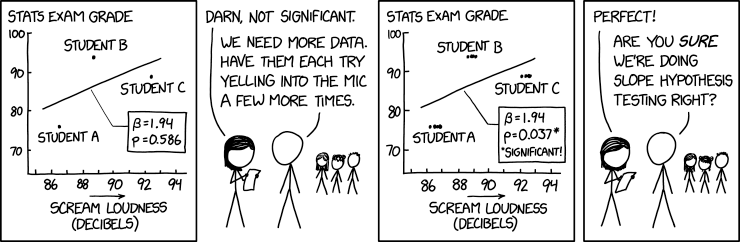
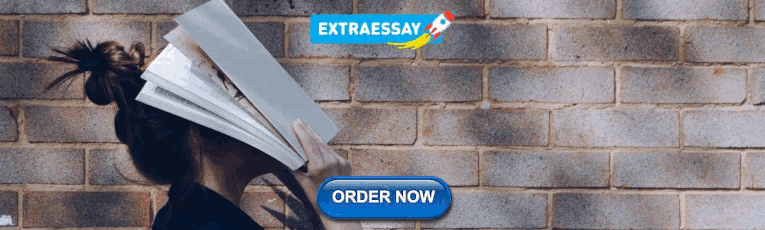
Slope Hypothesis Testing
"What? I can't hear--" "What? I said, are you sure--" "CAN YOU PLEASE SPEAK--"
2683: Fan Theories
Explanation [ edit ].
This is another comic on How to annoy people. Here Cueball annoys Megan , a Scientist, by referring to the hypotheses that there are subsurface oceans on some of Jupiter's Moons, as "fan theories".
A fan theory is an unofficial hypothesis about a fictional work from enthusiasts instead of the official authors. Fan theories may include non-canonical background information for, or continuations of, official narratives. Since humanity is not the "author" of the universe, all thoughts and writings on the nature of the universe are, almost by definition, non-canonical fan ideas.
In this comic, Cueball refers to scientific hypotheses as "fan theories." Scientists might consider this as undervaluing their work, because scientific hypotheses are usually the result of much more serious research than the authorship of fiction. Charismatic cranks often obtain undeserved recognition for supposedly scientific hypotheses, so being lumped in with charlatans or pseudoscientists could be perceived as insensitive to rigorous scientists. Once again, Randall has attempted to devise a new way to annoy scientists. However, many if not most professional scientists are aware of similarities between hypotheses pertaining to fiction and non-fiction.
By referring to rigorous scientific studies as "fan theories" Randall is also elevating the experience of fandom. Though they are typically not supported by research institutions, tenured faculty positions, or grant funding, fandom communities often demonstrate a high degree of skill in literary criticism including evidence gathering, philosophical interpretation, and generative writing. This continues the trend of building connections between disciplines in STEM, social science, and the humanities.
This comic appeared a few days after renewed suggestions that life may exist within one of Saturn's moons .
The title text may be facetious, having to do with unwelcoming or inconsiderate fandoms (or scientists who are annoyed at being called "fans of the universe"), or it could be sincere .
