Have a language expert improve your writing
Run a free plagiarism check in 10 minutes, generate accurate citations for free.
- Knowledge Base
Methodology
- Sampling Methods | Types, Techniques & Examples
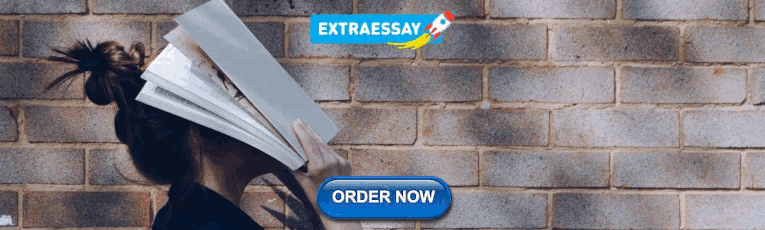
Sampling Methods | Types, Techniques & Examples
Published on September 19, 2019 by Shona McCombes . Revised on June 22, 2023.
When you conduct research about a group of people, it’s rarely possible to collect data from every person in that group. Instead, you select a sample . The sample is the group of individuals who will actually participate in the research.
To draw valid conclusions from your results, you have to carefully decide how you will select a sample that is representative of the group as a whole. This is called a sampling method . There are two primary types of sampling methods that you can use in your research:
- Probability sampling involves random selection, allowing you to make strong statistical inferences about the whole group.
- Non-probability sampling involves non-random selection based on convenience or other criteria, allowing you to easily collect data.
You should clearly explain how you selected your sample in the methodology section of your paper or thesis, as well as how you approached minimizing research bias in your work.
Table of contents
Population vs. sample, probability sampling methods, non-probability sampling methods, other interesting articles, frequently asked questions about sampling.
First, you need to understand the difference between a population and a sample , and identify the target population of your research.
- The population is the entire group that you want to draw conclusions about.
- The sample is the specific group of individuals that you will collect data from.
The population can be defined in terms of geographical location, age, income, or many other characteristics.
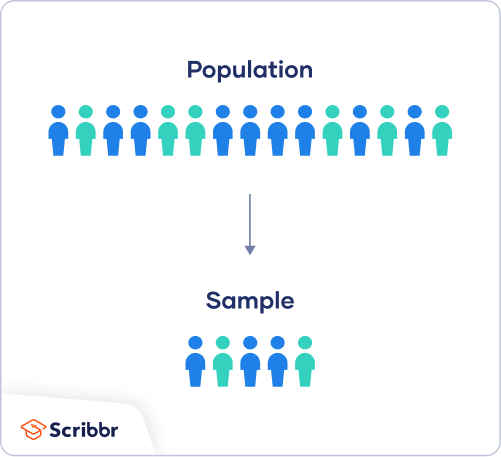
It is important to carefully define your target population according to the purpose and practicalities of your project.
If the population is very large, demographically mixed, and geographically dispersed, it might be difficult to gain access to a representative sample. A lack of a representative sample affects the validity of your results, and can lead to several research biases , particularly sampling bias .
Sampling frame
The sampling frame is the actual list of individuals that the sample will be drawn from. Ideally, it should include the entire target population (and nobody who is not part of that population).
Sample size
The number of individuals you should include in your sample depends on various factors, including the size and variability of the population and your research design. There are different sample size calculators and formulas depending on what you want to achieve with statistical analysis .
Prevent plagiarism. Run a free check.
Probability sampling means that every member of the population has a chance of being selected. It is mainly used in quantitative research . If you want to produce results that are representative of the whole population, probability sampling techniques are the most valid choice.
There are four main types of probability sample.
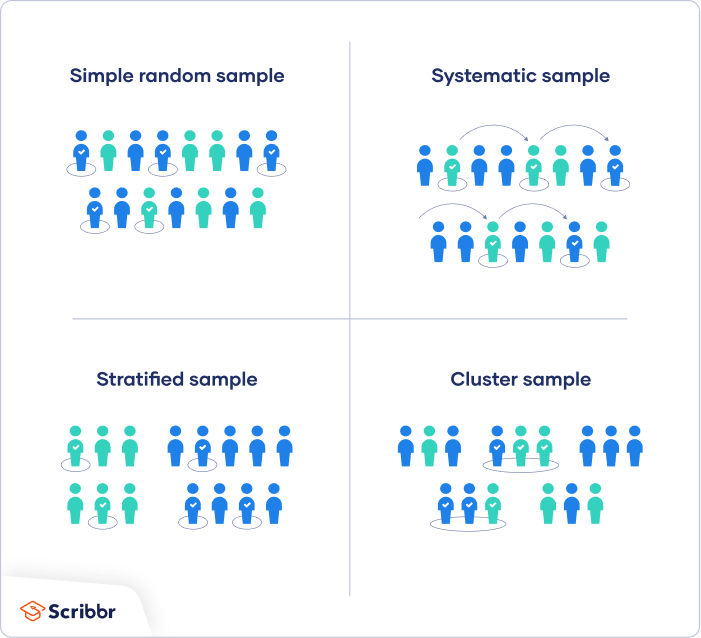
1. Simple random sampling
In a simple random sample, every member of the population has an equal chance of being selected. Your sampling frame should include the whole population.
To conduct this type of sampling, you can use tools like random number generators or other techniques that are based entirely on chance.
2. Systematic sampling
Systematic sampling is similar to simple random sampling, but it is usually slightly easier to conduct. Every member of the population is listed with a number, but instead of randomly generating numbers, individuals are chosen at regular intervals.
If you use this technique, it is important to make sure that there is no hidden pattern in the list that might skew the sample. For example, if the HR database groups employees by team, and team members are listed in order of seniority, there is a risk that your interval might skip over people in junior roles, resulting in a sample that is skewed towards senior employees.
3. Stratified sampling
Stratified sampling involves dividing the population into subpopulations that may differ in important ways. It allows you draw more precise conclusions by ensuring that every subgroup is properly represented in the sample.
To use this sampling method, you divide the population into subgroups (called strata) based on the relevant characteristic (e.g., gender identity, age range, income bracket, job role).
Based on the overall proportions of the population, you calculate how many people should be sampled from each subgroup. Then you use random or systematic sampling to select a sample from each subgroup.
4. Cluster sampling
Cluster sampling also involves dividing the population into subgroups, but each subgroup should have similar characteristics to the whole sample. Instead of sampling individuals from each subgroup, you randomly select entire subgroups.
If it is practically possible, you might include every individual from each sampled cluster. If the clusters themselves are large, you can also sample individuals from within each cluster using one of the techniques above. This is called multistage sampling .
This method is good for dealing with large and dispersed populations, but there is more risk of error in the sample, as there could be substantial differences between clusters. It’s difficult to guarantee that the sampled clusters are really representative of the whole population.
In a non-probability sample, individuals are selected based on non-random criteria, and not every individual has a chance of being included.
This type of sample is easier and cheaper to access, but it has a higher risk of sampling bias . That means the inferences you can make about the population are weaker than with probability samples, and your conclusions may be more limited. If you use a non-probability sample, you should still aim to make it as representative of the population as possible.
Non-probability sampling techniques are often used in exploratory and qualitative research . In these types of research, the aim is not to test a hypothesis about a broad population, but to develop an initial understanding of a small or under-researched population.
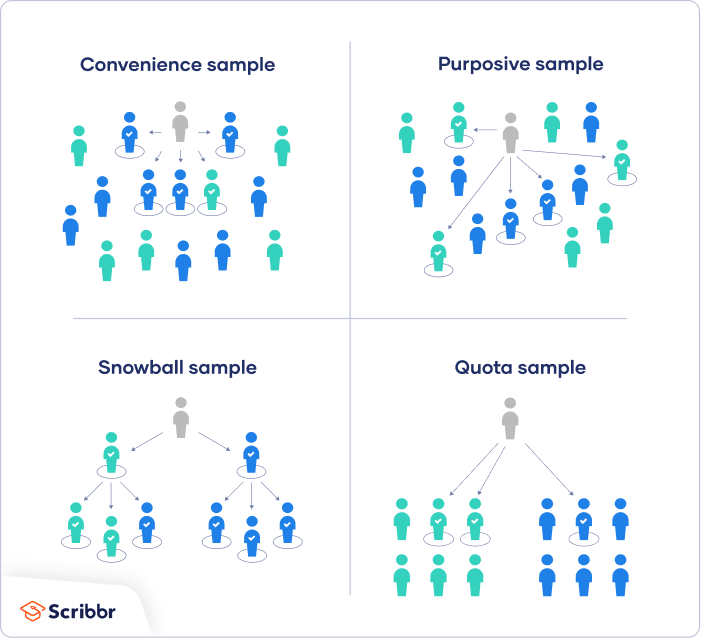
1. Convenience sampling
A convenience sample simply includes the individuals who happen to be most accessible to the researcher.
This is an easy and inexpensive way to gather initial data, but there is no way to tell if the sample is representative of the population, so it can’t produce generalizable results. Convenience samples are at risk for both sampling bias and selection bias .
2. Voluntary response sampling
Similar to a convenience sample, a voluntary response sample is mainly based on ease of access. Instead of the researcher choosing participants and directly contacting them, people volunteer themselves (e.g. by responding to a public online survey).
Voluntary response samples are always at least somewhat biased , as some people will inherently be more likely to volunteer than others, leading to self-selection bias .
3. Purposive sampling
This type of sampling, also known as judgement sampling, involves the researcher using their expertise to select a sample that is most useful to the purposes of the research.
It is often used in qualitative research , where the researcher wants to gain detailed knowledge about a specific phenomenon rather than make statistical inferences, or where the population is very small and specific. An effective purposive sample must have clear criteria and rationale for inclusion. Always make sure to describe your inclusion and exclusion criteria and beware of observer bias affecting your arguments.
4. Snowball sampling
If the population is hard to access, snowball sampling can be used to recruit participants via other participants. The number of people you have access to “snowballs” as you get in contact with more people. The downside here is also representativeness, as you have no way of knowing how representative your sample is due to the reliance on participants recruiting others. This can lead to sampling bias .
5. Quota sampling
Quota sampling relies on the non-random selection of a predetermined number or proportion of units. This is called a quota.
You first divide the population into mutually exclusive subgroups (called strata) and then recruit sample units until you reach your quota. These units share specific characteristics, determined by you prior to forming your strata. The aim of quota sampling is to control what or who makes up your sample.
If you want to know more about statistics , methodology , or research bias , make sure to check out some of our other articles with explanations and examples.
- Student’s t -distribution
- Normal distribution
- Null and Alternative Hypotheses
- Chi square tests
- Confidence interval
- Quartiles & Quantiles
- Cluster sampling
- Stratified sampling
- Data cleansing
- Reproducibility vs Replicability
- Peer review
- Prospective cohort study
Research bias
- Implicit bias
- Cognitive bias
- Placebo effect
- Hawthorne effect
- Hindsight bias
- Affect heuristic
- Social desirability bias
A sample is a subset of individuals from a larger population . Sampling means selecting the group that you will actually collect data from in your research. For example, if you are researching the opinions of students in your university, you could survey a sample of 100 students.
In statistics, sampling allows you to test a hypothesis about the characteristics of a population.
Samples are used to make inferences about populations . Samples are easier to collect data from because they are practical, cost-effective, convenient, and manageable.
Probability sampling means that every member of the target population has a known chance of being included in the sample.
Probability sampling methods include simple random sampling , systematic sampling , stratified sampling , and cluster sampling .
In non-probability sampling , the sample is selected based on non-random criteria, and not every member of the population has a chance of being included.
Common non-probability sampling methods include convenience sampling , voluntary response sampling, purposive sampling , snowball sampling, and quota sampling .
In multistage sampling , or multistage cluster sampling, you draw a sample from a population using smaller and smaller groups at each stage.
This method is often used to collect data from a large, geographically spread group of people in national surveys, for example. You take advantage of hierarchical groupings (e.g., from state to city to neighborhood) to create a sample that’s less expensive and time-consuming to collect data from.
Sampling bias occurs when some members of a population are systematically more likely to be selected in a sample than others.
Cite this Scribbr article
If you want to cite this source, you can copy and paste the citation or click the “Cite this Scribbr article” button to automatically add the citation to our free Citation Generator.
McCombes, S. (2023, June 22). Sampling Methods | Types, Techniques & Examples. Scribbr. Retrieved April 6, 2024, from https://www.scribbr.com/methodology/sampling-methods/
Is this article helpful?
Shona McCombes
Other students also liked, population vs. sample | definitions, differences & examples, simple random sampling | definition, steps & examples, sampling bias and how to avoid it | types & examples, what is your plagiarism score.

Sampling Methods & Strategies 101
Everything you need to know (including examples)
By: Derek Jansen (MBA) | Expert Reviewed By: Kerryn Warren (PhD) | January 2023
If you’re new to research, sooner or later you’re bound to wander into the intimidating world of sampling methods and strategies. If you find yourself on this page, chances are you’re feeling a little overwhelmed or confused. Fear not – in this post we’ll unpack sampling in straightforward language , along with loads of examples .
Overview: Sampling Methods & Strategies
- What is sampling in a research context?
- The two overarching approaches
Simple random sampling
Stratified random sampling, cluster sampling, systematic sampling, purposive sampling, convenience sampling, snowball sampling.
- How to choose the right sampling method
What (exactly) is sampling?
At the simplest level, sampling (within a research context) is the process of selecting a subset of participants from a larger group . For example, if your research involved assessing US consumers’ perceptions about a particular brand of laundry detergent, you wouldn’t be able to collect data from every single person that uses laundry detergent (good luck with that!) – but you could potentially collect data from a smaller subset of this group.
In technical terms, the larger group is referred to as the population , and the subset (the group you’ll actually engage with in your research) is called the sample . Put another way, you can look at the population as a full cake and the sample as a single slice of that cake. In an ideal world, you’d want your sample to be perfectly representative of the population, as that would allow you to generalise your findings to the entire population. In other words, you’d want to cut a perfect cross-sectional slice of cake, such that the slice reflects every layer of the cake in perfect proportion.
Achieving a truly representative sample is, unfortunately, a little trickier than slicing a cake, as there are many practical challenges and obstacles to achieving this in a real-world setting. Thankfully though, you don’t always need to have a perfectly representative sample – it all depends on the specific research aims of each study – so don’t stress yourself out about that just yet!
With the concept of sampling broadly defined, let’s look at the different approaches to sampling to get a better understanding of what it all looks like in practice.
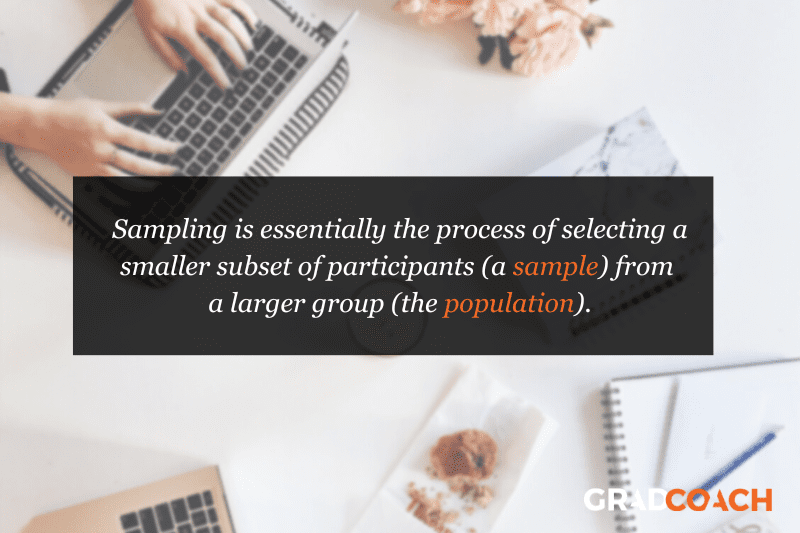
The two overarching sampling approaches
At the highest level, there are two approaches to sampling: probability sampling and non-probability sampling . Within each of these, there are a variety of sampling methods , which we’ll explore a little later.
Probability sampling involves selecting participants (or any unit of interest) on a statistically random basis , which is why it’s also called “random sampling”. In other words, the selection of each individual participant is based on a pre-determined process (not the discretion of the researcher). As a result, this approach achieves a random sample.
Probability-based sampling methods are most commonly used in quantitative research , especially when it’s important to achieve a representative sample that allows the researcher to generalise their findings.
Non-probability sampling , on the other hand, refers to sampling methods in which the selection of participants is not statistically random . In other words, the selection of individual participants is based on the discretion and judgment of the researcher, rather than on a pre-determined process.
Non-probability sampling methods are commonly used in qualitative research , where the richness and depth of the data are more important than the generalisability of the findings.
If that all sounds a little too conceptual and fluffy, don’t worry. Let’s take a look at some actual sampling methods to make it more tangible.
Need a helping hand?
Probability-based sampling methods
First, we’ll look at four common probability-based (random) sampling methods:
Importantly, this is not a comprehensive list of all the probability sampling methods – these are just four of the most common ones. So, if you’re interested in adopting a probability-based sampling approach, be sure to explore all the options.
Simple random sampling involves selecting participants in a completely random fashion , where each participant has an equal chance of being selected. Basically, this sampling method is the equivalent of pulling names out of a hat , except that you can do it digitally. For example, if you had a list of 500 people, you could use a random number generator to draw a list of 50 numbers (each number, reflecting a participant) and then use that dataset as your sample.
Thanks to its simplicity, simple random sampling is easy to implement , and as a consequence, is typically quite cheap and efficient . Given that the selection process is completely random, the results can be generalised fairly reliably. However, this also means it can hide the impact of large subgroups within the data, which can result in minority subgroups having little representation in the results – if any at all. To address this, one needs to take a slightly different approach, which we’ll look at next.
Stratified random sampling is similar to simple random sampling, but it kicks things up a notch. As the name suggests, stratified sampling involves selecting participants randomly , but from within certain pre-defined subgroups (i.e., strata) that share a common trait . For example, you might divide the population into strata based on gender, ethnicity, age range or level of education, and then select randomly from each group.
The benefit of this sampling method is that it gives you more control over the impact of large subgroups (strata) within the population. For example, if a population comprises 80% males and 20% females, you may want to “balance” this skew out by selecting a random sample from an equal number of males and females. This would, of course, reduce the representativeness of the sample, but it would allow you to identify differences between subgroups. So, depending on your research aims, the stratified approach could work well.
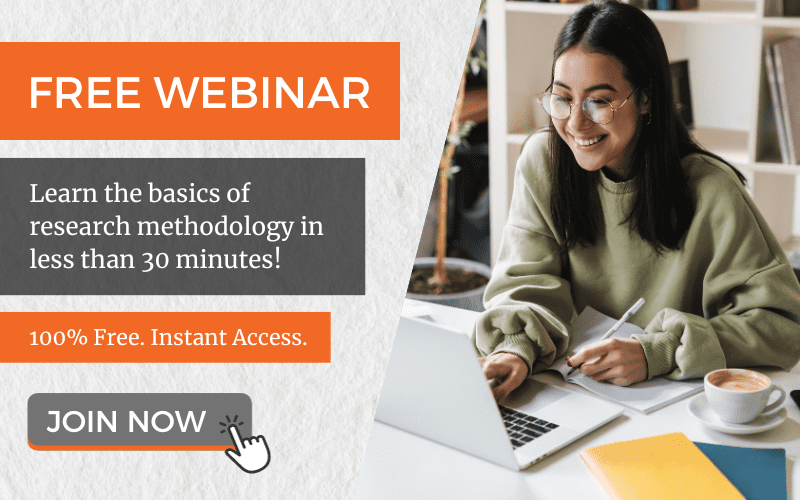
Next on the list is cluster sampling. As the name suggests, this sampling method involves sampling from naturally occurring, mutually exclusive clusters within a population – for example, area codes within a city or cities within a country. Once the clusters are defined, a set of clusters are randomly selected and then a set of participants are randomly selected from each cluster.
Now, you’re probably wondering, “how is cluster sampling different from stratified random sampling?”. Well, let’s look at the previous example where each cluster reflects an area code in a given city.
With cluster sampling, you would collect data from clusters of participants in a handful of area codes (let’s say 5 neighbourhoods). Conversely, with stratified random sampling, you would need to collect data from all over the city (i.e., many more neighbourhoods). You’d still achieve the same sample size either way (let’s say 200 people, for example), but with stratified sampling, you’d need to do a lot more running around, as participants would be scattered across a vast geographic area. As a result, cluster sampling is often the more practical and economical option.
If that all sounds a little mind-bending, you can use the following general rule of thumb. If a population is relatively homogeneous , cluster sampling will often be adequate. Conversely, if a population is quite heterogeneous (i.e., diverse), stratified sampling will generally be more appropriate.
The last probability sampling method we’ll look at is systematic sampling. This method simply involves selecting participants at a set interval , starting from a random point .
For example, if you have a list of students that reflects the population of a university, you could systematically sample that population by selecting participants at an interval of 8 . In other words, you would randomly select a starting point – let’s say student number 40 – followed by student 48, 56, 64, etc.
What’s important with systematic sampling is that the population list you select from needs to be randomly ordered . If there are underlying patterns in the list (for example, if the list is ordered by gender, IQ, age, etc.), this will result in a non-random sample, which would defeat the purpose of adopting this sampling method. Of course, you could safeguard against this by “shuffling” your population list using a random number generator or similar tool.
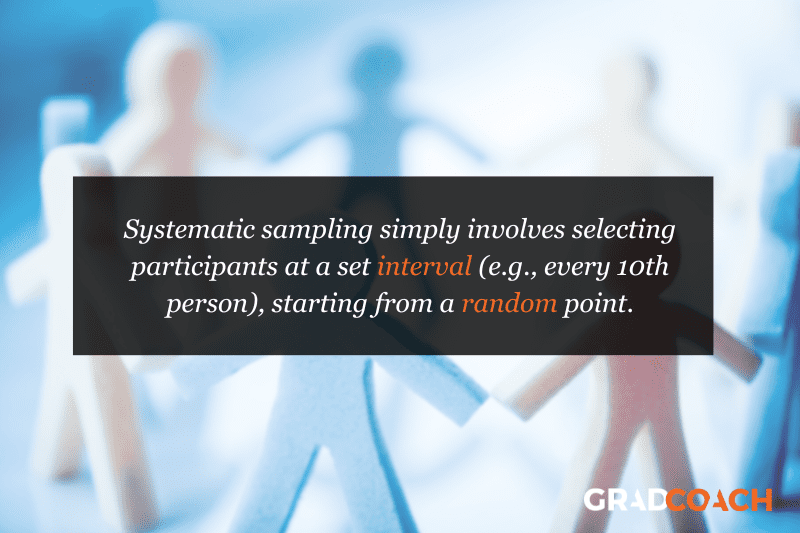
Non-probability-based sampling methods
Right, now that we’ve looked at a few probability-based sampling methods, let’s look at three non-probability methods :
Again, this is not an exhaustive list of all possible sampling methods, so be sure to explore further if you’re interested in adopting a non-probability sampling approach.
First up, we’ve got purposive sampling – also known as judgment , selective or subjective sampling. Again, the name provides some clues, as this method involves the researcher selecting participants using his or her own judgement , based on the purpose of the study (i.e., the research aims).
For example, suppose your research aims were to understand the perceptions of hyper-loyal customers of a particular retail store. In that case, you could use your judgement to engage with frequent shoppers, as well as rare or occasional shoppers, to understand what judgements drive the two behavioural extremes .
Purposive sampling is often used in studies where the aim is to gather information from a small population (especially rare or hard-to-find populations), as it allows the researcher to target specific individuals who have unique knowledge or experience . Naturally, this sampling method is quite prone to researcher bias and judgement error, and it’s unlikely to produce generalisable results, so it’s best suited to studies where the aim is to go deep rather than broad .
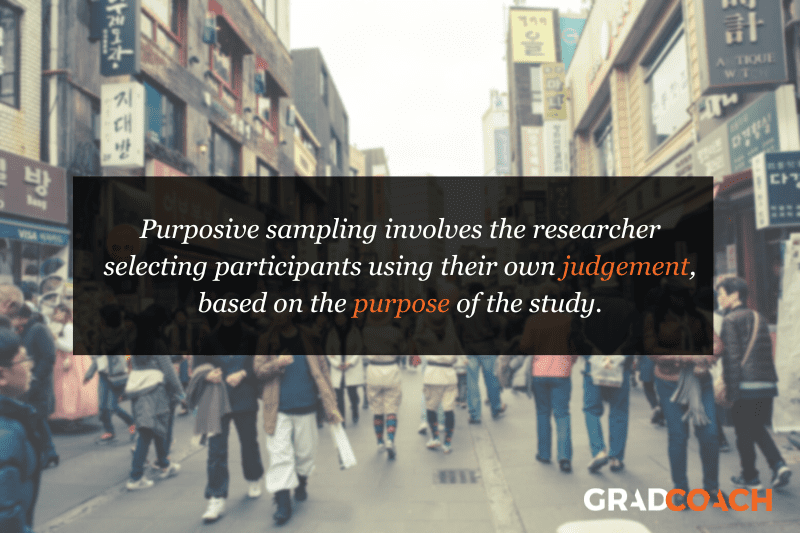
Next up, we have convenience sampling. As the name suggests, with this method, participants are selected based on their availability or accessibility . In other words, the sample is selected based on how convenient it is for the researcher to access it, as opposed to using a defined and objective process.
Naturally, convenience sampling provides a quick and easy way to gather data, as the sample is selected based on the individuals who are readily available or willing to participate. This makes it an attractive option if you’re particularly tight on resources and/or time. However, as you’d expect, this sampling method is unlikely to produce a representative sample and will of course be vulnerable to researcher bias , so it’s important to approach it with caution.
Last but not least, we have the snowball sampling method. This method relies on referrals from initial participants to recruit additional participants. In other words, the initial subjects form the first (small) snowball and each additional subject recruited through referral is added to the snowball, making it larger as it rolls along .
Snowball sampling is often used in research contexts where it’s difficult to identify and access a particular population. For example, people with a rare medical condition or members of an exclusive group. It can also be useful in cases where the research topic is sensitive or taboo and people are unlikely to open up unless they’re referred by someone they trust.
Simply put, snowball sampling is ideal for research that involves reaching hard-to-access populations . But, keep in mind that, once again, it’s a sampling method that’s highly prone to researcher bias and is unlikely to produce a representative sample. So, make sure that it aligns with your research aims and questions before adopting this method.
How to choose a sampling method
Now that we’ve looked at a few popular sampling methods (both probability and non-probability based), the obvious question is, “ how do I choose the right sampling method for my study?”. When selecting a sampling method for your research project, you’ll need to consider two important factors: your research aims and your resources .
As with all research design and methodology choices, your sampling approach needs to be guided by and aligned with your research aims, objectives and research questions – in other words, your golden thread. Specifically, you need to consider whether your research aims are primarily concerned with producing generalisable findings (in which case, you’ll likely opt for a probability-based sampling method) or with achieving rich , deep insights (in which case, a non-probability-based approach could be more practical). Typically, quantitative studies lean toward the former, while qualitative studies aim for the latter, so be sure to consider your broader methodology as well.
The second factor you need to consider is your resources and, more generally, the practical constraints at play. If, for example, you have easy, free access to a large sample at your workplace or university and a healthy budget to help you attract participants, that will open up multiple options in terms of sampling methods. Conversely, if you’re cash-strapped, short on time and don’t have unfettered access to your population of interest, you may be restricted to convenience or referral-based methods.
In short, be ready for trade-offs – you won’t always be able to utilise the “perfect” sampling method for your study, and that’s okay. Much like all the other methodological choices you’ll make as part of your study, you’ll often need to compromise and accept practical trade-offs when it comes to sampling. Don’t let this get you down though – as long as your sampling choice is well explained and justified, and the limitations of your approach are clearly articulated, you’ll be on the right track.
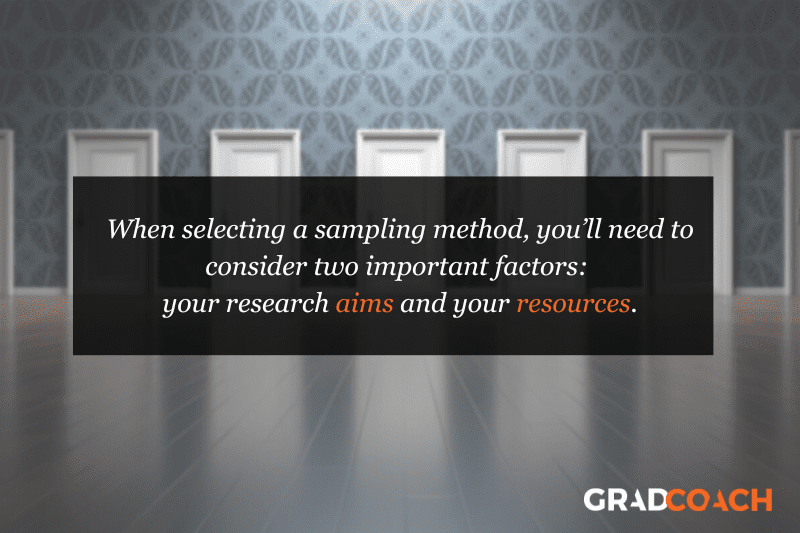
Let’s recap…
In this post, we’ve covered the basics of sampling within the context of a typical research project.
- Sampling refers to the process of defining a subgroup (sample) from the larger group of interest (population).
- The two overarching approaches to sampling are probability sampling (random) and non-probability sampling .
- Common probability-based sampling methods include simple random sampling, stratified random sampling, cluster sampling and systematic sampling.
- Common non-probability-based sampling methods include purposive sampling, convenience sampling and snowball sampling.
- When choosing a sampling method, you need to consider your research aims , objectives and questions, as well as your resources and other practical constraints .
If you’d like to see an example of a sampling strategy in action, be sure to check out our research methodology chapter sample .
Last but not least, if you need hands-on help with your sampling (or any other aspect of your research), take a look at our 1-on-1 coaching service , where we guide you through each step of the research process, at your own pace.
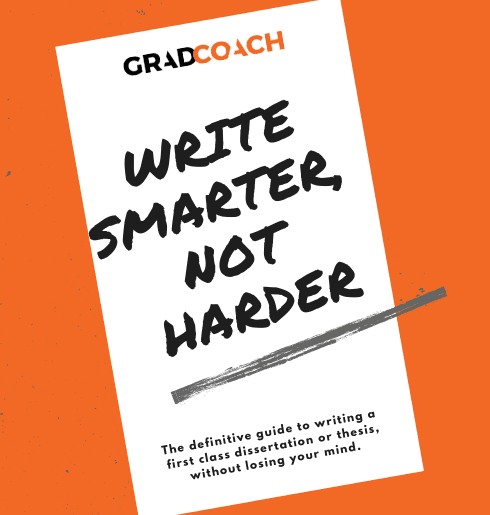
Psst… there’s more (for free)
This post is part of our dissertation mini-course, which covers everything you need to get started with your dissertation, thesis or research project.
You Might Also Like:
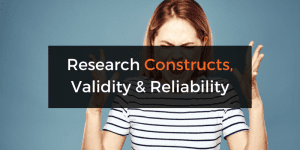
Excellent and helpful. Best site to get a full understanding of Research methodology. I’m nolonger as “clueless “..😉
Excellent and helpful for junior researcher!
Submit a Comment Cancel reply
Your email address will not be published. Required fields are marked *
Save my name, email, and website in this browser for the next time I comment.
- Print Friendly
Sampling Techniques for Quantitative Research
- First Online: 27 October 2022
Cite this chapter
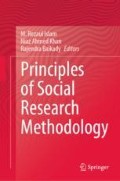
- Moniruzzaman Sarker ORCID: orcid.org/0000-0003-3595-5838 4 &
- Mohammed Abdulmalek AL-Muaalemi 5
3014 Accesses
8 Citations
In quantitative research, collecting data from an entire population of a study is impractical in many instances. It squanders resources like time and money which can be minimized by choosing suitable sampling techniques between probability and non-probability methods. The chapter outlines a brief idea about the different categories of sampling techniques with examples. Sensibly selecting among the sampling techniques allows the researcher to generalize the findings to a specific study context. Although probability sampling is more appealing to draw a representative sample, non-probability sampling techniques also enable the researcher to generalize the findings upon implementing the sampling strategy wisely. Moreover, adopting probability sampling techniques is not feasible in many situations. The chapter suggests selecting sampling techniques should be guided by research objectives, study scope, and availability of sampling frame rather than looking at the nature of sampling techniques.
- Sampling techniques
- Quantitative study
- Probability sampling
- Non-probability sampling
This is a preview of subscription content, log in via an institution to check access.
Access this chapter
- Available as PDF
- Read on any device
- Instant download
- Own it forever
- Available as EPUB and PDF
- Compact, lightweight edition
- Dispatched in 3 to 5 business days
- Free shipping worldwide - see info
- Durable hardcover edition
Tax calculation will be finalised at checkout
Purchases are for personal use only
Institutional subscriptions
Bryman, A., & Bell, E. (2015). Business research methods (4th ed.). Oxford University Press.
Google Scholar
Clottey, T. A., & Grawe, S. J. (2014). Non-response bias assessment in logistics survey research: Use fewer tests? International Journal of Physical Distribution & Logistics Management, 44 (5), 412–426. https://doi.org/10.1108/IJPDLM-10-2012-0314
Article Google Scholar
Collier, J. E., & Bienstock, C. C. (2007). An analysis of how nonresponse error is assessed in academic marketing research. Marketing Theory, 7 (2), 163–183. https://doi.org/10.1177/1470593107076865
Cooper, D. R., Schindler, P. S., & Sun, J. (2006). Business research methods (9th ed.). McGraw-Hill Irwin.
Creswell, J. W. (2014). Research design: Qualitative, quantitative, and mixed methods approaches (4th ed.). SAGE Publications, Incorporated.
Feild, L., Pruchno, R. A., Bewley, J., Lemay, E. P., & Levinsky, N. G. (2006). Using probability versus nonprobability sampling to identify hard-to-access participants for health-related research: Costs and contrasts. Journal of Aging and Health, 18 (4), 565–583. https://doi.org/10.1177/0898264306291420.
Hulland, J., Baumgartner, H., & Smith, K. M. (2017). Marketing survey research best practices: Evidence and recommendations from a review of JAMS articles. Journal of the Academy of Marketing Science, 46 (1), 92–108. https://doi.org/10.1007/s11747-017-0532-y
Lindner, J. R., Murphy, T. H., & Briers, G. E. (2001). Handling nonresponse in social science research. Journal of Agricultural Education, 42 (4), 43–53.
Malhotra, N. K., & Das, S. (2010). Marketing research: An applied orientation (6th ed.). Pearson Education.
Book Google Scholar
Marshall, M. N. (1996). Sampling for qualitative research. Family Practice, 13 (6), 522–526. https://doi.org/10.1093/fampra/13.6.522
Memon, M. A., Ting, H., Ramayah, T., Chuah, F., & Cheah, J.-H. (2017). A review of the methodological misconceptions and guidelines related to the application of structural equation modeling: A Malaysian scenario. Journal of Applied Structural Equation Modeling, 1 (1), i–xiii.
Rowley, J. (2014). Designing and using research questionnaires. Management Research Review, 37 (3), 308–330. https://doi.org/10.1108/MRR-02-2013-0027
Sarstedt, M., Bengart, P., Shaltoni, A. M., & Lehmann, S. (2018). The use of sampling methods in advertising research: A gap between theory and practice. International Journal of Advertising, 37 (4), 650–663. https://doi.org/10.1080/02650487.2017.1348329
Sax, L. J., Gilmartin, S. K., & Bryant, A. N. (2003). Assessing response rates and nonresponse bias in web and paper surveys. Research in Higher Education, 44 (4), 409–432. https://doi.org/10.1023/a:1024232915870
Seddon, P. B., & Scheepers, R. (2012). Towards the improved treatment of generalization of knowledge claims in IS research: Drawing general conclusions from samples. European Journal of Information Systems, 21 (1), 6–21.
Sekaran, U., & Bougie, R. (2016). Research methods for business: A skill building approach (7th ed.). John Wiley & Sons.
Zikmund, W. G., Babin, B. J., Carr, J. C., & Griffin, M. (2013). Business research methods (9th ed.). Cengage Learning.
Download references
Author information
Authors and affiliations.
Assistant Professor of Marketing, Southamton Malysia Business School, University of Southamton Malysia, Johor Bahru, Malaysia
Moniruzzaman Sarker
Faculty of Business and Accountancy, University of Malaya, Kuala Lumpur, Malaysia
Mohammed Abdulmalek AL-Muaalemi
You can also search for this author in PubMed Google Scholar
Corresponding author
Correspondence to Moniruzzaman Sarker .
Editor information
Editors and affiliations.
Centre for Family and Child Studies, Research Institute of Humanities and Social Sciences, University of Sharjah, Sharjah, United Arab Emirates
M. Rezaul Islam
Department of Development Studies, University of Dhaka, Dhaka, Bangladesh
Niaz Ahmed Khan
Department of Social Work, School of Humanities, University of Johannesburg, Johannesburg, South Africa
Rajendra Baikady
Rights and permissions
Reprints and permissions
Copyright information
© 2022 The Author(s), under exclusive license to Springer Nature Singapore Pte Ltd.
About this chapter
Moniruzzaman Sarker, AL-Muaalemi, M.A. (2022). Sampling Techniques for Quantitative Research. In: Islam, M.R., Khan, N.A., Baikady, R. (eds) Principles of Social Research Methodology. Springer, Singapore. https://doi.org/10.1007/978-981-19-5441-2_15
Download citation
DOI : https://doi.org/10.1007/978-981-19-5441-2_15
Published : 27 October 2022
Publisher Name : Springer, Singapore
Print ISBN : 978-981-19-5219-7
Online ISBN : 978-981-19-5441-2
eBook Packages : Social Sciences
Share this chapter
Anyone you share the following link with will be able to read this content:
Sorry, a shareable link is not currently available for this article.
Provided by the Springer Nature SharedIt content-sharing initiative
- Publish with us
Policies and ethics
- Find a journal
- Track your research
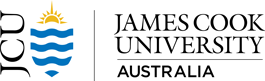
Want to create or adapt books like this? Learn more about how Pressbooks supports open publishing practices.
3.4 Sampling Techniques in Quantitative Research
Target population.
The target population includes the people the researcher is interested in conducting the research and generalizing the findings on. 40 For example, if certain researchers are interested in vaccine-preventable diseases in children five years and younger in Australia. The target population will be all children aged 0–5 years residing in Australia. The actual population is a subset of the target population from which the sample is drawn, e.g. children aged 0–5 years living in the capital cities in Australia. The sample is the people chosen for the study from the actual population (Figure 3.9). The sampling process involves choosing people, and it is distinct from the sample. 40 In quantitative research, the sample must accurately reflect the target population, be free from bias in terms of selection, and be large enough to validate or reject the study hypothesis with statistical confidence and minimise random error. 2
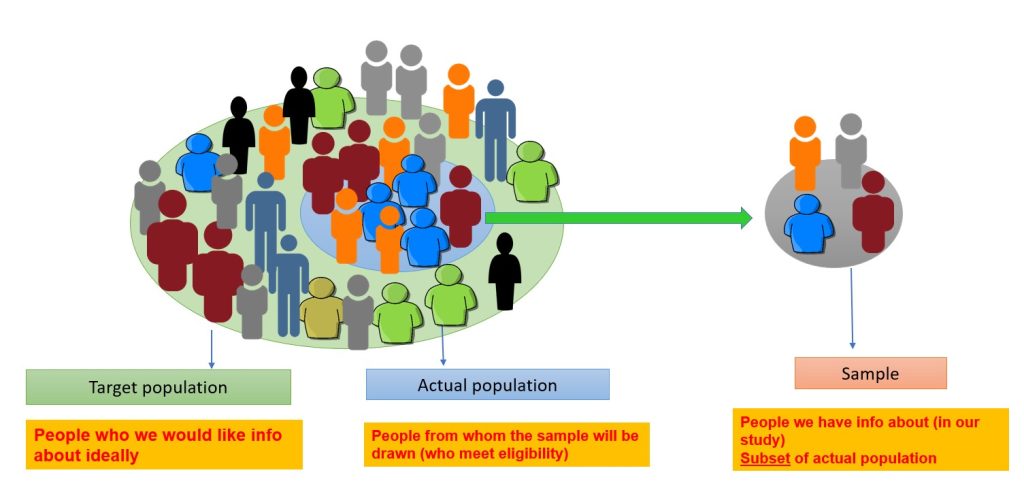
Sampling techniques
Sampling in quantitative research is a critical component that involves selecting a representative subset of individuals or cases from a larger population and often employs sampling techniques based on probability theory. 41 The goal of sampling is to obtain a sample that is large enough and representative of the target population. Examples of probability sampling techniques include simple random sampling, stratified random sampling, systematic random sampling and cluster sampling ( shown below ). 2 The key feature of probability techniques is that they involve randomization. There are two main characteristics of probability sampling. All individuals of a population are accessible to the researcher (theoretically), and there is an equal chance that each person in the population will be chosen to be part of the study sample. 41 While quantitative research often uses sampling techniques based on probability theory, some non-probability techniques may occasionally be utilised in healthcare research. 42 Non-probability sampling methods are commonly used in qualitative research. These include purposive, convenience, theoretical and snowballing and have been discussed in detail in chapter 4.
Sample size calculation
In order to enable comparisons with some level of established statistical confidence, quantitative research needs an acceptable sample size. 2 The sample size is the most crucial factor for reliability (reproducibility) in quantitative research. It is important for a study to be powered – the likelihood of identifying a difference if it exists in reality. 2 Small sample-sized studies are more likely to be underpowered, and results from small samples are more likely to be prone to random error. 2 The formula for sample size calculation varies with the study design and the research hypothesis. 2 There are numerous formulae for sample size calculations, but such details are beyond the scope of this book. For further readings, please consult the biostatistics textbook by Hirsch RP, 2021. 43 However, we will introduce a simple formula for calculating sample size for cross-sectional studies with prevalence as the outcome. 2
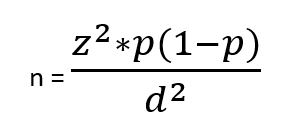
z is the statistical confidence; therefore, z = 1.96 translates to 95% confidence; z = 1.68 translates to 90% confidence
p = Expected prevalence (of health condition of interest)
d = Describes intended precision; d = 0.1 means that the estimate falls +/-10 percentage points of true prevalence with the considered confidence. (e.g. for a prevalence of 40% (0.4), if d=.1, then the estimate will fall between 30% and 50% (0.3 to 0.5).
Example: A district medical officer seeks to estimate the proportion of children in the district receiving appropriate childhood vaccinations. Assuming a simple random sample of a community is to be selected, how many children must be studied if the resulting estimate is to fall within 10% of the true proportion with 95% confidence? It is expected that approximately 50% of the children receive vaccinations
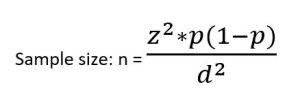
z = 1.96 (95% confidence)
d = 10% = 10/ 100 = 0.1 (estimate to fall within 10%)
p = 50% = 50/ 100 = 0.5
Now we can enter the values into the formula

