- Systematic review
- Open access
- Published: 19 June 2020
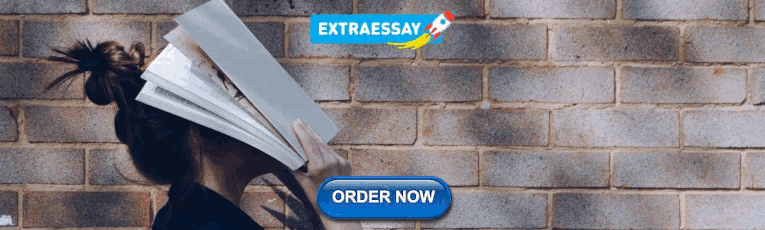
Quantitative measures of health policy implementation determinants and outcomes: a systematic review
- Peg Allen ORCID: orcid.org/0000-0001-7000-796X 1 ,
- Meagan Pilar 1 ,
- Callie Walsh-Bailey 1 ,
- Cole Hooley 2 ,
- Stephanie Mazzucca 1 ,
- Cara C. Lewis 3 ,
- Kayne D. Mettert 3 ,
- Caitlin N. Dorsey 3 ,
- Jonathan Purtle 4 ,
- Maura M. Kepper 1 ,
- Ana A. Baumann 5 &
- Ross C. Brownson 1 , 6
Implementation Science volume 15 , Article number: 47 ( 2020 ) Cite this article
17k Accesses
56 Citations
34 Altmetric
Metrics details
Public policy has tremendous impacts on population health. While policy development has been extensively studied, policy implementation research is newer and relies largely on qualitative methods. Quantitative measures are needed to disentangle differential impacts of policy implementation determinants (i.e., barriers and facilitators) and outcomes to ensure intended benefits are realized. Implementation outcomes include acceptability, adoption, appropriateness, compliance/fidelity, feasibility, penetration, sustainability, and costs. This systematic review identified quantitative measures that are used to assess health policy implementation determinants and outcomes and evaluated the quality of these measures.
Three frameworks guided the review: Implementation Outcomes Framework (Proctor et al.), Consolidated Framework for Implementation Research (Damschroder et al.), and Policy Implementation Determinants Framework (Bullock et al.). Six databases were searched: Medline, CINAHL Plus, PsycInfo, PAIS, ERIC, and Worldwide Political. Searches were limited to English language, peer-reviewed journal articles published January 1995 to April 2019. Search terms addressed four levels: health, public policy, implementation, and measurement. Empirical studies of public policies addressing physical or behavioral health with quantitative self-report or archival measures of policy implementation with at least two items assessing implementation outcomes or determinants were included. Consensus scoring of the Psychometric and Pragmatic Evidence Rating Scale assessed the quality of measures.
Database searches yielded 8417 non-duplicate studies, with 870 (10.3%) undergoing full-text screening, yielding 66 studies. From the included studies, 70 unique measures were identified to quantitatively assess implementation outcomes and/or determinants. Acceptability, feasibility, appropriateness, and compliance were the most commonly measured implementation outcomes. Common determinants in the identified measures were organizational culture, implementation climate, and readiness for implementation, each aspects of the internal setting. Pragmatic quality ranged from adequate to good, with most measures freely available, brief, and at high school reading level. Few psychometric properties were reported.
Conclusions
Well-tested quantitative measures of implementation internal settings were under-utilized in policy studies. Further development and testing of external context measures are warranted. This review is intended to stimulate measure development and high-quality assessment of health policy implementation outcomes and determinants to help practitioners and researchers spread evidence-informed policies to improve population health.
Registration
Not registered
Peer Review reports
Contributions to the literature
This systematic review identified 70 quantitative measures of implementation outcomes or determinants in health policy studies.
Readiness to implement and organizational climate and culture were commonly assessed determinants, but fewer studies assessed policy actor relationships or implementation outcomes of acceptability, fidelity/compliance, appropriateness, feasibility, or implementation costs.
Study team members rated most identified measures’ pragmatic properties as good, meaning they are straightforward to use, but few studies documented pilot or psychometric testing of measures.
Further development and dissemination of valid and reliable measures of policy implementation outcomes and determinants can facilitate identification, use, and spread of effective policy implementation strategies.
Despite major impacts of policy on population health [ 1 , 2 , 3 , 4 , 5 , 6 , 7 ], there have been relatively few policy studies in dissemination and implementation (D&I) science to inform implementation strategies and evaluate implementation efforts [ 8 ]. While health outcomes of policies are commonly studied, fewer policy studies assess implementation processes and outcomes. Of 146 D&I studies funded by the National Institutes of Health (NIH) through D&I funding announcements from 2007 to 2014, 12 (8.2%) were policy studies that assessed policy content, policy development processes, or health outcomes of policies, representing 10.5% of NIH D&I funding [ 8 ]. Eight of the 12 studies (66.7%) assessed health outcomes, while only five (41.6%) assessed implementation [ 8 ].
Our ability to explore the differential impact of policy implementation determinants and outcomes and disentangle these from health benefits and other societal outcomes requires high quality quantitative measures [ 9 ]. While systematic reviews of measures of implementation of evidence-based interventions (in clinical and community settings) have been conducted in recent years [ 10 , 11 , 12 , 13 ], to our knowledge, no reviews have explored the quality of quantitative measures of determinants and outcomes of policy implementation.
Policy implementation research in political science and the social sciences has been active since at least the 1970s and has much to contribute to the newer field of D&I research [ 1 , 14 ]. Historically, theoretical frameworks and policy research largely emphasized policy development or analysis of the content of policy documents themselves [ 15 ]. For example, Kingdon’s Multiple Streams Framework and its expansions have been widely used in political science and the social sciences more broadly to describe how factors related to sociopolitical climate, attributes of a proposed policy, and policy actors (e.g., organizations, sectors, individuals) contribute to policy change [ 16 , 17 , 18 ]. Policy frameworks can also inform implementation planning and evaluation in D&I research. Although authors have named policy stages since the 1950s [ 19 , 20 ], Sabatier and Mazmanian’s Policy Implementation Process Framework was one of the first such frameworks that gained widespread use in policy implementation research [ 21 ] and later in health promotion [ 22 ]. Yet, available implementation frameworks are not often used to guide implementation strategies or inform why a policy worked in one setting but not another [ 23 ]. Without explicit focus on implementation, the intended benefits of health policies may go unrealized, and the ability may be lost to move the field forward to understand policy implementation (i.e., our collective knowledge building is dampened) [ 24 ].
Differences in perspectives and terminology between D&I and policy research in political science are noteworthy to interpret the present review. For example, Proctor et al. use the term implementation outcomes for what policy researchers call policy outputs [ 14 , 20 , 25 ]. To non-D&I policy researchers, policy implementation outcomes refer to the health outcomes in the target population [ 20 ]. D&I science uses the term fidelity [ 26 ]; policy researchers write about compliance [ 20 ]. While D&I science uses the terms outer setting, outer context, or external context to point to influences outside the implementing organization [ 26 , 27 , 28 ], non-D&I policy research refers to policy fields [ 24 ] which are networks of agencies that carry out policies and programs.
Identification of valid and reliable quantitative measures of health policy implementation processes is needed. These measures are needed to advance from classifying constructs to understanding causality in policy implementation research [ 29 ]. Given limited resources, policy implementers also need to know which aspects of implementation are key to improve policy acceptance, compliance, and sustainability to reap the intended health benefits [ 30 ]. Both pragmatic and psychometrically sound measures are needed to accomplish these objectives [ 10 , 11 , 31 , 32 ], so the field can explore the influence of nuanced determinants and generate reliable and valid findings.
To fill this void in the literature, this systematic review of health policy implementation measures aimed to (1) identify quantitative measures used to assess health policy implementation outcomes (IOF outcomes commonly called policy outputs in policy research) and inner and outer setting determinants, (2) describe and assess pragmatic quality of policy implementation measures, (3) describe and assess the quality of psychometric properties of identified instruments, and (4) elucidate health policy implementation measurement gaps.
The study team used systematic review procedures developed by Lewis and colleagues for reviews of D&I research measures and received detailed guidance from the Lewis team coauthors for each step [ 10 , 11 ]. We followed the PRISMA reporting guidelines as shown in the checklist (Supplemental Table 1 ). We have also provided a publicly available website of measures identified in this review ( https://www.health-policy-measures.org/ ).
For the purposes of this review, policy and policy implementation are defined as follows. We deemed public policy to include legislation at the federal, state/province/regional unit, or local levels; and governmental regulations, whether mandated by national, state/province, or local level governmental agencies or boards of elected officials (e.g., state boards of education in the USA) [ 4 , 20 ]. Here, public policy implementation is defined as the carrying out of a governmental mandate by public or private organizations and groups of organizations [ 20 ].
Two widely used frameworks from the D&I field guide the present review, and a third recently developed framework that bridges policy and D&I research. In the Implementation Outcomes Framework (IOF), Proctor and colleagues identify and define eight implementation outcomes that are differentiated from health outcomes: acceptability, adoption, appropriateness, cost, feasibility, fidelity, penetration, and sustainability [ 25 ]. In the Consolidated Framework for Implementation Research (CFIR), Damschroder and colleagues articulate determinants of implementation including the domains of intervention characteristics, outer setting, inner setting of an organization, characteristics of individuals within organizations, and process [ 33 ]. Finally, Bullock developed the Policy Implementation Determinants Framework to present a balanced framework that emphasizes both internal setting constructs and external setting constructs including policy actor relationships and networks, political will for implementation, and visibility of policy actors [ 34 ]. The constructs identified in these frameworks were used to guide our list of implementation determinants and outcomes.
Through EBSCO, we searched MEDLINE, PsycInfo, and CINAHL Plus. Through ProQuest, we searched PAIS, Worldwide Political, and ERIC. Due to limited time and staff in the 12-month study, we did not search the grey literature. We used multiple search terms in each of four required levels: health, public policy, implementation, and measurement (Table 1 ). Table 1 shows search terms for each string. Supplemental Tables 2 and 3 show the final search syntax applied in EBSCO and ProQuest.
The authors developed the search strings and terms based on policy implementation framework reviews [ 34 , 35 ], additional policy implementation frameworks [ 21 , 22 ], labels and definitions of the eight implementation outcomes identified by Proctor et al. [ 25 ], CFIR construct labels and definitions [ 9 , 33 ], and additional D&I research and search term sources [ 28 , 36 , 37 , 38 ] (Table 1 ). The full study team provided three rounds of feedback on draft terms, and a library scientist provided additional synonyms and search terms. For each test search, we calculated the percentage of 18 benchmark articles the search captured. We determined a priori 80% as an acceptable level of precision.
Inclusion and exclusion criteria
This review addressed only measures of implementation by organizations mandated to act by governmental units or legislation. Measures of behavior changes by individuals in target populations as a result of legislation or governmental regulations and health status changes were outside the realm of this review.
There were several inclusion criteria: (1) empirical studies of the implementation of public policies already passed or approved that addressed physical or behavioral health, (2) quantitative self-report or archival measurement methods utilized, (3) published in peer-reviewed journals from January 1995 through April 2019, (4) published in the English language, (5) public policy implementation studies from any continent or international governing body, and (6) at least two transferable quantitative self-report or archival items that assessed implementation determinants [ 33 , 34 ] and/or IOF implementation outcomes [ 25 ]. This study sought to identify transferable measures that could be used to assess multiple policies and contexts. Here, a transferable item is defined as one that needed no wording changes or only a change in the referent (e.g., policy title or topic such as tobacco or malaria) to make the item applicable to other policies or settings [ 11 ]. The year 1995 was chosen as a starting year because that is about when web-based quantitative surveying began [ 39 ]. Table 2 provides definitions of the IOF implementation outcomes and the selected determinants of implementation. Broader constructs, such as readiness for implementation, contained multiple categories.
Exclusion criteria in the searches included (1) non-empiric health policy journal articles (e.g., conceptual articles, editorials); (2) narrative and systematic reviews; (3) studies with only qualitative assessment of health policy implementation; (4) empiric studies reported in theses and books; (5) health policy studies that only assessed health outcomes (i.e., target population changes in health behavior or status); (6) bill analyses, stakeholder perceptions assessed to inform policy development, and policy content analyses without implementation assessment; (7) studies of changes made in a private business not encouraged by public policy; and (8) countries with authoritarian regimes. We electronically programmed the searches to exclude policy implementation studies from countries that are not democratically governed due to vast differences in policy environments and implementation factors.
Screening procedures
Citations were downloaded into EndNote version 7.8 and de-duplicated electronically. We conducted dual independent screening of titles and abstracts after two group pilot screening sessions in which we clarified inclusion and exclusion criteria and screening procedures. Abstract screeners used Covidence systematic review software [ 40 ] to code inclusion as yes or no. Articles were included in full-text review if one screener coded it as meeting the inclusion criteria. Full-text screening via dual independent screening was coded in Covidence [ 40 ], with weekly meetings to reach consensus on inclusion/exclusion discrepancies. Screeners also coded one of the pre-identified reasons for exclusion.
Data extraction strategy
Extraction elements included information about (1) measure meta-data (e.g., measure name, total number of items, number of transferable items) and studies (e.g., policy topic, country, setting), (2) development and testing of the measure, (3) implementation outcomes and determinants assessed (Table 2 ), (4) pragmatic characteristics, and (5) psychometric properties. Where needed, authors were emailed to obtain the full measure and measure development information. Two coauthors (MP, CWB) reached consensus on extraction elements. For each included measure, a primary extractor conducted initial entries and coding. Due to time and staff limitations in the 12-month study, we did not search for each empirical use of the measure. A secondary extractor checked the entries, noting any discrepancies for discussion in consensus meetings. Multiple measures in a study were extracted separately.
Quality assessment of measures
To assess the quality of measures, we applied the Psychometric and Pragmatic Evidence Rating Scales (PAPERS) developed by Lewis et al. [ 10 , 11 , 41 , 42 ]. PAPERS includes assessment of five pragmatic instrument characteristics that affect the level of ease or difficulty to use the instrument: brevity (number of items), simplicity of language (readability level), cost (whether it is freely available), training burden (extent of data collection training needed), and analysis burden (ease or difficulty of interpretation of scoring and results). Lewis and colleagues developed the pragmatic domains and rating scales with stakeholder and D&I researchers input [ 11 , 41 , 42 ] and developed the psychometric rating scales in collaboration with D&I researchers [ 10 , 11 , 43 ]. The psychometric rating scale has nine properties (Table 3 ): internal consistency; norms; responsiveness; convergent, discriminant, and known-groups construct validity; predictive and concurrent criterion validity; and structural validity. In both the pragmatic and psychometric scales, reported evidence for each domain is scored from poor (− 1), none/not reported (0), minimal/emerging (1), adequate (2), good (3), or excellent (4). Higher values are indicative of more desirable pragmatic characteristics (e.g., fewer items, freely available, scoring instructions, and interpretations provided) and stronger evidence of psychometric properties (e.g., adequate to excellent reliability and validity) (Supplemental Tables 4 and 5 ).
Data synthesis and presentation
This section describes the synthesis of measure transferability, empiric use study settings and policy topics, and PAPERS scoring. Two coauthors (MP, CWB) consensus coded measures into three categories of item transferability based on quartile item transferability percentages: mostly transferable (≥ 75% of items deemed transferable), partially transferable (25–74% of items deemed transferable), and setting-specific (< 25% of items deemed transferable). Items were deemed transferable if no wording changes or only a change in the referent (e.g., policy title or topic) was needed to make the item applicable to the implementation of other policies or in other settings. Abstractors coded study settings into one of five categories: hospital or outpatient clinics; mental or behavioral health facilities; healthcare cost, access, or quality; schools; community; and multiple. Abstractors also coded policy topics to healthcare cost, access, or quality; mental or behavioral health; infectious or chronic diseases; and other, while retaining documentation of subtopics such as tobacco, physical activity, and nutrition. Pragmatic scores were totaled for the five properties, with possible total scores of − 5 to 20, with higher values indicating greater ease to use the instrument. Psychometric property total scores for the nine properties were also calculated, with possible scores of − 9 to 36, with higher values indicating evidence of multiple types of validity.
The database searches yielded 11,684 articles, of which 3267 were duplicates (Fig. 1 ). Titles and abstracts of the 8417 articles were independently screened by two team members; 870 (10.3%) were selected for full-text screening by at least one screener. Of the 870 studies, 804 were excluded at full-text screening or during extraction attempts with the consensus of two coauthors; 66 studies were included. Two coauthors (MP, CWB) reached consensus on extraction and coding of information on 70 unique quantitative eligible measures identified in the 66 included studies plus measure development articles where obtained. Nine measures were used in more than one included study. Detailed information on identified measures is publicly available at https://www.health-policy-measures.org/ .
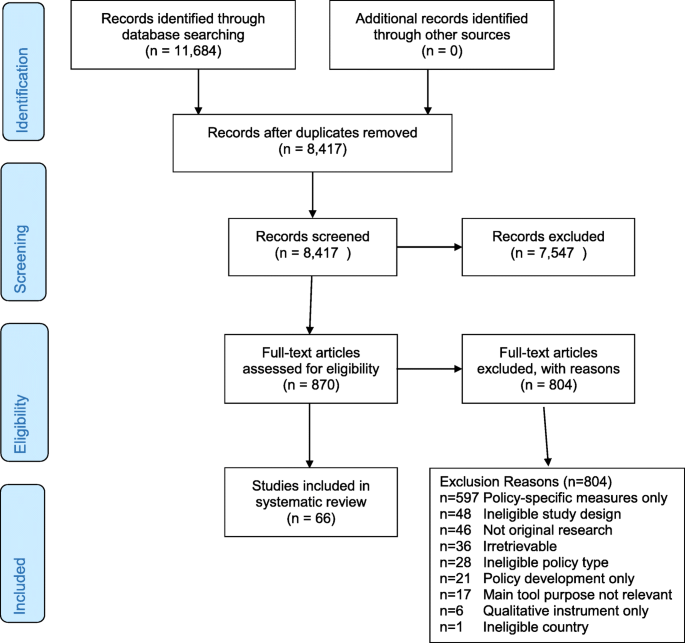
PRISMA flow diagram
The most common exclusion reason was lack of transferable items in quantitative measures of policy implementation ( n = 597) (Fig. 1 ). While this review focused on transferable measures across any health issue or setting, researchers addressing specific health policies or settings may find the excluded studies of interest. The frequencies of the remaining exclusion reasons are listed in Fig. 1 .
A variety of health policy topics and settings from over two dozen countries were found in the database searches. For example, the searches identified quantitative and mixed methods implementation studies of legislation (such as tobacco smoking bans), regulations (such as food/menu labeling requirements), governmental policies that mandated specific clinical practices (such as vaccination or access to HIV antiretroviral treatment), school-based interventions (such as government-mandated nutritional content and physical activity), and other public policies.
Among the 70 unique quantitative implementation measures, 15 measures were deemed mostly transferable (at least 75% transferable, Table 4 ). Twenty-three measures were categorized as partially transferable (25 to 74% of items deemed transferable, Table 5 ); 32 measures were setting-specific (< 25% of items deemed transferable, data not shown).
Implementation outcomes
Among the 70 measures, the most commonly assessed implementation outcomes were fidelity/compliance of the policy implementation to the government mandate (26%), acceptability of the policy to implementers (24%), perceived appropriateness of the policy (17%), and feasibility of implementation (17%) (Table 2 ). Fidelity/compliance was sometimes assessed by asking implementers the extent to which they had modified a mandated practice [ 45 ]. Sometimes, detailed checklists were used to assess the extent of compliance with the many mandated policy components, such as school nutrition policies [ 83 ]. Acceptability was assessed by asking staff or healthcare providers in implementing agencies their level of agreement with the provided statements about the policy mandate, scored in Likert scales. Only eight (11%) of the included measures used multiple transferable items to assess adoption, and only eight (11%) assessed penetration.
Twenty-six measures of implementation costs were found during full-text screening (10 in included studies and 14 in excluded studies, data not shown). The cost time horizon varied from 12 months to 21 years, with most cost measures assessed at multiple time points. Ten of the 26 measures addressed direct implementation costs. Nine studies reported cost modeling findings. The implementation cost survey developed by Vogler et al. was extensive [ 53 ]. It asked implementing organizations to note policy impacts in medication pricing, margins, reimbursement rates, and insurance co-pays.
Determinants of implementation
Within the 70 included measures, the most commonly assessed implementation determinants were readiness for implementation (61% assessed any readiness component) and the general organizational culture and climate (39%), followed by the specific policy implementation climate within the implementation organization/s (23%), actor relationships and networks (17%), political will for policy implementation (11%), and visibility of the policy role and policy actors (10%) (Table 2 ). Each component of readiness for implementation was commonly assessed: communication of the policy (31%, 22 of 70 measures), policy awareness and knowledge (26%), resources for policy implementation (non-training resources 27%, training 20%), and leadership commitment to implement the policy (19%).
Only two studies assessed organizational structure as a determinant of health policy implementation. Lavinghouze and colleagues assessed the stability of the organization, defined as whether re-organization happens often or not, within a set of 9-point Likert items on multiple implementation determinants designed for use with state-level public health practitioners, and assessed whether public health departments were stand-alone agencies or embedded within agencies addressing additional services, such as social services [ 69 ]. Schneider and colleagues assessed coalition structure as an implementation determinant, including items on the number of organizations and individuals on the coalition roster, number that regularly attend coalition meetings, and so forth [ 72 ].
Tables of measures
Tables 4 and 5 present the 38 measures of implementation outcomes and/or determinants identified out of the 70 included measures with at least 25% of items transferable (useable in other studies without wording changes or by changing only the policy name or other referent). Table 4 shows 15 mostly transferable measures (at least 75% transferable). Table 5 shows 23 partially transferable measures (25–74% of items deemed transferable). Separate measure development articles were found for 20 of the 38 measures; the remaining measures seemed to be developed for one-time, study-specific use by the empirical study authors cited in the tables. Studies listed in Tables 4 and 5 were conducted most commonly in the USA ( n = 19) or Europe ( n = 11). A few measures were used elsewhere: Africa ( n = 3), Australia ( n = 1), Canada ( n = 1), Middle East ( n = 1), Southeast Asia ( n = 1), or across multiple continents ( n = 1).
Quality of identified measures
Figure 2 shows the median pragmatic quality ratings across the 38 measures with at least 25% transferable items shown in Tables 4 and 5 . Higher scores are desirable and indicate the measures are easier to use (Table 3 ). Overall, the measures were freely available in the public domain (median score = 4), brief with a median of 11–50 items (median score = 3), and had good readability, with a median reading level between 8th and 12th grade (median score = 3). However, instructions on how to score and interpret item scores were lacking, with a median score of 1, indicating the measures did not include suggestions for interpreting score ranges, clear cutoff scores, and instructions for handling missing data. In general, information on training requirements or availability of self-training manuals on how to use the measures was not reported in the included study or measure development article/s (median score = 0, not reported). Total pragmatic rating scores among the 38 measures with at least 25% of items transferable ranged from 7 to 17 (Tables 4 and 5 ), with a median total score of 12 out of a possible total score of 20. Median scores for each pragmatic characteristic were the same across all measures as for the 38 mostly or partially transferable measures, with a median total score of 11 across all measures.
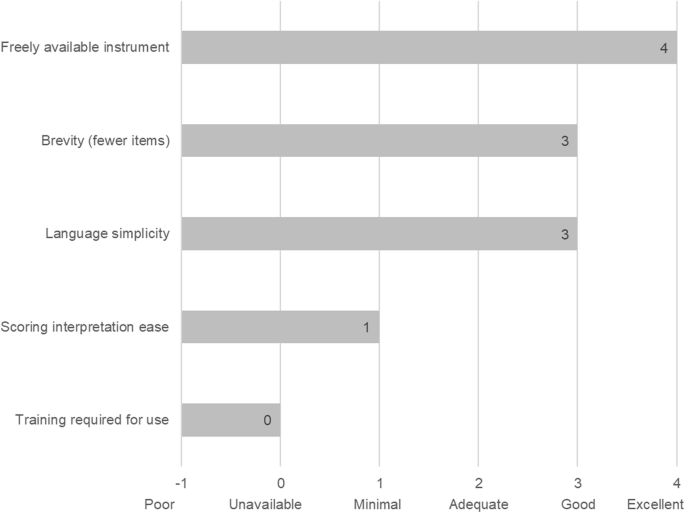
Pragmatic rating scale results across identified measures. Footnote: pragmatic criteria scores from Psychometric and Pragmatic Evidence Rating Scale (PAPERS) (Lewis et al. [ 11 ], Stanick et al. [ 42 ]). Total possible score = 20, total median score across 38 measures = 11. Scores ranged from 0 to 18. Rating scales for each domain are provided in Supplemental Table 4
Few psychometric properties were reported. The study team found few reports of pilot testing and measure refinement as well. Among the 38 measures with at least 25% transferable items, the psychometric properties from the PAPERS rating scale total scores ranged from − 1 to 17 (Tables 4 and 5 ), with a median total score of 5 out of a possible total score of 36. Higher scores indicate more types of validity and reliability were reported with high quality. The 32 measures with calculable norms had a median norms PAPERS score of 3 (good), indicating appropriate sample size and distribution. The nine measures with reported internal consistency mostly showed Cronbach’s alphas in the adequate (0.70 to 0.79) to excellent (≥ 90) range, with a median of 0.78 (PAPERS score of 2, adequate) indicating adequate internal consistency. The five measures with reported structural validity had a median PAPERS score of 2, adequate (range 1 to 3, poor to good), indicating the sample size was sufficient and the factor analysis goodness of fit was reasonable. Among the 38 measures, no reports were found for responsiveness, convergent validity, discriminant validity, known-groups construct validity, or predictive or concurrent criterion validity.
