People Analytics: 5 Real Case Studies
Maaike Ankum
Harness the power of HR analytics to improve business performance by learning from these organizations.
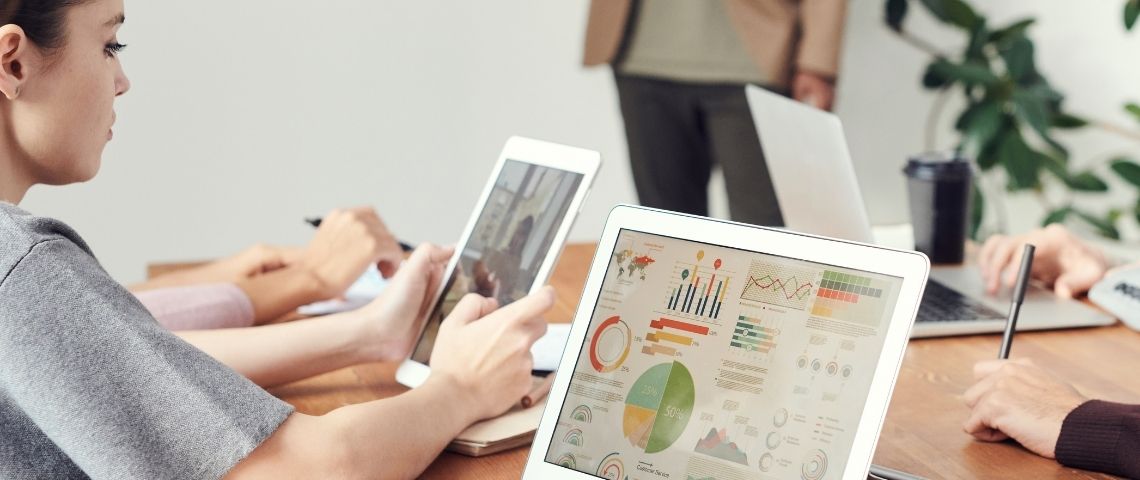
Data is essential to an organization’s growth and profitability. HR analytics turns the focus inwards on one of the biggest determining factors of a company’s success: their employees. Collect and analyze data about the talent within your organization and get the insights you need to increase productivity, reduce waste, and capitalize on unique opportunities.
As a leading expert in employee feedback solutions, Effectory encourages a data-driven approach to identifying opportunities for improvement within organizations. This includes refining strategies to hire the right talent, designing individual employee development plans, and enhancing employee engagement. We’re always available to talk with you about how we can make people analytics work for your organization.
This article will, first, outline the steps to implementing an effective people analytics strategy and, second, explore case studies from five prominent organizations that highlight the different ways in which HR analytics can be used to improve your business outcomes.
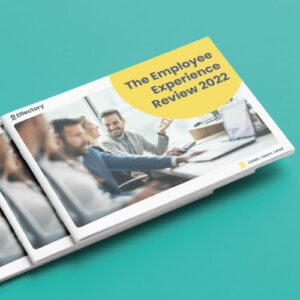
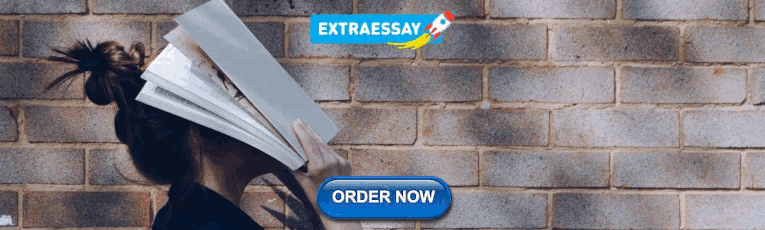
Download the Employee Experience Review
Discover how employees around the world are experiencing different parts of the employee journey and how this affects the employee experience.
The steps in an HR analytics strategy
Organizations need to have clearly defined processes to gather data, analyze it, identify patterns, and turn those insights into actionable initiatives. Though the terms are similar, HR reporting and HR analytics are not, in fact, interchangeable. They are actually the first and final steps, respectively, in the three-part process of people analytics.
1. Reporting
HR reporting is the foundation of people analytics, bringing together all the data your organization has tracked.
HR reports are objective and factual. They present data in a digestible way to highlight patterns, but do not derive conclusions.
2. Analysis
HR analysis is the investigative phase where your team draws connections among data points and develops hypotheses.
During analysis, you may need to identify gaps in available data and fill them in using new tools and initiatives like surveys, employee interviews, or game-like personality tests.
3. Analytics
This is where the people-data, HR reports, and analysis come together to give you a definitive picture of the problem or opportunity your organization is facing. Data and hypotheses are structured through analytics to provide actionable insights.
What is predictive people analytics?
The following step-by-step guide is another way to map out the people analytics process:
- Identify opportunities or areas for improvement
- Establish performance metrics
- Collect data
- Identify relevant patterns and develop hypotheses
- Fill the data gaps
- Analyze the results
- Implement data-driven strategies
Here are five real-world case studies that demonstrate different ways in which businesses have used people analytics to improve their bottom line.
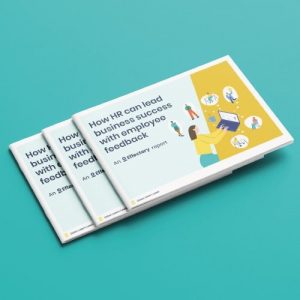
How HR can lead business success
HR and employee listening are crucial to business success. Here is how employee insights can help your organization.
Johnson & Johnson: Experience and retention
Area for improvement: American multinational Johnson & Johnson was looking to improve both employee performance and retention. At the time, their recruiters prioritized candidates with job experience in the industry, assuming they were more likely to stay with the company and would be quicker to make significant contributions. As a result, the company experienced a 10 percent decrease in new hires who had recently graduated from college. Data and analysis: Challenging these assumption, HR and the people analytics team at Johnson & Johnson compiled data on 47,000 employees to test the link between experience and turnover. The data revealed that employees hired right out of college actually remained with the organization “significantly longer” than more experienced candidates. Furthermore, there was no significant difference between the two groups’ contributions to the company. Their new hypothesis is that Johnson & Johnson’s two-year leadership development program may help reduce turnover among recent graduates. Analytics and outcome: Based on the people analytics, Johnson & Johnson increased hires of new graduates by 20 percent, effectively reducing turnover while maintaining performance.
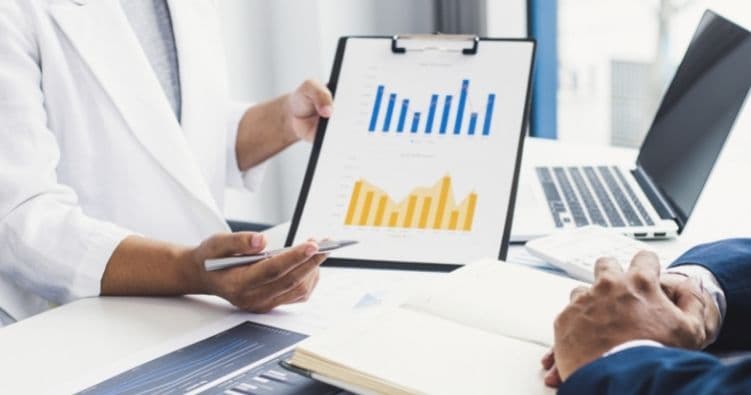
Clarks: Employee engagement
Area for improvement: C. & J. Clark is a British shoe manufacturer and retailer with approximately 1400 locations and over 13,000 employees. According to Chief People Officer, Belinda Deery, Clarks already had high levels of employee engagement relative to their industry but were looking to maximize return on investment, especially at the store level. Data and analysis: The HR analytics team used 450 data points to get an accurate picture of the relationship between employee engagement and overall business performance. The report and subsequent analysis revealed that for every 1 percent increase in employee engagement, business performance increased by 0.4 percent, confirming the correlation and the value of employee engagement. The analytics team also collected additional data from the company’s 100 best-performing stores.
Analytics and outcome: The combined findings of this two-step people analytics initiative enabled Clarks to implement multiple effective changes and programs that improved engagement and increased business performance. First, they drafted a replicable schema to create high-performing stores, including the ideal team size for optimum efficiency. The company also designed a store management development program and put together an employee engagement toolkit for managers. The CPO reported positive ongoing results after implementing these initiatives.
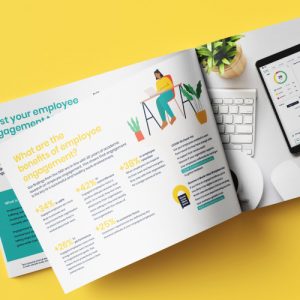
Global Employee Engagement Index™
A comprehensive overview of employee engagement with benchmarks from 57 countries with essential lessons for your HR strategy .
Credit Suisse: Predicting turnover
Area for improvement:
Credit Suisse is a financial services company based in Switzerland that employs over 47,000 people. To reduce turnover, they tried to predict which employees were most likely to leave the company and when. It’s been estimated that the cost of replacing an employee can be anywhere between 30 and 400 percent of an employee’s salary, depending on seniority and experience. This is a substantial loss for a company the size of Credit Suisse.
Data and analysis: Because the Credit Suisse workforce is so large and since they have strong data tracking practices, the analytics team had substantial information on who left the company, why, and after how long. The team dug deeper to explore “the specific circumstances prior to the points of departure” by tracking over 40 variables, such as performance ratings, the time spent in a given role, and the size of an employee’s team.
Analytics and outcome: The resulting predictive people analytics model gave Credit Suisse the ability to accurately predict how likely an employee is to leave the organization in the next year based on as few as ten indicators. With these predictors, Credit Suisse is able to identify risk factors and address these issues with employees before they result in attrition.
Use the insights of departing employees to uncover hidden truths about your organization and minimize further unnecessary departures.
E.On: Absenteeism
Based in Essen, Germany, E.On is an electric company that employs approximately 78,000 people. When absenteeism rose above the acceptable benchmark set by their HR department, E.On used people analytics to determine the factors driving increased unscheduled time off. Depending on whether an employee is paid hourly or with an annual salary, absenteeism can cost a business anywhere between $2,660 and $3,600 per employee, annually. For an organization of E.On’s size, that potentially adds up to hundreds of millions of dollars.
Data and analysis: The people analytics team at E.On put together 55 hypotheses. Based on the available data, the team tested 21 hypotheses and finally validated 11 of them. What they found was that the duration and timing of vacation had the biggest impact on the frequency of unplanned time off for the rest of the year. Contrary to popular belief, employees selling back their vacation time to the company did not appear to have any statistically significant impact on absenteeism.
Analytics and outcome: Based on these insights, E.On implemented policy changes that encouraged managers to be more accommodating with how employees schedule time off. Employees are encouraged to schedule multiple breaks throughout the year with at least one larger vacation—a combination that reduces absenteeism. The findings from this people analytics initiative also provided the HR team with additional insights they can use to test other variables in the future.
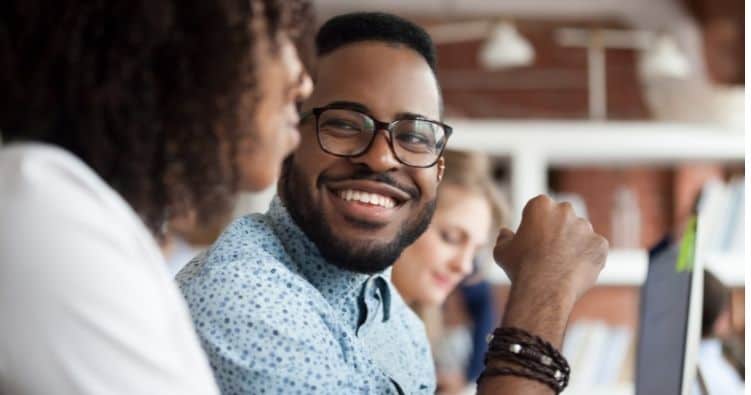
Cisco: Choosing an office location
Area for improvement: This multinational technology conglomerate based out of San Jose, California employs over 75,000 people. When the time came to open a new regional office, the company used people analytics to determine the best building and location for it. The goal was to avoid wasted space, create a positive work environment for employees, and attract the right talent to the organization. Data and analysis: With 266 Cisco offices across 87 countries, their People Planning, Analytics and Tools department were able to source data about office usage rates and average costs within the organization. Senior Director Ian Bailie and his team also looked at the building’s neighborhood and the location’s community to evaluate their respective potential impact on business performance. Most importantly, the analytics team explored the availability of talent, specifically from neighboring universities, relative to the number of competitors in the area.
Analytics and outcome: Because of Bailie and his team’s findings, the company decided to go with a different location than they first had their eye on. Their research found that the original choice would have left the recruitment team struggling to fill the necessary roles. The findings also changed Cisco’s process for opening new offices by bringing people analytics into the discussion much earlier. Planning, staffing, and operating new offices became much more resource efficient.
These are just a few real-world examples of how HR analytics can be used to improve business outcomes. People analytics focuses on an organization’s most valuable resource, its employees, and empowers them to do their best work to benefit the company and its larger goals. Prioritize data and regular reporting to gain meaningful insights and actionable takeaways. Talk to Effectory today to make people analytics a key part of your HR strategy .
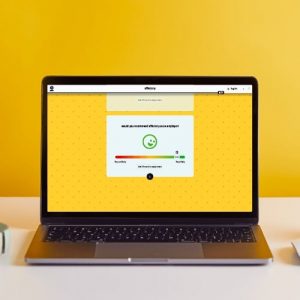
Book a free demo. See our solutions in action.
Effectory is Europe’s Leading provider of Employee Listening Solutions. Schedule a product demo and discover how to enhance your employees’ engagement.
Related themes
Related articles.
How to be great at people analytics
A decade ago, someone touting the benefits of “people analytics” probably would have been met with blank stares. Was there value to be gleaned from HR data? Absolutely. But firms were thinking more narrowly about the potential—focusing on core HR systems and gathering straightforward information, such as snapshots of regional head counts or the year’s average performance evaluation rating, rather than using analytics capabilities to manage talent and make evidence-based people decisions.
Today, however, the majority of large organizations have people analytics teams, 1 Innovation generation: The big HR tech disconnect 2019/20 report , Thomsons Online Benefits, July 24, 2019, thomsons.com. 70 percent of company executives cite people analytics as a top priority, 2 “How people analytics can change an organization,” Knowledge@Wharton, May 23, 2019, knowledge.wharton.upenn.edu. and there’s little argument that people analytics is a discipline that’s here to stay. What’s striking, though, is the different ways that firms have approached building their people analytics functions. Team size, composition, and organization vary widely, and priorities for capability development and maturation differ significantly.
Most companies still face critical obstacles in the early stages of building their people analytics capabilities, preventing real progress. The majority of teams are still in the early stages of cleaning data and streamlining reporting. Interest in better data management and HR technologies has been intensive, but most companies would agree that they have a long way to go.
Leaders at many organizations acknowledge that what they call their “analytics” is really basic reporting with little lasting impact. For example, a majority of North American CEOs indicated in a poll that their organizations lack the ability to embed data analytics in day-to-day HR processes consistently and to use analytics’ predictive power to propel better decision making. 3 Based on responses of participants at a McKinsey roundtable of 45 chief human-resources officers in the autumn of 2016. Frank Bafaro, Diana Ellsworth, and Neel Gandhi, “ The CEO’s guide to competing through HR ,” McKinsey Quarterly , July 24, 2017. This challenge is compounded by the crowded and fragmented landscape of HR technology, which few organizations know how to navigate.
So, while the majority of people analytics teams are still taking baby steps, what does it mean to be great at people analytics? We spoke with 12 people analytics teams from some of the largest global organizations in various sectors—technology, financial services, healthcare, and consumer goods—to try to understand what teams are doing, the impact they are having, and how they are doing it.
Stairway to impact
It helps to think about the growth trajectory of a people analytics team as a stairway with five steps (Exhibit 1). The best teams don’t climb directly from one step to the next one; they are constantly iterating—retracing their steps and climbing the same stairs again—at every level of the journey to the top.
To move from the first step of the stairway (poor data) to the second step (good data), an organization must focus on building a foundation of high-quality data. This usually means that data needs to be extracted from the transactional systems where it is entered and then reshaped, cleaned, and re-coded into a more manageable and easier-to-understand structure that is aligned to the goals of the people analytics team. The more that analysts and data scientists need to clean and recode data to make it usable for even simple analysis, the less efficient the analytics team will be and the longer it will take to develop its skills and capabilities. This is arguably the most difficult step to get right. Significant resources, time, and investment are required to identify and manage core HR data systems, establish a common language and consistent data structure, and determine a basic set of guidelines for data collection, processing, and engineering. These are iterative processes, with no definitive solutions; rather, the processes and their outcomes change as the internal and external talent environments shift, systems are retired and renewed, and links are established among HR teams such as recruiting, training and development, and employee benefits.
As the operating environment changes at an increasingly rapid pace, both capabilities and the technology used to manage and transform data need to be increasingly flexible. In people analytics, as in many other tech-enabled fields, taking an agile approach is now a fundamental requirement. People analytics teams must work together with their enterprise-wide technology groups in a rapid and nimble way to institute new technology platforms, evolve existing infrastructure, and maintain consistent enterprise-wide standards.
Once a strong data foundation is in place, the people analytics team can climb to the third step, making the useful data accessible to the organization and experimenting with new technologies to analyze and disseminate the data. The sophistication that organizations are able to achieve at this step is variable. At the simplest end of the spectrum, teams might focus on automating and visualizing HR dashboards via standard business-intelligence platforms such as Tableau, in order to generate standard reports or respond to ad hoc requests. More advanced teams might prioritize custom builds and software development for self-serve applications, perhaps using their own front-end developers.
It’s evident from our interviews that organizations arrive in different ways at the ability to put data and actionable insights into the hands of decision makers. At several points, organizations must make decisions related to technologies and platforms—decisions such as whether to use homegrown talent or third-party vendors—and the answers vary by organization. As one would expect, the ability to attain advanced automation and self-serve capabilities depends greatly on the quality and accessibility of the underlying data.
Teams that mastered descriptive and automated reporting at step three are ready to climb to step four and build advanced-analytics capabilities. Data scientists, rather than business-information specialists, use programming languages like R, Python, and Julia to join disparate sources of data, build models to help understand complex phenomena, and provide actionable recommendations to leaders making complex and strategic business decisions.
We spoke to people analytics teams at a handful of organizations that are experimenting heavily at this level of the stairway and still have significant room to grow as their companies become open to new statistical tools, scale their data-science talent bench, and pursue a wide range of use cases. While some companies employ “broad-spectrum” data scientists who work cross-functionally to support a wide range of business needs, we found that the most advanced teams have created specific subspecialties in data science (for example, natural-language processing, network analytics, and quantitative psychometrics). These allow people analytics teams to increase their impact on their organizations by providing the advanced insights necessary to support strategic decision making on diverse and complex types of talent issues.
No people analytics team we interviewed has been able to take a full fifth step to reach the top level of the stairway: creating reliable, consistent, and valid predictive analytics. Reliable predictions will enable people analytics teams to analyze and explore practical options for management action. While some organizations have built fit-for-purpose predictive models—mostly for workforce planning—implementing predictive analytics in the context of employee selection, development, or engagement decisions requires a substantially scaled-up data-science operation, massive amounts of highly accurate data (“very big data”), cutting-edge algorithmic technology, and organizational comfort with how to address the impact on fairness and bias.
Beyond the required resources and the complexity of the analytics techniques, the infrastructure also poses a challenge to scalability and could require the use of cloud services. Most of the teams we spoke with are still working from on-premise technological infrastructures and show few signs of migrating their data and analytics capabilities to cloud services in the near future.
Ingredients for success
Our conversations with people analytics teams in leading organizations reveal a set of six best-in-class ingredients that have helped to propel the teams’ impact, success, and continued growth. These ingredients fall into three main categories: data and data management, analytics capabilities, and operating models. If we were to build a leading people analytics team from scratch, this is what we would strive for.
Data and data management
All great analytics teams are enabled by strong data standards, engineering, and management, and our interviews confirmed that this is no different in people analytics.
Significant and dedicated data-engineering resources. We found that the greatest team differentiator was the level of dedicated data-engineering resources available to it for propelling data creation and quality control. The leading teams have full ownership of their own data repositories, allowing them to rapidly test new ideas, iterate, and reduce dependencies on enterprise-level technology resources.
An added benefit of dedicated data-engineering resources is that they enable strategic alignment. Data engineers who are steeped in the strategic context of their organization’s people analytics teams are able to design the data foundation and analytics solutions more thoughtfully and deliberately from the beginning.
Breadth and depth of data sources. Leading teams have invested heavily in a strong HR-data foundation but also have advanced ways of going beyond the core HR systems to use several additional internal sources of data. The most straightforward way might be seamlessly linking the HR data with finance data, though data priorities will differ depending on organizational context. A few teams have begun to step beyond relational databases to build graph databases 4 A type of NoSQL database, graph databases are able to model relationships within data in a powerful and flexible manner. For more, see Antonio Castro, Jorge Machado, Matthias Roggendorf, and Henning Soller, “ How to build a data architecture to drive innovation—today and tomorrow ,” June 3, 2020. for advanced network analytics. In addition, leading teams have a robust and flexible survey strategy for monitoring employee sentiment. They are also able to integrate their survey data with multiple other data sources to create multidimensional quantitative and psychometric models that help explain employee engagement trends and dynamics.