Given that people cannot be reported in decimal points, it is important to round up to the nearest whole number.
An Introduction to Research Methods for Undergraduate Health Profession Students Copyright © 2023 by Faith Alele and Bunmi Malau-Aduli is licensed under a Creative Commons Attribution-NonCommercial 4.0 International License , except where otherwise noted.
Have a language expert improve your writing
Run a free plagiarism check in 10 minutes, automatically generate references for free.
- Knowledge Base
- Methodology
- Sampling Methods | Types, Techniques, & Examples
Sampling Methods | Types, Techniques, & Examples
Published on 3 May 2022 by Shona McCombes . Revised on 10 October 2022.
When you conduct research about a group of people, it’s rarely possible to collect data from every person in that group. Instead, you select a sample. The sample is the group of individuals who will actually participate in the research.
To draw valid conclusions from your results, you have to carefully decide how you will select a sample that is representative of the group as a whole. There are two types of sampling methods:
- Probability sampling involves random selection, allowing you to make strong statistical inferences about the whole group. It minimises the risk of selection bias .
- Non-probability sampling involves non-random selection based on convenience or other criteria, allowing you to easily collect data.
You should clearly explain how you selected your sample in the methodology section of your paper or thesis.
Table of contents
Population vs sample, probability sampling methods, non-probability sampling methods, frequently asked questions about sampling.
First, you need to understand the difference between a population and a sample , and identify the target population of your research.
- The population is the entire group that you want to draw conclusions about.
- The sample is the specific group of individuals that you will collect data from.
The population can be defined in terms of geographical location, age, income, and many other characteristics.
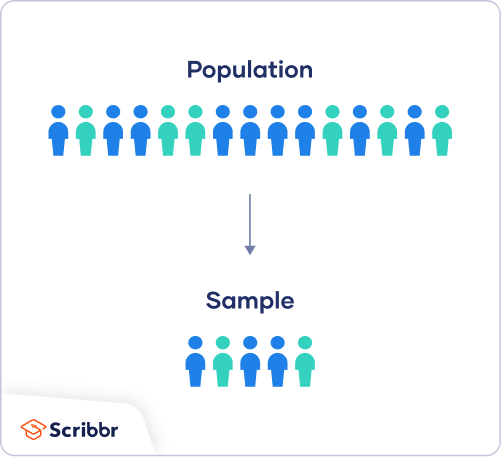
It is important to carefully define your target population according to the purpose and practicalities of your project.
If the population is very large, demographically mixed, and geographically dispersed, it might be difficult to gain access to a representative sample.
Sampling frame
The sampling frame is the actual list of individuals that the sample will be drawn from. Ideally, it should include the entire target population (and nobody who is not part of that population).
You are doing research on working conditions at Company X. Your population is all 1,000 employees of the company. Your sampling frame is the company’s HR database, which lists the names and contact details of every employee.
Sample size
The number of individuals you should include in your sample depends on various factors, including the size and variability of the population and your research design. There are different sample size calculators and formulas depending on what you want to achieve with statistical analysis .
Prevent plagiarism, run a free check.
Probability sampling means that every member of the population has a chance of being selected. It is mainly used in quantitative research . If you want to produce results that are representative of the whole population, probability sampling techniques are the most valid choice.
There are four main types of probability sample.
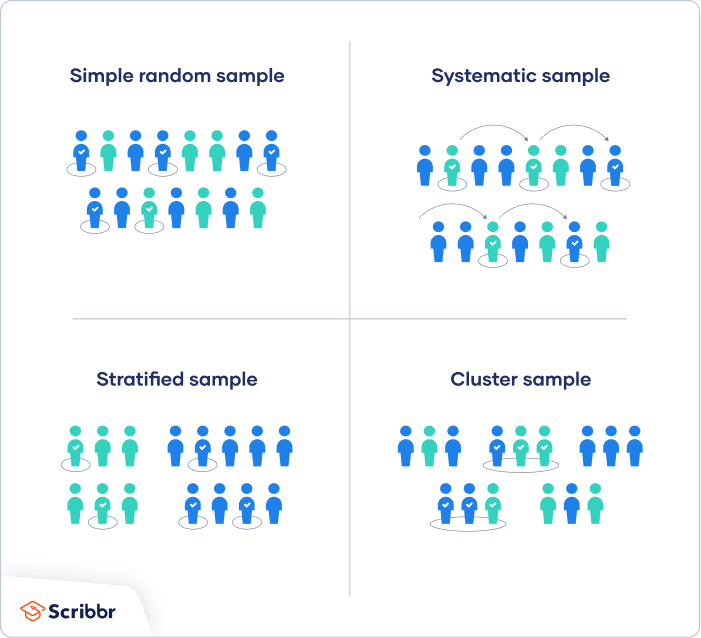
1. Simple random sampling
In a simple random sample , every member of the population has an equal chance of being selected. Your sampling frame should include the whole population.
To conduct this type of sampling, you can use tools like random number generators or other techniques that are based entirely on chance.
You want to select a simple random sample of 100 employees of Company X. You assign a number to every employee in the company database from 1 to 1000, and use a random number generator to select 100 numbers.
2. Systematic sampling
Systematic sampling is similar to simple random sampling, but it is usually slightly easier to conduct. Every member of the population is listed with a number, but instead of randomly generating numbers, individuals are chosen at regular intervals.
All employees of the company are listed in alphabetical order. From the first 10 numbers, you randomly select a starting point: number 6. From number 6 onwards, every 10th person on the list is selected (6, 16, 26, 36, and so on), and you end up with a sample of 100 people.
If you use this technique, it is important to make sure that there is no hidden pattern in the list that might skew the sample. For example, if the HR database groups employees by team, and team members are listed in order of seniority, there is a risk that your interval might skip over people in junior roles, resulting in a sample that is skewed towards senior employees.
3. Stratified sampling
Stratified sampling involves dividing the population into subpopulations that may differ in important ways. It allows you draw more precise conclusions by ensuring that every subgroup is properly represented in the sample.
To use this sampling method, you divide the population into subgroups (called strata) based on the relevant characteristic (e.g., gender, age range, income bracket, job role).
Based on the overall proportions of the population, you calculate how many people should be sampled from each subgroup. Then you use random or systematic sampling to select a sample from each subgroup.
The company has 800 female employees and 200 male employees. You want to ensure that the sample reflects the gender balance of the company, so you sort the population into two strata based on gender. Then you use random sampling on each group, selecting 80 women and 20 men, which gives you a representative sample of 100 people.
4. Cluster sampling
Cluster sampling also involves dividing the population into subgroups, but each subgroup should have similar characteristics to the whole sample. Instead of sampling individuals from each subgroup, you randomly select entire subgroups.
If it is practically possible, you might include every individual from each sampled cluster. If the clusters themselves are large, you can also sample individuals from within each cluster using one of the techniques above. This is called multistage sampling .
This method is good for dealing with large and dispersed populations, but there is more risk of error in the sample, as there could be substantial differences between clusters. It’s difficult to guarantee that the sampled clusters are really representative of the whole population.
The company has offices in 10 cities across the country (all with roughly the same number of employees in similar roles). You don’t have the capacity to travel to every office to collect your data, so you use random sampling to select 3 offices – these are your clusters.
In a non-probability sample , individuals are selected based on non-random criteria, and not every individual has a chance of being included.
This type of sample is easier and cheaper to access, but it has a higher risk of sampling bias . That means the inferences you can make about the population are weaker than with probability samples, and your conclusions may be more limited. If you use a non-probability sample, you should still aim to make it as representative of the population as possible.
Non-probability sampling techniques are often used in exploratory and qualitative research . In these types of research, the aim is not to test a hypothesis about a broad population, but to develop an initial understanding of a small or under-researched population.
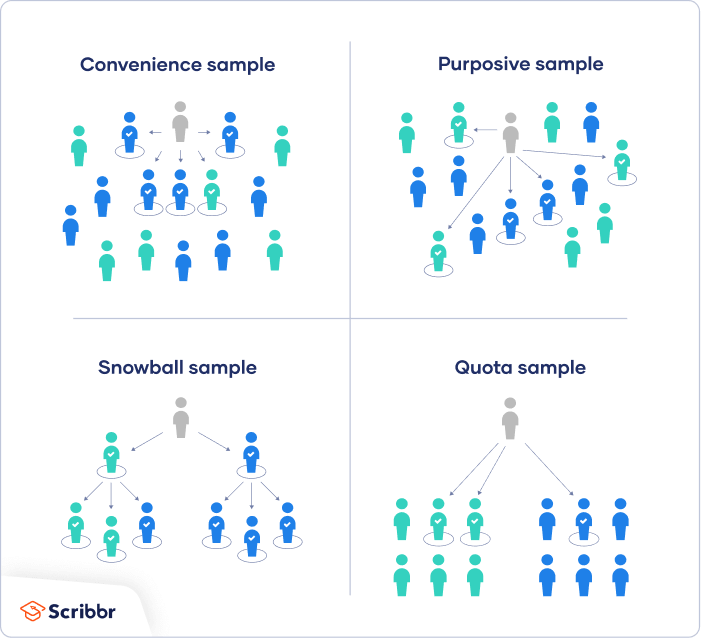
1. Convenience sampling
A convenience sample simply includes the individuals who happen to be most accessible to the researcher.
This is an easy and inexpensive way to gather initial data, but there is no way to tell if the sample is representative of the population, so it can’t produce generalisable results.
You are researching opinions about student support services in your university, so after each of your classes, you ask your fellow students to complete a survey on the topic. This is a convenient way to gather data, but as you only surveyed students taking the same classes as you at the same level, the sample is not representative of all the students at your university.
2. Voluntary response sampling
Similar to a convenience sample, a voluntary response sample is mainly based on ease of access. Instead of the researcher choosing participants and directly contacting them, people volunteer themselves (e.g., by responding to a public online survey).
Voluntary response samples are always at least somewhat biased, as some people will inherently be more likely to volunteer than others.
You send out the survey to all students at your university and many students decide to complete it. This can certainly give you some insight into the topic, but the people who responded are more likely to be those who have strong opinions about the student support services, so you can’t be sure that their opinions are representative of all students.
3. Purposive sampling
Purposive sampling , also known as judgement sampling, involves the researcher using their expertise to select a sample that is most useful to the purposes of the research.
It is often used in qualitative research , where the researcher wants to gain detailed knowledge about a specific phenomenon rather than make statistical inferences, or where the population is very small and specific. An effective purposive sample must have clear criteria and rationale for inclusion.
You want to know more about the opinions and experiences of students with a disability at your university, so you purposely select a number of students with different support needs in order to gather a varied range of data on their experiences with student services.
4. Snowball sampling
If the population is hard to access, snowball sampling can be used to recruit participants via other participants. The number of people you have access to ‘snowballs’ as you get in contact with more people.
You are researching experiences of homelessness in your city. Since there is no list of all homeless people in the city, probability sampling isn’t possible. You meet one person who agrees to participate in the research, and she puts you in contact with other homeless people she knows in the area.
A sample is a subset of individuals from a larger population. Sampling means selecting the group that you will actually collect data from in your research.
For example, if you are researching the opinions of students in your university, you could survey a sample of 100 students.
Statistical sampling allows you to test a hypothesis about the characteristics of a population. There are various sampling methods you can use to ensure that your sample is representative of the population as a whole.
Samples are used to make inferences about populations . Samples are easier to collect data from because they are practical, cost-effective, convenient, and manageable.
Probability sampling means that every member of the target population has a known chance of being included in the sample.
Probability sampling methods include simple random sampling , systematic sampling , stratified sampling , and cluster sampling .
In non-probability sampling , the sample is selected based on non-random criteria, and not every member of the population has a chance of being included.
Common non-probability sampling methods include convenience sampling , voluntary response sampling, purposive sampling , snowball sampling , and quota sampling .
Sampling bias occurs when some members of a population are systematically more likely to be selected in a sample than others.
Cite this Scribbr article
If you want to cite this source, you can copy and paste the citation or click the ‘Cite this Scribbr article’ button to automatically add the citation to our free Reference Generator.
McCombes, S. (2022, October 10). Sampling Methods | Types, Techniques, & Examples. Scribbr. Retrieved 2 April 2024, from https://www.scribbr.co.uk/research-methods/sampling/
Is this article helpful?
Shona McCombes
Other students also liked, what is quantitative research | definition & methods, a quick guide to experimental design | 5 steps & examples, controlled experiments | methods & examples of control.
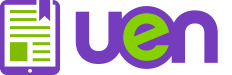
Part I: Sampling, Data Collection, & Analysis in Quantitative Research
In this module, we will focus on how quantitative research collects and analyzes data, as well as methods for obtaining sample population.
- Levels of Measurement
- Reliability and Validity
- Population and Samples
- Common Data Collection Methods
- Data Analysis
- Statistical Significance versus Clinical Significance
Objectives:
- Describe levels of measurement
- Describe reliability and validity as applied to critical appraisal of research
- Differentiate methods of obtaining samples for population generalizability
- Describe common data collection methods in quantitative research
- Describe various data analysis methods in quantitative research
- Differentiate statistical significance versus clinical significance
Levels of measurement
Once researchers have collected their data (we will talk about data collection later in this module), they need methods to organize the data before they even start to think about statistical analyses. Statistical operations depend on a variable’s level of measurement. Think about this similarly to shuffling all of your bills in some type of organization before you pay them. With levels of measurement, we are precisely recording variables in a method to help organize them.
There are four levels of measurement:
Nominal: The data can only be categorized
Ordinal: The data can be categorized and ranked
Interval: The data can be categorized, ranked, and evenly spaced
Ratio: The data can be categorized, ranked, even spaced, and has a natural zero
Going from lowest to highest, the 4 levels of measurement are cumulative. This means that they each take on the properties of lower levels and add new properties.
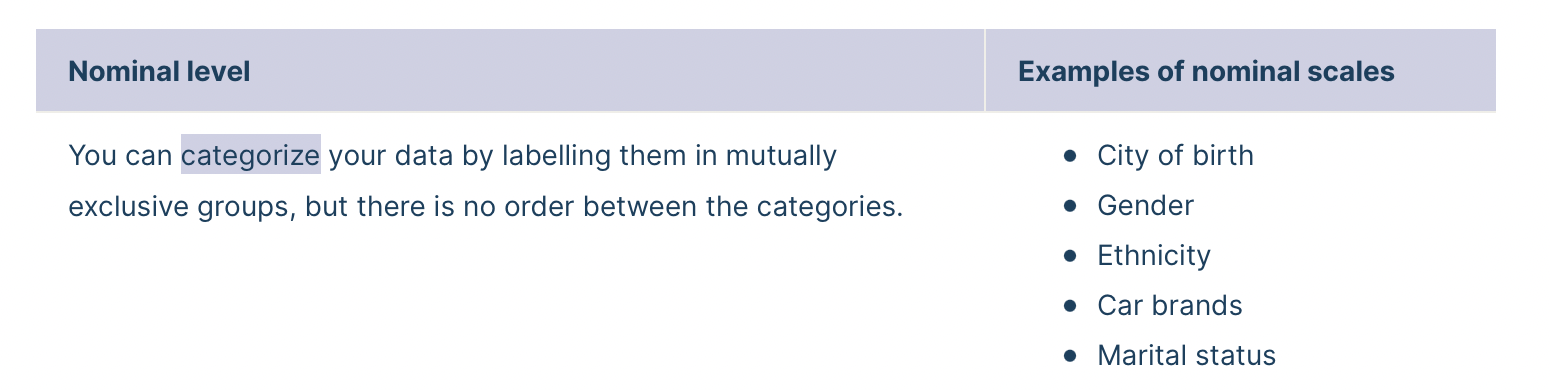
- A variable is nominal if the values could be interchanged (e.g. 1 = male, 2 = female OR 1 = female, 2 = male).
- A variable is ordinal if there is a quantitative ordering of values AND if there are a small number of values (e.g. excellent, good, fair, poor).
- A variable is usually considered interval if it is measured with a composite scale or test.
- A variable is ratio level if it makes sense to say that one value is twice as much as another (e.g. 100 mg is twice as much as 50 mg) (Polit & Beck, 2021).
Reliability and Validity as Applied to Critical Appraisal of Research
Reliability measures the ability of a measure to consistently measure the same way. Validity measures what it is supposed to measure. Do we have the need for both in research? Yes! If a variable is measured inaccurately, the data is useless. Let’s talk about why.
For example, let’s set out to measure blood glucose for our study. The validity is how well the measure can determine the blood glucose. If we used a blood pressure cuff to measure blood glucose, this would not be a valid measure. If we used a blood glucose meter, it would be a more valid measure. It does not stop there, however. What about the meter itself? Has it been calibrated? Are the correct sticks for the meter available? Are they expired? Does the meter have fresh batteries? Are the patient’s hands clean?
Reliability wants to know: Is the blood glucose meter measuring the same way, every time?
Validity is asking, “Does the meter measure what it is supposed to measure?” Construct validity: Does the test measure the concept that it’s intended to measure? Content validity: Is the test fully representative of what it aims to measure? Face validity: Does the content of the test appear to be suitable to its aims?
Leibold, 2020
Obtaining Samples for Population Generalizability
In quantitative research, a population is the entire group that the researcher wants to draw conclusions about.
A sample is the specific group that the researcher will actually collect data from. A sample is always a much smaller group of people than the total size of the population. For example, if we wanted to investigate heart failure, there would be no possible way to measure every single human with heart failure. Therefore, researchers will attempt to select a sample of that large population which would most likely reflect (AKA: be a representative sample) the larger population of those with heart failure. Remember, in quantitative research, the results should be generalizable to the population studied.
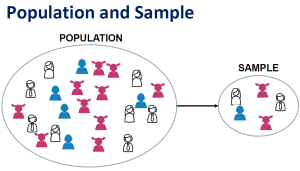
A researcher will specify population characteristics through eligibility criteria. This means that they consider which characteristics to include ( inclusion criteria ) and which characteristics to exclude ( exclusion criteria ).
For example, if we were studying chemotherapy in breast cancer subjects, we might specify:
- Inclusion Criteria: Postmenopausal women between the ages of 45 and 75 who have been diagnosed with Stage II breast cancer.
- Exclusion Criteria: Abnormal renal function tests since we are studying a combination of drugs that may be nephrotoxic. Renal function tests are to be performed to evaluate renal function and the threshold values that would disqualify the prospective subject is serum creatinine above 1.9 mg/dl.
Sampling Designs:
There are two broad classes of sampling in quantitative research: Probability and nonprobability sampling.
Probability sampling : As the name implies, probability sampling means that each eligible individual has a random chance (same probability) of being selected to participate in the study.
There are three types of probability sampling:
Simple random sampling : Every eligible participant is randomly selected (e.g. drawing from a hat).
Stratified random sampling : Eligible population is first divided into two or more strata (categories) from which randomization occurs (e.g. pollution levels selected from restaurants, bars with ordinances of state laws, and bars with no ordinances).
Systematic sampling : Involves the selection of every __ th eligible participant from a list (e.g. every 9 th person).
Nonprobability sampling : In nonprobability sampling, eligible participants are selected using a subjective (non-random) method.
There are four types of nonprobability sampling:
Convenience sampling : Participants are selected for inclusion in the sample because they are the easiest for the researcher to access. This can be due to geographical proximity, availability at a given time, or willingness to participate in the research.
Quota sampling : Participants are from a very tailored sample that’s in proportion to some characteristic or trait of a population. For example, the researcher could divide a population by the state they live in, income or education level, or sex. The population is divided into groups (also called strata) and samples are taken from each group to meet a quota.
Consecutive sampling : A sampling technique in which every subject meeting the criteria of inclusion is selected until the required sample size is achieved. Consecutive sampling is defined as a nonprobability technique where samples are picked at the ease of a researcher more like convenience sampling, only with a slight variation. Here, the researcher selects a sample or group of people, conducts research over a period, collects results, and then moves on to another sample.
Purposive sampling : A group of non-probability sampling techniques in which units are selected because they have characteristics that the researcher needs in their sample. In other words, units are selected “on purpose” in purposive sampling.
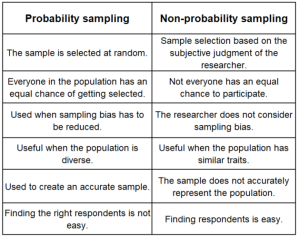
Common Data Collection Methods in Quantitative Research
There are various methods that researchers use to collect data for their studies. For nurse researchers, existing records are an important data source. Researchers need to decide if they will collect new data or use existing data. There is also a wealth of clinical data that can be used for non-research purposed to help answer clinical questions.
Let’s look at some general data collection methods and data sources in quantitative research.
Existing data could include medical records, school records, corporate diaries, letters, meeting minutes, and photographs. These are easy to obtain do not require participation from those being studied.
Collecting new data:
Let’s go over a few methods in which researcher can collect new data. These usually requires participation from those being studied.
Self-reports can be obtained via interviews or questionnaires . Closed-ended questions can be asked (“Within the past 6 months, were you ever a member of a fitness gym?” Yes/No) or open-ended questions such as “Why did you decide to join a fitness gym?” Important to remember (this sometimes throws students off) is that conducting interviews and questionnaires does not mean it is qualitative in nature! Do not let that throw you off in assessing whether a published article is quantitative or qualitative. The nature of the questions, however, may help to determine the type of research (quantitative or qualitative), as qualitative questions deal with ascertaining a very organic collection of people’s experiences in open-ended questions.
Advantages of questionnaires (compared to interviews):
- Questionnaires are less costly and are advantageous for geographically dispersed samples.
- Questionnaires offer the possibility of anonymity, which may be crucial in obtaining information about certain opinions or traits.
Advances of interviews (compared to questionnaires):
- Higher response rates
- Some people cannot fill out a questionnaire.
- Opportunities to clarify questions or to determine comprehension
- Opportunity to collect supplementary data through observation
Psychosocial scales are often utilized within questionnaires or interviews. These can help to obtain attitudes, perceptions, and psychological traits.
Likert Scales :
- Consist of several declarative statements ( items ) expressing viewpoints
- Responses are on an agree/disagree continuum (usually five or seven response options).
- Responses to items are summed to compute a total scale score.
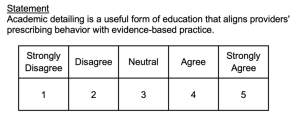
Visual Analog Scale:
- Used to measure subjective experiences (e.g., pain, nausea)
- Measurements are on a straight line measuring 100 mm.
- End points labeled as extreme limits of sensation
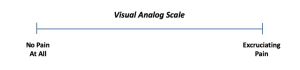
Observational Methods include the observation method of data collection involves seeing people in a certain setting or place at a specific time and day. Essentially, researchers study the behavior of the individuals or surroundings in which they are analyzing. This can be controlled, spontaneous, or participant-based research .
When a researcher utilizes a defined procedure for observing individuals or the environment, this is known as structured observation. When individuals are observed in their natural environment, this is known as naturalistic observation. In participant observation, the researcher immerses himself or herself in the environment and becomes a member of the group being observed.
Biophysiologic Measures are defined as ‘those physiological and physical variables that require specialized technical instruments and equipment for their measurement’. Biophysiological measures are the most common instruments for collecting data in medical science studies. To collect valid and reliable data, it is critical to apply these measures appropriately.
- In vivo refers to when research or work is done with or within an entire, living organism. Examples can include studies in animal models or human clinical trials.
- In vitro is used to describe work that’s performed outside of a living organism. This usually involves isolated tissues, organs, or cells.
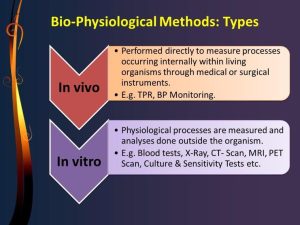
Let’s watch a video about Sampling and Data Collection that I made a couple of years ago.

