Guidelines for Reporting of Figures and Tables for Clinical Research in Urology
Affiliations.
- 1 Memorial Sloan Kettering Cancer Center, New York, New York.
- 2 Janssen Research & Development, Raritan, New Jersey.
- 3 Vanderbilt University School of Medicine, Nashville, Tennessee.
- 4 Southern Illinois University School of Medicine, Springfield, Illinois.
- 5 University of Chicago, Chicago, Illinois.
- 6 MD Anderson Cancer Center, University of Texas, Houston, Texas.
- 7 Cleveland Clinic, Cleveland, Ohio.
- PMID: 32441187
- DOI: 10.1097/JU.0000000000001096
In an effort to improve the presentation of and information within tables and figures in clinical urology research, we propose a set of appropriate guidelines. We introduce six principles: (1) include graphs only if they improve the reader's ability to understand the study findings; (2) think through how a graph might best convey information, do not just select a graph from preselected options on statistical software; (3) do not use graphs to replace reporting key numbers in the text of a paper; (4) graphs should give an immediate visual impression of the data; (5) make it beautiful; and (6) make the labels and legend clear and complete. We present a list of quick "dos and don'ts" for both tables and figures. Investigators should feel free to break any of the guidelines if it would result in a beautiful figure or a clear table that communicates data effectively. That said, we believe that the quality of tables and figures in the medical literature would improve if these guidelines were to be followed. Patient summary: A set of guidelines were developed for presenting figures and tables in urology research. The guidelines were developed by a broad group of statistical experts with special interest in urology.
Keywords: figures; guidelines; reporting guidelines; tables.
- Biomedical Research / standards*
- Computer Graphics / standards*
- Publishing / standards*
- Statistics as Topic / standards*
Thank you for visiting nature.com. You are using a browser version with limited support for CSS. To obtain the best experience, we recommend you use a more up to date browser (or turn off compatibility mode in Internet Explorer). In the meantime, to ensure continued support, we are displaying the site without styles and JavaScript.
- View all journals
- My Account Login
- Explore content
- About the journal
- Publish with us
- Sign up for alerts
- Open access
- Published: 11 April 2024
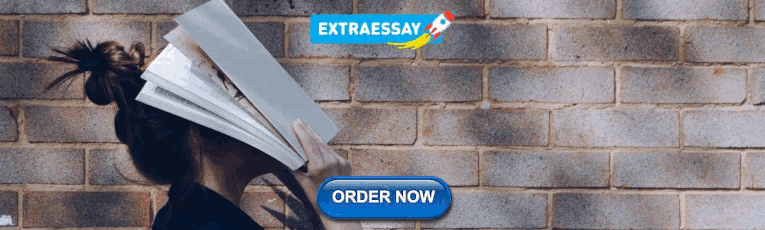
Reporting guidelines in medical artificial intelligence: a systematic review and meta-analysis
- Fiona R. Kolbinger ORCID: orcid.org/0000-0003-2265-4809 1 , 2 , 3 , 4 , 5 , 6 na1 ,
- Gregory P. Veldhuizen 1 na1 ,
- Jiefu Zhu 1 ,
- Daniel Truhn ORCID: orcid.org/0000-0002-9605-0728 7 &
- Jakob Nikolas Kather ORCID: orcid.org/0000-0002-3730-5348 1 , 8 , 9 , 10
Communications Medicine volume 4 , Article number: 71 ( 2024 ) Cite this article
328 Accesses
3 Altmetric
Metrics details
- Diagnostic markers
- Medical research
- Predictive markers
- Prognostic markers
The field of Artificial Intelligence (AI) holds transformative potential in medicine. However, the lack of universal reporting guidelines poses challenges in ensuring the validity and reproducibility of published research studies in this field.
Based on a systematic review of academic publications and reporting standards demanded by both international consortia and regulatory stakeholders as well as leading journals in the fields of medicine and medical informatics, 26 reporting guidelines published between 2009 and 2023 were included in this analysis. Guidelines were stratified by breadth (general or specific to medical fields), underlying consensus quality, and target research phase (preclinical, translational, clinical) and subsequently analyzed regarding the overlap and variations in guideline items.
AI reporting guidelines for medical research vary with respect to the quality of the underlying consensus process, breadth, and target research phase. Some guideline items such as reporting of study design and model performance recur across guidelines, whereas other items are specific to particular fields and research stages.