While it is common for people analytics teams to feel constrained by a lack of easily available data, leading teams are more creative with data, acquiring new sources or combining existing ones in new ways to attack the problem at hand. For example, time-sheet data could be transformed and loaded into a graph database and linked by activity or project codes to allow better analysis of teamwork and collaboration.
Analytics capabilities
Advanced people analytics projects can require both deep technical knowledge and the ability to integrate and translate across a wide array of expertise and input. The best teams are building their talent bench with breadth and depth.
Robust data-science function. As we expected, all the leading people analytics teams we interviewed have invested heavily in acquiring data-science talent, though their approaches differ. Some teams focus on hiring “all-around athletes,” while others prioritize specialized backgrounds such as quantitative psychometrics or natural-language processing. Leading teams have sizable data-science “pods” that span a wide range of advanced analytical methodologies, programming languages, and academic backgrounds. The best teams hire and develop specialists in specific disciplines of data science but nevertheless expect all of these individuals to operate in a nimble, cross-functional way in order to meet evolving needs.
Strong translation capability. Leading teams also complement their high-caliber technical talent with skilled “translators”: specialized “integrators,” who bridge the gap between business leaders and technical experts. They translate strategic challenges into analytic questions and use evidence-based practice to interpret insights derived from the analytics, engage stakeholders, and ultimately propel business changes. Translators often serve as an entry point to the people analytics team, helping to raise awareness of the team in the organization and build the team’s credibility. Some of the leading people analytics teams have built benches of internal consultants to partner directly with individual businesses on their specific problems.
Operating models
In a fast-developing field, people analytics teams need to deliver impact across the organization and stay ahead of the curve to maintain that impact into the future. The best teams align themselves well against organizational priorities while maintaining space for open experimentation and innovation.
Innovation as the norm. Members of leading teams are explicitly expected to explore and innovate beyond their day-to-day fulfillment of the needs of their clients. Some companies have rules of thumb for the percentage of time that teams spend on exploration as opposed to core foundational work. These expectations allow teams to fully experiment and build out proofs of concept.
This process can take a variety of forms, but the important distinction is that the areas of innovation need not directly support an existing business priority or client need; they might be purely exploratory. For example, some data scientists relish the extra time to play around in a sandbox and learn how analytic tools and services work in the cloud. Others might want to explore creative new ways to visualize data in order to equip business leaders with helpful insights. The goal is to ensure that all team members are constantly forming new ideas and looking for new ways to meet the analytic needs of the organization and thereby help it achieve its objectives.
Clear alignment with clients and organizational use cases. People analytics teams take different approaches to organizing themselves and aligning with different clients. What is consistent, however, is the presence of a mechanism for attaining an in-depth understanding of enterprise-wide priorities as well as the specific needs of individual clients. This mechanism creates feedback loops that enable continuous learning and iterative development, and it ensures that people analytics teams are working on the most pressing and high-impact topics.
A culture of trust, empowerment, and ownership is the critical foundation for ensuring that a people analytics team is aligned with its clients as well as the enterprise. People analytics teams routinely deal with urgent (and often ambiguous) client needs and questions, highly sensitive data, and challenges to extrapolating meaningful and actionable insights that will guide business decisions. The bar to entry for the best teams is high: members must own their work from end to end and be empowered to define the constraints of any analysis, protect privacy as well as fairness and equity, flag issues that arise, and use their own judgment to derive insights. Being reactive and incremental is not enough in human resources, where priorities change and the top ones require immediate attention.
Over time, as organizations become increasingly dependent on the quality of their insights, the best people analytics teams play a stronger role in shaping the HR agenda, influencing how the organization manages its talent at both a policy and a process level.
The pulse survey
The COVID-19 crisis provided a natural experiment for one large, global organization with a strong people analytics team to use the ingredients outlined in the previous section by rapidly creating a homegrown weekly pulse survey to track the opinions and feelings of tens of thousands of employees around the globe. This capability enabled the organization to better understand the best ways to support employees in a challenging time and a fully remote work environment.
Setting up the pulse survey required intensive collaboration between diverse, highly skilled individuals already embedded in the organization’s people analytics team as well as rapid and close collaboration with the leadership of the organization. Translators navigated the need to craft questions that engaged employees, gathered high-quality data to feed the analytic models, and communicated insights back to leaders who had urgent decisions to make about how to best support their workforce in an external environment that was highly unpredictable and changing week by week.
To speed the time to insights, data engineers established an automated and continuous link among weekly survey-response data, core HR data systems, and a broader set of additional data sources, including data sets that data engineers had developed and customized for this purpose. This process cleaned, tested, and prepared the data for analysis. In addition to rapidly providing analysts with weekly data to examine and synthesize, it fed these data to a prototype self-service reporting tool, which gave leaders the ability to directly investigate aggregated pulse data within six hours of the survey’s close.
The customized data sets supported both exploratory and targeted analyses and helped generate actionable insights for the leaders. Analyses were designed to build on the organization’s current understanding of the health of its employees, marrying new and existing information to yield new insights that guided various efforts. For example, specialists in natural language processing used structural topic modeling to identify and quantify topics in the free-text comments that employees submitted as part of the survey each week. Sentiment analysis was used to understand the emotion behind each topic. These results were then married to the demographic information prepared by data analysts, allowing managers, leaders, and other decision makers to understand how the conversations and associated feelings varied by subpopulation, such as parents and less tenured employees. The combination of data sources and analytic approaches ultimately revealed population-specific needs, which allowed the organization to target specific groups and tailor the type of support it offered to maximize impact.
Exhibit 2 is a view of the major topics generated from the free text of the employees who responded to the pulse surveys and how their emphasis on these topics changed over the course of two months of the crisis. At the beginning, employees were thankful for the health of their families and peers and had generic concerns about the developing situation, but as the crisis evolved, their thoughts crystallized into the more particular concerns of isolation, remote work, childcare, and work-life balance.
The ability to rapidly develop this capability, turn around a wide range of sophisticated analytics within 24 hours after the survey closed, and repeat the survey weekly did not come easily to the organization or the people analytics team. The capabilities required to pull it off were tightly rooted in the data, analytics, and operating-model ingredients that we have identified as the hallmarks of great people analytics teams.
Despite the vast differences that exist among organizations’ data quality, integration, and infrastructure, we all certainly have a lot to learn from each other. Answering the following questions will be helpful to leaders who want to identify where their organization’s people analytics is now and where they would like them to be:
- Where is the organization on the people analytics stairway? Where does it aspire to be in the next year, three years, and five years?
- How does the organizational context influence the mandate of the people analytics team?
- What ingredients does the organization possess today, and which does it need to build?
- How should the organization determine its priorities in building people analytics capabilities? For example, should it build to support certain specific internal use cases, or should it build a broad bench of capabilities to support an unpredictable or rapidly changing internal environment?
- If the organization had to get one thing right over the next 12 months, what would it be? What would get in the way of its getting there?
While no single model is the “correct” one for developing the capabilities of a people analytics team, leading teams seem to have a set of ingredients in common. While the past decade has brought about real change, even the best teams—those that iterate at each step of the stairway and learn as they ascend—have barely scratched the surface of what’s possible with people analytics.
Elizabeth Ledet is a partner in McKinsey’s Atlanta office; Keith McNulty is a director, people analytics and measurement, in the London office; Daniel Morales is a director of analytics in the Washington, DC, office; and Marissa Shandell is an alumna of the New York office.
Explore a career with us
Related articles.

A transformation of the learning function: Why it should learn new ways
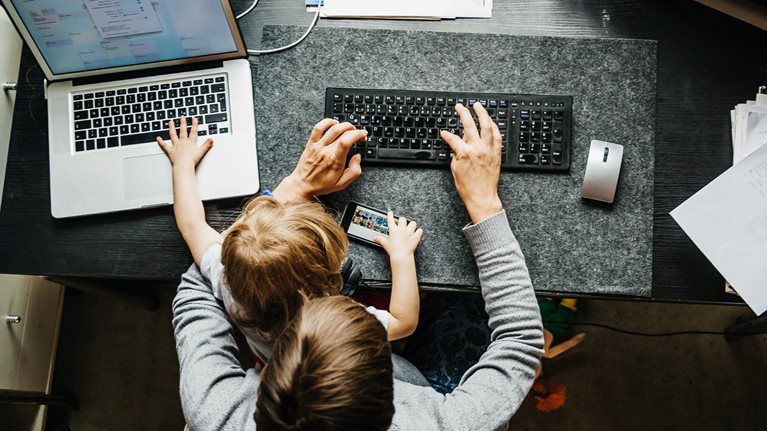
What 800 executives envision for the postpandemic workforce

The boss factor: Making the world a better place through workplace relationships
Boost your HR career with CHRMP certifications before the Fee Increase . Act now to secure your future! LAST DAY TODAY !!
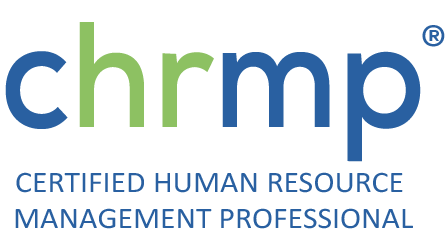
Top 10 HR Analytics Case Studies
Author by : chrmp.
- HR Analytics
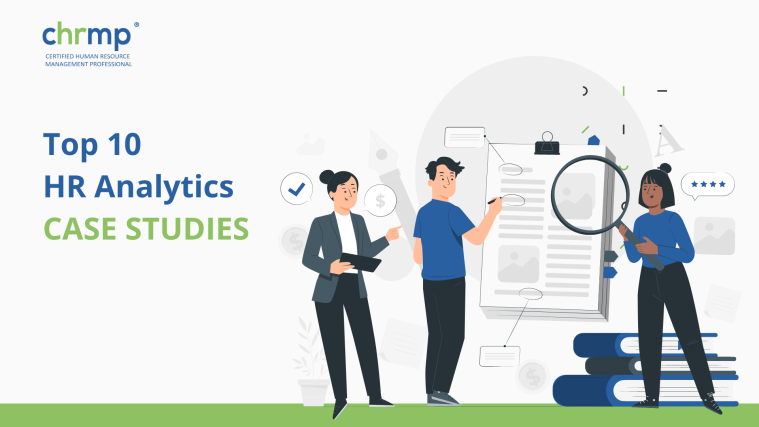
The power of data drives the fast-paced business world today. To stay abreast and ride the competition wave, it is important to harness the power of data. And HR Analytics is just the tool you need. It is a valuable tool to measure critical aspects of your organisation which impact its bottom line.
Whether you’re an HR professional, a business leader, or just someone curious about the role of HR Analytics in the workplace, this blog is for you. We’ll cover a range of industries and organizational sizes, showcasing the diversity of ways HR Analytics can be applied to drive results and also look at some HR analytics case studies.
Curious about how HR Analytics can drive business results? Well, look no further because, in this blog, we’ll be diving into the top 10 HR analytics case studies.
From boosting engagement to improving diversity, inclusion and productivity, these companies have achieved impactful results by leveraging HR data.
So sit back, grab a coffee, and get ready to be inspired!
Importance of HR Analytics in Modern Organizations
HR Analytics plays a critical role in modern organizations as it provides valuable insights into the people side of the business. With HR Analytics, organizations can track key metrics such as turnover, diversity and inclusion, and employee satisfaction, allowing them to identify areas for improvement and make data-backed changes.
Furthermore, HR Analytics can help organizations stay ahead of the curve by providing real-time insights into their HR practices, drives and campaigns, allowing them to respond quickly to emerging trends and challenges. Organizations can also design and implement more effective HR initiatives by leveraging HR data, such as training and development programs and talent management strategies.
In short, HR Analytics is essential for modern organizations looking to drive business results through their people. By providing a data-driven approach to HR, organizations can make informed decisions to improve employee outcomes and significantly impact the company’s bottom line.
Become a data-driven HR leader with our HR analytics course
- Drive business results with HR data.
- Master data collection, analysis, and reporting.
- Make informed decisions that impact your workforce.
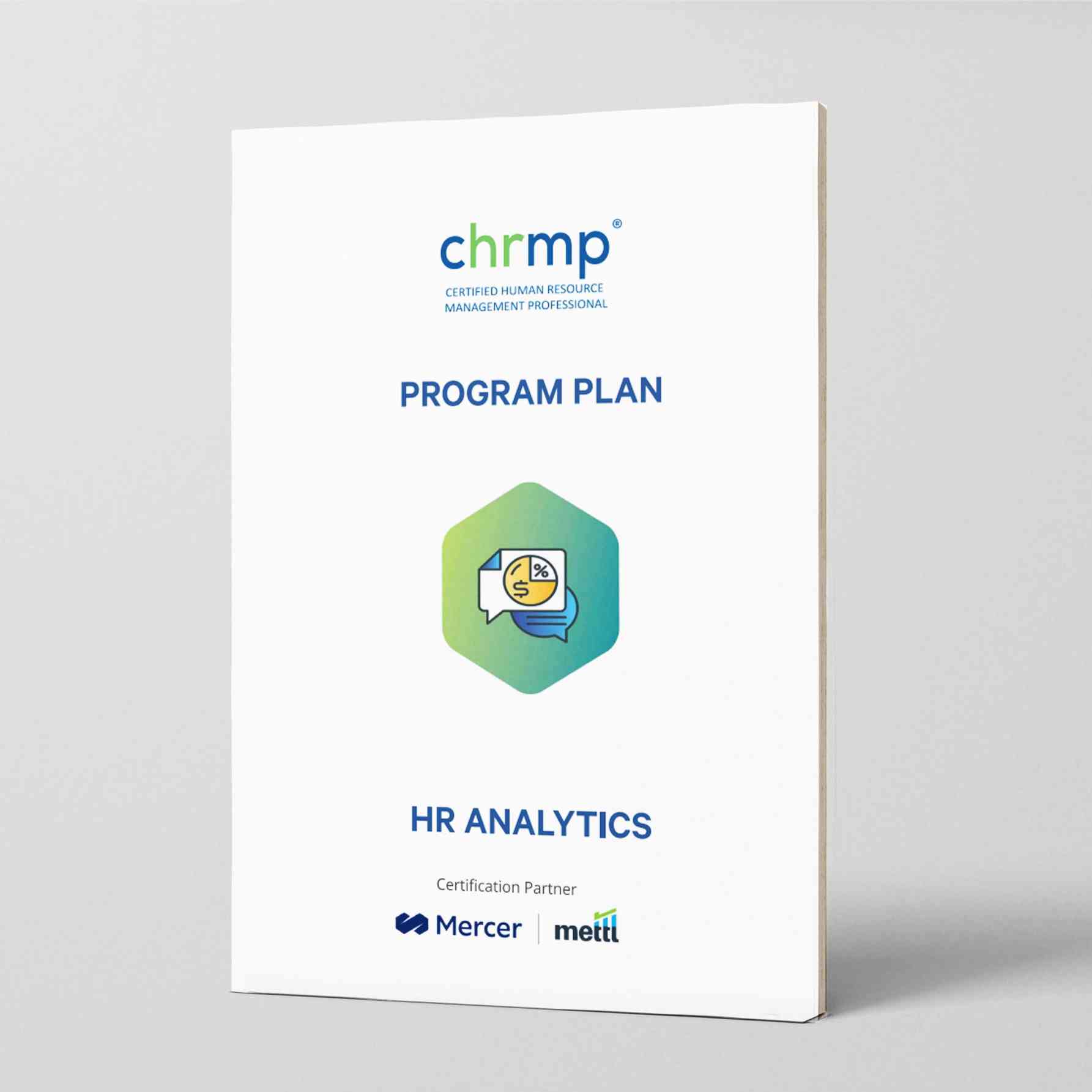
The 3 Steps in an HR Analytics Strategy
In today’s data-driven business world, organizations increasingly turn to HR Analytics to drive impactful changes and improve their people strategies.
An effective HR Analytics strategy begins with a clear plan outlining the steps to achieve our goals.
Here are the three basic steps in an HR analytics strategy:
Problem Statement
The first step is to define the problem or challenge you are trying to solve. This involves identifying areas in your organization where we need insights, such as employee turnover, diversity and inclusion, or employee engagement.
A clear problem statement helps to focus efforts and ensure that your HR Analytics strategy aligns with your overall business objectives and goals.
Approach and Solution
The next step is to determine the approach we will use to gather and analyze HR data. This involves selecting proper HR metrics to track and the tools and technologies to determine how we will collect and store our HR data. Our approach should align with our organizational culture and objectives.
The final step is to analyze and interpret the results of our HR Analytics efforts. This involves evaluating the impact of our HR initiatives, drives or campaigns and identifying areas for improvement. Communicating the results of our HR Analytics efforts to stakeholders within the organization is the key to gaining buy-in and support for future initiatives. By regularly tracking and reporting on HR metrics, organizations can continue to improve their HR practices.
In conclusion, these three steps – problem statement, approach and solution, and results – form the foundation of an effective HR Analytics strategy.
By following this process, organizations can ensure that their HR Analytics efforts align with their business objectives and drive meaningful impact for their people and businesses.
HR Analytics is a powerful tool to help organizations make informed decisions about their HR or people strategies. By analyzing data related to employee behavior, organizations can identify trends and make changes that improve outcomes from employees.
Here we will discuss ten HR Analytics case studies demonstrating the impact of data-driven HR strategies. Here’s a list of the top 10 HR analytics case studies:
Reducing road traffic accidents
A transportation company used HR Analytics to reduce road traffic accidents among its drivers. By analyzing data related to driver behavior and providing appropriate training to them, the company was able to identify and address the root cause of accidents and improve safety on the roads for every one.
Achieving an optimum staffing level
A manufacturing company used HR Analytics to optimize its staffing levels, reducing turnover, curbing absenteeism and sabbaticals and improving employee engagement and satisfaction. The company used data to track key metrics such as employee satisfaction, productivity, engagement and attendance, allowing it to make informed decisions about staffing levels .
A/B Testing Employee Training
A technology company used HR Analytics to test the effectiveness of its employee training programs. By using A/B testing, the company was able to determine which training methods were most effective and made improvements to the same, ultimately improving employee performance and engagement and curbing absenteeism at the same time. If the employees feel that the company is interested in their career development, growth and overall well being, this sentiment shows on their attendance records and performance .
Sick days at E.ON
Next, on our list of top ten HR analytics case studies is E.ON.
E.ON, a leading energy company, used HR Analytics to reduce the number of employee sick days.
By analyzing data related to employee health, the company identified the root cause of employee absenteeism which was ill health and implemented targeted interventions to improve employee wellbeing.
Engagement at Clarks
Clarks, a leading footwear company, used HR Analytics to improve employee engagement and retention. By tracking key metrics such as employee satisfaction, absenteeism, sabbaticals and turnover, the company was able to identify areas for improvement and make changes that led to higher levels of employee engagement , retention, reduction in turnover and absenteeism which in turn boosted employee and organisational performance .
Engagement at Shell
Shell, a global energy company, used HR Analytics to improve employee engagement and retention. By tracking employee satisfaction, absenteeism and turnover, the company was able to identify areas for improvement and implement targeted interventions, resulting in higher levels of employee engagement ,reduction in employee turnover and increase in their productivity and performance.
HR driving store performance
A retail company used HR Analytics to link HR practices with store performance. By tracking key metrics such as employee satisfaction, absenteeism, sick leave records and employee turnover, the company was able to identify the relationship between HR practices and store performance and make vital data driven changes in its people strategies.
Compensation and benefits at Clarks
Clarks deserves another spot in our top ten HR analytics case studies list because of it’s compensation and benefits.
Clarks used HR Analytics to optimize its compensation and benefits programs as compared to similar outfits in the industry. By analyzing data related to employee compensation and benefits, the company was able to make informed decisions about its programs and improve employee satisfaction and retention by improving compensation and benefits as compared to others in the same industry.
Opening a new office by Cisco
Cisco used HR Analytics to open a new office in a new location. By analyzing data related to employee locational preferences and commuting times, the company was able to make informed decisions about the venue of its new office .
Using automated listening during a hostile takeover and HR Analytics to understand employee sentiment during the same and by tracking employee engagement and satisfaction, the company was able to identify areas of concern and address them, ultimately improving employee morale and outcomes and reducing the impact of the takeover on the company’s workforce.
In conclusion, these HR Analytics case studies demonstrate the real-world impact that data-driven HR strategies can have on employee outcomes and business results. A major takeaway from these HR analytics case studies is that by using HR Analytics, organizations can make informed decisions about their people strategies, ultimately improving employee satisfaction and driving business success.
4 Key Emerging Trends in the Future of HR Analytics
The future of HR analytics is likely to be shaped by a number of emerging trends and technologies. Here are a few key areas to watch:
The new emerging trends and technology that are going to shape the future of HR Analytics are listed below:
Greater emphasis on predictive analytics:
The emphasis is on using predictive analytics to forecast future trends and identify potential areas of risk or opportunity as business outfits strive to be more proactive and agile with respect to their HR decision-making. Large volumes of data has to be analysed to identify patterns and trends, and using machine learning algorithms to make predictions based on historical data i.e . extrapolation of data.
