- CBSE Class 10th
- CBSE Class 12th
- UP Board 10th
- UP Board 12th
- Bihar Board 10th
- Bihar Board 12th
- Top Schools in India
- Top Schools in Delhi
- Top Schools in Mumbai
- Top Schools in Chennai
- Top Schools in Hyderabad
- Top Schools in Kolkata
- Top Schools in Pune
- Top Schools in Bangalore
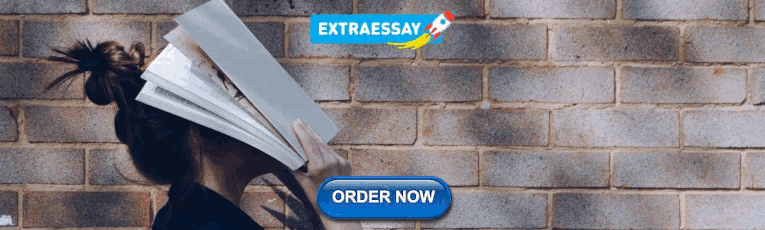
Products & Resources
- JEE Main Knockout April
- Free Sample Papers
- Free Ebooks
- NCERT Notes
- NCERT Syllabus
- NCERT Books
- RD Sharma Solutions
- Navodaya Vidyalaya Admission 2024-25
- NCERT Solutions
- NCERT Solutions for Class 12
- NCERT Solutions for Class 11
- NCERT solutions for Class 10
- NCERT solutions for Class 9
- NCERT solutions for Class 8
- NCERT Solutions for Class 7
- JEE Main 2024
- JEE Advanced 2024
- BITSAT 2024
- View All Engineering Exams
- Colleges Accepting B.Tech Applications
- Top Engineering Colleges in India
- Engineering Colleges in India
- Engineering Colleges in Tamil Nadu
- Engineering Colleges Accepting JEE Main
- Top IITs in India
- Top NITs in India
- Top IIITs in India
- JEE Main College Predictor
- JEE Main Rank Predictor
- MHT CET College Predictor
- AP EAMCET College Predictor
- GATE College Predictor
- KCET College Predictor
- JEE Advanced College Predictor
- View All College Predictors
- JEE Main Question Paper
- JEE Main Mock Test
- JEE Main Registration
- JEE Main Syllabus
- Download E-Books and Sample Papers
- Compare Colleges
- B.Tech College Applications
- GATE 2024 Result
- MAH MBA CET Exam
- View All Management Exams
Colleges & Courses
- MBA College Admissions
- MBA Colleges in India
- Top IIMs Colleges in India
- Top Online MBA Colleges in India
- MBA Colleges Accepting XAT Score
- BBA Colleges in India
- XAT College Predictor 2024
- SNAP College Predictor
- NMAT College Predictor
- MAT College Predictor 2024
- CMAT College Predictor 2024
- CAT Percentile Predictor 2023
- CAT 2023 College Predictor
- CMAT 2024 Registration
- TS ICET 2024 Registration
- CMAT Exam Date 2024
- MAH MBA CET Cutoff 2024
- Download Helpful Ebooks
- List of Popular Branches
- QnA - Get answers to your doubts
- IIM Fees Structure
- AIIMS Nursing
- Top Medical Colleges in India
- Top Medical Colleges in India accepting NEET Score
- Medical Colleges accepting NEET
- List of Medical Colleges in India
- List of AIIMS Colleges In India
- Medical Colleges in Maharashtra
- Medical Colleges in India Accepting NEET PG
- NEET College Predictor
- NEET PG College Predictor
- NEET MDS College Predictor
- DNB CET College Predictor
- DNB PDCET College Predictor
- NEET Application Form 2024
- NEET PG Application Form 2024
- NEET Cut off
- NEET Online Preparation
- Download Helpful E-books
- LSAT India 2024
- Colleges Accepting Admissions
- Top Law Colleges in India
- Law College Accepting CLAT Score
- List of Law Colleges in India
- Top Law Colleges in Delhi
- Top Law Collages in Indore
- Top Law Colleges in Chandigarh
- Top Law Collages in Lucknow
Predictors & E-Books
- CLAT College Predictor
- MHCET Law ( 5 Year L.L.B) College Predictor
- AILET College Predictor
- Sample Papers
- Compare Law Collages
- Careers360 Youtube Channel
- CLAT Syllabus 2025
- CLAT Previous Year Question Paper
- AIBE 18 Result 2023
- NID DAT Exam
- Pearl Academy Exam
Animation Courses
- Animation Courses in India
- Animation Courses in Bangalore
- Animation Courses in Mumbai
- Animation Courses in Pune
- Animation Courses in Chennai
- Animation Courses in Hyderabad
- Design Colleges in India
- Fashion Design Colleges in Bangalore
- Fashion Design Colleges in Mumbai
- Fashion Design Colleges in Pune
- Fashion Design Colleges in Delhi
- Fashion Design Colleges in Hyderabad
- Fashion Design Colleges in India
- Top Design Colleges in India
- Free Design E-books
- List of Branches
- Careers360 Youtube channel
- NIFT College Predictor
- UCEED College Predictor
- NID DAT College Predictor
- IPU CET BJMC
- JMI Mass Communication Entrance Exam
- IIMC Entrance Exam
- Media & Journalism colleges in Delhi
- Media & Journalism colleges in Bangalore
- Media & Journalism colleges in Mumbai
- List of Media & Journalism Colleges in India
- CA Intermediate
- CA Foundation
- CS Executive
- CS Professional
- Difference between CA and CS
- Difference between CA and CMA
- CA Full form
- CMA Full form
- CS Full form
- CA Salary In India
Top Courses & Careers
- Bachelor of Commerce (B.Com)
- Master of Commerce (M.Com)
- Company Secretary
- Cost Accountant
- Charted Accountant
- Credit Manager
- Financial Advisor
- Top Commerce Colleges in India
- Top Government Commerce Colleges in India
- Top Private Commerce Colleges in India
- Top M.Com Colleges in Mumbai
- Top B.Com Colleges in India
- IT Colleges in Tamil Nadu
- IT Colleges in Uttar Pradesh
- MCA Colleges in India
- BCA Colleges in India
Quick Links
- Information Technology Courses
- Programming Courses
- Web Development Courses
- Data Analytics Courses
- Big Data Analytics Courses
- RUHS Pharmacy Admission Test
- Top Pharmacy Colleges in India
- Pharmacy Colleges in Pune
- Pharmacy Colleges in Mumbai
- Colleges Accepting GPAT Score
- Pharmacy Colleges in Lucknow
- List of Pharmacy Colleges in Nagpur
- GPAT Result
- GPAT 2024 Admit Card
- GPAT Question Papers
- NCHMCT JEE 2024
- Mah BHMCT CET
- Top Hotel Management Colleges in Delhi
- Top Hotel Management Colleges in Hyderabad
- Top Hotel Management Colleges in Mumbai
- Top Hotel Management Colleges in Tamil Nadu
- Top Hotel Management Colleges in Maharashtra
- B.Sc Hotel Management
- Hotel Management
- Diploma in Hotel Management and Catering Technology
Diploma Colleges
- Top Diploma Colleges in Maharashtra
- UPSC IAS 2024
- SSC CGL 2024
- IBPS RRB 2024
- Previous Year Sample Papers
- Free Competition E-books
- Sarkari Result
- QnA- Get your doubts answered
- UPSC Previous Year Sample Papers
- CTET Previous Year Sample Papers
- SBI Clerk Previous Year Sample Papers
- NDA Previous Year Sample Papers
Upcoming Events
- NDA Application Form 2024
- UPSC IAS Application Form 2024
- CDS Application Form 2024
- CTET Admit card 2024
- HP TET Result 2023
- SSC GD Constable Admit Card 2024
- UPTET Notification 2024
- SBI Clerk Result 2024
Other Exams
- SSC CHSL 2024
- UP PCS 2024
- UGC NET 2024
- RRB NTPC 2024
- IBPS PO 2024
- IBPS Clerk 2024
- IBPS SO 2024
- Top University in USA
- Top University in Canada
- Top University in Ireland
- Top Universities in UK
- Top Universities in Australia
- Best MBA Colleges in Abroad
- Business Management Studies Colleges
Top Countries
- Study in USA
- Study in UK
- Study in Canada
- Study in Australia
- Study in Ireland
- Study in Germany
- Study in China
- Study in Europe
Student Visas
- Student Visa Canada
- Student Visa UK
- Student Visa USA
- Student Visa Australia
- Student Visa Germany
- Student Visa New Zealand
- Student Visa Ireland
- CUET PG 2024
- IGNOU B.Ed Admission 2024
- DU Admission
- UP B.Ed JEE 2024
- DDU Entrance Exam
- IIT JAM 2024
- IGNOU Online Admission 2024
- Universities in India
- Top Universities in India 2024
- Top Colleges in India
- Top Universities in Uttar Pradesh 2024
- Top Universities in Bihar
- Top Universities in Madhya Pradesh 2024
- Top Universities in Tamil Nadu 2024
- Central Universities in India
- CUET PG Admit Card 2024
- IGNOU Date Sheet
- CUET Mock Test 2024
- CUET Application Form 2024
- CUET PG Syllabus 2024
- CUET Participating Universities 2024
- CUET Previous Year Question Paper
- CUET Syllabus 2024 for Science Students
- E-Books and Sample Papers
- CUET Exam Pattern 2024
- CUET Exam Date 2024
- CUET Syllabus 2024
- IGNOU Exam Form 2024
- IGNOU Result
- CUET PG Courses 2024
Engineering Preparation
- Knockout JEE Main 2024
- Test Series JEE Main 2024
- JEE Main 2024 Rank Booster
Medical Preparation
- Knockout NEET 2024
- Test Series NEET 2024
- Rank Booster NEET 2024
Online Courses
- JEE Main One Month Course
- NEET One Month Course
- IBSAT Free Mock Tests
- IIT JEE Foundation Course
- Knockout BITSAT 2024
- Career Guidance Tool
Top Streams
- IT & Software Certification Courses
- Engineering and Architecture Certification Courses
- Programming And Development Certification Courses
- Business and Management Certification Courses
- Marketing Certification Courses
- Health and Fitness Certification Courses
- Design Certification Courses
Specializations
- Digital Marketing Certification Courses
- Cyber Security Certification Courses
- Artificial Intelligence Certification Courses
- Business Analytics Certification Courses
- Data Science Certification Courses
- Cloud Computing Certification Courses
- Machine Learning Certification Courses
- View All Certification Courses
- UG Degree Courses
- PG Degree Courses
- Short Term Courses
- Free Courses
- Online Degrees and Diplomas
- Compare Courses
Top Providers
- Coursera Courses
- Udemy Courses
- Edx Courses
- Swayam Courses
- upGrad Courses
- Simplilearn Courses
- Great Learning Courses
Access premium articles, webinars, resources to make the best decisions for career, course, exams, scholarships, study abroad and much more with
Plan, Prepare & Make the Best Career Choices
Covid 19 Essay in English
Essay on Covid -19: In a very short amount of time, coronavirus has spread globally. It has had an enormous impact on people's lives, economy, and societies all around the world, affecting every country. Governments have had to take severe measures to try and contain the pandemic. The virus has altered our way of life in many ways, including its effects on our health and our economy. Here are a few sample essays on ‘CoronaVirus’.
100 Words Essay on Covid 19
200 words essay on covid 19, 500 words essay on covid 19.
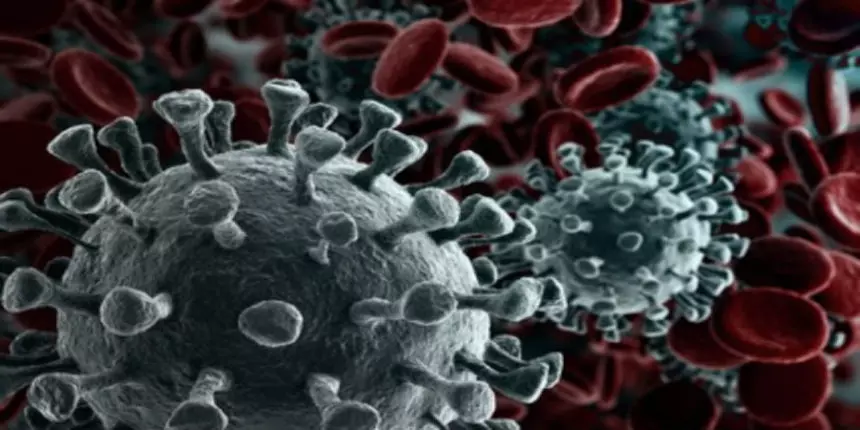
COVID-19 or Corona Virus is a novel coronavirus that was first identified in 2019. It is similar to other coronaviruses, such as SARS-CoV and MERS-CoV, but it is more contagious and has caused more severe respiratory illness in people who have been infected. The novel coronavirus became a global pandemic in a very short period of time. It has affected lives, economies and societies across the world, leaving no country untouched. The virus has caused governments to take drastic measures to try and contain it. From health implications to economic and social ramifications, COVID-19 impacted every part of our lives. It has been more than 2 years since the pandemic hit and the world is still recovering from its effects.
Since the outbreak of COVID-19, the world has been impacted in a number of ways. For one, the global economy has taken a hit as businesses have been forced to close their doors. This has led to widespread job losses and an increase in poverty levels around the world. Additionally, countries have had to impose strict travel restrictions in an attempt to contain the virus, which has resulted in a decrease in tourism and international trade. Furthermore, the pandemic has put immense pressure on healthcare systems globally, as hospitals have been overwhelmed with patients suffering from the virus. Lastly, the outbreak has led to a general feeling of anxiety and uncertainty, as people are fearful of contracting the disease.
My Experience of COVID-19
I still remember how abruptly colleges and schools shut down in March 2020. I was a college student at that time and I was under the impression that everything would go back to normal in a few weeks. I could not have been more wrong. The situation only got worse every week and the government had to impose a lockdown. There were so many restrictions in place. For example, we had to wear face masks whenever we left the house, and we could only go out for essential errands. Restaurants and shops were only allowed to operate at take-out capacity, and many businesses were shut down.
In the current scenario, coronavirus is dominating all aspects of our lives. The coronavirus pandemic has wreaked havoc upon people’s lives, altering the way we live and work in a very short amount of time. It has revolutionised how we think about health care, education, and even social interaction. This virus has had long-term implications on our society, including its impact on mental health, economic stability, and global politics. But we as individuals can help to mitigate these effects by taking personal responsibility to protect themselves and those around them from infection.
Effects of CoronaVirus on Education
The outbreak of coronavirus has had a significant impact on education systems around the world. In China, where the virus originated, all schools and universities were closed for several weeks in an effort to contain the spread of the disease. Many other countries have followed suit, either closing schools altogether or suspending classes for a period of time.
This has resulted in a major disruption to the education of millions of students. Some have been able to continue their studies online, but many have not had access to the internet or have not been able to afford the costs associated with it. This has led to a widening of the digital divide between those who can afford to continue their education online and those who cannot.
The closure of schools has also had a negative impact on the mental health of many students. With no face-to-face contact with friends and teachers, some students have felt isolated and anxious. This has been compounded by the worry and uncertainty surrounding the virus itself.
The situation with coronavirus has improved and schools have been reopened but students are still catching up with the gap of 2 years that the pandemic created. In the meantime, governments and educational institutions are working together to find ways to support students and ensure that they are able to continue their education despite these difficult circumstances.