In this systematic review, we sought to identify quantitative measures used to assess health policy implementation outcomes and determinants, rate the pragmatic and psychometric quality of identified measures, and point to future directions to address measurement gaps. In general, the identified measures are easy to use and freely available, but we found little data on validity and reliability. We found more quantitative measures of intra-organizational determinants of policy implementation than measures of the relationships and interactions between organizations that influence policy implementation. We found a limited number of measures that had been developed for or used to assess one of the eight IOF policy implementation outcomes that can be applied to other policies or settings, which may speak more to differences in terms used by policy researchers and D&I researchers than to differences in conceptualizations of policy implementation. Authors used a variety of terms and rarely provided definitions of the constructs the items assessed. Input from experts in policy implementation is needed to better understand and define policy implementation constructs for use across multiple fields involved in policy-related research.
We found several researchers had used well-tested measures of implementation determinants from D&I research or from organizational behavior and management literature (Tables 4 and 5 ). For internal setting of implementing organizations, whether mandated through public policy or not, well-developed and tested measures are available. However, a number of authors crafted their own items, with or without pilot testing, and used a variety of terms to describe what the items assessed. Further dissemination of the availability of well-tested measures to policy researchers is warranted [ 9 , 13 ].
What appears to be a larger gap involves the availability of well-developed and tested quantitative measures of the external context affecting policy implementation that can be used across multiple policy settings and topics [ 9 ]. Lack of attention to how a policy initiative fits with the external implementation context during policymaking and lack of policymaker commitment of adequate resources for implementation contribute to this gap [ 23 , 93 ]. Recent calls and initiatives to integrate health policies during policymaking and implementation planning will bring more attention to external contexts affecting not only policy development but implementation as well [ 93 , 94 , 95 , 96 , 97 , 98 , 99 ]. At the present time, it is not well-known which internal and external determinants are most essential to guide and achieve sustainable policy implementation [ 100 ]. Identification and dissemination of measures that assess factors that facilitate the spread of evidence-informed policy implementation (e.g., relative advantage, flexibility) will also help move policy implementation research forward [ 1 , 9 ].
Given the high potential population health impact of evidence-informed policies, much more attention to policy implementation is needed in D&I research. Few studies from non-D&I researchers reported policy implementation measure development procedures, pilot testing, scoring procedures and interpretation, training of data collectors, or data analysis procedures. Policy implementation research could benefit from the rigor of D&I quantitative research methods. And D&I researchers have much to learn about the contexts and practical aspects of policy implementation and can look to the rich depth of information in qualitative and mixed methods studies from other fields to inform quantitative measure development and testing [ 101 , 102 , 103 ].
Limitations
This systematic review has several limitations. First, the four levels of the search string and multiple search terms in each level were applied only to the title, abstract, and subject headings, due to limitations of the search engines, so we likely missed pertinent studies. Second, a systematic approach with stakeholder input is needed to expand the definitions of IOF implementation outcomes for policy implementation. Third, although the authors value intra-organizational policymaking and implementation, the study team restricted the search to governmental policies due to limited time and staffing in the 12-month study. Fourth, by excluding tools with only policy-specific implementation measures, we excluded some well-developed and tested instruments in abstract and full-text screening. Since only 12 measures had 100% transferable items, researchers may need to pilot test wording modifications of other items. And finally, due to limited time and staffing, we only searched online for measures and measures development articles and may have missed separately developed pragmatic information, such as training and scoring materials not reported in a manuscript.
Despite the limitations, several recommendations for measure development follow from the findings and related literature [ 1 , 11 , 20 , 35 , 41 , 104 ], including the need to (1) conduct systematic, mixed-methods procedures (concept mapping, expert panels) to refine policy implementation outcomes, (2) expand and more fully specify external context domains for policy implementation research and evaluation, (3) identify and disseminate well-developed measures for specific policy topics and settings, (4) ensure that policy implementation improves equity rather than exacerbating disparities [ 105 ], and (5) develop evidence-informed policy implementation guidelines.
Easy-to-use, reliable, and valid quantitative measures of policy implementation can further our understanding of policy implementation processes, determinants, and outcomes. Due to the wide array of health policy topics and implementation settings, sound quantitative measures that can be applied across topics and settings will help speed learnings from individual studies and aid in the transfer from research to practice. Quantitative measures can inform the implementation of evidence-informed policies to further the spread and effective implementation of policies to ultimately reap greater population health benefit. This systematic review of measures is intended to stimulate measure development and high-quality assessment of health policy implementation outcomes and predictors to help practitioners and researchers spread evidence-informed policies to improve population health and reduce inequities.
Availability of data and materials
A compendium of identified measures is available for dissemination at https://www.health-policy-measures.org/ . A link will be provided on the website of the Prevention Research Center, Brown School, Washington University in St. Louis, at https://prcstl.wustl.edu/ . The authors invite interested organizations to provide a link to the compendium. Citations and abstracts of excluded policy-specific measures are available on request.
Abbreviations
Consolidated Framework for Implementation Research
Cumulative Index of Nursing and Allied Health Literature
Dissemination and implementation science
Elton B. Stephens Company
Education Resources Information Center
Implementation Outcomes Framework
Psychometric and Pragmatic Evidence Rating Scale
Preferred Reporting Items for Systematic Reviews and Meta-Analyses
Purtle J, Dodson EA, Brownson RC. Policy dissemination research. In: Brownson RC, Colditz GA, Proctor EK, editors. Dissemination and Implementation Research in Health: Translating Science to Practice, Second Edition. New York: Oxford University Press; 2018.
Google Scholar
Brownson RC, Baker EA, Deshpande AD, Gillespie KN. Evidence-based public health. Third ed. New York, NY: Oxford University Press; 2018.
Guide to Community Preventive Services. About the community guide.: community preventive services task force; 2020 [updated October 03, 2019; cited 2020. Available from: https://www.thecommunityguide.org/ .
Eyler AA, Chriqui JF, Moreland-Russell S, Brownson RC, editors. Prevention, policy, and public health, first edition. New York, NY: Oxford University Press; 2016.
Andre FE, Booy R, Bock HL, Clemens J, Datta SK, John TJ, et al. Vaccination greatly reduces disease, disability, death, and inequity worldwide. Geneva, Switzerland: World Health Organization; 2008 February 2008. Contract No.: 07-040089.
Cheng JJ, Schuster-Wallace CJ, Watt S, Newbold BK, Mente A. An ecological quantification of the relationships between water, sanitation and infant, child, and maternal mortality. Environ Health. 2012;11:4.
PubMed PubMed Central Google Scholar
Levy DT, Li Y, Yuan Z. Impact of nations meeting the MPOWER targets between 2014 and 2016: an update. Tob Control. 2019.
Purtle J, Peters R, Brownson RC. A review of policy dissemination and implementation research funded by the National Institutes of Health, 2007-2014. Implement Sci. 2016;11:1.
Lewis CC, Proctor EK, Brownson RC. Measurement issues in dissemination and implementation research. In: Brownson RC, Ga C, Proctor EK, editors. Disssemination and Implementation Research in Health: Translating Science to Practice, Second Edition. New York: Oxford University Press; 2018.
Lewis CC, Fischer S, Weiner BJ, Stanick C, Kim M, Martinez RG. Outcomes for implementation science: an enhanced systematic review of instruments using evidence-based rating criteria. Implement Sci. 2015;10:155.
Lewis CC, Mettert KD, Dorsey CN, Martinez RG, Weiner BJ, Nolen E, et al. An updated protocol for a systematic review of implementation-related measures. Syst Rev. 2018;7(1):66.
Chaudoir SR, Dugan AG, Barr CH. Measuring factors affecting implementation of health innovations: a systematic review of structural, organizational, provider, patient, and innovation level measures. Implement Sci. 2013;8:22.
Rabin BA, Lewis CC, Norton WE, Neta G, Chambers D, Tobin JN, et al. Measurement resources for dissemination and implementation research in health. Implement Sci. 2016;11:42.
Nilsen P, Stahl C, Roback K, Cairney P. Never the twain shall meet?--a comparison of implementation science and policy implementation research. Implement Sci. 2013;8:63.
Sabatier PA, editor. Theories of the Policy Process. New York, NY: Routledge; 2019.
Kingdon J. Agendas, alternatives, and public policies, second edition. Second ed. New York: Longman; 1995.
Jones MD, Peterson HL, Pierce JJ, Herweg N, Bernal A, Lamberta Raney H, et al. A river runs through it: a multiple streams meta-review. Policy Stud J. 2016;44(1):13–36.
Fowler L. Using the multiple streams framework to connect policy adoption to implementation. Policy Studies Journal. 2020 (11 Feb).
Howlett M, Mukherjee I, Woo JJ. From tools to toolkits in policy design studies: the new design orientation towards policy formulation research. Policy Polit. 2015;43(2):291–311.
Natesan SD, Marathe RR. Literature review of public policy implementation. Int J Public Policy. 2015;11(4):219–38.
Sabatier PA, Mazmanian. Implementation of public policy: a framework of analysis. Policy Studies Journal. 1980 (January).
Sabatier PA. Theories of the Policy Process. Westview; 2007.
Tomm-Bonde L, Schreiber RS, Allan DE, MacDonald M, Pauly B, Hancock T, et al. Fading vision: knowledge translation in the implementation of a public health policy intervention. Implement Sci. 2013;8:59.
Roll S, Moulton S, Sandfort J. A comparative analysis of two streams of implementation research. Journal of Public and Nonprofit Affairs. 2017;3(1):3–22.
Proctor E, Silmere H, Raghavan R, Hovmand P, Aarons G, Bunger A, et al. Outcomes for implementation research: conceptual distinctions, measurement challenges, and research agenda. Admin Pol Ment Health. 2011;38(2):65–76.
Brownson RC, Colditz GA, Proctor EK, editors. Dissemination and implementation research in health: translating science to practice, second edition. New York: Oxford University Press; 2018.
Tabak RG, Khoong EC, Chambers DA, Brownson RC. Bridging research and practice: models for dissemination and implementation research. Am J Prev Med. 2012;43(3):337–50.
Rabin BA, Brownson RC, Haire-Joshu D, Kreuter MW, Weaver NL. A glossary for dissemination and implementation research in health. J Public Health Manag Pract. 2008;14(2):117–23.
PubMed Google Scholar
Lewis CC, Klasnja P, Powell BJ, Lyon AR, Tuzzio L, Jones S, et al. From classification to causality: advancing understanding of mechanisms of change in implementation science. Front Public Health. 2018;6:136.
Boyd MR, Powell BJ, Endicott D, Lewis CC. A method for tracking implementation strategies: an exemplar implementing measurement-based care in community behavioral health clinics. Behav Ther. 2018;49(4):525–37.
Glasgow RE. What does it mean to be pragmatic? Pragmatic methods, measures, and models to facilitate research translation. Health Educ Behav. 2013;40(3):257–65.
Glasgow RE, Riley WT. Pragmatic measures: what they are and why we need them. Am J Prev Med. 2013;45(2):237–43.
Damschroder LJ, Aron DC, Keith RE, Kirsh SR, Alexander JA, Lowery JC. Fostering implementation of health services research findings into practice: a consolidated framework for advancing implementation science. Implement Sci. 2009;4:50.
Bullock HL. Understanding the implementation of evidence-informed policies and practices from a policy perspective: a critical interpretive synthesis in: How do systems achieve their goals? the role of implementation in mental health systems improvement [Dissertation]. Hamilton, Ontario: McMaster University; 2019.
Watson DP, Adams EL, Shue S, Coates H, McGuire A, Chesher J, et al. Defining the external implementation context: an integrative systematic literature review. BMC Health Serv Res. 2018;18(1):209.
McKibbon KA, Lokker C, Wilczynski NL, Ciliska D, Dobbins M, Davis DA, et al. A cross-sectional study of the number and frequency of terms used to refer to knowledge translation in a body of health literature in 2006: a Tower of Babel? Implement Sci. 2010;5:16.
Terwee CB, Jansma EP, Riphagen II, de Vet HC. Development of a methodological PubMed search filter for finding studies on measurement properties of measurement instruments. Qual Life Res. 2009;18(8):1115–23.
Egan M, Maclean A, Sweeting H, Hunt K. Comparing the effectiveness of using generic and specific search terms in electronic databases to identify health outcomes for a systematic review: a prospective comparative study of literature search method. BMJ Open. 2012;2:3.
Dillman DA, Smyth JD, Christian LM. Internet, mail, and mixed-mode surveys: the tailored design method. Hoboken, NJ: John Wiley & Sons; 2009.
Covidence systematic review software. Melbourne, Australia: Veritas Health Innovation. https://www.covidence.org . Accessed Mar 2019.
Powell BJ, Stanick CF, Halko HM, Dorsey CN, Weiner BJ, Barwick MA, et al. Toward criteria for pragmatic measurement in implementation research and practice: a stakeholder-driven approach using concept mapping. Implement Sci. 2017;12(1):118.
Stanick CF, Halko HM, Nolen EA, Powell BJ, Dorsey CN, Mettert KD, et al. Pragmatic measures for implementation research: development of the Psychometric and Pragmatic Evidence Rating Scale (PAPERS). Transl Behav Med. 2019.
Henrikson NB, Blasi PR, Dorsey CN, Mettert KD, Nguyen MB, Walsh-Bailey C, et al. Psychometric and pragmatic properties of social risk screening tools: a systematic review. Am J Prev Med. 2019;57(6S1):S13–24.
Stirman SW, Miller CJ, Toder K, Calloway A. Development of a framework and coding system for modifications and adaptations of evidence-based interventions. Implement Sci. 2013;8:65.
Lau AS, Brookman-Frazee L. The 4KEEPS study: identifying predictors of sustainment of multiple practices fiscally mandated in children’s mental health services. Implement Sci. 2016;11:1–8.
Ekvall G. Organizational climate for creativity and innovation. European J Work Organizational Psychology. 1996;5(1):105–23.
Lövgren G, Eriksson S, Sandman PO. Effects of an implemented care policy on patient and personnel experiences of care. Scand J Caring Sci. 2002;16(1):3–11.
Dwyer DJ, Ganster DC. The effects of job demands and control on employee attendance and satisfaction. J Organ Behav. 1991;12:595–608.
Condon-Paoloni D, Yeatman HR, Grigonis-Deane E. Health-related claims on food labels in Australia: understanding environmental health officers’ roles and implications for policy. Public Health Nutr. 2015;18(1):81–8.
Patterson MG, West MA, Shackleton VJ, Dawson JF, Lawthom R, Maitlis S, et al. Validating the organizational climate measure: links to managerial practices, productivity and innovation. J Organ Behav. 2005;26:279–408.
Glisson C, Green P, Williams NJ. Assessing the Organizational Social Context (OSC) of child welfare systems: implications for research and practice. Child Abuse Negl. 2012;36(9):621–32.
Beidas RS, Aarons G, Barg F, Evans A, Hadley T, Hoagwood K, et al. Policy to implementation: evidence-based practice in community mental health--study protocol. Implement Sci. 2013;8(1):38.
Eisenberger R, Cummings J, Armeli S, Lynch P. Perceived organizational support, discretionary treatment, and job satisfaction. J Appl Psychol. 1997;82:812–20.
CAS PubMed Google Scholar
Eby L, George K, Brown BL. Going tobacco-free: predictors of clinician reactions and outcomes of the NY state office of alcoholism and substance abuse services tobacco-free regulation. J Subst Abus Treat. 2013;44(3):280–7.
Vogler S, Zimmermann N, de Joncheere K. Policy interventions related to medicines: survey of measures taken in European countries during 2010-2015. Health Policy. 2016;120(12):1363–77.
Wanberg CRB, Banas JT. Predictors and outcomes of openness to change in a reorganizing workplace. J Applied Psychology. 2000;85:132–42.
CAS Google Scholar
Hardy LJ, Wertheim P, Bohan K, Quezada JC, Henley E. A model for evaluating the activities of a coalition-based policy action group: the case of Hermosa Vida. Health Promot Pract. 2013;14(4):514–23.
Gavriilidis G, Östergren P-O. Evaluating a traditional medicine policy in South Africa: phase 1 development of a policy assessment tool. Glob Health Action. 2012;5:17271.
Hongoro C, Rutebemberwa E, Twalo T, Mwendera C, Douglas M, Mukuru M, et al. Analysis of selected policies towards universal health coverage in Uganda: the policy implementation barometer protocol. Archives Public Health. 2018;76:12.
Roeseler A, Solomon M, Beatty C, Sipler AM. The tobacco control network’s policy readiness and stage of change assessment: what the results suggest for moving tobacco control efforts forward at the state and territorial levels. J Public Health Manag Pract. 2016;22(1):9–19.
Brämberg EB, Klinga C, Jensen I, Busch H, Bergström G, Brommels M, et al. Implementation of evidence-based rehabilitation for non-specific back pain and common mental health problems: a process evaluation of a nationwide initiative. BMC Health Serv Res. 2015;15(1):79.
Rütten A, Lüschen G, von Lengerke T, Abel T, Kannas L, Rodríguez Diaz JA, et al. Determinants of health policy impact: comparative results of a European policymaker study. Sozial-Und Praventivmedizin. 2003;48(6):379–91.
Smith SN, Lai Z, Almirall D, Goodrich DE, Abraham KM, Nord KM, et al. Implementing effective policy in a national mental health reengagement program for veterans. J Nerv Ment Dis. 2017;205(2):161–70.
Carasso BS, Lagarde M, Cheelo C, Chansa C, Palmer N. Health worker perspectives on user fee removal in Zambia. Hum Resour Health. 2012;10:40.
Goldsmith REH, C.F. Measuring consumer innovativeness. J Acad Mark Sci. 1991;19(3):209–21.
Webster CA, Caputi P, Perreault M, Doan R, Doutis P, Weaver RG. Elementary classroom teachers’ adoption of physical activity promotion in the context of a statewide policy: an innovation diffusion and socio-ecologic perspective. J Teach Phys Educ. 2013;32(4):419–40.
Aarons GA, Glisson C, Hoagwood K, Kelleher K, Landsverk J, Cafri G. Psychometric properties and U.S. National norms of the Evidence-Based Practice Attitude Scale (EBPAS). Psychol Assess. 2010;22(2):356–65.
Gill KJ, Campbell E, Gauthier G, Xenocostas S, Charney D, Macaulay AC. From policy to practice: implementing frontline community health services for substance dependence--study protocol. Implement Sci. 2014;9:108.
Lavinghouze SR, Price AW, Parsons B. The environmental assessment instrument: harnessing the environment for programmatic success. Health Promot Pract. 2009;10(2):176–85.
Bull FC, Milton K, Kahlmeier S. National policy on physical activity: the development of a policy audit tool. J Phys Act Health. 2014;11(2):233–40.
Bull F, Milton K, Kahlmeier S, Arlotti A, Juričan AB, Belander O, et al. Turning the tide: national policy approaches to increasing physical activity in seven European countries. British J Sports Med. 2015;49(11):749–56.
Schneider EC, Smith ML, Ory MG, Altpeter M, Beattie BL, Scheirer MA, et al. State fall prevention coalitions as systems change agents: an emphasis on policy. Health Promot Pract. 2016;17(2):244–53.
Helfrich CD, Savitz LA, Swiger KD, Weiner BJ. Adoption and implementation of mandated diabetes registries by community health centers. Am J Prev Med. 2007;33(1,Suppl):S50-S65.
Donchin M, Shemesh AA, Horowitz P, Daoud N. Implementation of the Healthy Cities’ principles and strategies: an evaluation of the Israel Healthy Cities network. Health Promot Int. 2006;21(4):266–73.
Were MC, Emenyonu N, Achieng M, Shen C, Ssali J, Masaba JP, et al. Evaluating a scalable model for implementing electronic health records in resource-limited settings. J Am Med Inform Assoc. 2010;17(3):237–44.
Konduri N, Sawyer K, Nizova N. User experience analysis of e-TB Manager, a nationwide electronic tuberculosis recording and reporting system in Ukraine. ERJ Open Research. 2017;3:2.
McDonnell E, Probart C. School wellness policies: employee participation in the development process and perceptions of the policies. J Child Nutr Manag. 2008;32:1.
Mersini E, Hyska J, Burazeri G. Evaluation of national food and nutrition policy in Albania. Zdravstveno Varstvo. 2017;56(2):115–23.
Cavagnero E, Daelmans B, Gupta N, Scherpbier R, Shankar A. Assessment of the health system and policy environment as a critical complement to tracking intervention coverage for maternal, newborn, and child health. Lancet. 2008;371 North American Edition(9620):1284-93.
Lehman WE, Greener JM, Simpson DD. Assessing organizational readiness for change. J Subst Abus Treat. 2002;22(4):197–209.
Pankratz M, Hallfors D, Cho H. Measuring perceptions of innovation adoption: the diffusion of a federal drug prevention policy. Health Educ Res. 2002;17(3):315–26.
Cook JM, Thompson R, Schnurr PP. Perceived characteristics of intervention scale: development and psychometric properties. Assessment. 2015;22(6):704–14.
Probart C, McDonnell ET, Jomaa L, Fekete V. Lessons from Pennsylvania’s mixed response to federal school wellness law. Health Aff. 2010;29(3):447–53.
Probart C, McDonnell E, Weirich JE, Schilling L, Fekete V. Statewide assessment of local wellness policies in Pennsylvania public school districts. J Am Diet Assoc. 2008;108(9):1497–502.
Rakic S, Novakovic B, Stevic S, Niskanovic J. Introduction of safety and quality standards for private health care providers: a case-study from the Republic of Srpska, Bosnia and Herzegovina. Int J Equity Health. 2018;17(1):92.
Rozema AD, Mathijssen JJP, Jansen MWJ, van Oers JAM. Sustainability of outdoor school ground smoking bans at secondary schools: a mixed-method study. Eur J Pub Health. 2018;28(1):43–9.
Barbero C, Moreland-Russell S, Bach LE, Cyr J. An evaluation of public school district tobacco policies in St. Louis County, Missouri. J Sch Health. 2013;83(8):525–32.
Williams KM, Kirsh S, Aron D, Au D, Helfrich C, Lambert-Kerzner A, et al. Evaluation of the Veterans Health Administration’s specialty care transformational initiatives to promote patient-centered delivery of specialty care: a mixed-methods approach. Telemed J E-Health. 2017;23(7):577–89.
Spencer E, Walshe K. National quality improvement policies and strategies in European healthcare systems. Quality Safety Health Care. 2009;18(Suppl 1):i22–i7.
Assunta M, Dorotheo EU. SEATCA Tobacco Industry Interference Index: a tool for measuring implementation of WHO Framework Convention on Tobacco Control Article 5.3. Tob Control. 2016;25(3):313–8.
Tummers L. Policy alienation of public professionals: the construct and its measurement. Public Adm Rev. 2012;72(4):516–25.
Tummers L, Bekkers V. Policy implementation, street-level bureaucracy, and the importance of discretion. Public Manag Rev. 2014;16(4):527–47.
Raghavan R, Bright CL, Shadoin AL. Toward a policy ecology of implementation of evidence-based practices in public mental health settings. Implement Sci. 2008;3:26.
Peters D, Harting J, van Oers H, Schuit J, de Vries N, Stronks K. Manifestations of integrated public health policy in Dutch municipalities. Health Promot Int. 2016;31(2):290–302.
Tosun J, Lang A. Policy integration: mapping the different concepts. Policy Studies. 2017;38(6):553–70.
Tubbing L, Harting J, Stronks K. Unravelling the concept of integrated public health policy: concept mapping with Dutch experts from science, policy, and practice. Health Policy. 2015;119(6):749–59.