Increased use of artificial intelligence:
HR analytics is likely to be one of the areas that benefits the most as AI technologies continue to advance. Automatation of many tasks can be achieved using AI in HR analytics, such as data collection, cleaning, and analysis, freeing up HR professionals to focus on more strategic tasks. AI can also help identify and extrapolate data patterns and trends that might be difficult or impossible for humans to spot.
Focus on employee experience:
As organizations seek to create more engaging and fulfilling workplace experiences for their employees, there is likely to be an increased emphasis on using HR analytics to measure and enhance the employee experience. This could involve analyzing data on employee satisfaction, engagement, retention and productivity or turnover and absenteeism using which more effective training programs and policies can be designed.
Integration with other business functions:
As HR analytics becomes more sophisticated and integrated with other business functions, it is likely to become an even more powerful tool for driving business performance to greater heights. This could involve integrating HR analytics with financial, marketing, or supply chain analytics, for example, to gain a more comprehensive view of organizational performance as a whole.
In conclusion, the future of HR analytics is likely to be characterized by greater use of advanced technologies like AI, a focus on strategic data-driven decision-making, and a more holistic approach to understanding and enhancing the employee experience.
In conclusion, the use of HR Analytics has become increasingly important in modern organizations as they strive to make data-driven decisions about their people strategies.
The top 10 HR Analytics case studies demonstrate the tangible impact of data-driven HR strategies on employee outcomes and business results. From reducing road traffic accidents to improving employee engagement and retention, these HR analytics case studies show how organizations can use HR Analytics to make informed decisions about their people strategies and drive business success.
By using HR Analytics, organizations can gain a deeper understanding of their workforce and make changes towards better competitive compensation and benefits packages , providing better training development opportunities for career growth and medical well-being, resulting in a more engaged, productive, and productive, and satisfied workforce.
We hope you found inspiration from our list of HR analytics case studies.
Leave a Reply Cancel reply
Your email address will not be published. Required fields are marked *
Save my name, email, and website in this browser for the next time I comment.
Subscribe our Newsletter
You might also like.
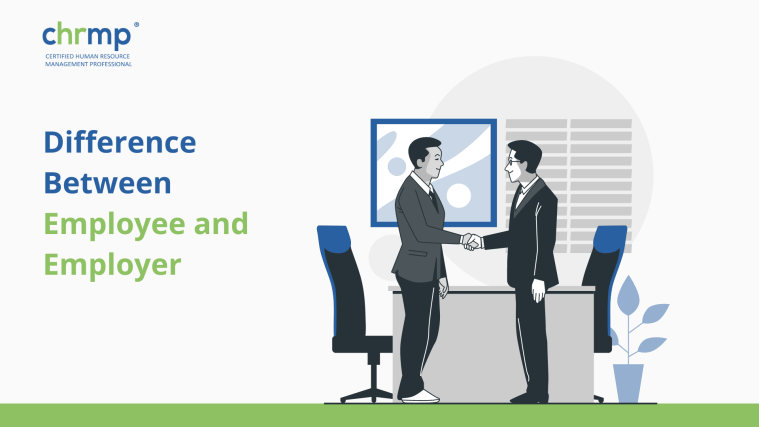
Top 9 Differences Between an Employee and Employer: A Comprehensive Guide
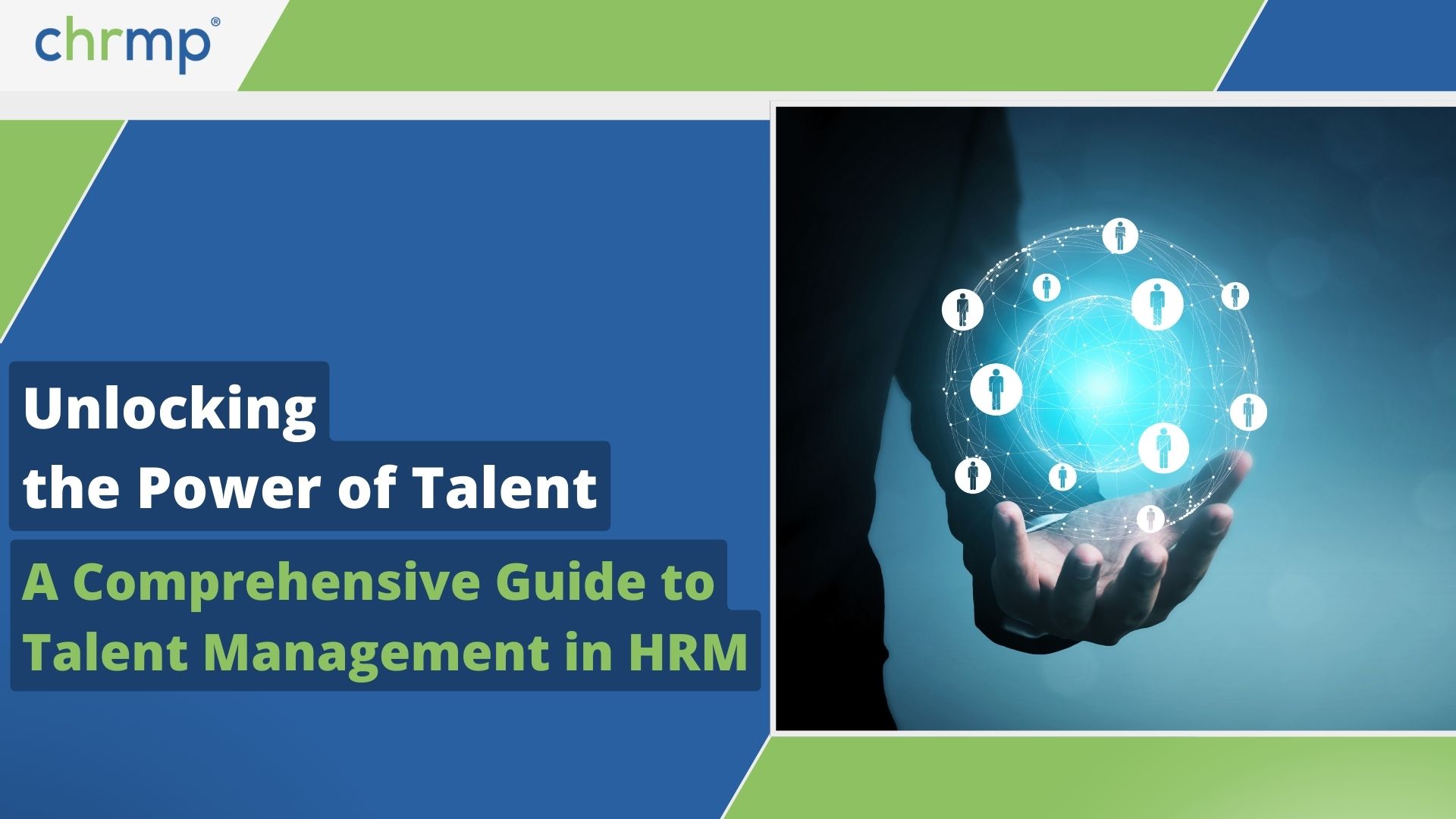
Unlocking the Power of Talent: A Comprehensive Guide to Talent Management in HRM
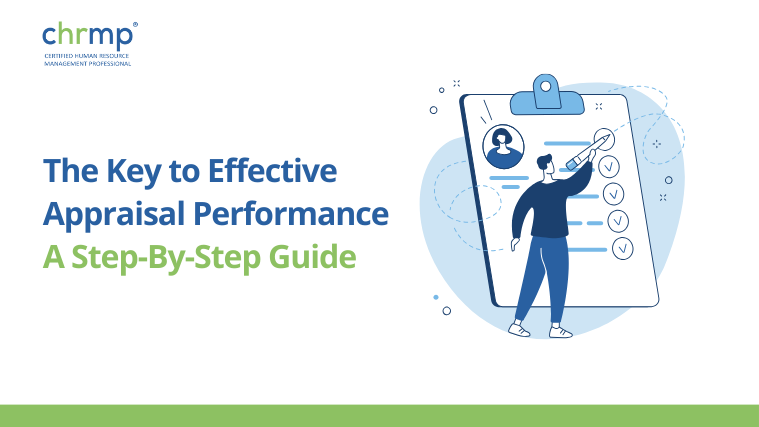
The Key To Effective Appraisal Performance- A Step-By-Step Guide
Become a certified human resource management professional, certifications.
- CHRMP Foundation
- HRBP Advanced
- CHRMP C&B Planning
- CHRMP Competency Mapping
- CHRMP Talent Acquisition
- CHRMP Talent Development
- CHRMP Tableau for HR
- HR Certification
- Competency Model
- For Fresh Graduates
- Career Change To HR
- Existing HR Professionals
- Employer/HR Manager
- Academy Login
- Academy Status Page
- Academy Roadmap
- Alumni Testimonials
- Know Your Facilitator
- International Affiliates
- Indian Affiliates
- Channel Partners
- CA Employee Nomination Form
- CHRMP Perspectives
- Webinar Series
- Support Center
- Covid-19 HR Policy
- Coronavirus Resources
- CHRMP L&D GPT
- Cookies Policy
- Privacy Policy
- Terms of Service
- Refund & Cancellation Policy
Most Popular Location for Our Online HR Courses
Get Your Program Plan
Fill in the below details to get a CHRMP HR Analytics Program Plan.
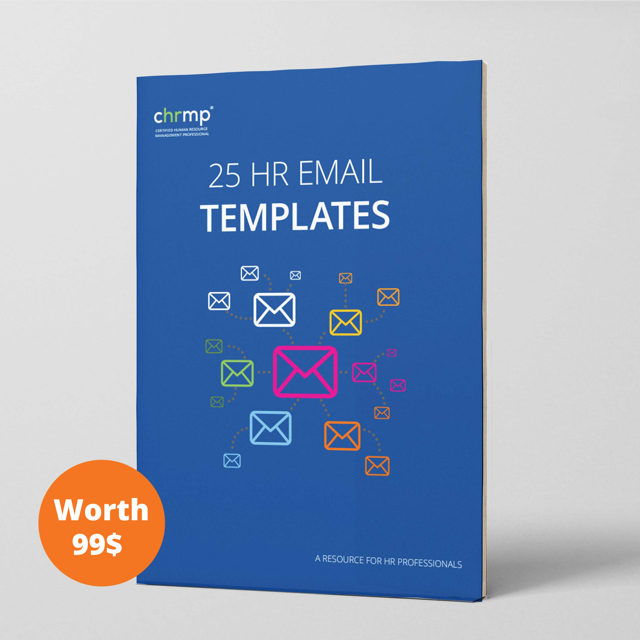
HR Made Easy
Grab Your 25 Free Email Templates Now
Bridging the gap: why, how and when HR analytics can impact organizational performance
Management Decision
ISSN : 0025-1747
Article publication date: 12 January 2022
Issue publication date: 19 December 2022
Despite the growth and adoption of human resource (HR) analytics, it remains unknown whether HR analytics can impact organizational performance. As such, this study aims to address this important issue by understanding why, how and when HR analytics leads to increased organizational performance and uncover the mechanisms through which this increased performance occurs.
Design/methodology/approach
Using data collected from 155 Irish organizations, structural equation modeling was performed to test the chain mediation model linking HR technology, HR analytics, evidence-based management (EBM) and organizational performance.
The study's findings support the proposed chain model, suggesting that access to HR technology enables HR analytics which facilitates EBM, which in turn enhances organizational performance.
Originality/value
This research contributes significantly to the HR analytics and EBM literature. First, the study extends our understanding of why and how HR analytics leads to higher organizational performance. Second, the authors identify that access to HR technology enables and is an antecedent of HR analytics. Finally, empirical evidence is offered to support EBM and its impact on organizational performance.
- Human resource (HR) analytics
- People analytics
- Evidence-based management
Organizational performance
- Human resource management
- Human capital analytics
McCartney, S. and Fu, N. (2022), "Bridging the gap: why, how and when HR analytics can impact organizational performance", Management Decision , Vol. 60 No. 13, pp. 25-47. https://doi.org/10.1108/MD-12-2020-1581
Emerald Publishing Limited
Copyright © 2021, Steven McCartney and Na Fu
Published by Emerald Publishing Limited. This article is published under the Creative Commons Attribution (CC BY 4.0) licence. Anyone may reproduce, distribute, translate and create derivative works of this article (for both commercial and non-commercial purposes), subject to full attribution to the original publication and authors. The full terms of this licence may be seen at http://creativecommons.org/licences/by/4.0/legalcode
Introduction
The concept and application of data and analytics in management have seen increasing attention as researchers and professionals aim to understand how data can be transformed into actionable insights leading to improved organizational performance ( Chierici et al. , 2019 ; Ferraris et al. , 2019 ; Santoro et al. , 2019 ; Singh and Del Giudice, 2019 ). Consequently, this interest has transcended various management disciplines, including human resources management (HRM), which is evidenced by the growing number of HR departments implementing HR analytics to improve decision-making ( Marler and Boudreau, 2017 ; Fernandez and Gallardo-Gallardo, 2020 ; McCartney et al ., 2020 ). Despite its increased popularity, HR analytics is not an entirely new concept ( Huselid, 2018 ). Rather, HR analytics has emerged from previous research on the impact of HR practices such as selection, training and performance management, which has a long history in social sciences, including industrial and organizational psychology, HRM and organizational behaviour [1] . What is new, however, is that HR analytics in contemporary organizations has shifted from “ assessing the levels associated with a particular workforce attribute (e.g. what is our cost per hire?) to understanding the impact of the workforce on the execution of firm strategy (e.g. how might an increase in the quality of our project managers affect our new product cycle time?) ” ( Huselid, 2018 , p. 680). In other words, HR analytics not only centers on investigating and improving elements of human capital but also applying analytical techniques coupled with people data to inform organizational strategy and improve performance.
Furthermore, the significant growth of access to HR technology, including human resource information systems (HRISs), cloud platforms and apps, has offered HR departments the ability to collect, manage and analyze large volumes of employee data, compared to earlier legacy IT systems ( Bondarouk and Brewster, 2016 ; Marler and Boudreau, 2017 ; Kim et al ., 2021 ). Such shift has also acted as a driver of HR analytics and increased its adoption within HR departments. For example, through the use of advanced HR technology to gather and analyze candidate and employee data, Google's HR analytics team has developed an evidence-based approach to improve its recruitment and selection process by identifying several elements of high performance that could predict a candidate's likelihood of success ( Harris et al ., 2011 ; Shrivastava et al ., 2018 ). Similarly, in addition to recruitment and selection, HR analytics offers organizations the ability to address various other HR challenges, including employee engagement, diversity and inclusion, and turnover ( Harris et al ., 2011 ; Andersen, 2017 ; Buttner and Tullar, 2018 ; Levenson, 2018 ; Simón and Ferreiro, 2018 ).
To date, the extant HR analytics literature has focused on many areas, including the current limitations and challenges facing the development of HR analytics ( Boudreau and Cascio, 2017 ; Levenson and Fink, 2017 ; Huselid, 2018 ; Minbaeva, 2018 ; Jeske and Calvard, 2020 ), best practices in developing and utilizing HR analytics ( Green, 2017 ; Falletta and Combs, 2020 ), and the impact and importance of analytical skills ( Kryscynski et al. , 2018 ; McCartney et al ., 2020 ). In addition, several reviews have been published offering a holistic view of the current state of HR analytics research ( Marler and Boudreau, 2017 ; Tursunbayeva et al ., 2018 ; Fernandez and Gallardo-Gallardo, 2020 ; Margherita, 2020 ). Despite the advancement of HR analytics literature and the number of case studies claiming that HR analytics allows organizations to improve their performance ( Marler and Boudreau, 2017 ; Fernandez and Gallardo-Gallardo, 2020 ; Margherita, 2020 ), research investigating how and to what extent HR analytics impacts and influences organizational performance remains scarce ( Huselid, 2018 ; Minbaeva, 2018 ). On this basis, this study seeks to understand how and why HR analytics influences organizational performance by theorizing and testing its underlying mechanisms.
This study draws on evidence-based management theory (EBM, Rousseau and Barends, 2011 ; Baba and HakemZadeh, 2012 ; Bezzina et al. , 2017 ), the resource-based view of the firm (RBV, Barney, 1991 ) and dynamic capabilities ( Teece et al ., 1997 ; Winter, 2003 ) as the underlying frameworks linking access to HR technology, HR analytics, EBM and organizational performance. These theoretical frameworks are justified as EBM is concerned with incorporating and deploying scientific and organizational facts coupled with expert and stakeholder judgment to make managerial decisions ( Rousseau and Barends, 2011 ; Baba and HakemZadeh, 2012 ). At the same time, HR analytics contributes to organizational evidence creation through acquiring and translating high-quality workforce data into information, resulting in critical organizational insights ( Marler and Boudreau, 2017 ; Minbaeva, 2018 ; Coron, 2021 ). Further, in line with previous studies exploring the performance impact of HR ( Delaney and Huselid, 1996 ; Guthrie, 2001 ; Fu et al. , 2017 ), this study integrates an RBV ( Barney, 1991 ) and dynamic capability ( Teece et al ., 1997 ) perspective to propose a chain model demonstrating that access to HR technology enables HR analytics (resource) which facilitates EBM (capability) which in turn enhances organizational performance.
By theorizing the chain model between access to HR technology, HR analytics, EBM and organizational performance, this study extends our understanding of why and how HR analytics leads to higher organizational performance. Additionally, this study addresses the conditional effect of HR technology as an antecedent of HR analytics. Finally, the study adds empirical evidence linking EBM to organizational performance, which at present is rare ( Baba and HakemZadeh, 2012 ). Together, these contributions offer a solid foundation for the strategic importance of HR analytics and EBM.
This paper's subsequent sections are structured as follows: First, the literature review and hypotheses section will summarize existing research in HR analytics, outline the five hypotheses tested within the paper and present the theoretical model. Second, the research methodology will describe the data collection process and offer a detailed explanation of the survey measures. Third, the research findings are presented, providing analysis and support for each of the hypotheses tested. Lastly, the paper's theoretical contributions to HR analytics and EBM are presented, implications for practice, limitations and areas for future research are discussed.
Literature review and hypotheses
Hr analytics: definition and development.
As a result of the ongoing digital transformation, many HR departments have begun to engage with workforce data to make data-driven decisions in areas such as recruitment and selection, performance measurement, diversity and inclusion and workforce planning ( Harris et al ., 2011 ; Kane, 2015 ; Rasmussen and Ulrich, 2015 ; Marler and Boudreau, 2017 ; Hamilton and Sodeman, 2020 ; Tursunbayeva et al. , 2021 ). This application of using workforce data to improve decision-making has been synonymously referred to by scholars as HR analytics ( Aral et al ., 2012 ; Rasmussen and Ulrich, 2015 ; Angrave et al. , 2016 ; Marler and Boudreau, 2017 ; McCartney et al ., 2020 ), people analytics ( Kane, 2015 ; Green, 2017 ; Nielsen and McCullough, 2018 ; Tursunbayeva et al ., 2018 ; Peeters et al ., 2020 ), talent analytics ( Harris et al ., 2011 ; Sivathanu and Pillai, 2020 ), human capital analytics ( Andersen, 2017 ; Boudreau and Cascio, 2017 ; Levenson and Fink, 2017 ; Minbaeva, 2018 ) and workforce analytics ( Huselid, 2018 ; Simón and Ferreiro, 2018 ).
Regardless of the term used, consistency exists in both academia and practice for the strategic importance of HR analytics as it provides organizations with data, information and insights to effectively make informed data-driven decisions ( Huselid, 2018 ; Minbaeva, 2018 ). For example, according to van den Heuvel and Bondarouk (2017) , HR analytics is the systematic identification and quantification of the people drivers of business outcomes to make better decisions. Equally important is the notion that these insights can be generated at varying levels of technological sophistication ( Margherita, 2020 ; Sivathanu and Pillai, 2020 ). For example, according to Margherita (2020) , HR analytics follows a linear three-stage maturity model. At its lowest level, “descriptive,” HR analytics focuses on using HR technology to generate reports and dashboards to answer questions concerning what has happened. Next, the “predictive” stage utilizes statistical techniques, advanced algorithms and machine learning to anticipate what might happen in the future and why. Lastly, the “prescriptive” stage centers on determining the optimal action that should be taken in response to the analysis.
This study adopts the HR analytics definition proposed by Marler and Boudreau (2017) , where HR analytics is “ an HR practice enabled by information technology that uses descriptive, visual, and statistical analyses of data related to HR processes, human capital, organizational performance, and external economic benchmarks to establish business impact and enable data-driven decision-making ” (p. 15). In light of this definition, this paper operationalizes HR analytics through the adoption of the human capital analytics framework ( Minbaeva, 2018 ), where HR analytics comprises of three dimensions: high-quality data, analytical competence and strategic ability to act. According to the human capital analytics framework, the dimension of high-quality data suggests that the data used for analytics needs to be accurate, consistent, timely and complete. For instance, organizations need to ensure that the data being used to conduct HR analytics is accurate. Without accurate data, the insights gained from the analytics will be unreliable and offer no benefit to the organization ( Minbaeva, 2018 ; Wamba et al. , 2019 ; Peeters et al ., 2020 ). Alternatively, inaccurate data could lead to implementing solutions that do not address the business's actual challenges. HR analytics also requires a high degree of analytical competence, referring to the analytics team's ability to apply statistical analysis and techniques to workforce data to transform data into valuable insights ( McCartney et al ., 2020 ). For example, the analytics team needs to frame relevant research questions and answer them through developing causal models and performing sophisticated statistical analysis ( Minbaeva, 2018 ). Moreover, the team needs to translate the insights gained into a compelling analytics narrative or story ( Andersen, 2017 ; Minbaeva, 2018 ; McCartney et al ., 2020 ). Lastly, the strategic ability to act refers to having the required managerial support to make decisions and implement solutions based on the data, information and insight gathered from HR analytics.