Transcript [ edit ]

To me, the title text seems to be referring to the opposite kind of fandom compared to what the current explanation says. 141.101.76.203 06:54, 11 October 2022 (UTC)
Given the constant stream of manchildren vandalizing this, could an admin please restrict editing access to logged in users? 172.68.110.143 07:50, 11 October 2022 (UTC)
I am 100% behind calling hypotheses 'fan theories'. Fephisto ( talk ) 16:45, 11 October 2022 (UTC)
To the person who inserted the {{ Citation needed }} initially unsuccessfully by [[citation needed]], and complained in their edit summaries that no other place uses our formatting (of {{}}s)... That's how Wikipedia implements it (or at least the actual Citation Needed... see below) and [[]]s/[]s are for more general hyperlinking (internal and external). You aren't thinking of some BBCode plugin for forums, are you? They will use [tags] and [tag][/tag], and I could imagine a popular tweak to the tag-handler to not require writing [sup][[u][color=#whatever]citation needed[/color][/u]][/sup] , or similar, every time someone wanted to parody (or properly apply?) the meme... ...although, reading your intention, did you instead intend to use the explainxkcd version that is {{ Actual citation needed }}? I can imagine another editor removing it, anyway, but I just moved it the more accepted side of the punctuation. 172.70.85.49 18:41, 11 October 2022 (UTC)
I think this comic might also be a comment on the following phenomenom: a lot of spectacular ideas that were once regarded as not really significant, "fringe", or even dubious by the mainstream scientific community two or three decades ago are now seriously pursued by science - mostly because the kids whose imagination was fired up by those ideas have now grown into scientists themselves, who can decide on their own what things to research. The idea that life might exist in the Europa ocean is a prime example for this: It was once mainly science fiction, popularized by Arthur C. Clarke in his 2010 and 2061 odyssey novels, but has since become a serious research subject. Similar things might be said about the time, energy and money that is nowadays devoted to SETI or to the search for primitive life on Mars. Scientists in the 60s and 70s would have probably fallen out of their chairs in shock if they knew. (note that I am all in favour of looking for aliens, but scientists in the 60s and 70s were much more interested in planetary geology and stuff like that). -- 172.70.247.38 09:01, 12 October 2022 (UTC)
The inclusion of Kuhn's work as part of the explanation seems odd. This may be because I don't know his work and thus don't see how this is connected to the comic and especially how merely mentioning it actually helps explaining the comic. I removed that part unless someone comes up with some actual explanation why this is relevant. Elektrizikekswerk ( talk ) 11:12, 12 October 2022 (UTC)
this suggests a "how to annoy" category w/ 2036 and 2654 (and possibly 2118 , though the "expert shouting at cueball" part is absent) -- 172.70.110.231 13:54, 12 October 2022 (UTC)
What does "franchise with an established fandom" mean? Could you guys please try to use a relatively simple vocabulary and define necessary jargon or specialized language, such as with link(s)? 172.69.22.163 19:46, 12 October 2022 (UTC)
It feels like the current explanation is missing a clear exposition of the key comparison between scientific research and fan theorising - that they both (a) start from a canonical body of work, (b) build on top of that with experimental theses that are then 'peer tested', and (c) may then in time end up becoming a part of the canon themselves. I can't quite work that up into a form that would flow nicely in the explanation, though. 172.70.91.58 13:45, 13 October 2022 (UTC)
By referring to scientists as fans, could Cueball be suggesting an author exists? 197.234.243.26 14:45, 13 October 2022 (UTC)
Does anyone else think that the astronomer in the the comic looks more like Danish than Megan? I guess her attitude and demeanor is more akin to a standard Megan, but idk. 15:55, 13 February 2023 (UTC)~ 3:55, 13 Feb 2023 (UTC)
Sorry if I'm a bit late, but I'd like to apologise on behalf of the "furry" vandals. My Species Doth Protest Too Much . I don't believe furries are "better than the rest of humanity combined." Your favorite aura doggo ( talk ) 03:59, 6 May 2023 (UTC)
Is there any fandom other than the universe fandom that makes fan theories that directly contradict the canon? 172.68.174.226 ( talk ) 04:10, 30 July 2023 (please sign your comments with ~~~~)
- Comics from 2022
- Comics from October
- Monday comics
- Comics featuring Ponytail
- Comics featuring Cueball
- Comics featuring White Hat
- Comics featuring Megan
- How to annoy
Navigation menu
Personal tools.
- Not logged in
- Contributions
- Create account
- View history
- Latest comic
- Community portal
- Recent changes
- Random page
- Browse comics
- What links here
- Related changes
- Special pages
- Printable version
- Permanent link
- Page information

- This page was last edited on 3 March 2023, at 19:15.
