- Open access
- Published: 27 October 2021
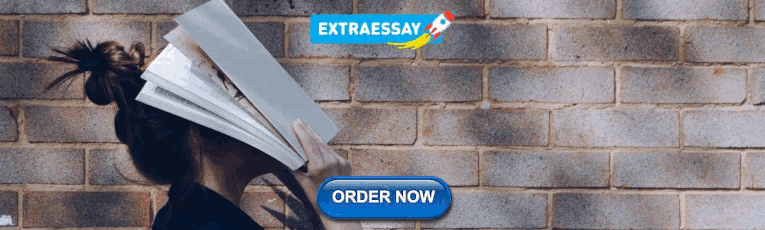
A narrative review on the validity of electronic health record-based research in epidemiology
- Milena A. Gianfrancesco 1 &
- Neal D. Goldstein ORCID: orcid.org/0000-0002-9597-5251 2
BMC Medical Research Methodology volume 21 , Article number: 234 ( 2021 ) Cite this article
10k Accesses
43 Citations
5 Altmetric
Metrics details
Electronic health records (EHRs) are widely used in epidemiological research, but the validity of the results is dependent upon the assumptions made about the healthcare system, the patient, and the provider. In this review, we identify four overarching challenges in using EHR-based data for epidemiological analysis, with a particular emphasis on threats to validity. These challenges include representativeness of the EHR to a target population, the availability and interpretability of clinical and non-clinical data, and missing data at both the variable and observation levels. Each challenge reveals layers of assumptions that the epidemiologist is required to make, from the point of patient entry into the healthcare system, to the provider documenting the results of the clinical exam and follow-up of the patient longitudinally; all with the potential to bias the results of analysis of these data. Understanding the extent of as well as remediating potential biases requires a variety of methodological approaches, from traditional sensitivity analyses and validation studies, to newer techniques such as natural language processing. Beyond methods to address these challenges, it will remain crucial for epidemiologists to engage with clinicians and informaticians at their institutions to ensure data quality and accessibility by forming multidisciplinary teams around specific research projects.
Peer Review reports
The proliferation of electronic health records (EHRs) spurred on by federal government incentives over the past few decades has resulted in greater than an 80% adoption-rate at hospitals [ 1 ] and close to 90% in office-based practices [ 2 ] in the United States. A natural consequence of the availability of electronic health data is the conduct of research with these data, both observational and experimental [ 3 ], due to lower overhead costs and lower burden of study recruitment [ 4 ]. Indeed, a search on PubMed for publications indexed by the MeSH term “electronic health records” reveals an exponential growth in biomedical literature, especially over the last 10 years with an excess of 50,000 publications.
An emerging literature is beginning to recognize the many challenges that still lay ahead in using EHR data for epidemiological investigations. Researchers in Europe identified 13 potential sources of “bias” (bias was defined as a contamination of the data) in EHR-based data covering almost every aspect of care delivery, from selective entrance into the healthcare system, to variation in care and documentation practices, to identification and extraction of the right data for analysis [ 5 ]. Many of the identified contaminants are directly relevant to traditional epidemiological threats to validity [ 4 ]. Data quality has consistently been invoked as a central challenge in EHRs. From a qualitative perspective, healthcare workers have described challenges in the healthcare environment (e.g., heavy workload), imperfect clinical documentation practices, and concerns over data extraction and reporting tools, all of which would impact the quality of data in the EHR [ 6 ]. From a quantitative perspective, researchers have noted limited sensitivity of diagnostic codes in the EHR when relying on discrete codings, noting that upon a manual chart review free text fields often capture the missed information, motivating such techniques as natural language processing (NLP) [ 7 ]. A systematic review of EHR-based studies also identified data quality as an overarching barrier to the use of EHRs in managing the health of the community, i.e. “population health” [ 8 ]. Encouragingly this same review also identified more facilitators than barriers to the use of EHRs in public health, suggesting that opportunities outweigh the challenges. Shortreed et al. further explored these opportunities discussing how EHRs can enhance pragmatic trials, bring additional sophistication to observational studies, aid in predictive modeling, and be linked together to create more comprehensive views of patients’ health [ 9 ]. Yet, as Shortreed and others have noted, significant challenges still remain.
It is our intention with this narrative review to discuss some of these challenges in further detail. In particular, we focus on specific epidemiological threats to validity -- internal and external -- and how EHR-based epidemiological research in particular can exacerbate some of these threats. We note that while there is some overlap in the challenges we discuss with traditional paper-based medical record research that has occurred for decades, the scale and scope of an EHR-based study is often well beyond what was traditionally possible in the manual chart review era and our applied examples attempt to reflect this. We also describe existing and emerging approaches for remediating these potential biases as they arise. A summary of these challenges may be found in Table 1 . Our review is grounded in the healthcare system in the United States, although we expect many of the issues we describe to be applicable regardless of locale; where necessary, we have flagged our comments as specific to the U.S.
Challenge #1: Representativeness
The selection process for how patients are captured in the EHR is complex and a function of geographic, social, demographic, and economic determinants [ 10 ]. This can be termed the catchment of the EHR. For a patient record to appear in the EHR the patient must have been registered in the system, typically to capture their demographic and billing information, and upon a clinical visit, their health details. While this process is not new to clinical epidemiology, what tends to separate EHR-based records from traditional paper-based records is the scale and scope of the data. Patient data may be available for longer periods of time longitudinally, as well as have data corresponding to interactions with multiple, potentially disparate, healthcare systems [ 11 ]. Given the consolidation of healthcare [ 12 ] and aggregated views of multiple EHRs through health information networks or exchanges [ 11 ] the ability to have a complete view of the patients’ total health is increasing. Importantly, the epidemiologist must ascertain whether the population captured within the EHR or EHR-derived data is representative of the population targeted for inference. This is particularly true under the paradigm of population health and inferring the health status of a community from EHR-based records [ 13 ]. For example, a study of Clostridium difficile infection at an urban safety net hospital in Philadelphia, Pennsylvania demonstrated notable differences in risk factors in the hospital’s EHR compared to national surveillance data, suggesting how catchment can influence epidemiologic measures [ 14 ]. Even health-related data captured through health information exchanges may be incomplete [ 15 ].
Several hypothetical study settings can further help the epidemiologist appreciate the relationship between representativeness and validity in EHR research. In the first hypothetical, an EHR-based study is conducted from a single-location federally qualified health center, and in the second hypothetical, an EHR-based study is conducted from a large academic health system. Suppose both studies occur in the same geographic area. It is reasonable to believe the patient populations captured in both EHRs will be quite different and the catchment process could lead to divergent estimates of disease or risk factor prevalence. The large academic health system may be less likely to capture primary care visits, as specialty care may drive the preponderance of patient encounters. However, this is not a bias per se : if the target of inference from these two hypothetical EHR-based studies is the local community, then selection bias becomes a distinct possibility. The epidemiologist must also consider the potential for generalizability and transportability -- two facets of external validity that respectively relate to the extrapolation of study findings to the source population or a different population altogether -- if there are unmeasured effect modifiers, treatment interference, or compound treatments in the community targeted for inference [ 16 ].
There are several approaches for ascertaining representativeness of EHR-based data. Comparing the EHR-derived sample to Census estimates of demography is straightforward but has several important limitations. First, as previously described, the catchment process may be driven by discordant geographical areas, especially for specialty care settings. Second and third, the EHR may have limited or inaccurate information on socioeconomic status, race, and ethnicity that one may wish to compare [ 17 , 18 ], and conversely the Census has limited estimates of health, chiefly disability, fertility, and insurance and payments [ 19 ]. If selection bias is suspected as a result of missing visits in a longitudinal study [ 20 ] or the catchment process in a cross-sectional study [ 21 ], using inverse probability weighting may remediate its influence. Comparing the weighted estimates to the original, non-weighted estimates provides insight into differences in the study participants. In the population health paradigm whereby the EHR is used as a surveillance tool to identify community health disparities [ 13 ], one also needs to be concerned about representativeness. There are emerging approaches for producing such small area community estimates from large observational datasets [ 22 , 23 ]. Conceivably, these approaches may also be useful for identifying issues of representativeness, for example by comparing stratified estimates across sociodemographic or other factors that may relate to catchment. Approaches for issues concerning representativeness specifically as it applies to external validity may be found in these references [ 24 , 25 ].
Challenge #2: Data availability and interpretation
Sub-challenge #2.1: billing versus clinical versus epidemiological needs.
There is an inherent tension in the use of EHR-based data for research purposes: the EHR was never originally designed for research. In the U.S., the Health Information Technology for Economic and Clinical Health Act, which promoted EHRs as a platform for comparative effectiveness research, was an attempt to address this deficiency [ 26 ]. A brief history of the evolution of the modern EHR reveals a technology that was optimized for capturing health details relevant for billing, scheduling, and clinical record keeping [ 27 ]. As such, the availability of data for fundamental markers of upstream health that are important for identifying inequities, such as socioeconomic status, race, ethnicity, and other social determinants of health (SDOH), may be insufficiently captured in the EHR [ 17 , 18 ]. Similarly, behavioral risk factors, such as being a sexual minority person, have historically been insufficiently recorded as discrete variables. It is only recently that such data are beginning to be captured in the EHR [ 28 , 29 ], or techniques such as NLP have made it possible to extract these details when stored in free text notes (described further in “ Unstructured data: clinical notes and reports ” section).
As an example, assessing clinical morbidities in the EHR may be done on the basis of extracting appropriate International Classification of Diseases (ICD) codes, used for billing and reimbursement in the U.S. These codes are known to have low sensitivity despite high specificity for accurate diagnostic status [ 30 , 31 ]. Expressed as predictive values, which depend upon prevalence, presence of a diagnostic code is a likely indicator of a disease state, whereas absence of a diagnostic code is a less reliable indicator of the absence of that morbidity. There may further be variation by clinical domain in that ICD codes may exist but not be used in some specialties [ 32 ], variation by coding vocabulary such as the use of SNOMED for clinical documentation versus ICD for billing necessitating an ontology mapper [ 33 ], and variation by the use of “rule-out” diagnostic codes resulting in false-positive diagnoses [ 34 , 35 , 36 ]. Relatedly is the notion of upcoding, or the billing of tests, procedures, or diagnoses to receive inflated reimbursement, which, although posited to be problematic in EHRs [ 37 ] in at least one study, has not been shown to have occurred [ 38 ]. In the U.S., the billing and reimbursement model, such as fee-for-service versus managed care, may result in varying diagnostic code sensitivities and specificities, especially if upcoding is occurring [ 39 ]. In short, there is potential for misclassification of key health data in the EHR.
Misclassification can potentially be addressed through a validation study (resources permitting) or application of quantitative bias analysis, and there is a rich literature regarding the treatment of misclassified data in statistics and epidemiology. Readers are referred to these texts as a starting point [ 40 , 41 ]. Duda et al. and Shepherd et al. have described an innovative data audit approach applicable to secondary analysis of observational data, such as EHR-derived data, that incorporates the audit error rate directly in the regression analysis to reduce information bias [ 42 , 43 ]. Outside of methodological tricks in the face of imperfect data, researchers must proactively engage with clinical and informatics colleagues to ensure that the right data for the research interests are available and accessible.
Sub-challenge #2.2: Consistency in data and interpretation
For the epidemiologist, abstracting data from the EHR into a research-ready analytic dataset presents a host of complications surrounding data availability, consistency and interpretation. It is easy to conflate the total volume of data in the EHR with data that are usable for research, however expectations should be tempered. Weiskopf et al. have noted such challenges for the researcher: in their study, less than 50% of patient records had “complete” data for research purposes per their four definitions of completeness [ 44 ]. Decisions made about the treatment of incomplete data can induce selection bias or impact precision of estimates (see Challenges #1 , #3 , and #4 ). The COVID-19 pandemic has further demonstrated the challenge of obtaining research data from EHRs across multiple health systems [ 45 ]. On the other hand, EHRs have a key advantage of providing near real-time data as opposed to many epidemiological studies that have a specific endpoint or are retrospective in nature. Such real-time data availability was leveraged during COVID-19 to help healthcare systems manage their pandemic response [ 46 , 47 ]. Logistical and technical issues aside, healthcare and documentation practices are nuanced to their local environments. In fact, researchers have demonstrated how the same research question analyzed in distinct clinical databases can yield different results [ 48 ].
Once the data are obtained, choices regarding operationalization of variables have the potential to induce information bias. Several hypothetical examples can help demonstrate this point. As a first example, differences in laboratory reporting may result in measurement error or misclassification. While the order for a particular laboratory assay is likely consistent within the healthcare system, patients frequently have a choice where to have that order fulfilled. Given the breadth of assays and reporting differences that may differ lab to lab [ 49 ], it is possible that the researcher working with the raw data may not consider all possible permutations. In other words, there may be lack of consistency in the reporting of the assay results. As a second example, raw clinical data requires interpretation to become actionable. A researcher interested in capturing a patient’s Charlson comorbidity index, which is based on 16 potential diagnoses plus the patient’s age [ 50 ], may never find such a variable in the EHR. Rather, this would require operationalization based on the raw data, each of which may be misclassified. Use of such composite measures introduces the notion of “differential item functioning”, whereby a summary indicator of a complexly measured health phenomenon may differ from group to group [ 51 ]. In this case, as opposed to a measurement error bias, this is one of residual confounding in that a key (unmeasured) variable is driving the differences. Remediation of these threats to validity may involve validation studies to determine the accuracy of a particular classifier, sensitivity analysis employing alternative interpretations when the raw data are available, and omitting or imputing biased or latent variables [ 40 , 41 , 52 ]. Importantly, in all cases, the epidemiologists should work with the various health care providers and personnel who have measured and recorded the data present in the EHR, as they likely understand it best.
Furthermore and related to “Billing versus Clinical versus Epidemiological Needs” section, the healthcare system in the U.S. is fragmented with multiple payers, both public and private, potentially exacerbating the data quality issues we describe, especially when linking data across healthcare systems. Single payer systems have enabled large and near-complete population-based studies due to data availability and consistency [ 53 , 54 , 55 ]. Data may also be inconsistent for retrospective longitudinal studies spanning many years if there have been changes to coding standards or practices over time, for example due to the transition from ICD-9 to ICD-10 largely occurring in the mid 2010s or the adoption of the Patient Protection and Affordable Care Act in the U.S. in 2010 with its accompanying changes in billing. Exploratory data analysis may reveal unexpected differences in key variables, by place or time, and recoding, when possible, can enforce consistency.
Sub-challenge #2.3: Unstructured data: clinical notes and reports
There may also be scenarios where structured data fields, while available, are not traditionally or consistently used within a given medical center or by a given provider. For example, reporting of adverse events of medications, disease symptoms, and vaccinations or hospitalizations occurring at different facility/health networks may not always be entered by providers in structured EHR fields. Instead, these types of patient experiences may be more likely to be documented in an unstructured clinical note, report (e.g. pathology or radiology report), or scanned document. Therefore, reliance on structured data to identify and study such issues may result in underestimation and potentially biased results.
Advances in NLP currently allow for information to be extracted from unstructured clinical notes and text fields in a reliable and accurate manner using computational methods. NLP utilizes a range of different statistical, machine learning, and linguistic techniques, and when applied to EHR data, has the potential to facilitate more accurate detection of events not traditionally located or consistently used in structured fields. Various NLP methods can be implemented in medical text analysis, ranging from simplistic and fast term recognition systems to more advanced, commercial NLP systems [ 56 ]. Several studies have successfully utilized text mining to extract information on a variety of health-related issues within clinical notes, such as opioid use [ 57 ], adverse events [ 58 , 59 ], symptoms (e.g., shortness of breath, depression, pain) [ 60 ], and disease phenotype information documented in pathology or radiology reports, including cancer stage, histology, and tumor grade [ 61 ], and lupus nephritis [ 32 ]. It is worth noting that scanned documents involve an additional layer of computation, relying on techniques such as optical character recognition, before NLP can be applied.
Hybrid approaches that combine both narrative and structured data, such as ICD codes, to improve accuracy of detecting phenotypes have also demonstrated high performance. Banerji et al. found that using ICD-9 codes to identify allergic drug reactions in the EHR had a positive predictive value of 46%, while an NLP algorithm in conjunction with ICD-9 codes resulted in a positive predictive value of 86%; negative predictive value also increased in the combined algorithm (76%) compared to ICD-9 codes alone (39%) [ 62 ]. In another example, researchers found that the combination of unstructured clinical notes with structured data for prediction tasks involving in-hospital mortality and 30-day hospital readmission outperformed models using either clinical notes or structured data alone [ 63 ]. As we move forward in analyzing EHR data, it will be important to take advantage of the wealth of information buried in unstructured data to assist in phenotyping patient characteristics and outcomes, capture missing confounders used in multivariate analyses, and develop prediction models.
Challenge #3: Missing measurements
While clinical notes may be useful to recover incomplete information from structured data fields, it may be the case that certain variables are not collected within the EHR at all. As mentioned above, it is important to remember that EHRs were not developed as a research tool (see “ Billing versus clinical versus epidemiological needs ” section), and important variables often used in epidemiologic research may not be typically included in EHRs including socioeconomic status (education, income, occupation) and SDOH [ 17 , 18 ]. Depending upon the interest of the provider or clinical importance placed upon a given variable, this information may be included in clinical notes. While NLP could be used to capture these variables, because they may not be consistently captured, there may be bias in identifying those with a positive mention as a positive case and those with no mention as a negative case. For example, if a given provider inquires about homelessness of a patient based on knowledge of the patient’s situation or other external factors and documents this in the clinical note, we have greater assurance that this is a true positive case. However, lack of mention of homelessness in a clinical note should not be assumed as a true negative case for several reasons: not all providers may feel comfortable asking about and/or documenting homelessness, they may not deem this variable worth noting, or implicit bias among clinicians may affect what is captured. As a result, such cases (i.e. no mention of homelessness) may be incorrectly identified as “not homeless,” leading to selection bias should a researcher form a cohort exclusively of patients who are identified as homeless in the EHR.
Not adjusting for certain measurements missing from EHR data can also lead to biased results if the measurement is an important confounder. Consider the example of distinguishing between prevalent and incident cases of disease when examining associations between disease treatments and patient outcomes [ 64 ]. The first date of an ICD code entered for a given patient may not necessarily be the true date of diagnosis, but rather documentation of an existing diagnosis. This limits the ability to adjust for disease duration, which may be an important confounder in studies comparing various treatments with patient outcomes over time, and may also lead to reverse causality if disease sequalae are assumed to be risk factors.
Methods to supplement EHR data with external data have been used to capture missing information. These methods may include imputation if information (e.g. race, lab values) is collected on a subset of patients within the EHR. It is important to examine whether missingness occurs completely at random or at random (“ignorable”), or not at random (“non-ignorable”), using the data available to determine factors associated with missingness, which will also inform the best imputation strategy to pursue, if any [ 65 , 66 ]. As an example, suppose we are interested in ascertaining a patient's BMI from the EHR. If men were less likely to have BMI measured than women, the probability of missing data (BMI) depends on the observed data (gender) and may therefore be predictable and imputable. On the other hand, suppose underweight individuals were less likely to have BMI measured; the probability of missing data depends on its own value, and as such is non-predictable and may require a validation study to confirm. Alternatively to imputing missing data, surrogate measures may be used, such as inferring area-based SES indicators, including median household income, percent poverty, or area deprivation index, by zip code [ 67 , 68 ]. Lastly, validation studies utilizing external datasets may prove helpful, such as supplementing EHR data with claims data that may be available for a subset of patients (see Challenge #4 ).
As EHRs are increasingly being used for research, there are active pushes to include more structured data fields that are important to population health research, such as SDOH [ 69 ]. Inclusion of such factors are likely to result in improved patient care and outcomes, through increased precision in disease diagnosis, more effective shared decision making, identification of risk factors, and tailoring services to a given population’s needs [ 70 ]. In fact, a recent review found that when individual level SDOH were included in predictive modeling, they overwhelmingly improved performance in medication adherence, risk of hospitalization, 30-day rehospitalizations, suicide attempts, and other healthcare services [ 71 ]. Whether or not these fields will be utilized after their inclusion in the EHR may ultimately depend upon federal and state incentives, as well as support from local stakeholders, and this does not address historic, retrospective analyses of these data.
Challenge #4: Missing visits
Beyond missing variable data that may not be captured during a clinical encounter, either through structured data or clinical notes, there also may be missing information for a patient as a whole. This can occur in a variety of ways; for example, a patient may have one or two documented visits in the EHR and then is never seen again (i.e. right censoring due to lost to follow-up), or a patient is referred from elsewhere to seek specialty care, with no information captured regarding other external issues (i.e. left censoring). This may be especially common in circumstances where a given EHR is more likely to capture specialty clinics versus primary care (see Challenge #1 ). A third scenario may include patients who appear, then are not observed for a long period of time, and then reappear: this case is particularly problematic as it may appear the patient was never lost to follow up but simply had fewer visits. In any of these scenarios, a researcher will lack a holistic view of the patient’s experiences, diagnoses, results, and more. As discussed above, assuming absence of a diagnostic code as absence of disease may lead to information and/or selection bias. Further, it has been demonstrated that one key source of bias in EHRs is “informed presence” bias, where those with more medical encounters are more likely to be diagnosed with various conditions (similar to Berkson’s bias) [ 72 ].
Several solutions to these issues have been proposed. For example, it is common for EHR studies to condition on observation time (i.e. ≥n visits required to be eligible into cohort); however, this may exclude a substantial amount of patients with certain characteristics, incurring a selection bias or limiting the generalizability of study findings (see Challenge #1 ). Other strategies attempt to account for missing visit biases through longitudinal imputation approaches; for example, if a patient missed a visit, a disease activity score can be imputed for that point in time, given other data points [ 73 , 74 ]. Surrogate measures may also be used to infer patient outcomes, such as controlling for “informative” missingness as an indicator variable or using actual number of missed visits that were scheduled as a proxy for external circumstances influencing care [ 20 ]. To address “informed presence” bias described above, conditioning on the number of health-care encounters may be appropriate [ 72 ]. Understanding the reason for the missing visit may help identify the best course of action and before imputing, one should be able to identify the type of missingness, whether “informative” or not [ 65 , 66 ]. For example, if distance to a healthcare location is related to appointment attendance, being able to account for this in analysis would be important: researchers have shown how the catchment of a healthcare facility can induce selection bias [ 21 ]. Relatedly, as telehealth becomes more common fueled by the COVID-19 pandemic [ 75 , 76 ], virtual visits may generate missingness of data recorded in the presence of a provider (e.g., blood pressure if the patient does not have access to a sphygmomanometer; see Challenge #3 ), or necessitate a stratified analysis by visit type to assess for effect modification.
Another common approach is to supplement EHR information with external data sources, such as insurance claims data, when available. Unlike a given EHR, claims data are able to capture a patient’s interaction with the health care system across organizations, and additionally includes pharmacy data such as if a prescription was filled or refilled. Often researchers examine a subset of patients eligible for Medicaid/Medicare and compare what is documented in claims with information available in the EHR [ 77 ]. That is, are there additional medications, diagnoses, hospitalizations found in the claims dataset that were not present in the EHR. In a study by Franklin et al., researchers utilized a linked database of Medicare Advantage claims and comprehensive EHR data from a multi-specialty outpatient practice to determine which dataset would be more accurate in predicting medication adherence [ 77 ]. They found that both datasets were comparable in identifying those with poor adherence, though each dataset incorporated different variables.
While validation studies such as those using claims data allow researchers to gain an understanding as to how accurate and complete a given EHR is, this may only be limited to the specific subpopulation examined (i.e. those eligible for Medicaid, or those over 65 years for Medicare). One study examined congruence between EHR of a community health center and Medicaid claims with respect to diabetes [ 78 ]. They found that patients who were older, male, Spanish-speaking, above the federal poverty level, or who had discontinuous insurance were more likely to have services documented in the EHR as compared to Medicaid claims data. Therefore, while claims data may help supplement and validate information in the EHR, on their own they may underestimate care in certain populations.
Research utilizing EHR data has undoubtedly positively impacted the field of public health through its ability to provide large-scale, longitudinal data on a diverse set of patients, and will continue to do so in the future as more epidemiologists take advantage of this data source. EHR data’s ability to capture individuals that traditionally aren’t included in clinical trials, cohort studies, and even claims datasets allows researchers to measure longitudinal outcomes in patients and perhaps change the understanding of potential risk factors.
However, as outlined in this review, there are important caveats to EHR analysis that need to be taken into account; failure to do so may threaten study validity. The representativeness of EHR data depends on the catchment area of the center and corresponding target population. Tools are available to evaluate and remedy these issues, which are critical to study validity as well as extrapolation of study findings. Data availability and interpretation, missing measurements, and missing visits are also key challenges, as EHRs were not specifically developed for research purposes, despite their common use for such. Taking advantage of all available EHR data, whether it be structured or unstructured fields through NLP, will be important in understanding the patient experience and identifying key phenotypes. Beyond methods to address these concerns, it will remain crucial for epidemiologists and data analysts to engage with clinicians and informaticians at their institutions to ensure data quality and accessibility by forming multidisciplinary teams around specific research projects. Lastly, integration across multiple EHRs, or datasets that encompass multi-institutional EHR records, add an additional layer of data quality and validity issues, with the potential to exacerbate the above-stated challenges found within a single EHR. At minimum, such studies should account for correlated errors [ 79 , 80 ], and investigate whether modularization, or submechanisms that determine whether data are observed or missing in each EHR, exist [ 65 ].
The identified challenges may also apply to secondary analysis of other large healthcare databases, such as claims data, although it is important not to conflate the two types of data. EHR data are driven by clinical care and claims data are driven by the reimbursement process where there is a financial incentive to capture diagnoses, procedures, and medications [ 48 ]. The source of data likely influences the availability, accuracy, and completeness of data. The fundamental representation of data may also differ as a record in a claims database corresponds to a “claim” as opposed to an “encounter” in the EHR. As such, the representativeness of the database populations, the sensitivity and specificity of variables, as well as the mechanisms of missingness in claims data may differ from EHR data. One study that evaluated pediatric quality care measures, such as BMI, noted inferior sensitivity based on claims data alone [ 81 ]. Linking claims data to EHR data has been proposed to enhance study validity, but many of the caveats raised in herein still apply [ 82 ].
Although we focused on epidemiological challenges related to study validity, there are other important considerations for researchers working with EHR data. Privacy and security of data as well as institutional review board (IRB) or ethics board oversight of EHR-based studies should not be taken for granted. For researchers in the U.S., Goldstein and Sarwate described Health Insurance Portability and Accountability Act (HIPAA)-compliant approaches to ensure the privacy and security of EHR data used in epidemiological research, and presented emerging approaches to analyses that separate the data from analysis [ 83 ]. The IRB oversees the data collection process for EHR-based research and through the HIPAA Privacy Rule these data typically do not require informed consent provided they are retrospective and reside at the EHR’s institution [ 84 ]. Such research will also likely receive an exempt IRB review provided subjects are non-identifiable.
Conclusions
As EHRs are increasingly being used for research, epidemiologists can take advantage of the many tools and methods that already exist and apply them to the key challenges described above. By being aware of the limitations that the data present and proactively addressing them, EHR studies will be more robust, informative, and important to the understanding of health and disease in the population.
Availability of data and materials
All data and materials used in this review are described herein.
Abbreviations
Body Mass Index
Electronic Health Record
International Classification of Diseases
Institutional review board/ethics board
Health Insurance Portability and Accountability Act
Natural Language Processing
Social Determinants of Health
Socioeconomic Status
Adler-Milstein J, Holmgren AJ, Kralovec P, et al. Electronic health record adoption in US hospitals: the emergence of a digital “advanced use” divide. J Am Med Inform Assoc. 2017;24(6):1142–8.
Article PubMed PubMed Central Google Scholar
Office of the National Coordinator for Health Information Technology. ‘Office-based physician electronic health record adoption’, Health IT quick-stat #50. dashboard.healthit.gov/quickstats/pages/physician-ehr-adoption-trends.php . Accessed 15 Jan 2019.
Cowie MR, Blomster JI, Curtis LH, et al. Electronic health records to facilitate clinical research. Clin Res Cardiol. 2017;106(1):1–9.
Article PubMed Google Scholar
Casey JA, Schwartz BS, Stewart WF, et al. Using electronic health records for population health research: a review of methods and applications. Annu Rev Public Health. 2016;37:61–81.
Verheij RA, Curcin V, Delaney BC, et al. Possible sources of bias in primary care electronic health record data use and reuse. J Med Internet Res. 2018;20(5):e185.
Ni K, Chu H, Zeng L, et al. Barriers and facilitators to data quality of electronic health records used for clinical research in China: a qualitative study. BMJ Open. 2019;9(7):e029314.
Coleman N, Halas G, Peeler W, et al. From patient care to research: a validation study examining the factors contributing to data quality in a primary care electronic medical record database. BMC Fam Pract. 2015;16:11.
Kruse CS, Stein A, Thomas H, et al. The use of electronic health records to support population health: a systematic review of the literature. J Med Syst. 2018;42(11):214.
Shortreed SM, Cook AJ, Coley RY, et al. Challenges and opportunities for using big health care data to advance medical science and public health. Am J Epidemiol. 2019;188(5):851–61.
In: Smedley BD, Stith AY, Nelson AR, editors. Unequal treatment: confronting racial and ethnic disparities in health care. Washington (DC) 2003.
Chaudhry B, Wang J, Wu S, et al. Systematic review: impact of health information technology on quality, efficiency, and costs of medical care. Ann Intern Med. 2006;144(10):742–52.
Cutler DM, Scott Morton F. Hospitals, market share, and consolidation. JAMA. 2013;310(18):1964–70.
Article CAS PubMed Google Scholar
Cocoros NM, Kirby C, Zambarano B, et al. RiskScape: a data visualization and aggregation platform for public health surveillance using routine electronic health record data. Am J Public Health. 2021;111(2):269–76.
Vader DT, Weldie C, Welles SL, et al. Hospital-acquired Clostridioides difficile infection among patients at an urban safety-net hospital in Philadelphia: demographics, neighborhood deprivation, and the transferability of national statistics. Infect Control Hosp Epidemiol. 2020;42:1–7.
Google Scholar
Dixon BE, Gibson PJ, Frederickson Comer K, et al. Measuring population health using electronic health records: exploring biases and representativeness in a community health information exchange. Stud Health Technol Inform. 2015;216:1009.
PubMed Google Scholar
Hernán MA, VanderWeele TJ. Compound treatments and transportability of causal inference. Epidemiology. 2011;22(3):368–77.
Casey JA, Pollak J, Glymour MM, et al. Measures of SES for electronic health record-based research. Am J Prev Med. 2018;54(3):430–9.
Polubriaginof FCG, Ryan P, Salmasian H, et al. Challenges with quality of race and ethnicity data in observational databases. J Am Med Inform Assoc. 2019;26(8-9):730–6.
U.S. Census Bureau. Health. Available at: https://www.census.gov/topics/health.html . Accessed 19 Jan 2021.
Gianfrancesco MA, McCulloch CE, Trupin L, et al. Reweighting to address nonparticipation and missing data bias in a longitudinal electronic health record study. Ann Epidemiol. 2020;50:48–51 e2.
Goldstein ND, Kahal D, Testa K, Burstyn I. Inverse probability weighting for selection bias in a Delaware community health center electronic medical record study of community deprivation and hepatitis C prevalence. Ann Epidemiol. 2021;60:1–7.
Gelman A, Lax J, Phillips J, et al. Using multilevel regression and poststratification to estimate dynamic public opinion. Unpublished manuscript, Columbia University. 2016 Sep 11. Available at: http://www.stat.columbia.edu/~gelman/research/unpublished/MRT(1).pdf . Accessed 22 Jan 2021.
Quick H, Terloyeva D, Wu Y, et al. Trends in tract-level prevalence of obesity in philadelphia by race-ethnicity, space, and time. Epidemiology. 2020;31(1):15–21.
Lesko CR, Buchanan AL, Westreich D, Edwards JK, Hudgens MG, Cole SR. Generalizing study results: a potential outcomes perspective. Epidemiology. 2017;28(4):553–61.
Westreich D, Edwards JK, Lesko CR, Stuart E, Cole SR. Transportability of trial results using inverse odds of sampling weights. Am J Epidemiol. 2017;186(8):1010–4.
Congressional Research Services (CRS). The Health Information Technology for Economic and Clinical Health (HITECH) Act. 2009. Available at: https://crsreports.congress.gov/product/pdf/R/R40161/9 . Accessed Jan 22 2021.
Hersh WR. The electronic medical record: Promises and problems. Journal of the American Society for Information Science. 1995;46(10):772–6.
Article Google Scholar
Collecting sexual orientation and gender identity data in electronic health records: workshop summary. Washington (DC) 2013.
Committee on the Recommended Social and Behavioral Domains and Measures for Electronic Health Records; Board on Population Health and Public Health Practice; Institute of Medicine. Capturing social and behavioral domains and measures in electronic health records: phase 2. Washington (DC): National Academies Press (US); 2015.
Goff SL, Pekow PS, Markenson G, et al. Validity of using ICD-9-CM codes to identify selected categories of obstetric complications, procedures and co-morbidities. Paediatr Perinat Epidemiol. 2012;26(5):421–9.
Schneeweiss S, Avorn J. A review of uses of health care utilization databases for epidemiologic research on therapeutics. J Clin Epidemiol. 2005;58(4):323–37.
Gianfrancesco MA. Application of text mining methods to identify lupus nephritis from electronic health records. Lupus Science & Medicine. 2019;6:A142.
National Library of Medicine. SNOMED CT to ICD-10-CM Map. Available at: https://www.nlm.nih.gov/research/umls/mapping_projects/snomedct_to_icd10cm.html . Accessed 2 Jul 2021.
Klabunde CN, Harlan LC, Warren JL. Data sources for measuring comorbidity: a comparison of hospital records and medicare claims for cancer patients. Med Care. 2006;44(10):921–8.
Burles K, Innes G, Senior K, Lang E, McRae A. Limitations of pulmonary embolism ICD-10 codes in emergency department administrative data: let the buyer beware. BMC Med Res Methodol. 2017;17(1):89.
Asgari MM, Wu JJ, Gelfand JM, Salman C, Curtis JR, Harrold LR, et al. Validity of diagnostic codes and prevalence of psoriasis and psoriatic arthritis in a managed care population, 1996-2009. Pharmacoepidemiol Drug Saf. 2013;22(8):842–9.
Hoffman S, Podgurski A. Big bad data: law, public health, and biomedical databases. J Law Med Ethics. 2013;41(Suppl 1):56–60.
Adler-Milstein J, Jha AK. Electronic health records: the authors reply. Health Aff. 2014;33(10):1877.
Geruso M, Layton T. Upcoding: evidence from medicare on squishy risk adjustment. J Polit Econ. 2020;12(3):984–1026.
Lash TL, Fox MP, Fink AK. Applying quantitative bias analysis to epidemiologic data. New York: Springer-Verlag New York; 2009.
Book Google Scholar
Gustafson P. Measurement error and misclassification in statistics and epidemiology: impacts and Bayesian adjustments. Boca Raton: Chapman and Hall/CRC; 2004.
Duda SN, Shepherd BE, Gadd CS, et al. Measuring the quality of observational study data in an international HIV research network. PLoS One. 2012;7(4):e33908.
Article CAS PubMed PubMed Central Google Scholar
Shepherd BE, Yu C. Accounting for data errors discovered from an audit in multiple linear regression. Biometrics. 2011;67(3):1083–91.
Weiskopf NG, Hripcsak G, Swaminathan S, et al. Defining and measuring completeness of electronic health records for secondary use. J Biomed Inform. 2013;46(5):830–6.
Kaiser Health News. As coronavirus strikes, crucial data in electronic health records hard to harvest. Available at: https://khn.org/news/as-coronavirus-strikes-crucial-data-in-electronic-health-records-hard-to-harvest/ . Accessed 15 Jan 2021.
Reeves JJ, Hollandsworth HM, Torriani FJ, Taplitz R, Abeles S, Tai-Seale M, et al. Rapid response to COVID-19: health informatics support for outbreak management in an academic health system. J Am Med Inform Assoc. 2020;27(6):853–9.
Grange ES, Neil EJ, Stoffel M, Singh AP, Tseng E, Resco-Summers K, et al. Responding to COVID-19: The UW medicine information technology services experience. Appl Clin Inform. 2020;11(2):265–75.
Madigan D, Ryan PB, Schuemie M, et al. Evaluating the impact of database heterogeneity on observational study results. Am J Epidemiol. 2013;178(4):645–51.
Lippi G, Mattiuzzi C. Critical laboratory values communication: summary recommendations from available guidelines. Ann Transl Med. 2016;4(20):400.
Charlson ME, Pompei P, Ales KL, MacKenzie CR. A new method of classifying prognostic comorbidity in longitudinal studies: development and validation. J Chronic Dis. 1987;40(5):373–83.
Jones RN. Differential item functioning and its relevance to epidemiology. Curr Epidemiol Rep. 2019;6:174–83.
Edwards JK, Cole SR, Troester MA, Richardson DB. Accounting for misclassified outcomes in binary regression models using multiple imputation with internal validation data. Am J Epidemiol. 2013;177(9):904–12.
Satkunasivam R, Klaassen Z, Ravi B, Fok KH, Menser T, Kash B, et al. Relation between surgeon age and postoperative outcomes: a population-based cohort study. CMAJ. 2020;192(15):E385–92.
Melamed N, Asztalos E, Murphy K, Zaltz A, Redelmeier D, Shah BR, et al. Neurodevelopmental disorders among term infants exposed to antenatal corticosteroids during pregnancy: a population-based study. BMJ Open. 2019;9(9):e031197.
Kao LT, Lee HC, Lin HC, Tsai MC, Chung SD. Healthcare service utilization by patients with obstructive sleep apnea: a population-based study. PLoS One. 2015;10(9):e0137459.
Article PubMed PubMed Central CAS Google Scholar
Jung K, LePendu P, Iyer S, Bauer-Mehren A, Percha B, Shah NH. Functional evaluation of out-of-the-box text-mining tools for data-mining tasks. J Am Med Inform Assoc. 2015;22(1):121–31.
Canan C, Polinski JM, Alexander GC, et al. Automatable algorithms to identify nonmedical opioid use using electronic data: a systematic review. J Am Med Inform Assoc. 2017;24(6):1204–10.
Iqbal E, Mallah R, Jackson RG, et al. Identification of adverse drug events from free text electronic patient records and information in a large mental health case register. PLoS One. 2015;10(8):e0134208.
Rochefort CM, Verma AD, Eguale T, et al. A novel method of adverse event detection can accurately identify venous thromboembolisms (VTEs) from narrative electronic health record data. J Am Med Inform Assoc. 2015;22(1):155–65.
Koleck TA, Dreisbach C, Bourne PE, et al. Natural language processing of symptoms documented in free-text narratives of electronic health records: a systematic review. J Am Med Inform Assoc. 2019;26(4):364–79.