Home » Sampling Methods – Types, Techniques and Examples
Sampling Methods – Types, Techniques and Examples
Table of Contents

Sampling refers to the process of selecting a subset of data from a larger population or dataset in order to analyze or make inferences about the whole population.
In other words, sampling involves taking a representative sample of data from a larger group or dataset in order to gain insights or draw conclusions about the entire group.
Sampling Methods
Sampling methods refer to the techniques used to select a subset of individuals or units from a larger population for the purpose of conducting statistical analysis or research.
Sampling is an essential part of the Research because it allows researchers to draw conclusions about a population without having to collect data from every member of that population, which can be time-consuming, expensive, or even impossible.
Types of Sampling Methods
Sampling can be broadly categorized into two main categories:
Probability Sampling
This type of sampling is based on the principles of random selection, and it involves selecting samples in a way that every member of the population has an equal chance of being included in the sample.. Probability sampling is commonly used in scientific research and statistical analysis, as it provides a representative sample that can be generalized to the larger population.
Type of Probability Sampling :
- Simple Random Sampling: In this method, every member of the population has an equal chance of being selected for the sample. This can be done using a random number generator or by drawing names out of a hat, for example.
- Systematic Sampling: In this method, the population is first divided into a list or sequence, and then every nth member is selected for the sample. For example, if every 10th person is selected from a list of 100 people, the sample would include 10 people.
- Stratified Sampling: In this method, the population is divided into subgroups or strata based on certain characteristics, and then a random sample is taken from each stratum. This is often used to ensure that the sample is representative of the population as a whole.
- Cluster Sampling: In this method, the population is divided into clusters or groups, and then a random sample of clusters is selected. Then, all members of the selected clusters are included in the sample.
- Multi-Stage Sampling : This method combines two or more sampling techniques. For example, a researcher may use stratified sampling to select clusters, and then use simple random sampling to select members within each cluster.
Non-probability Sampling
This type of sampling does not rely on random selection, and it involves selecting samples in a way that does not give every member of the population an equal chance of being included in the sample. Non-probability sampling is often used in qualitative research, where the aim is not to generalize findings to a larger population, but to gain an in-depth understanding of a particular phenomenon or group. Non-probability sampling methods can be quicker and more cost-effective than probability sampling methods, but they may also be subject to bias and may not be representative of the larger population.
Types of Non-probability Sampling :
- Convenience Sampling: In this method, participants are chosen based on their availability or willingness to participate. This method is easy and convenient but may not be representative of the population.
- Purposive Sampling: In this method, participants are selected based on specific criteria, such as their expertise or knowledge on a particular topic. This method is often used in qualitative research, but may not be representative of the population.
- Snowball Sampling: In this method, participants are recruited through referrals from other participants. This method is often used when the population is hard to reach, but may not be representative of the population.
- Quota Sampling: In this method, a predetermined number of participants are selected based on specific criteria, such as age or gender. This method is often used in market research, but may not be representative of the population.
- Volunteer Sampling: In this method, participants volunteer to participate in the study. This method is often used in research where participants are motivated by personal interest or altruism, but may not be representative of the population.
Applications of Sampling Methods
Applications of Sampling Methods from different fields:
- Psychology : Sampling methods are used in psychology research to study various aspects of human behavior and mental processes. For example, researchers may use stratified sampling to select a sample of participants that is representative of the population based on factors such as age, gender, and ethnicity. Random sampling may also be used to select participants for experimental studies.
- Sociology : Sampling methods are commonly used in sociological research to study social phenomena and relationships between individuals and groups. For example, researchers may use cluster sampling to select a sample of neighborhoods to study the effects of economic inequality on health outcomes. Stratified sampling may also be used to select a sample of participants that is representative of the population based on factors such as income, education, and occupation.
- Social sciences: Sampling methods are commonly used in social sciences to study human behavior and attitudes. For example, researchers may use stratified sampling to select a sample of participants that is representative of the population based on factors such as age, gender, and income.
- Marketing : Sampling methods are used in marketing research to collect data on consumer preferences, behavior, and attitudes. For example, researchers may use random sampling to select a sample of consumers to participate in a survey about a new product.
- Healthcare : Sampling methods are used in healthcare research to study the prevalence of diseases and risk factors, and to evaluate interventions. For example, researchers may use cluster sampling to select a sample of health clinics to participate in a study of the effectiveness of a new treatment.
- Environmental science: Sampling methods are used in environmental science to collect data on environmental variables such as water quality, air pollution, and soil composition. For example, researchers may use systematic sampling to collect soil samples at regular intervals across a field.
- Education : Sampling methods are used in education research to study student learning and achievement. For example, researchers may use stratified sampling to select a sample of schools that is representative of the population based on factors such as demographics and academic performance.
Examples of Sampling Methods
Probability Sampling Methods Examples:
- Simple random sampling Example : A researcher randomly selects participants from the population using a random number generator or drawing names from a hat.
- Stratified random sampling Example : A researcher divides the population into subgroups (strata) based on a characteristic of interest (e.g. age or income) and then randomly selects participants from each subgroup.
- Systematic sampling Example : A researcher selects participants at regular intervals from a list of the population.
Non-probability Sampling Methods Examples:
- Convenience sampling Example: A researcher selects participants who are conveniently available, such as students in a particular class or visitors to a shopping mall.
- Purposive sampling Example : A researcher selects participants who meet specific criteria, such as individuals who have been diagnosed with a particular medical condition.
- Snowball sampling Example : A researcher selects participants who are referred to them by other participants, such as friends or acquaintances.
How to Conduct Sampling Methods
some general steps to conduct sampling methods:
- Define the population: Identify the population of interest and clearly define its boundaries.
- Choose the sampling method: Select an appropriate sampling method based on the research question, characteristics of the population, and available resources.
- Determine the sample size: Determine the desired sample size based on statistical considerations such as margin of error, confidence level, or power analysis.
- Create a sampling frame: Develop a list of all individuals or elements in the population from which the sample will be drawn. The sampling frame should be comprehensive, accurate, and up-to-date.
- Select the sample: Use the chosen sampling method to select the sample from the sampling frame. The sample should be selected randomly, or if using a non-random method, every effort should be made to minimize bias and ensure that the sample is representative of the population.
- Collect data: Once the sample has been selected, collect data from each member of the sample using appropriate research methods (e.g., surveys, interviews, observations).
- Analyze the data: Analyze the data collected from the sample to draw conclusions about the population of interest.
When to use Sampling Methods
Sampling methods are used in research when it is not feasible or practical to study the entire population of interest. Sampling allows researchers to study a smaller group of individuals, known as a sample, and use the findings from the sample to make inferences about the larger population.
Sampling methods are particularly useful when:
- The population of interest is too large to study in its entirety.
- The cost and time required to study the entire population are prohibitive.
- The population is geographically dispersed or difficult to access.
- The research question requires specialized or hard-to-find individuals.
- The data collected is quantitative and statistical analyses are used to draw conclusions.
Purpose of Sampling Methods
The main purpose of sampling methods in research is to obtain a representative sample of individuals or elements from a larger population of interest, in order to make inferences about the population as a whole. By studying a smaller group of individuals, known as a sample, researchers can gather information about the population that would be difficult or impossible to obtain from studying the entire population.
Sampling methods allow researchers to:
- Study a smaller, more manageable group of individuals, which is typically less time-consuming and less expensive than studying the entire population.
- Reduce the potential for data collection errors and improve the accuracy of the results by minimizing sampling bias.
- Make inferences about the larger population with a certain degree of confidence, using statistical analyses of the data collected from the sample.
- Improve the generalizability and external validity of the findings by ensuring that the sample is representative of the population of interest.
Characteristics of Sampling Methods
Here are some characteristics of sampling methods:
- Randomness : Probability sampling methods are based on random selection, meaning that every member of the population has an equal chance of being selected. This helps to minimize bias and ensure that the sample is representative of the population.
- Representativeness : The goal of sampling is to obtain a sample that is representative of the larger population of interest. This means that the sample should reflect the characteristics of the population in terms of key demographic, behavioral, or other relevant variables.
- Size : The size of the sample should be large enough to provide sufficient statistical power for the research question at hand. The sample size should also be appropriate for the chosen sampling method and the level of precision desired.
- Efficiency : Sampling methods should be efficient in terms of time, cost, and resources required. The method chosen should be feasible given the available resources and time constraints.
- Bias : Sampling methods should aim to minimize bias and ensure that the sample is representative of the population of interest. Bias can be introduced through non-random selection or non-response, and can affect the validity and generalizability of the findings.
- Precision : Sampling methods should be precise in terms of providing estimates of the population parameters of interest. Precision is influenced by sample size, sampling method, and level of variability in the population.
- Validity : The validity of the sampling method is important for ensuring that the results obtained from the sample are accurate and can be generalized to the population of interest. Validity can be affected by sampling method, sample size, and the representativeness of the sample.
Advantages of Sampling Methods
Sampling methods have several advantages, including:
- Cost-Effective : Sampling methods are often much cheaper and less time-consuming than studying an entire population. By studying only a small subset of the population, researchers can gather valuable data without incurring the costs associated with studying the entire population.
- Convenience : Sampling methods are often more convenient than studying an entire population. For example, if a researcher wants to study the eating habits of people in a city, it would be very difficult and time-consuming to study every single person in the city. By using sampling methods, the researcher can obtain data from a smaller subset of people, making the study more feasible.
- Accuracy: When done correctly, sampling methods can be very accurate. By using appropriate sampling techniques, researchers can obtain a sample that is representative of the entire population. This allows them to make accurate generalizations about the population as a whole based on the data collected from the sample.
- Time-Saving: Sampling methods can save a lot of time compared to studying the entire population. By studying a smaller sample, researchers can collect data much more quickly than they could if they studied every single person in the population.
- Less Bias : Sampling methods can reduce bias in a study. If a researcher were to study the entire population, it would be very difficult to eliminate all sources of bias. However, by using appropriate sampling techniques, researchers can reduce bias and obtain a sample that is more representative of the entire population.
Limitations of Sampling Methods
- Sampling Error : Sampling error is the difference between the sample statistic and the population parameter. It is the result of selecting a sample rather than the entire population. The larger the sample, the lower the sampling error. However, no matter how large the sample size, there will always be some degree of sampling error.
- Selection Bias: Selection bias occurs when the sample is not representative of the population. This can happen if the sample is not selected randomly or if some groups are underrepresented in the sample. Selection bias can lead to inaccurate conclusions about the population.
- Non-response Bias : Non-response bias occurs when some members of the sample do not respond to the survey or study. This can result in a biased sample if the non-respondents differ from the respondents in important ways.
- Time and Cost : While sampling can be cost-effective, it can still be expensive and time-consuming to select a sample that is representative of the population. Depending on the sampling method used, it may take a long time to obtain a sample that is large enough and representative enough to be useful.
- Limited Information : Sampling can only provide information about the variables that are measured. It may not provide information about other variables that are relevant to the research question but were not measured.
- Generalization : The extent to which the findings from a sample can be generalized to the population depends on the representativeness of the sample. If the sample is not representative of the population, it may not be possible to generalize the findings to the population as a whole.
About the author
Muhammad Hassan
Researcher, Academic Writer, Web developer
You may also like

Probability Sampling – Methods, Types and...

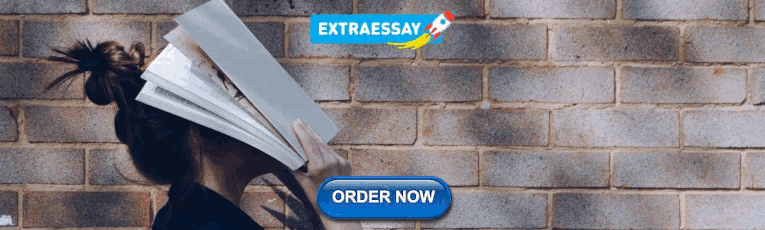
Quota Sampling – Types, Methods and Examples

Simple Random Sampling – Types, Method and...

Convenience Sampling – Method, Types and Examples

Purposive Sampling – Methods, Types and Examples

Systematic Sampling – Types, Method and Examples
If you're seeing this message, it means we're having trouble loading external resources on our website.
If you're behind a web filter, please make sure that the domains *.kastatic.org and *.kasandbox.org are unblocked.
To log in and use all the features of Khan Academy, please enable JavaScript in your browser.
Statistics and probability
Course: statistics and probability > unit 6.
- Picking fairly
- Using probability to make fair decisions
- Techniques for generating a simple random sample
- Simple random samples
- Techniques for random sampling and avoiding bias
- Sampling methods
Sampling methods review
- Samples and surveys
What are sampling methods?
Bad ways to sample.
- (Choice A) Convenience sampling A Convenience sampling
- (Choice B) Voluntary response sampling B Voluntary response sampling
Good ways to sample
- (Choice A) Simple random sampling A Simple random sampling
- (Choice B) Stratified random sampling B Stratified random sampling
- (Choice C) Cluster random sampling C Cluster random sampling
- (Choice D) Systematic random sampling D Systematic random sampling
Want to join the conversation?
- Upvote Button navigates to signup page
- Downvote Button navigates to signup page
- Flag Button navigates to signup page


What are quantitative research sampling methods?
The quantitative research sampling method is the process of selecting representable units from a large population. Quantitative research refers to the analysis wherein mathematical, statistical, or computational method is used for studying the measurable or quantifiable dataset. The core purpose of quantitative research is the generalization of a phenomenon or an opinion. This involves collecting and gathering information from a small group out of a population or universe.
To find out what drives Amazon’s popularity as the most preferred e-commerce company, a small group of Amazon’s customers can be surveyed. It will help arrive at a consensus on the most significant traits that make it successful.
Therefore, an assumption about a population is based on a small or selected dataset. In order to derive accurate results, it is essential to use an appropriate sampling method. The purpose of this article is to review different quantitative sampling methods and their applicability in different types of research.
Quantitative research sampling methods
By examining the nature of the small group, the researcher can deduce the behaviour of the larger population. Quantitative research sampling methods are broadly divided into two categories i.e.
- Probability sampling
- Non-probability sampling

Probability sampling method
In probability sampling, each unit in the population has an equal chance of being selected for the sample. The purpose is to identify those sample sets which majorly represent the characteristics of the population. Herein, all the characteristics of the population are required to be known. This is done through a process known as ‘listing’. This process of listing is called the sampling frame. As probability sampling is a type of random sampling, the generalization is more accurate.
Probability sampling is quite time-consuming and expensive. Hence, this method is only suitable in cases wherein the population are similar in characteristics, and the researcher has time, money, and access to the whole population. Probability sampling is further categorized into 4 types: simple random, systematic, stratified and cluster sampling. The figure below depicts the types of probability sampling.

The difference between and applicability of these sampling methods are depicted in the table below.
Non-probability sampling method
Non-probability-based quantitative research sampling method involves non-random selection of the sample from the entire population. All units of the population do not an equal chance of participating in the survey. Therefore, the results cannot be generalized for the population.
The non-probability technique of sampling is based on the subjective judgement of the researcher. Hence this method can be applied in cases wherein limited information about the population is available. Moreover, it requires less time and money. Non-probability sampling method can be of four types as shown below.