Donkin A, Goldblatt P, Allen J, Nathanson V, Marmot M. Global action on the social determinants of health. BMJ Glob Health. 2017;3(Suppl 1):e000603-e.
Baum F, Friel S. Politics, policies and processes: a multidisciplinary and multimethods research programme on policies on the social determinants of health inequity in Australia. BMJ Open. 2017;7(12):e017772-e.
Delany T, Lawless A, Baum F, Popay J, Jones L, McDermott D, et al. Health in All Policies in South Australia: what has supported early implementation? Health Promot Int. 2016;31(4):888–98.
Valaitis R, MacDonald M, Kothari A, O'Mara L, Regan S, Garcia J, et al. Moving towards a new vision: implementation of a public health policy intervention. BMC Public Health. 2016;16:412.
Bennett LM, Gadlin H, Marchand, C. Collaboration team science: a field guide. Bethesda, MD: National Cancer Institute, National Institutes of Health; 2018. Contract No.: NIH Publication No. 18-7660.
Mazumdar M, Messinger S, Finkelstein DM, Goldberg JD, Lindsell CJ, Morton SC, et al. Evaluating academic scientists collaborating in team-based research: a proposed framework. Acad Med. 2015;90(10):1302–8.
Brownson RC, Fielding JE, Green LW. Building capacity for evidence-based public health: reconciling the pulls of practice and the push of research. Annu Rev Public Health. 2018;39:27–53.
Brownson RC, Colditz GA, Proctor EK. Future issues in dissemination and implementation research. In: Brownson RC, Colditz GA, Proctor EK, editors. Dissemination and Implementation Research in Health: Translating Science to Practice. Second Edition ed. New York: Oxford University Press; 2018.
Thomson K, Hillier-Brown F, Todd A, McNamara C, Huijts T, Bambra C. The effects of public health policies on health inequalities in high-income countries: an umbrella review. BMC Public Health. 2018;18(1):869.
Download references
Acknowledgements
The authors are grateful for the policy expertise and guidance of Alexandra Morshed and the administrative support of Mary Adams, Linda Dix, and Cheryl Valko at the Prevention Research Center, Brown School, Washington University in St. Louis. We thank Lori Siegel, librarian, Brown School, Washington University in St. Louis, for assistance with search terms and procedures. We appreciate the D&I contributions of Enola Proctor and Byron Powell at the Brown School, Washington University in St. Louis, that informed this review. We thank Russell Glasgow, University of Colorado Denver, for guidance on the overall review and pragmatic measure criteria.
This project was funded March 2019 through February 2020 by the Foundation for Barnes-Jewish Hospital, with support from the Washington University in St. Louis Institute of Clinical and Translational Science Pilot Program, NIH/National Center for Advancing Translational Sciences (NCATS) grant UL1 TR002345. The project was also supported by the National Cancer Institute P50CA244431, Cooperative Agreement number U48DP006395-01-00 from the Centers for Disease Control and Prevention, R01MH106510 from the National Institute of Mental Health, and the National Institute of Diabetes and Digestive and Kidney Diseases award number P30DK020579. The findings and conclusions in this paper are those of the authors and do not necessarily represent the official positions of the Foundation for Barnes-Jewish Hospital, Washington University in St. Louis Institute of Clinical and Translational Science, National Institutes of Health, or the Centers for Disease Control and Prevention.
Author information
Authors and affiliations.
Prevention Research Center, Brown School, Washington University in St. Louis, One Brookings Drive, Campus Box 1196, St. Louis, MO, 63130, USA
Peg Allen, Meagan Pilar, Callie Walsh-Bailey, Stephanie Mazzucca, Maura M. Kepper & Ross C. Brownson
School of Social Work, Brigham Young University, 2190 FJSB, Provo, UT, 84602, USA
Cole Hooley
Kaiser Permanente Washington Health Research Institute, 1730 Minor Ave, Seattle, WA, 98101, USA
Cara C. Lewis, Kayne D. Mettert & Caitlin N. Dorsey
Department of Health Management & Policy, Drexel University Dornsife School of Public Health, Nesbitt Hall, 3215 Market St, Philadelphia, PA, 19104, USA
Jonathan Purtle
Brown School, Washington University in St. Louis, One Brookings Drive, Campus Box 1196, St. Louis, MO, 63130, USA
Ana A. Baumann
Department of Surgery (Division of Public Health Sciences) and Alvin J. Siteman Cancer Center, Washington University School of Medicine, 4921 Parkview Place, Saint Louis, MO, 63110, USA
Ross C. Brownson
You can also search for this author in PubMed Google Scholar
Contributions
Review methodology and quality assessment scale: CCL, KDM, CND. Eligibility criteria: PA, RCB, CND, KDM, SM, MP, JP. Search strings and terms: CH, PA, MP with review by AB, RCB, CND, CCL, MMK, SM, KDM. Framework selection: PA, AB, CH, MP. Abstract screening: PA, CH, MMK, SM, MP. Full-text screening: PA, CH, MP. Pilot extraction: PA, DNC, CH, KDM, SM, MP. Data extraction: MP, CWB. Data aggregation: MP, CWB. Writing: PA, RCB, JP. Editing: RCB, JP, SM, AB, CD, CH, MMK, CCL, KM, MP, CWB. The authors read and approved the final manuscript.
Corresponding author
Correspondence to Peg Allen .
Ethics declarations
Ethics approval and consent to participate.
Not applicable
Consent for publication
Competing interests.
The authors declare they have no conflicting interests.
Additional information
Publisher’s note.
Springer Nature remains neutral with regard to jurisdictional claims in published maps and institutional affiliations.
Supplementary information
Additional file 1: table s1.
. PRISMA checklist. Table S2 . Electronic search terms for databases searched through EBSCO. Table S3 . Electronic search terms for searches conducted through PROQUEST. Table S4: PAPERS Pragmatic rating scales. Table S5 . PAPERS Psychometric rating scales.
Rights and permissions
Open Access This article is licensed under a Creative Commons Attribution 4.0 International License, which permits use, sharing, adaptation, distribution and reproduction in any medium or format, as long as you give appropriate credit to the original author(s) and the source, provide a link to the Creative Commons licence, and indicate if changes were made. The images or other third party material in this article are included in the article's Creative Commons licence, unless indicated otherwise in a credit line to the material. If material is not included in the article's Creative Commons licence and your intended use is not permitted by statutory regulation or exceeds the permitted use, you will need to obtain permission directly from the copyright holder. To view a copy of this licence, visit http://creativecommons.org/licenses/by/4.0/ . The Creative Commons Public Domain Dedication waiver ( http://creativecommons.org/publicdomain/zero/1.0/ ) applies to the data made available in this article, unless otherwise stated in a credit line to the data.
Reprints and permissions
About this article
Cite this article.
Allen, P., Pilar, M., Walsh-Bailey, C. et al. Quantitative measures of health policy implementation determinants and outcomes: a systematic review. Implementation Sci 15 , 47 (2020). https://doi.org/10.1186/s13012-020-01007-w
Download citation
Received : 24 March 2020
Accepted : 05 June 2020
Published : 19 June 2020
DOI : https://doi.org/10.1186/s13012-020-01007-w
Share this article
Anyone you share the following link with will be able to read this content:
Sorry, a shareable link is not currently available for this article.
Provided by the Springer Nature SharedIt content-sharing initiative
- Implementation science
- Health policy
- Policy implementation
- Implementation
- Public policy
- Psychometric
Implementation Science
ISSN: 1748-5908
- Submission enquiries: Access here and click Contact Us
- General enquiries: [email protected]
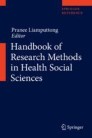
Handbook of Research Methods in Health Social Sciences pp 27–49 Cite as
Quantitative Research
- Leigh A. Wilson 2 , 3
- Reference work entry
- First Online: 13 January 2019
3980 Accesses
4 Citations
Quantitative research methods are concerned with the planning, design, and implementation of strategies to collect and analyze data. Descartes, the seventeenth-century philosopher, suggested that how the results are achieved is often more important than the results themselves, as the journey taken along the research path is a journey of discovery. High-quality quantitative research is characterized by the attention given to the methods and the reliability of the tools used to collect the data. The ability to critique research in a systematic way is an essential component of a health professional’s role in order to deliver high quality, evidence-based healthcare. This chapter is intended to provide a simple overview of the way new researchers and health practitioners can understand and employ quantitative methods. The chapter offers practical, realistic guidance in a learner-friendly way and uses a logical sequence to understand the process of hypothesis development, study design, data collection and handling, and finally data analysis and interpretation.
- Quantitative
- Epidemiology
- Data analysis
- Methodology
- Interpretation
This is a preview of subscription content, log in via an institution .
Buying options
- Available as PDF
- Read on any device
- Instant download
- Own it forever
- Available as EPUB and PDF
- Durable hardcover edition
- Dispatched in 3 to 5 business days
- Free shipping worldwide - see info
Tax calculation will be finalised at checkout
Purchases are for personal use only
Babbie ER. The practice of social research. 14th ed. Belmont: Wadsworth Cengage; 2016.
Google Scholar
Descartes. Cited in Halverston, W. (1976). In: A concise introduction to philosophy, 3rd ed. New York: Random House; 1637.
Doll R, Hill AB. The mortality of doctors in relation to their smoking habits. BMJ. 1954;328(7455):1529–33. https://doi.org/10.1136/bmj.328.7455.1529 .
Article Google Scholar
Liamputtong P. Research methods in health: foundations for evidence-based practice. 3rd ed. Melbourne: Oxford University Press; 2017.
McNabb DE. Research methods in public administration and nonprofit management: quantitative and qualitative approaches. 2nd ed. New York: Armonk; 2007.
Merriam-Webster. Dictionary. http://www.merriam-webster.com . Accessed 20th December 2017.
Olesen Larsen P, von Ins M. The rate of growth in scientific publication and the decline in coverage provided by Science Citation Index. Scientometrics. 2010;84(3):575–603.
Pannucci CJ, Wilkins EG. Identifying and avoiding bias in research. Plast Reconstr Surg. 2010;126(2):619–25. https://doi.org/10.1097/PRS.0b013e3181de24bc .
Petrie A, Sabin C. Medical statistics at a glance. 2nd ed. London: Blackwell Publishing; 2005.
Portney LG, Watkins MP. Foundations of clinical research: applications to practice. 3rd ed. New Jersey: Pearson Publishing; 2009.
Sheehan J. Aspects of research methodology. Nurse Educ Today. 1986;6:193–203.
Wilson LA, Black DA. Health, science research and research methods. Sydney: McGraw Hill; 2013.
Download references
Author information
Authors and affiliations.
School of Science and Health, Western Sydney University, Penrith, NSW, Australia
Leigh A. Wilson
Faculty of Health Science, Discipline of Behavioural and Social Sciences in Health, University of Sydney, Lidcombe, NSW, Australia
You can also search for this author in PubMed Google Scholar
Corresponding author
Correspondence to Leigh A. Wilson .
Editor information
Editors and affiliations.
Pranee Liamputtong
Rights and permissions
Reprints and permissions
Copyright information
© 2019 Springer Nature Singapore Pte Ltd.
About this entry
Cite this entry.
Wilson, L.A. (2019). Quantitative Research. In: Liamputtong, P. (eds) Handbook of Research Methods in Health Social Sciences. Springer, Singapore. https://doi.org/10.1007/978-981-10-5251-4_54
Download citation
DOI : https://doi.org/10.1007/978-981-10-5251-4_54
Published : 13 January 2019
Publisher Name : Springer, Singapore
Print ISBN : 978-981-10-5250-7
Online ISBN : 978-981-10-5251-4
eBook Packages : Social Sciences Reference Module Humanities and Social Sciences Reference Module Business, Economics and Social Sciences
Share this entry
Anyone you share the following link with will be able to read this content:
Sorry, a shareable link is not currently available for this article.
Provided by the Springer Nature SharedIt content-sharing initiative
- Publish with us
Policies and ethics
- Find a journal
- Track your research
Log in using your username and password
- Search More Search for this keyword Advanced search
- Latest content
- Current issue
- Write for Us
- BMJ Journals More You are viewing from: Google Indexer
You are here
- Volume 21, Issue 4
- How to appraise quantitative research
- Article Text
- Article info
- Citation Tools
- Rapid Responses
- Article metrics
This article has a correction. Please see:
- Correction: How to appraise quantitative research - April 01, 2019

- Xabi Cathala 1 ,
- Calvin Moorley 2
- 1 Institute of Vocational Learning , School of Health and Social Care, London South Bank University , London , UK
- 2 Nursing Research and Diversity in Care , School of Health and Social Care, London South Bank University , London , UK
- Correspondence to Mr Xabi Cathala, Institute of Vocational Learning, School of Health and Social Care, London South Bank University London UK ; cathalax{at}lsbu.ac.uk and Dr Calvin Moorley, Nursing Research and Diversity in Care, School of Health and Social Care, London South Bank University, London SE1 0AA, UK; Moorleyc{at}lsbu.ac.uk
https://doi.org/10.1136/eb-2018-102996
Statistics from Altmetric.com
Request permissions.
If you wish to reuse any or all of this article please use the link below which will take you to the Copyright Clearance Center’s RightsLink service. You will be able to get a quick price and instant permission to reuse the content in many different ways.
Introduction
Some nurses feel that they lack the necessary skills to read a research paper and to then decide if they should implement the findings into their practice. This is particularly the case when considering the results of quantitative research, which often contains the results of statistical testing. However, nurses have a professional responsibility to critique research to improve their practice, care and patient safety. 1 This article provides a step by step guide on how to critically appraise a quantitative paper.
Title, keywords and the authors
The authors’ names may not mean much, but knowing the following will be helpful:
Their position, for example, academic, researcher or healthcare practitioner.
Their qualification, both professional, for example, a nurse or physiotherapist and academic (eg, degree, masters, doctorate).
This can indicate how the research has been conducted and the authors’ competence on the subject. Basically, do you want to read a paper on quantum physics written by a plumber?
The abstract is a resume of the article and should contain:
Introduction.
Research question/hypothesis.
Methods including sample design, tests used and the statistical analysis (of course! Remember we love numbers).
Main findings.
Conclusion.
The subheadings in the abstract will vary depending on the journal. An abstract should not usually be more than 300 words but this varies depending on specific journal requirements. If the above information is contained in the abstract, it can give you an idea about whether the study is relevant to your area of practice. However, before deciding if the results of a research paper are relevant to your practice, it is important to review the overall quality of the article. This can only be done by reading and critically appraising the entire article.
The introduction
Example: the effect of paracetamol on levels of pain.
My hypothesis is that A has an effect on B, for example, paracetamol has an effect on levels of pain.
My null hypothesis is that A has no effect on B, for example, paracetamol has no effect on pain.
My study will test the null hypothesis and if the null hypothesis is validated then the hypothesis is false (A has no effect on B). This means paracetamol has no effect on the level of pain. If the null hypothesis is rejected then the hypothesis is true (A has an effect on B). This means that paracetamol has an effect on the level of pain.
Background/literature review
The literature review should include reference to recent and relevant research in the area. It should summarise what is already known about the topic and why the research study is needed and state what the study will contribute to new knowledge. 5 The literature review should be up to date, usually 5–8 years, but it will depend on the topic and sometimes it is acceptable to include older (seminal) studies.
Methodology
In quantitative studies, the data analysis varies between studies depending on the type of design used. For example, descriptive, correlative or experimental studies all vary. A descriptive study will describe the pattern of a topic related to one or more variable. 6 A correlational study examines the link (correlation) between two variables 7 and focuses on how a variable will react to a change of another variable. In experimental studies, the researchers manipulate variables looking at outcomes 8 and the sample is commonly assigned into different groups (known as randomisation) to determine the effect (causal) of a condition (independent variable) on a certain outcome. This is a common method used in clinical trials.
There should be sufficient detail provided in the methods section for you to replicate the study (should you want to). To enable you to do this, the following sections are normally included:
Overview and rationale for the methodology.
Participants or sample.
Data collection tools.
Methods of data analysis.
Ethical issues.
Data collection should be clearly explained and the article should discuss how this process was undertaken. Data collection should be systematic, objective, precise, repeatable, valid and reliable. Any tool (eg, a questionnaire) used for data collection should have been piloted (or pretested and/or adjusted) to ensure the quality, validity and reliability of the tool. 9 The participants (the sample) and any randomisation technique used should be identified. The sample size is central in quantitative research, as the findings should be able to be generalised for the wider population. 10 The data analysis can be done manually or more complex analyses performed using computer software sometimes with advice of a statistician. From this analysis, results like mode, mean, median, p value, CI and so on are always presented in a numerical format.
The author(s) should present the results clearly. These may be presented in graphs, charts or tables alongside some text. You should perform your own critique of the data analysis process; just because a paper has been published, it does not mean it is perfect. Your findings may be different from the author’s. Through critical analysis the reader may find an error in the study process that authors have not seen or highlighted. These errors can change the study result or change a study you thought was strong to weak. To help you critique a quantitative research paper, some guidance on understanding statistical terminology is provided in table 1 .
- View inline
Some basic guidance for understanding statistics
Quantitative studies examine the relationship between variables, and the p value illustrates this objectively. 11 If the p value is less than 0.05, the null hypothesis is rejected and the hypothesis is accepted and the study will say there is a significant difference. If the p value is more than 0.05, the null hypothesis is accepted then the hypothesis is rejected. The study will say there is no significant difference. As a general rule, a p value of less than 0.05 means, the hypothesis is accepted and if it is more than 0.05 the hypothesis is rejected.
The CI is a number between 0 and 1 or is written as a per cent, demonstrating the level of confidence the reader can have in the result. 12 The CI is calculated by subtracting the p value to 1 (1–p). If there is a p value of 0.05, the CI will be 1–0.05=0.95=95%. A CI over 95% means, we can be confident the result is statistically significant. A CI below 95% means, the result is not statistically significant. The p values and CI highlight the confidence and robustness of a result.
Discussion, recommendations and conclusion
The final section of the paper is where the authors discuss their results and link them to other literature in the area (some of which may have been included in the literature review at the start of the paper). This reminds the reader of what is already known, what the study has found and what new information it adds. The discussion should demonstrate how the authors interpreted their results and how they contribute to new knowledge in the area. Implications for practice and future research should also be highlighted in this section of the paper.
A few other areas you may find helpful are:
Limitations of the study.
Conflicts of interest.
Table 2 provides a useful tool to help you apply the learning in this paper to the critiquing of quantitative research papers.
Quantitative paper appraisal checklist
- 1. ↵ Nursing and Midwifery Council , 2015 . The code: standard of conduct, performance and ethics for nurses and midwives https://www.nmc.org.uk/globalassets/sitedocuments/nmc-publications/nmc-code.pdf ( accessed 21.8.18 ).
- Gerrish K ,
- Moorley C ,
- Tunariu A , et al
- Shorten A ,
Competing interests None declared.
Patient consent Not required.
Provenance and peer review Commissioned; internally peer reviewed.
Correction notice This article has been updated since its original publication to update p values from 0.5 to 0.05 throughout.
Linked Articles
- Miscellaneous Correction: How to appraise quantitative research BMJ Publishing Group Ltd and RCN Publishing Company Ltd Evidence-Based Nursing 2019; 22 62-62 Published Online First: 31 Jan 2019. doi: 10.1136/eb-2018-102996corr1
Read the full text or download the PDF:

An official website of the United States government
The .gov means it’s official. Federal government websites often end in .gov or .mil. Before sharing sensitive information, make sure you’re on a federal government site.
The site is secure. The https:// ensures that you are connecting to the official website and that any information you provide is encrypted and transmitted securely.
- Publications
- Account settings
Preview improvements coming to the PMC website in October 2024. Learn More or Try it out now .
- Advanced Search
- Journal List
- HHS Author Manuscripts

Quantitative Approaches for the Evaluation of Implementation Research Studies
Justin d. smith.
1 Center for Prevention Implementation Methodology (Ce-PIM) for Drug Abuse and HIV, Department of Psychiatry and Behavioral Sciences, Northwestern University Feinberg School of Medicine, 750 N Lake Shore Dr., Chicago, Illinois, USA.
Mohamed Hasan
2 Center for Healthcare Studies, Institute of Public Health and Medicine, Northwestern University Feinberg School of Medicine, 633 N St. Claire St., Chicago, Illinois, USA.
Authors’ contributions
Implementation research necessitates a shift from clinical trial methods in both the conduct of the study and in the way that it is evaluated given the focus on the impact of implementation strategies. That is, the methods or techniques to support the adoption and delivery of a clinical or preventive intervention, program, or policy. As strategies target one or more levels within the service delivery system, evaluating their impact needs to follow suit. This article discusses the methods and practices involved in quantitative evaluations of implementation research studies. We focus on evaluation methods that characterize and quantify the overall impacts of an implementation strategy on various outcomes. This article discusses available measurement methods for common quantitative implementation outcomes involved in such an evaluation—adoption, fidelity, implementation cost, reach, and sustainment—and the sources of such data for these metrics using established taxonomies and frameworks. Last, we present an example of a quantitative evaluation from an ongoing randomized rollout implementation trial of the Collaborative Care Model for depression management in a large primary healthcare system.
1. Background
As part of this special issue on implementation science, this article discusses quantitative methods for evaluating implementation research studies and presents an example of an ongoing implementation trial for illustrative purposes. We focus on what is called “summative evaluation,” which characterizes and quantifies the impacts of an implementation strategy on various outcomes ( Gaglio & Glasgow, 2017 ). This type of evaluation involves aggregation methods conducted at the end of a study to assess the success of an implementation strategy on the adoption, delivery, and sustainment of an evidence-based practice (EBP), and the cost associated with implementation ( Bauer, Damschroder, Hagedorn, Smith, & Kilbourne, 2015 ). These results help decision makers understand the overall worth of an implementation strategy and whether to upscale, modify, or discontinue ( Bauer et al., 2015 ). This topic complements others in this issue on formative evaluation (Elwy et al.) and qualitative methods (Hamilton et al.), which are also used in implementation research evaluation.
Implementation research, as defined by the United States National Institutes of Health (NIH), is “the scientific study of the use of strategies [italics added] to adopt and integrate evidence-based health interventions into clinical and community settings in order to improve patient outcomes and benefit population health. Implementation research seeks to understand the behavior of healthcare professionals and support staff, healthcare organizations, healthcare consumers and family members, and policymakers in context as key influences on the adoption, implementation and sustainability of evidence-based interventions and guidelines” ( Department of Health and Human Services, 2019 ). Implementation strategies are methods or techniques used to enhance the adoption, implementation, and sustainability of a clinical program or practice ( Powell et al., 2015 ).
To grasp the evaluation methods used in implementation research, one must appreciate the nature of this research and how the study designs, aims, and measures differ in fundamental ways from those methods with which readers will be most familiar—that is, evaluations of clinical efficacy or effectiveness trials. First, whereas clinical intervention research focuses on how a given clinical intervention—meaning a pill, program, practice, principle, product, policy, or procedure ( Brown et al., 2017 )—affects a health outcome at the patient level, implementation research focuses on how systems can take that intervention to scale in order to improve health outcomes of the broader community ( Colditz & Emmons, 2017 ). Thus, when implementation strategies are the focus, the outcomes evaluated are at the system level. Figure 1 illustrates the emphasis (foreground box) of effectiveness versus implementation research and the corresponding outcomes that would be included in the evaluation. This difference can be illustrated by “hybrid trials” in which effectiveness and implementation are evaluated simultaneously but with different outcomes for each aim ( Curran, Bauer, Mittman, Pyne, & Stetler, 2012 ; also see Landes et al., this issue).

Emphasis and Outcomes Evaluated in Clinical Effectiveness versus Implementation Research
Note. Adapted from a slide developed by C. Hendricks Brown.
2. Design Considerations for Evaluating Implementation Research Studies
The stark contrast between the emphasis in implementation versus effectiveness trials occurs largely because implementation strategies most often, but not always, target one or more levels within the system that supports the adoption and implementation of the intervention, such as the provider, clinic, school, health department, or even state or national levels ( Powell et al., 2015 ). Implementation strategies are discussed in this issue by Kirchner and colleagues. With the focus on levels within which patients who receive the clinical or preventive intervention are embedded, research designs in implementation research follow suit. The choice of a study design to evaluate an implementation strategy influences the confidence in the association drawn between a strategy and an observed effect ( Grimshaw, Campbell, Eccles, & Steen, 2000 ). Strong designs and methodologically-robust studies support the validity of the evaluations and provide evidence likely to be used by policy makers. Study designs are generally classified into observational (descriptive) and experimental/quasi-experimental.