- Privacy policy
- About explain xkcd
- Disclaimers

13.1 Understanding Null Hypothesis Testing
Learning objectives.
- Explain the purpose of null hypothesis testing, including the role of sampling error.
- Describe the basic logic of null hypothesis testing.
- Describe the role of relationship strength and sample size in determining statistical significance and make reasonable judgments about statistical significance based on these two factors.
The Purpose of Null Hypothesis Testing
As we have seen, psychological research typically involves measuring one or more variables in a sample and computing descriptive statistics for that sample. In general, however, the researcher’s goal is not to draw conclusions about that sample but to draw conclusions about the population that the sample was selected from. Thus researchers must use sample statistics to draw conclusions about the corresponding values in the population. These corresponding values in the population are called parameters . Imagine, for example, that a researcher measures the number of depressive symptoms exhibited by each of 50 adults with clinical depression and computes the mean number of symptoms. The researcher probably wants to use this sample statistic (the mean number of symptoms for the sample) to draw conclusions about the corresponding population parameter (the mean number of symptoms for adults with clinical depression).
Unfortunately, sample statistics are not perfect estimates of their corresponding population parameters. This is because there is a certain amount of random variability in any statistic from sample to sample. The mean number of depressive symptoms might be 8.73 in one sample of adults with clinical depression, 6.45 in a second sample, and 9.44 in a third—even though these samples are selected randomly from the same population. Similarly, the correlation (Pearson’s r ) between two variables might be +.24 in one sample, −.04 in a second sample, and +.15 in a third—again, even though these samples are selected randomly from the same population. This random variability in a statistic from sample to sample is called sampling error . (Note that the term error here refers to random variability and does not imply that anyone has made a mistake. No one “commits a sampling error.”)
One implication of this is that when there is a statistical relationship in a sample, it is not always clear that there is a statistical relationship in the population. A small difference between two group means in a sample might indicate that there is a small difference between the two group means in the population. But it could also be that there is no difference between the means in the population and that the difference in the sample is just a matter of sampling error. Similarly, a Pearson’s r value of −.29 in a sample might mean that there is a negative relationship in the population. But it could also be that there is no relationship in the population and that the relationship in the sample is just a matter of sampling error.
In fact, any statistical relationship in a sample can be interpreted in two ways:
- There is a relationship in the population, and the relationship in the sample reflects this.
- There is no relationship in the population, and the relationship in the sample reflects only sampling error.
The purpose of null hypothesis testing is simply to help researchers decide between these two interpretations.
The Logic of Null Hypothesis Testing
Null hypothesis testing is a formal approach to deciding between two interpretations of a statistical relationship in a sample. One interpretation is called the null hypothesis (often symbolized H 0 and read as “H-naught”). This is the idea that there is no relationship in the population and that the relationship in the sample reflects only sampling error. Informally, the null hypothesis is that the sample relationship “occurred by chance.” The other interpretation is called the alternative hypothesis (often symbolized as H 1 ). This is the idea that there is a relationship in the population and that the relationship in the sample reflects this relationship in the population.
Again, every statistical relationship in a sample can be interpreted in either of these two ways: It might have occurred by chance, or it might reflect a relationship in the population. So researchers need a way to decide between them. Although there are many specific null hypothesis testing techniques, they are all based on the same general logic. The steps are as follows:
- Assume for the moment that the null hypothesis is true. There is no relationship between the variables in the population.
- Determine how likely the sample relationship would be if the null hypothesis were true.
- If the sample relationship would be extremely unlikely, then reject the null hypothesis in favor of the alternative hypothesis. If it would not be extremely unlikely, then retain the null hypothesis .
