Advertisement
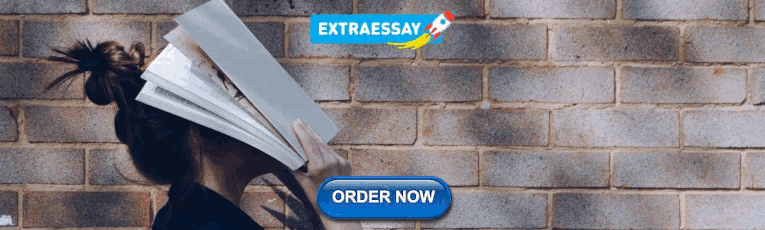
Using Social Media to Predict the Stock Market Crash and Rebound amid the Pandemic: The Digital ‘Haves’ and ‘Have-mores’
- Published: 15 September 2021
- Volume 9 , pages 5–31, ( 2022 )
Cite this article
- Chong Guan ORCID: orcid.org/0000-0002-7827-1498 1 ,
- Wenting Liu 2 &
- Jack Yu-Chao Cheng 3
8132 Accesses
17 Citations
2 Altmetric
Explore all metrics
Since the 2019 novel Coronavirus disease (COVID-19) spread across the globe, risks brought by the pandemic set in and stock markets tumbled worldwide. Amidst the bleak economic outlook, investors’ concerns over the pandemic spread rapidly through social media but wore out shortly. Similarly, the crash only caused a relatively short-lived bear market, which bottomed out and recovered quickly. Meanwhile, technology stocks have grabbed the spotlight as the digitally advanced sectors seemed to show resilience in this Coronavirus-plagued market. This paper aims to examine market sentiments using social media to predict the stock market performance before, during and after the March 2020 stock market crash. In addition, using the Organisation for Economic Co-operation and Development Taxonomy of Sectoral Digital-intensity Framework, we identified market sectors that have outperformed others as the market sentiment was impacted by the unfolding of the pandemic. The daily stock performance of a usable sample of 1619 firms from 34 sectors was first examined via a combination of hierarchical clustering and shape-based distance measure. This was then tested against a time series of daily price changes through augmented vector auto-regression. Results show that market sentiments towards the pandemic have significantly impacted the price differences. More interestingly, the stock performance across sectors is characterized by the level of digital intensity, with the most digitally advanced sectors demonstrating resilience against negative market sentiments on the pandemic. This research is among the first to demonstrate how digital intensity mitigates the negative effect of a crisis on stock market performance.
Similar content being viewed by others
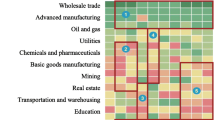
Building stock market resilience through digital transformation: using Google trends to analyze the impact of COVID-19 pandemic
Sensitivity of Stock Pricing to the Optimistic and Pessimistic Sentiment of Social Media: A Shreds of Evidence from Nifty Indices
Novel Method to Analyze and Forecast Social Impact on Macro- and Micro-Economies Using Social Media Data
Avoid common mistakes on your manuscript.
1 Introduction
The outbreak of 2019 novel Coronavirus disease (COVID-19) has brought widespread risks and investor concerns worldwide, with the stock prices in all major stock markets seeing sudden and unprecedented fall. The Coronavirus stock market crash was among the fastest falls in financial history [ 1 ]. Amidst the bleak economic outlook, investors’ concerns over the pandemic seemed to spread rapidly through social media but wore out shortly after that. Social media data measure people’s attention on unexpected incidents and can serve as a timely indicator that drives investment dynamics. For example, salient patterns exhibited in social media have been employed as an unconventional source of strategic information to predict stock market movements [ 2 ]. In fact, like the sudden surge and decline in social media postings on the pandemic, the crash only caused a relatively short-lived bear market, which bottomed out and recovered quickly.
Meanwhile, many countries have imposed lockdown measures in attempts to slow the spread of the life-threatening virus, from mandatory geographic quarantines, non-mandatory recommendations to working from home, closures of certain types of business entities, or bans on mass events and gatherings. These measures have brought those that are categorized as ‘non-essential’ brick-and-mortar business activities close to complete standstill. However, reports have suggested that even as most corporeal business activities halted, trade and consumption continued to grow online as the virus peaked [ 3 ]. This is also well reflected in the financial markets—technology stocks have grabbed the spotlight as the digitally intensive sectors seemed to show resilience in this Coronavirus-plagued market. Changes in consumer habits—an accelerated embrace of e-commerce, home entertainment and working from home [ 4 ]—have strengthened the already dominant positions of a few mega-cap technology companies, such as Amazon.com, PayPal and Alphabet, Google's parent company[ 5 ].
Data science, coupled with computational biology, is helping in myriad ways with applications including epidemiology, drug discovery, and molecular design for diagnostic and therapeutic purposes [ 6 , 7 , 8 ]. A number of data driven models, mathematical models, and predictive models have been developed for COVID-19 [ 9 , 10 , 11 , 12 , 13 ]. This paper aims to apply data science methods to predict the stock market performance using social media data before, during and after the March 2020 stock market crash. Specifically, using the Organisation for Economic Co-operation and Development (OECD) Taxonomy of Sectoral Digital-intensity Framework [ 14 ], we model market sentiments using cumulative Twitter postings on COVID-19 to predict the stock market performance and investigate what are the sectors that have outperformed others as market sentiments are impacted by the unfolding pandemic. In particular, we are interested to find out how digital intensity moderates the effect of market sentiments on firms’ stock prices, particularly due to largescale unanticipated events such as a pandemic.
This research contributes to the existing literature by examining the influence of market sentiments associated with COVID-19, as derived using cumulative social media postings, upon the stock market performance. While previous research has considered the use of social media to improve stock performance forecasting [ 15 , 16 ], this research is among the first few to demonstrate how digital intensity could mitigate the negative effect of market sentiments on stock market performance in a time of crisis.
The rest of the paper is organized as follows. The next section introduces the extant literature covering three fields of research: the impact of largescale unanticipated incidents on stock performance; the relationships between social media and the stock markets; and the sectoral digital intensity. This is then followed by a section that depicts the empirical study, detailing the research method and data analysis. The discussion section features the implications drawn from the key findings to both research and practice.
2 Literature Review
This research aims to investigate what are the sectors that have a competitive advantage despite the adverse effect of the pandemic. The subsequent sub-section looks at social media and financial markets, reviewing the literature on how social media data have been employed in examining investors’ sentiments and stock market performance. Furthermore, considering that digitally advanced firms performed ostensibly better in the financial markets, the OECD taxonomy of sectors by digital-intensity [ 14 ], which is adopted as the framework for classifying the sectors in the present study, is then introduced.
2.1 Effect of Largescale Unanticipated Events on Stock Markets
According to the Efficient Market Hypothesis (EMH), the aggregate decisions of all participants in the market should accurately reflect the value of public companies and their common shares at any given moment of time. Yet, empirical evidence has shown that, in violation of EMH, people tend to “overreact” to unanticipated and dramatic news events [ 17 , 18 ]. Over-reaction may occur in the short run when investors turn extremely pessimistic during downturns, or place too much emphasis on recent incidents while discounting historical data, which results in prices falling excessively on bad news [ 18 ].
In the current research, the COVID-19 pandemic is conceptualized as an instance of large-scale unanticipated adverse events. In the past, various studies have examined the impact of disasters or major incidents on stock markets. For instance, Barrett et al. [ 19 ] studied the effects of aviation accidents on stock returns in 1987; Shelor et al. [ 20 ] and Lee et al. [ 21 ] analysed the effects of natural disasters, such as hurricanes and earthquakes, on the stock markets. Cam and Ramiah [ 22 ] studied how investors react in the presence of unique large-scale disasters, such as the 9/11 terrorist attacks. Their results show considerable stock market overreactions, which are in agreement with an availability bias acknowledged in the behavioural finance literature, driven by the unprecedented loss and the successive high media attention these events typically receive [ 23 ].
Like other types of catastrophic events, COVID-19 is characterized by extreme uncertainty, whose transmission characteristics and consequences to human bodies were largely unknown. The rapid increase in new cases and death tolls have a significant emotional and material impact worldwide. The virus has certainly adjusted the investor perception on the micro and macroeconomic outlook, resulting in a ‘market-wide shock’. In fact, the impact of COVID-19 on the stock market is more significant than previous disasters due to its fast transmission across the world and it is expected to co-exist with humans over the long-term [ 24 ].
In the past, there have been many epidemics that impacted stock markets worldwide, such as Influenza, Ebola, and Severe Acute Respiratory Syndrome (SARS). All of these events have caused extensive impact on the financial markets. For the United States, a higher incidence of flu is associated with decreased trading, decreased volatility, decreased returns, and higher bid-ask spreads [ 25 ]. SARS infected more than 8000 people in 2003 and shaved 12.8% off the Standard & Poor (S&P) 500 over 38 trading days; Avian Influenza in 2004, Middle East Respiratory Syndrome (MERS) in 2012 and Ebola in 2013–2014 resulted in 5.8 to 7.3% drop to the S&P 500 over different lengths of time. Similarly, the market suffered a near 12.9% pullback in a span of 66 trading days due to the outbreak of Zika virus between 2015 and 2016 [ 26 ]. Nonetheless, none of the preceding epidemics were comparable to COVID-19 in terms of its number of cases infected and destruction to the economy and the financial markets. In order to stop the transmission, countries have shut down their business and people are advised to stay home. As a result, unemployment rates have increased; tourism and supply chains around the world have been disrupted [ 27 ]. Khurram, Liu and Hayfa [ 24 ] argue that COVID-19 has paused the economic cycle throughout the world, and that the health crisis has successfully induced the global financial crisis. Since the outbreak of COVID-19, major financial markets, such as the S&P 500, National Association of Securities Dealers Automated Quotations System (NASDAQ) 100, and Nikkei 225, have been confronted with a severe collision of nearly 30% in market values. Markets are close to collapse as they were during the Global Financial Crisis (GFC) between 2007 and 2009 [ 28 ], but more perilous [ 29 ]. In addition, the financial volatility index (VIX), also known as "Fear gauge," has moved to the highest level, while the 10-year treasury yield index in the US has dropped to record low figures [ 27 ]. According to an assessment by the Asian Development Bank (ADB), the global cost of COVID-19 could be $4.1 trillion.
While the economies are being hit hard, we observe an almost V-shaped recovery in several stock markets, despite the international gloomy economic outlook. There are many discussions on the possible reason behind the rapid rebound, such as fiscal stimulus from the Fed and Congress, expectations of a strong economic recovery and major technology stocks that help drive the gains [ 30 , 31 ]. This paper posits that the stock market rebound is partly attributed to the fading worries about the virus. The measures taken by nations around the world to manage COVID-19—restricting travel, shuttering nonessential businesses and mandatory social distancing policies—are having obvious impacts on slowing contagion. Coronavirus complacency arrives after overblown fears fuelled by media, which seem to contribute to the volatility of the stock market.
2.2 Social Media and Stock Markets
Most conventional research that examines the impact of largescale unanticipated events on stocks have mainly used intervention analysis [ 32 ] or event studies [ 33 ]. These incidents are typically operationalized as dummy variables, which are not able to directly assess the public attention to outlying events, nor quantify such effects. Some exceptions include Liu et al.’s [ 34 ] study on aviation accidents and Ding et al.’s [ 35 ] research on the impact of COVID-19. Both Liu et al. [ 34 ] and Ding et al. [ 35 ] determine market sentiments towards critical events using Search Indices, and assess their effects on stock prices.
Stock prices are influenced by a variety of fundamental factors. Investor sentiments play an important role in shaping the stock market [ 36 ]. Numerous professional and amateur analysts and investors use Twitter to post news articles and opinions, often more frequently than the mainstream news media [ 16 ]. As a meaningful channel for users to share information, social media have been integrated into many aspects of decision-making processes in our daily lives [ 37 ], including investment decisions [ 15 ]. Social media data reveal significant public interest, almost in real time. As such, it serves as a convenient and appropriate source to measure market sentiments.
Several extant studies have shown that social media data measure people’s attention and sentiments and provide timely feedback on investment dynamics. Bollen et al. [ 2 ] reveal that Twitter feeds are correlated to the value of the Dow Jones Industrial Average (DJIA) over time. Their work is in line with the findings of Gilbert and Karahalios [ 38 ], who construct public anxiety indices from online comments to estimate moves of the S&P 500. Several other important studies have also shed light on the various aspects of financial market performance in relation to social media. For example, Sprenger et al. [ 16 ] deploy machine learning algorithms to construct a different bullishness index, which is predictive of stock returns days later. Smailovic, Grcar, Lavrac, and Znidarsic [ 39 ] find sentiment (i.e., positive emotion) in Twitter postings to be predictive of stock returns. Saini and Sharma conduct a comparative analysis of various prediction techniques deployed to predict stock price using public sentiments from social media and other news sources [ 40 ]. These results confirmed the feasibility of using social media as a proxy for market sentiments.
Sul et al., [ 15 ] analyse the cumulative sentiments (positive and negative) in tweets on S&P 500 firms and model them against the stock returns of those firms. Cumulative posts that capture the collective interest of investors are an appropriate reflection of market sentiments and useful indicators for predicting investment decision making [ 15 ]. This study builds upon Sul et al. [ 15 ] in applying cumulative social media feeds as an indication of collective attention towards a largescale unanticipated incident, i.e. COVID-19, to study their impact on the stock performance across sectors.
2.3 Digital Intensity of Sectors
Despite the spread of COVID-19 resulting in the stalling of brick-and-mortar business activities, reports have shown that COVID-19 is accelerating the rise of the digital trade. Digitally-enabled companies are thriving in a time of crisis [ 3 , 41 ]. Firms that have undergone digitalisation are more capable to maintain some degree of business operation and revenue stream amidst the pandemic. Changes are affecting multiple aspects (e.g. product, process and business model innovation) and stages of innovation (e.g. research, development, commercialisation), the prevalence of which may differ across sectors [ 42 ].
Recent OECD work [ 14 ] benchmarks sectors in accordance to their level of digital intensity. The OECD’s Taxonomy of Digital Intensive Sectors is one of the most established sectorial digitalization frameworks [ 42 , 43 ]. This framework provides a systematic overview of digitalisation and its various manifestations: its technological indicators consist of Information and Communications Technology (ICT) equipment and software investment relative to total fixed investment; Purchases of ICT intermediate goods and services relative to output; Stock of robots per employee; Number of ICT specialists over total employment (also referred to as “ICT-specialist intensity”); and Propensity to engage in e-commerce sales. The indicators considered highlight how the degree of digitalisation in sectors is shaped by firms’ investments in various “digital” assets, the approach to interact with markets, the (type of) human capital and skills required, and the way production is operationalized.
There are 36 sectors in the OECD Structural Analysis (STAN) database, ranked by their intensity in the above-mentioned dimensions. For each indicator, cross-country averages are calculated at the sector level and used to benchmark each sector relative to all the others. Table 1 displays an overall summary of the sectors by quartile of digital intensity. Specifically, the taxonomy lists sectors according to their relative position in the overall economy’s ranking and categorizes them into “high”, “medium–high”, “medium–low” and “low” digital intensity, depending on whether sectors appear in the top 25% (or quartile, denoted as “high”), in the bottom 25% (“low”), or in between the two.
As illustrated in Table 1 , some sectors lag in the degree of digitalisation, such as agriculture, mining, and real estate. By contrast, the technology sector, media, financial services, and professional services are ahead of the curve. The uneven development of digitalisation matters as it is creating a new digital divide between the digital “haves” and “have-mores” across sectors. Firms with advanced digital assets and capabilities have been found to enjoy faster growth in revenue and profit margin and generate higher return to shareholders [ 14 ].
Digital transformation is a multifaceted phenomenon that has profound impact. Technologies have always served as key resources in handling issues with regards to complex product-service systems [ 44 ]. For manufacturing companies, a service-oriented transformation enabled by technological revolution is deemed to be important by both trade [ 45 ] and academic literature [ 46 ]. Technologies such as the Internet of things and artificial intelligence offer new pathways to innovative business models, transforming traditional manufacturing to smart manufacturing where the operations require minimal human intervention[ 47 ].
In a post-COVID-19 ‘new normal’, it is posited that in enhancing societal and economic resilience, digital connectivity has emerged as a crucial alternative to the physical equivalent [ 48 ]. It is observed that the stock price of Zoom Video Communications, Inc., a web conferencing platform provider, has risen from US$70 in early Jan 2020 to US$150 at the end of March 2020 amid the market crash. The “GAFAM” stocks—Google; Apple; Facebook; Amazon; and Microsoft—have significantly outperformed broader market indices because of travel restrictions and social distancing orders [ 5 ]. As many companies plunge into the financial unknown, sectors with a pre-existing digital ecosystem instil investors’ confidence in the companies and their stocks [ 3 , 49 ]. Thus, sectors that have embarked on digital transformation, the digital “have-mores”, are more resilient to the adverse effect of market sentiments from COVID-19, while other sectors that are succumbing with total standstill are amongst the most negatively affected.
3 Empirical Study
3.1 research design.
To differentiate the sectors that have performed relatively well from those that have been more heavily impacted by the unfolding of the pandemic, we carry out an empirical study using data from daily cumulative Twitter feeds on COVID-19 and stock prices. The OECD Taxonomy of Sectoral Digital-intensity Framework [ 14 ] is referenced to classify the sectors.
The study comprises two phases. The first Phase used hierarchical clustering and shape-based distance (SBD) to validate the categorisation of firms specified in the OECD Sectoral Digital-intensity Framework, extending the approaches by Sardá-Espinosa [ 50 ] and Paparrizos and Gravano [ 51 ]. The analysis identified two distinct clusters, i.e., a “sensitive” cluster and a “resilient” cluster. The second Phase modelled daily cumulative Twitter feeds on COVID-19 and stock price changes in an augmented vector auto-regression (VAR) [ 52 ]. The analysis compared the stock performance of firms across the two levels of digital intensity, i.e., low/medium, and high, according to the OECD Sectoral Digital-intensity Framework. The empirical investigation in the two phases built upon each other, supporting the notion that market sentiments towards COVID-19 as reflected in Twitter feeds affect the stock prices.
3.2 Data Sources and Analysis
Using data from FactSet, we selected close to 2000 firms listed on the NASDAQ according to the sectoral information in 2020. As one of the world’s largest stock exchange based on market capitalization, NASDAQ represents one of the most extensively studied stock exchange database among the academic community [ 53 , 54 ]. Data collection from such a popular platform facilitates gleaning findings that would hold both academic and practical significance. Sectors are selected according to the OECD Taxonomy of Sectoral Digital-intensity Framework [ 14 ]. The sample comprised four categories of firms: “high”, “medium–high”, “medium–low” and “low” digital intensity, depending on whether sectors appear in the top 25% (or quartile, denoted as “high”), in the bottom 25% (“low”), or in between the two.
From the initial sample, all entries with missing values were eliminated. Pharmaceutical and biopharmaceutical sectors are playing an essential role at the front lines against the virus. While global stock markets take a COVID-19 hit, pharmaceutical stocks are generally outperforming the others, with increased investments in the race to develop therapeutics and vaccines. Manufacturers of diagnostic kits, sanitizers and surgical masks are all ramping up production to meet unprecedented demand. As the present study mainly focuses on how digitally advanced sectors show resilience against negative market sentiments under crisis, firms belonging to the pharmaceutical and biopharmaceutical sectors were removed from the sample to avoid confounding effects. Additionally, we also removed hotels, resorts and cruise lines from the sample. As one of the hardest-hit industries, these sectors were largely affected by travel restrictions, social distancing and lockdowns, which are not relevant to the research focus of digitalisation. The final dataset consists of 1619 firms from 34 sectors.
Although these stocks in the sample cover only 31% of all stocks on the NASDAQ, they represent an economically significant segment of the total market as they account for close to 40% of total NASDAQ market capitalization in the first half of 2020. The average market capitalization of the sampled stocks is US$8,540 million, with a median value of US$643 million. The sampled time period (all trading days between early January to early May) allows a reasonable amount of time lag for the COVID-19 outbreak to generate public attention and stir market response. Table 2 summarizes the descriptive statistics of the firms in each sector. From the column of average daily price changes during the sample time period, some sectors (e.g., agricultural commodity, apparel/footwear retail, construction, etc.) exhibit negative price movement, whereas some sectors such as Internet retail and telecommunication that are likely to be resilient during the pandemic exhibit positive price change. Additionally, we examine the stock price trends of the top companies in two sampled industries (i.e., chemical products and Internet retail) before, during and after the March 2020 stock market crash (see Fig. 1 ). A mixed effect of the COVID-19 outbreak is observed in the chemical products sectors. In contrast, industries like Internet retail provide a silver lining amid the gloomy stock market with a stronger turn-around than other sectors. Stocks exhibit different degrees of rebound from the pandemic crash. In Phase 1, we intend to group together firms of similar performance in Coronavirus-plagued market and similar degree of rebound following the market crash.
