Advertisement
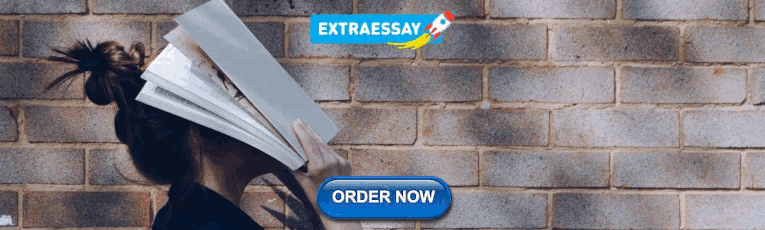
“Internet Addiction”: a Conceptual Minefield
- Open access
- Published: 19 September 2017
- Volume 16 , pages 225–232, ( 2018 )
Cite this article
You have full access to this open access article
- Francesca C. Ryding 1 &
- Linda K. Kaye 1
17k Accesses
56 Citations
19 Altmetric
Explore all metrics
With Internet connectivity and technological advancement increasing dramatically in recent years, “Internet addiction” (IA) is emerging as a global concern. However, the use of the term ‘addiction’ has been considered controversial, with debate surfacing as to whether IA merits classification as a psychiatric disorder as its own entity, or whether IA occurs in relation to specific online activities through manifestation of other underlying disorders. Additionally, the changing landscape of Internet mobility and the contextual variations Internet access can hold has further implications towards its conceptualisation and measurement. Without official recognition and agreement on the concept of IA, this can lead to difficulties in efficacy of diagnosis and treatment. This paper therefore provides a critical commentary on the numerous issues of the concept of “Internet addiction”, with implications for the efficacy of its measurement and diagnosticity.
Similar content being viewed by others
Internet Addiction
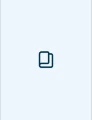
Avoid common mistakes on your manuscript.
What Is Internet Addiction (IA)?
Traditionally, the term addiction has been associated with psychoactive substances such as alcohol and tobacco; however, behaviours including the use of the Internet have more recently been identified as being addictive (Sim et al. 2012 ). The concept of IA is generally characterised as an impulse disorder by which an individual experiences intense preoccupation with using the Internet, difficulty managing time on the Internet, becoming irritated if disturbed whilst online, and decreased social interaction in the real world (Tikhonov and Bogoslovskii 2015 ). These features were initially proposed by Young ( 1998 ) based on the criteria for pathological gambling (Yellowlees and Marks 2007 ), and have since been adapted for consideration within the DSM-5. This has been well received by many working in the field of addiction (Király et al. 2015 ; Petry et al. 2014 ), and has been suggested to enable a degree of standardisation in the assessment and identification of IA (King and Delfabbro 2014 ). However, there is still debate and controversy surrounding this concept, in which researchers acknowledge much conceptual disparity and the need for further work to fully understand IA and its constituent disorders (Griffiths et al. 2014 ).
Much of the debate relates to the issue that IA is conceptualised as addiction to the Internet as a singular entity, although it incorporates an array of potential activities (Van Rooij and Prause 2014 ). That is, the Internet, in all its formats, whether accessed via PC, console, laptop or mobile device, is fundamentally a portal through which we access activities and services. Internet connectivity thus provides us with ways of accessing the following types of activities; play (e.g. online forms of gaming, gambling), work (accessing online resources, downloading software, emailing, website hosting), socialising (social networking sites, group chats, online dating), entertainment (film databases, porn, music), consumables (groceries, clothes), as well as many other activities and services. In this way, the Internet is a highly multidimensional and diverse environment which affords a multitude of experiences as a product of the specific virtual domain. Thus, it is questionable as to whether there is any degree of consistency in the concept of IA, in light of these diverse and specific affordances which may relate to Internet engagement. Indeed, it has been indicated that there are several distinct types of IA, including online gaming, social media, and online shopping (Kuss et al. 2013 ), and it has been claimed that through engagement in these behaviours, individuals may become addicted to these experiences, as opposed to the medium itself (Widyanto et al. 2011 ). Thus, IA is arguably too generalised as a concept to adequately capture these nuances. That is, an individual who spends excessive time online for shopping is qualitatively different from someone who watches or downloads porn excessively. These represent distinct behaviours which are arguably underpinned by different gratifications. Thus, the functionality of aspects of the Internet is a key consideration for research in this area (Tokunaga 2016 ). This is perhaps best approached from a uses and gratifications perspective (LaRose et al. 2003 ; Larose et al. 2001a ; Wegmann et al. 2015 ), to more fully understand the aetiology of IA (discussed subsequently). This is often best underpinned by the uses and gratifications theory (Larose et al. 2001a , 2003 ), which seeks to explain (media) behaviours by understanding their specific functions and how they gratify certain needs. Indeed, in the context of IA, this may be particularly useful to establish the extent to which certain Internet-based behaviours may be more or less functional in need gratification than others, and the extent to which it is Internet platform itself which is driving usage or indeed the constituent domains which it affords. If the former, then controlling Internet-based usage behaviour more generically is perhaps appropriate, however, a more specified approach may often be required given the diverse needs the online environment can afford users.
IA from a Gratifications Perspective
It is questionable on the extent to which IA is itself the “addiction” or whether its aetiology relates to other pre-existing conditions, which may be gratified through Internet domains (Caplan 2002 ). One particular theory that has been referenced throughout much developing research (King et al. 2012 ; Laier and Brand 2014 ) is the cognitive-behavioural model, proposed by Davis ( 2001 ). This model suggests that maladaptive cognitions precede the behavioural symptoms of IA (Davis 2001 ; Taymur et al. 2016 ). Since much research focuses on the comorbidity between IA and psychopathology (Orsal et al. 2013 ), this is particularly useful in underpinning the concept of IA, and perhaps provides support that IA is a manifestation of underlying disorders, due to its psychopathological aetiology (Taymur et al. 2016 ). Additionally, the cognitive-behavioural model also distinguishes between both specific and generalised pathological Internet use, in comparison to global Internet behaviours that would not otherwise exist outside of the Internet, such as surfing the web (Shaw and Black 2008 ). As such, it would assume those individuals who spend excessive time playing poker online, for example, are perhaps better categorised as problematic gamblers rather than as Internet addicts (Griffiths 1996 ). This has been particularly advantageous in the contribution to defining IA, as earlier literature tended to focus solely on either content-specific IA, or the amount of time spent online, rather than focussing as to why individuals are actually online (Caplan 2002 ). Indeed, this shows promise in resolving some of the aforementioned issues in the specificity of IA, as well as the likelihood of pre-existing conditions underpinning problematic behaviours on the Internet.
Much of the recent literature in the realm of IA has focused upon Internet Gaming Disorder (IGD) which has recently been included as an appendix as “a condition for further study” in the DSM-5 (American Psychiatric Association 2013 ). This has driven a wide range of research which has sought to establish the validity of IGD as an independent clinical condition (Kuss et al. 2017 ). Among the wealth of research papers surrounding this phenomenon, there remains large disparity within the academic community. Although some researchers claim there is consensus on IGD as a valid clinical disorder (Petry et al. 2014 ), others do not support this (e.g. Griffiths et al. 2016 ). As such, the academic literature has some way to go before more established claims can be made towards IGD as a valid construct, and indeed how this impacts upon clinical treatment.
One means by which researchers could move forward in this regard is to establish the validity of IGD to a wider range of gaming formats. That is, IGD research has predominantly defined the reference point in studies as “online games” or in some cases, is has been even less specific (Lemmens et al. 2015 ; Rehbein et al. 2015 ; Thomas and Martin 2010 ). Arguably, there are a range of forms of “online” gaming, including social networking site (SNS) games which are Internet-mediated and thus by definition, would appear under the remit of IGD. Indeed, links between SNS and gaming have been previously noted (Kuss and Griffiths 2017 ), although this has not specifically been empirically explored in the context of IGD symptomology. For example, causal form of gaming as is typically the case for SNS gaming have their own affordances in respect of where and how they are played, given these are often played on mobile devices rather than on more traditional PC or console platforms. Further, the demographics of who are most likely to play these games can vary from others forms of gaming which have predominated the IGD literature (Hull et al. 2013 ; Leaver and Wilson 2016 ). Accordingly, these affordances present additional nuances, which the literature has not yet fully accounted for in its exploration of IGD. Clearly, IGD relates to a specific form of Internet behaviour which may be conceptualised within IA, yet is paramount to understand it as a separate entity to ensure the conceptualisation and any associated treatment provision is sufficiently nuanced. Likewise, the same case can be made for many other Internet-based behaviours which may be best being established in respect of their functionality and gratification purposes for users.
IA as a Contextual Phenomenon
There is growing evidence suggesting that context is key towards the processes and cognitions associated with consumption of substances such as alcohol (Monk and Heim 2013 , 2014 ; Monk et al. 2016 ), highlighting some important implications towards understanding IA, as a form of behavioural addiction. That is, the study of IA has rarely been studied in respect of its contextual affordances, even though the combination of Internet connectivity (WiFi) and mobility (smartphones) means that the Internet may be accessed in many ways and in multiple contexts. It has been indicated by Griffiths ( 2000 ) that few studies consider the context of Internet use, despite many users spending a substantial amount of time on the Internet via the use of different platforms, such as mobile devices, as opposed to a computer (Hadlington 2015 ). It has been highlighted by Kawabe et al. ( 2016 ) that smartphone ownership in particular is rapidly increasing, and for some, smartphone devices have become a substitute for the computer (Aljomaa et al. 2016 ). It has also been suggested that the duration of usage on smartphones have been significantly associated with IA (Kawabe et al. 2016 ). This can largely be attributed to the advancement of smartphone technology, which permit them to function as a “one-stop-shop” for a variety of our everyday needs (checking the time, replying to emails, listening to music, interacting with others, playing games), and thus it is understandable that we are spending more of our time in using these devices. This further implicates research in IA, as this has often focussed on users’ Internet engagement through computers as opposed to mobile devices, albeit the numerous Internet subtypes accessible through mobile devices (Sinkkonen et al. 2014 ). One Internet subtype in particular which may facilitate addictive behaviours are social networking sites such as Facebook (Wu et al. 2013 ). Particularly, research has identified a positive relationship between daily usage of smartphones and addictive symptoms towards Facebook (Wu et al. 2013 ). This may also be the case for behaviours such as gaming through SNS which are typically accessed on mobile devices rather than computers. However, of critical interest here, is that addiction to these games has been argued to fall under the classification of IGD, despite being online via Facebook (Ryan et al. 2014 ). This indicates that the platform of Internet access is important in online behaviours, as well as implicating that further distinction between Internet subtypes should be made (particularly within SNS), to establish the different features of these, and how these affordances may be related to excessive usage. This issue is particularly pertinent given the increased interest in “smartphone addiction” (Kwon et al. 2013 ) in which the name assumes we are simply studying addiction to our smartphones themselves, not necessarily the functions they are affording to us. Research such as this is assuming the “problem” is the interaction with the technology (e.g. specific device) itself, when this is most likely not the case. Indeed, recent evidence highlights that different uses/functions of smartphones may be more likely to prompt users to feel more “attached” to the device than others, and that usage is often framed by one’s current context (Fullwood et al. 2017 ).
In addition to being able to access the Internet through multiple platforms, we are often reliant on the Internet for many everyday tasks, which poses a further issue in conceptualising what is “problematic” compared to “required” usage. The increased exposure to the Internet in both work and education make it difficult to avoid usage in such environments (Kiliҫer and Ҫoklar 2015 ; Uçak 2007 ), and it could be argued that the amount of time spent on the Internet for such contexts cannot be reflected as an addiction (LaRose et al. 2003 ). This is pertinent in light of much research, which tends to rely on metrics such as time spent online (e.g. average hours per week) as a variable in research paradigms. Particularly, this tends to be used to correlate against other psychological factors, such as depression or well-being, to indicate how “internet use” may be a problematic predictor of these outcomes (e.g. Sanders et al. 2000 ). In light of the aforementioned issues, this does not offer any degree of specificity in how time spent online is theoretically related to the outcomes variables of interest (Kardefelt-Winther 2014 ). Other studies have approached this with greater nuance by considering specific activities, such as number of emails sent and received in a given time period (Ford and Ford 2009 ; LaRose et al. 2001b ), or studied Internet use for a variety of different purposes, such as for health purposes and communication (Bessière et al. 2010 ). Further, other researchers have highlighted the distinction between behaviours such as smartphone “usage” versus “checking” (Andrews et al. 2015 ), whereby the latter may represent a more compulsive and less consciously driven and potentially more addictive form of behaviour than actual “usage”. These more nuanced approaches provide a more useful and theoretically insightful means of establishing how time spent online may be psychologically relevant as a concept. This suggests that future research which theorises on the impacts of “time spent online” (or “screen-time use”) should provide distinction between usage for work/education and leisure, and the gratification this engagement affords, to obtain greater nuance beyond the typical flawed metrics such as general time spent online.
A further compliment to the existing IA literature would be greater use of behavioural measures which garner users’ actual Internet-based behaviours. This is particularly relevant when considering that almost all existing research on smartphone addiction or problematic use, for example has been based on users’ self-reported usage, with no psychometric measure being validated against behavioural metrics. Worryingly, it has been noted that smartphone users grossly underestimate the amount of times they check their smartphone on a daily basis, with digital traces of their smartphone behaviours illuminating largely disparate findings (Andrews et al. 2015 ). Clearly, there is much opportunity to establish forms of Internet usage by capitalising on behavioural metrics and digital traces rather than relying on self-report which may not always be entirely accurate.
The concept of IA is more complex than it often theorised. Although there have been multiple attempts to define the characteristics of IA, there a numerous factors which require greater clarity in the theoretical underpinnings of this concept. Specifically, IA is often considered from the perspective that the Internet itself (and indeed the technology through which we access it) is harmful, with little specificity in how this functions in different ways for individual users, as well as the varying affordances which can be gained through it. Unfortunately, this aligns somewhat with typical societal conceptions of “technology is harmful” perspective, rather than considering the technology itself is simply a portal through which a psychological need is being served. This perspective is not a new phenomenon. Most new media has been subject to such moral panic and thus this serves a historical tradition within societal conception of new media. Indeed, this has been particularly relevant to violent videogames which scholars have discussed in respect of this issue (Ferguson 2008 ). Whilst many scholars recognise this notion through the application of a user and gratifications perspective, stereotypical conceptions of “technology is harmful” still remain. This raises the question about how we as psychologists can enable a cultural shift in these conceptions, to provide a more critical perspective on such issues. The pertinence of this surrounds two key issues; firstly that moving beyond a “technology is harmful” perspective, particularly for concerns over “Internet addiction” as one example, can enable a more critical insight into the antecedents of problematic behaviour to aid treatment, rather than simply revoking access from the Internet for such individuals. Arguably, this latter strategy would not always address the route of the issue and raises implications about the extent to which recidivism would occur upon reinstating Internet access. Secondly, on a more general level, diverging from an “anti-technology” perspective can enable researchers to draw out the nuances of specific Internet environments and their psychological impacts rather than battling with more blanket assumptions that “technology” (as a unitary concept) is presenting all individuals with the same issues and affordances, regardless of the specific virtual platform or context. In this way, we may be presented with more plentiful opportunities to more critically explore individuals and their interactions across many Internet-mediated domains and contexts.
Aljomaa, S. S., Al Qudah, M. F., Albursan, I. S., Bakhiet, S. F., & Abduljabbar, A. S. (2016). Smartphone addiction among university students in the light of some variables. Computers in Human Behavior, 61 , 155–164. https://doi.org/10.1016/j.chb.2016.03.041 .
Article Google Scholar
American Psychiatric Association. (2013). Diagnostic and statistical manual of mental disorders (5th ed.). Washington, DC: American Psychiatric Association.
Book Google Scholar
Andrews, S., Ellis, D. A., Shaw, H., & Piwek, L. (2015). Beyond self-report: tools to compare estimated and real-world smartphone use. PloS One, 10 , e0139004.
Article PubMed PubMed Central Google Scholar
Bessière, K., Pressman, S., Kiesler, S., & Kraut, R. (2010). Effects of Internet use on health and depression: a longitudinal study. Journal of Medical Internet Research, 12 (1), e6. https://doi.org/10.2196/jmir.1149 .
Caplan, S. E. (2002). Problematic Internet use and psychosocial well-being: development of a theory-based cognitive–behavioral measurement instrument. Computers in Human Behavior, 18 (5), 553–575. https://doi.org/10.1016/s0747-5632(02)00004-3 .
Davis, R. A. (2001). A cognitive-behavioral model of pathological Internet use. Computers in Human Behavior, 17 (2), 187–195. https://doi.org/10.1016/s0747-5632(00)00041-8 .
Ferguson, C. J. (2008). The school shooting/violent video game link: casual relationship or moral panic? Journal of Investigative Psychology and Offender Profiling, 5 , 25–37.
Ford, G. S., & Ford, S. G. (2009). Internet use and depression among the elderly . Phoenix Center Policy Paper Series. Retrieved April 26, 2016, from http://www.phoenix-center.org/pcpp/PCPP38Final.pdf .
Fullwood, C., Quinn, S., Kaye, L. K., & Redding, C. (2017). My Virtual friend: a qualitative analysis of the attitudes and experiences of Smartphone users: implications for Smartphone attachment. Computers in Human Behavior, 75 , 347–355. https://doi.org/10.1016/j.chb.2017.05.029 .
Griffiths, M. D. (1996). Gambling on the Internet: a brief note. Journal of Gambling Studies, 12 (4), 471–473.
Article CAS PubMed Google Scholar
Griffiths, M. (2000). Internet addiction - time to be taken seriously? Addiction Research & Theory, 8 (5), 413–418. https://doi.org/10.3109/16066350009005587 .
Griffiths, M. D., King, D. L., & Demetrovics, Z. (2014). DSM-5 Internet Gaming Disorder needs a unified approach to assessment. Neuropsychiatry, 4 (1), 1–4.
Griffiths, M. D., Van Rooij, A. J., Kardefelt-Winther, D., et al. (2016). Working towards an international consensus on criteria for assessing internet gaming disorder: a critical commentary on Petry et al. (2014). Addiction, 111 , 167–175.
Hadlington, L. J. (2015). Cognitive failures in daily life: exploring the link with Internet addiction and problematic mobile phone use. Computers in Human Behavior, 51 , 75–81. https://doi.org/10.1016/j.chb.2015.04.036 .
Hull, D. C., Williams, G. A., & Griffiths, M. D. (2013). Video game characteristics, happiness and flow as predictors of addiction among video game players: a pilot study. Journal of Behavioral Addictions, 2 (3), 145–152. https://doi.org/10.1556/JBA.2.2013.005 .
Kardefelt-Winther, D. (2014). A conceptual and methodological critique of internet addiction research: towards a model of compensatory internet use. Computers in Human Behavior, 31 , 351–354.
Kawabe, K., Horiuchi, F., Ochi, M., Oka, Y., & Ueno, S. (2016). Internet addiction: prevalence and relation with mental states in adolescents. Psychiatry and Clinical Neurosciences, 70 (9), 405–412. https://doi.org/10.1111/pcn.12402 .
Article PubMed Google Scholar
Kiliҫer, K., & Ҫoklar, A. N. (2015). Examining human value development of children with different habits of Internet usage. Hacettepe University of Education, 30 (1), 163–177.
Google Scholar
King, D. L., & Delfabbro, P. H. (2014). The cognitive psychology of Internet gaming disorder. Clinical Psychology Review, 34 (4), 298–308. https://doi.org/10.1016/j.cpr.2014.03.006 .
King, D. L., Delfabbro, P. H., Griffiths, M. D., & Gradisar, M. (2012). Cognitive-behavioral approaches to outpatient treatment of Internet addiction in children and adolescents. Journal of Clinical Psychology, 68 (11), 1185–1195. https://doi.org/10.1002/jclp.21918 .
Király, O., Griffiths, M. D., & Demetrovics, Z. (2015). Internet gaming disorder and the DSM-5: conceptualization, debates, and controversies. Current Addiction Reports, 2 (3), 254–262. https://doi.org/10.1007/s40429-015-0066-7 .
Kuss, D. J., & Griffiths, M. D. (2017). Social networking networking sites and addiction: ten lessons learned. International Journal of Environmental Research and Public Health, 14 , 311. https://doi.org/10.3390/ijerph14030311 .
Article PubMed Central Google Scholar
Kuss, D. J., van Rooij, A. J., Shorter, G. W., Griffiths, M. D., & van de Mheen, D. (2013). Internet addiction in adolescents: prevalence and risk factors. Computers in Human Behavior, 29 (5), 1987–1996. https://doi.org/10.1016/j.chb.2013.04.002 .
Kuss, D. J., Grittihs, M. D., & Pontes, H. M. (2017). DSM-5 diagnosis of Internet Gaming Disorder: some ways forward in overcoming issues and concerns in the gaming studies field. Journal of Behavioral Addictions. https://doi.org/10.1556/2006.6.2017.032 .
Kwon, M., Lee, J., Won, W., Park, J., Min, J., Hahn, C., Gu, X., Choi, J., & Kim, D. (2013). Development and validation of a smartphone addiction scale. PloS One, 8 (2), e56936. https://doi.org/10.1371/journal.pone.0056936 .
Article CAS PubMed PubMed Central Google Scholar
Laier, C., & Brand, M. (2014). Empirical evidence and theoretical considerations on factors contributing to cybersex addiction from a cognitive-behavioral view. Sexual Addiction & Compulsivity, 21 (4), 305–321. https://doi.org/10.1080/10720162.2014.970722 .
Larose, R., Mastro, D., & Eastin, M. S. (2001a). Understanding Internet usage: a social-cognitive approach to uses and gratifications. Social Science Computer Review, 19 (4), 395–413. https://doi.org/10.1177/089443930101900401 .