Brown et al. (2017) described three broad types of designs for implementation research. ( 1 ) Within-site designs involve evaluation of the effects of implementation strategies within a single service system unit (e.g., clinic, hospital). Common within-site designs include post, pre-post, and interrupted time series. While these designs are simple and can be useful for understanding the impact in a local context ( Cheung & Duan, 2014 ), they contribute limited generalizable knowledge due to the biases inherent small-sample studies with no direct comparison condition. Brown et al. describe two broad design types can be used to create generalizable knowledge as they inherently involve multiple units for aggregation and comparison using the evaluation methods described in this article. ( 2 ) Between-site designs involve comparison of outcomes between two or more service system units or clusters/groups of units. While they commonly involve the testing of a novel implementation strategy compared to routine practice (i.e., implementation as usual), they can also be head-to-head tests of two or more novel implementation strategies for the same intervention, which we refer to as a comparative implementation trial (e.g., Smith et al., 2019 ). ( 3 ) Within- and between-site designs add a time-based crossover for each unit in which they begin in one condition—usually routine practice—and then move to a second condition involving the introduction of the implementation strategy. We refer to this category as rollout trials, which includes the stepped-wedge and dynamic wait-list design ( Brown et al., 2017 ; Landsverk et al., 2017 ; Wyman, Henry, Knoblauch, & Brown, 2015 ). Designs for implementation research are discussed in this issue by Miller and colleagues.
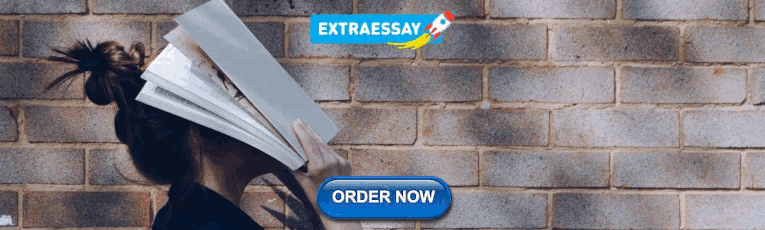
3. Quantitative Methods for Evaluating Implementation Outcomes
While summative evaluation is distinguishable from formative evaluation (see Elwy et al. this issue ), proper understanding of the implementation strategy requires using both methods, perhaps at different stages of implementation research ( The Health Foundation, 2015 ). Formative evaluation is a rigorous assessment process designed to identify potential and actual influences on the effectiveness of implementation efforts ( Stetler et al., 2006 ). Earlier stages of implementation research might rely solely on formative evaluation and the use of qualitative and mixed methods approaches. In contrast, later stage implementation research involves powered tests of the effect of one or more implementation strategies and are thus likely to use a between-site or a within- and between-site research design with at least one quantitative outcome. Quantitative methods are especially important to explore the extent and variation of change (within and across units) induced by the implementation strategies.
Proctor and colleagues (2011) provide a taxonomy of available implementation outcomes, which include acceptability, adoption, appropriateness, feasibility, fidelity, implementation cost, penetration/reach, and sustainability/sustainment. Table 1 in this article presents a modified version of Table 1 from Proctor et al. (2011) , focusing only on the quantitative measurement characteristics of these outcomes. Table 1 also includes the additional metrics of speed and quantity, which will be discussed in more detail in the case example. As noted in Table 1 , and by Proctor et al. (2011) , certain outcomes are more applicable at earlier versus later stages of implementation research. A recent review of implementation research in the field of HIV indicated that earlier stage implementation research was more likely to focus on acceptability and feasibility, whereas later stage testing of implementation strategies focused less on these and more on adoption, cost, penetration/reach, fidelity, and sustainability ( Smith et al., 2019 ). These sources of quantitative information are at multiple levels in the service delivery system, such as the intervention delivery agent, leadership, and key stakeholders in and outside of a particular delivery system ( Brown et al., 2013 ).
Quantitative Measurement Characteristics of Common Implementation Outcomes
Note. This table is modeled after Table 1 in the Proctor et al. (2011) article.
Methods for quantitative data collection include structured surveys; use of administrative records, including payor and health expenditure records; extraction from the electronic health record (EHR); and direct observation. Structured surveys are commonly used to assess attitudes and perceptions of providers and patients concerning such factors as the ability to sustain the intervention and a host of potential facilitators and barriers to implementation (e.g., Bertrand, Holtgrave, & Gregowski, 2009 ; Luke, Calhoun, Robichaux, Elliott, & Moreland-Russell, 2014 ). Administrative databases and the EHR are used to assess aspects of intervention delivery that result from the implementation strategies ( Bauer et al., 2015 ). Although the EHR supports automatic and cumulative data acquisition, its utility for measuring implementation outcomes is limited depending on the type of implementation strategy and the intervention. For example, it is well suited for gathering data on EHR-based implementation strategies, such as clinical decision supports and symptom screening, but less useful for behaviors that would not otherwise be documented in the EHR (e.g., effects of a learning collaborative on adoption of a cognitive behavioral therapy protocol). Last, observational assessment of implementation is fairly common but resource intensive, which limits its use outside of funded research. This is particularly germane to assessing fidelity of implementation, which is commonly observational in funded research but is rarely done when the intervention is adopted under real-world circumstances ( Schoenwald et al., 2011 ). The costs associated with observational fidelity measurement has led to promising efforts to automate this process with machine learning methods (e.g., Imel et al., 2019 ).
Quantitative evaluation of implementation research studies most commonly involves assessment of multiple outcome metrics to garner a comprehensive appraisal of the effects of the implementation strategy. This is due in large part to the interrelatedness and interdependence of these metrics. A shortcoming of the Proctor et al. (2011) taxonomy is that it does not specify relations between outcomes, rather they are simply listed. The RE-AIM evaluation framework ( Gaglio, Shoup, & Glasgow, 2013 ; Glasgow, Vogt, & Boles, 1999 ) is commonly used and includes consideration of the interrelatedness between both the implementtion outcomes and the clinical effectiveness of the intervention being implemented. Thus, it is particularly well-suited for effectiveness-implementation hybrid trials ( Curran et al., 2012 ; also see Landes et al., this issue). RE-AIM stands for Reach, Effectiveness (of the clinical or preventive intervention), Adoption, Implementation, and Maintenance. Each metric is important for determining the overall public health impact of the implementation, but they are somewhat interdependent. As such, RE-AIM dimensions can be presented in some combination, such as the “public health impact” metric (reach rate multiplied by the effect size of the intervention) ( Glasgow, Klesges, Dzewaltowski, Estabrooks, & Vogt, 2006 ). RE-AIM is one in a class of evaluation frameworks. For a review, see Tabak, Khoong, Chambers, and Brownson (2012) .
4. Resources for Quantitative Evaluation in Implementation Research
There are a number of useful resources for the quantitative measures used to evaluate implementation research studies. First is the Instrument Review Project affiliated with the Society for Implementation Research Collaboration ( Lewis, Stanick, et al., 2015 ). The results of this systematic review of measures indicated significant variability in the coverage of measures across implementation outcomes and salient determinants of implementation (commonly referred to as barriers and facilitators). The authors reviewed each identified measure for the psychometric properties of internal consistency, structural validity, predictive validity, having norms, responsiveness, and usability (pragmatism). Few measures were deemed high-quality and psychometrically sound due in large part to not using gold-standard measure development methods. This review is ongoing and a website ( https://societyforimplementationresearchcollaboration.org/sirc-instrument-project/ ) is continuously updated to reflect completed work, as well as emerging measures in the field, and is available to members of the society. A number of articles and book chapters provide critical discussions of the state of measurement in implementation research, noting the need for validation of instruments, use across studies, and pragmatism ( Emmons, Weiner, Fernandez, & Tu, 2012 ; Lewis, Fischer, et al., 2015 ; Lewis, Proctor, & Brownson, 2017 ; Martinez, Lewis, & Weiner, 2014 ; Rabin et al., 2016 ).
The RE-AIM website also includes various means of operationalizing the components of this evaluation framework ( http://www.re-aim.org/resources-and-tools/measures-and-checklists/ ) and recent reviews of the use of RE-AIM are also helpful when planning a quantitative evaluation ( Gaglio et al., 2013 ; Glasgow et al., 2019 ). Additionally, the Grid-Enabled Measures Database (GEM), hosted by the National Cancer Institute, has an ever-growing list of implementation-related measures (130 as of July, 2019) with a general rating by users ( https://www.gem-measures.org/public/wsmeasures.aspx?cat=8&aid=1&wid=11 ). Last, Rabin et al. (2016) provide an environmental scan of resources for measures in implementation and dissemination science.
5. Pragmatism: Reducing Measurement Burden
An emphasis in the field has been on finding ways to reduce the measurement burden on implementers, and to a lesser extent on implementation researchers to reduce costs and increase the pace of dissemination ( Glasgow et al., 2019 ; Glasgow & Riley, 2013 ). Powell et al. (2017) established criteria for pragmatic measures that resulted in four distinct categories: (1) acceptable, (2) compatible, (3) easy, and (4) useful; next steps are to develop consensus regarding the most important criteria and developing quantifiable rating criteria for assessing implementation measures on their pragmatism. Advancements have occurred using technology for the evaluation of implementation ( Brown et al., 2015 ). For example, automated and unobtrusive implementation measures can greatly reduce stakeholder burden and increase response rates. As an example, our group ( Wang et al., 2016 ) conducted a proof-of-concept demonstrating the use text analysis to automatically classify the completion of implementation activities using communication logs between implementer and implementing agency. As mentioned earlier in this article, researchers have begun to automate the assessment of implementation fidelity to such evidence-based interventions as motivational interviewing (e.g., Imel et al., 2019 ; Xiao, Imel, Georgiou, Atkins, & Narayanan, 2015 ), and this work is expanding to other intervention protocols to aid in implementation quality ( Smith et al., 2018 ).
6. Example of a Quantitative Evaluation of an Implementation Research Study
We now present the quantitative evaluation plan for an ongoing hybrid type II effectiveness-implementation trial (see Landes et al., this issue ) examining the effectiveness and implementation of the Collaborative Care Model (CCM; Unützer et al., 2002 ) for the management of depression in adult primary care clinics of Northwestern Medicine (Principal Investigator: Smith). CCM is a structure for population-based management of depression involving the primary care provider, a behavioral care manager, and a consulting psychiatrist. A meta-analysis of 79 randomized trials (n=24,308), concluded that CCM is more effective than standard care for short- and long-term treatment of depression ( Archer et al., 2012 ). CCM has also been shown to provide good economic value ( Jacob et al., 2012 ).
Our study involves 11 primary care practices in a rollout implementation design (see Figure 2 ). Randomization in roll-out designs occurs by start time of the implementation strategy, and ensures confidence in the results of the evaluation because known and unknown biases are equally distributed in the case and control groups ( Grimshaw et al., 2000 ). Rollout trials are both powerful and practical as many organizations feel it is unethical to withhold effective interventions, and roll-out designs reduce the logistic and resource demands of delivering the strategy to all units simultaneously. The co-primary aims of the study concern the effectiveness of CCM and its implementation, respectively: 1) Test the effectiveness of CCM to improve depression symptomatology and access to psychiatric services within the primary care environment; and 2) Evaluate the impact of our strategy package on the progressive improvement in speed and quantity of CCM implementation over successive clinics. We will use training and educational implementation strategies, provided to primary care providers, support staff (e.g., nurses, medical assistants), and to practice and system leadership, as well as monitoring and feedback to the practices. Figure 3 summarizes the quantitative evaluation being conducted in this trial using the RE-AIM framework.

Design and Timeline of Randomized Rollout Implementation Trial of CCM
Note. CCM = Collaborative Care Model. Clinics will have a staggered start every 3–4 months randomized using a matching scheme. Pre-implementation assessment period is 4 months. Evaluation of CCM implementation will be a minimum of 24 months at each clinic.

Summative Evaluation Metrics of CCM Implementation Using the RE-AIM Framework
Note. CCM = Collaborative Care Model. EHR = electronic health record.
7.1. EHR and other administrative data sources
As this is a Type 2effectiveness-implementation hybrid trial, Aim 1 encompasses both reach —an implementation outcome—of depression management by CCM within primary care—and the effectiveness of CCM at improving patient and service outcomes. Within RE-AIM, the Public Health Impact metric is effectiveness (effect size) multiplied by reach rate. EHR and administrative data are being used to evaluate the primary implementation outcomes of reach (i.e., the proportion of patients in the practice who are eligible for CCM and who are referred). The reach rates achieved after implementation of CCM can be compared to rates of mental health contact for patients with depression prior to implementation as well as to that achieved by other CCM implementation evaluations in the literature.
The primary effectiveness outcome of CCM is the reduction of patients’ depression symptom severity. De-identified longitudinal patient outcome data from the EHR—principally depression diagnosis and scores on the PHQ-9 ( Kroenke, Spitzer, & Williams, 2001 )—will be analyzed to evaluate the impact of CCM. Other indicators of the effectiveness of CCM will be evaluated as well but are not discussed here as they are likely to be familiar to most readers with knowledge of clinical trials. Service outcomes, from the Institute of Medicine’s Standards of Care ( Institute of Medicine Committee on Crossing the Quality Chasm, 2006 ), centered on providing care that is effective (providing services based on scientific knowledge to all who could benefit and refraining from providing services to those not likely to benefit), timely (reducing waits and sometimes harmful delays for both those who receive and those who give care), and equitable (providing care that does not vary in quality because of personal characteristics such as gender, ethnicity, geographic location, and socioeconomic status). We also sought to provide care that is safe, patient-centered, and efficient.
EHR data will also be used to determine adoption of CCM (i.e., the number of providers with eligible patients who refer to CCM). This can be accomplished by tracking patient screening results and intakes completed by the CCM behavioral care manager within the primary care clinician’s encounter record.
7.2. Speed and quantity of implementation
Achievement of Aim 2 requires an evaluation approach and an appropriate trial design to obtain results that can contribute to generalizable knowledge. A rigorous rollout implementation trial design, with matched-pair randomization to when the practice would change from usual care to CCM was devised. Figure 2 provides a schematic of the design with the timing of the crossover from standard practice to CCM implementation. The first thing one will notice about the design is that the sequential nature of the rollout in which implementation at earlier sites precedes the onset of implementation in later sites. This suggests the potential to learn from successes and challenges to improve implementation efficiency (speed) over time. We will use the Universal SIC® ( Saldana, Schaper, Campbell, & Chapman, 2015 ), a date-based, observational measure, to capture the speed of implementation of various activities needed to successfully implement CCM, such as “establishing a workflow”, “preparing for training”, and “behavioral care manager hired.” This measure is completed by practice staff and members of the implementation team based on their direct knowledge of precisely when the activity was completed. Using the completion date of each activity, we will analyze the time elapsed in each practice to complete each stage (Duration Score). Then, we will calculate the percentage of stages completed (Proportion Score). These scores can then be used in statistical analyses to understand the factors that contributed to timely stage completion, the number of stages that are important for successful program implementation by relating the SIC to other implementation outcomes, such as reach rate; and simply whether there was a degree of improvement in implementation efficiency and scale as the rollout took place. That is, were more stages completed more quickly by later sites compared to earlier ones in the rollout schedule. This analysis comprises the implementation domain of RE-AIM. It will be used in combination with other metrics from the EHR to determine the fidelity of implementation, which is consistent with RE-AIM.
7.3. Surveys
To understand the process and the determinants of implementation—the factors that impede or promote adoption and delivery with fidelity—a battery of surveys was administered at multiple time-points to key staff members in each practice. One challenge with large-scale implementation research is the need for measures to be both psychometrically sound as well as pragmatic. With this in mind, we adapted a set of questions for the current trial that were developed and validated in prior studies. This low-burden assessment is comprised of items from four validated implementation surveys concerning factors at the inner setting of the organization: the Implementation Leadership Scale ( Aarons, Ehrhart, & Farahnak, 2014 ), the Evidence-Based Practice Attitude Scale ( Aarons, 2004 ), the Clinical Effectiveness and Evidence-Based Practice Questionnaire ( Upton & Upton, 2006 ), and the Organizational Change Recipient’s Belief Scale ( Armenakis, Bernerth, Pitts, & Walker, 2007 ). In a prior study, we used confirmatory factor analysis to evaluate the four scales after shortening for pragmatism and tailoring the wording of the items (when appropriate) to the context under investigation in the study (Smith et al., under review). Further, different versions of the survey were created for administration to the various professional roles in the organization. Results showed that the scales were largely replicated after shortening and tailoring; internal consistencies were acceptable; and the factor structures were statistically invariant across professional role groups. The same process was undertaken for this study with versions of the battery developed for providers, practice leadership, support staff, and the behavioral care managers. The survey was administered immediately after initial training in the model and then again at 4, 12, and 24 months. Items were added after the baseline survey regarding the process of implementation thus far and the most prominent barriers and facilitators to implementation of CCM in the practice. Survey-based evaluation of maintenance in RE-AIM, also called sustainability, will occur via the Clinical Sustainability Assessment Tool ( Luke, Malone, Prewitt, Hackett, & Lin, 2018 ) to key decision makers at multiple levels in the healthcare system.
7.4. Cost of implementation
The costs incurred when adopting and delivering a new clinical intervention are a top reason attributed to lack of adoption of behavioral interventions ( Glasgow & Emmons, 2007 ). While cost-effectiveness and cost-benefit analyses demonstrate the long-term economic benefits associated with the effects of these interventions, they rarely consider the costs to the implementer associated with these endeavors as a unique component ( Ritzwoller, Sukhanova, Gaglio, & Glasgow, 2009 ). As such, decision makers value different kinds of economic evaluations, such as budget impact analysis, which assesses the expected short-term changes in expenditures for a health care organization or system in adopting a new intervention ( Jordan, Graham, Berkel, & Smith, 2019 ), and cost-effectiveness analysis from the perspective of the implementing system and not simply the individual recipient of the evidence-based intervention being implemented ( Raghavan, 2017 ). Eisman and colleagues ( this issue ) discuss economic evaluations in implementation research.
In our study, our economic approach focuses on the cost to Northwestern Medicine to deliver CCM and will incorporate reimbursement from payors to ensure that the costs to the system are recouped in such a way that it can be sustained over time under current models of compensated care. The cost-effectiveness of CCM has been established ( Jacob et al., 2012 ), but we will also quantify the cost of achieving salient health outcomes for the patients involved, such as cost to achieve remission as well as projected costs that would increase remission rates.
7. Conclusions
The field of implementation research has developed methods for conducting quantitative evaluation to summarize the overall, aggregate impact of implementation strategies on salient outcomes. Methods are still emerging to aid researchers in the specification and planning of evaluations for implementation studies (e.g., Smith, 2018 ). However, as noted in the case example, evaluations focused only on the aggregate results of a study should not be done in the absence of ongoing formative evaluations, such as in-protocol audit and feedback and other methods (see Elwy et al., this issue ),and mixed and/or qualitative methods (see Hamilton et al., this issue ). Both of which are critical for interpreting the results of evaluations that aggregate the results of a large trial and gaging the generalizability of the findings. In large part, the intent of quantitative evaluations of large trials in implementation research aligns with its clinical-level counterparts, but with the emphasis on the factors in the service delivery system associated with adoption and delivery of the clinical intervention rather than on the direct recipients of that intervention (see Figure 1 ). The case example shows how both can be accomplished in an effectiveness-implementation hybrid design (see Landes et al., this issue ). This article shows current thinking on quantitative outcome evaluation in the context of implementation research. Given the quickly-evolving nature of the field of implementation research, it is imperative for interested readers to consult the most up-to-date resources for guidance on quantitative evaluation.
- Quantitative evaluation can be conducted in the context of implementation research to determine impact of various strategies on salient outcomes.
- The defining characteristics of implementation research studies are discussed.
- Quantitative evaluation frameworks and measures for key implementation research outcomes are presented.
- Application is illustrated using a case example of implementing collaborative care for depression in primary care practices in a large healthcare system.
Acknowledgements
The authors wish to thank Hendricks Brown who provided input on the development of this article and to the members of the Collaborative Behavioral Health Program research team at Northwestern: Lisa J. Rosenthal, Jeffrey Rado, Grace Garcia, Jacob Atlas, Michael Malcolm, Emily Fu, Inger Burnett-Zeigler, C. Hendricks Brown, and John Csernansky. We also wish to thank the Woman’s Board of Northwestern Memorial Hospital, who generously provided a grant to support and evaluate the implementation and effectiveness of this model of care as it was introduced to the Northwestern Medicine system, and our clinical, operations, and quality partners in Northwestern Medicine’s Central Region.
This study was supported by a grant from the Woman’s Board of Northwestern Memorial Hospital and grant P30DA027828 from the National Institute on Drug Abuse, awarded to C. Hendricks Brown. The opinions expressed herein are the views of the authors and do not necessarily reflect the official policy or position of the Woman’s Board, Northwestern Medicine, the National Institute on Drug Abuse, or any other part of the US Department of Health and Human Services.
List of Abbreviations
Competing interests
None declared.
Declarations
Ethics approval and consent to participate
Not applicable. This study did not involve human subjects.
Availability of data and material
Not applicable.
Publisher's Disclaimer: This is a PDF file of an unedited manuscript that has been accepted for publication. As a service to our customers we are providing this early version of the manuscript. The manuscript will undergo copyediting, typesetting, and review of the resulting proof before it is published in its final citable form. Please note that during the production process errors may be discovered which could affect the content, and all legal disclaimers that apply to the journal pertain.