Effects of CoronaVirus on Economy
The outbreak of the coronavirus has had a significant impact on the global economy. The virus, which originated in China, has spread to over two hundred countries, resulting in widespread panic and a decrease in global trade. As a result of the outbreak, many businesses have been forced to close their doors, leading to a rise in unemployment. In addition, the stock market has taken a severe hit.
Effects of CoronaVirus on Health
The effects that coronavirus has on one's health are still being studied and researched as the virus continues to spread throughout the world. However, some of the potential effects on health that have been observed thus far include respiratory problems, fever, and coughing. In severe cases, pneumonia, kidney failure, and death can occur. It is important for people who think they may have been exposed to the virus to seek medical attention immediately so that they can be treated properly and avoid any serious complications. There is no specific cure or treatment for coronavirus at this time, but there are ways to help ease symptoms and prevent the virus from spreading.
Explore Career Options (By Industry)
- Construction
- Entertainment
- Manufacturing
- Information Technology
Bio Medical Engineer
The field of biomedical engineering opens up a universe of expert chances. An Individual in the biomedical engineering career path work in the field of engineering as well as medicine, in order to find out solutions to common problems of the two fields. The biomedical engineering job opportunities are to collaborate with doctors and researchers to develop medical systems, equipment, or devices that can solve clinical problems. Here we will be discussing jobs after biomedical engineering, how to get a job in biomedical engineering, biomedical engineering scope, and salary.
Data Administrator
Database professionals use software to store and organise data such as financial information, and customer shipping records. Individuals who opt for a career as data administrators ensure that data is available for users and secured from unauthorised sales. DB administrators may work in various types of industries. It may involve computer systems design, service firms, insurance companies, banks and hospitals.
Ethical Hacker
A career as ethical hacker involves various challenges and provides lucrative opportunities in the digital era where every giant business and startup owns its cyberspace on the world wide web. Individuals in the ethical hacker career path try to find the vulnerabilities in the cyber system to get its authority. If he or she succeeds in it then he or she gets its illegal authority. Individuals in the ethical hacker career path then steal information or delete the file that could affect the business, functioning, or services of the organization.
Data Analyst
The invention of the database has given fresh breath to the people involved in the data analytics career path. Analysis refers to splitting up a whole into its individual components for individual analysis. Data analysis is a method through which raw data are processed and transformed into information that would be beneficial for user strategic thinking.
Data are collected and examined to respond to questions, evaluate hypotheses or contradict theories. It is a tool for analyzing, transforming, modeling, and arranging data with useful knowledge, to assist in decision-making and methods, encompassing various strategies, and is used in different fields of business, research, and social science.
Geothermal Engineer
Individuals who opt for a career as geothermal engineers are the professionals involved in the processing of geothermal energy. The responsibilities of geothermal engineers may vary depending on the workplace location. Those who work in fields design facilities to process and distribute geothermal energy. They oversee the functioning of machinery used in the field.
Remote Sensing Technician
Individuals who opt for a career as a remote sensing technician possess unique personalities. Remote sensing analysts seem to be rational human beings, they are strong, independent, persistent, sincere, realistic and resourceful. Some of them are analytical as well, which means they are intelligent, introspective and inquisitive.
Remote sensing scientists use remote sensing technology to support scientists in fields such as community planning, flight planning or the management of natural resources. Analysing data collected from aircraft, satellites or ground-based platforms using statistical analysis software, image analysis software or Geographic Information Systems (GIS) is a significant part of their work. Do you want to learn how to become remote sensing technician? There's no need to be concerned; we've devised a simple remote sensing technician career path for you. Scroll through the pages and read.
Geotechnical engineer
The role of geotechnical engineer starts with reviewing the projects needed to define the required material properties. The work responsibilities are followed by a site investigation of rock, soil, fault distribution and bedrock properties on and below an area of interest. The investigation is aimed to improve the ground engineering design and determine their engineering properties that include how they will interact with, on or in a proposed construction.
The role of geotechnical engineer in mining includes designing and determining the type of foundations, earthworks, and or pavement subgrades required for the intended man-made structures to be made. Geotechnical engineering jobs are involved in earthen and concrete dam construction projects, working under a range of normal and extreme loading conditions.
Cartographer
How fascinating it is to represent the whole world on just a piece of paper or a sphere. With the help of maps, we are able to represent the real world on a much smaller scale. Individuals who opt for a career as a cartographer are those who make maps. But, cartography is not just limited to maps, it is about a mixture of art , science , and technology. As a cartographer, not only you will create maps but use various geodetic surveys and remote sensing systems to measure, analyse, and create different maps for political, cultural or educational purposes.
Budget Analyst
Budget analysis, in a nutshell, entails thoroughly analyzing the details of a financial budget. The budget analysis aims to better understand and manage revenue. Budget analysts assist in the achievement of financial targets, the preservation of profitability, and the pursuit of long-term growth for a business. Budget analysts generally have a bachelor's degree in accounting, finance, economics, or a closely related field. Knowledge of Financial Management is of prime importance in this career.
Product Manager
A Product Manager is a professional responsible for product planning and marketing. He or she manages the product throughout the Product Life Cycle, gathering and prioritising the product. A product manager job description includes defining the product vision and working closely with team members of other departments to deliver winning products.
Underwriter
An underwriter is a person who assesses and evaluates the risk of insurance in his or her field like mortgage, loan, health policy, investment, and so on and so forth. The underwriter career path does involve risks as analysing the risks means finding out if there is a way for the insurance underwriter jobs to recover the money from its clients. If the risk turns out to be too much for the company then in the future it is an underwriter who will be held accountable for it. Therefore, one must carry out his or her job with a lot of attention and diligence.
Finance Executive
Operations manager.
Individuals in the operations manager jobs are responsible for ensuring the efficiency of each department to acquire its optimal goal. They plan the use of resources and distribution of materials. The operations manager's job description includes managing budgets, negotiating contracts, and performing administrative tasks.
Bank Probationary Officer (PO)
Investment director.
An investment director is a person who helps corporations and individuals manage their finances. They can help them develop a strategy to achieve their goals, including paying off debts and investing in the future. In addition, he or she can help individuals make informed decisions.
Welding Engineer
Welding Engineer Job Description: A Welding Engineer work involves managing welding projects and supervising welding teams. He or she is responsible for reviewing welding procedures, processes and documentation. A career as Welding Engineer involves conducting failure analyses and causes on welding issues.
Transportation Planner
A career as Transportation Planner requires technical application of science and technology in engineering, particularly the concepts, equipment and technologies involved in the production of products and services. In fields like land use, infrastructure review, ecological standards and street design, he or she considers issues of health, environment and performance. A Transportation Planner assigns resources for implementing and designing programmes. He or she is responsible for assessing needs, preparing plans and forecasts and compliance with regulations.
An expert in plumbing is aware of building regulations and safety standards and works to make sure these standards are upheld. Testing pipes for leakage using air pressure and other gauges, and also the ability to construct new pipe systems by cutting, fitting, measuring and threading pipes are some of the other more involved aspects of plumbing. Individuals in the plumber career path are self-employed or work for a small business employing less than ten people, though some might find working for larger entities or the government more desirable.
Construction Manager
Individuals who opt for a career as construction managers have a senior-level management role offered in construction firms. Responsibilities in the construction management career path are assigning tasks to workers, inspecting their work, and coordinating with other professionals including architects, subcontractors, and building services engineers.
Urban Planner
Urban Planning careers revolve around the idea of developing a plan to use the land optimally, without affecting the environment. Urban planning jobs are offered to those candidates who are skilled in making the right use of land to distribute the growing population, to create various communities.
Urban planning careers come with the opportunity to make changes to the existing cities and towns. They identify various community needs and make short and long-term plans accordingly.
Highway Engineer
Highway Engineer Job Description: A Highway Engineer is a civil engineer who specialises in planning and building thousands of miles of roads that support connectivity and allow transportation across the country. He or she ensures that traffic management schemes are effectively planned concerning economic sustainability and successful implementation.
Environmental Engineer
Individuals who opt for a career as an environmental engineer are construction professionals who utilise the skills and knowledge of biology, soil science, chemistry and the concept of engineering to design and develop projects that serve as solutions to various environmental problems.
Naval Architect
A Naval Architect is a professional who designs, produces and repairs safe and sea-worthy surfaces or underwater structures. A Naval Architect stays involved in creating and designing ships, ferries, submarines and yachts with implementation of various principles such as gravity, ideal hull form, buoyancy and stability.
Orthotist and Prosthetist
Orthotists and Prosthetists are professionals who provide aid to patients with disabilities. They fix them to artificial limbs (prosthetics) and help them to regain stability. There are times when people lose their limbs in an accident. In some other occasions, they are born without a limb or orthopaedic impairment. Orthotists and prosthetists play a crucial role in their lives with fixing them to assistive devices and provide mobility.
Veterinary Doctor
Pathologist.
A career in pathology in India is filled with several responsibilities as it is a medical branch and affects human lives. The demand for pathologists has been increasing over the past few years as people are getting more aware of different diseases. Not only that, but an increase in population and lifestyle changes have also contributed to the increase in a pathologist’s demand. The pathology careers provide an extremely huge number of opportunities and if you want to be a part of the medical field you can consider being a pathologist. If you want to know more about a career in pathology in India then continue reading this article.
Speech Therapist
Gynaecologist.
Gynaecology can be defined as the study of the female body. The job outlook for gynaecology is excellent since there is evergreen demand for one because of their responsibility of dealing with not only women’s health but also fertility and pregnancy issues. Although most women prefer to have a women obstetrician gynaecologist as their doctor, men also explore a career as a gynaecologist and there are ample amounts of male doctors in the field who are gynaecologists and aid women during delivery and childbirth.
An oncologist is a specialised doctor responsible for providing medical care to patients diagnosed with cancer. He or she uses several therapies to control the cancer and its effect on the human body such as chemotherapy, immunotherapy, radiation therapy and biopsy. An oncologist designs a treatment plan based on a pathology report after diagnosing the type of cancer and where it is spreading inside the body.
Audiologist
The audiologist career involves audiology professionals who are responsible to treat hearing loss and proactively preventing the relevant damage. Individuals who opt for a career as an audiologist use various testing strategies with the aim to determine if someone has a normal sensitivity to sounds or not. After the identification of hearing loss, a hearing doctor is required to determine which sections of the hearing are affected, to what extent they are affected, and where the wound causing the hearing loss is found. As soon as the hearing loss is identified, the patients are provided with recommendations for interventions and rehabilitation such as hearing aids, cochlear implants, and appropriate medical referrals. While audiology is a branch of science that studies and researches hearing, balance, and related disorders.
Hospital Administrator
The hospital Administrator is in charge of organising and supervising the daily operations of medical services and facilities. This organising includes managing of organisation’s staff and its members in service, budgets, service reports, departmental reporting and taking reminders of patient care and services.
For an individual who opts for a career as an actor, the primary responsibility is to completely speak to the character he or she is playing and to persuade the crowd that the character is genuine by connecting with them and bringing them into the story. This applies to significant roles and littler parts, as all roles join to make an effective creation. Here in this article, we will discuss how to become an actor in India, actor exams, actor salary in India, and actor jobs.
Individuals who opt for a career as acrobats create and direct original routines for themselves, in addition to developing interpretations of existing routines. The work of circus acrobats can be seen in a variety of performance settings, including circus, reality shows, sports events like the Olympics, movies and commercials. Individuals who opt for a career as acrobats must be prepared to face rejections and intermittent periods of work. The creativity of acrobats may extend to other aspects of the performance. For example, acrobats in the circus may work with gym trainers, celebrities or collaborate with other professionals to enhance such performance elements as costume and or maybe at the teaching end of the career.
Video Game Designer
Career as a video game designer is filled with excitement as well as responsibilities. A video game designer is someone who is involved in the process of creating a game from day one. He or she is responsible for fulfilling duties like designing the character of the game, the several levels involved, plot, art and similar other elements. Individuals who opt for a career as a video game designer may also write the codes for the game using different programming languages.
Depending on the video game designer job description and experience they may also have to lead a team and do the early testing of the game in order to suggest changes and find loopholes.
Radio Jockey
Radio Jockey is an exciting, promising career and a great challenge for music lovers. If you are really interested in a career as radio jockey, then it is very important for an RJ to have an automatic, fun, and friendly personality. If you want to get a job done in this field, a strong command of the language and a good voice are always good things. Apart from this, in order to be a good radio jockey, you will also listen to good radio jockeys so that you can understand their style and later make your own by practicing.
A career as radio jockey has a lot to offer to deserving candidates. If you want to know more about a career as radio jockey, and how to become a radio jockey then continue reading the article.
Choreographer
The word “choreography" actually comes from Greek words that mean “dance writing." Individuals who opt for a career as a choreographer create and direct original dances, in addition to developing interpretations of existing dances. A Choreographer dances and utilises his or her creativity in other aspects of dance performance. For example, he or she may work with the music director to select music or collaborate with other famous choreographers to enhance such performance elements as lighting, costume and set design.
Videographer
Multimedia specialist.
A multimedia specialist is a media professional who creates, audio, videos, graphic image files, computer animations for multimedia applications. He or she is responsible for planning, producing, and maintaining websites and applications.
Social Media Manager
A career as social media manager involves implementing the company’s or brand’s marketing plan across all social media channels. Social media managers help in building or improving a brand’s or a company’s website traffic, build brand awareness, create and implement marketing and brand strategy. Social media managers are key to important social communication as well.
Copy Writer
In a career as a copywriter, one has to consult with the client and understand the brief well. A career as a copywriter has a lot to offer to deserving candidates. Several new mediums of advertising are opening therefore making it a lucrative career choice. Students can pursue various copywriter courses such as Journalism , Advertising , Marketing Management . Here, we have discussed how to become a freelance copywriter, copywriter career path, how to become a copywriter in India, and copywriting career outlook.
Careers in journalism are filled with excitement as well as responsibilities. One cannot afford to miss out on the details. As it is the small details that provide insights into a story. Depending on those insights a journalist goes about writing a news article. A journalism career can be stressful at times but if you are someone who is passionate about it then it is the right choice for you. If you want to know more about the media field and journalist career then continue reading this article.
For publishing books, newspapers, magazines and digital material, editorial and commercial strategies are set by publishers. Individuals in publishing career paths make choices about the markets their businesses will reach and the type of content that their audience will be served. Individuals in book publisher careers collaborate with editorial staff, designers, authors, and freelance contributors who develop and manage the creation of content.
In a career as a vlogger, one generally works for himself or herself. However, once an individual has gained viewership there are several brands and companies that approach them for paid collaboration. It is one of those fields where an individual can earn well while following his or her passion.
Ever since internet costs got reduced the viewership for these types of content has increased on a large scale. Therefore, a career as a vlogger has a lot to offer. If you want to know more about the Vlogger eligibility, roles and responsibilities then continue reading the article.
Individuals in the editor career path is an unsung hero of the news industry who polishes the language of the news stories provided by stringers, reporters, copywriters and content writers and also news agencies. Individuals who opt for a career as an editor make it more persuasive, concise and clear for readers. In this article, we will discuss the details of the editor's career path such as how to become an editor in India, editor salary in India and editor skills and qualities.
Linguistic meaning is related to language or Linguistics which is the study of languages. A career as a linguistic meaning, a profession that is based on the scientific study of language, and it's a very broad field with many specialities. Famous linguists work in academia, researching and teaching different areas of language, such as phonetics (sounds), syntax (word order) and semantics (meaning).