LaRose, R., Eastin, M. S., & Gregg, J. (2001b). Reformulating the Internet paradox: social cognitive explanations of Internet use and depression. Journal of Online Behavior, 1 (2). Retrieved Setpember 12, 2017 from http://psycnet.apa.org/record/2002-14047-001 .
LaRose, R., Lin, C. A., & Eastin, M. S. (2003). Unregulated Internet usage: Addiction, habit, or deficient self-regulation? Media Psychology, 5 (3), 225–253. https://doi.org/10.1207/s1532785xmep0503_01 .
Leaver, T., & Wilson, M. (2016). Social networks, casual games and mobile devices: the shifting contexts of gamers and gaming . London: Bloomsbury Publishing Inc..
Lemmens, J. S., Valkenburg, P. M., & Gentile, D. A. (2015). The internet gaming disorder scale. Psychological Assessment, 27 (2), 567–582.
Monk, R. L., & Heim, D. (2013). Environmental context effects on alcohol-related outcome expectancies, efficacy and norms: a field study. Psychology of Addictive Behaviors, 27 , 814–818.
Monk, R. L., & Heim, D. (2014). A real-time examination of context effects on alcohol cognitions. Alcoholism: Clinical and Experimental Research, 38 , 2452–2459.
Monk, R. L., Pennington, C. R., Campbell, C., Price, A., & Heim, D. (2016). Implicit alcohol-related expectancies and the effect of context. Journal of Studies on Alcohol and Drugs, 77 , 819–827.
Orsal, O., Unsal, A., & Ozalp, S. S. (2013). Evaluation of Internet addiction and depression among university students. Procedia - Social and Behavioral Sciences, 82 , 445–454. https://doi.org/10.1016/j.sbspro.2013.06.291 .
Petry, N. M., Rehbein, F., Gentile, D. A., Lemmens, J. S., Rumpf, H., Mӧßle, T., et al. (2014). An international consensus for assessing Internet Gaming Disorder using the new DSM-5 approach. Addiction, 109 (9), 1399–1406. https://doi.org/10.1111/add.12457 .
Rehbein, F., Kliem, S., Baier, D., Mossle, T., & Petry, N. M. (2015). Prevalence of internet gaming disorder in German adolescents: diagnostic contribution of the nine DSM-5 criteria in a state-wide representative sample. Addiction, 110 (5), 842–851.
Ryan, T., Chester, A., Reece, J., & Xenos, S. (2014). The uses and abuses of Facebook: a review of Facebook addiction. Journal of Behavioral Addictions, 3 (3), 133–148. https://doi.org/10.1556/jba.3.2014.016 .
Sanders, C. E., Field, T. M., Diego, M., & Kaplan, M. (2000). The relationship between Internet use to depression and social isolation among adolescents. Adolescence, 35 (138), 237–242.
CAS PubMed Google Scholar
Shaw, M., & Black, D. W. (2008). Internet addiction. CNS Drugs, 22 (5), 353–365. https://doi.org/10.2165/00023210-200822050-00001 .
Sim, T., Gentile, D. A., Bricolo, F., Serpelloni, G., & Gulamoydeen, F. (2012). A conceptual review of research on the pathological use of computers, video games, and the Internet. International Journal of Mental Health and Addiction, 10 (5), 748–769. https://doi.org/10.1007/s11469-011-9369-7 .
Sinkkonen, H.-M., Puhakka, H., & Meriläinen, M. (2014). Internet use and addiction among Finnish adolescents (15–19 years). Journal of Adolescence, 37 (2), 123–131. https://doi.org/10.1016/j.adolescence.2013.11.008 .
Taymur, I., Budak, E., Demirci, H., Akdağ, H. A., Güngör, B. B., & Özdel, K. (2016). A study of the relationship between internet addiction, psychopathology and dysfunctional beliefs. Computers in Human Behavior, 61 , 532–536. https://doi.org/10.1016/j.chb.2016.03.043 .
Thomas, N. J., & Martin, F. H. (2010). Video-arcade game, computer game and Internet activities of Australian students: participation participation habits and prevalence of addiction. Australian Journal of Psychology, 62 (2), 59–66.
Tikhonov, M. N., & Bogoslovskii, M. M. (2015). Internet addiction factors. Automatic Documentation and Mathematical Linguistics, 49 (3), 96–102. https://doi.org/10.3103/s0005105515030073 .
Tokunaga, R. S. (2016). An examination of functional difficulties from Internet use: Media habit and displacement theory explanations. Human Communication Research, 42 (3), 339–370. https://doi.org/10.1111/hcre.12081 .
Uçak, N. Ö. (2007). Internet use habits of students of the department of information management, Hacettepe University, Ankara. The Journal of Academic Librarianship, 33 (6), 697–707. https://doi.org/10.1016/j.acalib.2007.09.004 .
Van Rooij, A., & Prause, N. (2014). A critical review of “Internet addiction” criteria with suggestions for the future. Journal of Behavioral Addictions, 3 (4), 203–213. https://doi.org/10.1556/jba.3.2014.4.1 .
Wegmann, E., Stodt, B., & Brand, M. (2015). Addictive use of social networking sites can be explained by the interaction of Internet use expectancies, Internet literacy, and psychopathological symptoms. Journal of Behavioral Addictions, 4 (3), 155–162. https://doi.org/10.1556/2006.4.2015.021 .
Widyanto, L., Griffiths, M. D., & Brunsden, V. (2011). A psychometric comparison of the Internet Addiction Test, the Internet-Related Problem Scale, and self-diagnosis. Cyberpsychology, Behavior, and Social Networking, 14 (3), 141–149. https://doi.org/10.1089/cyber.2010.0151 .
Wu, A. M. S., Cheung, V. I., Ku, L., & Hung, E. P. W. (2013). Psychological risk factors of addiction to social networking sites among Chinese smartphone users. Journal of Behavioral Addictions, 2 (3), 160–166. https://doi.org/10.1556/jba.2.2013.006 .
Yellowlees, P. M., & Marks, S. (2007). Problematic Internet use or Internet addiction? Computers in Human Behavior, 23 (3), 1447–1453. https://doi.org/10.1016/j.chb.2005.05.004 .
Young, K. S. (1998). Internet addiction: the emergence of a new clinical disorder. Cyberpsychology & Behavior, 1 (3), 237–244. https://doi.org/10.1089/cpb.1998.1.237 .
Download references
Author information
Authors and affiliations.
Department of Psychology, Edge Hill University, St Helens Road, Ormskirk, Lancashire, L39 4QP, UK
Francesca C. Ryding & Linda K. Kaye
You can also search for this author in PubMed Google Scholar
Corresponding author
Correspondence to Linda K. Kaye .
Ethics declarations
Conflict of interest.
The authors declare that they have no conflicts of interest.
Rights and permissions
Open Access This article is distributed under the terms of the Creative Commons Attribution 4.0 International License (http://creativecommons.org/licenses/by/4.0/), which permits unrestricted use, distribution, and reproduction in any medium, provided you give appropriate credit to the original author(s) and the source, provide a link to the Creative Commons license, and indicate if changes were made.
Reprints and permissions
About this article
Ryding, F.C., Kaye, L.K. “Internet Addiction”: a Conceptual Minefield. Int J Ment Health Addiction 16 , 225–232 (2018). https://doi.org/10.1007/s11469-017-9811-6
Download citation
Published : 19 September 2017
Issue Date : February 2018
DOI : https://doi.org/10.1007/s11469-017-9811-6
Share this article
Anyone you share the following link with will be able to read this content:
Sorry, a shareable link is not currently available for this article.
Provided by the Springer Nature SharedIt content-sharing initiative
- Internet addiction
- Gratifications
- Find a journal
- Publish with us
- Track your research
ORIGINAL RESEARCH article
Predictive effect of internet addiction and academic values on satisfaction with academic performance among high school students in mainland china.
- Department of Applied Social Sciences, The Hong Kong Polytechnic University, Hong Kong, Hong Kong SAR, China
Academic performance occupies an important role in adolescent development. It reflects adolescents’ cognitive ability and also shapes their academic and career paths. Students who are satisfied with their school performance tend to show higher self-esteem, confidence, and motivation. Previous research has suggested that students’ problem behaviors, such as Internet Addiction (IA), and academic values, including intrinsic and utility values, could predict satisfaction with academic performance. However, the influence of IA and academic values has not been thoroughly explored in Chinese contexts where the pressure for academic success is heavy. This study examined the relationships between IA, academic values (intrinsic and utility value), and satisfaction with academic performance using two waves of data collected from secondary school students in four cities in mainland China. The matched sample included a total of 2,648 Grade 7 or 8 students (57.1% were boys with a mean age of 13.1 years at Wave 1). Participants completed the same questionnaire containing validated measures at both waves with a 1-year interval. In line with the hypotheses, multiple regression analyses showed that Wave 1 IA was a significant negative predictor of Wave 2 intrinsic value, utility value, and satisfaction with academic performance and their changes. Results of mediation analyses revealed that only intrinsic value, but not utility value, positively predicted satisfaction with academic performance. Structural equation modeling (SEM) analyses also showed similar findings. Two observations are concluded from the present findings: IA impaired students’ intrinsic value, utility value, and perceived satisfaction with academic performance; two aspects of academic values demonstrated different influences on satisfaction with academic performance. These findings provide implications for the promotion of academic satisfaction experienced by students and the prevention of negative effects of IA.
Introduction
The Internet has significantly changed people’s lives nowadays. Despite the profound benefits of the Internet, the public is aware of the negative influence of its overuse of misuse on health and well-being. One common problem is Internet addiction (IA), which refers to one’s inability to control Internet use that consequently causes social, psychological, academic, and work difficulties in life ( Chou and Hsiao, 2000 ). IA has drawn growing concerns of the public and professionals worldwide.
Among different age groups, adolescents are considered more vulnerable to IA as their cognitive ability, self-control, and coping strategies are not fully developed ( Long et al., 2018 ). Many studies have revealed that adolescents have a higher tendency to develop addictive behaviors such as playing online games or using social media in comparison to adults ( Long et al., 2018 ). As the Internet penetration rate has dramatically increased nowadays, more than 80% of the adolescent population in the United Kingdom, United States, and Asia can access the Internet ( Cerniglia et al., 2017 ). According to a national report, around 940 million Chinese people were Internet users, and among them were 172 million children and adolescents ( China Internet Network Information Center, 2020 ). Research has revealed a relatively high prevalence of IA among Chinese adolescents. Shek et al. (2008) conducted research with 6,121 Chinese primary and high school students in Hong Kong, revealing that around 20% of the respondents met the criteria for IA based on two assessment measures. The study of Tan et al. (2016) involving 1,772 high school students in southern China also showed that around 17.2% of participants demonstrating problematic Internet use.
Many studies have documented the negative impact of IA on different aspects of adolescent development, such as sleeping quality ( Tan et al., 2016 ), mental health ( Ko et al., 2012 ), subjective well-being ( Allen and Anderson, 2018 ), social development ( Cerniglia et al., 2017 ), emotional development ( Truzoli et al., 2020 ), and interpersonal relationship ( Zeng et al., 2021 ). For adolescents, IA is particularly associated with low levels of school performance. Empirical evidence showed that students with IA experience more academic failure than their counterparts ( Nemati and Matlabi, 2017 ). For example, students’ online gambling habits were positively related to low levels of school achievements and less prosocial behaviors ( Floros et al., 2015 ). Online pornography watching also impaired adolescents’ academic performance as it reduces their interest, concentration, and involvement in academic activities ( Beyens et al., 2015 ). Similar results were found among Chinese adolescents. For example, a longitudinal study examined the relationship between Internet behavior and students’ academic development based on a sample of 9,949 Chinese students revealed that IA could lead to lower academic achievement, dropout, and absenteeism ( Anthony et al., 2021 ). Another study evaluating IA and negative emotions also reported that IA negatively influenced academic problems by undermining students’ mental and psychological health ( Bu et al., 2021 ). The study of Bai et al. (2020) based on 1,794 adolescents from low-income families in China revealed that IA was linked to depression and detrimental to students’ academic performance.
In Chinese schools, students are evaluated publicly by peers and teachers in terms of whether their behavioral and academic performance reaches school standards, which largely influences students’ psychological health and adjustment ( Chen et al., 2012 ). Undoubtedly, academic performance is considered the most important standard in Chinese school context. Researchers have adopted different approaches to assess academic performance. Primarily, test scores are considered an objective indicator of academic performance and have been often used in previous studies. Although the use of test scores is helpful to suggest education improvement and school accountability, researchers have questioned whether test scores reflect the stable status of individual students’ overall development ( Goldhaber and Özek, 2019 ). An alternative is to use subjective indicators, such as perceived performance level, which reflects one’s overall subjective evaluation of normative performance level compared to peers ( Saw et al., 2016 ). Researchers have pointed out the importance of subjective perceptions of one’s academic performance for its close association with students’ psychological adjustment ( Haraldsen et al., 2020 ). Researchers also argued that satisfaction with perceived academic performance as an element of school adjustment provides a better indication of one’s appraisal of academic achievement in schools ( Shek, 2002 ). Research has shown that dissatisfaction with one’s academic performance constitutes developmental problems for adolescents, particularly when the failure occurs repetitively ( Enns et al., 2001 ; Lee et al., 2016 ). As the present study was interested in the roles of perceived academic values and motivation, we used satisfaction with academic performance as the indicator.
Scientific studies have been conducted to unravel the mechanisms of the negative impacts of IA on academic performance among adolescents. Earlier research has focused on the distraction and divergence behaviors in learning among students with IA, which often directly lead to a decline in school performance. Besides, anxiety and depression have been found to mediate the adverse effect of IA on academic performance ( Ko et al., 2012 ; Bai et al., 2020 ; Bu et al., 2021 ). Recent evidence suggests that IA may also interrupt students’ psychological learning process and create problems in academic values and motivation ( Reed and Reay, 2015 ; Truzoli et al., 2020 ). For example, problematic Internet use was found to exert a negative effect on academic motivation, learning productivity, and psychosocial status, which have negative effects on academic performance ( Truzoli et al., 2020 ).
Academic motivation includes intrinsic value and utility value ( Eccles, 1983 ; Neel and Fuligni, 2013 ). Intrinsic value involves a sense of satisfaction rooted in the study or learning procedure itself, while utility value refers to students’ sense of the instrumental value of the school courses (such as getting higher grades or material rewards) rather than finding the courses interesting. Ryan and Deci (2000) also categorized motivation into intrinsic motivation and extrinsic motivation. Intrinsic motivation refers to an individuals’ aspiration for doing something from the inner heart, while extrinsic motivation defines the concept of getting rewards from outside to stimulate someone to behave ( Benabou and Tirole, 2003 ).
Previous studies have found that IA may impair intrinsic value, as studying is often not as attractive as surfing the Internet ( Hanus and Fox, 2015 ; Reed and Reay, 2015 ). The various attractive and interesting sensory stimulations derived from the Internet could undermine students’ learning interest, self-control, and self-efficacy in learning. Wang et al. (2021) argued that problematic use of short-form video applications was associated with a sole focus on immediate hedonic rewards and a lack of understanding of future harmful consequences. The research of Anthony et al. (2021) found a close relationship between IA and a lack of interest in school learning. A study conducted with Chinese students also revealed the mediating role of intrinsic motivation in the positive relationship between social media use and academic performance ( Malik et al., 2020 ). Previous studies have mainly focused on the negative influence of IA and intrinsic value but paid less attention to the relationships between IA and utility value. Theoretically speaking, IA could also undermine utility value or extrinsic motivation as the intensive reinforcement and reward schedules in Internet activities (e.g., online games) provide instant extrinsic rewards to adolescents ( Truzoli et al., 2020 ), while students may not necessarily receive instant extrinsic rewards (e.g., high grades or praise) even if they study hard.
Although IA has been commonly considered a risk factor for academic values and performance, how the two types of academic values are associated with performance are less conclusive. Theoretically speaking, intrinsic value promotes academic performance through positive and active engagement in learning with enjoyment, autonomy, deep learning, task arrangement, and time spending in learning ( Vansteenkiste et al., 2005 ; Froiland and Oros, 2014 ; Liu et al., 2020 ). Intrinsic value is considered to have a relatively long-term effect on academic performance because it reinforces students’ self-concepts and values, which are vital for students to maintain healthy psychological status and deal with academic failures ( Cheo, 2017 ). On the contrary, utility value is constrained by the existence of external rewards and thus believed to have an instant but short-term positive effect on academic performance. In other words, once external rewards are terminated, utility value may become ineffective in stimulating adolescents’ continuous efforts into their study.
However, empirical evidence supporting the distinctive effects of the two types of values has been equivocal. For example, the study of Baker (2004) on university students found no significant relationships between intrinsic or extrinsic motivation and academic achievement. Some studies revealed that both intrinsic and utility value were positively linked to school performance ( Afzal et al., 2010 ). Some other studies revealed differential effects of intrinsic and utility value on academic outcomes ( Moneta and Siu, 2002 ). For example, a longitudinal study conducted with 13,799 Chinese high school students revealed the different effects of intrinsic and utility value on academic performance. Students with high levels of intrinsic value were more attentive, focused on learning interests, arranged flexible learning strategies, and spent more time learning to improve their academic performance ( Liu et al., 2020 ). It was argued that utility value might undermine the academic performance of students with high intrinsic value because utility value made students feel of being controlled, which damaged ones’ intrinsic values ( Wang and Guthrie, 2004 ; Liu et al., 2020 ). Similarly, Kuvaas et al. (2017) found that intrinsic value was positively associated with better performance, while extrinsic motivation showed a modest negative effect on performance. These inconclusive findings call for further exploration on how academic values might be differently related to adolescent development.
This study aimed to fill some research gaps. First, this study explored the effects of IA on perceived satisfaction of academic performance and the mediating roles of both intrinsic and utility values. This helps reveal the underlying mechanism of the influence of IA on academic performance and clarify the function of two types of academic values, which would fill the above-mentioned theoretical gaps. Second, most existing studies on adolescent IA and academic outcomes have been conducted with Western samples, hence calling for devoting more efforts to these issues in non-Western societies, particularly in Chinese contexts ( Shek et al., 2008 ; Shek and Yu, 2016 ). As academic excellence is highly emphasized in Chinese societies, academic motivation may be perceived differently. In fact, both intrinsic and utility values are emphasized in traditional Chinese culture. Regarding intrinsic value, Confucian stated that “wasn’t it a pleasure to learn and practice often?” (“xue er shi xi zhi, bu. yi yue hu?”) in the Analects of Confucius, highlighting the satisfaction of learning, practical application, and self-improvement ( Waley, 2005 ). As to utility value, the Chinese saying, “one who excels in the study can follow an official career” (“xue er you ze shi”), emphasizes the benefits of academic excellence in future career development. In contemporary Chines societies, “an official career” may no longer be the ultimate goal of studying. However, the value of education still receives great recognition among the public despite the development of ideology and philosophy in China ( Wang and Ross, 2010 ). At the national level, China’s Education Modernization 2035 plan sets the direction for developing the education sector to strengthen its overall capacity and international influence and makes China a powerhouse of education, human resources, and talents. At the family and individual levels, parents and students believe that “knowledge changes fate” and thus highly emphasize academic success ( Xiang, 2018 ). Third, as most studies have not collected longitudinal data, it is difficult to establish the causal relationships between IA and academic performance. In particular, although longitudinal studies have examined the antecedents of IA (e.g., Yu and Shek, 2013 ), limited research has examined the longitudinal prediction of IA on adolescent developmental outcomes in Chinese adolescents. This research aims to understand the relationship between IA and academic performance and examine the mediating role of academic motivation (intrinsic and utility values) in this relationship using two waves of data.
Research Hypotheses
Based on the literature, we proposed the following hypotheses for each research question.
Research Question 1 (RQ1)
What are the concurrent and longitudinal relationships between IA and academic motivation? Based on the previous findings ( Truzoli et al., 2020 ), we proposed that IA would be negatively associated with intrinsic value concurrently (Hypothesis 1a) and longitudinally (Hypothesis 1b). Besides, with reference to the existing literature ( Ryan and Deci, 2000 ; Truzoli et al., 2020 ), we expected negative concurrent and longitudinal relationships between IA and utility value (Hypotheses 1c,d, respectively).
Research Question 2 (RQ2)
What are the concurrent and longitudinal relationships between IA and satisfaction with academic performance? In line with studies conducted with Chinese students ( Anthony et al., 2021 ), we proposed that IA would be negatively related to satisfaction with academic performance concurrently (Hypothesis 2a) and longitudinally (Hypothesis 2b).
Research Question 3 (RQ3)
What are the concurrent and longitudinal relationships between academic motivation and satisfaction with academic performance? In line with previous research ( Anthony et al., 2021 ), we proposed that intrinsic value would be positively linked to satisfaction with academic performance concurrently (Hypothesis 3a) and longitudinally (Hypothesis 3b). Similarly, utility value would also show positive associations with satisfaction with academic performance concurrently (Hypothesis 3c) and over time (Hypothesis 3d).
Research Question 4 (RQ4)
Does academic motivation mediate the relationship between IA and satisfaction with academic performance? According to previous studies suggesting the mediating role of academic motivation ( Malik et al., 2020 ), we hypothesized that intrinsic value and utility value would mediate the impact of IA on satisfaction with academic performance (Hypotheses 4a,b, respectively).
Materials and Methods
Participants and procedure.
The data of this study were derived from a project examining adolescent adjustment and development in mainland China. The participants were recruited from four junior high schools in three provinces. Two waves of data were collected at the beginning of the school year of 2016/2017 (Wave 1) and 1 year later (Wave 2). A survey questionnaire was administered to students during school hours. Students were informed of the research aims, data collection, and the principles that the data collected will be anonymous, confidential, and only used for academic purposes. We obtained written consent from students, their parents, teachers, and school heads before data collection. This study has been reviewed and granted ethical approval by the authors’ university.