Table 2: Non-probability-based Quantitative research sampling method
The results of the quantitative research are mainly based on the information acquired from the sample. An effective sample yields a representable outcome. To draw valid and reliable conclusions, it is essential to carefully compute the sample size of the study and define the sampling technique of the study.
- McCombes, S. (2019) Understanding different sampling methods . Available at: https://www.scribbr.com/methodology/sampling-methods/ (Accessed: 7 February 2020).
- Click to share on Twitter (Opens in new window)
- Click to share on Facebook (Opens in new window)
- Click to share on LinkedIn (Opens in new window)
- Click to share on WhatsApp (Opens in new window)
- Click to share on Telegram (Opens in new window)
Notify me of follow-up comments by email.
1 thought on “What are quantitative research sampling methods?”
Proofreading.
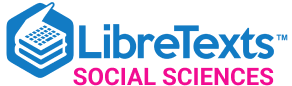
- school Campus Bookshelves
- menu_book Bookshelves
- perm_media Learning Objects
- login Login
- how_to_reg Request Instructor Account
- hub Instructor Commons
- Download Page (PDF)
- Download Full Book (PDF)
- Periodic Table
- Physics Constants
- Scientific Calculator
- Reference & Cite
- Tools expand_more
- Readability
selected template will load here
This action is not available.
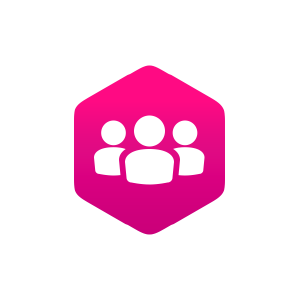
10.3: Sampling in quantitative research
- Last updated
- Save as PDF
- Page ID 25659
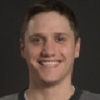
- Matthew DeCarlo
- Radford University via Open Social Work Education
Learning Objectives
- Describe how probability sampling differs from nonprobability sampling
- Define generalizability, and describe how it is achieved in probability samples
- Identify the various types of probability samples, and describe why a researcher may use one type over another
Quantitative researchers are often interested in making generalizations about groups larger than their study samples; they seek nomothetic causal explanations. While there are certainly instances when quantitative researchers rely on nonprobability samples (e.g., when doing exploratory research), quantitative researchers tend to rely on probability sampling techniques. The goals and techniques associated with probability samples differ from those of nonprobability samples. We’ll explore those unique goals and techniques in this section.
Probability sampling
Unlike nonprobability sampling, probability sampling refers to sampling techniques for which a person’s likelihood of being selected from the sampling frame is known. You might ask yourself why we should care about a potential participant’s likelihood of being selected for the researcher’s sample. The reason is that, in most cases, researchers who use probability sampling techniques are aiming to identify a representative sample from which to collect data. A representative sample is one that resembles the population from which it was drawn in all the ways that are important for the research being conducted. If, for example, you wish to be able to say something about differences between men and women at the end of your study, you better make sure that your sample doesn’t contain only women. That’s a bit of an oversimplification, but the point with representativeness is that if your population varies in some way that is important to your study, your sample should contain the same sorts of variation.
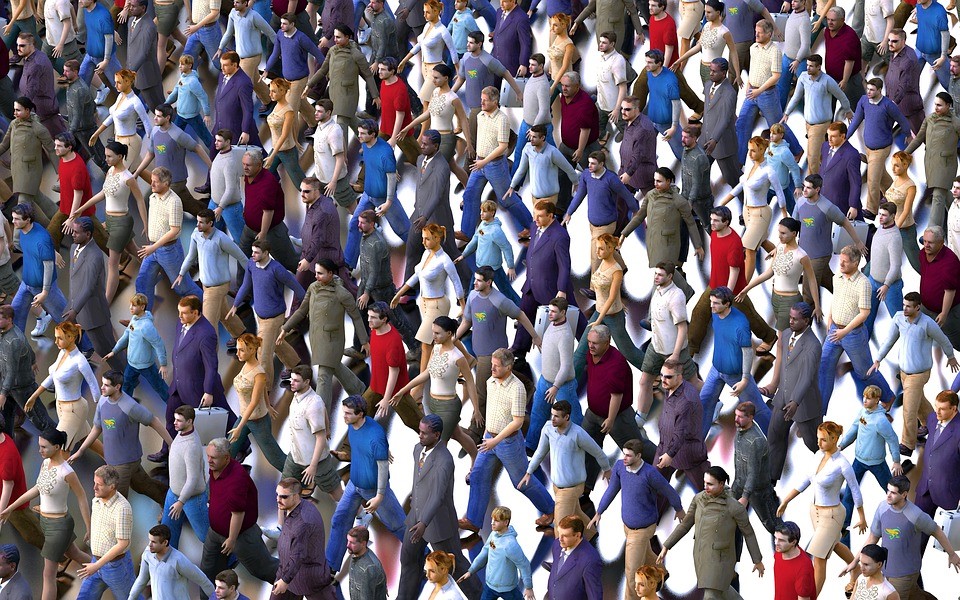
Obtaining a representative sample is important in probability sampling because of generalizability. In fact, generalizability is perhaps the key feature that distinguishes probability samples from nonprobability samples. Generalizability refers to the idea that a study’s results will tell us something about a group larger than the sample from which the findings were generated. In order to achieve generalizability, a core principle of probability sampling is that all elements in the researcher’s sampling frame have an equal chance of being selected for inclusion in the study. In research, this is the principle of random selection . Researchers use a computer’s random number generator to determine who from the sampling frame gets recruited into the sample.
Using random selection does not mean that your sample will be perfect. No sample is perfect. The only way to come with a perfect result would be to include everyone in the population in your sample, which defeats the whole point of sampling. Generalizing from a sample to a population always contains some degree of error. This is referred to as sampling error, a statistical calculation of the difference between results from a sample and the actual parameters of a population.
Generalizability is a pretty easy concept to grasp. Imagine a professor were to take a sample of individuals in your class to see if the material is too hard or too easy. The professor, however, only sampled individuals whose grades were over 90% in the class. Would that be a representative sample of all students in the class? That would be a case of sampling error—a mismatch between the results of the sample and the true feelings of the overall class. In other words, the results of the professor’s study don’t generalize to the overall population of the class.
Taking this one step further, imagine your professor is conducting a study on binge drinking among college students. The professor uses undergraduates at your school as her sampling frame. Even if that professor were to use probability sampling, perhaps your school differs from other schools in important ways. There are schools that are “party schools” where binge drinking may be more socially accepted, “commuter schools” at which there is little nightlife, and so on. If your professor plans to generalize her results to all college students, she will have to make an argument that her sampling frame (undergraduates at your school) is representative of the population (all undergraduate college students).
Types of probability samples
There are a variety of probability samples that researchers may use. These include simple random samples, systematic samples, stratified samples, and cluster samples. Let’s build on the previous example. Imagine we were concerned with binge drinking and chose the target population of fraternity members. How might you go about getting a probability sample of fraternity members that is representative of the overall population?
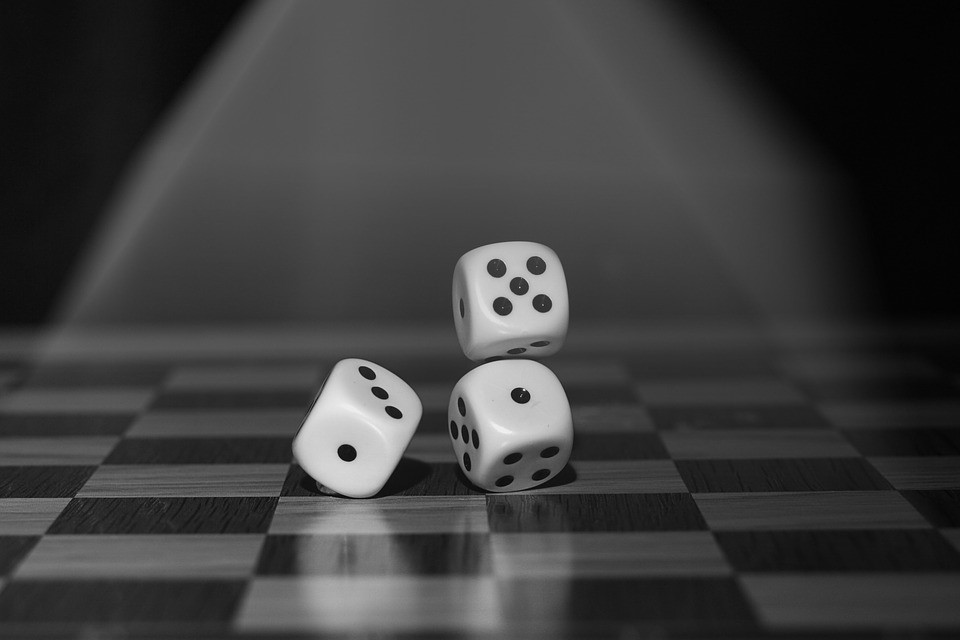
Simple random samples are the most basic type of probability sample. A simple random sample requires a real sampling frame—an actual list of each person in the sampling frame. Your school likely has a list of all of the fraternity members on campus, as Greek life is subject to university oversight. You could use this as your sampling frame. Using the university’s list, you would number each fraternity member, or element , sequentially and then randomly select the elements from which you will collect data.
True randomness is difficult to achieve, and it takes complex computational calculations to do so. Although you think you can select things at random, human-generated randomness is actually quite predictable, as it falls into patterns called heuristics. To truly randomly select elements, researchers must rely on computer-generated help. Many free websites have good pseudo-random number generators. A good example is the website Random.org, which contains a random number generator that can also randomize lists of participants. Sometimes, researchers use a table of numbers that have been generated randomly. There are several possible sources for obtaining a random number table. Some statistics and research methods textbooks offer such tables as appendices to the text.
As you might have guessed, drawing a simple random sample can be quite tedious. Systematic sampling techniques are somewhat less tedious but offer the benefits of a random sample. As with simple random samples, you must possess a list of everyone in your sampling frame. Once you’ve done that, to draw a systematic sample you’d simply select every kth element on your list. But what is k, and where on the list of population elements does one begin the selection process? k is your selection interval or the distance between the elements you select for inclusion in your study. To begin the selection process, you’ll need to figure out how many elements you wish to include in your sample. Let’s say you want to interview 25 fraternity members on your campus, and there are 100 men on campus who are members of fraternities. In this case, your selection interval, or k, is 4. To arrive at 4, simply divide the total number of population elements by your desired sample size. This process is represented in Figure 10.2.
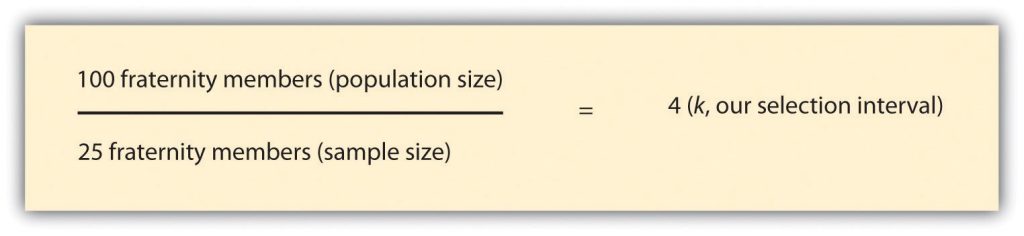
To determine where on your list of population elements to begin selecting the names of the 25 men you will interview, select a number between 1 and k, and begin there. If we select 3 as our starting point, we’d begin by selecting the third fraternity member on the list and then select every fourth member from there. This might be easier to understand if you can see it visually. Table 10.2 lists the names of our hypothetical 100 fraternity members on campus. You’ll see that the third name on the list has been selected for inclusion in our hypothetical study, as has every fourth name after that. A total of 25 names have been selected.
There is one clear instance in which systematic sampling should not be employed. If your sampling frame has any pattern to it, you could inadvertently introduce bias into your sample by using a systemic sampling strategy. (Bias will be discussed in more depth in the next section.) This is sometimes referred to as the problem of periodicity. Periodicity refers to the tendency for a pattern to occur at regular intervals. Let’s say, for example, that you wanted to observe binge drinking on campus each day of the week. Perhaps you need to have your observations completed within 28 days and you wish to conduct four observations on randomly chosen days. Table 10.3 shows a list of the population elements for this example. To determine which days we’ll conduct our observations, we’ll need to determine our selection interval. As you’ll recall from the preceding paragraphs, to do so we must divide our population size, in this case 28 days, by our desired sample size, in this case 4 days. This formula leads us to a selection interval of 7. If we randomly select 2 as our starting point and select every seventh day after that, we’ll wind up with a total of 4 days on which to conduct our observations. You’ll see how that works out in the following table.
Do you notice any problems with our selection of observation days in Table 1? Apparently, we’ll only be observing on Tuesdays. Moreover, Tuesdays may not be an ideal day to observe binge drinking behavior. Unless alcohol consumption patterns have changed significantly since I was in my undergraduate program, I would assume binge drinking is more likely to happen over the weekend.
In cases such as this, where the sampling frame is cyclical, it would be better to use a stratified sampling technique . In stratified sampling, a researcher will divide the study population into relevant subgroups and then draw a sample from each subgroup. In this example, we might wish to first divide our sampling frame into two lists: weekend days and weekdays. Once we have our two lists, we can then apply either simple random or systematic sampling techniques to each subgroup.
Stratified sampling is a good technique to use when, as in our example, a subgroup of interest makes up a relatively small proportion of the overall sample. In our example of a study of binge drinking, we want to include weekdays and weekends in our sample, but because weekends make up less than a third of an entire week, there’s a chance that a simple random or systematic strategy would not yield sufficient weekend observation days. As you might imagine, stratified sampling is even more useful in cases where a subgroup makes up an even smaller proportion of the sampling frame—for example, if we want to be sure to include in our study students who are in year five of their undergraduate program but this subgroup makes up only a small percentage of the population of undergraduates. There’s a chance simple random or systematic sampling strategy might not yield any fifth-year students, but by using stratified sampling, we could ensure that our sample contained the proportion of fifth-year students that is reflective of the larger population.
In this case, class year (e.g., freshman, sophomore, junior, senior, and fifth-year) is our strata , or the characteristic by which the sample is divided. In using stratified sampling, we are often concerned with how well our sample reflects the population. A sample with too many freshmen may skew our results in one direction because perhaps they binge drink more (or less) than students in other class years. Using stratified sampling allows us to make sure our sample has the same proportion of people from each class year as the overall population of the school.
Up to this point in our discussion of probability samples, we’ve assumed that researchers will be able to access a list of population elements in order to create a sampling frame. This, as you might imagine, is not always the case. Let’s say, for example, that you wish to conduct a study of binge drinking across fraternity members at each undergraduate program in your state. Just imagine trying to create a list of every single fraternity member in the state. Even if you could find a way to generate such a list, attempting to do so might not be the most practical use of your time or resources. When this is the case, researchers turn to cluster sampling. Cluster sampling occurs when a researcher begins by sampling groups (or clusters) of population elements and then selects elements from within those groups.
Let’s work through how we might use cluster sampling in our study of binge drinking. While creating a list of all fraternity members in your state would be next to impossible, you could easily create a list of all undergraduate colleges in your state. Thus, you could draw a random sample of undergraduate colleges (your cluster) and then draw another random sample of elements (in this case, fraternity members) from within the undergraduate college you initially selected. Cluster sampling works in stages. In this example, we sampled in two stages— (1) undergraduate colleges and (2) fraternity members at the undergraduate colleges we selected. However, we could add another stage if it made sense to do so. We could randomly select (1) undergraduate colleges (2) specific fraternities at each school and (3) individual fraternity members. As you might have guessed, sampling in multiple stages does introduce the possibility of greater error (each stage is subject to its own sampling error), but it is nevertheless a highly efficient method.
Jessica Holt and Wayne Gillespie (2008) [2] used cluster sampling in their study of students’ experiences with violence in intimate relationships. Specifically, the researchers randomly selected 14 classes on their campus and then drew a random subsample of students from those classes. But you probably know from your experience with college classes that not all classes are the same size. So, if Holt and Gillespie had simply randomly selected 14 classes and then selected the same number of students from each class to complete their survey, then students in the smaller of those classes would have had a greater chance of being selected for the study than students in the larger classes. Keep in mind, with random sampling the goal is to make sure that each element has the same chance of being selected. When clusters are of different sizes, as in the example of sampling college classes, researchers often use a method called probability proportionate to size (PPS). This means that they take into account that their clusters are of different sizes. They do this by giving clusters different chances of being selected based on their size so that each element within those clusters winds up having an equal chance of being selected.
To summarize, probability samples allow a researcher to make conclusions about larger groups. Probability samples require a sampling frame from which elements, usually human beings, can be selected at random from a list. The use of random selection reduces the error and bias present in nonprobability samples reviewed in the previous section, though some error will always remain. In relying on a random number table or generator, researchers can more accurately state that their sample represents the population from which it was drawn. This strength is common to all probability sampling approaches summarized in Table 10.4.
In determining which probability sampling approach makes the most sense for your project, it helps to know more about your population. A simple random sample and systematic sample are relatively similar to carry out. They both require a list all elements in your sampling frame. Systematic sampling is slightly easier in that it does not require you to use a random number generator, instead using a sampling interval that is easy to calculate by hand.
The relative simplicity of both approaches is counterweighted by their lack of sensitivity to characteristics in of your population. Stratified samples can better account for periodicity by creating strata that reduce or eliminate the effects of periodicity. Stratified samples also ensure that smaller subgroups are included in your sample, thus making your sample more representative of the overall population. While these benefits are important, creating strata for this purpose requires knowing information about your population before beginning the sampling process. In our binge drinking example, we would need to know how many students are in each class year to make sure our sample contained the same proportions. We would need to know that, for example, fifth-year students make up 5% of the student population to make sure 5% of our sample is comprised of fifth-year students. If the true population parameters are unknown, stratified sampling becomes significantly more challenging.
Common to each of the previous probability sampling approaches is the necessity of using a real list of all elements in your sampling frame. Cluster sampling is different. It allows a researcher to perform probability sampling in cases for which a list of elements is not available or pragmatic to create. Cluster sampling is also useful for making claims about a larger population, in our example, all fraternity members within a state. However, because sampling occurs at multiple stages in the process, in our example at the university and student level, sampling error increases. For many researchers, this weakness is outweighed by the benefits of cluster sampling.
Key Takeaways
- In probability sampling, the aim is to identify a sample that resembles the population from which it was drawn.
- There are several types of probability samples including simple random samples, systematic samples, stratified samples, and cluster samples.
- Probability samples usually require a real list of elements in your sampling frame, though cluster sampling can be conducted without one.
- Cluster sampling- a sampling approach that begins by sampling groups (or clusters) of population elements and then selects elements from within those groups
- Generalizability – the idea that a study’s results will tell us something about a group larger than the sample from which the findings were generated
- Periodicity- the tendency for a pattern to occur at regular intervals
- Probability proportionate to size- in cluster sampling, giving clusters different chances of being selected based on their size so that each element within those clusters has an equal chance of being selected
- Probability sampling- sampling approaches for which a person’s likelihood of being selected from the sampling frame is known
- Random selection- using a randomly generated numbers to determine who from the sampling frame gets recruited into the sample
- Representative sample- a sample that resembles the population from which it was drawn in all the ways that are important for the research being conducted
- Sampling error- a statistical calculation of the difference between results from a sample and the actual parameters of a population
- Simple random sampling- selecting elements from a list using randomly generated numbers
- Strata- the characteristic by which the sample is divided
- Stratified sampling- dividing the study population into relevant subgroups and then draw a sample from each subgroup
- Systematic sampling- selecting every kth element from a list
Image attributions
crowd men women by DasWortgewand CC-0
roll the dice by 955169 CC-0
- Figure 10.2 copied from Blackstone, A. (2012) Principles of sociological inquiry: Qualitative and quantitative methods. Saylor Foundation. Retrieved from: https://saylordotorg.github.io/text_...ative-methods/ Shared under CC-BY-NC-SA 3.0 License ( https://creativecommons.org/licenses/by-nc-sa/3.0/ ) ↵
- Holt, J. L., & Gillespie, W. (2008). Intergenerational transmission of violence, threatened egoism, and reciprocity: A test of multiple psychosocial factors affecting intimate partner violence. American Journal of Criminal Justice, 33 , 252–266. ↵
- (855) 776-7763
Training Maker
All Products
Qualaroo Insights
ProProfs.com
- Sign Up Free
Do you want a free Survey Software?
We have the #1 Online Survey Maker Software to get actionable user insights.
A Comprehensive Guide to Quantitative Research: Types, Characteristics, Methods & Examples
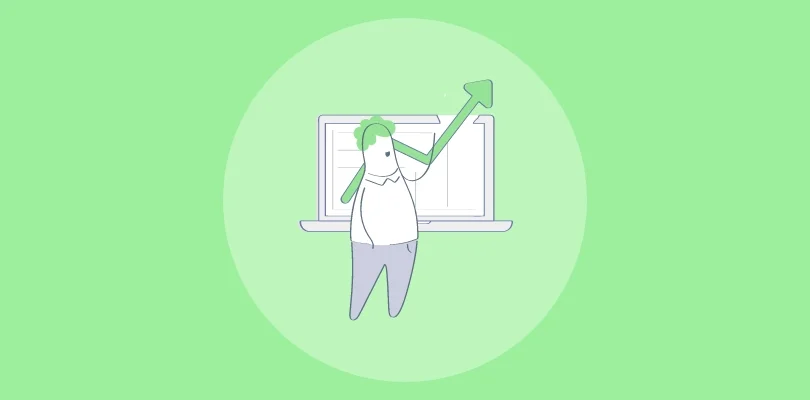
Step into the fascinating world of quantitative research, where numbers reveal extraordinary insights!
By gathering and studying data in a systematic way, quantitative research empowers us to understand our ever-changing world better. It helps understand a problem or an already-formed hypothesis by generating numerical data. The results don’t end here, as you can process these numbers to get actionable insights that aid decision-making.
You can use quantitative research to quantify opinions, behaviors, attitudes, and other definitive variables related to the market, customers, competitors, etc. The research is conducted on a larger sample population to draw predictive, average, and pattern-based insights.
Here, we delve into the intricacies of this research methodology, exploring various quantitative methods, their advantages, and real-life examples that showcase their impact and relevance.
Ready to embark on a journey of discovery and knowledge? Let’s go!
What Is Quantitative Research?
Quantitative research is a method that uses numbers and statistics to test theories about customer attitudes and behaviors. It helps researchers gather and analyze data systematically to gain valuable insights and draw evidence-based conclusions about customer preferences and trends.
Researchers use online surveys , questionnaires , polls , and quizzes to question a large number of people to obtain measurable and bias-free data.
In technical terms, quantitative research is mainly concerned with discovering facts about social phenomena while assuming a fixed and measurable reality.
Offering numbers and stats-based insights, this research methodology is a crucial part of primary research and helps understand how well an organizational decision is going to work out.
Let’s consider an example.
Suppose your qualitative analysis shows that your customers are looking for social media-based customer support . In that case, quantitative analysis will help you see how many of your customers are looking for this support.
If 10% of your customers are looking for such a service, you might or might not consider offering this feature. But, if 40% of your regular customers are seeking support via social media, then it is something you just cannot overlook.
Characteristics of Quantitative Research
Quantitative research clarifies the fuzziness of research data from qualitative research analysis. With numerical insights, you can formulate a better and more profitable business decision.
Hence, quantitative research is more readily contestable, sharpens intelligent discussion, helps you see the rival hypotheses, and dynamically contributes to the research process.
Let us have a quick look at some of its characteristics.
- Measurable Variables
The data collection methods in quantitative research are structured and contain items requiring measurable variables, such as age, number of family members, salary range, highest education, etc.
These structured data collection methods comprise polls, surveys, questionnaires, etc., and may have questions like the ones shown in the following image:
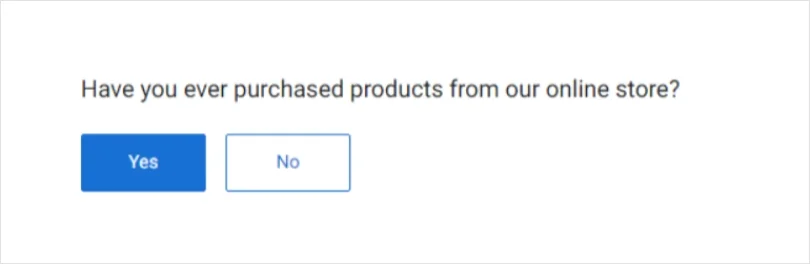
As you can see, all the variables are measurable. This ensures that the research is in-depth and provides less erroneous data for reliable, actionable insights.
- Sample Size
No matter what data analysis methods for quantitative research are being used, the sample size is kept such that it represents the target market.
As the main aim of the research methodology is to get numerical insights, the sample size should be fairly large. Depending on the survey objective and scope, it might span hundreds of thousands of people.
- Normal Population Distribution
To maintain the reliability of a quantitative research methodology, we assume that the population distribution curve is normal.
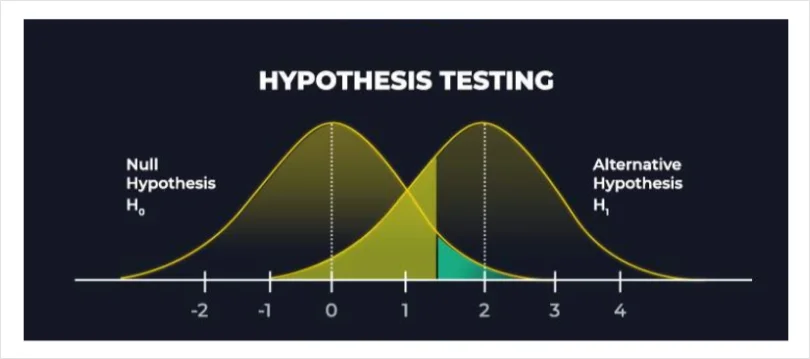
This type of population distribution curve is preferred over a non-normal distribution as the sample size is large, and the characteristics of the sample vary with its size.
This requires adhering to the random sampling principle to avoid the researcher’s bias in result interpretation. Any bias can ruin the fairness of the entire process and defeats the purpose of research.
- Well-Structured Data Representation
Data analysis in quantitative research produces highly structured results and can form well-defined graphical representations. Some common examples include tables, figures, graphs, etc., that combine large blocks of data.
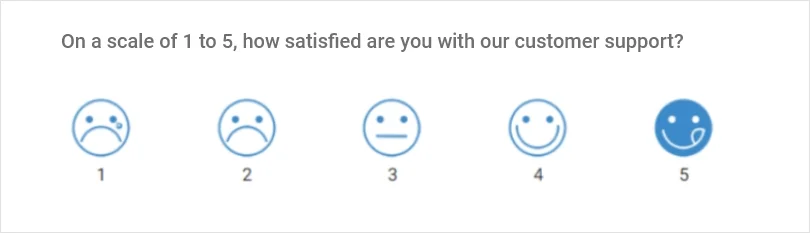
This way, you can discover hidden data trends, relationships, and differences among various measurable variables. This can help researchers understand the survey data and formulate actionable insights for decision-making.
- Predictive Outcomes
Quantitative analysis of data can also be used for estimations and prediction outcomes. You can construct if-then scenarios and analyze the data for the identification of any upcoming trends or events.
However, this requires advanced analytics and involves complex mathematical computations. So, it is mostly done via quantitative research tools that come with advanced analytics capabilities.
8 Best Practices to Conduct Quantitative Research
Here are some best practices to keep in mind while conducting quantitative research:
1. Define Research Objectives
There can be many ways to collect data via quantitative research methods that are chosen as per the research objective and scope. These methods allow you to build your own observations regarding any hypotheses – unknown, entirely new, or unexplained.
You can hypothesize a proof and build a prediction of outcomes supporting the same. You can also create a detailed stepwise plan for data collection, analysis, and testing.
Below, we explore quantitative research methods and discuss some examples to enhance your understanding of them.
2. Keep Your Questions Simple
The surveys are meant to reach people en-masse, and that includes a wide demographic range with recipients from all walks of life. Asking simple questions will ensure that they grasp what’s being asked easily.
Read More: Proven Tips to Avoid Leading and Loaded Questions in Your Survey
3. Develop a Solid Research Design
Choose an appropriate research design that aligns with your objectives, whether it’s experimental, quasi-experimental, or correlational. You also need to pay attention to the sample size and sampling technique such that it represents the target population accurately.
4. Use Reliable & Valid Instruments
It’s crucial to select or develop measurement instruments such as questionnaires, scales, or tests that have been validated and are reliable. Before proceeding with the main study, pilot-test these instruments on a small sample to assess their effectiveness and make any necessary improvements.
5. Ensure Data Quality
Implement data collection protocols to minimize errors and bias during data gathering. Double-check data entries and cleaning procedures to eliminate any inconsistencies or missing values that may affect the accuracy of your results. For instance, you might regularly cross-verify data entries to identify and correct any discrepancies.
6. Employ Appropriate Data Analysis Techniques
Select statistical methods that match the nature of your data and research questions. Whether it’s regression analysis, t-tests, ANOVA, or other techniques, using the right approach is important for drawing meaningful conclusions. Utilize software tools like SPSS or R for data analysis to ensure the accuracy and reproducibility of your findings.
7. Interpret Results Objectively
Present your findings in a clear and unbiased manner. Avoid making unwarranted causal claims, especially in correlational studies. Instead, focus on describing the relationships and patterns observed in your data.
8. Address Ethical Considerations
Prioritize ethical considerations throughout your research process. Obtain informed consent from participants, ensuring their voluntary participation and confidentiality of data. Comply with ethical guidelines and gain approval from a governing body if necessary.
Read More: How to Find Survey Participants & Respondents
Types of Quantitative Research Methods
Quantitative research is usually conducted using two methods. They are-
- Primary quantitative research methods
- Secondary quantitative research methods
1. Primary Methods
Primary quantitative research is the most popular way of conducting market research. The differentiating factor of this method is that the researcher relies on collecting data firsthand instead of relying on data collected from previous research.
There are multiple types of primary quantitative research. They can be distinguished based on three distinctive aspects, which are:
A. Techniques & Types of Studies:
- Survey Research
Surveys are the easiest, most common, and one of the most sought-after quantitative research techniques. The main aim of a survey is to widely gather and describe the characteristics of a target population or customers. Surveys are the foremost quantitative method preferred by both small and large organizations.
They help them understand their customers, products, and other brand offerings in a proper manner.
Surveys can be conducted using various methods, such as online polls, web-based surveys, paper questionnaires, phone calls, or face-to-face interviews. Survey research allows organizations to understand customer opinions, preferences, and behavior, making it crucial for market research and decision-making.
You can watch this quick video to learn more about creating surveys.
Surveys are of two types:
- Cross-Sectional Surveys Cross-sectional surveys are used to collect data from a sample of the target population at a specific point in time. Researchers evaluate various variables simultaneously to understand the relationships and patterns within the data.
- Cross-sectional surveys are popular in retail, small and medium-sized enterprises (SMEs), and healthcare industries, where they assess customer satisfaction, market trends, and product feedback.
- Longitudinal Surveys Longitudinal surveys are conducted over an extended period, observing changes in respondent behavior and thought processes.
- Researchers gather data from the same sample multiple times, enabling them to study trends and developments over time. These surveys are valuable in fields such as medicine, applied sciences, and market trend analysis.
Surveys can be distributed via various channels. Some of the most popular ones are listed below:
- Email: Sending surveys via email is a popular and effective method. People recognize your brand, leading to a higher response rate. With ProProfs Survey Maker’s in-mail survey-filling feature, you can easily send out and collect survey responses.
- Embed on a website: Boost your response rate by embedding the survey on your website. When visitors are already engaged with your brand, they are more likely to take the survey.
- Social media: Take advantage of social media platforms to distribute your survey. People familiar with your brand are likely to respond, increasing your response numbers.
- QR codes: QR codes store your survey’s URL, and you can print or publish these codes in magazines, signs, business cards, or any object to make it easy for people to access your survey.
- SMS survey: Collect a high number of responses quickly with SMS surveys. It’s a time-effective way to reach your target audience.
Read More: 24 Different Types of Survey Methods With Examples
2. Correlational Research:
Correlational research aims to establish relationships between two or more variables.
Researchers use statistical analysis to identify patterns and trends in the data, but it does not determine causality between the variables. This method helps understand how changes in one variable may impact another.
Examples of correlational research questions include studying the relationship between stress and depression, fame and money, or classroom activities and student performance.
3. Causal-Comparative Research:
Causal-comparative research, also known as quasi-experimental research, seeks to determine cause-and-effect relationships between variables.
Researchers analyze how an independent variable influences a dependent variable, but they do not manipulate the independent variable. Instead, they observe and compare different groups to draw conclusions.
Causal-comparative research is useful in situations where it’s not ethical or feasible to conduct true experiments.
Examples of questions for this type of research include analyzing the effect of training programs on employee performance, studying the influence of customer support on client retention, investigating the impact of supply chain efficiency on cost reduction, etc.
4. Experimental Research:
Experimental research is based on testing theories to validate or disprove them. Researchers conduct experiments and manipulate variables to observe their impact on the outcomes.
This type of research is prevalent in natural and social sciences, and it is a powerful method to establish cause-and-effect relationships. By randomly assigning participants to experimental and control groups, researchers can draw more confident conclusions.
Examples of experimental research include studying the effectiveness of a new drug, the impact of teaching methods on student performance, or the outcomes of a marketing campaign.
B. Data collection methodologies
After defining research objectives, the next significant step in primary quantitative research is data collection. This involves using two main methods: sampling and conducting surveys or polls.
Sampling methods:
In quantitative research, there are two primary sampling methods: Probability and Non-probability sampling.
Probability Sampling
In probability sampling, researchers use the concept of probability to create samples from a population. This method ensures that every individual in the target audience has an equal chance of being selected for the sample.
There are four main types of probability sampling:
- Simple random sampling: Here, the elements or participants of a sample are selected randomly, and this technique is used in studies that are conducted over considerably large audiences. It works well for large target populations.
- Stratified random sampling: In this method, the entire population is divided into strata or groups, and the sample members get chosen randomly from these strata only. It is always ensured that different segregated strata do not overlap with each other.
- Cluster sampling: Here, researchers divide the population into clusters, often based on geography or demographics. Then, random clusters are selected for the sample.
- Systematic sampling: In this method, only the starting point of the sample is randomly chosen. All the other participants are chosen using a fixed interval. Researchers calculate this interval by dividing the size of the study population by the target sample size.
Non-probability Sampling
Non-probability sampling is a method where the researcher’s knowledge and experience guide the selection of samples. This approach doesn’t give all members of the target population an equal chance of being included in the sample.
There are five non-probability sampling models:
- Convenience sampling: The elements or participants are chosen on the basis of their nearness to the researcher. The people in close proximity can be studied and analyzed easily and quickly, as there is no other selection criterion involved. Researchers simply choose samples based on what is most convenient for them.
- Consecutive sampling: Similar to convenience sampling, researchers select samples one after another over a significant period. They can opt for a single participant or a group of samples to conduct quantitative research in a consecutive manner for a significant period of time. Once this is over, they can conduct the research from the start.
- Quota sampling: With quota sampling, researchers use their understanding of target traits and personalities to form groups (strata). They then choose samples from each stratum based on their own judgment.
- Snowball sampling: This method is used where the target audiences are difficult to contact and interviewed for data collection. Researchers start with a few participants and then ask them to refer others, creating a snowball effect.
- Judgmental sampling: In judgmental sampling, researchers rely solely on their experience and research skills to handpick samples that they believe will be most relevant to the study.
Read More: Data Collection Methods: Definition, Types & Examples
C. Data analysis techniques
To analyze the quantitative data accurately, you’ll need to use specific statistical methods such as:
- SWOT Analysis: This stands for Strengths, Weaknesses, Opportunities, and Threats analysis. Organizations use SWOT analysis to evaluate their performance internally and externally. It helps develop effective improvement strategies.
- Conjoint Analysis: This market research method uncovers how individuals make complex purchasing decisions. It involves considering trade-offs in their daily activities when choosing from a list of product/service options.
- Cross-tabulation: A preliminary statistical market analysis method that reveals relationships, patterns, and trends within various research study parameters.
- TURF Analysis: Short for Totally Unduplicated Reach and Frequency Analysis, this method helps analyze the reach and frequency of favorable communication sources. It provides insights into the potential of a target market.
- By using these statistical techniques and inferential statistics methods like confidence intervals and margin of error, you can draw meaningful insights from your primary quantitative research that you can use in making informed decisions.
II. Secondary Quantitative Research Methods
- Secondary quantitative research, also known as desk research, is a valuable method that uses existing data, called secondary data.
- Instead of collecting new data, researchers analyze and combine already available information to enhance their research. This approach involves gathering quantitative data from various sources such as the internet, government databases, libraries, and research reports.
- Secondary quantitative research plays a crucial role in validating data collected through primary quantitative research. It helps reinforce or challenge existing findings.
Here are five commonly used secondary quantitative research methods:
A. Data Available on the Internet:
The Internet has become a vast repository of data, making it easier for researchers to access a wealth of information. Online databases, websites, and research repositories provide valuable quantitative data for researchers to analyze and validate their primary research findings.
B. Government and Non-Government Sources:
Government agencies and non-government organizations often conduct extensive research and publish reports. These reports cover a wide range of topics, providing researchers with reliable and comprehensive data for quantitative analysis.
C. Public Libraries:
While less commonly used in the digital age, public libraries still hold valuable research reports, historical data, and publications that can contribute to quantitative research.
D. Educational Institutions:
Educational institutions frequently conduct research on various subjects. Their research reports and publications can serve as valuable sources of information for researchers, validating and supporting primary quantitative research outcomes.
E. Commercial Information Sources:
Commercial sources such as local newspapers, journals, magazines, and media outlets often publish relevant data on economic trends, market research, and demographic analyses. Researchers can access this data to supplement their own findings and draw better conclusions.
Advantages of Quantitative Research Methods
Quantitative research data is often standardized and can be easily used to generalize findings for making crucial business decisions and uncover insights to supplement the qualitative research findings.
Here are some core benefits this research methodology offers.
Direct Result Comparison
As the studies can be replicated for different cultural settings and different times, even with different groups of participants, they tend to be extremely useful. Researchers can compare the results of different studies in a statistical manner and arrive at comprehensive conclusions for a broader understanding.
Replication
Researchers can repeat the study by using standardized data collection protocols over well-structured data sets. They can also apply tangible definitions of abstract concepts to arrive at different conclusions for similar research objectives with minor variations.
Large Samples
As the research data comes from large samples, the researchers can process and analyze the data via highly reliable and consistent analysis procedures. They can arrive at well-defined conclusions that can be used to make the primary research more thorough and reliable.
Hypothesis Testing
This research methodology follows standardized and established hypothesis testing procedures. So, you have to be careful while reporting and analyzing your research data , and the overall quality of results gets improved.
Proven Examples of Quantitative Research Methods
Below, we discuss two excellent examples of quantitative research methods that were used by highly distinguished business and consulting organizations. Both examples show how different types of analysis can be performed with qualitative approaches and how the analysis is done once the data is collected.
1. STEP Project Global Consortium / KPMG 2019 Global Family Business survey
This research utilized quantitative methods to identify ways that kept the family businesses sustainably profitable with time.
The study also identified the ways in which the family business behavior changed with demographic changes and had “why” and “how” questions. Their qualitative research methods allowed the KPMG team to dig deeper into the mindsets and perspectives of the business owners and uncover unexpected research avenues as well.
It was a joint effort in which STEP Project Global Consortium collected 26 cases, and KPMG collected 11 cases.
The research reached the stage of data analysis in 2020, and the analysis process spanned over 4 stages.
The results, which were also the reasons why family businesses tend to lose their strength with time, were found to be:
- Family governance
- Family business legacy
2. EY Seren Teams Research 2020
This is yet another commendable example of qualitative research where the EY Seren Team digs into the unexplored depths of human behavior and how it affected their brand or service expectations.
The research was done across 200+ sources and involved in-depth virtual interviews with people in their homes, exploring their current needs and wishes. It also involved diary studies across the entire UK customer base to analyze human behavior changes and patterns.
The study also included interviews with professionals and design leaders from a wide range of industries to explore how COVID-19 transformed their industries. Finally, quantitative surveys were conducted to gain insights into the EY community after every 15 days.
The insights and results were:
- A culture of fear, daily resilience, and hopes for a better world and a better life – these were the macro trends.
- People felt massive digitization to be a resourceful yet demanding aspect as they have to adapt every day.
- Some people wished to have a new world with lots of possibilities, and some were looking for a new purpose.
Enhance Your Quantitative Research With Cutting-Edge Software
While no single research methodology can produce 100% reliable results, you can always opt for a hybrid research method by opting for the methods that are most relevant to your objective.
This understanding comes gradually as you learn how to implement the correct combination of qualitative and quantitative research methods for your research projects. For the best results, we recommend investing in smart, efficient, and scalable research tools that come with delightful reporting and advanced analytics to make every research initiative a success.
These software tools, such as ProProfs Survey Maker, come with pre-built survey templates and question libraries and allow you to create a high-converting survey in just a few minutes.
So, choose the best research partner, create the right research plan, and gather insights that drive sustainable growth for your business.
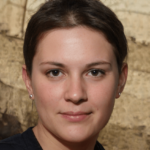
About the author
Emma David is a seasoned market research professional with 8+ years of experience. Having kick-started her journey in research, she has developed rich expertise in employee engagement, survey creation and administration, and data management. Emma believes in the power of data to shape business performance positively. She continues to help brands and businesses make strategic decisions and improve their market standing through her understanding of research methodologies.
Popular Posts in This Category
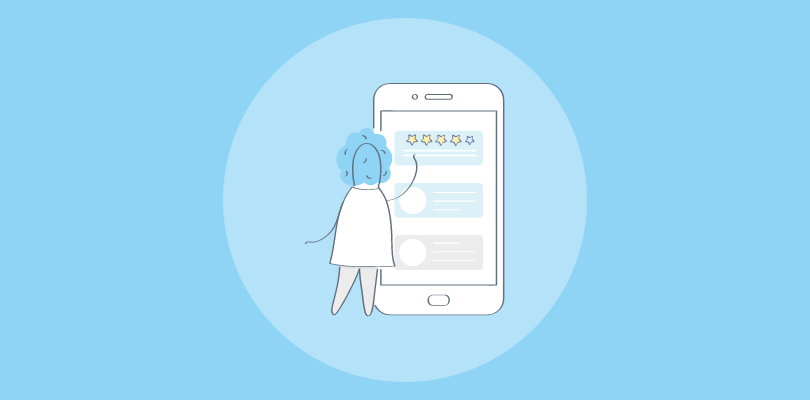
10 Best Patient Engagement Software to Consider in 2024
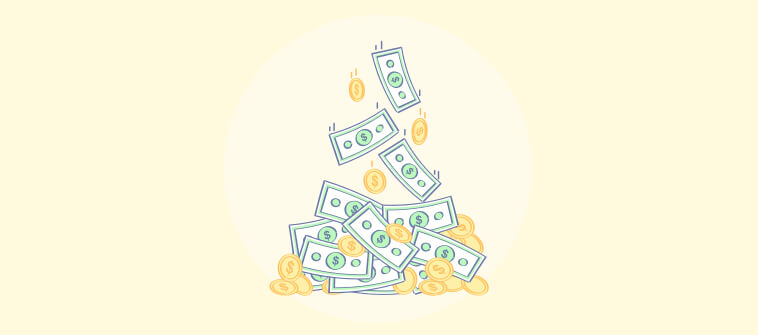
Importance of Customer Experience in 2024 – Billion Dollar Club Infographic
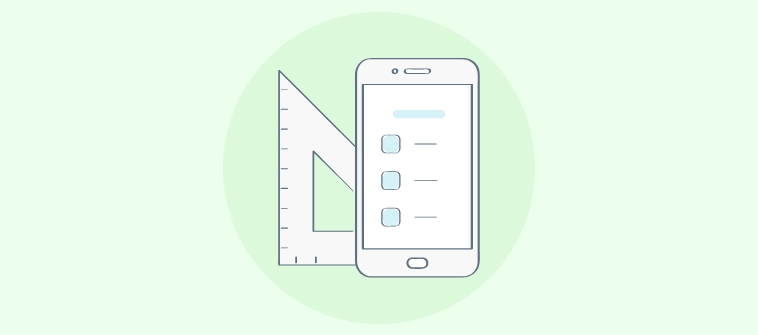
Likert Scale: Definition, Types, Questions & Advantages
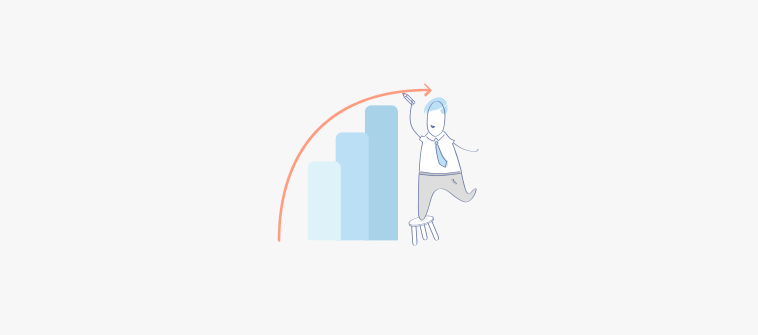
The Ultimate Conversational Marketing Guide
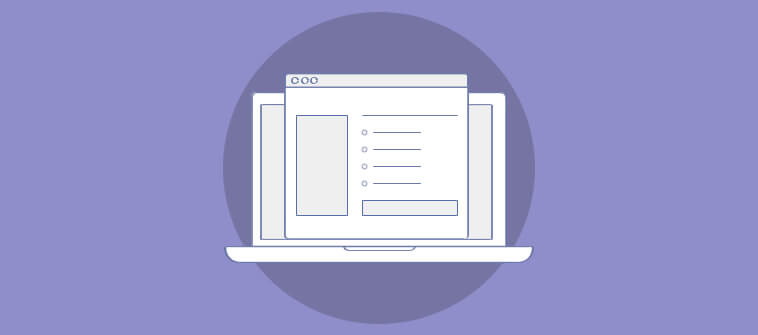
Create an Online Survey in 8 Easy Steps: A Detailed Guide
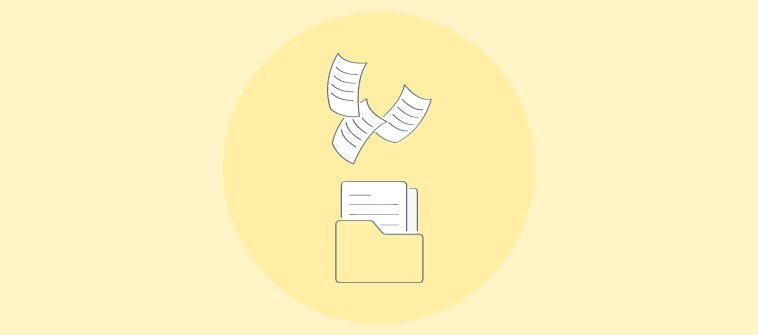
Quantitative Data: Types, Analysis & Examples