Wang L, Luo L, Wang Y, et al. Natural language processing for populating lung cancer clinical research data. BMC Med Inform Decis Mak. 2019;19(Suppl 5):239.
Banerji A, Lai KH, Li Y, et al. Natural language processing combined with ICD-9-CM codes as a novel method to study the epidemiology of allergic drug reactions. J Allergy Clin Immunol Pract. 2020;8(3):1032–1038.e1.
Zhang D, Yin C, Zeng J, et al. Combining structured and unstructured data for predictive models: a deep learning approach. BMC Med Inform Decis Mak. 2020;20(1):280.
Farmer R, Mathur R, Bhaskaran K, Eastwood SV, Chaturvedi N, Smeeth L. Promises and pitfalls of electronic health record analysis. Diabetologia. 2018;61:1241–8.
Haneuse S, Arterburn D, Daniels MJ. Assessing missing data assumptions in EHR-based studies: a complex and underappreciated task. JAMA Netw Open. 2021;4(2):e210184.
Groenwold RHH. Informative missingness in electronic health record systems: the curse of knowing. Diagn Progn Res. 2020;4:8.
Berkowitz SA, Traore CY, Singer DE, et al. Evaluating area-based socioeconomic status indicators for monitoring disparities within health care systems: results from a primary care network. Health Serv Res. 2015;50(2):398–417.
Kind AJH, Buckingham WR. Making neighborhood-disadvantage metrics accessible - the neighborhood atlas. N Engl J Med. 2018;378(26):2456–8.
Cantor MN, Thorpe L. Integrating data on social determinants of health into electronic health records. Health Aff. 2018;37(4):585–90.
Adler NE, Stead WW. Patients in context--EHR capture of social and behavioral determinants of health. N Engl J Med. 2015;372(8):698–701.
Chen M, Tan X, Padman R. Social determinants of health in electronic health records and their impact on analysis and risk prediction: a systematic review. J Am Med Inform Assoc. 2020;27(11):1764–73.
Goldstein BA, Bhavsar NA, Phelan M, et al. Controlling for informed presence bias due to the number of health encounters in an electronic health record. Am J Epidemiol. 2016;184(11):847–55.
Petersen I, Welch CA, Nazareth I, et al. Health indicator recording in UK primary care electronic health records: key implications for handling missing data. Clin Epidemiol. 2019;11:157–67.
Li R, Chen Y, Moore JH. Integration of genetic and clinical information to improve imputation of data missing from electronic health records. J Am Med Inform Assoc. 2019;26(10):1056–63.
Koonin LM, Hoots B, Tsang CA, Leroy Z, Farris K, Jolly T, et al. Trends in the use of telehealth during the emergence of the COVID-19 pandemic - United States, January-March 2020. MMWR Morb Mortal Wkly Rep. 2020;69(43):1595–9.
Barnett ML, Ray KN, Souza J, Mehrotra A. Trends in telemedicine use in a large commercially insured population, 2005-2017. JAMA. 2018;320(20):2147–9.
Franklin JM, Gopalakrishnan C, Krumme AA, et al. The relative benefits of claims and electronic health record data for predicting medication adherence trajectory. Am Heart J. 2018;197:153–62.
Devoe JE, Gold R, McIntire P, et al. Electronic health records vs Medicaid claims: completeness of diabetes preventive care data in community health centers. Ann Fam Med. 2011;9(4):351–8.
Schmajuk G, Li J, Evans M, Anastasiou C, Izadi Z, Kay JL, et al. RISE registry reveals potential gaps in medication safety for new users of biologics and targeted synthetic DMARDs. Semin Arthritis Rheum. 2020 Dec;50(6):1542–8.
Izadi Z, Schmajuk G, Gianfrancesco M, Subash M, Evans M, Trupin L, et al. Rheumatology Informatics System for Effectiveness (RISE) practices see significant gains in rheumatoid arthritis quality measures. Arthritis Care Res. 2020. https://doi.org/10.1002/acr.24444 .
Angier H, Gold R, Gallia C, Casciato A, Tillotson CJ, Marino M, et al. Variation in outcomes of quality measurement by data source. Pediatrics. 2014;133(6):e1676–82.
Lin KJ, Schneeweiss S. Considerations for the analysis of longitudinal electronic health records linked to claims data to study the effectiveness and safety of drugs. Clin Pharmacol Ther. 2016;100(2):147–59.
Goldstein ND, Sarwate AD. Privacy, security, and the public health researcher in the era of electronic health record research. Online J Public Health Inform. 2016;8(3):e207.
U.S. Department of Health and Human Services (HHS). 45 CFR 46. http://www.hhs.gov/ohrp/regulations-and-policy/regulations/45-cfr-46/index.html .
Download references
Acknowledgements
The authors thank Dr. Annemarie Hirsch, Department of Population Health Sciences, Geisinger, for assistance in conceptualizing an earlier version of this work.
Research reported in this publication was supported in part by the National Institute of Arthritis and Musculoskeletal and Skin Diseases of the National Institutes of Health under Award Number K01AR075085 (to MAG) and the National Institute Of Allergy And Infectious Diseases of the National Institutes of Health under Award Number K01AI143356 (to NDG). The content is solely the responsibility of the authors and does not necessarily represent the official views of the National Institutes of Health.
Author information
Authors and affiliations.
Division of Rheumatology, University of California School of Medicine, San Francisco, CA, USA
Milena A. Gianfrancesco
Department of Epidemiology and Biostatistics, Drexel University Dornsife School of Public Health, 3215 Market St., Philadelphia, PA, 19104, USA
Neal D. Goldstein
You can also search for this author in PubMed Google Scholar
Contributions
Both authors conceptualized, wrote, and approved the final submitted version.
Corresponding author
Correspondence to Neal D. Goldstein .
Ethics declarations
Ethics approval and consent to participate.
Not applicable
Consent for publication
Competing interests.
The authors have no competing interests to declare
Additional information
Publisher’s note.
Springer Nature remains neutral with regard to jurisdictional claims in published maps and institutional affiliations.
Rights and permissions
Open Access This article is licensed under a Creative Commons Attribution 4.0 International License, which permits use, sharing, adaptation, distribution and reproduction in any medium or format, as long as you give appropriate credit to the original author(s) and the source, provide a link to the Creative Commons licence, and indicate if changes were made. The images or other third party material in this article are included in the article's Creative Commons licence, unless indicated otherwise in a credit line to the material. If material is not included in the article's Creative Commons licence and your intended use is not permitted by statutory regulation or exceeds the permitted use, you will need to obtain permission directly from the copyright holder. To view a copy of this licence, visit http://creativecommons.org/licenses/by/4.0/ . The Creative Commons Public Domain Dedication waiver ( http://creativecommons.org/publicdomain/zero/1.0/ ) applies to the data made available in this article, unless otherwise stated in a credit line to the data.
Reprints and permissions
About this article
Cite this article.
Gianfrancesco, M.A., Goldstein, N.D. A narrative review on the validity of electronic health record-based research in epidemiology. BMC Med Res Methodol 21 , 234 (2021). https://doi.org/10.1186/s12874-021-01416-5
Download citation
Received : 02 July 2021
Accepted : 28 September 2021
Published : 27 October 2021
DOI : https://doi.org/10.1186/s12874-021-01416-5
Share this article
Anyone you share the following link with will be able to read this content:
Sorry, a shareable link is not currently available for this article.
Provided by the Springer Nature SharedIt content-sharing initiative
- Electronic health records
- Data quality
- Secondary analysis
BMC Medical Research Methodology
ISSN: 1471-2288
- General enquiries: [email protected]
Building Electronic Health Record Databases for Research
- Open Access
- First Online: 01 August 2020
Cite this chapter
You have full access to this open access chapter
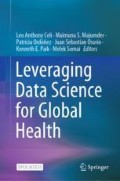
- Lucas Bulgarelli 7 ,
- Antonio Núñez-Reiz 8 &
- Rodrigo Octavio Deliberato 7
18k Accesses
This chapter presents information about the development and use of Electronic Health Record (EHR) Databases. There are petabytes of untapped research data hoarded within hospitals worldwide. There is enormous potential in the secondary analysis of this clinical data, leveraging data already collected in everyday medical practice, we could gain insight into the clinical decision-making process and it’s impact on patient outcomes. In this chapter we outline a high-level overview of some of the important considerations when building clinical research databases.
- Electronic health records
- Disease repositories
- Data mapping
- Data pipeline
- Deidentification
Download chapter PDF
Similar content being viewed by others
Patient Registries for Clinical Research
Biases in Electronic Health Records Data for Generating Real-World Evidence: An Overview
Ban Al-Sahab, Alan Leviton, … Bo Zhang
The main objectives include:
Understand the differences between traditional disease information repositories and EHR databases, and why they are useful
Review examples of current EHR clinical databases
Learn the necessary steps to develop an EHR clinical database
1 Background
1.1 introduction to clinical databases.
Health care information has traditionally been presented in “disease repositories”―a listing of manually collected disease specific information, often stored as aggregate registries. More recently, clinical databases have been developed, resulting in new ways to present, understand, and use health care data. Databases are defined as sets of routinely collected information, organized so it can be easily accessed, manipulated, and updated. Different from disease repositories, these new clinical databases are characterized by heterogeneous patient-level data, automatically gathered from the EHRs. They include many high-resolution variables originating from a large number of patients, thus allowing researchers to study both clinical interactions and decisions for a wide range of disease processes.
Two important phenomena accelerated the evolution of traditional disease repositories into new clinical databases. The first one is the global adoption of EHRs, in which paper-based systems are transformed into digital ones. Although the primary purpose of EHRs is not data collection, their implementation allows health systems to automatically gather large amounts of data (Bailly et al. 2018 ). Recognizing the enormous potential of the secondary analysis of these data for initiatives from quality improvement to treatment personalization, health and research institutions have started to leverage these novel clinical databases. The second phenomenon that supported the development of clinical databases is the extraordinary expansion of computational power that allowed the development of the necessary infrastructure to store vast amounts of diverse data, and the capacity to process it in a reasonable timeframe. These events enabled the emergence of the field of data science and machine learning. This new knowledge has been made accessible to a large and global audience through new massive open online courses (MOOCs), spurring substantial interest in analysis of large amounts of health data and the opportunity to crowdsource new machine learning techniques through available open source programming tools. (Sanchez-Pinto et al. 2018 ).
1.2 Goals for Database Creation
The main goal of creating a healthcare database is to put clinical information in a format that can be intuitively explored and rapidly processed, allowing researchers to extract valuable knowledge from the data. In a traditional database, there are relational structures built into store the data which guarantee consistency of the relationships between its entities (e.g. between patient and hospital visit). These structures are commonly referred to as “data models”, and consist of the definition of tables, fields, and requirements for that database. When developing such models, it is essential to capture meaningful representation of the concepts and processes we want to study. This can be a challenge in health care because there are many different actors, and faithfully representing their relationships is crucial to understand what is occurring and also to achieve relevant and reliable research conclusions. Another critical step when creating and maintaining a clinical database is incorporating data quality and security, so it can be appropriately and reliably used in secondary data analysis.
1.3 Examples of Clinical Databases Worldwide
1.3.1 m edical i nformation m art for i ntensive c are (mimic).
The M edical I nformation M art for I ntensive C are (MIMIC) (Johnson et al. 2016 ) is one of the most popular and widely used open access clinical databases worldwide. Launched in 2003, MIMIC originated from a partnership between the Massachusetts Institute of Technology (MIT) Laboratory for Computational Physiology, Philips Medical Systems, and Beth Israel Deaconess Medical Center, with funding from the National Institute of Biomedical Imaging and Bioengineering. It is currently in its third version and has de-identified data from 40,000 medical and surgical patients admitted to the Beth Israel Deaconess Medical Center (BIDMC). Originally created with the aim of leveraging machine learning in the healthcare setting to build advanced ICU patient monitoring and decision support systems, MIMIC’s main goal is to improve the efficiency, accuracy, and timeliness of clinical decision-making for ICU patients.
MIMIC has been used for many clinical studies from independent researchers (Aboelsoud et al. 2018 ; Johnson et al. 2018 ; Komorowski et al. 2018 ; Sandfort et al. 2018 ; Serpa Neto et al. 2018 ; Waudby-Smith et al. 2018 ; Block et al. 2018 ; Collins et al. 2014 ; Computing NCfB 2018 ; Deliberato et al. 2018 ; Dernoncourt et al. 2017 ; Desautels et al. 2016 ; Desautels et al. 2017 ; Farhan et al. 2016 ; Feng et al. 2018 ; Fleurence et al. 2014 ; Ghassemi et al. 2014 ; Johnson et al. 2016 ). Since its first version, MIMIC allowed researchers to freely access the data, after registering, completing a preliminary course on human research, and abiding by a data use agreement to avoid the potential misuse of clinical data. This has been one of the main reasons for its popularity in the clinical research community, along with the enormous quantity of diverse information for all patients in MIMIC, making complex cross-evaluating studies feasible. Another important feature for researchers is that individual patient consent has been waived by BIDMC’s Institutional Review Board, an essential and challenging prerequisite to allow for a clinical database to go public in the real world.
In addition to clinical data extracted from the EHR such as demographics, diagnoses, lab values, vital signs, events, and medications, there is a subset of patients with bedside monitor waveforms from ECG, EEG, and vital sign tracings that are stored in flat binary files with text header descriptors. MIMIC also maintains documentation of data structure and a public GitHub repository for researchers interested in working with the database. As result, new users can benefit from the work of others by accessing the available code, and are encouraged to contribute their own work, thereby strengthening and furthering the impact of MIMIC.
1.3.2 eICU C ollaborative R esearch D atabase (eICU-CRD)
Another example of an open-access database is the eICU Collaborative Research Database (eICU-CRD) (Pollard et al. 2018 ). This project is derived from a critical care telehealth initiative by Philips® Healthcare. The eICU-CRD was made freely available by the same team as MIMIC and features a distinct patient pool originating from 208 ICUs across the U.S. from 2014 to 2015. As a result, MIMIC and eICU-CRD are independent yet complementary. Similar to MIMIC, the main objective of the project is to boost collaboration in secondary analysis of electronic health records, through the creation of openly available repositories.
1.3.3 Other Databases for Collaborative Research
There are other clinical databases that can be used for collaborative research, although access is more restricted, and data tend to be more general and less granular than the clinical information available in MIMIC or eICU-CRD. One example is PCORnet (Collins et al. 2014 ), a patient-centered clinical research project that aims to build a national research network, linked by a common data platform and embedded in clinical care delivery systems (Collins et al. 2014 ; Fleurence et al. 2014 ). This network aims to provide enough data for studies of rare or uncommon clinical entities, that have been difficult to conduct with the “classical” model. Medical record collections from over 60 million patients allow for large-scale observational and interventional trials to be accomplished more easily (Block et al. 2018 ). Access to the data can be requested through their web platform “Front Door” and is granted with a case-by-case policy depending on the project.
Other initiatives aim to create common data models, enabling the construction of multiple databases using a common ontology, so that data from each source means the same thing. The Observational Medical Outcomes Partnership (OMOP) and i2b2 have been established using this concept and aim to translate healthcare concepts to a common language in order to facilitate the sharing of meaningful data across the compatible databases. OMOP is managed by a network called Observational Health Data Science and Informatics (OHDSI), a multi-stakeholder, interdisciplinary collaborative network that spans over 600 million patients. A list of databases ported to their model can be found at their website (Observational Health Data Sciences and Informatics (OHDSI) 2018 ; OMOP CDM 2018 ). The i2b2 tranSMART Foundation (Computing NCfB. i2b2 (Informatics for Integrating Biology and the Bedside) 2018 ) is a member-driven non-profit foundation with an open-source/open-data strategy. It provides an open-source data model similar to OMOP, and a list of databases can be found at their website (Computing NCfB 2018 ). Both OMOP and i2b2 have open source software tools to manage and access the data, and a very active community of users with forums where relevant information and implementation tips can be found.
2 Exercise: Steps for Building an EHR Database for Research
2.1 putting together the right team.
One of the most important steps at the start of any successful project is putting together the right team. Bringing together the range of skilled professionals with the required skills is essential when building an EHR database. One key role is that of a clinician with the knowledge to understand and decipher the highly specialized data collected in the EHR, especially because these data are often poorly organized within the EHR. Clinicians also have an important role in assessing the accuracy of the resulting database and working with data scientists to optimize its usability for targeted end-users.
Another critical member for the team is someone with substantial knowledge in data architecture, who can ensure consistency while modeling the highly complex data from EHRs. This person needs to work closely with the clinicians and data scientists to achieve a high quality, functional clinical database.
2.2 The Six Steps to Building an EHR Database
Once the multidisciplinary team has been formed, the next step is familiarizing everyone with the steps for building the database. This is important because the process of building a clinical database is iterative and continuous, as developers work to guarantee data quality and security. The six main stages for developing a clinical database are summarized in Fig. 4.1 .
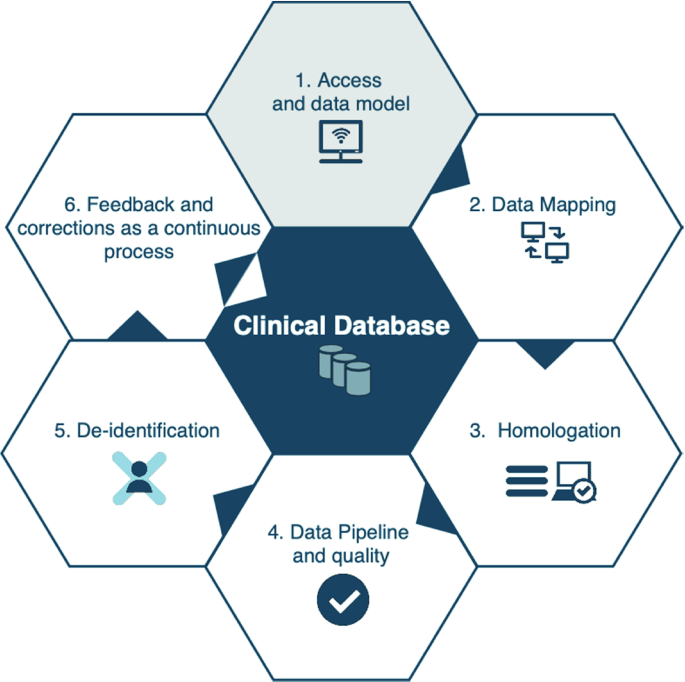
Main stages for the process of clinical database development
2.2.1 Step 1: Access and Data Model
At the start of the project, it can be helpful to acquire some resources to help with some of the laborious tasks that are inherent in the process of building clinical databases. For instance, if the clinicians building the database work at the hospital where the data is generated, obtaining access to a real time copy of the EHR source database (Step 1, Fig. 4.1 ) can facilitate mapping. In this scenario, clinicians can use one of their current patients to search for the information that the data architects are trying to map in the EHR system. This helps locate the data to be mapped in the source database. It also helps validate the mapping, by comparing the current reported values after the information is found. This resource is extremely valuable in the assessment of data consistency, since most of the data found in these source databases are used only for maintenance of system functionalities and have no clinical value, thus confusing the mapping process. Although obtaining real time copy of databases may be useful, it can be difficult to do in resource limited settings. In such cases, looking for other ways using the available computational infrastructure in order to acquire the data in a faster time frame is recommended, as any highly available data is valuable in building the database and creating a data-driven environment.
In addition to working with a copy of the EHR source database, the database development team needs to combine their skills in data architecture with their knowledge about the targeted uses of the database in order to find a data model that would fit all the stakeholders’ requirements (Step 1, Fig. 4.1 ). Balancing these needs is difficult, but critically important at this stage. While modeling all data to fit clinical or analytical mindsets might be desired, creating a model using high granularity and resolution data causes some limitations. Additionally, if conducting multicenter studies is one of the goals, the adoption of open-source health data models, or converging to a data model that can be used by prospective partners might be taken into consideration, as the use of common data models not only facilitates those studies, but also improves their reliability. It is important to emphasize that there is no ideal model and it is highly recommended to choose a common data model most likely to become part of the initiatives already in place in your institution, having an active voice in the process, and helping the community to decide the future direction of the model.
2.2.2 Data Mapping
With access to the EHR source database acquired and a data model determined, mapping the data will be main activity of both data architects and clinicians (Step 2, Fig. 4.1 ). This step is the longest in the process, so obtaining documentation from the source database will prove helpful and can shorten the time needed. Data architects will need to dive into the specifics of the source database, and work on the Extracting, Transform and Load (ETL) process, and fitting the data in the chosen data model. The clinicians’ role in this stage is to help the data architects in finding the information in the source database, by browsing through the EHR system and identifying where data is stored. The clinicians will also need to validate the data whenever new information is added to the ETL, verifying if the information being stored corresponds with their actual clinical meaning, making each iteration of the data mapping more reliable and consistent. If the clinicians do not work in the source hospital, their expertise will be used to validate the iterations based on whether the given value for each variable is reasonable for its type.
2.2.3 Homologation
If the validation steps during the iterations of data mapping were performed well, the next step, homologation (Step 3, Fig. 4.1 ), will be short and only require small adjustments to the mapping. Homologation consists of checking to make sure all the mapped data are correct, and have not been corrupted during the ETL process, as a result of improper deletion or modification of information, inclusion of irrelevant and confounding data, and inaccurate verification of correct clinical meaning. During this process, some of the clinicians’ current patients are randomly chosen and information from their latest stay is verified by comparing the information in their medical record to the mapped data. If real time access to the EHR source database was not obtained, this process can be more time consuming as the information from the randomly chosen patients needs to be adapted to the current conditions. If the clinicians on the database development team do not have access to the EHR system, they must homologate the records using their expert knowledge, as they did when validating the data mapping. It is very important that the database development team be thorough during the homologation process, as every piece of mapped information must be checked in order to guarantee the consistency of the data.
2.2.4 Data Pipeline and Quality
After completing the homologation process, the prototype of the database is essentially completed. The next step in the process is to establish the automatic input of the mapped data into a repository, by using a pipeline that assesses data quality (Step 4, Fig. 4.1 ). The pipeline is made up of sequentially executed computer tasks, scheduled and ordered according to desired intervals and availability of the source database, i.e. real-time or daily, in order to maintain the consistency of the data and the relationships between them. The last and most important task before the final incorporation of the data into the repository must be checking data quality, for example looking for values that differ significantly from current historical data, thereby preventing the inclusion of possibly corrupted data in studies utilizing the database.
2.2.5 De-identification
With the completion of the data pipeline, a usable database is in place. In order to have a clinical database that can be used by other researchers and applications, most institutions and governments require further development to comply with privacy policies and regulations. Additional steps, commonly referred to as de-identification, need to be included in the pipeline (Step 5, Fig. 4.1 ), in order to produce a database which complies with these requirements. For structured data, i.e. columns of a database, these methods rely on categorizing information, and then deleting or cryptographing the ones flagged as protected. For unstructured data, such as clinicians’ notes, various methods of natural language processing are used, from simple regular expressions, that are pattern matching sequences, to sophisticated neural networks, ultimately trying to identify all protected information throughout the free text for deletion or cryptography (Neamatullah et al. 2008 ; Dernoncourt et al. 2017 ). These methods have been included in software and services (Amazon Comprehend Medical 2018 ) to assist healthcare institutions to comply with patient privacy policies.
2.2.6 Feedback and Correction to Continually Improve and Maintain the Database
After completing the first version of the database, the process is not over. It is essential to understand that constructing a database is a continuous process, relying on continual user feedback to improve and maintain the integrity of the information. The users will have important insights on what can be improved in future versions. The data architect who will be responsible for the maintenance of the database must continually monitor the source database and the pipeline for any possible data corruption. Additional data quality assessments with expert knowledge from clinicians are recommended, who can provide ongoing input regarding whether the data is being properly populated in the database. This can help detect problems in the EHR system, source database or inform directives on how clinicians input information in the EHR system.
Aboelsoud, M., Siddique, O., Morales, A., Seol, Y., & Al-Qadi, M. (2018). Early biliary drainage is associated with favourable outcomes in critically-ill patients with acute cholangitis. Przeglad Gastroenterologiczny, 13 (1), 16–21.
PubMed PubMed Central Google Scholar
Amazon Comprehend Medical. Retrieved from December 2018, from https://aws.amazon.com/comprehend/medical/ .
Bailly, S., Meyfroidt, G., & Timsit, J. F. (2018). What’s new ICU in 2050: Big data and machine learning. Intensive Care Medicine, 44, 1524–1527.
Article Google Scholar
Block, J. P., Bailey, L. C., Gillman, M. W., Lunsford, D., Boone-Heinonen, J., Cleveland, L. P., et al. (2018). PCORnet antibiotics and childhood growth study: Process for cohort creation and cohort description. Academic Pediatric, 18 (5), 569–576.
Collins, F. S., Hudson, K. L., Briggs, J. P., & Lauer, M. S. (2014). PCORnet: Turning a dream into reality. Journal of the American Medical Informatics Association, 21 (4), 576–577.
Computing NCfB. (2018). i2b2 (Informatics for Integrating Biology and the Bedside). Retrieved October 2018, from https://www.i2b2.org .
Deliberato, R. O., Ko, S., Komorowski, M., de La Hoz Armengol, M. A., Frushicheva, M.P., & Raffa, J., et al. (2018, March). Severity of illness may misclassify critically ill obese patients. Crit Care, 46 (3), 394–400.
Google Scholar
Dernoncourt, F., Lee, J. Y., Uzuner, O., & Szolovitz, P. (2017). De-identification of patients notes with recurrent neural networks. Journal of the American Medical Informatics Association, 24 (3), 596–606.
PubMed Google Scholar
Desautels, T., Calvert, J., Hoffman, J., Jay, M., Kerem, Y., Shieh, L., et al. (2016). Prediction of sepsis in the intensive care unit with minimal electronic health record data: A machine learning approach. JMIR Med Inform., 4 (3), e28.
Desautels, T., Das, R., Calvert, J., Trivedi, M., Summers, C., Wales, D. J., et al. (2017). Prediction of early unplanned intensive care unit readmission in a UK tertiary care hospital: A cross-sectional machine learning approach. British Medical Journal Open, 7 (9), e017199.
Farhan, W., Wang, Z., Huang, Y., Wang, S., Wang, F., & Jiang, X. (2016). A predictive model for medical events based on contextual embedding of temporal sequences. JMIR Medical Informatics, 4 (4), e39.
Feng, M., McSparron, J. I., Kien, D. T., Stone, D. J., Roberts, D. H., Schwartzstein, R. M., et al. (2018). Transthoracic echocardiography and mortality in sepsis: Analysis of the MIMIC-III database. Intensive Care Medicine, 44 (6), 884–892.
Article CAS Google Scholar
Fleurence, R. L., Curtis, L. H., Califf, R. M., Platt, R., Selby, J. V., & Brown, J. S. (2014). Launching PCORnet, a national patient-centered clinical research network. Journal of the American Medical Informatics Association, 21 (4), 578–582.
Ghassemi, M., Marshall, J., Singh, N., Stone, D. J., & Celi, L. A. (2014). Leveraging a critical care database: Selective serotonin reuptake inhibitor use prior to ICU admission is associated with increased hospital mortality. Chest, 145 (4), 745–752.
Johnson, A. E., Pollard, T. J., Shen, L., Lehman, L. W., Feng, M., Ghassemi, M., et al. (2016). MIMIC-III, a freely accessible critical care database. Scientific Data, 3, 160035.
Johnson, A. E. W., Aboab, J., Raffa, J., Pollard, T. J., Deliberato, R. O., Celi, L. A., et al. (2018). A comparative analysis of sepsis identification methods in a electronic database. Critical Care, 46 (4), 494–499.
Komorowski, M., Celi, L. A., Badawi, O., Gordon, A. C., Faisal, A. A. (2018, October 22). The artificial intelligence clinician learns optimal treatment strategies for sepsis in intensive care. Nat Med. [Epub ahead of print].
List of databases which have been converted to OMOP CDM. (2018). Retrieved October 2018, from http://www.ohdsi.org/web/wiki/doku.php?id=resources:2018_data_network .
Neamatullah, I., Douglas, M. M., Lehman, L. W., Reisner, A., Villarroel, M., Long, W. J., et al. (2008). Automated de-identification of free text medical records. BMC Medical Informatics and Decision Making, 24 (8), 32.
Observational Health Data Sciences and Informatics (OHDSI) OMOP Common Data Model V5.0. Retrieved October 2018, from https://www.ohdsi.org .
Pollard, T. J., Johnson, A. E. W., Raffa, J. D., Celi, L. A., Mark, R. G., & Badawi, O. (2018). The eICU collaborative research database, a freely available multi-center database for critical care research. Scientific Data, 5, 180178.
Sanchez-Pinto, L. N., Luo, Y., Churpek, M. M. (2018, May 9). Big data and data science in critical care. Chest pii: S0012-3692(18)30725-6 [Epub ahead of print].
Sandfort,V., Johnson, A. E. W., Kunz, L. M., Vargas, J. D., Rosing, D. R. (2018). Prolonged elevated heart rate and 90-day survival in acutely Ill patients: Data from the MIMIC-III database. Journal of Intensive Care Medicine, 885066618756828.
Serpa Neto, A, Deliberato, R. O., Johnson, A. E. W., Bos, L. D., Amorim, P., Pereira, S. M., et al. (2018, October 5). Mechanical power of ventilation is associated with mortality in critically ill patients: an analysis of patients in two observational cohorts. Intensive Care Medicine [Epub ahead of print].
Waudby-Smith, I. E. R., Tran, N., Dubin, J. A., & Lee, J. (2018). Sentiment in nursing notes as an indicator of out-of-hospital mortality in intensive care patients. PLoS ONE, 13 (6), e0198687.
Download references
Author information
Authors and affiliations.
Laboratory for Computational Physiology, Massachusetts Institute of Technology, CA, USA
Lucas Bulgarelli & Rodrigo Octavio Deliberato
Servicio de Medicina Intensiva, Hospital Universitario Clínico San Carlos, Madrid, Spain
Antonio Núñez-Reiz
You can also search for this author in PubMed Google Scholar
Corresponding author
Correspondence to Rodrigo Octavio Deliberato .
Editor information
Editors and affiliations.
Massachusetts Institute of Technology, Cambridge, MA, USA
Leo Anthony Celi
Boston Children’s Hospital, Harvard Medical School, Boston, MA, USA
Maimuna S. Majumder
University of Puerto Rico Río Piedras, San Juan, PR, USA
Patricia Ordóñez
ScienteLab, Department of Global Health, University of Washington, Seattle, USA
Juan Sebastian Osorio
Institute for Medical Engineering and Science, Massachusetts Institute of Technology, Cambridge, MA, USA
Kenneth E. Paik
Imperial College London, London, UK
Melek Somai
Rights and permissions
Open Access This chapter is licensed under the terms of the Creative Commons Attribution 4.0 International License ( http://creativecommons.org/licenses/by/4.0/ ), which permits use, sharing, adaptation, distribution and reproduction in any medium or format, as long as you give appropriate credit to the original author(s) and the source, provide a link to the Creative Commons license and indicate if changes were made.
The images or other third party material in this chapter are included in the chapter's Creative Commons license, unless indicated otherwise in a credit line to the material. If material is not included in the chapter's Creative Commons license and your intended use is not permitted by statutory regulation or exceeds the permitted use, you will need to obtain permission directly from the copyright holder.
Reprints and permissions
Copyright information
© 2020 The Author(s)
About this chapter
Bulgarelli, L., Núñez-Reiz, A., Deliberato, R.O. (2020). Building Electronic Health Record Databases for Research. In: Celi, L., Majumder, M., Ordóñez, P., Osorio, J., Paik, K., Somai, M. (eds) Leveraging Data Science for Global Health. Springer, Cham. https://doi.org/10.1007/978-3-030-47994-7_4
Download citation
DOI : https://doi.org/10.1007/978-3-030-47994-7_4
Published : 01 August 2020
Publisher Name : Springer, Cham
Print ISBN : 978-3-030-47993-0
Online ISBN : 978-3-030-47994-7
eBook Packages : Computer Science Computer Science (R0)
Share this chapter
Anyone you share the following link with will be able to read this content:
Sorry, a shareable link is not currently available for this article.
Provided by the Springer Nature SharedIt content-sharing initiative
- Publish with us
Policies and ethics
- Find a journal
- Track your research
Suggestions or feedback?
MIT News | Massachusetts Institute of Technology
- Machine learning
- Social justice
- Black holes
- Classes and programs
Departments
- Aeronautics and Astronautics
- Brain and Cognitive Sciences
- Architecture
- Political Science
- Mechanical Engineering
Centers, Labs, & Programs
- Abdul Latif Jameel Poverty Action Lab (J-PAL)
- Picower Institute for Learning and Memory
- Lincoln Laboratory
- School of Architecture + Planning
- School of Engineering
- School of Humanities, Arts, and Social Sciences
- Sloan School of Management
- School of Science
- MIT Schwarzman College of Computing
Toward a smarter electronic health record
Press contact :, media download.
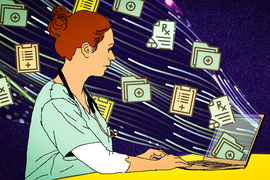
*Terms of Use:
Images for download on the MIT News office website are made available to non-commercial entities, press and the general public under a Creative Commons Attribution Non-Commercial No Derivatives license . You may not alter the images provided, other than to crop them to size. A credit line must be used when reproducing images; if one is not provided below, credit the images to "MIT."
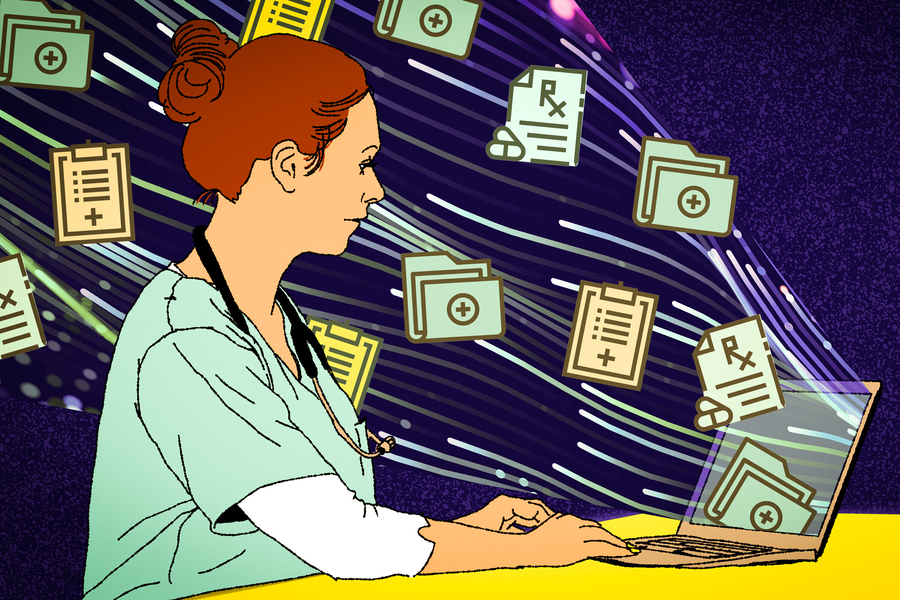
Previous image Next image
Electronic health records have been widely adopted with the hope they would save time and improve the quality of patient care. But due to fragmented interfaces and tedious data entry procedures, physicians often spend more time navigating these systems than they do interacting with patients.
Researchers at MIT and the Beth Israel Deaconess Medical Center are combining machine learning and human-computer interaction to create a better electronic health record (EHR). They developed MedKnowts, a system that unifies the processes of looking up medical records and documenting patient information into a single, interactive interface.
Driven by artificial intelligence, this “smart” EHR automatically displays customized, patient-specific medical records when a clinician needs them. MedKnowts also provides autocomplete for clinical terms and auto-populates fields with patient information to help doctors work more efficiently.
“In the origins of EHRs, there was this tremendous enthusiasm that getting all this information organized would be helpful to be able to track billing records, report statistics to the government, and provide data for scientific research. But few stopped to ask the deep questions around whether they would be of use for the clinician. I think a lot of clinicians feel they have had this burden of EHRs put on them for the benefit of bureaucracies and scientists and accountants. We came into this project asking how EHRs might actually benefit clinicians,” says David Karger, professor of computer science in the Computer Science and Artificial Intelligence Laboratory (CSAIL) and senior author of the paper.
The research was co-authored by CSAIL graduate students Luke Murray, who is the lead author, Divya Gopinath, and Monica Agrawal. Other authors include Steven Horng, an emergency medicine attending physician and clinical lead for machine learning at the Center for Healthcare Delivery Science of Beth Israel Deaconess Medical Center, and David Sontag, associate professor of electrical engineering and computer science at MIT and a member of CSAIL and the Institute for Medical Engineering and Science, and a principal investigator at the Abdul Latif Jameel Clinic for Machine Learning in Health. It will be presented at the Association for Computing Machinery Symposium on User Interface Software and Technology next month.
A problem-oriented tool
To design an EHR that would benefit doctors, the researchers had to think like doctors.
They created a note-taking editor with a side panel that displays relevant information from the patient’s medical history. That historical information appears in the form of cards that are focused on particular problems or concepts.
For instance, if MedKnowts identifies the clinical term “diabetes” in the text as a clinician types, the system automatically displays a “diabetes card” containing medications, lab values, and snippets from past records that are relevant to diabetes treatment.
Most EHRs store historical information on separate pages and list medications or lab values alphabetically or chronologically, forcing the clinician to search through data to find the information they need, Murray says. MedKnowts only displays information relevant to the particular concept the clinician is writing about.
“This is a closer match to the way doctors think about information. A lot of times, doctors will do this subconsciously. They will look through a medications page and only focus on the medications that are relevant to the current conditions. We are helping to do that process automatically and hopefully move some things out of the doctor’s head so they have more time to think about the complex part, which is determining what is wrong with the patient and coming up with a treatment plan,” Murray says.
Pieces of interactive text called chips serve as links to related cards. As a physician types a note, the autocomplete system recognizes clinical terms, such as medications, lab values, or conditions, and transforms them into chips. Each chip is displayed as a word or phrase that has been highlighted in a certain color depending on its category (red for a medical condition, green for a medication, yellow for a procedure, etc.)
Through the use of autocomplete, structured data on the patient’s conditions, symptoms, and medication usage is collected with no additional effort from the physician.
Sontag says he hopes the advance will “change the paradigm of how to create large-scale health datasets for studying disease progression and assessing the real-world effectiveness of treatments.”
In practice
After a year-long iterative design process, the researchers tested MedKnowts by deploying the software in the emergency department at Beth Israel Deaconess Medical Center in Boston. They worked with an emergency physician and four hospital scribes who enter notes into the electronic health record.
Deploying the software in an emergency department, where doctors operate in a high-stress environment, involved a delicate balancing act, Agrawal says.
“One of the biggest challenges we faced was trying to get people to shift what they currently do. Doctors who have used the same system, and done the same dance of clicks so many times, form a sort of muscle memory. Whenever you are going to make a change, there is a question of is this worth it? And we definitely found that some features had greater usage than others,” she says.
The Covid-19 pandemic complicated the deployment, too. The researchers had been visiting the emergency department to get a sense of the workflow, but were forced to end those visits due to Covid-19 and were unable to be in the hospital while the system was being deployed.
Despite those initial challenges, MedKnowts became popular with the scribes over the course of the one-month deployment. They gave the system an average rating of 83.75 (out of 100) for usability.
Scribes found the autocomplete function especially useful for speeding up their work, according to survey results. Also, the color-coded chips helped them quickly scan notes for relevant information.
Those initial results are promising, but as the researchers consider the feedback and work on future iterations of MedKnowts, they plan to proceed with caution.
“What we are trying to do here is smooth the pathway for doctors and let them accelerate. There is some risk there. Part of the purpose of bureaucracy is to slow things down and make sure all the i’s are dotted and all the t’s are crossed. And if we have a computer dotting the i’s and crossing the t’s for doctors, that may actually be countering the goals of the bureaucracy, which is to force doctors to think twice before they make a decision. We have to be thinking about how to protect doctors and patients from the consequences of making the doctors more efficient,” Karger says.
A longer-term vision
The researchers plan to improve the machine learning algorithms that drive MedKnowts so the system can more effectively highlight parts of the medical record that are most relevant, Agrawal says.
They also want to consider the needs of different medical users. The researchers designed MedKnowts with an emergency department in mind — a setting where doctors are typically seeing patients for the first time. A primary care physician who knows their patients much better would likely have some different needs.
In the longer-term, the researchers envision creating an adaptive system that clinicians can contribute to. For example, perhaps a doctor realizes a certain cardiology term is missing from MedKnowts and adds that information to a card, which would update the system for all users.
The team is exploring commercialization as an avenue for further deployment.
“We want to build tools that let doctors create their own tools. We don’t expect doctors to learn to be programmers, but with the right support they might be able to radically customize whatever medical applications they are using to really suit their own needs and preferences,” Karger says.
This research was funded by the MIT Abdul Latif Jameel Clinic for Machine Learning in Health.
Share this news article on:
Related links.
- David Karger
- David Sontag
- Computer Science and Artificial Intelligence Laboratory
- Abdul Latif Jameel Clinic for Machine Learning in Health
- Institute for Medical Engineering and Science
- Department of Electrical Engineering and Computer Science
Related Topics
- Health care
- Artificial intelligence
- Electrical Engineering & Computer Science (eecs)
- Computer Science and Artificial Intelligence Laboratory (CSAIL)
- Institute for Medical Engineering and Science (IMES)
Related Articles
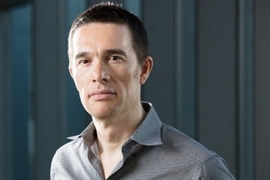
3 Questions: John Van Reenen on the impact of technology on health care workers
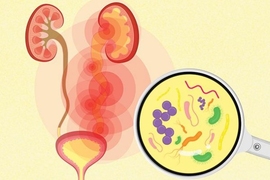
Algorithm reduces use of riskier antibiotics for UTIs
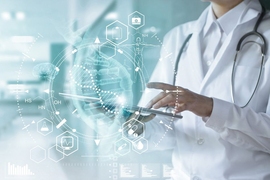
How well can computers connect symptoms to diseases?
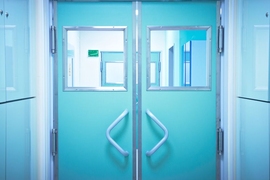
Model improves prediction of mortality risk in ICU patients
Previous item Next item
More MIT News
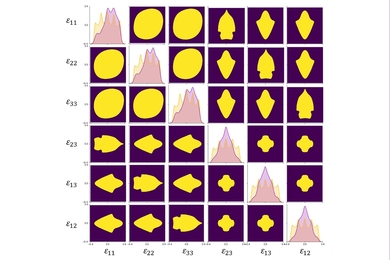
A first-ever complete map for elastic strain engineering
Read full story →
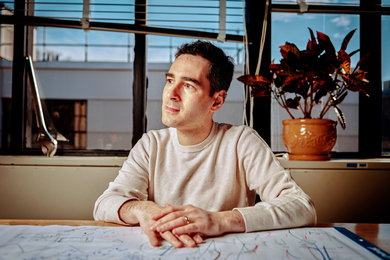
“Life is short, so aim high”
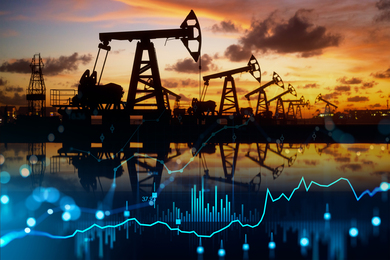
Shining a light on oil fields to make them more sustainable
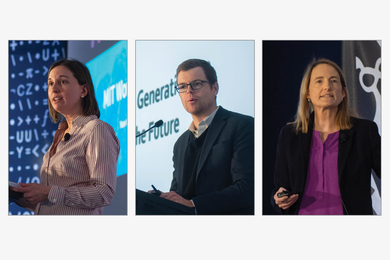
MIT launches Working Group on Generative AI and the Work of the Future
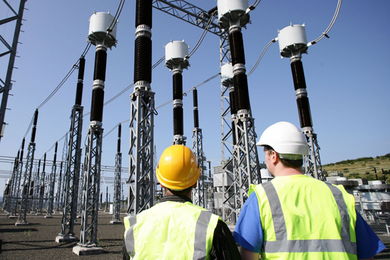
Atmospheric observations in China show rise in emissions of a potent greenhouse gas
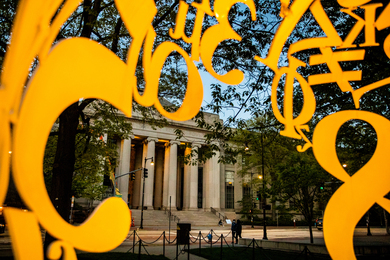
Second round of seed grants awarded to MIT scholars studying the impact and applications of generative AI
- More news on MIT News homepage →
Massachusetts Institute of Technology 77 Massachusetts Avenue, Cambridge, MA, USA
- Map (opens in new window)
- Events (opens in new window)
- People (opens in new window)
- Careers (opens in new window)
- Accessibility
- Social Media Hub
- MIT on Facebook
- MIT on YouTube
- MIT on Instagram