In total, 3,010 students completed the questionnaire at Wave 1. Among them, 1,362 were in Grade 7, and 1,648 were in Grade 8. The data at Wave 2 were collected from 2,648 students, including 1,305 Grade 8 students and 1,343 Grade 9 students. The matched sample consisted of 2,648 students (Boys = 1,513; Girls = 1,109) with a mean age of 13.12 years at Wave 1. The attrition rates were 4.2 and 18.5% for Grade 7 and Grade 8 students, respectively, which were more favorable compared with studies reported in longitudinal studies with adolescents ( Epstein and Botvin, 2000 ). Results of attrition analysis revealed non-significant differences between students in the matched sample ( n = 2,648) and the dropouts ( N = 362) in terms of age, IA, intrinsic and utility values, and satisfaction with academic performance in both grades.
Internet Addiction
The Chinese version of the Internet Addiction Scale developed by Young (1998) was adopted to evaluate participants’ IA symptoms. This scale has been used and validated in previous studies and showed good psychometric properties ( Shek et al., 2008 ; Yu and Shek, 2013 ; Chi et al., 2020 ). It includes 10 items assessing different IA symptoms, such as “Have you lied to family members, teachers, social workers, or others to conceal the extent of involvement with the Internet?” Participants indicated whether they exhibited each of the symptoms in the past 12 months on a dichotomous scale (i.e., yes/no). The total score equals the counts of “yes” answers to 10 questions. The values of Cronbach’s α of IA were 0.77 at Wave 1 and 0.80 at Wave 2.
Academic Values
Students’ academic values were measured via two aspects, including intrinsic value and utility value ( Eccles, 1983 ; Neel and Fuligni, 2013 ). This scale has been validated in the Chinese context ( Guo et al., 2017 ). Intrinsic value depicts how students perceive schoolwork as interesting and how much they like schoolwork in general. It includes two items: “In general, I find working on schoolwork is…” (1 = “very boring” and 5 = “very interesting”) and “How much do you like working on schoolwork?” (1 = “a little” and 5 = “a lot”). On the other hand, the utility value describes the perceived usefulness of schoolwork through three items: “Right now, how useful do you find things you learn in school to be in your everyday life,” “In the future, how useful do you think the things you have learned in school will be in your everyday life?” and “How useful do you think the things you have learned in school will be for what you want to be after you graduate” on a five-point Likert scale (1 = “not useful at all” and 5 = “very useful”). The Cronbach’s α estimates for the two scales ranged between 0.87 and 0.91 at the two waves, suggesting good internal consistency of the scales in this study.
Satisfaction With Academic Performance
Satisfaction with academic performance was measured by a single item, “I am satisfied with my academic performance as compared to my classmates,” on a six-point reporting scale (“1 = strongly disagree”; “6 = strongly agree”). This item was developed by authors based on literature ( Education and Manpower Bureau, 2003 ) and has been used in previous studies ( Shek, 2002 ).
Data Analysis
We first conducted descriptive analyses. Table 1 summarizes the means, SDs, and correlations among variables. Hierarchical multiple regression analyses were conducted to examine the concurrent and longitudinal relationships between research variables (RQ1, RQ2, and RQ3). This approach has been commonly adopted in the field ( Zhou et al., 2020 ; Dou and Shek, 2021 ). Particularly, we examined the longitudinal effects of IA at Wave 1 on academic outcomes at Wave 2 with the corresponding outcomes at Wave 1 controlled. By controlling the influence of the initial levels of academic outcomes, this method suggests the effect of the predictor variables on the dependent variables over time.
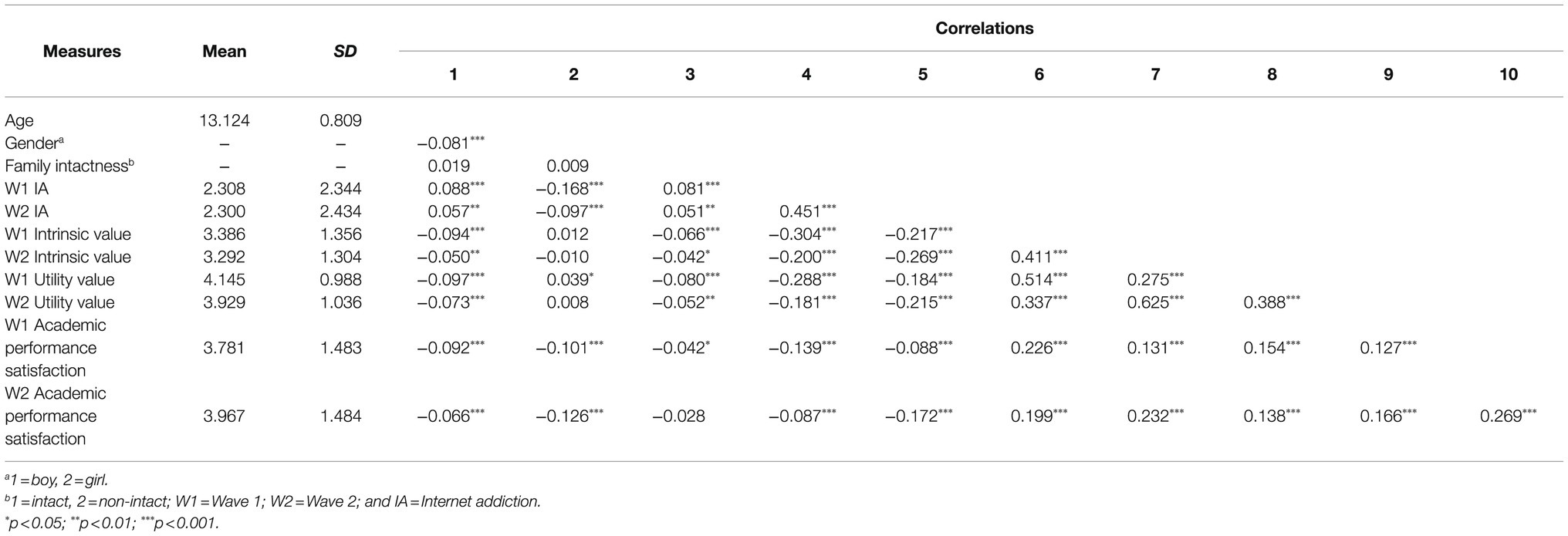
Table 1 . Descriptive and correlational analyses.
For RQ4, we first analyzed the mediational role of intrinsic and utility value through a series of regression models using PROCESS macro in SPSS ( Hayes, 2017 ). We calculated bias-corrected (BC) bootstrap 95% CIs using 2,000 re-samplings in the mediation analyses ( Hayes, 2017 ). We first examined the mediating effects of intrinsic and utility values in two models separately, and then simultaneously added them to one model. This conservative method is helpful to explore the relationships between research variables in line with research questions and also suggest potential interactions. Besides, we used Structural Equation Modeling (SEM) to test the complete hypothesized model via Lavaan package in R software ( Rosseel, 2012 ). SEM models can accommodate latent variables, multiple predictors, and outcomes, which allow a comprehensive analysis of the relationships between research variables. Multiple indices were used to indicate model goodness of fit, including Comparative Fit Index (“CFI”), Tucker-Lewis Index (“TLI”), Root Mean Square Error of Approximation (“RMSEA”), and Standardized Root Mean Square Residual (“SRMR”). Based on Hu and Bentler (1999) and Kline (2015) , the cutoff criteria should be above 0.90 for CFI and TFI values, and lower than 0.08 for RMSEA and SRMR values.
Descriptive Results and Correlations
Table 1 shows the means, SDs, and correlations for IA, intrinsic value, utility value, and satisfaction with school performance over the two time points. The correlations between the research variables were significant and in line with the hypotheses. IA was negatively associated with intrinsic and utility value concurrently and longitudinally ( r ranged between −0.20 and −0.30, p s < 0.001), and was negatively correlated with satisfaction with academic performance at each wave ( r s ranged between −0.09 and −0.17, p s < 0.001). Both intrinsic and utility values were positively correlated with satisfaction with academic performance at two waves ( r s ranged between 0.127 and 0.232, p s < 0.001).
Predictive Effects of IA on Academic Values
Results of hierarchical multiple regression analyses revealed significant concurrent negative effects of IA on intrinsic value (Wave 1: b = −0.30, p < 0.001, Cohen’s f 2 = 0.096; Wave 2: b = −0.27, p < 0.001, Cohen’s f 2 = 0.080, see Table 2 ) and utility value (Wave 1: b = −0.28, p < 0.001, and Cohen’s f 2 = 0.079; Wave 2: b = −0.21, p < 0.001, and Cohen’s f 2 = 0.046, see Table 3 ) at each wave after controlling gender, age, and family intactness. As to the longitudinal effect, Wave 1 IA had significant longitudinal effects on Wave 2 intrinsic value ( b = −0.21, p < 0.001, and Cohen’s f 2 = 0.042, see Table 4 ) and Wave 2 utility value ( b = −0.28, p < 0.001, and Cohen’s f 2 = 0.079, see Table 5 ). Additionally, after controlling Wave 1 intrinsic and utility values, IA at Wave 1 significantly predicted a decrease in both academic values over time ( b was −0.09 and −0.21, p s < 0.001, and Cohen’s f 2 was 0.007 and 0.046 for intrinsic and utility value, respectively, see Tables 4 , 5 ). Hypotheses 1a, 1b, 1c, and 1d were supported.
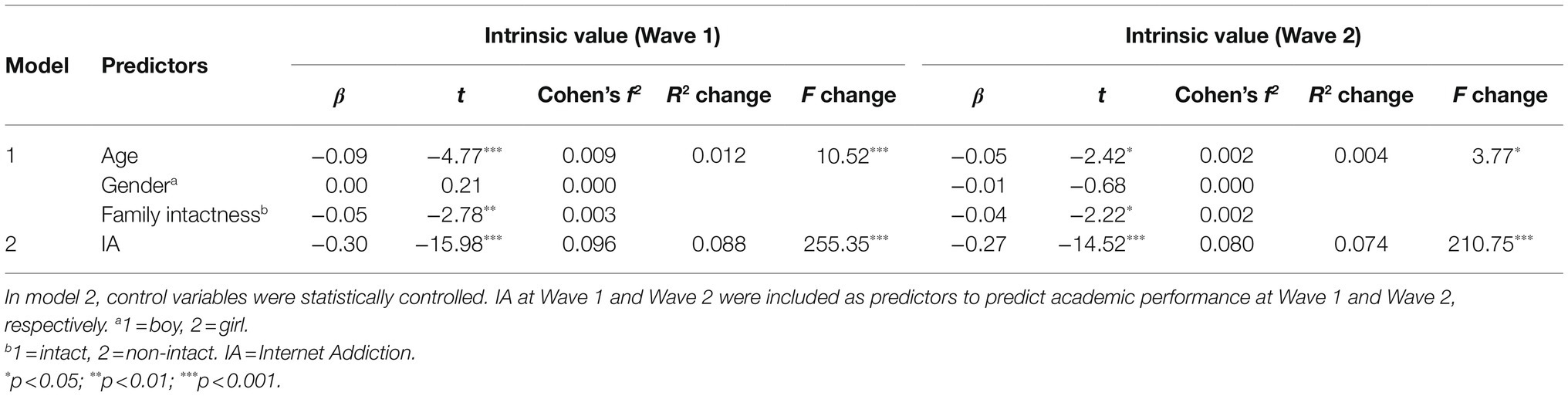
Table 2 . Cross-sectional regression analyses for intrinsic value.
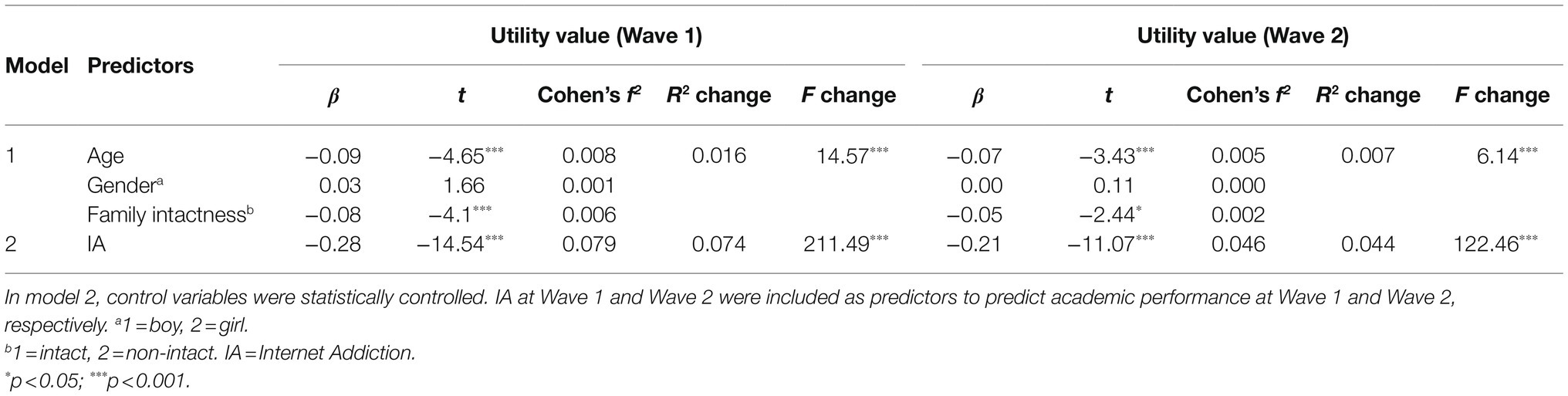
Table 3 . Cross-sectional regression analyses for utility value.
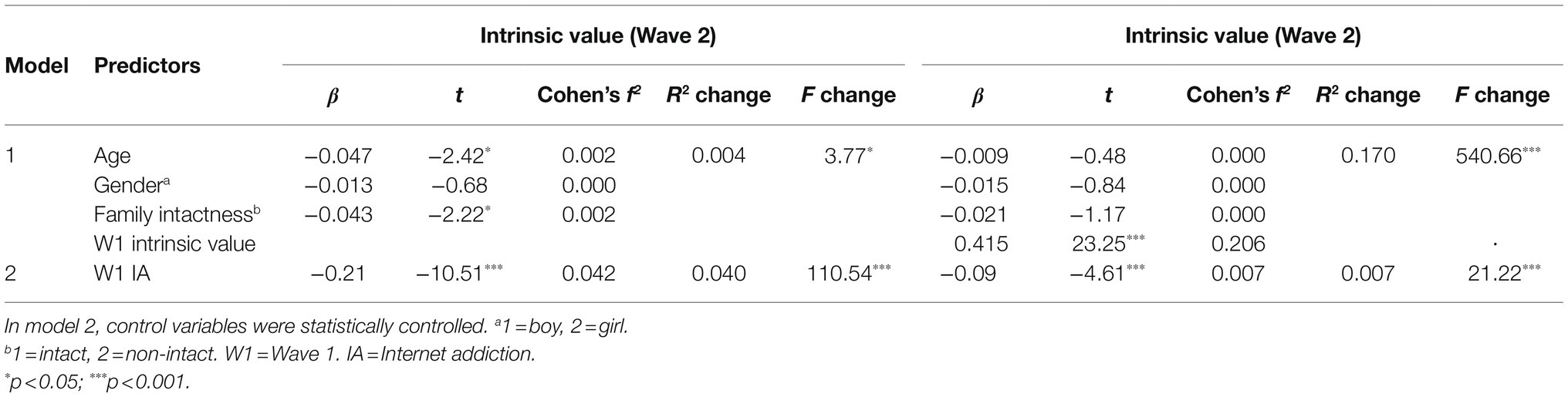
Table 4 . Longitudinal regression analyses for intrinsic value.
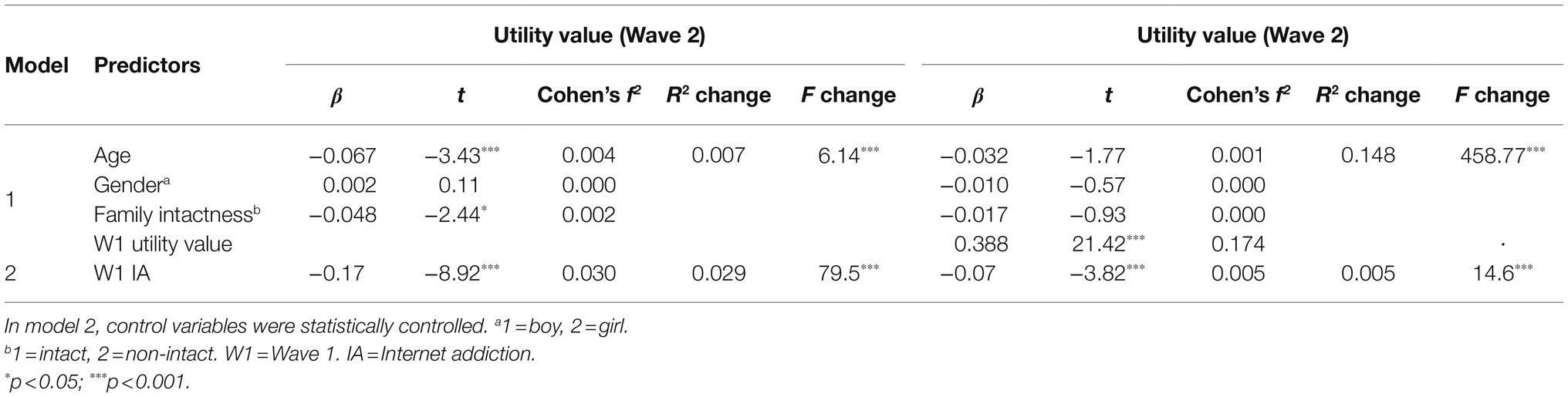
Table 5 . Longitudinal regression analyses for utility value.
Predictive Effects of IA on Satisfaction With Academic Performance
Results of multiple regression analyses demonstrated that IA had a significantly negative influence on satisfaction with academic performance at each wave ( b was −0.15 and − 0.18, p s < 0.001, and Cohen’s f 2 was 0.022 and 0.034 for Wave 1 and 2, respectively, see Table 6 ). In addition, IA showed significant and negative prediction on Wave 2 satisfaction with academic performance ( b = −0.11, p < 0.001, and Cohen’s f 2 = 0.011, see Table 6 ). After controlling Wave 1 satisfaction with academic performance, IA significantly predicted a decrease in satisfaction with academic performance ( b = −0.07, p < 0.001, and Cohen’s f 2 = 0.004, see Table 6 ). Hypotheses 2a and 2b were supported.
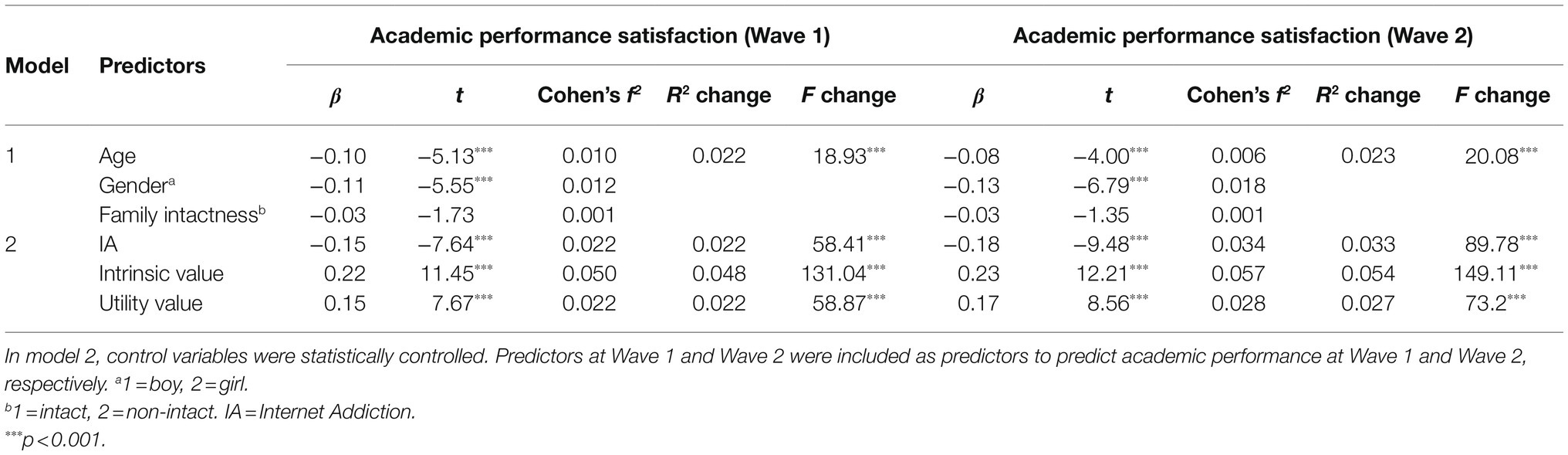
Table 6 . Cross-sectional regression analyses for academic performance.
Predictive Effects of Academic Values on Satisfaction With Academic Performance
Results of multiple regression analyses revealed that intrinsic value and utility value positively predicted each wave’s satisfaction with academic performance ( b ranged between 0.15 and 0.23, p s < 0.001, Cohen’s f 2 ranged from 0.022 to 0.057, see Table 6 ). Results also showed a longitudinal prediction of intrinsic value and utility value on satisfaction with performance ( b = 0.20 and 0.14, p s < 0.001, and Cohen’s f 2 = 0.039 and 0.019 for intrinsic and utility value, respectively, see Table 7 ). Moreover, both intrinsic and utility values predicted an increase in Wave 2 satisfaction with academic performance when Wave 1 satisfaction was controlled ( b = 0.15 and 0.10, p s < 0.001, and Cohen’s f 2 = 0.020 and 0.010 for intrinsic and utility value, respectively, see Table 7 ). Results supported Hypotheses 3a, 3b, 3c, and 3d.