Following this logic, we can begin to understand why Mehl and his colleagues concluded that there is no difference in talkativeness between women and men in the population. In essence, they asked the following question: “If there were no difference in the population, how likely is it that we would find a small difference of d = 0.06 in our sample?” Their answer to this question was that this sample relationship would be fairly likely if the null hypothesis were true. Therefore, they retained the null hypothesis—concluding that there is no evidence of a sex difference in the population. We can also see why Kanner and his colleagues concluded that there is a correlation between hassles and symptoms in the population. They asked, “If the null hypothesis were true, how likely is it that we would find a strong correlation of +.60 in our sample?” Their answer to this question was that this sample relationship would be fairly unlikely if the null hypothesis were true. Therefore, they rejected the null hypothesis in favor of the alternative hypothesis—concluding that there is a positive correlation between these variables in the population.
A crucial step in null hypothesis testing is finding the likelihood of the sample result if the null hypothesis were true. This probability is called the p value . A low p value means that the sample result would be unlikely if the null hypothesis were true and leads to the rejection of the null hypothesis. A p value that is not low means that the sample result would be likely if the null hypothesis were true and leads to the retention of the null hypothesis. But how low must the p value be before the sample result is considered unlikely enough to reject the null hypothesis? In null hypothesis testing, this criterion is called α (alpha) and is almost always set to .05. If there is a 5% chance or less of a result as extreme as the sample result if the null hypothesis were true, then the null hypothesis is rejected. When this happens, the result is said to be statistically significant . If there is greater than a 5% chance of a result as extreme as the sample result when the null hypothesis is true, then the null hypothesis is retained. This does not necessarily mean that the researcher accepts the null hypothesis as true—only that there is not currently enough evidence to reject it. Researchers often use the expression “fail to reject the null hypothesis” rather than “retain the null hypothesis,” but they never use the expression “accept the null hypothesis.”
The Misunderstood p Value
The p value is one of the most misunderstood quantities in psychological research (Cohen, 1994) [1] . Even professional researchers misinterpret it, and it is not unusual for such misinterpretations to appear in statistics textbooks!
The most common misinterpretation is that the p value is the probability that the null hypothesis is true—that the sample result occurred by chance. For example, a misguided researcher might say that because the p value is .02, there is only a 2% chance that the result is due to chance and a 98% chance that it reflects a real relationship in the population. But this is incorrect . The p value is really the probability of a result at least as extreme as the sample result if the null hypothesis were true. So a p value of .02 means that if the null hypothesis were true, a sample result this extreme would occur only 2% of the time.
You can avoid this misunderstanding by remembering that the p value is not the probability that any particular hypothesis is true or false. Instead, it is the probability of obtaining the sample result if the null hypothesis were true.

“Null Hypothesis” retrieved from http://imgs.xkcd.com/comics/null_hypothesis.png (CC-BY-NC 2.5)
Role of Sample Size and Relationship Strength
Recall that null hypothesis testing involves answering the question, “If the null hypothesis were true, what is the probability of a sample result as extreme as this one?” In other words, “What is the p value?” It can be helpful to see that the answer to this question depends on just two considerations: the strength of the relationship and the size of the sample. Specifically, the stronger the sample relationship and the larger the sample, the less likely the result would be if the null hypothesis were true. That is, the lower the p value. This should make sense. Imagine a study in which a sample of 500 women is compared with a sample of 500 men in terms of some psychological characteristic, and Cohen’s d is a strong 0.50. If there were really no sex difference in the population, then a result this strong based on such a large sample should seem highly unlikely. Now imagine a similar study in which a sample of three women is compared with a sample of three men, and Cohen’s d is a weak 0.10. If there were no sex difference in the population, then a relationship this weak based on such a small sample should seem likely. And this is precisely why the null hypothesis would be rejected in the first example and retained in the second.