- Aarons GA (2004). Mental health provider attitudes toward adoption of evidence-based practice: the Evidence-Based Practice Attitude Scale (EBPAS) . Ment Health Serv Res , 6 ( 2 ), 61–74. [ PMC free article ] [ PubMed ] [ Google Scholar ]
- Aarons GA, Ehrhart MG, & Farahnak LR (2014). The implementation leadership scale (ILS): Development of a brief measure of unit level implementation leadership . Implementation Science , 9 . doi: 10.1186/1748-5908-9-45 [ PMC free article ] [ PubMed ] [ CrossRef ] [ Google Scholar ]
- Archer J, Bower P, Gilbody S, Lovell K, Richards D, Gask L,… Coventry P (2012). Collaborative care for depression and anxiety problems . Cochrane Database of Systematic Reviews(10) doi: 10.1002/14651858.CD006525.pub2 [ PubMed ] [ CrossRef ] [ Google Scholar ]
- Armenakis AA, Bernerth JB, Pitts JP, & Walker HJ (2007). Organizational Change Recipients Beliefs Scale: Development of an Assessmetn Instrument . The Journal of Applied Behavioral Science , 42 , 481–505. doi:DOI: 10.1177/0021886307303654 [ CrossRef ] [ Google Scholar ]
- Bauer MS, Damschroder L, Hagedorn H, Smith J, & Kilbourne AM (2015). An introduction to implementation science for the non-specialist . BMC Psychology , 3 ( 1 ), 32. doi: 10.1186/s40359-015-0089-9 [ PMC free article ] [ PubMed ] [ CrossRef ] [ Google Scholar ]
- Bertrand JT, Holtgrave DR, & Gregowski A (2009). Evaluating HIV/AIDS programs in the US and developing countries In Mayer KH & Pizer HF (Eds.), HIV Prevention (pp. 571–590). San Diego: Academic Press. [ Google Scholar ]
- Brown CH, Curran G, Palinkas LA, Aarons GA, Wells KB, Jones L,… Cruden G (2017). An overview of research and evaluation designs for dissemination and implementation . Annual Review of Public Health , 38 ( 1 ), null. doi:doi: 10.1146/annurev-publhealth-031816-044215 [ PMC free article ] [ PubMed ] [ CrossRef ] [ Google Scholar ]
- Brown CH, Mohr DC, Gallo CG, Mader C, Palinkas L, Wingood G,… Poduska J (2013). A computational future for preventing HIV in minority communities: how advanced technology can improve implementation of effective programs . J Acquir Immune Defic Syndr , 63 . doi: 10.1097/QAI.0b013e31829372bd [ PMC free article ] [ PubMed ] [ CrossRef ] [ Google Scholar ]
- Brown CH, PoVey C, Hjorth A, Gallo CG, Wilensky U, & Villamar J (2015). Computational and technical approaches to improve the implementation of prevention programs . Implementation Science , 10 ( Suppl 1 ), A28. doi: 10.1186/1748-5908-10-S1-A28 [ CrossRef ] [ Google Scholar ]
- Cheung K, & Duan N (2014). Design of implementation studies for quality improvement programs: An effectiveness-cost-effectiveness framework . American Journal of Public Health , 104 ( 1 ), e23–e30. doi: 10.2105/ajph.2013.301579 [ PMC free article ] [ PubMed ] [ CrossRef ] [ Google Scholar ]
- Colditz GA, & Emmons KM (2017). The promise and challenges of dissemination and implementation research In Brownson RC, Colditz GA, & Proctor EK (Eds.), Dissemination and implementation research in health: Translating science to practice . New York, NY: Oxford University Press. [ Google Scholar ]
- Curran GM, Bauer M, Mittman B, Pyne JM, & Stetler C (2012). Effectiveness-implementation hybrid designs: Combining elements of clinical effectiveness and implementation research to enhance public health impact . Medical Care , 50 ( 3 ), 217–226. doi: 10.1097/MLR.0b013e3182408812 [ PMC free article ] [ PubMed ] [ CrossRef ] [ Google Scholar ]
- Department of Health and Human Services. (2019). PAR-19–274: Dissemination and Implementation Research in Health (R01 Clinical Trial Optional) . Retrieved from https://grants.nih.gov/grants/guide/pa-files/PAR-19-274.html
- Emmons KM, Weiner B, Fernandez ME, & Tu S (2012). Systems antecedents for dissemination and implementation: a review and analysis of measures . Health Educ Behav , 39 . doi: 10.1177/1090198111409748 [ PMC free article ] [ PubMed ] [ CrossRef ] [ Google Scholar ]
- Gaglio B, & Glasgow RE (2017). Evaluation approaches for dissemination and implementation research In Brownson R, Colditz G, & Proctor E (Eds.), Dissemination and Implementation Research in Health: Translating Science into Practice (2nd ed., pp. 317–334). New York: Oxford University Press. [ Google Scholar ]
- Gaglio B, Shoup JA, & Glasgow RE (2013). The RE-AIM Framework: A systematic review of use over time . American Journal of Public Health , 103 ( 6 ), e38–e46. doi: 10.2105/ajph.2013.301299 [ PMC free article ] [ PubMed ] [ CrossRef ] [ Google Scholar ]
- Glasgow RE, & Emmons KM (2007). How can we increase translation of research into practice? Types of evidence needed . Annual Review of Public Health , 28 , 413–433. [ PubMed ] [ Google Scholar ]
- Glasgow RE, Harden SM, Gaglio B, Rabin B, Smith ML, Porter GC,… Estabrooks PA (2019). RE-AIM Planning and Evaluation Framework: Adapting to New Science and Practice With a 20-Year Review . Frontiers in Public Health , 7 ( 64 ). doi: 10.3389/fpubh.2019.00064 [ PMC free article ] [ PubMed ] [ CrossRef ] [ Google Scholar ]
- Glasgow RE, Klesges LM, Dzewaltowski DA, Estabrooks PA, & Vogt TM (2006). Evaluating the impact of health promotion programs: using the RE-AIM framework to form summary measures for decision making involving complex issues . Health Education Research , 21 ( 5 ), 688–694. doi: 10.1093/her/cyl081 [ PubMed ] [ CrossRef ] [ Google Scholar ]
- Glasgow RE, & Riley WT (2013). Pragmatic measures: what they are and why we need them . Am J Prev Med , 45 . doi: 10.1016/j.amepre.2013.03.010 [ PubMed ] [ CrossRef ] [ Google Scholar ]
- Glasgow RE, Vogt TM, & Boles SM (1999). Evaluating the public health impact of health promotion interventions: The RE-AIM framework . American Journal of Public Health , 89 ( 9 ), 1322–1327. doi: 10.2105/AJPH.89.9.1322 [ PMC free article ] [ PubMed ] [ CrossRef ] [ Google Scholar ]
- Grimshaw J, Campbell M, Eccles M, & Steen N (2000). Experimental and quasi-experimental designs for evaluating guideline implementation strategies . Family practice , 17 Suppl 1 , S11–16. doi: 10.1093/fampra/17.suppl_1.s11 [ PubMed ] [ CrossRef ] [ Google Scholar ]
- Imel ZE, Pace BT, Soma CS, Tanana M, Hirsch T, Gibson J,… Atkins, D. C. (2019). Design feasibility of an automated, machine-learning based feedback system for motivational interviewing . Psychotherapy , 56 ( 2 ), 318–328. doi: 10.1037/pst0000221 [ PubMed ] [ CrossRef ] [ Google Scholar ]
- Institute of Medicine Committee on Crossing the Quality Chasm. (2006). Adaption to mental health and addictive disorder: Improving the quality of health care for mental and substanceuse conditions . Retrieved from Washington, D.C.: [ Google Scholar ]
- Jacob V, Chattopadhyay SK, Sipe TA, Thota AB, Byard GJ, & Chapman DP (2012). Economics of collaborative care for management of depressive disorders: A community guide systematic review . American Journal of Preventive Medicine , 42 ( 5 ), 539–549. doi: 10.1016/j.amepre.2012.01.011 [ PubMed ] [ CrossRef ] [ Google Scholar ]
- Jordan N, Graham AK, Berkel C, & Smith JD (2019). Budget impact analysis of preparing to implement the Family Check-Up 4 Health in primary care to reduce pediatric obesity . Prevention Science , 20 ( 5 ), 655–664. doi: 10.1007/s11121-018-0970-x [ PMC free article ] [ PubMed ] [ CrossRef ] [ Google Scholar ]
- Kroenke K, Spitzer R, & Williams JW (2001). The PHQ-9 . Journal of General Internal Medicine , 16 ( 9 ), 606–613. doi: 10.1046/j.1525-1497.2001.016009606.x [ PMC free article ] [ PubMed ] [ CrossRef ] [ Google Scholar ]
- Landsverk J, Brown CH, Smith JD, Chamberlain P, Palinkas LA, Ogihara M,… Horwitz SM (2017). Design and analysis in dissemination and implementation research In Brownson RC, Colditz GA, & Proctor EK (Eds.), Dissemination and implementation research in health: Translating research to practice (2nd ed., pp. 201–227). New York: Oxford University Press. [ Google Scholar ]
- Lewis CC, Fischer S, Weiner BJ, Stanick C, Kim M, & Martinez RG (2015). Outcomes for implementation science: an enhanced systematic review of instruments using evidence-based rating criteria . Implementation Science , 10 ( 1 ), 155. doi: 10.1186/s13012-015-0342-x [ PMC free article ] [ PubMed ] [ CrossRef ] [ Google Scholar ]
- Lewis CC, Proctor EK, & Brownson RC (2017). Measurement issues in dissemination and implementation research In Brownson RC, Colditz GA, & Proctor EK (Eds.), Dissemination and implementation research in health: Translating research to practice (2nd ed., pp. 229–244). New York: Oxford University Press. [ Google Scholar ]
- Lewis CC, Stanick CF, Martinez RG, Weiner BJ, Kim M, Barwick M, & Comtois KA (2015). The Society for Implementation Research Collaboration Instrument Review Project: A methodology to promote rigorous evaluation . Implementation Science , 10 ( 1 ), 2. doi: 10.1186/s13012-014-0193-x [ PMC free article ] [ PubMed ] [ CrossRef ] [ Google Scholar ]
- Luke DA, Calhoun A, Robichaux CB, Elliott MB, & Moreland-Russell S (2014). The Program Sustainability Assessment Tool: A new instrument for public health programs . Preventing Chronic Disease , 11 , E12. doi: 10.5888/pcd11.130184 [ PMC free article ] [ PubMed ] [ CrossRef ] [ Google Scholar ]
- Luke DA, Malone S, Prewitt K, Hackett R, & Lin J (2018). The Clinical Sustainability Assessment Tool (CSAT): Assessing sustainability in clinical medicine settings . Paper presented at the Conference on the Science of Dissemination and Implementation in Health, Washington, DC. [ Google Scholar ]
- Martinez RG, Lewis CC, & Weiner BJ (2014). Instrumentation issues in implementation science . Implement Sci , 9 . doi: 10.1186/s13012-014-0118-8 [ PMC free article ] [ PubMed ] [ CrossRef ] [ Google Scholar ]
- Powell BJ, Stanick CF, Halko HM, Dorsey CN, Weiner BJ, Barwick MA,… Lewis CC (2017). Toward criteria for pragmatic measurement in implementation research and practice: a stakeholder-driven approach using concept mapping . Implementation Science , 12 ( 1 ), 118. doi: 10.1186/s13012-017-0649-x [ PMC free article ] [ PubMed ] [ CrossRef ] [ Google Scholar ]
- Powell BJ, Waltz TJ, Chinman MJ, Damschroder LJ, Smith JL, Matthieu MM,… Kirchner JE (2015). A refined compilation of implementation strategies: results from the Expert Recommendations for Implementing Change (ERIC) project . Implement Sci , 10 . doi: 10.1186/s13012-015-0209-1 [ PMC free article ] [ PubMed ] [ CrossRef ] [ Google Scholar ]
- Proctor E, Silmere H, Raghavan R, Hovmand P, Aarons G, Bunger A,… Hensley M (2011). Outcomes for implementation research: conceptual distinctions, measurement challenges, and research agenda . Adm Policy Ment Health Ment Health Serv Res , 38 . doi: 10.1007/s10488-010-0319-7 [ PMC free article ] [ PubMed ] [ CrossRef ] [ Google Scholar ]
- Rabin BA, Lewis CC, Norton WE, Neta G, Chambers D, Tobin JN,… Glasgow RE (2016). Measurement resources for dissemination and implementation research in health . Implementation Science , 11 ( 1 ), 42. doi: 10.1186/s13012-016-0401-y [ PMC free article ] [ PubMed ] [ CrossRef ] [ Google Scholar ]
- Raghavan R (2017). The role of economic evaluation in dissemination and implementation research In Brownson RC, Colditz GA, & Proctor EK (Eds.), Dissemination and implementation research in health: Translating science to practice (2nd ed.). New York: Oxford University Press. [ Google Scholar ]
- Ritzwoller DP, Sukhanova A, Gaglio B, & Glasgow RE (2009). Costing behavioral interventions: a practical guide to enhance translation . Annals of Behavioral Medicine , 37 ( 2 ), 218–227. [ PubMed ] [ Google Scholar ]
- Saldana L, Schaper H, Campbell M, & Chapman J (2015). Standardized Measurement of Implementation: The Universal SIC . Implementation Science , 10 ( 1 ), A73. doi: 10.1186/1748-5908-10-s1-a73 [ CrossRef ] [ Google Scholar ]
- Schoenwald S, Garland A, Chapman J, Frazier S, Sheidow A, & Southam-Gerow M (2011). Toward the effective and efficient measurement of implementation fidelity . Admin Policy Mental Health Mental Health Serv Res , 38 . doi: 10.1007/s10488-010-0321-0 [ PMC free article ] [ PubMed ] [ CrossRef ] [ Google Scholar ]
- Smith JD (2018). An implementation research logic model: A step toward improving scientific rigor, transparency, reproducibility, and specification . Implementation Science , 14 ( Supp 1 ), S39. [ Google Scholar ]
- Smith JD, Berkel C, Jordan N, Atkins DC, Narayanan SS, Gallo C,… Bruening MM (2018). An individually tailored family-centered intervention for pediatric obesity in primary care: Study protocol of a randomized type II hybrid implementation-effectiveness trial (Raising Healthy Children study) . Implementation Science , 13 ( 11 ), 1–15. doi: 10.1186/s13012-017-0697-2 [ PMC free article ] [ PubMed ] [ CrossRef ] [ Google Scholar ]
- Smith JD, Li DH, Hirschhorn LR, Gallo C, McNulty M, Phillips GI,… Benbow ND (2019). Landscape of HIV implementation research funded by the National Institutes of Health: A mapping review of project abstracts (submitted for publication) . [ PMC free article ] [ PubMed ] [ Google Scholar ]
- Smith JD, Rafferty MR, Heinemann AW, Meachum MK, Villamar JA, Lieber RL, & Brown CH (under review). Evaluation of the factor structure of implementation research measures adapted for a novel context and multiple professional roles . [ PMC free article ] [ PubMed ] [ Google Scholar ]
- Stetler CB, Legro MW, Wallace CM, Bowman C, Guihan M, Hagedorn H,… Smith JL (2006). The role of formative evaluation in implementation research and the QUERI experience . Journal of General Internal Medicine , 21 ( 2 ), S1. doi : 10.1007/s11606-006-0267-9 [ PMC free article ] [ PubMed ] [ CrossRef ] [ Google Scholar ]
- Tabak RG, Khoong EC, Chambers DA, & Brownson RC (2012). Bridging research and practice: Models for dissemination and implementation research . American Journal of Preventive Medicine , 43 ( 3 ), 337–350. [ PMC free article ] [ PubMed ] [ Google Scholar ]
- The Health Foundation. (2015). Evaluation: What to consider. Commonly asked questions about how to approach evaluation of quality improvement in health care . Retrieved from London, England: https://www.health.org.uk/sites/default/files/EvaluationWhatToConsider.pdf [ Google Scholar ]
- Unützer J, Katon W, Callahan CM, Williams J, John W, Hunkeler E, Harpole L,… Investigators, f. t. I. (2002). Collaborative care management of late-life depression in the primary care setting: A randomized controlled trial . JAMA , 288 ( 22 ), 2836–2845. doi: 10.1001/jama.288.22.2836 [ PubMed ] [ CrossRef ] [ Google Scholar ]
- Upton D, & Upton P (2006). Development of an evidence-based practice questionnaire for nurses . Journal of Advanced Nursing , 53 ( 4 ), 454–458. [ PubMed ] [ Google Scholar ]
- Wang D, Ogihara M, Gallo C, Villamar JA, Smith JD, Vermeer W,… Brown CH (2016). Automatic classification of communication logs into implementation stages via text analysis . Implementation Science , 11 ( 1 ), 1–14. doi: 10.1186/s13012-016-0483-6 [ PMC free article ] [ PubMed ] [ CrossRef ] [ Google Scholar ]
- Wyman PA, Henry D, Knoblauch S, & Brown CH (2015). Designs for testing group-based interventions with limited numbers of social units: The dynamic wait-listed and regression point displacement designs . Prevention Science , 16 ( 7 ), 956–966. doi : 10.1007/s11121-014-0535-6 [ PMC free article ] [ PubMed ] [ CrossRef ] [ Google Scholar ]
- Xiao B, Imel ZE, Georgiou PG, Atkins DC, & Narayanan SS (2015). “Rate My Therapist”: Automated detection of empathy in drug and alcohol counseling via speech and language processing . PLoS ONE , 10 ( 12 ), e0143055. doi: 10.1371/journal.pone.0143055 [ PMC free article ] [ PubMed ] [ CrossRef ] [ Google Scholar ]
Click through the PLOS taxonomy to find articles in your field.
For more information about PLOS Subject Areas, click here .
Loading metrics
Open Access
Peer-reviewed
Research Article
The relationship between workload and burnout among nurses: The buffering role of personal, social and organisational resources
Roles Conceptualization, Data curation, Formal analysis, Investigation, Methodology, Project administration, Resources, Software, Validation, Visualization, Writing – original draft, Writing – review & editing
Affiliation Institute of Occupational, Social and Environmental Medicine, University Medical Center of the Johannes Gutenberg University Mainz, Mainz, Germany
Roles Conceptualization, Data curation, Investigation, Methodology, Project administration, Resources, Software, Writing – review & editing
Roles Conceptualization, Funding acquisition, Investigation, Resources, Writing – review & editing
Roles Conceptualization, Investigation, Methodology, Project administration, Resources, Supervision, Writing – review & editing
Affiliation Institute for Health Services Research in Dermatology and Nursing (IVDP), University Medical Center Hamburg-Eppendorf, Hamburg, Germany
Roles Conceptualization, Investigation, Methodology, Resources, Supervision, Writing – review & editing
Affiliations Institute for Health Services Research in Dermatology and Nursing (IVDP), University Medical Center Hamburg-Eppendorf, Hamburg, Germany, Department for Occupational Medicine, Hazardous Substances and Health Science, Institution for Accident Insurance and Prevention in the Health and Welfare Services (BGW), Hamburg, Germany
Roles Conceptualization, Funding acquisition, Investigation, Methodology, Project administration, Resources, Supervision, Writing – review & editing
¶ ‡ These authors are joint senior authors on this work.
Affiliations Institute of Occupational, Social and Environmental Medicine, University Medical Center of the Johannes Gutenberg University Mainz, Mainz, Germany, Federal Institute for Occupational Safety and Health (BAuA), Berlin, Germany
Roles Supervision, Writing – review & editing
* E-mail: [email protected]

- Elisabeth Diehl,
- Sandra Rieger,
- Stephan Letzel,
- Anja Schablon,
- Albert Nienhaus,
- Luis Carlos Escobar Pinzon,
- Pavel Dietz
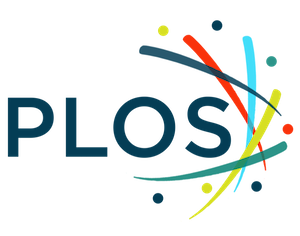
- Published: January 22, 2021
- https://doi.org/10.1371/journal.pone.0245798
- Peer Review
- Reader Comments
Workload in the nursing profession is high, which is associated with poor health. Thus, it is important to get a proper understanding of the working situation and to analyse factors which might be able to mitigate the negative effects of such a high workload. In Germany, many people with serious or life-threatening illnesses are treated in non-specialized palliative care settings such as nursing homes, hospitals and outpatient care. The purpose of the present study was to investigate the buffering role of resources on the relationship between workload and burnout among nurses. A nationwide cross-sectional survey was applied. The questionnaire included parts of the Copenhagen Psychosocial Questionnaire (COPSOQ) (scale ‘quantitative demands’ measuring workload, scale ‘burnout’, various scales to resources), the resilience questionnaire RS-13 and single self-developed questions. Bivariate and moderator analyses were performed. Palliative care aspects, such as the ‘extent of palliative care’, were incorporated to the analyses as covariates. 497 nurses participated. Nurses who reported ‘workplace commitment’, a ‘good working team’ and ‘recognition from supervisor’ conveyed a weaker association between ‘quantitative demands’ and ‘burnout’ than those who did not. On average, nurses spend 20% of their working time with palliative care. Spending more time than this was associated with ‘burnout’. The results of our study imply a buffering role of different resources on burnout. Additionally, the study reveals that the ‘extent of palliative care’ may have an impact on nurse burnout, and should be considered in future studies.
Citation: Diehl E, Rieger S, Letzel S, Schablon A, Nienhaus A, Escobar Pinzon LC, et al. (2021) The relationship between workload and burnout among nurses: The buffering role of personal, social and organisational resources. PLoS ONE 16(1): e0245798. https://doi.org/10.1371/journal.pone.0245798
Editor: Adrian Loerbroks, Universtiy of Düsseldorf, GERMANY
Received: July 30, 2020; Accepted: January 7, 2021; Published: January 22, 2021
Copyright: © 2021 Diehl et al. This is an open access article distributed under the terms of the Creative Commons Attribution License , which permits unrestricted use, distribution, and reproduction in any medium, provided the original author and source are credited.
Data Availability: According to the Ethics Committee of the Medical Association of Rhineland-Palatinate (Study ID: 837.326.16 (10645)), the Institute of Occupational, Social and Environmental Medicine of the University Medical Center of the University Mainz is specified as data holding organization. The institution is not allowed to share the data publically in order to guarantee anonymity to the institutions that participated in the survey because some institution-specific information could be linked to specific institutions. The data set of the present study is stored on the institution server at the University Medical Centre of the University of Mainz and can be requested for scientific purposes via the institution office. This ensures that data will be accessible even if the authors of the present paper change affiliation. Postal address: University Medical Center of the University of Mainz, Institute of Occupational, Social and Environmental Medicine, Obere Zahlbacher Str. 67, D-55131 Mainz. Email address: [email protected] .
Funding: The research was funded by the BGW - Berufsgenossenschaft für Gesundheitsdienst und Wohlfahrtspflege (Institution for Statutory Accident Insurance and Prevention in Health and Welfare Services). The funders had no role in study design, data collection and analysis, decision to publish, or preparation of the manuscript.
Competing interests: I have read the journal’s policy and the authors of this manuscript have the following competing interests: The project was funded by the BGW - Berufsgenossenschaft für Gesundheitsdienst und Wohlfahrtspflege (Institution for Statutory Accident Insurance and Prevention in Health and Welfare Services). The BGW is responsible for the health concerns of the target group investigated in the present study, namely nurses. Prof. Dr. A. Nienhaus is head of the Department for Occupational Medicine, Hazardous Substances and Health Science of the BGW and co-author of this publication. All other authors declare to have no potential conflict of interest. This does not alter our adherence to PLOS ONE policies on sharing data and materials.
Introduction
Our society has to face the challenge of a growing number of older people [ 1 ], combined with an expected shortage of skilled workers, especially in nursing care [ 2 ]. At the same time, cancer patients, patients with non-oncological diseases, multimorbid patients [ 3 ] and patients suffering from dementia [ 4 ] are to benefit from palliative care. In Germany, palliative care is divided into specialised and general palliative care ( Table 1 ). The German Society for Palliative Medicine (DGP) estimated that 90% of dying people are in need of palliative care, but only 10% of them are in need of specialised palliative care, because of more complex needs, such as complex pain management [ 5 ]. The framework of specialised palliative care encompasses specialist outpatient palliative care, inpatient hospices and palliative care units in hospitals. In Germany, most nurses in specialised palliative care have an additional qualification [ 6 ]. Further, nurses in specialist palliative care in Germany have fewer patients to care for than nurses in other fields which results in more time for the patients [ 7 ]. Most people are treated within general palliative care in non-specialized palliative care settings, which is provided by primary care suppliers with fundamental knowledge of palliative care. These are GPs, specialists (e.g. oncologists) and, above all, staff in nursing homes, hospitals and outpatient care [ 8 ]. Nurses in general palliative care have basic skills in palliative care from their education. However, there is no data available on the extent of palliative care they provide, or information on an additional qualification in palliative care. Palliative care experts from around the world consider the education and training of all staff in the fundamentals of palliative care to be essential [ 9 ] and a study conducted in Italy revealed that professional competency of palliative care nurses was positively associated with job satisfaction [ 10 ]. Thus, it is possible that the extent of palliative care or an additional qualification in palliative care may have implications on the working situation and health status of nurses. In Germany, there are different studies which concentrate on people dying in hospitals or nursing homes and the associated burden on the institution’s staff [ 11 , 12 ], but studies considering palliative care aspects concentrate on specialised palliative care settings [ 6 , 13 , 14 ]. Because the working conditions of nurses in specialised and general palliative care are somewhat different, as stated above, this paper focuses on nurses working in general palliative care, in other words, in non-specialized palliative care settings.
- PPT PowerPoint slide
- PNG larger image
- TIFF original image
https://doi.org/10.1371/journal.pone.0245798.t001
Burnout is a large problem in social professions, especially in health care worldwide [ 19 ] and is consistently associated with nurses intention to leave their profession [ 20 ]. Burnout is a state of emotional, physical, and mental exhaustion caused by a long-term mismatch of the demands associated with the job and the resources of the worker [ 21 ]. One of the causes for the alarming increase in nursing burnout is their workload [ 22 , 23 ]. Workload can be either qualitative (pertaining to the type of skills and/or effort needed in order to perform work tasks) or quantitative (the amount of work to be done and the speed at which it has to be performed) [ 24 ].
Studies analysing burnout in nursing have recognised different coping strategies, self-efficacy, emotional intelligence factors, social support [ 25 , 26 ], the meaning of work and role clarity [ 27 ] as protective factors. Studies conducted in the palliative care sector identified empathy [ 28 ], attitudes toward death, secure attachment styles, and meaning and purpose in life as protective factors [ 29 ]. Individual factors such as spirituality and hobbies [ 30 ], self-care [ 31 ], coping strategies for facing the death of a patient [ 32 ], physical activity [ 33 ] and social resources, like social support [ 33 , 34 ], the team [ 6 , 13 ] and time for patients [ 32 ] were identified, as effectively protecting against burnout. These studies used qualitative or descriptive methods or correlation analyses in order to investigate the relationship between variables. In contrast to this statistical approach, fewer studies examined the buffering/moderating role of resources on the relationship between workload and burnout in nursing. A moderator variable affects the direction and/or the strength of the relationship between two other variables [ 35 ]. A previous study has showed resilience as being a moderator for emotional exhaustion on health [ 36 ], and other studies revealed professional commitment or social support moderating job demands on emotional exhaustion [ 37 , 38 ]. Furthermore, work engagement and emotional intelligence was recognised as a moderator in the work demand and burnout relationship [ 39 , 40 ].