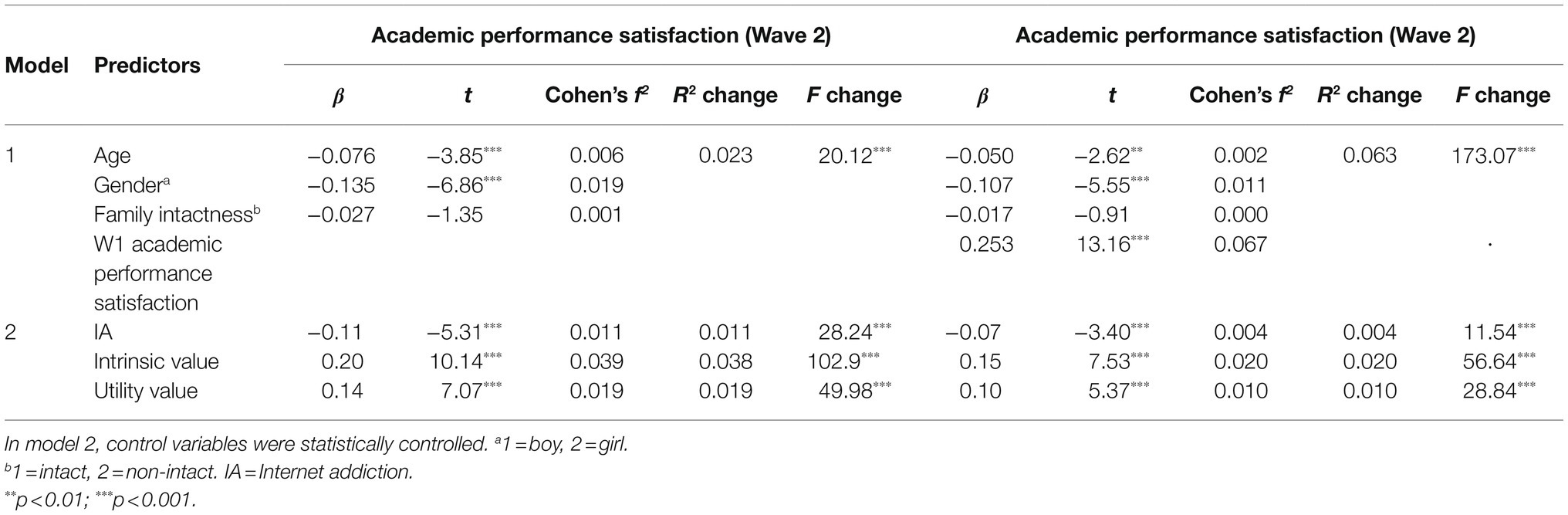
Table 7 . Longitudinal regression analyses for academic performance.
Mediating Roles of Academic Values
Results of mediation analyses via PROCESS are summarized in Table 8 . When intrinsic and utility values were examined in two separate models, results revealed significant mediating effects of both intrinsic value (see Model 1a in Table 8 ) and utility value (see Model 1b in Table 8 ). When they were added to the model simultaneously, results showed that IA at Wave 1 negatively predicted intrinsic value and utility value at Wave 2. However, only intrinsic value, not utility value, positively predicted satisfaction with academic performance, suggesting the potential mediating effect of intrinsic value only (see Model 2 in Table 8 ). The indirect effect of IA on academic performance via intrinsic value was significant ( b = −0.03, p < 0.001, see Model 2 in Table 8 ). The mediating effect of utility value was not significant (see Model 2 in Table 8 ).
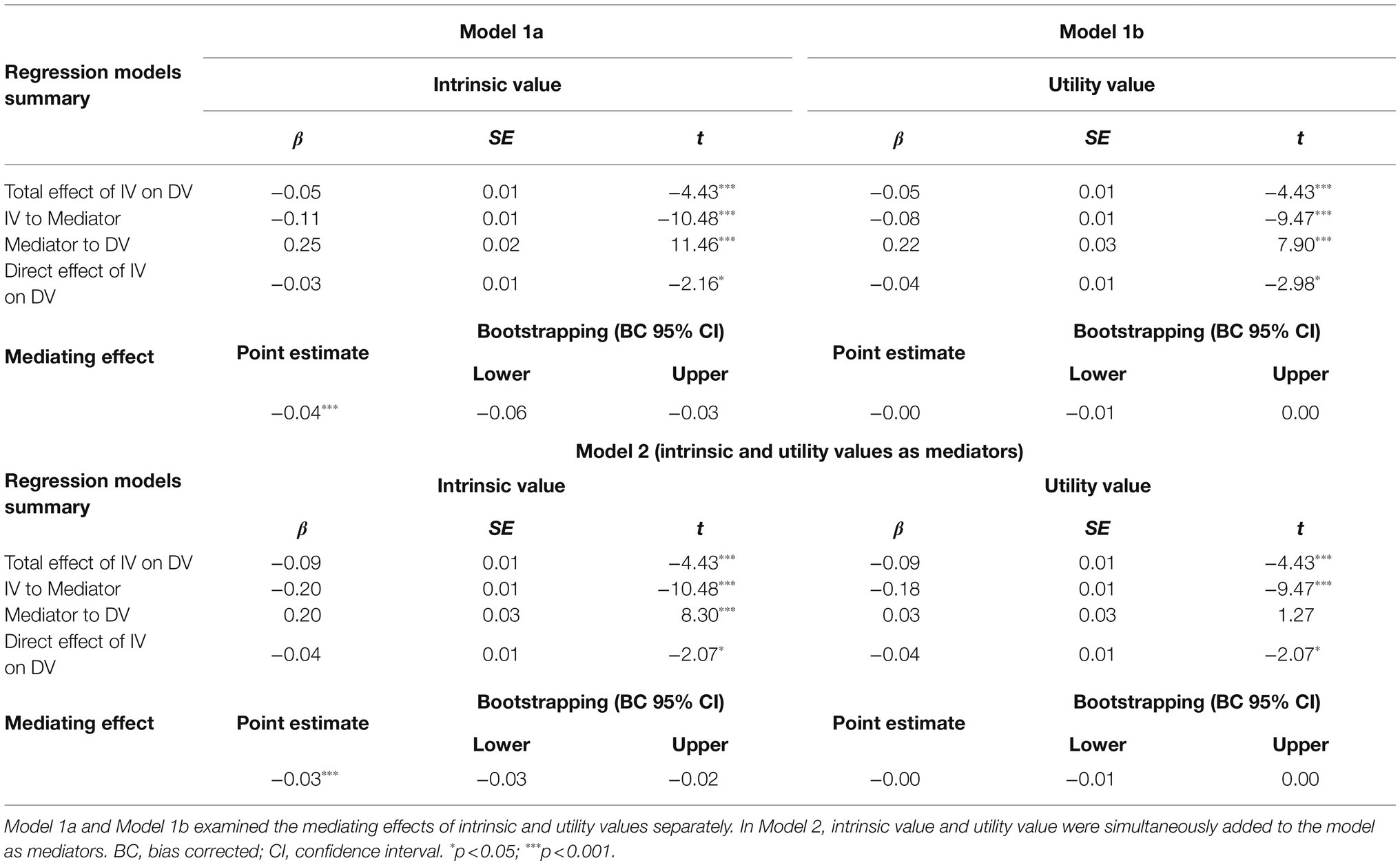
Table 8 . Longitudinal mediating effect analyses of intrinsic value and utility value at Wave 2 (the mediators) for the effect of IA at Wave 1 on academic performance satisfaction at Wave 2.
We further developed a SEM model to comprehensively understand the relationships between variables under investigation (see Figure 1 ). The SEM model included IA at Wave 1 and satisfaction with academic performance at Wave 2 as observed variables, and intrinsic and utility values at Wave 2 as latent variable. The SEM model showed adequate model fit ( χ 2 = 47.243, df = 9, CFI = 0.996, TFI = 0.990, NNFI = 0.990, RMSEA = 0.040, and SRMR = 0.011; Kline, 2015 ). Figure 1 shows the standardized coefficients in this model. IA at Wave 1 significantly and negatively predicted Wave 2 intrinsic value ( β = −0.09, p < 0.001), utility value ( β = −0.08, p < 0.001), but not satisfaction with academic performance ( p = 0.064). Wave 2 intrinsic value, but not utility value, demonstrated a significant and positive prediction on academic performance ( β = 0.33, p < 0.001). Results of SEM were in line with the PROCESS findings, which supported Hypothesis 4a but rejected Hypothesis 4b.
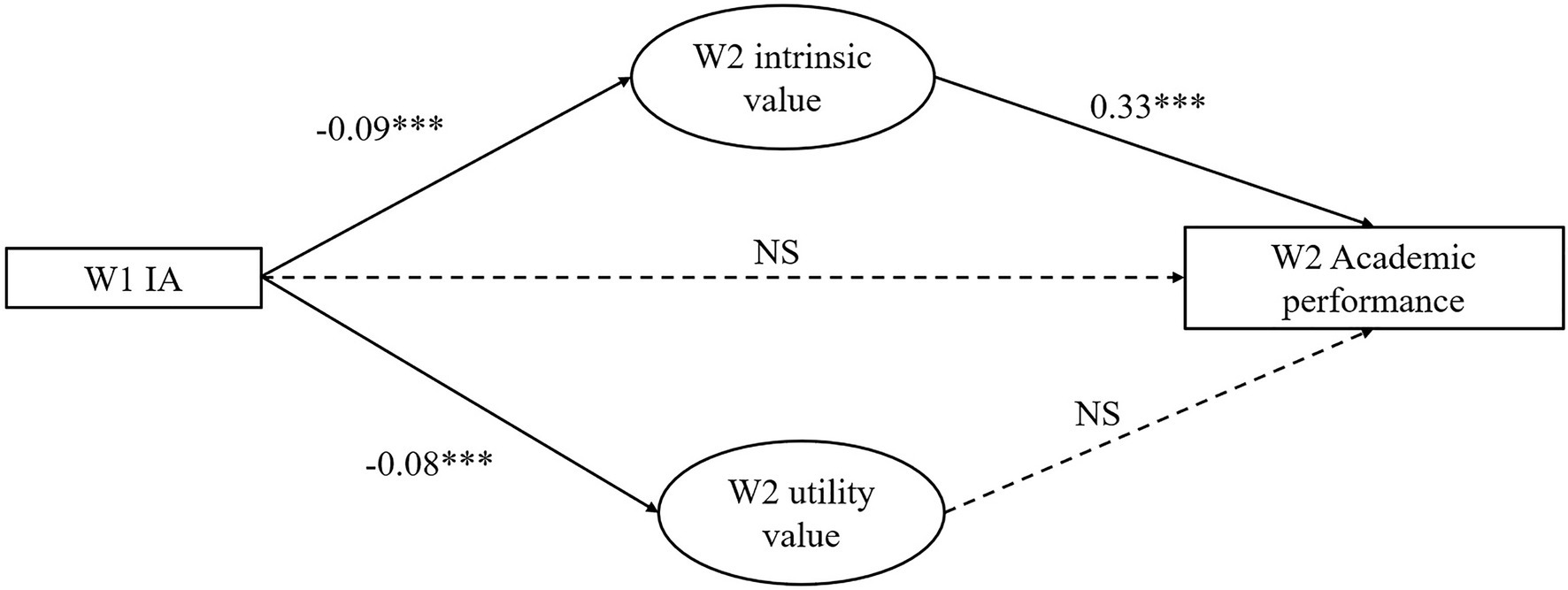
Figure 1 . Results of Structural equation modeling (SEM) model. *** p < 0.001.
In this study, we examined the predictive effect of IA on satisfaction with academic performance, with academic values hypothesized as mediators. With reference to the research gaps in the literature, this study has several strengths. First, instead of focusing on objective academic performance indexed by test scores, we adopted students’ satisfaction with academic performance, an indication of students’ appraisal of overall academic achievement, to better understand the research questions concerning students’ psychological motivation and values. Second, this study examined two potential mechanisms through which having IA symptoms potentially predict students’ satisfaction with academic performance through intrinsic and/or utility value. Third, a short-term longitudinal design was used to understand the predictive effects of IA on satisfaction with academic performance. Fourth, we employed a relatively large sample to enhance the generalizability of the findings. Fifth, as very few studies in this field have been conducted in the Chinese context, this study contributes to the understanding of the negative influence of IA on academic performance and the underlying mechanisms in an educational system that highly emphasizes academic success. Finally, analyses based on both multiple regression and SEM were used to address research questions in a comprehensive manner.
Findings based on multiple regression analyses generally support the proposed hypotheses, which are consistent with the existing literature. First, IA negatively predicted satisfaction with academic performance and its change over time. The findings support previous evidence suggesting negative associations between IA and academic performance ( Nemati and Matlabi, 2017 ; Anthony et al., 2021 ; Bu et al., 2021 ). Second, IA positively predicted both intrinsic and utility values and the changes over time. These findings are also in line with previous studies revealing negative influences of problem Internet use on students’ learning motivation ( Truzoli et al., 2020 ; Anthony et al., 2021 ). Third, results of multiple regression showed that both intrinsic and utility values positively predicted satisfaction with academic performance and its change over time. Although some previous studies have emphasized the downside of utility value on adolescent development, the results of the present study corroborate previous evidence highlighting the positive influence of both intrinsic and utility values ( Afzal et al., 2010 ). As mentioned earlier, Chinese cultures acknowledge both intrinsic and utility values of study. Although the education system in China has been criticized for the examination orientation, it is still perceived as the most accessible and fair approach for disadvantaged students to beat the odds and seek academic access ( Wang and Ross, 2010 ). For these students, schooling means much more than individual interests or satisfaction but “a future of comfort and dignity, a family responsibility and collective investment, and a path toward individual freedom and actualization” ( Xiang, 2018 , p. 81). These beliefs reflect instrumental value but are also rooted in spirits of hard-working and persistence that are vital for academic success. Finally, when both intrinsic and utility values were included in the mediation models, only intrinsic value, but not utility value, served as a mediator in the relationship between IA and satisfaction with academic performance. Results based on multiple regression and SEM are consistent, which generate triangulated findings for the study. The results are consistent with the widely held belief that intrinsic and utility values are distinct constructs and have different associations with adolescents’ maladjustment and psychological well-being ( Moneta and Siu, 2002 ). Students demonstrating more IA symptoms tended to regard school work as boring and consequently felt less satisfied with their academic performance, which is in agreement with previous findings ( Liu et al., 2020 ). Additionally, the mediating effect of utility value was not significant when intrinsic value was taken into account. One explanation is that utility value may include different subtypes depending on how one internalizes the extrinsic goals as a personal pursuit. If students regard the striving for performance excellence as a personal commitment, it reflects high levels of autonomy and self-determination ( Ryan and Deci, 2000 ). As results of the correlational analysis revealed a significant positive association between intrinsic and utility value, students may accept the utility of schooling and endorse the external goals. This finding echoes the idea that intrinsic value has an immediate effect on study performance, while ulitity value contributes to performance through its close association with intrinsic motivation ( Wang and Guthrie, 2004 ). We should also investigate the linkages between the two types of academic value in future studies.
There are several theoretical implications of the present findings. First, the study suggests that the negative effects of IA on academic values and satisfaction with academic performance concurrently and over time, which strengthens the theoretical proposition that IA has longitudinal adverse effects on academic outcomes ( Zhang et al., 2018 ). Second, the results underscore the importance of academic values, particularly intrinsic value, in mediating the influence of IA on satisfaction with academic performance. Students possessing high levels of intrinsic value perceive learning as exploratory, playful, and curiosity driven. According to Self-determination Theory ( Ryan and Deci, 2000 ), intrinsic value serves as “a natural wellspring of learning.” However, many online activities, including short videos, social media networks, and online games, have been designed or presented to be mentally stimulating to give users high levels and continual enjoyment ( King and Delfabbro, 2018 ). Students’ basic psychological needs for competence, autonomy, and relatedness may be better satisfied by Internet use rather than by traditional learning activities, which may lead to a decrease in their engagement in school work and an increase in Internet use ( Salmela-Aro et al., 2017 ; Zhang et al., 2018 ). As existing research has paid much attention to the direct relationship between the Internet and academic performance, our results highlight the importance of examining how psychological factors mediate the relationship between adolescent problem behaviors and their development and well-being in the long run.
The finding has practical implications for teachers and social workers to help adolescents and their parents understand the negative consequences of IA in undermining academic values (i.e., meaning of study) and academic performance. Given that many teaching and learning activities are online nowadays, adolescents and parents commonly hold the belief that Internet is an indispensable part of life, and thus it cannot be addictive and the “prolonged” use of IA is not problematic. Instead, adolescents should be aware of the potential dark side of Internet use, such as the adverse effects of IA on academic values and perceived school performance ( Salmela-Aro et al., 2017 ). Furthermore, to promote satisfaction with academic performance, we need to cultivate the meaning of studying in students. In school practices, it is trendy for teachers to adopt various pedagogical strategies to spark students’ intrinsic value and cultivate active learners. Utility value, on the contrary, is often regarded as ineffective or even detrimental in adolescent development and is often associated with unhealthy teaching or parenting styles, such as excessive involvement ( Rivers et al., 2012 ). As Benabou and Tirole argued, “external incentives are weak reinforcers in the short run, and negative reinforcers in the long run” (2003, p. 489). However, our results did not reveal any negative associations between utility value and intrinsic value or academic performance. As suggested by Lin et al. (2003) , we believe it is important that teachers and parents need not eliminate all perceived utility values for high performance, especially when students accepting utility value of schooling based on a sense of commitment and self-determination.
There are several limitations of the study. First, because only two waves of data were collected, the findings are based on a short-term longitudinal study. As such, more time points should be added in future studies. Second, the scale of academic values only included a few items for the two types of values. As suggested by Ryan and Deci (2000) , it is meaningful to explore different subtypes of extrinsic motivation based on the perceived locus of causality. We recommend that more items and subtypes of utility value should be examined in future studies. Third, the present study only adopted a subjective indicator of academic performance. We believe satisfaction with performance better reflects adolescents’ self-evaluation on schooling and is closely associated with their psychological well-being. Although satisfaction with academic performance is closely correlated with GPA ( Bradley, 2006 ), it would be helpful to include test scores and/or teacher-rated performance in future studies. Fourth, this study mainly focused on academic values as mediators. Other important factors, such as academic stress, could be taken into account in future studies ( Baker, 2004 ). Finally, only self-report data were collected, which may lead to common-method variance bias. Future studies should use multiple informants’ reports to assess adolescent IA symptoms and academic performance.
Data Availability Statement
The raw data supporting the conclusions of this article will be made available by the authors, without undue reservation.
Ethics Statement
The studies involving human participants were reviewed and approved by Human Subjects Ethics Subcommittee at The Hong Kong Polytechnic University. Written informed consent to participate in this study was provided by the participants’ legal guardian/next of kin.
Author Contributions
DS designed the research project and contributed to all the steps of the work. DD conducted data analyses, prepared the first draft, and revised the manuscript based on the comments and editing provided by DS. All authors contributed to the article and approved the submitted version.
This paper and the two-wave longitudinal study in the Tin Ka Ping Project P.A.T.H.S. were financially supported by Tin Ka Ping Foundation. The APC was funded by a start-up grant to DD (Project ID: P0035101).
Conflict of Interest
The authors declare that the research was conducted in the absence of any commercial or financial relationships that could be construed as a potential conflict of interest.
Publisher’s Note
All claims expressed in this article are solely those of the authors and do not necessarily represent those of their affiliated organizations, or those of the publisher, the editors and the reviewers. Any product that may be evaluated in this article, or claim that may be made by its manufacturer, is not guaranteed or endorsed by the publisher.
Afzal, H., Ali, I., Aslam Khan, M., and Hamid, K. (2010). A study of university students’ motivation and its relationship with their academic performance. SSRN Electron. J. 5:9. doi: 10.2139/ssrn.2899435
CrossRef Full Text | Google Scholar
Allen, J. J., and Anderson, C. A. (2018). Satisfaction and frustration of basic psychological needs in the real world and in video games predict internet gaming disorder scores and well-being. Comput. Hum. Behav. 84, 220–229. doi: 10.1016/j.chb.2018.02.034
Anthony, W. L., Zhu, Y. H., and Nower, L. (2021). The relationship of interactive technology use for entertainment and school performance and engagement: evidence from a longitudinal study in a nationally representative sample of middle school students in China. Comput. Hum. Behav. 122:106846. doi: 10.1016/j.chb.2021.106846
Bai, C., Chen, X. M., and Han, K. Q. (2020). Mobile phone addiction and school performance among Chinese adolescents from low-income families: A moderated mediation model. Child Youth Serv. Rev. 118:105406. doi: 10540610.1016/j.childyouth.2020.105406
Baker, S. R. (2004). Intrinsic, extrinsic, and amotivational orientations: their role in university adjustment, stress, well-being, and subsequent academic performance. Curr. Psychol. 23, 189–202. doi: 10.1007/s12144-004-1019-9
Benabou, R., and Tirole, J. (2003). Intrinsic and extrinsic motivation. Rev. Econ. Stud. 70, 489–520. doi: 10.1111/1467-937x.00253
Beyens, I., Vandenbosch, L., and Eggermont, S. (2015). Early adolescent boys’ exposure to internet pornography: relationships to pubertal timing, sensation seeking, and academic performance. J. Early Adolesc. 35, 1045–1068. doi: 10.1177/0272431614548069
Bradley, G. (2006). Work participation and academic performance: A test of alternative propositions. J. Educ. Work. 19, 481–501. doi: 10.1080/13639080600988756
Bu, H., Chi, X. L., and Qu, D. Y. (2021). Prevalence and predictors of the persistence and incidence of adolescent internet addiction in mainland China: A two-year longitudinal study. Addict. Behav. 122:107039. doi: 10703910.1016/j.addbeh.2021.107039
PubMed Abstract | CrossRef Full Text | Google Scholar
Cerniglia, L., Zoratto, F., Cimino, S., Laviola, G., Ammaniti, M., and Adriani, W. (2017). Internet addiction in adolescence: neurobiological, psychosocial and clinical issues. Neurosci. Biobehav. Rev. 76, 174–184. doi: 10.1016/j.neubiorev.2016.12.024
Chen, X., Huang, X., Wang, L., and Chang, L. (2012). Aggression, peer relationships, and depression in Chinese children. J. Child Psychol. Psychiatry 53, 1233–1241. doi: 10.1111/j.1469-7610.2012.02576.x
Cheo, R. (2017). Small rewards or Some encouragement? Using an experiment in China to test extrinsic motivation on academic performance. Singap. Econ. Rev. 62, 797–808. doi: 10.1142/S0217590817400276
Chi, X., Hong, X., and Chen, X. (2020). Profiles and sociodemographic correlates of internet addiction in early adolescents in southern China. Addict. Behav. 106:106385. doi: 10.1016/j.addbeh.2020.106385
China Internet Network Information Center (2020). Statistical Report on Internet Development in China.