Furthermore, we regard HR analytics as a valuable, rare, inimitable and non-substitutable resource for organizations based on the data, information and insights generated by HR analytics. This argument is justified by the many parallels that can be drawn and have been indirectly implied by scholars when it comes to HR analytics as an organizational resource ( Marler and Boudreau, 2017 ). For example, researchers and practitioners have discussed the value offered by HR analytics through its ability to allow HR to identify and address workforce challenges ( Marler and Boudreau, 2017 ; Huselid, 2018 ; Kryscynski et al. , 2018 ; McIver et al ., 2018 ; Minbaeva, 2018 ). In addition, the HR analytics literature has also referred to the rarity of high-quality HR analytics programs suggesting that many organizations struggle to utilize workforce data only offering basic reporting and descriptive statistics ( Angrave et al. , 2016 ; King, 2016 ; Andersen, 2017 ; Green, 2017 ; Levenson and Fink, 2017 ; Minbaeva, 2018 ). As such, effective HR analytics programs are rare at present. Concerning the imitability of HR analytics, according to Minbaeva (2018) , to utilize and conduct value-adding HR analytics, organizations need to have high-quality data, analytical capabilities and the strategic ability to act. However, it is difficult for HR departments to have all three elements given the low levels of technology, poor data quality, few resources, lack of analytical competencies and a lack of buy-in from senior management ( Andersen, 2017 ). Finally, HR analytics is its own stand-alone practice, meaning no available alternatives or substitutes can gain similar insights ( Falletta and Combs, 2020 ). Taken collectively, HR analytics meets the requirements set out by RBV, suggesting that HR analytics and the data, information and insight it creates, is a valuable resource for organizations with the potential to generate competitive advantage.
Linking HR analytics to EBM
The data, information and insights generated from HR analytics are not enough to generate competitive advantage alone. Instead, organizations must also deploy and incorporate the evidence effectively ( Sirmon et al ., 2007 ; Lin and Wu, 2014 ; Fu et al. , 2017 ). This idea is consistent with dynamic capabilities, which suggests that competitive advantage depends on an organization's capacity to successfully incorporate, develop and reconfigure its resources ( Teece et al ., 1997 ). Similarly, according to Baba and HakemZadeh (2012) , EBM is a dynamic process where evidence is first gathered and then interpreted, forming the foundation of managerial decision-making. Accordingly, this study adopts EBM theory to argue that the evidence and organizational facts generated by HR analytics can be used to make strategic decisions and facilitate EBM.
The idea of making decisions based on several sources of information, including organizational facts such as analytics, is a foundational element in evidence-based practice ( Walshe and Rundall, 2001 ; Briggs and McBeath, 2009 ; Rousseau and Barends, 2011 ; Baba and HakemZadeh, 2012 ; Coron, 2021 ). This decision-making methodology originated within the healthcare profession to better use scientific research to inform decision-making concerning patient care ( Walshe and Rundall, 2001 ; Pfeffer and Sutton, 2006 ; Briggs and McBeath, 2009 ; Baba and HakemZadeh, 2012 ; HakemZadeh and Baba, 2016 ). More recently, this approach to decision-making has been advocated for by various scholars suggesting that management decisions should be based on the combination of critical thinking coupled with the best sources of evidence ( Rousseau, 2006 ). These sources of information include scientific evidence found in peer-reviewed academic papers, organizational facts such as metrics and analytics, professional experience and judgment, and considering the outcome on affected stakeholders ( Rousseau, 2006 ; Rousseau and Barends, 2011 ; Baba and HakemZadeh, 2012 ; Bezzina et al. , 2017 ; Cassar and Bezzina, 2017 ). Moreover, according to Barends et al . (2014) , EBM comprises of six activities, including asking, acquiring, appraising, aggregating, applying and assessing. For example, organizations must translate an issue or problem into an answerable question (asking), systematically search for and retrieve the best available evidence (acquiring), critically judge the trustworthiness and relevance of the evidence (appraising), weigh and pull together the evidence (aggregating), incorporate the evidence into the decision-making process (applying) and evaluate the outcome of the decision (assessing).
HR analytics is positively associated with organizational EBM.
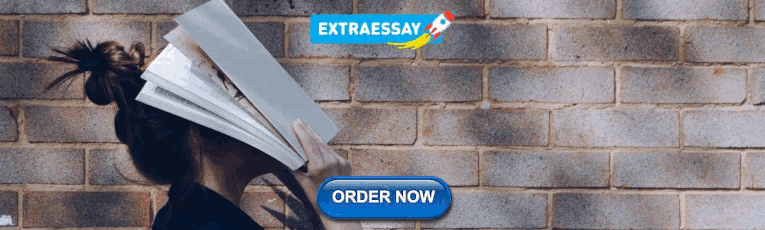
Linking EBM to organizational performance
Every day, managers and senior executives are faced with making critical decisions to improve the success of their organizations. Although some decision-makers will utilize a wide range of evidence to support their decisions, many justify their decisions based on gut feeling, outdated information, personal experience or a combination of the three ( Rousseau and Barends, 2011 ; Baba and HakemZadeh, 2012 ). As such, management scholars have urged for a shift in management decision-making, placing considerable emphasis on promoting EBM ( Pfeffer and Sutton, 2006 ; Rousseau, 2006 ; Briner et al ., 2009 ; Morrell and Learmonth, 2015 ; Rynes and Bartunek, 2017 ). This comes as a result of significant developments being made in healthcare toward the performance impact of EBM; specifically, the literature centered on healthcare quality and patient and hospital outcomes ( Melnyk et al. , 2014 ; Aloini et al. , 2018 ; Janati et al. , 2018 ; Roshanghalb et al. , 2018 ).
Organizational EBM is positively associated with organizational performance.
Building on this notion, HR analytics also generates evidence in the form of organizational facts, providing managers and executives with actionable insights which can be used as evidence in decision-making. Similarly, when organizations use and deploy the insights derived from HR analytics coupled with other sources of evidence to make decisions, it is likely to improve decision-making effectiveness, leading to higher organizational performance. This is evidenced in various case studies that have focused on how HR analytics facilitates evidence-based decision-making to improve HR and business performance ( Harris et al ., 2011 ; Rasmussen and Ulrich, 2015 ; Marler and Boudreau, 2017 ; Buttner and Tullar, 2018 ; Gelbard et al. , 2018 ; McIver et al ., 2018 ; Minbaeva, 2018 ). For example, a recent case study conducted by Simón and Ferreiro (2018) describes developing and implementing an HR analytics program at Inditex, a large Spanish multinational fashion retail group. In collaboration with the authors, Inditex developed key performance indicators centered around workforce analytics. Doing so led HR managers at Inditex to gain and apply critical evidence to make more informed decisions around their workforce, resulting in higher overall store performance ( Simón and Ferreiro, 2018 ). Similarly, Bank of America, working in collaboration with Humanyze (an HR analytics software provider), used HR analytics to improve HR and business outcomes ( Kane, 2015 ). To do so, Humanyze designed and developed ID badges for Bank of America employees, adding microphones, Bluetooth and infrared technology to facilitate workforce data collection ( Kane, 2015 ). Their findings determined that how employees interacted with their coworkers was the most significant factor in predicting productivity ( Kane, 2015 ). Based on this evidence, Bank of America implemented solutions to the working environment that led to increased team cohesion by 18%, a reduction in stress by 19% and a 23% increase in productivity ( Kane, 2015 ).
Organizational EBM mediates the relationship between HR analytics and organizational performance.
Access to HR technology enabling HR analytics
The rapid advancement of information technology has sparked a digital revolution, with organizations taking advantage of big data to address previously unknown opportunities ( Wamba et al. , 2015 ; Wamba et al. , 2017 ; Dubey et al. , 2019 ; Kim et al ., 2021 ). This is no exception for HR, as departments have shifted toward a more technology-enabled HR department ( Boudreau and Cascio, 2017 ; Marler and Boudreau, 2017 ; van den Heuvel and Bondarouk, 2017 ; McCartney et al ., 2020 ).
Accordingly, access to HR technology such as HRISs and other forms of electronic HRM (e-HRM) platforms have been a driving force in the implementation and growth of HR analytics ( Ashbaugh and Miranda, 2002 ; Dulebohn and Johnson, 2013 ; McIver et al ., 2018 ; Schiemann et al ., 2018 ; Kim et al ., 2021 ; Zhou et al. , 2021 ). For example, HRIS allows for capturing, storing, manipulating, retrieving and distributing HR data and are equipped with the functionality to generate reports on key performance indicators (KPIs) ( Hendrickson, 2003 ; Stone et al. , 2015 ). Furthermore, these systems can add more advanced analytics and reporting modules to predict short- and long-term workforce trends by incorporating big data, business intelligence and statistical applications ( Kapoor and Sherif, 2012 ; Stone et al. , 2015 ; van den Heuvel and Bondarouk, 2017 ; McIver et al ., 2018 ; Garcia-Arroyo and Osca, 2019 ; Mikalef et al. , 2019 ). More recently, advancements in HR technology platforms have led to integrating artificial intelligence (AI) solutions, including chatbots, for streamlining HR processes ( Buck and Morrow, 2018 ; van Esch and Black, 2019 ; Black and van Esch, 2020 ). As can be seen, HR technology evolves along a continuum from basic data collecting and storage (i.e. HRIS) to more robust platforms with AI and analysis capabilities. Although it is essential to distinguish among these differences, this study does not focus on the sophistication level of HR technology. Instead, this study aims to understand whether HR departments that have access to HR technology at any point on the continuum can adequately leverage it to enable HR analytics. As such, this study defines access to HR technology as an HR department that invests in and implements an HR software tool that allows for the recording, storage and perform analysis of data surrounding an organizations human resources that can be used by members of the department ( Aral et al ., 2012 ; Stone et al. , 2015 ; Marler and Boudreau, 2017 ; Kim et al ., 2021 ; Maamari and Osta, 2021 ).
Building on that notion, this study argues that access to HR technology is a driving force in HR analytics adoption and enables and acts as an antecedent to HR analytics. The justification for this is two-fold. First, HR technology serves as the foundation for HR analytics as it allows HR professionals timely access to workforce data that can be used to make more informed and data-driven decisions ( Lengnick-Hall and Mortiz, 2003 ; Johnson et al ., 2016 ; King, 2016 ; McIver et al ., 2018 ). For example, according to McIver et al. (2018) , HR technology enables the collection, cleaning and manipulation of various data types from several data sources that can be used to aid organizational decision-making. Thus, meeting the first element of the HR analytics framework high-quality data ( Minbaeva, 2018 ).
Second, HR technology facilitates the process of transforming workforce data into information, where executives, HR professionals and line managers can make strategic workforce decisions through its ability to conduct statistical and predictive analysis ( Aral et al ., 2012 ; Fernandez and Gallardo-Gallardo, 2020 ). According to van der Togt and Rasmussen (2017) , insights derived from HR analytics are enabled by HR technology as they can perform sophisticated statistical analyses such as regression on longitudinal and cross-functional data. Moreover, HR technology allows HR professionals to aggregate and perform predictive analytics, which would otherwise not be possible without HR technology ( Ulrich and Dulebohn, 2015 ). Current HR technology platforms also offer a wide range of functionality, allowing HR professionals to translate data into meaningful insights through their ability to generate dashboards, scorecards and data visualizations ( Ulrich and Dulebohn, 2015 ; Angrave et al. , 2016 ; Marler and Boudreau, 2017 ; McIver et al ., 2018 ). For instance, according to Ulrich and Dulebohn (2015) , dashboards and scorecards are descriptive analytics that HR professionals can utilize to compare and visualize various HR metrics over time. Similarly, McIver et al. (2018) suggest that dashboards offer HR professionals a way to efficiently illustrate workforce trends to help drive questions and take advantage of emerging workforce opportunities. Thus, meeting the two final elements of the HR analytics framework (analytical competence and strategic ability to act) ( Minbaeva, 2018 ).
Access to HR technology is positively associated with HR analytics.
As suggested above, access to HR technology enables HR analytics through its ability to collect and analyze workforce data. Building on this notion, and drawing upon the RBV of the firm ( Barney, 1991 ) and dynamic capabilities ( Teece et al ., 1997 ), this study proposes that HR analytics (i.e. high-quality data, analytical capabilities and strategic ability to act) is an organizational resource that can transform data into evidence in the form of organizational facts which is deployed through the organizational EBM capability leading to improved organizational performance.
Access to HR technology is positively linked with HR analytics which in turn facilitates organizational EBM, ultimately leading to organizational performance.
Figure 1 presents the theoretical model.
Research methodology
Data collection.
An online survey focusing on HR analytics and organizational performance was developed in collaboration with a large professional recruitment agency in Ireland. The survey was pilot-tested among several HR managers and senior managers with significant knowledge of the organization's performance metrics to ensure face validity. Some questions were minorly revised to achieve face validity. The survey was then distributed online to HR managers, business partners and senior management teams in 8,116 organizations. The organizations surveyed covered several sectors, including accounting, legal, banking and financial services, marketing, ICT, human resources and insurance sectors. After the initial email invitations were distributed, 51 organizations bounced back and 117 organizations chose to opt out of the survey, leaving 7,948 as the final population. Overall, a total of 260 responses were received, generating an overall response rate of 3%. After removing incomplete responses and organizations that completed less than one-third of the survey, the valid sample size was 155. The low response rate in this study was not surprising given that the response rate at the organizational level is much lower than that at the individual level and has been declining over time in management research ( Baruch and Holtom, 2008 ).
To examine the representativeness and detect the difference between the valid sample and the deleted responses, a one-way analysis of variance (ANOVA) was carried out. Similarly, a comparative analysis of early responses and late responses was conducted to determine the sample's representativeness ( Wilcox et al ., 1994 ). This is consistent with existing studies that have checked non-response bias by comparing demographic and contextual variables between early and late respondents ( Armstrong and Overton, 1977 ; Guthrie et al. , 2009 ; Fu et al. , 2017 ).
The ANOVA findings showed no significant difference in organizational size, organizational age, and sectors between the complete and incomplete respondents and no significant difference among early and late respondents. Therefore, we concluded our sample to be valid and continued our analysis with the 155 respondents representing 155 organizations.
Sample profile
Among the respondents, 53% were male, with 76% of respondents holding positions of HR managers/directors or senior managers. The average work tenure of respondents was nine years (SD = 8). Most organizations surveyed represented private organizations, with 88% of the respondents identifying as private. Concerning the industries represented, 30% of organizations belonged to the ICT industry, 25% were financial service firms and 13% were professional services, including accounting, architecture, and consulting and law firms. The remaining organizations represented industries, including construction, transport and communications.
Measurements
To measure organizational performance, seven items were adopted from Delaney and Huselid (1996) . Respondents were asked to rate their organization's performance relative to their competitors using a five-point Likert-type scale (1 = much weaker to 5 = much stronger). Example measures include “Ability to attract essential employees,” “Ability to retain essential employees,” “Quality of services” and “Customer service.” The reliability was assessed, showing a Cronbach's alpha of 0.87.
While concerns about the use of subjective performance data can be raised, several previously published studies examining HR and firm performance research have used self-reported performance measures ( Delaney and Huselid, 1996 ; Youndt et al. , 1996 ; Sun et al ., 2007 ; Takeuchi et al. , 2007 ; Chuang and Liao, 2010 ; Fu et al. , 2018 ). As the previous studies have shown, the rationale for using subjective performance data is partly due to the difficulty and inability to access the objective performance measures ( Gupta and Govindarajan, 1984 , 1986 ; Gupta, 1987 ). Similarly, the comparative method allows for more participants' responses rather than requiring respondents to provide exact figures ( Tomaskovic-Devey et al ., 1994 ). Finally, as evidenced by Wall et al . (2004) , subjective and objective measures of company performance are positively linked at 0.52.
Along with difficulty collecting objective performance, the organizations involved in this study represent several different service industries; therefore, financial performance, i.e. fee income, might not be the best indicator for firm performance. To validate the organizational performance measure, the authors conducted a second round of data collection six months later. Among the 155 organizations, only 36 responses were received. Respondents answered the same questions on organizational performance. The correlation between organizational performance at two-time points was significant ( r = 0.36, p < 0.05). Although the correlation was significant, the coefficient was not large. Upon reflection, we believe there might be a few factors influencing the low correlation coefficient. First, this study involves multiple industries, and industry-wide economic changes might be one factor [2] . Due to the limited sample, we would not be able to test this. Second, the relatively long-time lag (6 months) may also explain the changes as, within the last six months, organizations may have undergone several changes that have influenced their performance.
Organizational EBM
To measure organizational EBM, six items were developed based on EBM's definition in Rousseau (2006) and Barends et al. (2014) . Respondents were asked to indicate to what degree they agree or disagree with the following statements: “We translate an issue or problem into an answerable question” (asking), “We systematically search for and retrieve the best available evidence” (acquiring), “We critically judge the trustworthiness and relevance of the evidence we collect” (appraising), “We weigh and pull together the evidence” (aggregating), “We incorporate the evidence into the decision-making process” (applying) and “We evaluate the outcome of the decision” (assessing). Each item was evaluated on a five-point Likert scale (1 = strongly disagree, and 5 = strongly agree). The Cronbach's alpha was 0.93.
HR analytics
Given that no valid scale has been developed to measure HR analytics, this study applies the theoretical framework proposed by Minbaeva (2018) and adopts questions from established scales to reflect the theoretical definition. For the first dimension, high-quality data, we adopted five questions from Pipino et al . (2002) , which include: “The HR data we have is correct and reliable” (accuracy), “The HR data we have is sufficiently up to date” (timeliness), “The HR data we have is presented in the same format” (consistency), “The HR data we have is complete and no necessary data is missing” (completeness) and “The HR data we have is collected on a regular basis” (data process). The Cronbach's alpha for data quality was 0.91.
For the second dimension, analytical competency, we adopted five items from Kryscynski et al . (2018) . Example items include “Our HR Department translates data into useful insights”, “Our HR department identifies problems that can be solved with data” and “Our HR Department effectively uses HR analytics to create value for my organization.” The Cronbach's alpha for analytical capability was 0.95. Finally, the strategic ability to act was operationalized through three questions adopted from Minbaeva (2018) , including “Our HR Department has success stories to justify HR analytics projects”, “Our HR Department inspires relevant organizational stakeholders (e.g. senior management teams and line managers) to take action on the basis of their findings” and “The data-driven insights that we provide are used by our organization's stakeholders.” The Cronbach's alpha for analytical capability was 0.86.
Each of the three dimensions of the HR analytics’ measure was evaluated based on a five-point Likert scale (1 = strongly disagree and 5 = strongly agree). We conducted a second-order confirmatory factor analysis (CFA) for the HR analytics measure to examine the new scale's validity. The model fit indexes indicated an acceptable model fit for the second-order CFA with three first-order dimensions ( χ 2 / df = 171.65/72 = 2.38, p < 0.001; comparative fit index [CFI] = 0.95; Tucker–Lewis Index [TLI] = 0.93; root-mean-square error of approximation [RMSEA] = 0.09; and the standardized root mean square residual [SRMR] = 0.05). Considering HR analytics as a theorized higher-order concept in this study and the CFA's support for the higher-order factor structure, we treated HR analytics as one overall concept with three dimensions in the model test.
Access to HR technology
Three items were adapted from measures previously used by Aral et al . (2012) . The three items developed were “My organization has the necessary tools to conduct HR analytics,” “My organization invests in the tools needed to conduct HR analytics” and “My organization has the appropriate tools for performing HR analytics.” Respondents evaluated these statements based on a five-point Likert scale (1 = strongly disagree and 5 = strongly agree). The Cronbach's alpha was 0.93.
Control variables
In the analysis, we controlled several contextual variables with the potential to influence the use of HR analytics, EBM, HR technology and organizational performance such as organization size, organization age, organization type (multinational or domestic), sector and industries. Organization size was measured using three categories: 1 = small organizations (less than 50 employees), 2 = medium organizations (between 50 and 250 employees) and 3 = large organizations (more than 250 employees). Organization age was operationalized as the natural log of the actual organization age. Organization type (multinational or domestic) was measured using a dummy variable (1 = multinational companies; 0 = domestic companies). Sector was measured using a dummy variable (1 = private, 0 = public or semi-state). The industry was measured by four categories (1 = professional services including accounting, advertising, architecture, consulting and law firms; 2 = Information, communications and technology (ICT); 3 = financial services including banking, insurance, compliance and risk firms; and 4 = other services including education, healthcare, pharmaceutical etc.). Three dummy variables were created for the industry variable using ICT as the baseline category.