Of course, sometimes the result can be weak and the sample large, or the result can be strong and the sample small. In these cases, the two considerations trade off against each other so that a weak result can be statistically significant if the sample is large enough and a strong relationship can be statistically significant even if the sample is small. Table 13.1 shows roughly how relationship strength and sample size combine to determine whether a sample result is statistically significant. The columns of the table represent the three levels of relationship strength: weak, medium, and strong. The rows represent four sample sizes that can be considered small, medium, large, and extra large in the context of psychological research. Thus each cell in the table represents a combination of relationship strength and sample size. If a cell contains the word Yes , then this combination would be statistically significant for both Cohen’s d and Pearson’s r . If it contains the word No , then it would not be statistically significant for either. There is one cell where the decision for d and r would be different and another where it might be different depending on some additional considerations, which are discussed in Section 13.2 “Some Basic Null Hypothesis Tests”
Although Table 13.1 provides only a rough guideline, it shows very clearly that weak relationships based on medium or small samples are never statistically significant and that strong relationships based on medium or larger samples are always statistically significant. If you keep this lesson in mind, you will often know whether a result is statistically significant based on the descriptive statistics alone. It is extremely useful to be able to develop this kind of intuitive judgment. One reason is that it allows you to develop expectations about how your formal null hypothesis tests are going to come out, which in turn allows you to detect problems in your analyses. For example, if your sample relationship is strong and your sample is medium, then you would expect to reject the null hypothesis. If for some reason your formal null hypothesis test indicates otherwise, then you need to double-check your computations and interpretations. A second reason is that the ability to make this kind of intuitive judgment is an indication that you understand the basic logic of this approach in addition to being able to do the computations.
Statistical Significance Versus Practical Significance
Table 13.1 illustrates another extremely important point. A statistically significant result is not necessarily a strong one. Even a very weak result can be statistically significant if it is based on a large enough sample. This is closely related to Janet Shibley Hyde’s argument about sex differences (Hyde, 2007) [2] . The differences between women and men in mathematical problem solving and leadership ability are statistically significant. But the word significant can cause people to interpret these differences as strong and important—perhaps even important enough to influence the college courses they take or even who they vote for. As we have seen, however, these statistically significant differences are actually quite weak—perhaps even “trivial.”
This is why it is important to distinguish between the statistical significance of a result and the practical significance of that result. Practical significance refers to the importance or usefulness of the result in some real-world context. Many sex differences are statistically significant—and may even be interesting for purely scientific reasons—but they are not practically significant. In clinical practice, this same concept is often referred to as “clinical significance.” For example, a study on a new treatment for social phobia might show that it produces a statistically significant positive effect. Yet this effect still might not be strong enough to justify the time, effort, and other costs of putting it into practice—especially if easier and cheaper treatments that work almost as well already exist. Although statistically significant, this result would be said to lack practical or clinical significance.

“Conditional Risk” retrieved from http://imgs.xkcd.com/comics/conditional_risk.png (CC-BY-NC 2.5)
Key Takeaways
- Null hypothesis testing is a formal approach to deciding whether a statistical relationship in a sample reflects a real relationship in the population or is just due to chance.
- The logic of null hypothesis testing involves assuming that the null hypothesis is true, finding how likely the sample result would be if this assumption were correct, and then making a decision. If the sample result would be unlikely if the null hypothesis were true, then it is rejected in favor of the alternative hypothesis. If it would not be unlikely, then the null hypothesis is retained.
- The probability of obtaining the sample result if the null hypothesis were true (the p value) is based on two considerations: relationship strength and sample size. Reasonable judgments about whether a sample relationship is statistically significant can often be made by quickly considering these two factors.
- Statistical significance is not the same as relationship strength or importance. Even weak relationships can be statistically significant if the sample size is large enough. It is important to consider relationship strength and the practical significance of a result in addition to its statistical significance.
- Discussion: Imagine a study showing that people who eat more broccoli tend to be happier. Explain for someone who knows nothing about statistics why the researchers would conduct a null hypothesis test.
- The correlation between two variables is r = −.78 based on a sample size of 137.
- The mean score on a psychological characteristic for women is 25 ( SD = 5) and the mean score for men is 24 ( SD = 5). There were 12 women and 10 men in this study.
- In a memory experiment, the mean number of items recalled by the 40 participants in Condition A was 0.50 standard deviations greater than the mean number recalled by the 40 participants in Condition B.
