- Reference Manager
- Simple TEXT file
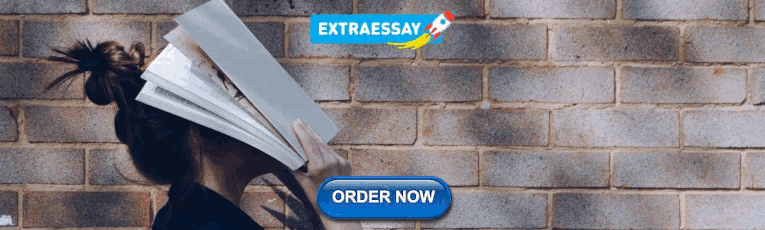
People also looked at
Original research article, what are task-sets: a single, integrated representation or a collection of multiple control representations.
- 1 Department Psychologie, Allgemeine und Experimentelle Psychologie I, Ludwig-Maximilians-Universität München, München, Germany
- 2 Birkbeck College, University of London, London, UK
Performing two randomly alternating tasks typically results in higher reaction times (RTs) following a task switch, relative to a task repetition. These task switch costs (TSC) reflect processes of switching between control settings for different tasks. The present study investigated whether task sets operate as a single, integrated representation or as an agglomeration of relatively independent components. In a cued task switch paradigm, target detection (present/absent) and discrimination (blue/green/right-/left-tilted) tasks alternated randomly across trials. The target was either a color or an orientation singleton among homogeneous distractors. Across two trials, the task and target-defining dimension repeated or changed randomly. For task switch trials, agglomerated task sets predict a difference between dimension changes and repetitions: joint task and dimension switches require full task set reconfiguration, while dimension repetitions permit re-using some control settings from the previous trial. By contrast, integrated task sets always require full switches, predicting dimension repetition effects (DREs) to be absent across task switches. RT analyses showed significant DREs across task switches as well as repetitions supporting the notion of agglomerated task sets. Additionally, two event-related potentials (ERP) were analyzed: the Posterior-Contralateral-Negativity (PCN) indexing spatial selection dynamics, and the Sustained-Posterior-Contralateral-Negativity (SPCN) indexing post-selective perceptual/semantic analysis. Significant DREs across task switches were observed for both the PCN and SPCN components. Together, DREs across task switches for RTs and two functionally distinct ERP components suggest that re-using control settings across different tasks is possible. The results thus support the “agglomerated-task-set” hypothesis, and are inconsistent with “integrated task sets.”
Introduction
Surviving in an environment in which both internal and external conditions change dynamically presupposes an ability to change between control settings for old and new tasks. A successful switch implies that the set of expectations about the environment (the topic of the present special issue) which was relevant in the previous task episode is replaced by one appropriate for the task at hand. Such switching processes are usually investigated in paradigms in which two or more different tasks vary across trials, requiring a change, on task-switch trials, in the internal control settings so as to fit the current task requirements. In cued task switching , prior to the stimulus display's onset, a cue is presented specifying the task to be performed on the upcoming trial. Across two consecutive trials, the task can either repeat or change. Reaction times (RTs) and errors are typically elevated for task switches relative to repetitions ( Allport et al., 1994 ; Rogers and Monsell, 1995 ). Such task switch costs (TSCs) imply the existence of extra, time-consuming processes invoked on task switch trials, but not (or to a lesser degree) on repetition trials. To comprehensively account for TSCs, answers to two related, yet separable questions are necessary: first, what cognitive mechanisms give rise to the TSCs, and, second, how are the representations on which these mechanisms operate organized? The present study focused on the latter issue—more precisely, on whether or not having to change some expectations automatically triggers a change in all expectations about task-relevant properties of the environment.
Determinants of TSCs
The available literature offers two dominant approaches to the question of what cognitive mechanisms give rise to TSCs. According to the first, TSCs reflect the extra time it takes to reconfigure control settings from the previously relevant to the currently relevant task demands ( Monsell and Driver, 2000 ; Monsell et al., 2003 ). Reconfiguration is achieved by means of a special executive function (or set of functions) which is active on task switches and inactive on task repetitions. An alternative approach assumes that TSCs reflect interference between the previously relevant and the currently required control settings, which are concurrently active on task switch trials ( Allport et al., 1994 ; Gilbert and Shallice, 2002 ; Waszak et al., 2003 ). TSCs arise because the interference is weaker on task repetition than on task switch trials. Finally, a hybrid, reconfiguration-interference account has also been proposed, postulating that TSCs reflect a mixture of reconfiguration and interference processes ( Meiran, 1996 , 2000 , but see Meiran et al., 2008 ). Critically, irrespectively of what mechanisms produce TSCs, all accounts assume that performance of a task is controlled by a set of representations that, following a task switch, are no longer appropriate. Thus, to meet the changed environmental demands, the control representations would have to change, too. Given this, the present study was designed to address the question of what representations change across task switches.
Conceptually, tasks can differ in all or some of the following respects: (i) criteria for spatial-attentional selection of the task-relevant stimulus; (ii) criteria for the identification of task-relevant stimulus properties; and (iii) task-appropriate stimulus-response (S-R) mappings. The set of cognitive representations specifying these criteria is considered collectively to constitute the task set. The available literature supports the notion of composite task set representations. For instance, Meiran (2000) demonstrated that while switching identification criteria can be performed in advance, actually performing the task is necessary for switching S-R mappings. Furthermore, Hübner et al. (2001) showed that the magnitude of TSCs increases with the number of task set components to be switched. Finally, a number of electrophysiological and imaging studies revealed neural correlates of a switch to co-vary with what task set component is being switched ( Rushworth et al., 2002 , 2005 ; Ravizza and Carter, 2008 ; Chiu and Yantis, 2009 ; Esterman et al., 2009 ; Hakun and Ravizza, 2012 ). In summary, the available evidence converges on the view that task sets consist of several dissociable representations controlling different cognitive processes in the stimulus-response chain. However, it remains unclear whether, on task switch trials, different components are changed relatively independently of each other, as would be predicted by the notion of “agglomerated task sets.”
Studies investigating whether or not it is possible to change only those task set components that require a change and to reuse shared components across different tasks yielded somewhat inconsistent findings. For example, Arrington et al. (2003) asked their participants to report, on different trials, either the stimulus height, width, color, or luminance. Smaller TSCs were found for switches across similar tasks (e.g., switching from color to luminance discrimination) relative to switches across dissimilar tasks (e.g., from height to luminance discrimination), suggesting that some reusing of control representations across different tasks is possible. By contrast, Vandierendonck et al. (2008) had their participants discriminate either the parity (odd/even) or the magnitude (greater/smaller than four) of stimuli consisting of several identical digits (e.g., five instances of the digit three). On different trials, either the number of digits or the digits themselves were task-relevant. The critical comparison was between trials on which both the identification criterion (parity vs. magnitude) and the stimulus attribute (number vs. digit) switched, and trials on which only one criterion switched (e.g., from digit parity to magnitude discrimination). Although partial switches in principle allowed for old control representations to be reused, no difference was observed between full and partial switches—indicating that, following a task switch, all task-set components are reset. Finally, in a study very similar to Kleinsorge (2004) ; Vandierendonck et al. (2008) (see also Kleinsorge and Heuer, 1999 ) observed partial repetition costs , that is, partial switches took actually more time to be implemented than full switches. Kleinsorge explained these findings by assuming a hierarchical organization of task sets, according to which having to change task set components situated earlier in the stimulus-response chain (e.g., identification criteria) would trigger a switch in all subsequent criteria (e.g., S-R mapping rules). In summary, the available literature suggests that following a task-switch, task-sets are sometime reset, sometimes switched, and sometimes reused. It should be borne in mind, however, that the various studies used (i) different paradigms and (ii) different types of switches. These differences will be discussed in more detail in the General Discussion.
Resetting, Switching or Reusing Task-Set Components
Depending on what tasks precisely vary across trials, task switching could necessitate changes of either all components (full switches) or only those components in which the two tasks differ (partial switches). To illustrate, consider a paradigm in which stimulus displays consist of many identical items with one of them (the singleton target) being different in either color or orientation from the rest. Participants are to, on different trials, either simply detect the presence vs. absence of the singleton target or discriminate its exact features, with a cue, presented prior to the display onset, announcing what task (detection or discrimination) is to be performed. Independently of the task sequence, the task-relevant dimension can also repeat or change. The dimension in which the singleton target is defined, although not informative about the response to be performed (i.e., knowing the dimension would not specify the exact response!), would be informative for both spatial-attentional selection and post-selective identification processes.
Concerning target selection, the available evidence suggests that when the dimension repeats across trials, spatial selection is speeded relative to dimension changes, as elaborated in the Dimension-Weighting Account of Müller and colleagues (e.g., Found and Müller, 1996 ; Müller and Krummenacher, 2006 ; Müller, 2010 ). Since both singleton detection and singleton discrimination tasks would require spatial selection, spatial selection processes for both tasks would be sensitive to the singleton dimension. Accordingly, dimension repetition effects (DREs) would be expected across (task repetition and switch) trials of both detection and discrimination tasks.
In contrast to the shared spatial selection, post-selective identification processes should differ between the two tasks. On the one hand, fast and accurate singleton detection can be achieved by simply determining the presence/absence of a singleton, while information about what the singleton features precisely are is not strictly necessary for performing the task. Consistent with this, there is evidence that the response-irrelevant singleton features are not processed up to the level at which they become available for explicit report ( Müller et al., 2004 ). On the other hand, encoding singleton features from a particular dimension is critical for the singleton discrimination task. With these differential task requirements in mind, it is likely that post-selective identification processes differ between tasks: the singleton dimension is important for identification in the discrimination, but not in the detection task (see Töllner et al., 2012b , for supporting EEG data).
Differences between detection and discrimination tasks would determine what can be and is reused across trials. Following performance of a target-present detection task, the dimension should have been encoded and thus be available for reuse only for spatial selection. Following a discrimination task, the dimension should have been encoded in and thus be available for reuse for both the spatial selection and post-selective identification processes. Thus, the task on trial n − 1, or prime trial, determines what is available for reusing. By contrast, the task on trial n , or probe trial, determines what is reused: in the detection task, reusing dimension information would facilitate just spatial selection, while in discrimination task reusing dimension information would facilitate both selection and identification processes. Consequently, on the hypothesis of agglomerated task sets, comparable DREs would be expected for detection → detection and detection → discrimination sequences, because across these sequences, only spatial selection criteria are available and reused. By contrast, following performance of the discrimination task, both spatial selection and identification criteria would be available for reuse, but they would be reused only on the current discrimination trial, predicting stronger DREs for discrimination → discrimination relative to discrimination → detection sequences.
In contrast to the notion of agglomerated task sets, that of integrated task sets would predict that following any switch, the task set would be reset; accordingly, there should not be a difference between full and partial switches. Finally, the notion of hierarchical task sets would predict a reversal of DREs, that is: switching from, e.g., detection to discrimination, would also switch the expected dimension, resulting in worse performance following dimension repetitions relative to dimension switches.
ERP Components Sensitive to the Spatial Selection and Post-Selective Identification Components
In the paradigm described above, preparatory adjustments with regard to the task-relevant (singleton) dimension are not possible since the task cue is not dimension-specific 1 . Consequently, the analyses of partial-switch effects for spatial selection and post-selective identification in the EEG domain have focused on areas and ERP components sensitive to the implementation of these processes.
As an index of spatial-attentional selection, parameters of the Posterior-Contralateral-Negativity (PCN, or N2-posterior-contralateral 2 ) have been analyzed. This component manifests as an increased negativity at posterior scalp electrode sites contralateral to the singleton position, relative to ipsilateral electrode sites, in the 175–300-ms time range post-stimulus. PCN parameters are considered to reflect the dynamics of spatial selection processes ( Luck and Hillyard, 1994 ; Eimer, 1996 ; Töllner et al., 2011 ). Importantly, PCN latencies are shorter for dimension repetitions relative to changes ( Töllner et al., 2008 ). On the assumption of agglomerated task sets, substantial DREs are expected for both task repetitions and switches. By contrast, the assumption of integrated tasks sets would predict no DRE across task switches.
The second component of interest was the Sustained Posterior Contralateral Negativity (SPCN), manifesting as an increased negativity over posterior electrodes contralateral to the target, relative to ipsilateral electrodes, starting from 350–400-ms post-stimulus. The SPCN component is considered to be sensitive to the processing demands following stimulus selection ( Jolicoeur et al., 2008 ). Importantly, the SPCN is weaker ( Mazza et al., 2007 ; Töllner et al., 2013 ) in tasks that do not necessarily require perceptual analysis following stimulus selection (e.g., singleton detection task), and more prominent (ibid.) in tasks that require stimulus analysis up to the feature levels (e.g., in singleton discrimination task). Thus, the available evidence predicts a stronger SPCN for the discrimination task, which requires explicit feature discrimination, relative to the detection task, in which post-selective processing is not necessary for an accurate response. Furthermore, the hypothesis of agglomerated task sets predicts stronger DREs in the SPCN time range on trials preceded by (a trial with) the discrimination task, in which post-selective identification should be sensitive to the dimensional identity of the singleton, relative to trials preceded by the detection task, for which it is sufficient to determine that the selected item is a singleton, without necessarily identifying its precise dimension or feature properties.
Participants
Sixteen males (mean age 29 years, 2 left-handed), all with normal color vision and normal or corrected-to-normal visual acuity, participated in the experiment for monetary compensation. All participants had extensive experience with psychophysical tasks and all were naïve as to the purpose of the experiment. Due to excessive eye blinking, two participants were excluded from the analyses.
Stimuli were presented on a 17″ CRT monitor with a 1024 × 768 pixels resolution and an 85-Hz refresh rate. Custom written C ++ code controlled stimulus presentation and recorded responses. The experiment was conducted in a dimly lit, acoustically and electromagnetically shielded room. Head-to-monitor distance was 60 cm.
Stimuli and Procedure
Stimulus displays (Figure 1 ) were presented on a gray background (19 cd/m 2 , CIE x = 0.292, y = 0.307) and consisted of 38 vertical yellow (0.388, 0.520) bars arranged around three concentric—inner, middle, and outer—circles with 8, 12, and 18 items, respectively. Single bars subtended 0.4 × 1.9° of visual angle, and the diameters of the three circles were 5, 10, and 15°, respectively. On target-present trials, one of the bars on the middle circle (excluding the two positions along the vertical meridian) was replaced by either a blue (0.275, 0.541) or a green (0.211, 0.263) color singleton target or a right-titled (12° clockwise from vertical) or left-tilted (12° counter-clockwise) orientation singleton target, matched in luminance to the distractor bars (68 cd/m 2 ).
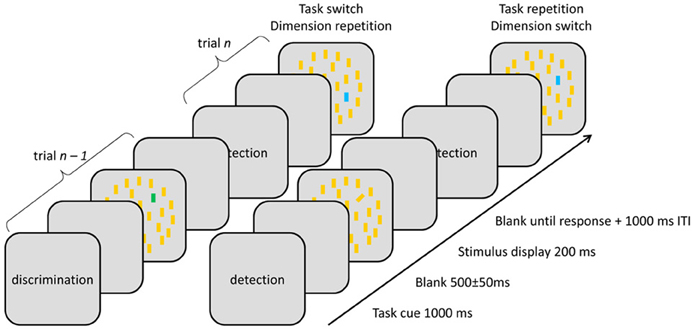
Figure 1. Illustration of stimulus displays across different inter-trial sequences .
Every trial started with a task cue (i.e., the word “detection” or “discrimination”) shown for 1000 ms, followed by a 500 ± 50-ms blank screen. Next, the stimulus display appeared for 200 ms, followed by a blank screen until response. In case of response errors, the standard intertrial interval (1000 ms) was doubled. Responses were given via pressing the left and right mouse keys using the left- and right-hand thumbs, respectively. Stimulus displays were identical for both tasks, the difference being that, in the detection task, participants were required to discern the presence (on 60% of detection task trials) vs. the absence of a singleton target by pressing the corresponding response key with two possible S-R mappings: (i) target-present → R 1 , -absent → R 2 and, respectively, (ii) target-absent → R 1 , -present → R 2 . In the discrimination task, a singleton was always present, with participants having to report the feature that distinguished it from distractors (blue, green, left-, and right tilted). Same-dimension singletons (e.g., blue and green) required different responses (e.g., R 1 and R 2 ), while singletons from different dimensions (e.g., blue and left-tilted) were mapped to a same response, resulting in four possible S-R mappings: (i) blue or left-tilted → R 1 , green or right-tilted → R 2 , (ii) green or left-tilted → R 1 , blue or right-tilted → R 2 , (iii) blue or right-tilted → R 1 , green or left-tilted → R 2 , and (iv) green or right-tilted → R 1 , blue or left-tilted → R 2 . The two possible S-R mappings in the detection task and the four S-R mappings in the discrimination task yielded eight different S-R mapping combinations, which were counterbalanced across participants.
The task (detection vs. discrimination) and the target's dimension (color vs. orientation) were randomly selected on every trial, resulting in four task sequences (detection on both prime and probe trials; discrimination on both trials; detection on prime, discrimination on probe trial; and discrimination on prime, detection on probe trial) and two dimension sequences (repetition/change) across consecutive trials. Relevant dimensions were sampled with equal probability, however, in order to ensure comparable numbers of target-present trials across the two tasks, the detection task was made more frequent (3:2 ratio).
Prior to experiment proper, participants received 1–4 practice blocks (80 trials per block); practice was terminated once a participant achieved ≤ 10% errors per block. All participants met the criterion after 2–4 blocks. Following practice, participants completed 1920 trials (in ca. 3 h), split in two equal-length sessions with a 15–30 min break in between.
EEG Recording and Analyses
The EEG was sampled at 1 KHz using Ag/AgCl active electrodes (actiCAP system; Brain Products, Munich) from 64 scalp sites, arranged according to the 10–10 System (American Electroencephalographic Society, 1994), and amplified using BrainAmp amplifiers (BrainProducts, Munich) with a 0.1–250-Hz bandpass filter. Impedances were kept below 5 kΩ. Electrodes were online referenced to FCz and re-referenced offline to averaged mastoids. Electrodes placed at the outer canthi of the eyes and the superior and inferior orbits monitored blinks and eye movements. Non-stereotyped noise was removed by visual inspection, followed by high-pass filtering using a Butterworth infinite impulse response filter at 0.5 Hz (24 dB/Oct). An infomax independent-component analysis was run to identify and remove effects of eye movements and blinks. Next, continuous EEG was epoched into −200–600 ms segments time-locked to stimulus display onset. Baseline correction was performed based on the −200–0 ms pre-stimulus interval. Target-absent trials, trials preceded by a target-absent trial, error response trials, as well as trials preceded by an error were excluded from the analyses. Finally, trials with (i) signals exceeding ±60 μV, (ii) bursts of electromyographic activity (permitted maximal voltage steps/sampling point of 50 μV), or (iii) activity lower than 0.5 μV within intervals of 500 ms (indicating dead channels) were removed from further analyses on an individual channel basis. The remaining trials (mean = 111 trials per participant per condition, SD = 5 trials) were sorted according to experimental conditions, and averaged on an individual-channel basis.
Data Analyses
Analysis of EEG signals focused on two event-related potentials (ERPs). The ERP components were quantified by subtracting ERPs measured at lateral parieto-occipital electrodes (PO7/PO8) ipsilateral to the target's location from contralateral ERPs. The PCN peak latencies and amplitudes were defined per participant as the maximum negative-going deflection in the time period 170–270 ms post-stimulus. SPCN amplitudes were defined as the average of the time window the 430–510-ms post-stimulus.
Mean RTs, error rates, PCN peak latencies and amplitudes, and SPCN mean amplitudes were computed for correct target-present probes for which the primes were also correct target-present trials. Dependent measures were submitted to repeated-measures ANOVAs (RANOVAs) in three different analyses. First, overall task differences and TSCs were assessed in a RANOVA with main terms for (i) task on probe trial (detection vs. discrimination) and (ii) task sequence (repetition vs. change) across prime and probe trials. The second analysis focused on indices of re-using processes across trials, that is, on dimension-repetition effects. Because re-use is expected to co-vary with what is available for re-using, which is determined on prime trials, the second set of analyses used a RANOVA with main terms for (i) task on prime trial, (ii) task on probe trial, and (iii) dimension sequence (repetition vs. change) across trials. Third, effects of response sequence on mean RTs and error rates were analyzed. Because only a small number of trials were available per cell for this analysis, the corresponding ERPs could not be examined.
Analyses of Overall Task Differences
Behavioral results.
Inspection of the overall mean RTs and error rates (shown in Table 1 ) revealed performance to be faster and more accurate for the detection task (mean RTs = 594 ms, mean error rate = 5.2%) than for the discrimination task (670 ms, 6.5%). Furthermore, task repetitions (598 ms, 4.5%) yielded faster and more accurate performance relative to task changes (666 ms, 7.1%), indicating substantial TSCs for both RTs and error rates (TSC RT = 68 ms, TSC errors = 2.6%). Finally, switching from discrimination to detection incurred greater TSCs (81 ms, 3%) than switching from detection to discrimination (57 ms, 2.3%).
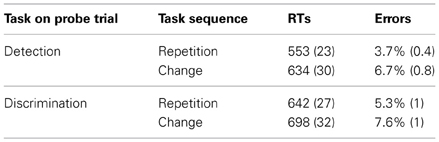
Table 1. Mean RTs (SE M ) and percentage of errors (SE M ) on probe trials for the two different tasks, dependent on the task sequence .
These observations were confirmed by RANOVAs of the mean RTs and error rates with main terms for task on probe trial (detection vs. discrimination) and task sequence (task- repetition vs. -change). The analysis of the mean RTs proved main effects of task, F (1,13) = 36.44, p < 0.01, η 2 p = 0.74, task sequence, F (1,13) = 18.65, p < 0.01, η 2 p = 0.60, as well as their interaction, F (1,13) = 6.18, p < 0.05, η 2 p = 0.32, to be significant. The RANOVA of the mean error rates yielded likewise a significant main effect of task sequence, F (1,13) = 20.94, p < 0.01, η 2 p = 0.62, without, however, any of the other effects reaching significance (all F < 2.17, all p > 0.16).
ERP results
The stimulus-locked ERP waves obtained for the detection and discrimination tasks are shown on Figure 2 . Figure 2A depicts the time course separately for electrodes contra- and ipsilateral to the target position, averaged across target positions 3 (left vs. right), while Figure 2B depicts the difference between contra- and ipsilateral electrodes. As can be seen from Figure 2B , substantial lateralization effects, confirmed by t -tests against zero, were observed in the PCN time range (170–270 ms) for both the detection (−2.17 μV, p < 0.01) and the discrimination task (−2.41, p < 0.01). Similar to what we already reported in Töllner et al. (2012a) , PCN amplitude was higher for the discrimination than for the detection task, with comparable PCN latencies across the two tasks (221 and 222 ms for detection and discrimination, respectively). The RANOVA of the PCN latencies with main terms for (i) task on probe trial and (ii) task sequence yielded no significant effects (all F < 1). The RANOVA of the PCN amplitudes yielded a significant main effect of task, F (1,13) = 6.61, p < 0.05, η 2 p = 0.43; the main effect of task sequence non-significant ( F < 1); the task × task sequence interaction approached significance, F (1,13) = 3.70, p = 0.08, η 2 p = 0.22), owing to the fact that switching task tended to increase the PCN amplitude for the detection task (−2.12 μV and −2.22 μV for detection → detection and discrimination → detection sequences, respectively), while tending to decrease the amplitude for the discrimination task (−2.52 and −2.29 μV for discrimination → discrimination and detection → discrimination sequences, respectively).
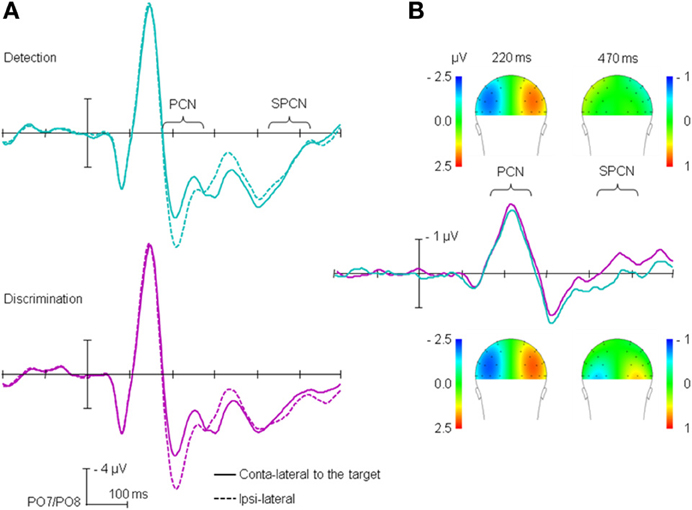
Figure 2. Stimulus-locked ERPs: (A) for the ipsi-and contralateral electrodes relative to the target position, separately for the detection and discrimination tasks; (B) difference between contra- and ipsilateral electrodes. For the purpose of the presentation, data are presented filtered with 30-Hz low-pass filter.
Furthermore, as Figure 2B shows, and as confirmed by t -tests against zero, lateralization effects in SPCN time range (430–510 ms) were observed for the discrimination task (mean amplitude = −0.36 μV, p < 0.01), but not for the detection task (0.14 μV, p = 0.18). A RANOVA of the SPCN mean amplitude with main terms for task and task sequence revealed the main effect of task to be significant, F (1,13) = 72.74, p < 0.01, η 2 p = 0.85, with no other effects reaching significance (all F < 1).
Analyses of Re-Using Control Settings Across Tasks
Figure 3 depicts the mean RT and error rate for a given task on the probe trial (detection, discrimination) dependent on the task on the prime trial (detection, discrimination), separately for dimension repetitions and changes (dimension sequence). As can be seen, RTs were faster for dimension repetitions (blue bars) than for dimension changes (red bars), in all conditions; error rates followed a similar pattern. These observations were confirmed by a RANOVA of the mean RTs, which revealed all three main effects (all F > 6.18, all p < 0.05), all two-way interactions (all F > 18.17, all p < 0.01), and the three-way interaction between task on prime trial, task on probe trial, and dimension sequence, F (1,13) = 21.27, p < 0.01, η 2 p = 0.62, to be significant. An analogous RANOVA of the error rates yielded the following significant effects: main effect of dimension sequence, F (1,13) = 11.39, p < 0.01, η 2 p = 0.47; task on probe trial × dimension sequence interaction, F (1,13) = 33.88, p < 0.05, η 2 p = 0.28; task on prime trial × task on probe trial interaction, F (1,13) = 20.94, p < 0.01, η 2 p = 0.62; and task on prime trial × task on probe trial × dimension sequence interaction, F (1,13) = 8.16, p < 0.5, η 2 p = 0.39.
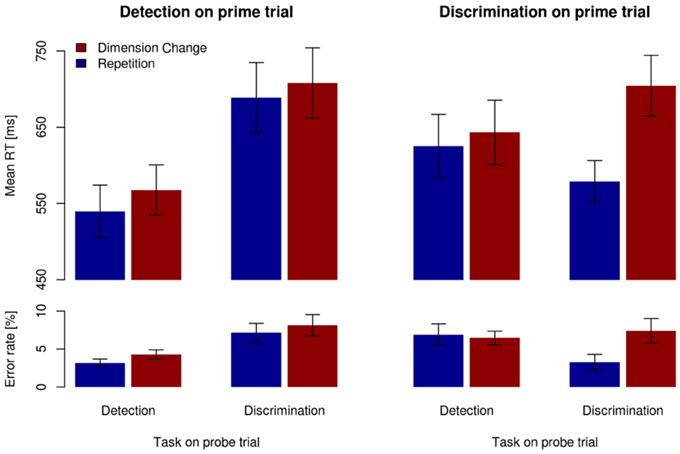
Figure 3. Mean RTs (upper panels) and error rates (lower panels) for the different task sequences, separately for dimension repetitions (blue bars) and changes (red bars). Vertical lines denote ±1SEM.
To further investigate the significant three-way interactions, separate RANOVAs were run dependent on the specific task on prime trials (detection and, respectively, discrimination), with main terms for task on probe trial and dimension sequence. With the detection task on prime trials (left-hand panels in Figure 3 ), the analysis of the (probe-trial) RTs revealed both main effects to be significant: task on probe trial [145-ms difference between discrimination and detection tasks, F (1,13) = 34.04, p < 0.01, η 2 p = 0.72] and dimension sequence [23-ms difference between dimension changes vs. -repetitions, F (1,13) = 22.00, p < 0.01, η 2 p = 0.63]; the interaction between the two was far from significance ( F < 1). The RANOVA of the error rates revealed a significant main effect of task on probe trials [3% difference between discrimination and detection, F (1,13) = 16.68, p < 0.01, η 2 p = 0.56], and a marginally significant main effect of dimension sequence, with dimension repetitions yielding 1.1% more accurate performance than dimension changes, F (1,13) = 4.35, p = 0.06; the interaction between the two was far from significance ( F < 1).
With the discrimination task on prime trials (right-hand panels in Figure 3 ), a RANOVA of the (probe-trial) RTs revealed the main effect of dimension sequence, F (1,13) = 36.32, p < 0.01, η 2 p = 0.81, and the task on probe trial × dimension sequence interaction, F (1,13) = 32.01, p < 0.01, η 2 p = 0.71, to be significant. The interaction was caused by the dimension repetition (vs. change) effect being much larger for discrimination → discrimination sequences (125 ms) than for discrimination → detection sequences (18 ms). For the errors (on probe trials), the main effect of dimension sequence proved significant, F (1,13) = 7.67, p < 0.05, η 2 p = 0.37, with accuracy being 1.4% higher for dimension repetitions relative to changes. The task on probe trial × dimension sequence interaction was also significant, F (1,13) = 8.31, p < 0.5, η 2 p = 0.40, with a stronger DRE for the discrimination relative to the detection task on the probe trial (3.2 vs. −0.3%, respectively).
In summary, significant DREs were observed in all experimental conditions. When the task on the prime trial required simple target detection, DREs were comparable for detection and discrimination tasks on the probe trial. By contrast, with the task on prime trial required target discrimination, stronger DREs were observed for the discrimination task, relative to the detection, to be performed on the probe trial.
Lateralized ERPs are depicted in Figure 4 for the probe trials, separately for the different tasks on prime and on probe trials, as well as across different dimension sequences. As can be seen from Figure 4 , the PCN latency was delayed for dimension changes (red lines) relative to dimension repetitions (blue lines), in all conditions. In the SPCN time range DREs were evident only for (probe) trials following the discrimination task.
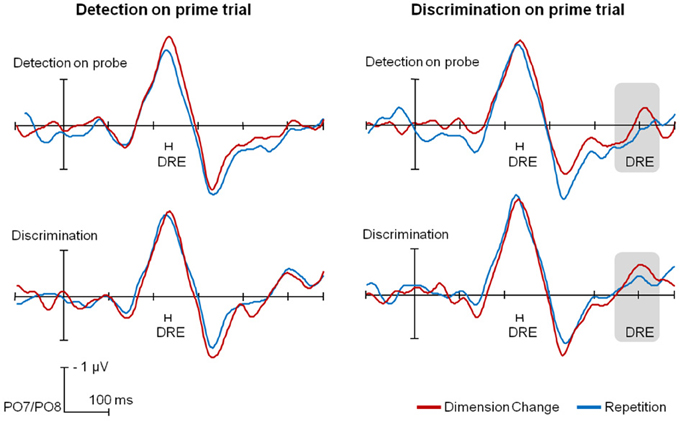
Figure 4. Group-averaged time course of PCN and SPCN components for the different task sequences, separately for dimension repetitions (blue) and changes (red). Significant dimension repetition effects for the peak PCN latency and the mean SPCN amplitude are indicated. For the purpose of presentation, a 30-Hz low-pass filter was applied; data analyses were performed over individual, unfiltered data.
PCN analyses
For (probe) trials preceded by the detection task (left-hand side of Figure 4 ), a RANOVA of the PCN peak latencies revealed only a significant main effect of dimension sequence, with dimension repetitions being 17 ms faster than dimension changes, F (1,13) = 15.41, p < 0.01, η 2 p = 0.54; no other effects reached significance (all F < 1). An analogous RANOVA for trials preceded by the discrimination task (right-hand side) also yielded only a significant main effect of dimension sequence [14-ms DRE, F (1,13) = 5.09, p < 0.05, η 2 p = 0.23], with no other effects reaching significance (all F < 1).
SPCN analyses
With the task on the prime trial requiring target detection, analysis of the (probe-trial) mean SPCN amplitudes revealed only a significant main effect of task on probe trial, with an overall stronger SPCN component for the discrimination relative to the detection task (−0.37 vs. 0.18 μV), F (1,13) = 25.70, p < 0.01, η 2 p = 0.66; no other effects reached significance (all F < 1.94, all p > 0.18). With the discrimination task on the prime trial, an analogous analysis also yielded a significant main effect of task on probe trial, F (1,13) = 30.16, p < 0.01, η 2 p = 0.70, with the significant SPCN for the discrimination relative to the insignificant SPCN for the detection task (−0.36 vs. 0.10 μ V). Importantly, the main effect of dimension sequence was also significant, F (1,13) = 5.33, p < 0.05, η 2 p = 0.29, with the SPCN amplitude being stronger for dimension changes relative to dimension repetitions (−0.23 vs. −0.02 μ V); the interaction task on probe trial x dimension sequence was far from significance ( F < 1).
In summary, analyses of the ERPs revealed longer PCN peak latencies for dimension changes vs. repetitions independently of the task on prime or probe trial; SPCN mean amplitudes were overall larger for the discrimination than for the detection task on probe trial, while significant DREs in the SPCN time interval were observed only for trials preceded by the discrimination task.
Analyses of Response-Sequence Effects
As can be seen from Table 2 , mean RTs were overall fastest for full repetitions (same task, dimension, and response), intermediate for partial repetitions, and slowest for full changes. By contrast, the errors varied as a function of task sequence. Importantly, though, there was no evidence that partial repetitions resulted in less accurate performance relative to full changes.
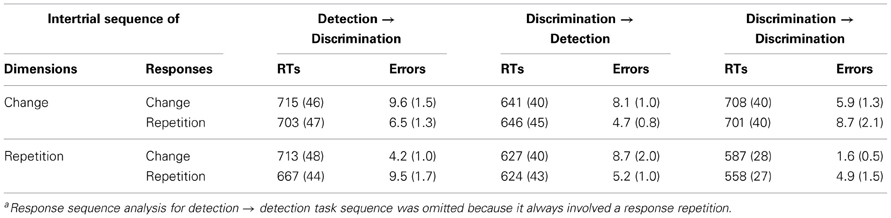
Table 2. Mean RTs (SE M ) and percentage of errors (SE M ) across different task-, dimension- and response a sequences .
These observations were confirmed by three-ways RANOVAs (task- × dimension- × response sequence) of the mean RTs and error rates with a focus on the main effect of and interactions involving response sequence. The RT analysis showed the main effect of response sequence to be significant, F (1,13) = 6.41, p < 0.05, η 2 p = 0.33, as well as the interaction of this factor with dimension sequence, F (1,13) = 9.04, p < 0.01, η 2 p = 0.41, owing to a larger response repetition benefit when the dimension repeated (30 ms, p < 0.01) rather than changed (5 ms, p = 0.49). The task sequence × response sequence interaction was marginally significant, F (2,26) = 1.67, p = 0.07, η 2 p = 0.18, suggestive of a larger response repetition benefit for the discrimination task (29 ms, p < 0.01, and 23 ms, p < 0.01, for detection → discrimination and discrimination → discrimination sequences, respectively) relative to the detection task (−1 ms, p = 0.93). The three-way interaction did not reach significance, F = 1.67, p = 0.27.
Concerning the error analysis, the main effect of response sequence was non-significant ( F < 1). However, response sequence interacted with dimension sequence, F (1,13) = 18.43, p < 0.01, η 2 p = 0.59, and task sequence, F (2,26) = 18.52, p < 0.01, η 2 p = 0.59; and the response- × dimension- × task sequence interaction was significant, F (2,26) = 8.55, p < 0.01, η 2 p = 0.40. Post-hoc analyses revealed the pattern of response sequence effects (repetition benefit vs. cost) to vary across task and dimension sequences. For discrimination → detection task sequences, dimension repetitions were associated with response repetition costs (−5.4%, p < 0.01), whereas dimension changes yielded response repetition benefits (4.1%, p < 0.01). By contrast, discrimination → detection task sequences resulted in response repetition benefits (3.5%, p < 0.01) independently of dimension sequence. Finally, discrimination → discrimination task sequences were associated with response repetition costs (−2.9%, p < 0.05) independently of dimension sequence.
In summary, analyses of response sequence effects on mean RTs showed either response repetition benefits or no effects of response sequence. This finding is at variance with hierarchical task sets, which predict response repetition costs following a task or dimension change. Integrated task sets, which predict no response sequence effects following a task or dimension switch, also account poorly for the present findings. The results for error rates were less consistent across task and dimension sequences, with different patterns of response sequence effects across different experimental conditions.
The present findings demonstrate that, consistent with “agglomerated task sets,” it is possible to reuse control settings across different tasks, and that the reusing is associated with distinct ERP components, depending on precisely which task set component (selection vs. identification criteria) is reused across tasks. In particular, switching tasks was revealed to be easier when the task-relevant dimension repeated relative to when it changed, as indexed by DREs on task switch trials. The notion of agglomerated task sets is fully compatible with existing computational models of cognitive control ( Logan and Gordon, 2001 ; Meiran et al., 2008 ). Both these accounts postulate several independent parameters influencing processes of selection, identification, and, respectively, responding. Importantly, since these parameters are independent, it is plausible that they can also be adjusted independently—which is the core assumption of the agglomerated-task-sets hypothesis. The compatibility with the computational models of cognitive control emphasizes another property of agglomerated task sets: their computational efficiency.
Note though that the DREs reported presently are consistent not only with agglomerated task sets, but also with two, relatively strong alternatives, namely: (i) switching between the detection and discrimination conditions did not involve a task switch, and (ii) the DREs are not dimension-specific, but rather feature- or response-specific. The former alternative would postulate that the two tasks used in the present study were effectively one task, in which participants always performed feature discrimination, but, depending on the cue, selected different responses. The latter alternative would imply that the substantial reusing of spatial selection and identification criteria (as revealed in the dimension-repetition effects) was not indicative of the reuse of task set components in general, but rather of the reuse of stimulus-response associations encountered on the previous trial ( Hommel et al., 2001 ; Dreisbach et al., 2006 , 2007 ).
The present findings argue against the hypothesis that no task switching took place in our paradigm. First, in the present study, substantial differences in mean RTs and errors rates were observed between the detection and discrimination tasks, suggesting that the two conditions were performed differently. Behavioral differences were accompanied by differences in the ERPs: a reliable SPCN component was observed for the discrimination, but not for the detection task. Taken together, these findings suggest that solving the detection and, respectively, discrimination tasks involved the use of different task sets. Second, switching between the two conditions incurred substantial switch costs. Importantly, the switch cost magnitude differed, with stronger costs for discrimination → detection relative to detection → discrimination sequences. The asymmetry in the switch costs—with switching to an easier, or dominant, task (presently, detection) being more effortful than switching to a more difficult task (presently, discrimination)—has frequently been reported in task-switching literature ( Allport et al., 1994 ; Wylie and Allport, 2000 ) and interpreted as an index of the interference between two concurrent task sets. On this background, it is likely that switching between the two tasks in the present study indeed involved task switch processes.
The second alternative explanation posits that DREs critically rely on repetitions of full stimulus-response episodes across trials, rather than on more abstract criterion repetitions. Studies investigating the role of S-R repetitions have typically found performance to be very good on full-repetition trials and worse on partial-repetition trials, that is, when either the stimulus or the response changed, while the other property repeated. Somewhat counter-intuitively, performance is typically better on full switches, that is, when both stimulus and response change, compared to partial repetitions ( Hommel, 1998 , 2005 ; Töllner et al., 2008 ; Zehetleitner et al., 2012 ). Applied to the present study, if DREs were actually S-R repetition effects, then changing the dimension and repeating the response (i.e., partial repetitions) should have resulted in worse performance relative to changing both the dimension and the response (full repetitions). However, analyses of response sequence effects showed this not to be the case, arguing that the DREs reported presently are not reducible to S-R repetition effects.
That partial-repetition costs were not prominent in the present study does not necessarily imply that the processes generating these effects were inactive; rather, the paradigm and dependent measures may not have been sensitive to these processes. Previous work investigating electrophysiological correlates of DREs in a single task paradigm ( Töllner et al., 2008 ) revealed partial repetition costs to correlate with ERP markers that were independent of the ERP markers for dimension and, respectively, response sequence effects. Importantly, the markers of partial-repetition costs were not investigated in the present study. Additionally, in a recent study of partial-repetition costs ( Zehetleitner et al., 2012 ), numerical simulations showed that three different sequence-sensitive mechanisms (dimension-, S-R mapping-, and motor-response-specific) combine and can produce any possible RT pattern, that is, with or without manifest partial repetition costs. Given this, until the boundary conditions for partial-repetition costs to arise are fully understood, it remains possible that the present paradigm simply did not meet these conditions.
Direction of Partial-Switch Effects: Reusing, Resetting, or Switching
While the present study, together with several previous investigations ( Arrington et al., 2003 ; Rangelov et al., 2011 , 2012 ), showed that reusing shared task set components across different tasks is possible, findings to the contrary have also been reported. In particular, Vandierendonck et al. (2008) failed to find any partial-switch effects, while Kleinsorge and colleagues (1999 , 2004 ) found partial-switch costs , relative to full switches, rather than benefits as reported presently. How can these disparate findings be reconciled?
A notable difference between the paradigms used in these investigations and the present study is in the stimulus material and the cueing procedure employed. More precisely, the previous studies used stimuli that were ambiguous with regard to both task-relevant stimulus attributes and identification criteria. Thus, cueing of both the relevant stimulus property (e.g., number or digit value in Vandierendonck et al., 2008 ) and identification criteria (parity vs. magnitude) was necessary on every trial (e.g., the string “number odd/even” served as a cue). This procedure produced partial overlap between cue strings on partial switches (e.g., “number odd/even” → “digit odd/even”) and no overlap on full switches (e.g., “number smaller/greater” → “digit odd/even”). As has been shown by several studies ( Logan and Schneider, 2006 ; Schneider and Logan, 2009 ), overlapping cues activate competing task sets, resulting in negative interference on partial-switch trials. As a consequence, any benefits from reusing shared control representations might have been masked by interference effects from overlapping cues, resulting in either insignificant partial-repetition effects or even partial-repetition costs. By contrast, the present study used cues that did not overlap between the different tasks. Furthermore, the task-relevant dimension was unambiguously specified by the stimulus displays themselves, so no dimension cues were necessary. Consequently, in the present experiment, the negative interference effects would have been minimal—and, correspondingly, partial-repetition effects turned out significant.
Electrophysiological Evidence for Separable Control Mechanisms
In contrast to the mixed behavioral findings regarding the direction of partial-switch effects, investigation of the ERP components related to the spatial selection and post-selective processing task components offers more conclusive findings. By assuming relative autonomy of the control systems for the different cognitive processes, “agglomerated task sets” predict the existence of multiple , relatively independent sources of DREs. Consistent with the prediction, EEG analyses revealed multiple ERP components to be sensitive to dimension sequences: dimension changes (relative to repetitions) resulted in longer PCN latencies as well as larger SPCN amplitudes.
Analyses of the PCN latencies showed dimension changes (relative to repetitions) to be associated with longer latencies independently of the task sequence (see also Töllner et al., 2008 , reporting similar findings in a single-task paradigm). This finding can be explained by assuming that both the detection and discrimination tasks required spatial selection processes, governed by a task set component sensitive to dimension sequences. Dimension repetitions would permit reusing control settings from the previous trial, generating DREs for the PCN latency. Importantly, on the agglomerated-task-set hypothesis, reusing settings is possible even across tasks that differ in other task set components—as evidenced by the present finding of DREs across task switches for the PCN timing.
Analyses of the SPCN amplitudes showed, consistent with our predictions, a more pronounced SPCN for discrimination relative to detection task trials. Apparently, the SPCN amplitude increases continuously with increases in demands for post-selective perceptual processing, from no SPCNs in singleton detection (present study) and singleton localization tasks ( Mazza et al., 2007 ) through singleton discrimination tasks (present study) to strong SPCNs in compound-search tasks ( Mazza et al., 2007 ; Töllner et al., 2013 ), in which one target dimension (e.g., color) is selection-relevant, whereas another dimension (e.g., orientation) is response-relevant.
Furthermore, the SPCN amplitude was sensitive to dimension sequence, but only on trials following the discrimination task. This finding is consistent with the conceptual analysis of the task sets for the detection and, respectively, the discrimination task and the functional interpretation of the SPCN component. Conceptual analysis of the detection task suggested that the singleton dimension is not mandatorily encoded in the post-selective identification settings (for the detection task) and it would not be available for reuse on the following trial, predicting no DRE for the SPCN component following detection task trials. By contrast, post-selective identification in the discrimination task necessarily involved determining the singleton dimension, predicting DREs for the SPCN component following trials of the discrimination task, consistent with the results of the present study.
The electrophysiological data are especially informative about the notion of a generalized switching mechanisms [as proposed by Kleinsorge and Heuer (1999) ], according to which one criterion switch enforces a switch in all criteria, rather than simple re-setting. If generalized switching were operating as a default task switch mechanism, then reconfiguring the spatial selection component should have triggered a switch in the post-selective identification component as well. This would predict an obligatory coupling between DREs for the PCN and SPCN parameters—a prediction that is not supported by the present findings. Thus, in summary, the present ERP findings are fully consistent with, and yet independent of the behavioral findings of DREs across task switches, in their support for the notion of agglomerated task sets.
Conclusions
The present study investigated the nature of task-set representations, defined as a set of criteria for spatial selection, post-selective identification, and S-R mapping rules. Two alternatives were considered: task sets as integral representations and task sets as agglomerations of relatively autonomous control settings guiding different processing stages. The key property of the “agglomerated-task-set” hypothesis is that following a task switch, different control instances can be reconfigured independently of each other, permitting reusing some of the control settings across different tasks. By contrast, the hypothesis of “integrated task sets” predicted no partial-switch effects, as changing any task set component would either reset all settings, or trigger a full switch. Consistent with agglomerated task sets, our findings demonstrate substantial partial-switch effects, operationalized as DREs. Most importantly, evidence of DREs over PCN latencies and SPCN amplitudes, two functionally distinct ERP components, further supports the idea of task sets as a collection of autonomous control instances governing processes of spatial selection and perceptual/symbolic analysis, respectively.
Conflict of Interest Statement
The authors declare that the research was conducted in the absence of any commercial or financial relationships that could be construed as a potential conflict of interest.
Acknowledgments
This research was supported by DFG grants RA 2191/1-1 (to Dragan Rangelov) and ZE 887/3-1 (to Michael Zehetleitner and Hermann J. Müller) as well as the German-Israeli Foundation for Scientific Research and Development grant 2011/158 (to Michael Zehetleitner and Hermann J. Müller).
1. ^ Note that most of the previous EEG studies on task switching used paradigms in which preparatory adjustments of the task set component of interest were possible, to some extent. This work typically revealed involvement of pre-frontal areas when preparing for a task switch ( Karayanidis et al., 2003 ; Nicholson et al., 2005 ; Karayanidis et al., 2009 ).
2. ^ As shown by Shedden and Nordgaard (2001 ; Töllner et al., 2012a ), the PCN is independent of both the amplitude and latency of the non-lateralized N2. Thus, to avoid potential confusion associated with the term “N2pc,” we prefer the neutral term “PCN.”
3. ^ Analyses of PCN and SPCN amplitudes revealed a significant target position effect for the PCN component (3.50 and 3.25 μV for left and right targets, respectively, F (1,13) = 4.81, p < 0.05, η 2 p = 0.27), and no difference between left and right targets in the SPCN component ( F = 1.62, p = 0.22). Most importantly, target position did not interact significantly with electrode site (ipsi- vs. contralateral), nor with any other experimental manipulation (all F s < 2.16, all p > 0.16)—indicating that the PCN and SPCN components were not specific for one visual hemifield.
Allport, D. A., Styles, E. A., and Hsieh, S. (1994). “Shifting intentional set: exploring the dynamic control of tasks,” in Attention and Performance , Vol. XV, eds C. Umilta and M. Moscovitch (Hillsdale, NJ: Erlbaum), 421–452.
Arrington, C. M., Altmann, E. M., and Carr, T. H. (2003). Tasks of a feather flock together: similarity effects in task switching. Mem. Cogn . 31, 781–789. doi: 10.3758/BF03196116
Pubmed Abstract | Pubmed Full Text | CrossRef Full Text
Chiu, Y.-C., and Yantis, S. (2009). A domain-independent source of cognitive control for task sets: shifting spatial attention and switching categorization rules. J. Neurosci . 29, 3930–3938. doi: 10.1523/JNEUROSCI.5737-08.2009
Dreisbach, G., Goschke, T., and Haider, H. (2006). Implicit task sets in task switching. J. Exp. Psychol. Learn. Mem. Cogn . 32, 1221–1233. doi: 10.1037/0278-7393.32.6.1221
Dreisbach, G., Goschke, T., and Haider, H. (2007). The role of task rules and stimulusresponse mappings in the task switching paradigm. Psychol. Res. Psychol. Forsch . 71, 383–392. doi: 10.1007/s00426-005-0041-3
Eimer, M. (1996). The N2pc component as an indicator of attentional selectivity. Electroencephalogr. Clin. Neurophysiol . 99, 225–234. doi: 10.1016/0013-4694(96)95711-9
Esterman, M., Chiu, Y.-C., Tamber-Rosenau, B. J., and Yantis, S. (2009). Decoding cognitive control in human parietal cortex. Proc. Natl. Acad. Sci. U.S.A . 106, 17974–17979. doi: 10.1073/pnas.0903593106
Found, A., and Müller, H. J. (1996). Searching for unknown feature targets on more than one dimension: investigating a “dimension-weighting” account. Percept. Psychophys . 58, 88–101. doi: 10.3758/BF03205479
Gilbert, S. J., and Shallice, T. (2002). Task switching: a PDP model. Cognit. Psychol . 44, 297–337. doi: 10.1006/cogp.2001.0770
Hakun, J. G., and Ravizza, S. M. (2012). Cognitive control: preparation of task switching components. Brain Res . 1451, 53–64. doi: 10.1016/j.brainres.2012.02.046
Hommel, B. (1998). Event files: evidence for automatic integration of stimulus-response episodes. Vis. Cogn . 5, 183. doi: 10.1080/713756773
CrossRef Full Text
Hommel, B. (2005). How much attention does an event file need. J. Exp. Psychol. Hum. Percept. Perform . 31, 1067–1082. doi: 10.1037/0096-1523.31.5.1067
Hommel, B., Müsseler, J., Aschersleben, G., and Prinz, W. (2001). The theory of event coding (TEC): a framework for perception and action planning. Behav. Brain Sci . 24, 849–878. doi: 10.1017/S0140525X01000103
Hübner, R., Futterer, T., and Steinhauser, M. (2001). On attentional control as a source of residual shift costs: evidence from two-component task shifts. J. Exp. Psychol. Learn. Mem. Cogn . 27, 640–653. doi: 10.1037/0278-7393.27.3.640
Jolicoeur, P., Brisson, B., and Robitaille, N. (2008). Dissociation of the N2pc and sustained posterior contralateral negativity in a choice response task. Brain Res . 1215, 160–172. doi: 10.1016/j.brainres.2008.03.059
Karayanidis, F., Coltheart, M., Michie, P. T., and Murphy, K. (2003). Electrophysiological correlates of anticipatory and poststimulus components of task switching. Psychophysiology 40, 329–348. doi: 10.1111/1469-8986.00037
Karayanidis, F., Mansfield, E. L., Galloway, K. L., Smith, J. L., Provost, A., and Heathcote, A. (2009). Anticipatory reconfiguration elicited by fully and partially informative cues that validly predict a switch in task. Cogn. Affect. Behav. Neurosci . 9, 202–215. doi: 10.3758/CABN.9.2.202
Kleinsorge, T. (2004). Hierarchical switching with two types of judgment and two stimulus dimensions. Exp. Psychol . 51, 145–149. doi: 10.1027/1618-3169.51.2.145
Kleinsorge, T., and Heuer, H. (1999). Hierarchical switching in a multi-dimensional task space. Psychol. Res . 62, 300–312. doi: 10.1007/s004260050060
Logan, G. D., and Gordon, R. D. (2001). Executive control of visual attention in dual-task situations. Psychol. Rev . 108, 393–434. doi: 10.1037/0033-295X.108.2.393
Logan, G. D., and Schneider, D. W. (2006). Priming or executive control? Associative priming of cue encoding increases “switch costs” in the explicit task-cuing procedure. Mem. Cogn . 34, 1250–1259. doi: 10.3758/BF03193269
Luck, S. J., and Hillyard, S. A. (1994). Spatial filtering during visual search: evidence from human electrophysiology. J. Exp. Psychol. Hum. Percept. Perform . 20, 1000–1014. doi: 10.1037/0096-1523.20.5.1000
Mazza, V., Turatto, M., Umiltà, C., and Eimer, M. (2007). Attentional selection and identification of visual objects are reflected by distinct electrophysiological responses. Exp. Brain Res . 181, 531–536. doi: 10.1007/s00221-007-1002-4
Meiran, N. (1996). Reconfiguration of processing mode prior to task performance. J. Exp. Psychol . 22, 1423–1442. doi: 10.1037/0278-7393.22.6.1423
Meiran, N. (2000). Modeling cognitive control in task-switching. Psychol. Res . 63, 234–249. doi: 10.1007/s004269900004
Meiran, N., Kessler, Y., and Adi-Japha, E. (2008). Control by action representation and input selection (CARIS): a theoretical framework for task switching. Psychol. Res . 72, 473–500. doi: 10.1007/s00426-008-0136-8
Monsell, S., and Driver, J. (2000). Control of Cognitive Processes: Attention and Performance XVIII . Available online at: http://eprints.ucl.ac.uk/18943/ (Accessed February 2, 2011).
Monsell, S., Sumner, P., and Waters, H. (2003). Task-set reconfiguration with predictable and unpredictable task switches. Mem. Cogn . 31, 327–342. doi: 10.3758/BF03194391
Müller, H. J., and Krummenacher, J. (2006). Visual search and selective attention. Vis. Cogn . 14, 389–410. doi: 10.1080/13506280500527676
Müller, H. J., Krummenacher, J., and Heller, D. (2004). Dimension-specific intertrial facilitation in visual search for pop-out targets: evidence for a top-down modulable visual short-term memory effect. Vis. Cogn . 11, 577–602. doi: 10.1080/13506280344000419
Müller, H. J., Töllner, T., Zehetleitner, M., Geyer, T., Rangelov, D., and Krummenacher, J. (2010). Dimension-based attention modulates feed-forward visual processing: a reply to Theeuwes (2010). Acta Psychol . 135, 117–122. doi: 10.1016/j.actpsy.2010.05.004
Nicholson, R., Karayanidis, F., Poboka, D., Heathcote, A., and Michie, P. T. (2005). Electrophysiological correlates of anticipatory task-switching processes. Psychophysiology 42, 540–554. doi: 10.1111/j.1469-8986.2005.00350.x
Rangelov, D., Müller, H. J., and Zehetleitner, M. (2011). Independent dimension-weighting mechanisms for visual selection and stimulus identification. J. Exp. Psychol . 37, 1369–1382. doi: 10.1037/a0024265
Rangelov, D., Müller, H. J., and Zehetleitner, M. (2012). The multiple-weighting-systems hypothesis: theory and empirical support. Attent. Percept. Psychophys . 74, 540–552. doi: 10.3758/s13414-011-0251-0252
Ravizza, S. M., and Carter, C. S. (2008). Shifting set about task switching: behavioral and neural evidence for distinct forms of cognitive flexibility. Neuropsychologia 46, 2924–2935. doi: 10.1016/j.neuropsychologia.2008.06.006
Rogers, R. D., and Monsell, S. (1995). Costs of a predictable switch between simple cognitive tasks. J. Exp. Psychol . 124, 207–231. doi: 10.1037/0096-3445.124.2.207
Rushworth, M. F. S., Passingham, R. E., and Nobre, A. C. (2002). Components of switching intentional set. J. Cogn. Neurosci . 14, 1139–1150. doi: 10.1162/089892902760807159
Rushworth, M. F. S., Passingham, R. E., and Nobre, A. C. (2005). Components of attentional set-switching. Exp. Psychol . 52, 83–98. doi: 10.1027/1618-3169.52.2.83
Schneider, D. W., and Logan, G. D. (2009). Selecting a response in task switching: testing a model of compound cue retrieval. J. Exp. Psychol. Learn. Mem. Cogn . 35, 122–136. doi: 10.1037/a0013744
Shedden, J. M., and Nordgaard, C. L. (2001). ERP time course of perceptual and post-perceptual mechanisms of spatial selection. Brain Res. Cogn. Brain Res . 11, 59–75. doi: 10.1016/S0926-6410(00)00064-1
Töllner, T., Conci, M., Rusch, T., and Müller, H. J. (2013). Selective manipulation of target identification demands in visual search: the role of stimulus contrast in CDA activations. J. Vis . 13:23. doi: 10.1167/13.3.23
Töllner, T., Gramann, K., Müller, H. J., Kiss, M., and Eimer, M. (2008). Electrophysiological markers of visual dimension changes and response changes. J. Exp. Psychol . 34, 531–542. doi: 10.1037/0096-1523.34.3.531
Töllner, T., Müller, H. J., and Zehetleitner, M. (2012a). Top-down dimensional weight set determines the capture of visual attention: evidence from the PCN component. Cereb. Cortex 22, 1554–1563. doi: 10.1093/cercor/bhr231
Töllner, T., Rangelov, D., and Müller, H. J. (2012b). How the speed of motor-response decisions, but not focal-attentional selection, differs as a function of task set and target prevalence. Proc. Natl. Acad. Sci. U.S.A . 109, E1990–E1999. doi: 10.1073/pnas.1206382109
Töllner, T., Zehetleitner, M., Gramann, K., and Müller, H. J. (2011). Stimulus saliency modulates pre-attentive processing speed in human visual cortex. PLoS ONE 6:e16276. doi: 10.1371/journal.pone.0016276
Vandierendonck, A., Christiaens, E., and Liefooghe, B. (2008). On the representation of task information in task switching: evidence from task and dimension switching. Mem. Cogn . 36, 1248–1261. doi: 10.3758/MC.36.7.1248
Waszak, F., Hommel, B., and Allport, A. (2003). Task-switching and long-term priming: role of episodic stimulus-task bindings in task-shift costs. Cogn. Psychol . 46, 361–413. doi: 10.1016/S0010-0285(02)00520-0
Wylie, G. R., and Allport, A. (2000). Task switching and the measurement of “switch costs.” Psychol. Res . 63, 212–233. doi: 10.1007/s004269900003
Zehetleitner, M., Rangelov, D., and Müller, H. J. (2012). Partial repetition costs persist in nonsearch compound tasks: evidence for multiple-weighting-systems hypothesis. Attent. Percept. Psychophys . 74, 879–890. doi: 10.3758/s13414-012-0287-y
Keywords: task switching, task sets, attentional selection, perceptual processing, electroencephalography, executive control
Citation: Rangelov D, Töllner T, Müller HJ and Zehetleitner M (2013) What are task-sets: a single, integrated representation or a collection of multiple control representations? Front. Hum. Neurosci . 7 :524. doi: 10.3389/fnhum.2013.00524
Received: 29 April 2013; Accepted: 13 August 2013; Published online: 03 September 2013.
Reviewed by:
Copyright © 2013 Rangelov, Töllner, Müller and Zehetleitner. This is an open-access article distributed under the terms of the Creative Commons Attribution License (CC BY) . The use, distribution or reproduction in other forums is permitted, provided the original author(s) or licensor are credited and that the original publication in this journal is cited, in accordance with accepted academic practice. No use, distribution or reproduction is permitted which does not comply with these terms.
*Correspondence: Dragan Rangelov, Department Psychologie, Allgemeine und Experimentelle Psychologie I, Ludwig-Maximilians-Universität München, Leopoldstr. 13, DE-80802 München, Germany e-mail: [email protected]
This article is part of the Research Topic
Attention, predictions and expectations, and their violation: attentional control in the human brain
Thank you for visiting nature.com. You are using a browser version with limited support for CSS. To obtain the best experience, we recommend you use a more up to date browser (or turn off compatibility mode in Internet Explorer). In the meantime, to ensure continued support, we are displaying the site without styles and JavaScript.
- View all journals
- My Account Login
- Explore content
- About the journal
- Publish with us
- Sign up for alerts
- Open access
- Published: 21 June 2019
EEG Decoding Reveals the Strength and Temporal Dynamics of Goal-Relevant Representations
- Jason Hubbard 1 ,
- Atsushi Kikumoto 1 &
- Ulrich Mayr ORCID: orcid.org/0000-0002-7512-4556 1
Scientific Reports volume 9 , Article number: 9051 ( 2019 ) Cite this article
5914 Accesses
23 Citations
8 Altmetric
Metrics details
- Cognitive control
An Author Correction to this article was published on 13 May 2020
This article has been updated
Models of action control assume that attentional control settings regulate the processing of lower-level stimulus/response representations. Yet, little is known about how exactly control and sensory/response representations relate to each other to produce goal-directed behavior. Addressing this question requires time-resolved information about the strength of the different, potentially overlapping representations, on a trial-by-trial basis. Using a cued task-switching paradigm, we show that information about relevant representations can be extracted through decoding analyses from the scalp electrophysiological signal (EEG) with high temporal resolution. Peaks in representational strength—indexed through decoding accuracy—proceeded from superficial task cues, to stimulus locations, to features/responses. In addition, attentional-set representations were prominent throughout almost the entire processing cascade. Trial-by-trial analyses provided detailed information about when and to what degree different representations predict performance, with attentional settings emerging as a strong and consistent predictor of within-individual and across-individual variability in performance. Also, the strength of attentional sets was related to target representations early in the post-stimulus period and to feature/response representations at a later period, suggesting control of successive, lower-level representations in a concurrent manner. These results demonstrate a powerful approach towards uncovering different stages of information processing and their relative importance for performance.
Similar content being viewed by others
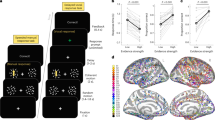
Intracranial electroencephalography reveals effector-independent evidence accumulation dynamics in multiple human brain regions
Sabina Gherman, Noah Markowitz, … Stephan Bickel
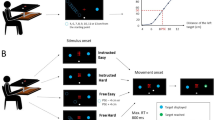
Theta but not beta activity is modulated by freedom of choice during action selection
Emeline Pierrieau, Sarah Kessouri, … Pierre-Michel Bernier
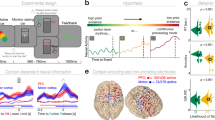
Ramping dynamics and theta oscillations reflect dissociable signatures during rule-guided human behavior
Jan Weber, Anne-Kristin Solbakk, … Randolph F. Helfrich
Introduction
The efficiency of information processing differs both moment to moment, and from one individual to the next. Such variability could reflect the quality of low-level, stimulus or response representations. Alternatively, it may arise from the strength of more abstract, attentional settings that instantiate or control sensory and response-related processes 1 , 2 , 3 . For example, in the experimental paradigm we used in the current work (see Fig. 1A ), participants were informed on each trial through auditory cues, which of two attentional settings to use 4 , 5 . For the Color task, they attended to the color singleton within the array of objects and responded via button press whether the exact color was orange or purple. Similarly, for the Orientation task, participants attended the orientation singleton and responded whether the line tilted to the left or to the right. In this situation, successful performance requires lower-level representations of the task cue, of the target location, and of the task-relevant feature/response. However, it may also require abstract attentional-set representations that differentiate between the color task context and the orientation task context and that ensure an adequate cascade of lower-level representations.
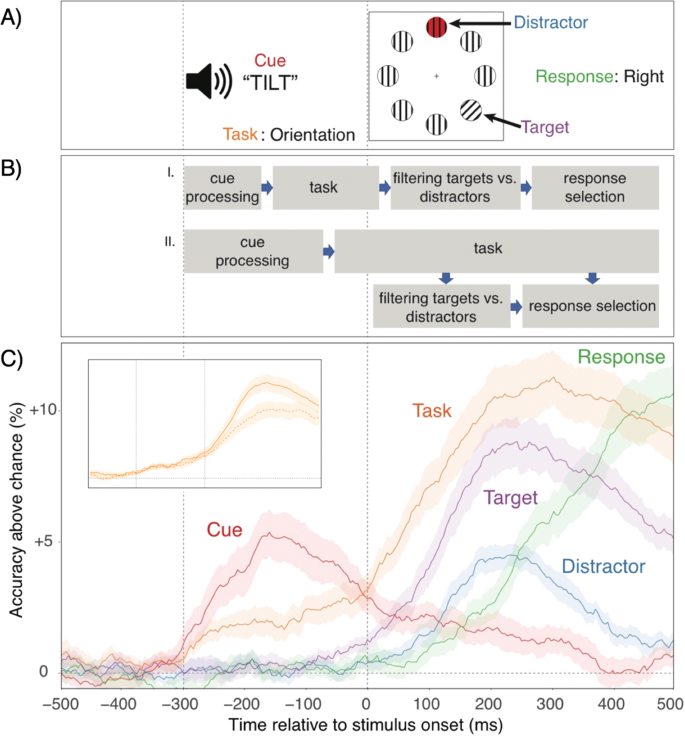
( A ) Stimulus timeline with each relevant task aspect. ( B ) Competing models specifying either a (I) preconfiguration or a (II) parallel-activation relationship between attentional set and stimulus/response representations. ( C ) Decoding accuracy of each aspect across time, relative to chance ( p = 0.5, except for target and distractor, where it was p = 0.25). In all figures, shaded regions specify 95% within-subject confidence intervals. The insert shows how task (i.e., attentional-set) decoding accuracy generalizes both within (filled line) and across target locations/responses (dotted line). Note, that in the current work we are particularly interested in within-individual variability in decoding accuracy and therefore the values presented here are based on averaged, trial-by-trial results. When performing decoding analyses based on averaged data, much higher decoding accuracy (>80% for some aspects) can be achieved.
Even though the existence of higher-level, task or rule representations is a common assumption in models of cognitive control 3 , 6 , 7 , 8 there are open questions about the degree to which such more abstract representations regulate performance, and how such regulation is achieved 4 , 5 , 9 , 10 . For example, as an alternative to the view that abstract, task-level representations are necessary to modulate lower-level processes, some authors have pointed out that when unambiguous, environmental stimuli (i.e., cues) distinguish between competing response options, superficial cue representations could be sufficient to constrain lower-level processes 4 . It is also currently not clear when exactly higher-level control occurs (see Fig. 1B ). Cue or task-set representations might be necessary to set up and preconfigure lower-level representations 11 , 12 . Alternatively, task sets may also become relevant only as competition between lower-level representations arises, in order to mold these representations in a goal-appropriate manner 3 . Addressing these and related issues requires methods that directly probe the status and functional relevance of goal-relevant representations with high temporal resolution.
Existing approaches, such as chronometric analyses of response-time (RT) patterns 13 , the analysis of averaged evoked EEG 14 , or fMRI BOLD signals 15 are of limited value for capturing temporal dynamics, or trial-to-trial variability (but see 16 , in the strength of different task-related representations. A particularly challenging problem is to identify representations that overlap in time, such as when attentional-set and stimulus/response representations occur and influence each other simultaneously. Moreover, because abstract attentional sets are not tied to specific stimuli or responses, they are particularly difficult to pin down with the existing methods.
The limited knowledge we have about the flow of information processing in humans, stands in contrast to advances from primate neurophysiological research. For example, in a recent study that served as a model for the current work, Siegel and colleagues 17 had monkeys perform a task-switching paradigm while multi-unit activity was recorded in critical anatomical areas along the entire sensory-motor processing stream 18 , 19 , 20 . The results suggest that abstract task rules, rather than superficial cue representations, control lower-level processes in a largely parallel manner. Specifically, the neural coding of cue information was very robust during the pre-stimulus phase, but then tampered off in the post-stimulus phase. In contrast, task-level information emerged concurrently with cue information, but then increased dramatically as stimulus and response choice information was processed during the entire response phase.
In humans it is currently not possible to match this type of primate, neurophysiological research in terms of both temporal and neuroanatomical resolution. However, recent work has suggested that a surprising amount of information about currently active representations can be extracted from EEG signals 21 , 22 , 23 , 24 , 25 . This approach is similar to the multi-voxel-pattern-classification method in fMRI research, where the pattern of activations across a set of voxels is used to classify specific task aspects (e.g., face vs. house stimuli). The degree to which such classification is possible, indicates the strength with which that aspect is encoded within the neural signal. Applied to EEG, the spatial pattern of the power in specific frequency bands (or of the raw EEG signal) across electrodes is used to categorize the task aspect in question. Recent work has shown that among other aspects, the focus of spatial attention 23 , the content of spatial working memory 22 , and even of semantic categories 24 can be decoded from the EEG signal. While EEG-based decoding results are limited in their spatial resolution (compared to fMRI), the decoding analyses can be repeated for every time point, thus yielding very high temporal resolution.
We applied the EEG decoding approach to the task-switching paradigm presented in Fig. 1A . Our goal was to track the temporal dynamics of activating the five, potentially relevant aspects: task cues, target and distractor locations, target feature/response, and the attentional/task set. In addition, we conducted our decoding analyses on the single-trial level, which provided information about a particular aspect’s decoding strength for each time point and each trial. This allowed us to examine with high temporal resolution the relative importance of lower-level, stimulus/response, versus higher-level attentional-set representations in predicting trial-by-trial variability in performance.
Participants
Consistent with the sample sizes used in other recent EEG-decoding experiments 22 , 23 , 24 , a total of 22 participants participated in this experiment. One participant was excluded for having EEG artifacts in excess of 30% of trials, and one was excluded due to an experimenter error that resulted in data loss, leaving a sample size of N = 20. Participants were compensated at a rate of $10 per hour, with additional incentives based on performance on the task. All experimental procedures were approved through the University of Oregon’s Human Subject Review Board, and performed in accordance with relevant guidelines/regulations. Informed consent was obtained from all participants.
Tasks and stimuli
We used a cued task switching paradigm that was closely modelled after a paradigm that we had previously used in the context of eye-tracking experiments 12 , 26 . On each trial, an auditory cue indicated which of the tasks, the Color task or the Orientation task, participants had to complete. Each task was paired with two auditory cues: “color” or “hue” for the Color task, and “tilt” or “lean” for the Orientation task. We used two sets of cues (Set A = “color” and “tilt”, Set B = “hue” and “lean”) that were alternated across consecutive trials 27 . Because with this procedure, both task-repeat and task-switch transitions were accompanied by cue transitions, it ensured that task-switch costs are not contaminated by superficial cue-priming effects 4 , 5 and that we could independently decode cue and task information 17 .
The stimulus array consisted of 8 circular gratings (diameter of each ~2.4 degrees) in a larger circular arrangement (diameter ~12.5 degrees). The stimulus array always contained six, neutral stimuli consisting of vertical, black and white gratings. In addition, there was (a) one color singleton stimulus with a vertical grating shaded in one of two colors, either “yellowish” or “reddish” and one (b) orientation singleton with a black grating oriented either 30 degrees to the left or the right. For the Color task, participants had to attend to the color singleton and press the left (z) key for a “yellowish” target and the right (/) key for the “reddish” target. For the Orientation task, participants had to attend to the orientation singleton and press the left (z) key for a left-tilted target and the right (/) key for the right-tilted target.
Each trial began with a 700 ms prestimulus interval with a fixation cross in the center of the screen. The auditory cue was presented in the last 300 ms of this interval, so that the stimulus array appeared as soon as the auditory cue completed. Participants were instructed to respond as quickly and accurately as possible. The stimuli remained on the screen until a response was made. In case of a mistake, an error tone was emitted for 100 ms. During the following inter-trial interval (ITI), which was jittered between 750 and 937 ms, participants were instructed to blink before the next trial began.
The experiment began with two single-task practice blocks (one for each task, order counter-balanced), and a task-switching practice block (20 trials), followed by 22 test blocks of 64 trials each. In order to incentivize participants to respond quickly and accurately, they were rewarded a small amount (0.5 cents) for each trial where they were faster than the 75 th % percentile of their RT distribution up to that point, but only if they maintained at least 90% accuracy for a given block 26 . At the end of each block, subjects were given feedback about their average RT and accuracy for that block. The RT distribution was determined separately for each task and switch condition after the first mixed-task block, and updated with each trial. All task aspects were determined randomly on a trial-by-trial basis. This includes the selection of tasks, yielding an average switch rate of p = 0.5.
Participants were seated approximately 70 cm from the screen, and instructed to keep their eyes at fixation and not blink throughout the trial.
EEG Recording and Preprocessing
Electroencephalographic (EEG) activity was recorded from 20 tin electrodes held in place by an elastic cap (Electrocap International) using the International 10/20 system. The 10/20 sites F3, Fz, F4, T3, C3, CZ, C4, T4, P3, PZ, P4, T5, T6, O1, and O2 were used along with five nonstandard sites: OL midway between T5 and O1; OR midway between T6 and O2; PO3 midway between P3 and OL; PO4 midway between P4 and OR; and POz midway between PO3 and PO4. The left-mastoid was used as reference for all recording sites. Data were re-referenced off-line to the average of all scalp electrodes. Electrodes placed ~1 cm to the left and right of the external canthi of each eye recorded horizontal electrooculogram (EOG) to measure horizontal saccades. To detect blinks, vertical EOG was recorded from an electrode placed beneath the left eye and reference to the left mastoid. The EEG and EOG were amplified with an SA Instrumentation amplifier with a bandpass filter of 0.01–80 Hz and were digitized at 250 Hz in LabView 6.1 running on a PC. Preprocessing was performed using the Signal Processing and EEGLAB 28 toolboxes in MATLAB. Trials including blinks (>80uv, window size = 200 ms, window step = 50 ms), large eye movements (>1°, window size = 200 ms, window step = 10 ms), and blocking of signals (range = −0.0 5uv to 0.05uv, window size = 200 ms) within the interval of −700 to +400 ms relative to the stimulus were rejected and excluded from further analysis, resulting in an average of 180 trials (12.4%) rejected across participants.
After the initial preprocessing, the single-trial EEG data were decomposed into a time-frequency representation via wavelet decomposition. The power spectrum of the EEG signal was obtained through a fast Fourier transform, which was then convolved with the power spectrum of complex Morlet wavelets, defined by ( \({e}^{2\pi ft}{e}^{-t2/(2\ast {\sigma }^{2})}\) ), where t is time, f is frequency, and σ is the width of each frequency band, set according to n / 2πf , with n increasing logarithmically from 3 to 8. This was repeated for the frequency bands between 2 and 31 Hz in logarithmically-spaced steps. The incremental number of wavelet cycles was used to balance between both temporally-based and frequency-based precision 29 . The results were then brought back into the temporal domain using an inverse Fourier transform. A frequency band-specific estimate at each time point was defined as the squared magnitude of the convolved signal Z (real([ z ( t )] 2 + imag[ z ( t )] 2 ) for power. Only power was considered for the present investigation, and for simplicity we focused on frequency bands that are most often presented in the literature: delta (2–3 Hz), theta (4–7 Hz), alpha (8–12 Hz), and beta (13–31 Hz). For each frequency band, we averaged the power signal across the range of interest. Note, that for the decoding analyses we did not differentiate between the four frequency bands.
Decoding analyses
With the decoding analyses, we examined the extent to which the spatial pattern of the EEG power across the scalp was predictive of each task aspect. The aspects we considered were the auditory cue (“color”/“hue” or “tilt”/“lean”, classified within tasks), the task (Color or Orientation), the target position (partitioned into 4 bins, coded 1–4), the distractor position (bins 1–4), and the response (left vs. right).
For the target/distractor location, we decoded positions based on the bin that each item appeared in (e.g., bin 1 = top and top-right position, bin 2 = right and bottom-right position, etc.). This ensured that the target and distractor occupied each combination of bins with equal frequency (including sharing the same bin), thus ensuring that successful distractor decoding is not simply due to the classifier decoding “not target position”.
Note also that in the current paradigm we cannot distinguish between the manual response and the specific target stimulus (e.g., left-tilted grating, or reddish grating) as they were confounded. Also, as we wanted to isolate the discriminability of each aspect regardless of any task differences, we performed the decoding separately within each task (except, of course in decoding the task set itself). The results from these analyses were then averaged. Previous work has established that different types of information are encoded in brain oscillations at particular frequency bands 22 , 30 , 31 , which motivated decomposing the raw EEG signal into the separate bands. However, in the present investigation we were agnostic to which bands encode which type of information, and thus concatenated all 4 bands together in the decoding analysis.
For each trial, we extracted a window centered around stimulus time onset, starting 500 ms before and extending 500 ms after the onset. The end of this interval corresponds to the 70 th percentile of the RT distribution, ensuring that at least 70% of trials are still in progress at that point. We performed the analyses separately for each 4 ms time point. Thus, in the decoding analyses, the features consisted of the estimate of power for each electrode at a single point in time, repeated for each frequency band (20 electrodes × 1 time point × 4 bands = 80 features). Prior to decoding, the EEG data were z-scored so that the mean of each trial’s data was 0 without baseline activity subtraction. We performed all analyses separately for each subject and then averaged results across subjects (e.g. 22 ). We used L2-regularized logistic regression, as implemented in the scikit-learn package in Python 32 , with a tolerance of 1 × 10 –4 and the inverse of the regularization strength (C) set to 1.0. Multi-class classification (which was necessary for target and distractor positions), was implemented as a series of binary classifications. For all decoding analyses, we used a 4-fold cross-validation procedure where 75% of trials were used in the training set, and the remaining 25% of trials were used as the test set, and this was repeated until each trial had an opportunity to be part of the test set. We also repeated the analyses with naïve Bayes, support vector machines, and random forests. The results were consistent across the different algorithms.
For the main decoding analysis (Fig. 1C in manuscript), we reported the decoding accuracy, averaged within subject, timepoint, and factors of interest (e.g., task), and then across subjects. We used decoding accuracy here as it is most consistent with how such results are presented in the literature. In contrast, Figs 2 – 5 are based on classifier confidence, which provide a continuous prediction score on the trial-by-trial level. Specifically, for each item in the test set, the classifier generates a probability (using the predict_proba function in scikit-learn), indicating the classifier’s confidence that the test observation belongs to each class. The class with the highest posterior corresponds to the prediction given by the classifier, and the sum of all probabilities for a single test observation equals 1. Average patterns of results based on classifier confidence are qualitatively very similar to those based on decoding accuracy, but are smoother and with tighter confidence intervals than for average decoding accuracy. Most importantly, the continuous score is better suited for the analyses of trial-to-trial variability as presented in Fig. 2 .
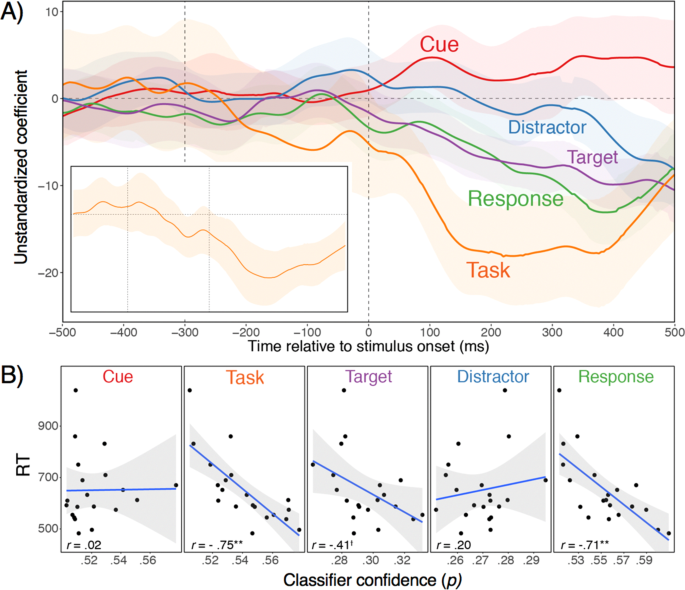
Within and between-individual relationships between decodability of all task aspects and RTs. ( A ) Coefficients from multilevel, linear models with logit-transformed classifier evidence from all task aspects for a given timepoint simultaneously predicting RTs. The insert shows the coefficient when the task-set predictor is based on the generalization scores presented in the insert to Fig. 1C . ( B ) T -values representing simple correlations between individuals’ average, logit-transformed classifier evidence for each task aspect and their average RT. ( C ) Scatterplots of relationships between subject-averaged classifier evidence and RTs during peak, average decoding accuracy periods for each task aspect. For task-level generalization scores (see insert to Fig. 1C ), the correlation remained very robust at 0.63 ( p < 0.01).
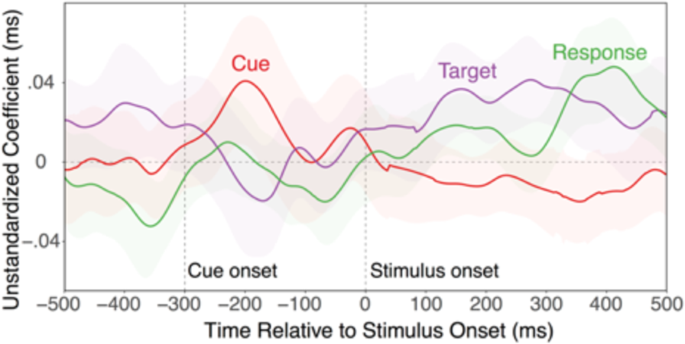
Coefficients representing the independent relationships between classifier confidence for task-level decoding on the one hand, and for cue, target, and responses on the other. For clarity of presentation, the relationship with distractor classifier confidence was omitted here, which hovered around 0 throughout.
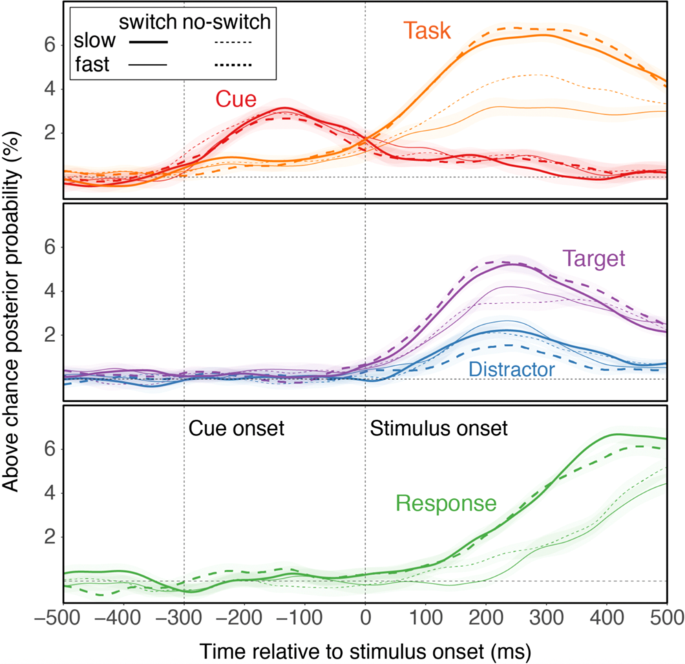
Average classifier confidence, separately for switch vs. no-switch trials and fast vs. slow RTs (determined via median split within individuals, tasks, and switch vs. no-switch trials).
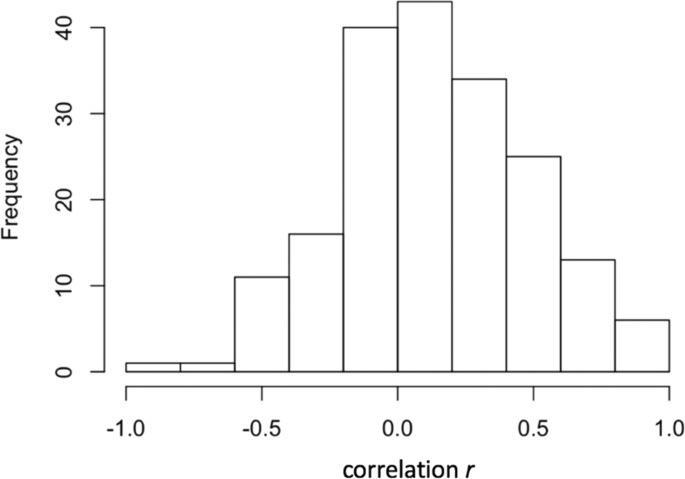
Histogram of correlations of task-contrast t-values across electrodes and frequency bands for all possible pairs of individual subjects (i.e., one correlation per pair).
For some of the subsequent analyses (e.g., Figs 2C and 5 ), we derived decoding scores that reflected the peaks of decoding accuracy for each feature. For these analyses, we identified the average time point with the maximum decoding accuracy for each aspect (see Fig. 1C ) and averaged the (logit-transformed) classifier confidence across a 150 ms window centered around that point. For the response aspect, the maximum decoding accuracy was towards the end of the 500 ms interval. Here, we simply averaged the period from 350–500 ms.
In the Supplemental Information, we provide additional analyses to (a) rule out the effects of eye-movements on task decoding, (b) examine task-specific effects on the encoding of lower-level features, and (c) provide additional information about trial-by-trial predictive relationships between decoding accuracy and behavior.
Table 1 contains mean RTs and error rates as a function of task and switch condition. We submitted RTs to a repeated-measures ANOVA with the factors task and switch. This yielded a modest, but highly significant switch effect, F (1,19) = 40.7, p < 0.001, but no effect of task, F (1,19) = 0.03, p = 0.86, and only a marginal task × switch interaction, F (1,19) = 3.33, p = 0.08. For error rates, we obtained a main effect for task, F (1,19) = 13.27, p = 0.002, with higher error rates for the color than for the orientation task, a main effect for switch, F (1,19) = 14.53, p = 0.001, and also a task × switch interaction, F (1,19) = 10.05, p = 0.005, with somewhat larger switch costs for the color task.
The magnitude of RT switch costs was relatively small. This is likely due to two design factors in our paradigm. First, to allow blinks between trials we used a relatively long response-stimulus interval (RSI) that ranged between 1450 and 1637 ms. Second, different from the more standard switching paradigm, where a central stimulus combines all task-relevant aspects, in the current paradigm target and distractors were spatially separated in order to allow decoding of target and distractor locations as task-related aspects. Given the spatial separation between targets and distractors, participants could use spatial attention to reduce or even eliminate stimulus-induced, between-task interference, which is critical for obtaining large task-switch costs 33 .
At least in terms of errors, the orientation task was somewhat easier than the color task, which may seem surprising given that color information is typically more salient than orientation/shape information. Likely this is due to the fact that the left-versus-right orientation discrimination has a compatible stimulus-response mapping (i.e., right tilt- > right response, left tilt- > left response), whereas the response mapping for the color task is arbitrary.
Representational dynamics
Figure 1C shows that decoding accuracy unfolds in a manner that is consistent with standard expectations about the flow of information––from cue encoding, to attentional-set activation, to relevant and irrelevant stimulus locations, and finally to feature/response codes. Remarkably, task-level (i.e., attentional set) information was decodable with high accuracy throughout almost the entire duration of the trial.
To ensure our conclusions regarding the decoding of the theoretically critical task feature are not compromised by multiple comparisons and faulty comparisons against chance 34 , 35 , we also conducted a cluster-based permutation test. First, we generated a series of t-values via t-tests against the chance level (33.3%) using decoding results with randomly shuffled labels. Then, we identified reliable clusters as neighboring time points that exceeded a primary threshold (cluster-defining threshold, alpha = 0.05) and retained the size of the maximum cluster. We computed a cluster p- value under the permutation distribution of cluster-level statistics (5000 permutations), which defined clusters as significant if their size was larger than the 95th (i.e., alpha = 0.05) largest member in the permutation distribution. According to these analyses, we identified a significant cluster expanding from −260 to 500 ms for the task feature (cluster- defining threshold, p < 0.05, corrected significance level, p < 0.001, critical statistics = 52 ms).
As mentioned in the Introduction, one prominent model suggests that the cognitive system does not actually rely on abstract task settings, but––at least when available-–uses superficial cue representations to resolve ambiguity between competing stimulus/response representations 4 , 9 . However, we found that cue decoding accuracy (i.e., discriminating between the two cues for each task) peaks during the pre-stimulus phase, but declines sharply once the stimulus is presented. This result is consistent with the view that cue representations are used to activate task- or attentional-set representations 5 , 27 and are less involved with actually regulating task-specific processes.
But how exactly are attentional sets instantiated? If such settings are critical for “preconfiguring” lower-level processes, stimulus representations would need to wait until they are firmly established 11 , 12 . Alternatively, task sets may be activated in parallel to low-level stimulus/response selection processes, biasing these in a goal-relevant direction 3 . As shown in Fig. 1C , there was above-chance task decoding during the prestimulus phase, which substantially increased once stimulus/response information became decodable. Thus, while the presence of prestimulus, task-level information indicates some role for preconfiguration, the overall pattern suggests that––consistent with the parallel-activation hypothesis––attentional settings become particularly critical once competition between conflicting stimulus/response representations needs to be resolved (see Fig. 1B ).
Determining the relevance of representations
Going beyond average activation trajectories, the trial-by-trial decoding approach allows determining at what point in the trial, which of the represented aspects drive performance. For this purpose, we entered logit-transformed classifier probabilities for the five aspects as simultaneous predictors into a linear mixed-effects model, predicting trial-by-trial RTs, with a random intercept and random coefficients for each subject using the lme4 package 36 . RTs were pre-whitened by removing any linear or quadratic trends across the blocks of the experiment. The coefficients shown in Fig. 2A represent the unique predictive power associated with each aspect, as a function of time in the trial. Note that negative coefficients imply that the greater the classifier confidence, the faster the RTs—indicating that classifier confidence can be interpreted as representational strength. Consistent with the view that attentional sets, and not superficial cues, control lower-level representations, we found that cue-related activity is largely irrelevant for performance, a result that also holds up when cue decoding accuracy is entered as the sole predictor. In contrast, attentional-set information became highly predictive of RTs during the post-stimulus phase, suggesting that fluctuations in the quality of attentional-sets are a major source of trial-to-trial variability in performance. Consistent with the parallel-activation account, attentional sets begin to predict RTs only once also the (independent) predictive power of stimulus and response information emerges. It is noteworthy that the predictive effect for task-level decoding emerges over and above the—also substantial—predictive effects for the target location and the feature/response aspect. Moreover, the aspects that we do not expect to predict performance (i.e., the cue and the distractor location) show no relationships. This result rules out the possibility that fast-RT trials are simply less noisy and therefore allow better decoding of any aspect.
We also looked at the degree to which the decoding accuracy for the different aspects is related to individual differences in RTs. We found that the temporal pattern of simple correlations between individuals’ average decoding accuracy for each task aspect and their average RT was very similar to the within-individual predictive pattern. As shown in Fig. 2B , decoding accuracy for attentional sets was a major source of individual differences in RTs for nearly the entire post-stimulus period, whereas stimulus location correlates early and the feature/response aspect late in the post-stimulus phase (Fig. 2B ). In addition, Fig. 2C shows the scatterplots for the correlations at each task aspects’ peak decoding accuracy (see Fig. 1C ). It is noteworthy that just as for the trial-to-trial relationships, the individual-differences relationships appear to express feature-specific effects on performance, rather than an unspecific, noise/decodability factor. With its small sample size, the current experiment was not originally designed to examine individual differences. Therefore, these analyses need to be treated as exploratory and require replication. Nevertheless, confidence in the results is strengthened by the fact that the relationships are strikingly robust and are, both in terms of involved features and their time course, highly consistent with the within-individual relationships.
Relationship between representations
The notion that cues activate task-level control settings, which in turn bias stimulus and eventually response representations, leads to a straightforward prediction about the sequence in which different lower-level representations should be related to the strength of attentional settings. Figure 3 presents for each timepoint the relationships between the classifier confidence for task-level information and each of the other aspects (to avoid clutter, we omit the distractor here, for which the relationship was close to zero throughout). As expected, early in the prestimulus phase, the strength of attentional sets was coupled with the strength of cue representation, likely indicating the activation of the attentional sets based on the cue 5 . Following stimulus onset, a correlation with the target location emerged and subsequently, a correlation with the response information. This pattern is again consistent with the parallel-activation account, where attentional sets coordinate lower-level representations in a concurrent manner (see Fig. 1B ).
Effects of task switching
In the results presented so far, we had ignored potential effects of trial-to-trial changes in tasks—typically of major interest in task-switching research 1 . In fact, our version of the task-switching paradigm was optimized towards EEG decoding analyses, not towards producing large switch effects (i.e., both the use of long inter-trial intervals and of spatially separate, task-related features can be expected to reduce between-task competition). Indeed, behavioral switch costs were small (see Table 1 ). Nevertheless, we examined the degree to which the switch/repeat contrast plays out in the decoding results. We constrained our analyses a-priori to the 150 ms intervals centered around the peak of the activation trajectories for each feature (see Fig. 1C ). In addition, given the strong relationship between RTs and decoding accuracy for task, stimulus locations, and response, we also conducted a median split into fast and slow RT trials. The median-split was conducted within each subject, task, and switch condition; values were than averaged across tasks and subjects, but presented separately for no-switch and switch trials. The dominant aspect in Fig. 4 is again the strong relationship between RTs on the one hand and task, target location, and response representations on the other. In addition, switching tasks led to weakened task-set representations, both in general (switch main effect: F (1,19) = 6.69, p = 0.018), but in particular on slow-RT trials (fast/slow × switch interaction: F (1,19) = 6.62, p = 0.019). Also, distractor representations were increased on switch trials, F (1,19) = 5.23, p = 0.034. Thus, at the time of peak attentional-set activation, decoding of task-level information was less robust on switch than on no-switch trials, whereas information related to the competing task was more strongly expressed.
How abstract are attentional sets?
In our paradigm, attentional settings are confounded with attention to different visual features (i.e., color versus orientation). Thus, the results reported so far do not allow strong conclusions about the question to what degree the task-level decoding reflects representations of abstract task rules, or the engagement of task-correlated sensory or response-related features. In fact, as shown in the Supplemental Material, there were marked between-task differences in the time course of encoding target locations, with color decoding being stronger and earlier than orientation decoding. Arguably, even if task-level decoding is driven by such aspects they would still be the consequence of top-down, task-level representations and thus indirectly reflect the strength of top-down control through such abstract representations. However, we can also conduct additional analyses to explore the abstractness of the information decoded on the level of tasks.
As a first step, we analyzed how task-set decoding generalizes across critical stimulus/response aspects. For this purpose, we split the data into four target positions by two features/responses (=8) bins. We then trained classifiers to discriminate task for each of these bins and tested for generalization both with left-out trials within the source bin and for the remaining seven bins. The insert in Fig. 1C presents the time-course of average, within-bin classification accuracy and across-bin classification accuracy (for each source bin, averaged across all seven generalization bins). As evident, accuracy for generalization analyses was somewhat reduced, but remained very robust. This result confirms that at least a substantial part of the decodable task-related information is indeed of a relatively abstract nature.
In a second step, we can ask to what degree the predictive power of task-level information (see Fig. 2A ) is associated with abstract information versus task-correlated, lower-level aspects. Thus, instead of task-set classifier confidence, we used the degree of generalization (see insert to Fig. 1C ) to predict RTs. As shown in the insert to Fig. 2A , the generalizable aspect of the decoded task-level representation remained a robust predictor of performance. In the Supplemental Material, we report an additional analysis, where we controlled in the predictive analyses (Fig. 2A ) for the degree to which lower-level representations generalized across tasks on a trial-by-trial basis (see Fig. S3 ). Again, we found no change to the overall predictive pattern, confirming that the decoded task-set representations were relatively abstract (Fig. 4A ).
Consistency of task decoding patterns
We used an individual-specific decoding approach because we had no a-priori predictions about the frequency bands and electrode locations that capture task-specific information. Nevertheless, it would be useful to know to what degree such an individual-specific approach is in fact warranted. Therefore, we computed for each subject, electrode, and frequency band the power across the 150 ms around the average, peak decoding accuracy for task sets (see Fig. 1C ) and compared these across the two tasks. We then correlated the resulting vector of 80 (20 electrode × 4 frequency bands) t -values for the task contrast for each participant with that of every other participant. Figure 5 shows the histogram of the resulting correlations; the average is r = 0.13 (computed by averaging z-transformed correlations and re-transforming the mean into an r coefficient) and the range, although tilted towards the positive direction is very large. This result suggests that the pattern separating the two tasks is fairly idiosyncratic and therefore justifies an individual-specific decoding approach.
When people need to respond to a given stimulus in a flexible, context-dependent manner, the flow of information processing cannot rely on sensory or response representations alone. Rather, stimulus and response selection processes have to be constrained by representations of the current context, goals, and/or stimulus-response rules. There is a substantial literature using behavioral 1 and neuroimaging methods 37 , 38 , 39 , 40 on how we select and change representations that enact top-down control. However, compared to the recent progress made using neurophysiological methods in primates (Siegel et al . 17 ), it has been much more difficult to precisely characterize the place and the relevance of such representations within the overall information processing cascade.
By decoding information about all potentially relevant features from EEG signals, we revealed a plausible sequence of active representations of target and distractor locations, as well as of response choices. For example, the timing of target/distractor location representations was highly consistent with recent work using eye-tracking to assess the dynamics of attentional allocation to task-relevant and irrelevant features 12 , 26 . More importantly, our results also reveal the time course for both cue and attentional-set representations. Task cues were highly decodable as soon as the cue was presented during the prestimulus phase, but were less strongly expressed once the stimulus appeared. In contrast, task representations exhibited the reverse pattern: Their activity ramped up only slowly during the post-cue/pre-stimulus phase, but showed a very strong presence during the entire stimulus-to-response.
The pattern of average, cue and attentional-set activation trajectories is of some theoretical interest by itself. For example, contrary to one prominent model 4 , the fact that task-level decoding accuracy is much higher than cue decoding accuracy (at least after stimulus presentation) suggests that task-set activity is more important than superficial cue information in controlling lower-level representations. Yet, average decoding accuracy allows no firm conclusions about the functional relevance of the different representations. This is where decoding scores on the single-trial level yield important, additional information. Using these scores to predict trial-to-trial variability in RTs, allowed us to determine the representations that drive performance in a time-resolved manner (Fig. 2 ). Interestingly, the pattern of predictive relationships indicates that cue representations do not explain variability in performance. In contrast, task representations emerged as a very robust predictor of trial-to-trial variability in RTs. As for the trajectories of decoding accuracy (see Fig. 1C ), the explanatory power of task-level representations was again largely limited to the post-stimulus phase and was most robust when also stimulus and response effects are particularly strong. Task-level information (but not cue information) also emerged as a robust predictor of inter-individual differences in performance—a result that will need to be confirmed with larger samples. In addition, an analysis of interrelationships between task and lower-level decoding scores (see Fig. 3 ) indicates that attentional sets were coupled in the post-stimulus phase initially with the target-location representations, and thereafter also with feature/response representations. Combined, these results strongly suggest that lower-level representations are configured through more abstract task or attentional settings, rather than through superficial cue representations. Further, the trajectory of average representational strength and the predictive pattern is most consistent with the parallel activation model (Fig. 1B ), where attentional sets can shape lower-level processes in a concurrent manner 3 .
Our results do not rule out the possibility of functionally relevant, preparatory activity before stimulus/response processing sets in. In fact, the use of cue information to retrieve the current attentional set is a necessary process that clearly happens within the cue-to-stimulus interval 5 . The early inter-relationship between strength of cue and task representations likely is an expression of this retrieval activity (Fig. 3 ). It is reasonable to assume that the 300 ms interval between cue and stimulus interval was sufficient to absorb major, within-individual or between-individual variability in the duration or quality of this process, thus preventing any predictive effects of prestimulus cue or task-set representations from revealing themselves. Also, it is very well possible that with longer preparatory intervals, greater proactive task-set activity might be observed. Both behaviorally and in EEG or fMRI neuroimaging studies, preparation effects are well documented 27 , 41 , 42 , 43 , 44 . However, the fact that task-set representations were strongest, and also most predictive of performance in the presence of stimulus and response representations suggests that a key function of attentional sets is to regulate these lower-level representations in a concurrent manner. This conclusion is also consistent with a large body of behavioral work suggesting that task-selection costs cannot be easily eliminated through opportunity for preparation 1 .
The most important limitation of the current work is that we cannot be certain that the decoded, task-level information reflects exclusively abstract rule-type representations. As the two different tasks required attention to different visual aspects and different S-R rules it is possible that between-task decoding is driven to a large part by these aspects (see Supplemental Material). Even if that was the case though, given that the bottom-up stimulation did not differ across tasks, these aspects must reflect the degree of task-related engagement, which at the very least is an indirect reflection of higher-level control representations.
We also report several results suggesting that the task decoding accuracy we observed does in fact represent the strength of relatively abstract, task or attentional settings. For example, in the predictive analyses, task-level classifier confidence explained substantial, within-individual variability in RTs over and above the predictive relationships between RTs and lower-level aspects. We also demonstrated that task representations generalized across target positions and features/responses. Furthermore, these generalization scores proved nearly as predictive of within-individual and between-individual variability in performance as the regular decoding accuracy.
Because we had no strong a-priori predictions about frequency bands or electrode sites that might carry the relevant information, we used a strict bottom-up approach to decode tasks/attentional sets. Obviously, decoding analyses could be restricted to specific frequency bands (or electrodes) to test predictions about which parts of the signal contain theoretically relevant information 45 . Frequency-specific decoding analyses would also address another limitation of the current approach. Time-frequency analysis comes with some degree of blurring of the precise temporal characteristics—which for example is the likely reason for the above-chance increase of decoding accuracy for the target feature before stimulus onset (see Fig. 1C ). Given that the degree of blurring is larger for lower than higher frequency bands and that there is no control over the contribution of each frequency band to the overall decoding accuracy, it is difficult to determine the degree to which temporal precision is compromised. When precise temporal relationships among features need to be assessed (e.g., which feature is activated first) then it would be useful to conduct decoding analyses in a frequency-specific manner. In the current case, however, none of our conclusions rest on precise, temporal comparisons.
In key aspects, our pattern of results was remarkably similar to earlier-mentioned results reported by Siegel et al . 17 . In particular, cue information was highly prominent during the pre-stimulus phase, but then tapered off in the post-stimulus phase. Task information emerged concurrently with cue information, but then increased dramatically as stimulus and response choice information was processed during the stimulus phase. The convergence of results across species and methods suggests that some of the same information that is conveyed through neuron-level recordings can also be extracted through scalp-level EEG signals. The fact that we were able to extract information about task-relevant features through relatively sparse recordings from the scalp is generally consistent with the fact that in Siegel et al ., both higher-level and lower-level aspects were represented throughout all cortical regions, albeit with varying strengths across regions.
To summarize, we show here that EEG-based, trial-by-trial decoding analyses can clarify the relative role and the temporal dynamics of both lower-level stimulus/response, as well as more abstract attentional-set representations. In particular, the ability to pinpoint the exact source of performance differences within a cascade of simultaneously evolving representations is a unique feature of this approach.
Change history
13 may 2020.
An amendment to this paper has been published and can be accessed via a link at the top of the paper.
Kiesel, A. et al . Control and interference in task switching—A review. Psychol Bull 136 , 849 (2010).
Article Google Scholar
Sakai, K. Task set and prefrontal cortex. Annu Rev Neurosci 31 , 219–245 (2008).
Article CAS Google Scholar
Gilbert, S. J. & Shallice, T. Task switching: A PDP model. Cogn Psychol 44 , 297–337, https://doi.org/10.1006/cogp.2001.0770 (2002).
Article PubMed Google Scholar
Logan, G. D. & Bundesen, C. Clever homunculus: Is there an endogenous act of control in the explicit task-cuing procedure? J Exp Psychol Hum Percept Perform 29 , 575 (2003).
Mayr, U. & Kliegl, R. Differential effects of cue changes and task changes on task-set selection costs. J Exp Psychol Learn Mem Cogn 29 , 362–372, https://doi.org/10.1037/0278-7393.29.36.362 (2003).
Badre, D., Kayser, A. S. & D’Esposito, M. Frontal cortex and the discovery of abstract action rules. Neuron 66 , 315–326 (2010).
Dosenbach, N. U., Fair, D. A., Cohen, A. L., Schlaggar, B. L. & Petersen, S. E. A dual-networks architecture of top-down control. Trends Cogn Sci 12 , 99–105 (2008).
Sakai, K. & Passingham, R. E. Prefrontal set activity predicts rule-specific neural processing during subsequent cognitive performance. J Neurosci 26 , 1211–1218 (2006).
Schneider, D. W. & Logan, G. D. Modeling task switching without switching tasks: a short-term priming account of explicitly cued performance. J Exp Psychol Gen 134 , 343 (2005).
Monsell, S. Task switching. Trends Cogn Sci 7 , 134–140, https://doi.org/10.1016/s1364-6613(03)00028-7 (2003).
Rogers, R. D. & Monsell, S. Costs of a predictible switch between simple cognitive tasks. J Exp Psychol Gen 124 , 207 (1995).
Mayr, U., Kuhns, D. & Rieter, M. Eye movements reveal dynamics of task control. J Exp Psychol Gen 142 , 489 (2013).
Rubinstein, J. S., Meyer, D. E. & Evans, J. E. Executive control of cognitive processes in task switching. J Exp Psychol Hum Percept Perform 27 , 763 (2001).
Jost, K., Mayr, U. & Rosler, F. Is task switching nothing but cue priming? Evidence from ERPs. Cogn Affect Behav Neurosci 8 , 74–84, https://doi.org/10.3758/cabn.8.1.74 (2008).
Braver, T. S., Reynolds, J. R. & Donaldson, D. I. Neural mechanisms of transient and sustained cognitive control during task switching. Neuron 39 , 713–726 (2003).
Ouyang, G., Hildebrandt, A., Sommer, W. & Zhou, C. Exploiting the intra-subject latency variability from single-trial event-related potentials in the P3 time range: a review and comparative evaluation of methods. Neurosci & Biobeha Rev 75 , 1–21 (2017).
Siegel, M., Buschman, T. J. & Miller, E. K. Cortical information flow during flexible sensorimotor decisions. Science 348 , 1352–1355 (2015).
Article ADS CAS Google Scholar
Wallis, J. D., Anderson, K. C. & Miller, E. K. Single neurons in prefrontal cortex encode abstract rules. Nature 411 , 953 (2001).
Donahue, C. H. & Lee, D. Dynamic routing of task-relevant signals for decision making in dorsolateral prefrontal cortex. Nature Neurosci 18 , 295–301 (2015).
Goodwin, S. J., Blackman, R. K., Sakellaridi, S. & Chafee, M. V. Executive control over cognition: stronger and earlier rule-based modulation of spatial category signals in prefrontal cortex relative to parietal cortex. J Neurosci 32 , 3499–3515 (2012).
Stokes, M. G., Wolff, M. J. & Spaak, E. Decoding rich spatial information with high temporal resolution. Trends Cogn Sci 19 , 636–638 (2015).
Foster, J. J., Sutterer, D. W., Serences, J. T., Vogel, E. K. & Awh, E. The topography of alpha-band activity tracks the content of spatial working memory. J Neurophysiol 115 , 168–177 (2016).
Samaha, J., Sprague, T. C. & Postle, B. R. Decoding and reconstructing the focus of spatial attention from the topography of alpha-band oscillations. J Cogn Neurosci 28 , 1090–1097(2016).
Murphy, B. et al . EEG decoding of semantic category reveals distributed representations for single concepts. Neuroimage 117 , 12–22 (2011).
Google Scholar
King, J. & Dehaene, S. Characterizing the dynamics of mental representations: the temporal generalization method. Trends Cogn Sci 18 , 203–210 (2014).
Kikumoto, A., Hubbard, J. & Mayr, U. Dynamics of task-set carry-over: evidence from eye-movement analyses. Psychon Bull Rev 23 , 899 (2016).
Monsell, S. & Mizon, G. A. Can the task-cuing paradigm measure an endogenous task-set reconfiguration process? J Exp Psychol Hum Percept Perform 32 , 493 (2006).
Delorme, A. & Makeig, S. EEGLAB: an open source toolbox for analysis of single-trial EEG dynamics including independent component analysis. J Neurosci Meth 134 , 9–21 (2004).
Cohen, M. X. Analyzing neural time series data: theory and practice . (MIT Press, 2014).
Buschman, T. J., Denovellis, E. L., Diogo, C., Bullock, D. & Miller, E. K. Synchronous oscillatory neural ensembles for rules in the prefrontal cortex. Neuron 76 , 838–846 (2012).
Fries, P. A mechanism for cognitive dynamics: neuronal communication through neuronal coherence. Trends Cogn Sci 9 , 474–480 (2005).
Pedregosa, F. et al . Scikit-learn: Machine learning in Python. J Mach Learn Res 12 , 2825–2830 (2011).
MathSciNet MATH Google Scholar
Mayr, U. Age differences in the selection of mental sets: The role of inhibition, stimulus ambiguity, and response-set overlap. Psychol and Aging 16 , 96–109, https://doi.org/10.1037//0882-7974.16.1.96 (2001).
Nichols, T. E. & Holmes, A. P. Nonparametric permutation tests for functional neuroimaging: a primer with examples. Hum Brain Mapp 15 , 1–25 (2002).
Maris, E. & Oostenveld, R. Nonparametric statistical testing of EEG-and MEG-data. J Neurosci Meth 164 , 177–190 (2007).
Bates, D., Maechler, M. & Dai, B. The lme4 package. Computer software manual]. Retrieved from http://cran.r-project.org/web/packages/lme4/lme4.pdf (2008).
Wager, T. D., Jonides, J. & Reading, S. Neuroimaging studies of shifting attention: a meta-analysis. Neuroimage 22 , 1679–1693 (2004).
Kim, C., Cilles, S. E., Johnson, N. F. & Gold, B. T. Domain general and domain preferential brain regions associated with different types of task switching: A Meta‐Analysis. Hum Brain Mapp 33 , 130–142 (2012).
Qiao, L., Zhang, L., Chen, A. & Egner, T. Dynamic Trial-by-Trial Re-Coding of Task-Set Representations in Frontoparietal Cortex Mediates Behavioral Flexibility. J Neurosci , 0935–0917 (2017).
Waskom, M. L., Kumaran, D., Gordon, A. M., Rissman, J. & Wagner, A. D. Frontoparietal representations of task context support the flexible control of goal-directed cognition. J Neurosci 34 , 10743–10755 (2014).
Meiran, N. Reconfiguration of stimulus task sets and response task sets during task switching. Control of cognitive processes: Attention and performance XVIII , 377–399 (2000).
Ruge, H., Jamadar, S., Zimmermann, U. & Karayanidis, F. The many faces of preparatory control in task switching: reviewing a decade of fMRI research. Hum Brain Mapp 34 , 12–35 (2013).
Manelis, A. & Reder, L. M. He Who is well prepared has half won the battle: an fMRI Study of task preparation. Cereb cortex 25 , 726–735 (2013).
Lavric, A., Mizon, G. A. & Monsell, S. Neurophysiological signature of effective anticipatory task‐set control: a task‐switching investigation. Eur J Neurosci 28 (5), 1016–1029 (2008).
Kikumoto, A. & Mayr, U. Decoding hierarchical control of sequential behavior in oscillatory EEG activity. eLife 7 , e38550 (2018).
Download references
Acknowledgements
This work was supported in part by National Institute of Aging grant R01 AG037564-01A1, as well by an Award by the Humboldt Foundation to Ulrich Mayr.
Author information
Authors and affiliations.
University of Oregon, Eugene, OR, 97403, United States
Jason Hubbard, Atsushi Kikumoto & Ulrich Mayr
You can also search for this author in PubMed Google Scholar
Contributions
J.H., A.K., U.M. jointly designed the experiment. J.H. took the lead with the analyses, aided by A.K. and supervised by U.M. U.M. took the lead with writing the paper, aided by J.H. and A.K.
Corresponding author
Correspondence to Ulrich Mayr .
Ethics declarations
Competing interests.
The authors declare no competing interests.
Additional information
Publisher’s note: Springer Nature remains neutral with regard to jurisdictional claims in published maps and institutional affiliations.
Supplementary information
Supplementary material, rights and permissions.
Open Access This article is licensed under a Creative Commons Attribution 4.0 International License, which permits use, sharing, adaptation, distribution and reproduction in any medium or format, as long as you give appropriate credit to the original author(s) and the source, provide a link to the Creative Commons license, and indicate if changes were made. The images or other third party material in this article are included in the article’s Creative Commons license, unless indicated otherwise in a credit line to the material. If material is not included in the article’s Creative Commons license and your intended use is not permitted by statutory regulation or exceeds the permitted use, you will need to obtain permission directly from the copyright holder. To view a copy of this license, visit http://creativecommons.org/licenses/by/4.0/ .
Reprints and permissions
About this article
Cite this article.
Hubbard, J., Kikumoto, A. & Mayr, U. EEG Decoding Reveals the Strength and Temporal Dynamics of Goal-Relevant Representations. Sci Rep 9 , 9051 (2019). https://doi.org/10.1038/s41598-019-45333-6
Download citation
Received : 03 January 2019
Accepted : 28 May 2019
Published : 21 June 2019
DOI : https://doi.org/10.1038/s41598-019-45333-6
Share this article
Anyone you share the following link with will be able to read this content:
Sorry, a shareable link is not currently available for this article.
Provided by the Springer Nature SharedIt content-sharing initiative
This article is cited by
No semantic information is necessary to evoke general neural signatures of face familiarity: evidence from cross-experiment classification.
- Alexia Dalski
- Gyula Kovács
- Géza Gergely Ambrus
Brain Structure and Function (2022)
Towards a Governance Framework for Brain Data
- Marcello Ienca
- Joseph J. Fins
- Philipp Kellmeyer
Neuroethics (2022)
By submitting a comment you agree to abide by our Terms and Community Guidelines . If you find something abusive or that does not comply with our terms or guidelines please flag it as inappropriate.
Quick links
- Explore articles by subject
- Guide to authors
- Editorial policies
Sign up for the Nature Briefing newsletter — what matters in science, free to your inbox daily.