We have analysed the working situation of nurses using the Rudow Stress-Strain-Resources model [ 41 ]. According to this model, the same stressor can lead to different strains in different people depending on available resources. These resources can be either individual, social or organisational. Individual resources are those resources which are owned by an individual. This includes for example personal capacities such as positive thinking as well as personal qualifications. Social resources consist of the relationships an individual has, this includes for example relationships at work as well as in his private life. Organisational resources refer to the concrete design of the workplace and work organisation. For example, nurses reporting a good working team may experience workload as less threatening and disruptive because a good working team gives them a feeling of security, stability and belonging. According to Rudow, individual, social or organisational resources can buffer/moderate the negative effects of job demands (stressors) on, for example, burnout (strain).
Nurses’ health may have an effect on the quality of the services offered by the health care system [ 42 ], therefore, it is of great interest to do everything possible to preserve their health. This may be achieved by reducing the workload and by strengthening the available resources. However, to the best of our knowledge, we are not aware of any study which considers palliative care aspects within general palliative care in Germany. Therefore, the aim of the study was to investigate the buffering role of resources on the relationship between workload (‘quantitative demands’) and burnout among nurses. Palliative care aspects, such as information on the extent of palliative care were incorporated to the analyses as covariates.
Study design and participants
An exploratory cross-sectional study was conducted in 2017. In Germany, there is no national register for nurses. Data for this study were collected from a stratified 10% random sample of a database with outpatient facilities, hospitals and nursing homes in Germany from the Institution for Statutory Accident Insurance and Prevention in Health and Welfare Services in Germany. This institution is part of the German social security system. It is the statutory accident insurer for nonstate institutions in the health and welfare services in Germany and thus responsible for the health concerns of the target group investigated in the present study, namely nurses. Due to data protection rules, this institution was also responsible for the first contact with the health facilities. 126 of 3,278 (3.8%) health facilities agreed to participate in the survey. They informed the study team about how many nurses worked in their institution, and whether the nurses would prefer to answer a paper-and-pencil questionnaire (with a pre-franked envelope) or an online survey (with an access code ). 2,982 questionnaires/access codes were sent out to the participating health facilities (656 to outpatient care, 160 to hospitals and 2,166 to nursing homes), where they were distributed to the nurses ( S1 Table ). Participation was voluntary and anonymous. Informed consent was obtained written at the beginning of the questionnaire. Approval to perform the study was obtained by the ethics committee of the State Chamber of Medicine in Rhineland-Palatinate (Clearance number 837.326.16 (10645)).
Questionnaire
The questionnaire contained questions regarding i) nurse’s sociodemographic information and information on current profession as well as ii) palliative care aspects. Furthermore, iii) parts of the German version of the Copenhagen Psychosocial Questionnaire (COPSOQ), iv) a resilience questionnaire [RS-13] and v) single questions relating to resources were added.
i) Sociodemographic information and information on current profession.
The nurse’s sociodemographic information and information on current profession included the variables ‘age’, ‘gender’, ‘marital status’, ‘education’, ‘professional qualification’, ‘working area’, ‘professional experience’ and ‘extent of employment’.
ii) Palliative care aspects.
Palliative care aspects included self-developed questions on ‘additional qualification in palliative care’, the ‘number of patients’ deaths within the last month (that the nurses cared for personally)’ and the ‘extent of palliative care’. The latter was evaluated by asking: how much of your working time (as a percentage) do you spend with care of palliative patients? The first two items were already used in the pilot study. The pilot study consisted of a qualitative part, where interviews with experts in general and specialised palliative care were performed [ 43 ]. These interviews were used to develop a standardized questionnaire which was used for a cross-sectional pilot survey [ 6 , 44 ].
iii) Copenhagen Psychosocial Questionnaire (COPSOQ).
The questionnaire included parts of the German standard version of the Copenhagen Psychosocial Questionnaire (COPSOQ) [ 45 ]. The COPSOQ is a valid and reliable questionnaire for the assessment of psychosocial work environmental factors and health in the workplace [ 46 , 47 ]. The scales selected were ‘quantitative demands’ (four items, for example: “Do you have to work very fast?”) measuring workload, ‘burnout’ (six items, for example: “How often do you feel emotionally exhausted?”), ‘meaning of work’ (three items, for example: “Do you feel that the work you do is important?”) and ‘workplace commitment’ (four items, for example: “Do you enjoy telling others about your place of work?”).
iv) Resilience questionnaire RS-13.
The RS-13 questionnaire is the short German version of the RS-25 questionnaire developed by Wagnild & Young [ 48 ]. The questionnaire postulates a two-dimensional structure of resilience formed by the factors “personal competence” and “acceptance of self and life”. The RS-13 questionnaire measures resilience with 13 items on a 7-point scale (1 = I do not agree, 7 = I totally agree with different statements) and has been validated in representative samples [ 49 , 50 ]. The results of the questionnaire were grouped into persons with low, moderate or high resilience.
v) Questions on resources.
Single questions on personal, social and organizational resources assessed the nurses’ views of these resources in being helpful in dealing with the demands of their work. Further, single questions collected the agreement to different statements such as ‘Do you receive recognition for your work from the supervisor? ’ (see Table 4 ). These resources were frequently reported in the pilot study by nurses in specialised palliative care [ 6 ].
Data preparation and analysis
The data from the paper-and-pencil and online questionnaires were merged, and data cleaning was done (e.g. questionnaires without specification to nursing homes, hospitals or outpatient care were excluded). The scales selected from the COPSOQ were prepared according to the COPSOQ guidelines. In general, COPSOQ items have a 5-point Likert format, which are then transformed into a 0 to 100 scale. The scale score is calculated as the mean of the items for each scale, if at least half of the single items had valid answers. Nurses who answered less than half of the items in a scale were recorded as missing. If at least half of the items were answered, the scale value was calculated as the average of the items answered [ 46 ]. High values for the scales ‘quantitative demands‘ and ‘burnout‘ were considered negative, while high values for the scales ‘meaning of work’ and ‘workplace commitment’ were considered positive. The proportion of missing values for single scale items was between 0.5% and 2.7%. Cronbach’s Alpha was used to assess the internal consistency of the scales. A Cronbach’s Alpha > 0.7 was regarded as acceptable [ 35 ]. The score of the RS-13 questionnaire ranges from 13 to 91. The answers were grouped according to the specifications in groups with low resilience (score 13–66), moderate resilience (67–72) and high resilience (73–91) [ 49 ]. The categorical resource variables were dichotomised (example: not helpful/little helpful vs. quite helpful/very helpful).
The study was conceptualised as an exploratory study. Consequently, no prior hypotheses were formulated, so the p-values merely enable the recognition of any statistically noteworthy findings [ 51 ]. Descriptive statistics (absolute and relative frequency, M = mean, SD = standard deviation) were used to depict the data. Bivariate analyses (Pearson correlation, t-tests, analysis of variance) were performed to infer important variables for the regression-based moderation analysis. Variables which did not fulfil all the conditions for linear regression analysis were recoded as categorical variables [ 35 ]. The variable ‘extent of palliative care’ was categorised as ‘≤ 20 percent of working time’ vs. ‘> 20 percent of working time’ due to the median of the variable (median = 20).
The first step with regard to the moderation analysis was to determine the resource variables. Therefore all resource variables that reached a p-value < 0.05 in the bivariate analysis with the scale ‘burnout’ were further analysed (scale ‘meaning of work’, scale ‘workplace commitment’, variables presented in Table 4 ). The moderator analysis was conducted using the PROCESS program developed by Andrew F. Hayes. First, scales were mean-centred to reduce possible scaling problems and multicollinearity. Secondly, for all significant resource variables the following analysis were done: the ‘quantitative demand’, one resource (one per model) and the interaction term between the ‘quantitative demand’ and the resource, as well as the covariates ‘age’, ‘gender’, ‘working area’, ‘extent of employment’, the ‘extent of palliative care’ and the ‘number of patient deaths within the last month’ were added to the moderator analysis, in order to control for confounding influence. If the interaction term between the ‘quantitative demand’ and the resource accounted for significantly more variance than without interaction term (change in R 2 denoted as ΔR 2 , p < 0.05), a moderator effect of the resource was present. The interaction of the variables (± 1 SD the mean or variable manifestation such as yes and no) was plotted.
All the statistical calculations were performed using the Statistical Package for Social Science (SPSS, version 23.5) and the PROCESS macro for SPSS (version 3.5 by Hayes) for the moderator analysis.
Of the 2,982 questionnaires/access codes sent out, 497 were eligible for the analysis. The response rate was 16.7% (response rate of outpatient care 14.6%, response rate of hospitals 18.1% and response rate of nursing homes 16.0%). Since only n = 29 nurses from hospitals participated, these were excluded from data analysis. After data cleaning , the final number of participants was n = 437.
Descriptive results
The basic characteristics of the study population are presented in Table 2 . The average age of the nurses was 42.8 years, and 388 (89.6%) were female. In total, 316 nurses answered the question how much working time they spend caring for palliative patients. Sixteen (5.1%) nurses reported spending no time caring for palliative patients, 124 (39.2%) nurses reported between 1% to 10%, 61 (19.30%) nurses reported between 11% to 20% and 115 (36.4%) nurses reported spending more than 20% of their working time for caring for palliative patients. Approximately one-third (n = 121, 27.7%) of the nurses in this study did not answer this question. One hundred seventeen (29.5%) nurses reported 4 or more patient deaths, 218 (54.9%) reported 1 to 3 patient deaths and 62 (15.6%) reported 0 patient deaths within the last month.
https://doi.org/10.1371/journal.pone.0245798.t002
Table 3 presents the mean values and standard deviations of the scales ‘quantitative demands’, ‘burnout’, and the resource scales ‘meaning of work’ and ‘workplace commitment’. All scales achieved a satisfactory level of internal consistency.
https://doi.org/10.1371/journal.pone.0245798.t003
Bivariate analyses
There was a strong positive correlation between the ‘quantitative demands’ and ‘burnout’ scales (r = 0.498, p ≤ 0.01), and a small negative correlation between ‘burnout’ and ‘meaning of work’ (r = -0.222, p ≤ 0.01) and ‘workplace commitment’ (r = -0.240, p ≤ 0.01). Regarding the basic and job-related characteristics of the sample shown in Table 2 , ‘burnout’ was significantly related to ‘extent of palliative care’ (≤ 20% of working time: n = 199, M = 46.06, SD = 20.28; > 20% of working time: n = 115, M = 53.80, SD = 20.24, t(312) = -3.261, p = 0.001). Furthermore, there was a significant effect regarding the ‘number of patient deaths during the last month’ (F (2, 393) = 5.197, p = 0.006). The mean of the burnout score was lower for nurses reporting no patient deaths within the last month than for nurses reporting four or more deaths (n = 62, M = 42.47, SD = 21.66 versus n = 116, M = 52.71, SD = 20.03). There was no association between ‘quantitative demands’ and an ‘additional qualification in palliative care’ (no qualification: n = 328, M = 55.77, SD = 21.10; additional qualification: n = 103, M = 54.39, SD = 20.44, p = 0.559).
The association between ‘burnout’ and the evaluated (categorical) resource variables is presented in Table 4 . Nurses mostly had a lower value on the ‘burnout’ scale when reporting various resources. Only the resources ‘family’, ‘religiosity/spirituality’, ‘gratitude of patients’, ‘recognition through patients/relatives’ and an ‘additional qualification in palliative care’ were not associated with ‘burnout’.
https://doi.org/10.1371/journal.pone.0245798.t004
Moderator analyses
In total, 16 moderation analyses were conducted. Table 5 presents the results of the moderation analyses where a significant moderation was found. For ‘workplace commitment’, there was a positive and significant association between ‘quantitative demands’ and ‘burnout’ (b = 0.47, SE = 0.051, p < 0.001). An increase of one value on the scale ‘quantitative demands’ increased the scale ‘burnout’ by 0.47. ‘Workplace commitment’ was negatively related to ‘burnout’, meaning that a higher degree of ‘workplace commitment’ was related to a lower level of ‘burnout’ (b = -0.11, SE = 0.048, p = 0.030). A model with the interaction term of ‘quantitative demands’ and the resource ‘workplace commitment’ accounted for significantly more variance in ‘burnout’ than a model without interaction term (ΔR 2 = 0.021, p = 0.004). The impact of ‘quantitative demands’ on ‘burnout’ was dependent on ‘workplace commitment’ (b = -0.01, SE = 0.002 p = 0.004). The variables explained 31.9% of the variance in ‘burnout’.
https://doi.org/10.1371/journal.pone.0245798.t005
Regarding the ‘good working team’ resource, the variables ‘quantitative demands’ and ‘burnout’ were positively and significantly associated (b = 0.76, SE = 0.154, p < 0.001), and the variables ‘good working team’ and ‘burnout’ were not associated (b = -3.15, SE = 3.52, p = 0.372). A model with the interaction term of ‘quantitative demands’ and the ‘good working team’ resource accounted for significantly more variance in ‘burnout’ than a model without interaction term (ΔR 2 = 0.011, p = 0.040). The ‘good working team’ resource moderated the impact of ‘quantitative demands’ on ‘burnout’ (b = -0.34, SE = 0.165, p = 0.004). The variables explained 29.7% of the variance in ‘burnout’.
The associations between ‘quantitative demands’ and ‘burnout’ (b = 0.63, SE = 0.085, p < 0.001), between ‘recognition supervisor’ and ‘burnout’ (b = -7.29, SE = 2.27, p = 0.001), and the interaction term of ‘quantitative demands’ and the resource ‘recognition supervisor’ (b = -0.34, SE = 0.108, p = 0.002) were significant. Again, a model with the interaction term accounted for significantly more variance in ‘burnout’ than a model without interaction term (ΔR 2 = 0.024, p = 0.002). ‘Recognition from supervisor’ influenced the impact of ‘quantitative demands’ on burnout for -0.34 on the 0 to 100 scale. The variables explained 33.7% of the variance in ‘burnout’.
Figs 1 – 3 demonstrates simple slopes of the interaction effects of ‘workplace commitment’ predicting ‘burnout’ at high, average and low levels ( Fig 1 ) respectively with and without the resource ‘good working team’ ( Fig 2 ) and ‘recognition from supervisor’ ( Fig 3 ). Higher ‘quantitative demands’ were associated with higher levels of ‘burnout’. At low ‘quantitative demands’, the ‘burnout’ level was quite similar for all nurses. However, when ‘quantitative demands’ increased, nurses who confirmed that they had the resources stated a lower ‘burnout’ level than nurses who denied having them. This trend is repeated by the resources ‘workplace commitment’, ‘good working team’ and ‘recognition from supervisor’.
https://doi.org/10.1371/journal.pone.0245798.g001
https://doi.org/10.1371/journal.pone.0245798.g002
https://doi.org/10.1371/journal.pone.0245798.g003
The palliative care aspect ‘extent of palliative care’ showed that spending more than 20 percent of working time in care for palliative patients increased burnout significantly by a value of approximately 5 on a 0 to 100 scale ( Table 5 ).
The aim of the present study was to analyse the buffering role of resources on the relationship between workload and burnout among nurses. This was done for the first time by considering palliative care aspects, such as information on the extent of palliative care.
The study shows that higher quantitative demands were associated with higher levels of burnout, which is in line with other studies [ 37 , 39 ]. Furthermore, the results of this study indicate that working in a good team, recognition from supervisor and workplace commitment is a moderator within the workload—burnout relationship. Although the moderator analyses revealed low buffering effect values, social resources were identified once more as important resources. This is consistent with the results of a study conducted in the field of specialised palliative care in Germany, where a good working team and workplace commitment moderated the impact of quantitative demands on nurses burnout [ 52 ]. A recently published review also describes social support from co-workers and supervisors as a fundamental resource in preventing burnout in nurses [ 53 ]. Workplace commitment was not only reported as a moderator between workload and health in the nurse setting [ 37 ], but also as a moderator between work stress and burnout [ 54 ] and between work stress and other health related aspects outside the nurse setting [ 55 ]. In the present study, the effect of high workload on burnout was reduced with increasing workplace commitment. Nurses reporting a high work commitment may experience workload as less threatening and disruptive because workplace commitment gives them a feeling of belonging, security and stability. However, there are also some correlation studies which observed no direct relationship between workplace commitment and burnout for occupations in the health sector [ 56 ]. A study from Serbia assessed workplace commitment by nurses and medical technicians as a protective factor against patient-related burnout, but not against personal and work-related burnout [ 57 ]. Furthermore, a study conducted in Estonia reported no relationship between workplace commitment and burnout amongst nurses [ 58 ]. As there are indications that workplace commitment is correlated with patient safety [ 59 ], the development and improving of workplace commitment needs further scientific investigation.
This study observed slightly higher burnout rates among nurses who reported a ‘good working team’ for low workload. This fact is not decisive for the interpretation of the moderation effect of this resource because moderation is present. When workload increased, nurses who confirmed that they worked in a good working team stated a lower burnout level. However, the result of the current study showed that a good working team is particularly important when workload increases, in the most extreme cases team work in palliative care is necessary to save a person’s life. Because team work in today’s health care system is essential, health care organisations should foster team work in order to enhance their clinical outcomes [ 60 ], improve the quality of patient care as well as health [ 61 ] and satisfaction of nurses [ 62 ].
The bivariate analysis revealed that nurses who reported getting recognition from colleagues, through the social context, salary and gratitude from relatives of patients stated a lower value on the burnout scale. This is in accordance with the results of a qualitative study, which indicated that the feeling of recognition, and that one’s work is useful and worthwhile, is very important for nurses and a source of satisfaction [ 63 ]. Furthermore, self-care, self-reflection [ 64 ] and professional attitude/dissociation seem to play an important role in preventing burnout. The bivariate analysis also revealed a relationship between resilience and burnout. Nurses with high resilience reported lower values on the burnout scale, but a buffering role of resilience on burnout was not assessed. The present paper focuses solely on quantitative demands and burnout. In future studies, the different fields of nursing demands, like organisational or emotional demands, should be assessed in relation to burnout, job satisfaction and health.
Finally, we observed whether the consideration of palliative care aspects is associated with burnout. The bivariate analysis revealed a relationship between the extent of palliative care, number of patient deaths within the last month and burnout. Using regression analyses, only the extent of palliative care was associated with burnout. Since, to the best of our knowledge, the present study is the first study to consider palliative care aspects within general palliative care in Germany, these variables need further scientific investigation, not only within the demand—burnout relationship but also between the demand—health and the demand—job satisfaction relationship. Furthermore, palliative care experts from around the world considered the education and training of all members of staff in the fundamentals of palliative care to be essential [ 9 ]. One-fourth of the respondents in the present study had an additional qualification in palliative care, which was not obligatory. We assessed a relationship between quantitative demands and burnout but no relationship between an additional qualification and quantitative demands nor burnout. Nevertheless, we assessed a protective effect of the additional qualification within the pilot study in specialised palliative care, in relation both to organisational demands and demands regarding the care of relatives [ 6 ]. This suggests that the additional qualification is a resource, but one which depends on the field of demand. Further analyses would be required to review benefits achieved by additional qualifications in general palliative care.
The variable extent of palliative care is the one with the most missing values in the survey, thus future analyses should not only study larger samples but also reconsider the question on extent of palliative care.
Finally, it can be said that the main contribution of the present study is to make palliative care aspects in non-specialised palliative care settings a subject of discussion.
Limitations
The following potential limitations need to be stated: although a random sample was drawn, the sample is not representative for general palliative care in Germany due to a low participation rate of the health facilities, a low response rate of the nurses, the different responses of the health facilities and the exclusion of hospitals. One possible explanation for the low participation rate of the health facilities is the sampling procedure and data protection rules, which did not allowed the study team to contact the institutions in the sample. Due to the low participation rate, the results of the present study may be labelled as preliminary. Further, the data are based on a detailed and anonymous survey, and therefore the potential for selection bias has to be considered. It is possible that the institutions and nurses with the highest burden had no time for or interest in answering the questionnaire. It is also possible that the institutions which care for a high number of palliative patients may have taken particular interest in the survey. Additionally, some items of the questionnaire were self-developed and not validated but were considered valuable for our study as they answered certain questions that standardized questionnaires could not. The moderator analyses revealed low effect values and the variance explained by the interaction terms is rather low. However, moderator effects are difficult to detect, therefore, even those explaining as little as one percent of the total variance should be considered [ 65 ]. Consequently, the additional amount of variance explained by the interaction in the current study (2% for workplace commitment and recognition of supervisor and 1% for good working team) is not only statistically significant but also practically and theoretically relevant. When considering the results of the current study, it must be taken into account that the present paper focuses solely on quantitative demands and burnout. In future studies, the different fields of nursing demands have to be carried out on the role of resources. This not only pertains for burnout, but also for other outcomes such as job satisfaction and health. Finally, the cross-sectional design does not allow for casual inferences. Longitudinal and interventional studies are needed to support causality in the relationships examined.
Conclusions
The present study provides support to a buffering role of workplace commitment, good working teams and recognition from supervisors on the relationship between workload and burnout. Initiatives to develop or improve workplace commitment and strengthen collaboration with colleagues and supervisors should be implemented in order to reduce burnout levels. Furthermore, the results of the study provides first insights that palliative care aspects in general palliative care may have an impact on nurse burnout, and therefore they have gone unrecognised for too long in the scientific literature. They have to be considered in future studies, in order to improve the working conditions, health and satisfaction of nurses. As our study was exploratory, the results should be confirmed in future studies.
Supporting information
S1 table. number of questionnaires sent out to facilites and response rate..
https://doi.org/10.1371/journal.pone.0245798.s001
Acknowledgments
We thank the nurses and the health care institutions for taking part in the study. We thank D. Wendeler, O. Kleinmüller, E. Muth, R. Amma and C. Kohring who were helpful in the recruitment of the participants and data collection.
- 1. OECD. Health at a glance 2015: OECD indicators. 2015th ed. Paris: OECD Publishing; 2015.
- View Article
- PubMed/NCBI
- Google Scholar
- 5. Melching H. Palliativversorgung—Modul 2 -: Strukturen und regionale Unterschiede in der Hospiz- und Palliativversorgung. Gütersloh; 2015.
- 8. German National Academy of Sciences Leopoldina and Union of German Academies of Sciences. Palliative care in Germany: Perspectives for practice and research. Halle (Saale): Deutsche Akademie der Naturforscher Leopoldina e. V; 2015.
- 12. George W, Siegrist J, Allert R. Sterben im Krankenhaus: Situationsbeschreibung, Zusammenhänge, Empfehlungen. Gießen: Psychosozial-Verl.; 2013.
- 15. Deutsche Krebsgesellschaft, Deutsche Krebshilfe, Arbeitsgemeinschaft der Wissenschaftlichen Medizinischen Fachgesellschaften (AWMF). Palliativmedizin für Patienten mit einer nicht heilbaren Krebserkrankung: Langversion 1.1; 2015.
- 16. Deutsche Gesellschaft für Palliativmedizin. Definitionen zur Hospiz- und Palliativversorgung. 2016. https://www.dgpalliativmedizin.de/images/DGP_GLOSSAR.pdf . Accessed 8 Sep 2020.
- 17. Deutscher Hospiz- und PalliativVerband e.V. Hospizarbeit und Palliativversorgung. 2020. https://www.dhpv.de/themen_hospiz-palliativ.html . Accessed 20 May 2020.
- 24. van Veldhoven Marc. Quantitative Job Demands. In: Peeters M, Jonge de J, editors. An introduction to contemporary work psychology. Chichester West Sussex UK: John Wiley & Sons; 2014. p. 117–143.
- 35. Field A. Discovering statistics using IBM SPSS statistics. 4th ed. Los Angeles, London, New Delhi, Singapore, Washington DC, Melbourne: SAGE; 2016.
- 41. Rudow B. Die gesunde Arbeit: Psychische Belastungen, Arbeitsgestaltung und Arbeitsorganisation. 3rd ed. Berlin, München, Boston: De Gruyter Oldenbourg; 2014.
- 45. Freiburger Forschungsstelle für Arbeits- und Sozialmedizin. Befragung zu psychosozialen Faktoren am Arbeitsplatz. 2016. https://www.copsoq.de/assets/COPSOQ-Standard-Fragebogen-FFAW.pdf . Accessed 6 Mar 2020.
- 60. O’Daniel M, Rosenstein AH. Patient Safety and Quality: An Evidence-Based Handbook for Nurses: Professional Communication and Team Collaboration. Rockville (MD); 2008.
- 61. Canadian Health Services Research Foundation. eamwork in healthcare: promoting effective teamwork in healthcare in Canada.: Policy synthesis and recommendations.; 2006.