An official website of the Department of Health & Human Services

- Search All AHRQ Sites
- Email Updates
Informing Improvement in Care Quality, Safety, and Efficiency
- Contact DHR

Electronic Medical Record Systems
Background | Areas of Current Investigation | AHRQ-Funded Projects | Selected EMR Resources |
Electronic medical record (EMR) systems, defined as "an electronic record of health-related information on an individual that can be created, gathered, managed, and consulted by authorized clinicians and staff within one health care organization," [ 1 ] have the potential to provide substantial benefits to physicians, clinic practices, and health care organizations. These systems can facilitate workflow and improve the quality of patient care and patient safety. Despite these benefits, widespread adoption of EMRs in the United States is low; a recent survey indicated that only 4 percent of ambulatory physicians reported having an extensive, fully functional electronic records system and 13 percent reported having a basic system. [ 2 ]
Among the most significant barriers to adoption are:
- High capital cost and insufficient return on investment for small practices and safety net providers.
- Underestimation of the organizational capabilities and change management required.
- Failure to redesign clinical process and workflow to incorporate the technology systems.
- Concern that systems will become obsolete.
- Lack of skilled resources for implementation and support.
- Concern that current market systems are potentially not meeting the needs of rural health centers or federally qualified health centers (FQHC).
- Concern regarding negative unintended consequences of technology.
Recognizing the role that EMRs can play in transforming health care, in 2003, the Institute of Medicine issued a group of eight key functions for safety, quality, and care efficiency that EMRs should support.
- Physician access to patient information, such as diagnoses, allergies, lab results, and medications.
- Access to new and past test results among providers in multiple care settings.
- Computerized provider order entry.
- Computerized decision-support systems to prevent drug interactions and improve compliance with best practices.
- Secure electronic communication among providers and patients.
- Patient access to health records, disease management tools, and health information resources.
- Computerized administration processes, such as scheduling systems.
- Standards-based electronic data storage and reporting for patient safety and disease surveillance efforts.
Areas of Current Investigation
Traditionally, the EMR vendor community has created systems that conform only to proprietary database formats, making it difficult for them to send and receive data from other, potentially competing products. The medical informatics community has realized the need for interoperability and thus has created standards for data coding and communication. The Office of the National Coordinator for Health IT (ONC) has funded several major initiatives to harmonize standards and create a certification process for EMRs so that different products can interoperate better and be easily and objectively compared. This will enable decisionmakers to adopt EMRs more easily.
In 2006, the U.S. Department of Health and Human Services (HHS) recognized initial criteria for certification of ambulatory EHR systems as recommended by the Certification Commission for Healthcare Information Technology (CCHIT) . The criteria were updated in 2010 ( PDF , 2.3 MB). These criteria will help reduce barriers for ambulatory providers to adopt EHR systems by ensuring confidence in purchased products. CCHIT certified products also meet requirements set forth by HHS in final physician self-referral law and anti-kickback statute rules, providing access to external means of implementing EHR systems.
Federal initiatives are under way to drive adoption of interoperable EMRs, including funding of the Agency for Healthcare Research and Quality (AHRQ) Health IT portfolio. The recent American Recovery and Reinvestment Act (ARRA) of 2009 ( PDF , 1 MB ) authorizes $34 billion to be distributed starting in 2011 as adoption incentives through Medicare and Medicaid to qualified providers who adopt and use certified EMRs. In addition, several States have recently promoted EMR adoption by mandates, initiatives, or funding programs through the disbursement of grants and loans within their States:
- The State of Minnesota is perhaps the most aggressive in promoting the adoption of standards-based electronic health records to support statewide electronic health information infrastructure. Minnesota has done this through a combination of legislative mandates and grants and loans programs.
- Missouri has established a fund for health IT development that is being made available to health care providers. Senate Bill 577 (2007) ( PDF , 2.29 MB ) states, "There is hereby created in the state treasury the "Health Care Technology Fund" which shall consist of all gifts, donations, transfers, and moneys appropriated by the general assembly, and bequests to the fund."
- Wisconsin has created a tax credit for health care providers who purchase EMRs in Senate Bill 40 (2007). Providers can claim up to 50 percent of the cost of the system with a maximum of $10 million a year ( PDF , 5.49 MB) .
AHRQ-Funded Projects
AHRQ has funded organizations across the country that are implementing and evaluating electronic medical and health record systems. Some of these include:
Title: Ambulatory Electronic Medical Record and Shared Access Principal Investigator: Michael DeLuca State: IL
Title: An Interactive Preventive Health Record (IPHR) to Promote Patient-Centered Care Principal Investigator: Alexander Krist State: VA
Title: A Partnership for Clinician EHR Use and Quality of Care Principal Investigator: Joanne Pohl State: MI
Title: Bringing Measurement to the Point of Care Principal Investigator: Winfred Wu State: NY
Title: Can Risk Score Alerts Improve Office Care for Chest Pain? Principal Investigator: Thomas Sequist State: MA
Title: Cardio HIT Phase II Principal Investigator: Karen Kmetik State: IL
Title: Conversational IT for Better, Safer Pediatric Primary Care Principal Investigator: William Adams State: MA
Title: Creating an Evidence Base for Vision Rehabilitation Principal Investigator: Cynthia Stuen State: NY
Title: Crossing the Quality Assessment Chasm: Aligning Measured and True Quality of Care Principal Investigator: Mark Weiner State: PA
Title: Crossing the Quality Chasm in Eastern Rural Kern County Principal Investigator: Kiki Nocella State: CA
Title: Developing and Using Valid Clinical Quality Metrics for HIT with HIE Principal Investigator: Rainu Kaushal State: NY
Title: eHealth Records to Improve Dental Care for Patients with Chronic Illnesses Principal Investigator: James Fricton State: MN
Title: Electronic Prescribing and Decision Support to Improve Rural Primary Care Quality Principal Investigator: James Veline State: SD
Title: Electronic Support for Public Health - Vaccine Adverse Event Reporting System (ESP:VAERS) Principal Investigator: Ross Lazarus State: MA
Title: Evaluation of a Computerized Clinical Decision Support System and EHR-Linked Registry to Improve Management of Hypertension in Community-Based Health Centers Principal Investigator: Helene Kopal State: NY
Title: Feedback of Treatment Intensification Data to Reduce Cardiovascular Disease Risk Principal Investigator: Joe Selby State: CA
Title: Harnessing Health IT to Prevent Medication-Induced Birth Defects Principal Investigator: Eleanor Schwarz State: PA
Title: Health Information Technology in the Nursing Home Principal Investigator: Jerry Gurwitz State: MA
Title: Impact of Health Information Technology on Clinical Care Principal Investigator: John Hsu State: CA
Title: Impact of Office-Based e-Prescribing on Prescribing Processes and Outcomes Principal Investigator: Michael Fischer State: MA
Title: Implementing a Low-Literacy, Multimedia IT System to Enhance Patient-Centered Cancer Care Principal Investigator: Elizabeth Hahn State: IL
Title: Improving Laboratory Monitoring in Community Practices: A Randomized Trial Principal Investigator: Steven Simon State: MA
Title: Improving Otitis Media Care with EHR-based Clinical Decision Support and Feedback Principal Investigator: Christopher Forrest State: PA
Title: Massachusetts Quality e-Measure Validation Study Principal Investigator: Eric Schneider State: MA
Title: Medication Monitoring for Vulnerable Populations via IT Principal Investigator: Christoph Lehmann State: MD
Title: Medication Safety in Primary Care Practice - Translating Research into Practice Principal Investigator: Steven Ornstein State: SC
Title: Monitoring Intensification of Treatment for Hyperglycemia and Hyperlipidemia Principal Investigator: Alexander Turchin State: MA
Title: Patient-Centered Online Disease Management Using a Personal Health Record System Principal Investigator: Paul Tang State: CA
Title: Pharmaceutical Safety Tracking (PhaST): Managing Medications for Patient Safety Principal Investigator: William Gardner State: OH
Title: Statewide Implementation of Electronic Health Records Principal Investigator: David W. Bates State: MA
Title: The BLUES Project: Improving Diabetes Outcomes in Mississippi with Health IT Principal Investigator: Karen Fox State: MS
Title: Tulare District Hospital Rural Health EMR Consortium Principal Investigator: Paul D. Galloway State: CA
Title: Using an Electronic Personal Health Record to Empower Patient with Hypertension Principal Investigator: Peggy Wagner State: GA
Title: Using Electronic Records to Detect and Learn from Ambulatory Diagnostic Errors Principal Investigator: Eric Thomas State: TX
Title: Using Health IT to Improve Ambulatory Chronic Disease Care Principal Investigator: David Mehr State: MO
Title: Using Information Technology to Provide Measurement Based Care for Chronic Illness Principal Investigator: Madhukar Trivedi State: TX
Title: Using IT for Patient-Centered Communication and Decision Making about Medications Principal Investigator: Michael Wolf State: IL
Title: Using IT to Improve the Quality of CVD Prevention & Management Principal Investigator: Thomas Vogt State: HI
Title: Using Precision Performance Measurement to Conduct Focused Quality Improvement Principal Investigator: David Baker State: IL
Title: VA Integrated Medication Manager Principal Investigator: Jonathan Nebeker State: UT
Selected EMR Resources
A Cost-Benefit Analysis of Electronic Medical Records in Primary Care Author(s): Wang SJ, Middleton B, Prosser LA, Bardon CG, Spurr CD, Carchidi PJ, Kittler AF, Goldszer RC, Fairchild DG, Sussman AJ, Kuperman GJ, Bates DW Source : Am J Med 2003 Apr 1;114(5):397-403. Summary : The purpose of this study was to estimate the net financial benefit or cost of implementing electronic medical record systems in primary care. Much of the data and conclusions are based on expert opinion and assumptions, with a minority of data from actual studies. We performed a cost-benefit study to analyze the financial effects of electronic medical record systems in ambulatory primary care settings from the perspective of the health care organization. The reference strategy for comparisons was the traditional paper-based medical record. The estimated net benefit from using an electronic medical record for a 5-year period was $86,400 per provider. Benefits accrue primarily from savings in drug expenditures, improved utilization of radiology tests, better capture of charges, and decreased billing errors. Implementation of an electronic medical record system in primary care can result in a positive financial return on investment to the health care organization.
AProposal for Electronic Medical Records in U.S. Primary Care Author(s): Bates DW, Ebell M, Gotlieb E, Zapp J, Mullins HC Source: J Am Med Inform Assoc (JAMIA) 2003 Jan-Feb;10(1):1-10. Summary : Delivery of excellent primary care?central to overall medical care?demands that providers have the necessary information when they give care. This paper, developed by the National Alliance for Primary Care Informatics, a collaborative group sponsored by a number of primary care societies, argues that providers' and patients' information and decision support needs can be satisfied only if primary care providers use electronic medical records (EMRs). Although robust EMRs are now available, only about 5 percent of U.S. primary care providers use them. Recently, with only modest investments, Australia, New Zealand, and England have achieved major breakthroughs in implementing EMRs in primary care. Substantial benefits realizable through routine use of EMRs include improved quality, safety, and efficiency, along with increased ability to conduct education and research. Nevertheless, barriers to adoption exist and must be overcome. Implementing specific policies can accelerate utilization of EMRs in the United States.
Electronic Health Record Systems: the Vehicle for Implementing Performance Measures Author(s) : O'Toole MF, Kmetik KS, Bossley H, Cahill JM, Kotsos TP, Schwamberger PA, Bufalino VJ Source : Am Heart Hosp J 2005 Spring; 3(2):88-93. Summary : Advances in information technology and recent national directives have the potential to support dramatic improvements in health care. Two key components are the implementation of functional electronic health record (EHR) systems and widely accepted, evidence-based clinical performance measures for physicians. Midwest Heart Specialists, a 55-physician cardiovascular group at 14 locations in northern Illinois, has utilized an outpatient electronic health record (HER) system since 1997. Since 2003, the group has integrated cardiovascular measurement sets developed by the American Medical Association-convened Physician Consortium for Performance Improvement into its EHR system. With this integration, the group was able to capture data needed for internal quality assessment and improvement as part of routine outpatient care without the need for additional resources. Critical disease-management data for decision support are available continuously, resulting in improvements in health care. The reporting of these standardized data could be the foundation to support quality-based reimbursement strategies and physician office-based, disease-management strategies.
How to Successfully Navigate Your EHR Implementation Author(s) : Adler KG Source : Fam Pract Manag 2007 Feb;14(2):33-9. Summary : This article identifies the three major do's and don'ts of electronic health record (EHR) system implementation and explores the application of these components. The author characterizes these areas as "the three T's": team, tactics, and technology. Each EHR implementation team should have a physician champion who motivates others, a skilled and collaborative project manager, broad stakeholder involvement, and specific, measurable goals. Tactics to employ for a successful implementation include: design a balanced scanning strategy, utilize a phased implementation, lighten workloads when going "live" and for a short period afterward, and enter data into the EHR electronically as much as possible. Havingproper speed and high network availability and capability, maintaining a test environment to mirror the live environment in case of problems, utilizing expert information technology (IT) advice when it comes to servers and networks, maintaining servers, and having a disaster recovery plan in place are all technology issues to address for successful implementation.
Medical Groups' Adoption of Electronic Health Records and Information Systems Author(s) : Gans D, Kralewski J, Hammons T, Dowd B Source : Health Aff (Millwood--Spring Hope) 2005 Sep-Oct;24(5):1323-33. Summary : We surveyed a nationally representative sample of medical group practices to assess their current use of information technology (IT). Our results suggest that adoption of electronic health records (EHRs) is progressing slowly, at least in smaller practices, although a number of group practices plan to implement an EHR within the next 2 years. Benefits of implementing an EHR include improved access to medical record information, workflow, patient communications, and accuracy for coding evaluation and management procedures. For those both with and without EHRs, the top five barriers were related to costs and concerns about physicians' support and their ability to use the new system. Overall, the process of choosing and implementing an EHR appears to be more complex and varied than we expected. This suggests a need for greater support for practices, particularly smaller ones, in this quest, if the benefits expected from EHRs are to be realized.
Physicians' Use of Electronic Medical Records: Barriers and Solutions Author(s) : Miller RH, Sim I Source : Health Aff (Millwood--Spring Hope) 2004 Mar-Apr;23(2):116-26. Summary : The electronic medical record (EMR) is an enabling technology that allows physician practices to pursue more powerful quality improvement programs than is possible with paper-based records. However, achieving quality improvement through EMR use is neither low-cost nor easy. Based on a qualitative study of physician practices that had implemented an EMR, we found that quality improvement depends heavily on physicians' use of the EMR?and not paper?for most of their daily tasks. The key barriers to physicians' use of EMRs include high initial costs and uncertain financial benefits; high initial physician time costs to learn the system; difficulties with technology, including EMR usability; and difficult complementary changes and inadequate assistance from both IT support and EMR vendors. We then suggest policy interventions to overcome these barriers, including providing work/practice support systems, improving electronic clinical data exchange, and providing financial rewards for quality improvement.
Primary Care Physician Time Utilization Before and After Implementation of an Electronic Health Record: A Time-motion Study Author(s) : Pizziferri L, Kittler AF, Volk LA, Honour MM, Gupta S, Wang S, Wang T, Lippincott M, Li Q, Bates DW Source : J Biomed Inform 2005 Jun;38(3):176-188. Summary : Despite benefits associated with the use of electronic health records (EHRs), one major barrier to adoption is the concern that EHRs may take longer for physicians to use than paper-based systems. To address this issue, we performed a time-motion study in five primary care clinics. Twenty physicians were observed and specific activities were timed during a clinic session before and after EHR implementation. Postimplementation, the adjusted mean overall time spent per patient during clinic sessions decreased by 0.5 min (p=0.86; 95 percent confidence interval [-5.05, 6.04]) from a preintervention adjusted average of 27.55 min (SE=2.1) to a post-intervention adjusted average of 27.05 min (SE=1.6). A majority of survey respondents believed EHR use results in quality improvement, yet only 29 percent reported that EHR documentation takes the same amount of time or less compared to the paper-based system. While the EHR did not require more time for physicians during a clinic session, further studies should assess the EHR's potential impact on nonclinic time.
The Impact of Electronic Health Records on Time Efficiency of Physicians and Nurses: A Systematic Review Author(s) : Poissant L, Pereira J, Tamblyn R, Kawasumi Y Source : J Am Med Inform Assoc (JAMIA) 2005 Sep-Oct;12(5):505-16. Summary : This systematic review examined the impact of electronic health records (EHRs) on documentation time of physicians and nurses. Twenty-three papers met our inclusion criteria; five were randomized controlled trials, six were posttest control studies, and 12 were one-group pretest/posttest designs. The use of bedside terminals and central station desktops saved nurses, respectively, 24.5 percent and 23.5 percent of their overall time spent documenting during a shift. Using bedside or point-of-care systems increased documentation time of physicians by 17.5 percent. In comparison, the use of central station desktops for computerized provider order entry (CPOE) was found to be inefficient, increasing the work time from 98.1 percent to 328.6 percent of physician's time per working shift. Studies conducting their evaluation process relatively soon after implementation of the EHR tended to demonstrate a reduction in documentation time; studies with a longer interval between implementation and the evaluation process observed an increase in time. This review highlighted that a goal of decreased documentation time in an EHR project is not likely to be realized.
The Value of Electronic Health Records in Solo or Small Group Practices Author(s) : Miller RH, West C, Brown TM, Sim I, Ganchoff C Source : Health Aff (Millwood--Spring Hope) 2005 Sep-Oct;24(5):1127-37. Summary : We conducted case studies of 14 solo or small-group primary care practices using electronic health record (EHR) software from two vendors. Initial EHR costs averaged $44,000 per full-time equivalent (FTE) provider, and ongoing costs averaged $8,500 per provider per year. The average practice paid for its EHR costs in 2.5 years and profited handsomely after that; however, some practices could not cover costs quickly; most providers spent more time at work initially, and some practices experienced substantial financial risks. Policies should be designed to provide incentives and support services to help practices improve the quality of their care by using EHRs. This article provides useful information for clinicians interested in purchasing and implementing an EHR, and for provider organizations and policymakers who may be involved in making decisions about EHR adoption. While only focusing on two EHR vendor systems is a limitation of this study, the numbers are consistent with other cost data from similar studies.
Using Diffusion of Innovation Concepts to Enhance Implementation of an Electronic Health Record to Support Evidence-Based Practice Author(s) : Geibert RC Source : Nurs Adm Q 2006 Jul-Sep;30(3):203-10. Summary : The article identifies the explosion of clinical data that is available and how difficult it is for clinicians to find answers to clinical questions. Electronic health records (EHRs) are used increasingly to assist clinicians in this process; however, resistance to the implementation of technology-assisted care is not uncommon. The article reviews the diffusion of innovation research and provides the nurse manager with suggestions for applying these concepts to enhance the implementation of an EHR that can support evidence-based practice. Five characteristics of innovations are discussed, as they help explain different rates of adoption. These characteristics are represented by the acronym TACOS: Trialability (Can we try this on a small scale first?), Advantage (Is this an important goal for our unit?), Compatibility (Will the practice work in our environment?), Observability (Can we see the practice in action at another site?), and Simplicity (How big a change will this be?). The five-stage, innovation-decision process is studied as it relates to EHR implementations.
- Director's Corner
- Current Priorities
- Executive Summary
- Research Spotlight
- Research Themes and Findings
- Research Dissemination
- Research Overview
- 2020 Year in Review
- 2019 Year in Review
- Engaging and Empowering Patients
- Optimizing Care Delivery for Clinicians
- Supporting Health Systems in Advancing Care Delivery
- Our Experts
- Search AHRQ-Funded Projects
- AHRQ-Funded Projects Map
- AHRQ Digital Healthcare Research Publications Database
- Health IT Survey Compendium
- Time and Motion Studies Database
- Health Information Security and Privacy Collaboration Toolkit
- Implementation in Independent Pharmacies
- Implementation in Physician Offices
- ePROs in Clinical Care
- Guide to Integrate Patient-Generated Digital Health Data into Electronic Health Records in Ambulatory Care Settings
- Children's Electronic Health Record (EHR) Format
- Project Resources Archives
- National Webinars
- Funding Opportunities
- Digital Healthcare Research Home
- 2018 Year in Review Home
- Research Summary
- Research Spotlights
- 2019 Year in Review Home
- Annual Report Home
Electronic health records to facilitate clinical research
Affiliations.
- 1 National Heart and Lung Institute, Imperial College London, Royal Brompton Hospital, Sydney Street, London, SW3 6HP, UK. [email protected].
- 2 Astra Zeneca R&D, Molndal, Sweden.
- 3 University of Turku, Turku, Finland.
- 4 Duke Clinical Research Institute, Durham, NC, USA.
- 5 Servier, Paris, France.
- 6 Robertson Centre for Biostatistics, University of Glasgow, Glasgow, UK.
- 7 University of Münster, Münster, Germany.
- 8 Daiichi-Sankyo, London, UK.
- 9 GlaxoSmithKline, Stockley Park, UK.
- 10 Boehringer-Ingelheim, Pharma GmbH & Co KG, Ingelheim, Germany.
- 11 Optum International, London, UK.
- 12 Bayer Pharma, Berlin, Germany.
- 13 Pfizer Ltd., Surrey, UK.
- 14 Institute of Health and Wellbeing, University of Glasgow, Glasgow, UK.
- 15 Food and Drug Administration, Silver Spring, MD, USA.
- 16 Campbell University College of Pharmacy and Health Sciences, Campbell, NC, USA.
- 17 Edwards LifeSciences, Nyon, Switzerland.
- 18 INSERM, Centre d'Investigation Clinique 9501 and Unité 961, Centre Hospitalier Universitaire, Nancy, France.
- 19 Department of Cardiology, Nancy University, Université de Lorraine, Nancy, France.
- 20 Glaxo Smith Kline, King of Prussia, Pennsylvania, USA.
- PMID: 27557678
- PMCID: PMC5226988
- DOI: 10.1007/s00392-016-1025-6
Electronic health records (EHRs) provide opportunities to enhance patient care, embed performance measures in clinical practice, and facilitate clinical research. Concerns have been raised about the increasing recruitment challenges in trials, burdensome and obtrusive data collection, and uncertain generalizability of the results. Leveraging electronic health records to counterbalance these trends is an area of intense interest. The initial applications of electronic health records, as the primary data source is envisioned for observational studies, embedded pragmatic or post-marketing registry-based randomized studies, or comparative effectiveness studies. Advancing this approach to randomized clinical trials, electronic health records may potentially be used to assess study feasibility, to facilitate patient recruitment, and streamline data collection at baseline and follow-up. Ensuring data security and privacy, overcoming the challenges associated with linking diverse systems and maintaining infrastructure for repeat use of high quality data, are some of the challenges associated with using electronic health records in clinical research. Collaboration between academia, industry, regulatory bodies, policy makers, patients, and electronic health record vendors is critical for the greater use of electronic health records in clinical research. This manuscript identifies the key steps required to advance the role of electronic health records in cardiovascular clinical research.
Keywords: Cardiovascular diseases; Clinical trials as topic; Electronic health records; Pragmatic clinical trials as topic.
Publication types
- Access to Information
- Cardiovascular Diseases* / diagnosis
- Cardiovascular Diseases* / epidemiology
- Cardiovascular Diseases* / therapy
- Clinical Trials as Topic / ethics
- Clinical Trials as Topic / methods*
- Comparative Effectiveness Research / ethics
- Comparative Effectiveness Research / methods*
- Confidentiality
- Data Accuracy
- Data Mining* / ethics
- Electronic Health Records* / ethics
- Medical Record Linkage
- Research Design*
- Systems Integration
Grants and funding
- MC_PC_13040/MRC_/Medical Research Council/United Kingdom
- MR/K007017/1/MRC_/Medical Research Council/United Kingdom
- Research article
- Open access
- Published: 06 January 2021
Electronic medical record implementation in tertiary care: factors influencing adoption of an electronic medical record in a cancer centre
- Anna Janssen ORCID: orcid.org/0000-0001-6611-9651 1 , 2 ,
- Candice Donnelly 1 , 2 ,
- Elisabeth Elder 3 ,
- Nirmala Pathmanathan 3 &
- Tim Shaw 1 , 2
BMC Health Services Research volume 21 , Article number: 23 ( 2021 ) Cite this article
10k Accesses
12 Citations
1 Altmetric
Metrics details
Electronic Medical Records (EMRs) are one of a range of digital health solutions that are key enablers of the data revolution transforming the health sector. They offer a wide range of benefits to health professionals, patients, researchers and other key stakeholders. However, effective implementation has proved challenging.
A qualitative methodology was used in the study. Interviews were conducted with 12 clinical and administrative staff of a cancer centre at one-month pre-launch and eight clinical and administrative staff at 12-months post-launch of an EMR. Data from the interviews was collected via audio recording. Audio recordings were transcribed, de-identified and analysed to identify staff experiences with the EMR.
Data from the pre-implementation interviews were grouped into four categories: 1) Awareness and understanding of EMR; 2) Engagement in launch process; 3) Standardisation and completeness of data; 4) Effect on workload. Data from the post-launch interviews were grouped into six categories: 1) Standardisation and completeness of data; 2) Effect on workload; 3) Feature completeness and functionality; 4) Interaction with technical support; 5) Learning curve; 6) Buy-in from staff. Two categories: Standardisation and completeness of data and effect on workload were common across pre and post-implementation interviews.
Findings from this study contribute new knowledge on barriers and enablers to the implementation of EMRs in complex clinical settings. Barriers to successful implementation include lack of technical support once the EMR has launched, health professional perception the EMR increases workload, and the learning curve for staff adequately familiarize themselves with using the EMR.
Peer Review reports
Digital technologies are being widely adopted in the healthcare, leading to a widespread transformation of the sector. This transformation has included an increase in the personalisation of health care [ 1 , 2 ] and potential for new approaches to facilitate research quality improvement initiatives and patient driven care [ 3 ]. Lately, digital technologies have been adopted by health sectors often for its better functioning and Electronic Medical Records (EMRs). EMRs are computerized information systems which collect, store and display patients’ information and typically replace paper-based medical records [ 4 ].
EMRs are key enablers of the data revolution transforming the health sector [ 5 ]. They incorporate a wide range of functionalities but can be broadly described as systems for presenting health, clinical or medical records in a digitised format [ 6 ]. The literature has acknowledged a number of benefits of using EMRs for healthcare professionals, patients, organisations and other stakeholders. Benefits include improving the quality of care for patients [ 6 ], improving timely access to data, and to potentially facilitate knowledge exchange for collaboration in multidisciplinary teams (MDTs) [ 7 ].
In spite of the wide range of potential benefits, physicians and other healthcare staffs can harness these supports only if EMRs are implemented [ 4 ]. However, it has been noted in the literature that there is a notable gap in understanding about the best ways to implement digital health [ 8 , 9 ]. The implementation of EMRs is particularly complex and requires a range of technical, human and organisational factors to be considered [ 7 ]. A comprehensive review of the literature identified eight categories of barriers to EMR adoption: Financial, Technical, Time, Psychological, Social, Legal, Organizational and Change process [ 4 ]. Financial, time/workload and technical barriers are the most frequently cited challenges in the literature [ 7 ].
Although the literature has identified some of the barriers and enablers to implementing EMRs in healthcare, much of this research has focused on primary care [ 10 ]. There is currently a dearth of literature on the implementation of EMRs in cancer care, which is a unique setting for implementing technology. The gold standard for the delivery of cancer care is the use of MDTs. MDTs describe a group of health professionals from a range of specialties who work together on a regular basis to deliver evidence-based care to patients [ 11 ]. Successful implementation of EMRs in a multidisciplinary setting requires consideration of the unique challenges to implementing EMRs for health professionals across a range of specialties, and for administrative staff.
This study described in this manuscript aimed to explore the barriers and enablers to implementing an EMR in a metropolitan tertiary cancer service.
Study design
A qualitative methodology was used to collect data on the experiences of clinical and administrative staff at pre- and post- launch of a new EMR.
Participants and study setting