What Is a Task and How Do You Know If You Have One or More?
- First Online: 29 November 2022
Cite this chapter
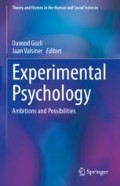
- Eliot Hazeltine 4 ,
- Tobin Dykstra 4 &
- Eric Schumacher 5
Part of the book series: Theory and History in the Human and Social Sciences ((THHSS))
548 Accesses
3 Citations
2 Altmetric
The burgeoning interest in how voluntary actions are organized into goal-based behaviors has produced a rich body of data showing that task representations are complex, integrating not only stimulus and response information but also internal (e.g., goals and relationships among actions) and external (e.g., task-irrelevant stimuli) context. The dominant description of task representations is the task set, a collection of SR associations, whose presence is inferred primarily through task-switch costs. However, we argue that this approach has serious limitations that are often ignored. First, task switch costs likely reflect numerous processes, including those relating to attention and inhibition, that vary across experimental procedures and complicate their interpretation. Second, the switch cost measure is coarse in that it groups transitions into a small number (usually two) of categories. As we demonstrate empirically, this procedure can produce misleading results. Observing a performance cost when a putative task boundary is crossed may be too coarse a measure to adequately describe how a task is organized. So, what exactly is a task? How do researchers know when two responses belong to the same or different tasks, and how do we determine when an effect relates to a change of task instead of some other central operation, such as attentional shifts or rebinding? In this chapter, we will examine the construct of a task and whether it is useful for understanding voluntary behavior.
This is a preview of subscription content, log in via an institution to check access.
Access this chapter
- Available as PDF
- Read on any device
- Instant download
- Own it forever
- Available as EPUB and PDF
- Compact, lightweight edition
- Dispatched in 3 to 5 business days
- Free shipping worldwide - see info
- Durable hardcover edition
Tax calculation will be finalised at checkout
Purchases are for personal use only
Institutional subscriptions
The blocks were included to examine the separate question of whether the decreases in RT associated with reducing the number of stimulus and response alternatives depended on which stimuli were removed from the set. This question is not related to our focus, which whether conventional measures of task switching capture the structure of task as determined by the complete set of transitional RTs.
The actual parameters used were as follows: inertia, 0.1; repulsion strength, 20,000.0; attraction strength, 10.0; maximum displacement, 10.0; auto-stabilized function, true; autostab strength, 80.0; autostab sensibility, 0.2; and gravity, 30.0. Only the repulsion strength was changed from the default value.
The Gephi software does not make an identical graph each time it is run. That is, the positions vary from run to run; although with networks this is simple, they are generally similar. The graphs we have chosen are highly typical of those produced by the software. Moreover, our conclusions are based on the strengths of the connection between responses, depicted by the edge thickness. This is a property of the data and does not change across runs.
Adam, J. J., Hommel, B., & Umilta, C. (2003). Preparing for perception and action (I): The role of grouping in the response-cuing paradigm. Cognitive Psychology, 46 , 302–358.
Article PubMed Google Scholar
Allport, A., Styles, E. A., & Hsieh, S. (1994). Shifting intentional set: Exploring the dynamic control of tasks. In C. Umilta & M. Moscovitch (Eds.), Attention and performance (Vol. attention and performance XV) (pp. 421–452). Harvard University Press.
Google Scholar
Arrington, C. M., & Logan, G. D. (2004). The cost of a voluntary task switch. Psychological Science, 15 , 610–615. https://doi.org/10.1111/j.0956-7976.2004.00728.x
Badre, D. (2008). Cognitive control, hierarchy, and the rostro-caudal organization of the frontal lobes. Trends in Cognitive Sciences, 12 (5), 193–200. https://doi.org/10.1016/j.tics.2008.02.004
Badre, D., & D’Esposito, M. (2009). Is the rostro-caudal axis of the frontal lobe hierarchical. Nature Reviews Neuroscience, 10 , 659–669.
Article PubMed PubMed Central Google Scholar
Badre, D., & Nee, D. E. (2018). Frontal cortex and the hierarchical control of behavior. Trends in Cognitive Sciences, 22 , 170–188. https://doi.org/10.1016/j.tics.2017.11.005
Badre, D., Bhandari, A., Keglovits, H., & Kikumoto, A. (2021). The dimensionality of neural representations for control. Current Opinion in Behavioral Sciences, 38 , 20–28.
Barcelo, F., Escera, C., Corral, M. J., & Periánez, J. A. (2006). Task switching and novelty processing activate a common neural network for cognitive control. Journal of Cognitive Neuroscience, 18 , 1734–1748.
Bartlett, F. C. (1932). Remembering: An experimental and social study . Cambridge University.
Bezdek, M. A., Godwin, C. A., Smith, D. M., Hazeltine, E., & Schumacher, E. H. (2019). How conscious aspects of task representation affect dynamic behavior in complex situations. Psychology of Consciousness: Theory, Research, and Practice, 6 , 225–241.
Botvinick, M. M., Braver, T. S., Barch, D. M., Carter, C. S., & Cohen, J. D. (2001). Conflict monitoring and cognitive control. Psychological Review, 108 , 624–652.
Braver, T. S. (2012). The variable nature of cognitive control: A dual mechanisms framework. Trends in Cognitive Sciences, 16 , 106–113.
Brewer, W. F., & Treyens, J. C. (1981). Role of schemata in memory for places. Cognitive Psychology, 13 , 207–230.
Article Google Scholar
Cohen, J. D., Dunbar, K., & McClelland, J. L. (1990). On the control of automatic processes: A parallel distributed processing account of the Stroop effect. Psychological Review, 97 , 332–361.
Collins, A. G. E., & Frank, M. J. (2016). Motor demands constrain cognitive rule structures. PLoS Computational Biology, 12 , e1004785. https://doi.org/10.1371/journal.pcbi.1004785
Cookson, S. L., Hazeltine, E., & Schumacher, E. H. (2016). Neural representations of stimulus-response associations during task preparation. Brain Research, 1648 , 496–505.
Cookson, S. L., Hazeltine, E., & Schumacher, E. H. (2020). Task structure boundaries affect response preparation. Psychological Research, 84 , 1610–1621. https://doi.org/10.1007/s00426-019-01171-9
Courtney, S. M., Roth, J. K., & Sala, J. B. (2007). A hierarchical biased competition model of domain-dependent working memory maitenance and executive control. In Working memory: Behavioural and neural correlates (pp. 369–383).
Derrfuss, J., Brass, M., Neumann, J., & von Cramon, D. Y. (2005). Involvement of the inferior frontal junction in cognition control: Meta-analyses of switching and Stroop studies. Human Brain Mapping, 25 , 22–34.
Desimone, R., & Duncan, J. (1995). Neural mechanisms of selective visual attention. Annual Review of Neuroscience, 18 , 193–222.
Dosenbach, N. U. F., Fair, D. A., Cohen, A. L., Schlaggar, B. L., & Petersen, S. E. (2008). A dual-networks architecture of top-down control. Trends in Cognitive Sciences, 12 (3), 99–105. https://doi.org/10.1016/j.tics.2008.01.001
Dreisbach, G. (2012). Mechanisms of cognitive control: The functional role of task rules. Current Directions in Psychological Science, 21 , 227–231.
Duncan, J. (2013). The structure of cognition: Attentional episodes in mind and brain. Neuron, 80 , 35–50.
Dykstra, T., Smith, D. M., Schumacher, E. H., & Hazeltine, E. (in prep). Measuring task structure with transitional response times: Task representations are more than task sets.
Eriksen, B. A., & Eriksen, C. W. (1974). Effects of noise letters upon the identification of a target letter in a nonsearch task. Perception & Psychophysics, 16 , 143–149.
Fitts, P. M., & Deininger, R. L. (1954). S-R compatibility: Correspondence among paired elements within stimulus and response codes. Journal of Experimental Psychology, 48 , 483–492.
Frings, C., Hommel, B., Koch, I., Rothermund, K., Dignath, D., Giesen, C., & Philipp, A. (2020). Binding and retrieval in action control (BRAC). Trends in Cognitive Sciences , 375–387. https://doi.org/10.1016/j.tics.2020.02.004
Fuster, J. M. (2008). The prefrontal cortex (4th ed.). Elsevier.
Gopher, D., Armony, L., & Greenshpan, Y. (2000). Switching tasks and attention policies. Journal of Experimental Psychology: General, 129 , 309–339.
Gozli, D. (2019). Experimental psychology and human agency . Springer.
Book Google Scholar
Grant, L. D., Cookson, S. L., & Weissman, D. H. (2020). Task sets serve as boundaries for the congruency sequence effect. Journal of Experimental Psychology: Human Perception and Performance, 46 , 798–812.
PubMed Google Scholar
Halvorson, K. M., & Hazeltine, E. (2015). Do small dual-task costs reflect ideomotor compatibility or the absence of crosstalk? Psychonomic Bulletin & Review, 22 , 1403–1409. https://doi.org/10.3758/s13423-015-0813-8
Hayes, A., Davidson, M., Keele, S. W., & Rafal, R. D. (1998). Toward a functional analysis of the basal ganglia. Journal of Cognitive Neuroscience, 10 , 178–198.
Hazeltine, E. (2005). Response-response compatibility during bimanual movements: Evidence for the conceptual coding of action. Psychonomic Bulletin & Review, 12 , 682–688.
Hazeltine, E., & Schumacher, E. H. (2016). In B. Ross (Ed.), Understanding central processes: The case against simple stimulus-response associations and for complex task representation (Vol. 64, pp. 195–245). Psychology of Learning and Motivation.
Hazeltine, E., Akçay, Ç., & Mordkoff, J. T. (2011a). Keeping Simon simple: Examining the relationship between sequential modulations and feature repetitions with two stimuli, two locations, and two responses. Acta Psychologia, 136 , 245–252.
Hazeltine, E., Lightman, E., Schwarb, H., & Schumacher, E. H. (2011b). The boundaries of sequential modulations: Evidence for set-level control. Journal of Experimental Psychology: Human Perception and Performance, 37 , 1898–1914.
Hirsch, P., Schwarzkopp, T., Declerck, M., Reese, S., & Koch, I. (2016). Age-related differences in task switching and task preparation: Exploring the role of task-set competition. Acta Psychologia, 170 , 66–71.
Hommel, B. (1998). Event files: Evidence for automatic integration of stimulus–response episodes. Visual Cognition, 5 , 183–216.
Hommel, B. (2004). Event files: Feature binding in and across perception and action. Trends in Cognitive Science, 8 , 494–500.
Hommel, B., Müsseler, J., Aschersleben, G., & Prinz, W. (2001). The theory of event coding (TEC). Behavioral and Brain Sciences, 24 , 849–878.
Hommel, B., Proctor, R. W., & Vu, K. L. (2004). A feature-integration account of sequential effects in the Simon task. Psychological Research, 68 , 1–17.
Jersild, A. T. (1927). Mental set and shift. Archives of Psychology, 89 .
Kiesel, A., Steinhauser, M., Wendt, M., Falkenstein, M., Jost, K., Philipp, A. M., & Koch, I. (2010). Control and interference in task switching-a review. Psychological Bulletin, 136 (5), 849–874. https://doi.org/10.1037/a0019842
Kikumoto, A., & Mayr, U. (2020). Conjunctive representations that integrate stimuli, responses, and rules are critical for action selection. Proceedings of the National Academy of Sciences, 117 , 10603–10608.
Kim, C., Cilles, S. E., Johnson, N. F., & Gold, B. T. (2012). Domain general and domain preferential brain regions associated with different types of task switching: A meta-analysis. Human Brain Mapping, 33 , 130–142.
Koch, I., Poljac, E., Müller, H., & Kiesel, A. (2018). Cognitive structure, flexibility, and plasticity in human multitasking – An integrative review of dual-task and task-switching research. Psychological Bulletin, 144 , 557–583. https://doi.org/10.1037/bul0000144
Koechlin, E., & Summerfield, C. (2007). An information theoretical approach to prefrontal executive function. Trends in Cognitive Sciences, 11 (6), 229–235. https://doi.org/10.1016/j.tics.2007.04.005
Kornblum, S., Hasbroucq, T., & Osman, A. (1990). Dimensional overlap: Cognitive basis for stimulus-response compatibility--A model and taxonomy. Psychological Review, 97 , 253–270.
Lien, M.-C., Ruthruff, E., Remington, R. W., & Johnston, J. C. (2005). On the limits of advance preparation for a task-switch: Do people prepare all of the task some of the time or some of the task all the time. Journal of Experimental Psychology: Human Perception and Performance, 31 , 299–315.
Logan, G. D. (2002). An instance theory of attention and memory. Psychological Review, 109 , 376–400.
Logan, G. D. (2004). Working memory, task switching, and executive control in the task span procedure. Journal of Experimental Psychology: General, 133 , 218–236. https://doi.org/10.1037/0096-3445.133.2.218
Logan, G. D., & Schneider, D. W. (2006). Interpreting instructional cues in task switching procedures: The role of mediator retrieval. Journal of Experimental Psychology: Learning, Memory and Cognition, 32 , 347–363. https://doi.org/10.1037/0278-7393.32.3.347
MacDonald, A. W., Cohen, J. D., Stenger, V. A., & Carter, C. S. (2000). Dissociating the role of the dorsolateral prefrontal cortex and anterior cingulate cortex in cognitive control. Science, 288 , 1835–1838.
Mayr, U. (2002). Inhibition of action rules. Psychonomic Bulletin & Review, 9 , 93–99.
Mayr, U., & Bryck, R. L. (2005). Sticky rules: Integration between abstract rules and specific actions. Journal of Experimental Psychology: Learning, Memory and Cognition, 31 , 337–350.
Mayr, U., Diedrichsen, J., Ivry, R. B., & Keele, S. W. (2006). Dissociating task-set selection from task-set inhibition in the prefrontal cortex. Journal of Cognitive Neuroscience, 18 , 14–21. https://doi.org/10.1162/089892906775250085
Meiran, N., Chorev, Z., & Sapir, A. (2000). Component processes in task switching. Cognitive Psychology, 41 , 211–253.
Miller, J. (1985). A hand advantage in preparation of simple keypress responses: Reply to reeve and Proctor (1984). Journal of Experimental Psychology: Human Perception and Performance, 11 , 221–233.
Miller, E. K., & Cohen, J. D. (2001). An integrative theory of prefrontal cortex function. Annual Review of Neuroscience, 24 , 167–202.
Mittelstädt, V., Miller, J., & Kiesel, A. (2018). Trading off switch costs and stimulus availability benefits: An investigation of voluntary task-switching behavior in a predictable dynamic multitasking environment. Memory & Cognition, 46 , 699–715.
Miyake, A., Friedman, N. P., Emerson, M. J., Witzki, A. H., Howerter, A., & Wager, T. D. (2000). The unity and diversity of executive functions and their contributions to complex “frontal lobe” tasks: A latent variable analysis. Cognitive Psychology, 41 , 49–100.
Monsell, S. (2003). Task switching. Trends in Cognitive Sciences, 7 , 134–140. https://doi.org/10.1016/S1364-6613(03)00028-7
Monsell, S., & Driver, J. (2000). Banishing the control homunculus. In S. Monsell & J. Driver (Eds.), Attention and performance XVIII: Control of cognitive processes (pp. 3–32). MIT Press.
Chapter Google Scholar
Murray, S., Krasich, K., Schooler, J. W., & Seli, P. (2020). What's in a task? Complications in the study of the task-unrelated-thought variety of mind wandering. Perspectives on Psychological Science, 15 , 572–588. https://doi.org/10.1177/1745691619897966
Norman, D. A. (1981). Categorization of action slips. Psychological Review, 88 , 1–15.
Norman, D. A., & Shallice, T. (1986). Attention to action: Willed and automatic control of behavior. In J. Davidson, G. E. Schwartz, & D. Shapiro (Eds.), Consciousness and self-regulation (Vol. 4, pp. 1–18). Plenum.
Petrides, M. (2006). The rostro-caudal axis of cognitive control procesing within lateral frontal cortex. In From monkey brain to human brain: A Fyssen Foundation symposium (pp. 293–314).
Poljac, E., Koch, I., & Bekkering, H. (2009). Dissociating restart cost and mixing cost in task switching. Psychological Research, 73 , 407–416.
Prinz, W. (1990). A common coding approach to perception and action. In O. Neumann & W. Prinz (Eds.), Relationships between perception and action (pp. 167–201). Springer-Verlag.
Qiao, L., Chen, A., & Egner, T. (2017). Dynamic trial-by-trial recoding of task-set representations in the frontoparietal cortex mediates behavioral flexibility. Journal of Neuroscience, 37 , 11037–11050. https://doi.org/10.1523/JNEUROSCI.0935-17.2017
Qiao, L., Xu, M., Zhang, L., Li, H., & Chen, A. (2020). Flexible adjustment of the effective connectivity between the fronto-parietal and visual regions supports cognitive flexibility. NeuroImage . https://doi.org/10.1016/j.neuroimage.2020.117158
Rescorla, R. A. (1988a). Behavioral studies of Pavlovian conditioning. Annual Review of Neuroscience, 11 , 329–352.
Rescorla, R. A. (1988b). Pavlovian conditioning: It's not what you think it is. American Psychologist, 43 , 151–160.
Rogers, R., & Monsell, S. (1995). The costs of a predictable switch between simple cognitive tasks. Journal of Experimental Psychology: Human Perception and Performance, 124 , 207–231.
Rosenbaum, D. A. (1980). Human movement initiation: Specification of arm, direction, and extent. Journal of Experimental Psychology: General, 109 , 444–474.
Rosenbaum, D. A. (1983). The movement precuing technique: Assumptions, applications, and extensions. In R. A. Magill (Ed.), Memory and control of action (pp. 230–274). North-Holland Publishing Company.
Ruthruff, E., Remington, R. W., & Johnston, J. C. (2001). Switching between simple cognitive tasks: The interaction of top-down and bottom-up factors. Journal of Experimental Psychology: Human Perception and Performance, 27 , 1404–1419.
Sakai, K. (2008). Task set and prefrontal cortex. In Annual review of neuroscience (Vol. 31, pp. 219–245). Annual Reviews.
Schmidt, J. R., De Houwer, J., & Rothermund, K. (2016). The parallel episodic processing (PEP) model 2.0: A single computational model of stimulus-response binding, contingency learning, power curves, and mixing costs. Cognitive Psychology, 91 , 82–108.
Schumacher, E. H., & Hazeltine, E. (2016). Hierarchical task representation: Task files and response selection. Current Directions in Psychological Science, 25 , 449–454. https://doi.org/10.1177/0963721416665085
Schumacher, E. H., & Schwarb, H. (2009). Parallel response selection disrupts sequence learning under dual-task conditions. Journal of Experimental Psychology: General, 138 , 270–290.
Shallice, T. (1982). Specific impairments of planning. Philosophical Transactions of the Royal Society of London B, 298 , 199–209.
Simon, J. R. (1969). Reactions towards the source of stimulation. Journal of Experimental Psychology, 81 , 174–176.
Smith, D. M., Dykstra, T., Hazeltine, E., & Schumacher, E. H. (2020). Task representation affects the boundaries of behavioral slowing following and error. Attention Perception & Psychophysics, 82 , 2315–2326. https://doi.org/10.3758/s13414-020-01985-5
Stroop, J. R. (1935). Studies of interference in serial verbal reactions. Journal of Experimental Psychology, 18 , 643–662.
Takacs, A., Mückschel, M., Roessner, V., & Beste, C. (2020). Decoding stimulus-response representations and their stability using EEG-based multivariate pattern analysis. Cerebral Cortex Communications . https://doi.org/10.1093/texcom/tgaa016
Tolman, E. C. (1932). Purposive behavior in animals and men . Century.
Tolman, E. C. (1948). Cognitive maps in rats and men. Psychological Review, 55 , 189–208. Retrieved from https://pdfs.semanticscholar.org/0874/a64d60a23a20303877e23caf8e1d4bb446a4.pdf
Tuckey, M. R., & Brewer, N. (2003). The influence of schemas, stimulus ambiguity, and interview schedule on eyewitness memory over time. Journal of Experimental Psychology: Applied, 9 , 101.
Verguts, T., & Notebaert, W. (2008). Hebbian learning of cognitive control: Dealing with specific and nonspecific adaptation. Psychological Review, 115 (2), 518–525. https://doi.org/10.1037/0033-295x.115.2.518
Waskom, M. L., Kumaran, D., Gordon, A. M., Rissman, J., & Wagner, A. D. (2014). Frontoparietal representations of task context support the flexible control of goal-directed cognition. Journal of Neuroscience, 34 , 10743–10755.
Weissman, D. H., Jiang, J., & Egner, T. (2014). Determinants of congruency sequence effects without learning and memory confounds. Journal of Experimental Psychology: Human Perception and Performance, 40 , 2022–2037.
Wifall, T., Hazeltine, E., & Mordkoff, J. T. (2015). The roles of stimulus and response uncertainty in forced-choice performance: An amendment of hick/Hyman law. Psychological Research Psychologische Forschung . https://doi.org/10.1007/s00426-015-0675-8
Wisniewski, D., Reverberi, C., Tusche, A., & Haynes, J.-D. (2015). The neural representation of voluntary task-set selection in dynamic environments. Cerebral Cortex, 25 , 4715–4726.
Woolgar, A., Hampshire, A., Thompson, R., & Duncan, J. (2011). Adaptive coding of task-relevant information in human frontoparietal cortex. Journal of Neuroscience, 31 , 14592–14599.
Wylie, G., & Allport, A. (2000). Task switching and the measurement of "switch costs". Psychological Research, 63 , 212–233.
Download references
Author information
Authors and affiliations.
Department of Psychological and Brain Sciences, University of Iowa, Iowa City, IA, USA
Eliot Hazeltine & Tobin Dykstra
Department of Psychology, Georgia Institute of Technology, Atlanta, GA, USA
Eric Schumacher
You can also search for this author in PubMed Google Scholar
Corresponding author
Correspondence to Eliot Hazeltine .
Editor information
Editors and affiliations.
University of Macau, Taipa, Macau S.A.R., China
Davood Gozli
Communication and Psychology, Aalborg University, AALBORG, Denmark
Jaan Valsiner
Rights and permissions
Reprints and permissions
Copyright information
© 2022 The Author(s), under exclusive license to Springer Nature Switzerland AG
About this chapter
Hazeltine, E., Dykstra, T., Schumacher, E. (2022). What Is a Task and How Do You Know If You Have One or More?. In: Gozli, D., Valsiner, J. (eds) Experimental Psychology. Theory and History in the Human and Social Sciences. Springer, Cham. https://doi.org/10.1007/978-3-031-17053-9_6
Download citation
DOI : https://doi.org/10.1007/978-3-031-17053-9_6
Published : 29 November 2022
Publisher Name : Springer, Cham
Print ISBN : 978-3-031-17052-2
Online ISBN : 978-3-031-17053-9
eBook Packages : Behavioral Science and Psychology Behavioral Science and Psychology (R0)
Share this chapter
Anyone you share the following link with will be able to read this content:
Sorry, a shareable link is not currently available for this article.
Provided by the Springer Nature SharedIt content-sharing initiative
- Publish with us
Policies and ethics
- Find a journal
- Track your research
Task set and prefrontal cortex
Affiliation.
- 1 Department of Cognitive Neuroscience, Graduate School of Medicine, The University of Tokyo, 7-3-1 Hongo, Bunkyo-ku, Tokyo 113-0033, Japan. [email protected]
- PMID: 18558854
- DOI: 10.1146/annurev.neuro.31.060407.125642
A task set is a configuration of cognitive processes that is actively maintained for subsequent task performance. Single-unit and brain-imaging studies have identified the neural correlates for task sets in the prefrontal cortex. Here I examine whether the neural data obtained thus far are sufficient to explain the behaviors that have been illustrated within the conceptual framework of task sets. I first discuss the selectivity of neural activity in representing a specific task. I then discuss the competitions between neural representations of task sets during task switch. Finally I discuss how, in neural terms, a task set is implemented to facilitate task performance. The processes of representing, updating, and implementing task sets occur in parallel at multiple levels of brain organization. Neural accounts of task sets demonstrate that the brain determines our thoughts and behaviors.
Publication types
- Research Support, Non-U.S. Gov't
- Action Potentials / physiology
- Behavior / physiology
- Brain Mapping
- Cognition / physiology*
- Mental Processes / physiology
- Nerve Net / physiology*
- Neurons / physiology*
- Prefrontal Cortex / anatomy & histology
- Prefrontal Cortex / physiology*
- Psychomotor Performance / physiology*
- Search Menu
- Browse content in Arts and Humanities
- Browse content in Archaeology
- Anglo-Saxon and Medieval Archaeology
- Archaeological Methodology and Techniques
- Archaeology by Region
- Archaeology of Religion
- Archaeology of Trade and Exchange
- Biblical Archaeology
- Contemporary and Public Archaeology
- Environmental Archaeology
- Historical Archaeology
- History and Theory of Archaeology
- Industrial Archaeology
- Landscape Archaeology
- Mortuary Archaeology
- Prehistoric Archaeology
- Underwater Archaeology
- Urban Archaeology
- Zooarchaeology
- Browse content in Architecture
- Architectural Structure and Design
- History of Architecture
- Residential and Domestic Buildings
- Theory of Architecture
- Browse content in Art
- Art Subjects and Themes
- History of Art
- Industrial and Commercial Art
- Theory of Art
- Biographical Studies
- Byzantine Studies
- Browse content in Classical Studies
- Classical History
- Classical Philosophy
- Classical Mythology
- Classical Literature
- Classical Reception
- Classical Art and Architecture
- Classical Oratory and Rhetoric
- Greek and Roman Epigraphy
- Greek and Roman Law
- Greek and Roman Archaeology
- Greek and Roman Papyrology
- Late Antiquity
- Religion in the Ancient World
- Digital Humanities
- Browse content in History
- Colonialism and Imperialism
- Diplomatic History
- Environmental History
- Genealogy, Heraldry, Names, and Honours
- Genocide and Ethnic Cleansing
- Historical Geography
- History by Period
- History of Agriculture
- History of Education
- History of Emotions
- History of Gender and Sexuality
- Industrial History
- Intellectual History
- International History
- Labour History
- Legal and Constitutional History
- Local and Family History
- Maritime History
- Military History
- National Liberation and Post-Colonialism
- Oral History
- Political History
- Public History
- Regional and National History
- Revolutions and Rebellions
- Slavery and Abolition of Slavery
- Social and Cultural History
- Theory, Methods, and Historiography
- Urban History
- World History
- Browse content in Language Teaching and Learning
- Language Learning (Specific Skills)
- Language Teaching Theory and Methods
- Browse content in Linguistics
- Applied Linguistics
- Cognitive Linguistics
- Computational Linguistics
- Forensic Linguistics
- Grammar, Syntax and Morphology
- Historical and Diachronic Linguistics
- History of English
- Language Acquisition
- Language Variation
- Language Families
- Language Evolution
- Language Reference
- Lexicography
- Linguistic Theories
- Linguistic Typology
- Linguistic Anthropology
- Phonetics and Phonology
- Psycholinguistics
- Sociolinguistics
- Translation and Interpretation
- Writing Systems
- Browse content in Literature
- Bibliography
- Children's Literature Studies
- Literary Studies (Asian)
- Literary Studies (European)
- Literary Studies (Eco-criticism)
- Literary Studies (Modernism)
- Literary Studies (Romanticism)
- Literary Studies (American)
- Literary Studies - World
- Literary Studies (1500 to 1800)
- Literary Studies (19th Century)
- Literary Studies (20th Century onwards)
- Literary Studies (African American Literature)
- Literary Studies (British and Irish)
- Literary Studies (Early and Medieval)
- Literary Studies (Fiction, Novelists, and Prose Writers)
- Literary Studies (Gender Studies)
- Literary Studies (Graphic Novels)
- Literary Studies (History of the Book)
- Literary Studies (Plays and Playwrights)
- Literary Studies (Poetry and Poets)
- Literary Studies (Postcolonial Literature)
- Literary Studies (Queer Studies)
- Literary Studies (Science Fiction)
- Literary Studies (Travel Literature)
- Literary Studies (War Literature)
- Literary Studies (Women's Writing)
- Literary Theory and Cultural Studies
- Mythology and Folklore
- Shakespeare Studies and Criticism
- Browse content in Media Studies
- Browse content in Music
- Applied Music
- Dance and Music
- Ethics in Music
- Ethnomusicology
- Gender and Sexuality in Music
- Medicine and Music
- Music Cultures
- Music and Religion
- Music and Culture
- Music and Media
- Music Education and Pedagogy
- Music Theory and Analysis
- Musical Scores, Lyrics, and Libretti
- Musical Structures, Styles, and Techniques
- Musicology and Music History
- Performance Practice and Studies
- Race and Ethnicity in Music
- Sound Studies
- Browse content in Performing Arts
- Browse content in Philosophy
- Aesthetics and Philosophy of Art
- Epistemology
- Feminist Philosophy
- History of Western Philosophy
- Metaphysics
- Moral Philosophy
- Non-Western Philosophy
- Philosophy of Science
- Philosophy of Action
- Philosophy of Law
- Philosophy of Religion
- Philosophy of Language
- Philosophy of Mind
- Philosophy of Perception
- Philosophy of Mathematics and Logic
- Practical Ethics
- Social and Political Philosophy
- Browse content in Religion
- Biblical Studies
- Christianity
- East Asian Religions
- History of Religion
- Judaism and Jewish Studies
- Qumran Studies
- Religion and Education
- Religion and Health
- Religion and Politics
- Religion and Science
- Religion and Law
- Religion and Art, Literature, and Music
- Religious Studies
- Browse content in Society and Culture
- Cookery, Food, and Drink
- Cultural Studies
- Customs and Traditions
- Ethical Issues and Debates
- Hobbies, Games, Arts and Crafts
- Lifestyle, Home, and Garden
- Natural world, Country Life, and Pets
- Popular Beliefs and Controversial Knowledge
- Sports and Outdoor Recreation
- Technology and Society
- Travel and Holiday
- Visual Culture
- Browse content in Law
- Arbitration
- Browse content in Company and Commercial Law
- Commercial Law
- Company Law
- Browse content in Comparative Law
- Systems of Law
- Competition Law
- Browse content in Constitutional and Administrative Law
- Government Powers
- Judicial Review
- Local Government Law
- Military and Defence Law
- Parliamentary and Legislative Practice
- Construction Law
- Contract Law
- Browse content in Criminal Law
- Criminal Procedure
- Criminal Evidence Law
- Sentencing and Punishment
- Employment and Labour Law
- Environment and Energy Law
- Browse content in Financial Law
- Banking Law
- Insolvency Law
- History of Law
- Human Rights and Immigration
- Intellectual Property Law
- Browse content in International Law
- Private International Law and Conflict of Laws
- Public International Law
- IT and Communications Law
- Jurisprudence and Philosophy of Law
- Law and Politics
- Law and Society
- Browse content in Legal System and Practice
- Courts and Procedure
- Legal Skills and Practice
- Primary Sources of Law
- Regulation of Legal Profession
- Medical and Healthcare Law
- Browse content in Policing
- Criminal Investigation and Detection
- Police and Security Services
- Police Procedure and Law
- Police Regional Planning
- Browse content in Property Law
- Personal Property Law
- Study and Revision
- Terrorism and National Security Law
- Browse content in Trusts Law
- Wills and Probate or Succession
- Browse content in Medicine and Health
- Browse content in Allied Health Professions
- Arts Therapies
- Clinical Science
- Dietetics and Nutrition
- Occupational Therapy
- Operating Department Practice
- Physiotherapy
- Radiography
- Speech and Language Therapy
- Browse content in Anaesthetics
- General Anaesthesia
- Neuroanaesthesia
- Browse content in Clinical Medicine
- Acute Medicine
- Cardiovascular Medicine
- Clinical Genetics
- Clinical Pharmacology and Therapeutics
- Dermatology
- Endocrinology and Diabetes
- Gastroenterology
- Genito-urinary Medicine
- Geriatric Medicine
- Infectious Diseases
- Medical Oncology
- Medical Toxicology
- Pain Medicine
- Palliative Medicine
- Rehabilitation Medicine
- Respiratory Medicine and Pulmonology
- Rheumatology
- Sleep Medicine
- Sports and Exercise Medicine
- Clinical Neuroscience
- Community Medical Services
- Critical Care
- Emergency Medicine
- Forensic Medicine
- Haematology
- History of Medicine
- Browse content in Medical Dentistry
- Oral and Maxillofacial Surgery
- Paediatric Dentistry
- Restorative Dentistry and Orthodontics
- Surgical Dentistry
- Medical Ethics
- Browse content in Medical Skills
- Clinical Skills
- Communication Skills
- Nursing Skills
- Surgical Skills
- Medical Statistics and Methodology
- Browse content in Neurology
- Clinical Neurophysiology
- Neuropathology
- Nursing Studies
- Browse content in Obstetrics and Gynaecology
- Gynaecology
- Occupational Medicine
- Ophthalmology
- Otolaryngology (ENT)
- Browse content in Paediatrics
- Neonatology
- Browse content in Pathology
- Chemical Pathology
- Clinical Cytogenetics and Molecular Genetics
- Histopathology
- Medical Microbiology and Virology
- Patient Education and Information
- Browse content in Pharmacology
- Psychopharmacology
- Browse content in Popular Health
- Caring for Others
- Complementary and Alternative Medicine
- Self-help and Personal Development
- Browse content in Preclinical Medicine
- Cell Biology
- Molecular Biology and Genetics
- Reproduction, Growth and Development
- Primary Care
- Professional Development in Medicine
- Browse content in Psychiatry
- Addiction Medicine
- Child and Adolescent Psychiatry
- Forensic Psychiatry
- Learning Disabilities
- Old Age Psychiatry
- Psychotherapy
- Browse content in Public Health and Epidemiology
- Epidemiology
- Public Health
- Browse content in Radiology
- Clinical Radiology
- Interventional Radiology
- Nuclear Medicine
- Radiation Oncology
- Reproductive Medicine
- Browse content in Surgery
- Cardiothoracic Surgery
- Gastro-intestinal and Colorectal Surgery
- General Surgery
- Neurosurgery
- Paediatric Surgery
- Peri-operative Care
- Plastic and Reconstructive Surgery
- Surgical Oncology
- Transplant Surgery
- Trauma and Orthopaedic Surgery
- Vascular Surgery
- Browse content in Science and Mathematics
- Browse content in Biological Sciences
- Aquatic Biology
- Biochemistry
- Bioinformatics and Computational Biology
- Developmental Biology
- Ecology and Conservation
- Evolutionary Biology
- Genetics and Genomics
- Microbiology
- Molecular and Cell Biology
- Natural History
- Plant Sciences and Forestry
- Research Methods in Life Sciences
- Structural Biology
- Systems Biology
- Zoology and Animal Sciences
- Browse content in Chemistry
- Analytical Chemistry
- Computational Chemistry
- Crystallography
- Environmental Chemistry
- Industrial Chemistry
- Inorganic Chemistry
- Materials Chemistry
- Medicinal Chemistry
- Mineralogy and Gems
- Organic Chemistry
- Physical Chemistry
- Polymer Chemistry
- Study and Communication Skills in Chemistry
- Theoretical Chemistry
- Browse content in Computer Science
- Artificial Intelligence
- Computer Architecture and Logic Design
- Game Studies
- Human-Computer Interaction
- Mathematical Theory of Computation
- Programming Languages
- Software Engineering
- Systems Analysis and Design
- Virtual Reality
- Browse content in Computing
- Business Applications
- Computer Security
- Computer Games
- Computer Networking and Communications
- Digital Lifestyle
- Graphical and Digital Media Applications
- Operating Systems
- Browse content in Earth Sciences and Geography
- Atmospheric Sciences
- Environmental Geography
- Geology and the Lithosphere
- Maps and Map-making
- Meteorology and Climatology
- Oceanography and Hydrology
- Palaeontology
- Physical Geography and Topography
- Regional Geography
- Soil Science
- Urban Geography
- Browse content in Engineering and Technology
- Agriculture and Farming
- Biological Engineering
- Civil Engineering, Surveying, and Building
- Electronics and Communications Engineering
- Energy Technology
- Engineering (General)
- Environmental Science, Engineering, and Technology
- History of Engineering and Technology
- Mechanical Engineering and Materials
- Technology of Industrial Chemistry
- Transport Technology and Trades
- Browse content in Environmental Science
- Applied Ecology (Environmental Science)
- Conservation of the Environment (Environmental Science)
- Environmental Sustainability
- Environmentalist Thought and Ideology (Environmental Science)
- Management of Land and Natural Resources (Environmental Science)
- Natural Disasters (Environmental Science)
- Nuclear Issues (Environmental Science)
- Pollution and Threats to the Environment (Environmental Science)
- Social Impact of Environmental Issues (Environmental Science)
- History of Science and Technology
- Browse content in Materials Science
- Ceramics and Glasses
- Composite Materials
- Metals, Alloying, and Corrosion
- Nanotechnology
- Browse content in Mathematics
- Applied Mathematics
- Biomathematics and Statistics
- History of Mathematics
- Mathematical Education
- Mathematical Finance
- Mathematical Analysis
- Numerical and Computational Mathematics
- Probability and Statistics
- Pure Mathematics
- Browse content in Neuroscience
- Cognition and Behavioural Neuroscience
- Development of the Nervous System
- Disorders of the Nervous System
- History of Neuroscience
- Invertebrate Neurobiology
- Molecular and Cellular Systems
- Neuroendocrinology and Autonomic Nervous System
- Neuroscientific Techniques
- Sensory and Motor Systems
- Browse content in Physics
- Astronomy and Astrophysics
- Atomic, Molecular, and Optical Physics
- Biological and Medical Physics
- Classical Mechanics
- Computational Physics
- Condensed Matter Physics
- Electromagnetism, Optics, and Acoustics
- History of Physics
- Mathematical and Statistical Physics
- Measurement Science
- Nuclear Physics
- Particles and Fields
- Plasma Physics
- Quantum Physics
- Relativity and Gravitation
- Semiconductor and Mesoscopic Physics
- Browse content in Psychology
- Affective Sciences
- Clinical Psychology
- Cognitive Neuroscience
- Cognitive Psychology
- Criminal and Forensic Psychology
- Developmental Psychology
- Educational Psychology
- Evolutionary Psychology
- Health Psychology
- History and Systems in Psychology
- Music Psychology
- Neuropsychology
- Organizational Psychology
- Psychological Assessment and Testing
- Psychology of Human-Technology Interaction
- Psychology Professional Development and Training
- Research Methods in Psychology
- Social Psychology
- Browse content in Social Sciences
- Browse content in Anthropology
- Anthropology of Religion
- Human Evolution
- Medical Anthropology
- Physical Anthropology
- Regional Anthropology
- Social and Cultural Anthropology
- Theory and Practice of Anthropology
- Browse content in Business and Management
- Business Strategy
- Business History
- Business Ethics
- Business and Government
- Business and Technology
- Business and the Environment
- Comparative Management
- Corporate Governance
- Corporate Social Responsibility
- Entrepreneurship
- Health Management
- Human Resource Management
- Industrial and Employment Relations
- Industry Studies
- Information and Communication Technologies
- International Business
- Knowledge Management
- Management and Management Techniques
- Operations Management
- Organizational Theory and Behaviour
- Pensions and Pension Management
- Public and Nonprofit Management
- Strategic Management
- Supply Chain Management
- Browse content in Criminology and Criminal Justice
- Criminal Justice
- Criminology
- Forms of Crime
- International and Comparative Criminology
- Youth Violence and Juvenile Justice
- Development Studies
- Browse content in Economics
- Agricultural, Environmental, and Natural Resource Economics
- Asian Economics
- Behavioural Finance
- Behavioural Economics and Neuroeconomics
- Econometrics and Mathematical Economics
- Economic Systems
- Economic Methodology
- Economic History
- Economic Development and Growth
- Financial Markets
- Financial Institutions and Services
- General Economics and Teaching
- Health, Education, and Welfare
- History of Economic Thought
- International Economics
- Labour and Demographic Economics
- Law and Economics
- Macroeconomics and Monetary Economics
- Microeconomics
- Public Economics
- Urban, Rural, and Regional Economics
- Welfare Economics
- Browse content in Education
- Adult Education and Continuous Learning
- Care and Counselling of Students
- Early Childhood and Elementary Education
- Educational Equipment and Technology
- Educational Strategies and Policy
- Higher and Further Education
- Organization and Management of Education
- Philosophy and Theory of Education
- Schools Studies
- Secondary Education
- Teaching of a Specific Subject
- Teaching of Specific Groups and Special Educational Needs
- Teaching Skills and Techniques
- Browse content in Environment
- Applied Ecology (Social Science)
- Climate Change
- Conservation of the Environment (Social Science)
- Environmentalist Thought and Ideology (Social Science)
- Natural Disasters (Environment)
- Social Impact of Environmental Issues (Social Science)
- Browse content in Human Geography
- Cultural Geography
- Economic Geography
- Political Geography
- Browse content in Interdisciplinary Studies
- Communication Studies
- Museums, Libraries, and Information Sciences
- Browse content in Politics
- African Politics
- Asian Politics
- Chinese Politics
- Comparative Politics
- Conflict Politics
- Elections and Electoral Studies
- Environmental Politics
- European Union
- Foreign Policy
- Gender and Politics
- Human Rights and Politics
- Indian Politics
- International Relations
- International Organization (Politics)
- International Political Economy
- Irish Politics
- Latin American Politics
- Middle Eastern Politics
- Political Methodology
- Political Communication
- Political Philosophy
- Political Sociology
- Political Theory
- Political Behaviour
- Political Economy
- Political Institutions
- Politics and Law
- Public Administration
- Public Policy
- Quantitative Political Methodology
- Regional Political Studies
- Russian Politics
- Security Studies
- State and Local Government
- UK Politics
- US Politics
- Browse content in Regional and Area Studies
- African Studies
- Asian Studies
- East Asian Studies
- Japanese Studies
- Latin American Studies
- Middle Eastern Studies
- Native American Studies
- Scottish Studies
- Browse content in Research and Information
- Research Methods
- Browse content in Social Work
- Addictions and Substance Misuse
- Adoption and Fostering
- Care of the Elderly
- Child and Adolescent Social Work
- Couple and Family Social Work
- Developmental and Physical Disabilities Social Work
- Direct Practice and Clinical Social Work
- Emergency Services
- Human Behaviour and the Social Environment
- International and Global Issues in Social Work
- Mental and Behavioural Health
- Social Justice and Human Rights
- Social Policy and Advocacy
- Social Work and Crime and Justice
- Social Work Macro Practice
- Social Work Practice Settings
- Social Work Research and Evidence-based Practice
- Welfare and Benefit Systems
- Browse content in Sociology
- Childhood Studies
- Community Development
- Comparative and Historical Sociology
- Economic Sociology
- Gender and Sexuality
- Gerontology and Ageing
- Health, Illness, and Medicine
- Marriage and the Family
- Migration Studies
- Occupations, Professions, and Work
- Organizations
- Population and Demography
- Race and Ethnicity
- Social Theory
- Social Movements and Social Change
- Social Research and Statistics
- Social Stratification, Inequality, and Mobility
- Sociology of Religion
- Sociology of Education
- Sport and Leisure
- Urban and Rural Studies
- Browse content in Warfare and Defence
- Defence Strategy, Planning, and Research
- Land Forces and Warfare
- Military Administration
- Military Life and Institutions
- Naval Forces and Warfare
- Other Warfare and Defence Issues
- Peace Studies and Conflict Resolution
- Weapons and Equipment