Google Scholar
Chou, C., and Hsiao, M.-C. (2000). Internet addiction, usage, gratification, and pleasure experience: the Taiwan college students’ case. Comput. Educ. 35, 65–80. doi: 10.1016/S0360-1315(00)00019-1
Dou, D., and Shek, D. T. L. (2021). Concurrent and longitudinal relationships between positive youth development attributes and adolescent internet addiction symptoms in Chinese mainland high school students. Int. J. Environ. Res. Public Health 18:1937. doi: 10.3390/ijerph18041937
Eccles, J. (1983). Expectancies, Values and Academic Behaviors. Achievement and Achievement Motives. San Francisco, CA: Free man.
Education and Manpower Bureau (2003). Users’ and training manual for measuring secondary students’ performance in affective and social domains.
Enns, M. W., Cox, B. J., Sareen, J., and Freeman, P. (2001). Adaptive and maladaptive perfectionism in medical students: a longitudinal investigation. Med. Educ. 35, 1034–1042. doi: 10.1046/j.1365-2923.2001.01044.x
Epstein, J. A., and Botvin, G. J. (2000). Methods to decrease attrition in longitudinal studies with adolescents. Psychol. Rep. 87, 139–140. doi: 10.2466/pr0.2000.87.1.139
Floros, G., Paradisioti, A., Hadjimarcou, M., Mappouras, D. G., Karkanioti, O., and Siomos, K. (2015). Adolescent online gambling in Cyprus: associated school performance and psychopathology. J. Gambl. Stud. 31, 367–384. doi: 10.1007/s10899-013-9424-3
Froiland, J. M., and Oros, E. (2014). Intrinsic motivation, perceived competence and classroom engagement as longitudinal predictors of adolescent reading achievement. Educ. Psychol. 34, 119–132. doi: 10.1080/01443410.2013.822964
Goldhaber, D., and Özek, U. (2019). How much should we rely on student test achievement as a measure of success? Educ. Res. 48, 479–483. doi: 10.3102/0013189X19874061
Guo, J., Marsh, H. W., Parker, P. D., Morin, A. J. S., and Dicke, T. (2017). Extending expectancy-value theory predictions of achievement and aspirations in science: dimensional comparison processes and expectancy-by-value interactions. Learn. Instr. 49, 81–91. doi: 10.1016/j.learninstruc.2016.12.007
Hanus, M. D., and Fox, J. (2015). Assessing the effects of gamification in the classroom: A longitudinal study on intrinsic motivation, social comparison, satisfaction, effort, and academic performance. Comput. Educ. 80, 152–161. doi: 10.1016/j.compedu.2014.08.019
Haraldsen, H. M., Solstad, B. E., Ivarsson, A., Halvari, H., and Abrahamsen, F. E. (2020). Change in basic need frustration in relation to perfectionism, anxiety, and performance in elite junior performers. Scand. J. Med. Sci. Sports 30, 754–765. doi: 10.1111/sms.13614
Hayes, A. F. (2017). Introduction to Mediation, Moderation, and Conditional Process Analysis: A Regression-Based Approach. New York: Guilford publications.
Hu, L. T., and Bentler, P. M. (1999). Cutoff criteria for fit indexes in covariance structure analysis: conventional criteria versus new alternatives. Struct. Equ. Model. Multidiscip. J. 6, 1–55. doi: 10.1080/10705519909540118
King, D., and Delfabbro, P. (2018). Internet Gaming Disorder: Theory, Assessment, Treatment, and Prevention. Cambridge, MA: Academic Press.
Kline, R. B. (2015). Principles and Practice of Structural Equation Modeling. 4th Edn . New York: The Guilford Press.
Ko, C. H., Yen, J. Y., Yen, C. F., Chen, C. S., and Chen, C. C. (2012). The association between internet addiction and psychiatric disorder: a review of the literature. Eur. Psychiatry 27, 1–8. doi: 10.1016/j.eurpsy.2010.04.011
Kuvaas, B., Buch, R., Weibel, A., Dysvik, A., and Nerstad, C. G. (2017). Do intrinsic and extrinsic motivation relate differently to employee outcomes? J. Econ. Psychol. 61, 244–258. doi: 10.1016/j.joep.2017.05.004
Lee, C. Y. S., Anderson, J. R., and Klimes-Dougan, B. (2016). Potentially traumatic experiences, academic performance, and psychological distress: The role of shame. J. Couns. Dev. 94, 41–50. doi: 10.1002/jcad.12060
Lin, Y.-G., McKeachie, W. J., and Kim, Y. C. (2003). College student intrinsic and/or extrinsic motivation and learning. Learn. Individ. Differ. 13, 251–258. doi: 10.1016/S1041-6080(02)00092-4
Liu, Y., Hau, K. T., Liu, H. Y., Wu, J., Wang, X. F., and Zheng, X. (2020). Multiplicative effect of intrinsic and extrinsic motivation on academic performance: A longitudinal study of Chinese students. J. Pers. 88, 584–595. doi: 10.1111/jopy.12512
Long, J., Liu, T., Liu, Y., Hao, W., Maurage, P., and Billieux, J. (2018). Prevalence and correlates of problematic online gaming: a systematic review of the evidence published in Chinese. Curr. Addict. Rep. 5, 359–371. doi: 10.1007/s40429-018-0219-6
Malik, M. J., Ahmad, M., Kamran, M. R., Aliza, K., and Elahi, M. Z. (2020). Student use of social media, academic performance, and creativity: the mediating role of intrinsic motivation. Interact. Technol. Smart Educ. 17, 403–415. doi: 10.1108/ITSE-01-2020-0005
Moneta, G. B., and Siu, C. M. (2002). Trait intrinsic and extrinsic motivations, academic performance, and creativity in Hong Kong college students. J. Coll. Stud. Dev. 43, 664–683.
Neel, C. G. O., and Fuligni, A. (2013). A longitudinal study of school belonging and academic motivation across high school. Child Dev. 84, 678–692. doi: 10.1111/j.1467-8624.2012.01862.x
Nemati, Z., and Matlabi, H. (2017). Assessing behavioral patterns of internet addiction and drug abuse among high school students. Psychol. Res. Behav. Manag. 10, 39–45. doi: 10.2147/Prbm.S123224
Reed, P., and Reay, E. (2015). Relationship between levels of problematic internet usage and motivation to study in university students. High. Educ. 70, 711–723. doi: 10.1007/s10734-015-9862-1
Rivers, J., Mullis, A. K., Fortner, L. A., and Mullis, R. L. (2012). Relationships between parenting styles and the academic performance of adolescents. J. Fam. Soc. Work. 15, 202–216. doi: 10.1080/10522158.2012.666644
Rosseel, Y. (2012). Lavaan: An R package for structural equation modeling and more. Version 0.5–12 (BETA). J. Stat. Softw. 48, 1–36. doi: 10.18637/jss.v048.i02
Ryan, R. M., and Deci, E. L. (2000). Intrinsic and extrinsic motivations: classic definitions and new directions. Contemp. Educ. Psychol. 25, 54–67. doi: 10.1006/ceps.1999.1020
Salmela-Aro, K., Upadyaya, K., Hakkarainen, K., Lonka, K., and Alho, K. (2017). The dark side of internet use: two longitudinal studies of excessive internet use, depressive symptoms, school burnout and engagement Among Finnish early and late adolescents. J. Youth Adolesc. 46, 343–357. doi: 10.1007/s10964-016-0494-2
Saw, A. E., Main, L. C., and Gastin, P. B. (2016). Monitoring the athlete training response: subjective self-reported measures trump commonly used objective measures: a systematic review. Br. J. Sports Med. 50, 281–291. doi: 10.1136/bjsports-2015-094758
Shek, D. T. (2002). Family functioning and psychological well-being, school adjustment, and problem behavior in Chinese adolescents with and without economic disadvantage. J. Genet. Psychol. 163, 497–502. doi: 10.1080/00221320209598698
Shek, D. T., Tang, V. M., and Lo, C. Y. (2008). Internet addiction in Chinese adolescents in Hong Kong: assessment, profiles, and psychosocial correlates. Sci. World J. 8, 776–787. doi: 10.1100/tsw.2008.104
Shek, D. T., and Yu, L. (2016). Adolescent internet addiction in Hong Kong: prevalence, change, and correlates. J. Pediatr. Adolesc. Gynecol. 29, S22–S30. doi: 10.1016/j.jpag.2015.10.005
Tan, Y., Chen, Y., Lu, Y., and Li, L. (2016). Exploring associations between problematic internet use, depressive symptoms and sleep disturbance among southern Chinese adolescents. Int. J. Environ. Res. Public Health 13:313. doi: 10.3390/ijerph13030313
Truzoli, R., Vigano, C., Galmozzi, P. G., and Reed, P. (2020). Problematic internet use and study motivation in higher education. J. Comput. Assist. Learn. 36, 480–486. doi: 10.1111/jcal.12414
Vansteenkiste, M., Simons, J., Lens, W., Soenens, B., and Matos, L. (2005). Examining the motivational impact of intrinsic versus extrinsic goal framing and autonomy-supportive versus internally controlling communication style on early adolescents’ academic achievement. Child Dev. 76, 483–501. doi: 10.1111/j.1467-8624.2005.00858.x
Waley, A. (2005). The Analects of Confucius. London: Routledge.
Wang, J. H. Y., and Guthrie, J. T. (2004). Modeling the effects of intrinsic motivation, extrinsic motivation, amount of reading, and past reading achievement on text comprehension between US and Chinese students. Read. Res. Q. 39, 162–186. doi: 10.1598/RRQ.39.2.2
Wang, Y., and Ross, H. (2010). Experiencing the change and continuity of the college entrance examination: A case study of Gaokao County, 1996-2010. Chin. Educ. Soc. 43, 75–93. doi: 10.2753/CED1061-1932430407
Wang, X., Zhao, S., Zhang, M. X., Chen, F., and Chang, L. (2021). Life history strategies and problematic use of short-form video applications. Evol. Psychol. Sci. 7, 39–44. doi: 10.1007/s40806-020-00255-9
Xiang, X. (2018). My future, my family, my freedom: meanings of schooling for poor, rural Chinese youth. Harv. Educ. Rev. 88, 81–102. doi: 10.17763/1943-5045-88.1.81
Young, K. S. (1998). Caught in the Net: How to Recognize the Signs of Internet Addiction--and a Winning Strategy for Recovery. New York: John Wiley and Sons.
Yu, L., and Shek, D. T. (2013). Internet addiction in Hong Kong adolescents: a three-year longitudinal study. J. Pediatr. Adolesc. Gynecol. 26, S10–S17. doi: 10.1016/j.jpag.2013.03.010
Zeng, G., Zhang, L., Fung, S.-F., Li, J., Liu, Y.-M., Xiong, Z.-K., et al. (2021). Problematic internet usage and self-esteem in Chinese undergraduate students: The mediation effects of individual affect and relationship satisfaction. Int. J. Environ. Res. Public Health 18:6949. doi: 10.3390/ijerph18136949
Zhang, Y., Qin, X., and Ren, P. (2018). Adolescents’ academic engagement mediates the association between internet addiction and academic achievement: The moderating effect of classroom achievement norm. Comput. Hum. Behav. 89, 299–307. doi: 10.1016/j.chb.2018.08.018
Zhou, Z., Shek, D. T. L., Zhu, X., and Dou, D. (2020). Positive youth development and adolescent depression: A longitudinal study based on mainland Chinese high school students. Int. J. Environ. Res. Public Health 17:4457. doi: 10.3390/ijerph17124457
Keywords: internet addiction, academic value, satisfaction with academic performance, high school students, mainland China
Citation: Dou D and Shek DTL (2021) Predictive Effect of Internet Addiction and Academic Values on Satisfaction With Academic Performance Among High School Students in Mainland China. Front. Psychol . 12:797906. doi: 10.3389/fpsyg.2021.797906
Received: 19 October 2021; Accepted: 24 November 2021; Published: 15 December 2021.
Reviewed by:
Copyright © 2021 Dou and Shek. This is an open-access article distributed under the terms of the Creative Commons Attribution License (CC BY) . The use, distribution or reproduction in other forums is permitted, provided the original author(s) and the copyright owner(s) are credited and that the original publication in this journal is cited, in accordance with accepted academic practice. No use, distribution or reproduction is permitted which does not comply with these terms.
*Correspondence: Daniel T. L. Shek, [email protected]
Disclaimer: All claims expressed in this article are solely those of the authors and do not necessarily represent those of their affiliated organizations, or those of the publisher, the editors and the reviewers. Any product that may be evaluated in this article or claim that may be made by its manufacturer is not guaranteed or endorsed by the publisher.
- Research article
- Open access
- Published: 06 January 2021
Prevalence and associated factors of internet addiction among undergraduate university students in Ethiopia: a community university-based cross-sectional study
- Yosef Zenebe ORCID: orcid.org/0000-0002-0138-6588 1 ,
- Kunuya Kunno 1 ,
- Meseret Mekonnen 1 ,
- Ajebush Bewuket 1 ,
- Mengesha Birkie 1 ,
- Mogesie Necho 1 ,
- Muhammed Seid 1 ,
- Million Tsegaw 1 &
- Baye Akele 2
BMC Psychology volume 9 , Article number: 4 ( 2021 ) Cite this article
34k Accesses
40 Citations
280 Altmetric
Metrics details
Internet addiction is a common problem in university students and negatively affects cognitive functioning, leads to poor academic performance and engagement in hazardous activities, and may lead to anxiety and stress. Behavioral addictions operate on a modified principle of the classic addiction model. The problem is not well investigated in Ethiopia. So the present study aimed to assess the prevalence of internet addiction and associated factors among university students in Ethiopia.
Main objective of this study was to assess the prevalence and associated factors of internet addiction among University Students in Ethiopia.
A community-based cross-sectional study was conducted among Wollo University students from April 10 to May 10, 2019. A total of 603 students were participated in the study using a structured questionnaire. A multistage cluster sampling technique was used to recruit study participants. A binary logistic regression method was used to explore associated factors for internet addiction and variables with a p value < 0.25 in the bivariate analysis were fitted to the multi-variable logistic regression analysis. The strength of association between internet addiction and associated factors was assessed with odds ratio, 95% CI and p value < 0.05 in the final model was considered significant.
The prevalence of internet addiction (IA) among the current internet users was 85% (n = 466). Spending more time on the internet (adjusted odds ratio (AOR) = 10.13, 95% CI 1.33–77.00)), having mental distress (AOR = 2.69, 95% CI 1.02–7.06), playing online games (AOR = 2.40, 95% CI 1.38–4.18), current khat chewing (AOR = 3.34, 95% CI 1.14–9.83) and current alcohol use (AOR = 2.32, 95% CI 1.09–4.92) were associated with internet addiction.
Conclusions
The current study documents a high prevalence of internet addiction among Wollo University students. Factors associated with internet addiction were spending more time, having mental distress, playing online games, current khat chewing, and current alcohol use. As internet addiction becomes an evident public health problem, carrying out public awareness campaigns may be a fruitful strategy to decrease its prevalence and effect. Besides to this, a collaborative work among stakeholders is important to develop other trendy, adaptive, and sustainable countermeasures.
Peer Review reports
Globally, more than three billion people use the internet daily with young people being the most common users [ 1 ]. In the field of medicine and healthcare, it helps in the practice of evidence-based medicine, research and learning, access to medical and online databases, handling patients in remote areas, and academic and recreational purposes [ 2 , 3 ].
In terms of classical psychology and psychiatry, IA is a relatively new phenomenon. The literature uses interchangeable references such as “compulsive Internet use”, “problematic Internet use”, “pathological Internet use”, and “Internet addiction”. The Psychologist Mark Griffiths, one of the widely recognized authorities in the sphere of addictive behavior, is the author of the most frequently quoted definition: “Internet addiction is a non-chemical behavioral addiction, which involves human–machine (computer-Internet) interaction” [ 4 , 5 ]. Internet addiction is a behavioural problem that has gained increasing scientific recognition in the last decade, with some researchers claiming it is a "21st Century epidemic"[ 6 ]. The psychopathologic symptoms of internet addiction includes Salience(the respondent most likely feels preoccupied with the Internet, hides the behaviour from others, and may display a loss of interest in other activities and/or relationships only to prefer more solitary time online), Excessive Use (the respondent engages in excessive online behaviour and compulsive usage, and is intermittently unable to control time online that he or she hides from others), Neglect Work (Job or school performance and productivity are most likely compromised due to the amount of time spent online), Anticipation(the respondent most likely thinks about being online when not at the computer and feels compelled to use the Internet when offline), Lack of Control(the respondent has trouble managing his or her online time, frequently stays online longer than intended, and others may complain about the amount of time he or she spends online) and Neglect Social Life (the respondent frequently forms new relationships with fellow online users and uses the Internet to establish social connections that may be missing in his or her life) [ 7 , 8 , 9 , 10 ].
Events during the adolescence period greatly influence a person's development and can determine their attitudes and behavior in later life [ 11 ]. The teenagers are often in conflict with authority and cultural and moral norms of society, certain developmental effects can trigger a series of defense mechanisms [ 12 ]. During adolescence, there is an increased risk of emotional crises, often accompanied by mood changes and periods of anxiety and depressive behavior, which some adolescents attempt to fight through withdrawal, avoidance of any extensive social contact, aggressive reactions, and addictive behaviour [ 13 , 14 ]. Adolescents are exceptionally vulnerable and receptive during this period and can become drawn to the Internet as a form of release. Over time, this can lead to addiction [ 15 ].
Relaxed access and social networking are two of the several aspects of the Internet development of addictive behaviour [ 16 ]. Internet addiction is a newly emerged behavioral problem of adults which was reported after problem behavior theory was proposed [ 17 ]. Behavioral addictions operate on a modified principle of the classic addiction model [ 18 , 19 , 20 ]. Others have reported, that there is a tendency for individuals to be multiply ''addicted'' and to have overlapping addictions between common substances such as alcohol and cigarettes and ''addictions'' to activities such as internet use, gambling, exercising, and television [ 21 ]. A key factor to both models of substance and behavioral addictions is the concept of psychological dependence, in which no physiological exchange, such as ingestion of a substance, occurs [ 18 , 22 ]. Internet addiction in puberty and young adults can negatively impact life satisfaction and engagement [ 23 ], which may negatively affect cognitive functioning [ 24 ], lead to poor academic performance [ 25 , 26 ], and engagement in hazardous activities [ 27 ]. Internet addiction is also related to depression, somatization, and obsessive–compulsive disorder [ 28 ]. It has been found that paranoid ideation, hostility, anxiety, depression, interpersonal sensitivity, and obsessive–compulsive average scores are higher in people with high Internet Addiction scores than those without Internet addiction [ 29 , 30 ].
College students are especially susceptible to developing a dependence on the Internet, more than most other segments of society. This can be qualified to numerous factors including the following: Availability of time; ease of use; the psychological and developmental characteristics of young adulthood; limited or no parental supervision; an expectation of Internet/computer use covertly if not, as some courses are Internet-dependent, from assignments and projects to link with peers and mentors; the Internet offering a way of escape from exam anxiety [ 31 ].
Studies have indicated that IA is associated with different factors. Socio-demographic factors such as age (having lower age) [ 32 ] and male gender [ 33 , 34 , 35 , 36 , 37 ]. Reason for internet use related factors such as making new friendships online [ 33 ], getting into relationships online [ 33 ], using the internet less for coursework/assignments [ 33 ], visiting pornographic sites [ 34 ] and playing online games [ 31 , 34 , 38 ]. Time related and other factors such as higher internet usage time [ 37 , 39 ],continuous availability online [ 33 , 35 , 39 ] and mode of internet access [ 35 ]. Clinical and substance related factors such as insomnia [ 40 ], attention deficient disorder and hyperactivity symptoms [ 41 ], being sexual inactive [ 32 ], low self-esteem [ 40 ], failure in academic performance [ 32 ], smoking [ 41 ], and potential addictive personal habits of, drinking alcohol or coffee, and taking drugs [ 34 ]. Besides, mental illness like depression, anxiety and psychological distress [ 35 , 36 , 37 , 39 , 40 ] are associated with internet addiction. This could be based on the application of a general strain theory framework whereby negative emotions that are secondary to depression, anxiety, and psychological distress will be associated positively with internet addiction [ 42 ].
Internet Addiction is now becoming a serious mental health problem among Chinese adolescents. The researchers identified 10.6% to 13.6% of Chinese college students as Internet addicts [ 43 , 44 ]. A study conducted among Taiwan college students reported that the prevalence of Internet Addiction was 15.3% [ 37 ].