Other researchers focus on specialities like computational linguistics, which seeks to better match human and computer language capacities, or applied linguistics, which is concerned with improving language education. Still, others work as language experts for the government, advertising companies, dictionary publishers and various other private enterprises. Some might work from home as freelance linguists. Philologist, phonologist, and dialectician are some of Linguist synonym. Linguists can study French , German , Italian .
Public Relation Executive
Travel journalist.
The career of a travel journalist is full of passion, excitement and responsibility. Journalism as a career could be challenging at times, but if you're someone who has been genuinely enthusiastic about all this, then it is the best decision for you. Travel journalism jobs are all about insightful, artfully written, informative narratives designed to cover the travel industry. Travel Journalist is someone who explores, gathers and presents information as a news article.
Quality Controller
A quality controller plays a crucial role in an organisation. He or she is responsible for performing quality checks on manufactured products. He or she identifies the defects in a product and rejects the product.
A quality controller records detailed information about products with defects and sends it to the supervisor or plant manager to take necessary actions to improve the production process.
Production Manager
Merchandiser.
A QA Lead is in charge of the QA Team. The role of QA Lead comes with the responsibility of assessing services and products in order to determine that he or she meets the quality standards. He or she develops, implements and manages test plans.
Metallurgical Engineer
A metallurgical engineer is a professional who studies and produces materials that bring power to our world. He or she extracts metals from ores and rocks and transforms them into alloys, high-purity metals and other materials used in developing infrastructure, transportation and healthcare equipment.
Azure Administrator
An Azure Administrator is a professional responsible for implementing, monitoring, and maintaining Azure Solutions. He or she manages cloud infrastructure service instances and various cloud servers as well as sets up public and private cloud systems.
AWS Solution Architect
An AWS Solution Architect is someone who specializes in developing and implementing cloud computing systems. He or she has a good understanding of the various aspects of cloud computing and can confidently deploy and manage their systems. He or she troubleshoots the issues and evaluates the risk from the third party.
Computer Programmer
Careers in computer programming primarily refer to the systematic act of writing code and moreover include wider computer science areas. The word 'programmer' or 'coder' has entered into practice with the growing number of newly self-taught tech enthusiasts. Computer programming careers involve the use of designs created by software developers and engineers and transforming them into commands that can be implemented by computers. These commands result in regular usage of social media sites, word-processing applications and browsers.
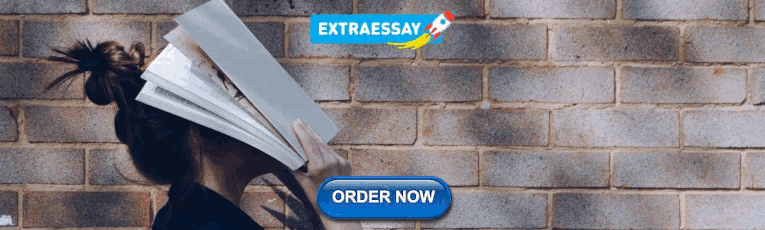
ITSM Manager
Information security manager.
Individuals in the information security manager career path involves in overseeing and controlling all aspects of computer security. The IT security manager job description includes planning and carrying out security measures to protect the business data and information from corruption, theft, unauthorised access, and deliberate attack
Business Intelligence Developer
Applications for admissions are open..

JEE Main Important Chemistry formulas
As per latest 2024 syllabus. Chemistry formulas, equations, & laws of class 11 & 12th chapters

Aakash iACST Scholarship Test 2024
Get up to 90% scholarship on NEET, JEE & Foundation courses

Resonance Coaching
Enroll in Resonance Coaching for success in JEE/NEET exams

TOEFL ® Registrations 2024
Thinking of Studying Abroad? Think the TOEFL® test. Register now & Save 10% on English Proficiency Tests with Gift Cards

ALLEN JEE Exam Prep
Start your JEE preparation with ALLEN

NEET 2024 Most scoring concepts
Just Study 32% of the NEET syllabus and Score upto 100% marks
Everything about Education
Latest updates, Exclusive Content, Webinars and more.
Download Careers360 App's
Regular exam updates, QnA, Predictors, College Applications & E-books now on your Mobile

Cetifications
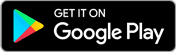
We Appeared in


An official website of the United States government
The .gov means it’s official. Federal government websites often end in .gov or .mil. Before sharing sensitive information, make sure you’re on a federal government site.
The site is secure. The https:// ensures that you are connecting to the official website and that any information you provide is encrypted and transmitted securely.
- Publications
- Account settings
Preview improvements coming to the PMC website in October 2024. Learn More or Try it out now .
- Advanced Search
- Journal List
- Elsevier - PMC COVID-19 Collection

Impact of COVID-19: A radical modal shift from public to private transport mode
Sanhita das.
a Department of Civil Engineering, Indian Institute of Technology Roorkee, Roorkee, Uttarakhand, 247667, India
Alice Boruah
b Department of Civil Engineering, Indian Institute of Technology Guwahati, Guwahati, Assam, 781039, India
Arunabha Banerjee
Rahul raoniar, suresh nama, akhilesh kumar maurya.
The unprecedented shock triggered by the COVID-19 pandemic has caused significant impact on public transportation services, travel behavior and mode choice preferences. Increasing risk of virus contagion in shared travel modes might result in a systemic shift from public transport to car commute. Such a shift causes increased congestions, emissions with a burden on the existing infrastructure. Given the urgent need of reconsideration of transport in a post-COVID world, this study presents insights into the possible shift from public transport to car commute due to the coronavirus crisis, potential factors influencing the mode shift, with emphasis being also laid on suitable strategies for promoting public transport use in the future world. Based on an online questionnaire survey conducted in India, results of logistic regression model indicate that commuters' socio-economic characteristics such as age, gender and monthly income tend to significantly influence mode switch preferences. In addition, trip characteristics including travel time, overcrowding and hygiene are strongly associated with mode shift preferences from public transport to car use. Commuters' perceptions on several strategies for promoting public transport have also been assessed, which will indeed pave the way for the formulation of post-COVID transport policies. In essence, efforts need to be directed towards restoring users’ confidence and trust by providing a safe, secure and healthy environment to the public transport users.
1. Introduction
The outbreak of a novel Coronavirus Disease (COVID-19) with virus strain Severe Acute Respiratory Syndrome Coronavirus 2 (SARS-CoV-2) has struck the global population with its epicenter initiation in Wuhan, China in December 2019 ( Huang et al., 2020 ). Due to lack of initial measures over travel restrictions across different countries, the spreading of the virus has gone unobstructed. Early confirmed COVID-19 cases outside China had travel links to Italy and Iran, and thereafter triggering the rapid spread of virus across 210 countries including India. Owing to alarming rise in virus spread, World Health Organization ( WHO, 2020 ) declared a worldwide emergency on March 11, 2020 to deal with the global pandemic scenario. By May 31st, WHO (2020) had confirmed a total of 62 lakh cases of infection globally, with death toll reaching 3.72 lakhs, and India contributing to 1,82,143 confirmed cases and 5164 deaths.
To curb transmission of the virus, national lockdown restrictions were imposed in India in several phases limiting all non-essential travel. The first phase of lockdown was implemented between 25th March and 14 th April, following which Phase 2, Phase 3 and Phase 4 lockdown were continued between 15th April to 3rd May, 4th May to 17th May and 18th May to 31st May respectively. This has led to drastic changes in people's travel patterns, daily activities, and a significant reduction in road traffic and ridership levels. Public transport ridership in some of the cities of Europe fell by 80% as transport operators reduced their services ( Bernhardt, 2020 ). According to a survey conducted by UITP and World Bank (2020) , the ban on bus operations in India had resulted in 90% reduction in ridership, where more than 60% bus operators believed that both demand and service levels would not be more than 50% of a pre-COVID scenario. Pillai (2020) highlighted that the capacity of public transport in Delhi reduced on an average by 73% during the pandemic. A report published by AAOCHAM and Primus Partners also indicated that the ridership of PT reduced by 34% across the Indian cities where out of 55% of the public transport users in the pre-pandemic period, only 21% were willing to prefer PT in post-pandemic world. According to the Community Mobility Reports of Google ( https://www.google.com/covid19/mobility/ ), a decline of 32.4% in public transport use was reported in India between February and May 2020, with the maximum reduction being observed as 59.9% in the month of April ( Bhaduri et al., 2020 ). A large decline in mobility was observed across cities due to the fear from COVID-19. This reduction in public transport usage has been the result of personal preferences and government measures to contain the virus spread.
As public transport (PT) brings people in close quarters in a confined space, the users become more vulnerable to the virus, thereby causing serious health concerns which ultimately lead to a loss in ridership. A survey conducted in the UK revealed that 72% of the surveyed respondents would not use public transport unless safety and hygiene measures are in place (Transport Focus, 2020 ), while 18% of the respondents were happy to resume services as soon as the government restrictions are lifted. Even 75% of Indian commuters reported public transport use as unsafe while only 1.7% of the commuters has perceived it as a safe mode of transport ( Pawar et al., 2020 ). As per Troko et al. (2011) , the close proximity with which individuals travel in shared travel models could lead to higher chance of getting infected. Although social distancing has become the new norm to reduce the risk of contagion ( Tirachini and Cats, 2020 ), people might rather shift from public transport to car commute due to concerns of hygiene and disease transmission in public transport use. This modal shift has been indicated in the recent literature where 5% of the surveyed respondents in India have shifted from public transport to private mode ( Pawar et al., 2020 ). Bucsky (2020) indicated that the dependence on car in Budapest during the pandemic has increased from 43% to 65%. Indeed car commuting in India is expected to increase to as much as 38% by the end of 2020. In particular, the number of car sales recorded in India in the month of July 2020 were found to be 69% higher than that in June 2020. Haas et al. (2020) also revealed that people in Netherlands prefer to use cars and tend to avoid public transport because of the corona crisis. Even a survey conducted in Australia indicated that private vehicle was considered as the most comfortable mode during pandemics and 42% of the respondents referred bus as the least comfortable one ( Beck and Hensher, 2020 ). A recent survey conducted by Pillai (2020) revealed that 55% of Indian shared transport users were more likely to own private cars in the near future, reflecting increase in car sales and skepticism over public transport use. Such a shift and high dependence on car could have negative consequences such as increased congestions, emissions, increased travel times, damage to the environment and accidents. Moreover, rise in car ownership levels in the near future will not only increase transport demand, but also can have negative effects in terms of accessibility and sustainability.
As a consequence, governments are facing challenges to develop a new future for smarter and more efficient public transport system to avert the impending crisis of congestion. In light of this, this paper fills a gap in the understanding of how the COVID-19 pandemic has impacted changes in public transportation and car use in India. It discusses the changes in travel behavior and its subsequent effect on mode switching behavior compared to the situation before the COVID-19 period. Specifically, this paper aims to evaluate the factors influencing commuters' choice to switch from public transport mode to private cars, their perceptions to associated risks, with proper emphasis being laid on strategies that could be implemented such that our public transportation system remains sustainable and resilient. Recognizing that developing effective policies for a future transport in a post-COVID world requires individuals’ mode choice decisions, this paper also presents several practical implications for public and private policymakers, as they chart a new path to reinstate public transport system post pandemic.
2. Research on mode choice determinants
Transport mode choice behavior is governed by individuals' characteristics, situational factors, travel characteristics, and a set of personal attitudes, habits, preferences, lifestyle, culture and (dis)abilities ( Chakrabarti, 2017 ). A plethora of literature on mode choice behavior reveals that travel time ( Chowdhury and Ceder, 2016 ; Almasri and Alraee, 2013 ), trip distance ( Nes, 2002 ; Cho, 2013 ), cost ( Meng et al., 2018 ), traveler characteristics such as gender ( Hanson, 2010 ; Mahadevia and Advani, 2016 ; Rosenbloom, 2006 ), age ( Almasri and Alraee, 2013 ; Liu et al., 2016 ), income ( Mahadevia and Advani, 2016 ; Ko et al., 2019 ), access to personal vehicle ( Chee and Fernandez, 2013 ; Chakrabarti, 2017 ), employment and education ( Liu et al., 2016 ) influence the transport mode choice. Indeed there exists a disagreement in gender-wise mode choice preferences. A study by Mahadevia and Advani (2016) indicated that males prefer using personal commute over transit, while the willingness of males for public transport use was indicated in Chee and Fernandez (2013) work.
Most studies reveal that travel time, speed, comfort, convenience and flexibility of trip making are important for choosing car over public transport ( Corpuz, 2007 ; Nurdden et al., 2007 ). Factors like accessibility, service and frequency are important for the car users to shift to public transport ( Lai and Chen, 2011 ; Corpuz, 2007 ). Research also indicates that car dependency increases among families with young children ( Ryley, 2006 ; Scheiner, 2014 ). Shorter travel time ( Eriksson et al., 2008 ; Vedagiri and Arasan, 2009 ), subsidized fare ( Nurdden, 2007 ; Gebeyehu and Takano, 2007 ) have been considered as key factors for making PT more attractive than private mode. A study by Basheer et al. (2019) indicated that car is most commonly used in combination with public transit for work-related travel, where sensitivity for time is high.
In particular, personal commute is often seen to dominate public transit in spaces where automobiles are relatively low-priced. But in places where land use policies are stringent, parking spaces are heavily priced and automobiles are expensive, shared modes of transit often rule ( Giuliano and Dargay, 2006 ). Efthymiou and Antoniou (2017) reported an increase in public transit use due to increased consciousness of environment, improvement in service, and increased costs of buying and maintaining a car. Among other factors, Clark et al. (2016) found that major lifestyle changes such as job change or moving home, as well as a pro-environmental attitude influence shifting from car to public transit use. The commuting policy literature has focused on the promotion of sustainable public transport mode. Indeed integrated planning taking land-use, consideration of all important factors promoting public transport as well as social practices, suitable network level design with introduction of bus rapid transit system, intelligent transportation systems and transits requiring less transfers, can result in a significant shift from car to public transportation services.
The peer-reviewed literature provides evidence that various factors influence the travel mode choice behavior. Very often, car is chosen as a preferred mode because of its speed, flexibility, comfort, and convenience ( Beirão and Cabral, 2007 ). Although the modal shift from public transport to car has been indicated in the literature related to pre-corona period, users’ preferences to choose a mode due to the coronavirus crisis and potential factors influencing such mode choice behavior can vary largely in a complicated manner. Specifically, to provide a comprehensive understanding of mode switching behavior of public transport users to car commute, the characteristics of the users (such as age, gender, income level and employment), trip features (including travel time, access distance, cost, safety, cleanliness and overcrowding) and vehicle characteristics (availability of personal vehicle) are considered in this study. Given the current global public health crisis, several policy measures to reconfigure public transportation services in a post-COVID world have also been discussed.
3. Methodology
3.1. approach and study area.
A stated-preference web-survey was conducted between April 29th and May 30th , 2020, during phase 2 (April 15th to May 3rd , 2020), phase 3 (May 4th to 17th 2020) and phase 4 (May 18th to 31st 2020) of nationwide lockdown declaration in India. The first wave of the survey was conducted well after the spread of the virus (more than 24, 75, 723 confirmed cases including 1,69,151 deaths had been reported worldwide on April 22nd). To gauge the impact of coronavirus spread on the transportation sector, the online questionnaire was distributed to the residents residing in different regions of the country via social media platform, email and professional networks.