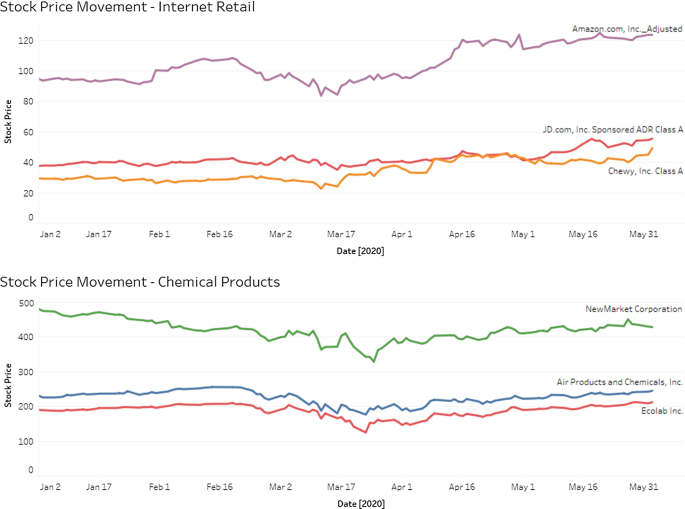
Stock Price Movement of Representative Industries. The stock prices of Amazon.com.Inc were scaled down (i.e., actual stock price/20) to fit into a comparable view with other companies in the Internet retail sector
In Phase 2, the daily cumulative Twitter postings on COVID-19 are collected from Tweet Binder [ 55 ]. Between mid-January 2020 to early May, there were 628,809,016 tweets generated on COVID-19 on Twitter [ 55 ], which captured the evolution of several keywords: #covid19 OR #Coronavirus OR Coronavirus OR #covid-19. In mid-March, Twitter showed an unprecedented increase in tweets related to the Coronavirus in a very short span of time (see Fig. 2 ). Cumulative Twitter postings have been used as a source of data in several studies [ 15 ], yet how such social media behaviours evolve during the course of a pandemic has not been extensively studied hitherto. We posit that such daily cumulative data are a timely reflection of the market attention on the catastrophic crisis. This research is the first to employ social media feeds to examine how critical incidents influence stock prices of firms across different sectors. Such data not only offer insights into investors' collective attention under unexpected incidents, but also reflect the changes over time.
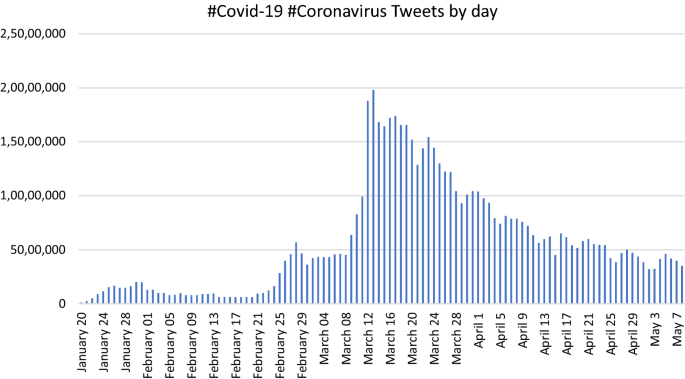
Source: Tweet Binder [ 46 ]
Twitter feeds related to COVID-19. The streamer captures the daily cumulative tweet counts by #covid19 OR #Coronavirus OR Coronavirus OR #covid-19 to reflect the evolution of tag words.
4.1 Phase 1 Results
Instead of comparing static descriptive indicators of firms, this study employs clustering methodology on dynamic stock time series from Jan 2020 to early June 2020 [ 56 ]. The choice of time-series clustering approaches depends on the elements of (dis)similarity or distance measures, clustering algorithms and evaluation [ 56 , 57 ]. Liao [ 58 ] summarizes that a majority of time-series clustering methods are adapted from static clustering algorithms with alteration of similarity or prototype extraction function, or transformation that converts dynamic series to obtain static features. Most time series clustering approaches are based on k-means and hierarchical clustering with different specifications of (dis)similarity measures suitable for the problems. However, k-means algorithm variations (e.g., fuzzy c-means) are more compatible to analyse time series of equal length [ 56 , 58 , 59 ]. Hierarchical clustering is more applicable to our dataset of daily market capitalization as not all the firms trade every day. It also requires a distance measure such as Dynamic Time Warping (DTW) distance to generate dissimilarity matrices [ 51 ].
Hierarchical clustering method groups objects into tree-based clusters including agglomerative method and divisive approach [ 60 ]. The agglomerative tree starts with single object clusters and iteratively groups them into larger clusters by dissimilarity measures. All objects will merge into one cluster unless stop criteria are defined. It will stop if all objects are grouped into one cluster or certain criteria are satisfied. For example, the agglomerative tree continues growing until it gets to the maximum number of clusters. The divisive tree starts with one cluster having all objects and iteratively splitting the cluster until all objects reach single object clusters. The visualized result of hierarchical clustering depicts a binary tree which is constructed by nodes and their children nodes. The children nodes under one parent node are more like each other compared to the children nodes that belong to another parent node. Similarity between clusters is defined in two ways, namely single linkage and complete linkage. The former takes the similarity of the closest pair, whereas the latter takes the similarity of the furthest pair [ 60 ]. DTW distance is a popular choice in the context of time-series clustering [ 50 , 56 ]. DTW compares time series where the timing or the tempo of the variations may vary between the series [ 61 ]. However, it is a computationally expensive option which takes multiple approaches of optimization to improve its time and memory efficiency [ 52 ].
We follow two steps to conduct hierarchical clustering using DTW distance [ 61 ]. The first step is to calculate the distance between time series using the DTW method. It is followed by calculating hierarchical cluster analysis over these dissimilarities. To calculate a DTW distance, considering any two time series to compare, \(X\left( {x_{1} ,x_{2} , \ldots , x_{n} } \right)\) and \(Y\left( {y_{1} ,y_{2} , \ldots , y_{m} } \right)\) , the first step is to compute a local cost matrix (lcm), which has all pairwise distances with \(n \times m\) dimensions. \(p\) corresponds to the \(l_{p}\) norm that defines the distance measures used to construct the lcm matrix. As the second step, an optimum path denoted as ϕ = {(1, 1),…,(n, m)} is searched to minimize the alignment between X and Y by iteratively looping through the lcm from lcm(1, 1) to lcm(n, m). For each step, the direction would be identified when the least cost increased under the chosen constraints [ 62 ]. The final distance \(DTW_{p} \left( {x, y} \right)\) is computed in Eq. ( 1 ) using m ϕ the per-step weighting coefficient and M ϕ the corresponding normalization constant.
We apply the DTW algorithm to all stock prices over the sample time period which are represented as numeric matrices and interpreted row-wise. The main outputs of the DTW algorithm are the minimum global distance between each pair of stock price time series. This distance matrix acts as inputs to enter hierarchical clustering. The hierarchical DTW clustering is conducted based on z-scores normalization with shape-based centroid. Another important decision is to choose the optimal number of clusters when using hierarchical clustering, however, this decision could be subjective. The recommended evaluation methods of clustering performance are to examine the cluster validity indices (CVIs) [ 63 , 64 ]. There are internal CVIs and external indices which are commonly used in assessing the clustering performance. The internal CVIs are prone to check cluster purity, while the external indices are used to validate the results based on a known accurate clustering result. Table 3 briefly summarizes the commonly used CVIs with their evaluation criteria.
Employing the listed methods, we test a different number of clusters on our data, ranging from two to seven clusters using a R technique proposed by Montero and Vilar [ 68 ]. Table 4 summarizes the clustering results. The consensus of two clusters is reached by fulfilling a majority of the CVI indices. The results show 547 firms belong to Cluster 1 with 1072 firms in Cluster 2.
By analysing the extracted members in each cluster, we examine the two clusters by comparing their stock time series patterns (see Fig. 3 ). A weaker softening trend of stock price is observed in Cluster 1 amid COVID-19 outbreak. The stock prices quickly level off and regain an upward tilt after 23rd March. Thus, these firms seem to be less sensitive to the COVID-19 pandemic. The stock prices in Cluster 2 show a L-shaped movement along the sample period, whereby a steeper decline is observed with a slower rate of recovery. The firms are more likely to be sensitive to the COVID-19 pandemic. Comparing the centroids of the two clusters (see Fig. 4 ), we observe a K-shaped recovery where different sectors recover at different rates and magnitudes and the path of recovery continues to diverge.
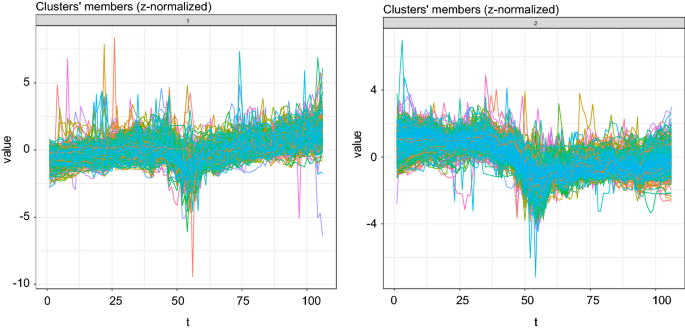
Members of Cluster 1 (left) and Cluster 2 (right)
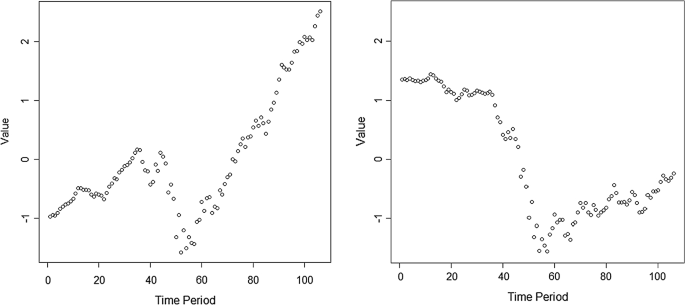
Centroid of Cluster 1 (left) and Cluster 2 (right)
To address the research query, the percentage of firms under the “Slow Recovery Cluster” and the “Better Recovery Cluster” is cross-tabulated with the level of digital transformation, with intensive and less intensive levels following the OECD taxonomy of digital-intensity. The OECD taxonomy categorizes sectors into “high”, “medium–high”, “medium–low” and “low” digital intensity depending on their relative position in the overall economies’ ranking. Quartile rankings is used to measure how a sector has performed against all other sectors in terms of digital intensity. The rankings range from "Top 25%" to "Bottom 25%". The top 25% sectors are assigned to "high", followed by the second highest 25% sectors denoted as “medium–high”. Those with the lowest 25% digital intensity are labelled as “low”, and the second lowest 25% are named “medium–low”. We assigned the sectors in the categories of “low”, “medium–low” and “medium–high” into Group 2 the “less digital intensive” set, whereas the sectors of “high” digital intensity are categorized as “digital intensive” into Group 1. This classification is in line with OECD’s methodology for implementation of the G20 roadmap for digitalisation, which compares sectors of operation that are among the top 25% of digital-intensive sectors versus not (Top Digital Intensive vs Less) [ 43 ].Transport equipment and telecommunications are members of the digital intensive sectors, whereas agricultural commodities and chemical products are among less digital intensive industries. Analysis is conducted to validate if the level of digital intensity is related with the two clusters, i.e., “Slow Recovery Cluster” and “Better Recovery Cluster”. The descriptive summary of the slow recovery cluster and the better recovery cluster can be found in Table 5 .
Consistent with expectation, a bigger portion of firms (47%) within the highly digitally transformed industries, such as telecommunications, Internet retail and professional services, falls under the “Better Recovery Cluster”, as indicated in Table 5 . 80% of the firms in the lower rung, including agriculture, fall in the “Slow Recovery Cluster”. In addition, Pearson \(\chi^{2}\) statistic is used to examine the two-way associations with the number of firms in the cells ( \(\chi^{2}\) (1) = 128.51, p < 0.001). We observed that the top industries which have 50% companies or above fall under the “better recovery cluster” including food retail (90%), Internet retail (77%), computer communications (69%) and major telecommunications (50%). All these sectors belong to the “digital intensive” category except for food retail. However, the strong performance of the food retail sector can be explained by the nature of the business. As the pandemic spreads across, the business is expected to operate and continue to provide essential goods to households and communities day by day. However, the urge for food retail to adopt digital transformation is clear because of increased online and mobile sales [ 69 ]. In comparison, the industries in the lower rung which has less than 20% of firms under the “better recovery cluster” include electric utilities (14%), movies/entertainment (14%), other transportation (14%), agricultural commodities/milling (12%), apparel/footwear retail (11%), construction materials (10%), water utilities (8%), real estate investment trusts (7%), real estate development (2%) and media conglomerates (0%). All these sectors are of less digital intensity except for the sector of other transportation. However, the financial performance of this sector is largely affected by the demand shock caused by COVID-19 crisis, excess supply, and significant oil price decline and is less likely to be linked to its digital intensity. The results show some directional support toward the relative impact of digital intensity. It indicates the degree of digital transformation (digital intensive, less digital intensive) is a relevant grouping variable which could be further tested in the Phase 2 analysis.
4.2 Phase 2 Results
From the plotted pair of proxies– the NASDAQ index and the daily cumulative Twitter postings on COVID-19, negatively correlated movement of the two proxies is present. Stock prices plummet when tweets about “COVID-19” increase. Instead of testing correlation between proxy variables, based on the results in Phase 1, we adopt VAR model to examine the mutual causality relationship between the daily cumulative Twitter postings on COVID-19 and stock price fluctuation under different levels of digital intensity [ 34 , 70 , 71 ]. One way is to test the effects of posting trends on the stock price fluctuation for each respective group of companies. The other way is to examine for each group of firms if the stock price fluctuation induces or reduces public concerns on COVID-19. We use Granger causality to identify the leading relationship between the stock price fluctuation and the daily cumulative twitter postings on COVID-19. We also investigate the timing and length of the effects. The algorithm is implemented in R (Fig. 5 ).
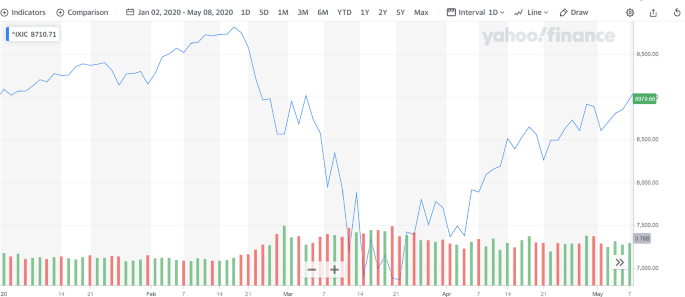
NASDAQ Composite (^IXIC) Charts
To ensure the validity of Granger-causality test, we need to validate the stationarity of both time series that enter the analysis because the non-stationarity of one or both time series would cause spurious causality [ 72 ]. We employ Augmented Dickey–Fuller (ADF) Test and Kwiatkowski–Phillips–Schmidt–Shin (KPSS) to check stationarity for both time series. The null hypothesis of the former test is that the time series are non-stationary. The null hypothesis for the latter is stationarity to conduct a cross-check. Table 6 presents the ADF and KPSS statistics for the daily cumulative Twitter postings on COVID-19 and the average daily stock price variation for each group of firms. The daily variation is the average of day-on-day price change of the firms in each group. The ADF and KPSS results show that the first-order difference eliminates stationarity for both time series. It also determines the order of integration and time series models [ 73 ].
Following the Toda-Yamamoto (TY) procedure, Eq. ( 2 ) and ( 3 ) are constructed. Y t denotes the average daily stock price variation in percentage. X t represents the daily cumulative Twitter postings on COVID-19. p denotes the lag order, and a p , b p , c p , d p , are the coefficients of Y t-p and X t-p with the constant terms a 0 and c 0 . \(u_{t}\) and \(v_{t}\) represent the error terms in Eq. ( 2 ) and ( 3 ) respectively. The null hypothesis for Eq. ( 2 ) is H 0 : b 1 = b 2 = … = b p = 0 with the alternative hypothesis H A : 'Not H 0 '. If H 0 can be rejected, X is the Granger-cause of Y. Equation ( 3 ) tests Granger causality from the other direction—that is, H 0 : d 1 = d 2 = … = d p = 0 and H A : 'Not H 0 '. Similarly, if H 0 could be rejected, Y is the Granger-cause of X. In another word, Eq. ( 2 ) is to see if the prior values of X together with Y’s own past values can predict Y better than solely using Y’s own history. Equation ( 3 ) is to see if the predictions of X can be better made on its own history values and Y’s past values than the predictions of X based on its own history.
Table 6 shows the first order differencing of X and Y eliminating the unit root, which determines the maximum order of integration as one, denoted I (1). According to the TY procedure, the VAR model uses the levels of the data without differencing. Another consideration for X and Y is to determine the appropriate lag length. According to the information criteria of Akaike Information Criterion (AIC), Hannan Quinn (HQ), Schwarz Criterion (SC) and Final Prediction Error (FPE), a lag of six is deemed appropriate for the model. Toda and Yamamoto [ 73 ] point out the advantage of the TY method is to save the cointegration test and avoid pre-test bias. Additionally, we need to ensure no residual serial correlation in the specified VAR model. We conduct a Portmanteau test and find Lag 6 removes residual serial autocorrelation.
The VAR model with Lag 6 is selected and 1 additional lag is added to each equation as the maximum order of integration—I(1). Then, we construct the augmented VAR model for Eq. ( 2 ) and Eq. ( 3 ). A Wald test is used to test the Granger causality hypothesis whether the coefficients of the first 6 lagged values of X in Eq. ( 2 ) and the coefficients of the first six lagged values of Y in Eq. ( 3 ) are zero. The coefficient of the 7th lag is not included because it is the additional lagged value to fix the asymptotics and ensure Wald test statistics of asymptotical chi-square distribution. When the null hypothesis of Wald test is rejected, a Granger causality is implied. The results are presented in Table 7 .
Table 7 shows that Twitter postings on COVID-19 Granger cause the stock price changes of firms in Group 1 and Group 2. The stock price fluctuation in Groups 1 and 2 also imposes an impact on cumulative Twitter postings on COVID-19. These results show that the fluctuation of stock prices would trigger a swing in market sentiment and vice versa. As a further investigation step, we test the magnitudes and the directions of these impacts using the augmented VAR models with the results in Tables 8 and 9 .
As we are interested to differentiate the impact of Twitter postings on stock price fluctuation among the firms of different levels of digital intensity, a detailed investigation of Eq. ( 2 ) is made on two groups of firms—one digital intensive (Group 1), the other one less digital intensive (Group 2). Consistent with our prediction, the cumulative Twitter postings are negatively related to the stock price fluctuation of firms in Group 1, lagged by two days. A two-period lag is found with stronger negative impact of Twitter postings on stock price in Group 2. This indicates cumulative Twitter postings would trigger stronger negative changes in stock prices of firms in Group 2 than those in Group 1. It is evident that digital intensity of firms mitigates the negative effect of market sentiments because of largescale unanticipated crisis on stock market performance. When stocks prices plummet amid COVID-19 pandemic, firms with high degree of digital intensity have out-performed the others.
The results of Eq. ( 3 ) show the impact of stock price variation on cumulative Twitter postings for firms in Group 1 and those in Group 2. As seen from Table 9 , stock price changes cause swings of cumulative Twitter postings in a negative direction for Group 1 and Group 2, lagged by one period. In terms of magnitude of the impact, stock price increase will lead to a deeper decline of cumulative Twitter postings in Group 1. The impact on cumulative Twitter postings is stronger for the firms in Group 1 than for those in Group 2.
5 Discussion
5.1 contributions to knowledge, methodology and practice.
This research has set out to examine how market sentiments affect the stock market performance under COVID-19. Market sentiments are modelled with cumulative social media postings on COVID-19, which reflect the overall consensus about the market as a whole. Performance is operationalised by the stock price differences of firms within the sectors. All sectors under investigation are organized according to OECD Digital-intensity Framework.
Analysis is conducted in two phases. Phase 1 establishes a two-group model on how stock prices adjust to the sudden emergence of the COVID-19 pandemic. Stock prices of a majority of firms across the most digitally advanced sectors have remained resilient when an unexpected crisis puts the market to test, while sectors that lag behind are among the most negatively impacted.