The prevalence of Problematic Internet Use (PIU) was greater among university students. For instance, the prevalence was 36.9 to 81% in Malaysian medical students by using the internet addiction questionnaire and Internet Addiction Diagnostic Questionnaire study instrument with a cut-offs point of ≥ 43 and 31to 79 respectively [ 45 , 46 ], 25.1% in American community university students by using the YIATstudy instrument with a cut-offs point of ≥ 40 [ 47 ], 40.7% in Iranian university students by utilizing the YIAT study instrument with a cut-offs point of ≥ 40 [ 48 ], 38.2–63.5% IA in Japanese university students as measured with the YIAT study instrument with a cut-offs point of ≥ 40 and ≥ 40 respectively [ 36 , 49 ], 16.8% IA in Lebanon University students by utilizing the YIAT study instrument with a cut-offs point of ≥ 50 [ 40 ], 35.4% IA in Nepal undergraduate students as measured with the YIAT study instrument with a cut-offs point of ≥ 40 [ 32 ], 40% IA in Jordan University students by utilizing the YIAT study instrument with a cut-offs point of ≥ 50 [ 50 ],19.85% to 42.9% IA in various parts of India as measured with the YIAT study instrument with a cut-offs point of 31to79, ≥ 50 and ≥ 50 respectively [ 33 , 35 , 39 ], 12% IA to 34.7% (PIU) in Greek University students by utilizing the Problematic Internet Use Diagnostic Test study instrument with no stated cut-offs point [ 34 ], 1.6% IA in Turkey students by using the Young’s Internet Addiction Scale study instrument with a cut-offs point of 70–100 [ 41 ].
In general, the main reason why youths are at particular risk of internet addiction is that they spend most of their time on online gaming and social applications like online social networking such as Twitter, Facebook, and telegrams [ 51 ].
Even though developing countries shares for a large magnitude of internet addiction, indicating the public health impact of the problem in the region, much is not known about the occurrence rate of the problem in these regions in general and Ethiopia in particular. As a result, trustworthy assessments of internet addiction in university students in these circumstances are required for delivering a focused intervention geared towards addressing the associated factors.
Moreover, it will be a ground for the expansion of national and international plans, procedures, and policy. At last but not least, the findings from this study will provide significant implications for counsellors and policymakers to prevent students' Internet addiction. Hence, this a community university-based cross-sectional study aimed and assessed the prevalence and associated factors of internet addiction among Wollo university students.
Research questions
The purpose of this study was to measure prevalence and associated factors of IA among undergraduate university students in Ethiopia. The specific research questions that guided the present study were:
What is the prevalence of IA among undergraduate university students in Ethiopia?
What are the associated factors of IA?
Methods and materials
Study area and period.
The study was done at Wollo University, Dessie campus that is found in South Wollo Zone, Amhara Regional State which is 401 kms far from Addis Ababa, Northeastern Ethiopia. It had 5 colleges and 2 schools and a total of 62 departments. The number of regular students in 2018/2019 is 7248; among these 4009 are males and 3239 are females. The study was conducted from April 10 to May 10/ 2019. The sample size was determined using single population proportion formula, taking a 50% prevalence of Internet Addiction with the following assumption: 95% CI, 5% margin of error, 10% non-response rate, and a design effect of 1.5. So, the final sample size was 603.
Sampling technique and procedure
A multistage cluster sampling technique was used to recruit study participants. In the first stage, by the use of the lottery method, two colleges (College of medicine and health sciences, and College of natural sciences, and one school (school of law)) were selected. In the second stage, 18 departments (9 from the college of medicine and health science, 8 from the college of natural science and 1 from the school of law) were selected. Students were selected proportionally from the given departments based on the number of students of a particular.
Study design
A community university-based cross-sectional study was carried out to assess the prevalence and associated factors of Internet Addiction among undergraduate students at Wollo University, Amhara Region, Ethiopia.
Inclusion and exclusion criteria
All generic regular undergraduate adult students whose ages were 18 years and above, and who were present at the time of data collection. Students who gave consent to the study were recruited. The study participants who are blind and severely ill were excluded from the study.
Study instruments
Self-administered, well-structured, and organized English version questionnaire was disseminated to students, and data were collected from the individual student. The questionnaires comprised six parts. The first part consisted of socio-demographic details; a structured questionnaire was used to assess sociodemographic characteristics. The second part consists of Young’s Internet Addiction Test (YIAT); a structured, self-administered questionnaire was used to assess Internet Addiction. The YIAT [ 7 ] is the most commonly used measure of Internet Addiction among adults [ 52 ]. It includes 20 questions with a scoring of 1–5 for each question and a total maximum score of 100. Based on scoring subjects would be classified into normal users (0–30), mild (31–49), moderate (50–79), and severe (80–100) Internet Addiction groups. Mild Internet addiction, moderate Internet addiction, and severe Internet Addiction were considered as having an Internet Addiction [ 53 , 54 , 55 ]. YIAT-20 showed that it is more reliable in University students. The Cronbach α in the present study was 0.89. The third part time-associated factors; a self-report structured questionnaire was prepared from different kinds of literature to assess time-associated factors (such as Internet use experience in months and Internet use per day in hours). The fourth part reasons for internet use; a structured questionnaire was used to assess the reasons for internet use. The fifth part psychoactive substance use-associated factors; a self-report questionnaire was used to assess the current use of psychoactive substances (Khat, Cigarette, Alcohol, and Cannabis), and the last part mental health problem-associated factors and it was assessed by Kessler10 (K10). The K10 scale [ 56 ] is a simple measure of mental distress. The K10-item scale, which has been translated into Amharic and validated in Ethiopia [ 57 ], was used to measure mental distress (depressive, anxiety, and somatic symptoms). The internal consistency of the K10 psychological distress scale in the present study was checked with a reliability assessment and was found to be 0.86 [ 58 , 59 , 60 ]. Scores will range from 10 to 50. A score under 20 is likely to be well, a score of 20–24 is likely to have mild mental distress, a score of 25–29 is likely to have moderate mental distress and a score of 30 and over are likely to have severe mental distress. Study participants with a score of 20 or more points on the K10 Likert scale were considered as having mental distress [ 61 ].
Data quality control
A structured self-administered questionnaire was developed in English and would be translated to Amharic language and again translated back into English to ensure consistency. Data collectors and supervisors would be trained for two days on the objective of the study, the content of the questionnaire, and the data collection procedure. Data would be pilot tested on 5% of the total sample size outside the study area and based on feedback obtained from the pilot test; the necessary modification would be done. During the study period, the collected data would be checked continuously daily for completeness by principal investigator and supervisor in the respective departments.
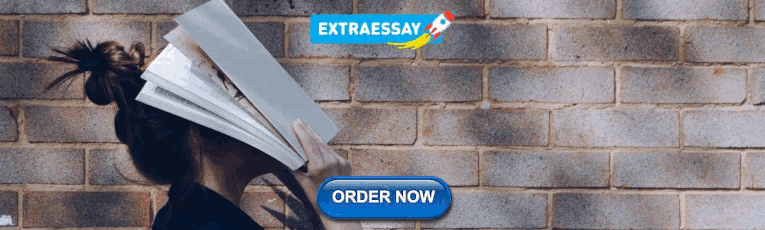
Data processing and analysis
Quantitative data would be cleaned, coded, and entered into Epi-data 3.1 and exported to SPSS version 25 for analysis. Descriptive data would be presented by a table, graphs, charts, and means. Multicollinearity test was checked by using standard error and there was no correlation between independent variables. The association between independent variables and Internet Addiction would be made using a binary logistic regression model and all independent variables having p value ≤ 0.25 would be included in multiple logistic regression models. A p value less than 0.05 and Adjusted Odds Ratio (AOR) with 95% Confidence Interval (CI) not inclusive of one would be considered as statically significant and would be used to determine predictors of Internet Addiction in the final model. Hosmer–Lemeshow test was done to check model fitness and the model was fit.
Socio-demographic characteristics of study participants
A total of 603 participants were involved with a response rate of 90.9% (n = 548). However, the rest 9.1% (n = 55) participants were excluded due to incomplete responses. The mean age of the respondents was 21.4 (SD 1.8) years, the minimum and maximum age of the participants was 18 years and 30 years respectively. More than half of, 291 (53.1%) of respondents were males. Many of the study participants had a practice of using the internet for more than twelve months, 321 (58.6%). About 501 (91.4%), 268 (48.9%), 433 (79%) were using the internet less than five hours per day, most common mode of internet access Wi-Fi, and log in and off occasionally during the day respectively. The study participants with current khat use, current cigarette smoking, current alcohol use, and current cannabis use were 19.0%, 11.3%, 25.4%; and 4.0% respectively. About 19.3% of the participants had mental distress (Table 1 ).
Prevalence of Internet addiction
The prevalence of IA was 466 (85%) of the 305(55.6%), 153(27.9%), 8(1.5%) mild, moderate, and severe Internet Addiction respectively. Nevertheless, the remaining 82 (15%) are free from Internet Addiction (Fig. 1 ). Participants who login permanently had a greater figure of IA than those who log in and off occasionally during the day (92.2% versus 83.1%). Those who used the internet for about six months had a greater prevalence of Internet Addiction than those who used greater than twelve months (91.6% versus 84.1%) (Table 2 ).
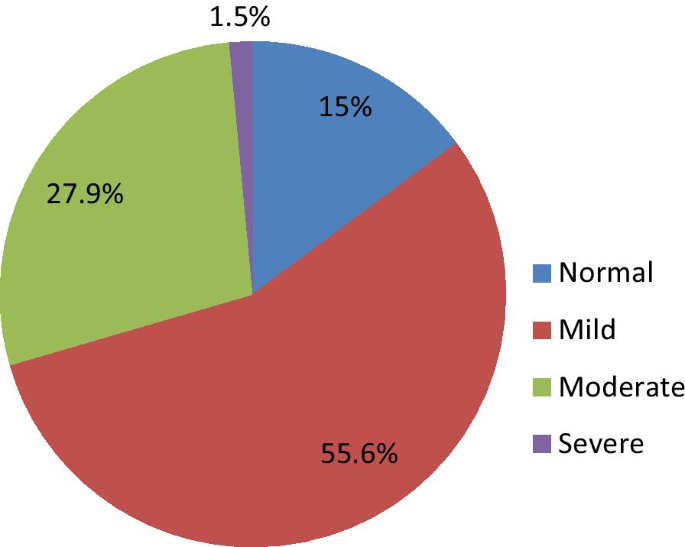
Internet Addiction by severity among undergraduate university students in Ethiopia, 2019 (n = 548)
Reasons for internet use among Wollo University students
The furthermost frequent reasons for internet use among Wollo University undergraduate students were using the internet for courses / assignments (93.6%), for social networks (Facebook, etc.) (85.6%), for reading / posting news (76.6%), for getting into relationships online (66.6%),for playing mobile games (44.5%), for downloading music or videos (65.7%), for watching videos (57.8%),for retrieving sexual information (22.8%), for chat rooms (47.6%) and for e-mail ( reading, writing) (49.8%) (Fig. 2 ).
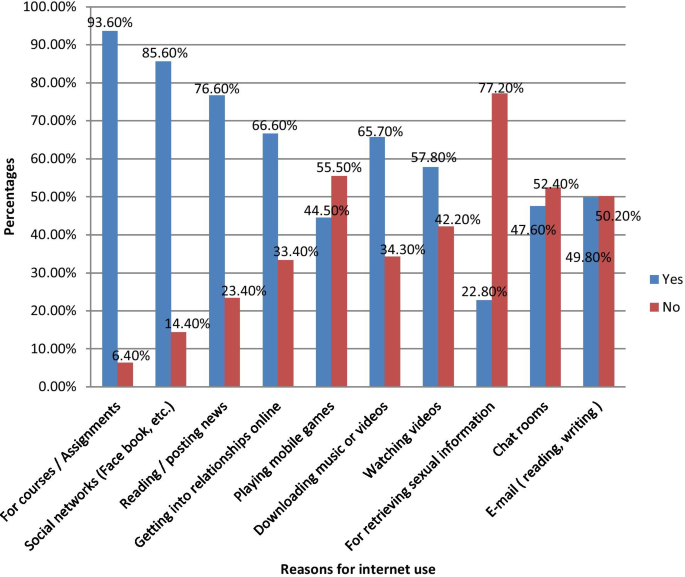
Reasons for internet use among undergraduate university students in Ethiopia, 2019 (n = 548)
Factors associated with internet addiction in the univariate analysis
Time related factors.
Duration of using the internet was associated with Internet Addiction i.e. students who used the internet for more than a year was 51% lower risks of having internet addiction than their counterparts (OR=0.49; CI 0.24–0.96). Respondents who were spending more time on the internet were more likely to develop Internet Addiction than their counterparts (OR=8.87; CI 1.21–65.25).
Mode of internet access was related to Internet Addiction i.e. those who used mobile internet were 45% lower risks of having Internet Addiction than those who used data cards (OR = 0.55; 95% CI 0.28–1.07).Participants who were permanently online were most likely to have Internet Addiction than those who were not (OR=2.39; 95% CI 1.16–4.93).
Reasons for internet use related factors
Study participants who played mobile games online were more likely to develop Internet Addiction than those who were not played mobile games (OR = 2.67; 95% CI 1.57–4.52). Those who downloaded music or videos were higher risks of having Internet Addiction than those who didn’t (OR = 1.62; 95% CI 1.00–2.61). Study participants who watched the video online were most likely to have Internet Addiction than those who didn’t watch (OR=1.94; 95% CI 1.21–3.12).
Psychoactive substance use related factors
Those who chewed khat currently were higher odds of having Internet Addiction than those who were not (OR = 5.33; 95% CI 1.90–14.91). Respondents who smoked cigarettes currently were more likely to have Internet Addiction than their counterparts (OR = 12.20; 95% CI 1.67–89.28).
Those who used alcohol currently were greater risks of having Internet Addiction than those who hadn't (OR = 2.76; 95% CI 1.38–5.51).
Mental health problem related factors
Study participants who had mental distress were four times more likely to develop Internet Addiction than those who didn't have mental distress (OR = 4.26; 95% CI 1.68–10.81) (Table 2 ).
Factors associated with internet addiction in the multivariate analysis
In the final model, spending more time on the internet, having mental distress and playing online games were the factors associated with Internet Addiction. Moreover, current khat chewing and current alcohol use were the independent predictors for Internet Addiction. Using the internet for more than twelve months and using the internet by mobile internet were negatively associated with Internet Addiction (Table 2 ).
Discussions
The present study aims to assess the prevalence and associated factors of Internet Addiction among undergraduate university students in Ethiopia. The prevalence of IA was 85% (n = 466). In the final model; spending more time on the internet, having mental distress and playing online games were the factors associated with Internet Addiction. Moreover, current khat chewing and current alcohol use were the independent predictors for Internet Addiction. Using the internet for more than twelve months and using the internet by mobile internet were negatively associated with Internet Addiction.
The prevalence of Internet Addiction in the present study was higher than the prevalence of Internet Addiction that was done in different universities such as three medical schools across three countries ( Croatia, India, and Nigeria) 49.7% [ 55 ], Malaysian 36.9% to 81% [ 45 , 46 ], American community 25.1% [ 47 ], Iran 12.5 to 40.7% [ 48 , 62 , 63 ], Japan 38.2% to 63.5% [ 36 , 49 ], Greek 12% to 30.1% [ 54 , 64 ], Jordan 40% [ 50 ], Lebanon 16.8% [ 40 ], Nepal 35.4% [ 32 ] and in different parts of India 19.85% to 42.9% [ 33 , 35 , 39 ]. The discrepancy might be due to the cut-off point of YIAT-20, instrument difference, mental health policy, a cultural difference like time utilization, the difference in study participants such as in our study the participants were from two colleges and one school, and all participants were internet users, sample size and the time difference between the studies. The study in Malaysian University was conducted among medical students only and focusing on mild Internet Addiction and moderate Internet Addiction and not on severe Internet addiction.
In our study spending more time on the internet was 10 times more likely to develop Internet Addiction than those who are spending less time. The finding of this study is in line with similar studies done on college students in Taiwan and three medical schools across three countries (Croatia, India, and Nigeria) [ 37 , 55 ]. The possible explanation for the association between Internet usage time and Internet Addiction is that it might be as much a symptom as it is a cause. However, this study design was cross-sectional and no causal relationship can be clarified, further studies ought to examine whether Internet usage time is an essential factor for determining Internet addiction.
Likewise, students who had mental distress were 2.7 times more likely to develop Internet Addiction as compared to their counterparts. Study findings in these areas showed that students who had mental distress were related to higher levels of Internet Addiction than students who hadn’t mental distress [ 35 , 36 , 39 , 40 , 41 , 50 ]. This could be due to the Khantzian’s [ 65 ] self-medication hypothesis, indicating that mentally distressed university students might come to rely on the Internet as a method for coping with their mental distress. Hence, they will devote more and more time on the Internet and headway toward addiction if their mental distress symptoms are not cured [ 66 ].Students who had playing online games were 2.4 times higher to have Internet Addiction than their counterparts. A similar finding was also reported in Greek University and others [ 34 , 38 , 54 , 67 ].
Furthermore, students who chewed khat currently were three times most likely to develop Internet Addiction than students who reported no current khat chewing which is in line with the study finding in Greek University students [ 34 ]. In this study, students who drank alcohol currently were 2.3 times most likely to have Internet Addiction as compared with students who didn’t drink alcohol. Other studies reported a similar finding [ 17 , 34 , 68 , 69 ]. Probable reasons involve; based on the problem behavior theory, the problem behaviors (Internet Addiction and substance abuse) are inter-related.
Students who used the internet by mobile internet were 60% of lower risks of having Internet Addiction as compared to those students who used data cards. This might be due to inadequate finance to use the internet on mobile internet. So, the students may refrain from using the internet through mobile internet. Students who used the internet for more than 12 months were 52% less likely to have Internet Addiction than their counterparts. The current finding is not supported by other studies in the world. The present study has limitations such as alpha inflation from multiple testing and the analysis did not account for the complex sampling strategy in adjusting the standard errors.
The current study documents a high prevalence of Internet Addiction among Wollo University students. The factors associated with Internet Addiction were spending more time on the internet, having mental distress, playing online games, current khat chewing, and current alcohol use. As internet addiction becomes an evident public health problem, carrying out public awareness campaigns on its severity and negative consequences of excruciating agonies may be a fruitful strategy to decrease its prevalence and effect. Campaign programs may aim at informing the adults on the phenomenon of internet addiction, knowing the possible risks and symptoms. Besides to this, a collaborative work among all stakeholders is important to develop other trendy, adaptive, ethical and sustainable countermeasures.
Availability of data and materials
The datasets supporting the conclusions of this article are not publicly available due to ethics regulations but may be available from the corresponding author upon reasonable request.
Abbreviations
Adjusted odds ratio
Confidence interval
Crude odds ratio
- Internet addiction
Statistical package for social science
Young’s internet addiction test
Bremer J. The internet and children: advantages and disadvantages. Child Adolesc Psychiat Clin. 2005;14(3):405–28.
Article Google Scholar
Swaminath G. Internet addiction disorder: fact or fad? Nosing into nosology. Indian J Psychiat. 2008;50(3):158.
Dargahi H, Razavi S. Internet addiction and its related factors: a study of an Iranian population. 2007.
Tereshchenko S, Kasparov E. Neurobiological risk factors for the development of internet addiction in adolescents. Behav Sci. 2019;9(6):62.
Article PubMed Central Google Scholar
Griffiths M. Does internet and computer" addiction" exist? Some case study evidence. CyberPsychol Behav. 2000;3(2):211–8.
Kuss D, Griffiths M. Internet addiction in psychotherapy. Berlin: Springer; 2014.
Google Scholar
Young KS. Internet addiction: the emergence of a new clinical disorder. Cyberpsychol Behav. 1998;1(3):237–44.
Morahan-Martin J, Schumacher P, editors. Incidence and correlates of pathological internet usage. Paper presented art the 105th annual convention of the American Psychological Association, Chicago, Illinois; 1997.
Young KS, De Abreu CN. Internet addiction. A handbook and guide to evaluation. 2011.
Scherer K. College life online of college life and development. Healthy Unhealthy Internet Use J. 1997;38(6):655–65.
Blos P. On adolescence: psychoanalytic interpretation. Reissue. New York, NY: Free Press; 1966.
Karacic S, Oreskovic S. Internet addiction through the phase of adolescence: a questionnaire study. JMIR Mental Health. 2017;4(2):e11.
Article PubMed PubMed Central Google Scholar
Achenbach TM, Becker A, Döpfner M, Heiervang E, Roessner V, Steinhausen HC, et al. Multicultural assessment of child and adolescent psychopathology with ASEBA and SDQ instruments: research findings, applications, and future directions. J Child Psychol Psychiat. 2008;49(3):251–75.
Article PubMed Google Scholar
Graovac M. Adolescent u obitelji. Medicina Fluminensis: Medicina Fluminensis. 2010;46(3):261–6.
ZboralskiACD K, OrzechowskaDEF A, TalarowskaDE M, DarmoszAB A, JaniakGF A, JaniakGF M, et al. The prevalence of computer and Internet addiction among pupils Rozpowszechnienie uzależnienia od komputera i Internetu. Postepy Hig Med Dosw(online). 2009;63:8–12.
Kuss DJ, Griffiths MD. Online social networking and addiction—a review of the psychological literature. Int J Environ Res Public Health. 2011;8(9):3528–52.
Ko CH, Yen J-Y, Yen CF, Chen CS, Weng CC, Chen CC. The association between Internet addiction and problematic alcohol use in adolescents: the problem behavior model. Cyberpsychol Behav. 2008;11(5):571–6.