- < Previous chapter
- Next chapter >
2 Tasks, Task Sets, and the Mapping Between Them
- Published: June 2014
- Cite Icon Cite
- Permissions Icon Permissions
This chapter asks what actually constitutes a task. It has long been suggested that task switching requires implementing a task set, but the nature of the task set itself often goes unspecified. How can we tell whether task set reconfiguration has occurred if we are not clear what a task set really is? It is argued here that task switching research—and its associated theorizing—has been hampered by poor definitions of what are tasks and task sets. The chapter provides a distinction between the two: a task is the representation of a set of instructions required to perform an activity accurately; a task set is the set of representations and processes that enable execution of the task. It argues that contrary to the often-held assumption that switching between two tasks in a task switching paradigm requires switching between two task sets, tasks and task sets do not necessarily have a one-to-one mapping. It provides recommendations for how to explore the nature of the relationship between tasks and task sets.
Signed in as
Institutional accounts.
- Google Scholar Indexing
- GoogleCrawler [DO NOT DELETE]
Personal account
- Sign in with email/username & password
- Get email alerts
- Save searches
- Purchase content
- Activate your purchase/trial code
Institutional access
- Sign in with a library card Sign in with username/password Recommend to your librarian
- Institutional account management
- Get help with access
Access to content on Oxford Academic is often provided through institutional subscriptions and purchases. If you are a member of an institution with an active account, you may be able to access content in one of the following ways:
IP based access
Typically, access is provided across an institutional network to a range of IP addresses. This authentication occurs automatically, and it is not possible to sign out of an IP authenticated account.
Sign in through your institution
Choose this option to get remote access when outside your institution. Shibboleth/Open Athens technology is used to provide single sign-on between your institution’s website and Oxford Academic.
- Click Sign in through your institution.
- Select your institution from the list provided, which will take you to your institution's website to sign in.
- When on the institution site, please use the credentials provided by your institution. Do not use an Oxford Academic personal account.
- Following successful sign in, you will be returned to Oxford Academic.
If your institution is not listed or you cannot sign in to your institution’s website, please contact your librarian or administrator.
Sign in with a library card
Enter your library card number to sign in. If you cannot sign in, please contact your librarian.
Society Members
Society member access to a journal is achieved in one of the following ways:
Sign in through society site
Many societies offer single sign-on between the society website and Oxford Academic. If you see ‘Sign in through society site’ in the sign in pane within a journal:
- Click Sign in through society site.
- When on the society site, please use the credentials provided by that society. Do not use an Oxford Academic personal account.
If you do not have a society account or have forgotten your username or password, please contact your society.
Sign in using a personal account
Some societies use Oxford Academic personal accounts to provide access to their members. See below.
A personal account can be used to get email alerts, save searches, purchase content, and activate subscriptions.
Some societies use Oxford Academic personal accounts to provide access to their members.
Viewing your signed in accounts
Click the account icon in the top right to:
- View your signed in personal account and access account management features.
- View the institutional accounts that are providing access.
Signed in but can't access content
Oxford Academic is home to a wide variety of products. The institutional subscription may not cover the content that you are trying to access. If you believe you should have access to that content, please contact your librarian.
For librarians and administrators, your personal account also provides access to institutional account management. Here you will find options to view and activate subscriptions, manage institutional settings and access options, access usage statistics, and more.
Our books are available by subscription or purchase to libraries and institutions.
- About Oxford Academic
- Publish journals with us
- University press partners
- What we publish
- New features
- Open access
- Rights and permissions
- Accessibility
- Advertising
- Media enquiries
- Oxford University Press
- Oxford Languages
- University of Oxford
Oxford University Press is a department of the University of Oxford. It furthers the University's objective of excellence in research, scholarship, and education by publishing worldwide
- Copyright © 2024 Oxford University Press
- Cookie settings
- Cookie policy
- Privacy policy
- Legal notice
This Feature Is Available To Subscribers Only
Sign In or Create an Account
This PDF is available to Subscribers Only
For full access to this pdf, sign in to an existing account, or purchase an annual subscription.