Phase 2 confirms that cumulative Twitter postings on COVID-19 are predictive of stock performance. There is further evidence to demonstrate that the sectoral digital intensity of firms can mitigate the adverse impact of market sentiments induced by largescale unanticipated events. As stock prices dipped amid the pandemic, the digital “have-mores” out-performed the rest and recovered quickly after the crash. Findings in Phase 2 also suggest that stock price changes can in turn influence market sentiments. That is, the market has responded more favourably towards the most digitally advanced firms and sectors and more negatively towards the laggards under crisis.
Governments worldwide have imposed lockdowns in the cities to slowdown the magnitude and speed at which the pandemic is developing. Such lockdowns have led to a near complete standstill of much corporeal economic activities. Yet, certain business trades and consumer purchases have not only continued online, but actually grown considerably. Demand for digital services and portable computing devices have risen as people switch to remote working and learning. Many are envisioning the emergence of a post-COVID-19 ‘new normal’, where there will be a strong prominence of digitalisation. In view of these developments, the market may have given eminence to and greater confidence in firms and sectors that are most digitally advanced as they are in a better position to sustain operations not only amid the pandemic, but also to leverage the full potential in the post-COVID-19 ‘new normal’. Thus, we observe a pronounced gap between the digital “haves” and “have-mores” in their stock market performance.
This study contributes to understanding about how digital intensity moderates the effect of market sentiments on firms’ stock prices, particularly due to largescale unanticipated events such as a pandemic. Researchers acknowledge the importance of alignment between a firm's business and digital strategies [ 74 ]. Information technology (IT) strategy and technological investments have been found to influence profitability and the market value of the firm [ 75 ]. While extant research has examined the use of technology and organizational performance [ 76 ], this study is among the first few to demonstrate how sectors and companies on the digital frontier can give rise to disproportionate gains in the stock market. While past research suggests that the stock market reacts more positively to announcements related to digital investments, such as appointments of chief digital officers [ 77 ] or engaging in e-commerce [ 78 ], our findings focus on sectoral digitalisation as the potential mitigating circumstances when bad news occurs.
The findings also suggest that people do take recent stock prices into consideration when posting online about COVID-19, and such posting behaviours will in turn drive future stock price changes. However, the magnitude of such effect depends on the level of sectoral digital intensity.
In terms of methodology, while the majority of conventional research have studied the impact of largescale unexpected incidents on stocks using intervention analysis [ 32 ] or event studies [ 33 ], this study demonstrated the feasibility of using cumulative social media postings on negative events to measure market sentiments, especially on largescale unanticipated events that can affect the stock prices of firms across sectors. This presents researchers with an alternative to quantify market sentiments and expand the research arsenal to incorporate social media trends, which can offer higher explanatory power.
5.2 Limitations and Future Research
This research has some limitations which offer opportunities for future investigation. Firstly, the study looks into how sectoral digital intensity mitigates the impact of negative market sentiments on the stock market, focusing on the period before, during and after the March 2020 COVID-19 stock market crash. It is possible that there is already a gap between the digital ‘Haves’ and ‘Have-mores’ in their stock performance before the crisis. Past research has confirmed that the stock market reacts positively to firms that embrace digital initiatives, such as appointments of newly created chief digital or data officer positions [ 77 ] or engaging in e-commerce [ 78 ]. Future studies can look into the long-run stock price performance of firms with effective digital strategies.
Secondly, after the March 2020 COVID-19 stock market crash, many of the major stock indices have regained much of their lost territory since early April, and such recovery so far has been fuelled by aggressive stimulus packages rolled out by governments to boost the economy. The resuming of business activities and trade is also underpinning market optimism and rebound. The confounding effects of government intervention and support are hard to capture within the current model. Future research can examine how such government intervention restores public confidence and accelerates the market rebound. Moreover, this research adopts a batch processing methodology. Future research can look into real-time prediction of stock performance using social media streaming [ 47 ].
Third, the literature on sectoral digital intensity suggests a large and widening divide among firms within each sector. We did not capture the within sector relative digital intensity in the current study. Future research should compare the digital “haves” and “have mores” within each sector.
Lastly, while the speed of innovation is accelerating economy-wise, not all firms within the same sectors are similarly equipped to respond to new challenges and tap into new opportunities. This research has not looked into the possible causes of the widening divide between sectors in digital intensity. With the entry of new digital start-ups and technology firms and the emergence of new market segments, sectoral boundaries are blurring. Future research may aim to uncover how the various conditions lead to an increasing divide between the “haves” and the “have-mores”. A longitudinal study to identify the changes in digital capabilities within each sector can help reveal the process of such changes.
Mazur M, Dang M, Vega M (2021) COVID-19 and the March 2020 stock market crash: evidence from S&P1500. Financ Res Lett 38:1090. https://doi.org/10.1016/j.frl.2020.101690
Article Google Scholar
Bollen J, Mao H, Zeng X (2011) Twitter mood predicts the stock market. J Comput Sci 2(1):1–8. https://doi.org/10.1016/j.jocs.2010.12.007
Huang X, Kuijpers D, Li L, Sha S (2020) Xia C (2020) How Chinese consumers are changing shopping habits in response to COVID-19, McKinsey & Company. McKinsey & Company Accessed 6:2020
Google Scholar
Sheth J (2020) Impact of Covid-19 on consumer behavior: Will the old habits return or die? J Bus Res 117:280–283. https://doi.org/10.1016/j.jbusres.2020.05.059
Kshetri N (2020) COVID-19 Meets Big Tech. Computer 53(08):10–13. https://doi.org/10.1109/MC.2020.2996698
Saxena N, Gupta P, Raman R, Rathore AS (2020) Role of data science in managing COVID-19 pandemic. Indian Chem Eng 62(4):385–395. https://doi.org/10.1080/00194506.2020.1855085
Sliwoski G, Kothiwale S, Meiler J, Lowe EW Jr (2013) Computational methods in drug discovery. Pharmacol Rev 66(1):334–395. https://doi.org/10.1124/pr.112.007336
Wong ZSY, Zhou J, Zhang Q (2019) Artificial Intelligence for infectious disease Big Data Analytics. Infect Dis Health 24(1):44–48. https://doi.org/10.1016/j.idh.2018.10.002
Mohamadou Y, Halidou A, Kapen PT (2020) A review of mathematical modeling, artificial intelligence and datasets used in the study, prediction and management of COVID-19. Appl Intell 50(11):3913–3925. https://doi.org/10.1007/s10489-020-01770-9
Wang P, Lu J-A, Jin Y, Zhu M, Wang L, Chen S (2020) Statistical and network analysis of 1212 COVID-19 patients in Henan, China. Int J Infect Dis 95:391–398. https://doi.org/10.1016/j.ijid.2020.04.051
Livadiotis G (2020) Statistical analysis of the impact of environmental temperature on the exponential growth rate of cases infected by COVID-19. PLoS ONE 15(5):e0233875–e0233875. https://doi.org/10.1371/journal.pone.0233875
Menebo MM (2020) Temperature and precipitation associate with Covid-19 new daily cases: A correlation study between weather and Covid-19 pandemic in Oslo. Norway Sci Total Environ 737:139659. https://doi.org/10.1016/j.scitotenv.2020.139659
Bragazzi NL, Dai H, Damiani G, Behzadifar M, Martini M, Wu J (2020) How Big Data and Artificial Intelligence Can Help Better Manage the COVID-19 Pandemic. Int J Environ Res Public Health 17(9):3176. https://doi.org/10.3390/ijerph17093176
Calvino F, Criscuolo C, Marcolin L, Squicciarini M (2018) A taxonomy of digital intensive sectors. OECD Science, Technology and Industry Working Papers. https://doi.org/10.1787/f404736a-en
Sul HK, Dennis AR, Yuan L (2017) Trading on Twitter: using social media sentiment to predict stock returns. Decis Sci 48(3):454–488. https://doi.org/10.1111/deci.12229
Sprenger TO, Tumasjan A, Sandner PG, Welpe IM (2014) Tweets and trades: the information content of stock microblogs. Eur Financ Manag 20(5):926–957. https://doi.org/10.1111/j.1468-036X.2013.12007.x
De Bondt WFM, Thaler R (1985) Does the stock market overreact? J Financ 40(3):793–805. https://doi.org/10.1111/j.1540-6261.1985.tb05004.x
Nofsinger JR (2005) Social mood and financial economics. J Behav Financ 6(3):144–160. https://doi.org/10.1207/s15427579jpfm0603_4
Barrett WB, Heuson AJ, Kolb RW, Schropp GH (1987) The adjustment of stock prices to completely unanticipated events. Financ Rev 22(4):345–354. https://doi.org/10.1111/j.1540-6288.1987.tb01258.x
Shelor RM, Anderson DC, Cross ML (1992) Gaining from loss: property-liability insurer stock values in the aftermath of the 1989 California earthquake. J Risk Insur 59(3):476–488. https://doi.org/10.2307/253059
Lee K-J, Lu S-L, Shih Y (2018) Contagion effect of natural disaster and financial crisis events on international stock markets. J Risk Financ Manag 11(2):16. https://doi.org/10.3390/jrfm11020016
Cam MA, Ramiah V (2014) The influence of systematic risk factors and econometric adjustments in catastrophic event studies. Rev Quant Finan Acc 42(2):171–189. https://doi.org/10.1007/s11156-012-0338-4
Pompian M (2012) Behavioral finance and wealth management: how to build investment strategies that account for investor biases. Wiley
Book Google Scholar
Shehzad K, Liu X, Kazouz H (2020) COVID-19’s disasters are perilous than Global Financial crisis: a rumour or fact? Financ Res Lett 36:101669. https://doi.org/10.1016/j.frl.2020.101669
McTier BC, Tse Y, Wald JK (2013) Do Stock Markets Catch the Flu? J Financ Quant Anal 48(3):979–1000. https://doi.org/10.1017/S0022109013000239
Li Y (2020) Market reactions to past virus scares show stocks may have more to lose. CNBC. https://www.cnbc.com/2020/01/28/market-reactions-to-major-virus-scares-show-stocks-have-more-to-lose.html
Leduc S, Liu Z (2020) The Uncertainty Channel of the Coronavirus. FRBSF. Accessed 30 March 2020
Tooze A (2020) The crisis has brought the economy to a near halt, and left millions of people out of work. But thanks to intervention on an unprecedented scale, a full-scale meltdown has been averted—for now. The Guardian. Accessed 2020 2020
Georgieva K (2020) IMF managing director Kristalina Georgieva's statement following a G20 ministerial call on the coronavirus emergency. International Monetary Fund. Accessed 23 March 2020 2020
Banerji G (2020) Why did stock markets rebound from Covid in record time? Here are five reasons. Wall Street J. Accessed 15 September 2020
Winck B (2020) Cries for more stimulus are overblown and stock investors should stop throwing ‘tantrums’ about it, says a Wall Street chief strategist. Business Insider. Accessed 2020
Box GEP, Tiao GC (1975) Intervention analysis with applications to economic and environmental problems. J Am Stat Assoc 70(349):70–79. https://doi.org/10.1080/01621459.1975.10480264
MacKinlay AC (1997) Event studies in economics and finance. J Econ Lit 35(1):13–39
Liu Y, Peng G, Hu L, Dong J, Zhang Q (2019) Using Google Trends and Baidu Index to analyze the impacts of disaster events on company stock price. Ind Manag Data Syst 120(2):350–365. https://doi.org/10.1108/IMDS-03-2019-0190
Ding D, Guan C, Chan CML, Liu W (2020) Building stock market resilience through digital transformation: using Google trends to analyze the impact of COVID-19 pandemic. Front Bus Res China 14:21. https://doi.org/10.1186/s11782-020-00089-z
Baker M, Wurgler J (2007) Investor Sentiment in the Stock Market. J Econ Perspect 21(2):129–152. https://doi.org/10.2139/ssrn.962706
boyd dm, Ellison NB, (2007) Social network sites: definition, history, and scholarship. J Comput-Mediat Comm 13(1):210–230. https://doi.org/10.1111/j.1083-6101.2007.00393.x
E G, K K Widespread worry and the stock market. In: ICWSM 2010 - Proceedings of the 4th International AAAI Conference on Weblogs and Social Media, Washington, DC, 2010. pp 58–65
Smailović J, Grčar M, Lavrač N, Žnidaršič M (2014) Stream-based active learning for sentiment analysis in the financial domain. Inf Sci 285:181–203. https://doi.org/10.1016/j.ins.2014.04.034
Saini A, Sharma A (2019) Predicting the unpredictable: an application of machine learning algorithms in Indian stock market. Ann Data Sci. https://doi.org/10.1007/s40745-019-00230-7
Baig A, Hall B, Jenkins P, Lamarre E (2020) McCarthy B (2020) The COVID-19 recovery will be digital: A plan for the first 90 days. McKinsey & Company Accessed 14:2020
Paunov C, Planes-Satorra S (2019) How are digital technologies changing innovation?: Evidence from agriculture, the automotive industry and retail. OECD Publishing, OECD Science. https://doi.org/10.1787/67bbcafe-en
OECD (2018) Towards the implementation of the G20 roadmap for digitalisation: skills, business dynamics and competition. Report prepared at the request of the 2017 G20 German Presidency
Neu WA, Brown SW (2005) Forming successful business-to-business services in goods-dominant firms. J Serv Res 8(1):3–17. https://doi.org/10.1177/1094670505276619
Noventum Service Management (2016) Manufacturers’ advanced services: IoT as the key to profitability and growth. White Paper. Accessed 2016
Coreynen W, Matthyssens P, Bockhaven WV (2017) Boosting servitization through digitization: Pathways and dynamic resource configurations for manufacturers. Ind Market Manag 60:42–53. https://doi.org/10.1016/j.indmarman.2016.04.012
Tien JM (2017) Internet of Things, Real-Time Decision Making, and Artificial Intelligence. Ann Data Sci 4(2):149–178. https://doi.org/10.1007/s40745-017-0112-5
Okuda A, Karazhanova A (2020) Digital Resilience Against COVID-19. United Nation ESCAP. Accessed 31 March 2020
Blackburn S, LaBerge L, O’Toole C (2020) Schneider J (2020) Digital strategy in a time of crisis. McKinsey & Company Accessed 22:2020
Sard´a-Espinosa A (2018) Comparing Time-Series Clustering Algorithms in R Using the dtwclust Package
Paparrizos J, Gravano L (2017) Fast and accurate time-series clustering. Acm T Database Syst 42(2):8. https://doi.org/10.1145/3044711
Shi Y, Tian YJ, Kou G, Peng Y, Li JP (2011) Optimization based data mining: theory and applications. Springer
Bordino I, Battiston S, Caldarelli G, Cristelli M, Ukkonen A, Weber I (2012) Web search queries can predict stock market volumes. PLoS ONE 7(7):e40014. https://doi.org/10.1371/journal.pone.0040014
Weng B, Lu L, Wang X, Megahed FM, Martinez W (2018) Predicting short-term stock prices using ensemble methods and online data sources. Expert Syst Appl 112:258–273. https://doi.org/10.1016/j.eswa.2018.06.016
Analytics T (2020) Covid 19—Twitter evolution. Tweet Binder. Accessed 8 May 2020 2020
Aghabozorgi S, Seyed Shirkhorshidi A, Ying Wah T (2015) Time-series clustering – A decade review. Inf Syst 53:16–38. https://doi.org/10.1016/j.is.2015.04.007
Olson DL, Shi Y (2007) Introduction to business data mining. McGraw-Hill/Irwin
Liao TW (2005) Clustering of time series data-a survey. Pattern Recognit 38(11):1857–1874. https://doi.org/10.1016/j.patcog.2005.01.025
Golay X, Kollias S, Stoll G, Meier D, Valavanis A, Boesiger P (1998) A new correlation-based fuzzy logic clustering algorithm for fMRI. Magn Reson Med 40(2):249–260. https://doi.org/10.1002/mrm.1910400211
Hastie T, Tibshirani R, Friedman J (2009) The elements of statistical learning. Springer
Giorgino T (2009) Computing and visualizing dynamic time warping alignments in R: the dtw package. J Stat Softw 31(7):1–24
Giorgino T (2009) Computing and visualizing dynamic time warping alignments in R: the dtw package. J Stat Softw 31(i07):1–24
Arbelaitz O, Gurrutxaga I, Muguerza J, Pérez JM, Perona I (2013) An extensive comparative study of cluster validity indices. Pattern Recognit 46(1):243–256. https://doi.org/10.1016/j.patcog.2012.07.021
Lei Y, Bezdek JC, Chan J, Vinh NX, Romano S, Bailey J (2017) Extending information-theoretic validity indices for fuzzy clustering. IEEE Trans Fuzzy Syst 25(4):1013–1018. https://doi.org/10.1109/TFUZZ.2016.2584644
Rousseeuw PJ (1987) silhouettes: a graphical aid to the interpretation and validation of cluster analysis. J Comput Appl Math 20:53–65. https://doi.org/10.1016/0377-0427(87)90125-7
Saitta S, Raphael B, Smith IFC (eds) (2007) A Bounded Index for Cluster Validity, vol 4571. Machine Learning and Data Mining in Pattern Recognition. MLDM 2007. Lecture Notes in Computer Science. Springer
Kim M, Ramakrishna RS (2005) New indices for cluster validity assessment. Pattern Recogn Lett 26:2353–2363
Montero P, Vilar JA (2014) TSclust: An R Package for Time Series Clustering. J Stat Softw 62(1):1–43
Markenson S (2020) Grocery’s Ecommerce Evolution during COVID-19. Food Marketing Institute. https://www.fmi.org/blog/view/fmi-blog/2020/06/23/grocery-s-ecommerce-evolution-during-covid-19
Tetlock PC (2007) Giving content to investor sentiment: the role of media in the stock market. J Financ 62(3):1139–1168. https://doi.org/10.1111/j.1540-6261.2007.01232.x
Deng S, Huang ZJ, Sinha AP, Zhao H (2018) The interaction between microblog sentiment and stock return: an empirical examination. MIS quart 42(3):895–918
He Z, Maekawa K (2001) On spurious Granger causality. Econ Lett 73(3):307–313. https://doi.org/10.1016/S0165-1765(01)00498-0
Toda HY, Yamamoto T (1995) Statistical inference in vector autoregressions with possibly integrated processes. J Econom 66(1–2):225–250. https://doi.org/10.1016/0304-4076(94)01616-8
Sabherwal R, Sabherwal S, Havakhor T, Steelman Z (2019) How does strategic alignment affect firm performance? the roles of information technology investment and environmental uncertainty. MIS Q 43(2):453–474. https://doi.org/10.25300/misq/2019/13626
Mithas S, Rust RT (2016) How information technology strategy and investments influence firm performance: conjecture and empirical evidence. MIS Q 40(1):223–245. https://doi.org/10.25300/misq/2016/40.1.10
Hanelt A, Firk S, Hildebrandt B, Kolbe LM (2021) Digital M&A, digital innovation, and firm performance: an empirical investigation. Eur J Inf Syst 30(1):3–26. https://doi.org/10.1080/0960085X.2020.1747365
Hendricks KB, Singhal VR (2001) The Long-Run Stock Price Performance of Firms with Effective TQM Programs. Manag Sci 47(3):359–368. https://doi.org/10.1287/mnsc.47.3.359.9773
Subramani M, Walden E (2001) The Impact of E-Commerce Announcements on the Market Value of Firms. Inf Syst Res 12(2):135–154. https://doi.org/10.1287/isre.12.2.135.9698
Download references
Author information
Authors and affiliations.
Office of Graduate Studies, Singapore University of Social Sciences, 463 Clementi Rd, Singapore, 599494, Singapore
School of Business, Singapore University of Social Sciences, 463 Clementi Rd, Singapore, 599494, Singapore
Wenting Liu
Intellectual Property Office of Singapore International, 1 Paya Lebar Link #11-03 PLQ 1, Paya Lebar Quarter, Singapore, 408533, Singapore
Jack Yu-Chao Cheng
You can also search for this author in PubMed Google Scholar
Corresponding author
Correspondence to Chong Guan .
Ethics declarations
Conflicts of interest.
The author declared they have no conflict of interest.
Additional information
Publisher's note.
Springer Nature remains neutral with regard to jurisdictional claims in published maps and institutional affiliations.