Common method bias
It was necessary to check whether common method bias was present in the study since all variables were collected from a single source. To address this concern, this study follows several recommendations made by Podsakoff et al . (2003) and Podsakoff et al . (2012) . For instance, before launching the survey, it was piloted with a group of HR managers and was revised and retested several times. Changes made as a result included the wording and order of the questions. Likewise, during the data analysis stage, we assessed the common method variance by carrying out a series of CFA to establish the validity of the studied variables. Likewise, we added one unrelated common factor to the CFA with enforced equal factor loadings to all items in evaluating the common method variance ( Podsakoff et al ., 2012 ). The squared regression estimates indicated a common variance of 3%, indicating no significant concern for common method bias.
Table 1 presents the descriptive statistics of the core variables in this study, including the mean, standard deviation and correlations.
Measurement models
Analysis was conducted using Mplus 8.0. A full measurement model was tested using three pre-calculated variables (data quality, analytical capability and strategic ability to act) loaded on one general factor representing HR analytics. EBM, HR technology and organizational performance items loaded on to their respective factors. The four-factor model showed a good model fit ( χ 2 / df = 236.93/143 = 1.66, p < 0.001; CFI = 0.95; CLI = 0.94; RMSEA = 0.07; SRMR = 0.07) with factor loadings higher than 0.55 ( p < 0.001). We then carried out χ 2 difference tests that compared this full measurement model to seven alternative nested models, as shown in Table 2 . The comparison results reveal that the model fit of the full measurement model was significantly better than the alternative models (all at p < 0.001), suggesting that the study's variables are distinct.
Structural models
We carried out the structural equation modeling in Mplus 8.0. Figure 2 presents the results.
Hypothesis 1 proposed that HR analytics would be positively linked to organizational EBM. Results in Figure 2 show that the standardized coefficient of organizational EBM on HR analytics was positive and significant ( β = 0.30, p < 0.05). Therefore, Hypothesis 1 was supported. Hypothesis 2 proposed that organizational EBM would be positively linked to organizational performance. Figure 2 shows that the standardized coefficient of organizational performance on EBM was 0.41 ( p < 0.001). Thus, Hypothesis 2 was supported.
Hypothesis 3 proposed the mediating role of organizational EBM in the relationship between HR analytics and organizational performance. According to Baron and Kenny (1986) and Hayes (2013) , three conditions need to be tested for the mediation model. The first two conditions include the significant relationships between the independent variable and the mediator and between the mediator and the dependent variable. The third condition requires the reduced relationship between the independent variable and dependent variable after including the mediator. Support for Hypotheses 1 and 2 met the first two conditions. The direct impact of organizational performance on HR analytics was 0.31 ( p < 0.05). After including the mediator–organizational EBM–the coefficient of organizational performance on HR analytics became non-significant ( β = 0.20, n.s.), meeting the third condition. To further test EBM's mediating effect in the relationship between HR analytics and organizational performance, this study adopted a bootstrapping test recommended by Hayes and Preacher (2014) . The bootstrapping test results reveal that the indirect effect of HR analytics and organizational performance through EBM was 0.16 ( p < 0.05), with a 95% confidence interval between 0.007 and 0.321. As such, Hypothesis 3 was supported, suggesting that EBM mediates the relationship between HR analytics and organizational performance.
Hypothesis 4 proposed that HR technology is positively associated with HR analytics. It is supported by the positive and significant coefficient for HR technology on HR analytics ( β = 0.71, p < 0.001). Hypothesis 5 proposed a chain model linking HR technology to organizational performance via the mediators of HR analytics and organizational EBM. The support for Hypotheses 1 to 4 confirms the significant impact of HR technology on HR analytics ( β = 0.71, p < 0.001), which in turn facilitates organizational EBM ( β = 0.30, p < 0.05), ultimately leading to organizational performance ( β = 0.41, p < 0.001). In addition, the indirect impact of organizational performance on HR technology via HR analytics and organizational EBM was calculated as 0.06 ( p < 0.05) with a 95% confidence interval between 0.0013 and 0.117. Therefore, Hypothesis 5 on the chain model of HR technology–HR analytics–organizational EBM–organizational performance was supported.
Despite the claimed importance of HR analytics, research investigating the performance impact of HR analytics on organizational performance remains underdeveloped ( Rasmussen and Ulrich, 2015 ; Baesens et al ., 2017 ; Levenson and Fink, 2017 ; Marler and Boudreau, 2017 ; Huselid, 2018 ; Greasley and Thomas, 2020 ). As such, this study sets out the first attempt to (1) theorize and establish the relationship between HR analytics and organizational performance; and (2) understand the process for how HR analytics can influence organizational performance. Drawing upon EBM ( Rousseau, 2006 ; Rousseau and Barends, 2011 ; Barends et al ., 2014 ), dynamic capabilities ( Teece et al ., 1997 ) and the RBV of the firm ( Barney, 1991 ), this study proposed a chain model where access to HR technology enables HR analytics which facilitates EBM, ultimately enhancing or improving organizational performance. Using a sample of 155 organizations based in Ireland, the structural equation modeling results provided full support for the theoretical chain model. Therefore, the study finds that HR technology enables HR analytics and acts as an antecedent to HR analytics, with HR analytics facilitating organization EBM, leading to higher organizational performance.
Theoretical contributions
The findings of this study make several contributions to the fields of HR analytics and EBM. First, this study offers a very timely investigation of whether HR analytics impacts organizational performance. Due to the growing interest in HR analytics, organizations have begun to buy into HR analytics, assembling HR analytics teams dedicated to using workforce data to make strategic workforce decisions ( Rasmussen and Ulrich, 2015 ; Andersen, 2017 ; McIver et al ., 2018 ). However, very little empirical evidence supports the impact HR analytics has on organizational performance ( Rasmussen and Ulrich, 2015 ; Marler and Boudreau, 2017 ; van der Togt and Rasmussen, 2017 ; McIver et al ., 2018 ). According to McIver et al. (2018) , despite the great enthusiasm for adopting HR analytics in practice, there remains a misunderstanding of how organizations can leverage and use HR analytics to increase organizational performance. Furthermore, King (2016) argues that although the practice of conducting HR analytics has risen in popularity, organizations should only begin to invest in HR analytics programs if they can demonstrate value and increase organizational performance. This research has responded to the above calls by seeking support for the positive effect of HR analytics on organizational performance and offers evidence of the performance impact of HR analytics.
Second, this study promotes current HR analytics research by providing evidence suggesting a relationship between HR technology and HR analytics. In recent years, scholars have theorized that HR technology is critical in enabling the HR analytics process. For example, Marler and Boudreau (2017) and McIver et al. (2018) have suggested that HR analytics are enabled by HR technology as it allows for the collection, manipulation, and reporting of structured and unstructured workforce data. Furthermore, several scholars have also begun to suggest that HR analytics are enabled by HR technology as they allow HR professionals to perform complex statistical analysis, leading to the development of predictive analytics and sophisticated people models ( Levenson, 2005 ; Ulrich and Dulebohn, 2015 ; Sharma and Sharma, 2017 ; van der Togt and Rasmussen, 2017 ). Despite these claims, evidence supporting the enabling role of HR technology in HR analytics has yet to be discussed in the extant HR analytics literature. Therefore, this paper supports these claims, indicating a link between HR technology and HR analytics, where HR technology is a critical component and antecedent to HR analytics.
Third, this study contributes to HR analytics research by exploring the process (i.e. the mediating role of EBM) through which HR analytics influences organizational performance. As reviewed earlier, the research examining the performance impact of HR analytics is scarce within the extant literature. Likewise, evidence illustrating the process of how HR analytics can influence organizational performance is non-existent, making the analysis of intervening variables essential both theoretically and empirically. We acknowledge that this is only the first step in identifying the underlying linkage between HR analytics and organizational performance; however, this study undoubtedly contributes to this endeavor.
Lastly, this study contributes toward EBM research significantly by identifying an antecedent of EBM (i.e. HR analytics), as well as offering evidence supporting the performance impact of EBM. To date, EBM research has seen increasing attention in both research and practice. However, there has been limited attention paid to directly address EBM's performance impact within the field of management, which is “of the utmost importance” ( Reay et al ., 2009 , p. 13). Moreover, the organizational level factors which drive EBM remain unknown. Thus, this paper contributes to EBM research by offering a critical organizational factor (HR analytics) that facilitates EBM within organizations.
Implications for practice
The findings offer several implications for practitioners. First, this study offers evidence for the positive impact of HR analytics on organizational performance, suggesting that investing in HR analytics and employing EBM practices can increase organizational performance. Second, the study provides supporting evidence for the critical role that access to HR technology plays in enhancing the impact of HR analytics on EBM. In other words, this study proposes that access to HR technology significantly impacts HR analytics and, in turn, EBM. Thus, HR analysts and managers must have the necessary tools to effectively transform and translate high-quality workforce data into organizational insights.
In addition, this study is significant for organizations looking to improve their current HR technology capabilities or are starting to implement or expand their current HR analytics activities. We find that HR technology offers HR managers and business partners the ability to run reports, create dashboards, visualizations, monitor KPIs and perform predictive analytics. Thus, providing several sources of additional information enabling evidence-based decision-making.
Lastly, this study suggests that establishing and cultivating a culture focused on decision-making has significant advantages for improving organizational performance. Likewise, we find support for HR analytics' important role in facilitating EBM. For instance, HR analytics offers information through mediums such as dashboards, scorecards and predictive analytics. According to Rousseau and Barends (2011) , these sources of organizational knowledge create a link between HR analytics and EBM, allowing HR managers and business partners to make more informed decisions about their workforce. Thus, we conclude that organizations should employ the organizational facts generated by HR analytics and incorporate them into their decision-making process.
Limitations and future research
Despite the significant implications for theory and practice in HR analytics, several limitations are evident in this study. First, this study adopted a cross-sectional design, which does not allow us to test for the causality between the studied variables. Second, the small sample size, response rate and context where it was conducted are limitations of the study. Future research is encouraged to collect longitudinal data among multi-industry, multi-sector and multi-country data sets. Doing so would allow for testing causality between the key variables and aid in the generalizability of the findings. Moreover, it would offer greater consideration to potential economic changes that may be impacting specific industries. Likewise, future studies may use more objective performance data such as return on investment (ROI), return on assets (ROA) or revenue growth rather than subjective measures to operationalize organizational performance similar to existing studies ( Crook et al. , 2011 ; Singh et al ., 2016 ; Omran et al. , 2021 ). The findings on the significant correlation coefficient between HR technology and HR analytics ( r = 0.66, p < 0.01) as well as the path coefficient of HR technology on HR analytics ( β = 0.71, p < 0.001) is another limitation of the study and raises concerns for the validity of the measurements. Accordingly, future research should adapt cross-disciplinary measures from the big data, marketing or information technology literature to better test this relationship. Lastly, the research study may have an issue with endogeneity. For example, according to Hill et al . (2021) and Semykina and Wooldridge (2010) , there are four causes of endogeneity, i.e. omitted variables, simultaneity, measurement error and selection. This study has addressed some sources, i.e. the omitted variables, by including control variables; and the measurement error issue at both the design (e.g. using valid scales) and analysis (e.g. addressing the CMV and using SEM) stages. However, future research should pay more attention to endogeneity in management research and adopt the recommendations and solutions proposed by previous studies (e.g. Larcker and Rusticus, 2010 ; Semykina and Wooldridge, 2010 ; Ketokivi and McIntosh, 2017 ; Hill et al. , 2021 ).
In addition, future research is warranted to further investigate the connection between HR technology and HR analytics. For instance, should HR technology be incorporated as a fourth component of HR analytics? Or does it only act as an enabler in the HR analytics process? Furthermore, as illustrated, organizations utilize various levels of HR technology in order to perform HR analytics. At the most basic level, HR departments rely on Excel and HRIS such as Workday, SuccessFactors, or BambooHR for reporting, generating HR metrics and dashboarding. In contrast, analytically mature HR departments will also utilize these platforms but will integrate them with more advanced forms of HR technology (e.g. business intelligence (BI) tools, AI-enabled platforms, open-source statistical packages) to enable predictive and prescriptive data analytics. This raises the question of whether the use of more advanced HR technology leads to more insightful HR analytics. And if so, how significant are these insights compared to those derived from basic level technology? Equally important is the notion that organizations currently engaging with HR analytics often rely on HR analytics teams to conduct the analytics (i.e. transforming and translating high-quality workforce data into organizational insights), rather than an individual employee ( Andersen, 2017 ; McIver et al ., 2018 ; McCartney et al ., 2020 ; Peeters et al ., 2020 ). However, very little attention has been paid to exploring the composition of HR analytics teams or their impact on HR practices and organizational performance ( McCartney et al ., 2020 ). For instance, given the complex range of skills required to transform and translate high-quality workforce data into organizational insights effectively, HR analytics teams require members to have various complimentary knowledge, skills, abilities and other characteristics (KSAOs) ( Andersen, 2017 ; McIver et al ., 2018 ; McCartney et al ., 2020 ). As such, it is essential that research examining the complimentary KSAOs and synergies among specific team members that enable the emergence of highly effective HR analytics teams be explored. Likewise, a significant way to move HR analytics research forward would be to explore how HR analytics teams can help develop or enhance HR practices and their effect on organizational performance.
While HR analytics is gaining increasing interest as a field of study, HR analytics is still a relatively new concept. As a result, scholars and practitioners are poised to conduct research highlighting how HR's digitalization and the growing amount of people data can impact HR decision-making and organizational outcomes. The present study sheds light on HR analytics research by identifying the impact of HR analytics on organizational performance. By doing so, we hope to see more research aiming to better understand how HR analytics adds value to organizations in the future.
Theoretical model
SEM results
Descriptive statistics and correlations of study variables
Note(s): N = 153, *** p < 0.001; χ 2 = chi-square discrepancy, df = degrees of freedom; CFI=comparative fit index; TLI = Tucker–Lewis Index; RMSEA = root mean square error of approximation; SRMR= standardized root mean square residual; = difference in chi-square, = difference in degrees of freedom. In all measurement models, error terms were free to covary to improve fit and help reduce bias in the estimated parameter values. All models are compared to the full measurement model
a = HR analytics and evidence-based management combined into a single factor
b = HR analytics and technology combined into a single factor
c = HR analytics, evidence-based management and technology combined into one factor
d = Evidence-based management and organizational performance combined into a single factor
e = HR analytics and organizational performance combined into a single factor
f = HR analytics, evidence-based management and organizational performance combined into a single factor
g = All factors combined into a single factor
The authors would like to thank one of the reviewers for this point.
Thanks are given to one of the reviewers who raised this point.
Aloini , D. , Cannavacciuolo , L. , Gitto , S. , Lettieri , E. , Malighetti , P. and Visintin , F. ( 2018 ), “ Evidence-based management for performance improvement in HealthCare ”, Management Decision , Vol. 56 No. 10 , pp. 2063 - 2068 , doi: 10.1108/MD-10-2018-004 .
Andersen , M.K. ( 2017 ), “ Human capital analytics: the winding road ”, Journal of Organizational Effectiveness , pp. 133 - 136 , doi: 10.1108/JOEPP-03-2017-0024 .
Angrave , D. , Charlwood , A. , Kirkpatrick , I. , Lawrence , M. and Stuart , M. ( 2016 ), “ HR and analytics: why HR is set to fail the big data challenge ”, Human Resource Management Journal , Vol. 26 No. 1 , pp. 1 - 11 , doi: 10.1111/1748-8583.12090 .
Aral , S. , Brynjolfsson , E. and Wu , L. ( 2012 ), “ Three-way complementarities: performance pay, human resource analytics, and information technology ”, Management Science , Vol. 58 No. 5 , pp. 913 - 931 , doi: 10.1287/mnsc.1110.1460 .
Armstrong , J.S. and Overton , T.S. ( 1977 ), “ Estimating nonresponse bias in mail surveys ”, Journal of Marketing Research , Vol. 14 No. 3 , p. 396 , doi: 10.2307/3150783 .
Ashbaugh , S. and Miranda , R. ( 2002 ), “ Technology for human resources management: seven questions and answers ”, Public Personnel Management , pp. 7 - 20 , doi: 10.1177/009102600203100102 .
Baba , V.V. and HakemZadeh , F. ( 2012 ), “ Toward a theory of evidence based decision making ”, Management Decision . doi: 10.1108/00251741211227546 .
Baesens , B. , De Winne , S. and Sels , L. ( 2017 ), “ Is your company ready for HR analytics? ”, MIT Sloan Management Review , Vol. 58 No. 2 , pp. 20 - 21 , doi: 10.1016/j.jsmc.2005.11.006 .
Barends , E. , Rousseau , D.M. and Briner , R.B. ( 2014 ), Evidence-Based Management: The Basic Principals , The Center for Evidence-Based Management , Amsterdam .
Barney , J.B. ( 1991 ), “ The resource based view of strategy: origins, implications, and prospects ”, Journal of Management , Vol. 17 No. 1 , pp. 97 - 211 .
Baron , R.M. and Kenny , D.A. ( 1986 ), “ The moderator–mediator variable distinction in social psychological research: conceptual, strategic, and statistical considerations ”, Journal of Personality and Social Psychology , Vol. 51 , pp. 1173 - 1182 .
Baruch , Y. and Holtom , B.C. ( 2008 ), “ Survey response rate levels and trends in organizational research ”, Human Relations , Vol. 61 No. 8 , pp. 1139 - 1160 , doi: 10.1177/0018726708094863 .
Bezzina , F. , Cassar , V. , Tracz-Krupa , K. , Przytuła , S. and Tipurić , D. ( 2017 ), “ Evidence-based human resource management practices in three EU developing member states: can managers tell truth from fallacy? ”, European Management Journal , Vol. 35 No. 5 , pp. 688 - 700 , doi: 10.1016/j.emj.2017.02.010 .
Black , J.S. and van Esch , P. ( 2020 ), “ AI-enabled recruiting: what is it and how should a manager use it? ”, Business Horizons , Vol. 63 No. 2 , pp. 215 - 226 , doi: 10.1016/j.bushor.2019.12.001 .
Bondarouk , T. and Brewster , C. ( 2016 ), “ Conceptualising the future of HRM and technology research ”, International Journal of Human Resource Management , Routledge , Vol. 27 No. 21 , pp. 2652 - 2671 , doi: 10.1080/09585192.2016.1232296 .
Boudreau , J. and Cascio , W. ( 2017 ), “ Human capital analytics: why are we not there? ”, Journal of Organizational Effectiveness: People and Performance , Vol. 4 No. 2 , pp. 119 - 126 , doi: 10.1108/JOEPP-03-2016-0029 .
Briggs , H.E. and McBeath , B. ( 2009 ), “ Evidence-based management: origins, challenges, and implications for social service administration ”, Administration in Social Work , Vol. 33 No. 3 , pp. 242 - 261 , doi: 10.1080/03643100902987556 .
Briner , R.B. , Denyer , D. and Rousseau , D.M. ( 2009 ), “ Evidence-based management: concept cleanup time? ”, Academy of Management Perspectives , Vol. 23 No. 4 , pp. 19 - 32 .
Buck , B. and Morrow , J. ( 2018 ), “ AI, performance management and engagement: keeping your best their best ”, Strategic HR Review , Vol. 17 No. 5 , pp. 261 - 262 , doi: 10.1108/shr-10-2018-145 .
Buttner , H. and Tullar , W. ( 2018 ), “ A representative organizational diversity metric: a dashboard measure for executive action ”, Equality, Diversity and Inclusion: An International Journal , Vol. 37 No. 3 , pp. 219 - 232 , doi: 10.1108/GM-12-2013-0140 .
Cassar , V. and Bezzina , F. ( 2017 ), “ Evidence-based HRM through analytics: reducing the propensity of HRM to become a Cinderella ”, Management Sciences , Vol. 4 No. 33 , pp. 3 - 10 .
Chierici , R. , Mazzucchelli , A. , Garcia-Perez , A. and Vrontis , D. ( 2019 ), “ Transforming big data into knowledge: the role of knowledge management practice ”, Management Decision , Vol. 57 No. 8 , pp. 1902 - 1922 , doi: 10.1108/MD-07-2018-0834 .
Chuang , C.H. and Liao , H. ( 2010 ), “ Strategic human resource management in service context: taking care of business by taking care of employees and customers ”, Personnel Psychology , Vol. 63 No. 1 , pp. 153 - 196 , doi: 10.1111/j.1744-6570.2009.01165.x .
Coron , C. ( 2021 ), “ Quantifying human resource management: a literature review ”, Personnel Review , ahead-of-print (ahead-of-print) , doi: 10.1108/pr-05-2020-0322 .
Crook , T.R. , Todd , S. , Combs , J. , Woehr , D. and Ketchen , D. ( 2011 ), “ Does human capital matter? A meta-analysis of the relationship between human capital and firm performance ”, Journal of Applied Psychology , Vol. 96 No. 3 , pp. 443 - 456 , doi: 10.1037/a0022147 .
Delaney , J.T. and Huselid , M.A. ( 1996 ), “ The impact of human resource management practices on perceptions of organizational performance ”, Academy of Management Journal , Vol. 39 No. 4 , pp. 949 - 969 .
