- Chorpita BF, Daleiden EL, Weisz JR. Identifying and selecting the common elements of evidence based interventions: a distillation and matching model. Mental Health Services Research. 2005; 7 :5–20. doi: 10.1007/s11020-005-1962-6. [ PubMed ] [ CrossRef ] [ Google Scholar ]
- Chorpita BF, Becker KD, Daleiden EL. Understanding the common elements of evidence-based practice: misconceptions and clinical examples. Journal of the American Academy of Child and Adolescent Psychiatry. 2007; 46 :647–652. doi: 10.1097/chi.0b013e318033ff71. [ PubMed ] [ CrossRef ] [ Google Scholar ]
- Cook BG, Cook SC. Unraveling evidence-based practices in special education. Journal of Special Education. 2013; 47 (2):71–82. doi: 10.1177/0022466911420877. [ CrossRef ] [ Google Scholar ]
- Cooper JO, Heron TE, Heward WL. Applied behavior analysis. 2. Upper Saddle River: Pearson; 2007. [ Google Scholar ]
- Detrich, R. (Chair) (2009). Evidence-based, empirically supported, best practice: What does it all mean? Symposium conducted at the annual meeting of the Association for Behavior Analysis International, Phoenix, AZ.
- Detrich R, Slocum TA, Spencer TD. Evidence-based education and best available evidence: Decision-making under conditions of uncertainty. In: Cook BG, Tankersley M, Landrum TJ, editors. Advances in learning and behavioral disabilities, 26. Bingly, UK: Emerald; 2013. pp. 21–44. [ Google Scholar ]
- Embry DD. Community-based prevention using simple, low-cost, evidence-based kernels and behavior vaccines. Journal of Community Psychology. 2004; 32 :575–591. doi: 10.1002/jcop.20020. [ CrossRef ] [ Google Scholar ]
- Embry DD, Biglan A. Evidence-based kernels: fundamental units of behavioral influence. Clinical Child and Family Psychology Review. 2008; 11 :75–113. doi: 10.1007/s10567-008-0036-x. [ PMC free article ] [ PubMed ] [ CrossRef ] [ Google Scholar ]
- Fisher WW, Piazza CC, Roane HS, editors. Handbook of applied behavior analysis. New York: Guilford Press; 2011. [ Google Scholar ]
- Fixsen DL, Naoom SF, Blase KA, Friedman RM, Wallace F. Implementation research: A synthesis of the literature (FMHI publication #231) Tampa: University of South Florida, Louis de la Parte Florida Mental Health Institute, The National Implementation Research Network; 2005. [ Google Scholar ]
- Goodheart CD. Evidence, endeavor, and expertise in psychology practice. In: Goodheart CD, Kazdin AE, Sternberg RJ, editors. Evidence-based psychotherapy: Where practice and research meet. Washington, D.C.: APA; 2006. pp. 37–61. [ Google Scholar ]
- Goodman KW. Ethics and evidence-based education: Fallibility and responsibility in clinical science. New York: Cambridge University Press; 2003. [ Google Scholar ]
- Heward WL, et al., editors. Focus on behavior analysis in education: Achievements, challenges, and opportunities. Upper Saddle River: Prentice Hall; 2005. [ Google Scholar ]
- Horner RH, Carr EG, Halle J, McGee G, Odom S, Wolery M. The use of single-subject research to identify evidence-based practice in special education. Exceptional Children. 2005; 71 (2):165–179. doi: 10.1177/001440290507100203. [ CrossRef ] [ Google Scholar ]
- Horner RH, Sugai G, Todd AW, Lewis-Palmer T. Schoolwide positive behavior support. In: Bambera LM, Kern L, editors. Individualized supports for students with problem behaviors: Designing positive behavior plans. New York: Guilford Press; 2005. pp. 359–390. [ Google Scholar ]
- Individuals with Disabilities Education Improvement Act of 2004, 70, Fed. Reg., (2005).
- Institute of Education Sciences, US. Department of Education. (n.d.). What Works Clearinghouse Procedures and Standards Handbook (No. Version 3.0). Washington DC. Retrieved from http://ies.ed.gov/ncee/wwc/pdf/reference_resources/wwc_procedures_v3_0_standards_handbook.pdf .
- Institute of Medicine . Crossing the quality chasm: A new health system for the 21st century. Washington, DC: National Academies Press; 2001. [ PubMed ] [ Google Scholar ]
- Johnston JM, Pennypacker HS. Strategies and tactics of behavioral research. 2. Hillsdale: Erlbaum; 1993. [ Google Scholar ]
- Jones RJ, Azrin NH. Behavioral engineering: stuttering as a function of stimulus duration during speech synchronization. Journal of Applied Behavior Analysis. 1969; 2 :223–229. doi: 10.1901/jaba.1969.2-223. [ PMC free article ] [ PubMed ] [ CrossRef ] [ Google Scholar ]
- Kazdin AE. Psychotherapy for children and adolescents: Directions for research and practice. New York: Oxford University Press; 2000. [ Google Scholar ]
- Kratochwill, T. R., Hitchcock, J., Horner, R. H., Levin, J. R., Odom, S. L., Rindskopf, D. M., & Shadish, W. R. (2010). Single-case designs technical documentation. Retrieved from http://ies.ed.gov/ncee/wwc/pdf/wwc_scd.pdf .
- Kratochwill TR, Hitchcock JH, Horner RH, Levin JR, Odom SL, Rindskopf DM, et al. Single-case intervention research design standards. Remedial & Special Education. 2013; 34 (1):26–38. doi: 10.1177/0741932512452794. [ CrossRef ] [ Google Scholar ]
- Madden GJ, Dube WV, Hackenberg TD, Hanley GP, Lattal KA, editors. American Psychological Association handbook of behavior analysis. Washington, DC: American Psychological Association; 2013. [ Google Scholar ]
- Maggin DM, O’Keeffe BV, Johnson AH. A quantitative synthesis of single-subject meta-analyses in special education, 1985–2009. Exceptionality. 2011; 19 :109–135. doi: 10.1080/09362835.2011.565725. [ CrossRef ] [ Google Scholar ]
- Maggin DM, Johnson AH, Chafouleas SM, Ruberto LM, Berggren M. A systematic evidence review of school-based group contingency interventions for students with challenging behavior. Journal of School Psychology. 2012; 50 :625–654. doi: 10.1016/j.jsp.2012.06.001. [ PubMed ] [ CrossRef ] [ Google Scholar ]
- McIntosh K, Filter KJ, Bennett JL, Ryan C, Sugai G. Principles of sustainable prevention: designing scale–up of School–wide Positive Behavior Support to promote durable systems. Psychology in the Schools. 2010; 47 (1):5–21. [ Google Scholar ]
- National Autism Center . National Standards Project: Findings and conclusions. Randolph: National Autism Center; 2009. [ Google Scholar ]
- No Child Left Behind Act of 2001, Pub. L. No. 107-110. (2002).
- Polsgrove L. Reflections on the past and future. Education and Treatment of Children. 2003; 26 :337–344. [ Google Scholar ]
- Riley-Tillman TC, Chafouleas SM. Using interventions that exist in the natural environment to increase treatment integrity and social influence in consultation. Journal of Educational & Psychological Consultation. 2003; 14 (2):139–156. doi: 10.1207/s1532768xjepc1402_3. [ CrossRef ] [ Google Scholar ]
- Sackett DL, Rosenberg WM, Gray JA, Haynes RB, Richardson WS. Evidence based medicine: what it is and what it isn’t. British Medical Journal. 1996; 312 (7023):71. doi: 10.1136/bmj.312.7023.71. [ PMC free article ] [ PubMed ] [ CrossRef ] [ Google Scholar ]
- Sackett DL, Straus SE, Richardson WS, Rosenberg W, Haynes RB, editors. Evidence-based medicine: How to teach and practice EBM. Edinburgh: Livingstone; 2000. [ Google Scholar ]
- Shanahan, T., Callison, K., Carriere, C., Duke, N.K., Pearson, P.D., Schatschneider, C., et al. (2010). Improving reading comprehension in kindergarten through 3rd grade: A practice guide (NCEE 2010-4038). Washington, DC: National Center for Education Evaluation and Regional Assistance, Institute of Education Sciences, U.S. Department of Education. http://ies.ed.gov/ncee/wwc/publications/practiceguides . Accessed 12 Sept 2013
- Sidman M. Tactics of scientific research: Evaluating experimental data in psychology. New York: Basic Books; 1960. [ Google Scholar ]
- Slocum, T. A., & Wilczynski, S. (2008). The unit of analysis in evidence-based practice . Invited paper presented at the meeting the Association for Behavior Analysis International, Chicago, Il.
- Slocum TA, Detrich R, Spencer TD. Evaluating the validity of systematic reviews to identify empirically supported treatments. Education and Treatment of Children. 2012; 35 :201–234. doi: 10.1353/etc.2012.0009. [ CrossRef ] [ Google Scholar ]
- Slocum TA, Spencer TD, Detrich R. Best available evidence: three complementary approaches. Education and Treatment of Children. 2012; 35 :27–55. [ Google Scholar ]
- Small RH. Maximize the likelihood of reimbursement when appealing managed care medical necessity denials. Getting Paid in Behavioral Healthcare. 2004; 9 (12):1–3. [ Google Scholar ]
- Smith T. What is evidence-based behavior analysis? The Behavior Analyst. 2013; 36 :7–33. [ PMC free article ] [ PubMed ] [ Google Scholar ]
- Strain PS, Barton EE, Dunap G. Lessons learned about the utility of social validity. Education and Treatment of Children. 2012; 35 (2):183–200. doi: 10.1353/etc.2012.0007. [ CrossRef ] [ Google Scholar ]
- Wilczynski SM. Risk and strategic decision-making in developing evidence-based practice guidelines. Education and Treatment of Children. 2012; 35 :291–311. doi: 10.1353/etc.2012.0012. [ CrossRef ] [ Google Scholar ]
- Wolf M. Social validity: the case for subjective measurement, or how applied behavior analysis is finding its heart. Journal of Applied Behavior Analysis. 1978; 11 :203–214. doi: 10.1901/jaba.1978.11-203. [ PMC free article ] [ PubMed ] [ CrossRef ] [ Google Scholar ]
- Zients, J. D. (2012). M-12-14. Memorandum to the heads of executive departs. From: Jeffrey D. Zients, Acting Director. Subject: use of evidence and evaluation in the 2014 Budget. www.whitehouse.gov/sites/default/files/omb/…/2012/m-12-14.pdf . Accessed 30 Sept 2012

- CREd Library , Research Design and Method
Treatment Fidelity in Single-Subject Designs
An overview of approaches and considerations for measuring treatment fidelity., cred library and ralf schlosser.
- October, 2014
DOI: 10.1044/cred-ssd-r101-001
Treatment fidelity, also called procedural integrity or treatment integrity, refers to the methodological strategies used to evaluate the extent to which an intervention is being implemented as intended. Maintaining high treatment fidelity helps ensure that changes observed during a study reflect an alteration of the subject’s behavior and not an alteration in the behavior of the experimenter.
Video: An Overview of Treatment Fidelity in Single-Subject Research
Well, there are a number of terms that are being used. One of them, as you know, is treatment fidelity. But there are other synonyms or more or less equivalent terms such as “procedural integrity” or “treatment integrity” or “procedural reliability” so there are four or five different terms that are being used. What it means though, as a concept related to treatment is that you measure that the intervention is implemented as you had planned. You have a treatment protocol, and you want to make sure the treatment is carried out as you had intended. Why is that important? It’s important because if you don’t know how the treatment was implemented, it becomes very difficult to know what the causal factor in the change was. So you reach a certain outcome, but you cannot really attribute it to something concrete because you don’t know how well the treatment was implemented. It affects internal validity. It affects external validity. It’s a very important aspect of treatment research.
How do you measure treatment fidelity?
You have to think about how the treatment breaks down into steps. You want those steps to be reflective of what the active ingredient of the intervention is. Sometimes you see in the literature there are steps laid out and they are measured, everything with good reliability, good treatment integrity, but the steps may not necessarily be reflective of what the key construct is. It’s important that those steps are related to what your theory of change is. You have a certain theory, and you want to make sure those steps are reflective of it. That’s one aspect. The other challenge is that you have to think about what method are you going to use to evaluate treatment fidelity. You can use self-monitoring. That is when the experimenter him or herself basically does check marks or takes notes. So that’s one method. The second method is when you have a second observer, and the second observer basically takes notes or records how well the experimenter does. That’s the second method. And the third method is when you have the experimenter take notes, and the second observer, and then you compare. And you derive what is called interobserver agreement on treatment fidelity. So the first and the second step are not mutually exclusive, you can do both. So that’s a big thing to think about in terms of measuring treatment fidelity. Another challenge is: How many sessions do you need? Do you need 100%, or is it okay to have maybe 20% to 30%? There are no clear-cut rules about this. But in general, journal editors and reviewers like to see above sort of 20%, at least, of the observations.
What advice do you have for developing and implementing a treatment fidelity protocol?
In the beginning when I started doing this kind of work, I was under the illusion that I could design this at the desk, and then the experimenter will implement it as I had planned. When you design an intervention at your desk, it all looks very deliberate and concrete and logical. Then you actually ask somebody to implement it, and there are steps missing, unforeseen circumstances happening, and the whole thing will fall apart. And you have to start from scratch. It’s an iterative process to develop a good treatment fidelity protocol. I have learned the hard way that you always have to pilot. For multiple reasons, pilot studies are really important, but related to treatment fidelity, it is essential. You don’t know if this is actually doable. You prepare a data collection sheet, and the observer says, “This is too cumbersome. I couldn’t keep up.” Especially if it’s done live. If you have recorded — you might want to think about that. Do I do live? Do I do video recording? These are pros and cons too. Live observation requires somebody to be there right then. And the video recording has added flexibility. You can watch it any time, it can be replayed. So you have to think about that, too. What I’ve observed in the real implementation is sometime the experimenter feels very conscious about being watched. They might feel anxious. “Am I doing the right thing?” The researcher has to be careful about how to approach this. This is not about “big brother is watching you.” It’s more about, “We’d like for you, as the experimenter to do the best job possible to deliver the intervention. So, we’d like to work with you and give you feedback, as you proceed with doing this. And we can help you do better, if needed.” You kind of phrase it like, “We are in this together. We’re trying to deliver the best intervention we can. Let’s make it happen.” So that kind of anxiety goes away.
How does your approach to treatment fidelity change through the course of a research program?
There’s a progression of research. There are many people who have written about this in our field. Initially, you want to have really good control, and really good treatment integrity. So that’s the primary objective — you want to have it as perfect as possible. But then, as you go into real practice, there are all kinds of constraints imposed on implementing an intervention that, it’s different from a study, as you know. There, it becomes sometimes important to do a study with less integrity. The treatment fidelity sort of becomes the independent variable that you’re manipulating. Can the same treatment outcomes be obtained by having less perfect implementation? Because, assuming the clinician is not a robot, and has to be responsive to what happens with the clients, you want them to be more flexible. But can you still obtain the same outcomes? You should follow this progression in measurement. Do multiple studies, and hopefully get to the point where we can reach outcomes in real-life settings with real-life expectations with what is reasonable in terms of fidelity.
~ From a video interview with Ralf Schlosser, Northeastern University.
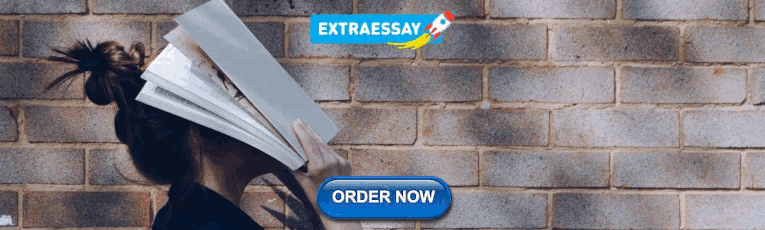
The Need for Special Consideration of Treatment Fidelity in Single-Subject Experimental Designs
A defining feature of single-case studies is that each condition remains in effect for extended periods of time (e.g., from several days to several weeks) to allow sufficient data to be collected from which judgments will be made. Thus, the possibility of implementation drift and of incorrect implementation is logically high. Another defining feature of single-case methods is intra-subject and/or inter-subject replication of the experimental conditions. Monitoring relevant variables across the course of an investigation can assist in assuring that the defining variables of the respective conditions are implemented similarly in each replication. Finally, applied single case studies often, but not always involve implementation by humans. […] The possibility of bias and drift are well known.
~ From Woolery (1994) .
Practically speaking, researchers expect that treatment agents will implement a treatment as planned. This is particularly acute in treatments that must be implemented by third parties such as teachers, parents, or research assistants. When significant behavior changes occur, the researcher often assumes that these changes were due to the intervention. However, it may well be the case that the treatment agent changed the intervention in ways unknown to the researcher and these changes were responsible for behavior change. In contrast, if significant behavior changes do not occur, then the researcher may assume falsely that the lack of change is due to an ineffective or inappropriate intervention. In this case, potentially effective treatments that would change behavior substantially if they were implemented properly may be discounted and eliminated from future consideration for similar problems. […] Stability in a dependent variable does not necessarily imply the stable application of the independent variable. [Further,] unless a researcher knows precisely what was done, how it was done, and how long it was done, then replication is impossible.
~ From Gresham (1996) .
Steps and Considerations for Measuring Treatment Fidelity
Provide clear, unambiguous, and comprehensive operational definitions of the independent variable(s). Consider the intervention across four dimensions: verbal, physical, spatial and temporal.
Determine the criteria for accuracy for each component of the independent variable.
Determine the number or percent of sessions for which it is practical to evaluate treatment fidelity.
Record the occurrence/nonoccurrence of the implementation of each component. Calculate the percentage implemented for each component across sessions (component integrity), and the percentage implemented for all components within sessions (session integrity).
Report treatment integrity data and/or methods when publishing the results of studies.
~ From Gresham, Gansle & Noell (1993) and Gresham (1996) .
Further Reading
Billingsley, F.F., White, O.R. & Munson, R. (1980). Procedural reliability: A rationale and an example. Behavioral Assessment, 2, 229–241.
Gresham, F.M. (1996). Treatment integrity in single-subject research. In Franklin, R.D., Allison, D.B. & Gorman, B.S. (Eds.), Design and analysis of single-case research (pp. 93–117). Hillsdale, NJ: Lawrence Erlbaum Associates, Inc.
Kovaleski, J.F. Treatment integrity protocols . RTI Action Network (Available from the RTI Action Network Website at www.rtinetwork.org).
Ralf Schlosser Northeastern University
The content of this page is based on selected clips from a video interview conducted at the ASHA Convention. Additional digested resources and references for further reading were selected by CREd Library staff.
Copyright © 2015 American Speech-Language-Hearing Association
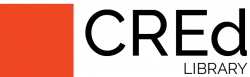
Clinical Research Education
More from the cred library, innovative treatments for persons with dementia, implementation science resources for crisp, when the ears interact with the brain, follow asha journals on twitter.