A total of 840 samples was obtained, among which 31% of the surveyed respondents were residing in the north-eastern region of the country, 25% in eastern region, 18% and 10% in northern and southern regions respectively, and the remaining 16% respondents were in the central and western region at the time of response. The survey is designed to collect information about possible changes in travel patterns post pandemic world and perceptions of the respondents to several policy measures that might help in containing the spread of virus on shared mode of transport.
3.2. Survey design
The questionnaire consisted of four sections which included both open-ended and close-ended questions. The first section indicated the purpose of the survey. The second section included current residence and location at the time of response, basic demographic and user characteristics in terms of age group, gender, profession, monthly income, number and type of personal vehicle(s) owned. In order to understand how commuters might change mode of transit post pandemic, trip characteristics before the lockdown is imperative. The third section therefore prompted the respondents to indicate their travel mode preferences during normal commute (before the coronavirus outbreak). In particular, this section inquired about the mode of travel generally used for different trip purposes, trip frequency of such trips (measured on the following scale “no trip”, “daily”, “3–5 times in a week”, “once a week” and “once a month” ), approximate travel time to work (indicators included “less than 15mins ”, “15- 30mins ”, “30- 45mins ” and “greater than 45mins ” ), distance from home to point of access to public transport services (measured as “ 0-500m ”, “ 500-1000m ”, “ 1000-2000m ”, “ 2000-3000m ” and “ more than 3000m ”) and factors that encourage respondents to choose cars over public transport during normal commute (considered factors were travel time, cost, personal safety, overcrowding and hygiene, all being measured on the scale of “ not important ”, “ important ” and “ very important ”).
The influence of COVID-19 on mode shift preferences can be assessed based on the dynamic changes on the transport demands, commuters' preferences in using a particular mode and perceived health risks associated with public transport use. To understand the impact of the pandemic on the mode shift preferences, the fourth section of the questionnaire prompted to indicate the preferred mode of travel post pandemic world for different trips, anticipated safer mode of travel (each travel mode was measured on the scale of “ safe ”, “ unsafe ” and “ not sure ”) and ranking of several possible policy measures for reviving public transportation services post pandemic world. In essence, seven different strategic measures were considered which included “ alternate seating arrangement with proper social distancing measures ”, “ limited passengers allowance ”, “ provision of personal protective equipment (PPE) kits ”, “ regular disinfection of buses at end-to-end stoppage ”, “ cashless fare transaction ”, “ reduce stoppage at areas attracting large crowd ” and “ real-time information of seat availability on the buses ” and the respondents’ preferences were measured based on the scale of “ not important”, “ important” and “ very important” . It further inquired about preferences of the respondents in using public transport post pandemic, if such strategic measures are implemented and the public transport system is redesigned (measured on the scale of “ yes ”, “ probably yes ”, “ probably no ”, “ no ” and “ definitely not ”).
The survey tests respondents' mode choice preferences during pre-pandemic and post-pandemic world in order to answer questions about mode switching behavior of public transport users to private cars. The possible decline in public transport ridership and different factors influencing the mode shift preferences are indicated by several factors including trip attributes, demographic characteristics, service quality and environment of the public transport system. Most importantly, this survey also attempted to address users’ perceptions to several possible policy interventions which could be implemented for reviving public transport ridership in the post-pandemic world.
3.3. Analytical approaches
Two approaches-logistic regressions and analytic hierarchy process were used to examine the mode choice preferences of the respondents in the post-pandemic scenario. Logistic regression model was considered to explain the mode switching behavior of the public transport users and their shift to car use post pandemic. Accordingly several explanatory variables were considered in the analysis that could potentially influence the decrease in public transport ridership and corresponding shift to car use. On the contrary, an analytic hierarchy process was utilized to rank several policy measures that the respondents considered important for reviving the public transportation system post pandemic. This approach is expected to reveal respondents’ perceptions to several strategic measures that the policy makers might consider for increasing the public transport ridership in the post-pandemic world.
3.3.1. Logistic regression models
Logistic regression model is employed to identify the significant factors affecting the mode switching behavior of public transport users to car commute. Owing to enhanced simplicity in model estimation and translation, and straightforward numerical frame, the model has been widely applied for mode choice decision model development. In the context of mode-switching behavior model, the theory of logistic regression model is that each respondent has a set of mode choice preferences (either selection of original mode or shift from original mode to new mode) and each mode choice preference has its own utility ( U in ) which is the ith mode choice preference of the nth respondent. In essence, each respondent chooses the mode with the highest utility and the utility of each respondent is linked to systematic utility ( V in ) and an unobserved component or error (ε), that is U in = V in + ε . The systematic component of utility V in can be defined as:
where α represents alternative specific constant in the utility function; γ 1 , γ 2 , γ 3 , … , γ K is a set of estimated coefficients of the considered explanatory variables; Y 1 , Y 2 , Y 3 , … , Y K is a set of explanatory variables considered in the mode switching behavior choice; K is the number of attributes. The probability of a respondent choosing a specific mode post pandemic is
Fig. 1 shows different explanatory variables used in the logistic regression analysis. The explanatory variables have been categorized into user, vehicle and trip characteristics to help explain mode switching behavior.

Research methodology.
3.3.2. Analytic hierarchy process
Concerning potential decline in public transport ridership, a new transport future in the post-COVID world requires provision of safe and quality public transport alternatives. Promoting public transport and implementation of policy measures entail choosing the right alternatives and making rational decisions in such a way that strategic alignment is maintained. The analytic hierarchy process (AHP) developed by Saaty (1980) is considered as one such technique used in decision-making process for complex environments, where human judgements, perceptions and consequences have long-term repercussions ( Bhushan and Rai, 2004 ). The AHP technique consists of decomposition of decision making process into a hierarchy of sub-problems and converting subjective assessments of relative importance of each criterion to a set of priority weights or scores.
The top level of hierarchy defines the objective of the decision making process that is, evaluation of several policy measures for promoting public transport in a post-COVID world. The second level of hierarchy defines the criteria and evaluation of their relative priorities, which include (A) alternate seating arrangement with proper social distancing measures, (B) limited passengers allowance, (C) provision of personal protective equipment (PPE) kits, (D) regular disinfection of buses at end-to-end stoppage, (E) cashless fare transaction, (F) reduce stoppage at areas attracting large crowd and (G) real-time information of seat availability on the buses. At the last level, the sub-criteria involves perceptions of the respondents to each criterion, categorized as important, not important and very important.
AHP creates a set of pair-wise comparison matrices between the sub-criteria on the same level, and the relative importance of each sub-criteria was assigned using Saaty scale ( Saaty, 1980 ). The first step involves construction of a comparison matrix and evaluation of relative importance of criterion j compared to k , assigned to ( j , k ) position of pair-wise comparison matrix. After that, the priority vector (or Eigen vector) for each of the criteria is computed by first normalizing each column of the matrix by summation of all the elements of the same column, and then calculating the mathematical average of all sub-criteria. The AHP then combines the weights and determines an overall score for each criteria, the highest score corresponding to the strategic measure one has to choose. A consistency index parameter is also considered to represent reliability level of the judgement. A step-by-step procedure can be found in Vargas (2010) , Longaray et al. (2015) and Sael et al. (2019) .
4. Public transit and car use in pre-pandemic and post-pandemic world: descriptive statistics
The descriptive analysis of public transport and car use among commuters during normal commute and post pandemic scenario is discussed in this section. A total of 840 observations were collected and analyzed after removing samples with missing demographics. In particular, the mode switching behavior of the surveyed respondents by different original mode choices and also the travel behavior among the car-owning households are investigated.
4.1. Respondent demographics
Table 1 reports sample demographics, highlighting mode switching behavior of the respondents involving public transport and cars. The data are aggregated by mode choice in the pre-pandemic and post-pandemic world with respect to respondent profile. The sample showed a higher percentage of male respondents, with 89.5% of the respondents aged between 19 and 45 years, 35% being students and reflecting consistent proportion of respondents’ monthly income. Although the gender split in the case study region is 51.54% male and 48.46% female ( Census India, 2011 ), the higher percentage of male respondents can be due to gender-wise travel patterns in India. Also, inadequate responses of the younger and elderly participants can be due to their unwillingness to be surveyed.
Respondent demographics.
The data suggest that females still tend to prefer public transport to cars during normal commute, while the reverse trend follows for males. This is in congruence with the modal choices of females indicated in Srinivasan (2008) and Mahadevia et al. (2012) . In both the cases, gender-wise travel patterns indicated drastic reduction in public transport mode choice post pandemic, with higher preferences in selecting car mode. When the respondents are segmented according to age, the data over the 30 group shows a higher preference for cars over public transport, while the under 30 group prefers public transport during normal commute trips. Even public transport is mostly favored by students and respondents with monthly income below ₹75000. According to the Wilbur Smith and Ministry of Urban Development (2008: xii), the public transport accessibility indices in the case study areas lie between 1.22 and 1.67, indicating better accessibility.
Socioeconomic heterogeneities are reflected in the surveyed dataset. Respondents who are mostly women, students, below the 30 age group and monthly income below ₹75000 prefer public transport during normal commute trips. Higher income respondents even show a tendency to use public transport because of better accessibility. Conversely, the mode switching behavior post pandemic indicates that public transport would still be favored by males, students, below the 30 age group and monthly income below ₹30000. This dataset reveals that respondents who are students, below the 30 age group exhibit similar travel patterns in both the scenario, although significant reduction in public transport trips could be observed post pandemic.
4.2. Change in share of overall trips post pandemic
While a reduction in public transport trips and increase in car trips is anticipated post pandemic, it still needs to be answered whether the final mode choice would imply sensitive dependence on the original mode. The dataset presented in Table 2 illustrates distinction in behavior when the original mode choice was shared transport (public transport and carpool) and those when it was not shared (private cars, motorize two-wheeler and cab) and active travel (bicycle and walk). The shared modes exhibit large mode switching behavior towards other unshared and active travel modes.
Mode shift from original mode choice to other modes for all trips.
Column (1) in Table 2 presents the percentage share of different travel modes reported by respondents during normal commute (in the pre-pandemic period). On the other hand, columns (2) and (3) indicate the percentage of original mode users preferring to use PT and car respectively post pandemic. While column (4) presents the percentage share of other travel modes excluding PT, car and a particular original travel mode; column (5) specifically indicates the percentage share of a given travel mode adopting the same mode post pandemic.
The unshared mode users and those with active travel mode show a higher preference towards using original mode post pandemic. More than 70% of the motorized two-wheeler (MTW) and car users retain the original mode choice. Interestingly, 24% of the respondents using PT during normal commute show a large shift towards other modes (59%), with only 23% of public transport users being attracted to use the original mode. Similar is the case observed for shared car users, where 18% of the shared cab users prefer using the original mode post pandemic. This indicates that increased exposure to health risks in shared transport could lead to behavioral shift from original mode choice. Those who are accustomed to use shared modes are more likely to adopt other modes of transport, although a small proportion of respondents would still be drawn by shared modes post pandemic. This suggests that suitable preventive measures and alternative strategies need to be followed for ensuring the safe health of the shared mode users post pandemic.
The data indicate that private car draws 18% users from public transport, 24% from cabs and 11% each from active travel mode, although it draws heavily from original car users (80%). This suggests a general trend of the respondents towards avoiding public transport mode and favoring other unshared modes post pandemic. However, preferences of some public transport users towards using the original mode could be attributed to better public transport accessibility and demographic characteristics such as vehicle ownership, profession, income and age group of the surveyed respondents, which might influence the mode choice decisions post pandemic. This provides evidence that although original mode choice influences final mode choice, there will be decreased public transport ridership post pandemic, thereby increasing the demand of other transport modes.
In particular, car commuting seems to be a more stable and attractive alternative to users. Over 80% of car commuters would still commute by cars post pandemic, drawing 18% share from the normal public transport users. This highlights the mode choice behavior of respondents post pandemic.
4.3. Travel behavior of commuters in car owning households
19% of commuters having car in their household reported using public transport as their normal commute mode before lockdown. While the percentage reveals a large reduction in using public transport services post pandemic, leaving the share of anticipated PT users less than 6%.
Table 3 shows that public transport users are generally students, comparatively younger, lower monthly income and lower access to cars in their households.
Characteristics of commuters in vehicle-owning households.
Respondents having access to cars (35.7%) still choose public transport during normal commute either because household car(s) is/are not available to them or they prefer using public transport services. Contrarily, 6% of the respondents still consider using public transport post pandemic and is mostly favored by 45% students having lower monthly income and lower average car household access. On average, no significant difference in public transport mode choice is observed between pre pandemic and post pandemic scenario with respect to male and younger respondents. The same holds true for car users.
While there is a significant reduction in PT ridership, the car commuting appears to be a more safe and suitable alternative, increasing the share of car ridership by almost 7% (29.2%–36.3%). Even respondents who are students, have lower monthly income and better car access in their households show a higher preference towards car commuting post pandemic, although no significant differences in their ridership could be observed for younger and male participants. This could be because of the disproportionately smaller number of female participants in the surveyed data. Also, because younger participants may be compelled to use public transport mode either due to unavailability of cars or not possessing driving license, no significant increment in car mode choice could be observed.
The data indicate that public transport users will prefer using the original mode for shorter trip time post pandemic. The relative distribution of trip time, disaggregated by change in commute mode for three different mode switching behavior (shift from public transport to public transport, public transport to car and car to car) is shown in Fig. 2 .

Shift in mode choice with respect to travel time.
39% of public transport users choose the original mode for trips involving lower travel time. Their preferences in selecting the same mode gradually decrease as trip travel time increases. By contrast, there is almost a consistent share of car ridership (24%–31%) across different trip times. This highlights that respondents who generally commute by cars tend to remain a car commuter irrespective of trip travel times.
Most importantly, the public transport users who are more exposed to health risks show a shift in commute mode from public transport to cars (varying from 18% to 32%) post pandemic. The mode shift occurs at all levels of trip times, with the largest share being observed for trips involving higher travel time (greater than 45 min).
5. Modelling results: shift from public transport to cars
The multinomial logistic (MNL) regression model has been considered for analyzing the mode switching behavior of public transport users and their shift to cars post pandemic. MNL is widely used for mode choice modelling due to enhanced simplicity and flexibility in handling multiple explanatory variables. Binary logit regressions were employed which take the value ‘1’ when respondents shift from public transport to cars and ‘0’ when public transport users choose the original mode. Several potential explanatory factors were investigated and the variables with the highest p-values were omitted until the pseudo R 2 values were found satisfactory.
The explanatory variables include respondents' demographics, travel patterns during normal commute and perceptions towards mode choice. Within respondents’ demographics, those of age, gender, monthly income, occupation and household vehicle access were defined as categorical variables. Information on travel characteristics such as number of trips usually made by the respondents, access distance to public transport services and average travel time required to make the trips using PT was included in the mode switching behavior model. As a way to investigate the probable factors encouraging users to choose cars over public transport services, five variables including travel time, cost, safety, avoiding crowd and hygiene were added. In particular, the ratings of each factor were considered to understand mode choice preferences of the respondents. Prior to model development, those variables with magnitudes of associations lower than 0.5 were further considered. Table 4 shows the logistic regression results for the mode switching behavior.
Logistic regression model of mode switching from public transport to car.
The results strongly suggest that the mode switching behavior of PT users to cars are influenced by distinct set of factors. In particular, it appears that high-income respondents (>₹75000) are more likely to use a car. Concerning age and gender, young respondents who are males are found to have a propensity for public transport use. This finding implies that the group of low income respondents who are young and mostly students tend to choose public transport post pandemic, even when they are exposed to increased health risks. Their decisions may be influenced by lower access to private cars in their households. As the degree of car ownership increases, respondents are more likely to switch to car.