Rights and permissions
Reprints and permissions
About this article
Guan, C., Liu, W. & Cheng, J.YC. Using Social Media to Predict the Stock Market Crash and Rebound amid the Pandemic: The Digital ‘Haves’ and ‘Have-mores’. Ann. Data. Sci. 9 , 5–31 (2022). https://doi.org/10.1007/s40745-021-00353-w
Download citation
Received : 30 December 2020
Revised : 16 June 2021
Accepted : 30 July 2021
Published : 15 September 2021
Issue Date : February 2022
DOI : https://doi.org/10.1007/s40745-021-00353-w
Share this article
Anyone you share the following link with will be able to read this content:
Sorry, a shareable link is not currently available for this article.
Provided by the Springer Nature SharedIt content-sharing initiative
- Stock market
- Social media
- Digital intensity
- Find a journal
- Publish with us
- Track your research
- Open access
- Published: 28 April 2021
Preventing crash in stock market: The role of economic policy uncertainty during COVID-19
- Peng-Fei Dai 1 , 2 ,
- Xiong Xiong 1 ,
- Zhifeng Liu ORCID: orcid.org/0000-0002-6158-4631 3 ,
- Toan Luu Duc Huynh 4 , 5 &
- Jianjun Sun 6
Financial Innovation volume 7 , Article number: 31 ( 2021 ) Cite this article
7090 Accesses
47 Citations
2 Altmetric
Metrics details
This paper investigates the impact of economic policy uncertainty (EPU) on the crash risk of US stock market during the COVID-19 pandemic. To this end, we use the GARCH-S (GARCH with skewness) model to estimate daily skewness as a proxy for the stock market crash risk. The empirical results show the significantly negative correlation between EPU and stock market crash risk, indicating the aggravation of EPU increase the crash risk. Moreover, the negative correlation gets stronger after the global COVID-19 outbreak, which shows the crash risk of the US stock market will be more affected by EPU during the epidemic.
Introduction
The economic downturn during the COVID-19 pandemic has resulted in a significant decline in the stock market. Some of the previous studies have examined the impacts of COVID-19 pandemic on the downside risks of stock market. One of the main conclusions is that, in general, the occurrence of COVID-19 causes a direct and significant drop in stock prices (Baker et al. 2020b , a ; Al-Awadhi et al. 2020 ; Ramelli and Wagner 2020 ; Zhang et al. 2020 ; Liu et al. 2020 ). Djurovic et al. ( 2020 ) points the Dow Jones Industrial Index has been dropping by 36.4% between February 18, 2020 and March 23, 2020. While from the perspective of volatility risk, COVID-19 will significantly increase the volatility of the stock market (Baek et al. 2020 ; Onali 2020 ; Papadamou et al. 2020 ). Different from the existing literature, this paper focuses on stock market crash risk. Mazur et al. ( 2020 ) and Ziemba ( 2020 ) study the US stock market crash during the Covid-19 period. However, they treat the crash risk as the extreme downside volatility. In our paper, following Chen et al. ( 2001 ) and Kräussl et al. ( 2016 ), we measure the crash risk by using the conditional skewness of the equity market return. It is a better way to simultaneously capture the asymmetry and negative extremes of the crash risk (Kim et al. 2011a , b ; Kim et al. 2011b ; Wen et al. 2019a , b ). And then, we conduct an empirical analysis to investigate the impacts of the economic policy uncertainty on the crash risk of the US stock market.
In fact, in the early days of the pandemic, the US stock market has experienced a plunge. From Fig. 1 , we can see that the stock market has undergone a severe impact from COVID-19. The S&P 500 plummets by one-third in a short period, from 3380 points on February 14, 2020 to 2237 points on March 23, 2020. Our subsequent empirical findings further confirm this intuitive conclusion that COVID-19 negatively affects stock market crash risk. We find that the severity of the pandemic, whose proxy is the growth rate of the daily new confirmed cases, does have a significant negative impact on the conditional skewness of the market return, i.e., the crash risk of stock market. It is also consistent with Liu et al. ( 2021 )’s work on the Chinese equity market, which indicates that the pandemic increases the crash risk of stock market.
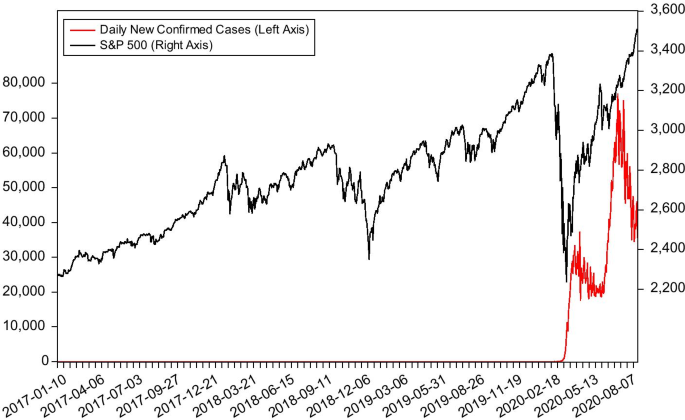
Daily new confirmed cases and S&P 500 Index
However, we have noticed that although the number of daily confirmed cases in the United States continued to rise in the following period, stock prices gradually returned to the level before the pandemic, and even hit a new high in the past three years. It indicates that the severity of the pandemic alone is not enough to explain the stock crash.
We argue that one of the reasons for this situation is the reduction of uncertainty in economic policies, which is believed to have a wide-ranging impact on economic and financial activities (Gulen and Ion 2016 ; Brogaard and Detzel 2015 ; Dai et al. 2021 ; Wen et al. 2019a , b ; Yousaf and Ali 2020 ). Figure 2 illustrates the evolving curves of uncertainty indices and S&P 500. As we can see from Fig. 2 , during the pandemic, economic policy uncertainty (EPU) and stock prices display the opposite trend. In the early stages of the pandemic, as the uncertainty caused by COVID-19 rose sharply, stock prices have also suffered a crash. However, with the successive government measures designed to deal with the pandemic, economic policy uncertainty has gradually decreased, which is what we believe a fundamental reason for the stock market rebound. In other words, we believe that the reduction of economic policy uncertainty during the pandemic will help reduce the crash risk of stock market. Our findings also support this hypothesis. We find that the conditional skewness reacts negatively to the change rate of economic policy uncertainty, indicating that the reduction of economic policy uncertainty can effectively reduce the crash risk of stock market. We further find that this effect only exists during the pandemic, and it is not significant during regular periods. It may mean that the stability of economic policies plays a more critical role in reducing the extremely negative impact of major crisis events.
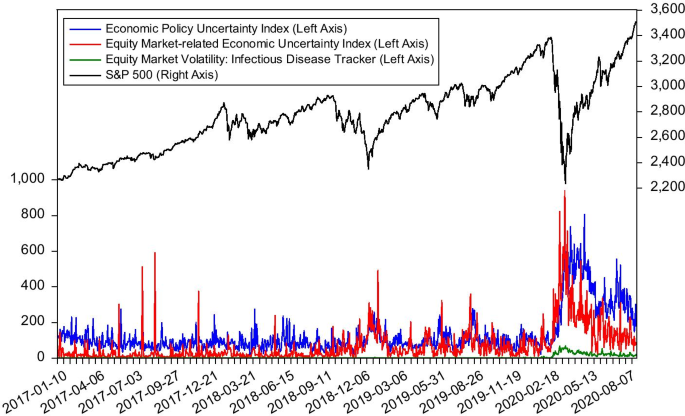
Economic policy uncertainty and the S&P 500 Index
Before explaining our main contributions, this paper objectives mainly focused on two main folds. First, the estimations of market crash would draw an attention to not only investors but also policymakers to understand how the markets move during this difficult time. Second, the followed regressions with predictive factors will also be our main aims and scopes. Therefore, this study is dedicated to examine the predictive factors of health situation (proxied by number of cases as well as deaths) and the Economic Policy Uncertainties. In doing so, this paper contributes to the extant literature in several ways. First, unlike existing research related to COVID-19, which mainly involves stock market returns and volatility, we focus our attention on the stock market crash risk. Return and volatility are the first and second moments of return distributions, respectively, and we use conditional skewness to measure the crash risk, thus paying attention to the third moment of stock market returns. Second, we confirm the conclusion that COVID-19 will increase the crash risk in the US stock market, which is initially drawn by Liu et al. ( 2021 ) in the Chinese stock market. We further find that the increase in the number of confirmed cases can only explain the worsening crash risk of stock market in the early stages of the pandemic. However, it cannot explain why in the middle and late stages, when the number of cases continues increasing, the crash risk will decrease instead. Therefore, the third contribution of our study is that we find that the stability of economic policies can provide a specific explanation for this puzzle. Our findings show that high economic policy uncertainty will increase the crash risk of the US stock market. In contrast, low uncertainty of economic policies can help reduce the likelihood of stock market crashes, especially during the extreme crisis of the COVID-19 global pandemic. More importantly, this paper has important policy implications. Just as our findings show the importance of economic policy stability in reducing the crash risk, we suggest that when facing major crisis events, policymakers should introduce event-response policies as soon as possible. It is helpful to reduce the adverse effects of economic policy uncertainty.
The rest of the paper is organized as follows. Section 2 provides a brief literature review regarding COVID-19 impacts on financial markets. Section 3 introduces the data and methodology. Section 4 shows the empirical results. Conclusions appear in Sect. 5 .
Brief literature review regarding COVID-19 impacts on financial markets
This section will acknowledges the current literature of COVID-19 pandemic and its impacts on the financial markets. When it comes to the comparison between COVID-19 event and other public health crises, Schell et al. ( 2020 ) indicated that the coronavirus outbreak exhibits the significant negative abnormal returns across the majority of equity markets while this phenomenon does not exist in the remaining events such as Ebola, Zika virus, and so forth. In the same vein, the study of Ambros et al. ( 2020 ) investigates the role of news on the stock markets returns and volatility. Although this paper sheds a new light on null results of potential channel between returns and pandemic news, the aforementioned paper provides an empirical evidence about the role of number of disease news significantly the European market volatility. This extant literature shapes our motivation to use the GARCH-S, capturing the skewness effects, to measure the possibility of market crashes during this unprecendented events. Instead of using the volatility index, proposed by Alizadeh et al. ( 2002 ), this paper would extend the the works of Chen et al. ( 2001 ) and Kräusslet al ( 2016 ) proxied by the conditional skewness for crashes on the onset of COVID-19 pandemic.
The novel indicator in terms of uncertainties regarding pandemic news, proposed by Baker et al. ( 2020b , a ), effectively explains the market returns and volatility in the United States. The followed studies is ongoing to contribute the new index by aggregating the wide range of economic uncertainties (Altig et al., 2020 ). Accordingly, the implied volatility rose considerably in February (2020) and fell gradually in the following months since the stock market recovered afterwards. Thus, these papers put the fundamental agruments how the uncertainties are associated with the the stock market jumps. Concomitantly, Wang et al. ( 2020 ) explained the efficiency of predictive power of both VIX and EPU on forecasting the equity markets during COVID-19. Interestingly, VIX contains the strongest predictive ability by using the different models. In contrast, the strand of literature also confirmed the gravity of Economic Policy Uncertainty (EPU) as risk factor to predict economic losses (Al‐Thaqeb et al., 2020 ; Youssef et al., 2021 ; Caggiano et al., 2020 ; Megaritis et al., 2021 ).
After reviewing the current literature, we found that the questions regarding the impacts of COVID-19 on the market crashes are still unanswered. Therefore, this paper contributes the ongoing discussion by three main folds. First, the advanced model of GARCH-S benefits the robust rather than using conventional approaches. Additionally, the persistent and autocorrelated skewness would help improve the model performance. Second, using both indicators, including the pandemic situation and Economic Policy Uncertainties, would provide insights about not only the health crisis management but also the market sentiment. Lastly, the studies of advanced economies broaden the current view how these financial markets reacted and jumps (Goodell and Huynh, 2020 ). However, the emerging markets are still a potential avenue for understanding. Hence, this paper sheds a new light on the Chinese markets, where has the fast resiliency after the economic shocks (Liu et al., 2021 ).
Data and methodology
Covid-19 related variables.
To measure the severity of the COVID-19 pandemic, we use the logarithmic growth rate of daily confirmed cases ( rCases ) in the US as the proxy. The initial dataset is from Our World in Data ( https://ourworldindata.org/coronavirus ). We also set a dummy variable, D_epid , to divide the whole sample into two periods: before- and after- the pandemic. We set the value of this dummy is one after January 21, 2020, the date when the first case of COVID-19 in the United States is confirmed, and zero otherwise.
Economic policy uncertainty indices
We use three economic policy uncertainty related indices based on daily newspaper coverage in the United States in our paper, all of which are proposed by Baker et al. ( 2016 ) or Baker et al. ( 2020b , a ). The first variable is the Economic Policy Uncertainty Index for United States ( EPU ), and the second is the Equity Market-related Economic Uncertainty Index ( EMU ). The third is a proxy for COVID-induced economic uncertainty, say, Equity Market Volatility: Infectious Disease Tracker ( EMV-ID ). We take the first variable as our main proxy of Economic Policy Uncertainty, and the other two are used in our robustness checks. In our empirical analysis, we use the logarithmic change rate of EPU indices, so that we get three change rates corresponding to the above indices, namely rEPU , rEMU , rEMV-ID , respectively. If the index has a zero value, we use log(Index + 1) when calculating the logarithm. All these data are retrieved from FRED, Federal Reserve Bank of St. Louis ( https://fred.stlouisfed.org/ ).
Measuring US stock market crash risk
In our paper, following the works of Chen et al. ( 2001 ) and Kräusslet al ( 2016 ), the crash risk of the US stock market is proxied by the conditional skewness ( Skew ), which is estimated from the GARCH-S (GARCH with skewness) model. The original idea of using skewness to measure crash risk was put forward by Chen et al. ( 2001 ). However, they only calculate a half-a-year horizon skewness from the daily data. To model the daily Euro crash risk, Kräussl et al. ( 2016 ) makes use of the Gram–Charlier series expansion method to estimate the conditional skewness. Our GARCH-S model is specified as follows:
where r t is the logarithmic return of the S&P 500 index, retrieved from Yahoo Finance ( https://finance.yahoo.com/ ), and h t is a classical GARCH(1,1) structure. Footnote 1 There are two residual forms in the model: the residual ε t and the standardized residual η t . I t−1 represents the information set at the time t . s t is the conditional skewness process and it is the key part in our model. In addition to the constant term, it consists of two parts: the autoregressive part and the lagged return shocks part. Both León et al. ( 2005 ) and Kräussl et al. ( 2016 ) use the Gram–Charlier series expansion to estimate the model. We will follow their works, and the difference is we truncate at the third moment.
Table 1 reports the estimation results of the GARCH-S model and the standard GARCH model. For comparison purposes, we calculate the estimation of GARCH(1,1) using the Gram-Charlier sequence expansion as well. As can be seen from Table 1 , the estimations from GARCH-S model are highly consistent with that from GARCH (1,1) model, which indicates that our model is robust. In general, most of the coefficients are significant, suggesting that the model can fit the data well. We focus on the conditional skewness process. As expected, the coefficient of the shock to skewness is positive and significant (0.0361 with a z-statistic 7.2590) and the coefficient of lagged skewness is also positive and significant (0.1544 with a z-statistic 5027.295). The structure of the conditional skewness is very similar to that in the variance case, indicating that the skewness is autocorrelated and persistent.
Descriptive statistics
All the data in our paper is daily, and the sample period is from January 2017 to August 2020. Table 2 shows the descriptive statistics of the variables we used, and all variables are stationary.
There are several worthy noting points from the descriptive statistics of our variables. First, our variables are stationary in the original level, implying the validity to be employed in the time series models to avoid the spurious results. In addition, the average changes in the number of infected cases are around 1.14%, while the highest growth rate is approximately 294%. To our great surprise, the movement of equity market uncertainties is likely to be dominant with the widest spread of 423% changes. Among the proxies for market shocks, this factor experiences the most significant deviation with the highest value in terms of Standard deviation.
Table 3 reports the correlations between any two variables considered in our empirical analysis. The Panel A, B and C of Table 3 show the results about the whole sample, subsample with ending date January 20, 2020 and subsample with starting date January 21, 2020 respectively. The Panel B of Table 3 reports the correlation of rEPU and Skew is not significant with value of − 0.0511; while the correlation of rEPU and Skew is significant at least 5% level with value of − 0.1975 reported in the Panel C of Table 3 . Moreover, the similar results about rEMU and rEMV_ID can be obtained by comparing the Panel B and Panel C of Table 3 . The results of correlation provide an intuitive reflection that the economic policy uncertainty does not affect the stock market crash risk before the break of COVID-19, while the economic policy uncertainty have an impact on the stock market crash risk since the break of COVID-19.
Model specifications
We use the simple time series model to conduct our empirical analysis. The dependent variable is the stock market crash risk, measured by conditional skewness estimated from the GARCH-S model. We have two main explanatory variables. The first is the logarithmic growth rate of daily confirmed cases, rCases , and the logarithmic change rate of the Economic Policy Uncertainty Index for the United States, rEPU . The alternative variables for rEPU will be used in the robustness checks . The primary model is specified as:
where p and q will be determined by the AIC or SC information criterion. In which, the c represents the constant term while \(\alpha ,\beta ,\lambda\) are the coefficients of the Skew, cases, and EPU, respectively. We also look at the previous period with the time-lagged ( t − 1) to see the predictive power with the error terms ( \(\varepsilon_{t} )\) .
In order to examine the potentially different roles that EPU may play during the pandemic, we add the interaction term of D_epid and rEPU in our model:
The denotations in Eq. ( 3 ) are similar to what we defined previously. However, instead of using one proxy to predict the market crashes, we also tried out to add the interaction terms, including the EPU and the period after the pandemic.
Empirical results
Covid-19 and stock market crash risk.
We start our empirical analysis by only considering the explanatory variable rCases , and the results are shown in Table 4 . As we expected, all the coefficient of rCases t-1 is negative and significant at 1% level, indicating the severity of the pandemic has a direct negative impact on the crash risk of the US stock market. This finding is also consistent with Liu et al. ( 2021 )’s work in the Chinese stock market. Furthermore, we also witness that the effects of the number of confirmed are not persistent because it only happens in one previous day after controlling the other previous days.
While the number of cases is used as the good predictive factor to market changes on the onset of COVID-19 pandemic (Zhang et al. 2020 ; Ashraf 2020a , 2020b ), our findings are the first evidence to contribute to the extant literature that this factor could predict the market crashes. However, Goodell ( 2020 ) might argue that other factors could drive the equity market shocks. One of the potential determinants is the uncertainties which were raised from the economic and policy responses. In doing so, we used the EPU suggested by Baker et al. ( 2016 ) and Baker et al. ( 2020b , a ) to explore the market situation in this difficult time.
Does and how does EPU matter?
We further consider the role of economic policy uncertainty in affecting the crash risk in this section. We first only add the explanatory variable rEPU into our model, and the results are reported in Table 5 . We can see that all the coefficients of \(rEPU_{t}\) except that in column (4) is negative and significant at 5% level. It is consistent with our exception that increased economic policy uncertainty will increase the possibility of a stock market crash. Although the existence of economic and policy uncertainties exhibit the negative impacts on the probability of market downturn, these effects are less pronounced when adding up with the different time horizons. This implies that the weakly predictive power of Baker et al. ( 2020b , a )’s proxy on the US market crash. Previously, the number of infected cases are likely to be robust when having different time zones while this magnitude of EPU is decreasing and turns into insignificant coefficients (in column 4). To our great surprise, we observed the strengthened effects of skewness, itself, by the increase in its coefficients (from 0.2032 to 0.2042, and significant at 1% level). The existing literature of Altig et al. ( 2020 ) offers insights about the different regimes in terms of EPU. In addition, several empirical evidence confirms the relationship between EPU and market volatilities by wave-let approaches (Choi 2020 ; Sharif et al. 2020 ) while our estimates shed new light on this linear dependence between market skewness and the standard proxy for the shaken markets. Notwithstanding its blur effects, in other words, improving the stability of economic policies will help prevent a stock market crash.
When considering the influence of rCases and rEPU at the same time, we use the Akaike information criterion (AIC) and Schwarz criterion (SC) to determine the lag length of the variables. The results are shown in Table 6 . Although the optimal lag lengths obtained according to different criteria are different, the conclusion that the severity of COVID-19 and EPU have negative influences on the crash risk of stock market is still holding. In terms of explanatory power, when the variables rCases and rEPU are added into the model simultaneously, its explanatory power has been significantly improved. For example, comparing the results in the first column of Table 6 with the results in the second column of Table 5 , we can see that after adding rEPU , the adj-R 2 increases from 0.0497 to 0.0504. While comparing the results from the second column of Table 6 with that in the first column of Table 5 , the Adj-R 2 has increased from 0.0479 to 0.0502. To sum up, our models remain robust when using the simultaneous appearance of both EPU and the number of infected cases. It is worth noting that both factors deteriorate the market by imposing the higher crashes on the onset of the pandemic outbreaks. However, we also have the caveat that these following estimates did not include the effects of the exact time of the pandemic. Schell et al. ( 2020 ) indicated that the adverse effects of COVID-19 are likely to be higher after the 21st January 2020, Public Health Risk Emergency of International Concern (PHEIC) announcements. In doing so, we will take a closer look at the market crash by adding the interactive terms to examine whether the EPU is strengthened after this event.