An official website of the United States government
The .gov means it’s official. Federal government websites often end in .gov or .mil. Before sharing sensitive information, make sure you’re on a federal government site.
The site is secure. The https:// ensures that you are connecting to the official website and that any information you provide is encrypted and transmitted securely.
- Publications
- Account settings
Preview improvements coming to the PMC website in October 2024. Learn More or Try it out now .
- Advanced Search
- Journal List
- Front Hum Neurosci
Task representation in individual and joint settings
Wolfgang prinz.
Department of Psychology, Max Planck Institute for Human Cognitive and Brain Sciences, Leipzig, Germany
This paper outlines a framework for task representation and discusses applications to interference tasks in individual and joint settings. The framework is derived from the Theory of Event Coding (TEC). This theory regards task sets as transient assemblies of event codes in which stimulus and response codes interact and shape each other in particular ways. On the one hand, stimulus and response codes compete with each other within their respective subsets (horizontal interactions). On the other hand, stimulus and response code cooperate with each other (vertical interactions). Code interactions instantiating competition and cooperation apply to two time scales: on-line performance (i.e., doing the task) and off-line implementation (i.e., setting the task). Interference arises when stimulus and response codes overlap in features that are irrelevant for stimulus identification, but relevant for response selection. To resolve this dilemma, the feature profiles of event codes may become restructured in various ways. The framework is applied to three kinds of interference paradigms. Special emphasis is given to joint settings where tasks are shared between two participants. Major conclusions derived from these applications include: (1) Response competition is the chief driver of interference. Likewise, different modes of response competition give rise to different patterns of interference; (2) The type of features in which stimulus and response codes overlap is also a crucial factor. Different types of such features give likewise rise to different patterns of interference; and (3) Task sets for joint settings conflate intraindividual conflicts between responses (what), with interindividual conflicts between responding agents (whom). Features of response codes may, therefore, not only address responses, but also responding agents (both physically and socially).
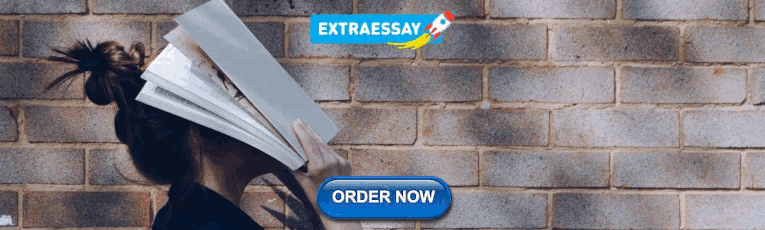
Tasks as Targets of Research
Experimental research in cognition and action relies on the study of performance in controlled environmental settings. Reaction time tasks provide a typical example of the way in which this research is conducted. The mentioned tasks require participants to perform certain actions in response to certain events, thereby following certain rules they have been instructed to follow (or, in somewhat more technical terms, to select and perform speeded responses to upcoming stimuli according to pre-specified mapping rules ). Task performance is assessed in terms of reaction time, that is, the time that elapses between stimulus presentation and response onset. Observed relationships between experimental conditions and task performance then lay the ground for theory building. Theories specify representational architectures and functional processing mechanisms that may underlie (and hence explain) the empirical observations reflecting these relationships.
In this approach tasks are tools, not targets of research. Tasks are understood as means for providing the information that theory requires, without being studied as ends in themselves. For instance, to run a memory experiment it is taken for granted that participants understand the instructions and implement an appropriate task set , that is a cognitive representation of the task, and that subserves both implementing the task and performing it. Researchers take the operation of task sets for granted in the same way that tailors take the workings of scissors and sewing machines for granted. Just as tailors use these tools for making garments and robes, researchers use tasks as tools for building theories. These theories address cognitive architectures and mechanisms, but not the tasks used to probe them. As long as the tools that researchers use are instrumental for attaining the desired targets, they can afford to be ignorant about their technical functionality.
While it is, on the one hand, perfectly justified to use tasks as tools for theory-driven research, it is, on the other hand, certainly no less legitimate to address tasks as targets of theory-driven research. This is what the present paper aims at. Importantly, theories of task representation and control will not only shed light on the workings of research tools in experimental settings, but also elucidate a central feature of human performance, viz. the interaction between bottom-up and top-down control of cognition and action. Tasks require us to pursue goals and perform goal-directed actions under appropriate circumstances. Thus, in a sense, we may see ourselves engaging in tasks all the time in everyday life. From this perspective research tools for experimental settings may be seen to instantiate a constituitive feature of action control in more natural settings. Understanding one will therefore translate into understanding the other.
More than a century ago Ach ( 1905 ) pioneered an approach to the representational underpinnings of task control. In his studies of simple arithmetic tasks the main focus was the issue of how a task’s goal (e.g., to multiply two digits) is represented besides the material objects to which it applies (i.e., the digits presented). In his theoretical account he introduced the famous notion of determining tendencies that were meant to instantiate intended goals, on top of reproductive tendencies meant to instantiate represented objects. Ach’s analysis was based on both, subjective experience (i.e., introspection) and objective performance (i.e., measurements). In the meantime reliance on subjective experience has, for a number of good reasons, become much weaker than reliance on objective performance. One of the classical instruments for objective assessment of task performance is provided by reaction time tasks, on which I will focus here. Typical issues that come to mind when we raise these tasks from tools to targets of enquiry include questions such as: How can we characterize task sets? How can they be acquired either through learning or verbal instruction? Which kinds of representational resources does their implementation require? How are stimuli and responses represented? How can mapping rules be instantiated in task sets? What does it take to move from representation to control, that is, from understanding a task to carrying it out?
The last two decades have witnessed the emergence of a wealth of literature on issues of cognitive control in reaction time tasks (Kiesel et al., 2010 ; Koch et al., 2010 ; Logan et al., 2014 ; Verbruggen et al., 2014 ). The main bulk of this literature is based on paradigms for the study of flexibility in task representation and control (e.g., in tool use, task switching and stop signal paradigms). Accordingly, the theoretical focus is on short-term changes within and between task sets, rather than structural and functional organization of task sets per se . While these paradigms study in some detail how task sets persist and change over time, they pay less attention to their microstructure, that is, the way in which the event representations involved in a given task set interact with each other and become shaped by the demands of the task at hand.
In this paper I argue for extending this approach. My aim is twofold. The first aim is to outline a framework for task set representation that addresses both, mapping relations between and content profiles within representations. Essentially, the framework claims that the implementation of a given task set does not only act to establish appropriate mappings ( between representations of pertinent events like stimuli, responding actions and action effects), but also appropriate feature profiles ( within event representations). In fact, it even argues that mappings between representations are instantiated through overlap of their feature profiles. The framework is derived from Ideomotor Theory and the Theory of Event Coding (TEC). Both view stimuli, responses and response outcomes as commensurate events that share a common representational domain (Prinz, 1987 , 1990 , 1997 ; Hommel et al., 2001 ; Prinz et al., 2009 ; Memelink and Hommel, 2013 ).
The second aim is to apply this framework to scenarios in which the task is embedded in a minimal social context. To pursue this aim, we will study experimental settings in which a reaction time task is shared between two participants (namely, task sharing settings). Findings from a number of recent studies have been understood to suggest that in such settings participants do not only represent their own share of the task, but also co-represent their partner’s share—even if that share is entirely irrelevant for their own performance (Sebanz et al., 2003 , 2005 , 2006 ). For instance, when a Simon task is divided between two participants (so that each takes care of one of the two relevant stimulus features and one of the two response key assigned to it), a spatial compatibility effect is observed for each of them—as in the standard version of the task in which a single participant takes care of both stimulus features and their assigned response keys. In contrast, no such effect is obtained when participants perform their task share alone, that is, without another being present. It has therefore been suggested that sharing a task with another invites implementing the full task; representing one’s own share and co-representing one’s partner’s share (Sebanz et al., 2005 ; Atmaca et al., 2008 ; Sebanz and Knoblich, 2009 ). This is where the second aim comes in; to find out what the role of co-representation can be in a broader framework for task representation.
The following discussion will take two major steps. The first step will outline a general framework for task representation, focusing on ways in which task demands shape representational content. The second step will then apply the logic of the framework to various kinds of interference paradigms, thereby gradually moving from unshared to shared settings. As we will see, the notion of co-representation will be lost in doing so, suggesting the radical conclusion that we don’t need it at all (Dolk et al., 2011 , 2013 ; Dolk and Prinz, in press ; Wenke et al., 2011 ).
A Framework for Task Representation
What does it take to carry out a reaction time task? Essentially, we may discern two levels of task description, external/observational and internal/theoretical. Adopting an external point of view, we may observe certain kinds of events taking place in the experimental setting. Typically, we observe an individual generating certain actions in response to certain stimuli presented to them, thereby following certain rules to which they have committed themselves. For instance, in the Simon task the individual may map red vs. green stimulus patches appearing on the screen to pressing left vs. right response keys mounted on the table, based on rules provided by preceding instructions.
Conversely, adopting an internal point of view, we may address the putative cognitive architecture whose structural and functional features are meant to account for observed performance. Typically, we invoke an architecture harboring stimulus representations, response representations, and linkages established between them. Stimulus representations are understood as internal placeholders for external stimulus events, generated and maintained on the sensory input side of the architecture. Response representations are understood as internal placeholders for external response events, generated and maintained on the motor output side. Finally, linkages between stimulus and response representations are understood as internal placeholders for the stimulus-to-response mappings required by the instruction.
Even though the internal description does not provide much more than an internalized version of the external account, it has over the past decades made its career as a fairly successful theoretical framework for the analysis of cognitive task performance. Building on early pioneers, for example, Broadbent, Posner, Sanders, and Welford, it has meanwhile reached the status of classical textbook wisdom (e.g., Broadbent, 1958 ; Welford, 1968 , 1980 ; Sternberg, 1969 ; Posner, 1986 ; Sanders, 1998 ). Nonetheless, it has not gone unchallenged. The critical challenge comes from observations on so-called privileged linkages between perception and action and related theoretical claims for a functional role of similarity in the operations subserving these linkages (Prinz, 1990 , 1997 ; Prinz and Hommel, 2002 ). The crucial observation here is that stimulus events can often more easily be linked to response events resembling them, as compared to non-resembling ones. For instance, in the Simon task a stimulus patch on the left side of the screen can be more easily and more efficiently linked to a key press response on the left side, as compared to the right side. The same holds true not only for a number of further instances of stimulus-response compatibility effects (e.g., Fitts and Seeger, 1953 ; Fitts and Deininger, 1954 ; Alluisi and Warm, 1990 ; Kornblum et al., 1990 ; Reeve and Proctor, 1990 ) but also for instances of action induction and action imitation (Lotze, 1852 ; Carpenter, 1874 ; Piaget, 1945/2013 ; Liberman et al., 1967 ; Meltzoff and Moore, 1977 ; Knuf et al., 2001 ; Prinz et al., 2005 ; for overviews cf., e.g., Prinz, 1987 , 1990 ; Meltzoff and Prinz, 2002 ; Hurley and Chater, 2005a , b ).
The classical framework has no obvious way to account for such similarity-based linkages between perception and action. This is because it regards linkages between input and output as arbitrary connections between incommensurate representations—incommensurate in the sense that they do not share any features on which the operation of similarity can be established. This is where the TEC and the notion of Common Coding comes into place (Prinz, 1990 , 1997 ; Hommel et al., 2001 ). They suggest extending the classical framework in a way that, atop of arbitrary linkages between incommensurate representations, also permits similarity-based linkages between commensurate representations.
Common Coding invokes an architecture of two parallel systems mediating between perception and action: a primary system for linking incommensurate sensory and motor representations, and a secondary one for linking commensurate cognitive representations of input events (stimuli) and output events (responses). While the primary system relies on arbitrary, contiguity-based linkages between input- and output- representations, the secondary system also allows for non-arbitrary, similarity-based linkages ( mapping vs. matching between input and output representations).
With these extensions in mind, let us now see how we can apply basic notions of Event Code Theory to issues of task representation and task set formation. In this section we discuss some basic claims concerning structure and function of the required representational machinery. The first, perhaps most important claim addresses the functional locus of that machinery. We posit that flexible cognitive task performance is mainly, if not entirely, organized by the secondary system. Unlike the primary sensory-motor system whose representational structure relies on long-term sensory and motor experience, the secondary, cognitive system is flexible enough to allow for creating short-lived and task-specific representational structures (task sets) and, at the same time, penetrable and transparent enough to allow for these structures being shaped and modulated through verbal communication (instructions) . Accordingly, our discussion will focus on ways in which the secondary system subserves task representation and performance.
Event Codes
The Theory of Event Codes (TEC) offers a framework for characterizing the basic structure of event representation in the secondary system (Prinz, 1990 ; Hommel et al., 2001 ). TEC views task sets as assemblies of event codes that are organized in specific ways. Thus, in order to understand how these assemblies are organized and how they work, we first need to understand what event codes are and how they interact with each other.
Representational function— The basic idea is simple (and perhaps trivial). Event codes are cognitive representations of a variety of things and events that an individual’s mental activity may address. Importantly, event codes may be placeholders for things and events in different modalities of existence (and even non-existence): things that happen , or ought to happen , in the past, present and future (and even those that do not happen ).
For the present discussion we take the workings of event codes for granted, without addressing the intricate issue as to how they may be generated and individuated from the continuous flow of information to which individuals are exposed. While this issue may be difficult to solve for natural scenarios, we may set it aside when discussing experimental settings. Such settings are actually designed in terms of well-defined things and events, so that we may assume that participants generate task-specific assemblies of event codes whose structure mirrors the structure of events that actually happen in these settings. While some of these events are explicitly mentioned in the instruction, others are introduced in other ways that give rise to the formation of pertinent codes. Accordingly, while we do not offer a detailed account of how these codes are generated, we do maintain that assemblies of task-specific event codes reflect the basic structure of task-specific events.
To fulfill their representational function, event codes need to be equal and mutually commensurate, even if the things and events they represent are incommensurate. How can this be possible? For instance, how can such diverse things like external stimulus events that are controlled and generated by the experimenter (e.g., a color patch appearing on the screen) and internal response events that are controlled and generated by the participant (e.g., her hand pressing a response key) be represented in a common format? As has been argued elsewhere (Prinz, 1992 ; Hommel et al., 2001 ; Memelink and Hommel, 2005 , 2006 , 2013 ; Prinz et al., 2009 ), distal reference is the key feature here. Event codes are commensurate in virtue of referring to distal events in the world and body. They are, in other words, placeholders for external events outside the representing system, but not for internal events that instantiate their representational function in that system (be it at the proximal level of sensors/effectors or the central level of the brain).
Structure— A convenient way of conceiving the structure of event codes is to regard them as feature compounds in semantic space (Hommel, 1997 , 2009 , 2013 ; Hommel et al., 2001 ). According to this view, event codes are instantiated in local, transient feature networks that are generated from resources provided by a global, permanent space of semantic features. For instance, a color patch on a screen may be represented by a compound of features, for example, red, round, small, at-top-of-screen, on-left-hand-side , etc. The formation of event codes thus requires and presupposes the existence of a global space of semantic features that provides the building blocks for their making. Notably, this space is semantic in the weak sense of codes being grounded in sensory and motor activity, not in the strong sense of codes serving as symbolic placeholders for external events.
Taking these building blocks for granted, we might examine how event codes are formed from them. To this end we outline their elementary structure and discuss ways in which they may change over time. A basic outline is depicted in Figure Figure1A. 1A . It shows a hypothetical feature compound which is characterized (i) through a particular set of features involved in it; and (ii) a particular pattern of connectivity interlinking them. It goes without saying that this sketch must be understood as an abstract, highly impoverished illustration of the underlying idea. The complexity of true event codes will exceed that of the sketch by several orders of magnitude (in terms of both, number of features and complexity of connectivity).

(A) Feature compound with equal weights; (B) Same compound with different weights (indicated by node size); (C) Same compound with one of the original features deleted (dotted) and a novel feature included (black).
At this point it should not go unnoticed what the sketch fails to show. It fails to show that the feature compounds instantiating event codes are local structures embedded in a global space. This embeddedness has important implications. In the same way as any given code comprises a number of features, any given feature contributes to a number of codes. As a result event codes may overlap with respect to their features. As we will discuss below, such feature overlap plays a crucial role for interactions between event codes in task set formation.
A structure such as the compound depicted in Figure Figure1A 1A can undergo various kinds of changes (Memelink and Hommel, 2013 ). While some such changes may pertain to the pattern of connectivity among given features, others, on which we focus here, pertain to the profile of their contributions to the compound. So far we have described in inclusion of features in event codes as an all-or-nothing affair. Figure Figure1B 1B shows a somewhat more realistic picture, assuming that features may differ in their relative contributions to a given code. While some features may be defining and essential, others may be more or less an accessory for representating an event. For instance, the color of the patch on the screen may be crucial to the task at hand whereas its shape may be irrelevant. Accordingly, when characteristics of things and events become relevant or irrelevant in particular settings, this will alter the relative weights with which corresponding features contribute to the event codes representing them. The pattern of feature weights thus specifies what we may call the weight profile of an event code. Below, we discuss ways in which that profile may become modulated by task context.
Restructuring weight profiles may be regarded as a weak way of altering the content of feature compounds. A more effective way is to alter feature profiles themselves, that is, to include new and/or delete old features altogether, as illustrated in Figure Figure1C. 1C . In one sense these alterations may also be considered weight changes (viz., down to zero and up to a value above zero, respectively). Yet, in another sense they have deeper implications than just weight alterations. This is because they furnish feature compounds with an enormous degree of structural flexibility; the capacity to grow and shrink on demand. As we will see below, this capacity turns out to be of utmost importance for tailoring the content of event codes to the demands of the task at hand.
Interactions— If feature weights and profiles can be modulated that way—what factors are modulating them? The basic claim here is that the structure of code profiles at a given time is mainly (if not entirely) determined by the history of interactions between these codes. According to this view, any event code that is involved in the representation of a given scenario becomes selectively tuned to the needs of efficient interaction with other codes involved in the same scenario.
Consider for illustration a simplified scheme with two intersecting and interacting event codes (Figure (Figure2A). 2A ). Each code exhibits both shared and unshared features. While shared features are common to both codes (and, hence, non-distinctive), unshared features are specific for each of them (and, hence, distinctive). Depending on the interaction in which the two codes are involved, such overlap may either be beneficial or detrimental. Overlap should be beneficial in scenarios requiring code cooperation, i.e., that both are jointly activated at the same time. In contrast, overlap should be detrimental in scenarios requiring code competition, i.e., that only one of them is activated (and the other silenced) at the same time. While cooperation requires that activating one code partially co-activates the other, competition requires that one must be shielded from being co-activated by the other.