- In another memory experiment, the mean scores for participants in Condition A and Condition B came out exactly the same!
- A student finds a correlation of r = .04 between the number of units the students in his research methods class are taking and the students’ level of stress.
- Cohen, J. (1994). The world is round: p < .05. American Psychologist, 49 , 997–1003. ↵
- Hyde, J. S. (2007). New directions in the study of gender similarities and differences. Current Directions in Psychological Science, 16 , 259–263. ↵

Share This Book
- Increase Font Size
- History of cooperation
- Areas of cooperation
- Procurement policy
- Useful links
- Becoming a supplier
- Procurement
- Rosatom newsletter
© 2008–2024Valtiollinen Rosatom-ydinvoimakonserni

- Rosatom Global presence
- Rosatom in region
- For suppliers
- Preventing corruption
- Press centre
Rosatom Starts Life Tests of Third-Generation VVER-440 Nuclear Fuel
- 16 June, 2020 / 13:00
This site uses cookies. By continuing your navigation, you accept the use of cookies. For more information, or to manage or to change the cookies parameters on your computer, read our Cookies Policy. Learn more
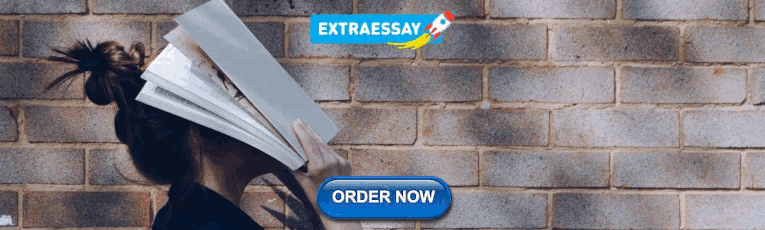
Toroidally focused ultrasonic flaw detectors
- Acoustic Methods
- Published: 28 July 2011
- Volume 47 , pages 308–310, ( 2011 )
Cite this article
- A. V. Shevelev 1 &
- Zh. V. Zatsepilova 2
33 Accesses
Explore all metrics
New-type toroidally focused ultrasonic flaw detectors, whose application provides an appreciable increase in the flaw detection rate with retention of high sensitivity to flaws, are considered. The construction of a flaw detector is presented, the sizes of a gauge for the formation of the toroidal surface of a lens are given, and the technology of the manufacturing of a toroidal lens is described.
This is a preview of subscription content, log in via an institution to check access.
Access this article
Price includes VAT (Russian Federation)
Instant access to the full article PDF.
Rent this article via DeepDyve
Institutional subscriptions
Similar content being viewed by others
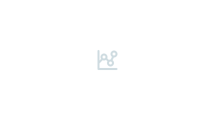
Remote diagnostics of soft solids using nonlinear acoustic methods
A. I. Korobov, M. Yu. Izosimova, … N. I. Odina
Ultrasonic Flaw Detection: Adjustment and Calibration of Equipment Using Samples with Cylindrical Drilling
L. Yu. Mogilner & Ya. G. Smorodinskii
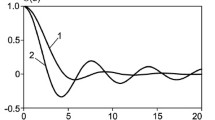
Influence of Pitch of Ultrasonic Antenna Array on Efficiency of Extraction of a Signal from Structural Noise in Flaw Detection
Zayed Saleh Salem Ali, V. G. Kartashev, … I. V. Sokolov
Ermolov, I.N., Aleshin, N.P., and Potapov, A.I., Nerazrushayushchii control’ (Nondestructive Testing), book 2: Akusticheskie metody kontrolya (Acoustic Testing), Moscow: Vysshaya shkola, 1991.
Google Scholar
Nerazrushayushchii kontrol’ (Spravochnik) (Nondestructive Testing: Handbook), Klyuev, V.V., Ed., vol. 3: Ul’trazvukovoi kontrol’ (Ultrasonic Testing), Moscow: Mashinostroenie, 2006.