Infected cases, EPU and the difficult time
Finally, we consider whether the impact of EPU on crash risk is different during the pandemic. We know that when a crisis event occurs, the government usually needs to formulate a series of policies to alleviate the negative impact of the crisis. Therefore, economic policy uncertainty during the COVID-19 crisis may play a different role from the normal period.
The relevant results are given in Table 7 . We can see that the coefficients of the interaction terms are negative and significant at the 5% level, while the coefficients of rEPU are not significant anymore. More accurately, take the results from column (1) for example, the coefficient of rEPU during the before-pandemic period is − 0.0062 with t-statistics − 1.5967 (not significant at 10% level). While during the pandemic period, the coefficient of rEPU is − 0.0408 with t-statistics = − 2.8666. It indicates that in a regular period, changes in economic policy uncertainty do not significantly correlate with the risk of stock market crashes. Moreover, during the pandemic, the increase in uncertainty caused by COVID-19 will increase the stock market crash risk. The timely formulation of response policies can reduce this uncertainty, thereby helping to prevent the possibility of extreme risks in the stock market.
We can draw some interesting conclusions from our findings with the predictive power of both factors, namely EPU and infected cases when confronted with the role of the policy announcement. First, our findings are also in line with the existing literature about the prominent time, which might induce market shocks (Schell et al. 2020 ; Goodell and Huynh 2020 ). More noticeably, while EPU did not have any correlation with equity market crashes before the coronavirus announcement, its effect turns out the observatory factor to the probability of the US equity market meltdown in the late period. It emphasizes the critical role of declaring the pandemic situation, which could drive the market changes. Second, the number of confirmed cases are still significant when we tried out different estimates. Therefore, our findings also confirm the extant literature of the predictive characteristic of a number of confirmed cases (Phan and Narayan 2020 ; Haroon and Rizvi 2020 ). Before offering policy implications, we offer some different robustness checks to ensure what our empirical results are not spurious.
Robustness results from alternative EPU indices
There are two alternative measures about EPU: rEMU and rEMV-ID , representing the equity market uncertainties for the normal time and the pandemic period. In this section, we use these two variables to redo our empirical analysis. The results are reported in Tables 8 and 9 , respectively. In general, the results are robust.
One of the main points from Table 9 is the significant coefficients of the number of confirmed cases ( rCases ) in one previous term, implying that the increase in cases predicts the higher likelihood of a market crash. Besides, the Economic Uncertainty Index negatively correlates with the market downturn when confronting with the post-event announcement of coronavirus pandemic. Ultimately, what we found before still holds true when replacing with Equity Market Volatility: Infectious Disease Tracker ( EMV-ID ). Notwithstanding the current empirical evidence employing this proxy (Bai et al. 2020 ; Bouri et al. 2020 ), our results indicate the effects of market uncertainties with various proxies on the market crashes. Therefore, our results are still robust for further policy implications and discussion.
Conclusions
This paper has examined the role of economic policy uncertainty in affecting the stock market crash. we use the conditional skewness from the GARCH-S model as the proxy for crash risk. Our findings shed new light that COVID-19 will increase the crash risk of the US stock market. Second, we find that the increase in economic policy uncertainty has the risk of triggering a stock market crash. However, further evidence suggests that this effect of EPU is significant only during the pandemic. It reminds us that proactive policies should be implemented in time during crisis events to reduce the economic policy uncertainty, which is very important to prevent the stock market from crashing effectively.
There are some policy implications which could be raised from these following findings and results. First, not only investors but also policymakers should pay necessary attention to the growing of infected cases. Our findings emphasize the predictive power of this factor to the market shocks because the rising cases are considered as the signal to the market movement to the negative side. Thus, the tracking record is mandatory to avoid the extreme event, which might cause huge losses when investing in this challenging time. Second, the role of news, mainly since January with restrictions of travelling, national curfews, the bans on public gatherings, and social distancing, would induce the harmful shakes of the market. Thus, it is an underlying mechanism to transmit the potential risk to the market crash after controlling other factors. Concomitantly, the process of reviewing the news content might help investors avoiding the sudden adverse shocks when the frequencies of negative words, relevant to the uncertainties because these elements are contributing to the market uncertainty and then its correlation with the market meltdown.
A caveat should be included in this analysis, that the COVID-19 pandemic is still in a nascent period of rapid growth. Thus, what may be right for the situation currently is likely to change until the other outbreak, the appearance of vaccine, or other factors, in some manner. Hence, this study just provides the preliminary results to understand how the infected cases and EPU are correlated with the market crash. Future research might focus on the dynamics of this effect. Thus, this avenue is still promising indeed.
Availability of data and materials
The datasets analyzed during the current study are available from the following websites, https://ourworldindata.org/coronavirus and https://fred.stlouisfed.org/ .
The F-statistics of ARCH test is 104.0580, which shows the GARCH structure is applicable here.
Al-Awadhi AM, Al-Saifi K, Al-Awadhi A, Alhamadi S (2020) Death and contagious infectious diseases: impact of the COVID-19 virus on stock market returns. J Behav Exp Financ 27:100326
Article Google Scholar
Alizadeh S, Brandt MW, Diebold FX (2002) Range-based estimation of stochastic volatility models. J Financ 57(3):1047–1091
Al‐Thaqeb SA, Algharabali BG, Alabdulghafour KT (2020) The pandemic and economic policy uncertainty. Int J Finance Econ
Altig D, Baker S, Barrero JM, Bloom N, Bunn P, Chen S, Mizen P (2020) Economic uncertainty before and during the COVID-19 pandemic. J Public Econ 191:104274
Ambros M, Frenkel M, Huynh TLD, Kilinc M (2020) COVID-19 pandemic news and stock market reaction during the onset of the crisis: evidence from high-frequency data. Appl Econ Lett 1–4
Ashraf BN (2020a) Economic impact of government interventions during the COVID-19 pandemic: International evidence from financial markets. J Behav Exp Financ 27:100371
Ashraf BN (2020b) Stock markets’ reaction to COVID-19: cases or fatalities? Res Int Bus Financ 54:101249
Baek S, Mohanty SK, Mina G (2020) COVID-19 and stock market volatility: an industry level analysis. Financ Res Lett 37:101748
Bai L, Wei Y, Wei G, Li X, Zhang S (2020) Infectious disease pandemic and permanent volatility of international stock markets: a long-term perspective. Finance Res Lett 101709
Baker SR, Bloom N, Davis SJ (2016) Measuring economic policy uncertainty. Q J Econ 131:1593–1636
Baker SR, Bloom N, Davis SJ, Kost K, Sammon M, Viratyosin T (2020a) The unprecedented stock market reaction to COVID-19. Rev Asset Pricing Stud 10(4):742–758
Baker SR, Bloom N, Davis S, Terry SJ (2020) Covid-induced economic uncertainty. National Bureau of Economic Research
Bouri E, Demirer R, Gupta R, Pierdzioch C (2020) Infectious diseases, market uncertainty and oil market volatility. Energies 13(16):4090
Brogaard J, Detzel A (2015) The asset-pricing implications of government economic policy uncertainty. Manage Sci 61:3–18
Caggiano G, Castelnuovo E, Kima R (2020) The global effects of Covid-19-induced uncertainty. Econ Lett 194:109392
Chen J, Hong H, Stein JC (2001) Forecasting crashes: trading volume, past returns, and conditional skewness in stock prices. J Financ Econ 61:345–381
Choi SY (2020) Industry volatility and economic uncertainty due to the COVID-19 pandemic: evidence from wavelet coherence analysis. Financ Res Lett 37:101783
Dai P-F, Xiong X, Zhou W-X (2021) A global economic policy uncertainty index from principal component analysis. Financ Res Lett 40:101686
Djurovic G, Djurovic V, Bojaj MM (2020) The macroeconomic effects of COVID-19 in Montenegro: a Bayesian VARX approach. Financ Innov 6:40
Goodell JW (2020) COVID-19 and finance: Agendas for future research. Financ Res Lett 35:101512
Goodell JW, Huynh TLD (2020) Did Congress trade ahead? Considering the reaction of US industries to COVID-19. Financ Res Lett 36:101578
Gulen H, Ion M (2016) Policy uncertainty and corporate investment. Rev Financ Stud 29(3):523–564
Google Scholar
Haroon O, Rizvi SAR (2020) Flatten the curve and stock market liquidity—an inquiry into emerging economies. Emerg Mark Financ Trade 56(10):2151–2161
Kim J, Li Y, Zhang L (2011) Corporate tax avoidance and stock price crash risk: firm-level analysis. J Financ Econ 100(3):639–662
Kim J, Li Y, Zhang L (2011) CFOs versus CEOs: equity incentives and crashes. J Financ Econ 101(3):713–730
Kräussl R, Lehnert T, Senulytė S (2016) Euro crash risk. J Empir Financ 38:417–428
León Á, Rubio G, Serna G (2005) Autoregresive conditional volatility, skewness and kurtosis. Q Rev Econ Finance 45:599–618
Liu Z, Zhang T, Zhang, G (2020) Heterogeneous stock market reactions to COVID-19: More hidden mechanisms. Available at SSRN 3697622
Liu Z, Huynh TDL, Dai P-F (2021) The impact of COVID-19 on the stock market crash risk in China. Res Int Bus Financ 57:101419
Mazur M, Dang M, Vega M (2020) COVID-19 and the March 2020 stock market crash. Evidence from S&P1500. Financ Res Lett 38:101690
Megaritis A, Vlastakis N, Triantafyllou A (2021) Stock market volatility and jumps in times of uncertainty. J Int Money Financ 113:102355
Onali E (2020) Covid-19 and stock market volatility. Available at SSRN 3571453
Papadamou S, Fassas A, Kenourgios D, Dimitriou D (2020) Direct and indirect effects of COVID-19 pandemic on implied stock market volatility: Evidence from Panel Data Analysis. MPRA Paper
Phan DHB, Narayan PK (2020) Country responses and the reaction of the stock market to COVID-19-A preliminary exposition. Emerg Mark Financ Trade 56(10):2138–2150
Ramelli S, Wagner AF (2020) Feverish stock price reactions to covid-19. Swiss Finance Institute Research Paper Series
Schell D, Wang M, Huynh TLD (2020) This time is indeed different: a study on global market reactions to public health crisis. J Behav Exp Finance 100349
Sharif A, Aloui C, Yarovaya L (2020) COVID-19 pandemic, oil prices, stock market, geopolitical risk and policy uncertainty nexus in the US economy: fresh evidence from the wavelet-based approach. Int Rev Financ Anal 70:101496
Wang J, Lu X, He F, Ma F (2020) Which popular predictor is more useful to forecast international stock markets during the coronavirus pandemic: VIX vs EPU? Int Rev Financ Anal 72:101596
Wen F, Xu L, Ouyang G, Kou G (2019) Retail investor attention and stock price crash risk: evidence from China. Int Rev Financ Anal 65:101376
Wen F, Zhao Y, Zhang M, Hu C (2019) Forecasting realized volatility of crude oil futures with equity market uncertainty. Appl Econ 51:6411–6427
Yousaf I, Ali S (2020) Discovering interlinkages between major cryptocurrencies using high-frequency data: new evidence from COVID-19 pandemic. Financ Innov 6:45
Youssef M, Mokni K, Ajmi AN (2021) Dynamic connectedness between stock markets in the presence of the COVID-19 pandemic: does economic policy uncertainty matter? Financ Innov 7(1):1–27
Zhang D, Hu M, Ji Q (2020) Financial markets under the global pandemic of COVID-19. Financ Res Lett 36:101528
Ziemba WT (2020) The COVID-19 Crash in the US Stock Market. Available at SSRN 3632410
Download references
Acknowledgements
Not applicable.
This research was supported by the National Natural Science Foundation of China (71861008, 72063005, U1811462, 71532009), the Natural Science Foundation of Hainan Province (718QN221, 2019RC151), and the Scientific Research Foundation of Hainan University (kyqd(sk)1809, kyqd1634). Toan Luu Duc Huynh acknowledges the generous funding from the University of Economics Ho Chi Minh City (Vietnam).
Author information
Authors and affiliations.
College of Management and Economics, Tianjin University, Tianjin, China
Peng-Fei Dai & Xiong Xiong
School of Business, East China University of Science and Technology, Shanghai, China
Peng-Fei Dai
Management School, Hainan University, Haikou, China
Zhifeng Liu
Chair of Behavioral Finance, WHU-Otto Beisheim School of Management, Vallendar, Germany
Toan Luu Duc Huynh
School of Banking, University of Economics Ho Chi Minh City, Ho Chi Minh City, Vietnam
School of Economics, Hainan University, Haikou, China
Jianjun Sun
You can also search for this author in PubMed Google Scholar
Contributions
P-FD conducted the conceptualization analysis, data collection and formal analysis. XX coordinated the writing on the different sections and managed the project administration. ZL was in charge of the software and wrote the methodology and the original draft. TLDH revised the original draft. JS provided the funding acquisition. All authors read and approved the final manuscript.
Corresponding author
Correspondence to Zhifeng Liu .
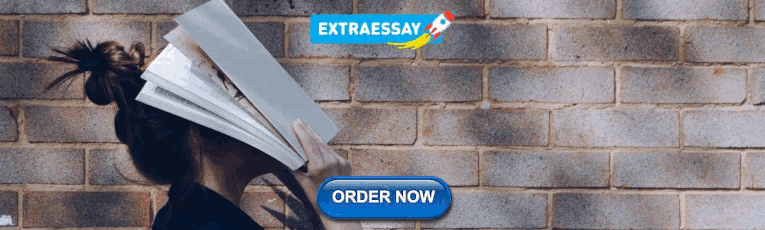
Ethics declarations
Competing interests.
The authors declare that they have no competing interests.
Additional information
Publisher's note.
Springer Nature remains neutral with regard to jurisdictional claims in published maps and institutional affiliations.
Rights and permissions
Open Access This article is licensed under a Creative Commons Attribution 4.0 International License, which permits use, sharing, adaptation, distribution and reproduction in any medium or format, as long as you give appropriate credit to the original author(s) and the source, provide a link to the Creative Commons licence, and indicate if changes were made. The images or other third party material in this article are included in the article's Creative Commons licence, unless indicated otherwise in a credit line to the material. If material is not included in the article's Creative Commons licence and your intended use is not permitted by statutory regulation or exceeds the permitted use, you will need to obtain permission directly from the copyright holder. To view a copy of this licence, visit http://creativecommons.org/licenses/by/4.0/ .
Reprints and permissions
About this article
Cite this article.
Dai, PF., Xiong, X., Liu, Z. et al. Preventing crash in stock market: The role of economic policy uncertainty during COVID-19. Financ Innov 7 , 31 (2021). https://doi.org/10.1186/s40854-021-00248-y
Download citation
Received : 14 December 2020
Accepted : 22 April 2021
Published : 28 April 2021
DOI : https://doi.org/10.1186/s40854-021-00248-y
Share this article
Anyone you share the following link with will be able to read this content:
Sorry, a shareable link is not currently available for this article.
Provided by the Springer Nature SharedIt content-sharing initiative
- Economic policy uncertainty
JEL Classification

An official website of the United States government
The .gov means it’s official. Federal government websites often end in .gov or .mil. Before sharing sensitive information, make sure you’re on a federal government site.
The site is secure. The https:// ensures that you are connecting to the official website and that any information you provide is encrypted and transmitted securely.
- Publications
- Account settings
Preview improvements coming to the PMC website in October 2024. Learn More or Try it out now .
- Advanced Search
- Journal List
- Elsevier - PMC COVID-19 Collection

COVID-19 and the march 2020 stock market crash. Evidence from S&P1500
Mieszko mazur.
a IESEG School of Management (LEM-CNRS 9221), Parvis de la Défense, 92800 Puteaux, France
b The University of Da Nang, University of Economics, 71 Ngu Hanh Son Street, Danang City 550000, Vietnam
Miguel Vega
This paper investigates the US stock market performance during the crash of March 2020 triggered by COVID-19. We find that natural gas, food, healthcare, and software stocks earn high positive returns, whereas equity values in petroleum, real estate, entertainment, and hospitality sectors fall dramatically. Moreover, loser stocks exhibit extreme asymmetric volatility that correlates negatively with stock returns. Firms react in a variety of different ways to the COVID-19 revenue shock. The analysis of the 8K and DEF14A filings of poorest performers reveals departures of senior executives, remuneration cuts, and (most surprisingly) newly approved cash bonuses and salary increases.
1. Introduction
March 2020 saw one of the most dramatic stock market crashes in history. In barely four trading days 2 , Dow Jones Industrial Average (DJIA) plunged 6,400 points, an equivalent of roughly 26%. The crash was caused by government's reaction to a novel coronavirus (COVID-19), a disease which originated in the Chinese city of Wuhan in December 2019 and quickly spread around the world causing a pandemic. Because the virus is highly contagious and fatal, the authorities imposed strict quarantines on their populations and ordered the shut-down of the bulk of business activity. At present, US economy seems to be affected most with the rate of unemployment reaching above 20% 3 .
In this paper we investigate the effect of COVID-19 on the stock market behavior during the crash of March 2020 using the universe of S&P1500 firms. Clearly, COVID-19 represents a massive revenue shock to the economy. Since most of the businesses are prohibited from remaining fully operational during the imposed quarantine, they choose to adjust their labor costs by laying off employees. Consequently, this leads to the sharp reduction in consumption and economic output, lowering the stream of expected future cash flows. Nevertheless, COVID-19, may not necessarily be equally detrimental to all firms and industries. Whereas most sectors suffer and their stock prices collapse, some other may benefit from the pandemic and the resulting lockdown. This paper attempts to answer these questions by examining the differential stock price reactions to the rapid spread of the coronavirus and the abrupt government interventions that triggered the crash. We also investigate the implications for the stock price volatility. Finally, by using hand-collected data we examine firms’ immediate responses to COVID-19.
We find that that approximately 90% of the S&P1500 stocks generate asymmetrically distributed large negative returns ( Fig. 1 ). When analyzing single-day extreme events, namely Black Monday, Black Thursday, and Black Monday II, we find that firms that operate in crude petroleum sector are hit hardest and lose over 60% of their market values in a day. In contrast, firms in natural gas and chemicals sectors improve their market valuations and earn positive returns of more than 10%, on average. Further, we study industry-level patterns and show that during March 2020 stock market crash the best performing industries include healthcare, food, software and technology, as well as natural gas. The superior performers in these industries yield a positive monthly return of over 20%. On the other hand, sectors including crude petroleum, real estate, as well as hospitality and entertainment experience rapid descent of their market capitalizations and plunge over 70%.

March 2020 stock returns.
The plot shows the relative frequency distribution of monthly stock returns for the universe of the S&P1500 firms in March 2020. The data are derived from Thomson Reuters Eikon.
Stock price crashes unfold extreme volatility. We document extreme asymmetric volatility for S&P1500 firms and find that volatility correlates negatively with realized stock returns ( Fig. 2 ). The highest level of volatility is observed for stocks in the crude petroleum sector whose prices tumble most. For example, Gulfport Energy displays the widest daily amplitude of price movement of roughly 130%. Entertainment and hospitality industries are highly volatile as well at an average level of about 20%. It is worth noting that in normal times, daily volatility is an order of magnitude lower.

March 2020 stock return and stock return volatility.
The plot shows the relationship between monthly stock returns and daily stock return volatility for the universe of the S&P1500 firms in March 2020. Because of its extreme value, Gulfport Energy data point has been omitted from the plot (see Table 2 ). The data are derived from Thomson Reuters Eikon.
In the final analysis, we investigate how poorest performers react to COVID-19 and the associated revenue shock. We examine the information disclosed in 8K and DEF14A reports filed with the Securities and Exchange Commission (SEC) in March and April 2020. We find that firms respond differently to profit uncertainty induced by COVID-19. Some of them reduce salaries for their top executives and board members. The other reduce the amount of dividend, however, dividends are never suspended. Surprisingly, a subset of firms increases salaries for senior executives or approve new bonus awards. Arguably, this latter behavior could be viewed as manifestation of poor corporate governance.