© 1997-2024 American Speech-Language-Hearing Association Privacy Notice Terms of Use
Patient Care
- Faculty+Staff
- Make an Appointment
- Access Epic CareLink
- Access the Network
- Get Directions
- Request Medical Records
- Find a Specialist
- Find Departments
- Search Jobs
- Donate or Volunteer
- Contact the Institute
- Refer a Patient
- Pay My Bill
Frequently Searched Terms and Topics
- Resources for Patients & Families
Faculty & Staff
Centers & programs.
- , Directions
General Information
Scientific support for applied behavior analysis from the neurobehavioral unit (nbu).
Over the past 40 years, an extensive body of literature has documented the successful use of ABA-based procedures to reduce problem behavior and increase appropriate skills for individuals with intellectual disabilities (ID), autism, and related disorders. The literature consists of numerous controlled studies employing single-case experimental designs, consecutive controlled case-series studies, controlled group studies, and some randomized controlled trials.
Types of Research Designs:
A number of different research designs are used to evaluate treatments and answer other questions about treatment procedures. Each type of design has its own scientific and practical strengths and limitations, and each is ideally suited to answer particular types of questions. The designs are discussed further below.
Single-case experimental designs:
Many studies demonstrating the outcomes obtained with ABA-based procedures use single-case experimental designs (also termed “single-subject designs”; Kazdin, 2010 & 2013) because this type of design is ideal for examining how the behavior of an individual changes as a function of changes in the environment – which is the subject of interest in the field of ABA. These studies often include a small number of individuals (typically between one to four). It should be noted that published studies using single-case experimental designs are not the same as “case reports” (often seen in clinical journals), which are typically simply descriptive in nature. Rather, studies using single-case designs are controlled studies where treatment is applied in a manner that allows one to demonstrate that the treatment was responsible for the change in behavior. These studies are methodologically rigorous because they involve direct observation of behavior and objective data collection where behaviors are defined and counted (often using a computerized data collection system). A second observer also collects data independently to ensure reliable and accurate data collection.
The most common type of single-case design is a reversal design, which involves the following: a pre-treatment baseline level of behavior is obtained, then treatment is applied, and after a change is observed, the treatment is withdrawn, then reapplied to replicate the treatment effect (Kazdin, 2010; Kratochwill & Levin, 2010). The “replication” of the treatment effect illustrates that the treatment (and not some other event) is responsible for the change. This type of design has excellent “internal validity,” which refers to the extent to which the change in behavior can be attributed to the intervention and not some other variable. Single-case designs are limited, however, in that one cannot determine the extent to which the findings for one study are applicable to other individuals or situations (that is, it has weak “external validity”). It is possible that only cases for which treatment was successful were included in the published study (a concern termed “publication bias”). On the other hand, the ABA literature spans four decades and describes the efficacy of these treatments across a wide range of populations, settings, and problems. Collectively, this extensive body of literature provides strong evidence supporting the external validity of ABA-based interventions.
In the field of ABA, single-case experimental designs are not reserved for exclusive use in research studies. Rather, their use represents good clinical practice. During assessment, single-case designs permit one to identify what factors cause the behavior in question. These findings are then prescriptive for developing an individualized treatment. In addition, single-case designs enable one to determine whether a prescribed treatment (or what particular elements of a treatment) is responsible for behavior change. Isolating the active ingredients of treatment is crucial in saving time and resources.
Consecutive controlled case series designs:
Consecutive controlled case-series studies describe a series of cases where single-case experimental designs were used (see Rooker et al., 2013 for a recent example). These studies describe all individuals encountered who were treated with a certain procedure (regardless of whether the treatment was effective or not), and thus have better external validity than cases involving fewer participants. Because all the cases in the series evaluated treatment using single-case experimental designs, consecutive controlled case-series studies have excellent internal validity as well. Moreover, because a large number of individuals are included, they provide an opportunity to answer other questions, including determining what characteristics predict good outcomes. Several large scale consecutive controlled case series studies describing ABA-based assessment and treatment procedures have been published, and their findings nicely correspond to the broader body of single-case studies describing smaller numbers of individuals.
Group designs:
In contrast to single-case experimental designs where the individual’s behavior change during treatment is compared to his/her own behavior without treatment, group designs evaluate treatments based on a comparison of a group of individuals receiving one treatment relative to another similar group of individuals who received no treatment (or a different treatment; Kazdin, 2003). In contrast to single-case designs, where the behaviors of an individual are observed extensively and repeatedly (often for many hours or days) before and after treatment, group designs involve fewer observations of each individual in the group but obtain these measures across large numbers of individuals. Statistical analyses are used to determine whether overall differences between the groups are large enough to conclude that they are not due to normal variation or “chance” (Cohen, Cohen, West, & Aiken, 2003).
The most rigorous type of group design is a randomized controlled trial, which involves randomly assigning participants to a particular group (e.g., treatment or no treatment), and observers who evaluate the outcomes of the treatment do not know whether the participant received treatment or not (i.e., observers are “blinded”). When certain types of treatments, such as medications are being evaluated, the participant may also be “blind” to which group s/he is assigned through the administration of an inactive pill (a placebo). Several group studies describing comprehensive ABA-based interventions for individuals with autism have been published, including some that have used randomization (e.g., Sallows & Graupner, 2005; Smith, Groen, & Wynn, 2000). The most appropriate design to use in a particular situation depends on numerous factors, including the research question, consideration of the relative costs and benefits to participants, and the current state of knowledge about the topic of interest.
Findings from Controlled Studies Employing Single-Case Experimental Designs:
Small-n controlled studies:.
Over a thousand studies reporting on ABA-based assessment and treatment techniques have been published since the 1960’s. As discussed in the “types of research designs” section above, these controlled studies have strong internal validity as they use experimental designs that permit one to conclude that the intervention was responsible for the change in behavior. Studies on topics relevant to the use of ABA with persons with intellectual and developmental disabilities are most frequently published in journals such as: Behavioral Interventions, Journal of Applied Behavior Analysis, Journal of Autism and Developmental Disorders, Journal of Intellectual Disability Research, Research in Developmental Disabilities, Research in Autism Spectrum Disorders. Topics of these studies include communication training, social skills training, behavioral assessment and treatment of problem behavior (e.g., self-injury, aggression), educational instruction, early intensive behavioral intervention, etc. For further information, the reader is referred to these journals or to an on-line search engine (i.e., PsychINFO, Google Scholar).
Consecutive Case-Series Studies:
As discussed on the types of research designs section above, consecutive controlled case-series studies describe a series of cases where single-case experimental designs were used with all individuals encountered (regardless of whether the treatment was effective or not).
Functional Analysis of Problem Behavior:
Focused ABA interventions for problem behavior are designed for each individual based on an understanding of what antecedents may “trigger” problem behavior and what consequences may reinforce (reward) it. Functional behavioral assessment can be performed using a range of procedures, including interviews, questionnaires, direct observation in the individual’s natural setting, and / or systematically presenting situations that can function as potential triggers or rewards and observing and recording how behavior changes with these events. This latter type of procedure, called a functional analysis, is the most rigorous type of functional behavioral assessment. In most cases, the results can reveal why problem behavior occurs and persists – and thus provides a foundation for focused interventions targeting these behaviors.
Literature reviews by Hanley, Iwata, and McCord (2003) and Beavers, Iwata, and Lerman (2013) collectively identified 435 peer-reviewed articles where functional analysis of problem behavior was reported. Studies listed below represent a sample of the large-scale consecutive controlled case series studies involving functional analysis. These studies demonstrate that functional analysis is highly effective in identifying the controlling variables for problem behavior.
Functional analysis across a variety of settings (inpatient, residential) Participants: 154 cases Results: Conclusive assessment results in over 90% of cases Reference: Iwata BA, Pace GM, Dorsey MF, Zarcone JR, Vollmer TR, Smith RG, Rodgers TA, Lerman DC, Shore BA, Mazalesk JL, et al. (1994). The functions of self-injurious behavior: An experimental-epidemiological analysis . Journal of Applied Behavior Analysis, 27(2) , 215-240.
Functional analysis in school settings Participants: 69 cases Results: Conclusive assessment results in over 90% of cases Reference: Mueller MM, Nkosi A, Hine JF. (2011). Functional analysis in public schools: A summary of 90 functional analyses . Journal of Applied Behavior Analysis, 44(4) , 807-818.
Functional analysis of severe problem behavior Participants: 176 cases with severe problem behavior Results: Conclusive assessment results in over 90% of cases References: Hagopian LP, Rooker GW , Jessel J, DeLeon IG . (2013). Initial functional analysis outcomes and modifications in pursuit of differentiation: A summary of 176 inpatient cases . Journal of Applied Behavior Analysis, 46(1) , 88-100.
ABA-Based Focused Treatment for Problem Behavior:
Studies employing rigorous single-case experimental designs describing ABA focused interventions for problem behavior have been reported for four decades. The following sample of large-scale consecutive controlled case series studies provide further support for the effectiveness of these interventions. Findings from these studies parallel findings from reviews and meta-analysis of small-n studies.
Functional communication training for treatment of problem behavior Participants: 21 inpatient cases with IDD Results: 80% or greater reduction in problem behavior in 90% of cases Reference: Hagopian LP , Fisher WW, Sullivan MT, Acquisto J, LeBlanc LA. (1998). Effectiveness of functional communication training with and without extinction and punishment: A summary of 21 inpatient cases . Journal of Applied Behavior Analysis, 31(2) , 211-235.
Function-based treatment for severe problem behavior Participants: 138 inpatient cases with IDD Results: 90% or greater reduction in problem behavior in over 83% of cases Reference: Asmus JM, Ringdahl JE, Sellers JA, Call NA, Andelman MS, Wacker DP. (2004). Use of a short-term inpatient model to evaluate aberrant behavior: Outcome data summaries from 1996 to 2001 . Journal of Applied Behavior Analysis, 37(3) , 283-304.
Functional-based treatment delivered by care providers (mostly parents) for severe problem behavior Participants: 42 outpatient cases with IDD Results: 80% or greater reduction in problem behavior in 95% of cases Reference: Kurtz PF , Fodstad JC, Huete JM, Hagopian LP . (2013). Caregiver- and staff-conducted functional analysis outcomes: A summary of 52 cases . Journal of Applied Behavior Analysis, 46(4) , 738-749.
Functional communication training for treatment of severe problem behavior Participants: 50 inpatient and outpatient cases with IDD Results: 80% or greater reduction in problem behavior in 86% of cases Reference: Rooker GW , Jessel J, Kurtz PF, Hagopian LP . (2013). Functional communication training with and without alternative reinforcement and punishment: An analysis of 58 applications . Journal of Applied Behavior Analysis, 46(4) , 708-722.
Review Papers:
Broadly speaking, review papers summarize the published literature on a specific topic (e.g., diagnosis, type of assessment or treatment procedure). The reader is referred to recent reviews on comprehensive and focused ABA-based interventions for problems associated with autism:
- Anderson C, Law JK , Daniels A, Rice C, Mandell DS, Hagopian L , Law PA. (2012). Occurrence and family impact of elopement in children with autism spectrum disorders . Pediatrics, 130(5) , 870-877.
- Dawson G, Burner K. (2011). Behavioral interventions in children and adolescents with autism spectrum disorder: A review of recent findings . Current Opinion in Pediatrics, 23(6) , 616-620.
- Doehring P, Reichow R, Palk T, Phillips C, Hagopian L . (2012). Behavioral approaches to managing severe problem behaviors in children with Autism Spectrum and Related Developmental Disorders: A descriptive analysis . Child and Adolescent Psychiatry Clinics of NA, 23(1), 25-40.
- Lang R, Mahoney R, El Zein F, Delaune E, Amidon M. (2011). Evidence to practice: Treatment of anxiety in individuals with autism spectrum disorders . Neuropsychiatric Disease and Treatment, 7 , 27-30.
- Myers SM, Johnson CP. (2007). Management of children with autism spectrum disorders . Pediatrics, 120(5) , 1162-1182.
- Reichow B, Volkmar FR. (2010). Social skills interventions for individuals with autism: Evaluation for evidence-based practices within a best evidence synthesis framework . Journal of Autism and Developmental Disorders, 40(2), 149-166.
Recent reviews on ABA-based procedures for persons with intellectual and developmental disabilities (IDD):
- Brosnan J, Healy O. (2011). A review of behavioral interventions for the treatment of aggression in individuals with developmental disabilities . Research in Developmental Disabilities, 32(2) , 437-446.
- Hanley GP, Iwata BA, McCord BE. (2003). Functional analysis of problem behavior: A review . Journal of Applied Behavior Analysis, 36(2) , 147–185.
- Kahng S, Iwata BA, Lewin AB. (2002). Behavioral treatment of self-injury, 1964 to 2000 . American Journal on Mental Retardation, 107(3) , 212-221.
- Lang R, Rispoli M, Machalicek W, White PJ, Kang S, Pierce N, Mulloy A, Fragale T, O'Reilly M, Sigafoos J, Lancioni G. (2009). Treatment of elopement in individuals with developmental disabilities: A systematic review . Research in Developmental Disabilities, 30(4) , 670-681.
- Lilienfeld SO. (2005). Scientifically unsupported and supported interventions for childhood psychopathology: A summary . Pediatrics, 115(3) , 761-764.
- Sturmey P. (2002). Mental retardation and concurrent psychiatric disorder: Assessment and treatment . Current Opinion in Psychiatry, 15 , 489-495.
- Tiger JH, Hanley GP, Bruzek J. (2008). Functional communication training: A review and practical guide . Behavior Analysis in Practice, 1(1) , 16-23.
Review articles indicating that treatments for autism and intellectual disability derived from ABA-based procedures are empirically supported treatments also have been published in non-behavioral journals. For example, the journal Current Opinion in Psychiatry is a journal designed to assist clinicians and researchers by synthesizing the psychiatric literature. An article that reviewed the assessment and treatment of individuals with intellectual disabilities and psychiatric disorders concluded that: "Interventions based on applied behavior analysis have the strongest empirical basis, although there is some evidence that other therapies have promise" (Sturmey, 2002, p. 489). Also, in the journal Pediatrics, the official journal of the American Academy of Pediatrics (AAP), an article offering guidelines on scientifically supported treatments for childhood psychiatric disorders concluded: "The most efficacious psychosocial treatment for autism is applied behavior analysis" (Lilienfeld, 2005, p. 762). The AAP issued a Clinical Report in Pediatrics regarding the management of children with autism, and the authors noted: “Children who receive early intensive behavioral treatment have been shown to make substantial, sustained gains in IQ, language, academic performance, and adaptive behavior as well as some measures of social behavior, and their outcomes have been significantly better than those of children in control groups” (Myers, & Johnson, 2007, p. 1164). In the Archives of Pediatric and Adolescent Medicine, Barbaresi et al. (2006) concluded, “ABA should be viewed as the optimal, comprehensive treatment approach in young children with ASD.”
Review papers finding support for ABA can be found in the following non-behavioral journals:
- Current Opinion in Psychiatry (Grey & Hastings, 2005; Sturmey, 2002)
- Scientific Review of Mental Health Practice (Herbert, Sharp, & Gaudiano, 2002)
- American Journal on Mental Retardation (Kahng, Iwata, & Lewin, 2002)
- Psychiatric Times (Erickson, Swiezy, Stigler, McDougle, & Posey, 2005)
- Archives of Pediatric and Adolescent Medicine (Barbaresi, Katusic, & Voigt, 2006)
- Child and Adolescent Psychiatric Clinics of North America (Doehring, Reichow, Palka, Phillips, & Hagopian, 2014)
Meta-Analyses:
In general, meta-analysis involves quantitative re-analysis of data reported in published studies. This requires standardizing treatment outcomes by statistically calculating “effect sizes” obtained within each study, for the purpose of evaluating data obtained across a group of studies on a particular treatment.
Similarly, seven meta-analyses (Campbell, 2003; Didden, Duker, & Korzilius, 1997; Harvey, Boer, Meyer, & Evans, 2009; Heyvaert, Maes, Van den Noortgate, Kuppens, & Onghena, 2012; Lundervold & Bourland, 1988; Ma, 2009; Weisz, Weiss, Han, Granger, & Morton, 1995) that collectively analyzed hundreds of studies concluded that ABA-based procedures were more effective for reducing problem behavior displayed by individuals with ID (as well as typically-developing individuals) than were alternative treatments. The large body of literature reviewed in these studies provides empirical evidence indicating that focused ABA interventions are effective at assessing and treating a variety of socially important behaviors emitted by individuals with a variety of diagnoses.
Furthermore, several meta-analytic studies also have found comprehensive ABA-based approaches for educating children with autism result in favorable outcomes (Eldevik, Hastings, Hughes, Jahr, Eikeseth, & Cross, 2010; Makrygianni & Reed, 2010; Reichow, 2012; Reichow, Barton, Boyd, & Hume, 2012; Virues-Ortega, 2010). In a recent meta-analytic study involving 22 studies, Virues-Ortega (2010) concluded: “Results suggest that long-term, comprehensive ABA intervention leads to (positive) medium to large effects in terms of intellectual functioning, language development, and adaptive behavior of individuals with autism” (p. 397).
Systematic Evaluative Reviews:
Systematic approaches for formally evaluating a body of research have been developed to determine if a particular intervention can be characterized as “empirically supported” or “established” based on the number, quality, and outcomes of published treatment studies. These efforts have been undertaken for the purpose of guiding clinical practice, influencing regulations and standards, providing priorities for funding (for both research and treatment), and guiding professional training (see Mesibov & Shea, 2011). For example, the American Psychological Association (Task Force Promoting Dissemination of Psychological Procedures, 1995) described a process to identify “empirically supported treatments.” Those interventions with the highest level of support are characterized as “well-established” (Chambless, et al, 1996).
Evaluations of the most commonly used focused ABA-based interventions (functional communication training and noncontingent reinforcement) indicated that these interventions meet criteria as “well-established” empiricially supported treatments (Carr, Severtson, & Lepper, 2009; Kurtz, Boelter, Jarmolowicz, Chin, & Hagopian, 2011). ABA-based treatments for pica (Hagopian, Rooker, & Rolider, 2011), and for treatment of phobic avoidance (Jennett & Hagopian, 2008) displayed by individuals with intellectual disabilities also have been characterized as “well-established.”
The National Standard Project of the National Autism Center developed a similar model to evaluate interventions for problems associated with autism (2009), which used the term “established” to describe interventions with the highest level of support. Using their evaluative method, the National Autism Center (2009) characterized comprehensive ABA-based interventions as being “established” treatments for autism.
Wong and colleagues (2013), as part of the Autism Evidence-Based Practice Review Group, describe a process for the identification of clinical practices that have sufficient empirical support to be termed “evidence-based.” The group stated in regards to the strength of evidence of ABA “Twenty-seven practices met the criteria for being evidence-based (see table 7, page 20)….evidence-based practices consist of interventions that are fundamental applied behavior analysis techniques (e.g., reinforcement, extinction, prompting), assessment and analytic techniques that are the basis for intervention (e.g., functional behavior assessment, task analysis), and combinations of primarily behavioral practices…”
Download Full Article

Want to create or adapt books like this? Learn more about how Pressbooks supports open publishing practices.
10.2 Single-Subject Research Designs
Learning objectives.
- Describe the basic elements of a single-subject research design.
- Design simple single-subject studies using reversal and multiple-baseline designs.