Bradley BP. Behavioural addictions: common features and treatment implications. Br J Addict. 1990;85(11):1417–9.
Holden C. 'Behavioral'addictions: do they exist?: American Association for the Advancement of Science; 2001.
Miele GM, Tilly SM, First M, Frances A. The definition of dependence and behavioural addictions. Br J Addict. 1990;85(11):1421–3.
Greenberg JL, Lewis SE, Dodd DK. Overlapping addictions and self-esteem among college men and women. Addict Behav. 1999;24(4):565–71.
Buckley RC. Adventure thrills are addictive. Front Psychol. 2015;6:1915.
PubMed PubMed Central Google Scholar
Shahnaz I, Karim A. The impact of internet addiction on life satisfaction and life engagement in young adults. Univ J Psychol. 2014;2(9):273–84.
Park M-H, Park E-J, Choi J, Chai S, Lee J-H, Lee C, et al. Preliminary study of Internet addiction and cognitive function in adolescents based on IQ tests. Psychiatry Res. 2011;190(2–3):275–81.
Akhter N. Relationship between internet addiction and academic performance among university undergraduates. Educ Res Rev. 2013;8(19):1793–6.
Usman N, Alavi M, Shafeq SM. Relationship between internet addiction and academic performance among foreign undergraduate students. Proced Soc Behav Sci. 2014;114:845–51.
Pfaff DW, Volkow ND. Neuroscience in the 21st century: from basic to clinical: Springer, Berlin, 2016.
Alavi SS, Alaghemandan H, Maracy MR, Jannatifard F, Eslami M, Ferdosi M. Impact of addiction to internet on a number of psychiatric symptoms in students of isfahan universities, iran, 2010. Int J Prevent Med. 2012;3(2):122.
Xiuqin HHZ, Mengchen L, Jinan W, Ying Z, Ran T. Mental health, personality, and parental rearing styles of adolescents with Internet addiction disorder. Cyberpsychol Behav Soc Netw. 2010;13(4):401–6.
Alavi SSAH, Maracy MR, Jannatifard F, Eslami M, Ferdosi M. Impact of addiction to internet on a number of psychiatric symptoms in students of Isfahan universities. Iran Int J Prev Med. 2012;3(2):122–7.
PubMed Google Scholar
Kandell JJ. Internet addiction on campus: The vulnerability of college students. Cyberpsychol Behav. 1998;1(1):11–7.
Bhandari PM, Neupane D, Rijal S, Thapa K, Mishra SR, Poudyal AK. Sleep quality, internet addiction and depressive symptoms among undergraduate students in Nepal. BMC Psychiat. 2017;17(1):106.
Krishnamurthy S, Chetlapalli SK. Internet addiction: prevalence and risk factors: A cross-sectional study among college students in Bengaluru, the Silicon Valley of India. Indian J Public Health. 2015;59(2):115.
Frangos CC, Frangos CC, Sotiropoulos I. Problematic internet use among Greek university students: an ordinal logistic regression with risk factors of negative psychological beliefs, pornographic sites, and online games. Cyberpsychol Behav Soc Netw. 2011;14(1–2):51–8.
Gedam SR, Ghosh S, Modi L, Goyal A, Mansharamani H. Study of internet addiction: Prevalence, pattern, and psychopathology among health professional undergraduates. Indian J Soc Psychiat. 2017;33(4):305.
Kitazawa M, Yoshimura M, Murata M, Sato-Fujimoto Y, Hitokoto H, Mimura M, et al. Associations between problematic internet use and psychiatric symptoms among university students in Japan. Psychiat Clin Neurosci. 2018;72(7):531–9.
Lin M-P, Ko H-C, Wu JY-W. Prevalence and psychosocial risk factors associated with Internet addiction in a nationally representative sample of college students in Taiwan. Cyberpsychol Behav Soc Netw. 2011;14(12):741–746.
Petry NM, O'Brien CP. Internet gaming disorder and the DSM‐5. 2013.
Gupta A, Khan AM, Rajoura O, Srivastava S. Internet addiction and its mental health correlates among undergraduate college students of a university in North India. J Family Med Primary Care. 2018;7(4):721.
Younes F, Halawi G, Jabbour H, El Osta N, Karam L, Hajj A, et al. Internet addiction and relationships with insomnia, anxiety, depression, stress and self-esteem in university students: a cross-sectional designed study. PLoS ONE. 2016;11(9):e0161126.
Seyrek S, Cop E, Sinir H, Ugurlu M, Şenel S. Factors associated with Internet addiction: Cross-sectional study of Turkish adolescents. Pediatr Int. 2017;59(2):218–22.
Jun S, Choi E. Academic stress and Internet addiction from general strain theory framework. Comput Hum Behav. 2015;49:282–7.
Chou C, Hsiao M-C. Internet addiction, usage, gratification, and pleasure experience: the Taiwan college students’ case. Comput Educ. 2000;35(1):65–80.
Yang T, Yu L, Oliffe JL, Jiang S, Si Q. Regional contextual determinants of internet addiction among college students: a representative nationwide study of China. Eur J Public Health. 2017;27(6):1032–7.
Ching SM, Awang H, Ramachandran V, Lim S, Sulaiman W, Foo Y, et al. Prevalence and factors associated with internet addiction among medical students-A cross-sectional study in Malaysia. Med J Malaysia. 2017;72(1):7–11.
Haque M, Rahman NAA, Majumder MAA, Haque SZ, Kamal ZM, Islam Z, et al. Internet use and addiction among medical students of Universiti Sultan Zainal Abidin. Malaysia Psychol Res Behav Manag. 2016;9:297.
Jelenchick LA, Becker T, Moreno MA. Assessing the psychometric properties of the internet addiction test (IAT) in US college students. Psychiat Res. 2012;196(2–3):296–301.
Bahrainian SA, Alizadeh KH, Raeisoon M, Gorji OH, Khazaee A. Relationship of Internet addiction with self-esteem and depression in university students. J Prevent Med Hygiene. 2014;55(3):86.
Tateno M, Teo AR, Shirasaka T, Tayama M, Watabe M, Kato TA. Internet addiction and self-evaluated attention-deficit hyperactivity disorder traits among Japanese college students. Psychiatry Clin Neurosci. 2016;70(12):567–72.
Al-Gamal E, Alzayyat A, Ahmad MM. Prevalence of internet addiction and its association with psychological distress and coping strategies among university students in Jordan. Perspect Psychiat Care. 2016;52(1):49–61.
Kuss DJ, Van Rooij AJ, Shorter GW, Griffiths MD, van de Mheen D. Internet addiction in adolescents: prevalence and risk factors. Comput Hum Behav. 2013;29(5):1987–96.
Frangos CC, Frangos CC, Sotiropoulos I, editors. A meta-analysis of the reliabilty of young’s internet addiction test. Proceedings of the World Congress on Engineering; 2012: World Congress on Engineering London, United Kingdom.
Young KS. Internet addiction test. Center for on-line addictions. 2009.
Tsimtsiou Z, Haidich A-B, Spachos D, Kokkali S, Bamidis P, Dardavesis T, et al. Internet addiction in Greek medical students: an online survey. Acad Psychiatry. 2015;39(3):300–4.
Balhara YPS, Gupta R, Atilola O, Knez R, Mohorović T, Gajdhar W, et al. Problematic internet use and its correlates among students from three medical schools across three countries. Acad Psychiatry. 2015;39(6):634–8.
Kessler RC, Barker PR, Colpe LJ, Epstein JF, Gfroerer JC, Hiripi E, et al. Screening for serious mental illness in the general population. Arch Gen Psychiat. 2003;60(2):184–9.
Kessler RC, Andrews G, Colpe LJ, Hiripi E, Mroczek DK, Normand S-L, et al. Short screening scales to monitor population prevalences and trends in non-specific psychological distress. Psychol Med. 2002;32(6):959–76.
Tadegge AD. The mental health consequences of intimate partner violence against women in Agaro Town, southwest Ethiopia. Trop Doct. 2008;38(4):228–9.
Hanlon C, Medhin G, Selamu M, Breuer E, Worku B, Hailemariam M, et al. Validity of brief screening questionnaires to detect depression in primary care in Ethiopia. J Affect Disord. 2015;186:32–9.
Fekadu A, Medhin G, Selamu M, Hailemariam M, Alem A, Giorgis TW, et al. Population level mental distress in rural Ethiopia. BMC Psychiat. 2014;14(1):194.
Andrews G, Slade T. Interpreting scores on the Kessler psychological distress scale (K10). Aust N Z J Public Health. 2001;25(6):494–7.
Arbabisarjou A, Gorgich EAC, Barfroshan S, Ghoreishinia G. The Association of internet addiction with academic achievement, emotional intelligence and strategies to prevention of them from student’s perspectives. Int J Hum Cult Stud (IJHCS). 2016;3(1):1656–66.
Gorgich EAC, Moftakhar L, Barfroshan S, Arbabisarjou A. Evaluation of internet addiction and mental health among medical sciences students in the southeast of Iran. Shiraz E Med J. 2018;19(1).
Frangos CCFC, Sotiropoulos I. A meta-analysis of the reliability of young’s internet addiction test. A meta-analysis of the reliability of young’s internet addiction test. WCE. 2012;1:368–71.
Khantzian EJ. The self-medication hypothesis of substance use disorders: A reconsideration and recent applications. Harvard Rev Psychiat. 1997;4(5):231–44.
Yen J-Y, Ko C-H, Yen C-F, Wu H-Y, Yang M-J. The comorbid psychiatric symptoms of Internet addiction: attention deficit and hyperactivity disorder (ADHD), depression, social phobia, and hostility. J Adolesc Health. 2007;41(1):93–8.
Association AP. Diagnostic and statistical manual of mental disorders (DSM-5®): American Psychiatric Pub; 2013.
Sajeev Kumar P, Prasad N, Raj Z, Abraham A. Internet addiction and substance use disorders in adolescent students-a cross sectional study. J Int Med Dent. 2015;2:172–9.
Ko C-H, Yen J-Y, Chen C-C, Chen S-H, Wu K, Yen C-F. Tridimensional personality of adolescents with internet addiction and substance use experience. Can J Psychiat. 2006;51(14):887–94.
Download references
Acknowledgements
We thank the Department of Psychiatry, College of Medicine and Health Sciences, Wollo University for supporting the research in different ways. We extend our heartfelt thanks to the student service directorate office for providing us the necessary information. We are grateful to all the students who participated in the study.
Not applicable.
Author information
Authors and affiliations.
Department of Psychiatry, College of Medicine and Health Sciences, Wollo University, Dessie, Ethiopia
Yosef Zenebe, Kunuya Kunno, Meseret Mekonnen, Ajebush Bewuket, Mengesha Birkie, Mogesie Necho, Muhammed Seid & Million Tsegaw
Department of Pharmacy, College of Medicine and Health Sciences, Wollo University, Dessie, Ethiopia
You can also search for this author in PubMed Google Scholar
Contributions
YZ and KK designed and supervised the study, carried out the analysis, and interpreted the data; MM, AB, MB, MNA, MS, MT, and BA assisted in the design, analysis, and interpretation of the data; and YZ wrote the manuscript. All authors contributed toward data analysis, drafting, and critically revising the paper and agree to be accountable for all aspects of the work. All authors read and approved the final manuscript.
Corresponding author
Correspondence to Yosef Zenebe .
Ethics declarations
Ethics approval and consent to participate.
The study was conducted after getting ethical clearance from Wollo University College of medicine and health science institutional review board with a certificate of approval number: CMHS/508/2019. A formal letter of permission was obtained from the student service directorate of Wollo University. The respondents were informed about the aim of the study. Confidentiality was maintained by giving codes for respondents rather than recording their names. The privacy of the respondents was also assured since the anonymous data collection procedure was followed. The data collectors have informed the clients that they had the full right to discontinue or refuse to participate in the study. Written consent was obtained from each participant before administering the questionnaire.
Consent for publication
Competing interests.
The authors report no conflicts of interest in this work.
Additional information
Publisher's note.
Springer Nature remains neutral with regard to jurisdictional claims in published maps and institutional affiliations.
Rights and permissions
Open Access This article is licensed under a Creative Commons Attribution 4.0 International License, which permits use, sharing, adaptation, distribution and reproduction in any medium or format, as long as you give appropriate credit to the original author(s) and the source, provide a link to the Creative Commons licence, and indicate if changes were made. The images or other third party material in this article are included in the article's Creative Commons licence, unless indicated otherwise in a credit line to the material. If material is not included in the article's Creative Commons licence and your intended use is not permitted by statutory regulation or exceeds the permitted use, you will need to obtain permission directly from the copyright holder. To view a copy of this licence, visit http://creativecommons.org/licenses/by/4.0/ . The Creative Commons Public Domain Dedication waiver ( http://creativecommons.org/publicdomain/zero/1.0/ ) applies to the data made available in this article, unless otherwise stated in a credit line to the data.
Reprints and permissions
About this article
Cite this article.
Zenebe, Y., Kunno, K., Mekonnen, M. et al. Prevalence and associated factors of internet addiction among undergraduate university students in Ethiopia: a community university-based cross-sectional study. BMC Psychol 9 , 4 (2021). https://doi.org/10.1186/s40359-020-00508-z
Download citation
Received : 21 May 2020
Accepted : 21 December 2020
Published : 06 January 2021
DOI : https://doi.org/10.1186/s40359-020-00508-z
Share this article
Anyone you share the following link with will be able to read this content:
Sorry, a shareable link is not currently available for this article.
Provided by the Springer Nature SharedIt content-sharing initiative
BMC Psychology
ISSN: 2050-7283
- General enquiries: [email protected]

An official website of the United States government
The .gov means it’s official. Federal government websites often end in .gov or .mil. Before sharing sensitive information, make sure you’re on a federal government site.
The site is secure. The https:// ensures that you are connecting to the official website and that any information you provide is encrypted and transmitted securely.
- Publications
- Account settings
Preview improvements coming to the PMC website in October 2024. Learn More or Try it out now .
- Advanced Search
- Journal List
- J Educ Health Promot
A study of internet addiction and its effects on mental health: A study based on Iranian University Students
Javad yoosefi lebni.
1 Health Education and Health Promotion, Health Institute, Kermanshah University of Medical Sciences, Kermanshah, Iran
Razie Toghroli
2 Social Determinants in Health Promotion Research Center, Hormozgan Health Institute, Hormozgan University of Medical Sciences, Bandar Abbas, Iran
Jaffar Abbas
3 Antai College of Economics and Management/School of Media and Communication, Shanghai Jiao Tong University, Shanghai-China
Nazila NeJhaddadgar
4 Department of Health Care Services and Health Education, School of Health, Ardabil University of Medical Science, Ardabil, Iran
Mohammad Reza Salahshoor
5 Department of Anatomical Sciences, Medical School, Kermanshah University of Medical Sciences, Kermanshah, Iran
Morteza Mansourian
6 Health Management and Economics Research Center, Iran University of Medical Sciences, Tehran, Iran
Hadi Darvishi Gilan
Neda kianipour.
7 Students Research Committee, Kermanshah University of Medical Sciences, Kermanshah, Iran
Fakhreddin Chaboksavar
Seyyed amar azizi, arash ziapour, introduction:.
The Internet has drastically affected human behavior, and it has positive and negative effects; however, its excessive usage exposes users to internet addiction. The diagnosis of students' mental dysfunction is vital to monitor their academic progress and success by preventing this technology through proper handling of the usage addiction.
MATERIALS AND METHODS:
This descriptive-analytical study selected 447 students (232 females and 215 males) of the first and second semesters enrolled at Kermanshah University of Medical Sciences, Iran, in 2018 by using Cochrane's sample size formula and stratified random sampling. The study applied Young's Internet Addiction Test and Goldberg General Health Questionnaire 28 for data collection. The study screened the data received and analyzed valid data set through the t -test and Pearson's correlation coefficient by incorporating SPSS Statistics software version 23.0.
The results of the current study specified that the total mean score of the students for internet addiction and mental health was 3.81 ± 0.88 and 2.56 ± 0.33, correspondingly. The results revealed that internet addiction positively correlated with depression and mental health, which indicated a negative relationship ( P > 0.001). The multiple regression analysis results showed students' five significant vulnerability predictors toward internet addiction, such as the critical reason for using the Internet, faculty, depression, the central place for using the Internet, and somatic symptoms.
CONCLUSIONS:
The study findings specified that students' excessive internet usage leads to anxiety, depression, and adverse mental health, which affect their academic performance. Monitoring and controlling students' internet addiction through informative sessions on how to use the Internet adequately is useful.
Introduction
In recent years, technological advancements have taken place in the modern world. In the complexity of today's world, internet use is playing a vital role in educational institutions to attain different learning skills, which have become a necessity for university students. However, scholars have shown concerns about the excessive use of this technology and the hidden risk factors of internet users, such as physical and mental health.[ 1 , 2 ] The Internet is an easy and quick medium of interaction to gain the required information for communication with others around the world. However, a lack of control over excessive internet use can disturb individuals' living standards and relationships between family members, and it can bring instability of feelings.[ 3 , 4 ] The users of the Internet have increased incredibly worldwide, with the peak of a digital industrial revolution in progress, and new technological revolution will undoubtedly create new problems and predicaments.[ 4 , 5 ] The history of internet users goes back some decades at present. The Internet has become one of the most fast-growing and transformative technologies. Globally, the users of the Internet have increased from 414 million in 2000, 665 million in 2002, and over 4.574 billion by December 31, 2019. The US National Science Foundation specified that the internet users enabled by smartphones access would increase to 5 billion in 2020.[ 6 , 7 ] In recent years, internet users in Iran have grown dramatically. According to the reported statistics, the Iranian users of the internet have risen from 11.0 million in 2006, 33.0 million in 2002, and over 62 million by July 1, 2019. Hence, the users of the Internet in Iran have increased drastically over 25 times, and recent research conducted in Iran indicated that the young population makes up the majority of internet users.[ 8 ] Previous research specified that the Iranian users devote 35% of their time to chat rooms, 28% to online games, 30% to checking E-mails, and 25% to surfing the net on averages, while connected to the Internet. Besides, another study reported that Iranian users spend 52 min/week as an average time, while linked to the Internet.[ 9 ]
Internet addiction disorder, pathological internet use, or problematic internet use typically refers to the questionable or compulsive use of the Internet, which results in substantial impairment in the function of individuals in their different life domains over prolonged time. Internet addiction and other relationships based on the usage of digital media and mental health are vital considerable research fields, arguments, and discussions among numerous experts and researchers in various disciplines. This addictive behavior has made controversy from the areas of scientific, medical, and technological communities. Internet addiction is an interdisciplinary phenomenon, and different researchers have investigated it from different perspectives from various disciplines, such as medicine, computer science, sociology, law, and psychology.[ 10 ] Some scholars have considered internet addiction as a social crisis, and it has attracted the attention of different researchers and experts. This phenomenon is a biological, psychological, social, economic, and cultural problem, which is impossible to be taken into account as a simple matter because different factors influence it.[ 11 ] The excessive and pathological use of the Internet refers to internet addiction.[ 6 ] Therefore, with the growing number of internet users and its widespread psychological and sociological implications, it is necessary to determine and recognize the contribution of predictive factors in internet addiction. By conducting pathological studies about internet addiction to judge the addictive behaviors, it would enable us to utilize this technology with a balanced approach better and more usefully.[ 12 ] Internet addiction generally refers to a type of applying the Internet, which leads to psychological, social, educational, or occupational problems in a person's life.[ 13 , 14 ] Scholars have described this phenomenon as internet addiction dysfunction[ 15 ] and the problematic application of the Internet,[ 16 ] or habitual use of the Internet,[ 17 ] which determines it as one of the forms of behavioral addiction.[ 18 ] Researchers have also described internet addiction as “the modern addiction.” In practice, this type of addiction is true dependency, like drug addiction and other kinds of dependency. Although this kind of dependency does not have the somatic problems of chemical addiction, its resultant social problems are like other types of addiction.[ 1 ] In the 2015 World Statistics report, the number of internet users and the population of countries were specified; it was reported that the total world population was 7,264,623,793, of which 3,079,339,857 were using the Internet, and the young made up the majority of users.[ 8 ] While taking into account many points of proper and practical use of the Internet and prevention of mental illness, these reported statistics underscore the importance of the Internet and social networks. Internet addiction is an etymological process of using the Internet that creates a psychological state in which the user's behavior is disturbed, thereby leading to a dysfunction in his/her cognitive status.[ 19 ] Mental health is one of the main pillars of healthy human societies, which plays a vital role in ensuring the dynamism and efficiency of any society. As university students are among the most prestigious layers of societies, they present future builders in any country, and newly arrived students in universities from far-away cities are the first who fall victim to internet addiction. The mental health of the students is essential for raising their learning and scientific awareness.[ 19 ] Mental health is a concept that reflects our thinking, feelings, and functioning in dealing with various life situations.[ 20 ] In this modern world, the disease patterns are shifting toward no communicable diseases, and the rising rate of mental dysfunction and the resultant costs imposed on societies have attracted the attention of health promotion specialists.[ 8 ] In this regard, the Global Burden of Disease statistics has introduced mental illnesses as one of the three primary causes of lost years of life due to disability.[ 21 ] According to the WHO, mental health is defined as one's ability to communicate with others harmoniously; modify the personal and social environment; and resolve conflicts and personal preferences logically, fairly, and appropriately.[ 22 ] Besides, the statistics announced by the WHO reported that 52 million people of different age groups suffer from severe illnesses worldwide and 250 million have mild mental dysfunction. In Iran, these statistics are not lower than those in other countries.[ 23 ] The results of the epidemiological studies conducted to examine psychiatric dysfunction in Iran are indicative of the variability of the prevalence of dysfunction between 11.9% and 30.2%.[ 24 , 25 ]
Concerning internet addiction, addressing the problems of individuals' mental health is of great importance. An earlier study conducted by Fallah reported that depression was more prevailing among internet users with addictive behavior as compared with average internet users. The finding specified that individuals having internet addiction showed anxious behavior and their mental health was more exposed to higher risks.[ 23 ] Lashgarara et al . described that 34% of university students had addictive behavior to the Internet based on the Young's categorization.[ 26 ]
In a previous study, Fonia et al . reported that students' mental health and internet addiction showed a negative relationship, and their internet addictive behavior was not significant. It was different from the students' gender and marital status variables.[ 27 ] Another study of Nastizai claimed that students' internet addiction developed a higher risk of mental health than ordinary users of the Internet.[ 28 ] Fonia et al . reported that there was a significant difference between internet addiction among male and female students.[ 27 ] Similarly, the relationship between internet addiction and users' mental health received more considerable attention, and previous studies have emphasized this matter, such as the investigations of Fallah Mehneh,[ 29 ] Alavi et al .,[ 30 ] Mirzaian et al .,[ 31 ] and Taheri Mobarakeh et al .[ 32 ] The tendency of using the Internet among students is higher, and they are more vulnerable to the risk of internet addiction. Thus, more attention to students' mental health needs should be considerable paid for their future as well as the development of the nation.[ 22 , 25 ] Universities need to pay attention to boosting students' mental health, personal growth, and well-being. Because internet addiction prevails worldwide, it also exists in Iran for several years, and young individuals have shown greater engagement toward internet use, while students make up the majority of internet users. The excessive use of the Internet leads to psychological injury, mental health damage, and other health problems. Experts have suggested necessary measures to prevent internet addiction among students and treat disorders and health problems where appropriate.[ 33 ] The present research emphasized investigating internet addiction and its effects on the mental health of medical students at Kermanshah University of Medical Sciences, and its findings provide valuable insights.