Dubey , R. , Gunasekaran , A. , Childe , S. , Papadopoulos , T. , Luo , Z. , Wamba , S.F. and Roubaud , D. ( 2019 ), “ Can big data and predictive analytics improve social and environmental sustainability? ”, Technological Forecasting and Social Change , Vol. 144 , pp. 534 - 545 , doi: 10.1016/j.techfore.2017.06.020 .
Dulebohn , J.H. and Johnson , R.D. ( 2013 ), “ Human resource metrics and decision support: a classification framework ”, Human Resource Management Review , Elsevier , Vol. 23 No. 1 , pp. 71 - 83 , doi: 10.1016/j.hrmr.2012.06.005 .
Falletta , S.V. and Combs , W.L. ( 2020 ), “ The HR analytics cycle: a seven-step process for building evidence-based and ethical HR analytics capabilities ”, Journal of Work-Applied Management , ahead-of-print (ahead-of-print) , doi: 10.1108/JWAM-03-2020-0020 .
Fernandez , V. and Gallardo-Gallardo , E. ( 2020 ), “ Tackling the HR digitalization challenge: key factors and barriers to HR analytics adoption ”, Competitiveness Review: An International Business Journal , ahead-of-print (ahead-of-print) , doi: 10.1108/CR-12-2019-0163 .
Ferraris , A. , Mazzoleni , A. , Devalle , A. and Couturier , J. ( 2019 ), “ Big data analytics capabilities and knowledge management: impact on firm performance ”, Management Decision , Vol. 57 No. 8 , pp. 1923 - 1936 , doi: 10.1108/MD-07-2018-0825 .
Fu , N. , Flood , P.C. , Bosak , J. , Rousseau , D. , Morris , T. and O’Regan , P. ( 2017 ), “ High performance work systems in professional service firms: examining the practice-resources-uses performance linkage ”, Human Resource Management , Vol. 56 No. 2 , pp. 329 - 352 , doi: 10.1002/hrm .
Fu , N. , Flood , P.C. , Rousseau , D. and Morris , T. ( 2018 ), “ Line managers as paradox navigators in HRM implementation: balancing consistency and individual responsiveness ”, Journal of Management , Vol. 46 No. 2 , pp. 203 - 233 , doi: 10.1177/0149206318785241 .
Garcia-Arroyo , J. and Osca , A. ( 2019 ), “ Big data contributions to human resource management: a systematic review ”, International Journal of Human Resource Management , p. 5192 , doi: 10.1080/09585192.2019.1674357 .
Gelbard , R. et al. ( 2018 ) “ Sentiment analysis in organizational work : towards an ontology of people analytics ”, (July 2017) , pp. 1 - 15 , doi: 10.1111/exsy.12289 .
Greasley , K. and Thomas , P. ( 2020 ) “ HR analytics : the onto-epistemology and politics of metricised HRM ”, (December 2019) , pp. 1 - 14 , doi: 10.1111/1748-8583.12283 .
Green , D. ( 2017 ), “ The best practices to excel at people analytics ”, Journal of Organizational Effectiveness: People and Performance , Vol. 4 No. 2 , pp. 137 - 144 , doi: 10.1108/JOEPP-03-2017-0027 .
Grundtvig , M. , Gullestad , L. , Hole , T. , Flønæs , B. and Westheim , A. ( 2011 ), “ Characteristics, implementation of evidence-based management and outcome in patients with chronic heart failure. Results from the Norwegian heart failure registry ”, European Journal of Cardiovascular Nursing , Vol. 10 No. 1 , pp. 44 - 49 , doi: 10.1016/j.ejcnurse.2010.04.001 .
Gupta , A.K. ( 1987 ), “ SBU strategies , corporate-SBU relations , and SBU effectiveness in strategy implementation ”, Academy of Management Journal , Vol. 30 No. 3 , pp. 477 - 500 .
Gupta , A.K. and Govindarajan , V. ( 1984 ), “ Business unit strategy, managerial characteristics, and business unit effectiveness at strategy implementation ”, Academy of Management Journal , Vol. 27 No. 1 , pp. 25 - 41 , doi: 10.5465/255955 .
Gupta , A.K. and Govindarajan , V. ( 1986 ), “ Resource sharing among SBUs : strategic antecedents and administrative implications ”, The Academy of Management Journal , Vol. 29 No. 4 , pp. 695 - 714 .
Guthrie , J.P. ( 2001 ), “ High involvement work practices, turnover and productivity: evidence from New Zealand ”, Academy of Management Journal , Vol. 44 , pp. 180 - 190 .
Guthrie , J.P. , Flood , P.C. , Liu , W. and MacCurtain , S. ( 2009 ), “ High performance work systems in Ireland: human resource and organizational outcomes ”, International Journal of Human Resource Management , Vol. 20 No. 1 , pp. 112 - 125 , doi: 10.1080/09585190802528433 .
HakemZadeh , F. and Baba , V.V. ( 2016 ), “ Toward a theory of collaboration for evidence-based management ”, Management Decision , Vol. 54 No. 10 , pp. 2587 - 2616 , doi: 10.1108/MD-06-2015-0243 .
Hamilton , R.H. and Sodeman , W.A. ( 2020 ), “ The questions we ask: opportunities and challenges for using big data analytics to strategically manage human capital resources ”, Business Horizons , Vol. 63 No. 1 , pp. 85 - 95 , doi: 10.1016/j.bushor.2019.10.001 .
Harris , J.G. , Craig , E. and Light , D.A. ( 2011 ), “ Talent and analytics: new approaches, higher ROI ”, Journal of Business Strategy , Vol. 32 No. 6 , pp. 4 - 13 , doi: 10.1108/02756661111180087 .
Hayes , A. ( 2013 ), Introduction to Mediation, Moderation, and Conditional Process Analysis , The Guilford Press , New York .
Hayes , A.F. and Preacher , K.J. ( 2014 ), “ Statistical mediation analysis with a multicategorical independent variable ”, British Journal of Mathematical and Statistical Psychology , Vol. 67 No. 3 , pp. 451 - 470 , doi: 10.1111/bmsp.12028 .
Hendrickson , A.R. ( 2003 ), “ Human resource information systems: backbone technology of contemporary human resources ”, Journal of Labor Research , Vol. 24 No. 3 , pp. 381 - 394 , doi: 10.1007/s12122-003-1002-5 .
Hill , A. , Johnson , S , Greco , L. , O’Boyle , E. and Walter , S. ( 2021 ), “ Endogeneity: a review and agenda for the methodology-practice divide affecting micro and macro research ”, Journal of Management , doi: 10.1177/0149206320960533 .
Huselid , M.A. ( 2018 ), “ The science and practice of workforce analytics: introduction to the HRM special issue ”, Human Resource Management , Vol. 57 No. 3 , pp. 679 - 684 , doi: 10.1002/hrm.21916 .
Janati , A. , Hasanpoor , E. , Hajebrahimi , S. and Sadeghi-Bazargani , H. ( 2018 ), “ Evidence-based management – healthcare manager viewpoints ”, International Journal of Health Care Quality Assurance , Vol. 31 No. 5 , pp. 436 - 448 , doi: 10.1108/IJHCQA-08-2017-0143 .
Jeske , D. and Calvard , T. ( 2020 ), “ Big data: lessons for employers and employees ”, Employee Relations , Vol. 42 No. 1 , pp. 248 - 261 , doi: 10.1108/ER-06-2018-0159 .
Johnson , R.D. , Lukaszewski , K.M. and Stone , D.L. ( 2016 ), “ The evolution of the field of human resource information systems: Co-Evolution of technology and HR processes ”, Communications of the Association for Information Systems , Vol. 38 No. 1 , pp. 533 - 553 , doi: 10.17705/1CAIS.03828 .
Kane , G. ( 2015 ), “ People analytics through super- charged ID ”, MIT Sloan Management Review , Vol. 56 No. 4 , available at: https://sloanreview.mit.edu/article/people-analytics-through-super-charged-id-badges/ .
Kapoor , B. and Sherif , J. ( 2012 ), “ Human resources in an enriched environment of business intelligence ”, Kybernetes , Vol. 44 No. 10 , pp. 1625 - 1637 , doi: 10.1108/K-01-2015-0009 .
Ketokivi , M. and McIntosh , C.N. ( 2017 ), “ Addressing the endogeneity dilemma in operations management research: theoretical, empirical, and pragmatic considerations ”, Journal of Operations Management , Vol. 52 , pp. 1 - 14 , doi: 10.1016/j.jom.2017.05.001 .
Kim , S. , Wang , Y. and Boon , C. ( 2021 ), “ Sixty years of research on technology and human resource management: looking back and looking forward ”, Human Resource Management , Vol. 60 No. 1 , pp. 229 - 247 , doi: 10.1002/hrm.22049 .
King , K.G. ( 2016 ), “ Data analytics in human resources: a case study and critical review ”, Human Resource Development Review , Vol. 15 No. 4 , pp. 487 - 495 , doi: 10.1177/1534484316675818 .
Kryscynski , D. , Reeves , C. , Stice-Lusvardi , R. , Ulrich , M. and Russell , G. ( 2018 ), “ Analytical abilities and the performance of HR professionals ”, Human Resource Management , Vol. 57 No. 3 , pp. 715 - 738 , doi: 10.1002/hrm.21854 .
Larcker , D.F. and Rusticus , T.O. ( 2010 ), “ On the use of instrumental variables in accounting research ”, Journal of Accounting and Economics , Vol. 49 No. 3 , pp. 186 - 205 , doi: 10.1016/j.jacceco.2009.11.004 .
Lengnick-Hall , M. and Mortiz , S. ( 2003 ), “ The impact of e-HR on the human resource management function ”, Journal of Labor Research , Vol. 24 No. 3 , pp. 365 - 379 .
Levenson , A. ( 2005 ), “ Harnessing the power of HR analytics ”, Strategic HR Review , Vol. 4 No. 3 , pp. 28 - 31 , doi: 10.1108/14754390580000607 .
Levenson , A. ( 2018 ), “ Using workforce analytics to improve strategy execution ”, Human Resource Management , Vol. 57 No. 3 , pp. 685 - 700 , doi: 10.1002/hrm.21850 .
Levenson , A. and Fink , A. ( 2017 ), “ Human capital analytics: too much data and analysis, not enough models and business insights ”, Journal of Organizational Effectiveness , Vol. 4 No. 2 , pp. 145 - 156 , doi: 10.1108/JOEPP-03-2017-0029 .
Lin , Y. and Wu , L.Y. ( 2014 ), “ Exploring the role of dynamic capabilities in firm performance under the resource-based view framework ”, Journal of Business Research , Vol. 67 No. 3 , pp. 407 - 413 , doi: 10.1016/j.jbusres.2012.12.019 .
Maamari , B.E. and Osta , A. ( 2021 ), “ The effect of HRIS implementation success on job involvement, job satisfaction and work engagement in SMEs ”, International Journal of Organizational Analysis . doi: 10.1108/IJOA-07-2020-2298 .
Margherita , A. ( 2020 ), “ Human resources analytics: a systematization of research topics and directions for future research ”, Human Resource Management Review , Elsevier , No. November , p. 100795 , doi: 10.1016/j.hrmr.2020.100795 .
Marler , J.H. and Boudreau , J.W. ( 2017 ), “ An evidence-based review of HR analytics ”, International Journal of Human Resource Management , Routledge , Vol. 28 No. 1 , pp. 3 - 26 , doi: 10.1080/09585192.2016.1244699 .
McCartney , S. , Murphy , C. and McCarthy , J. ( 2020 ), “ 21st century HR: a competency model for the emerging role of HR Analysts ”, Personnel Review , Vol. 50 No. 6 , pp. 1495 - 1513 .
McIver , D. , Lengnick-Hall , M.L. and Lengnick-Hall , C.A. ( 2018 ), “ A strategic approach to workforce analytics: integrating science and agility ”, Business Horizons , Vol. 61 No. 3 , pp. 397 - 407 , doi: 10.1016/j.bushor.2018.01.005 .
Melnyk , B.M. , Gallagher-Ford , L. , Long , L.E. and Fineout-Overholt , E. ( 2014 ), “ The establishment of evidence-based practice competencies for practicing registered nurses and advanced practice nurses in real-world clinical settings: proficiencies to improve healthcare quality, reliability, patient outcomes, and costs ”, Worldviews on Evidence-Based Nursing , Vol. 11 No. 1 , pp. 5 - 15 , doi: 10.1111/wvn.12021 .
Mikalef , P. , Boura , M. , Lekakos , G. and Krogstie , J. ( 2019 ), “ Big data analytics and firm performance: findings from a mixed-method approach ”, Journal of Business Research , Elsevier , Vol. 98 No. February , pp. 261 - 276 , doi: 10.1016/j.jbusres.2019.01.044 .
Minbaeva , D. ( 2018 ), “ Building credible human capital analytics for organizational competitive advantage ”, Human Resource Management , Vol. 57 No. 3 , pp. 701 - 713 , doi: 10.1002/hrm.21848 .
Morrell , K. and Learmonth , M. ( 2015 ), “ Against evidence-based management, for management learning ”, Academy of Management Learning and Education , Vol. 14 No. 4 , pp. 520 - 533 , doi: 10.5465/amle.2014.0346 .
Nielsen , C. and McCullough , N. ( 2018 ), “ How people analytics can help you change process, culture, and strategy ”, Harvard Business Review , No. May , pp. 2 - 6 .
Omran , M. , Khallaf , A. , Gleason , K. and Tahat , Y. ( 2021 ), “ Non-financial performance measures disclosure, quality strategy, and organizational financial performance: a mediating model ”, Total Quality Management and Business Excellence , Vol. 32 Nos 5-6 , pp. 652 - 675 , doi: 10.1080/14783363.2019.1625708 .
Peeters , T. , Paauwe , J. and Van De Voorde , K. ( 2020 ), “ People analytics effectiveness: developing a framework ”, Journal of Organizational Effectiveness , Vol. 7 No. 2 , pp. 203 - 219 , doi: 10.1108/JOEPP-04-2020-0071 .
Pfeffer , J. and Sutton , R.I. ( 2006 ), “ Evidence-based management ”, Harvard Business Review , pp. 63 - 74 .
Pipino , L.L. , Lee , Y.W. and Wang , R.Y. ( 2002 ), “ Data quality assessment ”, Communications of the ACM , Vol. 45 No. 4 , pp. 211 - 218 , doi: 10.1145/505248.506010 .
Podsakoff , P.M. , MacKenzie , S. , Lee , J. and Podsakoff , N.P. ( 2003 ), “ Common method biases in behavioral research: a critical review of the literature and recommended remedies ”, Journal of Applied Psychology , Vol. 88 No. 5 , pp. 879 - 903 , doi: 10.1037/0021-9010.88.5.879 .
Podsakoff , P.M. , MacKenzie , S.B. and Podsakoff , N.P. ( 2012 ), “ Sources of method bias in social science research and recommendations on how to control it ”, Annual Review of Psychology , Vol. 63 No. 1 , pp. 539 - 569 , doi: 10.1146/annurev-psych-120710-100452 .
Rasmussen , T. and Ulrich , D. ( 2015 ), “ Learning from practice: how HR analytics avoids being a management fad ”, Organizational Dynamics , Elsevier , Vol. 44 No. 3 , pp. 236 - 242 , doi: 10.1016/j.orgdyn.2015.05.008 .
Reay , T. , Berta , W. and Kohn , M.K. ( 2009 ), “ What's the evidence on evidence-based management? ”, Academy of Management Perspectives , Vol. 23 No. 4 , pp. 5 - 18 .
Roshanghalb , A. , Lettieri , E. , Aloini , D. , Cannavacciuolo , L. , Gitto , S. and Visintin , F. ( 2018 ), “ What evidence on evidence-based management in healthcare? ”, Management Decision , Vol. 56 No. 10 , pp. 2069 - 2084 , doi: 10.1108/MD-10-2017-1022 .
Rousseau , D.M. ( 2006 ), “ Is there such a thing as ‘evidence-based management’? ”, Academy of Management Review , Vol. 31 No. 2 , pp. 256 - 269 , doi: 10.5465/AMR.2006.22528177 .
Rousseau , D.M. and Barends , E.G.R. ( 2011 ), “ Becoming an evidence-based HR practitioner ”, Human Resource Management Journal , Vol. 21 No. 3 , pp. 221 - 235 , doi: 10.1111/j.1748-8583.2011.00173.x .
Rynes , S.L. and Bartunek , J.M. ( 2017 ), “ Evidence-based management: foundations, development, controversies and future ”, The Annual Review of Organizational Psychology and Organizational Behaviour , Vol. 4 , pp. 235 - 261 .
Santoro , G. , Fiano , F. , Bertoldi , B. and Ciampi , F. ( 2019 ), “ Big data for business management in the retail industry ”, Management Decision , Vol. 57 No. 8 , pp. 1980 - 1992 , doi: 10.1108/MD-07-2018-0829 .
Schiemann , W.A. , Seibert , J.H. and Blankenship , M.H. ( 2018 ), “ Putting human capital analytics to work: predicting and driving business success ”, Human Resource Management , Vol. 57 No. 3 , pp. 795 - 807 , doi: 10.1002/hrm.21843 .
Semykina , A. and Wooldridge , J.M. ( 2010 ), “ Estimating panel data models in the presence of endogeneity and selection ”, Journal of Econometrics , Vol. 157 No. 2 , pp. 375 - 380 , doi: 10.1016/j.jeconom.2010.03.039 .
Sharma , A. and Sharma , T. ( 2017 ), “ HR analytics and performance appraisal system: a conceptual framework for employee performance improvement ”, Management Research Review , Vol. 40 No. 6 , pp. 684 - 697 , doi: 10.1108/MRR-04-2016-0084 .
Shrivastava , S. , Nagdev , K. and Rajesh , A. ( 2018 ), “ Redefining HR using people analytics : the case of Google ”, Human Resource Management International Digest , Vol. 26 No. 2 , pp. 2 - 6 , doi: 10.1108/HRMID-06-2017-0112 .
Simón , C. and Ferreiro , E. ( 2018 ), “ Workforce analytics: a case study of scholar–practitioner collaboration ”, Human Resource Management , Vol. 57 No. 3 , pp. 781 - 793 , doi: 10.1002/hrm.21853 .
Singh , S.K. and Del Giudice , M. ( 2019 ), “ Big data analytics, dynamic capabilities and firm performance ”, Management Decision , Vol. 57 No. 8 , pp. 1729 - 1733 , doi: 10.1108/MD-08-2019-020 .
Singh , S. , Darwish , T.K. and Potočnik , K. ( 2016 ), “ Measuring organizational performance: a case for subjective measures ”, British Journal of Management , Vol. 27 No. 1 , pp. 214 - 224 , doi: 10.1111/1467-8551.12126 .
Sirmon , D.G. , Hitt , M.A. and Ireland , R.D. ( 2007 ), “ Managing firm resources in dynamic environments to create value: looking inside the Black box ”, Academy of Management Review , Vol. 32 No. 1 , pp. 273 - 292 .
Sivathanu , B. and Pillai , R. ( 2020 ) “ Technology and talent analytics for talent management – a game changer for organizational performance ”, Vol. 28 No. 2 , pp. 457 - 473 , doi: 10.1108/IJOA-01-2019-1634 .
Stone , D.L. , Deadrick , D.L. , Lukaszewski , K.M. and Johnson , R. ( 2015 ), “ The influence of technology on the future of human resource management ”, Human Resource Management Review , Vol. 25 No. 2 , pp. 216 - 231 , doi: 10.1016/j.hrmr.2015.01.002 .
Sun , L.-Y. , Aryee , S. and Law , K.S. ( 2007 ), “ High performance human resource practices, citizenship behaviour, and organizational performance: a relational perspective ”, Academy of Management Journal , Vol. 50 No. 3 , pp. 558 - 577 , doi: 10.5465/amj.2007.25525821 .
Takeuchi , R. , Lepak , D.P. , Wang , H. and Takeuchi , K. ( 2007 ), “ An empirical examination of the mechanisms mediating between high-performance work systems and the performance of Japanese organizations ”, Journal of Applied Psychology , Vol. 92 No. 4 , pp. 1069 - 1083 , doi: 10.1037/0021-9010.92.4.1069 .
Teece , D.J. , Pisano , G. and Shuen , A. ( 1997 ), “ Dynamic capabilities and strategic management ”, Strategic Management Journal , Vol. 18 No. 7 , pp. 509 - 533 .
Tomaskovic-Devey , D. , Leiter , J. and Thompson , S. ( 1994 ), “ Organizational survey nonresponse ”, Administration Science Quarterly , Vol. 39 No. 3 , pp. 439 - 457 .
Tursunbayeva , A. , Di Lauro , S. and Pagliari , C. ( 2018 ), “ People analytics—a scoping review of conceptual boundaries and value propositions ”, International Journal of Information Management , Vol. 43 , pp. 224 - 247 , doi: 10.1016/j.ijinfomgt.2018.08.002 .
Tursunbayeva , A. , Pagliari , C. , Di Lauro , S. and Antonelli , G. ( 2021 ), “ The ethics of people analytics: risks, opportunities and recommendations ”, Personnel Review , doi: 10.1108/PR-12-2019-0680 .