- Explain how single-subject research designs address the issue of internal validity.
- Interpret the results of simple single-subject studies based on the visual inspection of graphed data.
General Features of Single-Subject Designs
Before looking at any specific single-subject research designs, it will be helpful to consider some features that are common to most of them. Many of these features are illustrated in Figure 10.3 “Results of a Generic Single-Subject Study Illustrating Several Principles of Single-Subject Research” , which shows the results of a generic single-subject study. First, the dependent variable (represented on the y -axis of the graph) is measured repeatedly over time (represented by the x -axis) at regular intervals. Second, the study is divided into distinct phases, and the participant is tested under one condition per phase. The conditions are often designated by capital letters: A, B, C, and so on. Thus Figure 10.3 “Results of a Generic Single-Subject Study Illustrating Several Principles of Single-Subject Research” represents a design in which the participant was tested first in one condition (A), then tested in another condition (B), and finally retested in the original condition (A). (This is called a reversal design and will be discussed in more detail shortly.)
Figure 10.3 Results of a Generic Single-Subject Study Illustrating Several Principles of Single-Subject Research
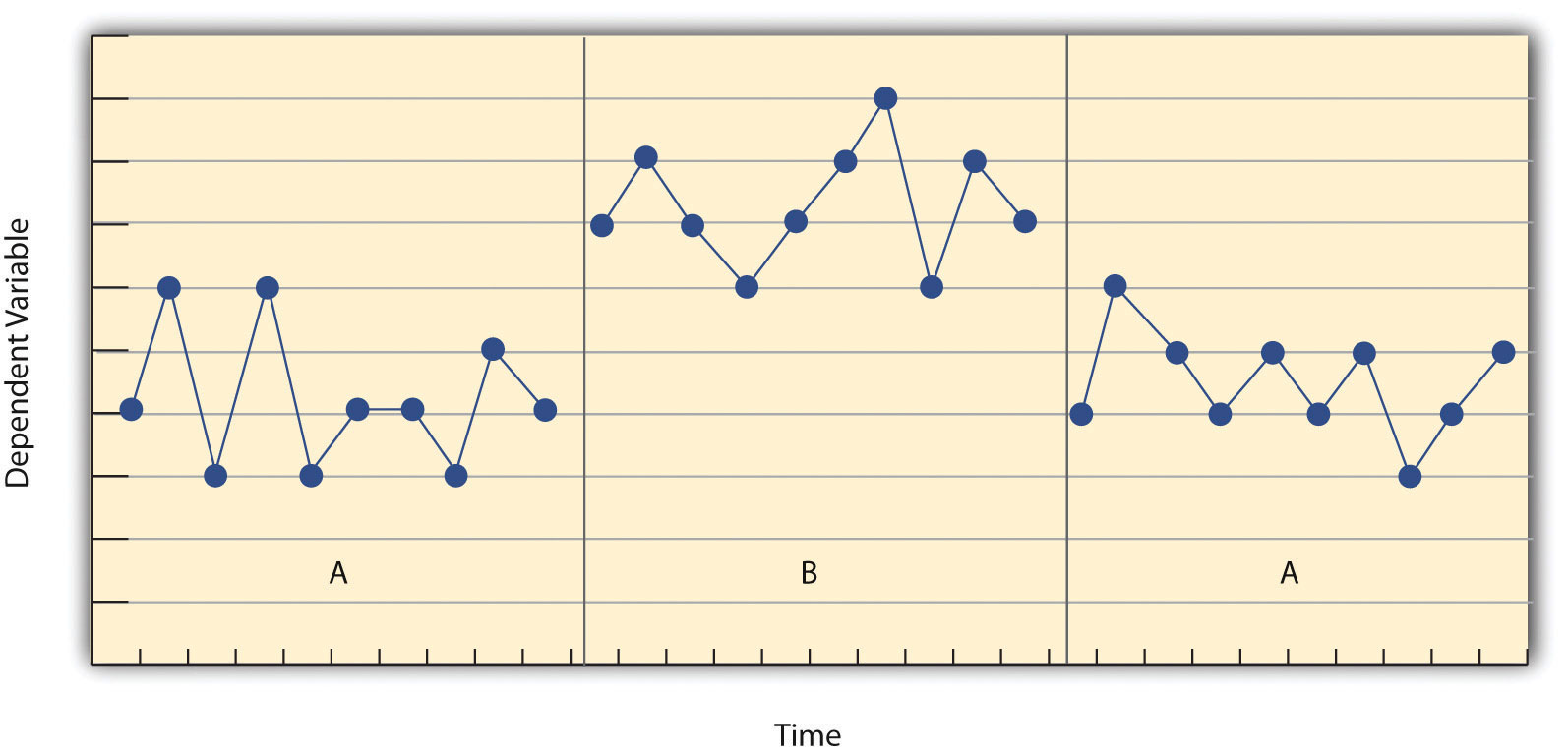
Another important aspect of single-subject research is that the change from one condition to the next does not usually occur after a fixed amount of time or number of observations. Instead, it depends on the participant’s behavior. Specifically, the researcher waits until the participant’s behavior in one condition becomes fairly consistent from observation to observation before changing conditions. This is sometimes referred to as the steady state strategy (Sidman, 1960). The idea is that when the dependent variable has reached a steady state, then any change across conditions will be relatively easy to detect. Recall that we encountered this same principle when discussing experimental research more generally. The effect of an independent variable is easier to detect when the “noise” in the data is minimized.
Reversal Designs
The most basic single-subject research design is the reversal design , also called the ABA design . During the first phase, A, a baseline is established for the dependent variable. This is the level of responding before any treatment is introduced, and therefore the baseline phase is a kind of control condition. When steady state responding is reached, phase B begins as the researcher introduces the treatment. There may be a period of adjustment to the treatment during which the behavior of interest becomes more variable and begins to increase or decrease. Again, the researcher waits until that dependent variable reaches a steady state so that it is clear whether and how much it has changed. Finally, the researcher removes the treatment and again waits until the dependent variable reaches a steady state. This basic reversal design can also be extended with the reintroduction of the treatment (ABAB), another return to baseline (ABABA), and so on.
The study by Hall and his colleagues was an ABAB reversal design. Figure 10.4 “An Approximation of the Results for Hall and Colleagues’ Participant Robbie in Their ABAB Reversal Design” approximates the data for Robbie. The percentage of time he spent studying (the dependent variable) was low during the first baseline phase, increased during the first treatment phase until it leveled off, decreased during the second baseline phase, and again increased during the second treatment phase.
Figure 10.4 An Approximation of the Results for Hall and Colleagues’ Participant Robbie in Their ABAB Reversal Design
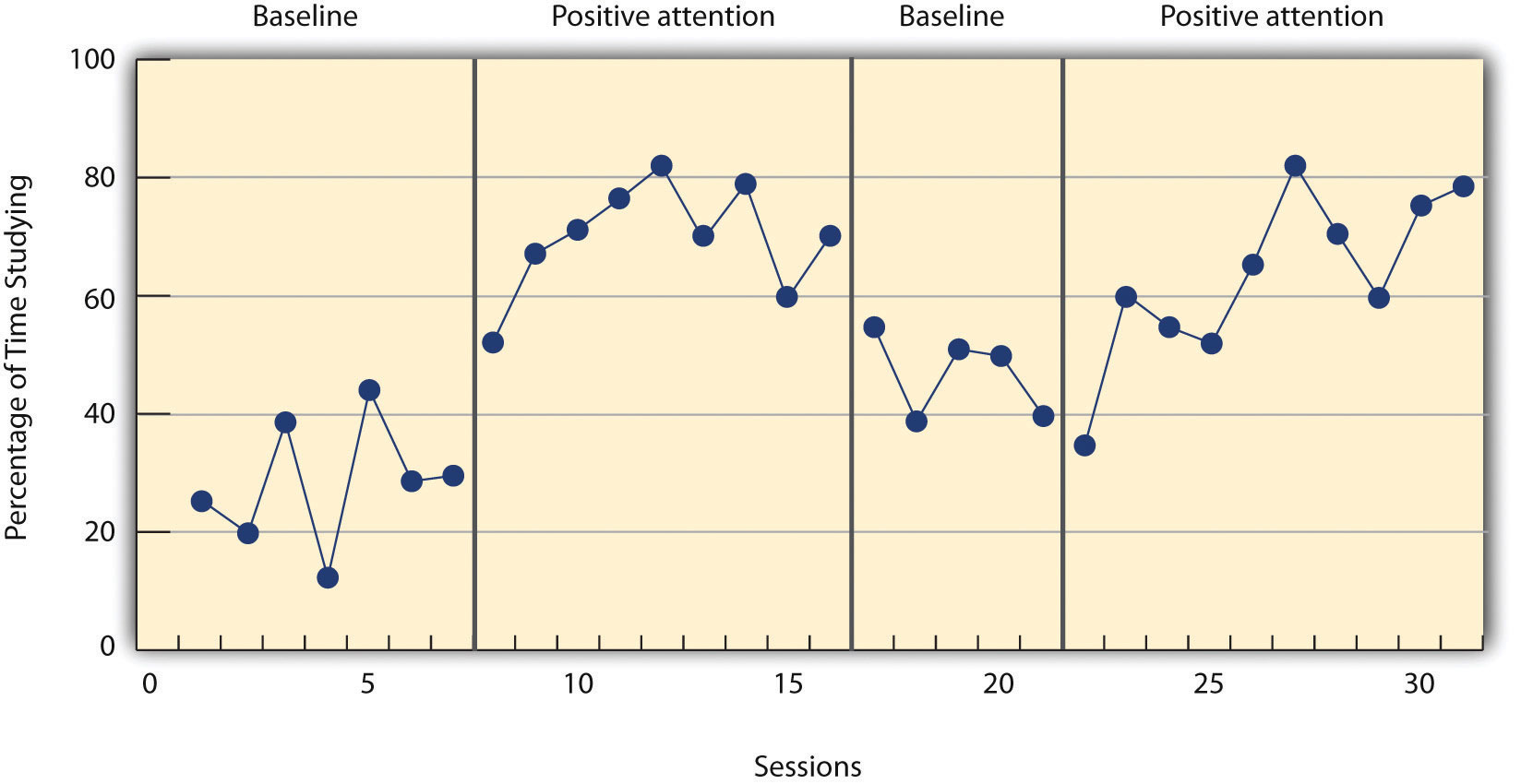
Why is the reversal—the removal of the treatment—considered to be necessary in this type of design? Why use an ABA design, for example, rather than a simpler AB design? Notice that an AB design is essentially an interrupted time-series design applied to an individual participant. Recall that one problem with that design is that if the dependent variable changes after the treatment is introduced, it is not always clear that the treatment was responsible for the change. It is possible that something else changed at around the same time and that this extraneous variable is responsible for the change in the dependent variable. But if the dependent variable changes with the introduction of the treatment and then changes back with the removal of the treatment, it is much clearer that the treatment (and removal of the treatment) is the cause. In other words, the reversal greatly increases the internal validity of the study.
There are close relatives of the basic reversal design that allow for the evaluation of more than one treatment. In a multiple-treatment reversal design , a baseline phase is followed by separate phases in which different treatments are introduced. For example, a researcher might establish a baseline of studying behavior for a disruptive student (A), then introduce a treatment involving positive attention from the teacher (B), and then switch to a treatment involving mild punishment for not studying (C). The participant could then be returned to a baseline phase before reintroducing each treatment—perhaps in the reverse order as a way of controlling for carryover effects. This particular multiple-treatment reversal design could also be referred to as an ABCACB design.
In an alternating treatments design , two or more treatments are alternated relatively quickly on a regular schedule. For example, positive attention for studying could be used one day and mild punishment for not studying the next, and so on. Or one treatment could be implemented in the morning and another in the afternoon. The alternating treatments design can be a quick and effective way of comparing treatments, but only when the treatments are fast acting.
Multiple-Baseline Designs
There are two potential problems with the reversal design—both of which have to do with the removal of the treatment. One is that if a treatment is working, it may be unethical to remove it. For example, if a treatment seemed to reduce the incidence of self-injury in a developmentally disabled child, it would be unethical to remove that treatment just to show that the incidence of self-injury increases. The second problem is that the dependent variable may not return to baseline when the treatment is removed. For example, when positive attention for studying is removed, a student might continue to study at an increased rate. This could mean that the positive attention had a lasting effect on the student’s studying, which of course would be good. But it could also mean that the positive attention was not really the cause of the increased studying in the first place. Perhaps something else happened at about the same time as the treatment—for example, the student’s parents might have started rewarding him for good grades.
One solution to these problems is to use a multiple-baseline design , which is represented in Figure 10.5 “Results of a Generic Multiple-Baseline Study” . In one version of the design, a baseline is established for each of several participants, and the treatment is then introduced for each one. In essence, each participant is tested in an AB design. The key to this design is that the treatment is introduced at a different time for each participant. The idea is that if the dependent variable changes when the treatment is introduced for one participant, it might be a coincidence. But if the dependent variable changes when the treatment is introduced for multiple participants—especially when the treatment is introduced at different times for the different participants—then it is extremely unlikely to be a coincidence.
Figure 10.5 Results of a Generic Multiple-Baseline Study
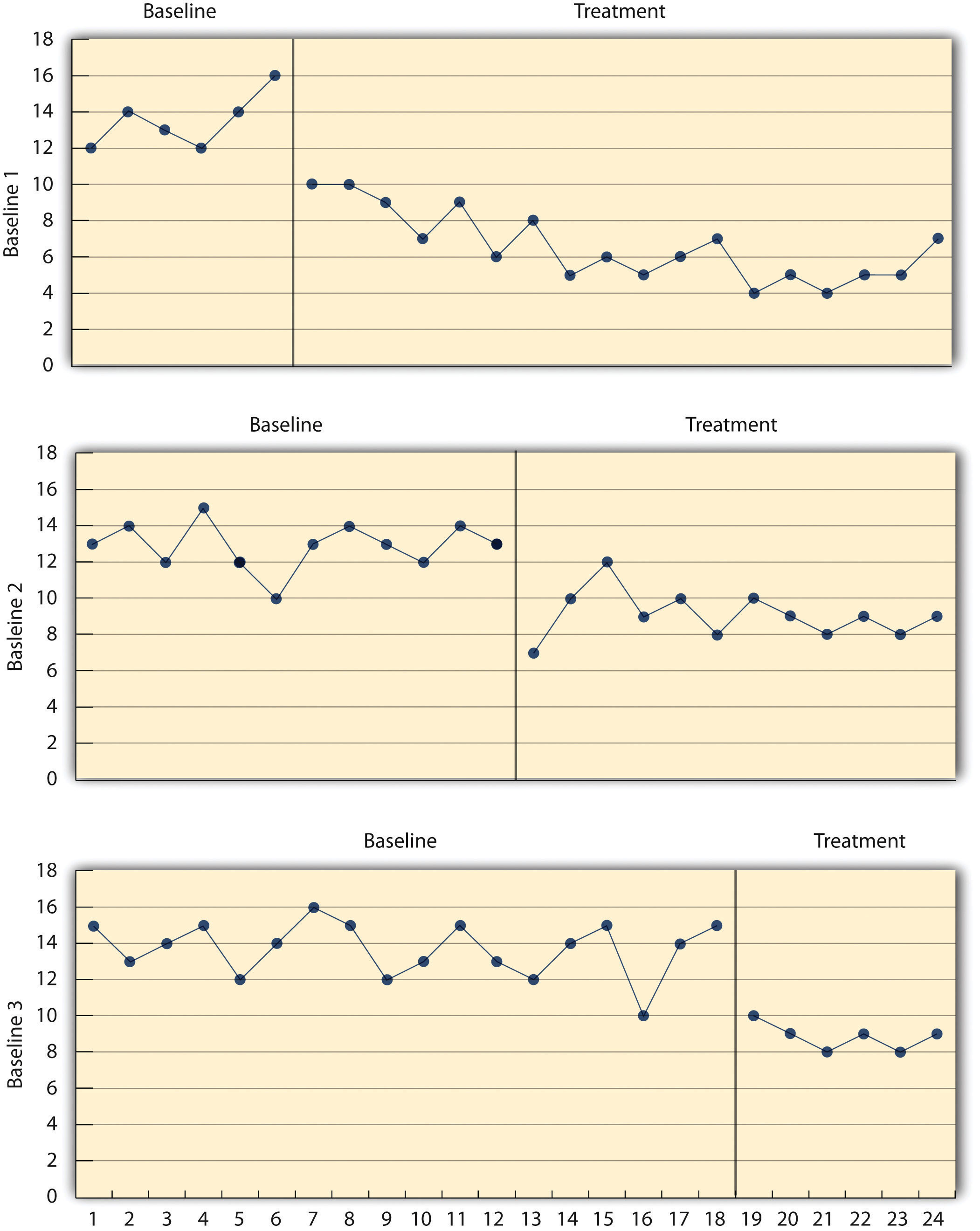
The multiple baselines can be for different participants, dependent variables, or settings. The treatment is introduced at a different time on each baseline.
As an example, consider a study by Scott Ross and Robert Horner (Ross & Horner, 2009). They were interested in how a school-wide bullying prevention program affected the bullying behavior of particular problem students. At each of three different schools, the researchers studied two students who had regularly engaged in bullying. During the baseline phase, they observed the students for 10-minute periods each day during lunch recess and counted the number of aggressive behaviors they exhibited toward their peers. (The researchers used handheld computers to help record the data.) After 2 weeks, they implemented the program at one school. After 2 more weeks, they implemented it at the second school. And after 2 more weeks, they implemented it at the third school. They found that the number of aggressive behaviors exhibited by each student dropped shortly after the program was implemented at his or her school. Notice that if the researchers had only studied one school or if they had introduced the treatment at the same time at all three schools, then it would be unclear whether the reduction in aggressive behaviors was due to the bullying program or something else that happened at about the same time it was introduced (e.g., a holiday, a television program, a change in the weather). But with their multiple-baseline design, this kind of coincidence would have to happen three separate times—a very unlikely occurrence—to explain their results.
In another version of the multiple-baseline design, multiple baselines are established for the same participant but for different dependent variables, and the treatment is introduced at a different time for each dependent variable. Imagine, for example, a study on the effect of setting clear goals on the productivity of an office worker who has two primary tasks: making sales calls and writing reports. Baselines for both tasks could be established. For example, the researcher could measure the number of sales calls made and reports written by the worker each week for several weeks. Then the goal-setting treatment could be introduced for one of these tasks, and at a later time the same treatment could be introduced for the other task. The logic is the same as before. If productivity increases on one task after the treatment is introduced, it is unclear whether the treatment caused the increase. But if productivity increases on both tasks after the treatment is introduced—especially when the treatment is introduced at two different times—then it seems much clearer that the treatment was responsible.
In yet a third version of the multiple-baseline design, multiple baselines are established for the same participant but in different settings. For example, a baseline might be established for the amount of time a child spends reading during his free time at school and during his free time at home. Then a treatment such as positive attention might be introduced first at school and later at home. Again, if the dependent variable changes after the treatment is introduced in each setting, then this gives the researcher confidence that the treatment is, in fact, responsible for the change.