Download references
Author information
Authors and affiliations.
Elektrostal Polytechnic Institute, Branch of the National University of Science and Technology “MISIS”, ul. Pervomaiskaya 7, Elektrostal, Moscow oblast, 144000, Russia
A. V. Shevelev
Elektrostal Heavy Engineering Plant JSC, ul. Krasnaya 19, Elektrostal, Moscow oblast, 144005, Russia
Zh. V. Zatsepilova
You can also search for this author in PubMed Google Scholar
Corresponding author
Correspondence to Zh. V. Zatsepilova .
Additional information
Original Russian Text © A.V. Shevelev, Zh.V. Zatsepilova, 2011, published in Defektoskopiya, 2011, Vol. 47, No. 5, pp. 19–22.
Rights and permissions
Reprints and permissions
About this article
Shevelev, A.V., Zatsepilova, Z.V. Toroidally focused ultrasonic flaw detectors. Russ J Nondestruct Test 47 , 308–310 (2011). https://doi.org/10.1134/S1061830911050093
Download citation
Received : 14 January 2011
Published : 28 July 2011
Issue Date : May 2011
DOI : https://doi.org/10.1134/S1061830911050093
Share this article
Anyone you share the following link with will be able to read this content:
Sorry, a shareable link is not currently available for this article.
Provided by the Springer Nature SharedIt content-sharing initiative
- ultrasonic flaw detectors
- focusing lens
- flaw detection
- nondestructive testing
- inspection of pipes
- Find a journal
- Publish with us
- Track your research
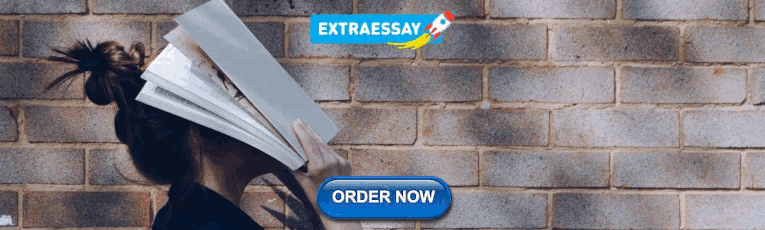
IMAGES
VIDEO
COMMENTS
xkcd: Slope Hypothesis Testing. A webcomic of romance, sarcasm, math, and language. What If? is on YouTube! The first video answers "What if we aimed Hubble at Earth?". Follow the What If? channel to be notified about new videos.
Explanation. "Slope hypothesis testing" is a method of testing the significance of a hypothesis involving a scatter plot. In this comic, Cueball and Megan are performing a study comparing student exam grades to the volume of their screams. Student A has the worst grade and softest scream, but Student B has the best grades and Student C the ...
Explain the xkcd jelly bean comic: What makes it funny? I see that one time out of the twenty total tests they run, p < 0.05 p < 0.05, so they wrongly assume that during one of the twenty tests, the result is significant ( 0.05 = 1/20 0.05 = 1 / 20 ). Hover text: "'So, uh, we did the green study again and got no link.
Slope Hypothesis Testing. ? What If? is now on YouTube! "What if we aimed the Hubble Telescope at Earth?". and follow xkcd's What If? The Video Series channel to be notified about each new video.
Explain xkcd is a wiki dedicated to explaining the webcomic xkcd. Go figure. 882: Significant. Explain xkcd: It's 'cause you're dumb. ... The comic and the comment above you are correct in saying that if the null hypothesis holds, 1 out of every 20 tests will produce a false positive: this is by definition of the p-value. ...
Explanation []. Miss Lenhart is teaching a science class and starts by formulating the fact that to perform any science you need to generate a hypothesis in order to test it.. The front row student, Cueball (presumably the rest of the students in the class are off panel), is thus prompted to ask the salient question of how one finds an original hypothesis. . By using a clever prompting ...