- Research article
- Open access
- Published: 18 May 2020
What feedback do reviewers give when reviewing qualitative manuscripts? A focused mapping review and synthesis
- Oliver Rudolf HERBER ORCID: orcid.org/0000-0003-3041-4098 1 ,
- Caroline BRADBURY-JONES 2 ,
- Susanna BÖLING 3 ,
- Sarah COMBES 4 ,
- Julian HIRT 5 ,
- Yvonne KOOP 6 ,
- Ragnhild NYHAGEN 7 ,
- Jessica D. VELDHUIZEN 8 &
- Julie TAYLOR 2 , 9
BMC Medical Research Methodology volume 20 , Article number: 122 ( 2020 ) Cite this article
14k Accesses
15 Citations
34 Altmetric
Metrics details
Peer review is at the heart of the scientific process. With the advent of digitisation, journals started to offer electronic articles or publishing online only. A new philosophy regarding the peer review process found its way into academia: the open peer review. Open peer review as practiced by BioMed Central ( BMC ) is a type of peer review where the names of authors and reviewers are disclosed and reviewer comments are published alongside the article. A number of articles have been published to assess peer reviews using quantitative research. However, no studies exist that used qualitative methods to analyse the content of reviewers’ comments.
A focused mapping review and synthesis (FMRS) was undertaken of manuscripts reporting qualitative research submitted to BMC open access journals from 1 January – 31 March 2018. Free-text reviewer comments were extracted from peer review reports using a 77-item classification system organised according to three key dimensions that represented common themes and sub-themes. A two stage analysis process was employed. First, frequency counts were undertaken that allowed revealing patterns across themes/sub-themes. Second, thematic analysis was conducted on selected themes of the narrative portion of reviewer reports.
A total of 107 manuscripts submitted to nine open-access journals were included in the FMRS. The frequency analysis revealed that among the 30 most frequently employed themes “writing criteria” (dimension II) is the top ranking theme, followed by comments in relation to the “methods” (dimension I). Besides that, some results suggest an underlying quantitative mindset of reviewers. Results are compared and contrasted in relation to established reporting guidelines for qualitative research to inform reviewers and authors of frequent feedback offered to enhance the quality of manuscripts.
Conclusions
This FMRS has highlighted some important issues that hold lessons for authors, reviewers and editors. We suggest modifying the current reporting guidelines by including a further item called “Degree of data transformation” to prompt authors and reviewers to make a judgment about the appropriateness of the degree of data transformation in relation to the chosen analysis method. Besides, we suggest that completion of a reporting checklist on submission becomes a requirement.
Peer Review reports
Peer review is at the heart of the scientific process. Reviewers independently examine a submitted manuscript and then recommend acceptance, rejection or – most frequently – revisions to be made before it gets published [ 1 ]. Editors rely on peer review to make decisions on which submissions warrant publication and to enhance quality standards. Typically, each manuscript is reviewed by two or three reviewers [ 2 ] who are chosen for their knowledge and expertise regarding the subject or methodology [ 3 ]. The history of peer review, often regarded as a “touchstone of modern evaluation of scientific quality” [ 4 ] is relatively short. For example, the British Medical Journal (now the BMJ ) was a pioneer when it established a system of external reviewers in 1893. But it was in the second half of the twentieth century that employing peers as reviewers became custom [ 5 ]. Then, in 1973 the prestigious scientific weekly Nature introduced a rigorous formal peer review system for every paper it printed [ 6 ].
Despite ever-growing concerns about its effectiveness, fairness and reliability [ 4 , 7 ], peer review as a central part of academic self-regulation is still considered the best available practice [ 8 ]. With the advent of digitisation in the late 1990s, scholarly publishing has changed dramatically with many journals starting to offer print as well as electronic articles or publishing online only [ 9 ]. The latter category includes for-profit journals such as BioMed Central ( BMC ) that have been online since their inception in 1999, with an ever evolving portfolio of currently over 300 peer-reviewed journals.
As compared to traditional print journals where individuals or libraries need to pay a fee for an annual subscription or for reading a specific article, open access journals such as BMC, PLoS ONE or BMJ Open are permanently free for everyone to read and download since the cost of publishing is paid by the author or an entity such as the university. Many, but not all, open access journals impose an article processing charge on the author, also known as the gold open access route, to cover the cost of publication. Depending on the journal and the publisher, article processing charges can range significantly between US$100 and US$5200 per article [ 10 , 11 ].
In the digital age, a new philosophy regarding the peer review process found its way into academia, questioning the anonymity of the closed system of peer-review as contrary to the demands for transparency [ 1 ]. The issue of reviewer bias, especially concerning gender and affiliation [ 12 ], led not only to the establishment of double-blind review but also to its extreme opposite: the open peer review system [ 8 ]. Although the term ‘open peer review’ has no standardised definition, scholars use the term to indicate that the identities of the authors and reviewers are disclosed and that reviewer reports are openly available [ 13 ]. In the late 1990s, the BMJ changed from a closed system of peer review to an open system [ 14 , 15 ]. During the same time, other publishers such as some journals in BMC followed the example of opening up their peer review.
While peer review reports have long been hidden from the public gaze [ 16 , 17 ], opening up the closed peer review system allows researchers to access reviewer comments, thus making it possible to study them. Since then, a number of articles have been published to assess reviews using quantitative research methods. For example, Landkroon et al. [ 18 ] assessed the quality of 247 reviews of 119 original articles using a 5-point Likert scale. Similarly, Henly and Dougherty [ 19 ] developed and applied a grading scale to assess the narrative portion of 464 reviews of 203 manuscripts using descriptive statistics. The retrospective cohort study by van Lent et al. [ 20 ] assessed peer review comments on drug trials from 246 manuscripts to investigate whether there is a relationship between the content of these comments and sponsorship using a generalised linear mixed model. Most recently, Davis et al. [ 21 ] evaluated reviewer grading forms for surgical journals with higher impact factors and compared them to surgical journals with lower impact factors using Fisher’s exact test.
Despite the readily available reviewer comments that are published alongside the final article of many open access journals, to the best of our knowledge no studies exist to date that used – besides quantitative methods – also qualitative methods to analyse the content of reviewers’ comments. Identifying (negative) reviewer comments will help authors to pay particular attention to these aspects and assist prospective qualitative researchers to understand the most common pitfalls when preparing their manuscript for submission. Thus, the aim of the study was to appraise the quality and nature of reviewers’ feedback in order to understand how reviewers engage with and influence the development of a qualitative manuscript. Our focus on qualitative research can be explained by the fact that we are passionate qualitative researchers with a history in determining the state of qualitative research in health and social science literature [ 22 ]. The following research questions were answered: (1) What are the frequencies of certain commentary types in manuscripts reporting on qualitative research? and (2) What are the nature of reviewers’ comments made on manuscripts reporting on qualitative research?
We conducted a focused mapping review and synthesis (FMRS) [ 22 , 23 , 24 , 25 ]. Most forms of review aim for breadth and exhaustive searches, but the FMRS searches within specific, pre-determined journals. While Platt [ 26 ] observed that ‘a number of studies have used samples of journal articles’, the distinctive feature of the FMRS is the purposive selection of journals. These are chosen for their likelihood to contain articles relevant to the field of inquiry – in this case qualitative research published in open access journals that operate an open peer-review process that involves posting the reviewer’s reports. It is these reports that we have analysed using thematic analysis techniques [ 27 ].
Currently there are over 70 BMC journals that have adopted open peer-review. The FMRS focused on reviewers’ reports published during the first quarter of 2018. Journals were selected using a three-stage process. First, we produced a list with all BMC journals that operate an open peer review process and will publish qualitative research articles ( n = 62). Second, from this list we selected journals that are general fields of practice and non-disease specific ( n = 15). Third, to ensure a sufficient number of qualitative articles, we excluded journals with less than 25 hits on the search term “qualitative” for the year 2018 (search date: 16 July 2018) because chances were considered too slim to contain sufficient articles of interest. At the end of the selection process, the following nine BMC journals were included in our synthesis: (1) BMC Complementary and Alternative Medicine , (2) BMC Family Practice , (3) BMC Health Services Research , (4) BMC Medical Education , (5) BMC Medical Ethics , (6) BMC Nursing , (7) BMC Public Health , (8) Health Research Policy and Systems , and (9) Implementation Science . Since these journals represent different subjects, a variety of qualitative papers written for different audiences was captured. Every article published within the timeframe was scrutinised against the inclusion and exclusion criteria (Table 1 ).
Development of the data extraction sheet
A validated instrument for the classification of reviewer comments does not exist [ 20 ]. Hence, a detailed classification system was developed and pilot tested considering previous research [ 20 ]. Our newly developed data extraction sheet consists of a 77-item classification system organised according to three dimensions: (1) scientific/technical content, (2) writing criteria/representation, and (3) technical criteria. It represents themes and sub-themes identified by reading reviewer comments from twelve articles published in open peer-review journals. For the development of the data extraction sheet, we randomly selected four articles containing qualitative research from each of the following three journals published between 2017 and 2018: BMC Nursing , BMC Family Practice and BMJ Open . We then analysed the reviews of manuscripts by systematically coding and categorising the reviewers’ free-text comments. Following the recommendation by Shashok [ 28 ], we initially organised the reviewer’s comments along two main dimensions, i.e., scientific content and writing criteria. Shashok [ 28 ] argues that when peer reviewers confuse content and writing, their feedback can be misunderstood by authors who may modify texts in unintentional ways to the detriment of the manuscript.
To check the comprehensiveness of our classification system, provisional themes and sub-themes were piloted using reviewer comments we had previously received from twelve of our own manuscripts that had been submitted to journals that operate blind peer-review. We wanted to account for potential differences in reviewers’ feedback (open vs. blind review). As a result of this quality enhancement procedure, three sub-themes and a further dimension (‘technical criteria’) were added. For reasons of clarity and comprehensibility, the dimension ‘scientific content’ was subdivided following the IMRaD structure. IMRaD is the most common organisational structure of an original research article comprising I ntroduction, M ethods, R esults a nd D iscussion [ 29 ]. Anchoring examples were provided for each theme/sub-theme. To account for reviewer comments unrelated to the IMRaD structure, a sub-category called ‘generic codes’ was created to collect more general comments. When reviewer comments could not be assigned to any of the existing themes/sub-themes, they were noted as “Miscellaneous”. Table 2 shows the final data extraction sheet including anchoring examples.
Data extraction procedure
Data extraction was accomplished by six doctoral students (coders). On average, each coder was allocated 18 articles. After reading the reviews, coders independently classified each comment using the classification system. In line with Day et al. [ 30 ] a reviewer comment was defined as “ a distinct statement or idea found in a review, regardless of whether that statement was presented in isolation or was included in a paragraph that contained several statements. ” Editor comments were not included. Reviewers’ comments were copied and pasted into the most appropriate item of the classification system following a set of pre-defined guidelines. For example, a reviewer comment could only be coded once by assigning it to the most appropriate theme/sub-theme. A separate data extraction sheet was used for each article. For the purpose of calibration, the first completed data extraction sheet from each coder together with the reviewer’s comments was sent to the study coordinator (ORH) who provided feedback on classifying the reviewer comments. The aim of the calibration was to ensure that all coders were working within the same parameters of understanding, to discuss the subtleties of the judgement process and create consensus regarding classifications. Although the assignment to specific themes/sub-themes is, by nature, a subjective process, difficult to assign comments were classified following discussion and agreement between coder and study coordinator to ensure reliability. Once all data extraction was completed, two experienced qualitative researchers (CB-J, JT) independently undertook a further calibration exercise of a random sub-sample of 20% of articles ( n = 22) to ensure consistency across coders. Articles were selected using a random number generator. For these 22 articles, classification discrepancies were resolved by consensus between coders and experienced researchers. Finally, all individual data extraction sheets were collated to create a comprehensive Excel spreadsheet with over 8000 cells that allowed tallying the reviewer’s comments across manuscripts for the purpose of data analysis. For each manuscript, a reviewer could have several remarks related to one type of comment. However, each type of comment was scored only once per category.
Finally, reviewer comments were ‘quantitized’ [ 31 ] by applying programming language (Python) to Jupyter Notebook, an open-source web application, to perform frequency counts of free-text comments regarding the 77 items. Among other data manipulation, we sorted elements of arrays in descending order of frequency using Pandas, counted the number of studies in which a certain theme/sub-theme occurred, conducted distinct word searches using NLTK 3 or grouped data according to certain criteria. The calculation of frequencies is a way to unite the empirical precision of quantitative research with the descriptive precision of qualitative research [ 32 ]. This quantitative transformation of qualitative data allowed extracting more meaning from our spreadsheet through revealing patterns across themes/sub-themes, thus giving indicators about which of them to analyse using thematic analysis.
A total of 109 manuscripts submitted to nine open-access journals were included in the FMRS. When scrutinising the peer review reports, we noticed that on one occasion the reviewer’s comments were missing [ 33 ]. For the remaining 108 manuscripts, reviewer comments were accessible via the journal’s pre-publication history. On close inspection, however, it became apparent that one article did not contain qualitative research, thus leaving ultimately 107 articles to work with ( supplementary file ). Considering that each manuscript could potentially be reviewed by multiple reviewers and underwent at least one round of revision, the total number of reviewer reports analysed amounted to 347 containing collectively 1703 reviewer comments. The level of inter-rater agreement for the 22 articles included in the calibration exercise was 97%. Disagreement was, for example, in relation to coding a comment as “miscellaneous” or as “confirmation/approval (from reviewer)”. For 18 out of 22 articles, there was 100% agreement for all types of comments.
Variation in number of reviewers
The number of reviewers invited by the editor to review a submitted manuscript varied greatly within and among journals. While the majority of manuscripts across journals had been reviewed by two to three reviewers, there were also significant variations. For example, the manuscript submitted to BMC Medical Education by Burgess et al. [ 34 ] had been reviewed by five reviewers whereas the manuscript submitted to BMC Public Health by Lee and Lee [ 35 ] had been reviewed by one reviewer only. Even within journals there was a huge variation. Among our sample, BMC Public Health had the greatest variance ranging from one to four reviewers. Besides, it was noted that additional reviewers were called in not until the second or even third revision of the manuscript. A summary of key information on journals included in the FMRS is provided in Table 3 .
“Quantitizing” reviewer comments
The frequency analysis revealed that the number of articles in which a certain theme/sub-theme occurred ranged from 1 to 79. Across all 107 articles, the types of comments most frequently reported were in relation to generic themes. Reviewer comments regarding “Adding information/detail/nuances”, “Clarification needed”, “Further explanation required” and “Confirmation/approval (from reviewer)” were used in 79, 79, 66 and 63 articles, respectively. The four most frequently used themes/sub-themes are composed of generic codes from dimension I (“Scientific/technical content”). Leaving all generic codes aside, it became apparent that among the 30 most frequently employed themes “Writing criteria” (dimension II) is the top ranking theme, followed by comments in relation to the “Methods” (dimension I) (Table 4 ).
Subsequently, we present key qualitative findings regarding “Confirmation/approval from reviewers” (generic), “Sampling” and “Analysis process” (methods), “Robust/rich data analysis and “Themes/sub-themes” (results) as well as findings that suggest an underlying quantitative mindset of the reviewers.
Confirmation/approval from reviewers (generic)
The theme “confirmation/approval from reviewers” ranks third among the top 30 categories. A total of 63 manuscripts contained at least one reviewer comment related to this theme. Overall, reviewers maintained a respectful and affirmative rhetoric when providing feedback. The vast majority of reviewers began their report by stating that the manuscript was well written. The following is a typical example:
“Overall, the paper is well written, and theoretically informed.” Article #14.
Reviewers then continued to add explicit praise for aspects or sections that were particularly innovative and/or well constructed before they started to put forward any negative feedback.
Sampling (methods)
Across all 107 articles there were 34 reviewer comments in relation to the sampling technique(s). Two major categories were identified: (1) composition of the sample and (2) identification and justification of selected participants. Regarding the former, reviewers raised several concerns about how the sample was composed. For instance, one reviewer wanted to know the reason for female predominance in the study and why an entire focus group was composed of females only. Another reviewer expressed strong criticism on the composition of the sample since only young, educated and non-minority white British participants were included in the study. The reviewer commented:
“ So a typical patient was young, educated and non-minority White British? The research studies these days should be inclusive of diverse types of patients and excluding patients because of their age and ethnicity is extremely concerning to me. This assumption that these individuals will “find it more difficult to complete questionnaires” is concerning ” Article #40.
This raised concerns of potentially excluding important diverse perspectives – such as extreme or deviant cases – from other participants. Similarly, some reviewers expressed concerns that relevant groups of people were not interviewed, calling into question that the findings were theoretically saturated. In terms of the identification of participants, reviewers raised questions regarding how the authors obtained the necessary characteristics to achieve purposive sampling or why only certain groups of people were included for interviews. Besides that, reviewers criticised that some authors did not mention their inclusion/exclusion criteria for selecting participants or did not specify their sampling method. For example:
“The authors state that they recruited a purposive sample of patients for the interviews. Concerning which variables was this sampling purposive? Are there any studies informing the patient selection process?” Article #61.
Hence, reviewers requested more detailed information on how participants were selected and to clearly state the type of sampling. Apart from the two key categories, reviewers made additional comments in relation to data saturation, transferability of findings, limitations of certain sampling methods and criticised the lack of description of participants who were approached but refused to participate in the study.
Details of analysis process (methods)
In 60 out of 107 articles, reviewers made comments in relation to the data analysis. The vast majority of comments stressed that authors provided scarce information about the analysis process. Hence, reviewers requested a more detailed description of the specific analysis techniques employed so that readers can obtain a better understanding of how the analysis was done to judge the trustworthiness of the findings. To this end, reviewers frequently requested an explicit statement on whether the analysis was inductive or deductive or iterative or sequential. One reviewer wrote the following comment:
“Please elaborate more on the qualitative analysis. The authors indicate that they used ‘iterative’ approaches. While this is certainly laudable, it is important to know how they moved from codes to themes (e.g. inductively? deductively?)” Article #5.
Since there are many approaches to analysing qualitative data, reviewers demanded sufficient detail in relation to the underlying theoretical framework used to develop the coding scheme, the analytic process, the researchers’ background (e.g. profession), the number of coders, data handling, length of interviews and whether data saturation occurred. Over a dozen reviewer comments were specifically in relation to the identification of themes/sub-themes. Reviewers requested a more detailed description on how the themes/sub-themes were derived from codes and whether they were developed by a second researcher working independently from each other.
“I would have liked to read how their themes were generated, what they were and how they assured robust practices in qualitative data analysis”. Article #43.
Besides that, some reviewers were in the opinion that the approach to analysis has led to a surface-level penetration of the data which was reflected in the Results section where themes were underexplored (for more detail see “ Robust/rich data analysis” below). Finally, reviewer comments that occurred infrequently included questions concerning the inter-rater reliability, competing interpretations of data, the use of computer software or the original interview language.
Robust/rich data analysis (results)
Among the 30 reviewer comments related to this theme/sub-theme, three key facets were observed: (1) greater analytical depth required, (2) suggestions for further analysis, and (3) themes are underexplored. In relation to the first point, reviewers requested more in-depth data analysis to strengthen the quality of the manuscript. Reviewers were in the opinion that authors reproduced interview data (raw data) in a reduced form with minimal or no interpretation, thus leaving the interpretation to the reader. Other reviewers referred to manuscripts as preliminary drafts that need to be further analysed to achieve greater analytical depth of themes, make links between themes or identify variations between respondents. In relation to the second point, several reviewers offered suggestions for further analysis. They provided detailed information on how to further explore the data and what additional results they would like to see in the revised version (e.g. group comparison, gender analysis). The latter aspect goes hand in hand with the third point. Several reviewers pointed out that the findings were shallow, simplistic or superficial at best; lacking the detailed descriptions of complex accounts from participants. For example:
“The results of the study are mostly descriptive and there is limited analysis. There is also absence of thick description, which one would expect in a qualitative study”. Article #34.
Even after the first revision, some manuscripts still lacked detailed analysis as the following comment from the same reviewer illustrates:
“I believe that the results in the revised version are still mostly descriptive and that there is limited analysis”. Article #34, R1.
Other, less frequently mentioned reviewer comments included lack of deviant cases or absence of relationships between themes.
Themes/sub-themes (results)
In total, there were 24 reviewer comments in relation to themes/sub-themes. More than half of the comments fell into one of the three categories: (1) themes/sub-themes are not sufficiently supported by data, (2) example/excerpt does not fit the stated theme, and (3) use of insufficient quotes to support theme/sub-theme. In relation to the first category, reviewers largely criticised that the data provided were insufficient to warrant being called a theme. Reviewers requested to provide data “from more than just one participant” to substantiate a certain theme or criticised that only a short excerpt was provided to support a theme. The second category dealt with reviewer comments that questioned whether the excerpts provided actually reflected the essence of a theme/sub-theme presented in the results section. The following reviewer comment exemplifies the issue:
“The data themes seem valid, but the data and narratives used to illustrate that don’t seem to fit entirely under each sub-heading”. Article #99.
Some reviewers provided alternative suggestions on how to call a theme/sub-theme or advised the authors to rethink if excerpts might be better placed under a different theme. The third category concerns themes/sub-themes that are not sufficiently supported by participants’ quotes. Reviewers perceived direct quotes as evidence to support a certain theme or as a means to add strength to the theme as the following example illustrates:
“Please provide at least one quote from each school leader and one quote from children to support this theme, if possible. It would seem that most, if not all, themes should reflect data from each participant group”. Article #88.
Hence, the absence of quotes prompted reviewers to request at least one quote to justify the existence of that theme. The inclusion of a rich set of quotes was perceived as strength of a manuscript. Finally, less frequently raised reviewer comments related to the discrimination of similar themes, the presentation of quotes in tables (rather than under the appropriate theme headings), the lack of defining a theme and reducing the number of themes.
Quantitative mindset
Some reviewers who were appointed by journal editors to review a manuscript containing qualitative research evaluated the quality of the manuscript from a perspective of a quantitative research paradigm. Some reviewers not only used terminology that is attuned to quantitative research, but also their judgements were based on a quantitative mindset. In particular, there were a number of reviewer comments published in BMC Health Services Research , BMC Medical Education and BMC Family Practice that demonstrated an apparent lack of understanding of the principles underlying qualitative inquiry of the person providing the review. First, several reviewers seemed to have confused the concept of generalisability with the concept of representativeness inherently associated with the positivist tradition. For instance, reviewers erroneously raised concerns about whether interviewees were “representative” of the “final target population” and requested the provision of detailed demographic characteristics.
“Need to better describe how the patients are representative of patients with chronic heart failure in the Netherlands generally. The declaration that “a representative group of patients were recruited” would benefit from stating what they were representative of.” Article # 66.
Similarly, another reviewer wanted to know from the authors how they ensured that the qualitative analysis was done objectively.
“The reader would benefit from a detailed description of […] how did the investigators ensure that they were objective in their analysis – objectivity and trustworthiness?” Article #22.
Furthermore, despite the fact that the paradigm wars have largely come to an end, hostility has not ceased on all fronts. In some reviewers the dominance and superiority of the quantitative paradigm over the qualitative paradigm is still present as the following comment illustrates:
“The main question and methods of this article is largely qualitative and does not seem to have significant implications for clinical practice, thus it may not be suitable to publish in this journal.” Article #45.
Finally, one reviewer apologised at the outset of the reviewer’s report for being unable to judge the data analysis due to the absence of sufficient knowledge in qualitative research.
Overall, in this FMRS we found that reviewers maintained a respectful and affirmative rhetoric when providing feedback. Yet, the positive feedback did not overshadow any key negative points that needed to be addressed in order to increase the quality of the manuscript. However, it should not be taken for granted that all reviewers are as courteous and generous as the ones included in our particular review, because as Taylor and Bradbury-Jones [ 36 ] observed there are many examples where reviewers can be unhelpful and destructive in their comments.
A key finding of this FMRS is that reviewers are more inclined to comment on the writing rather than the methodological rigour of a manuscript. This is a matter of concern, because Altman [ 37 ] – the originator of the EQUATOR (Enhancing the Quality and Transparency of Health Research) Network – has pointed out: “Unless methodology is described the conclusions must be suspect”. If we are to advance the quality of qualitative research then we need to encourage clarity and depth in reporting the rigour of research.
When reviewers did comment on the methodological aspects of an article, issues frequently commented on by reviewers were in relation to sampling, data analysis, robust/rich data analysis as reflected in the findings and themes/sub-themes that are insufficiently supported. Considerable work has been undertaken over the past decade trying to improve the reporting standards of qualitative research through the dissemination of qualitatively oriented reporting guidelines such as the ‘Standards for Reporting Qualitative Research’ (SRQR) [ 38 ] or the ‘Consolidated Criteria for Reporting Qualitative Research’ (COREQ) [ 39 ] with the aim of improving transparency of qualitative research. Although these guidelines appear to be comprehensive, some important issues identified in our study are not mentioned or only dealt with somewhat superficially: sampling for example. Neither COREQ nor SRQR shed light on the appropriateness of the sample composition, i.e., to critically question whether all relevant groups of people have been identified as potential participants or whether extreme or deviant cases were sought.