Data Analysis in Single-Subject Research
In addition to its focus on individual participants, single-subject research differs from group research in the way the data are typically analyzed. As we have seen throughout the book, group research involves combining data across participants. Group data are described using statistics such as means, standard deviations, Pearson’s r , and so on to detect general patterns. Finally, inferential statistics are used to help decide whether the result for the sample is likely to generalize to the population. Single-subject research, by contrast, relies heavily on a very different approach called visual inspection . This means plotting individual participants’ data as shown throughout this chapter, looking carefully at those data, and making judgments about whether and to what extent the independent variable had an effect on the dependent variable. Inferential statistics are typically not used.
In visually inspecting their data, single-subject researchers take several factors into account. One of them is changes in the level of the dependent variable from condition to condition. If the dependent variable is much higher or much lower in one condition than another, this suggests that the treatment had an effect. A second factor is trend , which refers to gradual increases or decreases in the dependent variable across observations. If the dependent variable begins increasing or decreasing with a change in conditions, then again this suggests that the treatment had an effect. It can be especially telling when a trend changes directions—for example, when an unwanted behavior is increasing during baseline but then begins to decrease with the introduction of the treatment. A third factor is latency , which is the time it takes for the dependent variable to begin changing after a change in conditions. In general, if a change in the dependent variable begins shortly after a change in conditions, this suggests that the treatment was responsible.
In the top panel of Figure 10.6 , there are fairly obvious changes in the level and trend of the dependent variable from condition to condition. Furthermore, the latencies of these changes are short; the change happens immediately. This pattern of results strongly suggests that the treatment was responsible for the changes in the dependent variable. In the bottom panel of Figure 10.6 , however, the changes in level are fairly small. And although there appears to be an increasing trend in the treatment condition, it looks as though it might be a continuation of a trend that had already begun during baseline. This pattern of results strongly suggests that the treatment was not responsible for any changes in the dependent variable—at least not to the extent that single-subject researchers typically hope to see.
Figure 10.6

Visual inspection of the data suggests an effective treatment in the top panel but an ineffective treatment in the bottom panel.
The results of single-subject research can also be analyzed using statistical procedures—and this is becoming more common. There are many different approaches, and single-subject researchers continue to debate which are the most useful. One approach parallels what is typically done in group research. The mean and standard deviation of each participant’s responses under each condition are computed and compared, and inferential statistical tests such as the t test or analysis of variance are applied (Fisch, 2001). (Note that averaging across participants is less common.) Another approach is to compute the percentage of nonoverlapping data (PND) for each participant (Scruggs & Mastropieri, 2001). This is the percentage of responses in the treatment condition that are more extreme than the most extreme response in a relevant control condition. In the study of Hall and his colleagues, for example, all measures of Robbie’s study time in the first treatment condition were greater than the highest measure in the first baseline, for a PND of 100%. The greater the percentage of nonoverlapping data, the stronger the treatment effect. Still, formal statistical approaches to data analysis in single-subject research are generally considered a supplement to visual inspection, not a replacement for it.
Key Takeaways
- Single-subject research designs typically involve measuring the dependent variable repeatedly over time and changing conditions (e.g., from baseline to treatment) when the dependent variable has reached a steady state. This approach allows the researcher to see whether changes in the independent variable are causing changes in the dependent variable.
- In a reversal design, the participant is tested in a baseline condition, then tested in a treatment condition, and then returned to baseline. If the dependent variable changes with the introduction of the treatment and then changes back with the return to baseline, this provides strong evidence of a treatment effect.
- In a multiple-baseline design, baselines are established for different participants, different dependent variables, or different settings—and the treatment is introduced at a different time on each baseline. If the introduction of the treatment is followed by a change in the dependent variable on each baseline, this provides strong evidence of a treatment effect.
- Single-subject researchers typically analyze their data by graphing them and making judgments about whether the independent variable is affecting the dependent variable based on level, trend, and latency.
Practice: Design a simple single-subject study (using either a reversal or multiple-baseline design) to answer the following questions. Be sure to specify the treatment, operationally define the dependent variable, decide when and where the observations will be made, and so on.
- Does positive attention from a parent increase a child’s toothbrushing behavior?
- Does self-testing while studying improve a student’s performance on weekly spelling tests?
- Does regular exercise help relieve depression?
- Practice: Create a graph that displays the hypothetical results for the study you designed in Exercise 1. Write a paragraph in which you describe what the results show. Be sure to comment on level, trend, and latency.
Fisch, G. S. (2001). Evaluating data from behavioral analysis: Visual inspection or statistical models. Behavioural Processes , 54 , 137–154.
Ross, S. W., & Horner, R. H. (2009). Bully prevention in positive behavior support. Journal of Applied Behavior Analysis , 42 , 747–759.
Scruggs, T. E., & Mastropieri, M. A. (2001). How to summarize single-participant research: Ideas and applications. Exceptionality , 9 , 227–244.
Sidman, M. (1960). Tactics of scientific research: Evaluating experimental data in psychology . Boston, MA: Authors Cooperative.
Research Methods in Psychology Copyright © 2016 by University of Minnesota is licensed under a Creative Commons Attribution-NonCommercial-ShareAlike 4.0 International License , except where otherwise noted.
Randomized single-case AB phase designs: Prospects and pitfalls
- Published: 18 July 2018
- Volume 51 , pages 2454–2476, ( 2019 )
Cite this article
- Bart Michiels 1 , 2 &
- Patrick Onghena 1
9813 Accesses
42 Citations
15 Altmetric
Explore all metrics
Single-case experimental designs (SCEDs) are increasingly used in fields such as clinical psychology and educational psychology for the evaluation of treatments and interventions in individual participants. The AB phase design , also known as the interrupted time series design , is one of the most basic SCEDs used in practice. Randomization can be included in this design by randomly determining the start point of the intervention. In this article, we first introduce this randomized AB phase design and review its advantages and disadvantages. Second, we present some data-analytical possibilities and pitfalls related to this design and show how the use of randomization tests can mitigate or remedy some of these pitfalls. Third, we demonstrate that the Type I error of randomization tests in randomized AB phase designs is under control in the presence of unexpected linear trends in the data. Fourth, we report the results of a simulation study investigating the effect of unexpected linear trends on the power of the randomization test in randomized AB phase designs. The implications of these results for the analysis of randomized AB phase designs are discussed. We conclude that randomized AB phase designs are experimentally valid, but that the power of these designs is sufficient only for large treatment effects and large sample sizes. For small treatment effects and small sample sizes, researchers should turn to more complex phase designs, such as randomized ABAB phase designs or randomized multiple-baseline designs.
Similar content being viewed by others
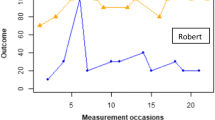
Quantitative Techniques and Graphical Representations for Interpreting Results from Alternating Treatment Design
Rumen Manolov, René Tanious & Patrick Onghena
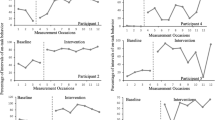
The Power to Explain Variability in Intervention Effectiveness in Single-Case Research Using Hierarchical Linear Modeling
Mariola Moeyaert, Panpan Yang & Xinyun Xu
Experimental Designs for Research on Adaptive Interventions: Singly and Sequentially Randomized Trials
Avoid common mistakes on your manuscript.
Introduction
Single-case experimental designs (SCEDs) can be used to evaluate treatment effects for specific individuals or to assess the efficacy of individualized treatments. In such designs, repeated observations are recorded for a single person on a dependent variable of interest, and the treatment can be considered as one of the levels of the independent variable (Barlow, Nock, & Hersen, 2009 ; Kazdin, 2011 ; Onghena, 2005 ). SCEDs are widely used as a methodological tool in various domains of science, including clinical psychology, school psychology, special education, and medicine (Alnahdi, 2015 ; Chambless & Ollendick, 2001 ; Gabler, Duan, Vohra, & Kravitz, 2011 ; Hammond & Gast, 2010 ; Kratochwill & Stoiber, 2000 ; Leong, Carter, & Stephenson, 2015 ; Shadish & Sullivan, 2011 ; Smith, 2012 ; Swaminathan & Rogers, 2007 ). The growing interest in these types of designs can be inferred from the recent publication of guidelines for reporting the results of SCEDs in various fields of the educational, behavioral, and health sciences (Shamseer et al., 2015 ; Tate et al., 2016 ; Vohra et al., 2015 ).
SCEDs are often confused with case studies or other nonexperimental research, but these types of studies should be clearly distinguished from each other (Onghena & Edgington, 2005 ). More specifically, SCEDs involve the deliberate manipulation of an independent variable, whereas such a manipulation is absent in nonexperimental case studies. In addition, the reporting of results from SCEDs usually involves visual and statistical analyses, whereas case studies are often reported in a narrative way.
SCEDs should also be distinguished from experimental designs that are based on comparing groups. The principal difference between SCEDs and between-subjects experimental designs concerns the definition of the experimental units. Whereas the experimental units in group-comparison studies refer to participants assigned to different groups, the experimental units in SCEDs refer to repeated measurements of specific entities under investigation (e.g., a person) that are assigned to different treatments (Edgington & Onghena, 2007 ). Various types of SCEDs exist. In the following section we will discuss the typology of single-case designs.
Typology of single-case experimental designs
A comprehensive typology of SCEDs can be constructed using three dimensions: (1) whether the design is a phase or an alternation design, (2) whether or not the design contains random assignment, and (3) whether or not the design is replicated. We will discuss each of these dimensions in turn.
Design type
Various types of SCEDs can be broadly categorized into two main types: phase designs and alternation designs (Heyvaert & Onghena, 2014 ; Onghena & Edgington, 2005 ; Rvachew & Matthews, 2017 ), although hybrids of both types are possible (see, e.g., Levin, Ferron, & Gafurov, 2014 ; Onghena, Vlaeyen, & de Jong, 2007 ). Phase designs divide the sequence of measurement occasions in a single-case experiment (SCE) into separate treatment phases, with each phase containing multiple measurements (Edgington, 1975a , 1980 ; Onghena, 1992 ). The basic building block of phase designs is the AB phase design that features a succession of a baseline phase (A) and a treatment phase (B). This basic design can be expanded by including more A phases or B phases leading to more complex phase designs such as ABA and ABAB phase designs. Furthermore, it is also possible to construct phase designs that compare more than two treatments (e.g., an ABC design). In contrast to phase designs, alternation designs do not feature distinct phases but rather involve rapid alternation of the experimental conditions throughout the course of the SCE. Consequently, these designs are intended for research situations in which rapid and frequent alternation of treatments is possible (Barlow & Hayes, 1979 ; Onghena & Edgington, 1994 ). Some common alternation designs include the completely randomized design (CRD), the randomized block design (RBD), and the alternating treatments design (ATD, Onghena, 2005 ). Manolov and Onghena ( 2017 ) provide a recent overview of the use of ATDs in published single-case research and discuss various data-analytical techniques for this type of design.
Random assignment
When treatment labels are randomly assigned to measurement occasions in an SCED, one obtains a randomized SCED. This procedure of random assignment in an SCED is similar to the way in which subjects are randomly assigned to experimental groups in a between-subjects design. The main difference is that in SCEDs repeated measurement occasions for one subject are randomized across two or more experimental conditions whereas in between-subjects designs individual participants are randomized across two or more experimental groups. The way in which SCEDs can be randomized depends on the type of design. Phase designs can be randomized by listing all possible intervention start points and then randomly selecting one of them for conducting the actual experiment (Edgington, 1975a ). Consider, for example, an AB design, consisting of a baseline (A) phase and a treatment (B) phase, with a total of ten measurement occasions and a minimum of three measurement occasions per phase. For this design there are six possible start points for the intervention, leading to the following divisions of the measurement occasions:
This type of randomization can also be applied to more complex phase designs, such as ABA or ABAB phase designs, by randomly selecting time points for all the moments of phase change in the design (Onghena, 1992 ). Alternation designs are randomized by imposing a randomization scheme on the set of measurement occasions, in which the treatment conditions are able to alternate throughout the experiment. The CRD is the simplest alternation design as it features “unrestricted randomization.” In this design, only the number of measurement occasions for each level of the independent variable has to be fixed. For example, if we consider a hypothetical SCED with two conditions (A and B) and three measurement occasions per condition, there are 20 possible randomizations \( \left(\genfrac{}{}{0pt}{}{6}{3}\right) \) using a CRD:
The randomizations schemes for an RBD or an ATD can be constructed by imposing additional constraints on the CRD randomization scheme. For example, an RBD is obtained by grouping measurement occasions in pairs and randomizing the treatment order within each pair. For the same number of measurement occasions as in the example above, an RBD yields 2 3 = 8 possible randomizations, which are a subset of the CRD randomizations.
This type of randomization can be useful to counter the effect of time-related confounding variables on the dependent variable, as the randomization within pairs (or blocks of a certain) size eliminates any time-related effects that might occur within these pairs. An ATD randomization scheme can be constructed from a CRD randomization scheme with the restriction that only a certain maximum number of successive measurement occasions are allowed to have the same treatment, which ensures rapid treatment alternation. Using the example of our hypothetical SCED, an ATD with a maximum number of two consecutive administrations of the same condition yields the following 14 randomizations:
Note again that all of these randomizations are a subset of the CRD randomizations. Many authors have emphasized the importance of randomizing SCEDs for making valid inferences (e.g., Dugard, 2014 ; Dugard, File, & Todman, 2012 ; Edgington & Onghena, 2007 ; Heyvaert, Wendt, Van den Noortgate, & Onghena, 2015 ; Kratochwill & Levin, 2010 ). The benefits and importance of incorporating random assignment in SCEDs are also stressed in recently developed guidelines for the reporting of SCE results, such as the CONSORT extension for reporting N -of-1 trials (Shamseer et al., 2015 ; Vohra et al., 2015 ) and the single-case reporting guideline in behavioral interventions statement (Tate et al., 2016 ). SCEDs that do not incorporate some form of random assignment are still experimental designs in the sense that they feature a deliberate manipulation of an independent variable, so they must still be distinguished from nonexperimental research such as case studies. That being said, the absence of random assignment in a SCED makes it harder to rule out alternative explanations for the occurrence of a treatment effect, thus weakening the internal validity of the design. In addition, it should be noted that the incorporation of randomization in SCEDs is still relatively rare in many domains of research.
Replication
It should be noted that research projects and single-case research publications rarely involve only one SCED, and that usually replication is aimed at. Kratochwill et al. ( 2010 ) noted that replication also increases the internal validity of an SCED. In this sense it is important to emphasize that randomization and replication should be used concurrently for increasing the internal validity of an SCED. Replication can occur in two different ways: simultaneously or sequentially (Onghena & Edgington, 2005 ). Simultaneous replication designs entail conducting multiple alternation or phase designs at the same time. The most widely used simultaneous replication design is the multiple baseline across participants design, which combines two or more phase designs (usually AB phase designs), in which the treatment is administered in a time-staggered manner across the individual participants (Hammond & Gast, 2010 ; Shadish & Sullivan, 2011 ). Sequential replication designs entail conducting individual SCEs sequentially in order to test the generalizability of the results to other participants, settings, or outcomes (Harris & Jenson, 1985 ; Mansell, 1982 ). Also for this part of the typology, it is possible to create hybrid designs by combining simultaneous and sequential features—for example, by sequentially replicating multiple-baseline across-participant designs or using a so-called “nonconcurrent multiple baseline design,” with only partial temporal overlap (Harvey, May, & Kennedy, 2004 ; Watson & Workman, 1981 ). Note that alternative SCED taxonomies have been proposed (e.g., Gast & Ledford, 2014 ). The focus of the present article is on the AB phase design, also known as the interrupted time series design (Campbell & Stanley, 1966 ; Cook & Campbell, 1979 ; Shadish, Cook, & Campbell, 2002 ).
The single-case AB phase design
The AB phase design is one of the most basic and practically feasible experimental designs for evaluating treatments in single-case research. Although widely used in practice, the AB phase design has received criticism for its low internal validity (Campbell, 1969 ; Cook & Campbell, 1979 ; Kratochwill et al., 2010 ; Shadish et al., 2002 ; Tate et al., 2016 ; Vohra et al., 2015 ). Several authors have rated the AB phase design as “quasi-experimental” or even “nonexperimental,” because the lack of a treatment reversal phase leaves the design vulnerable to the internal validity threats of history and maturation (Kratochwill et al., 2010 ; Tate et al., 2016 ; Vohra et al., 2015 ). History refers to the confounding influence of external factors on the treatment effect during the course of the experiment, whereas maturation refers to changes within the subject during the course of the experiment that may influence the treatment effect (Campbell & Stanley, 1966 ). These confounding effects can serve as alternative explanations for the occurrence of a treatment effect other than the experimental manipulation and as such threaten the internal validity of the SCED. Kratochwill et al. argue that the internal validity threats of history and maturation are mitigated when SCEDs contain at least two AB phase pair repetitions. More specifically, their argument is that the probability that history effects (e.g., the participant turns ill during the experiment) occurring simultaneously with the introduction of the treatment is smaller when there are multiple introductions of the treatment than in the situation in which there is only one introduction of the treatment. Similarly, to lessen the impact of potential maturation effects (e.g., spontaneous improvement of the participant yielding an upward or downward trend in the data) on the internal validity of the SCED, Kratochwill et al. argue that an SCED should be able to record at least three demonstrations of the treatment effect. For these reasons, they argue that only phase designs with at least two AB phase pair repetitions (e.g., an ABAB design) are valid SCEDs, and that designs with only one AB phase pair repetition (e.g., an AB phase design) are inadequate for drawing valid inferences. Similarly, Tate et al. and Vohra et al. do not consider the AB phase design as a valid SCED. More specifically, Tate et al. consider the AB phase design as a quasi-experimental design, and Vohra et al. even regard the AB phase design as a nonexperimental design, putting it under the same label as case studies. In contrast, the SCED classification by Logan, Hickman, Harris, and Heriza ( 2008 ) does include the AB phase design as a valid design.
Rather than using discrete classifications, we propose a gradual view of evaluating the internal validity of an SCED. In the remainder of this article we will argue that randomized AB phase designs have an important place in the methodological toolbox of the single-case researcher as valid SCEDs. It is our view that the randomized AB phase design can be used as a basic experimental design for situations in which this design is the only feasible way to collect experimental data (e.g., when evaluating treatments that cannot be reversed due to the nature of the treatment or because of ethical concerns). We will build up this argument in several steps. First, we will explain how random assignment strengthens the internal validity of AB phase designs as compared to AB phase designs without random assignment, and discuss how the internal validity of randomized AB phase designs can be increased further through the use of replication and formal statistical analysis. Second, after mentioning some common statistical techniques for analyzing randomized AB phase designs we will discuss the use of a statistical technique that can be directly derived from the random assignment that is present in randomized AB phase designs: the randomization test (RT). In addition we will discuss some potential data-analytical pitfalls that can occur when analyzing randomized AB phase designs and argue how the use of the RT can mitigate some of these pitfalls. Furthermore, we will provide a worked example of how AB phase designs can be randomized and subsequently analyzed with the RT using the randomization method proposed by Edgington ( 1975a ). Third, we will demonstrate the validity of the RT when analyzing randomized AB phase designs containing a specific manifestation of a maturation effect: An unexpected linear trend that occurs in the data yielding a gradual increase in the scores of the dependent variable that is unrelated to the administration of the treatment. More specifically we will show that the RT controls the Type I error rate when unexpected linear trends are present in the data. Finally, we will also present the results of a simulation study that investigated the power of the RT when analyzing randomized AB phase designs containing various combinations of unexpected linear trends in the baseline phase and/or treatment phase. Apart from controlled Type I error rates, adequate power is another criterion for the usability of the RT for specific types of datasets. Previous research already investigated the effect of different levels of autocorrelation on the power of the RT in randomized AB phase designs but only for data without trend (Ferron & Ware, 1995 ). However, a study by Solomon ( 2014 ) showed that trend is quite common in single-case research, making it important to investigate the implications of trend effects on the power of the RT.