Two overlapping event codes, instantiated as intersecting feature compounds (C1 and C2 with red and blue edges, respectively) . Overlap is indicated by feature nodes on which red and blue edges converge (= shared features). (A) Initial scheme with equal feature weights. (B–E) Possible structural changes under conditions of code cooperation (B,C) and code competition (D,E) . (B) Strengthening shared features through (i) increasing weights of old features (node size); and (ii) including new features that are shared (black); (C) Weakening unshared features through weight reduction and deletion (dotted); (D) Weakening shared features through weight reduction and deletion; (E) Strengthening unshared features through weight increase and inclusion of novel features.
The scheme depicted in Figure Figure2A 2A invites obvious measures for improving the efficiency of both cooperation and competition. These measures rely on altering the structure of code profiles according to given requirements. Measures supporting cooperation are shown in Figures 2B,C . They may pertain to the overlap zone (strengthening weights and creating new features that are shared) or to non-overlap zones (weakening weights and deleting unshared features). Likewise, measures supporting competition are shown in Figures 2D,E . Again, they may pertain to the overlap zone (weakening weights and deleting shared features) or to non-overlap zones (strengthening weights and creating novel, unshared features).
In sum, we see two basic factors at work in tailoring profiles of feature assemblies to context conditions: initial pattern of overlap (shared vs. unshared features) and required mode of interaction (cooperation vs. competition). We claim that these two factors enter learning algorithms for optimizing the efficiency of event codes for processing under given conditions of overlap and interaction. As we will see in the subsequent section, these algorithms play an important role as tools for the formation of task sets. To understand this role we do not need to specify how they work in detail. The only thing we need to accept is that they exist and perform their function.
The application of these ideas to task sets and task set formation is fairly straightforward. As indicated above, the concept of task set refers to the representational underpinnings of performance in S-R mapping tasks. Our framework claims that event codes provide the basic equipment from which task sets are made. More specifically, it regards task sets as particular kinds of assemblies of such codes. Of these assemblies we demand that they must be organized in ways that allow us to derive basic patterns of task performance from basic patterns of code interaction.
The classical approach to capturing the functional logic of S-R mapping tasks addresses three fundamental sets of task components (cf., e.g., Garner, 1962 , 1974 ; Kornblum et al., 1990 ; Kornblum, 1992 ): stimulus set (the set of possible stimulus events), response set (the set of possible responding actions), and mapping rules (the set of prescriptions for assigning responding actions to stimulus events).
While these components address task structure and off-line implementation, basic operations address task performance and on-line execution: stimulus presentation (presenting an element from the stimulus set) and response selection (selecting an element from the response set). Seen from the participant’s perspective, stimulus presentation is under external control (i.e., the experimenter’s protocol), whereas response selection is under internal control (i.e., the participant’s action decision).
Task implementation and performance are thus both grounded in interactions among event codes that are involved in task sets (summarized under the notion of intentional weighting by Memelink and Hommel, 2013 ). As illustrated in Figure Figure3, 3 , these interactions transform the initial structure of event code assemblies into a final, task-specific structure. In essence, we may discern two basic kinds of such interactions, horizontal and vertical. Both aim at optimizing task set structure and function. At the level of implementation they tailor the content profiles of stimulus and response codes to the demands of the task at hand. At the level of performance they strengthen cooperation and weaken competition.

Interactions between event codes in task set formation. (A) Initial structure of a hypothetical event code assembly, with two partially overlapping stimulus codes (S 1 /S 2 ) and two partially overlapping response codes (R 1 /R 2 ). (B) Final structure of the same assembly, with initial horizontal overlap among stimulus and response codes deleted (unconnected dotted features) and new vertical overlap between stimulus and response codes created. Note that the final structure of the assembly differentiates between wanted and unwanted S-R assignments (wanted: S 1 –R 1 and S 2 –R 2 ; unwanted: S 1 –R 2 and S 2 –R 1 ). To illustrate, consider a task requiring left key presses in response to squares (S 1 →R 1 ) and right key presses in response to circles (S 2 →R 2 ). An efficient task set must, on the one hand, weaken, or delete features that are shared among the competing stimuli and the competing responses (like, e.g., the closedness of the two shapes and the downward-directed push of the two key presses, respectively). On the other hand, it must implement the required mappings by creating overlap between distinctive features of stimuli and responses (such as, e.g., straight lines/left key vs. curved lines/right key ) and creating new functional features instantiating these mappings.
Horizontal Interactions
Primarily, a task requires identification of stimulus events and selection of appropriate actions for response. Thus, when viewed in terms of event codes and event code assemblies, each of these two operations seems to entail the selection of one element from a set of competing elements. In terms of the scheme depicted in Figure Figure3, 3 , such selective competition requires horizontal interactions within stimulus and response codes, respectively. Stimulus identification selects one particular stimulus code from the set of alternative stimulus codes involved in the task. Likewise, response selection selects one particular response code from the set of alternative response codes.
As discussed above, code competition requires structuring the underlying event code assemblies accordingly. An obvious way of achieving this is to strengthen distinctiveness among competing codes through weakening their mutual overlap. As a result of such distinctive tuning, conflict will be reduced and the efficiency of selection will increase. Importantly, this applies to operations, stimulus identification, and response selection (triggered by external presentation and internal decision, respectively).
Vertical Interactions
So far we have not yet addressed the role of mapping rules. Mapping rules are prescriptive. Essentially, they require that activation of particular stimulus codes must be followed by activation of particular response codes. Thus, in order to instatiate these rules, the involved event code assemblies need to establish some kind of selective cooperation between stimulus and response codes. Selective cooperation tunes stimulus codes to assigned actions and/or response codes to assigned stimuli. With reference to the scheme in Figure Figure3, 3 , selective cooperation requires vertical interactions between stimulus and response codes. These interactions may take two complementary forms, aiming at facilitating wanted and impeding unwanted mappings, respectively.
When a task comprises n stimuli and m responses, the total number of possible S-R–mappings equals n × m . Within the total set of possible mappings, instructions introduce a distinction between two subsets: that of wanted, correct mappings and that of unwanted, incorrect mappings. Whilst wanted mappings yield correct responses, unwanted mappings yield errors. Efficient task sets thus require both, to facilitate wanted und impede unwanted mappings.
Wanted mappings— Implementation of wanted mappings requires selective cooperation between particular stimulus and response codes. An obvious way of achieving this is to create selective overlap between the involved codes. As an example, we may once again consider a simple choice task that requires pressing a left vs. right key in response to a red vs. green color patch. A mapping such as this is initially entirely arbitrary. Before the task is administered, linkages between features such as left–red or right–green do not exist. Pertinent event codes are, at this time, entirely disjunct. This is where instructions intervene. Instructions act to create patterns of selective overlap. These zones of overlap instantiate the required mappings between stimulus events and responding actions. Basically, we may imagine two ways of creating such overlap: integration of old features and generation of new ones.
The first option is to integrate given stimulus features into response codes and/or given response features into stimulus codes. As a result, red becomes integrated into the response code for left key presses, and/or left becomes integrated into the stimulus code for red patches. The second option is to generate new features and assign them to stimulus and response codes that are required to cooperate. As a result, the new feature xyz becomes integrated into both the stimulus code for red patches and the response code for left key presses.
At first glance such feature assignments appear to be fairly arbitrary and strange. Yet, we need to remind ourselves that features are freefloaters and not naturally and intrinsically tied to the stimulus or the response domain. Each feature can therefore, at least in principle, become integrated into each kind of code. Furthermore we also need to remind ourselves that features are semantic elements that may not only address physical properties of things and events (such as colors of patches or locations of response keys), but also functional properties (such as locations belonging to given colors or colors belonging to given locations etc.). Such functional features can be seen to represent the fact that the two events are assigned to each other in the current task.
Instructions thus act to create new overlap, either based on old or new features. In virtue of such overlap, codes for red patches will co-activate codes for left key presses and likewise will codes for left key presses co-activate codes for red patches. Of course, this presupposes that the weights of the features that make up for the new overlap are strong enough to warrant such co-activation.
Unwanted Mappings— Nonetheless, creating new overlap is just one side of the coin. The other side pertains to deleting old overlap. While selective generation and strengthening of new overlap aims at facilitating wanted mappings, selective deletion and weakening of initially given, old overlap aims at impeding unwanted mappings.
Selective deletion comes into effect under conditions of unwanted shared features. Under these conditions feature overlap arises between stimulus and response codes that are not assigned to each other by instructions, so that their co-activation may drive wrong, unwanted responses. A typical example is provided by choice tasks with incompatible assignments between stimulus and response events. Consider a task in which tones presented to the left vs. right ear require right vs. left key presses as responses. In a setting such as this the two tones will initially share strong spatial features with the two keys: left and right tones will invite, as it were, left and right responses. However, since task instructions require the reverse assignments, these natural, compatible mappings ( left-left and right-right ) are in conflict with the required incompatible mappings ( left-right and right-left ). Thus, in order to implement an efficient task set, two complementary measures must be taken. Initial, natural overlap needs to be weakened or deleted, and new overlap needs to be generated and strengthened.
In any case, incompatible assignments must be expected to yield poorer performance than compatible assignments. This may rely entirely upon initial overlap, without the need to weaken or strengthen anything. This is in fact what numerous studies have shown. Relative to neutral assignments that exhibit no initial overlap, incompatible assignments yield slower responses and more errors, whereas compatible assignments lead to faster responses and fewer errors (Alluisi and Warm, 1990 ; Hommel et al., 2001 ). Importantly, this is not only true for overlap of spatial and physical features, but also of semantic and symbolic features (Stroop, 1935 ; LeMay and Simon, 1969 ; Hedge and Marsh, 1975 ; Simon et al., 1981 ; Virzi and Egeth, 1985 ; Prinz, 1990 ; MacLeod, 1991 ; Hommel, 1997 ; van Maanen et al., 2009 ). We may, therefore, conclude that our framework is in line with these basic findings. It accounts for both the detrimental effects of incompatibility and the beneficial effects of compatibility.
Event code theory views task sets as event code assemblies in which event codes from two different sources interact and shape each other: stimulus codes, generated from external sources and response codes generated from internal sources. In each trial the task requires identification of the given stimulus and selection of the required response. On the one hand, stimulus codes and response codes compete with each other within their respective sets, to the effect of weakening and deleting initial code overlap ( horizontal interactions ). At the same time particular stimuli have to be mapped onto particular responses, which requires cooperation between stimulus and response codes. Selective strengthening of vertical overlap facilitates wanted assignments, whereas selective weakening impedes unwanted assignments ( vertical interactions ).
Importantly, these interactions apply to two time scales: on-line performance and off-line implementation. While performance-related operations apply to performing the task (online execution: identification, mapping, selection), implementation-related operations apply to setting the task (offline learning: shaping code profiles according to task demands).
One way of viewing this framework is in terms of attentional mechanisms. Similar mechanisms have been proposed to account for selective attention. For instance, the idea of code competition and selection is widespread in the attentional literature (e.g., Logan, 1988 , 2002 , 2007 ; Bundesen, 1990 , 1998 ; Desimone and Duncan, 1995 ; Schneider, 2013 ; Rosenbaum, 2014 ). The same applies to the notion of codes as feature compounds whose structure and composition may change on demand (Barsalou, 1999 ; Hommel et al., 2001 ; Memelink and Hommel, 2005 , 2006 ; Hommel, 2010 ). Yet, while the basic logic is very much the same, the functional domain is different. Theories of attention apply these ideas to the domain of perceptual processing, but our framework applies them to the broader domain of interactions between perception and action in task representation. Thus, when viewed from an attentional perspective, our framework can be seen to deal with attentional mechanisms operating in task implementation and performance.
Application to Interference Paradigms
In this section we move on to apply the framework of event code theory to more complex tasks where the number of stimuli exceeds the number of responses mapped to them. In a task scenario such as this the elements of the stimulus set may differ in features that are irrelevant for response selection. For instance, when stimuli are color patches that can either be red or green and, at the same time, either take the shape of a circle or square, the resulting set of possible stimulus events comprises four elements that differ on two feature dimensions, namely color and shape. According to instructions, color may, for instance, be relevant for response selection (i.e., determine the choice between left and right key presses), whereas shape may be irrelevant (or vice versa under reverse instructions).
For a task such as this, our framework leads us to expect (among other things) that relevant features become strengthened in the underlying stimulus codes, whereas irrelevant features become weakened, or even deleted. As a result, response selection should become mainly, or even exclusively, controlled by relevant features. Yet on the other hand, if it appears that irrelevant features cannot be entirely silenced or deleted, their unwanted processing may also impact response selection.
The Logic of Interference
Interference paradigms are designed to provoke such impact. In typical interference tasks stimulus and response codes overlap with respect to features that take two conflicting roles at a time: irrelevant elements in stimulus codes (requiring weakening) and relevant elements in response codes (requiring strengthening). An example is provided by the Simon task (Simon and Rudell, 1967 ; Simon, 1968 , 1969 , 1990 ; Umiltà and Nicoletti, 1990 ). In this task participants are required to select one of two keys, for instance in response to the color of a stimulus patch which may appear on the left- or the right-hand side of the screen. Color is thus a relevant feature of stimulus events, wheras their location on the screen is entirely irrelevant in the sense that it should play no role for response selection at all. Yet on the other hand, the location of the key to be pressed (mounted on the left- or the right - hand side of the table) is the crucial distinctive feature in which the two competing actions differ. The task can therefore be seen to instantiate a conflict between two roles of a feature: one and the same feature (e.g., being located on the right-hand side ) is entirely irrelevant for stimulus identification, but highly relevant, if not indispensable for response competition.
The pronounced interference effect that is regularly observed in this task indicates that participants find it in fact impossible to effectively ignore the location of the color patch. Instead, the effect thus suggests that the required weakening/deletion of the irrevant stimulus feature does not occur. It looks as if the strong role that this feature plays for response selection spills over into stimulus identification.
This dilemma is illustrated in Figure Figure4. 4 . The upper panels show a stimulus code and two competing response codes (e.g., representing a red patch and a left vs. right key press, respectively). Location features in response codes are highlighted, reflecting their importance for response competition. Panel (b) indicates the wanted assignment, indicated by a novel feature that is shared by the stimulus code and the assigned response codes (e.g., instantiating the prescription to press the left key in response to a red patch).

Event code assemblies involved in the Simon task. (A/B) Control condition (where stimulus location is constant across trials) (A) Initial assembly of non-intersecting stimulus and response codes (stimulus code S and two competing response codes, R 1 and R 2 , with location features L/R highlighted). (B) New overlap between S and R 1 instantiates the required mapping. (C,D) Interference condition (where stimulus location varies across trials) (C) Compatible trial; stimulus location (L) supports the required response. (D) Incompatible trial; stimulus location (R) supports the opposite response. See text for further explanation.
While the upper panels address trials in which the stimulus is presented in a neutral location, the lower two address compatible vs. incompatible trials in which stimuli occur in locations that do or do not correspond to response locations, respectively. Since location features exhibit high weights (arising from response competition), they become automatically (and unavoidably) integrated into pertinent stimulus codes. This will either strengthen the activation of the wanted response or induce activation of the competing unwanted response (in the compatible and the incompatible case, respectively). Relative to neutral trials, response conflict is thus reduced on compatible trials and increased on incompatible trials.
Accordingly, the crucial factor driving the Simon effect derives from a dilemma inherent in the requirement of forming efficient task sets. Efficient response competition requires strong weights for features in which competing responses differ. At the same time efficient stimulus identification requires weak weights for features that are irrelevant.
As long as the features to which these conflicting requirements pertain are different (e.g., stimulus shapes and response locations ), no conflict will arise. However, conflict will become unavoidable, when they are the same (e.g., stimulus locations and response locations ). Stimulus- and response codes will then overlap with respect to theses features and the requirement of strengthening their weights for one code will counteract that of weakening their weights for the other code. In particular, a strong role that a feature plays for the formation of efficient response codes will automatically strengthen its role for stimulus code formation, thus preventing it from becoming weakened or deleted, as optimal task set efficiency would require. As a result, stimulus identification will interfere with response selection.
To examine the explanatory power of this simple principle we now move on to study a variety of interference tasks. The tasks that are included in our examination differ in two major respects: mode of response competition and type of overlapping features . Event code theory posits that these two factors must act as modulators of task set formation and ensuing performance in interference paradigms.
A special focus of our examination will consider social paradigms—that is, paradigms in which a task is shared between two participants. As will become apparent below, these paradigms are certainly special in the sense of relying on special modes of response competition and special types of interfering features. Nonetheless, our framework can account for them without introducing any special assumptions. (cf. Dolk and Prinz, in press ; Dolk et al., 2011 , 2014a ).
Modes of Response Competition
Our framework considers response conflict a crucial driving force of task set formation and the ensuing competition a critical constraint of task set structure. Accordingly, we must expect that the mode of that competition impacts both; task set formation and task performance. Here we examine three major types of response conflict and competition: choice, selective response, and joint selective response.
Choice tasks require decisions between alternative actions. For instance, in the case of binary choices on which we concentrate here, choice tasks require decisions between two actions that are both explicitly represented. Since one of them has to be chosen and performed on each given trial, we address them as Go/Go tasks . In contrast, selective response tasks require decisions between performing and witholding a single, explicitly represented action. Since one of these two options has to be chosen on each given trial, we address them as Go/No-go tasks . Finally, joint selective response tasks combine two individuals performing two complementary selective reponse tasks. In these tasks, too, both individuals have to make choices between responding vs. withholding. However, since their choices are complementary, the combined task amounts to a choice between individuals, that is, which of them responds on a given trial. Therefore, we may address such tasks as I-go/You-go tasks .
While choice and selective response tasks are classical tools of mental chronometry (Donders, 1868/1969 ; Wirth, 1927 ), the joint selective response task is a newcomer to the field (Sebanz et al., 2003 , 2005 , 2006 ). In the following sections we concentrate on applications of these tasks to interference paradigms, comparing them in terms of requirements for response competition and ensuing implications for task implementation and performance. In this discussion we will mainly focus on the Simon task as a prototypical interference paradigm and a testcase for examination and comparison.
Choices: Go/Go Tasks
Classical interference tasks are choice tasks, instantiating competition among a set of responses mapped to a set of stimuli. Here we may confine ourselves to summarizing previously discussed topics from ealier sections. As we have seen, a typical Simon task requires selecting and pushing one of two keys in response to the color, for example, of a stimulus patch on the screen. Interference may then arise from overlap between features of required responses (e.g., left or right key presses) and irrelevant features of presented stimuli (e.g., left or right on screen). Since the overlap supports correct/wanted responses on compatible trials, but incorrect/unwanted responses on incompatible trials, it facilitates and impedes response selection accordingly. The resulting performance difference produces the Simon effect.
Choice interference tasks thus instantiate the above-mentioned dilemma between the efficiency requirements of stimulus and response code formation. Features that must play a strong role in one kind of code cannot be weak or even absent in the other. Given the structure of choice tasks, there is no obvious way to resolve the dilemma. This may in fact account for the remarkable persistence of the interference effect in such tasks (MacLeod and Dunbar, 1988 ; MacLeod, 1991 ; van Maanen et al., 2009 ).
Selective Responses: Go/No-go Tasks
Let us now see what our framework predicts when we alter the requirements of response competition. Consider a Go/No-go version of the Simon task in which participants again respond to one of the two colors ( say, red ), but withhold responding to the other color ( say, green ). In a setting like this the stimulus set comprises the same four combinations of (task-relevant) colors and (task-irrelevant) locations as before. In contrast, the structure of the response set is entirely different. Each trial requires a decision between two options: to push or not push the response key. Conflict and competition thus apply to the options Go vs. No-go , that is responding vs. withholding response. Recent evidence has shown that processing mechanisms for withholding are closely related to those for responding, so that withholding a response can also be regarded as a response (Kühn et al., 2009 ; Kühn and Brass, 2010 ). If one adopts this view, the Go/No-go task may still be seen to instantiate a conflict between two competing response options. Yet, the two options are not spatially distinct anymore: they do not differ in terms of spatial locations to which they pertain.
Our framework predicts that the Simon effect must disappear under these conditions. Since there is no response competition that highlights location features anymore, there is no strengthening of feature weights and, hence, no mandatory inclusion of location features in pertinent stimulus codes. Instead, these features can now be weakened or deleted, as the instruction to ignore them suggests. This is in fact what severalstudies have shown: the Simon effect is abolished in the Go/No-go task (e.g., Hommel, 1996 ; Ansorge and Wühr, 2004 ).
At this point we may tentatively conclude that event code theory offers a framework that helps us to understand the functional difference between Go/Go and Go/No-go versions of the Simon task. More specifically we may conclude that the mode of response competion inherent in these versions drives task set formation and determines task set structure.
Joint Selective Responses: I-go/You-go Tasks
Can the framework likewise account for performance in the joint selective response task? Before we address this question, let us first see what the task requires and how it works. The literature offers two basic accounts to capture the functional logic of the task: individual and social. They differ not only in descriptive terms, but also suggest different explanations that are associated with fairly diverging theoretical backgrounds (Dolk and Prinz, in press ; Wenke et al., 2011 ; Dolk et al., 2014a ).
Individual account— According to the individual account, the task combines two participants who are required to perform two independent, complementary Go/No-go tasks. Typically, the two are seated next to each other, with one of two response keys assigned to each of them. Instructions may require, for instance, that one responds to green, but withholds to red stimuli whereas the other does the reverse, that is, responds to red, but withholds to green stimuli. Each participant’s share of the task is thus, in terms of task requirements, completely equivalent to the regular, single Go/No-go task as discussed above. In other words, for each of them response competition is competing between the options of pushing or not pushing one and the same response key. Accordingly, the individual perspective leads us to expect that the joint selective response setting should exhibit similar interference effects as the regular, single Go/No-go setting.
Social account— The social account takes a broader perspective. Essentially, it regards the two individual Go/No-go tasks as two complementary components of a common Go/Go task that is shared between two individuals. Within this common task each of the two paricipants is responsible for one of the two stimulus colors and one of the two response keys assigned to them. Since stimulus color dictates which of the two response keys must be pushed, the full task is seen to instantiate conflict and competition between two spatially distinct response options. Accordingly, the social perspective leads us to expect that the joint selective response setting should exhibit similar interference effects as the regular, single Go/Go setting.
As indicated, the two accounts are rooted in quite divergent theoretical and metatheoretical backgrounds (cf. Prinz, 2012 , Ch. 2). In particular, they differ deeply in terms of their implied beliefs regarding the nature and representational basis of human sociality. The individual account believes in solipsistic closure. It regards human agents as closed, encapsulated systems that, when brought into social scenarios, tend to concentrate on their own agenda, without taking much notice of others or even coordinating or sharing their agendas with them. In contrast, the social account believes in open-mindedness. It regards agents as open, interactive systems that, when brought into social scenarios, are keen on taking notice of others and opening their own agenda for coordinating and sharing it with them. Sociality is thus a primary, constitutive ingredient of human nature for the social account, while it plays only a secondary, derived role for the individual account.
Combined account— Setting such big issues aside, we now take a more pragmatic look at the joint task from the perspective of event code theory. This framework suggests an account that combines individual and social elements (Baess and Prinz, in prep.; Dolk and Prinz, in press ; Wenke et al., 2011 ; Dolk et al., 2014a ).
The combined account once more builds up on response conflict and competition as the key factor driving task set formation and shaping task set structure. It combines two elements, What and Who (Jeannerod, 1999 , 2003 , 2006 ; Decety and Chaminade, 2003 ). On the one hand, it claims that response competition takes the form of a What- conflict: in each trial participants decide between responding and withholding—as the individual account suggests. On the other hand, it claims that the What- conflict is superimposed by a Who- conflict, that is, a conflict between the two agents—as the social account suggests.
The interindividual conflict becomes particularly apparent when we focus on overt responses. When viewed in terms of overt events, trials can be seen to instantiate choices between the responding agents: either I respond or You respond. For each participant, though, this interindividual conflict is perfectly correlated, and hence completely confounded with the individual Go/No-go conflict: when I go You withhold, and when You go I withhold. The task thus conflates two modes of response competition: individual selective response and interindividual choice. This is why we may refer to it as an I-go/You-go task.
Under such conditions predictions of task performance are difficult to make. Basically, we must expect that task performance exhibits signatures of both modes of response competition. Mixed evidence along these lines is indeed what studies of the joint selective response task show (cf. Dolk and Prinz, in press ).
On the one hand, there is strong evidence in support of the claim that the joint task is, in functional terms, still a selective response task, not a choice task. This conclusion is suggested by comparisons of response speed. As is known since Donders’ pioneering studies, response times for choices are substantially longer than for selective responses. The difference may range in the order of up to 200 ms—in fact a gigantic amount of time in terms of the standards of mental chronometry. Given this background, the observation of joint selective responses being substantially faster than choices and exhibiting the same time regime as regular selective reponses, seems to suggest that the embedding of Go/No-go tasks in joint settings does not alter the workings of the underlying task set machinery in a fundamental way. It would appear that joint selective responses are processed in the same way as regular selective responses, unlike choice responses.
On the other hand, evidence also exists in support of the claim that the joint task must be regarded as a choice task. This is because a Simon effect is regularly observed in this task. The size of the effect is weaker than in classical choice tasks, but it is highly reliable (Sebanz et al., 2003 , 2005 , 2006 ). We may therefore conclude that interference arises in the joint, but not in the regular version of the selective response task. This seems to indicate that individual task set formation becomes modulated by social context.
Our framework can readily account for these observations and conclusions. The central claim is that social context acts to shape response code assemblies, which then act back on stimulus code assemblies and interactions between the two.
The critical contribution that social context makes in shaping competing responses comes from integrating new distinctive features. In the joint task such new features can be derived from the fact that the two competing responses are distributed between two agents who differ from each other in several respects. Accordingly, any feature in which the agents and/or their responses differ is now a candidate for becoming included in their respective response codes. The joint task thus offers new distinctive features for inclusion in response codes—features that do not exist in the regular individual task.
Location features provide an obvious example. When two agents share a Simon task, they are seated on two chairs next to each other in front of the screen. They are, in other words, distinguished by their spatial locations in the scenario. Relative to the other, one is the left and the other the right agent. Accordingly, these features offer themselves for inclusion in the competing response codes. If this happens they will, on the one hand, increase code distinctiveness and improve the efficiency of response selection (as illustrated in Figure Figure2E). 2E ). However, if they overlap with irrelevant stimulus features, they will also give rise to interference (as illustrated in Figures 4C,D ), resulting in a (albeit weak) Simon effect. Here we return to the dilemma inherent in interference tasks: interference is the consequence of the same feature requiring inclusion in response codes and deletion from stimulus codes.
Looking back, we can now specify more precisely the way in which the combined account integrates individual and social elements. There are two basic claims. The first is that individuals concentrate on representing their own share of the task, without representing the other agent’s share. This is the individual element. The second is that they still form representations of agents and actions involved in the task scenario, exploiting them for shaping and optimizing their own task sets. This is the social element (Dolk and Prinz, in press ; Dolk et al., 2014a ).
In sum, the combined account does not believe in the romantic idea of task co-representation, claiming that agents share common representations of the full task in which they participate. The combined account posits shaping rather than sharing task sets. This notion reflects the pragmatic idea that representations of co-actors and their actions help agents to shape representations of their own task (Dolk and Prinz, in press ).
Types of Overlapping Features
Up to this point we have treated interference paradigms as a uniform category and discussed the Simon task as a prototypical example. Yet, when we consider a broader range of tasks, we need to extend our framework to account for different types of overlapping features and patterns of interference effects associated with them.
More specifically, we may discern three major paradigms that differ in terms of the stimulus-related features whose overlap with response-related features gives rise to interference: the Simon task (where overlap pertains to spatial locations), the Eriksen flanker task (where it pertains to categorical assignments), and a face perception task (where it pertains to personal identities).
Since we have used the Simon task as a testbed for applying event code theory to interference paradigms, we may start summarizing what has been discussed hitherto concerning spatial interference effects. Interference in the Choice version of this task arises from overlap between spatial features associated with stimuli and responses (i.e., left vs. right locations). This overlap acts to facilitate and impede response selection on in compatible and incompatible trials, respectively. The resulting difference produces the Simon effect.
In the following, we focus on Selective Response s. Firstly, we discuss fundamental mechanisms of spatial interference. Here we consider both, Individual and Joint Selective Response tasks. Secondly, we go beyond basic mechanism, focusing on the impact of Social Modulators on spatial interference.
Individual selective responses: Go/No-go— As we have seen the interference effect disappears in the Individual Selective Response version of the Simon task. Our framework offers a simple explanation for this observation, based on the fact that in Go/No-go task response conflict applies to options that do not differ in terms of spatial locations (viz., pushing vs. not pushing a given response key). Thus, since response codes do not carry location features, overlap between stimulus and response locations cannot emerge, and spatial interference effects cannot be obtained.
This view claims that the crucial difference between Choice and Selective Response lies in different roles of spatial response features. These features play a strong role in Choice , but no role at all in Selective Response . An obvious way of testing this claim is to run a Go/No-go task scenario where spatial layout gives rise to localizing the involved response key and/or the act of pushing it on a left-right dimension. This should furnish the underlying response code with corresponding location features.
One way of achieving this is to introduce an accessory reference object or event and integrate it into the spatial layout of the task scenario. If the object or event is sufficiently salient, it should act as a reference for localizing the key press on the left/right dimension, thus furnishing the underlying response code with a location feature pertaining to that dimension. The accessory reference is thus expected to play a similar role for Selective Responses as the competing response does for Choices : to lay the ground for referential coding of response locations and feature overlap and interference arising from such coding.
This prediction has been confirmed in recent studies. Dolk et al. ( 2011 ) showed that a Simon effect in a Go/No-go task cannot only be obtained when a co-actor is introduced (i.e., under Joint Go/No-go conditions; see below), but also when, instead of their co-actor’s response key, another salient object (e.g., a Japanese waving cat or a ticking metronome) is mounted on the left- or right-hand side of the participant’s response key (Dolk et al., 2013 ; see also Dolk et al., 2011 ). These studies demonstrate that any attention-capturing object may serve the function of a spatial reference for furnishing response keys with location features.
These findings support the idea of whether spatial interference is obtained in the Selective Response task and whether it is determined by the spatial layout of the task scenario. If the scenario harbors a salient reference, participants will localize their responses accordingly, thus furnishing their response codes with location features. Once established, location features in response codes will give rise to spatial interference, due to their overlap with location features in stimulus codes.
Joint selective responses: I-go/You-go— The scope of this explanatory approach is evidently not restricted to the individual task. It can also be applied to the Joint Go/No-go task. In fact it was initially conceived to account for the Joint task and only then tested on the Individual task (e.g., Guagnano et al., 2010 ; Dolk et al., 2011 ).
Essentially, this approach treats actors, co-actors and actions as physical objects and events that are specified by their locations in the spatial layout of the task scenario. More specifically, the claim is that the co-actor and/or his response serve as a reference for localizing the actor and/or her own response—provided that the co-actor’s involvement in the task is sufficiently salient. For instance, it has been shown that interference is obtained when the co-actor is actively involved in the task, not when he/she is passively sitting by the actor’s side (Sebanz et al., 2005 , 2006 ). Likewise, interference seems to depend on distance of the reference: a Simon effect only obtaines when the co-actor is seated close-by, not when he/she is seated at a distance (Guagnano et al., 2010 ).
Referential coding can thus account for the emergence of interference in the Joint Go/No-go task. However we should realize that the notion of referential coding is grossly underspecified. For instance, we are unclear as to which objects and events qualify as references. There are several candidate items, such as response keys and their sounds, arms, hands and fingers as they operate response keys, torsos to which these limbs are attached, and seats carrying them etc.
Under normal conditions the relative locations of these items are perfectly correlated: a response key on the left is operated by a hand on the left, which is, in turn, attached to a torso on the left, etc. Still, we have limited knowledge as to their respective contributions to spatial coding; does location coding mainly pertain to response devices—or to agents and their bodies? First evidence seems to suggest that efficient location coding may in fact involve both, keys and seats at the same time (Dittrich et al., 2013 ).
Social modulators— So far we have concentrated on co-actors and their actions as physical objects and events. Yet, the combined approach outlined above adopts a more comprehensive view that goes beyond the physical domain. As have seen, this approach posits that the What- conflict between Go and No-go is in the Joint task superimposed by a Who- conflict between I and You . Accordingly, it predicts a critical role for what we may call Self/Other overlap : any features in which the two agents differ or overlap can be expected to play a functional role in resolving the combined I-go/You-go conflict.
According to this view, spatial location is just one such distinctive feature. Beyond their accidental seating in the experimental scenario, the two agents will also differ in a number of non-spatial and non-physical respects, be it in terms of transient states or permanent traits. Thus, when two individuals meet to engage in a joint task, they will immediately form impressions about each other, including intuitions concerning Self/Other overlap . These intuitions will adress both, features that are common to them (e.g., female, German, tall) and features that distinguish them (e.g., dressed in black vs. white, speaking with Bavarian vs. Saxonian accent, friendly vs. unfriendly behavior, etc.).
Taking this into account, their seating in the experimental scenario does no more than add one particular transient state to that list, viz. sitting left vs. right of the other. Even if we assume that such spatial features are coded automatically, their contribution to the formation of pertinent response codes are likely to depend on their relative role in the total list of distinctive features instantiating Self/Other overlap . The shorter the list, the stronger their contribution will be, and vice versa : When the two agents resemble each other in many respects, the list of distinctive features will be short, so that the added value of distinctive location features will be substantial. Conversely, when the agents differ in many respects, the list will be long, so that the added value of distinctive location features can only be marginal.
Accordingly, the framework predicts that agent similarity, or Self/Other overlap , must modulate spatial interference in the Joint Selective Response task. This prediction was recently confirmed in several studies. One line of evidence stems from studies combining in-group and out-group members in the Joint task. Here it was shown that a Simon effect is obtained when two (mutually similar) in-group members interact, but not when two (dissimilar) outgroup members interact (Müller et al., 2011 ). A further line of evidence pertains to the role of interpersonal relationships. Factors such as positive mood and positive relationships between co-acting individuals are known to increase perceived self-other overlap (e.g., Davis et al., 1996 ; cf. Heider, 1958 ). As we must therefore expect, such factors also act to increase spatial interference in the Joint task (Hommel et al., 2009 ; Kuhbandner et al., 2010 ).
As a concluding remark it should be noted that social modulation of spatial interference is also predicted by the co-representation account. The claim here is that individuals are more prone and/or more efficient in sharing common task representations with similar as compared to dissimilar others. In contrast, our framework relies entirely on shaping individual task representations according to the requirements emerging from spatial and social characteristics of the task scenario.
Assignments
It is sometimes argued that interference in the Simon task may be special because spatial features may play a privileged role in sensorimotor processing which may perhaps be hardware-rooted. For instance one may argue that spatial interference arises from physical features whose coding requires no more than just discriminating between two well-separated locations of stimulus and response events.
In contrast, semantic interference relies on arbitrary categorical assignments. The Eriksen flanker task (Eriksen and Eriksen, 1974 ; Eriksen, 1995 ) provides a paradigm for the study of interference arising from short-lived stimulus-response assignments. In the flanker task participants respond to two (or more) targets (e.g., letters, shapes, etc.) by pressing one of two response keys. Targets are surrounded by distracting flankers that are either (1) the same as the target (identical trials); (2) perceptually different from the target, but assigned to the same response (compatible trials); (3) perceptually different and assigned to the competing opposite response (incompatible trials); or (4) perceptually different and not assigned to any response (neutral trials).
To illustrate, we may refer to a study by Atmaca et al. ( 2011 ). In their experiments a string of five letters were presented in each trial: a target in the center, surrounded by four identical flankers (two on either side). In a given trial, one of four letters could appear in the target position: H and K (assigned to one of two response keys) and S and C (assigned to the opposite key). As an example for explaining the four task conditions, we may consider trials on which letter H served as target. In identical trials the same letter also served for flankers (yielding HHHHH ), whereas in neutral trials the same function was served by a letter that was never used as target (and therefore never assigned to a response key, e.g., UUHUU ). These two conditions were considered control conditions. Experimental conditions were provided by compatible and incompatible trials. In compatible trials flankers were physically different from the target but assigned to the same response category (yielding KKHKK ), wheras in incompatible trials they were both physically and categorically different (yielding, e.g., SSHSS ).
As with the Simon task this paradigm has generated a large amount of literature pertaining to various task versions and research questions. (cf., e.g., Eriksen, 1995 ; Purmann et al., 2011 ; Davelaar, 2013 ). The common finding is that flanker-related processing interferes with target-related processing, and that it does so despite the fact that task instructions advise ignoring flankers altogether.
Firstly, and perhaps not too surprisingly, response times for target categorization are substantially shorter in identical than in neutral trials, indicating that identical flankers may facilitate target processing. Secondly, and perhaps more interestingly, response times in compatible trials are often faster than in neutral trials, indicating that interference may not only arise from physical features but also from semantic, categorical features. Thirdly, and even more interestingly, responses are usually much slower in incompatible than in neutral trials, supporting and strengthening the same conclusion.
Unfortunately, contrasts involving the so-called neutral condition are less conclusive than this brief summary suggests. This is because two factors are confounded in such contrasts: assignment and valence. The assignment factor refers to the functional contrast of interest here: flankers may be assigned or not assigned to a response. The valence factor refers to a different functional contrast: flankers may be bivalent or univalent. Assigned flankers are always bivalent, that is, they can occur in the task in both roles; flankers and targets. Conversely, unassigned flankers are univalent, that is, they can only occur as flankers throughout the task. Thus, since univalent flankers are certainly easier to ignore than bivalent flankers, neutral trials cannot be expected to deliver a truly neutral reference for compatible and incompatible trials. This is in fact in line with results from most experiments: responses in so-called neutral trials are often as fast as in compatible trials, or even faster.
As with the Simon task we must therefore concentrate on the net compatibility effect, i.e., the contrast between compatible and incompatible trials. This contrast adresses assignments, unconfounded with valences. The sole difference between compatible and incompatible trials pertains to the response category to which the flankers in the string are assigned. In the compatible case both target and flankers drive wanted responses. Conversely, in the incompatible case the target and flankers drive wanted and unwanted responses, respectively. As a result, interference obtains: relative to the compatible case, response conflict is stronger in the incompatible case, weakening performance accordingly.
Flanker interference is grounded on semantic features—semantic in the sense of instantiating arbitrary assignments between stimulus letters and response keys. Notably, these assignments are acquired within the task. It is only by following instructions that participants learn that H and K belong to one response category and S and C to the other. In this respect semantic interference is indeed fundamentally different from spatial interference. While spatial interference relies on overlap between physical features that are inherent in the task layout, semantic interference relies on arbitrary stimulus-response assignments that are only established by task instructions. Thus, unlike spatial features that can be derived from overt perception of the task layout, semantic features must be derived from covert knowledge of task-specific assignments.
Our framework offers a simple and convenient way of conceiving the representational underpinnings of that knowledge. The underlying assumption is that new assignments are instantiated through new features. According to this view, implementing new assignments into a task set is tantamount to creating a novel feature that forms part of both, the stimulus code and the response code assigned to it (cf. Figures Figures2B, 2B , ,3B). 3B ). This feature then instantiates the wanted assignment. It instantiates both, the prescriptive assignment that the stimulus must be followed by the response and the descriptive assignment that the response may be linked to the stimulus.
Such features stand for the assignment as such: these are what they represent and what they mean. Their functional significance for the task set will then depend on the relative weights that they attain in the feature compounds that make up stimulus and response codes. The flanker task suggests that verbal instructions can drive their weights to a level strong enough to give rise to substantial interference effects.
Individual selective responses: Go/No-go— As seen above, the transition from Go/Go to Go/No-go is in the Simon task associated with a loss of response-related location features. The loss is critical since spatial interference builds on such features. However, in the flanker task we are facing a different functional situation. In this task semantic assignment features become partially lost and partially preserved on that transition. For instance, when instructions require responding to H and K and withholding response to any other target, the assignment of H and K to the response key is preserved, whereas any other letter-key-assignments are lost. Unlike key-related location features, key-related assignments do not require the opposite key as a reference. Thus, when the opposite key is removed, the remaining key looses its location, but not its assignment.
As a result we must expect to see a positive, not a negative compatibility effect in the Go/No-go task. There can be no negative effect as there is no longer any functional difference between neutral and incompatible trials: incompatible trials (such as SSHSS or CCKCC ) are, in functional terms, neutral trials. Conversely, the positive effect must be preserved as the covert assignment knowledge on which it relies is unaffected.
In practice, however, the above-mentioned confound forces us to concentrate on the net compatibility effect, that is, the contrast between compatible and incompatible trials. With respect to this contrast we must expect that a compatibility effect should still be obtained. This is because flankers contribute to driving the Go response on compatible, but not on incompatible trials. This prediction is confirmed by task performance data (e.g., Atmaca et al., 2011 , Experiments 1 and 3).
A further prediction is that the compatibility effect must depend on prior experience with other versions of the task. For instance, when participants perform a Go/No-go task after performing other task versions in which incompatible flankers were assigned to overt responses, they may be expected to carry over these previously acquired assignments to the new task. An example is provided by Experiment 3 in the study by Atmaca et al. ( 2011 ), in which the Go/No-go task could be administered either before or after a different version of the task in which the same incompatible flankers were assigned to response keys. While interference was otained in both conditions, it was modulated by previously acquired assignments. The compatibility effect in a current Go/No-go task increased substantially when assignments acquired in a preceding task could be carried over to the current task.
In sum, these observations suggest that, unlike the Simon task, some interference is preserved when we move from choices to selective responses. This difference in performance reflects a difference in the features that give rise to interference. While spatial features anchored in the overt task layout are lost in this way, semantic features anchored in covert knowledge are preserved.
Joint selective response: I-go/You-go— For this task the combined account claims that the individual conflict between responding and withholding ( Go/No-go ) is superimposed by a social conflict between the two responding agents ( I/You ). Agents may differ in many ways. As we have seen, crucial features driving the joint Simon effect pertain to co-actors’ spatial locations. Their overlap with corresponding stimulus features gives rise to spatial interference.
In the flanker task the agents also differ in terms of spatial locations, although in this task there is no overlap between stimulus and response locations. Instead, overlap now applies to flanker assignments. In compatible and incompatible trials flankers are assigned to I and You , respectively. While the assignment of compatible flankers to oneself also applies to the individual task, the assignment of incompatible flankers to one’s co-actor is only established in the joint task. As a result, flanker interference must be expected to increase in the joint task. As in the individual task, compatible flankers must be expected to help, but atop of this incompatible flankers must also be expected to hurt now.
Experimental evidence confirms this prediction. Net flanker interference is always higher in Joint Go/No-go tasks than in Individual Go/No-go tasks (Atmaca et al., 2011 ). Remarkably, this applies to both; tasks involving human and non-human co-actors, suggesting that flankers may not only become assigned to real agents who perform the task, but also to non-performing pseudo-agents (Dolk et al., 2014b ). Taken together, the evidence from these studies supports the claim that in the Joint task intraindividual conflict between responding and withholding becomes correlated and conflated with interindividual conflict between onself and one’s co-actor.
As a final example we consider a paradigm for the study of interference arising from irrelevant face information (Baess and Prinz, in prep.; see also Philipp and Prinz, 2010 ). The task required Go/No-go decisions, dependent on the color of circles presented on the screen (e.g., Go for white and No-go for black circles, or vice versa). The circles were superimposed on background faces whose identity were irrelevant for task and therefore had to be ignored. Nonetheless, the critical manipulation pertained to the identity of the irrelevant faces on which the relevant circles were superimposed. Three kinds of trials were randomly intermixed, varying in the identity of the background face and instantiating different degrees of face/agent overlap: own face, familiar face and neutral face . In own-face trials circles were superimposed onto a photo showing the face of the responding agent. In familiar-face trials they were superimposed onto photos showing familiar individuals such as co-actors, friends or siblings. Neutral-face trials showed entirely unfamiliar faces for control.
Individual selective responses: Go/No-go— In this study a reliable own-face advantage was obtained throughout: Go responses on own-face trials were always faster than in neutral-face trials. Results for familiar faces depended on the depth of familiarity. When photos showed faces of deeply familiar individuals such as siblings or friends, a familiarity advantage was obtained. In this case Go responses for familiar faces were just as fast as for own faces. No such advantage was obtained when photos showed faces of superficially familiar individuals such as experimental co-actors. In this case, Go responses were just as slow as for neutral faces.
To account for the own-face advantage , we need to address the question of what kinds of features faces and Go/No-go responses have in common and what own faces have that neutral, foreign faces don’t have. One kind of such features pertains to personal identity . On the stimulus side, the role of identity features is fairly obvious: more than any other body part a person’s face bears the signature of his/her identity. Moreover, since faces are salient pop-out stimuli that are hard to ignore, we may assume that features pertaining to facial identity are automatically processed in this task.
The role of these features is perhaps less obvious on the response side. However, the conflict between responding vs. withholding is conflated with an asymmetry in terms of agent identity. Go responses are overt actions that require an agent performing them: when there is an overt action there must also be an agent. Response codes for Go responses must therefore specify both, the action ( What ) and the agent performing it ( Who ). Conversely, No-go responses do not imply any overt actions that require an agent performing them: when there is no overt action there can be no agent either. Response codes for No -go responses can therefore neither specify the action nor the agent.
This asymmetry may explain why Go responses are faster on own-face trials than in neutral-face trials. When the photo shows one’s own face, the identity of the responding agent (as specified by the response code) overlaps with the identity of the stimulus face (as specified by the stimulus code). No such overlap applies when the photo shows a neutral face. This functional difference may give rise to the own-face advantage. Its phenomenal counterpart is the intuition in which pictures of participants’ own face entail action impulses that pictures of neutral faces don’t: for them, it is as if seeing their own face prompts their own action.
A related, though more formal type of features that stimulus faces may share with Go/No-go responses pertains to dimensional polarity . The Go/No-go dimension is unipolar in the sense that one response option is well defined (positive polarity: push the key in front of you), whereas the other is defined by default (negative polarity: do nothing). The same applies to the face dimension: one’s own face is singular and well defined, whereas other faces are defined by default. As a result, the positive polarity of one’s own face matches the positive polarity of the Go response. Such polarity correspondence may likewise contribute to the own-face advantage (Seymour, 1971 ; Proctor et al., 1992 ; Proctor and Cho, 2006 ).
To account for the familiarity advantage we may again resort to overlap of identity features. If it is true that response codes for Go responses address both, the overt action and oneself as responding agent, we should not be surprised to see that overlap between face and agent identity is not an all-or-none affair, but may be graded according to the social and semantic distance between the face on the screen and oneself as responding agent. Deep familiarity that relies on long-term acquaintance (e.g., siblings or friends) seems to create strong overlap—being no weaker than familiarity with one’s own face. In contrast, superficial familiarity that builds on short-term acquaintance (e.g., experimental co-actors first encountered a few minutes before) seems to be insufficient in creating any overlap at all.
Taken together, the results suggest considering own-face advantage as a special case of familiarity advantage . In this paradigm interference arises from overlap between identities of stimulus faces and responding agents, and the features instantiating them in stimulus and response codes. Interference in the face task thus relies on features whose assignment to stimulus and response codes builds on previously acquired knowledge. This explains why interference is obtained in the Individual Go/No-go task: critical features are derived from internal, knowledge-based resources, not from external, stimulus-based resources (as in the Eriksen task, but unlike the Simon task).
Joint selective responses: I-go/You-go— Finally, when we move from the individual to the joint version of the Go/No-go task, agent asymmetry ( I vs. No-one ) becomes agent competition ( I vs. You ). Agent competition should support agent differentiation, that is, increase weights of distinctive features for the identities of the two competing agents. Since these response-related identity features overlap with stimulus-induced identity features, the basic prediction is that identity-based interference should increase when one moves from the individual to joint Go/No-go task.
In the study by Baess and Prinz (in prep.) the evidence in support of this prediction was mixed. An increase of own-face advantage was in fact obtained when the joint task was shared with a hitherto unknown co-actor. However, no such increase was obtained when the task was shared with a sibling or friend. In other words, the prediction was confirmed under conditions of shallow, but not deep familiarity.
To account for this surprising finding, the authors suggested resorting to the role of familiarity for Self/Other overlap (Baess and Prinz, in prep.). When actors and co-actors are closely related to each other (i.e., siblings or friends), their representations of themselves and of their co-actors will strongly overlap. Due to this overlap a photo of their own face will not only prime their own Go response, but also their co-actor’s Go response to some degree (hence, their own No-go response conflated with it). As shown in Figure Figure5, 5 , (horizontal) self/other overlap must thus be expected to modulate the efficiency of priming arising from (vertical) face/agent overlap. Critically, these two factors have opposite effects on response competition. On one hand, response competition decreases as face/agent overlap increases, but on the other hand it increases as self/other overlap increases. The speed of the Go response must therefore reflect the combined effect of two counteracting factors that may cancel each other.