Interestingly, the model estimates suggest that respondents prefer to use cars as the number of trips increases. Public transport users appear to be more likely to use private cars for their daily commute, while they still consider using transit for trips with once in a month or longer. Regardless of the accessibility to public transport services, results show that access distance has a significant positive association with the switching preferences to cars. Although better accessibility to transit use appears to exert a positive impact on its use, the results reveal that respondents prefer to avoid transit use, even when the access distance is lower. Another interesting finding is that travel time has a significant positive association with the mode switching choice. Specifically, respondents would mostly prefer to use a private car regardless of the travel time required to reach the destination. This is also supported by Ko et al. (2019) .
With respect to respondents’ mode choice preferences, travel time is found to be significant at 0.05 significance level, with increased travel time being positively associated with mode switching choices. Because car-based travel is often considered to be faster than public transport, respondents consider travel time as an important determinant in the transport mode shift from transit to cars. On the other hand, cost of travel is significant at 0.1 significance level, which is somewhat expected because respondents usually rely on public transit because of cheaper costs, thereby reducing its share of commuters as cost increases. Although the variable safety was not found to be significant, the negative association suggests that respondents who are more concerned about personal safety and security still prefer to use public transport over cars. In particular, this variable considers only personal safety and is not related to health risks associated with coronavirus.
Interestingly, overcrowding and hygiene/cleanliness were found as the significant factors influencing the mode switching behavior of respondents. One possible explanation is that a crowded bus may lead to increased dissatisfaction (in terms of psychological, emotional distress or loss of privacy) on the passengers during travelling ( Li and Hensher, 2011 , 2013 ). Thus, it is more likely that respondents’ preferences on car use will increase in order to avoid crowding in public transport. Moreover, perceptions related to cleanliness are found to have a significant association with the mode switching behavior. Literature highlights that service environment (or cleanliness) has a major impact on mode choice for public transport ( Li and Hensher, 2013 ; Shaaban and Kim, 2016 ; Chen and Li, 2017 ), signifying that private cars providing cleaner service environment than public transport attract users to switch to car use.
6. Policy analysis
6.1. empirical insights.
The major empirical findings and their research and policy implications are discussed in this section. In the context of socio-demographic characteristics, respondents who are students below the age group of 30years, with monthly income lower than ₹15000 show a higher preference for public transport use during both pre-pandemic and post-pandemic scenario. Even higher income respondents who prefer to use public transport during normal commute are more likely to switch to car transportation post pandemic world.
Modelling results suggest that car demand will be primarily driven by travel characteristics and respondents' preferences towards mode choice. While 80% of car commuters would still commute by cars post pandemic, this study indicates that it would draw 18% share from the normal public transport users. Although socio-demographics do not factor heavily, the car demand will increase with the increase in trip frequency, travel time and respondents’ access to cars in their household. Given the importance of short trips, public transport can still be considered for trips with travel time lower than 15 min. But, respondents who are sensitive to service quality and service environment (such as crowding and cleanliness) show a higher propensity to switch to cars.
Although public transport proves to be an efficient, reliable and cheaper transport mode providing better accessibility, several factors relating to trip characteristics, socio-demographics and respondents’ attitudes influence switching to car commuting post pandemic. Under this circumstance, it would be necessary to promote public transport ridership by providing quality PT services, cleaner and a safe environment, which could possibly reduce health risks.
6.2. Possible strategies to revive public transport systems post pandemic
While public transport is believed to be an efficient, safe and sustainable mode of transport, a significant reduction in public transport ridership is anticipated after resumption of services. Most importantly, public transport provides a lifeline to vast numbers of low and middle-income Indian urban families. This suggests that appropriate policies need to be implemented for building users' confidence in public transport such that the excessive burden on road traffic is reduced. In particular, developing any effective policies require prior understanding of users' perceptions and preferences towards the proposed interventions. Recognizing this, this work has further attempted to gain insights into respondents’ preferences to a number of policy measures which, if considered, might revive the public transport system or reduce switching to car transportation.
Based on the questionnaire survey, respondents evaluated the importance of several possible policy measures which could attract them to use the public transport post pandemic. A total of seven strategic measures are considered which include (A) alternate seating arrangement with proper social distancing measures, (B) limited passengers allowance, (C) provision of personal protective equipment (PPE) kits, (D) regular disinfection of buses at end-to-end stoppage, (E) cashless fare transaction, (F) reduce stoppage at areas attracting large crowd and (G) real-time information of seat availability on the buses. Respondents indicated to what extent these proposed strategies are important for using public transport services which in turn might provide a safe and healthy environment to the users.
An analytic hierarchy process (AHP) is used in this study to rank several considered strategies ( Saaty, 1980 ; Mayo and Taboada, 2020 ). AHP uses a hierarchical model consisting of goals, criteria and alternatives. It involves pairwise comparisons of the considered strategies at each level with respect to an element from the upper hierarchy level. Based on the principle of hierarchical decomposition, the priority weights are estimated to provide the overall priority of each considered strategy. Prior to understanding users’ preferences to several PT reviving strategies, it is important to demystify which policy strategies can fly and for which segments of population. Accordingly, the priority weights and individual rankings (given in parentheses) of each strategy for each demographic segment are estimated, as presented in Table 5 .
Priority weights and ranking (given in parentheses) of seven strategies.
The results indicated that factor F ranked the highest in 9 out of 12 sub-segments while factor B and D were the least influential. This implies that respondents seem to be mostly motivated from strategies prioritizing reduced bus stoppage at areas attracting large crowd. This preferred choice is quite understandable because respondents value service quality and environment more for using public transit. This is in line with the significant factors obtained in Table 4 . In particular, a higher density of bus stops reduces travel time of the users as well as allows more PT passengers, which impact users’ riding comfort and safety. Concerning socio-demographics, both male and female respondents aged less than 45 years of all professions prioritize factor F. Interestingly, middle to higher income respondents indicated that PT would be preferred if measure F was considered.
Factor E relating to “cashless fare transaction” and factor A favoring “seating arrangement with proper social distancing measures” ranked second and third respectively. One possible explanation is that respondents are usually more concerned about transmission of virus through the exchange of physical money. Therefore, cashless and contactless payment seem to be a more effective public-health measure for middle to high income group respondents aged less than 30years. On the other hand, both male and female respondents prioritize alternate seating arrangement over cashless payment transaction. A total of 7 in 12 sub-segments rank alternate seating arrangement as the third priority such that social distancing is ensured among the travelers. Although it would be challenging to implement such strategy in Indian contexts where public transport modes are always crowded, such initiatives on social distancing rules would rebuild users’ confidence in using public transport.
Moving beyond, provision of PPE kits (strategy C) and real-time information on seat availability (strategy G) are ranked 4th and 5th respectively. Wearing masks and gloves on public transport are important for the safety of all the travelers and the driver. Moreover, real-time information on seat availability of the transit modes can be made available to the travelers either through an app or occupancy information on the public transport itself. Interestingly, female respondents rank seat availability information as 3rd while reduced bus stops being still considered as the first priority. Conversely, strategy B relating to “limited passengers’ allowance” and strategy D “regular disinfection of buses at end-to-end stoppage” were considered as the least priority strategies among all demographic segments.
To summarize, respondents indicated that the strategy “reduce stoppage at areas attracting large crowd” is the most influential for retaining public transport ridership post pandemic scenario. Cashless and contactless payment was the next priority, followed by alternate seating arrangement with proper social distancing measures, provision of PPE kits, real-time information on seat availability, limited passengers’ allowance and regular disinfection of buses. Furthermore, when the respondents were asked about their likelihood to use public transport if such strategies are implemented, 25.6% of respondents replied “yes” while 53.6%, 16.7%, 3.6% and 0.5% respondents indicated “probably yes”, “probably no”, “no” and “definitely not” respectively. This signifies that implementing such policy measures by reassuring safety to the public transport users can still retain public transport ridership in a post-COVID world.
6.3. Policy implications
Due to the ongoing pandemic, transport policy makers are facing myriad of challenges to reconcile social safety and protection with global economic growth. Clear directives and formulating new policies based on users' preferences provide an opportunity to reinforce the new transport future in a post-pandemic world. In particular, the impact of individual behavioral changes and their perceptions to travel choice has indicated an unprecedented decline in public transport ridership. Recognizing that reconfiguring or formulating transport policies should be based on users’ perceptions and mode choice preferences, several possible policy implications obtained from this research are summarized below.
6.3.1. Safeguard safety
During the pandemic the onus of responsibility has been placed on the individuals for their own and others' health and wellbeing. Although shared trips run a significant risk in such a public health crisis, several policy measures targeting public transport users will be effective to reassure users’ safety during operation. In this line, reconfiguring the layout of seats with alternate seating arrangement, cashless and contactless fare transaction, provision of PPE kits and hand sanitizer dispensers can be considered to safeguard safety to the public transit users. Even respondents considered reconfiguring seating arrangement of buses as one of the influential strategies to retain the PT ridership in a post-COVID world, followed by provision of PPE kits (or wearing masks and gloves, etc.).
6.3.2. Service environment
Modelling results showed that overcrowding and hygiene/cleanliness are the significant factors influencing switch of public transport users to car commuting. This suggests that policy formulations designed with careful consideration of service environment are more likely to be effective in the context of promoting safe and healthy journeys to the travelers. Some of the interventions might include limited allowance of passengers prohibiting overcrowded public transit, frequent cleaning of vehicles and handgrips in buses, social distancing markers to guide the onboard passengers and separating bus drivers from the boarded passengers.
6.3.3. Service innovations
The provision of quality transit service and technological innovations can be effective in ensuring healthy and safe service to the public transport users. Results of analytic hierarchy process indicated real-time digital information on seat availability as the fifth influential strategy that respondents might prefer to use public transport in a post pandemic world. An adequate provision of real-time passenger information through mobile phone apps or LED information displays on the transit services can essentially alert passengers to seat availability or overcrowding such that they can plan their journey safely and comfortably. Such technological interventions can make transit services more passenger-oriented and reliable, while keeping passengers and drivers’ health and safety as top priority.
6.3.4. Reliable system
Results showed that respondents' mode switching behavior is strongly associated with travel time. In essence, respondents are more likely to switch to private car commute when the travel time on public transport increases. As indicated in the analytic hierarchy process results, strict public transport restriction measures such as eliminating stoppage at bus stops attracting large crowd might be needed to ensure limited passengers entry on the buses, thereby reducing their average travel time. The policy measures need to suit users’ attitudes and behavior, and build confidence about returning to public transportation in a post COVID world.
Given the global pandemic, integrating cashless and contactless payments, real-time information data, ensuring safety measures, reconfiguring the internal layout of the transit services with provision of a healthy service environment, can be effective and attractive for reviving public transport ridership in the face of global public health crisis. Additionally, several interventions at the tactical and operation level such as changes in time tables, redesigning PT services to avoid crowding, real-time information of vehicles and crowding at stations, communication and awareness to the passenger, accessibility and safe operation of PT, service frequencies and rigid hygienic routines can improve the long-term resilience of public transport sector and customer retention in the post-covid world.
7. Conclusions
This study attempted to explore to what extent the COVID-19 pandemic and government's policies to curb the transmission of the virus in India will impact the mode switching behavior from public transport dependence to car use. Recognizing that developing effective policy measures should be based on travelers' mode choice behaviors, an online questionnaire survey is conducted from approximately 840 Indian residents aged 16 years and older. The survey data coupled with statistical approaches provided an understanding of behavioral patterns, attitudes, mode choice preferences and perceptions of the survey participants.
Our findings show that over 80% of car commuters would commute by cars post pandemic, drawing 18% share from the public transport users. Although 19% respondents of car-owning households reported using public transport as their normal commute mode, a significant decline in public transport use has been reported post pandemic, leaving the share to only 6%. Yet, younger participants below 30 age group would still prefer using PT services either due to unavailability of cars or not possessing driving license. Interestingly, travel time has been found as an influential factor in the understanding of mode switching behavior. While 39% of public transport users would still choose the same mode for shorter trips (less than 15 min), they show a large shift in commute mode from public transport to cars as trip time increases, with the largest share being observed for trips greater than 45 min.
Public transport services being the most impacted transport sector, several potential explanatory variables that could influence the mode switching behavior of public transport users from PT to car use were further investigated. Respondents' socio-economic characteristics are found to be significantly associated with mode shift choice. In particular, high-income (>₹75000) and elderly respondents, with access to private cars in their households are more likely to use a car. Regardless of the accessibility to PT services, PT users show a stronger propensity to car use for their daily commute as travel time to work increases. Most importantly, overcrowding and hygiene/cleanliness are the significant factors influencing the mode switching behavior to cars, as crowding and unhygienic service environment increase the risk of exposure to the virus. These findings reflect that the COVID-19 crisis might show a behavioral shift on travel preferences, which can have long-lasting effects on people's mobility with increased burden on road infrastructure. In this light, the crisis might form a window of opportunities for policy makers to revamp the public transport services by implementing suitable policy measures.
This research has explored several policy strategies based on respondents' perceptions and behavioral attitudes in the near future. Utilizing analytic hierarchy process, several proposed policy measures were ranked and the priority weights indicated that reducing frequency of bus stops at areas attracting large crowd is the most influential factor for retaining public transport ridership post pandemic scenario. Cashless and contactless payment was the next priority, followed by alternate seating arrangement with proper social distancing measures, provision of PPE kits, real-time information on seat availability, limited passengers' allowance and regular disinfection of buses. It is important for the policy makers to address the expectations of the users and build users’ confidence in public transport use by providing a safe and healthy service environment. Given the governmental policies to restrict public transport use, it is important to closely observe the changes in mobility patterns and the negative impact of the pandemic on the existing road infrastructure as the car dependence continues to rise in a post-pandemic world.
Author’s Contribution
Sanhita Das: Conceptualization, Methodology, Formal analysis, Investigation, Writing – original draft, Visualization, Alice Boruah: Investigation, Resources, Writing – original draft, Arunabha Banerjee: Investigation, Resources, Writing – original draft, Rahul Raoniar: Investigation, Resources, Suresh Nama: Resources, Akhilesh Kumar Maurya: Writing – review & editing, Visualization, Supervision.
- Almasri E., Alraee S. Factors affecting mode choice of work trips in developing cities—gaza as a case study. J. Transport. Technol. 2013; 3 (4):247–259. [ Google Scholar ]
- Basheer M.A., van der Waerden P., Kochan B., Bellemans T., Shah S.A.R. Multi-stage trips: an exploration of factors affecting mode combination choice of travelers in England. Transport Pol. 2019; 81 :95–105. [ Google Scholar ]
- Beck M.J., Hensher D.A. Insights into the impact of COVID-19 on household travel and activities in Australia–The early days under restrictions. Transport Pol. 2020; 96 :76–93. [ PMC free article ] [ PubMed ] [ Google Scholar ]
- Beirão G., Cabral J.S. Understanding attitudes towards public transport and private car: a qualitative study. Transport Pol. 2007; 14 (6):478–489. [ Google Scholar ]
- Bernhardt Decline in ridership, adapted timetables and disinfection-robots – the impact of Corona/COVID-10 on public transport. 2020. https://www.urban-transport-magazine.com/en/decline-in-ridership-adapted-timetables-and-disinfection-robots-the-impact-of-corona-Covid-10-on-public-transport/ Retrieved on 02 August 2020 from.