Randomized AB phase designs are valid single-case experimental designs
There are several reasons why the use of randomized AB phase designs should be considered for conducting single-case research. First of all, the randomized AB phase design contains all the required elements to fit the definition of an SCED: A design that involves repeated measurements on a dependent variable and a deliberate experimental manipulation of an independent variable. Second, the randomized AB phase design is the most feasible single-case design for treatments that cannot be withdrawn for practical or ethical reasons and also the most cost-efficient and the most easily implemented of all phase designs (Heyvaert et al., 2017 ). Third, if isolated randomized AB phase designs were dismissed as invalid, and if only a randomized AB phase design was feasible, given the very nature of psychological and educational interventions that cannot be reversed or considered undone, then practitioners would be discouraged from using an SCED altogether, and potentially important experimental evidence would never be collected.
We acknowledge that the internal validity threats of history and maturation have to be taken into account when drawing inferences from AB phase designs. Moreover we agree with the views from Kratochwill et al. ( 2010 ) that designs with multiple AB phase pairs (e.g., an ABAB design) offer better protection from threats to internal validity than designs with only one AB phase pair (e.g., the AB phase design). However, we also argue that the internal validity of the basic AB phase design can be strengthened in several ways.
First, the internal validity of the AB phase design (as well as other SCEDs) can be increased considerably by incorporating random assignment into the design (Heyvaert et al., 2015 ). Random assignment can neutralize potential history effects in SCEDs as random assignment of measurement occasions to treatment conditions allows us to statistically control confounding variables that may manifest themselves throughout the experiment. In a similar vein, random assignment can also neutralize potential maturation effects because any behavioral changes that might occur within the subject are unrelated to the random allocation of measurement occasions to treatment conditions (Edgington, 1996 ). Edgington ( 1975a ) proposed a way to incorporate random assignment into the AB phase design. Because the phase sequence in a AB phase design is fixed, random assignment should respect this phase structure. Therefore, Edgington ( 1975a ) proposed to randomize the start point of the treatment phase. In this approach the researcher initially specifies the total number of measurement occasions to be included in the design along with limits for the minimum number of measurement occasions to be included in each phase. This results in a range of potential start points for the treatment phase. The researcher then randomly selects one of these start points to conduct the actual experiment. By randomizing the start point of the treatment phase in the AB phase design it becomes possible to evaluate the treatment effect for each of the hypothetical start points from the randomization process and to compare these hypothetical treatment effects to the observed treatment effect from the start point that was used for the actual experiment. Under the assumption that potential confounding effects such as history and maturation are constant for the various possible start points of the treatment phase these effects are made less plausible as alternative explanations in case a statistically significant treatment effect is found. As such, incorporating random assignment into the AB phase design can also provide a safeguard for threats against internal validity without the need for adding extra phases to the design. This method of randomizing start points in AB phase designs can easily be extended to more complex phase designs such as ABA or ABAB designs by generating random start points for each moment of phase change in the design (Levin et al., 2014 ; Onghena, 1992 ).
Second, the internal validity of randomized AB phase designs can be increased further by replications, and replicated randomized AB phase designs are acceptable by most standards (e.g., Kratochwill et al., 2010 ; Tate et al., 2016 ). When a treatment effect can be demonstrated across multiple replicated randomized AB phase designs, it lowers the probability that this treatment effect is caused by history or maturation effects rather than by the treatment itself. In fact, when multiple randomized AB phase designs are replicated across participants and the treatment is administered in a staggered manner across the participants, one obtains a multiple-baseline across-participant design, which is accepted as a valid SCED according to many standards (Kratochwill et al., 2010 ; Logan et al., 2008 ; Tate et al., 2016 ; Vohra et al., 2015 ).
Third, one can increase the chance of making valid inferences from randomized AB phase designs by analyzing them statistically with adequate statistical techniques. Many data-analytical techniques for single-case research focus mainly on analyzing randomized AB phase designs and strengthening the resulting inferences (e.g., interrupted time series analysis, Borckardt & Nash, 2014 ; Gottman & Glass, 1978 ; nonoverlap effect size measures, Parker, Vannest, & Davis, 2011 ; multilevel modeling, Van den Noortgate & Onghena, 2003 ). Furthermore, one can analyze the randomized AB phase design using a statistical test that is directly derived from the random assignment that is present in the design: the RT (Kratochwill & Levin, 2010 ; Onghena & Edgington, 2005 ).
Data analysis of randomized AB phase designs: techniques and pitfalls
Techniques for randomized AB phase designs can be broadly categorized in two groups: visual analysis and statistical analysis (Heyvaert et al., 2015 ). Visual analysis refers to inspecting the observed data for changes in level, phase overlap, variability, trend, immediacy of the effect, and consistency of data patterns across similar phases (Horner, Swaminathan, Sugai, & Smolkowski, 2012 ). The advantages of visual analysis are that it is quick, intuitive, and requires little methodological knowledge. The main disadvantages of visual analysis are that small but systematic treatment effects are hard to detect (Kazdin, 2011 ) and that it is associated with low interrater agreement (e.g., Bobrovitz & Ottenbacher, 1998 ; Ximenes, Manolov, Solanas, & Quera, 2009 ). Although visual analysis remains widely used for analyzing randomized AB phase designs (Kazdin, 2011 ), there is a general consensus that visual analysis should be used concurrently with supplementary statistical analyses to corroborate the results (Harrington & Velicer, 2015 ; Kratochwill et al., 2010 ).
Techniques for the statistical analysis of randomized AB phase designs can be divided into three groups: effect size calculation, statistical modeling, and statistical inference. Effect size (ES) calculation involves evaluating treatment ESs by calculating formal ES measures. One can discern proposals that are based on calculating standardized mean difference measures (e.g., Busk & Serlin, 1992 ; Hedges, Pustejovsky, & Shadish, 2012 ), proposals that are based on calculating overlap between phases (see Parker, Vannest, & Davis, 2011 , for an overview), proposals that are based on calculating standardized or unstandardized regression coefficients (e.g., Allison & Gorman, 1993 ; Solanas, Manolov, & Onghena, 2010 ; Van den Noortgate & Onghena, 2003 ), and proposals that are based on Bayesian methods (Rindskopf, Shadish, & Hedges, 2012 ; Swaminathan, Rogers, & Horner, 2014 ). Statistical modeling refers to constructing an adequate description of the data by fitting the data to a statistical model. Some proposed modeling techniques include interrupted time series analysis (Borckardt & Nash, 2014 ; Gottman & Glass, 1978 ), generalized mixed models (Shadish, Zuur, & Sullivan, 2014 ), multilevel modeling (Van den Noortgate & Onghena, 2003 ), Bayesian modeling techniques (Rindskopf, 2014 ; Swaminathan et al., 2014 ), and structural equation modeling (Shadish, Rindskopf, & Hedges, 2008 ).
Statistical inference refers to assessing the statistical significance of treatment effects through hypothesis testing or by constructing confidence intervals for the parameter estimates (Heyvaert et al., 2015 ; Michiels, Heyvaert, Meulders, & Onghena, 2017 ). On the one hand, inferential procedures can be divided into parametric and nonparametric procedures, and on the other hand, they can be divided into frequentist and Bayesian procedures. One possibility for analyzing randomized AB phase designs is to use parametric frequentist procedures, such as statistical tests and confidence intervals based on t and F distributions. The use of these procedures is often implicit in some of the previously mentioned data-analytical proposals, such as the regression-based approach of Allison and Gorman ( 1993 ) and the multilevel model approach of Van den Noortgate and Onghena ( 2003 ). However, it has been shown that data from randomized AB phase designs often violate the specific distributional assumptions made by these parametric procedures (Shadish & Sullivan, 2011 ; Solomon, 2014 ). As such, the validity of these parametric procedures is not guaranteed when they are applied to randomized AB phase designs. Bayesian inference can be either parametric or nonparametric, depending on the assumptions that are made for the prior and posterior distributions of the Bayesian model employed. De Vries and Morey ( 2013 ) provide an example of parametric Bayesian hypothesis testing for the analysis of randomized AB phase designs.
An example of a nonparametric frequentist procedure that has been proposed for the analysis of randomized AB phase designs is the RT (e.g., Bulté & Onghena, 2008 ; Edgington, 1967 ; Heyvaert & Onghena, 2014 ; Levin, Ferron, & Kratochwill, 2012 ; Onghena, 1992 ; Onghena & Edgington, 1994 , 2005 ). The RT can be used for statistical inference based on random assignment. More specifically, the test does not make specific distributional assumptions or an assumption of random sampling, but rather obtains its validity from the randomization that is present in the design. When measurement occasions are randomized to treatment conditions according to the employed randomization scheme, a statistical reference distribution for a test statistic S can be calculated. This reference distribution can be used for calculating nonparametric p values or for constructing nonparametric confidence intervals for S by inverting the RT (Michiels et al., 2017 ). The RT is also flexible with regard to the choice of the test statistic (Ferron & Sentovich, 2002 ; Onghena, 1992 ; Onghena & Edgington, 2005 ). For example, it is possible to use an ES measure based on standardized mean differences as the test statistic in the RT (Michiels & Onghena, 2018 ), but also ES measures based on data nonoverlap (Heyvaert & Onghena, 2014 ; Michiels, Heyvaert, & Onghena, 2018 ). This freedom to devise a test statistic that fits the research question makes the RT a versatile statistical tool for various research settings and treatment effects (e.g., with mean level differences, trends, or changes in variability; Dugard, 2014 ).
When using inferential statistical techniques for randomized AB phase designs, single-case researchers can encounter various pitfalls with respect to reaching valid conclusions about the efficacy of a treatment. A first potential pitfall is that single-case data often violate the distributional assumptions of parametric hypothesis tests (Solomon, 2014 ). When distributional assumptions are violated, parametric tests might inflate or deflate the probability of Type I errors in comparison to the nominal significance level of the test. The use of RTs can provide a safeguard from this pitfall: Rather than invoking distributional assumptions, the RT procedure involves the derivation of a reference distribution from the observed data. Furthermore, an RT is exactly valid by construction: It can be shown that the probability of committing a Type I error using the RT is never larger than the significance level α , regardless of the number of measurement occasions or the distributional properties of the data (Edgington & Onghena, 2007 ; Keller, 2012 ). A second pitfall is the presence of serial dependencies in the data (Shadish & Sullivan, 2011 ; Solomon, 2014 ). Serial dependencies can lead to inaccurate variance estimates in parametric hypothesis tests, which in turn can result in either too liberal or too conservative tests. The use of RTs can also provide a solution for this pitfall. Although the presence of serial dependencies does affect the power of the RT (Ferron & Onghena, 1996 ; Ferron & Sentovich, 2002 ; Levin et al., 2014 ; Levin et al., 2012 ), the Type I error of the RT will always be controlled at the nominal level, because the serial dependency is identical for each element of the reference distribution (Keller, 2012 ). A third pitfall that can occur when analyzing randomized AB phase designs is that these designs typically employ a small number of measurement occasions (Shadish & Sullivan, 2011 ). As such, statistical power is an issue with these designs. A fourth pitfall to analyzing single-case data is the presence of an unexpected data trend (Solomon, 2014 ). One way that unexpected data trends can occur is through maturation effects (e.g., a gradual reduction in pain scores of a patient due to a desensitization effect). In a subsequent section of this article, we will show that the RT does not alter the probability of a Type I error above the nominal level for data containing general linear trends, and thus it also mitigates this pitfall.
Analyzing randomized AB phase designs with randomization tests: a hypothetical example
For illustrative purposes, we will discuss the steps involved in constructing a randomized AB phase design and analyzing the results with an RT by means of a hypothetical example. In a first step, the researcher chooses the number of measurement occasions to be included in the design and the minimum number of measurement occasions to be included in each separate phase. For this illustration we will use the hypothetical example of a researcher planning to conduct a randomized AB phase design with 26 measurement occasions and a minimum of three measurement occasions in each phase. In a second step, the design can be randomized using the start point randomization proposed by Edgington ( 1975a ). This procedure results in a range of potential start points for the treatment throughout the course of the SCE. Each individual start point gives rise to a unique division of measurement occasions into baseline and treatment occasions in the design (we will refer to each such a division as an assignment ). The possible assignments for this particular experiment can be obtained by placing the start point at each of the measurement occasions, respecting the restriction of at least three measurement occasions in each phase. There are 21 possible assignments, given this restriction (not all assignments are listed):
AAABBBBBBBBBBBBBBBBBBBBBBB
AAAABBBBBBBBBBBBBBBBBBBBBB
AAAAABBBBBBBBBBBBBBBBBBBBB
AAAAAAAAAAAAAAAAAAAAABBBBB
AAAAAAAAAAAAAAAAAAAAAABBBB
AAAAAAAAAAAAAAAAAAAAAAABBB
Suppose that the researcher randomly selects the assignment with the 13th measurement occasion as the start point of the B phase for the actual experiment: AAAAAAAAAAAABBBBBBBBBBBBBB. In a third step, the researcher chooses a test statistic that will be used to quantify the treatment effect. In this example, we will use the absolute difference between the baseline phase mean and the treatment phase mean as a test statistic. In a fourth step, the actual experiment with the randomly selected start point is conducted, and the data are recorded. Suppose that the recorded data of the experiment are 0, 2, 2, 3, 1, 3, 3, 2, 2, 2, 2, 2, 6, 7, 5, 8, 5, 6, 5, 7, 4, 6, 8, 5, 6, and 7. Figure 1 displays these hypothetical data graphically. In a fifth step, the researcher calculates the randomization distribution, which consists of the value of the test statistic for each of the possible assignments. The randomization distribution for the present example consists of 21 values (not all values are listed; the observed value is marked in bold):
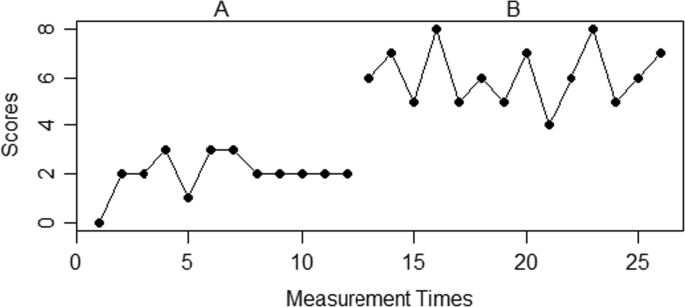
Data from a hypothetical AB design
In a final step, the researcher can calculate a two-sided p value for the observed test statistic by determining the proportion of test statistics in the randomization distribution that are at least as extreme as the observed test statistic. In this example, the observed test statistic is the most extreme value in the randomization distribution. Consequently, the p value is 1/21, or .0476. This p value can be interpreted as the probability of observing the data (or even more extreme data) under the null hypothesis that the outcome is unrelated to the levels of the independent variable. Note that the calculation of two-sided p values are preferable if the treatment effects can go in both directions. Alternatively, the randomization test can also be inverted, in order to obtain a nonparametric confidence interval of the observed treatment effect (Michiels et al., 2017 ). The benefit of calculating confidence intervals over p values is that the former conveys the same information as the latter, with the advantage of providing a range of “plausible values” for the test statistic in question (du Prel, Hommel, Röhrig, & Blettner, 2009 ).
The Type I error of the randomization test for randomized AB phase designs in the presence of unexpected linear trend
One way in which a maturation effect can manifest itself in an SCED is through a linear trend in the data. Such a linear trend could be the result of a sensitization or desensitization effect that occurs in the participant, yielding an unexpected upward or downward trend throughout the SCE that is totally unrelated to the experimental manipulation of the design. The presence of such an unexpected data trend can seriously diminish the power of hypothesis tests in which the null and alternative hypotheses are formulated in terms of differences in mean level between phases, to the point that they become useless. A convenient property of the start point randomization of the randomized AB phase design in conjunction with the RT analysis is that the RT offers nominal Type I error rate protection for data containing linear trends under the null hypothesis that there is no differential effect of the treatment on the A phase and the B phase observations. Before illustrating this property with a simple derivation, we will demonstrate that, in contrast to the RT, a two-sample t test greatly increases the probability of a Type I error for data with a linear trend. Suppose that we have a randomized AB phase design with ten measurement occasions (with five occasions in the A phase and five in the B phase). Suppose there is no intervention effect and we just have a general linear time trend (“maturation”):
A t test on these data with a two-sided alternative hypothesis results in a t value of 5 for eight degrees of freedom, and a p value of .0011, indicating a statistically significant difference between the means at any conventional significance level. In contrast, an RT on these data produces a p value of 1, which is quite the opposite from a statistically significant treatment effect. The p value of 1 can be explained by looking at the randomization distribution for this particular example (assuming a minimum of three measurement occasions per case):
The test statistic values for all randomizations are identical, leading to a maximum p value of 1. The result for the RT in this hypothetical example is reassuring, and it can be shown that the RT with differences between means as the test statistic guarantees Type I error rate control in the presence of linear trends, whereas the rejection rate of the t test increases dramatically with increasing numbers of measurement occasions.
The nominal Type I error rate protection of the RT in a randomized AB phase design for data containing a linear trend holds in a general way. If the null hypothesis is true, the data from a randomized AB phase design with a linear trend can be written as
with Y t being the dependent variable score at time t , β 0 being the intercept, β 1 being the slope of the linear trend, ε t being the residual error, T being the time variable, and t being the time index. Assuming that the errors have a zero mean, the expected value for these data is
In a randomized AB phase design, these scores are divided between an A phase ( \( {\widehat{Y}}_{\mathrm{At}} \) ) and a B phase ( \( {\widehat{Y}}_{\mathrm{Bt}} \) ):
and with n A + n B = n . The mean of the expected A phase scores ( \( {\widehat{\overline{Y}}}_{\mathrm{A}} \) ) and the mean of the expected B phase scores ( \( {\widehat{\overline{Y}}}_{\mathrm{B}} \) ) are equal to
Consequently, the difference between \( {\widehat{\overline{Y}}}_{\mathrm{B}} \) and \( {\widehat{\overline{Y}}}_{\mathrm{A}} \) equals
which simplifies to
This derivation shows that, under the null hypothesis, \( {\widehat{\overline{Y}}}_{\mathrm{B}}-{\widehat{\overline{Y}}}_{\mathrm{A}} \) is expected to be a constant for every assignment of the randomized AB phase design. The expected difference between means, \( {\widehat{\overline{Y}}}_{\mathrm{B}}-{\widehat{\overline{Y}}}_{\mathrm{A}} \) , is only a function of the slope of the linear trend, β 1 , and the total number of measurement occasions, n . This implies that the expected value of the test statistic for each random start point is identical if the null hypothesis is true, exactly what is needed for Type I error rate control. In contrast, the rejection rate of the t test will increase with increasing β 1 and increasing n , because the difference between means constitutes the numerator of the t test statistic, and the test will only refer to Student’s t distribution with n – 2 degrees of freedom. The t test will therefore detect a difference between means that is merely the result of a general linear trend.