Materials and Methods
This descriptive-analytical study selected 447 students (232 females and 215 males) of the first and second semesters enrolled at Kermanshah University of Medical Sciences, in 2018 (May 2017–October 2018) by applying Cochrane's sample size formula and stratified random sampling methods. This method draws the statistical population according to the hierarchy of the types of population units. The study applied Young's Internet Addiction Test (IAT) and Goldberg General Health Questionnaire (GHQ-28) for data collection. The study screened the data received and analyzed valid data set through the t -test and Pearson's correlation coefficient by incorporating SPSS Statistics software version 24.0. Hence, the authors randomly selected nine faculties (medicine, dentistry, pharmaceutical medicine, nursing and midwifery, paramedics, public health, nutrition sciences, and food industries, and self-governing college). In the next step, we selected majors and classes from each faculty (as many as the number of research samples) and evaluated the data. The inclusion criterion was the right to choose the courses freely, and the investigators have excluded incomplete questionnaires from the study. Besides, we assured participants about the confidentiality of the collected information and lack of disclosure of their personal information. Besides, the ethical principles employed in the present study included critical steps, such as obtaining the necessary permits, retaining the right for the schools under investigation to either accept or reject to participate in the research study, and ensuring confidentiality and nondisclosure of agreement. The investigators distributed the questionnaires among the target respondents. The authors explained the objectives of the present study to the target individuals and obtained informed consent from all participants before to execute the research. Not to mention, the exclusion criteria were the sample's disinterest in participating in the study and handing over incomplete questionnaires.
Demographic questionnaire
The first section of the self-administered instrument contained the demographics and comprised questions on gender, age, marital status, place of residence, faculty, education, having a personal computer, central location, and time of using the Internet, and the primary reason for using the Internet.
Internet Addiction Test
Young developed this 20-item scale for measuring the internet addiction, which affects a variety of aspects in users' lives.[ 17 ] The study applied the Persian translation of Young's IAT developed by Alavi et al .[ 30 ] The questions showed the scores on a selected 5-point Likert scale (5 = always, 4 = usually, 3 = most of the time, 2 = sometimes, and 1 = seldom). The ranges of minimum and maximum scores showed 20–100. The scores divided internet users into the following three groups: typical users indicating a score of 20–49; at-risk users, specifying a score of 50–79; and the internet users having an addiction with a score of 80–100. The highest scores represent the highest levels of students' dependency on the Internet, which leads to addictive behavior. Recent studies evidenced that a score of 50 or above shows internet addiction. Besides, this study confirmed the questionnaire's validity from three experts by applying the content validity index (0.84), while the survey confirmed reliability through the t -test (0.88). The Cronbach's alpha provided a satisfactory value (0.87) with a sample of twenty medical students within 2-week process of data collection. The findings of the reliability and validity of this instrument/tool are consistent with the results of previous studies, which indicated over 90%.[ 34 , 35 , 36 , 37 , 38 ]
The General Health Questionnaire
This prospective study used GHQ-28 for gathering data.[ 39 ] The study screened the data received and analyzed valid data set through the t -test and Pearson's correlation coefficient by using the SPSS Statistics software version 24.0. The self-reported Goldberg's 28-item questionnaire examines the individual's mental health in the recent month and includes symptoms such as abnormal thoughts and feelings and aspects of visible behavior. This questionnaire consists of the following four subscales: somatic symptoms (questions 1–7), anxiety (questions 8–14), social dysfunction (questions 15–21), and depression (questions 22–28). Each subscale contains seven questions that measure the various aspects of mental health, ranging from somatic to psychological dysfunction.[ 39 ] The questions presented scores with a 4-point Likert scale (0 = not at all, 1 = average, 2 = more than average, and 3 = far more than average). The minimum and maximum ranges illustrated 0–84, which showed categories into four levels of mental health, for instance, normal (0–22), weak (21–40), balanced (41–60), and severe (61–84). The highest ratings/scores represented the lowest level of students' mental health status. The study examined and confirmed the questionnaire's reliability for each section by using content validity (0.80). The sought the opinion from three experts by using the content validity index (0.80) and confirmed reliability through several tests, such as test-retest (0.87). The study calculated the Cronbach's alpha (0.93) value from the sample of twenty medical students. The results derived from the tests of reliability and validity of this instrument are in line with the findings of previous global studies, which specified the same results.[ 9 , 40 , 41 , 42 ]
The study applied descriptive statistics (percentage, mean, and standard deviation) and inferential statistics ( t -test and Pearson's correlation coefficient) to analyze the data received by using the SPSS Statistics software (version 23.0, SPSS Inc., Chicago, IL, USA), and applied required analysis at the statistical significance level of 0.05 ( P < 0.01).
Ethical consideration
This study is the part of the research project (IR.KUMS.REC.1397.108, No. 97056) sponsored by the Deputy of Research and Technology from Kermanshah University of Medical Sciences, Iran. The authors maintained all the protocols before performing all the procedures engaged in this study involving human participants in accordance with the ethical standards of the institutional research committee and with the 1964 Helsinki declaration and its later amendments or comparable ethical standards.
The total population comprised of 447 participants in the present study, including 215 male (48.1%) and 232 female (51.9%) students. The average age of the respondents under investigation was 23.47 ± 4.58 years, and the majority of respondents' age ranged between 19 and 24 years (69.6%). Concerning the marital status, there were 360 single participants (80.50%) in this population. The second majority of the study indicated bachelor's degree students (202 students or 45.20%), and the majority of the discipline was a school of medicine (71 students or 15.90%). The majority of the dormitory students comprised 48.30% (216/447). The study findings specified that 356 students possessed their computers (356/447 = 79.60%), and the number of students using the Internet at their dormitories comprised 205 respondents (205/447 = 45.90%). The results indicated that the majority of the students used the Internet either in the morning or in the evening (367/447 = 82.10%).
The main reason for using the internet application was chatting with friends and family members. The mean and standard deviation of students with internet addiction was 3.81 ± 0.88. Besides, the mean and standard deviation of students' mental health was 2.56 ± 0.33, which stated that the general mental health of students was not in good condition. Regarding the mental health of the students' sample, the study results indicated that the highest and lowest rates showed linkage to depression with a mean and standard deviation of 2.84 ± 0.21 and somatic dysfunction with a mean and standard deviation of 2.16 ± 0.79, respectively, as indicated in Table 1 . This specific study applied the Pearson's correlation coefficient to determine the relationship between the students' internet addiction and mental health. The results of the correlation matrix demonstrated that they did not statistically significantly correlate with each other ( P < 0.001, r = 0.052). The study results specified that students' depression and somatic symptoms had the highest ( P = 0.001, r = 0.166) and lowest ( P > 0.001, r = 0.006) relationships with internet addiction, as indicated in Table 2 .
Internet addiction and mental health scores for different genders
The results of Pearson’s correlation coefficient between internet addiction and mental health among students
**Correlation was significant at the 0.01 level (two tailed)
The present study aimed to investigate internet addiction and its effects on the mental health of medical students at Kermanshah University of Medical Sciences. The results of the present study demonstrated that 45.5% of students at Kermanshah University of Medical Sciences were addicted to the Internet. This finding was concurrent with the results of studies conducted by Farhadinia et al .,[ 43 ] Sepehrian and Jokar,[ 44 ] Fonia et al .,[ 27 ] and Dargahi and Razavi.[ 45 ] Those who use the Internet more than others can replace stronger relationships in real life with low-quality social relationships, thereby resulting in more loneliness and depression. To further explicate the matter, the Internet may serve as a substitute for lives without vitality. Loneliness and isolation may cause people to spend more time on the Internet, thereby decreasing the quality of their social relationships.
As for the demographic characteristics, the results demonstrated that there was a significant difference between male and female students in terms of internet addiction. In addition, 23% of male students were internet addicts, which exceeded that of female students by 22.4%. In this study, male students should be given priority in prevention programs for internet addiction. These results were consistent with the results of studies conducted by Alavi et al .,[ 30 ] Orsal et al .,[ 46 ] and Fonia et al .,[ 27 ] whereas inconsistent with the results of studies performed by Atashpour et al .[ 47 ] and Shahbazirad and Mirderikvand.[ 48 ] Male students seem to have more internet addiction than girls. In fact, the present research, in line with the findings of previous studies, shows that men are more exposed to internet addiction, not because of biological differences between the two genders, but due to different social and environmental factors to which each gender is exposed. According to the results, it seems that this finding can be an alarm at the increase in this disorder among students, and it is better that proper planning be done in this area in cooperation with university officials.
Based on the results of the present study, the mean score of male students' mental health was higher than that of female students, and no significant difference was seen between gender and mental health. However, the finding of the present study was consistent with the results of studies conducted by Taji and Verdinejad,[ 49 ] Namdar et al .,[ 50 ] and Imani et al .[ 51 ] In studies done by Asadi et al .,[ 52 ] Gorgich et al .,[ 9 ] Fonia et al .,[ 27 ] and Xu and Liu,[ 23 ] it was expressed that female students had more mental disorders than male students, which was inconsistent with the results of the present study. It should be noted that the mean score of male students' mental health was higher than that of female students, possibly due to men's ability to communicate with others in the society and university, the ability to deal with problems and difficulties, and the ability to earn money, as well as women's excessive emotional dependency on their families and lack of social security in the society.
The results of this study revealed that half of the students had poor mental health, and there was a significant difference between the mean scores of depression and internet addiction. These results were consistent with the results of studies done by Nastizai,[ 28 ] Anderson et al .,[ 12 ] and Chung and Wong.[ 53 ] In a study done by Abdollahi on nursing students at Tehran University of Medical Sciences, it was shown that 32.1% of students had suspected mental disorders, which was 29.7% in women and 34.3% in men.[ 54 ] In addition, Rafiei and Mosavipour showed that 67.9% of students at Arak University of Medical Sciences had symptoms of mental disorders, and only 32.1% of them had normal mental health.[ 55 ] Similarly, Masoudi et al . concluded that 52.4% of students at Tehran University of Medical Sciences were suffering from mental health disorders.[ 56 ] Similarly, in a study done by Yavarian et al . on students at Uromia University of Medical Sciences, it was demonstrated that 45.8% of students had different degrees of mental health disorders. In their study, it was also revealed that 10%, 0.5%, and 3.2% of students had severe disorders in terms of somatic symptoms, anxiety and insomnia, and depression, respectively. This finding was concurrent with the results of the present study.[ 57 ] It seems that the different prevalence of psychiatric disorders in various studies can be attributed to several factors, including the differences in groups under study.
The results revealed that internet addiction and mental health were negatively related, which was consistent with the results of studies conducted by Shahbazirad and Mirderikvand,[ 48 ] Fallah Mehneh,[ 58 ] and Mousavomoghadam et al .[ 59 ] Hosseini et al . showed that 4.2% of students at Payam Noor University of Charm had severe addiction to the Internet. In addition, a significant relationship was observed between internet addiction and mental health.[ 60 ] Similarly, in a study performed by Farhadinia et al ., it was demonstrated that internet addiction and mental health significantly correlated among the students of Lorestan University of Medical Sciences,[ 43 ] which seems to lay the groundwork for the internet addicts. Some people resort to the Internet to reduce their depression. In this case, the Internet may provide a substitute for the joyless lives of depressed people, or they may get depressed as a result of internet addiction. In other words, the internet addicts will experience the negative consequences, such as depression.
In justifying the relationship between depression and internet addiction, it can be expressed that the excessive use of the Internet can lead to social isolation and depression through reducing familial, social, and local connection. Therefore, depression may occur as a result of internet addiction, and in this case, the internet addicts experience the resultant negative consequences, such as depression.[ 28 ]
The results revealed that the five major predictors of vulnerability to internet addiction in university students were as follows: the key reason for using the Internet, faculty, depression, the main place for using the Internet, and somatic symptoms.
Limitations of the study
Concerning the limitations, this study evaluated medical students in the classrooms based on different groups. The discussions among students could present bias in their feedback. There was no face-to-face interview session, and the data set reported on a self-reported questionnaire, which increases the risk of social desirability biases among medical students of medicine, dentistry, and pharmaceutical departments at self-governing Education Incubator of Kermanshah, Iran. The limitations of the current study specify that researchers can consider a large sample size based on medical students as well as other departments to execute their investigations in western part of Iran. Scholars can find experimental and longitudinal methods with larger samples to examine different results.
Conclusions
According to the findings of the present study, it can be concluded that students' excessive use of the Internet leads to depression, anxiety, and reduced mental health, thereby affecting their academic performance. Hence, it is suggested that further monitoring and control be exercised on how the Internet is used by university students, and they should be informed of the detrimental effects of this technology in the case of misuse or overuse. The findings of the present study are also indicative of the significance of preventative measures in the form of educational and counseling programs for students regarding the proper and practical use of the Internet. In addition, addressing the issues and problems relating to communication technologies, such as the Internet, can lay the groundwork for proper education and instigate parents' and families' further attention to proper and effective use of the Internet.
Financial support and sponsorship
Conflicts of interest.
There are no conflicts of interest.
Acknowledgments
The authors hereby bestow their gratitude to the students in the for-profit Schools of Medicine, Dentistry, Paramedics, Nursing and Midwifery, Paramedicine, Nutrition Sciences and Food Industries, Public Health and Self-Governing College in Kermanshah University of Medical Sciences for their participation in the present study.
- My Shodhganga
- Receive email updates
- Edit Profile
Shodhganga : a reservoir of Indian theses @ INFLIBNET
- Shodhganga@INFLIBNET
- Andhra University
- Department of Psychology and Parapsychology
Items in Shodhganga are licensed under Creative Commons Licence Attribution-NonCommercial-ShareAlike 4.0 International (CC BY-NC-SA 4.0).

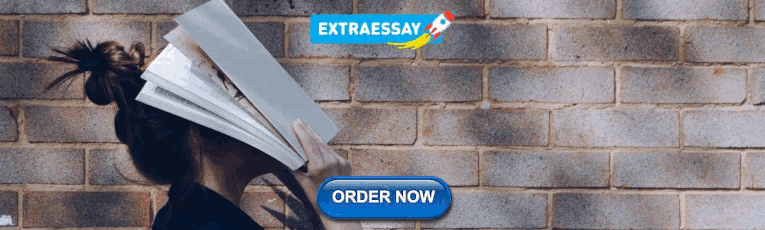
COMMENTS
INTRODUCTION. Over the last 15 years, the number of Internet users has increased by 1000%[], and at the same time, research on addictive Internet use has proliferated.Internet addiction has not yet been understood very well, and research on its etiology and natural history is still in its infancy[].Currently, it is estimated that between 0.8% of young individuals in Italy[] and 8.8% of Chinese ...
Introduction. The internet has become an indispensable part of modern society and its use has grown exponentially, causing internet addiction to become a growing concern across all age groups and countries.[] Uncontrolled use of the internet significantly affects not only individuals' quality of life and social functioning but impacts their physical and psychological health.[2,3] Despite its ...
For Internet addiction, we used 'Internet addiction (网络成瘾),' 'addiction (成瘾),' 'Internet abuse (网络滥用),' and 'Internet dependence (网络依赖).' The English-language articles were retrieved from the Google Scholar, ProQuest Dissertations, Taylor Francis, Springer, Web of Science, PsycINFO, EBSCO, Elsevier SDOL.
The term internet addiction was established by Young (1998a) to describe many types of excessive internet use, but has largely been replaced by more specific definitions, with gaming as the focal point of parental worries and researchers' interest (Bergmark & Bergmark, 2009). This problem was picked up by the two arguably most influential ...
Other scales were utilised to screen participants for Social Media Addiction (n:1), Internet Gaming Disorder (n:5), Smartphone Addiction (n:6), and Internet Addiction (n:12). One scale was used to screen participants for both Internet Addiction and Internet Gaming Disorder [30]. The screening instrument list is shown in Table 1. Of the studies ...
Internet addiction (IA), described as a behavioral addiction, manifested as repeated and unrestrained use of the internet, induces a series of cognitive and social impairments (1-3). With the advancement of the internet, IA is widely prevelant among teenagers in many countries around the world, reaching a maximum of 57.5% (4-6).
With Internet connectivity and technological advancement increasing dramatically in recent years, "Internet addiction" (IA) is emerging as a global concern. However, the use of the term 'addiction' has been considered controversial, with debate surfacing as to whether IA merits classification as a psychiatric disorder as its own entity, or whether IA occurs in relation to specific ...
Internet a ddiction during adolescence is a psychological phenomenon that has negative effects on mental health and. development. According to the results of the review, addiction is associated ...
ON THE NATURE OF INTERNET ADDICTION What is it and how is it measured? ACADEMIC DISSERTATION Academic dissertation to be publicly discussed, by due permission of the Faculty of Behavioural Sciences at the University of Helsinki in the Main Building Hall 5 (Fabianinkatu 33) on the 12 th of June, 2015 at 12 o clock. Helsinki 2015
The association between internet addiction, insomnia, and depression was explored in a study that observed that internet addiction and sleep quality independently mediated 16.5% and 30.9% indirect effect of each other on depression. Conclusion. Internet addiction among youth has become increasingly a great concern.
Predictive Effects of Academic Values on Satisfaction With Academic Performance. Results of multiple regression analyses revealed that intrinsic value and utility value positively predicted each wave's satisfaction with academic performance (b ranged between 0.15 and 0.23, ps < 0.001, Cohen's f 2 ranged from 0.022 to 0.057, see Table 6).Results also showed a longitudinal prediction of ...
Internet addiction (IA) is an extreme form of this phenomenon, an inability to avoid using the Internet that has adverse effects on various life aspects ... ProQuest was searched for thesis, dissertations, and studies presented at conferences. The search was performed using MESH and free keywords. The keywords selected for the search were ...
Background Internet addiction is a common problem in university students and negatively affects cognitive functioning, leads to poor academic performance and engagement in hazardous activities, and may lead to anxiety and stress. Behavioral addictions operate on a modified principle of the classic addiction model. The problem is not well investigated in Ethiopia. So the present study aimed to ...
attitudes predict Internet addiction and nomophobia. The sample included 250 college students (140 Caucasian, 110 African American) between the ages of 18 and 22. Participants completed the Horizontal & Vertical Individualism Collectivism II scale, the Internet Addiction Test, and the Nomophobia Questionnaire. The results revealed that
Internet addiction is expressed by Young (1996) as not being able to restrain one's desire to use the internet, and the time away from the internet feeling meaningless. It may lead to withdrawal symptoms (extreme irritability and aggression) similar to other addictions. Caplan (2002) simply defined internet addiction as the generally
The side effects of the excessiveness led to anxiety, depression, hea lth problems, school. absenteeism, lying, fatigue, unemployment, decreased job productivity, and social isolation. The ...
The results of the current study specified that the total mean score of the students for internet addiction and mental health was 3.81 ± 0.88 and 2.56 ± 0.33, correspondingly. The results revealed that internet addiction positively correlated with depression and mental health, which indicated a negative relationship (P > 0.001). The multiple ...
Results Table 1: Respondents’ Internet Addiction Level according to the modified criteria of Young’s (1998) Internet Addiction Test (IAT) No Level Frequency Percentage 1 Severe 6 5.0 2 Moderate 83 69.2 3 Mild 31 25.8 Total 120 100.0 Based on the total score, the respondents were categorized into 3 categories: mild, moderate and ...
Shodhganga. The Shodhganga@INFLIBNET Centre provides a platform for research students to deposit their Ph.D. theses and make it available to the entire scholarly community in open access. Shodhganga@INFLIBNET. Bundelkhand University. Department of Psychology.
Therefore, it newlineis important to assess the level of internet addiction and its associated psychological factors in them. The newlinemain objective of this research is to study the prevalence of internet addiction and its association with newlinedepression, anxiety, stress and the well-being. newline newline: dc.format.extent: dc.language ...
Adolescents using the internet for 7 and 5-6 hours constitute only 6,6% and 9,4% of study. participants respectively (Demir & Kutlu, 2018). Furthermore, it is impossible to tell for certain. how ...