An official website of the United States government
The .gov means it’s official. Federal government websites often end in .gov or .mil. Before sharing sensitive information, make sure you’re on a federal government site.
The site is secure. The https:// ensures that you are connecting to the official website and that any information you provide is encrypted and transmitted securely.
- Publications
- Account settings
Preview improvements coming to the PMC website in October 2024. Learn More or Try it out now .
- Advanced Search
- Journal List
- An Bras Dermatol
- v.91(3); May-Jun 2016
Sampling: how to select participants in my research study? *
Jeovany martínez-mesa.
1 Faculdade Meridional (IMED) - Passo Fundo (RS), Brazil.
David Alejandro González-Chica
2 University of Adelaide - Adelaide, Australia.
Rodrigo Pereira Duquia
3 Universidade Federal de Ciências da Saúde de Porto Alegre (UFCSPA) - Porto Alegre (RS), Brazil.
Renan Rangel Bonamigo
João luiz bastos.
4 Universidade Federal de Santa Catarina (UFSC) - Florianópolis (RS), Brazil.
In this paper, the basic elements related to the selection of participants for a health research are discussed. Sample representativeness, sample frame, types of sampling, as well as the impact that non-respondents may have on results of a study are described. The whole discussion is supported by practical examples to facilitate the reader's understanding.
To introduce readers to issues related to sampling.
INTRODUCTION
The essential topics related to the selection of participants for a health research are: 1) whether to work with samples or include the whole reference population in the study (census); 2) the sample basis; 3) the sampling process and 4) the potential effects nonrespondents might have on study results. We will refer to each of these aspects with theoretical and practical examples for better understanding in the sections that follow.
TO SAMPLE OR NOT TO SAMPLE
In a previous paper, we discussed the necessary parameters on which to estimate the sample size. 1 We define sample as a finite part or subset of participants drawn from the target population. In turn, the target population corresponds to the entire set of subjects whose characteristics are of interest to the research team. Based on results obtained from a sample, researchers may draw their conclusions about the target population with a certain level of confidence, following a process called statistical inference. When the sample contains fewer individuals than the minimum necessary, but the representativeness is preserved, statistical inference may be compromised in terms of precision (prevalence studies) and/or statistical power to detect the associations of interest. 1 On the other hand, samples without representativeness may not be a reliable source to draw conclusions about the reference population (i.e., statistical inference is not deemed possible), even if the sample size reaches the required number of participants. Lack of representativeness can occur as a result of flawed selection procedures (sampling bias) or when the probability of refusal/non-participation in the study is related to the object of research (nonresponse bias). 1 , 2
Although most studies are performed using samples, whether or not they represent any target population, census-based estimates should be preferred whenever possible. 3 , 4 For instance, if all cases of melanoma are available on a national or regional database, and information on the potential risk factors are also available, it would be preferable to conduct a census instead of investigating a sample.
However, there are several theoretical and practical reasons that prevent us from carrying out census-based surveys, including:
- Ethical issues: it is unethical to include a greater number of individuals than that effectively required;
- Budgetary limitations: the high costs of a census survey often limits its use as a strategy to select participants for a study;
- Logistics: censuses often impose great challenges in terms of required staff, equipment, etc. to conduct the study;
- Time restrictions: the amount of time needed to plan and conduct a census-based survey may be excessive; and,
- Unknown target population size: if the study objective is to investigate the presence of premalignant skin lesions in illicit drugs users, lack of information on all existing users makes it impossible to conduct a census-based study.
All these reasons explain why samples are more frequently used. However, researchers must be aware that sample results can be affected by the random error (or sampling error). 3 To exemplify this concept, we will consider a research study aiming to estimate the prevalence of premalignant skin lesions (outcome) among individuals >18 years residing in a specific city (target population). The city has a total population of 4,000 adults, but the investigator decided to collect data on a representative sample of 400 participants, detecting an 8% prevalence of premalignant skin lesions. A week later, the researcher selects another sample of 400 participants from the same target population to confirm the results, but this time observes a 12% prevalence of premalignant skin lesions. Based on these findings, is it possible to assume that the prevalence of lesions increased from the first to the second week? The answer is probably not. Each time we select a new sample, it is very likely to obtain a different result. These fluctuations are attributed to the "random error." They occur because individuals composing different samples are not the same, even though they were selected from the same target population. Therefore, the parameters of interest may vary randomly from one sample to another. Despite this fluctuation, if it were possible to obtain 100 different samples of the same population, approximately 95 of them would provide prevalence estimates very close to the real estimate in the target population - the value that we would observe if we investigated all the 4,000 adults residing in the city. Thus, during the sample size estimation the investigator must specify in advance the highest or maximum acceptable random error value in the study. Most population-based studies use a random error ranging from 2 to 5 percentage points. Nevertheless, the researcher should be aware that the smaller the random error considered in the study, the larger the required sample size. 1
SAMPLE FRAME
The sample frame is the group of individuals that can be selected from the target population given the sampling process used in the study. For example, to identify cases of cutaneous melanoma the researcher may consider to utilize as sample frame the national cancer registry system or the anatomopathological records of skin biopsies. Given that the sample may represent only a portion of the target population, the researcher needs to examine carefully whether the selected sample frame fits the study objectives or hypotheses, and especially if there are strategies to overcome the sample frame limitations (see Chart 1 for examples and possible limitations).
Examples of sample frames and potential limitations as regards representativeness
Sampling can be defined as the process through which individuals or sampling units are selected from the sample frame. The sampling strategy needs to be specified in advance, given that the sampling method may affect the sample size estimation. 1 , 5 Without a rigorous sampling plan the estimates derived from the study may be biased (selection bias). 3
TYPES OF SAMPLING
In figure 1 , we depict a summary of the main sampling types. There are two major sampling types: probabilistic and nonprobabilistic.