The result of this derivation can be further clarified by comparing the null hypotheses that are evaluated in both the RT and the t test. The null hypothesis of the t test states that there is no difference in means between the A phase observations and the B phase observations, whereas the null hypothesis of the RT states that there is no differential effect of the levels of the independent variable (i.e., the A and B observations) on the dependent variable. A data set with a perfect linear trend such as the one displayed above yields a mean level difference between the A phase observations and the B phase observations, but no differential effect between the A phase observations and the B phase observations (i.e., the trend effect is identical for both the A phase and the B phase observations). For this reason, the null hypothesis of the t test gets rejected, whereas the null hypothesis of the RT is not. Consequently, we can conclude that the RT is better suited for detecting unspecified treatment effects than is the t test, because its null hypothesis does not specify the nature of the treatment effect. Note that the t test, in contrast to the RT, assumes a normal distribution, homogeneity of variances, and independent errors, assumptions that are often implausible for SCED data. It is also worth noting that, with respect to the prevention of Type I errors, the RT also has a marked advantage over visual analysis, as the latter technique offers no way to prevent such errors when dealing with unexpected treatment effects. Consequently, we argue that statistical analysis using RTs is an essential technique for achieving valid conclusions from randomized AB phase designs.
The effect of unexpected linear trends on the power of the randomization test in randomized AB phase designs: a simulation study
In the previous section, we showed the validity of the randomized AB phase design and the RT with respect to the Type I error for data containing unexpected linear trends. Another criterion for the usability of the RT for specific types of data sets, apart from controlled Type I error rates, is adequate power. In this section we focus on the power of the RT in the randomized AB phase design when the data contain unexpected linear trends. Previous research has not yet examined the effect of unexpected linear data trends on the power of the RT in randomized AB phase designs. However, Solomon ( 2014 ) investigated the presence of linear trends in a large sample of published single-case research and found that the single-case data he surveyed were characterized by moderate levels of linear trend. As such, it is important to investigate the implications of unexpected data trends for the power of the RT in randomized AB phase designs.
When assessing the effect of linear trend on the power of the RT, we should make a distinction between the situation in which a data trend is expected and the situation in which a data trend is not expected. Edgington ( 1975b ) proposed a specific type of RT for the former situation. More specifically, the proposed RT utilizes a test statistic that takes the predicted trend into account, in order to increase its statistical power. Using empirical data from completely randomized designs, Edgington ( 1975b ) illustrated that such an RT can be quite powerful when the predicted trend is accurate. Similarly, a study by Levin, Ferron, and Gafurov ( 2017 ) showed that the power of the RT can be increased for treatment effects that are delayed and/or gradual in nature, by using adjusted test statistics that account for these types of effects. Of course, in many realistic research situations, data trends are either unexpected or are expected but cannot be accurately predicted. Therefore, we performed a Monte Carlo simulation study to investigate the effect of unexpected linear data trends on the power of the RT when it is used to assess treatment effects in randomized AB phase designs. A secondary goal was to provide guidelines for the number of measurement occasions to include in a randomized AB phase design, in order to achieve sufficient power for different types of data patterns containing trends and various treatment effect sizes. Following the guidelines by Cohen ( 1988 ), we defined “sufficient power” as a power of 80% or more.
The Monte Carlo simulation study contained the following factors: mean level change, a trend in the A phase, a trend in the B phase, autocorrelation in the residuals, and the number of measurement occasions for each data set. We used the model of Huitema and McKean ( 2000 ) to generate the data. This model uses the following regression equation:
Y t being the outcome at time t , with t = 1, 2, . . . , n A , n A +1, . . . , n A + n B ,
n A being the number of observations in the A phase,
n B being the number of observations in the B phase,
β 0 being the regression intercept,
T t being the time variable that indicates the measurement occasions,
D t being the value of the dummy variable indicating the treatment phase at time t ,
[ T t – ( n A +1)] D t being the value of the slope change variable at time t ,
β 1 being the regression coefficient for the A phase trend,
β 2 being the regression coefficient for the mean level treatment effect,
β * 3 being the regression coefficient for the slope change variable, and
ε t being the error at time t .
In this simulation study, we will sample ε t from a standard normal distribution or from a first-order autoregressive model (AR1) model.
The A phase trend, the treatment effect, and the B phase slope change correspond to the β 1 , β 2 , and β * 3 regression coefficients of the Huitema–McKean model, respectively. Note that β * 3 of the Huitema–McKean model indicates the amount of slope change in the B phase relative to the A phase trend. For our simulation study, we defined a new parameter (denoted by β 3 ) that indicates the value of the trend in the B phase independent of the level of trend in the A phase. The relation between β * 3 and β 3 can be written as follows: β 3 = β * 3 + β 1 . To include autocorrelation in the simulated data sets, the ε t s were generated from an AR1 model with different values for the AR parameter. Note that residuals with an autocorrelation of 0 are equivalent to the residuals from a standard normal distribution. The power of the RT was evaluated for two different measures of ES: an absolute mean difference statistic (MD) and an immediate treatment effect index (ITEI).
The MD is defined as
with \( \overline{A} \) being the mean of all A phase observations and \( \overline{B} \) being the mean of all B phase observations. The ITEI is defined as
with \( {\overline{A}}_{ITEI} \) being the mean of the last three A phase observations before the introduction of the treatment and \( {\overline{B}}_{ITEI} \) being the mean of the first three B phase observations after the introduction of the treatment. For each of the simulation factors, the following levels were used in the simulation study:
β 1 : 0, .25, .50
β 2 : – 4, – 1, 0, 1, 4
β 3 : – .50, – .25, 0, .25, .50
AR1: – .6, – .3, 0, .3, .6.
N : 30, 60, 90, 120
ES: MD, ITEI
The β 1 and β 3 values were based on a survey by Solomon ( 2014 ), who calculated trend values through linear regression for a large number of single-case studies. A random-effects meta-analysis showed that the mean standardized trend regression weight for all analyzed data was .37, with a 95% confidence interval of [.28 ; .43]. On the basis of these results, we defined a “small” trend as a standardized regression weight of .25 and a “large” trend as a standardized regression weight of .50. Note that we included upward trends (i.e., β 3 values with a positive sign) as well as downward trends in the B phase (i.e., β 3 with a negative sign), in order to account for data patterns with A phase trends and B phase trends that go in opposite directions. It was not necessary to also include downward trends in the A phase, because this would lead to some data patterns being just mirror images (when only the direction of the A phase trend as compared to the B phase trend was considered) in the full factorial crossing of all included parameter values. The full factorial combination of these three β 1 values and five β 3 values resulted in 15 different data patterns containing an A phase trend and/or a B phase trend. Table 1 provides an overview of these 15 data patterns, and Fig. 2 illustrates the data patterns visually. Note that the data patterns in Fig. 2 only serve to illustrate the described A phase trends and/or B phase trends, as these patterns do not contain any data variability nor a mean level treatment effect. Hereafter, we will use the numbering in Table 1 to refer to each of the 15 data patterns individually.
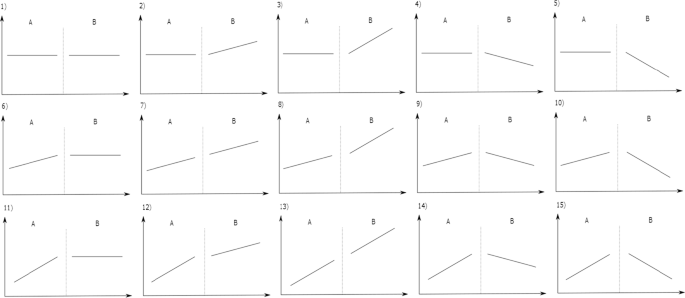
Fifteen AB data patterns containing an A phase trend and/or a B phase trend
The values for β 2 were based on the standardized treatment effects reported by Harrington and Velicer ( 2015 ), who used interrupted time series analyses on a large number of empirical single-case data sets published in the Journal of Applied Behavioral Analysis. The Huitema–McKean model is identical to the interrupted time series model of Harrington and Velicer when the autoregressive parameter of the latter model is zero. We collected the d values (which correspond to standardized β 2 values in the Huitema–McKean model) reported in Table 1 of Harrington and Velicer’s study, and defined β 2 = 1 as a “small” treatment effect and β 2 = 4 as a “large” treatment effect. These values were the 34th and 84th percentiles of the empirical d distribution, respectively. The AR1 parameter values were based on a survey by Solomon ( 2014 ), who reported a mean absolute autocorrelation of .36 across a large number of single-case data sets. On the basis of this value, we defined .3 as a realistic AR1 parameter value. To obtain an additional “bad case scenario” condition with respect to autocorrelation, we doubled the empirical value of .3. Both the AR1 values of .3 and .6 were included with negative and positive signs in the simulation study, in order to assess the effects of both negative and positive autocorrelation. The numbers of measurement occasions of the simulated data sets were either 30, 60, 90, or 120. We chose a lower limit of 30 measurement occasions because this is the minimum number of measurement occasions that is needed in a randomized AB phase design with at least five measurement occasions in each phase to achieve a p value equal to .05 or smaller. The upper limit of 120 measurement occasions was chosen on the basis of a survey by Harrington and Velicer that showed that SCEDs rarely contain more than 120 measurement occasions.
The ES measures used in this simulation study are designed to quantify two important aspects of evaluating treatment effects of single-case data, according to the recommendations of the What Works Clearinghouse (WWC) Single-Case Design Standards (Kratochwill et al., 2010 ). The first aspect is the overall difference in level between phases, which we quantified using the absolute mean difference between all A phase observations and all B phase observations. Another important indicator for treatment effectiveness in randomized AB phase designs is the immediacy of the treatment effect (Kratochwill et al., 2010 ). For this aspect of the data, we calculated an immediate treatment effect index (ITEI). On the basis of the recommendation by Kratochwill et al., we defined the ITEI in a randomized AB phase design as the average difference between the last three A observations and the first three B observations. Both ESs were used as the test statistic in the RT for this simulation study. In accordance with the WWC standards’ recommendation that a “phase” should consist of five or more measurement occasions (Kratochwill et al., 2010 ), we took a minimum limit of five measurement occasions per phase into account for the start point randomization in the RT. A full factorial crossing of all six simulation factors yielded 3,750 simulation conditions. The statistical power of the RT for each condition was calculated by generating 1,000 data sets and calculating the proportion of rejected null hypotheses at a 5% significance level across these 1,000 replications.
The results will be presented in two parts. To evaluate the effect of the simulation factors on the power of the RT, we will present the main effects of each simulation factor. Apart from a descriptive analysis of the statistical power in the simulation conditions, we will also look at the variation between conditions using a multiway analysis of variance (ANOVA). We will limit the ANOVA to main effects because the interaction effects between the simulation factors were small and difficult to interpret. For each main effect, we will calculate eta-squared ( η 2 ) in order to identify the most important determinants of the results. Second, we will report the power for each specific AB data pattern that was included in the simulation study for both the MD and the ITEI.
Main effects
The results from the multiway ANOVA indicated that all simulation factors had a statistically significant effect on the power of the RT at the .001 significance level. Table 2 displays the η 2 values for the main effect of each simulation factor, indicating the relative importance of these factors in determining the power of the RT, in descending order.
Table 2 shows that by far the largest amount of variance was explained by the size of the treatment effect ( β 2 ). Of course, this result is to be expected, because the size of the treatment effect ranged from 0 to 4 (in absolute value), which is a very large difference. The large amount of variance explained by the treatment effect size also accounts for the large standard deviations for the power levels of the other main effects (displayed in Tables 4 – 8 in the Appendix). To visualize the effect of the simulation factors on the RT’s power, we plotted the effect of each simulation factor in interaction with the size of the treatment effect ( β 2 ) while averaging the power across all other simulation factors in the simulation study in Fig. 3 . The means and standard deviations of the levels of the main effect for each experimental factor (averaged across all other simulation factors, including the size of the treatment effect) can be found in Tables 4 – 8 in the Appendix.
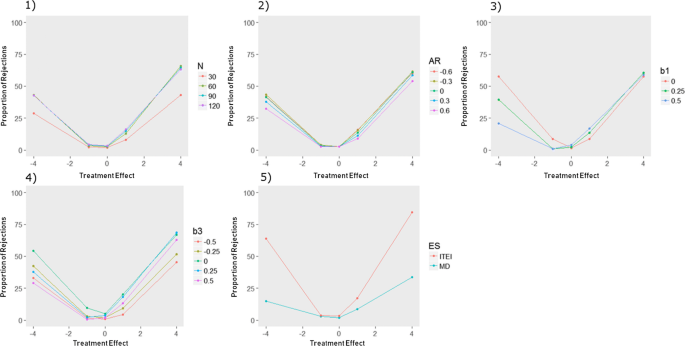
Effects of the simulation factors of the simulation study in interaction with the size of the treatment effect: (1) the number of measurement occasions, (2) the level of autocorrelation, (3) the A phase trend, (4) the B phase trend, and (5) the test statistic used in the randomization test. The proportions of rejections for the conditions in which the treatment effect is zero are the Type I error rates. N = number of measurement occasions, AR = autoregression parameter, β 1 = A phase trend regression parameter, β 3 = B phase trend regression parameter, ES = effect size measure
Panels 1–5 in Fig. 3 show the main effects of the number of measurement occasions, the level of autocorrelation, the size of the A phase trend, the size of the B phase trend, and the effect size measure used, respectively, on the power of the RT. We will summarize the results concerning the main effects for each of these experimental factors in turn.
Number of measurement occasions
Apart from the obvious result that an increase in the number of measurement occasions increases the power of the RT, we can also see that the largest substantial increase in average power occurs when increasing the number of measurement occasions from 30 to 60. In contrast, increasing the number of measurement occasions from 60 to 90, or even from 90 to 120, yields only very small increases in average power.
Level of autocorrelation
The main result for this experimental factor is that the presence of positive autocorrelation in the data decreases the power, whereas the presence of negative autocorrelation increases the power. However, Table 2 shows that the magnitude of this effect is relatively small as compared to the other effects in the simulation study.
Effect size measure
The results show that the ITEI on average yields larger power than does the MD for the types of data patterns that were used in this simulation study.
A phase trend (β 1 )
On average, the power of the randomized AB phase design is reduced when there is an A phase trend in the data, and this reduction increases when the A phase trend gets larger.
B phase trend (β 3 )
The presence of B phase trend in the data reduces the power of the RT, as compared to data without a B phase trend. In addition, the power reduction increases as the B phase trend gets larger. Furthermore, the increase in the reduction of power is larger for downward B phase trends than for upward B phase trends for data that also contain an upward A phase trend. Because the A phase trends in this simulation study were all upward trends, we can conclude that the power reduction associated with the presence of B phase trend is larger when the B phase trend has a direction opposite the direction of the A phase trend than in the situation in which both trends have the same direction. Similarly, it is also evident across all panels of Fig. 3 that the power of the RT is lower for treatment effects that have a direction opposite to the direction of the A phase trend.
Finally, the conditions in Fig. 3 in which the treatment effect is zero show that the manipulation of each experimental factor did not inflate the Type I error rate of the RT above the nominal significance level. However, this result is to be expected, as the RT provides guaranteed nominal Type I error control.
Trend patterns
In this section we will discuss the power differences between the different types of data patterns in the simulation study. In addition, we will pay specific attention to the differences between the MD and the ITEI in the different data patterns, as the ES measure that was used in the RT was the experimental factor that explained the most variance in the ANOVA apart from the size of the treatment effect. Figure 4a contains the power graphs for Data Patterns 1–5, Fig. 4b contains the power graphs for Data Patterns 6–10, and Fig. 4c contains the power graphs for Data Patterns 11–15.
Data patterns with no A phase trend (Data Patterns 1–5): The most important results regarding Data Patterns 1–5 can be summarized in the following bullet points:
For data patterns without any trend (Data Pattern 1), the average powers of the MD and the ITEI are similar.
The average power of the ITEI is substantially larger than the average power of the MD for data patterns with any type of B phase trend (Data Patterns 2–5).
Comparison of Data Patterns 2 and 3 shows that the average power advantage of the ITEI as compared to the MD in data patterns with an upward B phase trend increases as the B phase trend grows larger.
The average power of the MD in Data Patterns 2–5 is very low.
The average power graphs for Data Patterns 1–5 are symmetrical, which means that the results for negative and positive mean level treatment effects are similar.
Data patterns with an A phase trend of .25 (Data Patterns 6–10):
For all five of these data patterns, the ITEI has a large average power advantage as compared to the MD, for both positive and negative treatment effects.
The average powers of both the ITEI and the MD are higher when the treatment effect has the same direction as the A phase trend, as compared to when the effects go in opposite directions.
The average power difference between the MD and the ITEI is larger when the A phase trend and the treatment effect go in opposite directions than when they have the same direction.
When the A phase trend and the B phase trend have the same value (Data Pattern 7), the average power advantage of the ITEI relative to the MD disappears, but only for positive treatment effects.
The average power of the MD is extremely low in nearly all data patterns.
Data patterns with an A phase trend of .50 (Data Patterns 11–15):
In comparison to Data Patterns 6–10, the overall average power drops due to the increased size of the A phase trend (for both the ITEI and the MD and for both positive and negative treatment effects).
For all five data patterns, the ITEI has a large average power advantage over the MD, for both positive and negative treatment effects.
When the A phase trend and the B phase trend have the same value (Data Pattern 13), the average power advantage of the ITEI relative to the MD disappears, but only for positive treatment effects.
The average power of the MD is extremely low for all types of treatment effects in all data patterns (except for Data Pattern 13). In contrast, the ITEI still has substantial average power, but only for positive treatment effects.
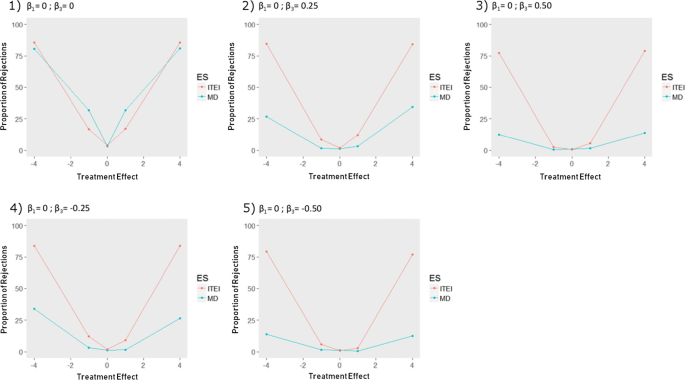
a Power graphs for the five AB data patterns without an A phase trend. β 1 and β 3 represent the trends in the A and B phases, respectively. b Power graphs for the five AB data patterns with an upward A phase trend of .25. β 1 and β 3 represent the trends in the A and B phases, respectively. c Power graphs for the five AB data patterns with an upward A phase trend of .5. β 1 and β 3 represent the trends in the A and B phases, respectively
The most important results regarding differences between the individual data patterns and between the MD and the ITEI can be summarized as follows:
The presence of A phase trend and/or B phase trend in the data decreases the power of the RT, as compared to data without such trends, and the decrease is proportional to the magnitude of the trend.