Ulrich , D. and Dulebohn , J.H. ( 2015 ), “ Are we there yet? What's next for HR? ”, Human Resource Management Review , Elsevier , Vol. 25 No. 2 , pp. 188 - 204 , doi: 10.1016/j.hrmr.2015.01.004 .
van den Heuvel , S. and Bondarouk , T. ( 2017 ), “ The rise (and fall?) of HR analytics ”, Journal of Organizational Effectiveness: People and Performance , Vol. 4 No. 2 , pp. 157 - 178 , doi: 10.1002/chir .
van der Togt , J. and Rasmussen , T.H. ( 2017 ), “ Toward evidence-based HR ”, Journal of Organizational Effectiveness , Vol. 4 No. 2 , pp. 127 - 132 , doi: 10.1108/JOEPP-02-2017-0013 .
van Esch , P. and Black , J.S. ( 2019 ), “ Factors that influence new generation candidates to engage with and complete digital, AI-enabled recruiting ”, Business Horizons , Vol. 62 No. 6 , pp. 729 - 739 , doi: 10.1016/j.bushor.2019.07.004 .
Wall , T.D. , Michie , J. , Patterson , M. , Wood , S.J. , Sheehan , M. , Clegg , C.W. and West , M. ( 2004 ), “ On the validity of subjective measures of company performance ”, Personnel Psychology , Vol. 57 , pp. 95 - 118 .
Walshe , K. and Rundall , T.G. ( 2001 ), “ Evidence-based management: from theory to practice in health care ”, Milbank Quarterly , Vol. 79 No. 3 , pp. 429 - 457 , doi: 10.1111/1468-0009.00214 .
Wamba , S.F. , Akter , S. , Edwards , A. , Chopin , G. and Gnanzou , D. ( 2015 ), “ How “big data” can make big impact: findings from a systematic review and a longitudinal case study ”, International Journal of Production Economics , Elsevier , Vol. 65 , pp. 234 - 246 .
Wamba , S.F. , Akter , S. , Trinchera , L. and De Bourmont , M. ( 2019 ), “ Turning information quality into firm performance in the big data economy ”, Management Decision , Vol. 57 No. 8 , pp. 1756 - 1783 , doi: 10.1108/MD-04-2018-0394 .
Wamba , S.F. , Gunasekaran , A. , Akter , S. , Ren , S.J. , Dubey , R. and Childe , S.J. ( 2017 ), “ Big data analytics and firm performance: effects of dynamic capabilities ”, Journal of Business Research , Vol. 70 , pp. 356 - 365 , doi: 10.1016/j.jbusres.2016.08.009 .
Wilcox , J.B. , Bellenger , D.N. and Rigdon , E.E. ( 1994 ), “ Assessing sample representativeness in industrial surveys ”, Journal of Business and Industrial Marketing , Vol. 9 No. 2 , pp. 51 - 61 , doi: 10.1108/08858629410059834 .
Winter , S.G. ( 2003 ), “ Understanding dynamic capabilities ”, Strategic Management Journal , Vol. 24 No. 10 SPEC ISS. , pp. 991 - 995 , doi: 10.1002/smj.318 .
Youndt , M.A. , Snell , S.A. , Dean , J.W. and Lepak , D.P. ( 1996 ), “ Human resource management, manufacturing strategy, and firm performance ”, Academy of Management Journal , Vol. 39 No. 4 , pp. 836 - 866 .
Zhou , Y. , Liu , G. , Chang , X. and Wang , L. ( 2021 ), “ The impact of HRM digitalization on firm performance: investigating three-way interactions ”, Asia Pacific Journal of Human Resources , Vol. 59 No. 1 , pp. 20 - 43 , doi: 10.1111/1744-7941.12258 .
Acknowledgements
The authors would like to express their appreciation to Professor Randolph-Seng and the two anonymous reviewers for their valuable feedback and suggestions that significantly improved the paper.
Data availability statement : The data supporting the findings of this study are available at Reserved DOI: 10.17632/hfk7fxt9fm.2.
Corresponding author
Related articles, we’re listening — tell us what you think, something didn’t work….
Report bugs here
All feedback is valuable
Please share your general feedback
Join us on our journey
Platform update page.
Visit emeraldpublishing.com/platformupdate to discover the latest news and updates
Questions & More Information
Answers to the most commonly asked questions here
making HR better, one HR pro at a time
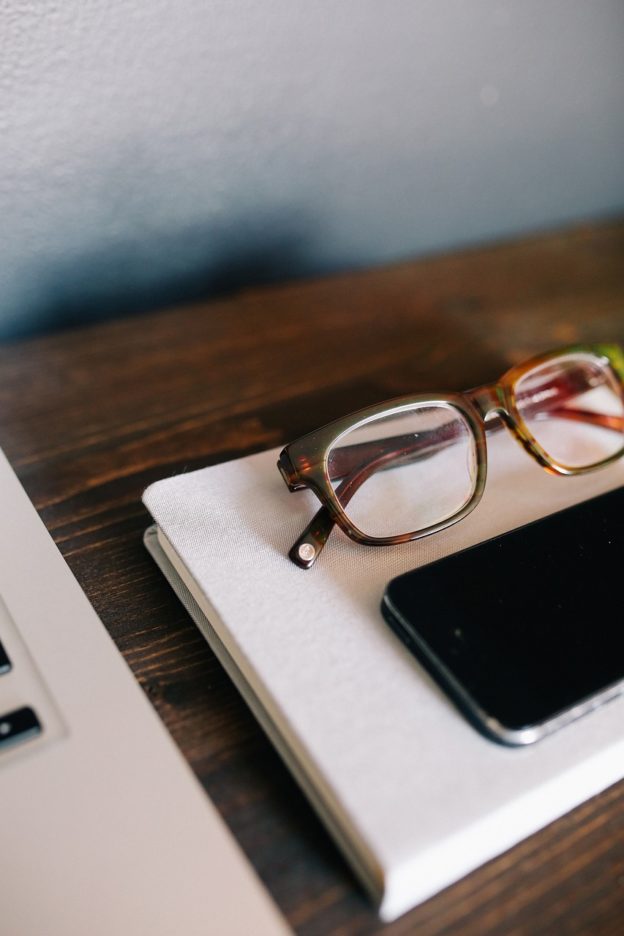
13+ HR Case Studies: Recruiting, Learning, Analytics, and More
Reposting a piece from the blog over at Lighthouse Research because I know not all of you subscribe over there!
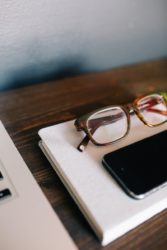
While much of the work we do at Lighthouse Research & Advisory focuses on quantitative research studies, we do a fair amount of qualitative research as well. We’ve collected case studies over time (and continue to) that highlight interesting approaches and examples of innovation within human capital management. The list below offers a wide variety of industries, examples, and flavors for you to learn from.
Want to see another topic or example not listed here? Comment below and and I will see what we can do to find that for you!
Wal-Mart, Automation, and Compassion Training
Walmart’s Fastest Growing Line of Business is Delivering Experiences
The Motley Fool: Blending Talent Management and Engagement
Motley Fool: The Coolest Talent Processes You’ve Never Heard Of
Chipotle: How Internal Mobility Reduced Turnover by 64%
Internal promotion-how Chipotle reduced turnover by 64%
Adtran: Using Hackathons for Employer Branding, Employee Development, and Retention
Using Hackathons for Branding and Retention
Stout Advisory: Performance Management, Peer Feedback, and Employee Engagement
How to Radically Change Your Performance Management Practice [Podcast]
H&R Block: Seasonal Hiring, Strategic Recruiting, and Hiring Manager Communications
Patagonia: measuring the roi of hr programs, hr strategy, employee perks and benefits.
Measuring the ROI of HR Programs is Critical: Here’s How Patagonia Does It
Hot Chicken Takeover: Employee Benefits, Corporate Culture, Leadership, and Social Responsibility
Can a Business Grow Competitively While Doing Social Good? [Podcast]
AlliedUniversal: Talent Acquisition, Employee Referrals, and High-Volume Hiring
How Does AlliedUniversal Hire 90,000 Workers a Year? Referrals and PURPOSE [Podcast]
Duie Pyle: Remote Worker Engagement, Blue Collar Challenges, and Competitive Recruiting
Talent Lessons from the Transportation Industry [Podcast]
Ohio Living: Core Values, Company Culture, and Employee Recognition
We’re Only Human 39: Ohio Living Serves 70,000 Clients Annually with Core Values
Cox Enterprises: HR Analytics, Business Impact, and Strategy
We’re Only Human 53: How to Partner with Your Talent Analytics Team
McDonald’s: Learning Measurement, Business Impact, and ROI
Southwest airlines: corporate culture, employee perks, and employee engagement.
We’re Only Human 40: How Southwest Airlines Lives and Breathes Corporate Culture
HJF: HR Technology Selection and Implementation, HR Leadership, and Modernization
We’re Only Human 55:The HR Leader’s First Year on the Job

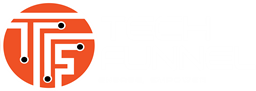
5 HR Analytics Case Studies with Tangible Organizational Benefits

HR analytics are improving business processes for every sector but there are still many organizations that are cautious about the true effectiveness of this data. If you’re looking to use HR analytics to improve your bottom line , retention and turnover rates, employee engagement efforts or just to ensure the long-term success of your business, use these HR analytics case studies to gain a better understanding of just how HR analytics can ensure the success of your organization.
What HR analytics case studies can provide you with tangible organizational benefits?
Use these case studies on HR to gain a better understanding of why HR analytics are essential to the success of your company.
HR Analytics Case Studies
1. employee leave predictions at credit suisse.
In March of 2015, The Wall Street Journal published one of the first articles detailing how employers can effectively predict which potential hires might stay with their company longer, thus, saving the company a lot of money. This now extremely popular HR metric, known as employee churn analytics, not only helps employers determine who might quit the business but also explains why these employees may quit. Since the introduction of employee churn analytics at Credit Suisse, managers at organizations today are better trained to retain high performing employees and reduce turnover risk factors.
2. Employee Turnover at Experian
While there are many case studies on HR , this case study not only improved turnover rates but saved Experian millions of dollars. The credit reporting site, Experian, was having major issues with employee turnover with levels being 4% higher than what they aspired them to be. Experian sought out to build a predictive model that included 200 attributes to predict the flight risk of employees, including team size and structure, supervisor performance, and length of business commute. By discovering what attributes they could change to reduce employee turnover, Experian was able to save an estimated $8,000,000 – $10,000,000.
3. Talent Retention at Nielsen
Back in 2015, Nielsen, a leading global information & measurement company, created a predictive model that was designed to help them retain key talent at the company. This predictive model informed Nielsen that the first year employees work for their company is the most important. Nielsen discovered that when they approached employees at risk of leaving the company with a job change, they were able to keep 40% of flight risk employees.
4. Employee Absence at E.ON
E.ON, a European holding company, was noticing a large number of employee absences and decided to tackle the issue using people analytics. To tackle their employee absenteeism, E.ON had their analytics team formulate 55 hypotheses to solve the issue, tested 21 of them and ended up validating 11 of them. E.ON found that employees who didn’t take a long holiday once a year or take a personal day or two every now and then increased absenteeism and the best way to solve this issue was for managers to improve holiday approval policies.
5. Employee Engagement at Clarks
Clarks, the large shoe retailer, decided to look into the relationship between employee engagement and the financial success of the company and discovered some rather interesting information. First, they discovered that engaged employees leads to higher business performance . They also discovered that there was an optimum team size that should be met in their stores and that the length of tenure of a store manager was a major indicator of employee performance. Thanks to the insights Clarks gained from their investigation, they were able to create a blueprint for high-performing stores and create an engagement toolkit that managers can use to improve employee performance.
While there are many HR analytics case studies available to gain a better understanding of just why HR analytics are essential to the long-term success of your business , it’s important to learn from different case studies on HR to have the best understanding of how to properly run your business. As long as you link employee attitudes directly to business outcomes, prioritize business interventions that offer the greatest impact, ensure that managers are focusing on areas that will improve business outcomes the most, and improve key business drivers, you can ensure that your organization will be successful for years to come.
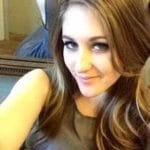
Emily Pribanic | Emily is a graduate of the University of North Texas. She has her B.A. in Advertising with a concentration in Copywriting. Emily has been writing since she was young and has a creative imagination. She lives in Dallas, Texas with her family and two cats.
Emily Pribanic | Emily is a graduate of the University of North Texas. She has her B.A. in Advertising with a concentration in Copywriting. Emily has been writing s...
Related Posts.
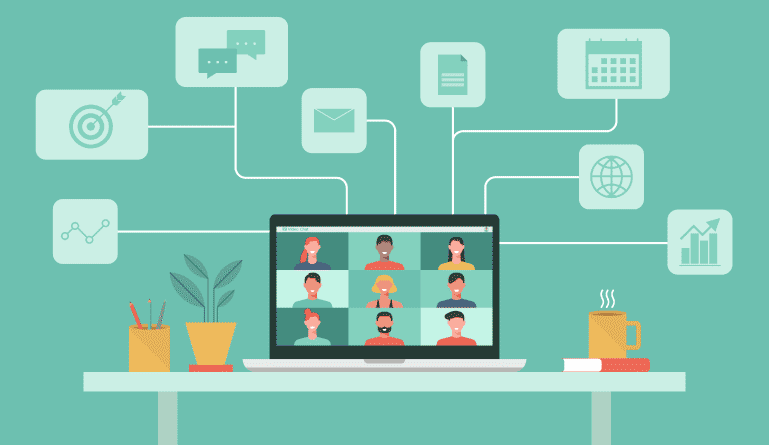
Optimizing Remote Collaboration: Tools and Strategies for Effective Virtual Teams
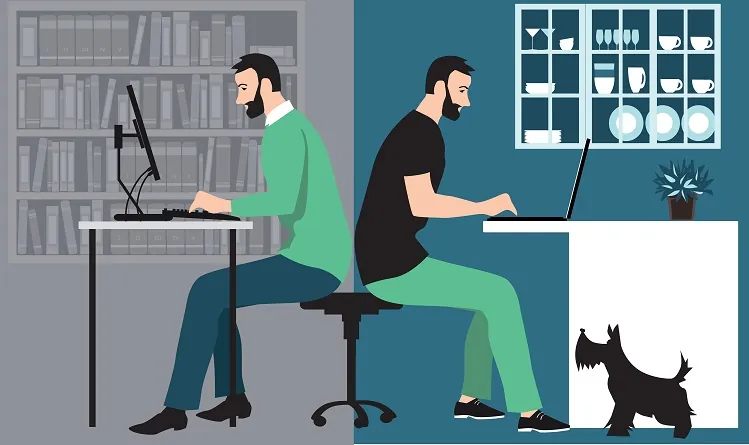
The Future of Remote Work: Navigating the Hybrid Workforce
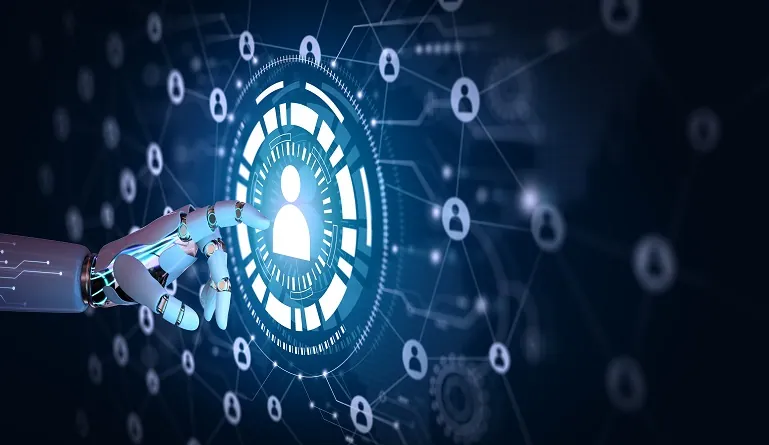
Revolutionize HR: 10 Tasks to Automate with AI in 2024
Latest read.
Error: Contact form not found.
Latest Watch
Phone Login
Looks like you already have an account with this ID. You can try logging in
Forgot password?
Back to login
Register Now
This Email id already exist please try loging in
Create an account to find courses best suited to your profile
- September 26, 2022
Best HR Case Studies
Drop your details to know more about programme
- Mobile Number *
- State * State* Andaman and Nicobar Andhra Pradesh Arunachal Pradesh Assam Bihar Chandigarh Chhattisgarh Dadra and Nagar Haveli Daman and Diu Delhi Goa Gujarat Haryana Himachal Pradesh Jammu and Kashmir Srinagar Jharkhand Karnataka Kerala Lakshadweep Madhya Pradesh Maharashtra Manipur Meghalaya Mizoram Nagaland Odisha Puducherry Punjab Rajasthan Sikkim Tamil Nadu Telangana Tripura Uttar Pradesh Uttarakhand West Bengal
- I accept Terms and Conditions
Last date of application: 14/07/2022
HR as a function has undeniable importance from a business management perspective. With the advancement in technology, 2022 saw a huge technological shift in this aspect of business management as well. Apart from digitizing all other business aspects, organizations have begun to incorporate technology and data into HR practices as well.
HR Analytics Case Studies with Business Impact and its benefits are listed below:
An american mnc reduces attrition using people analytics and forecasting.
Case: This American MNC is a client of PeopleStrong and is suffering from a high turnover of employees at five locations. The company intended to install analytics in order to evaluate the main drivers of attrition and do forecasting for their occurrence at different business locations.
Solution: An integrated tool for workforce analytics was created and implemented. This tool could capture attrition results and their drivers and do a forecasting based on trends.
Also Read: Executive Development Program In Human Resource Management From XLRI Jamshedpur
Result: The forecasting report predicted that 500 of the 5000 employees were going to quit in the next 6 months. Better employee retention policies were designed which included rewards and incentives apart from better people strategies. Even though 250 people still left, the figure was 50% lower than the prediction.
Under Armour digitized employee recruitment and enhanced employee experience
Case: Under Armour, an American organization dealing with the manufacture of sports and casual apparel and footwear, is a global company. With more than 130 global outlets and 8500 employees, their ATS system received more than 30,000 resumes in a month. Thus, hiring was a cumbersome process for them as well as candidates applying for a job.
Solution: They engaged in a digital recruitment system called Hirevue. With Hirevue, managers could create interviews with candidates with the help of pre-recorded questions. This screening process helped managers call in only employees who met their requirements for webcam or mobile recorded interviews.
Result: Managers could now hire new employees much more quickly. There was a 35% reduction in time in the overall interview to the hiring process. Talent quality also improved.
These above case studies show the emerging trend of incorporating analytics in the HR function of business management . This can also be seen to have positive results in the recruitment and retention processes.
Human resource management is quite a recent term. Employees are treated with a lot of respect and regard nowadays compared to earlier. There were times when workers were considered to be expendable and they had few rights. Working conditions were miserable and people had no say in how organizations are operated or in the way they were treated. The industrial revolution is what brought changes. Companies started realizing that keeping employees loyal was essential for running businesses smoothly.
Caring For Employees During The Industrial Revolution
Courses for human resources certification online teach that before the industrial revolution there were hardly any large industries and a need for managing workers was not felt. Working conditions were dangerous for them and pay was hardly commensurate with what work they did. In the late 1900s, companies like the UK-based Cadbury and Jacob from Ireland appointed welfare officers. These firms introduced a system of payment during sick leaves and cheap housing for employees.
Also Read: Executive Development Program In HR Analytics From XLRI
It was F W Taylor during the early twentieth century who introduced a system for managing staff. He believed that people could be trained to become experts in certain jobs. The famous carmaker Ford adopted his methods. Tools in manpower management like job analysis, employee selection procedures, and training methods were introduced during this period. Certain fast food organizations also adopted Taylor’s theories. His mistake was that he did not think people can get bored with doing the same job.
Employee Management During The World Wars
Two events that changed many things for us are the first and second world wars. Employee unions had been formed during the first world war. As men went to fight wars, women came to be seen more in workplaces. In your HR training certification by IIM Raipur , you will learn how companies had to think about managing workers and form new rules. Recruitment, dismissal, bonus, and absence from work came under the scope of manpower management.
Researchers like Elton May opined that factors like motivation, job satisfaction, leadership skills, and group dynamics could influence performance. The improvement in the economy after the war saw many firms adopting a more flexible approach to staff members. Big companies used employee benefits to lure and retain people. Personnel and welfare work was in full swing during the second world war, but it was done in a bureaucratic style as government-run firms influenced law-making.
The Post-War Scenario
The 60s were not good times for industrial relations as it was found that none of the entities involved in negotiation had skills to discuss issues of employees. As the decade came to an end, employment opportunities improved, and along with this, people management techniques began to be used. When you study human resources certification online courses you will know that terms like motivation, organizational behavior, and management training were heard more commonly.
Also Read: Executive Development Program In Talent Management
In the seventies, much was talked about rewarding employees. The next two decades saw economies sliding and companies becoming less profitable. But it was also then that many organizations realized the importance of retaining people. They began looking at workers as an asset that must be taken care of if the firm wants to have an edge over competitors. Humans started to be regarded as resources that need to be effectively managed. Human Resource Management was born.
The Nineties To Now
It is no more only personnel management and administrative tasks for workforce heads. The HR training certification by IIM Raipur will tell you that it is more about employee engagement and development that people managers are tasked with now. Human resource departments are strengthening the culture in an organization and finding people who can fit that environment. They are also tasked with ensuring that every employee gets an opportunity to use his or her talents for the benefit of their companies.