Similarly, lack of in-depth data analysis has been identified as another weakness where uninterpreted (raw) data were presented as if they were findings. However, existing reporting guidelines are not sharp enough to distinguish between findings and data. While findings are researchers’ interpretations of the data they collected, data consist of empirical, uninterpreted material researchers offer as their findings [ 32 ]. Hence, we suggest modifying the current reporting guidelines by including a further item to the checklist called “Degree of data transformation”. The suggested checklist item might prompt both authors and reviewers to make a judgment about the degree to which data have been transformed, i.e., interpretively removed from data as given. The rationale for the new item is to raise authors’ and reviewers’ awareness for the appropriateness of the degree of data transformation in relation to the chosen analysis method. For example, findings derived from content analysis remain close to the data as they were given to the research; they are often organised into surface classification systems and summarised in brief text. Findings derived from grounded theory, however, should offer a coherent model or line of argument which addresses causality or the fundamental nature of events or experiences [ 32 ].
Besides that, some reviewers put forward comments that we refer to as aligning with a ‘quantitative mindset’. Such reviewers did not appear to understand that rather than aspiring to statistical representativeness, in qualitative research participants are selected purposefully for the contribution they can make towards the phenomenon under study [ 40 ]. Hence, the generalisability of qualitative findings beyond an immediate group of participants is judged by similarities between the time, place, people or other social contexts [ 41 ] rather than in relation to the comparability of the demographic variables. It is the fit of the topic or the comparability of the problem that is of concern [ 40 ].
The majority of issues that reviewers picked up on are already mentioned in reporting guidelines, so there is no reason why these were omitted by researchers. Many journals now insist on alignment with COREQ criteria, so there is an important question to be asked as to why this is not always happening. We suggest that completion of an established reporting checklist (e.g. COREQ, SRQR) on submission becomes a requirement.
In this FMRS we have made judgements about fellow peer reviewers and found their feedback to be constructive, but also, among some, we found some lack of grasp of the essence of the qualitative endeavor. Some reviewers did not seem to understand that objectivity and representative sampling are the antithesis of subjectivity, reflexivity and data saturation. We acknowledge though, that individual reviewers might have varying levels of experience and competence both in terms of qualitative research, but also in the reviewing process. We found one reviewer who apologised at the outset of the reviewer’s report for being unable to judge the data analysis due to their absence of sufficient knowledge in qualitative research. In line with Spigt and Arts [ 42 ], we appreciate the honesty of that reviewer for being transparent about their skillset. The lessons here we feel are for more experienced reviewers to offer support and reviewing mentorship to those who are less experienced and for reviewers to emulate the honesty of the reviewer as discussed here, by being open about their capabilities within the review process.
Based on our findings, we have a number of recommendations for both researchers and reviewers. For researchers reporting qualitative studies, we suggest that particular attention is paid to reporting of sampling techniques, both in the characteristics and composition of the sample, and how participants were selected. This is an issue that the reviewers in our FMRS picked up on, so forewarned is forearmed. But it is also crucially important that sampling matters are not glossed over, so this constitutes good practice in research reporting as well. Second, it seems that qualitative researchers do not give sufficient detail about analytic techniques and underlying theoretical frameworks. The latter has been pointed out before [ 25 ], but both these aspects were often the subject of reviewer comments.
Our recommendation for reviewers is simply to be honest. If qualitative research is not an area of expertise, then it is better to decline to undertake the review, than to apply a quantitative lens in the assessment of a qualitative piece of work. It is inappropriate to ask for details about validity and generalisability and shows a lack of respect to qualitative researchers. We are well beyond the arguments about quantitative versus qualitative [ 43 ]. It is totally appropriate to comment on background and findings and any obvious deficiencies. Finally, our recommendation to editors is a difficult one, because as editors ourselves we know how challenging it can be to find willing reviewers. When selecting reviewers however, it is as important to bear in mind the methodological aspects of an article and its subject, and to select reviewers with appropriate methodological expertise. Some journals make it a requirement for quantitative articles to be reviewed by a statistical expert and we think this is good practice. When it comes to qualitative articles however, the methodological expertise of reviewers may not be so stringently noted and applied. Editors could make a difference here and help to push up the quality of qualitative reviews.
Strengths and weaknesses
Since we had only access to reviewer’s comments of articles that were finally published in open access journals, we are unable to compare them to types of comments related to rejected submissions. Thus, this study was limited to manuscripts that were sent out for external peer review and were finally published. Furthermore, the chosen study design of analysing only reviewer comments of published articles with an open system of peer review did not allow direct comparison with reviewer comments derived from blind-review.
FMRS provides a snap-shot of a particular issue at one particular time [ 23 ]. To that end, findings might be different in another review undertaken in a different time period. However, as a contemporary profile of reviewing within qualitative research, the current findings provide useful insights for authors of qualitative reports and reviewers alike. Further research should focus on comparing reviewer comments taken from an open and closed system of peer review in order to identify similarities and differences between the two models of peer review.
A limitation is that we reviewed open access journals because this was the only way of accessing a range of comments. The alternative that we did consider was to use the feedback provided by reviewers on our own manuscripts. However, this would have lacked the transparency and traceability associated with this current FMRS, which we consider to be a strength. That said, there may be an inherent problem in having reviewed open access peer review comments, where both the author and reviewer are known. Reviewers are unable to ‘hide behind’ the anonymity of blind peer review and this might reflect, at least in part, why their comments as analysed for this review were overwhelmingly courteous and constructive. This is at odds with the comments that one of us has received as part of a blind peer review: ‘silly, silly, silly’ [ 36 ].
This FMRS has highlighted some important issues in the field of qualitative reviewing that hold lessons for authors, reviewers and editors. Authors of qualitative reports are called upon to follow guidelines on reporting and any amendments that these might contain as recommended by the findings of our review. Humility and transparency are required among reviewers when it comes to accepting to undertake a review and an honest appraisal of their capabilities in understanding the qualitative endeavor. Journal editors can assist this by thoughtful and judicious selection of reviewers. Ultimately, all those involved with the publication process can drive up the quality of individual qualitative articles and the synergy is such that this can make a significant impact on quality across the field.
Availability of data and materials
The datasets used and/or analysed during the current study are available from the corresponding author on reasonable request.
Abbreviations
BioMed central
British medical journal
Consolidated criteria for reporting qualitative research
Enhancing the quality and transparency of health research
Focused mapping review and synthesis
Introduction, methods, results and discussion
Natural language toolKit
Standards for reporting qualitative research
Gannon F. The essential role of peer review (editorial). EMBO Rep. 2001;21(91):743.
Article Google Scholar
Mungra P, Webber P. Peer review process in medical research publications: language and content comments. Engl Specif Purp. 2010;29:43–53.
Turcotte C, Drolet P, Girard M. Study design, originality, and overall consistency influence acceptance or rejection of manuscripts submitted to the journal. Can J Anaesth. 2004;51:549–56.
Van der Wall EE. Peer review under review: room for improvement? Neth Heart J. 2009;17:187.
Burnham JC. The evolution of editorial peer review. JAMA. 1990;263:1323–9.
Article CAS Google Scholar
Baldwin M. Credibility, peer review, and Nature , 1945-1990. Notes Rec R Soc Lond. 2015;69:337–52.
Lee CJ, Sugimoto CR, Zhang G, Cronin B. Bias in peer review. J Assoc Inf Sci Technol. 2013;64:2–17.
Horbach SPJM, Halffman W. The changing forms and expectations of peer review. Res Integr Peer Rev. 2018;3:8.
Oermann MH, Nicoll LH, Chinn PL, Ashton KS, Conklin JL, Edie AH, et al. Quality of articles published in predatory nursing journals. Nurs Outlook. 2018;66:4–10.
University of Cambridge. How much do publishers charge for Open Access? (2019) https://www.openaccess.cam.ac.uk/paying-open-access/how-much-do-publishers-charge-open-access Accessed 26 Jun 2019.
Elsevier. Open access journals. (2018) https://www.elsevier.com/about/open-science/open-access/open-access-journals Accessed 28 Oct 2018.
Peters DP, Ceci SJ. Peer-review practices of psychological journals: the fate of published articles, submitted again. Behav Brain Sci. 1982;5:187–95.
Ross-Hellauer T. What is open peer review? A systematic review. F1000 Res. 2017;6:588.
Smith R. Opening up BMJ peer review. A beginning that should lead to complete transparency. BMJ. 1999;318:4–5.
Brown HM. Peer review should not be anonymous. BMJ. 2003;326:824.
Gosden H. “Thank you for your critical comments and helpful suggestions”: compliance and conflict in authors’ replies to referees’ comments in peer reviews of scientific research papers. Iberica. 2001;3:3–17.
Google Scholar
Swales J. Occluded genres in the academy. In: Mauranen A, Ventola E, editors. Academic writing: intercultural and textual issues. Amsterdam: John Benjamins Publishing Company; 1996. p. 45–58.
Chapter Google Scholar
Landkroon AP, Euser AM, Veeken H, Hart W, Overbeke AJ. Quality assessment of reviewers' reports using a simple instrument. Obstet Gynecol. 2006;108:979–85.
Henly SJ, Dougherty MC. Quality of manuscript reviews in nursing research. Nurs Outlook. 2009;57:18–26.
Van Lent M, IntHout J, Out HJ. Peer review comments on drug trials submitted to medical journals differ depending on sponsorship, results and acceptance: a retrospective cohort study. BMJ Open. 2015. https://doi.org/10.1136/bmjopen-2015-007961 .
Davis CH, Bass BL, Behrns KE, Lillemoe KD, Garden OJ, Roh MS, et al. Reviewing the review: a qualitative assessment of the peer review process in surgical journals. Res Integr Peer Rev. 2018;3:4.
Bradbury-Jones C, Breckenridge J, Clark MT, Herber OR, Wagstaff C, Taylor J. The state of qualitative research in health and social science literature: a focused mapping review and synthesis. Int J Soc Res Methodol. 2017;20:627–45.
Bradbury-Jones C, Breckenridge J, Clark MT, Herber OR, Jones C, Taylor J. Advancing the science of literature reviewing in social research: the focused mapping review and synthesis. Int J Soc Res Methodol. 2019. https://doi.org/10.1080/13645579.2019.1576328 .
Taylor J, Bradbury-Jones C, Breckenridge J, Jones C, Herber OR. Risk of vicarious trauma in nursing research: a focused mapping review and synthesis. J Clin Nurs. 2016;25:2768–77.
Bradbury-Jones C, Taylor J, Herber OR. How theory is used and articulated in qualitative research: development of a new typology. Soc Sci Med. 2014;120:135–41.
Platt J. Using journal articles to measure the level of quantification in national sociologies. Int JSoc Res Methodol. 2016;19:31–49.
Braun V, Clarke V. Using thematic analysis in psychology. Qual Res Psychol. 2006;3:77–101.
Shashok K. Content and communication: how can peer review provide helpful feedback about the writing? BMC Med Res Methodol. 2008;8:3.
Hall GM. How to write a paper. 2nd ed. London: BMJ Publishing Group; 1998.
Day FC, Dl S, Todd C, Wears RL. The use of dedicated methodology and statistical reviewers for peer review: a content analysis of comments to authors made by methodology and regular reviewers. Ann Emerg Med. 2002;40:329–33.
Tashakkori A, Teddlie C. Mixed methodology: combining qualitative and quantitative approaches. London: Sage Publications; 1998.
Sandelowski M, Barroso J. Handbook for synthesizing qualitative research. New York: Springer Publishing Company; 2007.
Jonas K, Crutzen R, Krumeich A, Roman N, van den Borne B, Reddy P. Healthcare workers’ beliefs, motivations and behaviours affecting adequate provision of sexual and reproductive healthcare services to adolescents in Cape Town, South Africa: a qualitative study. BMC Health Serv Res. 2018;18:109.
Burgess A, Roberts C, Sureshkumar P, Mossman K. Multiple mini interview (MMI) for general practice training selection in Australia: interviewers’ motivation. BMC Med Educ. 2018;18:21.
Lee S-Y, Lee EE. Cancer screening in Koreans: a focus group approach. BMC Public Health. 2018;18:254.
Taylor J, Bradbury-Jones C. Writing a helpful journal review: application of the 6 C’s. J Clin Nurs. 2014;23:2695–7.
Altman D. My journey to EQUATOR: There are no degrees of randomness. EQUATOR Network. 2016 https://www.equator-network.org/2016/02/16/anniversary-blog-series-1/ Accessed 17 Jun 2019.
O’Brien BC, Harris IB, Beckman TJ, Reed DA, Cook DA. Standards for reporting qualitative research: a synthesis of recommendations. Acad Med. 2014;89:1245–51.
Tong A, Sainsbury P, Craig J. Consolidated criteria for reporting qualitative research (COREQ): a 32-item checklist for interviews and focus groups. Int J Qual Health Care. 2007;19:349–57.
Morse JM. Editorial: Qualitative generalizability. Qual Health Res. 1999;9:5–6.
Leung L. Validity, reliability, and generalizability in qualitative research. J Family Med Prim Care. 2015;4:324–7.
Spigt M, Arts ICW. How to review a manuscript. J Clin Epidemiol. 2010;63:1385–90.
Griffiths P, Norman I. Qualitative or quantitative? Developing and evaluating complex interventions: time to end the paradigm war. Int J Nurs Stud. 2013;50:583–4.
Download references
Acknowledgments
The support of Daniel Rütter in compiling data and providing technical support is gratefully acknowledged. Furthermore, we would like to thank Holger Hönings for applying general-purpose programming language to allow for a quantification of reviewer comments in the MS Excel spreadsheet.
Author information
Authors and affiliations.
Institute of General Practice, Centre for Health and Society, Medical Faculty of the Heinrich Heine University Düsseldorf, Moorenstr. 5, 40225, Düsseldorf, Germany
Oliver Rudolf HERBER
School of Nursing, College of Medical and Dental Sciences, University of Birmingham, Birmingham, UK
Caroline BRADBURY-JONES & Julie TAYLOR
Institute of Health and Care Sciences, Sahlgrenska Academy at the University of Gothenburg, Gothenburg, Sweden
Susanna BÖLING
Florence Nightingale Faculty of Nursing, Midwifery & Palliative Care, King’s College London, London, UK
Sarah COMBES
Institute of Applied Nursing Sciences, Department of Health, University of Applied Sciences FHS St.Gallen, St. Gallen, Switzerland
Julian HIRT
Cardiology department, Radboud University Medical Centre, Nijmegen, the Netherlands
Yvonne KOOP
Division of Emergencies and Critical Care, Oslo University Hospital/Institute of Health and Society, Faculty of Medicine, University of Oslo, Oslo, Norway
Ragnhild NYHAGEN
Hogeschool Utrecht, Utrecht, the Netherlands
Jessica D. VELDHUIZEN
Birmingham Women’s and Children’s Hospitals NHS Foundation Trust, Birmingham, UK
Julie TAYLOR
You can also search for this author in PubMed Google Scholar
Contributions
All authors have made an intellectual contribution to this research paper. ORH conducted the qualitative analysis and wrote the first draft of the paper. SB, SC, JH, YK, RN and JDV extracted and classified each comment using the classification system. CB-J and JT independently undertook a calibration exercise of a random sub-sample of articles ( n = 22) to ensure consistency across coders. All co-authors (CB-J, SB, SC, JH, YK, RN, JDV and JT) have input into drafts and have read and approved the final version of the manuscript.
Corresponding author
Correspondence to Oliver Rudolf HERBER .
Ethics declarations
Ethics approval and consent to participate.
Not applicable.
Consent for publication
Competing interests.
The authors declare that they have no competing interests.
Additional information
Publisher’s note.
Springer Nature remains neutral with regard to jurisdictional claims in published maps and institutional affiliations.
Supplementary information
Additional file 1.
References of all manuscripts included in the analysis ( n = 107).
Rights and permissions
Open Access This article is licensed under a Creative Commons Attribution 4.0 International License, which permits use, sharing, adaptation, distribution and reproduction in any medium or format, as long as you give appropriate credit to the original author(s) and the source, provide a link to the Creative Commons licence, and indicate if changes were made. The images or other third party material in this article are included in the article's Creative Commons licence, unless indicated otherwise in a credit line to the material. If material is not included in the article's Creative Commons licence and your intended use is not permitted by statutory regulation or exceeds the permitted use, you will need to obtain permission directly from the copyright holder. To view a copy of this licence, visit http://creativecommons.org/licenses/by/4.0/ . The Creative Commons Public Domain Dedication waiver ( http://creativecommons.org/publicdomain/zero/1.0/ ) applies to the data made available in this article, unless otherwise stated in a credit line to the data.
Reprints and permissions
About this article
Cite this article.
HERBER, O.R., BRADBURY-JONES, C., BÖLING, S. et al. What feedback do reviewers give when reviewing qualitative manuscripts? A focused mapping review and synthesis. BMC Med Res Methodol 20 , 122 (2020). https://doi.org/10.1186/s12874-020-01005-y
Download citation
Received : 27 August 2019
Accepted : 04 May 2020
Published : 18 May 2020
DOI : https://doi.org/10.1186/s12874-020-01005-y
Share this article
Anyone you share the following link with will be able to read this content:
Sorry, a shareable link is not currently available for this article.
Provided by the Springer Nature SharedIt content-sharing initiative
- Open access publishing
- Peer review
- Manuscript review
- reviewer’s report
- Qualitative analysis
- Qualitative research
BMC Medical Research Methodology
ISSN: 1471-2288
- General enquiries: [email protected]
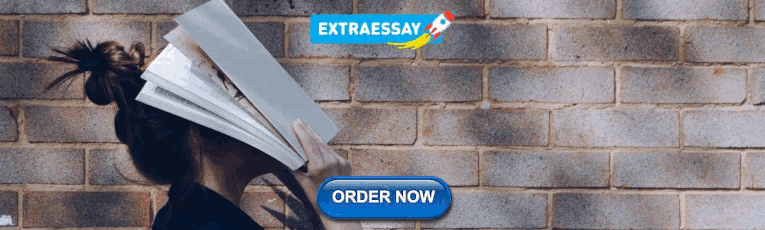
IMAGES
VIDEO
COMMENTS
Second, quantitative research may exploit the bottom-up generalization strategy that is inherent to many qualitative approaches. This offers a new perspective on unsuccessful replications by treating them not as scientific failures, but as a valuable source of information about the scope of a theory. ... Journal Dev. Educ. Glob. Learn. 7, 6 ...
Unlike research questions in quantitative research, research questions in qualitative research are usually continuously reviewed and reformulated. The central question and associated subquestions are stated more than the hypotheses. 15 The central question broadly explores a complex set of factors surrounding the central phenomenon, aiming to ...
Background Identifying determinants of health and understanding their role in health production constitutes an important research theme. We aimed to document the state of recent multi-country research on this theme in the literature. Methods We followed the PRISMA-ScR guidelines to systematically identify, triage and review literature (January 2013—July 2019). We searched for studies that ...
The quantitative research method consists of: 1) survey, 2) correlational, 3) experimental, 4) causalcomparative or ex post facto (Apuke, 2017). The quantitative correlational method is used to ...
Qualitative and quantitative research is collected and analysed at the same time in a parallel or complementary manner. Integration can occur at three points: ... Provenance and peer review: Not commissioned; externally peer reviewed. ... field-specific studies on the state of the art of mixed-methods research. Journal of Mixed-methods Research ...
There were several inclusion criteria: (1) empirical studies of the implementation of public policies already passed or approved that addressed physical or behavioral health, (2) quantitative self-report or archival measurement methods utilized, (3) published in peer-reviewed journals from January 1995 through April 2019, (4) published in the ...
The peer review process includes a detailed review by experts in the field, who will assess the methods used, sample size, and limitations of the study. A study may be rejected for publication if it does not meet the rigorous standards of the journal or is deemed to be poorly conducted (see also Chap. 59, "Critical Appraisal of Quantitative ...
Differences in methodological strengths and weaknesses between quantitative and qualitative research are discussed, followed by a data mining exercise on 1,089 journal articles published in Adult Education Quarterly, Studies in Continuing Education, and International Journal of Lifelong Learning. A categorization of quantitative adult education ...
To set the stage for this project, we will begin with a brief review of the literature on triangulation coding in mixed methods research and evaluation. ... A discussion and case study of the unplanned triangulation of quantitative and qualitative research methods. International Journal of Social Research Methodology, 1, 47-63. Crossref ...
American Sociological Review, 21, 683-690. Crossref. ISI. Google Scholar. ... Living within blurry boundaries: The value of distinguishing between qualitative and quantitative research. Journal of Mixed Methods Research, 12(3), 268-279. Crossref. ISI. Google Scholar. Morgan D. L. (2018b). Rebuttal. Journal of ... Social Media Peer Communication ...
This article focuses on the application of quantitative methods in schoolscape research, including a discussion of its advantages and disadvantages. This article seeks to rehabilitate the quantitative by re-theorizing the landscape in linguistic landscape (LL), moving from an area based study of visible forms to a poststructuralist and ...
Adhering to PRISMA guidelines, we conducted a systematic review of peer-reviewed articles indexed within Scopus, Medline, ProQuest Political Science and Public Administration, and PsycINFO. Original English-language quantitative or mixed-methods research or methods papers that explicitly applied intersectionality theoretical frameworks were ...
Scope and Aims (Adapted from journals' mission statements) Impact Factor*. American Journal of Evaluation. American Evaluation Association/Sage. Explores decisions and challenges related to conceptualizing, designing and conducting evaluations. Offers original articles about the methods, theory, ethics, politics, and practice of evaluation.
Ranked #500 out of 1,415 "Sociology and Political Science" journals. Founded in 2009, the Journal of the Society for Social Work and Research ( JSSWR) is the flagship publication of the Society for Social Work and Research (SSWR), a freestanding organization founded in 1994 to advance social work research. JSSWR is a peer-reviewed ...
This paper examines research on peer review between 1969 and 2015 by looking at records indexed from the Scopus database. Although it is often argued that peer review has been poorly investigated, we found that the number of publications in this field doubled from 2005. A half of this work was indexed as research articles, a third as editorial notes and literature reviews and the rest were ...
Title, keywords and the authors. The title of a paper should be clear and give a good idea of the subject area. The title should not normally exceed 15 words 2 and should attract the attention of the reader. 3 The next step is to review the key words. These should provide information on both the ideas or concepts discussed in the paper and the ...
3. Quantitative Methods for Evaluating Implementation Outcomes. While summative evaluation is distinguishable from formative evaluation (see Elwy et al. this issue), proper understanding of the implementation strategy requires using both methods, perhaps at different stages of implementation research (The Health Foundation, 2015).Formative evaluation is a rigorous assessment process designed ...
Burnout is a large problem in social professions, especially in health care worldwide [] and is consistently associated with nurses intention to leave their profession [].Burnout is a state of emotional, physical, and mental exhaustion caused by a long-term mismatch of the demands associated with the job and the resources of the worker [].One of the causes for the alarming increase in nursing ...
A quantitative research study collects numerical data that must be analyzed to help draw the study's conclusions. ... Lam C. (2013) An overview of experimental and quasi-experimental research in technical communication journals (1992-2011). IEEE Transactions on Professional Communication 56 ... Review of analysis techniques in mental health ...
2. Questionnaire researchers, journal editors and reviewers should be more careful and suspicious about using published measures in management research. Designing new questionnaires is tricky and time consuming, so it is tempting to use and re-use existing ones for practical and legitimation reasons (Scherbaum & Meade, 2009). Moreover, the use ...
The first step in the critique process is for the reader to browse the abstract and article for an overview. During this initial review a great deal of information can be obtained. The abstract should provide a clear, concise overview of the study. During this review it should be noted if the title, problem statement, and research question (or ...
Background Peer review is at the heart of the scientific process. With the advent of digitisation, journals started to offer electronic articles or publishing online only. A new philosophy regarding the peer review process found its way into academia: the open peer review. Open peer review as practiced by BioMed Central (BMC) is a type of peer review where the names of authors and reviewers ...
We offer best-practice recommendations for journal reviewers, editors, and authors regarding data collection and preparation. Our recommendations are applicable to research adopting different epistemological and ontological perspectives—including both quantitative and qualitative approaches—as well as research addressing micro (i.e., individuals, teams) and macro (i.e., organizations ...