Sampling types used in scientific studies
NONPROBABILISTIC SAMPLING
In the context of nonprobabilistic sampling, the likelihood of selecting some individuals from the target population is null. This type of sampling does not render a representative sample; therefore, the observed results are usually not generalizable to the target population. Still, unrepresentative samples may be useful for some specific research objectives, and may help answer particular research questions, as well as contribute to the generation of new hypotheses. 4 The different types of nonprobabilistic sampling are detailed below.
Convenience sampling : the participants are consecutively selected in order of apperance according to their convenient accessibility (also known as consecutive sampling). The sampling process comes to an end when the total amount of participants (sample saturation) and/or the time limit (time saturation) are reached. Randomized clinical trials are usually based on convenience sampling. After sampling, participants are usually randomly allocated to the intervention or control group (randomization). 3 Although randomization is a probabilistic process to obtain two comparable groups (treatment and control), the samples used in these studies are generally not representative of the target population.
Purposive sampling: this is used when a diverse sample is necessary or the opinion of experts in a particular field is the topic of interest. This technique was used in the study by Roubille et al, in which recommendations for the treatment of comorbidities in patients with rheumatoid arthritis, psoriasis, and psoriatic arthritis were made based on the opinion of a group of experts. 6
Quota sampling: according to this sampling technique, the population is first classified by characteristics such as gender, age, etc. Subsequently, sampling units are selected to complete each quota. For example, in the study by Larkin et al., the combination of vemurafenib and cobimetinib versus placebo was tested in patients with locally-advanced melanoma, stage IIIC or IV, with BRAF mutation. 7 The study recruited 495 patients from 135 health centers located in several countries. In this type of study, each center has a "quota" of patients.
"Snowball" sampling : in this case, the researcher selects an initial group of individuals. Then, these participants indicate other potential members with similar characteristics to take part in the study. This is frequently used in studies investigating special populations, for example, those including illicit drugs users, as was the case of the study by Gonçalves et al, which assessed 27 users of cocaine and crack in combination with marijuana. 8
PROBABILISTIC SAMPLING
In the context of probabilistic sampling, all units of the target population have a nonzero probability to take part in the study. If all participants are equally likely to be selected in the study, equiprobabilistic sampling is being used, and the odds of being selected by the research team may be expressed by the formula: P=1/N, where P equals the probability of taking part in the study and N corresponds to the size of the target population. The main types of probabilistic sampling are described below.
Simple random sampling: in this case, we have a full list of sample units or participants (sample basis), and we randomly select individuals using a table of random numbers. An example is the study by Pimenta et al, in which the authors obtained a listing from the Health Department of all elderly enrolled in the Family Health Strategy and, by simple random sampling, selected a sample of 449 participants. 9
Systematic random sampling: in this case, participants are selected from fixed intervals previously defined from a ranked list of participants. For example, in the study of Kelbore et al, children who were assisted at the Pediatric Dermatology Service were selected to evaluate factors associated with atopic dermatitis, selecting always the second child by consulting order. 10
Stratified sampling: in this type of sampling, the target population is first divided into separate strata. Then, samples are selected within each stratum, either through simple or systematic sampling. The total number of individuals to be selected in each stratum can be fixed or proportional to the size of each stratum. Each individual may be equally likely to be selected to participate in the study. However, the fixed method usually involves the use of sampling weights in the statistical analysis (inverse of the probability of selection or 1/P). An example is the study conducted in South Australia to investigate factors associated with vitamin D deficiency in preschool children. Using the national census as the sample frame, households were randomly selected in each stratum and all children in the age group of interest identified in the selected houses were investigated. 11
Cluster sampling: in this type of probabilistic sampling, groups such as health facilities, schools, etc., are sampled. In the above-mentioned study, the selection of households is an example of cluster sampling. 11
Complex or multi-stage sampling: This probabilistic sampling method combines different strategies in the selection of the sample units. An example is the study of Duquia et al. to assess the prevalence and factors associated with the use of sunscreen in adults. The sampling process included two stages. 12 Using the 2000 Brazilian demographic census as sampling frame, all 404 census tracts from Pelotas (Southern Brazil) were listed in ascending order of family income. A sample of 120 tracts were systematically selected (first sampling stage units). In the second stage, 12 households in each of these census tract (second sampling stage units) were systematically drawn. All adult residents in these households were included in the study (third sampling stage units). All these stages have to be considered in the statistical analysis to provide correct estimates.
NONRESPONDENTS
Frequently, sample sizes are increased by 10% to compensate for potential nonresponses (refusals/losses). 1 Let us imagine that in a study to assess the prevalence of premalignant skin lesions there is a higher percentage of nonrespondents among men (10%) than among women (1%). If the highest percentage of nonresponse occurs because these men are not at home during the scheduled visits, and these participants are more likely to be exposed to the sun, the number of skin lesions will be underestimated. For this reason, it is strongly recommended to collect and describe some basic characteristics of nonrespondents (sex, age, etc.) so they can be compared to the respondents to evaluate whether the results may have been affected by this systematic error.
Often, in study protocols, refusal to participate or sign the informed consent is considered an "exclusion criteria". However, this is not correct, as these individuals are eligible for the study and need to be reported as "nonrespondents".
SAMPLING METHOD ACCORDING TO THE TYPE OF STUDY
In general, clinical trials aim to obtain a homogeneous sample which is not necessarily representative of any target population. Clinical trials often recruit those participants who are most likely to benefit from the intervention. 3 Thus, the more strict criteria for inclusion and exclusion of subjects in clinical trials often make it difficult to locate participants: after verification of the eligibility criteria, just one out of ten possible candidates will enter the study. Therefore, clinical trials usually show limitations to generalize the results to the entire population of patients with the disease, but only to those with similar characteristics to the sample included in the study. These peculiarities in clinical trials justify the necessity of conducting a multicenter and/or global studiesto accelerate the recruitment rate and to reach, in a shorter time, the number of patients required for the study. 13
In turn, in observational studies to build a solid sampling plan is important because of the great heterogeneity usually observed in the target population. Therefore, this heterogeneity has to be also reflected in the sample. A cross-sectional population-based study aiming to assess disease estimates or identify risk factors often uses complex probabilistic sampling, because the sample representativeness is crucial. However, in a case-control study, we face the challenge of selecting two different samples for the same study. One sample is formed by the cases, which are identified based on the diagnosis of the disease of interest. The other consists of controls, which need to be representative of the population that originated the cases. Improper selection of control individuals may introduce selection bias in the results. Thus, the concern with representativeness in this type of study is established based on the relationship between cases and controls (comparability).
In cohort studies, individuals are recruited based on the exposure (exposed and unexposed subjects), and they are followed over time to evaluate the occurrence of the outcome of interest. At baseline, the sample can be selected from a representative sample (population-based cohort studies) or a non-representative sample. However, in the successive follow-ups of the cohort member, study participants must be a representative sample of those included in the baseline. 14 , 15 In this type of study, losses over time may cause follow-up bias.
Researchers need to decide during the planning stage of the study if they will work with the entire target population or a sample. Working with a sample involves different steps, including sample size estimation, identification of the sample frame, and selection of the sampling method to be adopted.
Financial Support: None.
* Study performed at Faculdade Meridional - Escola de Medicina (IMED) - Passo Fundo (RS), Brazil.
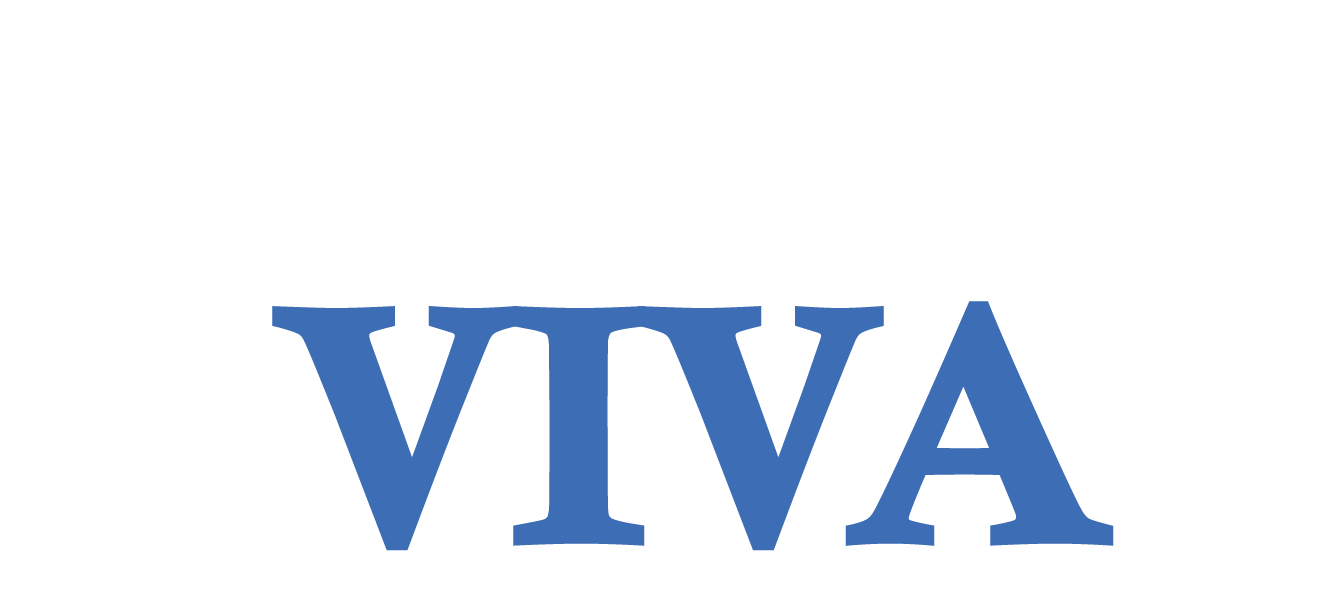
Want to create or adapt books like this? Learn more about how Pressbooks supports open publishing practices.
18 10. Quantitative sampling
Chapter Outline
- The sampling process (25 minute read time)
- Sampling approaches for quantitative research (15 minute read time)
- Sample quality (24 minute read time)
Content warning: examples contain references to addiction to technology, domestic violence and batterer intervention, cancer, illegal drug use, LGBTQ+ discrimination, binge drinking, intimate partner violence among college students, child abuse, neocolonialism and Western hegemony.
10.1 The sampling process
Learning Objectives
Learners will be able to…
- Decide where to get your data and who you might need to talk to
- Evaluate whether it is feasible for you to collect first-hand data from your target population
- Describe the process of sampling
- Apply population, sampling frame, and other sampling terminology to sampling people your project’s target population
One of the things that surprised me most as a research methods professor is how much my students struggle with understanding sampling. It is surprising because people engage in sampling all the time. How do you learn whether you like a particular food, like BBQ ribs? You sample them from different restaurants! Obviously, social scientists put a bit more effort and thought into the process than that, but the underlying logic is the same. By sampling a small group of BBQ ribs from different restaurants and liking most of them, you can conclude that when you encounter BBQ ribs again, you will probably like them. You don’t need to eat all of the BBQ ribs in the world to come to that conclusion, just a small sample. [1] Part of the difficulty my students face is learning sampling terminology, which is the focus of this section.
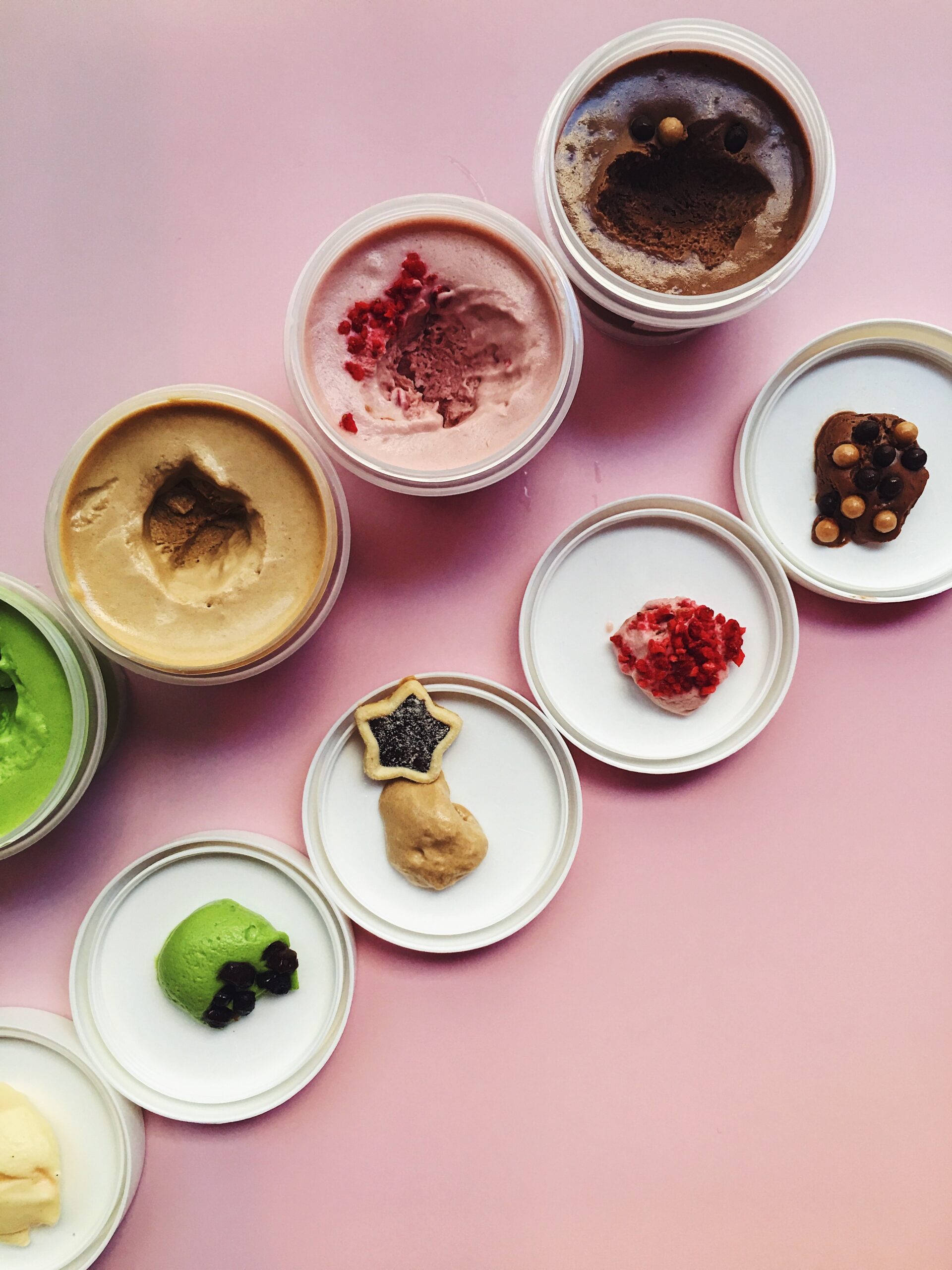
Who is your study about and who should you talk to?
At this point in the research process, you know what your research question is. Our goal in this chapter is to help you understand how to find the people (or documents) you need to study in order to find the answer to your research question. It may be helpful at this point to distinguish between two concepts. Your unit of analysis is the entity that you wish to be able to say something about at the end of your study (probably what you’d consider to be the main focus of your study). Your unit of observation is the entity (or entities) that you actually observe, measure, or collect in the course of trying to learn something about your unit of analysis.
It is often the case that your unit of analysis and unit of observation are the same. For example, we may want to say something about social work students (unit of analysis), so we ask social work students at our university to complete a survey for our study (unit of observation). In this case, we are observing individuals , i.e. students, so we can make conclusions about individual s .
On the other hand, our unit of analysis and observation can differ. We could sample social work students to draw conclusions about organizations or universities. Perhaps we are comparing students at historically black colleges and universities (HBCUs) and primarily white institutions (PWIs). Even though our sample was made up of individual students from various colleges (our unit of observation), our unit of analysis was the university as an organization. Conclusions we made from individual-level data were used to understand larger organizations.
Similarly, we could adjust our sampling approach to target specific student cohorts. Perhaps we wanted to understand the experiences of black social work students in PWIs. We could choose either an individual unit of observation by selecting students, or a group unit of observation by studying the National Association of Black Social Workers .
Sometimes the units of analysis and observation differ due to pragmatic reasons. If we wanted to study whether being a social work student impacted family relationships, we may choose to study family members of students in social work programs who could give us information about how they behaved in the home. In this case, we would be observing family members to draw conclusions about individual students.
In sum, there are many potential units of analysis that a social worker might examine, but some of the most common include i ndividuals, groups, and organizations. Table 10.1 details examples identifying the units of observation and analysis in a hypothetical study of student addiction to electronic gadgets.
First-hand vs. second-hand knowledge
Your unit of analysis will be determined by your research question. Specifically, it should relate to your target population. Your unit of observation, on the other hand, is determined largely by the method of data collection you use to answer that research question. Let’s consider a common issue in social work research: understanding the effectiveness of different social work interventions. Who has first-hand knowledge and who has second-hand knowledge? Well, practitioners would have first-hand knowledge about implementing the intervention. For example, they might discuss with you the unique language they use help clients understand the intervention. Clients, on the other hand, have first-hand knowledge about the impact of those interventions on their lives. If you want to know if an intervention is effective, you need to ask people who have received it!
Unfortunately, student projects run into pragmatic limitations with sampling from client groups. Clients are often diagnosed with severe mental health issues or have other ongoing issues that render them a vulnerable population at greater risk of harm. Asking a person who was recently experiencing suicidal ideation about that experience may interfere with ongoing treatment. Client records are also confidential and cannot be shared with researchers unless clients give explicit permission. Asking one’s own clients to participate in the study creates a dual relationship with the client, as both clinician and researcher, and dual relationship have conflicting responsibilities and boundaries.
Obviously, studies are done with social work clients all the time. But for student projects in the classroom, it is often required to get second-hand information from a population that is less vulnerable. Students may instead choose to study clinicians and how they perceive the effectiveness of different interventions. While clinicians can provide an informed perspective, they have less knowledge about personally receiving the intervention. In general, researchers prefer to sample the people who have first-hand knowledge about their topic, though feasibility often forces them to analyze second-hand information instead.
Population: Who do you want to study?
In social scientific research, a population is the cluster of people you are most interested in. It is often the “who” that you want to be able to say something about at the end of your study. While populations in research may be rather large, such as “the American people,” they are more typically more specific than that. For example, a large study for which the population of interest is the American people will likely specify which American people, such as adults over the age of 18 or citizens or legal permanent residents. Based on your work in Chapter 2, you should have a target population identified in your working question. That might be something like “people with developmental disabilities” or “students in a social work program.”
It is almost impossible for a researcher to gather data from their entire population of interest. This might sound surprising or disappointing until you think about the kinds of research questions that social workers typically ask. For example, let’s say we wish to answer the following question: “How does gender impact attendance in a batterer intervention program?” Would you expect to be able to collect data from all people in batterer intervention programs across all nations from all historical time periods? Unless you plan to make answering this research question your entire life’s work (and then some), I’m guessing your answer is a resounding no. So, what to do? Does not having the time or resources to gather data from every single person of interest mean having to give up your research interest?
- What is your population, the people you want to make conclusions about?
- Do your unit of analysis and unit of observation differ or are they the same?
- Can you ethically and practically get first-hand information from the people most knowledgeable about the topic, or will you rely on second-hand information from less vulnerable populations?
Setting: Where will you go to get your data?
While you can’t gather data from everyone, you can find some people from your target population to study. The first rule of sampling is: go where your participants are. You will need to figure out where you will go to get your data. For many student researchers, it is their agency, their peers, their family and friends, or whoever comes across students’ social media posts or emails asking people to participate in their study.
Each setting (agency, social media) limits your reach to only a small segment of your target population who has the opportunity to be a part of your study. This intermediate point between the overall population and the sample of people who actually participate in the researcher’s study is called a sampling frame . A sampling frame is a list of people from which you will draw your sample.
But where do you find a sampling frame? Answering this question is the first step in conducting human subjects research. Social work researchers must think about locations or groups in which your target population gathers or interacts. For example, a study on quality of care in nursing homes may choose a local nursing home because it’s easy to access. The sampling frame could be all of the residents of the nursing home. You would select your participants for your study from the list of residents. Note that this is a real list. That is, an administrator at the nursing home would give you a list with every resident’s name or ID number from which you would select your participants. If you decided to include more nursing homes in your study, then your sampling frame could be all the residents at all the nursing homes who agreed to participate in your study.
Let’s consider some more examples. Unlike nursing home patients, cancer survivors do not live in an enclosed location and may no longer receive treatment at a hospital or clinic. For social work researchers to reach participants, they may consider partnering with a support group that services this population. Perhaps there is a support group at a local church survivors may attend. Without a set list of people, your sampling frame would simply be the people who showed up to the support group on the nights you were there. Similarly, if you posted an advertisement in an online peer-support group for people with cancer, your sampling frame is the people in that group.
More challenging still is recruiting people who are homeless, those with very low income, or those who belong to stigmatized groups. For example, a research study by Johnson and Johnson (2014) [2] attempted to learn usage patterns of “bath salts,” or synthetic stimulants that are marketed as “legal highs.” Users of “bath salts” don’t often gather for meetings, and reaching out to individual treatment centers is unlikely to produce enough participants for a study, as the use of bath salts is rare. To reach participants, these researchers ingeniously used online discussion boards in which users of these drugs communicate. Their sampling frame included everyone who participated in the online discussion boards during the time they collected data. Another example might include using a flyer to let people know about your study, in which case your sampling frame would be anyone who walks past your flyer wherever you hang it—usually in a strategic location where you know your population will be.
In conclusion, sampling frames can be a real list of people like the list of faculty and their ID numbers in a university department, which allows you to clearly identify who is in your study and what chance they have of being selected. However, not all sampling frames allow you to be so specific. It is also important to remember that accessing your sampling frame must be practical and ethical, as we discussed in section 2.3 and in Chapter 8. For studies that present risks to participants, approval from gatekeepers and the university’s institutional review board (IRB) is needed.
Criteria: What characteristics must your participants have/not have?
Your sampling frame is not just everyone in the setting you identified. For example, if you were studying MSW students who are first-generation college students, you might select your university as the setting, but not everyone in your program is a first-generation student. You need to be more specific about which characteristics or attributes individuals either must have or cannot have before they participate in the study. These are known as inclusion and exclusion criteria, respectively.
Inclusion criteria are the characteristics a person must possess in order to be included in your sample. If you were conducting a survey on LGBTQ+ discrimination at your agency, you might want to sample only clients who identify as LGBTQ+. In that case, your inclusion criteria for your sample would be that individuals have to identify as LGBTQ+.
Comparably, exclusion criteria are characteristics that disqualify a person from being included in your sample. In the previous example, you could think of cisgenderism and heterosexuality as your exclusion criteria because no person who identifies as heterosexual or cisgender would be included in your sample. Exclusion criteria are often the mirror image of inclusion criteria. However, there may be other criteria by which we want to exclude people from our sample. For example, we may exclude clients who were recently discharged or those who have just begun to receive services.
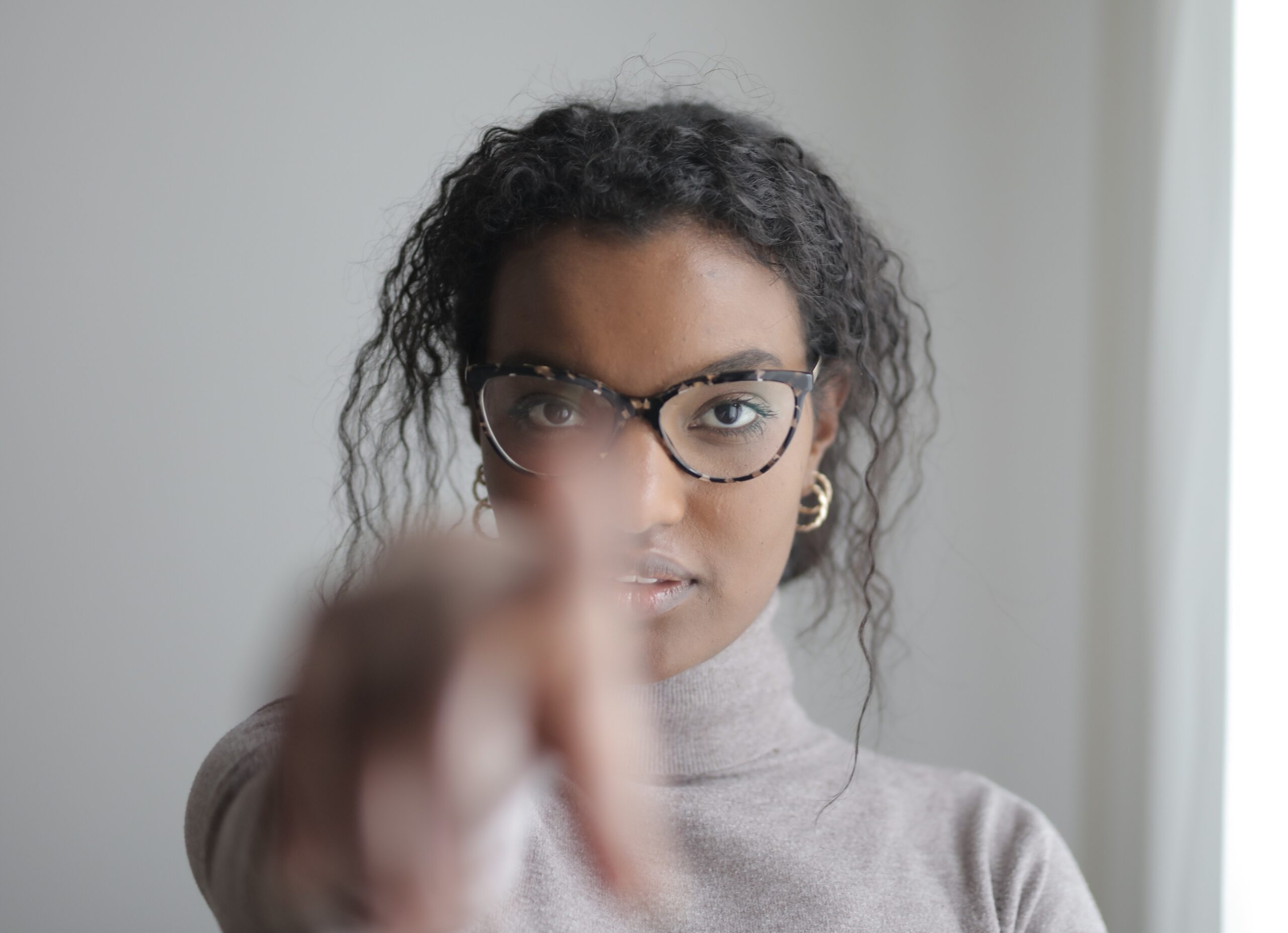
Recruitment: How will you ask people to participate in your study?
Once you have a location and list of people from which to select, all that is left is to reach out to your participants. Recruitment refers to the process by which the researcher informs potential participants about the study and asks them to participate in the research project. Recruitment comes in many different forms. If you have ever received a phone call asking for you to participate in a survey, someone has attempted to recruit you for their study. Perhaps you’ve seen print advertisements on buses, in student centers, or in a newspaper. I’ve received many emails that were passed around my school asking for participants, usually for a graduate student project. (As an aside, researchers sometimes speak of “research karma.” If you participate in others’ research studies, they will participate in yours.) As we learn more about specific types of sampling, make sure your recruitment strategy makes sense with your sampling approach. For example, if you put up a flyer in the student health office to recruit student athletes for your study, you may not be targeting your recruitment efforts to settings where your target population is likely to see your recruitment materials.
Recruiting human participants
Sampling is the first time in which you will contact potential study participants. Before you start this process, it is important to make sure you have approval from your university’s institutional review board as well as any gatekeepers at the locations in which you plan to conduct your study. As we discussed in section 10.1, the first rule of sampling is to go where your participants are. If you are studying domestic violence, reach out to local shelters, advocates, or service agencies. Gatekeepers will be necessary to gain access to your participants. For example, a gatekeeper can forward your recruitment email across their employee email list. Review our discussion of gatekeepers in Chapter 2 before proceeding with contacting potential participants as part of recruitment.
Recruitment can take many forms. You may show up at a staff meeting to ask for volunteers. You may send a company-wide email. Each step of this process should be vetted by the IRB as well as other stakeholders and gatekeepers. You will also need to set reasonable expectations for how many reminders you will send to the person before moving on. Generally, it is a good idea to give people a little while to respond, though reminders are often accompanied by an increase in participation. Pragmatically, it is a good idea for you to think through each step of the recruitment process and how much time it will take to complete it.
For example, as a graduate student, I conducted a study of state-level disabilities administrators in which I was recruiting a sample of very busy people and had no financial incentives to offer them for participating in my study. It helped for my research team to bring on board a well-known agency as a research partner, allowing them to review and offer suggestions on our survey and interview questions. This collaborative process took time and had to be completed before sampling could start. Once sampling commenced, I pulled contact names from my collaborator’s database and public websites, and set a weekly schedule of email and phone contacts. I would contact the director once via email. Ten days later, I would follow up via email and by leaving a voicemail with their administrative support staff. Ten days after that, I would reach out to state administrators in a different office via email and then again via phone, if needed. The process took months to complete and required a complex Excel tracking document.
Recruitment will also expose your participants to the informed consent information you prepared. For students going through the IRB, there are templates you will have to follow in order to get your study approved. For students whose projects unfold under the supervision of their department, rather than the IRB, you should check with your professor on what the expectations are for getting participant consent. In the aforementioned study, I used our IRB’s template to create a consent form but did not include a signature line. The IRB allowed me to collect my data without a signature, as there was little risk of harm from the study. It was imperative to review consent information before completing the survey and interview with participants. Only when the participant is totally clear on the purpose, risks and benefits, confidentiality protections, and other information detailed in Chapter 8, can you ethically move forward with including them in your sample.
Sampling available documents
As with sampling humans, sampling documents centers around the question: which documents are the most relevant to your research question, in that which will provide you first-hand knowledge. Common documents analyzed in student research projects include client files, popular media like film and music lyrics, and policies from service agencies. In a case record review, the student would create exclusion and inclusion criteria based on their research question. Once a suitable sampling frame of potential documents exists, the researcher can use probability or non-probability sampling to select which client files are ultimately analyzed.
Sampling documents must also come with consent and buy-in from stakeholders and gatekeepers. Assuming you have approval to conduct your study and access to the documents you need, the process of recruitment is much easier than in studies sampling humans. There is no informed consent process with documents, though research with confidential health or education records must be done in accordance with privacy laws such as the Health Insurance Portability and Accountability Act and the Family Educational Rights and Privacy Act . Barring any technical or policy obstacles, the gathering of documents should be easier and less time consuming than sampling humans.
Sample: Who actually participates in your study?
Once you find a sampling frame from which you can recruit your participants and decide which characteristics you will include and exclude, you will recruit people using a specific sampling approach, which we will cover in Section 10.2. At the end, you’re left with the group of people you successfully recruited from your sampling frame to participate in your study, your sample . If you are a participant in a research project—answering survey questions, participating in interviews, etc.—you are part of the sample in that research project.
Visualizing sampling terms
Sampling terms can be a bit daunting at first. However, with some practice, they will become second nature. Let’s walk through an example from a research project of mine. I collected data for a research project related to how much it costs to become a licensed clinical social worker (LCSW) in each state. Becoming an LCSW is necessary to work in private clinical practice and is used by supervisors in human service organizations to sign off on clinical charts from less credentialed employees, and to provide clinical supervision. If you are interested in providing clinical services as a social worker, you should become familiar with the licensing laws in your state.
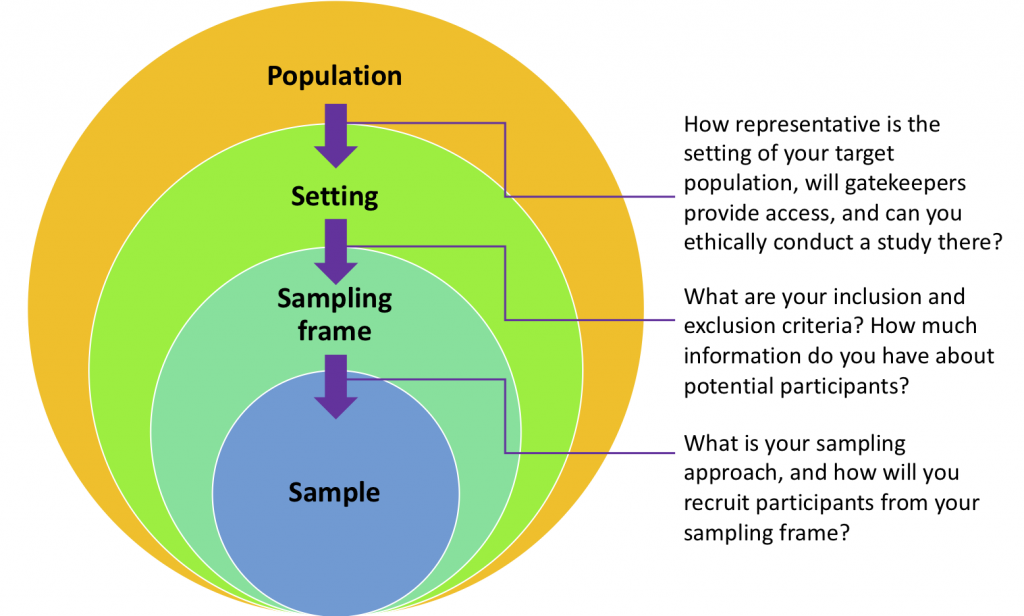
Using Figure 10.1 as a guide, my population is clearly clinical social workers, as these are the people about whom I want to draw conclusions. The next step inward would be a sampling frame. Unfortunately, there is no list of every licensed clinical social worker in the United States. I could write to each state’s social work licensing board and ask for a list of names and addresses, perhaps even using a Freedom of Information Act request if they were unwilling to share the information. That option sounds time-consuming and has a low likelihood of success. Instead, I tried to figure out a convenient setting social workers are likely to congregate. I considered setting up a booth at a National Association of Social Workers (NASW) conference and asking people to participate in my survey. Ultimately, this would prove too costly, and the people who gather at an NASW conference may not be representative of the general population of clinical social workers. I finally discovered the NASW membership email list, which is available to advertisers, including researchers advertising for research projects. While the NASW list does not contain every clinical social worker, it reaches over one hundred thousand social workers regularly through its monthly e-newsletter, a large proportion of social workers in practice, so the setting was likely to draw a representative sample. To gain access to this setting from gatekeepers, I had to provide paperwork showing my study had undergone IRB review and submit my measures for approval by the mailing list administrator.