- Bhaduri E., Manoj B.S., Wadud Z., Goswami A.K., Choudhury C.F. Modelling the effects of COVID-19 on travel mode choice behaviour in India. Transportation Research Interdisciplinary Perspectives. 2020; 8 :100273. [ Google Scholar ]
- Bhushan N., Rai K. Springer; New York: 2004. Strategic Decision Making: Applying the Analytic Hierarchy Process. [ Google Scholar ]
- Bucsky P. Transportation Research Interdisciplinary Perspectives; 2020. Modal Share Changes Due to COVID-19: the Case of Budapest; p. 100141. [ PMC free article ] [ PubMed ] [ Google Scholar ]
- Census India . 2011. Gender Composition, India Series 1, Office of the Registrar General and Census Commissioner. https://censusindia.gov.in/census_and_you/gender_composition.aspx New Delhi. Retrieved from. [ Google Scholar ]
- Chakrabarti S. How can public transit get people out of their cars? An analysis of transit mode choice for commute trips in Los Angeles. Transport Pol. 2017; 54 :80–89. [ Google Scholar ]
- Chee W.L., Fernandez J.L. Factors that influence the choice of mode of transport in Penang: a preliminary analysis. Procedia-Social and Behavioral Sciences. 2013; 91 :120–127. [ Google Scholar ]
- Chen J., Li S. Mode choice model for public transport with categorized latent variables. Mathematical Problems in Engineering, 2017. 2017 doi: 10.1155/2017/7861945. Article ID 7861945. [ CrossRef ] [ Google Scholar ]
- Cho H.D. University of Florida; 2013. The Factors that Affect Long-Distance Travel Mode Choice Decisions and Their Implications for Transportation Policy. [ Google Scholar ]
- Chowdhury S., Ceder A.A. Users' willingness to ride an integrated public-transport service: a literature review. Transport Pol. 2016; 48 :183–195. [ Google Scholar ]
- Clark B., Chatterjee K., Melia S. Changes to commute mode: the role of life events, spatial context and environmental attitude. Transport. Res. Pol. Pract. 2016; 89 :89–105. [ Google Scholar ]
- Corpuz G. 30th Australasian Transport Research Forum. 2007, September. Public transport or private vehicle: factors that impact on mode choice. 11. [ Google Scholar ]
- Efthymiou D., Antoniou C. Understanding the effects of economic crisis on public transport users' satisfaction and demand. Transport Pol. 2017; 53 :89–97. [ Google Scholar ]
- Eriksson L., Friman M., Gärling T. Stated reasons for reducing work-commute by car. Transport. Res. F Traffic Psychol. Behav. 2008; 11 :427–433. [ Google Scholar ]
- Transport Focus . Express & Star News; 2020. Growing Safety Concerns Among Public Transport Users – Survey. 21 May 2020. [ Google Scholar ]
- Gebeyehu M., Takano S. Diagnostic evaluation of public transportation mode choice in Addis Ababa. Journal of Public Transportation. 2007; 10 (4):27–50. [ Google Scholar ]
- Giuliano G., Dargay J. Car ownership, travel and land use: a comparison of the US and Great Britain. Transport. Res. Pol. Pract. 2006; 40 (2):106–124. [ Google Scholar ]
- Haas M., Faber R., Hamersma M. Transportation Research Interdisciplinary Perspectives; 2020. How COVID-19 and the Dutch ‘intelligent Lockdown’change Activities, Work and Travel Behaviour: Evidence from Longitudinal Data in the Netherlands; p. 100150. [ PMC free article ] [ PubMed ] [ Google Scholar ]
- Hanson S. Gender and mobility: new approaches for informing sustainability. Gend. Place Cult. 2010; 17 (1):5–23. [ Google Scholar ]
- Huang C., Wang Y., Li X., Ren L., Zhao J., Hu Y., et al. Clinical features of patients infected with 2019 novel coronavirus in Wuhan, China. Lancet 2020. 2020; 395 :497–506. doi: 10.1016/S0140-6736(20)30183-5. [ PMC free article ] [ PubMed ] [ CrossRef ] [ Google Scholar ]
- Ko J., Lee S., Byun M. Exploring factors associated with commute mode choice: an application of city-level general social survey data. Transport Pol. 2019; 75 :36–46. [ Google Scholar ]
- Lai W.T., Chen C.F. Behavioral intentions of public transit passengers—the roles of service quality, perceived value, satisfaction and involvement. Transport Pol. 2011; 18 (2):318–325. [ Google Scholar ]
- Li Z., Hensher D.A. Crowding and public transport: a review of willingness to pay evidence and its relevance in project appraisal. Transport Pol. 2011; 18 (6):880–887. [ Google Scholar ]
- Li Z., Hensher D.A. Crowding in public transport: a review of objective and subjective measures. Journal of Public Transportation. 2013; 16 (2):6. [ Google Scholar ]
- Liu Y., Hong Z., Liu Y. Do driving restriction policies effectively motivate commuters to use public transportation? Energy Pol. 2016; 90 :253–261. [ Google Scholar ]
- Longaray A.A., Gois J.D.D.R., da Silva Munhoz P.R. Proposal for using AHP method to evaluate the quality of services provided by outsourced companies. Procedia Computer Science. 2015; 55 :715–724. [ Google Scholar ]
- Mahadevia D., Advani D. Gender differentials in travel pattern–the case of a mid-sized city, Rajkot, India. Transport. Res. Transport Environ. 2016; 44 :292–302. [ Google Scholar ]
- Mahadevia D., Joshi R., Datey A. UNEP Risoe Centre on Energy, Climate and Sustainable Development, Technical University of Denmark; Roskilde: 2012. Accessibility and Sustainability of Bus Rapid Transit in India. [ Google Scholar ]
- Mayo F.L., Taboada E.B. Ranking factors affecting public transport mode choice of commuters in an urban city of a developing country using analytic hierarchy process: the case of Metro Cebu, Philippines. Transportation Research Interdisciplinary Perspectives. 2020; 4 :100078. [ Google Scholar ]
- Meng M., Rau A., Mahardhika H. Public transport travel time perception: effects of socioeconomic characteristics, trip characteristics and facility usage. Transport. Res. Pol. Pract. 2018; 114 :24–37. [ Google Scholar ]
- Nes V.R. 2002. Design of Multimodal Transport Networks: A Hierarchical Approach. [ Google Scholar ]
- Nurdden A., Rahmat R.A., Ismail A. Effect of transportation polices on modal shift from private car to public transport in Malaysia. J. Appl. Sci. 2007; 7 (7):1013–1018. [ Google Scholar ]
- Pawar D.S., Yadav A.K., Akolekar N., Velaga N.R. Impact of physical distancing due to novel coronavirus (SARS-CoV-2) on daily travel for work during transition to lockdown. Transportation Research Interdisciplinary Perspectives. 2020:100203. doi: 10.1016/j.trip.2020.100203. [ PMC free article ] [ PubMed ] [ CrossRef ] [ Google Scholar ]
- Pillai S. Hindustan Times; 2020. Public Transit Use Likely to Fall Sharply after Lockdown: Study. 29 May 2020. [ Google Scholar ]
- Rosenbloom S. Is the driving experience of older women changing? Safety and mobility consequences over time. Transport. Res. Rec. 2006; 1956 (1):127–132. [ Google Scholar ]
- Ryley T. Use of non-motorised modes and life stage in Edinburgh. J. Transport Geogr. 2006; 14 (5):367–375. [ Google Scholar ]
- Saaty T.L. RWS publications; USA: 1980. The Analytical Hierarchy Process, Planning, Priority. Resource Allocation. [ Google Scholar ]
- Sael N., Hamim T., Benabbou F. Implementation of the analytic hierarchy process for student profile Analysis. International Journal of Emerging Technologies in Learning (iJET) 2019; 14 (15):78–93. [ Google Scholar ]
- Scheiner J. The gendered complexity of daily life: effects of life-course events on changes in activity entropy and tour complexity over time. Travel Behaviour and Society. 2014; 1 (3):91–105. [ Google Scholar ]
- Shaaban K., Kim I. Assessment of the taxi service in Doha. Transport. Res. Pol. Pract. 2016; 88 :223–235. [ Google Scholar ]
- Srinivasan S. Gendered mobilities; 2008. A Spatial Exploration of the Accessibility of Low-Income Women: Chengdu, China and Chennai, India; pp. 143–158. [ Google Scholar ]
- Tirachini A., Cats O. COVID-19 and public transportation: current assessment, prospects, and research needs. Journal of Public Transportation. 2020; 22 (1):1. [ PMC free article ] [ PubMed ] [ Google Scholar ]
- Troko J., Myles P., Gibson J., Hashim A., Enstone J., Kingdon S., et al. Is public transport a risk factor for acute respiratory infection? BMC Infect. Dis. 2011; 11 (1):1–6. [ PMC free article ] [ PubMed ] [ Google Scholar ]
- UITP, World Bank Bus operations in India: what has been the impact of COVID-19? 2020. https://www.uitp.org/news/bus-operations-india-what-has-been-impact-covid-19 Retrieved on.
- Vargas R.V. Project Management Institute; Washington, DC. Newtown Square, PA: 2010. Using the Analytic Hierarchy Process (Ahp) to Select and Prioritize Projects in a Portfolio. Paper presented at PMI® Global Congress 2010—North America. [ Google Scholar ]
- Vedagiri P., Arasan V.T. Estimating modal shift of car travelers to bus on introduction of bus priority system. Journal of transportation systems engineering and information technology. 2009; 9 (6):120–129. [ Google Scholar ]
- WHO Coronavirus disease (COVID-19) dashboard. 2020. https://covid19.who.int/
Thank you for visiting nature.com. You are using a browser version with limited support for CSS. To obtain the best experience, we recommend you use a more up to date browser (or turn off compatibility mode in Internet Explorer). In the meantime, to ensure continued support, we are displaying the site without styles and JavaScript.
- View all journals
- My Account Login
- Explore content
- About the journal
- Publish with us
- Sign up for alerts
- Open access
- Published: 31 October 2023
MultiCOVID: a multi modal deep learning approach for COVID-19 diagnosis
- Max Hardy-Werbin 1 , 3 ,
- José Maria Maiques 2 ,
- Marcos Busto 2 ,
- Isabel Cirera 3 ,
- Alfons Aguirre 3 ,
- Nieves Garcia-Gisbert 1 ,
- Flavio Zuccarino 2 ,
- Santiago Carbullanca 2 ,
- Luis Alexander Del Carpio 2 ,
- Didac Ramal 2 ,
- Ángel Gayete 2 ,
- Jordi Martínez-Roldan 4 ,
- Albert Marquez-Colome 5 ,
- Beatriz Bellosillo 1 , 6 &
- Joan Gibert 1 , 6
Scientific Reports volume 13 , Article number: 18761 ( 2023 ) Cite this article
899 Accesses
2 Altmetric
Metrics details
- Computational biology and bioinformatics
- Health care
The rapid spread of the severe acute respiratory syndrome coronavirus 2 led to a global overextension of healthcare. Both Chest X-rays (CXR) and blood test have been demonstrated to have predictive value on Coronavirus Disease 2019 (COVID-19) diagnosis on different prevalence scenarios. With the objective of improving and accelerating the diagnosis of COVID-19, a multi modal prediction algorithm (MultiCOVID) based on CXR and blood test was developed, to discriminate between COVID-19, Heart Failure and Non-COVID Pneumonia and healthy (Control) patients. This retrospective single-center study includes CXR and blood test obtained between January 2017 and May 2020. Multi modal prediction models were generated using opensource DL algorithms. Performance of the MultiCOVID algorithm was compared with interpretations from five experienced thoracic radiologists on 300 random test images using the McNemar–Bowker test. A total of 8578 samples from 6123 patients (mean age 66 ± 18 years of standard deviation, 3523 men) were evaluated across datasets. For the entire test set, the overall accuracy of MultiCOVID was 84%, with a mean AUC of 0.92 (0.89–0.94). For 300 random test images, overall accuracy of MultiCOVID was significantly higher (69.6%) compared with individual radiologists (range, 43.7–58.7%) and the consensus of all five radiologists (59.3%, P < .001). Overall, we have developed a multimodal deep learning algorithm, MultiCOVID, that discriminates among COVID-19, heart failure, non-COVID pneumonia and healthy patients using both CXR and blood test with a significantly better performance than experienced thoracic radiologists.
Similar content being viewed by others
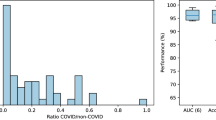
Assessing clinical applicability of COVID-19 detection in chest radiography with deep learning
João Pedrosa, Guilherme Aresta, … Aurélio Campilho
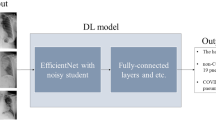
Deep learning model for the automatic classification of COVID-19 pneumonia, non-COVID-19 pneumonia, and the healthy: a multi-center retrospective study
Mizuho Nishio, Daigo Kobayashi, … Takamichi Murakami
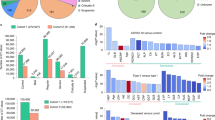
Open resource of clinical data from patients with pneumonia for the prediction of COVID-19 outcomes via deep learning
Wanshan Ning, Shijun Lei, … Zheng Wang
Introduction
The outbreak of Coronavirus Disease 2019 (COVID-19), caused by severe acute respiratory syndrome coronavirus 2 (SARS-CoV-2), stroke the worldwide population with more than 200 million cases and 4.5 million deaths by August 2021. The rapid spread of the pandemic led to a global overexertion of health care and research facilities in order to counteract the growing rate of infection. However, a collapse of the sanitary system was imminent and inevitable worldwide, and new technologies were needed to speed up the diagnostic process.
The reference for COVID-19 diagnosis is the detection of SARS-CoV-2 viral RNA by real-time polymerase chain reaction (RT-PCR). However, the massive requests for sample processing at the beginning of the pandemic caused serious delays to obtain results.
As lung involvement is one of the main causes of morbidity and mortality in SARS-CoV-2 infection, a quick identification of characteristic findings in chest imaging can support the diagnosis and speed up the identification of COVID-19 positive patients at the emergency units.
Several studies have shown that implementation of deep learning (DL) tools to detect chest X-rays (CXR) findings typically associated with SARS-CoV-2 infection, deliver comparable results to those acquired by interpretation of radiologists. However, most of the trained models have a drop in their prediction performance when tested over external datasets 1 . In addition, one of the main hurdles to overcome when training an algorithm to detect Sars-CoV-2 infection in CXR is the similarity of findings with other entities like bacterial pneumonias or heart failure 2 . On the other hand, models based on laboratory results of peripheral blood also give predictive results on diagnosis 3 and prognosis 4 .
A key fact to highlight is how the incursion of COVID-19 caused a dramatic drop in the emergency room consultations of other pathologies. Later on, after the initial peak, the decline of the COVID-19 prevalence made the non-COVID diseases emerge once again at the hospitals. This is relevant due to the challenge of performing an efficient differential diagnosis with selected pathologies during a pandemic. It is well known that the predictive value of a diagnostic test is conditioned by the prevalence of the disease and that of COVID varies widely throughout the different waves of the pandemic 5 . A multicategory approach that takes into account differential diagnoses that are more stable in their prevalence could reduce this variability.
With the objective of improving and accelerating the diagnosis of COVID-19, we developed a tool to assist physicians in reaching a diagnosis. This tool is a multi-modal prediction algorithm (MultiCOVID) based on CXR and blood test with the ability to discriminate between COVID-19, Heart Failure (HF), Non-COVID Pneumonia (NCP) and healthy (Control) samples.