Discussion. IMHO the current explanation is misleading. The p-value describes how well the experiment output fits hypothesis. The hypothesis can be that the experiment output is random. The low p-values point out that the experiment output fits well with behavior predicted by the hypothesis. The higher the p-value the more the observed and ...
Null hypothesis. The null hypothesis, mentioned in the title text, is the hypothesis in a statistical analysis that indicates that the effect investigated by the analysis does not occur, i.e. 'null' as in zero effect. For example, the null hypothesis for this study might be "The vaccine has no effect on whether subjects catch COVID."
Congrats, your comment is the only thing that pops up when I Google "Toyota Sakenda". Wtf does this mean. 43. Dont_Think_So • 2 yr. ago. Toyota Process: A way to track and avoid manufacturing issues by looking at statistics of defects. Sakenda: Japanese for "scream". Guess we need an "explain xkcd comments" site too.
The null hypothesis is the hypothesis in a statistical analysis that indicates that the effect investigated by the analysis does not occur, i.e. 'null' as in zero effect. For example, the null hypothesis for a study about cell phones and cancer risk might be "Cell phones have no effect on cancer risk." The alternative hypothesis, by contrast ...
A webcomic of romance, sarcasm, math, and language. What If? is now on YouTube! Check out the first video for the answer to "What if we aimed the Hubble Telescope at Earth?" and follow xkcd's What If? The Video Series channel to be notified about each new video. Slope Hypothesis Testing. |<.
Fan theories may include non-canonical background information for, or continuations of, official narratives. Since humanity is not the "author" of the universe, all thoughts and writings on the nature of the universe are, almost by definition, non-canonical fan ideas. In this comic, Cueball refers to scientific hypotheses as "fan theories."
But then Megan and Cueball ask the scientists to test whether only one color of jelly beans is responsible. They test 20 different colors, each at a significance level of 5%. If the probability that each trial gives. a false positive result is 1 in 20, then by testing 20 different colors it is now likely that at least one jelly bean test will ...
This is known as null hypothesis significance testing. Before they start the experiment, they choose a threshold known as the probability level. In the comic, the scientists choose a probability level of 5%. The probability level indicates the probability of obtaining test results at least as extreme as the results actually observed, assuming ...
The hypothesis testing framework 1.Start with two hypotheses about the population: thenull hypothesisand thealternative hypothesis 2.Choose a sample, collect data, and analyze the data 3.Figure out how likely it is to see data like what we got/observed, IF the null hypothesis were true 4.If our data would have been extremely unlikely if the null
A crucial step in null hypothesis testing is finding the likelihood of the sample result if the null hypothesis were true. This probability is called the p value. A low p value means that the sample result would be unlikely if the null hypothesis were true and leads to the rejection of the null hypothesis. A p value that is not low means that ...
Round table 2021. "Electrostal" Metallurgical plant" JSC has a number of remarkable time-tested traditions. One of them is holding an annual meeting with customers and partners in an extеnded format in order to build development pathways together, resolve pressing tasks and better understand each other. Although the digital age ...
The life tests started after successful completion of hydraulic tests (hydraulic filling) of the mock-up with the aim to determine RK3+ hydraulic resistance. Life tests are carried out on a full-scale research hot run-in test bench V-440 and will last for full 1500 hours. The aim of tests is to study mechanical stability of RK3+ components ...
Alexandrov, who represented the USSR at the Bikini "Crossroads" tests in 1946 and in UN discussions on atomic energy in 1947; the latter presumably was A. I. Leipunski, a well-known Russian nuclear physicist. When Krebs was then flown to Moscow (without his consent) he learned that the German groups worked as an independent organization under
New-type toroidally focused ultrasonic flaw detectors, whose application provides an appreciable increase in the flaw detection rate with retention of high sensitivity to flaws, are considered. The construction of a flaw detector is presented, the sizes of a gauge for the formation of the toroidal surface of a lens are given, and the technology of the manufacturing of a toroidal lens is described.