The EMR was implemented in November 2017 in a breast cancer service within Western Sydney Local Health District. The cancer service is located across three hospitals within Western Sydney Local Health District (LHD). The main centre is co-located with a screening clinic and registers approximately 500 new breast cancer patients per year. Staff at the cancer centre had used another EMR platform for 10 years prior to the launch of the new EMR investigated in this study. The existing platform was a bespoke SQL database that no longer met the needs of the service. This EMR was predominantly used by administrative staff who entered data from paper records completed by clinical staff as well as clinicians doing follow-up with patients, had restricted interoperability with the main hospital EMR (Cerner) and was not used widely across the various disciplines within the service. The new EMR was developed in a Cerner Millennium platform with bespoke built multidisciplinary breast cancer fields for data entry at point-of-care. The development was a collaboration between key clinical representatives of the breast cancer centre and the LHD Information Communication and Technology service over a period of several years.
All clinical and administrative staff within the service were eligible to participate in the study, but due to the size of the center not all staff opted to participate. A purposeful sample were recruited to participate in 30–60 min phone interviews about their experiences with the EMR. A total of 12 participants consented to participate in the pre-launch interviews, three in administrative or research roles and nine in clinical roles. A total of eight participants consented to participate in the post-launch interviews, two in administrative or research roles and six in clinical roles. Participants were from a range of disciplines including medical oncology, radiation oncology, surgery, pathology, nursing and clinical administration.
Potential participants were recruited via email to participate in the study. Consent was provided either through return of a signed consent form, or via confirmation in the email response. At the start of each interviewee consent to participate in the interview and consent to record the interview was once again confirmed.
Interviews were conducted with staff within the cancer centre one-month pre-launch and twelve months post-launch of the EMR. Potential participants for the pre-implementation interviews were identified by members of the Advisory Committee that oversee the development of data improvement projects within the organisation. A member of the research team contacted all eligible participants via email and scheduled a pre-launch interview. All participants who completed a pre-launch interview were invited to participate in an interview post-launch.
Both pre and post launch interviews were conducted face to face or via phone at a time convenient to the participant. Interviews took between 30 and 60 min and were conducted by a researcher experienced in qualitative methods. Interviews were audio recorded. Audio recordings were transcribed by a commercial transcription service, prior to de-identification by one member of the research team.
Refer to Additional file 1 to see the interview guide.
Analysis was conducted to identify key themes and subthemes. These themes were then refined to reduce redundancy and emphasise prominent groupings. During analysis, illustrative quotes were identified and grouped them by themes and sub-themes.
The study was granted ethical approval by the Western Sydney Local Health District Human Research Ethics Committee’s Executive Committee (protocol/approval number:4678). All participants provided written consent to participate in the study by return of an email, verbal consent was confirmed at the start of each interview.
Pre-implementation interview findings
Pre-implementation interview findings were grouped into 4 categories, awareness and understanding of electronic medical record, engagement in launch process, effect on workload, standardisation and completeness of data.
Refer to Table 1 for exemplar quotes related to these themes.
All participants were aware of the upcoming EMR launch within the service. The majority of participants were enthusiastic about the potential of the EMR, but many acknowledged uncertainty in what the additional capabilities and functionalities of the new EMR would be.
The participants who held the greatest knowledge of the functionality of the new EMR had been involved in the design and implementation of the EMR. Outside of this group, almost all participants indicated a basic understanding that the EMR would replace the current process of using paper records at point of care with digitised data entry into the EMR. It was also widely understood that the new EMR would change the data entry process to be completed by clinical staff rather than administrative staff. Some participants also indicated that the new EMR would replace the existing database used to store clinical notes, anticipating that this would be useful for research and quality improvement activities.
Finally, a number of participants discussed the use of the new EMR in MDT meetings instead of the existing process where administrative staff manually extract and collate relevant patient information for discussion. Most of the participants who understood this application of the EMR were clinical staff. Most participants thought the use of the EMR in MDT meetings would be beneficial, particularly for early career clinicians who would be able to learn from the process of using the record in meetings.
All participants indicated that they had been engaged on some level during the implementation process for the new EMR. Most participants had been involved in training sessions in the weeks prior to the EMR launch, or had been provided an opportunity to participate even if they hadn’t attended. A minority of participants indicated they had an active role in the design of the new EMR in the 18-month development period.
Although participants were happy with the level of engagement they had with the development and implementation of the EMR, the majority weren’t sure whether their needs had been captured in the final EMR design. A minority of participants indicated that features they had requested were not being made available in the initial launch of the EMR, but anticipated the inclusion of those features in the future. Other participants felt their requests for particular EMR features had been included, but were not sure how what they had requested would function once the EMR was made available to them. This was because request for features were often made prior to using the EMR or when there had only been limited use of the EMR. It was anticipated that there would be a need to refine the design once the EMR had launched in order to align it with the needs of the service.
Participants perceived the EMR to be beneficial for improving the quality of clinical data collected within their centre in the future. This was one of the most consistently stated benefits of implementing a new EMR. The anticipated benefits of the improved data collection and quality varied across participants, but included a reduction in incomplete data entry for patient records due to mandatory fields, a more holistic view of the patient record and opportunities to access data for research purposes.
A minority of participants raised concerns about the EMR’s effect on standardisation and data completeness. Some participants raised concerns that the EMR was not integrated with other clinical data repositories within the local health district (outside of the NSW Health EMR) and that interoperability would continue to be an issue. Another participant raised concern about the limitations of the EMR to capture data that was appropriate for their discipline specialty.
The majority of participants discussed the perceived effect of the EMR on their workload. Generally, participants were concerned that the EMR may negatively impact workload, particularly for clinical staff who would be required to complete data entry tasks. Although workload concerns were raised frequently, multiple participants also acknowledged that this issue was likely to resolve after launch when both clinical and administrative staff became more familiar with the technology and integrated it into their routine.
Although most participants felt the EMR would increase workload, a minority of participants felt that the EMR implementation could decrease their workload. Currently, a number of participants described having to manage and search through a large number of paper files to access information. Participants anticipated that being able to access information digitally would be more efficient. Other participants felt it would be beneficial to be able to access data in the EMR remotely allowing more flexibility over where work was undertaken.
Post-launch interview findings
Post-implementation interview findings were grouped into 5 categories, feature completeness and functionality, learning curve, buy-in from staff.
Refer to Table 2 for exemplar quotes related to these themes.
A total of eight interviews were conducted with staff within the cancer center. Of these interviews, three were undertaken with administrative staff and five were undertaken with clinical staff. Clinical staff represented a range of specialties including radiation oncology, surgery and pathology.
Data analysed from the interviews was classified into six categories: 1) Standardisation of documentation and completeness of data; 2) Effect on workload; 3) Feature completeness and functionality; 4) Interaction with technical support; 5) Learning curve; 6) Buy-in from staff.
Both administrative and clinical staff indicated benefits of interoperability with the main hospital EMR and centre-wide access to the same information in the EMR. This was valuable when patients had been transferred to the centre from other hospital departments as patient information was easily accessible, enabling more efficient internal transfer for patients. It was also noted as a useful reference for finding initial consult information for returning patients that the clinical staff had not seen for a long time.
The EMR was noted as valuable for improving completeness of data on MDT recommendations. The new process of live data entry during MDT meetings allows all members to ensure the accuracy of the data in real-time, thus reducing recall bias that may have occurred when data was entered from notes after the MDT meeting. The centre had also installed three large LCD screens in the MDT meeting room to easily visualise patient information from multiple systems at the same time as viewing the EMR. One participant noted that data entry during the MDT meeting led to increased recording of longer-term recommendations for patients.
The EMR was perceived to have both positive and negative impacts on workload. A participant reported that the EMR increased efficiency by making information more accessible. Once familiar with the platform, it was described as relatively easy to navigate the EMR and input patient data. With regards to follow-up patients, data entry was noted as particularly efficient, only taking a few minutes, though it was more time consuming for new patients. Other benefits included decreased time in finding and reviewing information prior to a patient consultation, when compared with the previous process of manually searching through paper records.
Participants noted increased efficiency and reduced risk of errors in the new process of clinical staff entering data directly into the EMR rather than the previous process of administrative staff transcribing paper records. One participant noted the increased accountability for clinical staff to input data into the EMR. However, concerns were raised that some senior clinicians had experienced an increased workload as they complete EMR data entry themselves, due to uncertainty regarding whether early career doctors were inputting data consistently.
Although some participants felt the EMR was more efficient, others felt it increased workload. Concern was also raised about the unexpected issues when the new EMR did not allow staff to complete simple tasks that were previously automated, including the inability to bulk-print letters. The EMR was described as having increased workload for administrative staff due to increased time spent on auditing and completing the letters automatically generated from the EMR, following-up clinical staff for completion of data needed to generate the letters and repetitively scanning documents in to the EMR.
A number of participants raised concerns about issues with features, or functionality of features in the EMR, and ranged in degree of severity, from usability to issues effecting data quality and workload. Some of these issues included not having drop down menus to select a doctor or provider name in the EMR or generating letters from the incorrect clinical encounter. Letter generation was a largely cited concern. Participants noted that the process of letter generation was cumbersome and often the letter contained the incorrect content. Furthermore, the letter layout was described as unprofessional by some interviewees as the template put constraints on the content and structure on the letter, preventing clinicians to generate their patient letters in a preferred style.
A significant concern, was the lack of the patient summary page which provided a single-page overview of the patients treatment and other clinical interactions in the EMR. The inclusion of a patient summary view was an important feature of the new EMRs design, and its sub-optimal implementation was a perceived barrier to use of the new EMR. This feature was intended for quick and easy access to pertinent patient information in one instance. The patient summary was also intended to be used for MDT meetings. Participants noted that due to a lack of the ‘patient summary’ page, the required navigation across tabs to retrieve all the information needed was time consuming.
Another major functionality issue was lack of auto completion of fields in the EMR. Concerns were raised about GP information not auto-populating as it did in the previous system. As this functionality was also not successfully executed, an unexpected technical issue that occurred was that patient information did not transfer across clinical encounters. There were some issues that occurred in the first 3 months of implementation when forms were not auto-populating and there was a loss of data. Finally, some participants noted that not all forms suited the type of appointment e.g. second appointment for surgical decision, making it difficult to know where the data should be entered appropriately. Another highlighted issue was the lack of flexibility in the EMR drop-down menus or pre-set tick box options. One clinical staff member noted this was particularly concerning as these options should incorporate emerging evidence, such as new drugs.
There were some concerns about the difficulty in remembering how to enter data into the new EMR fields, particularly for staff that didn’t interact with it regularly. The platform was different to that found in other clinical settings which also meant new staff had a learning curve to familiarise themselves with how to use the system. However, it was noted that it had typically taken one to 2 weeks to overcome the learning curve. After this time, the EMR usability was reported positively. One participant noted that the EMR may be challenging to learn to use for relief staff who may only use it for one or 2 weeks.
Some participants raised concerns about lack of buy-in to the EMR across clinical disciplines that work within and in collaboration within the centre, which led to gaps in available information. There were also concerns that some people had ceased using the EMR because of issues with the data fields. These issues included drop down menus in the EMR not including treatment options the clinicians wanted to use, and other issues relating to navigating the EMR.
This study presents findings describing the implementation of EMR in a cancer care centre. The findings are broadly aligned with the literature on barriers to implementation of EMRs, particularly in regard to the central role of Information Technology (IT) support during the post-implementation period [ 2 , 7 ]. However, findings from this study suggested that staff were prepared to overlook challenges in functionality or technical support due to a perception the system would become beneficial over time as system errors would be addressed.
Findings from this study showed that prior to launch, study participants were generally enthusiastic about the EMR and optimistic it would improve processes. A core component of this enthusiasm was the perceived engagement during the pre-implementation process. A number of participants were provided an opportunity to be involved in the design of the EMR, and all participants noted they had an opportunity to participate in EMR training sessions. Whilst research has showed the value of involving health professionals in EMR to improve uptake [ 12 ], there is little research describing the important role of delivering flexible training to staff on the use of the new system in the lead up to implementation. The value of using education and training as a tool in successful implementation has been acknowledged in the literature [ 12 ], with findings from this study showing it is as important when implementing digital technologies as in other areas.
The literature has shown that clinician resistance is a major barrier to EMR implementation as they are the largest user group of EMR systems [ 4 ]. However, findings from this study identified some perceived benefits that have not previously been recognized in the literature. The EMR was particularly well received by early career doctors who had found it easier for documentation, time saving, effective for improving record completeness and beneficial for guiding clinical consultations in highlighting what should be recorded. Clinical staff also felt the EMR enabled senior specialists to act in a more traditional consultant role, with early career doctors completing data entry. By incorporating the EMR into MDT meetings there was a perceived benefit to early career doctors as they had an opportunity to engage more actively with patient records and ask questions about treatment decisions. The central role of informal education opportunities in the training and mentorship of early career doctors has been acknowledged in the literature [ 13 , 14 ]. Furthermore, there literature on health professions education has acknowledged the untapped potential of EMR data and other clinical data sets for supporting high-quality learning environments [ 15 ]. Findings from this study suggest placing an emphasis on these benefits when implementing digital technologies to enhance EMR uptake.
Although findings from this study identified benefits of the EMR, there were also a number of challenges identified which acted as barriers to engagement with the new system. These challenges included feature completeness and reliability, as well as poor communication from IT regarding feasibility of achieving required program features and in addressing EMR issues once implemented. Interestingly, the major challenges implementing the EMR were external to the control of the clinical team implementing the system. The literature has noted that lack of infrastructure and technical supports is a major barrier preventing the harnessing of EMR data by the staff [ 16 ], and for making health data actionable more broadly [ 15 ]. Findings from this study emphasise the enormity of overcoming resourcing and infrastructure challenges if digital technologies are to be effectively implemented and sustained in the health sector. Finally, the challenges faced when successfully implementing an EMR in this study highlight the need for technical and clinical experts to collaborate closely from the outset when developing digital technologies for healthcare, in order for those technologies to capture the complexity of clinical care.
A limitation of this study is that, although all staff at the study site were invited to participate, not all chose to. As a result the perspectives of these staff members might not have been captured in this study. Further, not all participants who participated in pre-implementation interviews chose to participate in post-implementation interviews. As such some of the perspectives of interviewees pre-implementation are not captured in the post-implementation findings.
Digital technologies such as EMRs have great potential to improve the quality, equity and cost of healthcare. However, the health sector is still struggling to implement these technologies in a way that leads to sustained use. Barriers to successful implementation of EMRs include lack of technical support, perceived increase in workload and a learning curve to adequately familiarise with the feature set of the EMR. Enablers of successful implementation include clinician engagement in the design and roll-out of the EMR, and training and upskilling of all end-users of the EMR. Although there may be challenges in the usability of EMRs right after its implementation, staff will be encouraged to use it if they perceive improved features of EMR are imminent and has potential benefit to patient care and workflow. However, it is important that continuous supports are provided to ensure buy-in from health professional is not lost.
Availability of data and materials
The datasets used and/or analysed during the current study are available from the corresponding author on reasonable request.
Abbreviations
Electronic Medical Record/s
Clauser SB, Wagner EH, Bowles EJA, Tuzzio L, Greene SM. Improving modern cancer care through information technology. Am J Prev Med. 2011;40(5):S198–207.
Article Google Scholar
Miller RH, Sim I. Physicians’ use of electronic medical records: barriers and solutions. Health Aff. 2004;23(2):116–26.
Baldwin JL, Singh H, Sittig DF, Giardina TD. Patient portals and health apps: Pitfalls, promises, and what one might learn from the other. Healthcare. 2017: Elsevier;2017:81–5.
Boonstra A, Broekhuis M. Barriers to the acceptance of electronic medical records by physicians from systematic review to taxonomy and interventions. BMC Health Serv Res. 2010;10(1):231.
Kayyali B, Knott D, Van Kuiken S. The big-data revolution in US health care: accelerating value and innovation. Mc Kinsey Co. 2013;2(8):1–13.
Google Scholar
Nguyen L, Bellucci E, Nguyen LT. Electronic health records implementation: an evaluation of information system impact and contingency factors. Int J Med Inform. 2014;83(11):779–96.
McGinn CA, Grenier S, Duplantie J, Shaw N, Sicotte C, Mathieu L, Leduc Y, Légaré F, Gagnon M-P. Comparison of user groups' perspectives of barriers and facilitators to implementing electronic health records: a systematic review. BMC Med. 2011;9(1):46.
Devlin AM, McGee-Lennon M, O’Donnell CA, Bouamrane M-M, Agbakoba R, O’Connor S, Grieve E, Finch T, Wyke S, Watson N. Delivering digital health and well-being at scale: lessons learned during the implementation of the Dallas program in the United Kingdom. J Am Med Inform Assoc. 2016;23(1):48–59.
Murray E, Burns J, May C, Finch T, O'Donnell C, Wallace P, Mair F. Why is it difficult to implement e-health initiatives? A qualitative study. Implement Sci. 2011;6(1):6.
Fleming NS, Culler SD, McCorkle R, Becker ER, Ballard DJ. The financial and nonfinancial costs of implementing electronic health records in primary care practices. Health Aff. 2011;30(3):481–9.
Janssen A, Brunner M, Keep M, Hines M, Nagarajan SV, Kielly-Carroll C, Dennis S, McKeough Z, Shaw T. Interdisciplinary eHealth practice in cancer care: a review of the literature. Int J Environ Res Public Health. 2017;14(11):1289.
Bain E, Bubner T, Ashwood P, Van Ryswyk E, Simmonds L, Reid S, Middleton P, Crowther CA. Barriers and enablers to implementing antenatal magnesium sulphate for fetal neuroprotection guidelines: a study using the theoretical domains framework. BMC Pregnancy Childbirth. 2015;15(1):176.
Cowan F, Flint S. The importance of mentoring for junior doctors. BMJ. 2012;345:e7813.
Fournier M, Tourian L. “A peer mentoring initiative across medical residency programs”. Mental Health and Social Inclusion. 2019;24(1):33–40. https://doi.org/10.1108/MHSI-09-2019-0027 .
Wang YC, DeSalvo K. Timely, granular, and actionable: informatics in the public health 3.0 era. Am J Public Health. 2018;108(7):930–4.
Tolar M, Balka E. Beyond individual patient care: enhanced use of EMR data in a primary care setting. ITCH. 2011;2011:143–7.
Janssen A, Donnelly C, Elder E, Pathmanathan N, Shaw T. Factors Influencing Implementation of an Electronic Medical Record in a Tertiary Cancer Centre. Stud Health Technol Inform. 2019;266:95–100. https://doi.org/10.3233/SHTI190779 .
Download references
Acknowledgements
The authors wish to thank the cancer MDT leaders and members for volunteering their time to participate in the study.
Preliminary results of this study where presented at the Health Informatics Conference 2019 [ 17 ].
All authors listed in this publication conducted the research as part of the salaried position supported by their hiring organisation. No additional funding was provided for this research study.
Author information
Authors and affiliations.
Faculty of Medicine and Health, Charles Perkins Centre, The University of Sydney, Level 2, Charles Perkins Centre D17, Sydney, NSW, Australia
Anna Janssen, Candice Donnelly & Tim Shaw
Sydney West Translational Cancer Research Centre, Westmead Hospital, Sydney, NSW, Australia
Westmead Breast Cancer Institute, Western Sydney Local Health District, Sydney, NSW, Australia
Elisabeth Elder & Nirmala Pathmanathan
You can also search for this author in PubMed Google Scholar
Contributions
Authors AJ and CD wrote where involved in study design, data collection and coordination of manuscript drafting. Authors TS, EE and NP where involved in study design and manuscript drafting. All authors have read and approved the manuscript.
Corresponding author
Correspondence to Anna Janssen .
Ethics declarations
Ethics approval and consent to participate.
Permission to conduct this study was granted by human research ethics committees of the University of Sydney, the Western Sydney Local Health District, and the Nepean Blue Mountains Local Health District. Participants were consented to participate, as per the requirements of the ethics approval. Potential participants were recruited via email to participate in the study. Consent was provided either through return of a signed consent form, or via confirmation in the email response. At the start of each interviewee consent to participate in the interview and consent to record the inter-view was once again confirmed. All authors have read and approved the manuscript.
Consent for publication
Consent for publication is not necessary for this publication.
Competing interests
The authors declare that they have no competing interests.
Additional information
Publisher’s note.
Springer Nature remains neutral with regard to jurisdictional claims in published maps and institutional affiliations.
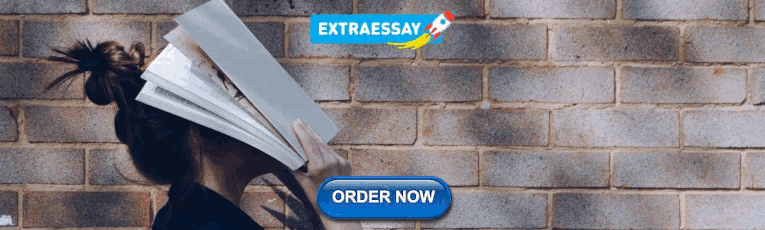
Supplementary Information
Additional file 1., rights and permissions.
Open Access This article is licensed under a Creative Commons Attribution 4.0 International License, which permits use, sharing, adaptation, distribution and reproduction in any medium or format, as long as you give appropriate credit to the original author(s) and the source, provide a link to the Creative Commons licence, and indicate if changes were made. The images or other third party material in this article are included in the article's Creative Commons licence, unless indicated otherwise in a credit line to the material. If material is not included in the article's Creative Commons licence and your intended use is not permitted by statutory regulation or exceeds the permitted use, you will need to obtain permission directly from the copyright holder. To view a copy of this licence, visit http://creativecommons.org/licenses/by/4.0/ . The Creative Commons Public Domain Dedication waiver ( http://creativecommons.org/publicdomain/zero/1.0/ ) applies to the data made available in this article, unless otherwise stated in a credit line to the data.
Reprints and permissions
About this article
Cite this article.
Janssen, A., Donnelly, C., Elder, E. et al. Electronic medical record implementation in tertiary care: factors influencing adoption of an electronic medical record in a cancer centre. BMC Health Serv Res 21 , 23 (2021). https://doi.org/10.1186/s12913-020-06015-6
Download citation
Received : 04 June 2020
Accepted : 13 December 2020
Published : 06 January 2021
DOI : https://doi.org/10.1186/s12913-020-06015-6
Share this article
Anyone you share the following link with will be able to read this content:
Sorry, a shareable link is not currently available for this article.
Provided by the Springer Nature SharedIt content-sharing initiative
- Multidisciplinary care
- Clinical informatics
- Electronic medical records
- Implementation
- Digital health
BMC Health Services Research
ISSN: 1472-6963
- General enquiries: [email protected]
- Alzheimer's disease & dementia
- Arthritis & Rheumatism
- Attention deficit disorders
- Autism spectrum disorders
- Biomedical technology
- Diseases, Conditions, Syndromes
- Endocrinology & Metabolism
- Gastroenterology
- Gerontology & Geriatrics
- Health informatics
- Inflammatory disorders
- Medical economics
- Medical research
- Medications
- Neuroscience
- Obstetrics & gynaecology
- Oncology & Cancer
- Ophthalmology
- Overweight & Obesity
- Parkinson's & Movement disorders
- Psychology & Psychiatry
- Radiology & Imaging
- Sleep disorders
- Sports medicine & Kinesiology
- Vaccination
- Breast cancer
- Cardiovascular disease
- Chronic obstructive pulmonary disease
- Colon cancer
- Coronary artery disease
- Heart attack
- Heart disease
- High blood pressure
- Kidney disease
- Lung cancer
- Multiple sclerosis
- Myocardial infarction
- Ovarian cancer
- Post traumatic stress disorder
- Rheumatoid arthritis
- Schizophrenia
- Skin cancer
- Type 2 diabetes
- Full List »
share this!
March 26, 2024
This article has been reviewed according to Science X's editorial process and policies . Editors have highlighted the following attributes while ensuring the content's credibility:
fact-checked
peer-reviewed publication
trusted source
Optimizing electronic health records: Study reveals improvements in departmental productivity
by Marshall University
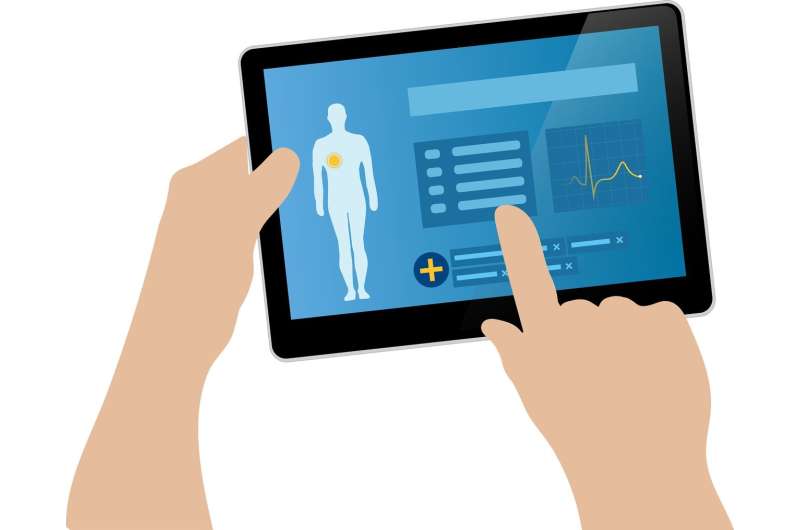
In a study published in the Annals of Family Medicine , researchers at the Marshall University Joan C. Edwards School of Medicine identify transformative effects of electronic health record (EHR) optimization on departmental productivity. With the universal implementation of EHR systems, the study sheds light on the importance of collaborative efforts between clinicians and information technology (IT) experts in maximizing the potential of these digital tools.
The study, led by a team of health care professionals in a family medicine department, embarked on a department-wide EHR optimization initiative in collaboration with IT specialists over a four-month period. Unlike previous efforts that primarily focused on institutional-level successes, this study delved deep into the intricacies of EHR interface development and its impact on clinical workflow.
"There has been a longstanding disconnect between EHR developers and end-users, resulting in interfaces that often fail to capture the intricacies of clinical workflows," said Adam M. Franks, M.D., interim chair of family and community health at the Joan C. Edwards School of Medicine and lead researcher on the study. "Our study aimed to bridge this gap and demonstrate the tangible benefits of collaborative optimization efforts."
The methodology involved an intensive quality improvement process engaging clinicians and clinical staff at all levels. Four categories of optimizations emerged: accommodations (adjustments made by the department to fit EHR workflows); creations (novel workflows developed by IT); discoveries (previously unnoticed workflows within the EHR); and modifications (changes made by IT to existing workflows).
Key findings from the study showed significant improvements in productivity: The optimization efforts led to remarkable enhancements in departmental productivity. Monthly charges increased from 0.74 to 1.28, while payments surged from 0.83 to 1.58. Although monthly visit ratios also increased from 0.65 to 0.98, the change was not statistically significant.
The study also revealed that a significant number of solutions to EHR usability issues were already embedded within the system, emphasizing the need for thorough exploration and understanding of existing workflows.
Finally, accommodation optimizations underscored the necessity for better collaboration between EHR developers and end-users before implementation, highlighting the potential for more user-centric design approaches.
"Our study not only demonstrates the efficacy of departmental collaboration with IT for EHR optimization but also underscores the importance of detailed workflow analysis in enhancing productivity," Franks said.
The research provides valuable insights for health care institutions aiming to maximize the potential of their EHR systems, with implications for improving patient care, efficiency and overall organizational performance.
In addition to Franks, co-authors on the study included Charles Clements, M.D., Tammy Bannister, M.D., Adrienne Mays-Kingston, M.D., Ashley Beaty, MSN, RN-BC, Alperen Korkmaz, M.Ed., John A. Parker, M.D., and Stephen M. Petrany, M.D.
Explore further
Feedback to editors