Once I gained access from gatekeepers, my setting became the members of the NASW membership list. I decided to recruit 5,000 participants because I knew that people sometimes do not read or respond to email advertisements, and I figured maybe 20% would respond, which would give me around 1,000 responses. Figuring out my sample size was a challenge, because I had to balance the costs associated with using the NASW newsletter. As you can see on their pricing page , it would cost money to learn personal information about my potential participants, which I would need to check later in order to determine if my population was representative of the overall population of social workers. For example, I could see if my sample was comparable in race, age, gender, or state of residence to the broader population of social workers by comparing my sample with information about all social workers published by NASW. I presented my options to my external funder as:
- I could send an email advertisement to a lot of people (5,000), but I would know very little about them and they would get only one advertisement.
- I could send multiple advertisements to fewer people (1,000) reminding them to participate, but I would also know more about them by purchasing access to personal information.
- I could send multiple advertisements to fewer people (2,500), but not purchase access to personal information to minimize costs.
In your project, there is no expectation you purchase access to anything, and if you plan on using email advertisements, consider places that are free to access like employee or student listservs. At the same time, you will need to consider what you can know or not know about the people who will potentially be in your study, and I could collect any personal information we wanted to check representativeness in the study itself. For this reason, we decided to go with option #1. When I sent my email recruiting participants for the study, I specified that I only wanted to hear from social workers who were either currently receiving or recently received clinical supervision for licensure—my inclusion criteria. This was important because many of the people on the NASW membership list may not be licensed or license-seeking social workers. So, my sampling frame was the email addresses on the NASW mailing list who fit the inclusion criteria for the study, which I figured would be at least a few thousand people. Unfortunately, only 150 licensed or license-seeking clinical social workers responded to my recruitment email and completed the survey. You will learn in Section 10.3 why this did not make for a very good sample.
From this example, you can see that sampling is a process. The process flows sequentially from figuring out your target population, to thinking about where to find people from your target population, to figuring out how much information you know about potential participants, and finally to selecting recruiting people from that list to be a part of your sample. Through the sampling process, you must consider where people in your target population are likely to be and how best to get their attention for your study. Sampling can be an easy process, like calling every 100th name from the phone book, or challenging, like standing every day for a few weeks in an area in which people who are homeless gather for shelter. In either case, your goal is to recruit enough people who will participate in your study so you can learn about your population.
What about sampling non-humans?
Many student projects do not involve recruiting and sampling human subjects. Instead, many research projects will sample objects like client charts, movies, or books. The same terms apply, but the process is a bit easier because there are no humans involved. If a research project involves analyzing client files, it is unlikely you will look at every client file that your agency has. You will need to figure out which client files are important to your research question. Perhaps you want to sample clients who have a diagnosis of reactive attachment disorder. You would have to create a list of all clients at your agency (setting) who have reactive attachment disorder (your inclusion criteria) then use your sampling approach (which we will discuss in the next section) to select which client files you will actually analyze for your study (your sample). Recruitment is a lot easier because, well, there’s no one to convince but your gatekeepers, the managers of your agency. However, researchers who publish chart reviews must obtain IRB permission before doing so.
Key Takeaways
- The first rule of sampling is to go where your participants are. Think about virtual or in-person settings in which your target population gathers. Remember that you may have to engage gatekeepers and stakeholders in accessing many settings, and that you will need to assess the pragmatic challenges and ethical risks and benefits of your study.
- Consider whether you can sample documents like agency files to answer your research question. Documents are much easier to “recruit” than people!
- Researchers must consider which characteristics are necessary for people to have (inclusion criteria) or not have (exclusion criteria), as well as how to recruit participants into the sample.
- Social workers can sample individuals, groups, or organizations.
- Sometimes the unit of analysis and the unit of observation in the study differ. In student projects, this is often true as target populations may be too vulnerable to expose to research whose potential harms may outweigh the benefits.
- One’s recruitment method has to match one’s sampling approach, as will be explained in the next chapter.
- Based on what you know right now, how representative of your population are potential participants in the setting?
- How much information can you reasonably know about potential participants before you recruit them?
- Are there gatekeepers and what kinds of concerns might they have?
- Are there any stakeholders that may be beneficial to bring on board as part of your research team for the project?
- What interests might stakeholders and gatekeepers bring to the project and would they align with your vision for the project?
- What ethical issues might you encounter if you sampled people in this setting?
- For the settings you’ve identified, how might you recruit participants?
- Identify your inclusion criteria and exclusion criteria, and assess whether you have enough information on whether people in each setting will meet them.
10.2 Sampling approaches for quantitative research
- Determine whether you will use probability or non-probability sampling, given the strengths and limitations of each specific sampling approach
- Distinguish between approaches to probability sampling and detail the reasons to use each approach
Sampling in quantitative research projects is done because it is not feasible to study the whole population, and researchers hope to take what we learn about a small group of people (your sample) and apply it to a larger population. There are many ways to approach this process, and they can be grouped into two categories—probability sampling and non-probability sampling. Sampling approaches are inextricably linked with recruitment, and researchers should ensure that their proposal’s recruitment strategy matches the sampling approach.
Probability sampling approaches use a random process, usually a computer program, to select participants from the sampling frame so that everyone has an equal chance of being included. It’s important to note that random means the researcher used a process that is truly random . In a project sampling college students, standing outside of the building in which your social work department is housed and surveying everyone who walks past is not random. Because of the location, you are likely to recruit a disproportionately large number of social work students and fewer from other disciplines. Depending on the time of day, you may recruit more traditional undergraduate students, who take classes during the day, or more graduate students, who take classes in the evenings.
In this example, you are actually using non-probability sampling . Another way to say this is that you are using the most common sampling approach for student projects, availability sampling . Also called convenience sampling, this approach simply recruits people who are convenient or easily available to the researcher. If you have ever been asked by a friend to participate in their research study for their class or seen an advertisement for a study on a bulletin board or social media, you were being recruited using an availability sampling approach.
There are a number of benefits to the availability sampling approach. First and foremost, it is less costly and time-consuming for the researcher. As long as the person you are attempting to recruit has knowledge of the topic you are studying, the information you get from the sample you recruit will be relevant to your topic (although your sample may not necessarily be representative of a larger population). Availability samples can also be helpful when random sampling isn’t practical. If you are planning to survey students in an LGBTQ+ support group on campus but attendance varies from meeting to meeting, you may show up at a meeting and ask anyone present to participate in your study. A support group with varied membership makes it impossible to have a real list–or sampling frame–from which to randomly select individuals. Availability sampling would help you reach that population.
Availability sampling is appropriate for student and smaller-scale projects, but it comes with significant limitations. The purpose of sampling in quantitative research is to generalize from a small sample to a larger population. Because availability sampling does not use a random process to select participants, the researcher cannot be sure their sample is representative of the population they hope to generalize to. Instead, the recruitment processes may have been structured by other factors that may bias the sample to be different in some way than the overall population.
So, for instance, if we asked social work students about their level of satisfaction with the services at the student health center, and we sampled in the evenings, we would get most likely get a biased perspective of the issue. Students taking only night classes are much more likely to commute to school, spend less time on campus, and use fewer campus services. Our results would not represent what all social work students feel about the topic. We might get the impression that no social work student had ever visited the health center, when that is not actually true at all. Sampling bias will be discussed in detail in Section 10.3.
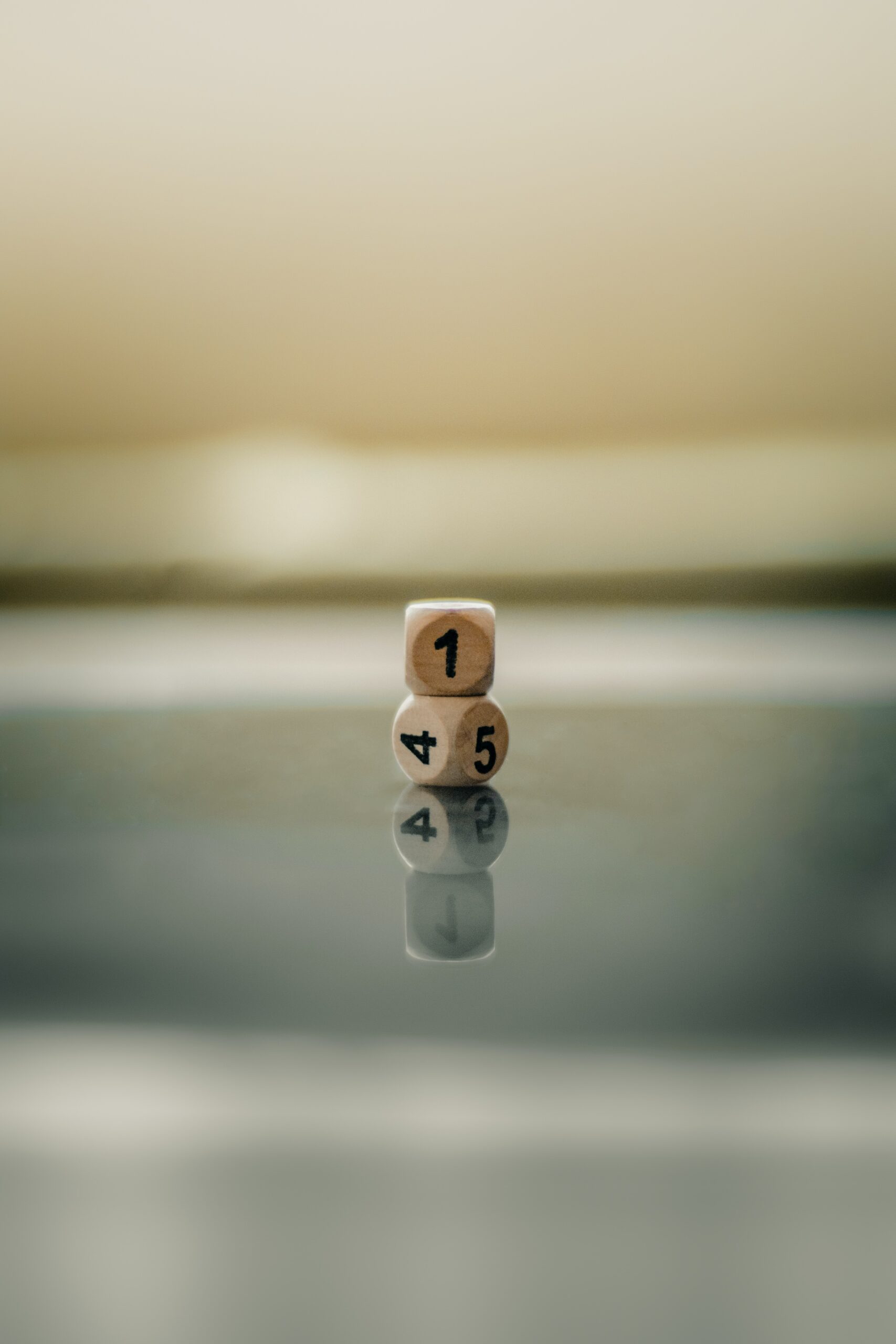
Approaches to probability sampling
What might be a better strategy is getting a list of all email addresses of social work students and randomly selecting email addresses of students to whom you can send your survey. This would be an example of simple random sampling . It’s important to note that you need a real list of people in your sampling frame from which to select your email addresses. For projects where the people who could potentially participate is not known by the researcher, probability sampling is not possible. It is likely that administrators at your school’s registrar would be reluctant to share the list of students’ names and email addresses. Always remember to consider the feasibility and ethical implications of the sampling approach you choose.
Usually, simple random sampling is accomplished by assigning each person, or element , in your sampling frame a number and selecting your participants using a random number generator. You would follow an identical process if you were sampling records or documents as your elements, rather than people. True randomness is difficult to achieve, and it takes complex computational calculations to do so. Although you think you can select things at random, human-generated randomness is actually quite predictable, as it falls into patterns called heuristics . To truly randomly select elements, researchers must rely on computer-generated help. Many free websites have good pseudo-random number generators. A good example is the website Random.org , which contains a random number generator that can also randomize lists of participants. Sometimes, researchers use a table of numbers that have been generated randomly. There are several possible sources for obtaining a random number table. Some statistics and research methods textbooks provide such tables in an appendix.
Though simple, this approach to sampling can be tedious since the researcher must assign a number to each person in a sampling frame. Systematic sampling techniques are somewhat less tedious but offer the benefits of a random sample. As with simple random samples, you must possess a list of everyone in your sampling frame. Once you’ve done that, to draw a systematic sample you’d simply select every k th element on your list. But what is k , and where on the list of population elements does one begin the selection process?
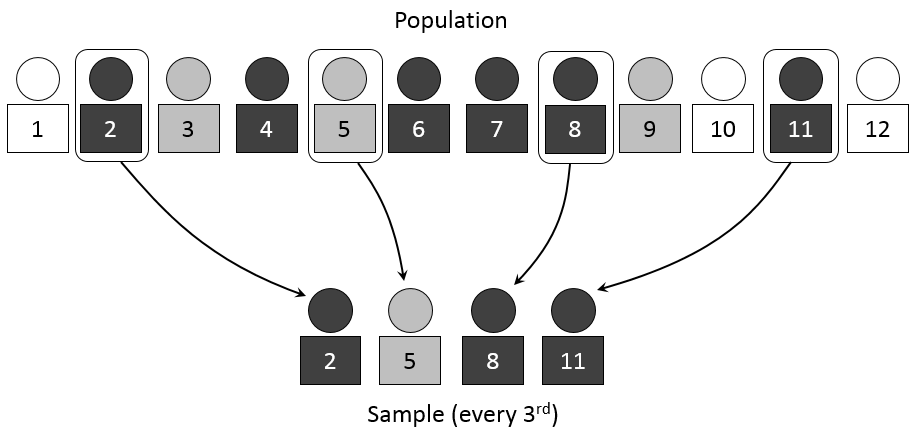
k is your selection interval or the distance between the elements you select for inclusion in your study. To begin the selection process, you’ll need to figure out how many elements you wish to include in your sample. Let’s say you want to survey 25 social work students and there are 100 social work students on your campus. In this case, your selection interval, or k , is 4. To get your selection interval, simply divide the total number of population elements by your desired sample size. Systematic sampling starts by randomly selecting a number between 1 and k to start from, and then recruiting every kth person. In our example, we may start at number 3 and then select the 7th, 11th, 15th (and so forth) person on our list of email addresses. In Figure 10.2, you can see the researcher starts at number 2 and then selects every third person for inclusion in the sample.
There is one clear instance in which systematic sampling should not be employed. If your sampling frame has any pattern to it, you could inadvertently introduce bias into your sample by using a systemic sampling strategy. (Bias will be discussed in more depth in section 10.3.) This is sometimes referred to as the problem of periodicity. Periodicity refers to the tendency for a pattern to occur at regular intervals.
To stray a bit from our example, imagine we were sampling client charts based on the date they entered a health center and recording the reason for their visit. We may expect more admissions for issues related to alcohol consumption on the weekend than we would during the week. The periodicity of alcohol intoxication may bias our sample towards either overrepresenting or underrepresenting this issue, depending on our sampling interval and whether we collected data on a weekday or weekend.
Advanced probability sampling techniques
Returning again to our idea of sampling student email addresses, one of the challenges in our study will be the different types of students. If we are interested in all social work students, it may be helpful to divide our sampling frame, or list of students, into three lists—one for traditional, full-time undergraduate students, another for part-time undergraduate students, and one more for full-time graduate students—and then randomly select from these lists. This is particularly important if we wanted to make sure our sample had the same proportion of each type of student compared with the general population.
This approach is called stratified random sampling . In stratified random sampling, a researcher will divide the study population into relevant subgroups or strata and then draw a sample from each subgroup, or stratum. Strata is the plural of stratum, so it refers to all of the groups while stratum refers to each group. This can be used to make sure your sample has the same proportion of people from each stratum. If, for example, our sample had many more graduate students than undergraduate students, we may draw incorrect conclusions that do not represent what all social work students experience.
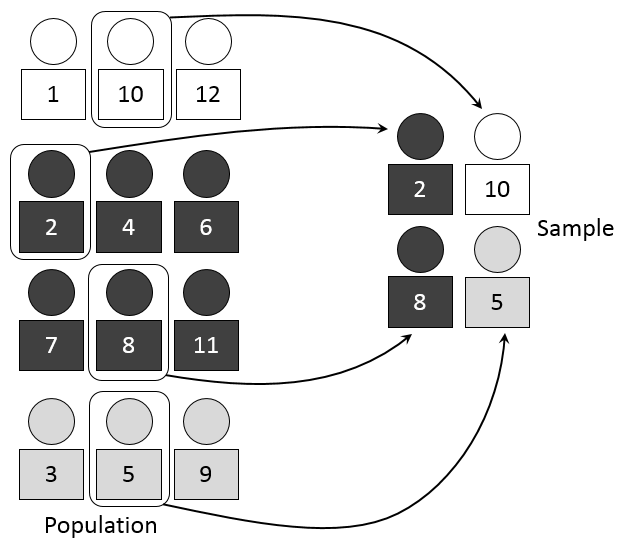
Generally, the goal of stratified random sampling is to recruit a sample that makes sure all elements of the population are included sufficiently that conclusions can be drawn about them. Usually, the purpose is to create a sample that is identical to the overall population along whatever strata you’ve identified. In our sample, it would be graduate and undergraduate students. Stratified random sampling is also useful when a subgroup of interest makes up a relatively small proportion of the overall sample. For example, if your social work program contained relatively few Asian students but you wanted to make sure you recruited enough Asian students to conduct statistical analysis, you could use race to divide people into subgroups or strata and then disproportionately sample from the Asian students to make sure enough of them were in your sample to draw meaningful conclusions. Statistical tests may have a minimum number
Up to this point in our discussion of probability samples, we’ve assumed that researchers will be able to access a list of population elements in order to create a sampling frame. This, as you might imagine, is not always the case. Let’s say, for example, that you wish to conduct a study of health center usage across students at each social work program in your state. Just imagine trying to create a list of every single social work student in the state. Even if you could find a way to generate such a list, attempting to do so might not be the most practical use of your time or resources. When this is the case, researchers turn to cluster sampling. Cluster sampling occurs when a researcher begins by sampling groups (or clusters) of population elements and then selects elements from within those groups.
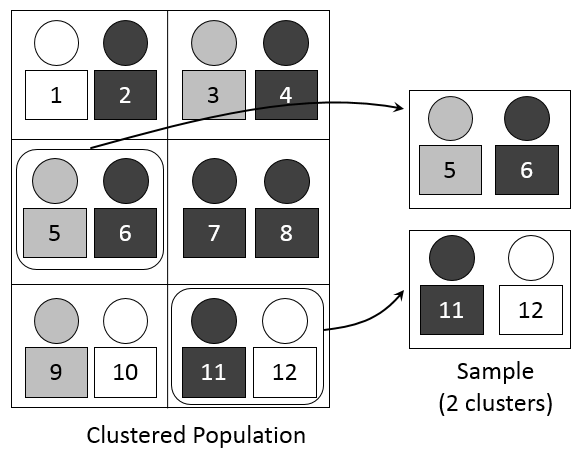
Let’s work through how we might use cluster sampling. While creating a list of all social work students in your state would be next to impossible, you could easily create a list of all social work programs in your state. Then, you could draw a random sample of social work programs (your cluster) and then draw another random sample of elements (in this case, social work students) from each of the programs you randomly selected from the list of all programs.
Cluster sampling often works in stages. In this example, we sampled in two stages—(1) social work programs and (2) social work students at each program we selected. However, we could add another stage if it made sense to do so. We could randomly select (1) states in the United States (2) social work programs in that state and (3) individual social work students. As you might have guessed, sampling in multiple stages does introduce a greater possibility of error. Each stage is subject to its own sampling problems. But, cluster sampling is nevertheless a highly efficient method.
Jessica Holt and Wayne Gillespie (2008) [3] used cluster sampling in their study of students’ experiences with violence in intimate relationships. Specifically, the researchers randomly selected 14 classes on their campus and then drew a random sub-sample of students from those classes. But you probably know from your experience with college classes that not all classes are the same size. So, if Holt and Gillespie had simply randomly selected 14 classes and then selected the same number of students from each class to complete their survey, then students in the smaller of those classes would have had a greater chance of being selected for the study than students in the larger classes. Keep in mind, with random sampling the goal is to make sure that each element has the same chance of being selected. When clusters are of different sizes, as in the example of sampling college classes, researchers often use a method called probability proportionate to size (PPS). This means that they take into account that their clusters are of different sizes. They do this by giving clusters different chances of being selected based on their size so that each element within those clusters winds up having an equal chance of being selected.
To summarize, probability samples allow a researcher to make conclusions about larger groups. Probability samples require a sampling frame from which elements, usually human beings, can be selected at random from a list. The use of random selection reduces the error and bias present in non-probability samples, which we will discuss in greater detail in section 10.3, though some error will always remain. In relying on a random number table or generator, researchers can more accurately state that their sample represents the population from which it was drawn. This strength is common to all probability sampling approaches summarized in Table 10.2.
In determining which probability sampling approach makes the most sense for your project, it helps to know more about your population. A simple random sample and systematic sample are relatively similar to carry out. They both require a list all elements in your sampling frame. Systematic sampling is slightly easier in that it does not require you to use a random number generator, instead using a sampling interval that is easy to calculate by hand.
However, the relative simplicity of both approaches is counterweighted by their lack of sensitivity to characteristics of your population. Stratified samples can better account for periodicity by creating strata that reduce or eliminate its effects. Stratified sampling also ensure that smaller subgroups are included in your sample, thereby making your sample more representative of the overall population. While these benefits are important, creating strata for this purpose requires having information about your population before beginning the sampling process. In our social work student example, we would need to know which students are full-time or part-time, graduate or undergraduate, in order to make sure our sample contained the same proportions. Would you know if someone was a graduate student or part-time student, just based on their email address? If the true population parameters are unknown, stratified sampling becomes significantly more challenging.
Common to each of the previous probability sampling approaches is the necessity of using a real list of all elements in your sampling frame. Cluster sampling is different. It allows a researcher to perform probability sampling in cases for which a list of elements is not available or feasible to create. Cluster sampling is also useful for making claims about a larger population (in our previous example, all social work students within a state). However, because sampling occurs at multiple stages in the process, (in our previous example, at the university and student level), sampling error increases. For many researchers, the benefits of cluster sampling outweigh this weaknesses.
Matching recruitment and sampling approach
Recruitment must match the sampling approach you choose in section 10.2. For many students, that will mean using recruitment techniques most relevant to availability sampling. These may include public postings such as flyers, mass emails, or social media posts. However, these methods would not make sense for a study using probability sampling. Probability sampling requires a list of names or other identifying information so you can use a random process to generate a list of people to recruit into your sample. Posting a flyer or social media message means you don’t know who is looking at the flyer, and thus, your sample could not be randomly drawn. Probability sampling often requires knowing how to contact specific participants. For example, you may do as I did, and contact potential participants via phone and email. Even then, it’s important to note that not everyone you contact will enter your study. We will discuss more about evaluating the quality of your sample in section 10.3.
- Probability sampling approaches are more accurate when the researcher wants to generalize from a smaller sample to a larger population. However, non-probability sampling approaches are often more feasible. You will have to weigh advantages and disadvantages of each when designing your project.
- There are many kinds of probability sampling approaches, though each require you know some information about people who potentially would participate in your study.
- Probability sampling also requires that you assign people within the sampling frame a number and select using a truly random process.
- Identify one of the sampling approaches listed in this chapter that might be appropriate to answering your question and list the strengths and limitations of it.
- Describe how you will recruit your participants and how your plan makes sense with the sampling approach you identified.
- Examine one of the empirical articles from your literature review. Identify what sampling approach they used and how they carried it out from start to finish.
10.3 Sample quality
- Assess whether your sampling plan is likely to produce a sample that is representative of the population you want to draw conclusions about
- Identify the considerations that go into producing a representative sample and determining sample size
- Distinguish between error and bias in a sample and explain the factors that lead to each
Okay, so you’ve chosen where you’re going to get your data (setting), what characteristics you want and don’t want in your sample (inclusion/exclusion criteria), and how you will select and recruit participants (sampling approach and recruitment). That means you are done, right? (I mean, there’s an entire section here, so probably not.) Even if you make good choices and do everything the way you’re supposed to, you can still draw a poor sample. If you are investigating a research question using quantitative methods, the best choice is some kind of probability sampling, but aside from that, how do you know a good sample from a bad sample? As an example, we’ll use a bad sample I collected as part of a research project that didn’t go so well. Hopefully, your sampling will go much better than mine did, but we can always learn from what didn’t work.
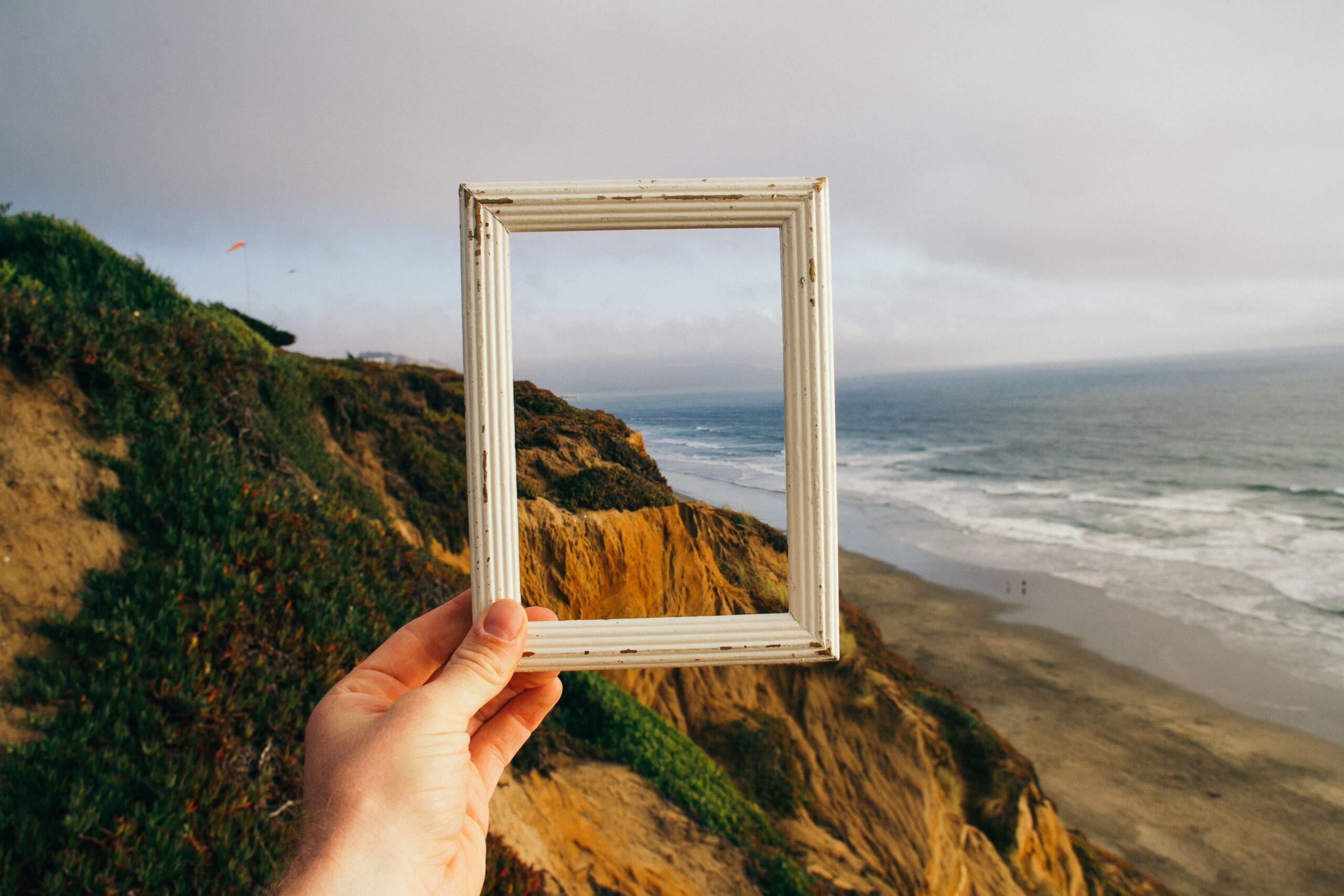
Representativeness
A representative sample is, “a sample that looks like the population from which it was selected in all respects that are potentially relevant to the study” (Engel & Schutt, 2011). [4] For my study on how much it costs to get an LCSW in each state, I did not get a sample that looked like the overall population to which I wanted to generalize. My sample had a few states with more than ten responses and most states with no responses. That does not look like the true distribution of social workers across the country. I could compare the number of social workers in each state, based on data from the National Association of Social Workers, or the number of recent clinical MSW graduates from the Council on Social Work Education. More than that, I could see whether my sample matched the overall population of clinical social workers in gender, race, age, or any other important characteristics. Sadly, it wasn’t even close. So, I wasn’t able to use the data to publish a report.
- Will the sample of people (or documents) look like the population to which you want to generalize?
- Specifically, what characteristics are important in determining whether a sample is representative of the population? How do these characteristics relate to your research question?
- Consider returning to this question once you have completed the sampling process and evaluate whether the sample in your study was similar to what you designed in this section.
Many of my students erroneously assume that using a probability sampling technique will guarantee a representative sample. This is not true. Engel and Schutt (2011) identify that probability sampling increases the chance of representativeness; however, it does not guarantee that the sample will be representative. If a representative sample is important to your study, it would be best to use a sampling approach that allows you to control the proportion of specific characteristics in your sample. For instance, stratified random sampling allows you to control the distribution of specific variables of interest within your sample. However, that requires knowing information about your participants before you hand them surveys or expose them to an experiment.
In my study, if I wanted to make sure I had a certain number of people from each state (state being the strata), making the proportion of social workers from each state in my sample similar to the overall population, I would need to know which email addresses were from which states. That was not information I had. So, instead I conducted simple random sampling and randomly selected 5,000 of 100,000 email addresses on the NASW list. There was less of a guarantee of representativeness, but whatever variation existed between my sample and the population would be due to random chance. This would not be true for an availability or convenience sample. While these sampling approaches are common for student projects, they come with significant limitations in that variation between the sample and population is due to factors other than chance. We will discuss these non-random differences later in the chapter when we talk about bias. For now, just remember that the representativeness of a sample is helped by using random sampling, though it is not a guarantee.
- Before you start sampling, do you know enough about your sampling frame to use stratified random sampling, which increases the potential of getting a representative sample?
- Do you have enough information about your sampling frame to use another probability sampling approach like simple random sampling or cluster sampling?
- If little information is available on which to select people, are you using availability sampling? Remember that availability sampling is okay if it is the only approach that is feasible for the researcher, but it comes with significant limitations when drawing conclusions about a larger population.
Assessing representativeness should start prior to data collection. I mentioned that I drew my sample from the NASW email list, which (like most organizations) they sell to advertisers when companies or researchers need to advertise to social workers. How representative of my population is my sampling frame? Well, the first question to ask is what proportion of my sampling frame would actually meet my exclusion and inclusion criteria. Since my study focused specifically on clinical social workers, my sampling frame likely included social workers who were not clinical social workers, like macro social workers or social work managers. However, I knew, based on the information from NASW marketers, that many people who received my recruitment email would be clinical social workers or those working towards licensure, so I was satisfied with that. Anyone who didn’t meet my inclusion criteria and opened the survey would be greeted with clear instructions that this survey did not apply to them.
At the same time, I should have assessed whether the demographics of the NASW email list and the demographics of clinical social workers more broadly were similar. Unfortunately, this was not information I could gather. I had to trust that this was likely to going to be the best sample I could draw and the most representative of all social workers.