Interaction between (vertical) face/agent and (horizontal) self/other overlap . Due to vertical overlap between stimulus and response codes, a photo of one’s own face ( OF ) primes one’s own Go response ( I-go ). As long as horizontal overlap between response codes is weak the competing code ( You-go ) is unaffected by the vertical overlap (panel A , unfamiliar co-actors). However, when horizontal overlap is substantial, one’s own face will also prime the competing code ( You-go ; panel B , familiar co-actors).
At first glance, this finding and the account offered for it seem to be at variance with observations and explanations discussed in the above-mentioned studies on the role of self/other overlap in the joint Simon task. As reported, agent overlap in these studies was always correlated positively with interference. This would lead one to expect strong interference for siblings and friends (where self/other overlap must be fairly strong), but weak or no interference for unknown co-actors (where agent overlap must be much weaker).
A closer look reveals that there is no contradiction at all, however. This is because differences in agent overlap must be associated with differences in the relative contributions of response- vs. agent-related features to the combined response/agent codes. More specifically, an increase in agent overlap must be expected to weaken the weights of agent-related features, to the effect of strengthening the relative weights of response-related features. Such restructuring of feature weights must lead to different consequences for the Simon task and the face/agent interference task. Since Simon interference relies on overlap between stimulus and response-related features, strengthening the role of response-related features must lead to stronger interference. Conversely, since face/agent interference relies on overlap between stimulus and agent-related features, weakening the role of agent-related features must lead to weaker interference. This is why the finding regarding the role of self/other overlap in the face/agent interference task is entirely compatible with seemingly conflicting findings concerning its role in the Simon task.
Event code theory claims that interference arises in tasks in which the same features adopt two roles at a time: that of irrelevant elements in stimulus codes and that of relevant elements in response codes. In this case conflict becomes unavoidable. Since stimulus and response codes overlap in these features, the requirement of strengthening feature weights for one code must counteract the requirement of weakening them for the other. In particular, must a strong role that features gain from processing irrelevant stimulus information counteract their suppression for efficient response selection? This is the dilemma on which interference builds.
In this section we applied this principle to a variety of interference paradigms, including joint tasks that are shared between two participants. In one aspect, since our framework considers response competition the chief driver of interference, different modes of such competition must give rise to differnt patterns of interference. However, since it also claims a crucial role for features in which stimulus and response codes overlap, different kinds of such features must likewise be associated with different patterns of interference.
Concerning the role of social context for task set formation, two major observations have emerged, one of which pertains to response competition. Task sets for shared tasks seem to instantiate a special mode of response competition in which an intraindividual conflict between responding and withholding is conflated with an interindividual conflict between the two agents, yielding an I-go/You-go conflict. The other observation pertains to the nature of features whose overlap gives rise to interference. Such features may, in some paradigms, refer to basic elements for example, key locations, body parts and body movements. However, in other (if not all) social paradigms they also refer to the agents themselves—both physically (e.g., being seated alongside each other) and non-physically (e.g., being related to each other as siblings or aquainted as friends).
Taken together, the two observations suggest a prominent role of agent-related features for task set formation in social context. When a task assigns competing actions to competing agents, task set formation will adress both actions and agents. As a result, response codes will include features refering to the agents who respond, not just the actions through which they respond.
The Benefits of Matching
As discussed above, the notion of matching plays a key role in the Event Code Theory of task set formation. Unlike mapping operations, which are thought to rely on extrinsic, arbitrary linkages, matching operations are thought to rely on intrinsic, content-based interactions between stimulus and response codes. This raises the issue of how mapping and matching are related.
There are two seemingly contradictory answers to this question, one at the level of representing world events through mind codes, and the other at the level of formation of these codes from elementary features.
The answer at the representational level invokes mapping-through-matching . This principle claims that incommensurate objects can become represented by commensurate codes in the mind, so that code similarity, or overlap plays a role in their interaction. In line with this idea, our framework claims that S-R mappings are instantiated through creating selective overlap between stimulus and response codes (as illustrated in Figures 2B,C , ,3B, 3B , ,4B). 4B ). Once implemented, the overlap mediates interactions between stimulus and response codes in performance. It thus accounts for arbitrary relationships between incommensurate events (e.g., stimulus colors and response keys) through similarity relationships beween their commensurate event codes.
The concept that objects and events that are coupled to each other may be represented by codes whose overlap indicates their coupling, has recently gained support from fMRI studies addressing the shaping of cortical representations through temporal regularities. Results showed that several brain areas encoded regular couplings between objects and events through increasing the overlap of their cortical representations (Schapiro et al., 2012 , 2013 ). These findings suggest a functional role for representational similarity: couplings between objects and events become instantiated through overlap between their representations. Our framework claims that the same principle holds for the coupling of stimuli and responses in task sets.
Mapping-through-matching holds for represention of events in the world through codes in the mind/brain. In contrast, the reverse principle seems to hold for the formation of event codes from features. At this level matching-through-mapping applies. Event codes build on local feature compounds that rely on arbitrary mappings of selected features (e.g., their selective inclusion/exclusion or their selective strengthening/weakening of their weights in these compounds). Therefore, networks of arbitrary mappings are required at the feature level to enable similarity-based matchings at the code level. High-level matching depends on a powerful machinery for low-level mapping (Prinz, 1984 , 1987 , 2013 ; Catmur et al., 2007 ; Heyes, 2010 ; Pulvermüller et al., 2014 ).
This raises the issue of what the functional benefits of matching may be, that is, what matching can do for cognitive systems that mapping cannot—a question reminiscent of the classical debate of the role of similarity and contiguity for the formation of associations (e.g., Warren, 1921 ; Turner, 1967 ). Matching relies on similarity or overlap, whereas mapping relies on established linkages. Matching-based systems can therefore be more flexible than mapping-based systems. They can still be functional when pre-established codes and linkages do not exist or work, for instance when novel, unfamiliar stimuli are presented or new responses are required. This seems to be the crucial asset for which cognitive systems invest substantial ressources when constructing sophisticated devices for matching. Still, we should not forget that matching at code level always requires and presupposes machinery for mapping at feature level.
Conflict of Interest Statement
The author declares that the research was conducted in the absence of any commercial or financial relationships that could be construed as a potential conflict of interest.
- Ach N. (1905). Über die Willenstätigkeit und das Denken [About the Activity of Will and Thinking]. Göttingen: Vandenhoeck and Ruprecht. [ Google Scholar ]
- Alluisi E. A., Warm J. S. (1990). “ Things that go together: a review of stimulus-response compatibility and related effects ,” in Stimulus-Response Compatibility—An Integrated Perspective , eds Proctor R. W., Reeve T. G. (Amsterdam: North-Holland; ), 3–20. [ Google Scholar ]
- Ansorge U., Wühr P. (2004). A response-discrimination account of the Simon effect . J. Exp. Psychol. Hum. Percept. Perform. 30 , 365–377. 10.1037/0096-1523.30.2.365 [ PubMed ] [ CrossRef ] [ Google Scholar ]
- Atmaca S., Sebanz N., Knoblich G. (2011). The joint flanker effect: sharing tasks with real and imagined co-actors . Exp. Brain Res. 211 , 371–385. 10.1007/s00221-011-2709-9 [ PMC free article ] [ PubMed ] [ CrossRef ] [ Google Scholar ]
- Atmaca S., Sebanz N., Prinz W., Knoblich G. (2008). Action co-representation: the joint SNARC effect . Soc. Neurosci. 3 , 410–420. 10.1080/17470910801900908 [ PubMed ] [ CrossRef ] [ Google Scholar ]
- Barsalou L. W. (1999). Perceptual symbol systems . Behav. Brain Sci. 22 , 577–609. 10.1017/s0140525x99002149 [ PubMed ] [ CrossRef ] [ Google Scholar ]
- Broadbent D. E. (1958). Perception and Communication. Oxford: Pergamon Press. [ Google Scholar ]
- Bundesen C. (1990). A theory of visual attention . Psychol. Rev. 97 , 523–547. 10.1037/0033-295x.97.4.523 [ PubMed ] [ CrossRef ] [ Google Scholar ]
- Bundesen C. (1998). A computational theory of visual attention . Philos. Trans. R. Soc. Lond. B Biol. Sci. 353 , 1271–1281. 10.1098/rstb.1998.0282 [ PMC free article ] [ PubMed ] [ CrossRef ] [ Google Scholar ]
- Carpenter W. B. (1874). Principles of Mental Physiology: With Their Applications to the Training and Discipline of the Mind and the Study of Its Morbid Conditions. London: King. [ Google Scholar ]
- Catmur C., Walsh V., Heyes C. (2007). Sensorimotor learning configures the human mirror system . Curr. Biol. 17 , 1527–1531. 10.1016/j.cub.2007.08.006 [ PubMed ] [ CrossRef ] [ Google Scholar ]
- Davelaar E. J. (2013). When the ignored gets bound: sequential effects in the flanker task . Front. Psychol. 3 :552. 10.3389/fpsyg.2012.00552 [ PMC free article ] [ PubMed ] [ CrossRef ] [ Google Scholar ]
- Davis M. H., Conklin L., Smith A., Luce C. (1996). Effects of perspective taking on the cognitive representation of persons: a merging of self and other . J. Pers. Soc. Psychol. 70 , 713–726. 10.1037//0022-3514.70.4.713 [ PubMed ] [ CrossRef ] [ Google Scholar ]
- Decety J., Chaminade T. (2003). When the self represents the other: a new cognitive neuroscience view on psychological identification . Conscious. Cogn. 12 , 577–596. 10.1016/s1053-8100(03)00076-x [ PubMed ] [ CrossRef ] [ Google Scholar ]
- Desimone R., Duncan J. (1995). Neural mechanisms of selective visual attention . Annu. Rev. Neurosci. 18 , 193–222. 10.1146/annurev.neuro.18.1.193 [ PubMed ] [ CrossRef ] [ Google Scholar ]
- Dittrich K., Dolk T., Rothe-Wulf A., Klauer K. C., Prinz W. (2013). Keys and seats: spatial response coding underlying the social Simon effect . Atten. Percept. Psychophys. 75 , 1725–1736. 10.3758/s13414-013-0524-z [ PubMed ] [ CrossRef ] [ Google Scholar ]
- Dolk T., Hommel B., Colzato L. S., Schütz-Bosbach S., Prinz W., Liepelt R. (2011). How “social” is the social Simon effect? Front. Psychol. 2 :84. 10.3389/fpsyg.2011.00084 [ PMC free article ] [ PubMed ] [ CrossRef ] [ Google Scholar ]
- Dolk T., Hommel B., Colzato L. S., Schütz-Bosbach S., Prinz W., Liepelt R. (2014a). The joint Simon effect: a review and theoretical integration . Front. Psychol. 5 :974. 10.3389/fpsyg.2014.00974 [ PMC free article ] [ PubMed ] [ CrossRef ] [ Google Scholar ]
- Dolk T., Hommel B., Prinz W., Liepelt R. (2013). The (not so) social Simon effect: a referential coding account . J. Exp. Psychol. Hum. Percept. Perform. 39 , 1248–1260. 10.1037/a0031031 [ PubMed ] [ CrossRef ] [ Google Scholar ]
- Dolk T., Hommel B., Prinz W., Liepelt R. (2014b). The joint flanker effect: less social than previously thought . Psychon. Bull. Rev. 21 , 1224–1230. 10.3758/s13423-014-0583-8 [ PubMed ] [ CrossRef ] [ Google Scholar ]
- Dolk T., Prinz W. (in press). “ What it takes to share a task: sharing versus shaping task representations ,” in Shared Representations: Sensorimotor Foundations of Social Life , eds Obhi S. S., Cross E. S. (Cambridge: Cambridge University Press; ). [ Google Scholar ]
- Donders F. C. (1868/1969). “ On the speed of mental processes ,” in Proceedings of the Donders Centenary Symposium on Reaction Time: Attention and Performance II , ed Koster W. G. (Amsterdam: North-Holland; ), 412–431. [ Google Scholar ]
- Eriksen C. W. (1995). “ The flankers task and response competition: a useful tool for investigating a variety of cognitive problems ,” in Visual Selective Attention , eds Bundesen C., Shibuya H. (Hillsdale, NJ: Erlbaum; ), 101–118. [ Google Scholar ]
- Eriksen B. A., Eriksen C. W. (1974). Effects of noise letters upon the identification of a target letter in a nonsearch task . Percept. Psychophys. 16 , 143–149 10.3758/bf03203267 [ CrossRef ] [ Google Scholar ]
- Fitts P. M., Deininger R. L. (1954). S-R compatibility: correspondence among paired elements within stimulus and response codes . J. Exp. Psychol. 48 , 483–492. 10.1037/h0054967 [ PubMed ] [ CrossRef ] [ Google Scholar ]
- Fitts P. M., Seeger C. M. (1953). S-R compatibility: spatial characteristics of stimulus and response codes . J. Exp. Psychol. 46 , 199–210. 10.1037/h0062827 [ PubMed ] [ CrossRef ] [ Google Scholar ]
- Garner W. R. (1962). Uncertainty and Structure as Psychological Concepts. New York: Wiley. [ Google Scholar ]
- Garner W. R. (1974). The Processing of Information and Structure. Potomac, MD: Erlbaum. [ Google Scholar ]
- Guagnano D., Rusconi E., Umiltà C. (2010). Sharing a task or sharing space? On the effect of the confederate in action coding in a detection task . Cognition 114 , 348–355. 10.1016/j.cognition.2009.10.008 [ PubMed ] [ CrossRef ] [ Google Scholar ]
- Hedge A., Marsh N. W. (1975). The effects of irrelevant spatial correspondence on two-choice response time . Acta Psychol. (Amst) 39 , 427–439. 10.1016/0001-6918(75)90041-4 [ PubMed ] [ CrossRef ] [ Google Scholar ]
- Heider F. (1958). The Psychology of Interpersonal Relations. New York: Wiley. [ Google Scholar ]
- Heyes C. (2010). Where do mirror neurons come from? Neurosci. Biobehav. Rev. 34 , 575–583. 10.1016/j.neubiorev.2009.11.007 [ PubMed ] [ CrossRef ] [ Google Scholar ]
- Hommel B. (1996). S-R compatibility effects without response uncertainty . Q. J. Exp. Psychol. 49A , 546–571 10.1080/027249896392496 [ CrossRef ] [ Google Scholar ]
- Hommel B. (1997). “ Toward an action-concept model of stimulus-response compatibility ,” in Theoretical Issues in Stimulus-Response Compatibility , eds Hommel B., Prinz W. (Amsterdam: North-Holland; ), 281–320. [ Google Scholar ]
- Hommel B. (2009). Action control according to TEC (theory of event coding) . Psychol. Res. 73 , 512–526. 10.1007/s00426-009-0234-2 [ PMC free article ] [ PubMed ] [ CrossRef ] [ Google Scholar ]
- Hommel B. (2010). “ Grounding attention in action control: the intentional control of selection ,” in Effortless Attention: A New Perspective in the Cognitive Science of Attention and Action , ed Bruya B. J. (Cambridge, MA: MIT Press; ), 121–140. [ Google Scholar ]
- Hommel B. (2013). “ Ideomotor action control: on the perceptual grounding of voluntary actions and agents ,” in Action Science: Foundations of an Emerging Discipline , eds Prinz W., Beisert M., Herwig A. (Cambridge, MA: MIT Press; ), 113–136. [ Google Scholar ]
- Hommel B., Colzato L. S., van den Wildenberg W. P. M. (2009). How social are task representations? Psychol. Sci. 20 , 794–798. 10.1111/j.1467-9280.2009.02367.x [ PubMed ] [ CrossRef ] [ Google Scholar ]
- Hommel B., Müsseler J., Aschersleben G., Prinz W. (2001). The theory of event coding (TEC): a framework for perception and action planning . Behav. Brain Sci. 24 , 849–878; discussion 878–937. 10.1017/s0140525x01000103 [ PubMed ] [ CrossRef ] [ Google Scholar ]
- Hurley S., Chater N. (eds) (2005a). Mechanisms of Imitation and Imitation in Animals (Vol. 1). Cambridge, MA: MIT Press. [ Google Scholar ]
- Hurley S., Chater N. (eds) (2005b). Imitation, Human Development and Culture. (Vol. 2). Cambridge, MA: MIT Press. [ Google Scholar ]
- Jeannerod M. (1999). The 25th Bartlett Lecture. To act or not to act: perspectives on the representation of actions . Q. J. Exp. Psychol. A 52 , 1–29. 10.1080/713755803 [ PubMed ] [ CrossRef ] [ Google Scholar ]
- Jeannerod M. (2003). The mechanisms of self-recognition in humans . Behav. Brain Res. 142 , 1–15. 10.1016/s0166-4328(02)00384-4 [ PubMed ] [ CrossRef ] [ Google Scholar ]
- Jeannerod M. (2006). Motor Cognition: What Actions Tell the Self. Oxford: Oxford University Press. [ Google Scholar ]
- Kiesel A., Steinhauser M., Wendt M., Falkenstein M., Jost K., Philipp A. M., et al.. (2010). Control and interference in task switching–a review . Psychol. Bull. 136 , 849–874. 10.1037/a0019842 [ PubMed ] [ CrossRef ] [ Google Scholar ]
- Knuf L., Aschersleben G., Prinz W. (2001). An analysis of ideomotor action . J. Exp. Psychol. Gen. 130 , 779–798. 10.1037/0096-3445.130.4.779 [ PubMed ] [ CrossRef ] [ Google Scholar ]
- Koch I., Gade M., Schuch M., Philipp A. M. (2010). The role of inhibition in task switching: a review . Psychon. Bull. Rev. 17 , 1–14. 10.3758/PBR.17.1.1 [ PubMed ] [ CrossRef ] [ Google Scholar ]
- Kornblum S. (1992). “ Dimensional overlap and dimensional relevance in stimulus-response and stimulus-stimulus compatibility ,” in Tutorials in Motor Behavior (Vol. 2), eds Stelmach G. E., Requin J. (Amsterdam, NL: Elsevier; ), 743–777. [ Google Scholar ]
- Kornblum S., Hasbroucq T., Osman A. (1990). Dimensional overlap: cognitive basis for stimulus-response compatibility–a model and taxonomy . Psychol. Rev. 97 , 253–270. 10.1037//0033-295x.97.2.253 [ PubMed ] [ CrossRef ] [ Google Scholar ]
- Kuhbandner C., Pekrun R., Maier M. A. (2010). The role of positive and negative affect in the “mirroring” of other persons’ actions . Cogn. Emot. 24 , 1182–1190 10.1080/02699930903119196 [ CrossRef ] [ Google Scholar ]
- Kühn S., Brass M. (2010). The cognitive representation of intending not to act: evidence for specific non-action-effect binding . Cognition 117 , 9–16. 10.1016/j.cognition.2010.06.006 [ PubMed ] [ CrossRef ] [ Google Scholar ]
- Kühn S., Elsner B., Prinz W., Brass M. (2009). Busy doing nothing: evidence for non-action-effect binding . Psychon. Bull. Rev. 16 , 542–549. 10.3758/PBR.16.3.542 [ PubMed ] [ CrossRef ] [ Google Scholar ]
- LeMay R. P., Simon J. R. (1969). Temporal and symbolic S-R compatibility in a sequential information-processing task . J. Exp. Psychol. 80 , 558–560 10.1037/h0027447 [ CrossRef ] [ Google Scholar ]
- Liberman A. M., Cooper F. S., Shankweiler D. P., Studdert-Kennedy M. (1967). Perception of speech code . Psychol. Rev. 74 , 431–461. 10.1037/h0020279 [ PubMed ] [ CrossRef ] [ Google Scholar ]
- Logan G. D. (1988). Toward an instance theory of automatization . Psychol. Rev. 95 , 492–527 10.1037/0033-295x.95.4.492 [ CrossRef ] [ Google Scholar ]
- Logan G. D. (2002). An instance theory of attention and memory . Psychol. Rev. 109 , 376–400. 10.1037/0033-295x.109.2.376 [ PubMed ] [ CrossRef ] [ Google Scholar ]
- Logan G. D. (2007). What it costs to implement a plan: plan-level and task-level contributions to switch costs . Mem. Cognit. 35 , 591–602. 10.3758/bf03193297 [ PubMed ] [ CrossRef ] [ Google Scholar ]
- Logan G. D., Van Zandt T., Verbruggen F., Wagenmakers E.-J. (2014). On the ability to inhibit thought and action: general and special theories of an act of control . Psychol. Rev. 121 , 66–95. 10.1037/a0035230 [ PubMed ] [ CrossRef ] [ Google Scholar ]
- Lotze R. H. (1852). Medicinische Psychologie oder Physiologie der Seele [Medical Psychology or Physiology of the Soul]. Leipzig: Weidmann. [ Google Scholar ]
- MacLeod C. M. (1991). Half a century of research on the Stroop effect: an integrative review . Psychol. Bull. 109 , 163–203. 10.1037/0033-2909.109.2.163 [ PubMed ] [ CrossRef ] [ Google Scholar ]
- MacLeod C. M., Dunbar K. (1988). Training and Stroop-like interference: evidence for a continuum of automaticity . J. Exp. Psychol. Learn. Mem. Cogn. 14 , 126–135. 10.1037//0278-7393.14.1.126 [ PubMed ] [ CrossRef ] [ Google Scholar ]
- Meltzoff A. N., Moore M. K. (1977). Imitation of facial and manual gestures by human neonates . Science 198 , 75–78. 10.1126/science.897687 [ PubMed ] [ CrossRef ] [ Google Scholar ]
- Meltzoff A. N., Prinz W. (eds) (2002). The Imitative Mind: Development, Evolution and Brain Bases. Cambridge: Cambridge University Press. [ Google Scholar ]
- Memelink J., Hommel B. (2005). Attention, instruction and response representation . Eur. J. Cogn. Psychol. 17 , 674–685 10.1080/09541440540000059 [ CrossRef ] [ Google Scholar ]
- Memelink J., Hommel B. (2006). Tailoring perception and action to the task at hand . Eur. J. Cogn. Psychol. 18 , 579–592 10.1080/09541440500423228 [ CrossRef ] [ Google Scholar ]
- Memelink J., Hommel B. (2013). Intentional weighting: a basic principle in cognitive control . Psychol. Res. 77 , 249–259. 10.1007/s00426-012-0435-y [ PMC free article ] [ PubMed ] [ CrossRef ] [ Google Scholar ]
- Müller B. C. N., Kühn S., van Baaren R. B., Dotsch R., Brass M., Dijksterhuis A. (2011). Perspective taking eliminates differences in co-representation of out-group members’ actions . Exp. Brain Res. 211 , 423–428. 10.1007/s00221-011-2654-7 [ PMC free article ] [ PubMed ] [ CrossRef ] [ Google Scholar ]
- Philipp A. M., Prinz W. (2010). Evidence for a role of the responding agent in the joint compatibility effect . Q. J. Exp. Psychol. (Hove) 63 , 2159–2171. 10.1080/17470211003802426 [ PubMed ] [ CrossRef ] [ Google Scholar ]
- Piaget J. (1945/2013). Play, Dreams and Imitation in Childhood. London: Taylor and Francis. [ Google Scholar ]
- Posner M. I. (1986). Chronometric Explorations of Mind. Hillsdale, NJ: Erlbaum. [ Google Scholar ]
- Prinz W. (1984). “ Modes of linkage between perception and action ,” in Cognition and Motor Processes , eds Prinz W., Sanders A. F. (Berlin: Springer; ), 185–193. [ Google Scholar ]
- Prinz W. (1987). “ Ideo-motor action ,” in Perspectives on Perception and Action , eds Heuer H., Sanders A. F. (Hillsdale: Erlbaum; ), 47–76. [ Google Scholar ]
- Prinz W. (1990). “ A common coding approach to perception and action ,” in Relationships between Perception and Action: Current Approaches , eds Neumann O., Prinz W. (Berlin: Springer; ), 167–201. [ Google Scholar ]
- Prinz W. (1992). Why don’t we perceive our brain states? Eur. J. Cogn. Psychol. 4 , 1–20 10.1080/09541449208406240 [ CrossRef ] [ Google Scholar ]
- Prinz W. (1997). Perception and action planning . Eur. J. Cogn. Psychol. 9 , 129–154 10.1080/713752551 [ CrossRef ] [ Google Scholar ]
- Prinz W. (2012). Open Minds: The Social Making of Agency and Intentionality. Cambridge, MA: MIT Press. [ Google Scholar ]
- Prinz W. (2013). “ Common coding ,” in Encyclopedia of the Mind , eds Pashler H., Ferreira F., Kinsbourne M., Zemel R. (Los Angeles: Sage; ), 161–163. [ Google Scholar ]
- Prinz W., Aschersleben G., Koch I. (2009). “ Cognition and action ,” in Oxford Handbook of Human Action , eds Morsella E., Bargh J. A., Gollwitzer P. M. (Oxford: Oxford University Press; ), 35–71. [ Google Scholar ]
- Prinz W., De Maeght S., Knuf L. (2005). “ Intention in action ,” in Attention in Action: Advances from Cognitive Neuroscience , eds Humphreys G. W., Riddoch M. J. (Hove: Psychology Press; ), 93–107. [ Google Scholar ]
- Prinz W., Hommel B. (eds) (2002). Common Mechanisms in Perception and Action: Attention and Performance XIX. Oxford: Oxford University Press. [ Google Scholar ]
- Proctor R. W., Cho Y. S. (2006). Polarity correspondence: a general principle for performance of speeded binary classification tasks . Psychol. Bull. 132 , 416–442. 10.1037/0033-2909.132.3.416 [ PubMed ] [ CrossRef ] [ Google Scholar ]
- Proctor R. W., Reeve T. G., Van Zandt T. (1992). “ Salient-features coding in response selection ,” in Tutorials in Motor Behavior (Vol. 2), eds Stelmach G. E., Requin J. (Amsterdam, NL: Elsevier; ), 727–741. [ Google Scholar ]
- Pulvermüller F., Moseley R. L., Egorova N., Shebani Z., Boulenger V. (2014). Motor cognition-motor semantics: action perception theory of cognition and communication . Neuropsychologia 55 , 71–84. 10.1016/j.neuropsychologia.2013.12.002 [ PubMed ] [ CrossRef ] [ Google Scholar ]
- Purmann S., Badde S., Luna-Rodriguez A., Wendt M. (2011). Adaptation to frequent conflict in the Eriksen Flanker task: an ERP study . J. Psychophysiol. 25 , 50–59 10.1027/0269-8803/a000041 [ CrossRef ] [ Google Scholar ]
- Reeve T. G., Proctor R. W. (1990). “ The salient-features coding principle for spatial- and symbolic-compatibility effects ,” in Stimulus-Response Compatibility–An Integrated Perspective , eds Proctor R. W., Reeve T. G. (Amsterdam: North-Holland; ), 163–182. [ Google Scholar ]
- Rosenbaum D. A. (2014). It’s a Jungle in There: How Competition and Cooperation in the Brain Shape the Mind. Oxford, NY: Oxford University Press. [ Google Scholar ]
- Sanders A. F. (1998). Elements of Human Performance: Reaction Processes and Attention in Human Skill. Mahwah, NJ: Erlbaum. [ Google Scholar ]
- Schapiro A. C., Kustner L. V., Turk-Browne N. B. (2012). Shaping of object representations in the human medial temporal lobe based on temporal regularities . Curr. Biol. 22 , 1622–1627. 10.1016/j.cub.2012.06.056 [ PMC free article ] [ PubMed ] [ CrossRef ] [ Google Scholar ]
- Schapiro A. C., Rogers T. T., Cordova N., Turk-Browne N. B., Botvinick M. M. (2013). Neural representations of events arise from temporal community structure . Nat. Neurosci. 16 , 486–492. 10.1038/nn.3331 [ PMC free article ] [ PubMed ] [ CrossRef ] [ Google Scholar ]
- Schneider W. X. (2013). Selective visual processing across competition episodes: a theory of task-driven visual attention and working memory . Philos. Trans. R. Soc. Lond. B Biol. Sci. 368 :20130060. 10.1098/rstb.2013.0060 [ PMC free article ] [ PubMed ] [ CrossRef ] [ Google Scholar ]
- Sebanz N., Bekkering H., Knoblich G. (2006). Joint action: bodies and minds moving together . Trends Cogn. Sci. 10 , 70–76. 10.1016/j.tics.2005.12.009 [ PubMed ] [ CrossRef ] [ Google Scholar ]
- Sebanz N., Knoblich G. (2009). Prediction in joint action: what, when and where . Top. Cogn. Sci. 1 , 353–367. 10.1111/j.1756-8765.2009.01024.x [ PubMed ] [ CrossRef ] [ Google Scholar ]
- Sebanz N., Knoblich G., Prinz W. (2003). Representing others’ actions: just like one’s own? Cognition 8 , B11–B21. 10.1016/s0010-0277(03)00043-x [ PubMed ] [ CrossRef ] [ Google Scholar ]
- Sebanz N., Knoblich G., Prinz W. (2005). How two share a task: corepresenting stimulus-response mappings . J. Exp. Psychol. Hum. Percept. Perform. 31 , 1234–1246. 10.1037/0096-1523.31.6.1234 [ PubMed ] [ CrossRef ] [ Google Scholar ]
- Seymour P. H. K. (1971). Perceptual and judgmental bias in classification of word-shape displays . Acta Psychol. (Amst) 35 , 461–477. 10.1016/0001-6918(71)90004-7 [ PubMed ] [ CrossRef ] [ Google Scholar ]
- Simon J. R. (1968). Effect of ear stimulated on reaction time and movement time . J. Exp. Psychol. 78 , 344–346. 10.1037/h0026288 [ PubMed ] [ CrossRef ] [ Google Scholar ]
- Simon J. R. (1969). Reactions toward the source of simulation . J. Exp. Psychol. 81 , 174–176 10.1037/h0027448 [ PubMed ] [ CrossRef ] [ Google Scholar ]
- Simon J. R. (1990). “ The effects of an irrelevant directional cue on human information processing ,” in Stimulus-Response Compatibility–An Integrated Perspective , eds Proctor R. W., Reeve T. G. (Amsterdam: North-Holland; ), 31–86. [ Google Scholar ]
- Simon J. R., Rudell A. P. (1967). Auditory S-R compatibility: the effect of an irrelevant cue on information processing . J. Appl. Psychol. 51 , 300–304. 10.1037/h0020586 [ PubMed ] [ CrossRef ] [ Google Scholar ]
- Simon J. R., Sly P., Vilapakkam S. (1981). Effect of compatibility of S-R mapping on reactions toward the stimulus source . Acta Psychol. (Amst) 47 , 63–81. 10.1016/0001-6918(81)90039-1 [ PubMed ] [ CrossRef ] [ Google Scholar ]
- Sternberg S. (1969). The discovery of processing stages: extensions of Donders’ method . Acta Psychol. 30 , 276–315 10.1016/0001-6918(69)90055-9 [ CrossRef ] [ Google Scholar ]
- Stroop J. R. (1935). Studies of interference in serial verbal reactions . J. Exp. Psychol. 18 , 643–662 10.1037/h0054651 [ CrossRef ] [ Google Scholar ]
- Turner M. B. (1967). Philosophy and the Science of Behavior. New York: Appleton-Century-Crofts. [ Google Scholar ]
- Umiltà C., Nicoletti R. (1990). “ Spatial stimulus-response compatibility ,” in Stimulus-Response Compatibility–An Integrated Perspective , eds Proctor R. W., Reeve T. G. (Amsterdam: North-Holland; ), 89–116. [ Google Scholar ]
- van Maanen L., van Rijn H., Borst J. P. (2009). Stroop and picture-word interference are two sides of the same coin . Psychol. Bull. Rev. 16 , 987–999. 10.3758/PBR.16.6.987 [ PubMed ] [ CrossRef ] [ Google Scholar ]
- Verbruggen F., McLaren I. P. L., Chambers C. D. (2014). Banishing the control homunculi in studies of action control and behavior change . Perspect. Psychol. Sci. 9 , 497–524. 10.1177/1745691614526414 [ PMC free article ] [ PubMed ] [ CrossRef ] [ Google Scholar ]
- Virzi R. A., Egeth H. E. (1985). Toward a translational model of stroop interference . Mem. Cognit. 13 , 304–319. 10.3758/bf03202499 [ PubMed ] [ CrossRef ] [ Google Scholar ]
- Warren H. C. (1921). A History of the Association Psychology. New York: Scribner. [ Google Scholar ]
- Welford A. T. (1968). Fundamentals of Skill. London: Methuen. [ Google Scholar ]
- Welford A. T. (ed) (1980). Reaction Times. London: Academic Press. [ Google Scholar ]
- Wenke D., Atmaca S., Holländer A., Liepelt R., Baess P., Prinz W. (2011). What is shared in joint action? Issues of co-representation, response conflict and agent identification . Rev. Philos. Psychol. 2 , 147–172 10.1007/s13164-011-0057-0 [ CrossRef ] [ Google Scholar ]
- Wirth W. (1927). “ Die Reaktionszeiten [Reaction times] ,” in Handbuch der Normalen und Pathologischen Physiologie: Spezielle Physiologie des Zentralnervensystems der Wirbeltiere [Handbook of Normal and Pathological Physiology: Specific Physiology of the Central Nervous System] (Vol. 10), eds Bethe A., von Bergmann G., Embden G., Ellinger A. (Berlin: Springer; ), 525–599. [ Google Scholar ]
The Nature of Task Set Representations in Working Memory
Ieee account.
- Change Username/Password
- Update Address
Purchase Details
- Payment Options
- Order History
- View Purchased Documents
Profile Information
- Communications Preferences
- Profession and Education
- Technical Interests
- US & Canada: +1 800 678 4333
- Worldwide: +1 732 981 0060
- Contact & Support
- About IEEE Xplore
- Accessibility
- Terms of Use
- Nondiscrimination Policy
- Privacy & Opting Out of Cookies
A not-for-profit organization, IEEE is the world's largest technical professional organization dedicated to advancing technology for the benefit of humanity. © Copyright 2024 IEEE - All rights reserved. Use of this web site signifies your agreement to the terms and conditions.
Advertisement
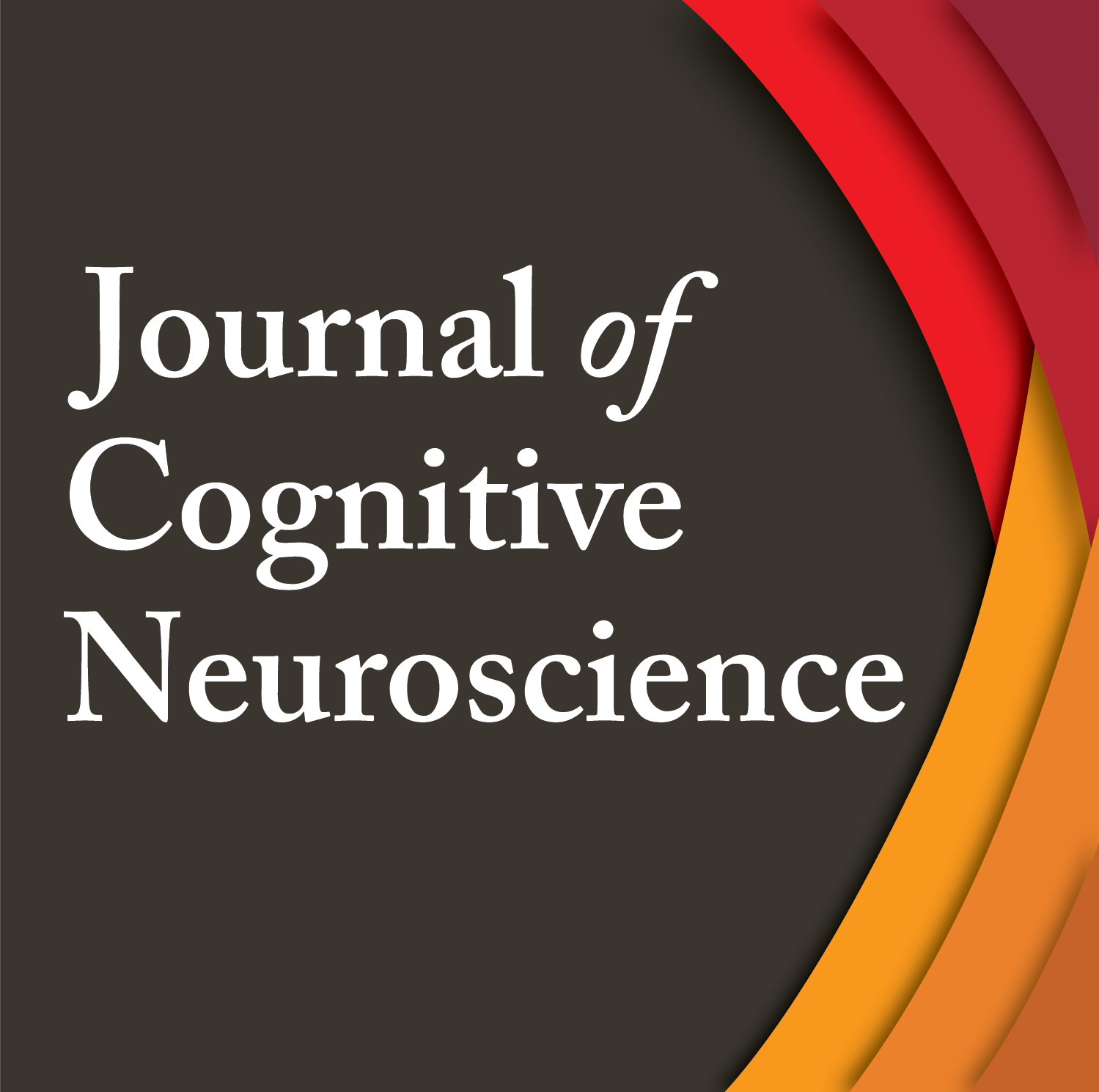
- Previous Article
The Nature of Task Set Representations in Working Memory
- Cite Icon Cite
- Permissions
- Article contents
- Figures & tables
- Supplementary Data
- Peer Review
- Search Site
Atsushi Kikumoto , Ulrich Mayr; The Nature of Task Set Representations in Working Memory. J Cogn Neurosci 2017; 29 (11): 1950–1961. doi: https://doi.org/10.1162/jocn_a_01173
Download citation file:
- Ris (Zotero)
- Reference Manager
Selection and preparation of action plans (task sets) is often assumed to occur in working memory (WM). Yet, the absence of consistent evidence that WM capacity and task selection efficiency is correlated raises questions about the functional relationship between these two aspects of executive control. We used the EEG-derived contralateral delay activity (CDA) to index the WM load of task sets. In Experiment 1, we found a CDA set size effect (2 vs. 4 stimulus–response [S-R] rules) for high-WM, but not for low-WM, individuals when S-R sets were novel. In contrast, when only four task sets were presented throughout the experiment, we observed a sustained yet set size-independent use of WM for high-WM participants. Moreover, Experiment 2 showed an increase of the CDA in situations with task conflict, and this effect was larger the more that participants experienced RT conflict effects. Combined, these results indicate that even highly familiar S-R settings are maintained in WM, albeit in a compressed manner, presumably through cues to long-term memory representations. Finally, participants with low-WM capacity represented even familiar tasks in a load-dependent manner, suggesting that the establishment of effective retrieval structures itself is a capacity-limited process.
Client Account
Sign in via your institution, email alerts, related articles, related book chapters, affiliations.
- Online ISSN 1530-8898
- Print ISSN 0898-929X
A product of The MIT Press
Mit press direct.
- About MIT Press Direct
Information
- Accessibility
- For Authors
- For Customers
- For Librarians
- Direct to Open
- Open Access
- Media Inquiries
- Rights and Permissions
- For Advertisers
- About the MIT Press
- The MIT Press Reader
- MIT Press Blog
- Seasonal Catalogs
- MIT Press Home
- Give to the MIT Press
- Direct Service Desk
- Terms of Use
- Privacy Statement
- Crossref Member
- COUNTER Member
- The MIT Press colophon is registered in the U.S. Patent and Trademark Office
This Feature Is Available To Subscribers Only
Sign In or Create an Account
Main content
Links to this project.
Loading projects and components...
Multi-level decoding of task set representations in neurophysiological data during cognitive flexibility
- Fork this Project
- Duplicate template
- View Forks (1)
- Bookmark Remove from bookmarks
- Request access Log in to request access
- Ádám Takács
Date created: 2021-01-22 11:46 AM | Last Updated: 2021-11-12 08:17 AM
Category: Project
Description: To achieve goals, cognitive flexibility is important. Cognitive theories assume that the representation of task rules is central to understand cognitive flexibility. However, how this representational dynamic is implemented on a neurophysiology level is widely elusive. Using EEG-based MVPA-methods we show that relevant representational content is evident at multiple levels in the neurophysiological signal (i.e., during stimulus-related processing, response selection and motor response execution), occurs parallel in time and relates to different brain regions. The intensity with which these different representational levels are processed dynamically decreases and increases. The temporal stability of these representations can, however, hardly explain differences in behavioral performance. Rather, inhibitory control processes associated with left orbito-frontal regions and inferior parietal regions and selectively acting on invalid motor response representations are relevant. The study shows how propositions from cognitive theories stressing the importance of mental representations during cognitive flexibility are implemented on a neurophysiological level.
Link other OSF projects
- Registrations
Loading citations...
Get more citations
Example datasets for STAR Protocol
Loading logs...
Recent Activity
Start managing your projects on the OSF today.
Free and easy to use, the Open Science Framework supports the entire research lifecycle: planning, execution, reporting, archiving, and discovery.
Copyright © 2011-2024 Center for Open Science | Terms of Use | Privacy Policy | Status | API TOP Guidelines | Reproducibility Project: Psychology | Reproducibility Project: Cancer Biology
Europe PMC requires Javascript to function effectively.
Either your web browser doesn't support Javascript or it is currently turned off. In the latter case, please turn on Javascript support in your web browser and reload this page.
Search life-sciences literature (43,943,223 articles, preprints and more)
- Free full text
- Citations & impact
- Similar Articles
What are task-sets: a single, integrated representation or a collection of multiple control representations?
Author information, affiliations.
- Rangelov D 1
ORCIDs linked to this article
- Rangelov D | 0000-0001-7648-6447
Frontiers in Human Neuroscience , 03 Sep 2013 , 7: 524 https://doi.org/10.3389/fnhum.2013.00524 PMID: 24027513 PMCID: PMC3759751
Abstract
Free full text , dragan rangelov.
1 Department Psychologie, Allgemeine und Experimentelle Psychologie I, Ludwig-Maximilians-Universität München, München, Germany
Thomas Töllner
Hermann j. müller.
2 Birkbeck College, University of London, London, UK
Michael Zehetleitner
Performing two randomly alternating tasks typically results in higher reaction times (RTs) following a task switch, relative to a task repetition. These task switch costs (TSC) reflect processes of switching between control settings for different tasks. The present study investigated whether task sets operate as a single, integrated representation or as an agglomeration of relatively independent components. In a cued task switch paradigm, target detection (present/absent) and discrimination (blue/green/right-/left-tilted) tasks alternated randomly across trials. The target was either a color or an orientation singleton among homogeneous distractors. Across two trials, the task and target-defining dimension repeated or changed randomly. For task switch trials, agglomerated task sets predict a difference between dimension changes and repetitions: joint task and dimension switches require full task set reconfiguration, while dimension repetitions permit re-using some control settings from the previous trial. By contrast, integrated task sets always require full switches, predicting dimension repetition effects (DREs) to be absent across task switches. RT analyses showed significant DREs across task switches as well as repetitions supporting the notion of agglomerated task sets. Additionally, two event-related potentials (ERP) were analyzed: the Posterior-Contralateral-Negativity (PCN) indexing spatial selection dynamics, and the Sustained-Posterior-Contralateral-Negativity (SPCN) indexing post-selective perceptual/semantic analysis. Significant DREs across task switches were observed for both the PCN and SPCN components. Together, DREs across task switches for RTs and two functionally distinct ERP components suggest that re-using control settings across different tasks is possible. The results thus support the “agglomerated-task-set” hypothesis, and are inconsistent with “integrated task sets.”
- Introduction
Surviving in an environment in which both internal and external conditions change dynamically presupposes an ability to change between control settings for old and new tasks. A successful switch implies that the set of expectations about the environment (the topic of the present special issue) which was relevant in the previous task episode is replaced by one appropriate for the task at hand. Such switching processes are usually investigated in paradigms in which two or more different tasks vary across trials, requiring a change, on task-switch trials, in the internal control settings so as to fit the current task requirements. In cued task switching , prior to the stimulus display's onset, a cue is presented specifying the task to be performed on the upcoming trial. Across two consecutive trials, the task can either repeat or change. Reaction times (RTs) and errors are typically elevated for task switches relative to repetitions (Allport et al., 1994 ; Rogers and Monsell, 1995 ). Such task switch costs (TSCs) imply the existence of extra, time-consuming processes invoked on task switch trials, but not (or to a lesser degree) on repetition trials. To comprehensively account for TSCs, answers to two related, yet separable questions are necessary: first, what cognitive mechanisms give rise to the TSCs, and, second, how are the representations on which these mechanisms operate organized? The present study focused on the latter issue—more precisely, on whether or not having to change some expectations automatically triggers a change in all expectations about task-relevant properties of the environment.
Determinants of TSCs
The available literature offers two dominant approaches to the question of what cognitive mechanisms give rise to TSCs. According to the first, TSCs reflect the extra time it takes to reconfigure control settings from the previously relevant to the currently relevant task demands (Monsell and Driver, 2000 ; Monsell et al., 2003 ). Reconfiguration is achieved by means of a special executive function (or set of functions) which is active on task switches and inactive on task repetitions. An alternative approach assumes that TSCs reflect interference between the previously relevant and the currently required control settings, which are concurrently active on task switch trials (Allport et al., 1994 ; Gilbert and Shallice, 2002 ; Waszak et al., 2003 ). TSCs arise because the interference is weaker on task repetition than on task switch trials. Finally, a hybrid, reconfiguration-interference account has also been proposed, postulating that TSCs reflect a mixture of reconfiguration and interference processes (Meiran, 1996 , 2000 , but see Meiran et al., 2008 ). Critically, irrespectively of what mechanisms produce TSCs, all accounts assume that performance of a task is controlled by a set of representations that, following a task switch, are no longer appropriate. Thus, to meet the changed environmental demands, the control representations would have to change, too. Given this, the present study was designed to address the question of what representations change across task switches.
Conceptually, tasks can differ in all or some of the following respects: (i) criteria for spatial-attentional selection of the task-relevant stimulus; (ii) criteria for the identification of task-relevant stimulus properties; and (iii) task-appropriate stimulus-response (S-R) mappings. The set of cognitive representations specifying these criteria is considered collectively to constitute the task set. The available literature supports the notion of composite task set representations. For instance, Meiran ( 2000 ) demonstrated that while switching identification criteria can be performed in advance, actually performing the task is necessary for switching S-R mappings. Furthermore, Hübner et al. ( 2001 ) showed that the magnitude of TSCs increases with the number of task set components to be switched. Finally, a number of electrophysiological and imaging studies revealed neural correlates of a switch to co-vary with what task set component is being switched (Rushworth et al., 2002 , 2005 ; Ravizza and Carter, 2008 ; Chiu and Yantis, 2009 ; Esterman et al., 2009 ; Hakun and Ravizza, 2012 ). In summary, the available evidence converges on the view that task sets consist of several dissociable representations controlling different cognitive processes in the stimulus-response chain. However, it remains unclear whether, on task switch trials, different components are changed relatively independently of each other, as would be predicted by the notion of “agglomerated task sets.”
Studies investigating whether or not it is possible to change only those task set components that require a change and to reuse shared components across different tasks yielded somewhat inconsistent findings. For example, Arrington et al. ( 2003 ) asked their participants to report, on different trials, either the stimulus height, width, color, or luminance. Smaller TSCs were found for switches across similar tasks (e.g., switching from color to luminance discrimination) relative to switches across dissimilar tasks (e.g., from height to luminance discrimination), suggesting that some reusing of control representations across different tasks is possible. By contrast, Vandierendonck et al. ( 2008 ) had their participants discriminate either the parity (odd/even) or the magnitude (greater/smaller than four) of stimuli consisting of several identical digits (e.g., five instances of the digit three). On different trials, either the number of digits or the digits themselves were task-relevant. The critical comparison was between trials on which both the identification criterion (parity vs. magnitude) and the stimulus attribute (number vs. digit) switched, and trials on which only one criterion switched (e.g., from digit parity to magnitude discrimination). Although partial switches in principle allowed for old control representations to be reused, no difference was observed between full and partial switches—indicating that, following a task switch, all task-set components are reset. Finally, in a study very similar to Kleinsorge ( 2004 ); Vandierendonck et al. ( 2008 ) (see also Kleinsorge and Heuer, 1999 ) observed partial repetition costs , that is, partial switches took actually more time to be implemented than full switches. Kleinsorge explained these findings by assuming a hierarchical organization of task sets, according to which having to change task set components situated earlier in the stimulus-response chain (e.g., identification criteria) would trigger a switch in all subsequent criteria (e.g., S-R mapping rules). In summary, the available literature suggests that following a task-switch, task-sets are sometime reset, sometimes switched, and sometimes reused. It should be borne in mind, however, that the various studies used (i) different paradigms and (ii) different types of switches. These differences will be discussed in more detail in the General Discussion.
Resetting, switching or reusing task-set components
Depending on what tasks precisely vary across trials, task switching could necessitate changes of either all components (full switches) or only those components in which the two tasks differ (partial switches). To illustrate, consider a paradigm in which stimulus displays consist of many identical items with one of them (the singleton target) being different in either color or orientation from the rest. Participants are to, on different trials, either simply detect the presence vs. absence of the singleton target or discriminate its exact features, with a cue, presented prior to the display onset, announcing what task (detection or discrimination) is to be performed. Independently of the task sequence, the task-relevant dimension can also repeat or change. The dimension in which the singleton target is defined, although not informative about the response to be performed (i.e., knowing the dimension would not specify the exact response!), would be informative for both spatial-attentional selection and post-selective identification processes.
Concerning target selection, the available evidence suggests that when the dimension repeats across trials, spatial selection is speeded relative to dimension changes, as elaborated in the Dimension-Weighting Account of Müller and colleagues (e.g., Found and Müller, 1996 ; Müller and Krummenacher, 2006 ; Müller, 2010 ). Since both singleton detection and singleton discrimination tasks would require spatial selection, spatial selection processes for both tasks would be sensitive to the singleton dimension. Accordingly, dimension repetition effects (DREs) would be expected across (task repetition and switch) trials of both detection and discrimination tasks.
In contrast to the shared spatial selection, post-selective identification processes should differ between the two tasks. On the one hand, fast and accurate singleton detection can be achieved by simply determining the presence/absence of a singleton, while information about what the singleton features precisely are is not strictly necessary for performing the task. Consistent with this, there is evidence that the response-irrelevant singleton features are not processed up to the level at which they become available for explicit report (Müller et al., 2004 ). On the other hand, encoding singleton features from a particular dimension is critical for the singleton discrimination task. With these differential task requirements in mind, it is likely that post-selective identification processes differ between tasks: the singleton dimension is important for identification in the discrimination, but not in the detection task (see Töllner et al., 2012b , for supporting EEG data).
Differences between detection and discrimination tasks would determine what can be and is reused across trials. Following performance of a target-present detection task, the dimension should have been encoded and thus be available for reuse only for spatial selection. Following a discrimination task, the dimension should have been encoded in and thus be available for reuse for both the spatial selection and post-selective identification processes. Thus, the task on trial n − 1, or prime trial, determines what is available for reusing. By contrast, the task on trial n , or probe trial, determines what is reused: in the detection task, reusing dimension information would facilitate just spatial selection, while in discrimination task reusing dimension information would facilitate both selection and identification processes. Consequently, on the hypothesis of agglomerated task sets, comparable DREs would be expected for detection → detection and detection → discrimination sequences, because across these sequences, only spatial selection criteria are available and reused. By contrast, following performance of the discrimination task, both spatial selection and identification criteria would be available for reuse, but they would be reused only on the current discrimination trial, predicting stronger DREs for discrimination → discrimination relative to discrimination → detection sequences.
In contrast to the notion of agglomerated task sets, that of integrated task sets would predict that following any switch, the task set would be reset; accordingly, there should not be a difference between full and partial switches. Finally, the notion of hierarchical task sets would predict a reversal of DREs, that is: switching from, e.g., detection to discrimination, would also switch the expected dimension, resulting in worse performance following dimension repetitions relative to dimension switches.
ERP components sensitive to the spatial selection and post-selective identification components
In the paradigm described above, preparatory adjustments with regard to the task-relevant (singleton) dimension are not possible since the task cue is not dimension-specific 1 . Consequently, the analyses of partial-switch effects for spatial selection and post-selective identification in the EEG domain have focused on areas and ERP components sensitive to the implementation of these processes.
As an index of spatial-attentional selection, parameters of the Posterior-Contralateral-Negativity (PCN, or N2-posterior-contralateral 2 ) have been analyzed. This component manifests as an increased negativity at posterior scalp electrode sites contralateral to the singleton position, relative to ipsilateral electrode sites, in the 175–300-ms time range post-stimulus. PCN parameters are considered to reflect the dynamics of spatial selection processes (Luck and Hillyard, 1994 ; Eimer, 1996 ; Töllner et al., 2011 ). Importantly, PCN latencies are shorter for dimension repetitions relative to changes (Töllner et al., 2008 ). On the assumption of agglomerated task sets, substantial DREs are expected for both task repetitions and switches. By contrast, the assumption of integrated tasks sets would predict no DRE across task switches.
The second component of interest was the Sustained Posterior Contralateral Negativity (SPCN), manifesting as an increased negativity over posterior electrodes contralateral to the target, relative to ipsilateral electrodes, starting from 350–400-ms post-stimulus. The SPCN component is considered to be sensitive to the processing demands following stimulus selection (Jolicoeur et al., 2008 ). Importantly, the SPCN is weaker (Mazza et al., 2007 ; Töllner et al., 2013 ) in tasks that do not necessarily require perceptual analysis following stimulus selection (e.g., singleton detection task), and more prominent (ibid.) in tasks that require stimulus analysis up to the feature levels (e.g., in singleton discrimination task). Thus, the available evidence predicts a stronger SPCN for the discrimination task, which requires explicit feature discrimination, relative to the detection task, in which post-selective processing is not necessary for an accurate response. Furthermore, the hypothesis of agglomerated task sets predicts stronger DREs in the SPCN time range on trials preceded by (a trial with) the discrimination task, in which post-selective identification should be sensitive to the dimensional identity of the singleton, relative to trials preceded by the detection task, for which it is sufficient to determine that the selected item is a singleton, without necessarily identifying its precise dimension or feature properties.
Participants
Sixteen males (mean age 29 years, 2 left-handed), all with normal color vision and normal or corrected-to-normal visual acuity, participated in the experiment for monetary compensation. All participants had extensive experience with psychophysical tasks and all were naïve as to the purpose of the experiment. Due to excessive eye blinking, two participants were excluded from the analyses.
Stimuli were presented on a 17″ CRT monitor with a 1024 × 768 pixels resolution and an 85-Hz refresh rate. Custom written C ++ code controlled stimulus presentation and recorded responses. The experiment was conducted in a dimly lit, acoustically and electromagnetically shielded room. Head-to-monitor distance was 60 cm.
Stimuli and procedure
Stimulus displays (Figure (Figure1) 1 ) were presented on a gray background (19 cd/m 2 , CIE x = 0.292, y = 0.307) and consisted of 38 vertical yellow (0.388, 0.520) bars arranged around three concentric—inner, middle, and outer—circles with 8, 12, and 18 items, respectively. Single bars subtended 0.4 × 1.9° of visual angle, and the diameters of the three circles were 5, 10, and 15°, respectively. On target-present trials, one of the bars on the middle circle (excluding the two positions along the vertical meridian) was replaced by either a blue (0.275, 0.541) or a green (0.211, 0.263) color singleton target or a right-titled (12° clockwise from vertical) or left-tilted (12° counter-clockwise) orientation singleton target, matched in luminance to the distractor bars (68 cd/m 2 ).