Researchers produce grafts that replicate the human ear
17 hours ago

An infamous 'inflammasome'—a rogue protein complex—appears to underlie a rare and disabling autoimmune disorder
Mar 29, 2024

Researchers discover skin biomarkers in infants that predict early development of food allergies

Veterans help provide greater insight into Klinefelter and Jacobs syndromes

High-resolution images reveal similarities in protein structures between Alzheimer's disease and Down syndrome

How blocking a neural receptor responsible for addiction could reduce alcohol use

Study finds few hospitals promoting potentially predatory medical payment products

COVID-19 research: Study reveals new details about potentially deadly inflammation

Enhanced melanoma vaccine offers improved survival for men

How music choices can affect productivity
Related stories.

New workflow tool tackles modelling hurdles of data, scenario and collaboration management
Dec 10, 2021

AI-generated responses to patient portal messages are feasible, usable
Mar 25, 2024

Closing the referral loop: Perspectives and experiences of primary care and specialist physicians
Mar 4, 2024

Promise of mobile technology provided to hospitalists not impacting patient care
Jan 4, 2022

New study shows daily, consistent parental reading in the first year of life improves infants' language scores
Feb 14, 2023

Small primary care practices that received training in quality measures to enhance care improved patient outcomes
Nov 9, 2021
Recommended for you

Prescribing alcohol use disorder medications upon discharge from alcohol-related hospitalizations works

Researchers demonstrate technique for identifying single cancer cells in blood for the first time

Private and secure generative AI tool supports operations and research in a cancer center

Artificial Intelligence tool successfully predicts fatal heart rhythm
Mar 28, 2024

Predicting infection risk in childhood cancer

AI-driven attention mechanisms aid in streamlining cancer pathology reporting
Let us know if there is a problem with our content.
Use this form if you have come across a typo, inaccuracy or would like to send an edit request for the content on this page. For general inquiries, please use our contact form . For general feedback, use the public comments section below (please adhere to guidelines ).
Please select the most appropriate category to facilitate processing of your request
Thank you for taking time to provide your feedback to the editors.
Your feedback is important to us. However, we do not guarantee individual replies due to the high volume of messages.
E-mail the story
Your email address is used only to let the recipient know who sent the email. Neither your address nor the recipient's address will be used for any other purpose. The information you enter will appear in your e-mail message and is not retained by Medical Xpress in any form.
Newsletter sign up
Get weekly and/or daily updates delivered to your inbox. You can unsubscribe at any time and we'll never share your details to third parties.
More information Privacy policy
Donate and enjoy an ad-free experience
We keep our content available to everyone. Consider supporting Science X's mission by getting a premium account.
E-mail newsletter
- Article Information
eAppendix. Questions and Response Options in the 2019 National Electronic Health Records Survey
See More About
Select your interests.
Customize your JAMA Network experience by selecting one or more topics from the list below.
- Academic Medicine
- Acid Base, Electrolytes, Fluids
- Allergy and Clinical Immunology
- American Indian or Alaska Natives
- Anesthesiology
- Anticoagulation
- Art and Images in Psychiatry
- Artificial Intelligence
- Assisted Reproduction
- Bleeding and Transfusion
- Caring for the Critically Ill Patient
- Challenges in Clinical Electrocardiography
- Climate and Health
- Climate Change
- Clinical Challenge
- Clinical Decision Support
- Clinical Implications of Basic Neuroscience
- Clinical Pharmacy and Pharmacology
- Complementary and Alternative Medicine
- Consensus Statements
- Coronavirus (COVID-19)
- Critical Care Medicine
- Cultural Competency
- Dental Medicine
- Dermatology
- Diabetes and Endocrinology
- Diagnostic Test Interpretation
- Drug Development
- Electronic Health Records
- Emergency Medicine
- End of Life, Hospice, Palliative Care
- Environmental Health
- Equity, Diversity, and Inclusion
- Facial Plastic Surgery
- Gastroenterology and Hepatology
- Genetics and Genomics
- Genomics and Precision Health
- Global Health
- Guide to Statistics and Methods
- Hair Disorders
- Health Care Delivery Models
- Health Care Economics, Insurance, Payment
- Health Care Quality
- Health Care Reform
- Health Care Safety
- Health Care Workforce
- Health Disparities
- Health Inequities
- Health Policy
- Health Systems Science
- History of Medicine
- Hypertension
- Images in Neurology
- Implementation Science
- Infectious Diseases
- Innovations in Health Care Delivery
- JAMA Infographic
- Law and Medicine
- Leading Change
- Less is More
- LGBTQIA Medicine
- Lifestyle Behaviors
- Medical Coding
- Medical Devices and Equipment
- Medical Education
- Medical Education and Training
- Medical Journals and Publishing
- Mobile Health and Telemedicine
- Narrative Medicine
- Neuroscience and Psychiatry
- Notable Notes
- Nutrition, Obesity, Exercise
- Obstetrics and Gynecology
- Occupational Health
- Ophthalmology
- Orthopedics
- Otolaryngology
- Pain Medicine
- Palliative Care
- Pathology and Laboratory Medicine
- Patient Care
- Patient Information
- Performance Improvement
- Performance Measures
- Perioperative Care and Consultation
- Pharmacoeconomics
- Pharmacoepidemiology
- Pharmacogenetics
- Pharmacy and Clinical Pharmacology
- Physical Medicine and Rehabilitation
- Physical Therapy
- Physician Leadership
- Population Health
- Primary Care
- Professional Well-being
- Professionalism
- Psychiatry and Behavioral Health
- Public Health
- Pulmonary Medicine
- Regulatory Agencies
- Reproductive Health
- Research, Methods, Statistics
- Resuscitation
- Rheumatology
- Risk Management
- Scientific Discovery and the Future of Medicine
- Shared Decision Making and Communication
- Sleep Medicine
- Sports Medicine
- Stem Cell Transplantation
- Substance Use and Addiction Medicine
- Surgical Innovation
- Surgical Pearls
- Teachable Moment
- Technology and Finance
- The Art of JAMA
- The Arts and Medicine
- The Rational Clinical Examination
- Tobacco and e-Cigarettes
- Translational Medicine
- Trauma and Injury
- Treatment Adherence
- Ultrasonography
- Users' Guide to the Medical Literature
- Vaccination
- Venous Thromboembolism
- Veterans Health
- Women's Health
- Workflow and Process
- Wound Care, Infection, Healing
Others Also Liked
- Download PDF
- X Facebook More LinkedIn
Gaffney A , Woolhandler S , Cai C, et al. Medical Documentation Burden Among US Office-Based Physicians in 2019 : A National Study . JAMA Intern Med. 2022;182(5):564–566. doi:10.1001/jamainternmed.2022.0372
Manage citations:
© 2024
- Permissions
Medical Documentation Burden Among US Office-Based Physicians in 2019 : A National Study
- 1 Department of Medicine, Cambridge Health Alliance, Cambridge, Massachusetts
- 2 Harvard Medical School, Boston, Massachusetts
- 3 Hunter College, City University of New York, New York
- 4 Public Citizen Health Research Group, Washington, District of Columbia
- 5 Department of Medicine, Brigham and Women’s Hospital, Boston, Massachusetts
Many physicians perceive that medical documentation is onerous, detracts from face time with patients, and drives burnout. 1 , 2 We assessed the burden of medical documentation on US office-based physicians.
In this cross-sectional study, we analyzed office-based physician responses to the 2019 National Electronic Health Records Survey, which collects nationally representative data on the use and burdens of the electronic health record (EHR). 3 The overall participation rate was 37.7%. 3 The Cambridge Health Alliance Institutional Review Board deemed this analysis of publicly available, deidentified data exempt from review. Respondents provided informed consent at the time of data collection. Reporting followed the STROBE reporting guideline.
We evaluated the time physicians devoted to documentation outside office hours based on the question, “On average, how many hours per day do you spend outside of normal office hours documenting clinical care in your medical record system?” (none, <1, 1-2, >2-4, or >4 hours). We estimated mean daily hours devoted to documentation outside office hours by recoding categorical responses at the midpoint (for the highest category, the minimum) of response. We also explored 5 measures of satisfaction with the EHR and time spent documenting. For the complete question text and an explanation of how some response categories were combined for this analysis, see the eAppendix in the Supplement . We assessed the proportion of physicians with each outcome stratified by specialty and then the correlation among 3 physician and practice characteristics and mean documentation time outside office hours: (1) EHR use; (2) staff support for documentation (eg, scribes); and (3) participation in an Accountable Care Organization or pay-for-performance program (hereafter, value-based purchasing [VBP] participation).
Physician responses on satisfaction with documentation are summarized as weighted percentages and time spent on documentation as means with 95% CIs. Analyses were performed using Stata SE, version 16.1 (StataCorp LLC) survey procedures module. Two-sided P < .05 indicated statistical significance.
The 1524 respondents (78.6% aged ≥50 years; 69.9% men) represented 301 603 physicians nationwide. Across primary care, surgical, and medical specialties, 64.1% of respondents reported satisfaction with the EHR; 64.5% found documentation to be easy ( Table 1 ). However, 58.1% disagreed that the time spent documenting was appropriate and did not reduce time spent with patients, and 84.7% agreed that documentation solely for billing purposes increased total documentation time. Differences by physician specialty were not significant.
Overall, physicians spent a mean of 1.77 (95% CI, 1.67-1.87) hours daily completing documentation outside office hours ( Table 2 ). Differences by staff support for documentation (eg, scribes) were small and nonsignificant. Physicians who used EHRs spent a mean of 1.84 (95% CI, 1.74-1.94) h/d documenting outside office hours, significantly more than the 1.10 (95% CI, 0.83-1.37) h/d among those who did not use EHRs ( P < .001). Physicians participating in VBP spent 2.02 (95% CI, 1.86-2.19) h/d on documentation outside office hours vs 1.58 (95% CI, 1.47-1.69) h/d among those who did not ( P < .001). Assuming a 5-day work week and 47-week work year, 4 we estimated US physicians spent 125 million hours documenting outside office hours in 2019.
This cross-sectional study reveals that documentation burdens US physicians. Most respondents indicated that documentation time is inappropriate and takes time from patients.
The large majority of US physicians reported that billing-related tasks exacerbate their documentation burden, which suggests that payment strategies specific to the US, vs to EHRs per se, account for some of the overload. 5 Relative to EHR users in other nations, US physicians spend more time documenting in the EHR, 5 with outpatient notes approximately 4 times longer. 1
Per our analysis, VBP participation is associated with a higher documentation burden, a finding consonant with the doubling in length of EHR ambulatory notes in the VBP era (among EHR users). 1 Surprisingly, we did not find that scribe use was associated with less overall documentation time; however, this does not address whether scribe users saw more patients.
A limitation of our study is that data were cross-sectional, precluding firm causal inference. Moreover, all data were self-reported by physicians, and the survey did not define documentation . 3 Reassuringly, our estimate of time spent documenting outside office hours is comparable with some previous estimates based on other approaches. 6 Reconsideration of the US approach to medical documentation may be warranted.
Accepted for Publication: January 30, 2022.
Published Online: March 28, 2022. doi:10.1001/jamainternmed.2022.0372
Corresponding Author: Adam Gaffney, MD, MPH, Cambridge Health Alliance/Harvard Medical School, Pulmonary and Critical Care Medicine, 1943 Cambridge St, Cambridge, MA 02139 ( [email protected] ).
Author Contributions: Dr Gaffney had full access to all of the data in the study and takes responsibility for the integrity of the data and the accuracy of the data analysis.
Concept and design: Gaffney, Woolhandler, Bor, McCormick, D. U. Himmelstein.
Acquisition, analysis, or interpretation of data: Gaffney, Cai, J. Himmelstein, McCormick, D. U. Himmelstein.
Drafting of the manuscript: Gaffney.
Critical revision of the manuscript for important intellectual content: All authors.
Statistical analysis: Gaffney, Woolhandler, D. U. Himmelstein.
Obtained funding: Bor.
Administrative, technical, or material support: Bor.
Supervision: Woolhandler, D. U. Himmelstein.
Conflict of Interest Disclosures: Dr Gaffney reported serving as a leader of the Physicians for a National Health Program (PNHP), a nonprofit organization that favors coverage expansion through a single-payer program; he has not received compensation from PNHP, but some of his travel on behalf of the organization was previously reimbursed by it. His spouse is an employee of Treatment Action Group, a nonprofit research and policy think tank focused on HIV, tuberculosis, and hepatitis C treatment. Dr Woolhandler served as an unpaid policy adviser to Senators Bernie Sanders and Elizabeth Warren and cofounded PNHP but receives no financial compensation from the organization. Dr Cai reported receiving personal fees from the US House of Representatives, Office of Pramila Jayapal, as a summer health policy fellow and nonfinancial support from PNHP as a summer research intern and board member outside the submitted work. Dr J. Himmelstein reported receiving grants from the Institutional National Research Service outside the submitted work. No other disclosures were reported.
- Register for email alerts with links to free full-text articles
- Access PDFs of free articles
- Manage your interests
- Save searches and receive search alerts