Our paper extends the literature on stock market crashes by providing insights into the March 2020 collapse. At least 20 percent decline in the main index can be defined as a stock market crash ( Mishkin and White, 2002 ). On October 28-29, 1929 DJIA declined by 24.5%, whereas on October 19, 1987, by 22.6%. For the sake of comparison, the sequence of panic selling on March 9, 12, 16 and 23 of 2020 led to the cumulative 26% percent drop of DJIA. Interestingly, financial crisis of 2007-2009 did not produce a sharp fall of comparable magnitude and the stock market decline was, instead, extended in time ( Anand, Puckett, Irvine, and Venkataraman, 2013 ). Further, the March 2020 stock market crash does not reflect the bursting of asset price bubble. To the contrary, perhaps for the first time in economic history the crash occurs when fundamentals are sound and the slump in market capitalizations is rather due to the lockdown of populations and the shutdown of most of manufacturing and service business. Next, we complement existing studies and find extreme negative asymmetries in stock return volatility ( Chen, Hong, and Stein, 2001 ). Further, our study suggests that March 2020 stock market crash may uncover corporate governance scandals similarly to the ones that emerged in the early 2000s ( Nelson, Price, and Rountree, 2008 ; Bhagat and Bolton, 2013 ). Finally, our paper extends emerging literature on COVID-19 and financial markets ( Akhtaruzzaman, Boubaker, and Sensoy, 2020 ; Akhtaruzzaman, Boubaker, Lucey, and Sensoy, 2020 ; Baker, Bloom, Davis, Kost, Sammon, and Viratyosin, 2020 ; Zhang, Hu, and Ji, 2020 ).
The paper is organized as follows. Section 2 describes the data, Section 3 presents the result, and Section 4 concludes.
We use the universe of the Standard and Poor's (S&P) 1,500 firms for the month of March 2020. Stock price and trading volume data come from Thomson Reuters (TR) Eikon. To derive complementary information, we match these data by ticker with CRSP Daily Stock File and Compustat Index Constituents. For the industry-level analysis we exclude very few firms that could not be matched due to ticker incompatibility. For example, in TR Eikon, Aqua America's ticker is WTRG, whereas in CRSP it is WTR. Moreover, we exclude firms that do IPOs in March 2020 (e.g., Otis Worldwide), as IPO stocks begin trading in the middle of the month. Finally, we hand collect information on fundamentals from 8K and DEF14A filings available on SEC's EDGAR.
3.1. Panic selling: Black monday, Black tuesday, Black monday II
Quite surprisingly, natural gas companies are among winner stocks earning between +17% and +11% daily on Black Monday and Black Tuesday. One possible reason for the increase in stock prices of these firms is that for crude petroleum producers natural gas is a byproduct extracted only during extraction of oil. Since oil prices declined sharply in March 2020, crude producers decided to reduce the output of oil and therefore they automatically reduced production of natural gas. Needless to say, this had a positive impact on market prices of natural gas and expected future cashflows of natural gas producers. Similarly, firms in food industry (e.g., United Natural Foods) and chemicals (e.g., Kraton) experience a significant price jump of 20% in a single day.
At the other extreme, crude petroleum stocks plunge drastically by more than 60%. Stocks in hospitality, real estate, and entertainment sectors suffer a decline of similar magnitude. For example, Eldorado Resorts or EPR Properties each lose more than 60% of their values.
3.2. March 2020 stock market crash and industry-level performance
The best performing industries include healthcare and medical devices, which is understandable on the basis that virtually every country in the world is going through catastrophic mortality deterioration due to COVID-19 ( Table 2 ). Another well performing sector is food and grocery distribution that is currently benefiting from the upward shift in demand, as restaurants and eateries have been shut down for the public. Software and technology sector has performed equally well. For example, providers of resources for the remote work (e.g., Citrix) and multinational networking services (e.g., Netgear) experience an unusual surge in demand. This is due to the fact that a large fraction of employees moved to online working, which spurred the need for appropriate software and hardware. Finally, natural gas industry is another big winner for the reasons already stated above. Overall, each of these these industries earn a monthly return of over +20%.
Industry return and volatility during the March 2020 stock market crash.
To identify industry clusters, we use the top and bottom 2% of the S&P1500 firms sorted by monthly stock return estimated for March 2020. For ease of exposition, we merge related industries into one. We report the industry, once it appears more than twice within each 2% tail. Ret. denotes monthly return using daily data. Sigma denotes average daily volatility. The data are derived from Thomson Reuters Eikon.
Among the industries that perform the worst are crude petroleum and oil services (-77%), real estate (-72%), and hospitality and entertainment (-70%).
What explains rapid declines in stock prices for the great majority of industries? Many sectors are in a position of strength and despite that their values collapse. Theoretical underpinning for these findings relies arguably on the theory of economic relationships between linked firms, where a shock to one firm has a resulting effect on all the linked partners (e.g., customers and suppliers) ( Bernanke, 1983 ; Cohen and Frazzini, 2008 ). Therefore, even for unrelated industries, a revenue shock to one firm may have a negative revenue effect on all economically related firms, precipitating a cascade of price declines in the stock market.
3.3. Additional analyses
Following the event-study methodology described in Peterson (1989) , we assess firm and industry performance relative to the benchmark on Black Monday, Black Thursday, and Black Monday II ( Table 3 ). In comparison to raw returns presented in Tables 1 and and2 , 2 , we expect the event-study returns to be significantly higher for well- and poor-performing stocks and industries during the indicated event days, due to the highly negative returns on the market as a whole. As expected, for example, on Black Monday II, the abnormal return to superior performers is roughly +32%, whereas the equal-weighted raw return is +18.7%. Likewise, the return to the worst performing stocks on the same day is -36.3%, whereas the equal-weighted raw return is considerably lower (-51.4%) ( Table 1 ).
Single-day returns during the March 2020 stock market crash.
The data are derived from Thomson Reuters Eikon. Ret. denotes daily return.
Additional analyses.
This table reports event-study results. The event dates are defined as in Table 1 . Abnormal returns are measured over a single trading day on March 9, 12, and 16, 2020 using the mean-adjusted return model relative to the S&P500, S&P400, or S&P600 value-weighted index depending on the index constituent stock. Superior (Worst) performers are grouped based on the identification used in Table 1 . The industries are defined as in Table 2 . AR denotes mean abnormal return. ***, **, * indicate significance at the 1, 5, and 10% levels, respectively.
3.4. Extreme volatility
Stocks that exhibit extreme negative returns during the March 2020 crash exhibit also extreme volatility ( Fig. 1 ). Crude petroleum stocks are particularly volatile irrespective of firm market capitalization. In one instance (Gulfport Energy) the level of volatility reaches staggering 130% ( Table 4 ). Entertainment and hospitality stocks are also marked by high volatility in the neighborhood of 20%. A similar picture emerges when we analyze volatility at the industry level ( Table 2 ). High return industries are significantly less volatile (6% to 9%), whereas industries that plummet experience excessive volatility (around 20%).
Volatility during the March 2020 stock market crash.
Close price is the close stock price on March 31, 2020. Sigma denotes average daily volatility. The data are derived from Thomson Reuters Eikon.
Existing finance theories suggest that asymmetric volatility (increase of volatility together with negative returns) can be explained either by heterogenous believes and short-sale constraints of certain types of investors ( Hong and Stein, 1999 ), or alternatively, by operating and financial leverage effects ( Schwert, 1989 ; Bekaert and Wu, 1997 ). The context of COVID-19 stock market crash, however, differs from other crashes that occurred in the past. At present, most of the listed firms have valuable assets in place and strong free cash flow potential indicating that the collapse of stock prices did not occur due to popping of the bubble. Our results suggest that a better model of extreme volatility would be one that recognizes that fact.
3.5. Firms’ response to COVID-19
COVID-19 represents a revenue shock to majority of industries that remain shut down during the quarantine period. From this perspective, March 2020 stock market crash does not occur due to weak economic fundamentals. Nevertheless, because of suppressed consumer spending, firms revise downwards their earnings prospects. Consequently, this leads to market's reassessment of firms’ values and a large fall of stock prices as demonstrated in this study.
We analyze disclosures made by firms filing current reports (8K) and proxy statements (DEF14A) in March through April 2020. We choose a subset of crude oil firms affected most by March 2020 stock market crash. As expected and indicated in Table 5 , firms decide to lower the amount of dividend payouts, reduce compensation for senior executives and directors, cut capital expenditures as well as other costs. Dividends, however, are never reduced to zero, meaning that despite economic contraction, firms that have been affected most by COVID-19 continue paying out cash to investors. Most surprisingly, some firms increase salaries for senior management and award cash bonuses approved by boards as late as March 2020 (e.g., Gulfport Energy). Moreover and equally intriguing, some firms choose not to respond at all to the current crisis (e.g., Oasis Petroleum).
Firms’ response to COVID-19.
This table summarizes firms’ responses to the revenue shock caused by the COVID-19 pandemic. We select a set of crude petroleum producers, as this sector has been hit hardest by the March 2020 stock market crash. The data are hand-collected from 8K and DEF14 reports filed with the SEC in March and April 2020. Ret. denotes monthly return using daily data estimated for March 2020.
The question is why we observe this differential reaction to COVID-19 for firms in the same sector. As shown theoretically, corporate governance has a profound impact on firm decision-making ( Adams and Ferreira, 2007 ). Firms may have weak, management-friendly boards unaccountable to shareholders they represent ( Almazan and Suarez, 2003 ). Our observations seem to be consistent with these theories. For instance, Gulfport Energy has recently been targeted by an activist investor Firefly who criticized it for not scheduling shareholders’ meeting to discuss capital allocation and strategy decisions under COVID-19. Gulfport was also condemned in the early March 2020 for having incompetent and inattentive board. A deeper insight into the sample firms’ corporate governance shows that, for example, Oasis Petroleum has two directors that used to hold managerial positions in the same organization at the same time. Also, in the last fiscal year its nominating and corporate governance committees were significantly less active than compensation committee. Obviously, weak boards would allow managers to appropriate resources in the form of e.g., higher remuneration, and would overall pay less attention to shareholders’ interests.
4. Conclusion
The collapse of stock prices in March 2020 marks one of the biggest stock market crashes in history. As measured by DJIA, the market fell 26% in four days. The crash was caused by COVID-19 pandemic and government's dramatic response to it. According to the latest statistics, the US GDP decreased 4.8 percent in the first quarter of 2020 and the unemployment rate spiked to above 20%.
In this study, we show that during March 2020 stock market crash stocks in healthcare, food, natural gas, and software sectors perform abnormally well generating high returns, whereas firms operating in crude petroleum, real estate, entertainment and hospitality sectors plummet considerably losing more than 70% of their market capitalizations. Loser stocks have more asymmetric movements and exhibit extreme volatility that correlates negatively with stock returns. We also show that a subset of poorest performers respond to the revenue shock adequately by cutting costs, including remuneration for top management and board members, whereas others increase salaries and implement new cash awards. The latter behavior may signal poor corporate governance and indicates a fruitful area for future research.
Author Statement
Mieszko Mazur, Man Dang, and Miguel Vega contributed equally to the development of the manuscript
2 March 9, 12, 16 and 23, 2020
3 https://faculty.fuqua.duke.edu/~charvey/Audio/COVID/COVID-Harvey.html
- Adams R.B., Ferreira D. A theory of friendly boards. J. Finance. 2007; 62 (1):217–250. [ Google Scholar ]
- Akhtaruzzaman M, Boubaker S, Sensoy A. Financial contagion during COVID–19 crisis. Finance Res. Let. 2020 forthcoming. [ PMC free article ] [ PubMed ] [ Google Scholar ]
- Akhtaruzzaman, M, Boubaker, S, Lucey, B.M. and Sensoy, A, 2020. Is gold a hedge or safe haven asset during COVID–19 crisis?Available at SSRN: https://ssrn.com/abstract=3621358 .
- Almazan A., Suarez J. Entrenchment and severance pay in optimal governance structures. J. Finance. 2003; 58 (2):519–547. [ Google Scholar ]
- Anand A., Puckett A., Irvine P., Venkataraman K. Market crashes and institutional trading: evidence from US equities during the financial crisis of 2007-08. J. Financ. Econ. 2013; 108 :773–797. [ Google Scholar ]
- Baker S.R., Bloom, N., Davis, S.J., Kost, K.J., Sammon, M.C., Viratyosin, T., 2020. The unprecedented stock market impact of COVID-19. NBER Working Paper.
- Bekaert, G. and Wu, G., 1997. Asymmetry volatility and risk in equity markets (No. 6022). NBER Working Paper.
- Bernanke, B.S., 1983. Non-monetary effects of the financial crisis in the propagation of the great depression (No. w1054). NBER Working Paper.
- Bhagat S., Bolton B. Director ownership, governance, and performance. J. Financ. Quant. Anal. 2013; 48 (1):105–135. [ Google Scholar ]
- Chen J., Hong H., Stein J.C. Forecasting crashes: trading volume, past returns, and conditional skewness in stock prices. J. Financ. Econ. 2001; 61 (3):345–381. [ Google Scholar ]
- Cohen L., Frazzini A. Economic links and predictable returns. J. Finance. 2008; 63 (4):1977–2011. [ Google Scholar ]
- Hong, H. and Stein, J.C., 1999. Differences of opinion, rational arbitrage and market crashes, NBER Working Paper.
- Mishkin, F.S., and E.N. White, 2002. US stock market crashes and their aftermath: implications for monetary policy. NBER Working Paper.
- Nelson K.K., Price R.A., Rountree B. The market reaction to Arthur Andersen's role in the Enron scandal: loss of reputation or confounding effects? J. Account. Econ. 2008; 46 (2-3):279–293. [ Google Scholar ]
- Peterson P.P. Event studies: a review of issues and methodology. Q. J. Bus. Econ. 1989:36–66. [ Google Scholar ]
- Schwert G.W. Why does stock market volatility change over time? J. Finance. 1989; 44 (5):1115–1153. [ Google Scholar ]
- Zhang, D., Hu, M., Ji, Q., 2020 Financial markets under the global pandemic of COVID-19. Finance Res. Lett. Forthcoming. [ PMC free article ] [ PubMed ]

Stock Market Crash of 1929
October 1929.

The Roaring Twenties roared loudest and longest on the New York Stock Exchange. Share prices rose to unprecedented heights. The Dow Jones Industrial Average increased six-fold from sixty-three in August 1921 to 381 in September 1929. After prices peaked, economist Irving Fisher proclaimed, “stock prices have reached ‘what looks like a permanently high plateau.’” 1
The epic boom ended in a cataclysmic bust. On Black Monday, October 28, 1929, the Dow declined nearly 13 percent. On the following day, Black Tuesday, the market dropped nearly 12 percent. By mid-November, the Dow had lost almost half of its value. The slide continued through the summer of 1932, when the Dow closed at 41.22, its lowest value of the twentieth century, 89 percent below its peak. The Dow did not return to its pre-crash heights until November 1954.
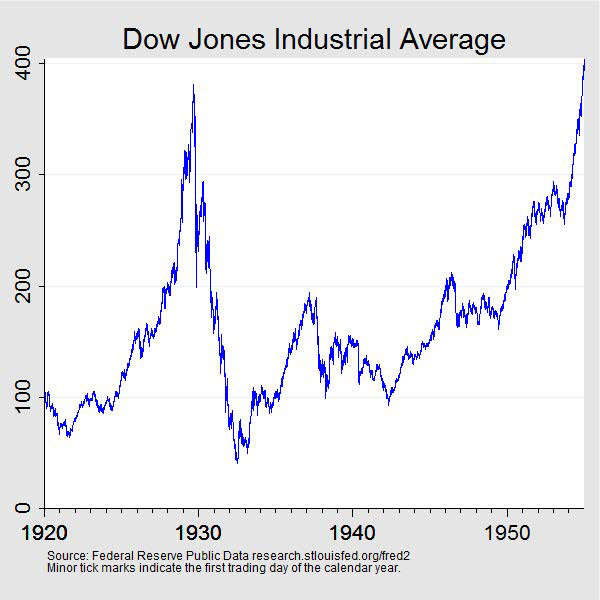
The financial boom occurred during an era of optimism. Families prospered. Automobiles, telephones, and other new technologies proliferated. Ordinary men and women invested growing sums in stocks and bonds. A new industry of brokerage houses, investment trusts, and margin accounts enabled ordinary people to purchase corporate equities with borrowed funds. Purchasers put down a fraction of the price, typically 10 percent, and borrowed the rest. The stocks that they bought served as collateral for the loan. Borrowed money poured into equity markets, and stock prices soared.
Skeptics existed, however. Among them was the Federal Reserve. The governors of many Federal Reserve Banks and a majority of the Federal Reserve Board believed stock-market speculation diverted resources from productive uses, like commerce and industry. The Board asserted that the “Federal Reserve Act does not … contemplate the use of the resources of the Federal Reserve Banks for the creation or extension of speculative credit” (Chandler 1971, 56). 2
The Board’s opinion stemmed from the text of the act. Section 13 authorized reserve banks to accept as collateral for discount loans assets that financed agricultural, commercial, and industrial activity but prohibited them from accepting as collateral “notes, drafts, or bills covering merely investments or issued or drawn for the purpose of carrying or trading in stocks, bonds or other investment securities, except bonds and notes of the Government of the United States” (Federal Reserve Act 1913).
Section 14 of the act extended those powers and prohibitions to purchases in the open market. 3
These provisions reflected the theory of real bills, which had many adherents among the authors of the Federal Reserve Act in 1913 and leaders of the Federal Reserve System in 1929. This theory indicated that the central bank should issue money when production and commerce expanded, and contract the supply of currency and credit when economic activity contracted.
The Federal Reserve decided to act. The question was how. The Federal Reserve Board and the leaders of the reserve banks debated this question. To rein in the tide of call loans, which fueled the financial euphoria, the Board favored a policy of direct action. The Board asked reserve banks to deny requests for credit from member banks that loaned funds to stock speculators. 4 The Board also warned the public of the dangers of speculation.
The governor of the Federal Reserve Bank of New York, George Harrison, favored a different approach. He wanted to raise the discount lending rate. This action would directly increase the rate that banks paid to borrow funds from the Federal Reserve and indirectly raise rates paid by all borrowers, including firms and consumers. In 1929, New York repeatedly requested to raise its discount rate; the Board denied several of the requests. In August the Board finally acquiesced to New York’s plan of action, and New York’s discount rate reached 6 percent. 5
The Federal Reserve’s rate increase had unintended consequences. Because of the international gold standard, the Fed’s actions forced foreign central banks to raise their own interest rates. Tight-money policies tipped economies around the world into recession. International commerce contracted, and the international economy slowed (Eichengreen 1992; Friedman and Schwartz 1963; Temin 1993).
The financial boom, however, continued. The Federal Reserve watched anxiously. Commercial banks continued to loan money to speculators, and other lenders invested increasing sums in loans to brokers. In September 1929, stock prices gyrated, with sudden declines and rapid recoveries. Some financial leaders continued to encourage investors to purchase equities, including Charles E. Mitchell, the president of the National City Bank (now Citibank) and a director of the Federal Reserve Bank of New York. 6 In October, Mitchell and a coalition of bankers attempted to restore confidence by publicly purchasing blocks of shares at high prices. The effort failed. Investors began selling madly. Share prices plummeted.

Funds that fled the stock market flowed into New York City’s commercial banks. These banks also assumed millions of dollars in stock-market loans. The sudden surges strained banks. As deposits increased, banks’ reserve requirements rose; but banks’ reserves fell as depositors withdrew cash, banks purchased loans, and checks (the principal method of depositing funds) cleared slowly. The counterpoised flows left many banks temporarily short of reserves.
To relieve the strain, the New York Fed sprang into action. It purchased government securities on the open market, expedited lending through its discount window, and lowered the discount rate. It assured commercial banks that it would supply the reserves they needed. These actions increased total reserves in the banking system, relaxed the reserve constraint faced by banks in New York City, and enabled financial institutions to remain open for business and satisfy their customers’ demands during the crisis. The actions also kept short term interest rates from rising to disruptive levels, which frequently occurred during financial crises.
At the time, the New York Fed’s actions were controversial. The Board and several reserve banks complained that New York exceeded its authority. In hindsight, however, these actions helped to contain the crisis in the short run. The stock market collapsed, but commercial banks near the center of the storm remained in operation (Friedman and Schwartz 1963).