Illustration of stimulus displays across different inter-trial sequences .
Every trial started with a task cue (i.e., the word “detection” or “discrimination”) shown for 1000 ms, followed by a 500 ± 50-ms blank screen. Next, the stimulus display appeared for 200 ms, followed by a blank screen until response. In case of response errors, the standard intertrial interval (1000 ms) was doubled. Responses were given via pressing the left and right mouse keys using the left- and right-hand thumbs, respectively. Stimulus displays were identical for both tasks, the difference being that, in the detection task, participants were required to discern the presence (on 60% of detection task trials) vs. the absence of a singleton target by pressing the corresponding response key with two possible S-R mappings: (i) target-present → R 1 , -absent → R 2 and, respectively, (ii) target-absent → R 1 , -present → R 2 . In the discrimination task, a singleton was always present, with participants having to report the feature that distinguished it from distractors (blue, green, left-, and right tilted). Same-dimension singletons (e.g., blue and green) required different responses (e.g., R 1 and R 2 ), while singletons from different dimensions (e.g., blue and left-tilted) were mapped to a same response, resulting in four possible S-R mappings: (i) blue or left-tilted → R 1 , green or right-tilted → R 2 , (ii) green or left-tilted → R 1 , blue or right-tilted → R 2 , (iii) blue or right-tilted → R 1 , green or left-tilted → R 2 , and (iv) green or right-tilted → R 1 , blue or left-tilted → R 2 . The two possible S-R mappings in the detection task and the four S-R mappings in the discrimination task yielded eight different S-R mapping combinations, which were counterbalanced across participants.
The task (detection vs. discrimination) and the target's dimension (color vs. orientation) were randomly selected on every trial, resulting in four task sequences (detection on both prime and probe trials; discrimination on both trials; detection on prime, discrimination on probe trial; and discrimination on prime, detection on probe trial) and two dimension sequences (repetition/change) across consecutive trials. Relevant dimensions were sampled with equal probability, however, in order to ensure comparable numbers of target-present trials across the two tasks, the detection task was made more frequent (3:2 ratio).
Prior to experiment proper, participants received 1–4 practice blocks (80 trials per block); practice was terminated once a participant achieved ≤ 10% errors per block. All participants met the criterion after 2–4 blocks. Following practice, participants completed 1920 trials (in ca. 3 h), split in two equal-length sessions with a 15–30 min break in between.
EEG recording and analyses
The EEG was sampled at 1 KHz using Ag/AgCl active electrodes (actiCAP system; Brain Products, Munich) from 64 scalp sites, arranged according to the 10–10 System (American Electroencephalographic Society, 1994), and amplified using BrainAmp amplifiers (BrainProducts, Munich) with a 0.1–250-Hz bandpass filter. Impedances were kept below 5 kΩ. Electrodes were online referenced to FCz and re-referenced offline to averaged mastoids. Electrodes placed at the outer canthi of the eyes and the superior and inferior orbits monitored blinks and eye movements. Non-stereotyped noise was removed by visual inspection, followed by high-pass filtering using a Butterworth infinite impulse response filter at 0.5 Hz (24 dB/Oct). An infomax independent-component analysis was run to identify and remove effects of eye movements and blinks. Next, continuous EEG was epoched into −200–600 ms segments time-locked to stimulus display onset. Baseline correction was performed based on the −200–0 ms pre-stimulus interval. Target-absent trials, trials preceded by a target-absent trial, error response trials, as well as trials preceded by an error were excluded from the analyses. Finally, trials with (i) signals exceeding ±60 μV, (ii) bursts of electromyographic activity (permitted maximal voltage steps/sampling point of 50 μV), or (iii) activity lower than 0.5 μV within intervals of 500 ms (indicating dead channels) were removed from further analyses on an individual channel basis. The remaining trials (mean = 111 trials per participant per condition, SD = 5 trials) were sorted according to experimental conditions, and averaged on an individual-channel basis.
Data analyses
Analysis of EEG signals focused on two event-related potentials (ERPs). The ERP components were quantified by subtracting ERPs measured at lateral parieto-occipital electrodes (PO7/PO8) ipsilateral to the target's location from contralateral ERPs. The PCN peak latencies and amplitudes were defined per participant as the maximum negative-going deflection in the time period 170–270 ms post-stimulus. SPCN amplitudes were defined as the average of the time window the 430–510-ms post-stimulus.
Mean RTs, error rates, PCN peak latencies and amplitudes, and SPCN mean amplitudes were computed for correct target-present probes for which the primes were also correct target-present trials. Dependent measures were submitted to repeated-measures ANOVAs (RANOVAs) in three different analyses. First, overall task differences and TSCs were assessed in a RANOVA with main terms for (i) task on probe trial (detection vs. discrimination) and (ii) task sequence (repetition vs. change) across prime and probe trials. The second analysis focused on indices of re-using processes across trials, that is, on dimension-repetition effects. Because re-use is expected to co-vary with what is available for re-using, which is determined on prime trials, the second set of analyses used a RANOVA with main terms for (i) task on prime trial, (ii) task on probe trial, and (iii) dimension sequence (repetition vs. change) across trials. Third, effects of response sequence on mean RTs and error rates were analyzed. Because only a small number of trials were available per cell for this analysis, the corresponding ERPs could not be examined.
Analyses of overall task differences
Behavioral results.
Inspection of the overall mean RTs and error rates (shown in Table Table1) 1 ) revealed performance to be faster and more accurate for the detection task (mean RTs = 594 ms, mean error rate = 5.2%) than for the discrimination task (670 ms, 6.5%). Furthermore, task repetitions (598 ms, 4.5%) yielded faster and more accurate performance relative to task changes (666 ms, 7.1%), indicating substantial TSCs for both RTs and error rates (TSC RT = 68 ms, TSC errors = 2.6%). Finally, switching from discrimination to detection incurred greater TSCs (81 ms, 3%) than switching from detection to discrimination (57 ms, 2.3%).
Mean RTs (SE M ) and percentage of errors (SE M ) on probe trials for the two different tasks, dependent on the task sequence .
These observations were confirmed by RANOVAs of the mean RTs and error rates with main terms for task on probe trial (detection vs. discrimination) and task sequence (task- repetition vs. -change). The analysis of the mean RTs proved main effects of task, F (1,13) = 36.44, p < 0.01, η 2 p = 0.74, task sequence, F (1,13) = 18.65, p < 0.01, η 2 p = 0.60, as well as their interaction, F (1,13) = 6.18, p < 0.05, η 2 p = 0.32, to be significant. The RANOVA of the mean error rates yielded likewise a significant main effect of task sequence, F (1,13) = 20.94, p < 0.01, η 2 p = 0.62, without, however, any of the other effects reaching significance (all F < 2.17, all p > 0.16).
ERP results
The stimulus-locked ERP waves obtained for the detection and discrimination tasks are shown on Figure Figure2. 2 . Figure Figure2A 2A depicts the time course separately for electrodes contra- and ipsilateral to the target position, averaged across target positions 3 (left vs. right), while Figure Figure2B 2B depicts the difference between contra- and ipsilateral electrodes. As can be seen from Figure Figure2B, 2B , substantial lateralization effects, confirmed by t -tests against zero, were observed in the PCN time range (170–270 ms) for both the detection (−2.17 μV, p < 0.01) and the discrimination task (−2.41, p < 0.01). Similar to what we already reported in Töllner et al. ( 2012a ), PCN amplitude was higher for the discrimination than for the detection task, with comparable PCN latencies across the two tasks (221 and 222 ms for detection and discrimination, respectively). The RANOVA of the PCN latencies with main terms for (i) task on probe trial and (ii) task sequence yielded no significant effects (all F < 1). The RANOVA of the PCN amplitudes yielded a significant main effect of task, F (1,13) = 6.61, p < 0.05, η 2 p = 0.43; the main effect of task sequence non-significant ( F < 1); the task × task sequence interaction approached significance, F (1,13) = 3.70, p = 0.08, η 2 p = 0.22), owing to the fact that switching task tended to increase the PCN amplitude for the detection task (−2.12 μV and −2.22 μV for detection → detection and discrimination → detection sequences, respectively), while tending to decrease the amplitude for the discrimination task (−2.52 and −2.29 μV for discrimination → discrimination and detection → discrimination sequences, respectively).

Stimulus-locked ERPs: (A) for the ipsi-and contralateral electrodes relative to the target position, separately for the detection and discrimination tasks; (B) difference between contra- and ipsilateral electrodes. For the purpose of the presentation, data are presented filtered with 30-Hz low-pass filter.
Furthermore, as Figure Figure2B 2B shows, and as confirmed by t -tests against zero, lateralization effects in SPCN time range (430–510 ms) were observed for the discrimination task (mean amplitude = −0.36 μV, p < 0.01), but not for the detection task (0.14 μV, p = 0.18). A RANOVA of the SPCN mean amplitude with main terms for task and task sequence revealed the main effect of task to be significant, F (1,13) = 72.74, p < 0.01, η 2 p = 0.85, with no other effects reaching significance (all F < 1).
Analyses of re-using control settings across tasks
Figure Figure3 3 depicts the mean RT and error rate for a given task on the probe trial (detection, discrimination) dependent on the task on the prime trial (detection, discrimination), separately for dimension repetitions and changes (dimension sequence). As can be seen, RTs were faster for dimension repetitions (blue bars) than for dimension changes (red bars), in all conditions; error rates followed a similar pattern. These observations were confirmed by a RANOVA of the mean RTs, which revealed all three main effects (all F > 6.18, all p < 0.05), all two-way interactions (all F > 18.17, all p < 0.01), and the three-way interaction between task on prime trial, task on probe trial, and dimension sequence, F (1,13) = 21.27, p < 0.01, η 2 p = 0.62, to be significant. An analogous RANOVA of the error rates yielded the following significant effects: main effect of dimension sequence, F (1,13) = 11.39, p < 0.01, η 2 p = 0.47; task on probe trial × dimension sequence interaction, F (1,13) = 33.88, p < 0.05, η 2 p = 0.28; task on prime trial × task on probe trial interaction, F (1,13) = 20.94, p < 0.01, η 2 p = 0.62; and task on prime trial × task on probe trial × dimension sequence interaction, F (1,13) = 8.16, p < 0.5, η 2 p = 0.39.

Mean RTs (upper panels) and error rates (lower panels) for the different task sequences, separately for dimension repetitions (blue bars) and changes (red bars). Vertical lines denote ±1SEM.
To further investigate the significant three-way interactions, separate RANOVAs were run dependent on the specific task on prime trials (detection and, respectively, discrimination), with main terms for task on probe trial and dimension sequence. With the detection task on prime trials (left-hand panels in Figure Figure3), 3 ), the analysis of the (probe-trial) RTs revealed both main effects to be significant: task on probe trial [145-ms difference between discrimination and detection tasks, F (1,13) = 34.04, p < 0.01, η 2 p = 0.72] and dimension sequence [23-ms difference between dimension changes vs. -repetitions, F (1,13) = 22.00, p < 0.01, η 2 p = 0.63]; the interaction between the two was far from significance ( F < 1). The RANOVA of the error rates revealed a significant main effect of task on probe trials [3% difference between discrimination and detection, F (1,13) = 16.68, p < 0.01, η 2 p = 0.56], and a marginally significant main effect of dimension sequence, with dimension repetitions yielding 1.1% more accurate performance than dimension changes, F (1,13) = 4.35, p = 0.06; the interaction between the two was far from significance ( F < 1).
With the discrimination task on prime trials (right-hand panels in Figure Figure3), 3 ), a RANOVA of the (probe-trial) RTs revealed the main effect of dimension sequence, F (1,13) = 36.32, p < 0.01, η 2 p = 0.81, and the task on probe trial × dimension sequence interaction, F (1,13) = 32.01, p < 0.01, η 2 p = 0.71, to be significant. The interaction was caused by the dimension repetition (vs. change) effect being much larger for discrimination → discrimination sequences (125 ms) than for discrimination → detection sequences (18 ms). For the errors (on probe trials), the main effect of dimension sequence proved significant, F (1,13) = 7.67, p < 0.05, η 2 p = 0.37, with accuracy being 1.4% higher for dimension repetitions relative to changes. The task on probe trial × dimension sequence interaction was also significant, F (1,13) = 8.31, p < 0.5, η 2 p = 0.40, with a stronger DRE for the discrimination relative to the detection task on the probe trial (3.2 vs. −0.3%, respectively).
In summary, significant DREs were observed in all experimental conditions. When the task on the prime trial required simple target detection, DREs were comparable for detection and discrimination tasks on the probe trial. By contrast, with the task on prime trial required target discrimination, stronger DREs were observed for the discrimination task, relative to the detection, to be performed on the probe trial.
Lateralized ERPs are depicted in Figure Figure4 4 for the probe trials, separately for the different tasks on prime and on probe trials, as well as across different dimension sequences. As can be seen from Figure Figure4, 4 , the PCN latency was delayed for dimension changes (red lines) relative to dimension repetitions (blue lines), in all conditions. In the SPCN time range DREs were evident only for (probe) trials following the discrimination task.