An official website of the United States government
The .gov means it’s official. Federal government websites often end in .gov or .mil. Before sharing sensitive information, make sure you’re on a federal government site.
The site is secure. The https:// ensures that you are connecting to the official website and that any information you provide is encrypted and transmitted securely.
- Publications
- Account settings
Preview improvements coming to the PMC website in October 2024. Learn More or Try it out now .
- Advanced Search
- Journal List
- Yearb Med Inform
- (Suppl 1); 2016

Electronic Health Records: Then, Now, and in the Future
Describe the state of Electronic Health Records (EHRs) in 1992 and their evolution by 2015 and where EHRs are expected to be in 25 years. Further to discuss the expectations for EHRs in 1992 and explore which of them were realized and what events accelerated or disrupted/derailed how EHRs evolved.
Literature search based on “Electronic Health Record”, “Medical Record”, and “Medical Chart” using Medline, Google, Wikipedia Medical, and Cochrane Libraries resulted in an initial review of 2,356 abstracts and other information in papers and books. Additional papers and books were identified through the review of references cited in the initial review.
By 1992, hardware had become more affordable, powerful, and compact and the use of personal computers, local area networks, and the Internet provided faster and easier access to medical information. EHRs were initially developed and used at academic medical facilities but since most have been replaced by large vendor EHRs. While EHR use has increased and clinicians are being prepared to practice in an EHR-mediated world, technical issues have been overshadowed by procedural, professional, social, political, and especially ethical issues as well as the need for compliance with standards and information security. There have been enormous advancements that have taken place, but many of the early expectations for EHRs have not been realized and current EHRs still do not meet the needs of today’s rapidly changing healthcare environment.
The current use of EHRs initiated by new technology would have been hard to foresee. Current and new EHR technology will help to provide international standards for interoperable applications that use health, social, economic, behavioral, and environmental data to communicate, interpret, and act intelligently upon complex healthcare information to foster precision medicine and a learning health system.
Introduction
Translation of ancient Egyptian hieroglyphic inscriptions and papyri from 1,600-3,000 BC indicate the use of medical records. However, paper medical records were not steadily used until 1900-1920. Medical record, medical chart, and health record are different terms used to describe the documentation of a patient’s medical history and care. In recent years, the term health record has received prominence based on the fact that a patient’s medical information should include health and lifestyle information beyond just episodic medical encounters. Traditionally, health records were written on paper, maintained in folders divided into sections based on the type of note, and only one copy was available. New computer technology developed in the 1960s and 1970s laid the foundation for the development of the Electronic Heath Record (EHR). The use of EHRs has not only made patients’ medical information easier to read and available from almost any location in the world, but also changed the format of health records, and thus changed health care.
Thousands of published studies report on EHRs increasing use, clinical decision support’s (CDS) ability to improve or not improve the healthcare process or clinical outcomes, evaluation methods, implementation/adoption, clinical trial patient identification, numerous new applications, and unintended consequences. Information on specific studies is beyond the scope of this paper and not included. For the 25 th anniversary of the Yearbook of the International Medical Informatics Association (IMIA), this paper focuses on the overall state and use of EHRs in 1992 and how they have evolved by 2015. This paper also discusses the expectations for EHRs in 1992 and which of these were realized, what events accelerated or disrupted/derailed EHR evolution. Finally, we will discuss the expected state of EHRs in the 25 years.
State of EHRs in 1992
Initially, EHRs were developed and used at a number of academic inpatient and outpatient medical facilities [ 1-10 ], but none contained all the information in the paper chart and most EHRs today are still a hybrid collection of computerized and paper data [ 11 , 12 ] ( Table 1 ). Some EHRs developed between 1971 and 1992 were developed with hierarchical or relational databases, around or added to hospital billing and scheduling systems while others such as COSTAR, PROMIS, TMR, and HELP were developed as clinical systems to help improve medical care and for use in medical research [ 3 , 13-15 ]. While some EHRs were developed on minicomputers, most were initially developed on large mainframe computers and in either case had limited storage, which required the use of removable disk packs and/or tape for extra data storage, nightly downtimes for database back-up, and dedicated/wired terminals. Only a few early EHRs allowed physician entry of orders, prescriptions, and notes and data entry was through keyboards focused mostly on laboratory and medication review [ 16 ]. While usually hospital-based, many of the early EHRs had features and functionalities that are still used and important today.
Capabilities and use of EHRs in 1992 and 2015
As inadequacies of the paper record became increasingly more apparent in 1992 [ 17 ], the Institute of Medicine advocated a shift from a paper-based to an electronic medical record [ 12 ]. However, the widespread use of EHRs was delayed by high costs, data entry errors, poor initial physicians’ acceptance, and lack of any real incentive. The goal of replacing the entire paper chart with an electronic record was considered problematic due to the large initial costs resulting in the view that only key data should be computerized. As a result, the EHR would complement and not replace the paper record [ 18 ]. Others also felt the costs to implement EHRs for general practices would outweigh any gains in efficiency, which also could be achieved through better use of paper records [ 19 ].
In the late 1980’s and early 1990’s, hardware became more affordable, powerful, and compact and the use of personal computers, local area networks, and the Internet provided faster and easier access to medical information and initiated the use of web-based EHRs [ 20 ]. The personal computer provided a mouse interface which made use easier with pull-down menus, pop-up lists, buttons, multi-page forms, and scrolling fields. Other features included help screens, control functions, audit trails, and export of data to statistical packages for analyses. An initial obstacle for EHR ease of use was the need for portable computers [ 21 ] and soon computers were being classified as laptop, palmtop, notebook, and pen-based [ 22 ].
EHR Use in 1992
Early use of EHRs also included data interchange for claims processing [ 23 ] and image scanning as a method for document capture [ 24 ]. Each of these efforts saved time by eliminating filing and retrieval of charts, photocopying, and chart location control [ 25 ]. More clinical use began when the physician workstation became the term used for personal computers integrated with EHRs that allowed access to physician notes, orders, consults, laboratory results, radiological studies, direct patient measurements, nursing assessments and notes, and patient care procedures. Workstations interfaced with tools such as drug references, clinical manuals, textbooks of medicine, literature search engines, CDS, and electronic communication [ 26 , 27 ]. Data also could be represented in a variety of graphical formats which especially facilitated the management of critically ill patients. While not widespread, new applications and functionalities were being developed and used. For example, physicians began to use electronic documentation but many did not believe that computerization saved time, although they appreciated its value for administrative functions and for producing printouts [ 28 ]. Networks of microcomputer workstations were used to write all inpatient orders linked to an EHR. While this significantly lowered patient charges and hospital costs, the systems required more physician time than did the paper charts [ 29 ]. Likewise, initial attempts at nurse charting failed because they required time-consuming manual data entry. Automated management of patient records became available through the development of patient data management systems which could be connected to bedside monitoring devices to record and interpret patient data in the EHR [ 30 ]. Some specific areas such as admitting, pharmacy, laboratory, surgery, radiology, respiratory therapy, and infectious diseases were either interfaced with or completely developed within EHRs [ 31 ]. However, laws still required hospitals and practitioners to be accountable for the accuracy and completeness of medical records and thus all documents had to be reviewed and signed. While regulatory and accrediting agencies restricted the auto-authentication of medical records, electronic signatures could and were being used within EHRs [ 32 ].
Soon, the use of EHRs produced massive amounts of health care data which were found to be valuable for epidemiologic review. However, secondary use of EHR data soon elucidated issues with the quality of these data for research and evaluation. Use of data for purposes other than those for which the data was collected showed that poor quality data often led to significant misinformation and potential patient harm [ 33 ].
Clinical Decision Support
It was recognized that medical information in EHRs could be used for CDS which generated a new major domain of medical informatics. Knowledge bases began to be designed as separate databases and used within EHRs. Some academic EHRs included knowledge bases to feed CDS functions while few of the commercial systems did by 1992 [ 12 , 34 , 35 ]. Early CDS included drug-allergy, drug-drug, and abnormal laboratory test results while other areas were being covered as well [ 36 , 37 ]. As an effort to influence physician decisions, CDS was built within Physician Order Entry (POE) [ 38 ] and required physicians to respond to computer-generated reminders to improve their compliance with preventive care protocols [ 39 ]. The benefit of sharing Medical Logic Modules (MLMs) was being tried in order to reduce efforts by avoiding that each group replicates what others had developed and evaluated. The Arden syntax [ 40 ] was an initial effort to show that CDS rules could be shared between different hospital EHRs with different databases and data dictionaries. Thus, in order to use data for CDS, numerous efforts to code data were emphasized and the use of “free-text” data was avoided whenever possible.
The Need for Standards
The increase of third party applications being used within EHRs required additional and specific interfaces to be developed. It soon became obvious that standards were needed. By 1992, Health Level Seven (HL7) [ 41 ] and IEEE P1157 [ 42 ] were being used as the main interface standards by homegrown EHRs and vendors to interface with other systems. The advantage of this approach was to reduce the ambiguity in data element definitions. As a number of EHRs and other applications began to be interfaced, the HL7 standard was refined and expanded into additional domains and became the most practical solution to aggregate ancillary systems like laboratory, microbiology, electrocardiogram, echocardiography, and other results into a central EHR [ 43 ]. The need for a consistent message format or syntax was recognized as only part of the problem. The need to use standardized dictionary codes (semantics) was spear headed by the National Library of Medicine and the development of the Universal Medical Language System (UMLS) reminding us today that computer systems come and go, but data last forever [ 44 ].
Data from medical devices such as ventilators, bedside monitors, and infusion pumps are critical for patient care. Manual entry of sporadic medical device data did not meet the needs of clinicians. The IEEE P1073 Medical Information Bus (MIB) standard developed to facilitate the interfacing with any type of medical device was only used by a small number of facilities due to the limited participation by medical device vendors [ 45 ]. However, Picture Archiving and Communication Systems (PACS) were being successfully used and found to improve the access, storage, and transfer of digital images [ 46 ].
Data Exchange and Networks
Data ownership and privacy became common issues whenever data storage and data exchange through computer networks were planned [ 19 ]. Despite these challenges, a number of community health information networks were in the developmental stages or at varying degrees of operability [ 47 ], and Singapore developed a national computer network for the medical and health community [ 48 ]. However, the technology raised several important ethical problems including data control and patient dignity along with other important ethical matters requiring solutions including data ownership, data liability, informed consent to use and retrieve, security, and access [ 49 ]. Other extensive views of EHR capabilities, functionalities, and use in 1992 can be found in The Computer-Based Patient Record: An Essential Technology for Health Care, Revised Edition [ 12 ] and in the paper by Reed Gardner on the past and future of clinical information systems in this anniversary edition of the Yearbook [ 50 ].
EHR Use and Evolution by 2015
Large health care organizations and government agencies are recognizing the value of information in EHRs to determine optimal patterns of care [ 51 ]. However, growing issues facing healthcare coverage, privacy, and especially the security of EHRs remain crucial obstacles for their acceptance. Patients, providers, and healthcare facilities continue to demand assurance that these records are securely protected [ 52 , 53 ]. Thus, as EHR use has increased over time, technical issues continued to be overshadowed by procedural, professional, social, political, and especially ethical issues and the need for compliance with standards and information security [ 54 ].
A 2004 random sample of healthcare facilities from across the U.S. found that 13% of respondents had an EHR system fully implemented while 10% did not have or did not plan to have an EHR system. The majority of respondents (62%) used a vendor EHR system and most were not aware or slightly aware of the ASTM E1384 standards for the minimum data elements that should be included in all EHR systems [ 55 ]. Some healthcare facilities used a single EHR for nearly all care they provided while others used EHRs from more than one vendor or a homegrown EHR [ 56 ].
The number of academic institution developed EHRs in use have declined and been replaced by large vendor EHRs since 1992 [ 57 ]. However, the number of EHR-related articles published by academic institutions has significantly increased. From 1991-2005, most articles were published in English and were from North America, followed by the UK, Switzerland, the Netherlands, and Norway [ 58 ]. Since 2005, EHR innovation and development and published articles have steadily increased from most European countries, Australia, and Asia, including the establishment of an IMIA journal solely dedicated to applied clinical informatics [ 59 , 60 ].
Most EHRs are now web/client-server-based, use relational databases, data access and entry screens are navigated using mouselike scrolling and pointer devices. In the U.S., the increase in EHR adoption was stimulated by the 2009 stimulus plan’s Meaningful Use initiative. Electronically sharing medical information from one facility to another has become more frequent and many medical organizations have implemented EHRs and health information exchange (HIE) networks [ 61 ]. The Veterans Administration in the U.S. Health Exchange has advanced HIE interoperability standards and patient consent policies [ 62 ]. In an effort to reach the same goal, the Canada Health Infoway initiative helped develop interoperable EHRs nationwide [ 63 ]. Australia launched a personally controlled EHR designed around the needs of consumers [ 64 ]. Estonia implemented a nationwide electronic health record system which gives full access to its citizens [ 65 ]. EHRs are implemented and used in ambulatory care in sub-Saharan Africa [ 66 ]; and Hong Kong’s web-based electronic patient record system allows integrated, real time patient-based information to be shared in clinics, and private and public hospitals [ 67 ]. To exchange a common set of data elements, a common terminology such as SNOMED and LOINC, common data structures, and a common transport standard, the HL7 Clinical Document Architecture (CDA) and The American Society for Testing and Materials (ASTM International) Continuity of Care Record (CCR) are being used [ 68 ]. Today, the ASTM CCR is usually viewed within an HL7 message. Remote follow-up of implanted medical devices can be made through data exchanged via an HL7/XML communication protocol to a patient’s clinical information stored in the local EHR [ 69 , 70 ].
New Functionality and Use
EHR records are now being created, used, edited, and viewed by multiple independent entities including primary care physicians, hospitals, insurance companies, and patients. EHRs are increasingly being used in primary-care exam rooms to document and access patients’ records along with online medical information and decision-making tools, and prescribe medications. They have changed the dynamics of the patient-clinician interaction through clinician-patient email, virtual consults, and telemedicine [ 71-75 ].
Additional parts of the paper chart continue to be incorporated into the EHR since 1992. Digital pathology includes the information technology that allows for the management of data and images [ 76 , 77 ]. Likewise, results from laboratories that are external to the health care enterprise are being integrated into the EHR [ 78 ]. Providers are now using order sets, voice recognition, barcodes, and documentation templates to directly enter information into the EHR and decision support rules along with computerized provider order entry (CPOE) are used daily [ 79 , 81 ]. Mobile devices with high-resolution cameras are also used by clinicians to capture images from the bedside and insert them into the EHR [ 82 ]. Medication ordering is now coupled with CDS and includes e-prescribing interfaces to local pharmacies [ 83 ]. The inclusion of mental and behavioral health data in the EHR has significantly improved the quality of medical care and increased the use of medical services among patients [ 84 , 85 ]. However, there is mounting pressure to decrease or remove the need for a patient’s consent to access their medical information. Mental health information is sensitive and potentially damaging if privacy is breached and can result in patients being reluctant to seek treatment if they cannot be assured of confidentiality [ 86 ]. Access to behavioral health data becomes even more complicated due to variations in states, countries, and international laws.
While more information is being stored in EHRs and shared with multiple facilities, EHRs are now also being used in nursing homes. This additional environment has been a challenge due to the multiplicity of different providers in a home, the physical structure of the facilities, multiple facility organizations, and high costs of implementation and maintenance. However, EHRs in nursing homes have improved communication between providers, consultants, hospital, and nursing home staff [ 87 ], and nursing homes are increasingly introducing EHRs into nursing practice [ 88 ]. Also, EHRs are being used by home health or hospice care [ 89 ], organizations that provide care to the homeless population [ 90 ], and departments of corrections [ 91 ].
The increased demand by patients to access their healthcare data has led to more personal use of the EHR [ 92 ]. Personal Health Records (PHRs) are now interfaced with applications within EHRs and are used by most large facilities and vendors [ 93 ]. Many patients are taking a more active role in managing their medical data which is essential for patient-centered care [ 94 ]. Patient-centered-care allows patients to add personal stories of key life events both medical and non-medical that enable clinicians to better understand what matters and is important to patients, and what are their personal health goals and care preferences [ 95 , 96 ]. Recent reports have highlighted the need for efforts to better understand the collection and use of this information in the EHR [ 97 ] and there is a lack of consensus on how PHR success will be determined [ 98 , 99 ]. There are no comprehensive laws or procedures regarding patients’ access rights to EHRs [ 100 ]. Another problem may be that the use of PHRs may be challenging for some older adults or those of lower socioeconomic status and with low health literacy [ 101 ].
Family health histories are also being entered into EHRs. They are used to assess disease risk and offer insight into the interplay between inherited and social factors relevant to patient care [ 102 ]. Additionally, the human genome has now been decoded and whole-exome and whole-genome sequencing results are now being stored in the EHR [ 103 , 104 ]. DNA results can be provided to the practitioner, used for CDS, and collected data in the EHR serve as a source of phenotypic information for analytic purposes [ 105 ]. Together, these data sources make the EHR the paramount source of phenotypic information and genomic research [ 106 ]. However, there remain challenges in extracting phenotypic data that sometimes need to be inferred from multiple sources. But, recent work has made progress in translating “omic” data into patient care and CDS using pharmacogenomics data [ 107 , 108 ]. Biobanks are now linked to personal and family health information in EHRs to accurately identify subjects with specific diseases and phenotypes and to identify genotype-phenotype associations [ 109 ].
In addition to patient and family health benefits, public health has found increased value in EHRs. Biosurveillance systems are linked to EHRs to detect events of public health significance [ 110 ]. Adapting EHR systems to serve public health needs provides the possibility of enormous advances in public health practice and policy [ 111 ]. Distributed networks for public health surveillance allow public health to initiate custom queries against participating EHRs while the data remain behind each practice’s firewall [ 112 ].
CDS applications continue to be promoted as one of the key features of EHRs. Diagnostic decision support systems were some of the earliest examples of medical informatics innovations. Computer-based diagnostic systems are available commercially and studies have shown that these computer programs have strengths and limitations [ 113 , 114 ]. In addition, a comprehensive taxonomy of front-end CDS tools has been developed and validated. A subsequent survey of commercial EHR vendors and leading healthcare institutions revealed a small core set of common CDS tools, but identified significant variability in the remainder of CDS content [ 115 ].
Modern patient care depends on a number of different medical devices in order to monitor a patient’s vital signs, infuse medications, and maintain life support. Data from medical devices such as ventilators, infusion pumps, bedside and portable monitors, and others are interfaced to EHRs through custom interface applications [ 116 , 117 ]. Not only is this data valuable for a patient’s EHR, but it is essential for CDS applications to help prevent patient harm. Integrated Development Environments are now used along with new programming languages to facilitate and accelerate the development of CDS and other applications. A comprehensive discussion of current CDS capabilities can be found in Greenes’s “Clinical Decision Support” [ 118 ].
The increased amount of patient information stored in the EHR continues to include a large variety of free-text documents. This spawned the need for the use of Natural Language Processing (NLP) to read and code free-text data which has become common within most EHRs [ 119-121 ]. NLP and inference rules have acceptable performance for understanding and finding previously hidden information in dictated documents and especially for adding problems to the problem list [ 122 ].
The growing amount and availability of EHR data also present new opportunities for discovering new knowledge about diseases. In the past decade, there has been an increase in big- data and text mining focused on the identification of disease associations [ 123 ]. Big-data offers the promise of large-scale EHR data analysis of outcomes, patterns, temporal trends, and correlations. Many think the evolution of Big Data analytics will use EHR data and move us from description and reporting diseases to forecasting, predictive modeling, and decision optimization [ 124 ].
However, EHRs are often considered as incomplete. In 2011, fewer than 5% of anesthesia departments used an EMR that was anesthesia specific [ 125 , 126 ], primary care functions frequently remained unsupported [ 127 ], and pediatricians reported the absence of pediatric data and functions in EHRs [ 128-130 ]. Not all available patient information is entered due to physicians’ and other providers’ complaints about increased workload and the inflexibility of EHRs and CPOE [ 131 ]. These complaints spawned the use of medical scribes, who are trained medical information managers specialized in charting physician encounters in real-time and assisting in the navigation of the EHR [ 132 ]. Implementation also continues to be limited in rural areas due to financial barriers [ 133 ]. Open source technology is becoming a solution to overcome the problems of high-cost and inflexibility associated with the proprietary EHRs. Open source EHRs are being used in a number of countries across the world and mainly in Sub-Saharan Africa and South America [ 134-137 ]. While open source EHRs may have limited capabilities currently, they offer a better option than paper and continue to improve their capabilities, functionalities, and user acceptance [ 138 ].
Still, healthcare providers are under increasing pressure to enable widespread access to their EHRs for the patients they serve. The meaningful use incentive program in the U.S. has been a significant driver encouraging this access. Elsewhere, the cloud has become extremely efficient and successful at establishing digital identities for individuals and making EHRs interoperable across heterogeneous systems. As the healthcare industry contemplates providing patients more access to their EHRs, the solution could leverage existing cloud technology [ 139 , 140 ].
EHR data generated in the care of patients are also widely used to support clinical research and quality improvement [ 141 ]. The enormous amount of data being collected by EHRs has generated additional value when integrated and stored in enterprise data warehouses (EDWs). The EDW allows all data from organizations with numerous inpatient and outpatient facilities to be integrated and analyzed [ 142 ]. These data are not only an essential tool for management and strategic decision making, but also for enhanced data exploration, cohort identification, population management, and patient specific CDS. Patient data that was previously stored on removable disk packs or tape are now stored online; birth to death.
Implementation and Value
As stated, this paper focuses on the overall state and use of EHRs in 1992 and their evolution as of 2015. Some of the functionality and use described resulted in common use and opened new domains for most EHRs that have become standard while others may have only been reported in a single paper and later found to have little value or were never implemented outside the original facility. Perceived value and use are often dependent on the workflow of a medical specialty or facility. Thus, published work describing EHR capabilities was included, although it was beyond the scope of this paper, to comment directly on value or sustainability for each case. Further, an unsuccessful initial development may be used to identify new and useful functionality for future improvement.
Addressing Hurdles to Optimal Use of the EHR
Some healthcare professionals continue to develop workarounds and rely on paper alternatives rather than using EHRs [ 143 ]. Understanding of the reasons why EHR functionalities vary and why there is a need for workarounds remains important to facilitate user-centered design and create needed education for EHR use [ 144 , 145 ]. There also continues to be discussion on the right balance between information overload and the right-and helpful-information [ 146 ] including the need for restrictions on the use of copy and paste to limit the growing challenge of ‘note bloat’ [ 147 ]. Moreover, many studies have demonstrated unintended negative consequences of the use of EHRs on the quality of care [ 148-151 ]. Research on the application of human factors to the design and development of EHRs has been used to improve their use and prevent unintentional consequences [ 152 ]. A centralized, nonpartisan board with an appropriate legal and regulatory infrastructure to ensure the safety of EHRs has been proposed to facilitate the identification of EHR-related adverse events and errors and potentially create a safer and more effective health care delivery system [ 153 ].
With the advanced capabilities of EHRs, the enormous amount of information that may not always be easy to locate and the potential of unintended consequences, education and training have become major issues. Clinicians are now being prepared to practice in an EHR-mediated world including electronic medical education [ 154-156 ]. Some medical schools are including lectures on EHR use. Educational systems have been integrated into the EHR [ 157 ]. Students of health professions and practicing professionals can access and interact with a set of representative solutions using an interdisciplinary EHR educational portal [ 158 ]. Likewise, medical simulation centers have been integrated into EHRs which provide realistic environments for usability testing, training, and evaluation of human-computer interactions [ 159 ]. A major role of simulation training is also to test the efficacy and safety of EHR-user interfaces, identify implementation gaps, and improve their safety [ 160-162 ].
Many medical school curricula have also added content on information retrieval [ 163 ]. Linking the EHR to the digital library and thus allowing the automatic integration of clinical data with relevant knowledge-based information to support informed decisions has been an important informatics goal [ 164 ]. Online information resources are now linked to EHRs and the role of medical librarians has changed to support this effort [ 165 ]. Infobuttons are forms of CDS and education tools that use information about the clinical context (institution, user, patient) in which an information need arises to provide direct access to relevant information from knowledge resources that accept context parameters from an EHR. Using the knowledge base of resources and information needs, these tools generate a set of links that direct the user to relevant information [ 166 ]. Infobuttons are standards-based, open source Web services that disseminate specific information in multiple EHR systems anticipating clinicians’ questions and providing automated links to relevant information in knowledge resources [ 167 ]. Additional history and information of EHR capabilities and functionality can be found in the chapter “Electronic Health Record Systems” in “Biomedical Informatics: Computer Applications in Health Care and Biomedicine” [ 168 ].
Expectations for and Realization of EHRs in 1992
The changes in the use and handling of EHR information initiated by new technology, especially the microcomputer and internet would have been hard to predict prior to 1992. New technologies and events have had and will continue to have a profound effect on the development and use of future EHRs ( Table 2 ). Even prior to the 1990s, it was expected that EHRs would become technically and economically feasible on a broad scale [ 169 ] and their data would be transmitted across high speed digital networks in response to user and patient needs [ 170 ]. Those are common daily occurrences in many places around the world and modern medical care is dependent on this ability. The Institute of Medicine’s 1991 recommendations stated that by the year 2000, each physician’s office should use an EHR in order to improve patient care and its provided rules and regulations focused on patient privacy and confidentially have only been partially realized as of 2015 [ 12 ]. Likewise, while EHR data in form of text, images, and voice are available instantaneously via the internet from virtually anywhere in the world, providers still do not have all the patient information they need [ 171 , 172 ]. However, it is hard to downplay the enormous advancements that have taken place. Meaningful Use in the U.S. has had a positive influence on the implementation of EHRs in more hospitals and primary care physician offices [ 173 ]. While, some feel EHR adoption is outpacing published evidence of improvement in patient care, there is a growing body of descriptive literature regarding incentives, benefits, risks, costs of adoption, and utilization.
Expectations and realization of EHRs in 1992
The expectation to have standards for EHR data including secondary uses for population data, communications, decision support, terminology, and data exchange has seen constant advances and progress, but still requires further work especially as new technology and innovation continue and the need for open services has become paramount [ 42 , 44 , 174 , 175 ]. However, the number of organizations and vendors implementing HL7 has increased and the standard has continued to be refined and expanded along with new domain-specific areas [ 41 ].
Likewise, the expected reduction in administrative costs of the healthcare system through claims processing of EHR data utilizing data exchange standards has been realized [ 23 ]. At the same time, the IEEE P1073 MIB standard that had promised to facilitate the exchange of data between EHRs and medical devices was not supported by the medical device vendors and is currently not used [ 45 ].
The expectations for voice recognition have been realized and voice recognition has been accepted as a common method for dictated data entry into EHRs [ 176 ]. The improved and extended use of PACS image acquisition devices and medical image processing and management has had a positive impact on patient care [ 46 ]. The EHR was initially expected to provide the ability for computer diagnosis. While some definite advances have been made, computer diagnosis failed to reach the initial expectations of 1992.
The expectation to have EHRs used in the ambulatory setting has been realized and EHRs have been interfaced to or combined with hospital EHRs [ 177 ]. The use of EHRs in the ambulatory setting has been associated with increased adherence to recommended care [ 178 ]. This has been accomplished in part by the expected use of portable computing devices that can access patient information and allow patient information to be charted in the busy medical office [ 21 , 22 ].
The expectation that EHRs would only complement and not replace the traditional office record, unfortunately, continues to be reality in many facilities around the world [ 18 ]. However, the expectation had implied that the EHR could be implemented in a modular step-by-step fashion rather than all at once with its high associated costs.
It had been expected that EHRs would eliminate data duplication [ 179 ] and save time by automatically issuing routine reports, improve clinical practice, simplify quality assurance, ease data collection, generate reports, treatment plans, and medication orders without removing records from circulation [ 180 ]. While we see some of these expectations realized today, we still suffer from data duplication and many clinicians do not believe EHRs are saving time.
Finally, we expected that health care reform and the changing healthcare environment would require medical data to be in electronic form that could be accessed by providers, hospitals, government organizations, and private health care management. Thus, the “successful” medical practitioners in the future would need to change the way in which they provide care and would be those whose practices have “life-long” EHRs that allow comprehensive medical record keeping, inclusive of patient charting, billing, coding, scheduling, and data reporting to third parties [ 181-183 ]. We have seen that the healthcare reform and the changing environment worldwide have definitely accelerated the implementation and use of EHRs. However, how we measure success was not defined back in 1992. A number of events and technologies helped to accelerate the development of EHRs. Unfortunately, there also has been a number that disrupted or derailed that effort ( Table 3 ).
Events and technologies that helped to accelerate and disrupt or derailed planned development of EHRs
Where Are EHRs Expected to Be in the Next 25 years?
Current EHRs do not meet the needs of today’s distributed systems and of the rapidly changing healthcare environment [ 184 , 185 ]. The ability of applications to communicate, interpret, and act intelligently upon complex healthcare information has assumed paramount importance [ 186 ]. The future lies in the development of flexible component-based architectures that can operate seamlessly within the workflow of a healthcare environment ( Table 4 ). Much of EHR adoption continues in an environment shaped by paper chart thinking, which continues to limit successs [ 187 ]. Further research is needed to understand human-technology integration factors that may be causing clinicians to continue to rely on paper alternatives to the EHR [ 143 ]. More research is needed to determine how to integrate the EHR into patient encounters more effectively [ 188 ] and provide clinicians with a greater control of the EHR resulting in greater flexibility to fit to their needs and preferences [ 189 ]. Many physicians are optimistic about the future benefits of EHRs, but are frustrated with the non-intuitive and multi-click interfaces and cumbersome data searches of existing EHRs [ 190 ].
Design and capabilities of EHRs in the next 25 years
While the goal of creating integrated EHRs is within our reach, its success will depend chiefly on the creation and especially adoption of standards by all international parties [ 191 ]. HIE which will enable data integration, semantic interoperability, and CDS across multiple EHRs has been a desired but largely unattained aim of clinical informatics, especially in commercial EHR systems [ 192 , 193 ]. The opportunity for enabling such scalable CDS will require vendor-supported, web-based CDS development platforms along with vendor-supported application programming interfaces (APIs) allowing the use of innovative, pluggable, interchangeable, and API-based CDS applications. This will probably require initially using custom EHR APIs and then moving towards standardized EHR APIs which are already starting to be supported by major commercial EHR vendors [ 194 ]. Web applications that run on the Substitutable Medical Apps, Reusable Technologies (SMART) platform are starting to be used. The SMART platform is an example of an emerging framework that enables EHR systems to behave as “iPhone like platforms” by exhibiting an API for easy addition and deletion of third party applications [ 195 ].
Genetic testing has had limited impact on routine clinical care. Widespread adoption of future EHRs will provide the needed methods of disseminating genetic testing into diverse care settings [ 196 ]. However, the successful integration of genomic data will require significant redesign of existing EHRs. The future EHRs will be able to manage the size and complexity of genetic test results, use standards for combining clinical and genetic data, and eliminate the current limitations in the capacity to store and analyze genetic data. Related challenges of the uncertainty in the interpretation of results and privacy concerns specific to genetic testing will be resolved and EHRs will provide the genetic, environmental, and lifestyle data needed for precision medicine and the improvement of patient care [ 197 ].
The capabilities of NLP will continue to advance and its use will be needed to code the increasing dictated free-text produced by the concomitant advances and use of voice recognition. Data storage capabilities to handle the massive amount of patient information will increase as storage capacities will increase and the related costs decrease. Transforming clinical data into knowledge to improve patient care has been the goal of biomedical informatics professionals [ 198 ]. With the increase in the amount of electronic healthcare data, the potential for knowledge discovery will be substantial if data are managed in innovative and effective ways [ 199 ]. Some data sets will be so large or complex that traditional data processing applications will be inadequate. This will require the creation of new big data applications especially for data sharing. Analyses of this “big data” will provide the next step to transform healthcare data into actionable knowledge.
Displaying large amounts of irrelevant patient information can lead to information overload and user error. Next-generation interfaces for EHRs will use CDS to synthesize fragments of evidence documented throughout the entire record to understand the etiology of a disease and of its clinical manifestation in individual patients. Context-based EHRs will employ biomedical ontologies and disease models as sources of domain knowledge to identify relevant parts of the record to display [ 200 ]. Usability, the ultimate goal of recording and managing patient data, will require improved technical considerations in addition to appropriate methodologies for medical data management [ 201 ]. Since EHRs will continue to have the potential to increase medical errors, built-in EHR applications that detect physician-level usage of EHR features, and clinical simulations will be needed to analyze human-computer interaction in real healthcare settings [ 162 , 202 ]. As EHRs continue to change and improve, engineering and reengineering will still be needed in order to increase their beneficial potential while at the same time improve their safety.
The fundamental role and purpose of the EHR in the future will be a data repository based on international standard APIs for the retrieval and storage of data. It will be coupled with facility and vendor provided, and user selected applications for data review and entry and especially CDS. In addition to health data, social, economic, behavioral, and environmental data will play a vital role in providing and especially improving healthcare. The applications will be interchangeable, not dependent on EHR versions and updates, and will facilitate innovation like the current Smart Phone applications [ 203 ].
EHR safety concerns involving both unsafe technology and unsafe use will persist in the future. Mounting pressure will require EHR implementations to build a robust infrastructure to monitor and learn from adverse events, errors, and potential adverse events and errors [ 204 ]. These errors will be electronically and securely reported to a centralized, nonpartisan body (like a patient safety organization) whose purpose will be to find ways for future prevention rather than punishment [ 153 ].
Tourism as well as international business travel creates health risks for individuals and populations [ 205 ]. International HIE standards like those proposed by the International Medical Informatics Association will provide travelers with their complete personal medical information interfaced with their primary EHR [ 206 ]. This information will be available on mobile phones and other future mobile devices. Applications will allow that information to be securely uploaded to any standards-based EHR using state of the art cloud-like technologies to provide current medical care. New information, including patient entered, will be stored in the primary EHR [ 207 , 208 ]. This will be coupled with tele-health which will allow the patients’ primary care physicians to directly see and communicate with other healthcare providers in urban as well as rural areas worldwide through computerized language translation and the preservation of clinical meaning [ 209 ]. Thus, it will be necessary to develop specifications for privacy and trust agreements between international partners [ 210 , 211 ].
Use of mobile devices with high-resolution cameras by clinicians to capture images from the bedside and incorporate them into the EHR will increase. However, secure and efficient ways to manage and share digital images are lacking to date. Future clinical image applications should more closely link clinical images and documentation and should consider enabling secure transmission over public WiFi or cellular networks. The capability to share data and harness their potential to generate knowledge rapidly and inform decisions will have transformative effects improving health. The infrastructure to achieve this goal at scale --marrying technology, process, and policy-- is commonly referred to as the Learning Health System [ 212 ]. We have been extremely fortunate to have the foresight and perseverance of a number of early biomedical informatics pioneers. While, we hope for a totally electronic and clinician accepted EHR within the next 25 years, we’ll have to wait and see. However, based on the current growth in education and training of numerous talented and innovative people, the future looks bright.
Acknowledgments
I’d like to thank Kyle V. Johnson, Stephen Howe, James F. Lloyd and Jacob S. Tripp for input on classifying events that have either accelerated or disrupted/derailed EHR development.
This paper is in the following e-collection/theme issue:
Published on 29.3.2024 in Vol 26 (2024)
Identification of Predictors for Clinical Deterioration in Patients With COVID-19 via Electronic Nursing Records: Retrospective Observational Study
Authors of this article:

Original Paper
- Sumi Sung 1 , PhD ;
- Youlim Kim 2 , MPH ;
- Su Hwan Kim 3 , PhD ;
- Hyesil Jung 4 , PhD
1 Department of Nursing Science, Research Institute of Nursing Science, Chungbuk National University, Cheongju, Chungcheongbuk-do, Republic of Korea
2 Department of Radiation Oncology, College of Medicine, Seoul National University, Seoul, Republic of Korea
3 Department of Information Statistics, Gyeongsang National University, Jinju, Gyeongsangnam-do, Republic of Korea
4 Department of Nursing, College of Medicine, Inha University, Incheon, Republic of Korea
Corresponding Author:
Hyesil Jung, PhD
Department of Nursing
College of Medicine
Inha University
100 Inha-ro, Michuhol-gu
Incheon, 22212
Republic of Korea
Phone: 82 32 860 8206
Fax:82 32 874 5880
Email: [email protected]
Background: Few studies have used standardized nursing records with Systematized Nomenclature of Medicine–Clinical Terms (SNOMED CT) to identify predictors of clinical deterioration.
Objective: This study aims to standardize the nursing documentation records of patients with COVID-19 using SNOMED CT and identify predictive factors of clinical deterioration in patients with COVID-19 via standardized nursing records.
Methods: In this study, 57,558 nursing statements from 226 patients with COVID-19 were analyzed. Among these, 45,852 statements were from 207 patients in the stable (control) group and 11,706 from 19 patients in the exacerbated (case) group who were transferred to the intensive care unit within 7 days. The data were collected between December 2019 and June 2022. These nursing statements were standardized using the SNOMED CT International Edition released on November 30, 2022. The 260 unique nursing statements that accounted for the top 90% of 57,558 statements were selected as the mapping source and mapped into SNOMED CT concepts based on their meaning by 2 experts with more than 5 years of SNOMED CT mapping experience. To identify the main features of nursing statements associated with the exacerbation of patient condition, random forest algorithms were used, and optimal hyperparameters were selected for nursing problems or outcomes and nursing procedure–related statements. Additionally, logistic regression analysis was conducted to identify features that determine clinical deterioration in patients with COVID-19.
Results: All nursing statements were semantically mapped to SNOMED CT concepts for “clinical finding,” “situation with explicit context,” and “procedure” hierarchies. The interrater reliability of the mapping results was 87.7%. The most important features calculated by random forest were “oxygen saturation below reference range,” “dyspnea,” “tachypnea,” and “cough” in “clinical finding,” and “oxygen therapy,” “pulse oximetry monitoring,” “temperature taking,” “notification of physician,” and “education about isolation for infection control” in “procedure.” Among these, “dyspnea” and “inadequate food diet” in “clinical finding” increased clinical deterioration risk (dyspnea: odds ratio [OR] 5.99, 95% CI 2.25-20.29; inadequate food diet: OR 10.0, 95% CI 2.71-40.84), and “oxygen therapy” and “notification of physician” in “procedure” also increased the risk of clinical deterioration in patients with COVID-19 (oxygen therapy: OR 1.89, 95% CI 1.25-3.05; notification of physician: OR 1.72, 95% CI 1.02-2.97).
Conclusions: The study used SNOMED CT to express and standardize nursing statements. Further, it revealed the importance of standardized nursing records as predictive variables for clinical deterioration in patients.
Introduction
As of September 27, 2023, the World Health Organization reported more than 770 million confirmed cases of COVID-19, including approximately 6.9 million deaths [ 1 ]. In South Korea, among 34,436,586 confirmed cases between January 3, 2020, and August 31, 2023, there were 35,812 deaths attributed to COVID-19 [ 2 ]. Among them, older patients or those with underlying diseases or comorbidities died due to severe conditions during the beginning of COVID-19; however, other cases exhibited initially mild symptoms that gradually worsened, causing death. Therefore, early detection of aggravating factors and symptoms is crucial for timely intervention and treatment.
COVID-19 symptoms include fever, cough, sore throat, nasal congestion, malaise, headache or muscle pain, dehydration, pneumonia, sepsis, and shortness of breath [ 3 ]. COVID-19 mortality prediction relies on patient age, blood oxygen saturation, and body temperature [ 4 ]. Through a systematic evaluation and external validation of 22 prognostic models for COVID-19 [ 5 ], admission oxygen saturation on room air and age have been shown to be strong predictors of clinical deterioration and mortality, respectively, in adults hospitalized with COVID-19.
The electronic health records (EHR) system contains detailed information about symptoms, problems, and services or care provided to patients with COVID-19. Previous studies have used EHR data to predict the COVID-19 prognosis [ 6 - 8 ]. However, nursing documentation records, which account for a substantial portion of EHR data, are underused for research owing to their low quality of documentation [ 9 , 10 ]. Unlike other EHR data, nursing documentation lacks standardization than other data such as diagnoses, laboratory, and medication data. The common data model (CDM) of the Observational Medical Outcome Partnership (OMOP) is designed to standardize the structure and content of observational data from multiple sites for efficient analyses that can produce reliable evidence; it is rarely used to standardize nursing documentation. One study [ 11 ] standardized 6277 nursing statements data using Systematized Nomenclature of Medicine-Clinical Terms (SNOMED CT) and converted them into the OMOP CDM to develop a fall-prediction model along with other CDM data. SNOMED CT is the most comprehensive multilingual clinical terminology [ 12 , 13 ] used by more than 40 countries and 30,000 individuals or organizations. The SNOMED CT International Edition, published on April 30, 2023, includes 360,942 concepts, 1,595,980 descriptions (synonyms) of concepts, and 3,261,032 relationships between concepts. Despite these advancements, there is a lack of empirical studies analyzing patient outcomes using standardized nursing documentation or records with SNOMED CT.
The American Nurses Association in 2015 and 2018 position statements also recommended the use of SNOMED CT to code nursing assessments or outcomes to facilitate the interoperability of nursing data. Several studies have examined the content coverage of SNOMED CT in the nursing domain by mapping standardized nursing terminologies, such as the Nursing Intervention Classification, International Classification for Nursing Practice (ICNP), Clinical Care Classification System, North American Nursing Diagnosis Association-International, and Omaha system, into SNOMED CT [ 14 - 18 ]. Thoroddsen et al [ 19 ] described the nursing care of patients with COVID-19 using ICNP and SNOMED CT. SNOMED CT outperformed ICNP in representing COVID-19 diagnoses and interventions. In particular, SNOMED CT comprehensively covered nursing interventions for patients with COVID-19. However, prior research only evaluated its usability or content coverage in the nursing domain, with limited analysis of specific phenomena or problems. Therefore, analyzing specific phenomena or problems using standardized nursing documentation data with SNOMED CT is necessary.
This study aimed to (1) standardize the nursing documentation records of patients with COVID-19 using SNOMED CT and (2) identify predictive factors of clinical deterioration in patients with COVID-19 using standardized nursing records.
Study Design and Setting
This retrospective observational study used data extracted from a clinical data warehouse (CDW) at a tertiary hospital with 1782 beds in Seoul, South Korea. The hospital operated 2 general wards and 4 disaster intensive care units (DICU) to treat COVID-19.
In this study, the clinical outcome is the patient’s clinical deterioration. We defined clinical deterioration as an event in which patients with COVID-19 were transferred to the ICU from general wards.
Study Participants
In total, 460 patients with COVID-19 were admitted to 2 general wards for infectious disease treatment at the hospital between December 2019 and June 2022. Patients transferred from or to other general wards or ICUs other than the DICU were excluded. Among the 460 patients, 19 patients were transferred to the DICU because of their deteriorating clinical condition. The mean length of stay (LOS) in the general ward of the 441 patients who were not transferred to the DICU during hospitalization was 8.71 days. The mean and maximum LOS of the 19 patients transferred to the DICU were 3.11 and 7 days, respectively.
Considering LOS differences between the 2 groups, the final analysis of the study included 226 admitted patients with COVID-19 at 2 general wards who were discharged or transferred to the DICU within 7 days between December 2019 and June 2022. Among these, 207 patients who were discharged in a stable clinical condition from the hospital within 7 days were assigned to the control group. A total of 19 patients who were transferred to the DICU within 7 days because of their deteriorating clinical conditions were assigned to the case group.
Data Sources
Using CDW at a study hospital, the general characteristics of patients including age, gender, and initial respiratory symptoms were extracted from initial nursing assessment records. The patient’s acuity level and nursing statements were extracted from the nursing records. A total of 57,558 nursing statements from 226 patients were extracted. Among these, 45,852 nursing statements were obtained from the nursing records of 207 patients in the control group. Of the remaining records, 11,706 nursing statements were obtained from 19 patients in the case group.
Mapping to International Standard Terminology, SNOMED CT
Nursing statements were standardized by mapping them to SNOMED CT concepts. The 57,558 nursing statements consisted of 1776 unique nursing statements which were interface terminologies developed by Seoul National University Hospital. Among the 1776 unique nursing statements, 260 unique nursing statements which were recorded 50,867 in total (cumulative recorded rate of 90%) were selected as the mapping source. Among these 260 unique statements, the control group used 237 nursing statements, and the case group used 204 nursing statements.
According to the mapping guide in the previous study [ 20 ], 2 experts with more than 5 years of SNOMED CT mapping experience performed the mapping. The scope of the map was defined to restrict mapping to the precoordinated SNOMED CT concept only. Korean nursing statements were translated into English, and search terms were chosen considering the clinical context. SNOMED CT concepts are organized into 19 top-level concepts or hierarchies under the root concept. Among them, the Clinical finding (finding) hierarchy contains concepts related to symptoms and disorders. The procedure hierarchy contains concepts related to activities performed in the provision of health care and regime or therapy. The Situation with explicit context hierarchy includes concepts that specifically define the context information of a clinical finding or procedure [ 21 ]. Therefore, the nursing statements in nursing problems or outcomes and diagnosis domains were mapped to concepts of “clinical finding” and “situation with explicit context” hierarchies, and those in the nursing interventions domain were mapped to concepts of “procedure” hierarchy within the SNOMED CT International edition, released on November 30, 2022. If a SNOMED CT concept semantically consistent with the nursing statement could not be found, it was mapped to broader precoordinated concepts.
The mapping results were classified according to the level of correspondence as 3 types: “exact map,” “narrow to broad map,” and “no map.” Mappings were categorized as follows: “exact map” when the meaning of the nursing statement matched an equivalent SNOMED CT concept, “narrow to broad map” when the meaning of the nursing statement matched a broader SNOMED CT concept, and “no map” when there was no broad match concept for the meaning of the nursing statement.
Internal validation of the final map was conducted by calculating interrater reliability. Two experts reviewed the mapping results. If the mapper and reviewer selected the same result, the map was deemed correct. If the maps differed, the results were evaluated via a group discussion, and one of them was selected.
Statistical Analyses
The general characteristics of patients (eg, gender, age, and patient acuity level) in both the case and control groups were explored. For patients in the case group transferred to the DICU (average ward LOS, 3.1 days), nursing statements documented up to the third day were extracted. The total number of nursing statements was divided by the LOS to calculate the mean number of nursing statements per day. Thereafter, the data set was separated according to the top-level hierarchies of SNOMED CT mapped to nursing statements, “clinical finding” or “situation with explicit context,” and “procedure.” Among the data set, we excluded SNOMED CT concepts that do not describe the patient’s clinical problems, such as no breathlessness, free of symptoms, no sputum, and no cough. To identify key features of nursing statements associated with the exacerbation of patient condition, random forest algorithms were used, and optimal hyperparameters were selected with 2 data sets (“clinical finding” or “situation with explicit context” and “procedure”).
To assess the effects of the features identified by random forest algorithms on the exacerbation of patient conditions, we performed logistic regression. Patient DICU transfer status was the dependent variable, and the top 5 features from each hierarchy identified by random forest algorithms were independent variables at a significance level of α=.05. Considering the small sample size for binary logistic regression analysis, the model was fitted using a modified estimation procedure known as Firth correction [ 22 ]. R (version 4.2.2; R Foundation for Statistical Computing) was used for all analyses.
Ethical Considerations
This study was approved by the Institutional Review Board of the Seoul National University Hospital (H-2207-097-1341). The requirement for informed consent was waived according to the relevant guidelines and regulations of the institutional review board. Identifiers, such as the patient’s ID and name, were encrypted so that individuals could not be identified during the data analysis. Participant compensation was not offered since this study was a retrospective observational study.
SNOMED CT Mapping Results
The results of mapping nursing statements to SNOMED CT are presented in Table 1 . The interrater reliability of the mapping results was excellent (87.7%). Of the 260 unique nursing statements, 157 were on nursing problems or outcomes, 16 were on nursing diagnoses, and 87 were on nursing intervention statements. A total of 138 (87.8%) of nursing problems or outcomes statements were mapped to concepts within “clinical finding” and “situation with explicit context” hierarchies on SNOMED CT. Through a validation process, 19 nursing problems and outcome statements were mapped into concepts within the procedure hierarchy. All 16 nursing diagnosis statements were mapped to clinical finding concepts. Among the 87 nursing intervention statements, 85 (97.7%) nursing intervention statements were mapped to concepts within the procedure hierarchy. Only 2 statements were mapped to the clinical finding concepts, “267038008 |Edema|” and “29658002 |Oxygen supply absent|.” Of the 260 nursing statements, 244 (93.8%) nursing statements were classified as “exact map” and 16 (6.2%) nursing statements were classified as “broad map.” The mapped concepts with high frequency are presented in Multimedia Appendix 1 .
a The number of concepts was calculated after removing duplicated concepts.
Clinical Characteristics
The clinical characteristics of the study participants are presented in Table 2 . The mean age was 55.9 (SD 17.3) years, and the mean LOS was 5.3 (SD 2.0) days. Patients in the control group were hospitalized in the ward for approximately 5.04 (SD 1.6) days. Patients in the case group were admitted to the ward for approximately 3.1 (SD 1.9) days. Overall, 119 (52.7%) patients were male. A total of 197 (86.7%) patients had a patient acuity level of 4, and 26 (11.5%) patients had a patient acuity level of 3. Additionally, 59 (26.1%) patients had a cough, 47 (20.8%) patients had sputum, and 26 (11.5%) patients had dyspnea as their initial symptoms.
b Chi-square.
c LOS: length of stay.
d DICU: disaster intensive care units.
e N/A: not applicable.
Feature Selection via Random Forest
The feature importance calculated using the random forest method is shown in Figure 1 . The most important feature in “clinical finding” showed “449171008 |Oxygen saturation below reference range (finding)|.” Prominent concepts that express respiratory issues included “267036007 |Dyspnea (finding)|,” “271823003 |Tachypnea (finding)|,” and “49727002 |Cough (finding)|.”