Also Read: Why is it Important to Study Human Resource Management?
HR managers are more focused on workers than on processes. This department is also gaining more importance as management’s realize a need to attract and retain the best talents available in the market. HR leaders find themselves among the C-suite as their role in getting the best out of employees is increasing. They must understand the needs of a more diverse, multicultural, and multigenerational workforce and ensure to fulfill them. Retention of good hands has assumed much importance nowadays.
The Future Of HR Management
The human resources certification online courses will teach that it is not just enough to employ and retain people, but they must also be trained and developed. The speed at which new technologies emerge, there is a need to keep employees abreast of modern developments. HR managers must continuously update themselves with modern technology and arrange training programs to empower workers with new skills. The journey of staff members in an enterprise will be that of continuous learning.
Acquiring best talents and retaining them will remain the focus of any progressive organization. People managers will have to find innovative means to attract those who are equipped with the latest skills required for a job. Engaging with prospective employees through social media platforms will be practiced by more HR heads. There will be increased use of automation for screening resumes and conducting initial interviews. This will speed up the process and reduce costs.
HR departments will be trying innovative methods to improve employee experience in the company. They will find out the requirements of the new breed of recruits. Learning opportunities will be improved. Promotions and salary hikes will no longer be based on experience or seniority. New procedures for evaluating employees will be used. Getting HR training certification by IIM Raipur will teach new methods that are used by global enterprises for appraisal and rewarding.
Looking at the evolution of human resource management can show you that there has been a shift from looking at employees as only a means to achieve company objectives, treating them as individuals, and satisfying their needs. There is a realization that it is equally important to ensure that their goals are achieved and these objectives are in line with that of the organization. HR departments will play a more important role as retaining good talent becomes crucial. Combining the human force with machines and using that synergy will be highly important in the future.
More Information:
Executive Program In Business Management
Professional Certificate Program In General Management
How to grow your career in Human Resource Management?
Executive Program In Supply Chain Management During Uncertain Times
Professional Certificate Program In Supply Chain Strategy And Management
Executive Development Program In Project Management For Senior Professionals
Want to know how can this course help in your profile?
Talk to our counsellors to find a course best for your career.
- Will get in touch with you soon
Let us call you back
We'll contact you asap.
- Select a Course Select a course Post Graduate Diploma In Finance Doctor Of Business Administration Doctorate of Business Administration Certificate Program in Applied Data Science and Deep Learning Executive Development Programme in Leadership in Sales & Marketing – The CMO Programme Postgraduate Certificate In Business Analytics B- 6 New Digital Marketing Job Linked Bootcamp Executive Development Programme in Strategic Decision Making in the Digital Era Professional Certificate Program in Health Care Management with immersion Advanced Certificate in Digital Marketing and Communication S4 Advanced Certificate in Digital Marketing and Communication S3 Advanced Certificate in Digital Marketing and Communication S2 Executive Development Programme in Applied Finance Executive Development Programme in Talent Management Executive Certificate Program in HR Analytics Advanced Certificate in Supply Chain Management MBA from Staffordshire Business School Professional Certificate program in Cyber Security Doctor of Business Administration in Emerging Technologies Executive Development Program in HR Analytics from XLRI B6 Executive Development Programme in Digital HR Transformation & AI-Driven HR Analytics MICA-MBMC-14-1223 Professional Certificate Program in Business Analytics B8 Professional Certificate Program In Marketing And Sales Management Executive Development Program in Advanced Financial Management MS in Full Stack Artificial Intelligence and Machine Learning MS in Full Stack Artificial Intelligence and Machine Learning MS in Full Stack Artificial Intelligence and Machine Learning Advanced Program in Strategic Management for Business Excellence -B4 Executive Certificate Program in Supply Chain Management and Analytics Master of Business Administration (MBA) Liverpool Business School -immersion MBA (Global) | Deakin Business School- With immersion Executive Development Program in Human Resource Management from XLRI Jamshedpur -B15(Copy) Advanced Certificate in Sales Forecasting and Demand Planning EXECUTIVE DEVELOPMENT PROGRAMME IN DIGITAL TRANSFORMATION STRATEGIES Executive Development Program in Human Resource Management from XLRI Jamshedpur -B15 Executive Development Program in Financial Analytics B-6 Full Stack Development Bootcamp Professional Certificate Program in Health Care Management Executive Development Program in Talent Management from XLRI- B12 Executive Certificate Programme in Advanced Strategic Management & Innovation Executive Development Programme in Driving Growth – The CXO Programme B-2 Executive Development Program in Project Management For Senior Professionals from XLRI Jamshedpur -B10 Ecommerce Supply Chain Management and Analytics Professional Certificate Program in General Management Batch 6 Master of Science in Computer Science Master of Science in Data Science Executive Post Graduate Programme in Machine Learning & AI Executive Development Programme in Strategic Marketing Management (Batch 2) Advanced Certificate Programme in Big Data Programming Advanced Certificate Program in Devops Advanced Certificate Programme in Cloud Backend Development Advanced Certificate Programme in Blockchain Executive Development Programme in Strategic Brand Management -B2 Advanced Certificate Programme in Cyber Security Executive Program in Supply Chain Management During Uncertain Times -B3 Executive Program in Marketing Strategy -B4 Executive Post Graduate Program in Full Stack Software Development Post Graduate Diploma in Management Post Graduate Certificate in Product Management Leadership and Management in New Age Business Executive Development Program in Data Science using Python, R & Excel B-10 Postgraduate Certificate In Business Analytics B- 6 Postgraduate Certificate in Human Capital Leadership -B-2 Executive Development Program in Digital Marketing B-7 100% Job-Guarantee Post Graduate Certificate in Software Engineering Full Stack Development Bootcamp - 100% job opportunities in MAANG/Top product companies Advanced General Management Program Professional Certificate Programme in HR Management and Analytics Executive Post Graduate Programme in Data Science Executive Post-Graduate Programme in Human Resource Management Professional Certificate Program in Data Science and Business Analytics Executive Post Graduate Program in Data Science and Machine Learning PROFESSIONAL CERTIFICATE PROGRAM IN MARKETING AND SALES MANAGEMENT -BATCH 8 Advanced Program in Leadership in the Digital Era -b3 Executive Development Program in Transformational Leadership -B5 Executive Program in Business Management - Batch 3 Professional Certificate Program in Business Analytics from IIM Kozhikode -B7 Executive Development Program in Strategic Management from XLRI -Batch6 Executive Development Programme In Business Analytics and Big Data Executive Certificate Program in Business Analytics and Big Data Advanced Certificate in Managing Brands and Marketing Communication -B-13 Executive Development Program in Human Resource Management from XLRI Jamshedpur -B14 Executive Development Program in Leadership & Change Management b-9 Certificate Programme In Operations Management And Analytics Professional Certificate Program in Supply Chain Strategy and Management - B5 Executive Development Program in Advanced Financial Management Executive Certificate Program in Applied Financial Risk Management -batch-3 Advanced Certificate in Digital Marketing and Communication Global Doctor of Business Administration Executive Development Programme in Strategic Marketing Management (Batch 1) Executive Development Program in HR Analytics -Batch 5 Executive Development Programme in Strategic Brand Management Master of Business Administration (MBA) Liverpool Business School Professional Certificate Program in Business Analytics from IIM Kozhikode Advanced Program in Strategic Management for Business Excellence Executive Development Program in Financial Analytics Advanced Certificate in Advertising Management and Public Relations Executive Development Programme Digital HR Transformation & AI-Driven HR Analytics Executive Program in Business Management Executive Program in Supply Chain Management During Uncertain Times CERTIFICATE PROGRAMME IN STARTUP BOOT CAMP Professional Certificate Program in Supply Chain Strategy and Management CERTIFICATE PROGRAMME IN INDUSTRIAL DESIGN, INNOVATION AND ENTREPRENEURSHIP Executive Development Program in HR Analytics B4 Executive Program in Marketing Strategy
Call us to get more information
Our counsellors will call you back in next 24 hours to help you with courses best suited for your career
- Human Capital Leadership XLRI Jamshedpur
*I hereby authorize Talentedge to contact me. It will override my registry on the NCPR.
Fee Structure
Program Fees
INR /- +GST
EMI Partners
No Cost EMI - 9 Months
Standard emi - 12 months, standard emi - 18 months, standard emi - 24 months.
* I accept Privacy Policy and Terms & Conditions. I appoint MyMoneyMantra as authorized representative to receive my credit information from Experian for the purpose of providing access to credit & targeted offers ('End Use Purpose') as defined in given Terms & Conditions.
* Loan Processing fee to be paid directly to the Loan Provider.
Request a call back
Let us help you guide towards your career path
- Non-biased career guidance
- Counselling based on your skills and preference
- No repetitive calls, only as per convenience
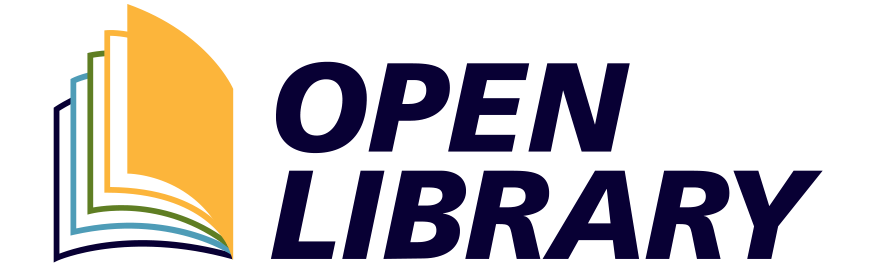
Want to create or adapt books like this? Learn more about how Pressbooks supports open publishing practices.
2.14 Case Study: The Power of HR Analytics for ACME Inc.
Case study: the power of hr analytics for acme inc..
ACME Inc. has a problem, a big problem. It is losing employees at a rate of 18% per year. Benchmarks in their industry are at 9%. For the past few years, new government regulations have been making the industry more competitive and profits are on a steady decline. The CEO has identified curbing the high turnover rate as a primary objective for the HR department.
The VP of Human Resources has hired a team of data analysts to look at the issue. The team performed advanced analysis on the anonymous corporate employee data integrated from several HR information systems. The dataset contains common and specific HR-oriented features for approximately 1,560 individual employees. Topics include demographics, satisfaction with the job and the company, absences, salary, and even travelling schedule. For each individual record, there is also information determining whether the corresponding employee left the company at the end of analyzed period. This information is used to identify key features connected to attrition. By identifying data patterns that can predict current employee behaviour in terms of attrition, the company can develop strategies to address the issue(s).
For the purpose of the case-study, the dataset was inspected and using the logistic regression method, two different questions were answered:
- Which specific factors increase or decrease the probability of attrition?
- Which individual employees across different jobs are at high risk of attrition?
Regarding the first question, advanced modelling techniques like neural networks were able to identify “drivers” that influence the target variable: risk of attrition. See the article: What Drives Employee Turnover? Part 2 for a list of the drivers of employee turnover. This kind of information provides seemingly straightforward insight. However, to deliver more thorough and usable conclusions, it is necessary to go a little deeper. One possible way to do that is to perform a separate analysis for different job types and roles. The next figure (2.13.1) shows the importance of various factors for three different job categories: technician, scientist and salesman.
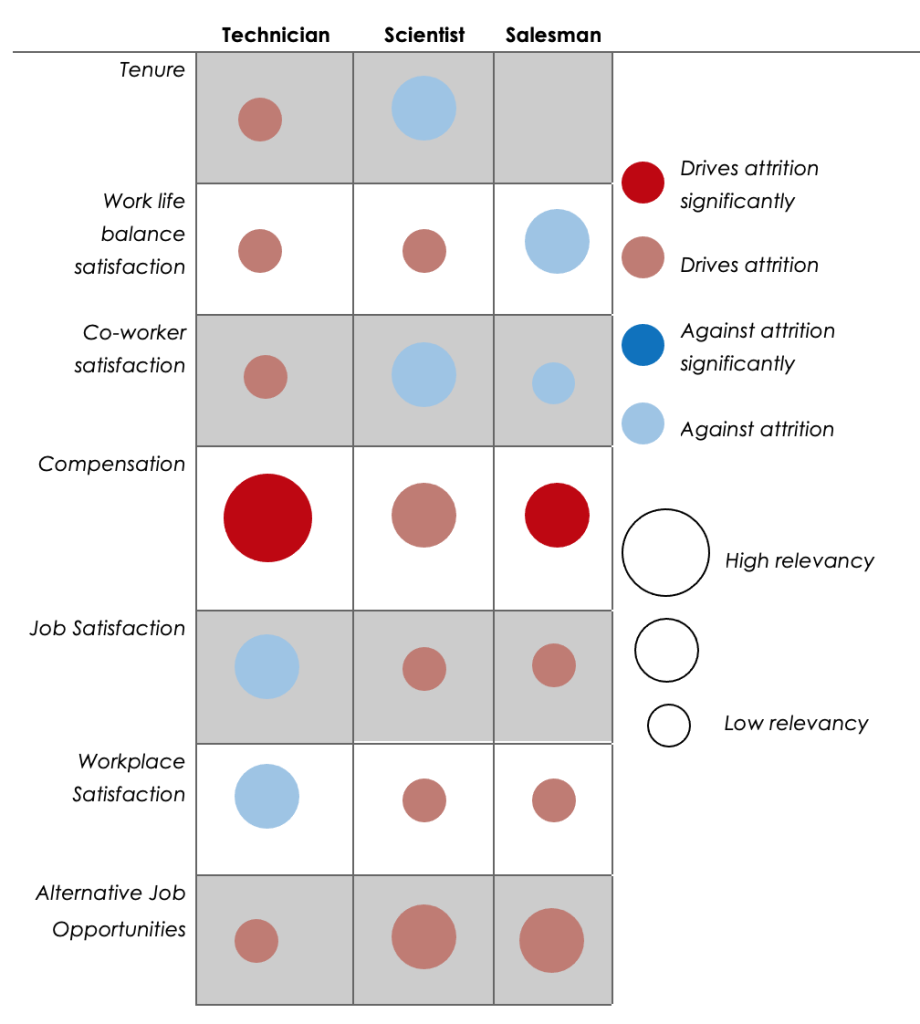
In order to answer the second question -who are the employees most at risk of leaving, a prediction model was developed and applied. For validation purposes, one-third of the dataset was separated to test the model accuracy. The rest was used to train the model and perform previous analysis. The newly-developed model is able to predict 88.9% of employees with “left-the-company” flags.

Now equipped with this information, the VP of Human Resources is working at addressing the issues with a concrete and aggressive plan of action to curb turnover.
- What are the factors that made this advanced analysis successful?
- Research prediction model and discuss what makes is a successful model to predict risk of employees leaving. Why was this step important to ACME Inc.
- Following the data analysis, what are the first action steps (name 5 steps) that the VP of Human Resources can take to address the issues in a concrete manner?
“ Chapter 11: HR Analytics ” from Human Resources Management – 2nd Ontario Edition by Elizabeth Cameron is licensed under a Creative Commons Attribution-NonCommercial-ShareAlike 4.0 International License .
Human Resources Management - 3rd Edition Copyright © 2023 by Debra Patterson is licensed under a Creative Commons Attribution-NonCommercial-ShareAlike 4.0 International License , except where otherwise noted.
Share This Book
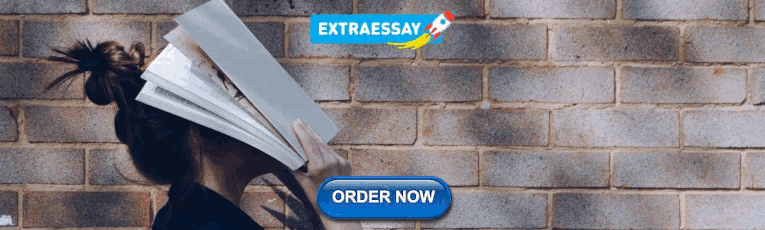
IMAGES
VIDEO
COMMENTS
The full details of this HR analytics case study and the statistical tests can be found here. 7. Achieving an optimum staffing level. Another interesting HR analytics case study was about reaching optimum staffing levels. A large mining company in Zimbabwe was concerned about losing money because of over or understaffed departments.
Establish performance metrics. Collect data. Identify relevant patterns and develop hypotheses. Fill the data gaps. Analyze the results. Implement data-driven strategies. Here are five real-world case studies that demonstrate different ways in which businesses have used people analytics to improve their bottom line.
People analytics—the application of advanced analytics and large data sets to talent management—is going mainstream.Five years ago, it was the provenance of a few leading companies, such as Google (whose former senior vice president of people operations wrote a book about it).Now a growing number of businesses are applying analytics to processes such as recruiting and retention, uncovering ...
Case studies with business impact. People analytics has transformed from trend to an essential part of HR's toolkit. Confidence in the discipline is high — just look at the people analytics technology market, which was valued at $5.6 billion in 2022, and only expected to grow. If you're still not sure if investing time or budget in people ...
Ingredients for success. Our conversations with people analytics teams in leading organizations reveal a set of six best-in-class ingredients that have helped to propel the teams' impact, success, and continued growth. These ingredients fall into three main categories: data and data management, analytics capabilities, and operating models.
The top 10 HR Analytics case studies demonstrate the tangible impact of data-driven HR strategies on employee outcomes and business results. From reducing road traffic accidents to improving employee engagement and retention, these HR analytics case studies show how organizations can use HR Analytics to make informed decisions about their ...
This is evidenced in various case studies that have focused on how HR analytics facilitates evidence-based decision-making to improve HR and business performance (Harris et al., 2011; ... While HR analytics is gaining increasing interest as a field of study, HR analytics is still a relatively new concept. As a result, scholars and practitioners ...
Valuing people. This case study provides insight into Coca-Cola Enterprises' (CCE) data analytics journey. Given the complexity of the CCE operation, its global footprint and various business units, a team was needed to provide a centralised HR reporting and analytics service to the business. This led to the formation of a HR analytics team ...
Internal promotion-how Chipotle reduced turnover by 64%. Internal promotion is a valuable, yet underutilized, tool to engage employees and managers in the recruiting process, provide career growth, and save on costs associated with bringing in external talent. As I alluded yesterday, the content covered at Hire Minds was astonishing.
Gamification can be a good way to enhance an already existing digital process. 5. Global Energy Firm. Making Digital HR a reality. While it's important to focus on providing digital platforms to improve your customer experience, it's just as important to recognize the importance of Digital HR to improve your employee experience.
HR Analytics Case Studies. 1. Employee Leave Predictions at Credit Suisse. In March of 2015, The Wall Street Journal published one of the first articles detailing how employers can effectively predict which potential hires might stay with their company longer, thus, saving the company a lot of money. This now extremely popular HR metric, known ...
Human Resources analytics underscores the value of HR data by emphasizing how people create value for the organization, so that value can be captured and leveraged. The research discusses transition of HR analytics with a linear three-stage maturity model and explains progression from traditional HR analytics to new age HR analytics.
Apply Your Skills with a Power BI Case Study. In this Power BI case study, you will be exploring a dataset for a fictitious software company called Atlas Labs. This course focuses on helping you import, analyze and visualize Human Resources data in Power BI. Building on your existing knowledge of the platform, you'll learn how to effectively ...
HR Analytics Case Studies with Business Impact and its benefits are listed below: An American MNC reduces attrition using people analytics and forecasting . Case: This American MNC is a client of PeopleStrong and is suffering from a high turnover of employees at five locations. The company intended to install analytics in order to evaluate the ...
in HR. Being an emerging important to show the value deliver to organizations. In this case study we have collected some of People Analytics case studies come across in the past Each one connected to a specific business imperative. You can find the originalpublication of each case study in. 1. CREDIT SUISSE. Saving money by predicting who will ...
In this Power BI case study, you will be exploring a dataset for a fictitious software company called Atlas Labs. This course focuses on helping you import, analyze and visualize Human Resources data in Power BI. Building on your existing knowledge of the platform, you'll learn how to effectively work with Power BI using example data.
In this intermediate-level Power BI live training, you'll learn how to solve an interview case study in Power BI using an HR dataset and uncover insights for a fictitious software company. You'll explore the data and build relationships between tables in your data model. You'll then use DAX to create new measures and columns that will ...
Case Study: The Power of HR Analytics for ACME Inc. ACME Inc. has a problem, a big problem. It is losing employees at a rate of 18% per year. Benchmarks in their industry are at 9%. For the past few years, new government regulations have been making the industry more competitive and profits are on a steady decline.
For more real-world HR analytics examples, you can refer to the case studies we published in the past. Here are links to three of them: Case Study 1: Key Drivers of Retail Sales Performance; Case Study 2: Reducing Workplace Accidents Using People Analytics; Case Study 3: How We Determined Optimal Staffing Levels
Analyzing HR Data in Tableau. In this Tableau case study, you will explore a dataset for a fictitious software company called Atlas Labs. This course focuses on helping you import, analyze and visualize Human Resources data in Tableau. Building on your existing knowledge of the platform, you'll learn how to effectively work with Tableau using ...
2. Provides a basis for business agility. A business's success depends on its ability to adapt its people and processes during periods of uncertainty or change. Many HR software vendors offer ...