Treatment effects that go in the same direction as the A phase trend can be detected with higher power than treatment effects that go in the opposite direction from the A phase trend.
The ITEI yields higher power than does the MD in data sets with trends, especially for large trends and trends that have a direction opposite from the direction of the treatment effect.
An additional result regarding the magnitude of the power in the simulation study is that none of the conditions using 30 measurement occasions reached a power of 80% or more. Also, all conditions that reached a power of 80% or more contained large treatment effects ( β 2 = 4). The analysis of the main effects showed that designs with 90 or 120 measurement occasions only yielded very small increases in power as compared to designs with 60 measurement occasions. Table 3 contains an overview of the average powers for large positive and large negative mean level treatment effects ( β 2 = |4|) for each of the 15 different data patterns with 60 measurement occasions, for both the MD and the ITEI (averaged over the levels of autocorrelation in the data).
Upon inspecting Table 3 , one can see that for detecting differences in mean level (i.e., the simulation conditions using the MD as the test statistic), the randomized AB phase design only has sufficient power for data patterns without any trend (Data Pattern 1) or for data patterns in which the A phase trend and the B phase trend are equal (Data Patterns 7 and 13) and in which the treatment effect is in the same direction as the A phase trend. With respect to detecting immediate treatment effects, one can see that the randomized AB phase design had sufficient power for all the data patterns with no A phase trend included in the simulation study, provided that the treatment effect was large (Data Patterns 1–5). For data patterns with A phase trend, the randomized AB phase design also has sufficient power, provided that the treatment effect is in the same direction as the A phase trend. When the treatment effect is in the opposite direction from the A phase trend, the randomized AB phase design only has sufficient power when both the A phase trend and the B phase trend are small (Data Patterns 6, 7, and 9). It is also important to note that the RT only has sufficient power for large treatment effects.
Discussion and future research
In this article we have argued that randomized AB phase designs are an important part of the methodological toolbox of the single-case researcher. We discussed the advantages and disadvantages of these designs in comparison with more complex phase designs, such as ABA and ABAB designs. In addition, we mentioned some common data-analytical pitfalls when analyzing randomized AB phase designs and discussed how the RT as a data-analytical technique can lessen the impact of some of these pitfalls. We demonstrated the validity of the RT in randomized AB phase designs containing unexpected linear trends and investigated the implications of unexpected linear data trends for the power of the RT in randomized AB phase designs. To cover a large number of potential empirical data patterns with linear trends, we used the model of Huitema and McKean ( 2000 ) for generating data sets. The power was assessed for both the absolute mean phase difference (MD, designed to evaluate differences in level) and the immediate treatment effect index (ITEI, designed to evaluate the immediacy of the effect) as the test statistic in the RT. In addition, the effect of autocorrelation on the power of the RT in randomized AB phase designs was investigated by incorporating residual errors with different levels of autocorrelation into the Huitema–McKean model.
The results showed that the presence of any combination of A phase trend and/or B phase trend reduced the power of the RT in comparison to data patterns without trend. In addition, the results showed that the ITEI yielded substantially higher power in the RT than did the MD for randomized AB phase designs containing linear trend. Autocorrelation only had a small effect on the power of the RT, with positive autocorrelation diminishing the power of the RT and negative autocorrelation increasing its power. Furthermore, the results showed that none of the conditions using 30 measurement occasions reached a power of 80% or more. However, the power increased dramatically when the number of measurement occasions was increased to 60. The main effect of number of measurement occasions showed that the power of randomized AB phase designs with 60 measurement occasions hardly benefits from an increase to 90 or even 120 measurement occasions.
The overarching message of this article is that the randomized AB phase design is a potentially valid experimental design. More specifically, the use of repeated measurements, a deliberate experimental manipulation, and random assignment all increase the probability that a valid inference regarding the treatment effect of an intervention for a single entity can be made. In this respect, it should be noted that the internal validity of an experimental design is also dependent on all plausible rival hypotheses, and that it is difficult to make general statements regarding the validity of a design, regardless of the research context. As such, we recommend that single-case researchers should not reject randomized AB phase designs out of hand, but consider how such designs can be used in a valid manner for their specific purposes.
The results from this simulation study showed that the randomized AB phase design has relatively low power: A power of 80% or more is only reached when treatment effects are large and the design contains a substantial number of measurement occasions. These results echo the conclusions of Onghena ( 1992 ), who investigated the power of randomized AB phase designs for data without trend or autocorrelation. That being said, this simulation study also showed that it is possible to achieve a power of 80% or more for specific data patterns containing unexpected linear trends and/or autocorrelation, at least for large effect sizes.
One possibility for increasing the power of the RT for data sets with trends may be the use of adjusted test statistics that accurately predict the trend (Edgington, 1975b ; Levin et al., 2017 ). Rather than predicting the trend before the data are collected, another option might be to specify an adjusted test statistic after data collection using masked graphs (Ferron & Foster-Johnson, 1998 ).
Recommendations with regard to an appropriate number of measurement occasions for conducting randomized AB phase designs should be made cautiously, for several reasons. First, the manipulation of the treatment effect in this simulation study was very large and accounted for most of the variability in the power. Consequently, the expected size of the treatment effect is an important factor in selecting the number of measurement occasions for the randomized AB phase design. Of course, the size of the treatment effect cannot be known beforehand, but it is plausible that effect size magnitudes vary depending on the specific domain of application. Second, we did not investigate possible interactions between the various experimental factors, because these would be very difficult to interpret. However, these potential interactions might have an effect on the power of different types of data patterns, making it more difficult to formulate general recommendations. Taking the previous disclaimers into account, we can state that randomized AB phase designs in any case should contain more than 30 measurement occasions to achieve adequate power. Note that Shadish and Sullivan ( 2011 ) reported that across a survey of 809 published SCEDs, the median number of measurement occasions was 20, and that 90.6% of the included SCEDs had fewer than 50 data points. It is possible that randomized AB phase designs with fewer than 60 measurement occasions may also have sufficient power in specific conditions we simulated, but we cannot verify this on the basis of the present results. As we previously mentioned, we do not recommend implementing randomized AB phase designs with more than 60 measurement occasions, since the extra practical burden this entails does not outweigh the very small increase in power it yields.
Although we advocate the use of randomization in SCEDs, readers should note that some authors oppose to this practice, as well as the use of RTs, because it conflicts with response-guided experimentation (Joo, Ferron, Beretvas, Moeyaert, & Van den Noortgate, 2017 ; Kazdin, 1980 ). According to this approach, decisions to implement, withdraw, or alter treatments are often based on the observed data patterns during the course of the experiment (e.g., starting the treatment only after the baseline phase has stabilized). Response-guided experimentation conflicts with the use of RTs, because RTs require prespecifying the start of the treatment in a random fashion. In response to this criticism, Edgington ( 1980 ) proposed an RT in which only part of the measurement occasions of the SCE are randomized, thus giving the researcher control over the nonrandomized part.
Some additional remarks concerning the present simulation study are in order. First, although this simulation study showed that the randomized AB phase design has relatively low power, we should mention that multiple randomized AB phase designs can be combined in a multiple-baseline, across-participant design that increases the power of the RT considerably (Onghena & Edgington, 2005 ). More specifically, a simulation study has shown that under most conditions, the power to detect a standardized treatment effect of 1.5 for designs with four participants and a total of 20 measurement occasions per participant is already 80% or more (Ferron & Sentovich, 2002 ). A more recent simulation study by Levin, Ferron, and Gafurov ( 2018 ) investigating several different randomization test procedures for multiple-baseline designs showed similar results. Another option to obtain phase designs with more statistical power would be to extend the basic AB phase design to an ABA or ABAB design. Onghena ( 1992 ) has developed an appropriate randomization test for such extended phase designs.
Second, it is important to realize that the MD and ITEI analyses used in this simulation study quantify two different aspects of the difference between the phases. The MD aims to quantify overall level differences between the A phase and the B phase, whereas the ITEI aims to quantify the immediate treatment effect after the implementation of the treatment. The fact that the power of the RT in randomized AB phase designs is generally higher for the ITEI than for the MD indicates that the randomized AB phase design is mostly sensitive to immediate changes in the dependent variable after the treatment has started. Kratochwill et al. ( 2010 ) argued that immediate treatment effects are more reliable indicators of a functional relation between the outcome variable and the treatment than are gradual or delayed treatment effects. In this sense, the use of a randomized AB phase design is appropriate to detect such immediate treatment effects.
Third, in this article we assumed a research situation in which a researcher is interested in analyzing immediate treatment effects and differences in mean level, but in which unexpected linear trends in the data hamper such analyses. In this context it is important to mention that over the years multiple proposals have been made concerning how to deal with the presence of trends in the statistical analysis of single-case data. These proposals include RTs for predicted trends (Edgington, 1975b ), calculating measures of ES that control for trend (e.g., the percentage of data points exceeding the baseline median; Ma, 2006 ), calculating ESs that incorporate the trend into the treatment effect itself (e.g., Tau-U; Parker, Vannest, Davis, & Sauber, 2011 ), and quantifying trend separately from a mean level shift effect, which is an approach adopted by most regression-based techniques (e.g., Allison & Gorman, 1993 ; Van den Noortgate & Onghena, 2003 ), and also by slope and level change (SLC; Solanas et al., 2010 ), which is a nonparametric technique to isolate the trend from the mean level shift effect in SCEDs. The possibilities to deal with trends in single-case data are numerous and beyond of the scope of the present article.
The present study has a few limitations that we will now mention. First of all, the results and conclusions of this simulation study are obviously limited to the simulation conditions that were included. Because we simulated a large number of data patterns, we had to compromise on the number of levels of some simulation factors in order to keep the simulation study computationally manageable. For example, we only used three different treatment effect sizes (in absolute value) and four different numbers of measurement occasions. Moreover, the incremental differences between the different values of these factors were quite large. Second, this simulation study only considered the 15 previously mentioned data patterns generated from the Huitema–McKean model, featuring constant and immediate treatment effects and linear trends. We did not simulate data patterns with delayed or gradual treatment effects or nonlinear trends. An interesting avenue for future research would be to extend the present simulation study to delayed and/or gradual treatment effects and nonlinear trends. Third, in this simulation study we only investigated randomized AB phase designs. Future simulation studies could investigate the effect of unexpected trends in more complex phase designs, such as ABA and ABAB designs or multiple-baseline designs. Fourth, we only used test statistics designed to evaluate two aspects of single-case data: level differences and the immediacy of the effect. Although these are important indicators of treatment effectiveness, other aspects of the data might provide additional information regarding treatment efficacy. More specifically, data aspects such as variability, nonoverlap, and consistency of the treatment effect must also be evaluated in order to achieve a fuller understanding of the data (Kratochwill et al., 2010 ). In this light, more research needs to be done evaluating the power of the RT using test statistics designed to quantify trend, variability, and consistency across phases. Future research could focus on devising an RT test battery consisting of multiple RTs with different test statistics, each aimed at quantifying a different aspect of the data at hand. In such a scenario, the Type I error rate across multiple RTs could be controlled at the nominal level using multiple testing corrections. A final limitation of this simulation study is that the data were generated using a random-sampling model with the assumption of normally distributed errors. It is also possible to evaluate the power of the RT in a random assignment model (cf. conditional power; Keller, 2012 ; Michiels et al., 2018 ). Future research could investigate whether the results of the present simulation study would still hold in a conditional power framework.
The AB phase design has been commonly dismissed as inadequate for research purposes because it allegedly cannot control for maturation and history effects. However this blanket dismissal of AB phase designs fails to discern between randomized and nonrandomized versions of the design. The present article has demonstrated that the randomized AB phase design is a potentially internally valid experimental design that can be used for assessing the effect of a treatment in a single participant when the treatment is irreversible or cannot be withdrawn due to ethical reasons. We showed that randomized AB phase designs can be analyzed with randomization tests to assess the statistical significance of the mean level changes and immediate changes in the outcome variable by using appropriate test statistics for each type of effect. The results of a simulation study showed that the power with which mean level changes and immediate changes can be evaluated depends on the specific type of data pattern that is analyzed. We concluded that for nearly every data pattern in this simulation study that included an upward A phase trend, a positive treatment effect, and/or a downward or upward B phase trend, it was possible to detect immediate treatment effects with sufficient power using the RT. In any case, randomized AB phase designs should contain more than 30 measurement occasions to provide adequate power in the RT. Researchers should be aware that the randomized AB phase design generally has low power, even for large sample sizes. For this reason, we recommend that researchers use single-case phase designs with more power (such as randomized multiple-baseline designs or a serially replicated randomized AB phase design) whenever possible, as they have a higher statistical-conclusion validity. When an AB phase design is the only feasible option, researchers should consider the benefits of randomly determining the intervention point. It is far better to perform the randomized AB phase design, which can provide tentative information about a treatment effect, than not to perform an SCED study at all.
Allison, D. B., & Gorman, B. S. (1993). Calculating effect sizes for meta-analysis: The case of the single case. Behaviour Research and Therapy , 31 , 621–631.
PubMed Google Scholar
Alnahdi, G. H. (2015). Single-subject design in special education: Advantages and limitations. Journal of Research in Special Educational Needs , 15 , 257–265.
Google Scholar
Barlow, D. H., & Hayes, S. C. (1979). Alternating treatments design: One strategy foßr comparing the effects of two treatments in a single subject. Journal of Applied Behavior Analysis , 12 , 199–210.
PubMed PubMed Central Google Scholar
Barlow, D. H., Nock, M. K., & Hersen, M. (2009). Single case experimental designs: Strategies for studying behavior change (3rd ed.). Boston, MA: Pearson.
Bobrovitz, C. D., & Ottenbacher, K. J. (1998). Comparison of visual inspection and statistical analysis of single-subject data in rehabilitation research. American Journal of Physical Medicine and Rehabilitation 77 , 94–102.
Borckardt, J. J., & Nash, M. R. (2014). Simulation modelling analysis for small sets of single-subject data collected over time. Neuropsychological Rehabilitation , 24 , 492–506.
Bulté, I., & Onghena, P. (2008). An R package for single-case randomization tests. Behavior Research Methods , 40 , 467–478. https://doi.org/10.3758/BRM.40.2.467
Article PubMed Google Scholar
Busk, P. L., & Serlin, R. C. (1992). Meta-analysis for single-case research. In T. R. Kratochwill, J. R. Levin (Eds.), Single-case research design and analysis: New directions for psychology and education (pp. 187–212). Hillsdale, NJ: Erlbaum.
Campbell, D. T. (1969). Reforms as experiments. American Psychologist , 24 , 409–429. https://doi.org/10.1037/h0027982
Article Google Scholar
Campbell, D. T., & Stanley, J. C. (1966). Experimental and quasi- experimental designs for research. Boston, MA: Houghton Mifflin.
Chambless, D. L., & Ollendick, T. H. (2001). Empirically supported psychological interventions: Controversies and evidence. Annual Review of Psychology , 52 , 685–716.
Cohen, J. (1988). Statistical power analysis for the behavioral sciences (2nd ed.). Hillsdale, NJ: Erlbaum.
Cook, T. D., & Campbell, D. T. (1979). Quasi-experimentation: Design and analysis issues for field settings. Chicago, IL: Rand McNally.
de Vries, R. M., & Morey, R. D. (2013). Bayesian hypothesis testing for single-subject designs. Psychological Methods , 18 , 165–185. https://doi.org/10.1037/a0031037
du Prel, J., Hommel, G., Röhrig, B., & Blettner, M. (2009). Confidence interval or p -value? Deutsches Ärzteblatt International , 106 , 335–339.
Dugard, P. (2014). Randomization tests: A new gold standard? Journal of Contextual Behavioral Science , 3 , 65–68.
Dugard, P., File, P., & Todman, J. (2012). Single-case and small-n experimental designs: A practical guide to randomization tests (2nd ed.). New York, NY: Routledge.
Edgington, E. S. (1967). Statistical inference from N = 1 experiments. Journal of Psychology , 65 , 195–199.
Edgington, E. S. (1975a). Randomization tests for one-subject operant experiments. Journal of Psychology , 90 , 57–68.
Edgington, E. S. (1975b). Randomization tests for predicted trends. Canadian Psychological Review , 16 , 49–53.
Edgington, E. S. (1980). Overcoming obstacles to single-subject experimentation. Journal of Educational Statistics , 5 , 261–267.
Edgington, E. S. (1996). Randomized single-subject experimental designs. Behaviour Research and Therapy , 34 , 567–574.
Edgington, E. S., & Onghena, P. (2007). Randomization tests (4th ed.). Boca Raton, FL: Chapman & Hall/CRC.
Ferron, J., & Foster-Johnson, L. (1998). Analyzing single-case data with visually guided randomization tests. Behavior Research Methods, Instruments, & Computers , 30 , 698–706. https://doi.org/10.3758/BF03209489
Ferron, J., & Onghena, P. (1996). The power of randomization tests for single-case phase designs. Journal of Experimental Education , 64 , 231–239.
Ferron, J., & Sentovich, C. (2002). Statistical power of randomization tests used with multiple-baseline designs. Journal of Experimental Education , 70 , 165–178.
Ferron, J., & Ware, W. (1995). Analyzing single-case data: The power of randomization tests. Journal of Experimental Education , 63 , 167–178.
Gabler, N. B., Duan, N., Vohra, S., & Kravitz, R. L. (2011). N -of-1 trials in the medical literature: A systematic review. Medical Care , 49 , 761–768.
Gast, D.L., & Ledford, J.R. (2014). Single case research methodology: Applications in special education and behavioral sciences (2nd ed.).New York, NY: Routledge.
Gottman, J. M., & Glass, G. V. (1978). Analysis of interrupted time-series experiments. In T. R. Kratochwill (Ed.), Single-subject research: Strategies for evaluating change (pp. 197–237). New York, NY: Academic Press.
Hammond, D., & Gast, D. L. (2010). Descriptive analysis of single-subject research designs: 1983–2007. Education and Training in Autism and Developmental Disabilities , 45 , 187–202.
Harrington, M., & Velicer, W. F. (2015). Comparing visual and statistical analysis in single-case studies using published studies. Multivariate Behavioral Research , 50 , 162–183.
Harris, F. N., & Jenson, W. R. (1985). Comparisons of multiple- baseline across persons designs and AB designs with replications: Issues and confusions. Behavioral Assessment , 7 , 121–127.
Harvey, M. T., May, M. E., & Kennedy, C. H. (2004). Nonconcurrent multiple baseline designs and the evaluation of educational systems. Journal of Behavioral Education , 13 , 267–276.
Hedges, L. V., Pustejovsky, J. E., & Shadish, W. R. (2012). A standardized mean difference effect size for single case designs. Research Synthesis Methods , 3 , 324–239.