Among procedure concepts, “57485005 |Oxygen therapy|” was the most important feature, followed by “284034009 |Pulse oximetry monitoring|,” “56342008 |Temperature taking (procedure)|,” “428426009 |Notification of physician|,” and “737612005 |Education about isolation for infection control|.”
Association Between Patient’s DICU Transfer and Selected Features
Table 3 presents the results of logistic regression analysis, which explored the association between patient DICU transfer status and features in both “clinical finding” or “situation with explicit context” and “procedure” selected via random forest. In “clinical finding,” patients with dyspnea and inadequate food diet were more likely to experience DICU transfer (dyspnea: odds ratio [OR] 5.99, 95% CI 2.25-20.29; inadequate food diet: OR 10.0, 95% CI 2.71-40.84). Patients with infectious disease in nursing records were less likely to be associated with the DICU transfer in patients with COVID-19 (OR 0.32, 95% CI 0.16-0.66). In the procedure, patients with “oxygen therapy” and “notification of physician” were more likely to experience DICU transfer (oxygen therapy: OR 1.89, 95% CI 1.25-3.05; notification of physician: OR 1.72, 95% CI 1.02-2.97). Patients with “temperature taking” were less likely to experience DICU transfer (OR 0.36, 95% CI 0.18-0.70).
a SNOMED CT: Systematized Nomenclature of Medicine-Clinical Terms.
Principal Results
This study standardized the nursing statements of patients with COVID-19 in a hospital in South Korea by mapping them to SNOMED CT, an international standard terminology. Using these standardized statements, machine learning analysis with a random forest was conducted. Concepts that could predict patient deterioration in nursing problems or outcomes and intervention domains were identified. In the nursing problems or outcomes domain, concepts in nursing statements related to dyspnea, tachypnea, and oxygen saturation below the reference range were associated with patient deterioration. In nursing interventions, concepts in nursing statements related to respiratory intervention, including oxygen therapy, pulse oximetry monitoring, and physician notification, were associated with DICU transfer. These concepts were different from the findings of another study that analyzed nursing statements of patients with ovarian cancer. Kim et al [ 23 ] analyzed standardized nursing statements of patients who underwent curative surgery for epithelial ovarian cancer, and urination, food supply, bowel mobility, and pain were identified as the most common concepts.
To the best of our knowledge, this study is the first to identify predictive factors from nursing records standardized using SNOMED CT. Although previous studies [ 24 - 29 ] have used standardized nursing records such as the North American Nursing Diagnosis Association-International, Nursing Intervention Classification, Nursing Outcomes Classification, and ICNP to predict the patient’s condition or explore the nursing care provided, no study has predicted patient clinical deterioration using SNOMED CT–standardized nursing records. To achieve interoperable health information exchange, the Centers for Medicare and Medicaid Services and Office of the National Coordinator for Health Information Technology recommended the use of SNOMED CT and Logical Observation Identifier Names and Codes (LOINC) as reference terminologies. In 2020, the Office of the National Coordinator for Health Information Technology introduced the United States Core Data for Interoperability, a standardized set of health data classes and constituent data elements that recommended the use of international standard terminologies, including SNOMED CT and LOINC [ 30 ]. Consequently, standardization of nursing records using reference terminology before conducting data analysis is required in the nursing field.
Our study comprehensively standardized all nursing statements in study participants using SNOMED CT concepts. Several previous studies have evaluated SNOMED CT coverage in various health care domains, such as frailty and wound care [ 31 - 34 ]. In nursing, efforts have been made to map ICNP 7-axis concepts, nursing problem lists, and ICU nursing flowsheets to SNOMED CT [ 16 , 32 , 35 - 37 ], which provides the most detailed semantic expression compared with other standard terminologies [ 19 ]. Notably, this study unexpectedly mapped 19 nursing statements on nursing assessments or outcomes to the “procedure” hierarchy, despite the lack of corresponding concepts in the “clinical finding” or “situation with explicit context” hierarchies. This was due to the fact that the problem or phenomenon was the result of specific nursing procedures or practices. For example, the statement “a patient is applying a mattress to prevent bedsores” in nursing assessment or outcome was mapped to concept “733920005 |Provision of pressure-relieving mattress|” in the procedure hierarchy.
The identified features affecting patient deterioration (DICU transfer) in the hierarchy of “clinical finding” included respiratory issues such as low oxygen saturation, dyspnea, and tachypnea. Izquierdo et al [ 7 ] reported patient signs and symptoms, especially tachypnea, to be reliable predictors of DICU admission, which is consistent with our results. In addition, inadequate diet was significantly associated with DICU transfer, potentially due to the inability of patients to eat independently owing to their deteriorated condition. These findings highlighted the importance of signs and symptoms in nursing records for predicting a patient’s deteriorating clinical condition. Alternatively, nursing statements related to “infectious disease” within “clinical finding” and “temperature taking” within “procedure” decreased the risk of clinical deterioration in patients with COVID-19. Since patients with COVID-19 were included as study participants, it was valid that the concept of “infectious disease” appeared frequently in nursing statements, but repeated studies are needed to explain the finding that the more statements related to this concept, the lower the risk of clinical deterioration. Considering previous studies [ 4 , 38 ] reporting “fever” as a significant factor affecting deterioration in patients with COVID-19, our study revealed that increased nursing activity of “temperature taking” notably reduced patient deterioration risk. This suggested that nurses may have preemptively frequently monitored temperature to prevent fever; however, to accurately interpret this result, analyzing “body temperature” data is necessary. The features identified in this study highlight the potential of nursing records as a valuable real time predictor of a patient’s clinical condition.
Limitations
This study had some limitations. First, the analyzed data were extracted from a single tertiary university hospital, which limits the generalizability of this study. Second, sample bias is possible because patients admitted for more than 7 days to the ward were excluded. Third, other clinical findings or nursing interventions may not have been documented as nursing statements in the nursing records. Considering that this study extracted only structured nursing statements stored in the CDW, records written in free text were not included in the analysis. Fourth, as SNOMED CT concepts were used in the analysis, the results may vary depending on the hierarchical structure or level of the mapped SNOMED CT concept. For example, concepts such as “chest pain,” “headache,” and “pain” derived through random forest are connected in a hierarchical structure where “chest pain” and “headache” are subcategories of the “pain” concept. If “chest pain” and “headache” were grouped together as “pain,” the effect of “pain” on clinical deterioration might have been significant.
Conclusions
This study showed that standardized nursing records are an important source of data that can be used to predict clinical deterioration in patients with COVID-19. In total, 260 nursing statements were mapped to the SNOMED CT, including 109 concepts in the clinical finding hierarchy, 73 concepts in the procedure hierarchy, and 22 concepts in “situation” with an explicit context hierarchy. Among the standardized nursing statements, key clinical findings were respiratory issues, including low oxygen saturation, dyspnea, and tachypnea. The primary procedure-related features included oxygen therapy, pulse oximetry, and temperature monitoring. In specific, low oxygen saturation, dyspnea, tachypnea, and oxygen therapy are associated with the risk of clinical deterioration in patients with COVID-19. This study validates the use of nursing records as variables for predicting the deterioration of patients with COVID-19. Future research should investigate the integration of standardized nursing records with diagnoses, laboratory, and medication data to develop a highly reliable predictive model.
Acknowledgments
This study was supported by an Institute of Information and Communications Technology Planning and Evaluation grant funded by the Korean government (MSIT; 2021-0-00312, “Development of Non-Face-to-face Patient Infection Activity Prediction and Protection Management SW Technology at Home and Community Treatment Centers for Effective Response to Infectious Disease”) and the National Research Foundation of Korea funded by the Ministry of Science and Information Communication Technology (NRF-2021R1A2C1091261).
Data Availability
The data analyzed during this study are not readily available because of hospital regulation restrictions and patient privacy concerns. Requests to access the data sets should be directed to the corresponding author.
Authors' Contributions
SS and HJ conceptualized the study and reviewed and edited the manuscript. SS, SHK, and HJ developed the study methodology and drafted the manuscript. SS, SHK, YK, and HJ conducted formal analyses. HJ supervised the study and acquired funding. All the authors reviewed the results and approved the final version of the manuscript. Generative AI was not used in any part of the manuscript writing.
Conflicts of Interest
None declared.
The mapped concepts with high frequency.
- WHO Coronavirus (COVID-19) dashboard. World Health Organization. 2023. URL: https://covid19.who.int/ [accessed 2023-09-27]
- Coronavirus disease 19 (COVID-19) occurrence status. Korea Disease Control and Prevention Agency. URL: https://ncov.kdca.go.kr/bdBoardList_Real.do [accessed 2023-09-27]
- Clinical management of severe acute respiratory infection when novel coronavirus (2019-nCoV) infection is suspected: interim guidance. World Health Organization. 2020. URL: https://iris.who.int/handle/10665/330893 [accessed 2023-09-27]
- Yadaw AS, Li YC, Bose S, Iyengar R, Bunyavanich S, Pandey G. Clinical features of COVID-19 mortality: development and validation of a clinical prediction model. Lancet Digit Health. 2020;2(10):e516-e525. [ FREE Full text ] [ CrossRef ] [ Medline ]
- Gupta RK, Marks M, Samuels THA, Luintel A, Rampling T, Chowdhury H, et al. Systematic evaluation and external validation of 22 prognostic models among hospitalised adults with COVID-19: an observational cohort study. Eur Respir J. 2020;56(6):2003498. [ FREE Full text ] [ CrossRef ] [ Medline ]
- Campbell TW, Wilson MP, Roder H, MaWhinney S, Georgantas RW, Maguire LK, et al. Predicting prognosis in COVID-19 patients using machine learning and readily available clinical data. Int J Med Inform. 2021;155:104594. [ FREE Full text ] [ CrossRef ] [ Medline ]
- Izquierdo JL, Ancochea J, Savana COVID-19 Research Group, Soriano JB. Clinical characteristics and prognostic factors for intensive care unit admission of patients with COVID-19: retrospective study using machine learning and natural language processing. J Med Internet Res. 2020;22(10):e21801. [ FREE Full text ] [ CrossRef ] [ Medline ]
- Gong K, Wu D, Arru CD, Homayounieh F, Neumark N, Guan J, et al. A multi-center study of COVID-19 patient prognosis using deep learning-based CT image analysis and electronic health records. Eur J Radiol. 2021;139:109583. [ FREE Full text ] [ CrossRef ] [ Medline ]
- Wang N, Hailey D, Yu P. Quality of nursing documentation and approaches to its evaluation: a mixed-method systematic review. J Adv Nurs. 2011;67(9):1858-1875. [ CrossRef ] [ Medline ]
- Fennelly O, Grogan L, Reed A, Hardiker NR. Use of standardized terminologies in clinical practice: a scoping review. Int J Med Inform. 2021;149:104431. [ FREE Full text ] [ CrossRef ] [ Medline ]
- Jung H, Yoo S, Kim S, Heo E, Kim B, Lee HY, et al. Patient-level fall risk prediction using the observational medical outcomes partnership's common data model: pilot feasibility study. JMIR Med Inform. 2022;10(3):e35104. [ FREE Full text ] [ CrossRef ] [ Medline ]
- Lee D, de Keizer N, Lau F, Cornet R. Literature review of SNOMED CT use. J Am Med Inform Assoc. 2014;21(e1):e11-e19. [ FREE Full text ] [ CrossRef ] [ Medline ]
- Park HA, Yu SJ, Jung H. Strategies for adopting and implementing SNOMED CT in Korea. Healthc Inform Res. 2021;27(1):3-10. [ FREE Full text ] [ CrossRef ] [ Medline ]
- Park HT, Lu DF, Konicek D, Delaney C. Nursing interventions classification in systematized nomenclature of medicine clinical terms: a cross-mapping validation. Comput Inform Nurs. 2007;25(4):198-208; quiz 209. [ CrossRef ] [ Medline ]
- Park HA, Lundberg C, Coenen A, Konicek D. Evaluation of the content coverage of SNOMED CT representing ICNP seven-axis version 1 concepts. Methods Inf Med. 2011;50(5):472-478. [ CrossRef ] [ Medline ]
- Kim TY, Coenen A, Hardiker N. Semantic mappings and locality of nursing diagnostic concepts in UMLS. J Biomed Inform. 2012;45(1):93-100. [ FREE Full text ] [ CrossRef ] [ Medline ]
- Monsen KA, Finn RS, Fleming TE, Garner EJ, LaValla AJ, Riemer JG. Rigor in electronic health record knowledge representation: lessons learned from a SNOMED CT clinical content encoding exercise. Inform Health Soc Care. 2016;41(2):97-111. [ CrossRef ] [ Medline ]
- Kim J, Yao Y, Macieira TGR, Keenan G. An examination of the coverage of the SNOMED CT coded nursing problem list subset. JAMIA Open. 2019;2(3):386-391. [ FREE Full text ] [ CrossRef ] [ Medline ]
- Thoroddsen A, Rúnarsdóttir ER, Örlygsdóttir B. Description of COVID-19 patients and mapping nursing data to ICNP 2021 reference set in SNOMED CT. Int Nurs Rev. 2023;70(1):28-33. [ FREE Full text ] [ CrossRef ] [ Medline ]
- Sung S, Park HA, Jung H, Kang H. A SNOMED CT mapping guideline for the local terms used to document clinical findings and procedures in electronic medical records in South Korea: methodological study. JMIR Med Inform. 2023;11:e46127. [ FREE Full text ] [ CrossRef ] [ Medline ]
- SNOMED CT editorial guide. SNOMED CT International. 2023. URL: https://confluence.ihtsdotools.org/display/DOCEG/SNOMED+CT+Editorial+Guide [accessed 2023-10-25]
- van Smeden M, de Groot JAH, Moons KGM, Collins GS, Altman DG, Eijkemans MJC, et al. No rationale for 1 variable per 10 events criterion for binary logistic regression analysis. BMC Med Res Methodol. 2016;16(1):163. [ FREE Full text ] [ CrossRef ] [ Medline ]
- Kim K, Han Y, Jeong S, Doh K, Park HA, Lee K, et al. Prediction of postoperative length of hospital stay based on differences in nursing narratives in elderly patients with epithelial ovarian cancer. Methods Inf Med. 2019;58(6):222-228. [ CrossRef ] [ Medline ]
- Azzolin K, Mussi CM, Ruschel KB, de Souza EN, de Fátima Lucena A, Rabelo-Silva ER. Effectiveness of nursing interventions in heart failure patients in home care using NANDA-I, NIC, and NOC. Appl Nurs Res. 2013;26(4):239-244. [ CrossRef ] [ Medline ]
- Escalada-Hernández P, Muñoz-Hermoso P, González-Fraile E, Santos B, González-Vargas JA, Feria-Raposo I, et al. A retrospective study of nursing diagnoses, outcomes, and interventions for patients with mental disorders. Appl Nurs Res. 2015;28(2):92-98. [ CrossRef ] [ Medline ]
- da Silva Thomé E, Centena RC, da Silva Behenck A, Marini M, Heldt E. Applicability of the NANDA-I and nursing interventions classification taxonomies to mental health nursing practice. Int J Nurs Knowl. 2014;25(3):168-172. [ CrossRef ] [ Medline ]
- Yang MJ, Kim HY, Ko E, Kim HK. Identification of nursing diagnosis-outcome-intervention linkages for inpatients in the obstetrics department nursing unit in South Korea. Int J Nurs Knowl. 2019;30(1):12-20. [ CrossRef ] [ Medline ]
- Palomar-Aumatell X, Subirana-Casacuberta M, Mila-Villarroel R. Critical care nursing interventions and the time required for their completion in intensive care units: a Delphi study. Intensive Crit Care Nurs. 2017;43:87-93. [ CrossRef ] [ Medline ]
- Sung S, Jung H, Kim Y. Exploring nursing care for patients with COVID-19 using international classification for nursing practice-based nursing records. Comput Inform Nurs. 2024;42(2):127-135. [ FREE Full text ] [ CrossRef ] [ Medline ]
- United States Core Data for Interoperability (USCDI). Office of the National Coordinator for Health Information Technology. 2023. URL: https://www.healthit.gov/isa/united-states-core-data-interoperability-uscdi#uscdi-v1 [accessed 2023-09-27]
- Thandi M, Brown S, Wong ST. Mapping frailty concepts to SNOMED CT. Int J Med Inform. 2021;149:104409. [ CrossRef ] [ Medline ]
- Block LJ, Wong ST, Handfield S, Hart R, Currie LM. Comparison of terminology mapping methods for nursing wound care knowledge representation. Int J Med Inform. 2021;153:104539. [ CrossRef ] [ Medline ]
- Hüsers J, Przysucha M, Esdar M, John SM, Hübner UH. Expressiveness of an international semantic standard for wound care: mapping a standardized item set for leg ulcers to the systematized nomenclature of medicine-clinical terms. JMIR Med Inform. 2021;9(10):e31980. [ FREE Full text ] [ CrossRef ] [ Medline ]
- Block L, Handfield S. Mapping wound assessment data elements in SNOMED CT. In: Sermeus W, Procter PM, Weber P, editors. Nursing Informatics 2016: eHealth for All: Every Level Collaboration—From Project to Realization. Amsterdam, Netherlands. IOS Press; 2016;1078-1079.
- Matney SA, Warren JJ, Evans JL, Kim TY, Coenen A, Auld VA. Development of the nursing problem list subset of SNOMED CT®. J Biomed Inform. 2012;45(4):683-688. [ FREE Full text ] [ CrossRef ] [ Medline ]
- So EY, Park HA. Exploring the possibility of information sharing between the medical and nursing domains by mapping medical records to SNOMED CT and ICNP. Healthc Inform Res. 2011;17(3):156-161. [ FREE Full text ] [ CrossRef ] [ Medline ]
- Park HA, Lundberg CB, Coenen A, Konicek DJ. Evaluation of the content coverage of SNOMED-CT to represent ICNP version 1 catalogues. In: Saranto K, Brennan PF, Park HA, Tallberg M, Ensio A, editors. Connecting Health and Humans. Amsterdam, Netherland. IOS Press; 2009;303-307.
- Chen SL, Feng HY, Xu H, Huang SS, Sun JF, Zhou L, et al. Patterns of deterioration in moderate patients with COVID-19 from jan 2020 to mar 2020: a multi-center, retrospective cohort study in China. Front Med (Lausanne). 2020;7:567296. [ FREE Full text ] [ CrossRef ] [ Medline ]
Abbreviations
Edited by T de Azevedo Cardoso; submitted 03.10.23; peer-reviewed by J Lee, N Hardiker, D Liu; comments to author 19.10.23; revised version received 26.10.23; accepted 27.02.24; published 29.03.24.
©Sumi Sung, Youlim Kim, Su Hwan Kim, Hyesil Jung. Originally published in the Journal of Medical Internet Research (https://www.jmir.org), 29.03.2024.
This is an open-access article distributed under the terms of the Creative Commons Attribution License (https://creativecommons.org/licenses/by/4.0/), which permits unrestricted use, distribution, and reproduction in any medium, provided the original work, first published in the Journal of Medical Internet Research, is properly cited. The complete bibliographic information, a link to the original publication on https://www.jmir.org/, as well as this copyright and license information must be included.
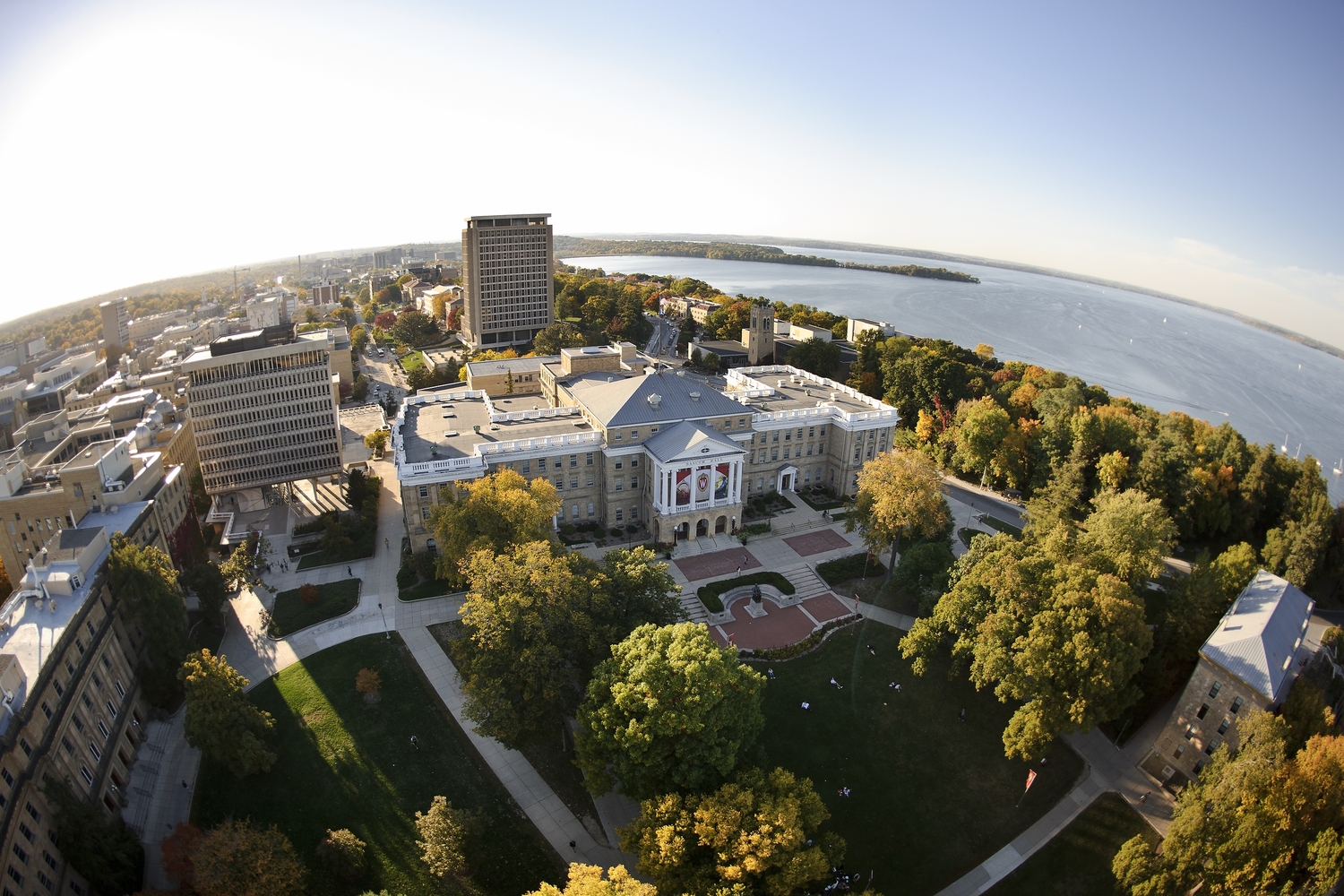
Research Coordinator
- Madison, Wisconsin
- SCHOOL OF MEDICINE AND PUBLIC HEALTH/DEPARTMENT OF MEDICINE
- Staff-Full Time
- Staff-Part Time
- Opening at: Mar 26 2024 at 13:30 CDT
- Closing at: Apr 9 2024 at 23:55 CDT
Job Summary:
The Researcher will provide support for program activities of Dr. M Brennan, MD, MS, health services researcher in the division of Infectious Disease, Department of Medicine, aiming to improve diabetic foot ulcer outcomes and health equity. The research coordinator's work would be largely focused on rural health equity, but the overall program also aims to improve health equity for patients who identify as racial or ethnic minorities. This multidisciplinary research program includes projects in claims and electronic medical record data analysis, qualitative research, and clinical health systems interventions using implementation science. This position offers an opportunity for professional growth within a small, collaborative research environment. The research coordinator will be working directly with the PI. The position is ideal for recent graduates taking a year or so off prior to considering graduate training in the health sciences/medicine. The researcher coordinator is responsible for consenting rural research participants; data collection; historical chart abstraction; data management; regulatory compliance; interfacing with clinical staff; qualitative coding; and bibliographic and scientific writing support. The main work will center around an ongoing 3-year pilot of an integrated care algorithm to improve diabetic foot ulcer outcomes for patients in 3 rural Wisconsin clinics. It will involve travel to these clinics to consent participants and review medical records. While applicants must have a valid US driver's license, a car/mileage reimbursement will be provided. The successful applicant will be highly motivated and able to take initiative, as key research activities will take place while the research coordinator is by themselves at the rural clinics.
Responsibilities:
- 75% Assists with conducting experiments or interviews, collecting and analyzing data, and documenting results according to established protocols
- 10% Assists in composing and organizing research reports and manuscripts to provide updates on unit objectives
- 5% Schedules logistics and secures resources for research supplies, equipment, and facilities
- 10% Tracks supply and equipment inventory levels and places replenishment orders
Institutional Statement on Diversity:
Diversity is a source of strength, creativity, and innovation for UW-Madison. We value the contributions of each person and respect the profound ways their identity, culture, background, experience, status, abilities, and opinion enrich the university community. We commit ourselves to the pursuit of excellence in teaching, research, outreach, and diversity as inextricably linked goals. The University of Wisconsin-Madison fulfills its public mission by creating a welcoming and inclusive community for people from every background - people who as students, faculty, and staff serve Wisconsin and the world. For more information on diversity and inclusion on campus, please visit: Diversity and Inclusion
Preferred Bachelor's Degree
Qualifications:
Required: *Candidate should have knowledge of study design, data collection and management, and compliance *Proficiency with Microsoft Word, Excel & PowerPoint *Excellent written and verbal communication *Detail-oriented and is well organized Preferred: *Experience with: NVivo, REDCap, or comparable data tools, Epic or other electronic health record *Prior healthcare exposure
License/Certification:
Required Drivers License - Valid and Meets UW Risk Management Standards Travel is required. Must provide a valid driver's license. Employees may use their own transportation or receive approval for University Car Fleet usage. Employment is conditional pending the results of a Pre-hire Driver Authorization Check. See https://businessservices.wisc.edu/managing-risk/driver-authorization-and-insurance/driver-authorization/#become-authorized-driver (click on "Become an authorized driver;" then click on "Required criteria").
Work Schedule:
Monday-Friday, daytime hours, to meet the needs of the division.
Full or Part Time: 80% - 100% It is anticipated this position requires work be performed in-person, onsite, at a designated campus work location.
Appointment Type, Duration:
Terminal, 12 month appointment. This position has the possibility to be extended or converted to an ongoing appointment based on need and/or funding
Minimum $18.00 HOURLY Depending on Qualifications
Additional Information:
The successful applicant will be responsible for ensuring eligibility for employment in the United States on or before the effective date of the appointment. University sponsorship is not available for this position. This position has been identified as a position of trust with access to vulnerable populations. The selected candidate will be required to pass an initial caregiver check to be eligible for employment under the Wisconsin Caregiver Law and every four years.
How to Apply:
To apply for this position, please click on the "Apply Now" button. You will be asked to upload a current resume/CV and a cover letter briefly describing your qualifications and experience as it relates to this role. You will also be asked to provide contact information for three (3) references, including your current/most recent supervisor during the application process. References will not be contacted without prior notice.
Charles Amble [email protected] 608-262-5609 Relay Access (WTRS): 7-1-1. See RELAY_SERVICE for further information.
Official Title:
Research Coordinator(RE034)
Department(s):
A53-MEDICAL SCHOOL/MEDICINE/INFECT DIS
Employment Class:
University Staff-Ongoing
Job Number:
The university of wisconsin-madison is an equal opportunity and affirmative action employer..
You will be redirected to the application to launch your career momentarily. Thank you!
Frequently Asked Questions
Applicant Tutorial
Disability Accommodations
Pay Transparency Policy Statement
Refer a Friend
You've sent this job to a friend!
Website feedback, questions or accessibility issues: [email protected] .
Learn more about accessibility at UW–Madison .
© 2016–2024 Board of Regents of the University of Wisconsin System • Privacy Statement
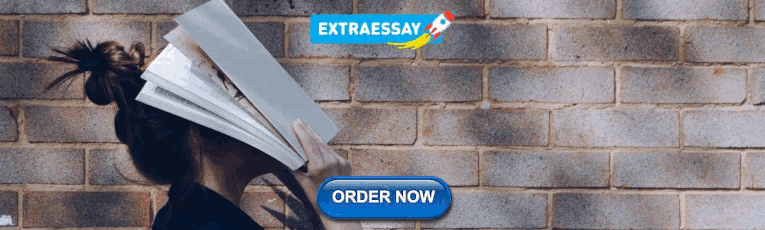
IMAGES
VIDEO
COMMENTS
Abstract. Electronic health records (EHRs) provide opportunities to enhance patient care, embed performance measures in clinical practice, and facilitate clinical research. Concerns have been raised about the increasing recruitment challenges in trials, burdensome and obtrusive data collection, and uncertain generalizability of the results.
The proliferation of electronic health records (EHRs) spurred on by federal government incentives over the past few decades has resulted in greater than an 80% adoption-rate at hospitals [] and close to 90% in office-based practices [] in the United States.A natural consequence of the availability of electronic health data is the conduct of research with these data, both observational and ...
The last several decades have seen an expanding role for EHRs. The 1960s and 1970s saw development of the first clinical information systems, such as The Medical Record at Duke University, the Computer Stored Ambulatory Record at Massachusetts General Hospital, and Health Evaluation through Logical Processing at Intermountain Healthcare in Utah. 2 In the early 1970s, the Department of Veterans ...
Electronic health records (EHRs) are now widely adopted in the United States, but health systems have barely begun using them to deliver high-value care. More directed and rigorous research is needed to fulfill the promise of EHRs to not only store information but also support the delivery of better care. This article describes 4 potential benefits of EHR-based research: improving clinical ...
This chapter presents information about the development and use of Electronic Health Record (EHR) Databases. There are petabytes of untapped research data hoarded within hospitals worldwide. There is enormous potential in the secondary analysis of this clinical data,...
The electronic medical record (EMR) and electronic health record (EHR) are platforms utilized within both clinical care and clinical research settings to collect data ultimately used by a clinical research information system (CRIS) to support clinical research. This chapter will review the concepts of the EMR, EHR, CRIS; the architecture of an ...
Abstract. Background: The increased use of electronic health records (EHRs) has resulted in new opportunities for research, but also raises concerns regarding privacy, confidentiality, and patient awareness.Because public trust is essential to the success of the research enterprise, patient perspectives are essential to the development and implementation of ethical approaches to the research ...
1. Introduction. In the early 1990s, a trend in the shift from paper-based health records to electronic records started; this was in response to advances in technology as well as the advocacy of the Institute of Medicine in the United States [1,2].As a result of the inadequacies of paper-based health records gradually becoming evident to the healthcare industry [], electronic records have ...
This research was funded by the MIT Abdul Latif Jameel Clinic for Machine Learning in Health. MedKnowts, a "smart" electronic health record system, can help doctors work more efficiently by presenting relevant information from a patient's medical history, autocompleting medical terms as a clinician types, and auto-populating repetitive ...
1. Introduction. Electronic health records (EHRs) are an appealing source of health information for researchers, including data scientists. EHRs capture data recorded during a health encounter, including patient demographics, laboratory orders and results, medical imaging reports, physiologic measurements, medication records, caregiver and procedure notes, and diagnosis and procedural codes ...
Although the benefits of EHR are well-received and Health Information Technology for Economic and Clinical Health (HITECH) Act encourages the use of EHR to improve care quality and efficiency, prior studies show mixed results of implementing EHR. 3 Recent studies suggest that full adoption of EHR might not be sufficient to ensure the benefits of EHRs; instead, meaningful use 4 or meaningful ...
AHRQ's digital healthcare research program explores topics such as the use and adoption of electronic health records. Create Accurate Reports from Electronic Health Records (EHRs) Developing and Assessing the Validity of Claims-based Indicators of Frailty & Functional Disabilities in Electronic Health Records.
Implementation of an electronic medical record system in primary care can result in a positive financial return on investment to the health care organization. AProposal for Electronic Medical Records in U.S. Primary Care Author(s): Bates DW, Ebell M, Gotlieb E, Zapp J, Mullins HC Source: J Am Med Inform Assoc (JAMIA) 2003 Jan-Feb;10(1):1-10.
Abstract. Electronic health records (EHRs) provide opportunities to enhance patient care, embed performance measures in clinical practice, and facilitate clinical research. Concerns have been raised about the increasing recruitment challenges in trials, burdensome and obtrusive data collection, and uncertain generalizability of the results.
Background Electronic Medical Records (EMRs) are one of a range of digital health solutions that are key enablers of the data revolution transforming the health sector. They offer a wide range of benefits to health professionals, patients, researchers and other key stakeholders. However, effective implementation has proved challenging. Methods A qualitative methodology was used in the study ...
Health Professionals readiness to implement electronic medical record system at three hospitals in Ethiopia: a cross sectional study: Ref. 49: Jawhari et al. 2016: Barriers and facilitators to Electronic Medical Record (EMR) use in an urban slum: Ref. 50: Sharikh et al. 2020: The impact of electronic medical records' functions on the quality ...
An electronic health record (EHR) holds detailed longitudinal information about a patient's health status and general clinical history, a large portion of which is stored as unstructured, free text. Existing approaches to model a patient's trajectory focus mostly on structured data and a subset of single-domain outcomes.
More information: Adam M. Franks et al, Optimization of Electronic Health Record Usability Through a Department-Led Quality Improvement Process, The Annals of Family Medicine (2024). DOI: 10.1370 ...
In this cross-sectional study, we analyzed office-based physician responses to the 2019 National Electronic Health Records Survey, which collects nationally representative data on the use and burdens of the electronic health record (EHR). 3 The overall participation rate was 37.7%. 3 The Cambridge Health Alliance Institutional Review Board deemed this analysis of publicly available ...
Objectives. Describe the state of Electronic Health Records (EHRs) in 1992 and their evolution by 2015 and where EHRs are expected to be in 25 years. Further to discuss the expectations for EHRs in 1992 and explore which of them were realized and what events accelerated or disrupted/derailed how EHRs evolved.
All Info for H.R.7848 - 118th Congress (2023-2024): To update the National Action Plan for Adverse Drug Event Prevention to consider advances in pharmacogenomic research and testing, to improve electronic health records for pharmacogenomic information, and for other purposes.
Background: Few studies have used standardized nursing records with Systematized Nomenclature of Medicine-Clinical Terms (SNOMED CT) to identify predictors of clinical deterioration. Objective: This study aims to standardize the nursing documentation records of patients with COVID-19 using SNOMED CT and identify predictive factors of clinical deterioration in patients with COVID-19 via ...
The research coordinator's work would be largely focused on rural health equity, but the overall program also aims to improve health equity for patients who identify as racial or ethnic minorities. This multidisciplinary research program includes projects in claims and electronic medical record data analysis, qualitative research, and clinical ...