Group-averaged time course of PCN and SPCN components for the different task sequences, separately for dimension repetitions (blue) and changes (red). Significant dimension repetition effects for the peak PCN latency and the mean SPCN amplitude are indicated. For the purpose of presentation, a 30-Hz low-pass filter was applied; data analyses were performed over individual, unfiltered data.
PCN analyses
For (probe) trials preceded by the detection task (left-hand side of Figure Figure4), 4 ), a RANOVA of the PCN peak latencies revealed only a significant main effect of dimension sequence, with dimension repetitions being 17 ms faster than dimension changes, F (1,13) = 15.41, p < 0.01, η 2 p = 0.54; no other effects reached significance (all F < 1). An analogous RANOVA for trials preceded by the discrimination task (right-hand side) also yielded only a significant main effect of dimension sequence [14-ms DRE, F (1,13) = 5.09, p < 0.05, η 2 p = 0.23], with no other effects reaching significance (all F < 1).
SPCN analyses
With the task on the prime trial requiring target detection, analysis of the (probe-trial) mean SPCN amplitudes revealed only a significant main effect of task on probe trial, with an overall stronger SPCN component for the discrimination relative to the detection task (−0.37 vs. 0.18 μV), F (1,13) = 25.70, p < 0.01, η 2 p = 0.66; no other effects reached significance (all F < 1.94, all p > 0.18). With the discrimination task on the prime trial, an analogous analysis also yielded a significant main effect of task on probe trial, F (1,13) = 30.16, p < 0.01, η 2 p = 0.70, with the significant SPCN for the discrimination relative to the insignificant SPCN for the detection task (−0.36 vs. 0.10 μ V). Importantly, the main effect of dimension sequence was also significant, F (1,13) = 5.33, p < 0.05, η 2 p = 0.29, with the SPCN amplitude being stronger for dimension changes relative to dimension repetitions (−0.23 vs. −0.02 μ V); the interaction task on probe trial x dimension sequence was far from significance ( F < 1).
In summary, analyses of the ERPs revealed longer PCN peak latencies for dimension changes vs. repetitions independently of the task on prime or probe trial; SPCN mean amplitudes were overall larger for the discrimination than for the detection task on probe trial, while significant DREs in the SPCN time interval were observed only for trials preceded by the discrimination task.
Analyses of response-sequence effects
As can be seen from Table Table2, 2 , mean RTs were overall fastest for full repetitions (same task, dimension, and response), intermediate for partial repetitions, and slowest for full changes. By contrast, the errors varied as a function of task sequence. Importantly, though, there was no evidence that partial repetitions resulted in less accurate performance relative to full changes.
Mean RTs (SE M ) and percentage of errors (SE M ) across different task-, dimension- and response a sequences .
These observations were confirmed by three-ways RANOVAs (task- × dimension- × response sequence) of the mean RTs and error rates with a focus on the main effect of and interactions involving response sequence. The RT analysis showed the main effect of response sequence to be significant, F (1,13) = 6.41, p < 0.05, η 2 p = 0.33, as well as the interaction of this factor with dimension sequence, F (1,13) = 9.04, p < 0.01, η 2 p = 0.41, owing to a larger response repetition benefit when the dimension repeated (30 ms, p < 0.01) rather than changed (5 ms, p = 0.49). The task sequence × response sequence interaction was marginally significant, F (2,26) = 1.67, p = 0.07, η 2 p = 0.18, suggestive of a larger response repetition benefit for the discrimination task (29 ms, p < 0.01, and 23 ms, p < 0.01, for detection → discrimination and discrimination → discrimination sequences, respectively) relative to the detection task (−1 ms, p = 0.93). The three-way interaction did not reach significance, F = 1.67, p = 0.27.
Concerning the error analysis, the main effect of response sequence was non-significant ( F < 1). However, response sequence interacted with dimension sequence, F (1,13) = 18.43, p < 0.01, η 2 p = 0.59, and task sequence, F (2,26) = 18.52, p < 0.01, η 2 p = 0.59; and the response- × dimension- × task sequence interaction was significant, F (2,26) = 8.55, p < 0.01, η 2 p = 0.40. Post-hoc analyses revealed the pattern of response sequence effects (repetition benefit vs. cost) to vary across task and dimension sequences. For discrimination → detection task sequences, dimension repetitions were associated with response repetition costs (−5.4%, p < 0.01), whereas dimension changes yielded response repetition benefits (4.1%, p < 0.01). By contrast, discrimination → detection task sequences resulted in response repetition benefits (3.5%, p < 0.01) independently of dimension sequence. Finally, discrimination → discrimination task sequences were associated with response repetition costs (−2.9%, p < 0.05) independently of dimension sequence.
In summary, analyses of response sequence effects on mean RTs showed either response repetition benefits or no effects of response sequence. This finding is at variance with hierarchical task sets, which predict response repetition costs following a task or dimension change. Integrated task sets, which predict no response sequence effects following a task or dimension switch, also account poorly for the present findings. The results for error rates were less consistent across task and dimension sequences, with different patterns of response sequence effects across different experimental conditions.
The present findings demonstrate that, consistent with “agglomerated task sets,” it is possible to reuse control settings across different tasks, and that the reusing is associated with distinct ERP components, depending on precisely which task set component (selection vs. identification criteria) is reused across tasks. In particular, switching tasks was revealed to be easier when the task-relevant dimension repeated relative to when it changed, as indexed by DREs on task switch trials. The notion of agglomerated task sets is fully compatible with existing computational models of cognitive control (Logan and Gordon, 2001 ; Meiran et al., 2008 ). Both these accounts postulate several independent parameters influencing processes of selection, identification, and, respectively, responding. Importantly, since these parameters are independent, it is plausible that they can also be adjusted independently—which is the core assumption of the agglomerated-task-sets hypothesis. The compatibility with the computational models of cognitive control emphasizes another property of agglomerated task sets: their computational efficiency.
Note though that the DREs reported presently are consistent not only with agglomerated task sets, but also with two, relatively strong alternatives, namely: (i) switching between the detection and discrimination conditions did not involve a task switch, and (ii) the DREs are not dimension-specific, but rather feature- or response-specific. The former alternative would postulate that the two tasks used in the present study were effectively one task, in which participants always performed feature discrimination, but, depending on the cue, selected different responses. The latter alternative would imply that the substantial reusing of spatial selection and identification criteria (as revealed in the dimension-repetition effects) was not indicative of the reuse of task set components in general, but rather of the reuse of stimulus-response associations encountered on the previous trial (Hommel et al., 2001 ; Dreisbach et al., 2006 , 2007 ).
The present findings argue against the hypothesis that no task switching took place in our paradigm. First, in the present study, substantial differences in mean RTs and errors rates were observed between the detection and discrimination tasks, suggesting that the two conditions were performed differently. Behavioral differences were accompanied by differences in the ERPs: a reliable SPCN component was observed for the discrimination, but not for the detection task. Taken together, these findings suggest that solving the detection and, respectively, discrimination tasks involved the use of different task sets. Second, switching between the two conditions incurred substantial switch costs. Importantly, the switch cost magnitude differed, with stronger costs for discrimination → detection relative to detection → discrimination sequences. The asymmetry in the switch costs—with switching to an easier, or dominant, task (presently, detection) being more effortful than switching to a more difficult task (presently, discrimination)—has frequently been reported in task-switching literature (Allport et al., 1994 ; Wylie and Allport, 2000 ) and interpreted as an index of the interference between two concurrent task sets. On this background, it is likely that switching between the two tasks in the present study indeed involved task switch processes.
The second alternative explanation posits that DREs critically rely on repetitions of full stimulus-response episodes across trials, rather than on more abstract criterion repetitions. Studies investigating the role of S-R repetitions have typically found performance to be very good on full-repetition trials and worse on partial-repetition trials, that is, when either the stimulus or the response changed, while the other property repeated. Somewhat counter-intuitively, performance is typically better on full switches, that is, when both stimulus and response change, compared to partial repetitions (Hommel, 1998 , 2005 ; Töllner et al., 2008 ; Zehetleitner et al., 2012 ). Applied to the present study, if DREs were actually S-R repetition effects, then changing the dimension and repeating the response (i.e., partial repetitions) should have resulted in worse performance relative to changing both the dimension and the response (full repetitions). However, analyses of response sequence effects showed this not to be the case, arguing that the DREs reported presently are not reducible to S-R repetition effects.
That partial-repetition costs were not prominent in the present study does not necessarily imply that the processes generating these effects were inactive; rather, the paradigm and dependent measures may not have been sensitive to these processes. Previous work investigating electrophysiological correlates of DREs in a single task paradigm (Töllner et al., 2008 ) revealed partial repetition costs to correlate with ERP markers that were independent of the ERP markers for dimension and, respectively, response sequence effects. Importantly, the markers of partial-repetition costs were not investigated in the present study. Additionally, in a recent study of partial-repetition costs (Zehetleitner et al., 2012 ), numerical simulations showed that three different sequence-sensitive mechanisms (dimension-, S-R mapping-, and motor-response-specific) combine and can produce any possible RT pattern, that is, with or without manifest partial repetition costs. Given this, until the boundary conditions for partial-repetition costs to arise are fully understood, it remains possible that the present paradigm simply did not meet these conditions.
Direction of partial-switch effects: reusing, resetting, or switching
While the present study, together with several previous investigations (Arrington et al., 2003 ; Rangelov et al., 2011 , 2012 ), showed that reusing shared task set components across different tasks is possible, findings to the contrary have also been reported. In particular, Vandierendonck et al. ( 2008 ) failed to find any partial-switch effects, while Kleinsorge and colleagues ( 1999 , 2004 ) found partial-switch costs , relative to full switches, rather than benefits as reported presently. How can these disparate findings be reconciled?
A notable difference between the paradigms used in these investigations and the present study is in the stimulus material and the cueing procedure employed. More precisely, the previous studies used stimuli that were ambiguous with regard to both task-relevant stimulus attributes and identification criteria. Thus, cueing of both the relevant stimulus property (e.g., number or digit value in Vandierendonck et al., 2008 ) and identification criteria (parity vs. magnitude) was necessary on every trial (e.g., the string “number odd/even” served as a cue). This procedure produced partial overlap between cue strings on partial switches (e.g., “number odd/even” → “digit odd/even”) and no overlap on full switches (e.g., “number smaller/greater” → “digit odd/even”). As has been shown by several studies (Logan and Schneider, 2006 ; Schneider and Logan, 2009 ), overlapping cues activate competing task sets, resulting in negative interference on partial-switch trials. As a consequence, any benefits from reusing shared control representations might have been masked by interference effects from overlapping cues, resulting in either insignificant partial-repetition effects or even partial-repetition costs. By contrast, the present study used cues that did not overlap between the different tasks. Furthermore, the task-relevant dimension was unambiguously specified by the stimulus displays themselves, so no dimension cues were necessary. Consequently, in the present experiment, the negative interference effects would have been minimal—and, correspondingly, partial-repetition effects turned out significant.
Electrophysiological evidence for separable control mechanisms
In contrast to the mixed behavioral findings regarding the direction of partial-switch effects, investigation of the ERP components related to the spatial selection and post-selective processing task components offers more conclusive findings. By assuming relative autonomy of the control systems for the different cognitive processes, “agglomerated task sets” predict the existence of multiple , relatively independent sources of DREs. Consistent with the prediction, EEG analyses revealed multiple ERP components to be sensitive to dimension sequences: dimension changes (relative to repetitions) resulted in longer PCN latencies as well as larger SPCN amplitudes.
Analyses of the PCN latencies showed dimension changes (relative to repetitions) to be associated with longer latencies independently of the task sequence (see also Töllner et al., 2008 , reporting similar findings in a single-task paradigm). This finding can be explained by assuming that both the detection and discrimination tasks required spatial selection processes, governed by a task set component sensitive to dimension sequences. Dimension repetitions would permit reusing control settings from the previous trial, generating DREs for the PCN latency. Importantly, on the agglomerated-task-set hypothesis, reusing settings is possible even across tasks that differ in other task set components—as evidenced by the present finding of DREs across task switches for the PCN timing.
Analyses of the SPCN amplitudes showed, consistent with our predictions, a more pronounced SPCN for discrimination relative to detection task trials. Apparently, the SPCN amplitude increases continuously with increases in demands for post-selective perceptual processing, from no SPCNs in singleton detection (present study) and singleton localization tasks (Mazza et al., 2007 ) through singleton discrimination tasks (present study) to strong SPCNs in compound-search tasks (Mazza et al., 2007 ; Töllner et al., 2013 ), in which one target dimension (e.g., color) is selection-relevant, whereas another dimension (e.g., orientation) is response-relevant.
Furthermore, the SPCN amplitude was sensitive to dimension sequence, but only on trials following the discrimination task. This finding is consistent with the conceptual analysis of the task sets for the detection and, respectively, the discrimination task and the functional interpretation of the SPCN component. Conceptual analysis of the detection task suggested that the singleton dimension is not mandatorily encoded in the post-selective identification settings (for the detection task) and it would not be available for reuse on the following trial, predicting no DRE for the SPCN component following detection task trials. By contrast, post-selective identification in the discrimination task necessarily involved determining the singleton dimension, predicting DREs for the SPCN component following trials of the discrimination task, consistent with the results of the present study.
The electrophysiological data are especially informative about the notion of a generalized switching mechanisms [as proposed by Kleinsorge and Heuer ( 1999 )], according to which one criterion switch enforces a switch in all criteria, rather than simple re-setting. If generalized switching were operating as a default task switch mechanism, then reconfiguring the spatial selection component should have triggered a switch in the post-selective identification component as well. This would predict an obligatory coupling between DREs for the PCN and SPCN parameters—a prediction that is not supported by the present findings. Thus, in summary, the present ERP findings are fully consistent with, and yet independent of the behavioral findings of DREs across task switches, in their support for the notion of agglomerated task sets.
- Conclusions
The present study investigated the nature of task-set representations, defined as a set of criteria for spatial selection, post-selective identification, and S-R mapping rules. Two alternatives were considered: task sets as integral representations and task sets as agglomerations of relatively autonomous control settings guiding different processing stages. The key property of the “agglomerated-task-set” hypothesis is that following a task switch, different control instances can be reconfigured independently of each other, permitting reusing some of the control settings across different tasks. By contrast, the hypothesis of “integrated task sets” predicted no partial-switch effects, as changing any task set component would either reset all settings, or trigger a full switch. Consistent with agglomerated task sets, our findings demonstrate substantial partial-switch effects, operationalized as DREs. Most importantly, evidence of DREs over PCN latencies and SPCN amplitudes, two functionally distinct ERP components, further supports the idea of task sets as a collection of autonomous control instances governing processes of spatial selection and perceptual/symbolic analysis, respectively.
Conflict of interest statement
The authors declare that the research was conducted in the absence of any commercial or financial relationships that could be construed as a potential conflict of interest.
- Acknowledgments
This research was supported by DFG grants RA 2191/1-1 (to Dragan Rangelov) and ZE 887/3-1 (to Michael Zehetleitner and Hermann J. Müller) as well as the German-Israeli Foundation for Scientific Research and Development grant 2011/158 (to Michael Zehetleitner and Hermann J. Müller).
1 Note that most of the previous EEG studies on task switching used paradigms in which preparatory adjustments of the task set component of interest were possible, to some extent. This work typically revealed involvement of pre-frontal areas when preparing for a task switch (Karayanidis et al., 2003 ; Nicholson et al., 2005 ; Karayanidis et al., 2009 ).
2 As shown by Shedden and Nordgaard ( 2001 ; Töllner et al., 2012a ), the PCN is independent of both the amplitude and latency of the non-lateralized N2. Thus, to avoid potential confusion associated with the term “N2pc,” we prefer the neutral term “PCN.”
3 Analyses of PCN and SPCN amplitudes revealed a significant target position effect for the PCN component (3.50 and 3.25 μV for left and right targets, respectively, F (1,13) = 4.81, p < 0.05, η 2 p = 0.27), and no difference between left and right targets in the SPCN component ( F = 1.62, p = 0.22). Most importantly, target position did not interact significantly with electrode site (ipsi- vs. contralateral), nor with any other experimental manipulation (all F s < 2.16, all p > 0.16)—indicating that the PCN and SPCN components were not specific for one visual hemifield.
- Allport D. A., Styles E. A., Hsieh S. (1994). “Shifting intentional set: exploring the dynamic control of tasks,” in Attention and Performance , Vol. XV , eds Umilta C., Moscovitch M. (Hillsdale, NJ: Erlbaum; ), 421–452 [ Google Scholar ]
- Arrington C. M., Altmann E. M., Carr T. H. (2003). Tasks of a feather flock together: similarity effects in task switching . Mem. Cogn . 31 , 781–789 10.3758/BF03196116 [ Abstract ] [ CrossRef ] [ Google Scholar ]
- Chiu Y.-C., Yantis S. (2009). A domain-independent source of cognitive control for task sets: shifting spatial attention and switching categorization rules . J. Neurosci . 29 , 3930–3938 10.1523/JNEUROSCI.5737-08.2009 [ Europe PMC free article ] [ Abstract ] [ CrossRef ] [ Google Scholar ]
- Dreisbach G., Goschke T., Haider H. (2006). Implicit task sets in task switching . J. Exp. Psychol. Learn. Mem. Cogn . 32 , 1221–1233 10.1037/0278-7393.32.6.1221 [ Abstract ] [ CrossRef ] [ Google Scholar ]
- Dreisbach G., Goschke T., Haider H. (2007). The role of task rules and stimulusresponse mappings in the task switching paradigm . Psychol. Res. Psychol. Forsch . 71 , 383–392 10.1007/s00426-005-0041-3 [ Abstract ] [ CrossRef ] [ Google Scholar ]
- Eimer M. (1996). The N2pc component as an indicator of attentional selectivity . Electroencephalogr. Clin. Neurophysiol . 99 , 225–234 10.1016/0013-4694(96)95711-9 [ Abstract ] [ CrossRef ] [ Google Scholar ]
- Esterman M., Chiu Y.-C., Tamber-Rosenau B. J., Yantis S. (2009). Decoding cognitive control in human parietal cortex . Proc. Natl. Acad. Sci. U.S.A . 106 , 17974–17979 10.1073/pnas.0903593106 [ Europe PMC free article ] [ Abstract ] [ CrossRef ] [ Google Scholar ]
- Found A., Müller H. J. (1996). Searching for unknown feature targets on more than one dimension: investigating a “dimension-weighting” account . Percept. Psychophys . 58 , 88–101 10.3758/BF03205479 [ Abstract ] [ CrossRef ] [ Google Scholar ]
- Gilbert S. J., Shallice T. (2002). Task switching: a PDP model . Cognit. Psychol . 44 , 297–337 10.1006/cogp.2001.0770 [ Abstract ] [ CrossRef ] [ Google Scholar ]
- Hakun J. G., Ravizza S. M. (2012). Cognitive control: preparation of task switching components . Brain Res . 1451 , 53–64 10.1016/j.brainres.2012.02.046 [ Abstract ] [ CrossRef ] [ Google Scholar ]
- Hommel B. (1998). Event files: evidence for automatic integration of stimulus-response episodes . Vis. Cogn . 5 , 183 10.1080/713756773 [ CrossRef ] [ Google Scholar ]
- Hommel B. (2005). How much attention does an event file need . J. Exp. Psychol. Hum. Percept. Perform . 31 , 1067–1082 10.1037/0096-1523.31.5.1067 [ Abstract ] [ CrossRef ] [ Google Scholar ]
- Hommel B., Müsseler J., Aschersleben G., Prinz W. (2001). The theory of event coding (TEC): a framework for perception and action planning . Behav. Brain Sci . 24 , 849–878 10.1017/S0140525X01000103 [ Abstract ] [ CrossRef ] [ Google Scholar ]
- Hübner R., Futterer T., Steinhauser M. (2001). On attentional control as a source of residual shift costs: evidence from two-component task shifts . J. Exp. Psychol. Learn. Mem. Cogn . 27 , 640–653 10.1037/0278-7393.27.3.640 [ Abstract ] [ CrossRef ] [ Google Scholar ]
- Jolicoeur P., Brisson B., Robitaille N. (2008). Dissociation of the N2pc and sustained posterior contralateral negativity in a choice response task . Brain Res . 1215 , 160–172 10.1016/j.brainres.2008.03.059 [ Abstract ] [ CrossRef ] [ Google Scholar ]
- Karayanidis F., Coltheart M., Michie P. T., Murphy K. (2003). Electrophysiological correlates of anticipatory and poststimulus components of task switching . Psychophysiology 40 , 329–348 10.1111/1469-8986.00037 [ Abstract ] [ CrossRef ] [ Google Scholar ]
- Karayanidis F., Mansfield E. L., Galloway K. L., Smith J. L., Provost A., Heathcote A. (2009). Anticipatory reconfiguration elicited by fully and partially informative cues that validly predict a switch in task . Cogn. Affect. Behav. Neurosci . 9 , 202–215 10.3758/CABN.9.2.202 [ Abstract ] [ CrossRef ] [ Google Scholar ]
- Kleinsorge T. (2004). Hierarchical switching with two types of judgment and two stimulus dimensions . Exp. Psychol . 51 , 145–149 10.1027/1618-3169.51.2.145 [ Abstract ] [ CrossRef ] [ Google Scholar ]
- Kleinsorge T., Heuer H. (1999). Hierarchical switching in a multi-dimensional task space . Psychol. Res . 62 , 300–312 10.1007/s004260050060 [ CrossRef ] [ Google Scholar ]
- Logan G. D., Gordon R. D. (2001). Executive control of visual attention in dual-task situations . Psychol. Rev . 108 , 393–434 10.1037/0033-295X.108.2.393 [ Abstract ] [ CrossRef ] [ Google Scholar ]
- Logan G. D., Schneider D. W. (2006). Priming or executive control? Associative priming of cue encoding increases “switch costs” in the explicit task-cuing procedure . Mem. Cogn . 34 , 1250–1259 10.3758/BF03193269 [ Abstract ] [ CrossRef ] [ Google Scholar ]
- Luck S. J., Hillyard S. A. (1994). Spatial filtering during visual search: evidence from human electrophysiology . J. Exp. Psychol. Hum. Percept. Perform . 20 , 1000–1014 10.1037/0096-1523.20.5.1000 [ Abstract ] [ CrossRef ] [ Google Scholar ]
- Mazza V., Turatto M., Umiltà C., Eimer M. (2007). Attentional selection and identification of visual objects are reflected by distinct electrophysiological responses . Exp. Brain Res . 181 , 531–536 10.1007/s00221-007-1002-4 [ Europe PMC free article ] [ Abstract ] [ CrossRef ] [ Google Scholar ]
- Meiran N. (1996). Reconfiguration of processing mode prior to task performance . J. Exp. Psychol . 22 , 1423–1442 10.1037/0278-7393.22.6.1423 [ CrossRef ] [ Google Scholar ]
- Meiran N. (2000). Modeling cognitive control in task-switching . Psychol. Res . 63 , 234–249 10.1007/s004269900004 [ Abstract ] [ CrossRef ] [ Google Scholar ]
- Meiran N., Kessler Y., Adi-Japha E. (2008). Control by action representation and input selection (CARIS): a theoretical framework for task switching . Psychol. Res . 72 , 473–500 10.1007/s00426-008-0136-8 [ Abstract ] [ CrossRef ] [ Google Scholar ]
- Monsell S., Driver J. (2000). Control of Cognitive Processes: Attention and Performance XVIII . Available online at: http://eprints.ucl.ac.uk/18943/ (Accessed February 2, 2011).
- Monsell S., Sumner P., Waters H. (2003). Task-set reconfiguration with predictable and unpredictable task switches . Mem. Cogn . 31 , 327–342 10.3758/BF03194391 [ Abstract ] [ CrossRef ] [ Google Scholar ]
- Müller H. J., Krummenacher J. (2006). Visual search and selective attention . Vis. Cogn . 14 , 389–410 10.1080/13506280500527676 [ CrossRef ] [ Google Scholar ]
- Müller H. J., Krummenacher J., Heller D. (2004). Dimension-specific intertrial facilitation in visual search for pop-out targets: evidence for a top-down modulable visual short-term memory effect . Vis. Cogn . 11 , 577–602 10.1080/13506280344000419 [ CrossRef ] [ Google Scholar ]
- Müller H. J., Töllner T., Zehetleitner M., Geyer T., Rangelov D., Krummenacher J. (2010). Dimension-based attention modulates feed-forward visual processing: a reply to Theeuwes (2010) . Acta Psychol . 135 , 117–122 10.1016/j.actpsy.2010.05.004 [ Abstract ] [ CrossRef ] [ Google Scholar ]
- Nicholson R., Karayanidis F., Poboka D., Heathcote A., Michie P. T. (2005). Electrophysiological correlates of anticipatory task-switching processes . Psychophysiology 42 , 540–554 10.1111/j.1469-8986.2005.00350.x [ Abstract ] [ CrossRef ] [ Google Scholar ]
- Rangelov D., Müller H. J., Zehetleitner M. (2011). Independent dimension-weighting mechanisms for visual selection and stimulus identification . J. Exp. Psychol . 37 , 1369–1382 10.1037/a0024265 [ Abstract ] [ CrossRef ] [ Google Scholar ]
- Rangelov D., Müller H. J., Zehetleitner M. (2012). The multiple-weighting-systems hypothesis: theory and empirical support . Attent. Percept. Psychophys . 74 , 540–552 10.3758/s13414-011-0251-0252 [ Abstract ] [ CrossRef ] [ Google Scholar ]
- Ravizza S. M., Carter C. S. (2008). Shifting set about task switching: behavioral and neural evidence for distinct forms of cognitive flexibility . Neuropsychologia 46 , 2924–2935 10.1016/j.neuropsychologia.2008.06.006 [ Europe PMC free article ] [ Abstract ] [ CrossRef ] [ Google Scholar ]
- Rogers R. D., Monsell S. (1995). Costs of a predictable switch between simple cognitive tasks . J. Exp. Psychol . 124 , 207–231 10.1037/0096-3445.124.2.207 [ Abstract ] [ CrossRef ] [ Google Scholar ]
- Rushworth M. F. S., Passingham R. E., Nobre A. C. (2002). Components of switching intentional set . J. Cogn. Neurosci . 14 , 1139–1150 10.1162/089892902760807159 [ Abstract ] [ CrossRef ] [ Google Scholar ]
- Rushworth M. F. S., Passingham R. E., Nobre A. C. (2005). Components of attentional set-switching . Exp. Psychol . 52 , 83–98 10.1027/1618-3169.52.2.83 [ Abstract ] [ CrossRef ] [ Google Scholar ]
- Schneider D. W., Logan G. D. (2009). Selecting a response in task switching: testing a model of compound cue retrieval . J. Exp. Psychol. Learn. Mem. Cogn . 35 , 122–136 10.1037/a0013744 [ Europe PMC free article ] [ Abstract ] [ CrossRef ] [ Google Scholar ]
- Shedden J. M., Nordgaard C. L. (2001). ERP time course of perceptual and post-perceptual mechanisms of spatial selection . Brain Res. Cogn. Brain Res . 11 , 59–75 10.1016/S0926-6410(00)00064-1 [ Abstract ] [ CrossRef ] [ Google Scholar ]
- Töllner T., Conci M., Rusch T., Müller H. J. (2013). Selective manipulation of target identification demands in visual search: the role of stimulus contrast in CDA activations . J. Vis . 13 :23 10.1167/13.3.23 [ Abstract ] [ CrossRef ] [ Google Scholar ]
- Töllner T., Gramann K., Müller H. J., Kiss M., Eimer M. (2008). Electrophysiological markers of visual dimension changes and response changes . J. Exp. Psychol . 34 , 531–542 10.1037/0096-1523.34.3.531 [ Europe PMC free article ] [ Abstract ] [ CrossRef ] [ Google Scholar ]
- Töllner T., Müller H. J., Zehetleitner M. (2012a). Top-down dimensional weight set determines the capture of visual attention: evidence from the PCN component . Cereb. Cortex 22 , 1554–1563 10.1093/cercor/bhr231 [ Abstract ] [ CrossRef ] [ Google Scholar ]
- Töllner T., Rangelov D., Müller H. J. (2012b). How the speed of motor-response decisions, but not focal-attentional selection, differs as a function of task set and target prevalence . Proc. Natl. Acad. Sci. U.S.A . 109 , E1990–E1999 10.1073/pnas.1206382109 [ Europe PMC free article ] [ Abstract ] [ CrossRef ] [ Google Scholar ]
- Töllner T., Zehetleitner M., Gramann K., Müller H. J. (2011). Stimulus saliency modulates pre-attentive processing speed in human visual cortex . PLoS ONE 6 :e16276 10.1371/journal.pone.0016276 [ Europe PMC free article ] [ Abstract ] [ CrossRef ] [ Google Scholar ]
- Vandierendonck A., Christiaens E., Liefooghe B. (2008). On the representation of task information in task switching: evidence from task and dimension switching . Mem. Cogn . 36 , 1248–1261 10.3758/MC.36.7.1248 [ Abstract ] [ CrossRef ] [ Google Scholar ]
- Waszak F., Hommel B., Allport A. (2003). Task-switching and long-term priming: role of episodic stimulus-task bindings in task-shift costs . Cogn. Psychol . 46 , 361–413 10.1016/S0010-0285(02)00520-0 [ Abstract ] [ CrossRef ] [ Google Scholar ]
- Wylie G. R., Allport A. (2000). Task switching and the measurement of “switch costs.” Psychol. Res . 63 , 212–233 10.1007/s004269900003 [ Abstract ] [ CrossRef ] [ Google Scholar ]
- Zehetleitner M., Rangelov D., Müller H. J. (2012). Partial repetition costs persist in nonsearch compound tasks: evidence for multiple-weighting-systems hypothesis . Attent. Percept. Psychophys . 74 , 879–890 10.3758/s13414-012-0287-y [ Abstract ] [ CrossRef ] [ Google Scholar ]
Full text links
Read article at publisher's site: https://doi.org/10.3389/fnhum.2013.00524
Citations & impact
Impact metrics, citations of article over time, smart citations by scite.ai smart citations by scite.ai include citation statements extracted from the full text of the citing article. the number of the statements may be higher than the number of citations provided by europepmc if one paper cites another multiple times or lower if scite has not yet processed some of the citing articles. explore citation contexts and check if this article has been supported or disputed. https://scite.ai/reports/10.3389/fnhum.2013.00524, article citations, a gestalt account of human behavior is supported by evidence from switching between single and dual actions..
Huestegge L , Pieczykolan A , Koch I
Sci Rep , 13(1):21201, 01 Dec 2023
Cited by: 0 articles | PMID: 38040736 | PMCID: PMC10692127
Auditory attention switching and judgment switching: Exploring multicomponent task representations.
Seibold JC , Nolden S , Oberem J , Fels J , Koch I
Atten Percept Psychophys , 80(7):1823-1832, 01 Oct 2018
Cited by: 3 articles | PMID: 29959662
Saliency maps for finding changes in visual scenes?
Liesefeld HR , Liesefeld AM , Müller HJ , Rangelov D
Atten Percept Psychophys , 79(7):2190-2201, 01 Oct 2017
Cited by: 4 articles | PMID: 28718177
Item-based selection is in good shape in visual compound search: A view from electrophysiology.
Töllner T , Rangelov D
Behav Brain Sci , 40:e156, 01 Jan 2017
Cited by: 0 articles | PMID: 29342607
Hierarchical task organization in dual tasks: evidence for higher level task representations.
Hirsch P , Nolden S , Philipp AM , Koch I
Psychol Res , 82(4):759-770, 11 Mar 2017
Cited by: 9 articles | PMID: 28285364
Similar Articles
To arrive at the top five similar articles we use a word-weighted algorithm to compare words from the Title and Abstract of each citation.
A Gratton-like effect concerning task order in dual-task situations.
Strobach T , Kübler S , Schubert T
Acta Psychol (Amst) , 217:103328, 13 May 2021
Cited by: 3 articles | PMID: 33991794
Shifting the set of stimulus selection when switching between tasks.
Wendt M , Luna-Rodriguez A , Jacobsen T
Psychol Res , 82(1):134-145, 27 Jul 2017
Cited by: 1 article | PMID: 28752317
Electrophysiological evidence for preparatory reconfiguration before voluntary task switches but not cued task switches.
Kang MS , Diraddo A , Logan GD , Woodman GF
Psychon Bull Rev , 21(2):454-461, 01 Apr 2014
Cited by: 5 articles | PMID: 23979831 | PMCID: PMC3933470
Free full text in Europe PMC
The multiple-weighting-systems hypothesis: theory and empirical support.
Rangelov D , Müller HJ , Zehetleitner M
Atten Percept Psychophys , 74(3):540-552, 01 Apr 2012
Cited by: 8 articles | PMID: 22160820
Optimal monitoring strategies for guiding when to switch first-line antiretroviral therapy regimens for treatment failure in adults and adolescents living with HIV in low-resource settings.
Chang LW , Harris J , Humphreys E
Cochrane Database Syst Rev , (4):CD008494, 14 Apr 2010
Cited by: 13 articles | PMID: 20393969
Europe PMC is part of the ELIXIR infrastructure
Help | Advanced Search
Computer Science > Computer Vision and Pattern Recognition
Title: fsrt: facial scene representation transformer for face reenactment from factorized appearance, head-pose, and facial expression features.
Abstract: The task of face reenactment is to transfer the head motion and facial expressions from a driving video to the appearance of a source image, which may be of a different person (cross-reenactment). Most existing methods are CNN-based and estimate optical flow from the source image to the current driving frame, which is then inpainted and refined to produce the output animation. We propose a transformer-based encoder for computing a set-latent representation of the source image(s). We then predict the output color of a query pixel using a transformer-based decoder, which is conditioned with keypoints and a facial expression vector extracted from the driving frame. Latent representations of the source person are learned in a self-supervised manner that factorize their appearance, head pose, and facial expressions. Thus, they are perfectly suited for cross-reenactment. In contrast to most related work, our method naturally extends to multiple source images and can thus adapt to person-specific facial dynamics. We also propose data augmentation and regularization schemes that are necessary to prevent overfitting and support generalizability of the learned representations. We evaluated our approach in a randomized user study. The results indicate superior performance compared to the state-of-the-art in terms of motion transfer quality and temporal consistency.
Submission history
Access paper:.
- Other Formats
References & Citations
- Google Scholar
- Semantic Scholar
BibTeX formatted citation

Bibliographic and Citation Tools
Code, data and media associated with this article, recommenders and search tools.
- Institution
arXivLabs: experimental projects with community collaborators
arXivLabs is a framework that allows collaborators to develop and share new arXiv features directly on our website.
Both individuals and organizations that work with arXivLabs have embraced and accepted our values of openness, community, excellence, and user data privacy. arXiv is committed to these values and only works with partners that adhere to them.
Have an idea for a project that will add value for arXiv's community? Learn more about arXivLabs .
- Skip to main content
- Keyboard shortcuts for audio player
NPR defends its journalism after senior editor says it has lost the public's trust

David Folkenflik

NPR is defending its journalism and integrity after a senior editor wrote an essay accusing it of losing the public's trust. Saul Loeb/AFP via Getty Images hide caption
NPR is defending its journalism and integrity after a senior editor wrote an essay accusing it of losing the public's trust.
NPR's top news executive defended its journalism and its commitment to reflecting a diverse array of views on Tuesday after a senior NPR editor wrote a broad critique of how the network has covered some of the most important stories of the age.
"An open-minded spirit no longer exists within NPR, and now, predictably, we don't have an audience that reflects America," writes Uri Berliner.
A strategic emphasis on diversity and inclusion on the basis of race, ethnicity and sexual orientation, promoted by NPR's former CEO, John Lansing, has fed "the absence of viewpoint diversity," Berliner writes.
NPR's chief news executive, Edith Chapin, wrote in a memo to staff Tuesday afternoon that she and the news leadership team strongly reject Berliner's assessment.
"We're proud to stand behind the exceptional work that our desks and shows do to cover a wide range of challenging stories," she wrote. "We believe that inclusion — among our staff, with our sourcing, and in our overall coverage — is critical to telling the nuanced stories of this country and our world."
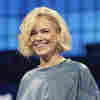
NPR names tech executive Katherine Maher to lead in turbulent era
She added, "None of our work is above scrutiny or critique. We must have vigorous discussions in the newsroom about how we serve the public as a whole."
A spokesperson for NPR said Chapin, who also serves as the network's chief content officer, would have no further comment.
Praised by NPR's critics
Berliner is a senior editor on NPR's Business Desk. (Disclosure: I, too, am part of the Business Desk, and Berliner has edited many of my past stories. He did not see any version of this article or participate in its preparation before it was posted publicly.)
Berliner's essay , titled "I've Been at NPR for 25 years. Here's How We Lost America's Trust," was published by The Free Press, a website that has welcomed journalists who have concluded that mainstream news outlets have become reflexively liberal.
Berliner writes that as a Subaru-driving, Sarah Lawrence College graduate who "was raised by a lesbian peace activist mother ," he fits the mold of a loyal NPR fan.
Yet Berliner says NPR's news coverage has fallen short on some of the most controversial stories of recent years, from the question of whether former President Donald Trump colluded with Russia in the 2016 election, to the origins of the virus that causes COVID-19, to the significance and provenance of emails leaked from a laptop owned by Hunter Biden weeks before the 2020 election. In addition, he blasted NPR's coverage of the Israel-Hamas conflict.
On each of these stories, Berliner asserts, NPR has suffered from groupthink due to too little diversity of viewpoints in the newsroom.
The essay ricocheted Tuesday around conservative media , with some labeling Berliner a whistleblower . Others picked it up on social media, including Elon Musk, who has lambasted NPR for leaving his social media site, X. (Musk emailed another NPR reporter a link to Berliner's article with a gibe that the reporter was a "quisling" — a World War II reference to someone who collaborates with the enemy.)
When asked for further comment late Tuesday, Berliner declined, saying the essay spoke for itself.
The arguments he raises — and counters — have percolated across U.S. newsrooms in recent years. The #MeToo sexual harassment scandals of 2016 and 2017 forced newsrooms to listen to and heed more junior colleagues. The social justice movement prompted by the killing of George Floyd in 2020 inspired a reckoning in many places. Newsroom leaders often appeared to stand on shaky ground.
Leaders at many newsrooms, including top editors at The New York Times and the Los Angeles Times , lost their jobs. Legendary Washington Post Executive Editor Martin Baron wrote in his memoir that he feared his bonds with the staff were "frayed beyond repair," especially over the degree of self-expression his journalists expected to exert on social media, before he decided to step down in early 2021.
Since then, Baron and others — including leaders of some of these newsrooms — have suggested that the pendulum has swung too far.

Author Interviews
Legendary editor marty baron describes his 'collision of power' with trump and bezos.
New York Times publisher A.G. Sulzberger warned last year against journalists embracing a stance of what he calls "one-side-ism": "where journalists are demonstrating that they're on the side of the righteous."
"I really think that that can create blind spots and echo chambers," he said.
Internal arguments at The Times over the strength of its reporting on accusations that Hamas engaged in sexual assaults as part of a strategy for its Oct. 7 attack on Israel erupted publicly . The paper conducted an investigation to determine the source of a leak over a planned episode of the paper's podcast The Daily on the subject, which months later has not been released. The newsroom guild accused the paper of "targeted interrogation" of journalists of Middle Eastern descent.
Heated pushback in NPR's newsroom
Given Berliner's account of private conversations, several NPR journalists question whether they can now trust him with unguarded assessments about stories in real time. Others express frustration that he had not sought out comment in advance of publication. Berliner acknowledged to me that for this story, he did not seek NPR's approval to publish the piece, nor did he give the network advance notice.
Some of Berliner's NPR colleagues are responding heatedly. Fernando Alfonso, a senior supervising editor for digital news, wrote that he wholeheartedly rejected Berliner's critique of the coverage of the Israel-Hamas conflict, for which NPR's journalists, like their peers, periodically put themselves at risk.
Alfonso also took issue with Berliner's concern over the focus on diversity at NPR.
"As a person of color who has often worked in newsrooms with little to no people who look like me, the efforts NPR has made to diversify its workforce and its sources are unique and appropriate given the news industry's long-standing lack of diversity," Alfonso says. "These efforts should be celebrated and not denigrated as Uri has done."
After this story was first published, Berliner contested Alfonso's characterization, saying his criticism of NPR is about the lack of diversity of viewpoints, not its diversity itself.
"I never criticized NPR's priority of achieving a more diverse workforce in terms of race, ethnicity and sexual orientation. I have not 'denigrated' NPR's newsroom diversity goals," Berliner said. "That's wrong."
Questions of diversity
Under former CEO John Lansing, NPR made increasing diversity, both of its staff and its audience, its "North Star" mission. Berliner says in the essay that NPR failed to consider broader diversity of viewpoint, noting, "In D.C., where NPR is headquartered and many of us live, I found 87 registered Democrats working in editorial positions and zero Republicans."
Berliner cited audience estimates that suggested a concurrent falloff in listening by Republicans. (The number of people listening to NPR broadcasts and terrestrial radio broadly has declined since the start of the pandemic.)
Former NPR vice president for news and ombudsman Jeffrey Dvorkin tweeted , "I know Uri. He's not wrong."
Others questioned Berliner's logic. "This probably gets causality somewhat backward," tweeted Semafor Washington editor Jordan Weissmann . "I'd guess that a lot of NPR listeners who voted for [Mitt] Romney have changed how they identify politically."
Similarly, Nieman Lab founder Joshua Benton suggested the rise of Trump alienated many NPR-appreciating Republicans from the GOP.
In recent years, NPR has greatly enhanced the percentage of people of color in its workforce and its executive ranks. Four out of 10 staffers are people of color; nearly half of NPR's leadership team identifies as Black, Asian or Latino.
"The philosophy is: Do you want to serve all of America and make sure it sounds like all of America, or not?" Lansing, who stepped down last month, says in response to Berliner's piece. "I'd welcome the argument against that."
"On radio, we were really lagging in our representation of an audience that makes us look like what America looks like today," Lansing says. The U.S. looks and sounds a lot different than it did in 1971, when NPR's first show was broadcast, Lansing says.
A network spokesperson says new NPR CEO Katherine Maher supports Chapin and her response to Berliner's critique.
The spokesperson says that Maher "believes that it's a healthy thing for a public service newsroom to engage in rigorous consideration of the needs of our audiences, including where we serve our mission well and where we can serve it better."
Disclosure: This story was reported and written by NPR Media Correspondent David Folkenflik and edited by Deputy Business Editor Emily Kopp and Managing Editor Gerry Holmes. Under NPR's protocol for reporting on itself, no NPR corporate official or news executive reviewed this story before it was posted publicly.
- Anniston/Gadsden
Alabama among 7 states targeted by new Justice Department carjacking task force
- Published: Apr. 18, 2024, 11:09 a.m.
- Carol Robinson | [email protected]
The U.S. Department of Justice announced Thursday that Alabama is one of seven states where a carjacking task force will be launched to focus resources on what they described as a public safety threat.
The newly formed federal task forces will be led by the U.S. Attorneys’ Offices, the FBI, and the Bureau of Alcohol, Tobacco, Firearms and Explosives, working alongside state, local, Tribal, and territorial law.
In Alabama, the task force will be in the Northern District, which includes Birmingham, Tuscaloosa, and Huntsville.
Other states include Oregon, Texas, California, Louisiana, Mississippi, and Washington.
DOJ officials said carjacking and other violent crime task forces have proven to be an effective part of successful violent crime reduction strategies by focusing on significant crime drivers and taking violent offenders off the streets.
The task forces will work to use federal sentencing enhancements for cases where adults use juveniles to commit carjackings, as well as work with state and local authorities to ensure the appropriate handling of juvenile suspects.
The task forces will also assist state and local officials who wish to seek Justice Department grant funding for juvenile intervention and diversion resources.
The initiative is based on best practices learned from similar carjacking task forces for the District of Columbian, the Northern District of Illinois, the Eastern District of Pennsylvania, and the Middle District of Florida.
For example, carjackings in Philadelphia declined by 31% from 2022 to 2023, and armed carjackings are down 28% in the District of Columbia this year compared to the same period in 2023. In Chicago, carjackings decreased 29% from a high in 2021 through the end of 2023.
“The Justice Department has no higher priority than keeping our communities safe, Deputy Attorney General Lisa Monaco said in a Thursday’s news release.
“We do so by targeting the most significant drivers of violent crime and by acting as a force multiplier for our state and local law enforcement partners. We’re seeing results — with violent crime declining broadly nationwide.”
“When prosecutors, officers, agents, and analysts come together to crunch data, share intelligence, and apply best practices,” Monaco said, “we can make real progress in the fight against all forms of violent crime, including carjacking.”
If you purchase a product or register for an account through a link on our site, we may receive compensation. By using this site, you consent to our User Agreement and agree that your clicks, interactions, and personal information may be collected, recorded, and/or stored by us and social media and other third-party partners in accordance with our Privacy Policy.
Advertisement
Supported by
Trump to Meet an Embattled Johnson, Putting Their Tortured Ties on Display
The presumptive Republican presidential nominee and the G.O.P. speaker, at odds over many issues, are making common cause on “election integrity.”
- Share full article

By Annie Karni
Reporting from the Capitol
Speaker Mike Johnson may not have a functional majority in Congress, but his job is similar to the Republicans who preceded him in at least one respect: The duties include the difficult task of managing Donald J. Trump.
Mr. Johnson on Friday will travel to Mar-a-Lago, Mr. Trump’s Florida estate, to join him for what the speaker has billed as a “major announcement on election integrity.” No further details have been forthcoming.
The two men had been planning to get together for a political meeting, but Mr. Johnson’s team suggested a joint public appearance on a topic Mr. Trump cares deeply about, according to two people familiar with the planning.
It will afford Mr. Johnson the opportunity to stand shoulder to shoulder with Mr. Trump at a precarious moment in his speakership, as he works to corral a minuscule and deeply divided majority around a legislative agenda many of them oppose — all while facing the threat of an ouster from Representative Marjorie Taylor Greene, a far-right Georgia Republican and ride-or-die Trump ally. Making matters even trickier, Mr. Trump, the former president and presumptive Republican presidential nominee, is helping to undermine that agenda.
Even so, Republicans generally consider it good and politically helpful to be physically near Mr. Trump.
“It’s about Trump embracing Johnson,” former Speaker Newt Gingrich said of Friday’s joint appearance. “This is Trump saying, ‘He is the speaker, I am his friend, we are together.’ That’s a pretty important thing for him. He just has to endure.”
Mr. Trump does think of Mr. Johnson, who defended him in two impeachment trials and played a key role in his efforts to overturn the 2020 election , as something like a friend, people close to him said. He likes the Louisiana Republican, and likes his loyalty even more. (He especially appreciated that Mr. Johnson quickly endorsed him after becoming speaker, a move that his predecessor Kevin McCarthy always resisted). The two speak regularly, and Mr. Trump has even come around on some of the congressional endorsements Mr. Johnson has lobbied him on.
Still, if this is what an embrace looks like, it’s not clear that it’s so much better than the alternative.
Mr. Trump earlier this week weighed in against legislation that Mr. Johnson put forward to extend an expiring warrantless surveillance law that national security officials say is crucial to fighting terrorism and gathering intelligence. Mr. Trump urged lawmakers to “kill” the law undergirding the program, and ultraconservatives in the House banded together to block it from coming to the House floor in an embarrassing defeat for Mr. Johnson.
The speaker managed on Friday to get the measure back on track in the House by shortening the extension to two years from five, placating far-right Republicans who believe Mr. Trump would be president again once it expired.
The former president has also said it is “stupid” for the United States to send aid to Ukraine and railed against doing so, even as Mr. Johnson has made it clear that it is a top priority of his to bring up a bill to provide an infusion of American military assistance to Kyiv.
The dynamic means that even as they present a united front at Mar-a-Lago, the pair will be at odds on many issues they could be asked about. Such is life with Mr. Trump. And for Republican speakers, it always has been.
For a short time after Mr. Trump first arrived in the White House in 2017, he deferred to Republican leaders on Capitol Hill on their legislative efforts, which included trying to repeal Obamacare and seeking tax cuts. Former Speaker Paul D. Ryan, who had refused to campaign for Mr. Trump, benefited from the fact that the new president had a personal interest in the success of a shared Republican agenda.
Even so, managing the relationship required both hand-holding and hand-wringing about who was going to be the last person in Mr. Trump’s ear. In 2018, for instance, Mr. Trump threatened to veto a big spending bill that had been approved by Congress. Mr. Ryan had to work to convince him to sign it.
“It would be these fire drills where you had to send five or six people to walk him back,” recalled Brendan Buck, who served as a top adviser to Mr. Ryan. “You’re always going to be fighting the last person who talked to him, emotional whims, the thing he read. It’s a constant battle you always have to be fighting.”
That same year, Mr. Trump almost scuttled a version of the surveillance legislation he tanked this week when he tweeted criticism of it — breaking with his administration — apparently after watching a segment on Fox News. “Everyone was calling him, the national security adviser rushed over, people were rushing over to the White House,” Mr. Buck recalled. Mr. Trump eventually walked back his post 90 minutes later.
But Mr. Trump now has less of a stake in the Republican agenda in Congress — it’s not his. And he is not surrounded by a national security apparatus that can weigh in and help keep him on a track that is more in line with that of party leaders in Congress.
As he settled into the White House, Mr. Trump also started keeping his own counsel more and relying less on congressional leaders for direction. He was not interested in taking Mr. Ryan’s advice, for instance, about trying to avoid a government shutdown at the end of 2018.
Mr. McCarthy spent years nurturing his relationship with Mr. Trump, going so far as to sort out his favorite flavors of Starburst to curry favor. He visited the former president at Mar-a-Lago after the Jan. 6, 2021, mob attack on the Capitol in an attempt to smooth over any divisions.
Mr. McCarthy had an up-and-down relationship with Mr. Trump during his short tenure as speaker. He succeeded in steering some of Mr. Trump’s endorsements during the 2022 midterm campaigns, and his biggest win might have been keeping the former president silent during his negotiations with the White House over the debt ceiling — Mr. Trump waited until after the deal was signed to criticize it.
Mostly, Mr. McCarthy benefited from timing: The former president was not yet the presumed Republican nominee during his tenure and was less involved in the agenda in Washington.
Mr. Johnson may have the worst of both worlds: Mr. Trump is not the president, so he does not have a shared interest in the Republican speaker’s legislative success, but at this stage of the presidential campaign, he is attuned enough to potentially complicate anything he tries to do. Mr. Trump continues to carry enough influence with Republicans on Capitol Hill that his opposition can be enough to sink a bill outright, and Mr. Johnson has not had as long to cement their relationship.
“Johnson has the hardest speakership of anyone since maybe the beginning of the Civil War,” Mr. Gingrich said. “His major goal has to be to hold the system together to get to an election in which Trump increases Republican turnout.”
People close to Mr. Trump said he values Mr. Johnson’s political insights and has deferred to him at times on endorsements. Mr. Johnson lobbied him hard to back Representative Mike Bost, Republican of Illinois, over Darren Bailey, a competitive challenger running a spirited MAGA campaign. It was a difficult endorsement for Mr. Trump to come around to, people familiar with it said, but he ultimately did so at Mr. Johnson’s urging.
Ms. Greene, for her part, said she would not back down on her criticism of Mr. Johnson or drop the threat to try to oust him, even if Mr. Trump gives the speaker a public boost.
“Things like that don’t bother me,” she said of Mr. Trump hosting an event with Mr. Johnson. Of the speaker, she added: “Right now, he does not have my support.”
Annie Karni is a congressional correspondent for The Times. She writes features and profiles, with a recent focus on House Republican leadership. More about Annie Karni
Our Coverage of the 2024 Election
Presidential Race
President Biden’s campaign has featured initiatives aimed at young people , union workers and environmentalists as well as calling for tariffs on Chinese steel , but it is not clear that they will be sufficient to rekindle support in those groups.
American voters absorbed their first view of a split-screen campaign: President Biden sprinting across one of the country’s top battleground states and former President Donald J. Trump sitting in a New York courtroom.
Robert F. Kennedy Jr. announced that he had secured a spot on the ballot in Michigan, as more than a dozen members of his family endorsed President Biden. Additionally, activists who worked with Kennedy at an environmental nonprofit group urged him to drop his presidential bid .
A Generation Gap: Many older Black voters see moral and political reasons to vote, but younger Black voters feel far less motivated to cast a ballot for Democrats or even at all .
Vice-Presidential Calculations: As Trump sifts through potential running mates, he has peppered some advisers and associates with a direct question: Which Republican could best help him raise money ?
Embracing the Jan. 6 Rioters: Trump initially disavowed the attack on the Capitol, but he is now making it a centerpiece of his campaign .
Mobilizing the Left: Amid the war in Gaza, the pro-Palestinian movement has grown into a powerful, if disjointed, political force in the United States. Democrats are feeling the pressure .
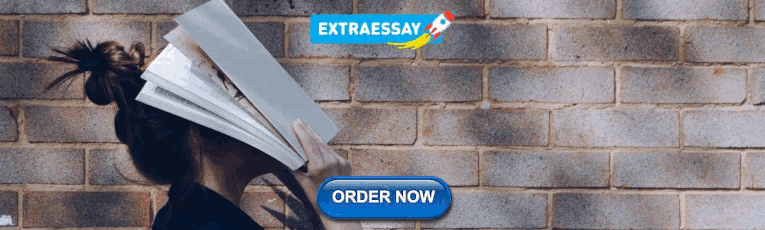
IMAGES
VIDEO
COMMENTS
The representations of each set of tasks are close to four vertices of a square. As a result, the top two principal components have similar eigenvalues and are therefore interchangeable.
The present study investigated the nature of task-set representations, defined as a set of criteria for spatial selection, post-selective identification, and S-R mapping rules. Two alternatives were considered: task sets as integral representations and task sets as agglomerations of relatively autonomous control settings guiding different ...
Introduction "Cognitive control" describes a set of processes that allows individuals to coordinate thoughts and actions in accordance with internal goals (Miller and Cohen, 2001).A key component of cognitive control is the capacity to flexibly configure, and switch between, "task sets," rule representations that define currently relevant stimulus attributes and determine how they map ...
Reinforcement learning relies on representation of tasks as sequences of states. Designing the correct state space for each task is critical in RL 26, 27, 28. First, different state ...
However, the fact that task-set representations were strongest, and also most predictive of performance in the presence of stimulus and response representations suggests that a key function of ...
regions in the dynamic reconfiguration of task -set representations from trial-to-trial. In the present study, we generated a set of fundamental predictions about neural task-set representations based on the cognitive literature, and tested those hypotheses by combining fMRI during a cued task switching protocol with MVPA and representational
Here we measured human brain activity during task switching and tracked the similarity of neural task-set representations from trial to trial. We show that frontal and parietal brain regions flexibly recode changing task sets in a trial-by-trial fashion, and that task-set similarity over consecutive trials predicts behavior. ...
we derive Hypothesis 1 as follows: task-set representations on switch trials should be more labile/less stable than on repeat trials (see, Classification accuracy and distance to the hyperplane analysis). Second, the basic assumption of task-set recoding within a common set of brain regions implies Hypothesis 2 as follows: task-set representa-
For task switch trials, agglomerated task sets predict a difference between dimension changes and repetitions: joint task and dimension switches require full task set reconfiguration, while dimension repetitions permit re-using some control settings from the previous trial.
Indeed, in a recent cued task-switching study using MVPA classification, reward incentives (which varied trial-by-trial and were precued) increased the distinctiveness of frontoparietal task-set representations, and these task-set coding changes explained individual differences in performance improvement on incentivized trials [52].
The dominant description of task representations is the task set, a collection of SR associations, whose presence is inferred primarily through task-switch costs. However, we argue that this approach has serious limitations that are often ignored. First, task switch costs likely reflect numerous processes, including those relating to attention ...
The present study examined the cognitive representation of tasks ("task sets") using the task-switching paradigm. To do so, we manipulated the task-set components "judgment" (i. e., stimulus categories) and "response modality" orthogonally in two-componential switching experiments. In Experiment 1, we additionally manipulated the type of cues, whereas we manipulated the cue-stimulus interval ...
A task set is a configuration of cognitive processes that is actively maintained for subsequent task performance. Single-unit and brain-imaging studies have identified the neural correlates for task sets in the prefrontal cortex. ... I then discuss the competitions between neural representations of task sets during task switch. Finally I ...
It is argued here that task switching research—and its associated theorizing—has been hampered by poor definitions of what are tasks and task sets. The chapter provides a distinction between the two: a task is the representation of a set of instructions required to perform an activity accurately; a task set is the set of representations and ...
Results indicate that even highly familiar S-R settings are maintained in working memory, albeit in a compressed manner, presumably through cues to long-term memory representations, suggesting that the establishment of effective retrieval structures itself is a capacity-limited process. Selection and preparation of action plans (task sets) is often assumed to occur in working memory (WM).
For instance, to run a memory experiment it is taken for granted that participants understand the instructions and implement an appropriate task set, that is a cognitive representation of the task, and that subserves both implementing the task and performing it. Researchers take the operation of task sets for granted in the same way that ...
Task representations are critical for cognitive control and adaptive behavior. The hierarchical organization of task representations allows humans to maintain goals, integrate information across varying contexts, and select potential responses. In this study we characterized the structure and interactive dynamics of task representations that facilitate cognitive control. Human participants ...
Here we measured human brain activity during task switching and tracked the similarity of neural task-set representations from trial to trial. We show that frontal and parietal brain regions flexibly recode changing task sets in a trial-by-trial fashion, and that task-set similarity over consecutive trials predicts behavior.
The Nature of Task Set Representations in Working Memory Abstract: Selection and preparation of action plans (task sets) is often assumed to occur in working memory (WM). Yet, the absence of consistent evidence that WM capacity and task selection efficiency is correlated raises questions about the functional relationship between these two ...
Abstract. Selection and preparation of action plans (task sets) is often assumed to occur in working memory (WM). Yet, the absence of consistent evidence that WM capacity and task selection efficiency is correlated raises questions about the functional relationship between these two aspects of executive control. We used the EEG-derived contralateral delay activity (CDA) to index the WM load of ...
To achieve goals, cognitive flexibility is important. Cognitive theories assume that the representation of task rules is central to understand cognitive flexibility. However, how this representational dynamic is implemented on a neurophysiology level is widely elusive. Using EEG-based MVPA-methods we show that relevant representational content is evident at multiple levels in the ...
Search worldwide, life-sciences literature Search. Advanced Search
The human brain's remarkable motor adaptability stems from the formation of context representations and the use of a common context representation (e.g., an invariant task structure across task contexts) derived from structural learning. However, direct evaluation of context representations and structural learning in sensorimotor tasks remains limited. This study aimed to rigorously ...
The present study examined the cognitive representation of tasks ("task sets") using the task-switching paradigm. To do so, we manipulated the task-set components "judgment" (i.e., stimulus ...
The task of face reenactment is to transfer the head motion and facial expressions from a driving video to the appearance of a source image, which may be of a different person (cross-reenactment). Most existing methods are CNN-based and estimate optical flow from the source image to the current driving frame, which is then inpainted and refined to produce the output animation. We propose a ...
Despite the advantages of their low-resource settings, traditional sparse retrievers depend on exact matching approaches between high-dimensional bag-of-words (BoW) representations of both the queries and the collection. As a result, retrieval performance is restricted by semantic discrepancies and vocabulary gaps. On the other hand, transformer-based dense retrievers introduce significant ...
A veteran NPR editor publicly questions whether the public radio network has, in its push for greater diversity and representation, overlooked conservative viewpoints.
The U.S. Department of Justice announced Thursday that Alabama is one of seven states where a carjacking task force will be launched to focus resources on what they described as a public safety ...
The presumptive Republican presidential nominee and the G.O.P. speaker, at odds over many issues, are making common cause on "election integrity."