Heyvaert, M., Moeyaert, M.,Verkempynck, P., Van den Noortgate, W., Vervloet, M., Ugille M., & Onghena, P. (2017). Testing the intervention effect in single-case experiments: A Monte Carlo simulation study. Journal of Experimental Education , 85 , 175–196.
Heyvaert, M., & Onghena, P. (2014). Analysis of single-case data: Randomisation tests for measures of effect size. Neuropsychological Rehabilitation , 24 , 507–527.
Heyvaert, M., Wendt, O., Van den Noortgate, W., & Onghena, P. (2015). Randomization and data-analysis items in quality standards for single-case experimental studies. Journal of Special Education , 49 , 146–156.
Horner, R. H., Swaminathan, H., Sugai, G., & Smolkowski, K. (2012). Considerations for the systematic analysis and use of single-case research. Education & Treatment of Children , 35 , 269–290.
Huitema, B. E., & McKean, J. W. (2000). Design specification issues in time- series intervention models. Educational and Psychological Measurement , 60 , 38–58.
Joo, S.-H., Ferron, J. M., Beretvas, S. N., Moeyaert, M., & Van den Noortgate, W. (2017). The impact of response-guided baseline phase extensions on treatment effect estimates. Research in Developmental Disabilities . https://doi.org/10.1016/j.ridd.2017.12.018
Kazdin, A. E. (1980). Obstacles in using randomization tests in single-case experimentation. Journal of Educational Statistics , 5 , 253–260.
Kazdin, A. E. (2011). Single-case research designs: Methods for clinical and applied settings (2nd ed.). New York, NY: Oxford University Press.
Keller, B. (2012). Detecting treatment effects with small samples: The power of some tests under the randomization model. Psychometrika , 2 , 324–338.
Kratochwill, T. R., Hitchcock, J., Horner, R. H., Levin, J. R., Odom, S. L., Rindskopf, D. M., & Shadish, W. R. (2010). Single-case designs technical documentation. Retrieved from the What Works Clearinghouse website: http://ies.ed.gov/ncee/wwc/pdf/wwc_scd.pdf .
Kratochwill, T. R., & Levin, J. R. (2010). Enhancing the scientific credibility of single-case intervention research: Randomization to the rescue. Psychological Methods , 15 , 124–144. https://doi.org/10.1037/a0017736
Kratochwill, T. R., & Stoiber, K. C. (2000). Empirically supported interventions and school psychology: Conceptual and practical issues: Part II. School Psychology Quarterly , 15 , 233–253.
Leong, H. M., Carter, M., & Stephenson, J. (2015). Systematic review of sensory integration therapy for individuals with disabilities: Single case design studies. Research in Developmental Disabilities , 47 , 334–351.
Levin, J. R., Ferron, J. M., & Gafurov, B. S. (2014). Improved randomization tests for a class of single-case intervention designs. Journal of Modern Applied Statistical Methods , 13 , 2–52.
Levin, J. R., Ferron, J. M., & Gafurov, B. S. (2017). Additional comparisons of randomization-test procedures for single-case multiple-baseline designs: Alternative effect types. Journal of School Psychology , 63 , 13–34.
Levin, J. R., Ferron, J. M., & Gafurov, B. S. (2018). Comparison of randomization-test procedures for single-case multiple-baseline designs. Developmental Neurorehabilitation , 21 , 290–311. https://doi.org/10.1080/17518423.2016.1197708
Levin, J. R., Ferron, J. M., & Kratochwill, T. R. (2012). Nonparametric statistical tests for single-case systematic and randomized ABAB … AB and alternating treatment intervention designs: New developments, new directions. Journal of School Psychology , 50 , 599–624.
Logan, L. R., Hickman, R. R., Harris, S. R., & Heriza, C. B. (2008). Single-subject research design: Recommendations for levels of evidence and quality rating. Developmental Medicine and Child Neurology , 50 , 99–103.
Ma, H. H. (2006). An alternative method for quantitative synthesis of single-subject research: Percentage of data points exceeding the median. Behavior Modification , 30 , 598–617.
Manolov, R., & Onghena, P. (2017). Analyzing data from single-case alternating treatments designs. Psychological Methods . Advance online publication. https://doi.org/10.1037/met0000133
Mansell, J. (1982). Repeated direct replication of AB designs. Journal of Behavior Therapy and Experimental Psychiatry , 13 , 261–262.
Michiels, B., Heyvaert, M., Meulders, A., & Onghena, P. (2017). Confidence intervals for single-case effect size measures based on randomization test inversion. Behavior Research Methods , 49 , 363–381. https://doi.org/10.3758/s13428-016-0714-4
Michiels, B., Heyvaert, M., & Onghena, P. (2018). The conditional power of randomization tests for single-case effect sizes in designs with randomized treatment order: A Monte Carlo simulation study. Behavior Research Methods , 50 , 557–575. https://doi.org/10.3758/s13428-017-0885-7
Michiels, B., & Onghena, P. (2018). Nonparametric meta-analysis for single-case research: Confidence intervals for combined effect sizes. Behavior Research Methods . https://doi.org/10.3758/s13428-018-1044-5
Onghena, P. (1992). Randomization tests for extensions and variations of ABAB single-case experimental designs: A rejoinder. Behavioral Assessment , 14 , 153–171.
Onghena, P. (2005). Single-case designs. In B. Everitt & D. Howell (Eds.), Encyclopedia of statistics in behavioral science (Vol. 4, pp. 1850–1854). Chichester, UK: Wiley.
Onghena, P., & Edgington, E. S. (1994). Randomization tests for restricted alternating treatments designs. Behaviour Research and Therapy , 32 , 783–786.
Onghena, P., & Edgington, E. S. (2005). Customization of pain treatments: Single-case design and analysis. Clinical Journal of Pain , 21 , 56–68.
Onghena, P., Vlaeyen, J. W. S., & de Jong, J. (2007). Randomized replicated single-case experiments: Treatment of pain-related fear by graded exposure in vivo. In S. Sawilowsky (Ed.), Real data analysis (pp. 387–396). Charlotte, NC: Information Age.
Parker, R. I., Vannest, K. J., & Davis, J. L. (2011). Effect size in single-case research: a review of nine nonoverlap techniques. Behavior Modification , 35 , 303–322.
Parker, R. I., Vannest, K. J., Davis, J. L., & Sauber, S. B. (2011). Combining nonoverlap and trend for single-case research: Tau-U. Behavior Therapy , 42 , 284–299.
Rindskopf, D. (2014). Nonlinear Bayesian analysis for single case designs. Journal of School Psychology , 52 , 179–189.
Rindskopf, D., Shadish, W. R., & Hedges, L. V. (2012). A simple effect size estimator for single-case designs using WinBUGS. Washington DC: Society for Research on Educational Effectiveness.
Rvachew, S., & Matthews, T. (2017). Demonstrating treatment efficacy using the single subject randomization design: A tutorial and demonstration. Journal of Communication Disorders , 67 , 1–13.
Shadish, W. R., Cook, T. D., & Campbell, D. T. (2002). Experimental and quasi-experimental designs for generalized causal inference. New York, NY: Houghton Mifflin.
Shadish, W. R., Rindskopf, D. M., & Hedges, L. V. (2008). The state of the science in the meta-analysis of single-case experimental designs . Evidence-Based Communication Assessment and Intervention , 2 , 188–196.
Shadish, W. R., & Sullivan, K. J. (2011). Characteristics of single-case designs used to assess intervention effects in 2008. Behavior Research Methods , 43 , 971–980. https://doi.org/10.3758/s13428-011-0111-y
Shadish, W. R., Zuur, A. F., & Sullivan, K. J. (2014). Using generalized additive (mixed) models to analyze single case designs. Journal of School Psychology , 52 , 149–178.
Shamseer, L., Sampson, M., Bukutu, C., Schmid, C. H., Nikles, J., Tate, R., … the CENT Group. (2015). CONSORT extension for reporting N-of-1 trials (CENT) 2015: Explanation and elaboration. British Medical Journal, 350, h1793.
Smith, J. D. (2012). Single-case experimental designs: A systematic review of published research and current standards. Psychological Methods , 17 , 510–550. https://doi.org/10.1037/a0029312
Solanas, A., Manolov, R., & Onghena, P. (2010). Estimating slope and level change in N = 1 designs. Behavior Modification , 34 , 195–218.
Solomon, B. G. (2014). Violations of assumptions in school-based single-case data: Implications for the selection and interpretation of effect sizes. Behavior Modification , 38 , 477–496.
Swaminathan, H., & Rogers, H. J. (2007). Statistical reform in school psychology research: A synthesis. Psychology in the Schools , 44 , 543–549.
Swaminathan, H., Rogers, H. J., & Horner, R. H. (2014). An effect size measure and Bayesian analysis of single-case designs. Journal of School Psychology , 52 , 213–230.
Tate, R. L., Perdices, M., Rosenkoetter, U., Shadish, W., Vohra, S., Barlow, D. H., … Wilson, B. (2016). The Single-Case Reporting guideline In Behavioural interventions (SCRIBE) 2016 statement. Aphasiology, 30, 862–876.
Van den Noortgate, W., & Onghena, P. (2003). Hierarchical linear models for the quantitative integration of effect sizes in single-case research. Behavior Research Methods, Instruments, & Computers , 35 , 1–10. https://doi.org/10.3758/BF03195492
Vohra, S., Shamseer, L., Sampson, M., Bukutu, C., Schmid, C. H., Tate, R., … the CENT Group. (2015). CONSORT extension for reporting N-of-1 trials (CENT) 2015 Statement. British Medical Journal, 350, h1738.
Watson, P. J., & Workman, E. A. (1981). The non-concurrent multiple baseline across-individuals design: An extension of the traditional multiple baseline design. Journal of Behavior Therapy and Experimental Psychiatry , 12 , 257–259.
Ximenes, V. M., Manolov, R., Solanas, A., & Quera, V. (2009). Factors affecting visual inference in single-case designs. Spanish Journal of Psychology , 12 , 823–832.
Download references
Author note
This research was funded by the Research Foundation–Flanders (FWO), Belgium (Grant ID: G.0593.14). The authors assure that all research presented in this article is fully original and has not been presented or made available elsewhere in any form.
Author information
Authors and affiliations.
Faculty of Psychology and Educational Sciences, KU Leuven–University of Leuven, Leuven, Belgium
Bart Michiels & Patrick Onghena
Methodology of Educational Sciences Research Group, Tiensestraat 102, Box 3762, B-3000, Leuven, Belgium
Bart Michiels
You can also search for this author in PubMed Google Scholar
Corresponding author
Correspondence to Bart Michiels .
Electronic supplementary material
Appendix: descriptive results (means and standard deviations) of the main effects in the simulation study, rights and permissions.
Reprints and permissions
About this article
Michiels, B., Onghena, P. Randomized single-case AB phase designs: Prospects and pitfalls. Behav Res 51 , 2454–2476 (2019). https://doi.org/10.3758/s13428-018-1084-x
Download citation
Published : 18 July 2018
Issue Date : December 2019
DOI : https://doi.org/10.3758/s13428-018-1084-x
Share this article
Anyone you share the following link with will be able to read this content:
Sorry, a shareable link is not currently available for this article.
Provided by the Springer Nature SharedIt content-sharing initiative
- Single-case experimental design
- Interrupted time series design
- Linear trend
- Randomization test
- Power analysis
- Find a journal
- Publish with us
- Track your research
3 Dimensions of a Single-case Study Design
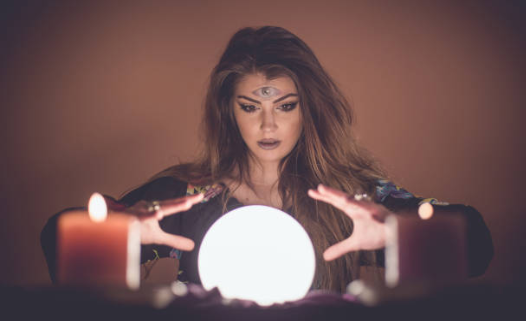
Prediction, verification and replication.
Prediction involves anticipating what you think will happen in the future. Verification is showing that dependent variables (DVs) would not change without intervention (independent variables: IVs). Replication involves taking away the intervention, reintroducing it, and obtaining similar outcomes.
Level, trend and variability have to do with the visual analysis of graphed data.
Studies across participants, settings or behaviors are ways to set up multiple baseline designs.
Stimulus, response and consequence make up the “three-term contingency.”
Leave a Reply
Leave a reply cancel reply.
Your email address will not be published. Required fields are marked *
Save my name, email, and website in this browser for the next time I comment.
Start Services:
Mailing address:.

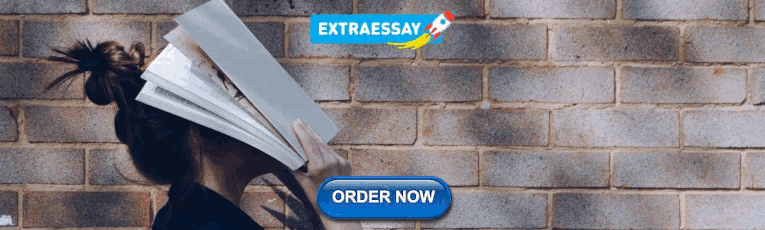
IMAGES
VIDEO
COMMENTS
The purpose of this article is to describe single-case studies, and contrast them with case studies and randomized clinical trials. We will highlight current research designs, analysis techniques, and quality appraisal tools relevant for single-case rehabilitation research. ... A recent study used an ABA reversal SC study to determine the ...
Many of these features are illustrated in Figure 10.2, which shows the results of a generic single-subject study. First, the dependent variable (represented on the y -axis of the graph) is measured repeatedly over time (represented by the x -axis) at regular intervals. Second, the study is divided into distinct phases, and the participant is ...
When choosing a single-subject experimental design, ABA researchers are looking for certain characteristics that fit their study. First, individuals serve as their own control in single subject research. In other words, the results of each condition are compared to the participant's own data. If 3 people participate in the study, each will ...
This systematic review evaluates single-case research design studies investigating applied behavior analytic (ABA) interventions for people with Down syndrome (DS). One hundred twenty-five studies examining the efficacy of ABA interventions on increasing skills and/or decreasing challenging behaviors met inclusion criteria.
Given that the unit of analysis is each case (i.e., participant), a single study could be conceptualized as a series of single-case experiments. Perhaps a better label for these designs would be "intrasubject replication designs" . Second, SCDs are not limited to interventions that produce large, immediate changes in behavior.
The purpose of this article is to describe single-case studies, and contrast them with case studies and randomized clinical trials Lobo, M. A., Moeyaert, M., Baraldi Cunha, A., & Babik, I. (2017). Single-case design, analysis, and quality assessment for intervention research.
To search for SSRDs using keywords or phrases, follow the steps here, consulting the sample screen shots. (Look below for the reason why this works.) First, go to APA PsycInfo. Second, copy and paste this set of terms describing different types of SSRDs into an APA PsycInfo search box, and choose "Abstract" in the drop-down menu.
We used multi-level meta-analysis to synthesize the results of 137 single-case design studies on SIB treatment for 245 individuals with autism and/or intellectual disabilities. Analyses compare the effects of various behavioral and medical treatments for SIB and assess associations between treatment effects and participant- and study-level ...
Abstract. This systematic review evaluates single-case research design studies investigating applied behavior analytic (ABA) interventions for people with Down syndrome (DS). One hundred twenty-five studies examining the efficacy of ABA interventions on increasing skills and/or decreasing challenging behaviors met inclusion criteria. The What Works Clearinghouse standards and Risk of Bias in N ...
Single-case Experimental Designs in Clinical Settings. W.C. Follette, in International Encyclopedia of the Social & Behavioral Sciences, 2001 2 Characteristics of Single-case Design. Single-case designs study intensively the process of change by taking many measures on the same individual subject over a period of time. The degree of control in single-case design experiments can often lead to ...
Tactics of Scientific Research (Sidman, 1960) provides a visionary treatise on single-case designs, their scientific underpinnings, and their critical role in understanding behavior. Since the foundational base was provided, single-case designs have proliferated especially in areas of application where they have been used to evaluate interventions with an extraordinary range of clients ...
The Institute for Education Science (IES) has recognized the contribution single case designs can make toward identifying effective practices and has recently established standards for evaluating the quality of single case design studies (Institute of Educational Sciences, n.d.; Kratochwill et al. 2013).
Types of Single Case Designs Rather than one single design model, SCD is a class of multiple designs. Although the basic SCD has many variations, there are two classes that warrant further description regarding their applicability to home visiting studies: (1) withdrawal/reversal designs and (2) multiple baseline designs.
A special issue of Perspectives on Behavior Science focused on methodological advances needed for single-case research is a timely contribution to the field. There are growing efforts to both articulate professional standards for single-case methods (Kratochwill et al., 2010; Tate et al., 2016), and advance new procedures for analysis and interpretation of single-case studies (Manolov ...
A defining feature of single-case studies is that each condition remains in effect for extended periods of time (e.g., from several days to several weeks) to allow sufficient data to be collected from which judgments will be made. ... Treatment integrity in applied behavior analysis with children. Journal of Applied Behavior Analysis, 262, 257 ...
Many studies demonstrating the outcomes obtained with ABA-based procedures use single-case experimental designs (also termed "single-subject designs"; Kazdin, 2010 & 2013) because this type of design is ideal for examining how the behavior of an individual changes as a function of changes in the environment - which is the subject of ...
Reversal Designs. The most basic single-subject research design is the reversal design, also called the ABA design. During the first phase, A, a baseline is established for the dependent variable. This is the level of responding before any treatment is introduced, and therefore the baseline phase is a kind of control condition.
Single-case design studies in music therapy: resurrecting experimental evidence in small group and individual music therapy clinical settings. J Music Ther, 51 (2014), pp. 293-309. CrossRef View in Scopus Google Scholar ... Applied behavior analysis in acquired brain injury rehabilitation: a meta-analysis of single-case design intervention ...
Abstract. Single-case experimental designs (SCEDs) represent a family of research designs that use experimental methods to study the effects of treatments on outcomes. The fundamental unit of analysis is the single case—which can be an individual, clinic, or community—ideally with replications of effects within and/or between cases.
The clinician in practice is apt to select the AB 1 B 2, changing criterion design. The single case approach provides a means of measuring the increased amount of an intervention. For example, in Kazdin, 2 increased expected levels of quiz performance are used across math objectives 1, 2, 3 and 4 as measured during daily school sessions. 3, 5.
Single-case experimental designs (SCEDs) are increasingly used in fields such as clinical psychology and educational psychology for the evaluation of treatments and interventions in individual participants. The AB phase design, also known as the interrupted time series design, is one of the most basic SCEDs used in practice. Randomization can be included in this design by randomly determining ...
3 Dimensions of a Single-case Study Design. Prediction, verification and replication. Prediction involves anticipating what you think will happen in the future. Verification is showing that dependent variables (DVs) would not change without intervention (independent variables: IVs). Replication involves taking away the intervention ...
Using variations on the single-subject experimental designs as described previously, researchers can flexibly complete efficacy or effectiveness studies, dismantling studies, parametric studies, or any other evaluations that can be addressed using large-sample designs—and can do so within or between subjects (Nock et al., 2007).