While New York’s actions protected commercial banks, the stock-market crash still harmed commerce and manufacturing. The crash frightened investors and consumers. Men and women lost their life savings, feared for their jobs, and worried whether they could pay their bills. Fear and uncertainty reduced purchases of big ticket items, like automobiles, that people bought with credit. Firms – like Ford Motors – saw demand decline, so they slowed production and furloughed workers. Unemployment rose, and the contraction that had begun in the summer of 1929 deepened (Romer 1990; Calomiris 1993). 7
While the crash of 1929 curtailed economic activity, its impact faded within a few months, and by the fall of 1930 economic recovery appeared imminent. Then, problems in another portion of the financial system turned what may have been a short, sharp recession into our nation’s longest, deepest depression.
From the stock market crash of 1929, economists – including the leaders of the Federal Reserve – learned at least two lessons. 8
First, central banks – like the Federal Reserve – should be careful when acting in response to equity markets. Detecting and deflating financial bubbles is difficult. Using monetary policy to restrain investors’ exuberance may have broad, unintended, and undesirable consequences. 9
Second, when stock market crashes occur, their damage can be contained by following the playbook developed by the Federal Reserve Bank of New York in the fall of 1929.
Economists and historians debated these issues during the decades following the Great Depression. Consensus coalesced around the time of the publication of Milton Friedman and Anna Schwartz’s A Monetary History of the United States in 1963. Their conclusions concerning these events are cited by many economists, including members of the Federal Reserve Board of Governors such as Ben Bernanke, Donald Kohn and Frederic Mishkin.
In reaction to the financial crisis of 2008 scholars may be rethinking these conclusions. Economists have been questioning whether central banks can and should prevent asset market bubbles and how concerns about financial stability should influence monetary policy. These widespread discussions hearken back to the debates on this issue among the leaders of the Federal Reserve during the 1920s.
- 1 Irving Fisher’s quote appeared in the New York Times on October 16, 1929, p. 8. Fisher made the comment in a speech at the monthly dinner of the Purchasing Agents Association at the Builders Exchange Club, 2 Park Avenue. At the time, Fisher was one of the nation’s most well-known and widely quoted economists. His comments came in response to a prediction on September 5 at the Annual National Business Conference by rival financial prognosticator, Roger Babson, that “sooner or later a crash is coming, and it may be terrific.” Babson’s comment was followed by a sharp decline in stock-market prices known as the Babson break. Fisher reiterated his faith in the stock market in a speech before the District of Columbia Bankers Association on October 23.
- 2 The Board made these statements in its famous letter from February 2, 1929. For the text of the letter and discussion of its implications see Chandler 1971, pp. 56-58.
- 3 In addition to assets that could serve as collateral for discount loans, Section 14 authorized open market purchases of (a) gold coins, bullion, and certificates, and (b) securities issued and guaranteed by “any State, county, district, political subdivision, or municipality in the continental United States” (Federal Reserve Act 1913).
- 4 These requests appear in the Board’s letter of February 2, 1929. See Chandler 1971, pp. 56-58.
- 5 In this brief essay, we focus on the clash of opinions between the leaders of the Federal Reserve Board in Washington, DC, and leaders of the Federal Reserve Bank of New York. Several of the authors that we cite also highlight this line of debate. We should note that leaders throughout the Federal Reserve System vigorously debated this issue, and differences of opinion existed between the Board and leaders of many banks and also within those leadership groups. At times, for example, members of the Federal Reserve Board disagreed with each other about the appropriate course of action; policy proposals frequently passed only with split votes and after vigorous discussion and dissent. Differences of opinion also existed among the board of directors of the Federal Reserve Bank of New York and between leaders in New York, Washington, and other cities. An overview of the system-wide debate appears in Chandler 1971, pp. 54-70.
- 6 Galbraith characterizes Mitchell as one of the two prominent “prophets” of the stock market boom, the other being Irving Fisher.
- 7 The account that we present is, in our opinion, the academic consensus, although on this issue we note that Meltzer (2003, 252-257) and other scholars suggest that the crash was a symptom, not a contributing force, to the contraction in 1929.
- 8 Friedman and Schwartz (1963) outline these lessons in their coverage of the stock market crash. Meltzer (2003) reaches similar conclusions in his history of the Federal Reserve. Chairmen of the Federal Reserve, including Bernanke and Greenspan, echoed these sentiments in their writings and speeches in recent decades.
- 9 Irrational exuberance is, of course, a phrase popularized by Alan Greenspan and typically cited to this speech.
Bibliography
Bernanke, Ben, “ Asset Price ‘Bubbles’ and Monetary Policy .” Remarks before the New York Chapter of the National Association for Business Economics, New York, NY, October 15, 2002.
Calomiris, Charles W. “Financial Factors in the Great Depression.” The Journal of Economic Perspectives 7, no. 2 (Spring 1993): 61-85.
Chandler, Lester V. American Monetary Policy, 1928-1941 . New York: Harper and Row, 1971.
Eichengreen, Barry. Golden Fetters: The Gold Standard and the Great Depression, 1919 –1929 . Oxford: Oxford University Press, 1992.
Federal Reserve Act, 1913. Pub. L. 63-43, ch. 6, 38 Stat. 251 (1913).
Friedman, Milton and Anna Schwartz. A Monetary History of the United States . Princeton: Princeton University Press, 1963.
Galbraith, John Kenneth. The Great Crash of 1929 . New York: Houghton Mifflin, 1954.
Greenspan, Alan, “ The Challenge of Central Banking in a Democratic Society ,” Remarks at the Annual Dinner and Francis Boyer Lecture of The American Enterprise Institute for Public Policy Research, Washington, DC, December 5, 1996.
Klein, Maury. “The Stock Market Crash of 1929: A Review Article.” Business History Review 75, no. 2 (Summer 2001): 325-351.
Kohn, Donald, “ Monetary policy and asset prices ,” Speech at “Monetary Policy: A Journey from Theory to Practice,” a European Central Bank Colloquium held in honor of Otmar Issing, Frankfurt, Germany, March 16, 2006.
Meltzer, Allan. A History of the Federal Reserve, Volume 1, 1913-1951 . Chicago: University of Chicago Press, 2003.
Mishkin, Frederic, “ How Should We Respond to Asset Price Bubbles? ” Comments at the Wharton Financial Institutions Center and Oliver Wyman Institute's Annual Financial Risk Roundtable, Philadelphia, PA, May 15, 2008.
Romer, Christina. “The Great Crash and the Onset of the Great Depression.” Quarterly Journal of Economics 105, no. 3 (August 1990): 597-624.
Temin, Peter. “Transmission of the Great Depression.” Journal of Economic Perspectives 7, no. 2 (Spring 1993): 87-102.
Written as of November 22, 2013. See disclaimer .
Related Essays
- Banking Panics of 1931-33
- Stock Market Crash of 1987
- The Great Depression
Related People

George L. Harrison President
New York Fed
1928 – 1940
Federal Reserve History

FinancialResearch.gov
Working papers, crash narratives.
By Dasol Kim, William Goetzmann, and Robert Shiller
Published: December 28, 2023
Share on Facebook Share on Linked In Logo for Twitter
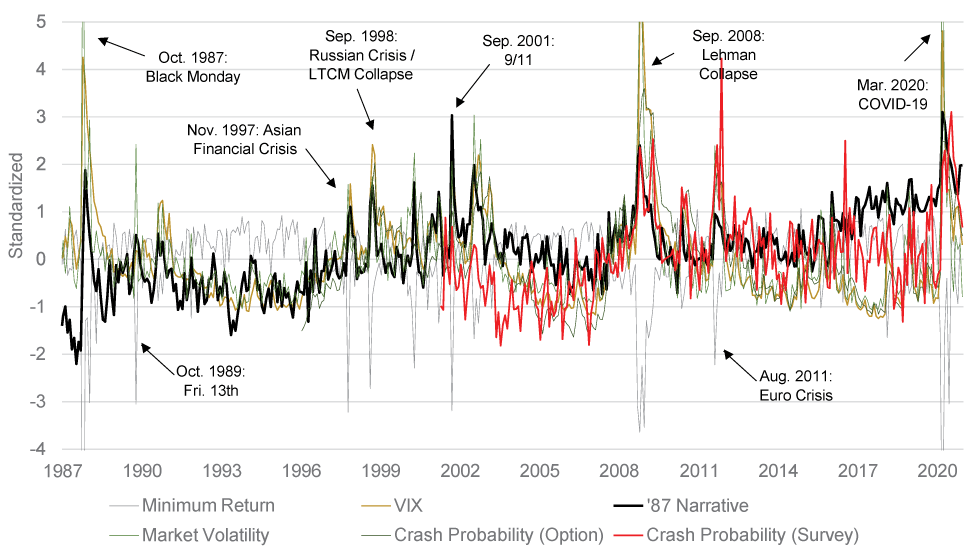
The financial press is a conduit for popular narratives that reflect collective memory about historical events. Some collective memories relate to major stock market crashes, and investors may rely on associated narratives, or crash narratives, to inform their current beliefs and choices. Using recent advances in computational linguistics, we develop a higher-order measure of narrativity based on newspaper articles that appear following major crashes. We provide evidence that crash narratives propagate broadly once they appear in news articles and significantly explain predictive variation in market volatility. We exploit investor heterogeneity using survey data to distinguish the effects of narrativity and fundamental conditions and find consistent evidence. Finally, we develop a measure of pure narrativity to examine when the financial press is more likely to employ narratives (Working Paper no. 23-10).
The financial press is a conduit for popular narratives that reflect collective memory about historical events. Some collective memories relate to major stock market crashes, and investors may rely on associated narratives, or crash narratives, to inform their current beliefs and choices. Using recent advances in computational linguistics, we develop a higher-order measure of narrativity based on newspaper articles that appear following major crashes. We provide evidence that crash narratives propagate broadly once they appear in news articles and significantly explain predictive variation in market volatility. We exploit investor heterogeneity using survey data to distinguish the effects of narrativity and fundamental conditions and find consistent evidence. Finally, we develop a measure of pure narrativity to examine when the financial press is more likely to employ narratives.
Keywords: Narrative Finance, Crash Beliefs, Investor Surveys JEL Classifications: E03, G00, G02, G11, G23
Back to Working Papers
You are now leaving the OFR’s website.
You will be redirected to:
You are now leaving the OFR Website. The website associated with the link you have selected is located on another server and is not subject to Federal information quality, privacy, security, and related guidelines. To remain on the OFR Website, click 'Cancel'. To continue to the other website you selected, click 'Proceed'. The OFR does not endorse this other website, its sponsor, or any of the views, activities, products, or services offered on the website or by any advertiser on the website.
Thank you for visiting www.financialresearch.gov.
Stock Market Crash: S&P 500 Falls Below 5,000
W hile the benchmark S&P 500 index has consistently defied gravity, it may not be exempt from the golden rule: what goes up must eventually come down. Indeed, the loss of the psychologically important 5,000-point level may be more than just a blip on the radar. Instead, it raises the possibility of a stock market crash.
On the technical front, Lawrence G. McMillan, president of MacMillan Analysis, sounded the alarm about a support line breaking down. Per an article published by MarketWatch , the S&P 500 fellow below a support line established at 5,050 points. “That changes the investment picture to a large extent,” wrote the investment commodity trading advisor. McMillan added the following:
“The 5,050-5,180 area had been a strong base for the launch to new all-time highs in late March. So, the fact that the market has not been able to stem the tide of the selling that began with the CPI number two weeks ago and has continued with geopolitical worries is significant. Once that 5,050 area was broken, some fairly heavy technical selling came in.”
The expert mentioned that the index closed below 5,050 for two consecutive days, with April 18 being the second day. Therefore, this circumstance has officially eliminated its “core” bullish position. While that doesn’t necessarily equate to a stock market crash, the odds are moving in an unfavorable direction.
Worrisome Fundamentals Highlight the Possibility of a Stock Market Crash
To be sure, the technical situation reflects the fundamental backdrop — and that too does not look compelling. In particular, flaring tensions in the Middle East may lead to events that could potentially trigger a stock market crash.
Last weekend, Iran launched a barrage of missiles and drones against Israel. Government officials claimed it did so in response to a bombing of Iran’s consulate in Syria , an attack which Iran pinned on Israel. Reports have emerged that Israel has now struck back at Iran, which again raises the specter of a stock market crash.
As The Wall Street Journal reports, the scope of the Israeli response was “narrow.” Significantly, Iranian media outlets are downplaying the incident, suggesting that both sides want to cool tensions. However, as the WSJ pointed out, a miscalculation may metastasize into a broader regional conflict.
Should such a miscalculation occur, a fear exists that global oil supplies could be disrupted. That may have an inflationary impact, representing a possible trigger for a stock market crash.
What makes the problem challenging is that energy prices contributed to a hotter-than-expected inflation reading. And that may have downwind implications for consumer sentiment. Content streaming giant Netflix (NASDAQ: NFLX ) was the first of the mega-capitalization technology firms to report earnings. Unfortunately, soft revenue guidance for the current quarter led to a sharp drop in its share price.
Why It Matters
A stubbornly hot inflationary environment also clouds the Federal Reserve’s monetary policy aim of lowering interest rates. Subsequently, several popular growth-oriented tech enterprises, including Super Micro Computer (NASDAQ: SMCI ) and Nvidia (NASDAQ: NVDA ) have floundered. Again, it’s not proof of a stock market crash, but the wind is not blowing in the right direction.
On the date of publication, Josh Enomoto did not have (either directly or indirectly) any positions in the securities mentioned in this article. The opinions expressed in this article are those of the writer, subject to the InvestorPlace.com Publishing Guidelines .
A former senior business analyst for Sony Electronics, Josh Enomoto has helped broker major contracts with Fortune Global 500 companies. Over the past several years, he has delivered unique, critical insights for the investment markets, as well as various other industries including legal, construction management, and healthcare. Tweet him at @EnomotoMedia.
Bank of America's top global strategist warns of growing bubble risk in the stock market as the economy heads for a no-landing scenario — and shares 3 charts showing the market could be headed for trouble
- Bank of America's Michael Hartnett predicts a no-landing scenario for the US economy for now.
- But the longer this scenario plays out, the higher recession risks become.
- Signs of trouble ahead include falling small-business optimism and a drop in high-yield bonds.
Michael Hartnett , Bank of America's top global strategist, thinks a no-landing scenario is the most-likely outcome for the US economy in the months ahead. That means the labor market would remain strong, but inflation would also stay above the Federal Reserve's long-term goal of 2%.
While that's fine for now, Hartnett warns it's a path that eventually leads to trouble for the economy and stocks. The longer inflation stays elevated, the longer the Fed has to keep policy restrictive or even tighten further, putting the economy at risk of a recession as businesses and consumers slow borrowing and spending.
"We say rising no landing risks = rising hard landing risks," Hartnett said in an April 11 note. "Monetary tightening resumes (markets now pricing in 15% probability of Fed hike) & contagion risks form from REITs, regional banks, small cap up."
By many measures, the US economy looks strong. The unemployment rate is at a historically low 3.8% and monthly job growth is steady. Consumer spending , which makes up about two-thirds of the US economy, is also still robust. Household balance sheets are strong, too, with home values at all-time highs and stocks hovering just off of record levels.
But some cracks are beginning to show. Delinquencies on credit card and auto loans are rising, as are layoff announcements. As Hartnett pointed out, small-business optimism is poor, with hiring plans among them at eight-year lows. This is concerning because small businesses make up two-thirds of the US labor market.
Another sign that the economy could be headed for trouble is the sudden drop in high-yield bond prices in recent months. High-yield bonds carry a higher risk of default, so investors demand higher yields in a shaky economic environment. Bond yields rise when their prices drop.
Related stories
The iShares iBoxx $ High Yield Corporate Bond ETF (HYG) just hit its 200-day moving average, which Hartnett said was "ominous." The fund's price dipped below its 200-day moving average in 2020 and 2022, when the economy slowed and stocks underperformed.
This, then, could be a signal that stocks are headed for a downtrend as the S&P 500 sits near all-time highs.
Hartnett said there are other signs, too, that stocks could be in bubble territory. One is that the tech-heavy Nasdaq index is rising at the same time as 10-year Treasury yields, which has historically only happened during bubble or recovery periods.
Are we headed for a hard landing?
Market consensus has shifted from a hard landing in 2022 to a soft landing in 2023 and 2024. But like Hartnett's view, outlooks may begin to shift more to the bear case in the months ahead, with the Fed likely to leave rates higher for longer.
As recent as December, investors were anticipating that the central bank would make its first rate cut in March. But as jobs data has come in hot and inflation has remained above 3%, the Fed is now expected to cut in July. Some are even ruling out cuts for the entirety of 2024.
"We are firmly in the camp of no rate cuts in 2024," said Michael Landsberg, chief investment officer at Landsberg Bennett Private Wealth Management, in a memo on Thursday. "While it's unlikely to occur, there is actually a strong case to be made for the Fed to raise interest rates in 2024 given elevated inflation, low unemployment, high stock prices, bitcoin surging and the re-emergence of IPOs."
Geopolitical tensions are also high at the moment, as conflicts continue in Ukraine and the Middle East. This has caused oil prices to surge since December, threatening to kick off another bout of global inflation.
Despite geopolitical and monetary policy risks in the post-pandemic era, however, the US economy has so far managed to skirt a recession, proving bearish forecasters wrong.
It may continue to do so. But as Hartnett argues, the longer a no-landing scenario — where inflation stays high — plays out, the higher the risks of recession and a bear market become.
Watch: What happens when the US debt reaches critical levels?
- Main content
The stock market is headed for a hard 'reset' that could take years to recover from, CIO says
- Stocks are poised for a "reset," according to Technical Traders' Chris Vermeulen.
- Defensive areas of the market are rallying, which is typical in a late-stage bull market.
- Bull markets are inevitably followed by bear markets and a financial reset, Vermeuelen warned.

Stocks have been in the midst of a long bull market, but there are signs that it's finally going to run out of steam and will inevitably be followed by a bear market and a difficult "reset," according to Chris Vermeulen,CIO of Technical Traders.
In an interview with Bloomberg , the investment chief pointed to the recent run-up in defensive assets, like precious metals, energy stocks, and industrial stocks. Those areas all typically do well in the late stage of a bull market, which is inevitably followed by a bear market or a "financial reset," Vermeulen said.
Investors are likely heading into another bear market, similar to the ones that followed the dot-com bubble and the 2008 financial crisis, he predicted. That could end up sparking painful stock losses for investors, with people seeing their wealth decline as much as 30%-50% over the next year, he warned.
"I think we're coming into a major market top, more or less a financial reset," Vermeulen said Tuesday. "It's short-term, temporarily painful. But we need markets to reset. We need regular pullbacks and corrections in order for the market to keep going up."
That reset could also come with a recession, Vermeulen said, with industrial stocks in particular signaling a slowdown for the economy. While the sector has done well in recent months, buyers of industrial goods typically upgrade their equipment at the end of an economic growth cycle, due to "huge delays" between slowing business and orders for new machinery.
"They don't realize we're coming to the end of a growth cycle, and the music is about to stop," Vermeulen said of US firms. "Industrial stocks have just continued to muscle their way higher. They're hitting all-time highs, and that is a sign that we're going to see these companies eventually start to slow down."
Investors remain concerned over a potential recession, especially as inflation has remained stubbornly elevated and the Fed looks poised to keep interest rates higher for longer. The economy has a 58% chance of tipping into recession by March of next year, per the latest estimate from the New York Fed .
- Main content
- Today's news
- Reviews and deals
- Climate change
- 2024 election
- Fall allergies
- Health news
- Mental health
- Sexual health
- Family health
- So mini ways
- Unapologetically
- Buying guides
Entertainment
- How to Watch
- My Portfolio
- Stock Market
- Biden Economy
- Stocks: Most Actives
- Stocks: Gainers
- Stocks: Losers
- Trending Tickers
- World Indices
- US Treasury Bonds
- Top Mutual Funds
- Highest Open Interest
- Highest Implied Volatility
- Stock Comparison
- Advanced Charts
- Currency Converter
- Investment Ideas
- Research Reports
- Basic Materials
- Communication Services
- Consumer Cyclical
- Consumer Defensive
- Financial Services
- Industrials
- Real Estate
- Mutual Funds
- Credit Cards
- Balance transfer cards
- Cash-back cards
- Rewards cards
- Travel cards
- Personal Loans
- Student Loans
- Car Insurance
- Options 101
- Good Buy or Goodbye
- Options Pit
- Yahoo Finance Invest
- EV Deep Dive
- Fantasy football
- Pro Pick 'Em
- College Pick 'Em
- Fantasy baseball
- Fantasy hockey
- Fantasy basketball
- Download the app
- Daily fantasy
- Scores and schedules
- GameChannel
- World Baseball Classic
- Premier League
- CONCACAF League
- Champions League
- Motorsports
- Horse racing
- Newsletters
New on Yahoo
- Privacy Dashboard
Yahoo Finance
Why the magnificent 7's 'momentum is collapsing'.