- Before you start, what do you know about your setting and potential participants?
- You want to avoid throwing out half of the surveys you get back because the respondents aren’t a part of your target population. This is a common error I see in student proposals.
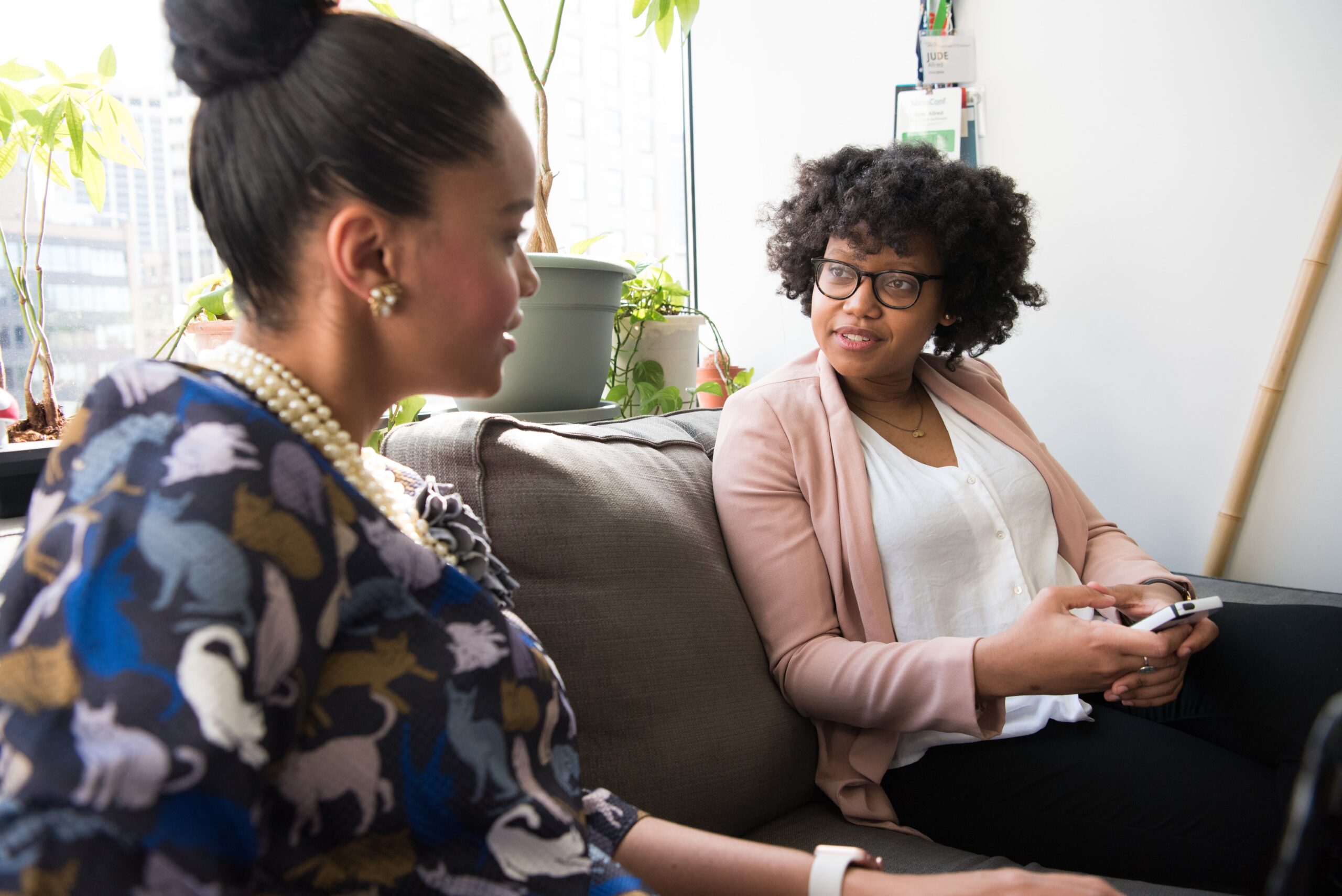
Many of you will sample people from your agency, like clients or staff. Let’s say you work for a children’s mental health agency, and you wanted to study children who have experienced abuse. Walking through the steps here might proceed like this:
- Think about or ask your coworkers how many of the clients at your agency have experienced this issue. If it’s common, then clients at your agency would probably make a good sampling frame for your study. If not, then you may want to adjust your research question or consider a different agency to sample. You could also change your target population to be more representative with your sample. For example, while your agency’s clients may not be representative of all children who have survived abuse, they may be more representative of abuse survivors in your state, region, or county. In this way, you can draw conclusions about a smaller population, rather than everyone in the world who is a victim of child abuse.
- Think about those characteristics that are important for individuals in your sample to have or not have. Obviously, the variables in your research question are important, but so are the variables related to it. Take a look at the empirical literature on your topic. Are there different demographic characteristics or covariates that are relevant to your topic?
- All of this assumes that you can actually access information about your sampling frame prior to collecting data. This is a challenge in the real world. Even if you ask around your office about client characteristics, there is no way for you to know for sure until you complete your study whether it was the most representative sampling frame you could find. When in doubt, go with whatever is feasible and address any shortcomings in sampling within the limitations section of your research report. A good project is a done project.
- While using a probability sampling approach helps with sample representativeness, it does not guarantee it. Due to random variation, samples may differ across important characteristics. If you can feasibly use a probability sampling approach, particularly stratified random sampling, it will help make your sample more representative of the population.
- Even if you choose a sampling frame that is representative of your population and use a probability sampling approach, there is no guarantee that the sample you are able to collect will be representative. Sometimes, people don’t respond to your recruitment efforts. Other times, random chance will mean people differ on important characteristics from your target population. ¯\_(ツ)_/¯
In agency-based samples, the small size of the pool of potential participants makes it very likely that your sample will not be representative of a broader target population. Sometimes, researchers look for specific outcomes connected with sub-populations for that reason. Not all agency-based research is concerned with representativeness, and it is still worthwhile to pursue research that is relevant to only one location as its purpose is often to improve social work practice.
Sample size
Let’s assume you have found a representative sampling frame, and that you are using one of the probability sampling approaches we reviewed in section 10.2. That should help you recruit a representative sample, but how many people do you need to recruit into your sample? As with many questions about sample quality, students should keep feasibility in mind. The easiest answer I’ve given as a professor is, “as many as you can, without hurting yourself.” While your quantitative research question would likely benefit from hundreds or thousands of respondents, that is not likely to be feasible for a student who is working full-time, interning part-time, and in school full-time. Don’t feel like your study has to be perfect, but make sure you note any limitations in your final report.
To the extent possible, you should gather as many people as you can in your sample who meet your criteria. But why? Let’s think about an example you probably know well. Have you ever watched the TV show Family Feud ? Each question the host reads off starts with, “we asked 100 people…” Believe it or not, Family Feud uses simple random sampling to conduct their surveys the American public. Part of the challenge on Family Feud is that people can usually guess the most popular answers, but those answers that only a few people chose are much harder. They seem bizarre, and are more difficult to guess. That’s because 100 people is not a lot of people to sample. Essentially, Family Feud is trying to measure what the answer is for all 327 million people in the United States by asking 100 of them. As a result, the weird and idiosyncratic responses of a few people are likely to remain on the board as answers, and contestants have to guess answers fewer and fewer people in the sample provided. In a larger sample, the oddball answers would likely fade away and only the most popular answers would be represented on the game show’s board.
In my ill-fated study of clinical social workers, I received 87 complete responses. That is far below the hundred thousand licensed or license-eligible clinical social workers. Moreover, since I wanted to conduct state-by-state estimates, there was no way I had enough people in each state to do so. For student projects, samples of 50-100 participants are more than enough to write a paper (or start a game show), but for projects in the real world with real-world consequences, it is important to recruit the appropriate number of participants. For example, if your agency conducts a community scan of people in your service area on what services they need, the results will inform the direction of your agency, which grants they apply for, who they hire, and its mission for the next several years. Being overly confident in your sample could result in wasted resources for clients.
So what is the right number? Theoretically, we could gradually increase the sample size so that the sample approaches closer and closer to the total size of the population (Bhattacherjeee, 2012). [5] But as we’ve talked about, it is not feasible to sample everyone. How do we find that middle ground? To answer this, we need to understand the sampling distribution . Imagine in your agency’s survey of the community, you took three different probability samples from your community, and for each sample, you measured whether people experienced domestic violence. If each random sample was truly representative of the population, then your rate of domestic violence from the three random samples would be about the same and equal to the true value in the population.
But this is extremely unlikely, given that each random sample will likely constitute a different subset of the population, and hence, the rate of domestic violence you measure may be slightly different from sample to sample. Think about the sample you collect as existing on a distribution of infinite possible samples. Most samples you collect will be close to the population mean but many will not be. The degree to which they differ is associated with how much the subject you are sampling about varies in the population. In our example, samples will vary based on how varied the incidence of domestic violence is from person to person. The difference between the domestic violence rate we find and the rate for our overall population is called the sampling error .
An easy way to minimize sampling error is to increase the number of participants in your sample, but in actuality, minimizing sampling error relies on a number of factors outside of the scope of a basic student project. You can see this online textbook for more examples on sampling distributions or take an advanced methods course at your university, particularly if you are considering becoming a social work researcher. Increasing the number of people in your sample also increases your study’s power , or the odds you will detect a significant relationship between variables when one is truly present in your sample. If you intend on publishing the findings of your student project, it is worth using a power analysis to determine the appropriate sample size for your project. You can follow this excellent video series from the Center for Open Science on how to conduct power analyses using free statistics software. A faculty members who teaches research or statistics could check your work. You may be surprised to find out that there is a point at which you adding more people to your sample will not make your study any better.
Honestly, I did not do a power analysis for my study. Instead, I asked for 5,000 surveys with the hope that 1,000 would come back. Given that only 87 came back, a power analysis conducted after the survey was complete would likely to reveal that I did not have enough statistical power to answer my research questions. For your projects, try to get as many respondents as you feasibly can, but don’t worry too much about not reaching the optimal amount of people to maximize the power of your study unless you goal is to publish something that is generalizable to a large population.
A final consideration is which statistical test you plan to use to analyze your data. We have not covered statistics yet, though we will provide a brief introduction to basic statistics in this textbook. For now, remember that some statistical tests have a minimum number of people that must be present in the sample in order to conduct the analysis. You will complete a data analysis plan before you begin your project and start sampling, so you can always increase the number of participants you plan to recruit based on what you learn in the next few chapters.
- How many people can you feasibly sample in the time you have to complete your project?
One of the interesting things about surveying professionals is that sometimes, they email you about what they perceive to be a problem with your study. I got an email from a well-meaning participant in my LCSW study saying that my results were going to be biased! She pointed out that respondents who had been in practice a long time, before clinical supervision was required, would not have paid anything for supervision. This would lead me to draw conclusions that supervision was cheap, when in fact, it was expensive. My email back to her explained that she hit on one of my hypotheses, that social workers in practice for a longer period of time faced fewer costs to becoming licensed. Her email reinforced that I needed to account for the impact of length of practice on the costs of licensure I found across the sample. She was right to be on the lookout for bias in the sample.
One of the key questions you can ask is if there is something about your process that makes it more likely you will select a certain type of person for your sample, making it less representative of the overall population. In my project, it’s worth thinking more about who is more likely to respond to an email advertisement for a research study. I know that my work email and personal email filter out advertisements, so it’s unlikely I would even see the recruitment for my own study (probably something I should have thought about before using grant funds to sample the NASW email list). Perhaps an older demographic that does not screen advertisements as closely, o r those whose NASW account was linked to a personal email with fewer junk filters would be more likely to respond. To the extent I made conclusions about clinical social workers of all ages based on a sample that was biased towards older social workers, my results would be biased. This is called selection bias , or the degree to which people in my sample differ from the overall population.
Another potential source of bias here is nonresponse bias . Because people do not often respond to email advertisements (no matter how well-written they are), my sample is likely to be representative of people with characteristics that make them more likely to respond. They may have more time on their hands to take surveys and respond to their junk mail. To the extent that the sample is comprised of social workers with a lot of time on their hands (who are those people?) my sample will be biased and not representative of the overall population.
It’s important to note that both bias and error describe how samples differ from the overall population. Error describes random variations between samples, due to chance. Using a random process to recruit participants into a sample means you will have random variation between the sample and the population. Bias creates variance between the sample and population in a specific direction, such as towards those who have time to check their junk mail. Bias may be introduced by the sampling method used or due to conscious or unconscious bias introduced by the researcher (Rubin & Babbie, 2017). [6] A researcher might select people who “look like good research participants,” in the process transferring their unconscious biases to their sample. They might exclude people from the sampling from who “would not do well with the intervention.” Careful researchers can avoid these, but unconscious and structural biases can be challenging to root out.
- Identify potential sources of bias in your sample and brainstorm ways you can minimize them, if possible.
Critical considerations
Think back to you undergraduate degree. Did you ever participate in a research project as part of an introductory psychology or sociology course? Social science researchers on college campuses have a luxury that researchers elsewhere may not share—they have access to a whole bunch of (presumably) willing and able human guinea pigs. But that luxury comes at a cost—sample representativeness. One study of top academic journals in psychology found that over two-thirds (68%) of participants in studies published by those journals were based on samples drawn in the United States (Arnett, 2008). [7] Further, the study found that two-thirds of the work that derived from US samples published in the Journal of Personality and Social Psychology was based on samples made up entirely of American undergraduate students taking psychology courses.
These findings certainly raise the question: What do we actually learn from social science studies and about whom do we learn it? That is exactly the concern raised by Joseph Henrich and colleagues (Henrich, Heine, & Norenzayan, 2010), [8] authors of the article “The Weirdest People in the World?” In their piece, Henrich and colleagues point out that behavioral scientists very commonly make sweeping claims about human nature based on samples drawn only from WEIRD (Western, Educated, Industrialized, Rich, and Democratic) societies, and often based on even narrower samples, as is the case with many studies relying on samples drawn from college classrooms. As it turns out, robust findings about the nature of human behavior when it comes to fairness, cooperation, visual perception, trust, and other behaviors are based on studies that excluded participants from outside the United States and sometimes excluded anyone outside the college classroom (Begley, 2010). [9] This certainly raises questions about what we really know about human behavior as opposed to US resident or US undergraduate behavior. Of course, not all research findings are based on samples of WEIRD folks like college students. But even then, it would behoove us to pay attention to the population on which studies are based and the claims being made about those to whom the studies apply.
Another thing to keep in mind is that just because a sample may be representative in all respects that a researcher thinks are relevant, there may be relevant aspects that didn’t occur to the researcher when she was drawing her sample. You might not think that a person’s phone would have much to do with their voting preferences, for example. But had pollsters making predictions about the results of the 2008 presidential election not been careful to include both cell phone-only and landline households in their surveys, it is possible that their predictions would have underestimated Barack Obama’s lead over John McCain because Obama was much more popular among cell phone-only users than McCain (Keeter, Dimock, & Christian, 2008). [10] This is another example of bias.
Putting it All Together
So how do we know how good our sample is or how good the samples gathered by other researchers are? While there might not be any magic or always-true rules we can apply, there are a couple of things we can keep in mind as we read the claims researchers make about their findings.
First, remember that sample quality is determined only by the sample actually obtained, not by the sampling method itself. A researcher may set out to administer a survey to a representative sample by correctly employing a random sampling approach with impeccable recruitment materials. But, if only a handful of the people sampled actually respond to the survey, the researcher should not make claims like their sample went according to plan.
Another thing to keep in mind, as demonstrated by the preceding discussion, is that researchers may be drawn to talking about implications of their findings as though they apply to some group other than the population actually sampled. Whether the sampling frame does not match the population or the sample and population differ on important criteria, the resulting sampling error can lead to bad science.
We’ve talked previously about the perils of generalizing social science findings from graduate students in the United States and other Western countries to all cultures in the world, imposing a Western view as the right and correct view of the social world. As consumers of theory and research, it is our responsibility to be attentive to this sort of (likely unintentional) bait and switch. And as researchers, it is our responsibility to make sure that we only make conclusions from samples that are representative. A larger sample size and probability sampling can improve the representativeness and generalizability of the study’s findings to larger populations, though neither are guarantees.
Finally, keep in mind that a sample allowing for comparisons of theoretically important concepts or variables is certainly better than one that does not allow for such comparisons. In a study based on a nonrepresentative sample, for example, we can learn about the strength of our social theories by comparing relevant aspects of social processes. We talked about this as theory-testing in Chapter 5.
At their core, questions about sample quality should address who has been sampled, how they were sampled, and for what purpose they were sampled. Being able to answer those questions will help you better understand, and more responsibly interpret, research results. For your study, keep the following questions in mind.
- Are your sample size and your sampling approach appropriate for your research question?
- How much do you know about your sampling frame ahead of time? How will that impact the feasibility of different sampling approaches?
- What gatekeepers and stakeholders are necessary to engage in order to access your sampling frame?
- Are there any ethical issues that may make it difficult to sample those who have first-hand knowledge about your topic?
- Does your sampling frame look like your population along important characteristics? Once you get your data, ask the same question of the sample you successfully recruit.
- What about your population might make it more difficult or easier to sample?
- Are there steps in your sampling procedure that may bias your sample to render it not representative of the population?
- If you want to skip sampling altogether, are there sources of secondary data you can use? Or might you be able to answer you questions by sampling documents or media, rather than people?
- The sampling plan you implement should have a reasonable likelihood of producing a representative sample. Student projects are given more leeway with nonrepresentative samples, and this limitation should be discussed in the student’s research report.
- Researchers should conduct a power analysis to determine sample size, though quantitative student projects should endeavor to recruit as many participants as possible. Sample size impacts representativeness of the sample, its power, and which statistical tests can be conducted.
- The sample you collect is one of an infinite number of potential samples that could have been drawn. To the extent the data in your sample varies from the data in the entire population, it includes some error or bias. Error is the result of random variations. Bias is systematic error that pushes the data in a given direction.
- Even if you do everything right, there is no guarantee that you will draw a good sample. Flawed samples are okay to use as examples in the classroom, but the results of your research would have limited applicability to the community and society.
- Historically, samples were drawn from dominant groups and generalized to all people. This shortcoming is a limitation of some social science literature and should be considered a colonialist scientific practice.
- I clearly need a snack. ↵
- Johnson, P. S., & Johnson, M. W. (2014). Investigation of “bath salts” use patterns within an online sample of users in the United States. Journal of psychoactive drugs , 46 (5), 369-378. ↵
- Holt, J. L., & Gillespie, W. (2008). Intergenerational transmission of violence, threatened egoism, and reciprocity: A test of multiple psychosocial factors affecting intimate partner violence. American Journal of Criminal Justice, 33 , 252–266. ↵
- Engel, R. & Schutt (2011). The practice of research in social work (2nd ed.) . California: SAGE ↵
- Bhattacherjee, A. (2012). Social science research: Principles, methods, and practices . Retrieved from: https://scholarcommons.usf.edu/cgi/viewcontent.cgi?article=1002&context=oa_textbooks ↵
- Rubin, C. & Babbie, S. (2017). Research methods for social work (9th edition) . Boston, MA: Cengage. ↵
- Arnett, J. J. (2008). The neglected 95%: Why American psychology needs to become less American. American Psychologist , 63, 602–614. ↵
- Henrich, J., Heine, S. J., & Norenzayan, A. (2010). The weirdest people in the world? Behavioral and Brain Sciences , 33, 61–135. ↵
- Newsweek magazine published an interesting story about Henrich and his colleague’s study: Begley, S. (2010). What’s really human? The trouble with student guinea pigs. Retrieved from http://www.newsweek.com/2010/07/23/what-s-really-human.html ↵
- Keeter, S., Dimock, M., & Christian, L. (2008). Calling cell phones in ’08 pre-election polls. The Pew Research Center for the People and the Press . Retrieved from http://people-press.org/files/legacy-pdf/cell-phone-commentary.pdf ↵
entity that a researcher wants to say something about at the end of her study (individual, group, or organization)
the entities that a researcher actually observes, measures, or collects in the course of trying to learn something about her unit of analysis (individuals, groups, or organizations)
the larger group of people you want to be able to make conclusions about based on the conclusions you draw from the people in your sample
the list of people from which a researcher will draw her sample
the people or organizations who control access to the population you want to study
Inclusion criteria are general requirements a person must possess to be a part of your sample.
characteristics that disqualify a person from being included in a sample
the process by which the researcher informs potential participants about the study and attempts to get them to participate
the group of people you successfully recruit from your sampling frame to participate in your study
sampling approaches for which a person’s likelihood of being selected from the sampling frame is known
sampling approaches for which a person’s likelihood of being selected for membership in the sample is unknown
researcher gathers data from whatever cases happen to be convenient or available
(as in generalization) to make claims about a large population based on a smaller sample of people or items
selecting elements from a list using randomly generated numbers
the units in your sampling frame, usually people or documents
selecting every kth element from your sampling frame
the tendency for a pattern to occur at regular intervals
dividing the study population into subgroups based on a characteristic (or strata) and then drawing a sample from each subgroup
the characteristic by which the sample is divided in stratified random sampling
a sampling approach that begins by sampling groups (or clusters) of population elements and then selects elements from within those groups
in cluster sampling, giving clusters different chances of being selected based on their size so that each element within those clusters has an equal chance of being selected
"a sample that looks like the population from which it was selected in all respects that are potentially relevant to the study" (Engel & Schutt, 2011)
the set of all possible samples you could possibly draw for your study
the odds you will detect a significant relationship between variables when one is truly present in your sample
The bias that occurs when those who respond to your request to participate in a study are different from those who do not respond to you request to participate in a study.
Graduate research methods in social work Copyright © 2020 by Matthew DeCarlo, Cory Cummings, Kate Agnelli is licensed under a Creative Commons Attribution-NonCommercial-ShareAlike 4.0 International License , except where otherwise noted.
Share This Book
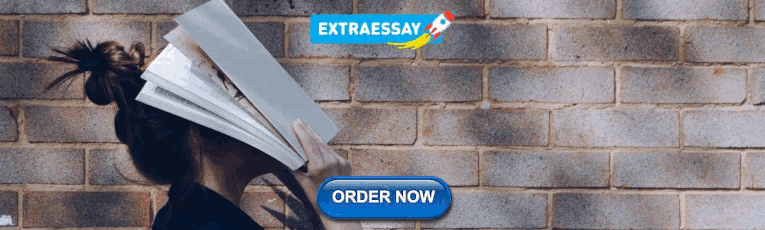
IMAGES
VIDEO
COMMENTS
Example: Sampling frame You are doing research on working conditions at a social media marketing company. Your population is all 1000 employees of the company. ... It is mainly used in quantitative research. If you want to produce results that are representative of the whole population, probability sampling techniques are the most valid choice.
Probability-based sampling methods are most commonly used in quantitative research, especially when it's important to achieve a representative sample that allows the researcher to generalise their findings. Non-probability sampling, on the other hand, refers to sampling methods in which the selection of participants is not statistically random.
The sampling technique in quantitative research comes from its ability to draw small units of the population (i.e., sample size) and generalize it to the population (Seddon & Scheepers, 2012).In a study, specifically in behavioural research where the number of population elements is too large, collecting data from every element of a population is unreal.
Sample size calculation. In order to enable comparisons with some level of established statistical confidence, quantitative research needs an acceptable sample size. 2 The sample size is the most crucial factor for reliability (reproducibility) in quantitative research. It is important for a study to be powered - the likelihood of identifying a difference if it exists in reality. 2 Small ...
1. Simple random sampling. In a simple random sample, every member of the population has an equal chance of being selected. Your sampling frame should include the whole population. To conduct this type of sampling, you can use tools like random number generators or other techniques that are based entirely on chance.
Chapter 8: Quantitative Sampling I. Introduction to Sampling a. The primary goal of sampling is to get a representative sample, or a small collection of units or cases from a much larger collection or population, such that the researcher can study the smaller group and produce accurate generalizations about the larger group. Researchers
Obtaining Samples for Population Generalizability. In quantitative research, a population is the entire group that the researcher wants to draw conclusions about.. A sample is the specific group that the researcher will actually collect data from. A sample is always a much smaller group of people than the total size of the population.
Purposive sampling Example: ... The data collected is quantitative and statistical analyses are used to draw conclusions. Purpose of Sampling Methods. The main purpose of sampling methods in research is to obtain a representative sample of individuals or elements from a larger population of interest, in order to make inferences about the ...
Cluster sampling- she puts 50 into random groups of 5 so we get 10 groups then randomly selects 5 of them and interviews everyone in those groups --> 25 people are asked. 2. Stratified sampling- she puts 50 into categories: high achieving smart kids, decently achieving kids, mediumly achieving kids, lower poorer achieving kids and clueless ...
Availability sampling is appropriate for student and smaller-scale projects, but it comes with significant limitations. The purpose of sampling in quantitative research is to generalize from a small sample to a larger population. Because availability sampling does not use a random process to select participants, the researcher cannot be sure ...
Here are two quantitative research examples: ... The following data collection methods are commonly used in primary quantitative research: Sampling: The most common type is probability sampling, in which a sample is chosen from a larger population using some form of random selection, that is, every member of the population has an equal chance ...
The quantitative research sampling method is the process of selecting representable units from a large population. Quantitative research refers to the analysis wherein mathematical, statistical, or computational method is used for studying the measurable or quantifiable dataset. The core purpose of quantitative research is the generalization of ...
This is referred to as sampling error, a statistical calculation of the difference between results from a sample and the actual parameters of a population. Generalizability is a pretty easy concept to grasp. Imagine a professor were to take a sample of individuals in your class to see if the material is too hard or too easy.
Another type of sampling discussed by some authors is "systematic random sample.". The steps for this method are: Make a list of all the potential recruits. Using a random method (described earlier) to select a starting point (example number 4) Select this number and every fifth number from this starting point.
After defining research objectives, the next significant step in primary quantitative research is data collection. This involves using two main methods: sampling and conducting surveys or polls. Sampling methods: In quantitative research, there are two primary sampling methods: Probability and Non-probability sampling.
INTRODUCTION. Scientific research is usually initiated by posing evidenced-based research questions which are then explicitly restated as hypotheses.1,2 The hypotheses provide directions to guide the study, solutions, explanations, and expected results.3,4 Both research questions and hypotheses are essentially formulated based on conventional theories and real-world processes, which allow the ...
The essential topics related to the selection of participants for a health research are: 1) whether to work with samples or include the whole reference population in the study (census); 2) the sample basis; 3) the sampling process and 4) the potential effects nonrespondents might have on study results. We will refer to each of these aspects ...
The sampling process (25 minute read time); Sampling approaches for quantitative research (15 minute read time); Sample quality (24 minute read time); Content warning: examples contain references to addiction to technology, domestic violence and batterer intervention, cancer, illegal drug use, LGBTQ+ discrimination, binge drinking, intimate partner violence among college students, child abuse ...