Materials and methods
We retrospectively collected CXR images and hemogram values from 8578 samples from 6123 patients and healthy subjects (mean age 66 ± 18 years of standard deviation, 3523 men) from Parc Salut Mar (PSMAR) Consortium, Barcelona, Spain. Four cohorts were designed: (i) 1171 samples from patients diagnosed with COVID-19 by RT-PCR from March to May 2020; (ii) 1008 samples of patients who suffered an episode of heart failure between 2012 to 2019; (iii) 490 samples of patients diagnosed with non-COVID pneumonia (NCP) from 2018 to 2019; (iv) 5909 samples of standard preoperatory studies of healthy subjects from 2017 to 2019 (Fig. 1 ). HR and NCP diagnosis were selected as defined by the International Classification of Diseases, Tenth Revision (ICD-10) code. All the CXR images from groups i-iii were validated by two independent radiologists (MB and JM).
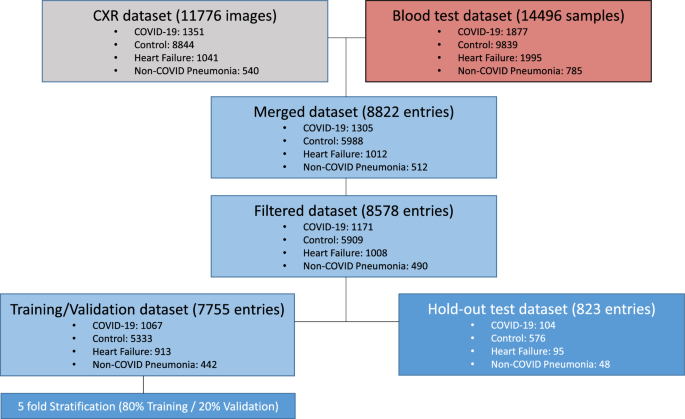
Flowchart for sample selection and patient inclusion in the study and breakdown of training, validation, and hold-out test data sets. Around 25,000 entries were obtained using both CXR images and blood test in a time wise manner. The whole dataset totals 8822 entries of paired CXR and blood test data. Samples with low completeness (less than 80% of blood test data available) were discarded for the model building.
Acquisition of blood sample and image data
We included CXR images performed in a period ranging from 1 day before the patient’s diagnosis to 7 days after. The images were filtered to include only frontal projections regardless of the quality and the radiography system used. Blood sample results were collected within a range of 2 days before or 7 days after the CXR acquisition date using PSMAR lab record system, except for control samples whose measurements ranged for 2 weeks. If two or more blood test results were collected, measurements were averaged.
CXR images and blood test results were combined in the same dataset and split into train/validation set (90%), and hold-out test (10%) set. For training/validation split, we divided the dataset in training (80%) and validation (20%) sets with 5 different random seeds. We ensured that there were no cross-over patients between groups.
Deep learning models
Detailed description of the models, training policy and image preprocessing are provided in Supplementary Material . In brief, segmentation model is based on a U-Net architecture 6 . The CXR-only classification model consists of a validated Convolutional neural network (CNN) resnet-34 architecture 7 . Tabular only-model is an Attention-based network (TabNet) 8 . Joint model is a multi-modal deep learning algorithm which merges the CXR-only and the Blood-only models and uses both CXR image and blood tests as input values. It uses Gradient Blending in order to prevent overfitting and improve generalization 9 . MultiCOVID model is an ensemble predictor of 5 different Joint models that would classify independently between the different classes. Then it uses majority vote to assign a final classification. The whole pipeline development and training was performed using fastai deep learning API 10 .
Comparison with thoracic radiologist interpretations
Hold-out test dataset consisting of 300 samples (ensuring no patient overlap with training or validation sets) was used for expert interpretation. Each sample consisted of a CXR with matched blood results. Expert interpretations were independently provided by five board-certified thoracic radiologists (FZ, SC, LdC, DR, AG) with 2–30 years post-residency training experience. Radiologists were able to check both non segmented images and blood test results without any other additional information in a platform created ad-hoc for prediction. They provided a classification for each image in one of the four categories (COVID-19, control, HF and NCP). A consensus interpretation for the radiologist was obtained by the majority vote for each paired CHX-blood test analyzed.
Statistical analysis
A two-tailed t-test P value was reported when clinical and population blood test differences were assessed. McNemar–Bowker test was used to compare model performance against radiologist majority vote using FDR correction. Plotting and statistical analyses were performed using the packages ggplot, ggpubr and rcompanion in R, version 3.6 (R Core Team; R Foundation for Statistical Computing).
Ethical approval
The study was designed to use radiology images and associated clinical/demographic/ laboratory patient information already collected for the purpose of performing clinical COVID-19 research by Hospital del Mar. The study was conducted in accordance with the relevant institutional guidelines and regulations. The experimental protocols, data acquisition and analysis were approved by the Parc de Salut Mar Clinical Research Ethics Committee (2020/9199/I). Informed consent was obtained, when possible, from patients or legal representatives or waived by the local Parc de Salut Mar Clinical Research Ethics Committee (2020/9199/I) if informed consent was not available due to the pandemic situation.
Patient characteristics
A total of 8578 samples were evaluated across datasets. Patient characteristics and blood test parameters are shown in Table 1 . A highly significant difference in age was found between the cohort of patients with heart failure (82.8 ± 10 years) and the other three cohorts (66.0 ± 16 years for COVID-19 samples, 63.2 ± 18 years for control samples and 67.8 ± 17 years for NCP samples, P < 0.001 for each comparison) and was not considered as a valid variable for further classification.
Whole CXR models learn spurious characteristics for classification
Previous studies have demonstrated that deep learning (DL)-based algorithms should be rigorously evaluated due to their ability to learn non relevant features in order to increase its prediction accuracy 1 . For this reason, we first developed a segmentation algorithm able to segment lung parenchyma at a 95%-pixel accuracy. Then, after segmentation, we evaluated the accuracy of the algorithms for three complementary datasets: non-segmented images, segmented regions and excluded regions. After a few training epochs the three different models achieved nonrandom accuracies between 67 and 74% (Fig. 2 A). However, attention map exploration on the images showed that the different models based their predictions not only inside but also outside of the lung parenchyma (Fig. 2 B).
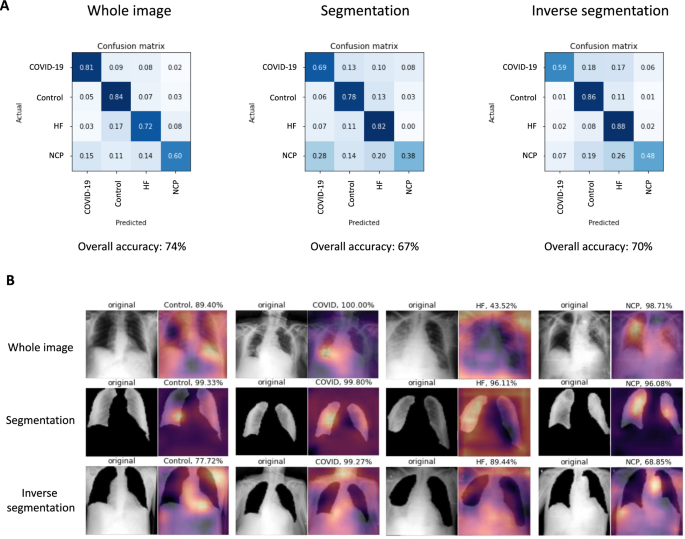
Performance of visual models on whole CXR images. ( A ) Confusion matrix and overall accuracy using whole image, segmented and inverse segmented images, respectively for each category tested. ( B ) Raw image and Grad-CAM heatmap representation of an image for each category and model trained.
These observations showed that, although there are important features outside the lung parenchyma that may help the model to classify between the different entities (eg. heart size), there are other elements (eg. oxygen nasal cannulas or intravenous (IV) catheters) that might confound the model. Thus, we decided to first segment all the CXR before training our models for prediction of diagnosis. In order to accomplish this task, we generated a 785-radiology level lung segmentation dataset and trained a U-net model to regenerate the whole CXR dataset keeping only the lung parenchyma.
Performance of single and multimodal models
In order to evaluate the prediction capacity of both segmented CXR and blood sample data, we built different DL models using both sources alone or in combination. Metrics comparison of all the single vision (CXR-only) and tabular (Blood-only) models are detailed in Supplementary Material . As expected, CXR-only models had a more robust prediction of all 4 categories tested compared to Blood-only models (Fig. 3 ). This difference is stronger in the classes with less samples (HF, and NCP) where CXR-only models could identify features in the CXR images which are characteristic of these two entities whereas this was not possible with Blood-only models.
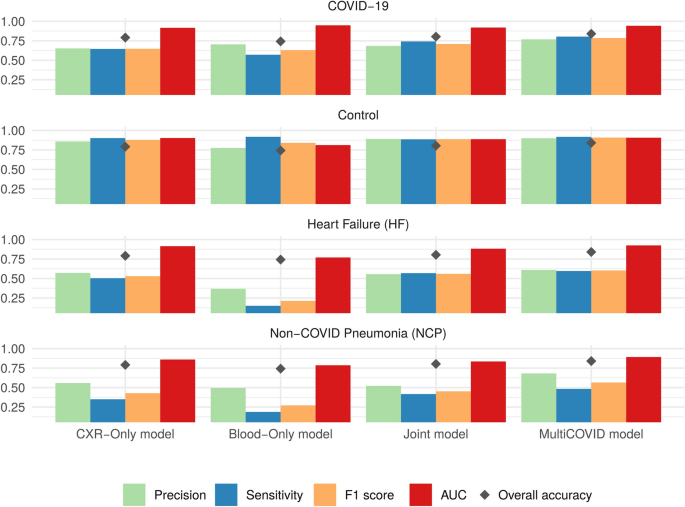
Performance of different models on the entries from hold-out test datasets. Means for precision (green), sensitivity (blue), F1 score (yellow), AUC (red) and accuracy (black diamond) for each model type and category assessed, respectively. CXR-only models use only CXR images for 4 category classification. Blood-only models use blood test a source of information. Joint model uses both CXR and blood test as input for classification and MultiCOVID is the majority vote of 5 different Joint models.
Model interpretability of Blood-only models by analyzing feature importance using Shapley Additive explanations 12 showed that patient classification was related to two different axes: the immune compartment and the red blood cell (RBC) compartment, respectively (Fig. 4 A). The first axis seems to be strongly associated with COVID-19 classification and shows a specific signature looking at the blood counts (Fig. 4 B-top). However, the second axis seems to subdivide patients between COVID-19/Control and HF/NCP, although COVID-19 blood counts seems to be statistically different from Control samples, too (Fig. 4 B-bottom).
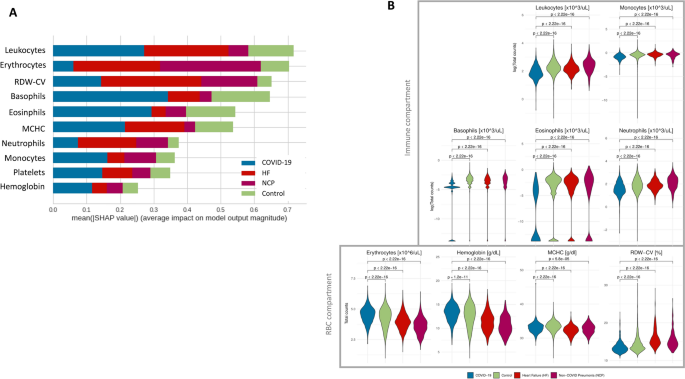
Blood-only model interpretability by SHAP analysis. ( A ) Summary plot showing the mean absolute SHAP value of the ten most important features for the four classes. ( B ) Blood test values of the different features identified by SHAP analysis. RDW-CV: red cell distribution width; MCHC: Mean Corpuscular Hemoglobin Concentration; RBC: red blood cells.
The combination of CXR and blood tests using multimodal models that combine inputs from tabular and image data to perform a global prediction, slightly increased the prediction capacity of the single models even when DL tabular models are worse than machine learning (ML—XGBoost) models alone (Supplementary Table 1 ). This underpins the concept that adding new sources of information to the data could increase the ability of the models to generate better predictions 13 . Moreover, the joint approach used for building MultiCOVID algorithm resulted on an improved performance in the majority of the metrics analyzed (Fig. 3 and Supplementary Table 1 ).
Comparison with expert thoracic radiologists
Finally, we compared the performance of MultiCOVID algorithm with the interpretation of expert chest radiologists. This comparison was performed with 300 CXR randomly selected from the hold-out test set that were independently reviewed by 5 radiologists together with the blood test results. The independent results from radiologists showed an accuracy ranging from 43.7 to 58.7%. This value rose to 59.3% (178/300) when the consensus interpretation of all 5 radiologists based on the majority vote was considered. Of note, the overall accuracy achieved by MultiCOVID was 69.6% (209/300) that was significantly higher than consensus interpretation ( P < 0.001). In addition, for COVID-19 prediction individually, MultiCOVID showed similar sensitivity to the radiologists’ consensus but with a much higher specificity, leading to significantly better performance when discerning between COVID-19 versus Control and COVID-19 vs HF patients ( P < 0.05 for both comparisons; Fig. 5 ).
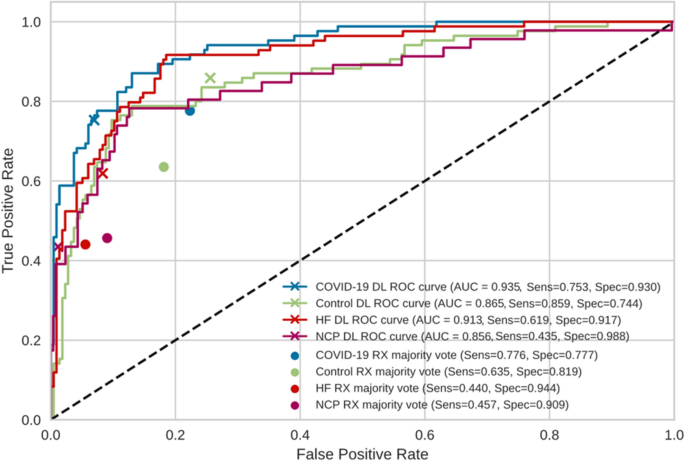
Comparison of the performance of MultiCOVID model with consensus expert radiologist interpretations on random sample of 300 images from the test set. The receiver operating characteristic (ROC) curves for each category (COVID-19 – blue; Control – green; Heart Failure (HF) – red and Non-COVID Pneumonia (NCP) – magenta) are shown for MultiCOVID (DL) and for the consensus interpretation of radiologists (majority vote). Sensitivity (Sens) and specificity (Spec) are also plotted for each category assessed. DL: deep learning.
Diagnosis of COVID-19 is an evolving challenge. During the beginning of the pandemic and the successive peaks with high prevalence rates, a prompt and effective diagnosis was critical for proper patient isolation and evaluation. However, since the prevalence of the COVID-19 cases oscillated, showing fewer cases between waves, and more non-COVID cases, it was important to differentiate patients with other diseases than COVID-19 presenting similar visual characteristics in the CXR.
During patient assessment in the emergency room, clinicians take into account different inputs for a proper diagnosis. First, the anamnesis, symptoms, vitals and physical findings guide the physician to an initial assumption. Based on this information, additional tests are requested (CXR, blood test, ECG and SARS-CoV-2 detection). The integration of these results allows the team to diagnose a patient accurately. However, this process is time consuming and sometimes findings are difficult to interpret, leading to misdiagnosis.