The run may be over for the seven stocks that drove the lion's share of the stock market rally over the past year.
UBS Investment Bank's chief US equity strategist Jonathan Golub downgraded six of the "Magnificent Seven" stocks — Apple ( AAPL ), Alphabet ( GOOGL , GOOG ), Microsoft ( MSFT ), Amazon ( AMZN ), Meta ( META ), and Nvidia ( NVDA ) — from Overweight to Neutral in a new research note on Monday.
His call comes as the Magnificent Seven, which also includes Tesla ( TSLA ), just endured its largest weekly market cap loss in history. All seven of the Big Tech leaders are off their recent highs, a decline punctuated by a 10% single-day drawdown for Nvidia on Friday, its worst one-day price performance since March 2020, though the AI darling rebounded 4% on Monday.
Golub, who rates sectors within the S&P 500 ( ^GSPC ), not individual stocks, remains Overweight on technology outside of the six stocks named in his note.
But for the large companies that have grown earnings significantly over the past year, Golub believes the tide may be shifting and other areas are set to outperform the largest stocks in the S&P 500.
"Our downgrade of the Big 6 — from Overweight to Neutral — is not predicated on extended valuations, or doubts about AI," Golub wrote.
"Rather, it is an acknowledgment of the difficult comps and cyclical forces weighing on these stocks. These forces do not apply to other TECH+ companies or the rest of the market in the same way."
Earnings for the S&P 500 have largely been driven by profit growth at the large tech firms. That's expected to play out again during first quarter reports , with FactSet projecting Amazon, Alphabet, Meta, Microsoft, and Nvidia will combine for earnings growth of roughly 64%. Meanwhile, the other 495 companies in the S&P 500 are expected to report an earnings decline of 6%.
But over the course of the year, this is expected to shift.
Consensus estimates from FactSet show earnings growth for those five companies is set to end the year just shy of 20% year over year in the fourth quarter, reflecting significantly slower growth than their prior pace.
By that point, consensus expects the other 495 companies to grow earnings by about 17% compared with the prior year, a significant uptick from their current growth rate.
"Investors attribute the run in mega cap stocks to animal spirits and the impact of AI," Golub wrote. "However, our work indicates that surging earnings momentum (change in forward growth projections) fueled this upside. Unfortunately, this momentum is collapsing."
Tesla is expected to report quarterly results on Tuesday, with results from Meta, Microsoft, and Alphabet coming later this week.
This shift in where earnings are growing most could be "disruptive in the near term," Golub added.
But given increasing signs that the US economy is growing faster than expected this year , Golub thinks a broadening out in earnings performance over the next year keeps his call for the S&P 500 to end the year at 5,400 in play. The benchmark index closed at 5,010 on Monday.
"This target remains supported by broadly positive fundamentals and a robust economy," Golub wrote.
Josh Schafer is a reporter for Yahoo Finance. Follow him on X @_joshschafer .
Click here for in-depth analysis of the latest stock market news and events moving stock prices .
Read the latest financial and business news from Yahoo Finance
Recommended Stories
Ftse 100 surges to record high as middle east tensions ease.
The FTSE 100 has closed at a record high after fears about an escalating conflict in the Middle East eased.
Real Estate Commission Settlement Gets Early Approval. Expect Changes in July.
A federal judge has preliminarily approved a settlement in a high-profile lawsuit over real estate commissions, clearing the way for sweeping changes starting this summer in the way buyers and sellers work with their agents. The changes are likely to increase transparency around real estate agent fees—but the revised rules’ ultimate impact on commission rates has yet to be seen. Judge Stephen R. Bough of the Western District of Missouri granted preliminary approval to the settlement agreement in a Missouri antitrust case about broker commissions, according to court documents filed Tuesday.
Investors Love This Under the Radar Emerging Markets ETF
EMXC has seen inflows of nearly $4 billion this year.
Analyst revises Google parent Alphabet's stock price target before earnings
Here's what could happen next to Alphabet's shares.
Virginia man makes three times the minimum wage, but can’t afford to live. Here are 3 ways to stretch your money
He says working 90 hours is ‘not the goal.’
4 Big Earnings Reports Will Drive ETFs This Week
These stocks make up 15% of SPY.
Advertisement
Supported by
‘It’s Clearly Bleak’: Stocks Notch Longest Losing Streak in Months
A rally at the start of the year has given way to worries on Wall Street about economics and geopolitics.
- Share full article
S&P 500

By Joe Rennison
Stocks suffered their longest losing streak of the year, as geopolitical turmoil rattled Wall Street and investors slashed their bets on the Federal Reserve cutting interest rates any time soon.
The S&P 500 fell 0.9 percent on Friday, its sixth consecutive decline, marking its worst run since October 2022.
The slide dragged the S&P 500 down by just over 3 percent for the week, a third straight weekly decline. By that measure, it is the longest weekly losing streak for the index since September, when concerns over rising government debt and a potential government shutdown compounded worries about the effects of high interest rates.
Those fears dissipated toward the end of last year as inflation cooled and investors began to bet that the Fed would soon cut rates, prompting a ferocious stock rally in the first three months of 2024.
But this month, worries that stubborn inflation would lead the Fed to keep rates high have returned, compounded by the widening conflict in the Middle East, with Israel striking Iran early on Friday .
“It’s clearly bleak,” said Andrew Brenner, head of international fixed income at National Alliance Securities.
Investors have pulled roughly $21 billion out of funds that invest in U.S. stocks over the two weeks through Wednesday, according to data from EPFR Global, which tracks fund flows. That compares to an inflow of around $80 billion for the year through early April. And the unease is not just apparent in the stock market.
U.S. government bond yields, which underpin interest rates for a wide variety of loans, have been rising. The average rate on 30-year mortgages, the most popular home loan in the United States, rose above 7 percent on Thursday for the first time this year.
The dollar is also markedly higher, putting pressure on countries that import goods from the United States and issue dollar-denominated debt. And oil prices, stoked by geopolitical tensions, are up more than 13 percent since the start of the year.
“There is nothing that looks good right now,” Mr. Brenner said.
Recent reports showing hotter-than-expected inflation have altered investors’ forecasts for the Fed , which has kept its key rate near a two-decade high. “The recent data have clearly not given us greater confidence and instead indicate that it’s likely to take longer than expected to achieve that confidence,” Jerome H. Powell, the Fed chair, said at an event in Washington on Tuesday.
Economists at Société Générale no longer expect the Fed to cut rates this year. BNP Paribas and Wells Fargo economists have also dialed down their expectations for cuts.
Traders in futures markets, which allow investors to bet on where interest rates are headed, are wagering on one, and perhaps two, quarter-point cuts by the end of the year. At the start of the year, traders were expecting six cuts over that period.
At first, the shift appeared to be welcomed by stock investors. A strong economy, all else equal, is good for the stock market, and while some inflation data had started to buck the trend earlier this year it wasn’t enough to disrupt the broader cooling that took hold in 2023. But recent inflation reports have continued to disappointed investors and economists and become harder to ignore.
John Williams, the president of the New York Fed, said this week that it was possible that another increase, rather than a cut, to rates might be warranted if inflation remained sticky, even if that wasn’t what he considered the most likely scenario. Other officials have noted that the Fed may have to wait until much later this year, or even 2025, to begin easing rates.
So far, worries have yet to intensify to the point of threatening the strength of the U.S. economy. Although the S&P 500 has fallen 5.5 percent this month, it remains more than 4 percent higher for the year.
And a recent survey of fund managers around the world by Bank of America showed the most optimism since January 2022, with respondents expecting global growth to accelerate. The biggest risk, according to the respondents, is a rise in inflation that could keep interest rates elevated, squeezing growth abroad and at home.
Reflecting some of those worries, the Russell 2000 index that tracks smaller companies that tend to be more sensitive to the outlook for the domestic economy, has slumped to a loss of roughly 4 percent for the year.
“I think the recent selling pressure is just the beginning of a larger move,” said Peter Tchir, head of macro strategy at Academy Securities.
An earlier version of this article misstated the number of consecutive weeks the S&P 500 is set to drop. The market moves this week have set the stock index up for a third straight weekly decline, not a fourth.
How we handle corrections
Joe Rennison writes about financial markets, a beat that ranges from chronicling the vagaries of the stock market to explaining the often-inscrutable trading decisions of Wall Street insiders. More about Joe Rennison
- Election 2024
- Entertainment
- Newsletters
- Photography
- Personal Finance
- AP Investigations
- AP Buyline Personal Finance
- AP Buyline Shopping
- Press Releases
- Israel-Hamas War
- Russia-Ukraine War
- Global elections
- Asia Pacific
- Latin America
- Middle East
- Election Results
- Delegate Tracker
- AP & Elections
- Auto Racing
- 2024 Paris Olympic Games
- Movie reviews
- Book reviews
- Personal finance
- Financial Markets
- Business Highlights
- Financial wellness
- Artificial Intelligence
- Social Media
Stock market today: Tumbling tech stocks drag Wall Street to the finish line of another losing week
People pass the New York Stock Exchange, right, on Wednesday, April 17, 2024 in New York. Global shares are trading mixed after most U.S. stocks slipped on firmer expectations that the Fed is committed to its current rate practices.(AP Photo/Peter Morgan)
- Copy Link copied
NEW YORK (AP) — The worst week for big technology stocks since the COVID crash in 2020 dragged Wall Street on Friday across the finish line of another losing week.
The S&P 500 dropped 0.9% to close out its third straight losing week. That’s its longest such streak since September, before it broke into a romp that sent it to a string of records this year.
The Nasdaq composite sank 2%. The Dow Jones Industrial Average, which has less of an emphasis on tech, was an outlier and rose 211 points, or 0.6%.
The market’s worst performers included several stocks that had been its biggest stars. Super Micro Computer lost more than a fifth of its value, dropping 23.1%. The company, which sells server and storage systems used in AI and other computing, had soared nearly 227% for the year coming into the day.
Nvidia, another stock that has surged to dizzying heights due to Wall Street’s frenzy around artificial-intelligence technology, also gave up some of its big recent gains. It slumped 10% and was the heaviest single weight on the S&P 500, by far, because of its huge size.
Tech stocks in the S&P 500 broadly lost 7.3% this week for their worst performance since March 2020 as some global giants reported discouraging trends. ASML, a Dutch company that’s a major supplier to the semiconductor industry, reported weaker-than-expected orders for the start of 2024, for example.
The larger threat was a dawning, dispiriting acknowledgement sweeping Wall Street that interest rates may likely stay high for longer.
AP AUDIO: Stock market today: Wall Street limps toward its longest weekly losing streak since September
Wall Street is limping toward its longest weekly losing streak since September. AP business correspondent Damian Troise has more.
Top Fed officials said this week that they could hold interest rates at their high level for a while. That’s a letdown for traders after the Fed had signaled earlier that three cuts to interest rates could be possible this year.
High rates hurt prices for all kinds of investments. Some of the hardest hit tend to be those seen as the most expensive and which make investors wait the longest for big growth, which can make tech stocks vulnerable.
Lower rates had earlier appeared to be on the horizon after inflation cooled sharply last year. But a string of reports this year showing inflation has remained hotter than expected has raised worries about stalled progress.
Fed officials are adamant that they want to see additional proof inflation is heading down toward their 2% target before lowering the Fed’s main interest rate, which is at its highest level since 2001.
Traders are now largely forecasting just one or two cuts to rates this year, according to data from CME Group, down from expectations for six or more at the start of the year. They’re also betting on the possibility of no cuts to rates this year.
But Brian Jacobsen, chief economist at Annex Wealth Management, expects inflation to moderate as U.S. households that have become “hypersensitive to price hikes” by businesses begin slowing their spending.
“The giant sucking sound of optimism (escaping) from the market is due to the Fed’s lack of foresight and irrational focus on where inflation has been instead of where it’s going,” he said.
Because interest rates look unlikely to offer much help in the near term, companies are under even more pressure to deliver growth in profits. The recent drops in price have cooled a bit of the criticism that stocks had grown too expensive, but they won’t look cheap unless either prices fall further or profits jump higher.
Netflix sank 9.1% despite reporting stronger profits for the latest quarter than expected. Analysts called it a mostly solid performance, but the streaming giant disappointed some investors by saying it will stop giving updates on its subscriber numbers every three months, beginning next year.
Helping to limit the market’s losses was American Express, which rose 6.2%. It reported stronger profit for the latest quarter than analysts expected. Fifth Third Bancorp rose 5.9% after it likewise topped expectations.
All told, the S&P 500 dropped 43.89 points to 4,967.23. It’s 5.5% below its record set late last month.
The Dow Jones Industrial Average rose 211.02 to 37,986.40, and the Nasdaq composite fell 319.49 to 15,282.01.
In the oil market, a barrel of Brent crude pulled back to $87.29 after briefly leaping above $90 overnight on worries about fighting in the Middle East . Iranian troops fired air defenses at a major air base and a nuclear site during an apparent Israeli drone attack , raising worries in the market. But crude prices pared their gains as traders questioned how Iran would respond.
In the bond market, the yield on the 10-year Treasury eased to 4.62% from 4.64% late Thursday to trim its gain for the week. It had been down more overnight, when worries were spiking about a potentially broadening war in the Middle East.
In markets abroad, stock indexes were mixed in Europe after falling more sharply in Asia.
AP Writers Matt Ott and Zimo Zhong contributed.
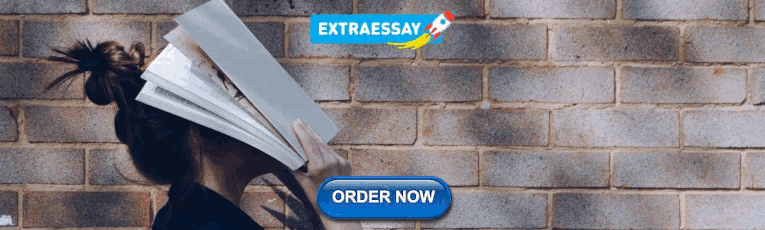
IMAGES
VIDEO
COMMENTS
This paper argues that the stock market crash of 2008, triggered by a collapse in house prices, caused the Great Recession. The paper has three parts. First, it provides evidence of a high correlation between the value of the stock market and the unemployment rate in U.S. data since 1929. Second, it compares a new model of the economy developed ...
The aim of this study is to investigate quantitatively whether share prices deviated from company fundamentals in the stock market crash of 2008. For this purpose, we use a large database containing the balance sheets and share prices of 7796 worldwide companies for the period 2004-2013.
The first half of the year 2020 saw one of the most dramatic stock market crashes in history. The crash was caused by the virus that originated in Wuhan, China. ... and very limited research has been carried out to study the impact of such events. ... The unprecedented stock market impact of Covid-19 (Working Paper No. 26945). National Bureau ...
We analyze how investor expectations about economic growth and stock returns changed during the February-March 2020 stock market crash induced by the COVID-19 p ... National Bureau of Economic Research (NBER); Centre for Economic Policy Research (CEPR) ... Giglio, Stefano and Maggiori, Matteo and Stroebel, Johannes and Utkus, Stephen P., Inside ...
This paper uses multivariate Hawkes processes to model the transactions behavior of the US stock market as measured by the 30 Dow Jones Industrial Average individual stocks before, during and after the 36-min May 6, 2010, Flash Crash. The basis for our analysis is the excitation matrix, which describes a complex network of interactions among the stocks. Using high-frequency transactions data ...
This paper aims to examine market sentiments using social media to predict the stock market performance before, during and after the March 2020 stock market crash. In addition, using the Organisation for Economic Co-operation and Development Taxonomy of Sectoral Digital-intensity Framework, we identified market sectors that have outperformed ...
The stock market crash of October 1929 was one of the sharpest and most abrupt collapses. 18 On two days October 28-29, the Dow Jones fell a total of 24 percent, down 19.6 percent for the
This paper investigates the impact of economic policy uncertainty (EPU) on the crash risk of US stock market during the COVID-19 pandemic. To this end, we use the GARCH-S (GARCH with skewness) model to estimate daily skewness as a proxy for the stock market crash risk. The empirical results show the significantly negative correlation between EPU and stock market crash risk, indicating the ...
1. Introduction. March 2020 saw one of the most dramatic stock market crashes in history. In barely four trading days 2, Dow Jones Industrial Average (DJIA) plunged 6,400 points, an equivalent of roughly 26%.The crash was caused by government's reaction to a novel coronavirus (COVID-19), a disease which originated in the Chinese city of Wuhan in December 2019 and quickly spread around the ...
Stock Price Crash Risk: Review of the Empirical Literature. Ahsan Habib, M. Hasan, Haiyan Jiang. Published 11 March 2017. Economics, Business. S&P Global Market Intelligence Research Paper Series. We survey the burgeoning literature on the determinants and consequences of firm‐specific future stock price crash risk.
For example, the stock market crash of October 19, 1987, is an event that represents the largest one-day decline in the Dow Jones Industrial Average (DJIA) Index since the stock market crash of October 24, 1929. Figure 1 displays monthly counts of references in The Wall Street Journal to the crashes of October 24, 1929, and October 19, 1987.
research papers that the stock market volatility has significantly changed post financial crisis of 2008 (Varma , 2009; Ramlall, 2009, 2010; Karuna nayake et al., 2010).
"The Stock Market Crash of 1929: A Review Article." Business History Review 75, no. 2 (Summer 2001): 325-351. Kohn, Donald, " Monetary policy and asset prices ," Speech at "Monetary Policy: A Journey from Theory to Practice," a European Central Bank Colloquium held in honor of Otmar Issing, Frankfurt, Germany, March 16, 2006.
surpassing 20%. 2. In this paper, we investigate the effect of CO VID-19 on the stock market behavior. during the crash of March 2020 using the universe of S&P1500 firms. Clearly, COVID-19 rep ...
Crash Narratives. By Dasol Kim, William Goetzmann, and Robert Shiller. Published: December 28, 2023. View paper. The financial press is a conduit for popular narratives that reflect collective memory about historical events. Some collective memories relate to major stock market crashes, and investors may rely on associated narratives, or crash ...
Second, using a rolling window multifractal, asymmetric, detrending cross-correlation model with lag, it is found that within four weeks before a stock market crash, the speed at which stock price information is absorbed is accelerated; however, the content of the stock price information is gradually reduced, noise traders are active, and the ...
The panic began again on Black Monday (October 28), with the market closing down 12.8 percent. On Black Tuesday (October 29) more than 16 million shares were traded. The Dow lost another 12 percent and closed at 198—a drop of 183 points in less than two months. Prime securities tumbled like the issues of bogus gold mines. General Electric fell from 396 on September 3 to 210 on October 29.
The 1987 stock market crash was a major systemic shock. Not only did the prices of many financial assets tumble, but market functioning was severely impaired. This paper reviews the events surrounding the crash and discusses the response of the Federal Reserve, which responded in a number of ways to support the operation of financial markets ...
Reports have emerged that Israel has now struck back at Iran, which again raises the specter of a stock market crash. As The Wall Street Journal reports, the scope of the Israeli response was ...
Why the U.S. Needs the Stock Market to Crash The boy who cried wolf was ultimately right April 15, 2024 By Michael A. Gayed Apr 15, 2024, 2:54 pm EDT April 15, 2024
Bank of America's top global strategist warns of growing bubble risk in the stock market as the economy heads for a no-landing scenario — and shares 3 charts showing the market could be headed ...
People's wealth could take a huge hit as the stock market peaks after one of the longest bull markets ever, according to an investment chief. Menu icon A vertical stack of three evenly spaced ...
The run may be over for the seven stocks that drove the lion's share of the stock market rally over the past year. UBS Investment Bank's chief US equity strategist Jonathan Golub downgraded six of ...
It is decidedly not a good look for U.S. stocks. Rallying at the open but finishing lower on the day sends a bad message about market health. Doing it for a fourth session in a row, as the S&P 500 ...
Stocks suffered their longest losing streak of the year, as geopolitical turmoil rattled Wall Street and investors slashed their bets on the Federal Reserve cutting interest rates any time soon.
Iranian troops fired air defenses at a major air base and a nuclear site during an apparent Israeli drone attack, raising worries in the market. But crude prices pared their gains as traders questioned how Iran would respond. In the bond market, the yield on the 10-year Treasury eased to 4.62% from 4.64% late Thursday to trim its gain for the week.