To improve this diagnostic process, we have developed and trained a multimodal deep learning algorithm based in a multiple input approach combining CXR images together with blood sample data to identify COVID-19 diagnosis with high sensitivity. This way we were able to manage the increased complexity of the dataset. These data from multiple sources are somehow correlated and complementary to each other and could reflect patterns that are not present in single models alone 13 .
Hence, MultiCOVID is fed by two of the most common and fast clinical tests requested in the emergency room (CXR and Blood test) and can predict the presence of three different diseases (COVID-19, heart failure and non-COVID pneumonia) with similar CXR characteristics.
Analysis of single models shows the importance of model interpretation. While CXR-only models could identify patterns outside the lung parenchyma that could diminish its generalization capacity 9 , Blood-only models could point to interesting population of cells that are differently represented in COVID-19 patients, leveraging its prediction capacity. In this context, the immune compartment plays an important role in the COVID-19 response, and it has been already published that COVID-19 patients present fewer overall leukocytes counts and, more concretely, eosinophil counts 14 , 15 . Furthermore, oxygen transport seems to be somehow affected, modulating the red cell population. In this regard, in our work we found significant differences in the erythrocyte count and the hemoglobin concentration. Although most of the studies correlate the reduction of this values to severe COVID-19 patients 16 , this is the first dataset to compare them in these four different categories at the time of diagnosis.
Moreover, although a huge amount of literature about COVID-19 diagnosis and prognosis has been published using only blood tests 17 , 18 , 19 , 20 or CXR 21 , 22 , 23 , 24 , 25 , 26 , 27 , 28 this is the first study that combines both parameters and compares its prediction capacity at diagnosis. Of note, only one previously published study integrates both blood test and CXR severity scores in order to determine in-hospital death of COVID-19 patients 29 . Hence, it is clear that merging both sources of data leads to a better prediction performance when compared with the two single models alone and that this difference is more pronounced where the number of cases is scarce. It is important to stress that this combination of data sources addresses the variable prevalence of COVID-19 cases during the pandemic, which is an issue that could not be solved in previous studies 23 , 24 .
Our study has several limitations. First, the algorithm was evaluated on a single center; thus, there was likely some degree of bias. Additionally, the sample collection was performed in different time periods for each group of patients, which could present some kind of differences in the CXR image acquisition although this was partially solved using the lung segmentation model which removes the noise signal present outside the lung parenchyma. And finally, model performance could be influenced by potential shifts in the disease landscape due to COVID-19 variants and vaccination efforts, which could influence the generalizability and interpretation of our findings.
Conclusions
We have developed a multimodal deep learning algorithm, MultiCOVID, that discriminates among COVID-19, heart failure, non-COVID pneumonia and healthy patients using both CXR and blood test with a significantly better performance than experienced thoracic radiologists.
Our approach and results suggest an innovative scenario where COVID-19 prediction could be identified from other similar diseases and facilitate triage within the emergency room in a COVID-19 low prevalence situation.
Data availability
Our code base is provided on GitHub at https://github.com/Tato14/MultiCOVID , including weights for each of the individually trained neural network architectures and respective model weights for the weighted ensemble model. The datasets used and analyzed during the current study will be available from the corresponding author on reasonable request. In order to correct samples bias 11 , additional metadata information present in the DICOM image headers from the CXR would be also available upon request.
Abbreviations
Deep learning
Chest X-rays
Area under the receiver operating characteristic curve
Coronavirus disease 2019
Reverse-transcription polymerase chain reaction
Severe acute respiratory syndrome coronavirus 2
Heart failure
Non-COVID pneumonia
DeGrave, A. J., Janizek, J. D. & Lee, S.-I. AI for radiographic COVID-19 detection selects shortcuts over signal. Nat. Mach. Intell. 3 , 610–619 (2021).
Article Google Scholar
Cleverley, J., Piper, J. & Jones, M. M. The role of chest radiography in confirming covid-19 pneumonia. BMJ https://doi.org/10.1136/bmj.m2426 (2020).
Article PubMed Google Scholar
Avila, E., Kahmann, A., Alho, C. & Dorn, M. Hemogram data as a tool for decision-making in COVID-19 management: Applications to resource scarcity scenarios. PeerJ 8 , e9482 (2020).
Article PubMed PubMed Central Google Scholar
Razavian, N. et al. A validated, real-time prediction model for favorable outcomes in hospitalized COVID-19 patients. npj Digit. Med. 3 , 130 (2020).
Trevethan, R. Sensitivity, specificity, and predictive values: Foundations, pliabilities, and pitfalls in research and practice. Front. Public Heal. 5 , 307 (2017).
Ronneberger, O., Fischer, P. & Brox, T. U-Net: Convolutional Networks for Biomedical Image Segmentation 234–241 (2015). https://doi.org/10.1007/978-3-319-24574-4_28 .
He, K., Zhang, X., Ren, S. & Sun, J. Deep residual learning for image recognition. in 2016 IEEE Conference on Computer Vision and Pattern Recognition (CVPR) 770–778 (IEEE, 2016). https://doi.org/10.1109/CVPR.2016.90 .
Arik, S. Ö. & Pfister, T. TabNet: Attentive interpretable tabular learning. Proc. AAAI Conf. Artif. Intell. 35 (8), 6679–6687 (2021).
Google Scholar
Wang, W., Tran, D. & Feiszli, M. What makes training multi-modal classification networks hard?. In Proceedings / IEEE Computer Society Conference on Computer Vision and Pattern Recognition 12692–12702 (2019) https://doi.org/10.1109/CVPR42600.2020.01271 .
Howard, J. & Gugger, S. Fastai: A layered API for deep learning. Information 11 , 108 (2020).
Garcia Santa Cruz, B., Bossa, M. N., Sölter, J. & Husch, A. D. Public Covid-19 X-ray datasets and their impact on model bias—A systematic review of a significant problem. Med. Image Anal. 74 , 102225 (2021).
Lundberg, S. M. et al. From local explanations to global understanding with explainable AI for trees. Nat. Mach. Intell. 2 , 56–67 (2020).
Ngiam, J. et al. Multimodal deep learning. ICML (2011).
Tan, Y., Zhou, J., Zhou, Q., Hu, L. & Long, Y. Role of eosinophils in the diagnosis and prognostic evaluation of COVID-19. J. Med. Virol. 93 , 1105–1110 (2021).
Article CAS PubMed Google Scholar
Rahman, A. et al. Hematological abnormalities in COVID-19: A narrative review. Am. J. Trop. Med. Hyg. 104 , 1188–1201 (2021).
Article CAS PubMed PubMed Central Google Scholar
Lippi, G. & Mattiuzzi, C. Hemoglobin value may be decreased in patients with severe coronavirus disease 2019. Hematol. Transfus. Cell Ther. 42 , 116–117 (2020).
Kukar, M. et al. COVID-19 diagnosis by routine blood tests using machine learning. Sci. Rep. 11 , 10738 (2021).
Article ADS CAS PubMed PubMed Central Google Scholar
Bayat, V. et al. A Severe acute respiratory syndrome coronavirus 2 (SARS-CoV-2) prediction model from standard laboratory tests. Clin. Infect. Dis. 73 , e2901–e2907 (2021).
Soltan, A. A. S. et al. Rapid triage for COVID-19 using routine clinical data for patients attending hospital: Development and prospective validation of an artificial intelligence screening test. Lancet Digit. Heal. 3 , e78–e87 (2021).
Article CAS Google Scholar
Chen, J. et al. Distinguishing between COVID-19 and influenza during the early stages by measurement of peripheral blood parameters. J. Med. Virol. 93 , 1029–1037 (2021).
Hwang, E. J. et al. Deep learning for chest radiograph diagnosis in the emergency department. Radiology 293 , 573–580 (2019).
Wang, L., Lin, Z. Q. & Wong, A. COVID-Net: A tailored deep convolutional neural network design for detection of COVID-19 cases from chest X-ray images. Sci. Rep. 10 , 19549 (2020).
Wehbe, R. M. et al. DeepCOVID-XR: An artificial intelligence algorithm to detect COVID-19 on chest radiographs trained and tested on a large U.S. clinical data set. Radiology 299 , E167–E176 (2021).
Zhang, R. et al. Diagnosis of coronavirus disease 2019 pneumonia by using chest radiography: Value of artificial intelligence. Radiology 298 , E88–E97 (2021).
Baikpour, M. et al. Role of a chest x-ray severity score in a multivariable predictive model for mortality in patients with COVID-19: A single-center, retrospective study. J. Clin. Med. 11 , 2157 (2022).
Nishio, M. et al. Deep learning model for the automatic classification of COVID-19 pneumonia, non-COVID-19 pneumonia, and the healthy: A multi-center retrospective study. Sci. Rep. 12 , 8214 (2022).
Sun, Y. et al. Use of machine learning to assess the prognostic utility of radiomic features for in-hospital COVID-19 mortality. Sci. Rep. 13 , 7318 (2023).
Nishio, M., Noguchi, S., Matsuo, H. & Murakami, T. Automatic classification between COVID-19 pneumonia, non-COVID-19 pneumonia, and the healthy on chest X-ray image: Combination of data augmentation methods. Sci. Rep. 10 , 17532 (2020).
Garrafa, E. et al. Early prediction of in-hospital death of COVID-19 patients: A machine-learning model based on age, blood analyses, and chest x-ray score. Elife 10 , e70640 (2021).
Download references
Author information
Authors and affiliations.
Cancer Research Program, IMIM (Hospital del Mar Medical Research Institute), Barcelona, Spain
Max Hardy-Werbin, Nieves Garcia-Gisbert, Beatriz Bellosillo & Joan Gibert
Radiology Department, Hospital del Mar, Barcelona, Spain
José Maria Maiques, Marcos Busto, Flavio Zuccarino, Santiago Carbullanca, Luis Alexander Del Carpio, Didac Ramal & Ángel Gayete
Emergency Department, Hospital del Mar, Barcelona, Spain
Max Hardy-Werbin, Isabel Cirera & Alfons Aguirre
Innovation and Digital Transformation Department, Hospital del Mar, Barcelona, Spain
Jordi Martínez-Roldan
Information Systems Department, Hospital del Mar, Barcelona, Spain
Albert Marquez-Colome
Pathology Department, Hospital del Mar, Barcelona, Spain
Beatriz Bellosillo & Joan Gibert
You can also search for this author in PubMed Google Scholar
Contributions
M.H.-W.: Data curation , Validation, Formal analysis, Investigation, Project administration, Supervision, Roles/Writing—original draft, Writing—review & editing; J.M.M.: Data curation , Formal analysis, Investigation, Validation, Roles/Writing—original draft, Writing—review & editing; M.B.: Data curation, Formal analysis, Investigation Validation, Roles/Writing—original draft, Writing—review & editing; I.C.: Data curation, Validation, Roles/Writing—original draft, Writing—review & editing; A.A.: Data curation, Validation, Roles/Writing—original draft, Writing—review & editing; N.G.-G.: Investigation, Visualization, Project administration, Roles/Writing—original draft, Writing—review & editin; F.Z.: Validation, Roles/Writing—original draft, Writing—review & editing; S.C.: Validation, Roles/Writing—original draft, Writing—review & editing, L.A.D.C.: Validation, Roles/Writing—original draft, Writing—review & editing, D.R.: Validation, Roles/Writing—original draft, Writing—review & editing; Á.G.: Validation, Roles/Writing—original draft, Writing—review & editin; J.M.-R.: Project administration, Supervision, Roles/Writing—original draft, Writing—review & editing; A.M.-C.: Data curation, Project administration, Supervision, Roles/Writing—original draft, Writing—review & editing; B.B.: Data curation, Formal analysis, Investigation, Project administration, Supervision, Roles/Writing—original draft, Writing—review & editin; J.G.: Data curation, Formal analysis; Investigation, Visualization, Project administration, Supervision, Roles/Writing—original draft, Writing—review & editing.
Corresponding author
Correspondence to Joan Gibert .
Ethics declarations
Competing interests.
The authors declare no competing interests.
Additional information
Publisher's note.
Springer Nature remains neutral with regard to jurisdictional claims in published maps and institutional affiliations.
Supplementary Information
Supplementary information 1., supplementary table 1., rights and permissions.
Open Access This article is licensed under a Creative Commons Attribution 4.0 International License, which permits use, sharing, adaptation, distribution and reproduction in any medium or format, as long as you give appropriate credit to the original author(s) and the source, provide a link to the Creative Commons licence, and indicate if changes were made. The images or other third party material in this article are included in the article's Creative Commons licence, unless indicated otherwise in a credit line to the material. If material is not included in the article's Creative Commons licence and your intended use is not permitted by statutory regulation or exceeds the permitted use, you will need to obtain permission directly from the copyright holder. To view a copy of this licence, visit http://creativecommons.org/licenses/by/4.0/ .
Reprints and permissions
About this article
Cite this article.
Hardy-Werbin, M., Maiques, J.M., Busto, M. et al. MultiCOVID: a multi modal deep learning approach for COVID-19 diagnosis. Sci Rep 13 , 18761 (2023). https://doi.org/10.1038/s41598-023-46126-8
Download citation
Received : 10 February 2023
Accepted : 27 October 2023
Published : 31 October 2023
DOI : https://doi.org/10.1038/s41598-023-46126-8
Share this article
Anyone you share the following link with will be able to read this content:
Sorry, a shareable link is not currently available for this article.
Provided by the Springer Nature SharedIt content-sharing initiative
By submitting a comment you agree to abide by our Terms and Community Guidelines . If you find something abusive or that does not comply with our terms or guidelines please flag it as inappropriate.
Quick links
- Explore articles by subject
- Guide to authors
- Editorial policies
Sign up for the Nature Briefing: Translational Research newsletter — top stories in biotechnology, drug discovery and pharma.

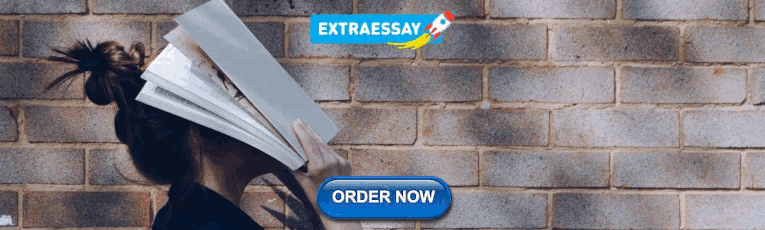
IMAGES
VIDEO
COMMENTS
COVID-19 measures decreased mobility by half in Budapest, Hungary. •. Modal share of public transport decreased dramatically due to COVID-19 measures. •. Car usage increased to 65% from 43% in the modal share. •. Bicycle usage saw the greatest growth rate by more than doubling its share in the modal share. •.
The unprecedented shock triggered by the COVID-19 pandemic has caused significant impact on public transportation services, travel behavior and mode choice preferences. Increasing risk of virus contagion in shared travel modes might result in a systemic shift from public transport to car commute. Such a shift causes increased congestions ...
For 300 random test images, overall accuracy of MultiCOVID was significantly higher (69.6%) compared with individual radiologists (range, 43.7–58.7%) and the consensus of all five radiologists ...
This article analyses the uses of modal auxiliary verbs in reporting Covid-19 in selected articles in Daily Nation newspaper. Covid-19 is a member of the beta coronaviruses that can cause acute severe respiratory symptoms or possibly mild, cold-like symptoms. The reporting of the disease was done in the newspaper in Kenya every day from the first time it was reported in the country on 13th ...