Conclusions
Our analysis highlights the importance of reporting guidelines in clinical AI research and underscores the need for common standards that address the identified variations and gaps in current guidelines. Overall, this comprehensive overview could help researchers and public stakeholders reinforce quality standards for increased reliability, reproducibility, clinical validity, and public trust in AI research in healthcare. This could facilitate the safe, effective, and ethical translation of AI methods into clinical applications that will ultimately improve patient outcomes.
Plain Language Summary
Artificial Intelligence (AI) refers to computer systems that can perform tasks that normally require human intelligence, like recognizing patterns or making decisions. AI has the potential to transform healthcare, but research on AI in medicine needs clear rules so caregivers and patients can trust it. This study reviews and compares 26 existing guidelines for reporting on AI in medicine. The key differences between these guidelines are their target areas (medicine in general or specific medical fields), the ways they were created, and the research stages they address. While some key items like describing the AI model recurred across guidelines, others were specific to the research area. The analysis shows gaps and variations in current guidelines. Overall, transparent reporting is important, so AI research is reliable, reproducible, trustworthy, and safe for patients. This systematic review of guidelines aims to increase the transparency of AI research, supporting an ethical and safe progression of AI from research into clinical practice.
Similar content being viewed by others
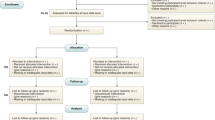
Reporting guidelines for clinical trial reports for interventions involving artificial intelligence: the CONSORT-AI extension
Xiaoxuan Liu, Samantha Cruz Rivera, … The SPIRIT-AI and CONSORT-AI Working Group
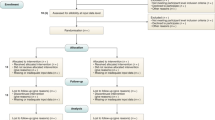
Guidelines for clinical trial protocols for interventions involving artificial intelligence: the SPIRIT-AI extension
Samantha Cruz Rivera, Xiaoxuan Liu, … SPIRIT-AI and CONSORT-AI Consensus Group
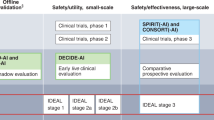
Reporting guideline for the early-stage clinical evaluation of decision support systems driven by artificial intelligence: DECIDE-AI
Baptiste Vasey, Myura Nagendran, … the DECIDE-AI expert group
Introduction
The field of Artificial Intelligence (AI) is rapidly growing and its applications in the medical field have the potential to revolutionize the way diseases are diagnosed and treated. Despite the field still being in its relative infancy, deep learning algorithms have already proven to perform at parity with or better than current gold standards for a variety of tasks related to patient care. For example, deep learning models perform on par with human experts in classification of skin cancer 1 , aid in both the timely identification of patients with sepsis 2 and respective adaptation of the treatment strategy 3 , and can identify genetic alterations from histopathological imaging across different cancer types 4 . Due to the black box nature of many AI-based investigations, it is critical that the methodology and results of the findings are reported in a thorough, transparent and reproducible manner. However, despite this need, such measures are often omitted 5 . High reporting standards are vital in ensuring that public trust, medical efficacy and scientific integrity are not compromised by erroneous, often overly positive performance metrics due to flaws such as skewed data selection or methodological errors such as data leakage.
To address these challenges, numerous reporting guidelines have been developed to regulate AI-related research in preclinical, translational, and clinical settings. A reporting guideline is a set of criteria and recommendations designed to standardize the reporting of research methodologies and findings. These guidelines aim to ensure the inclusion of minimum essential information within research studies and thereby enhance transparency, reproducibility, and the overall quality of research reporting 6 , 7 . While clinical treatment guidelines typically describe a summary of standards of care based on existing medical evidence, there is no universal standard approach for the development of reporting guidelines regarding what information should be provided when attempting to publish findings from a scientific investigation. Consequently, the quality of reporting guidelines can vary depending on the methods used to reach consensus as well as the individuals involved in the process. The Delphi method, when employed by a panel of authoritative experts in the relevant field, is generally considered to be the most appropriate means of obtaining high-quality agreement 8 . This method describes a structured technique in which experts cycle through several rounds of questionnaires, with each round resulting in an updated questionnaire that is provided to participants along with a summary of responses in the subsequent iteration. This pattern is repeated until consensus is reached.
Another factor to consider when developing reporting guidelines for medical AI is their scope. Reporting guidelines may be specific to the unique needs of a single clinical specialty or intended to be more general in nature. In addition, due to the highly dynamic nature of AI research, these guidelines require frequent reassessment to safeguard against obsolescence. As a consequence of the breadth of stakeholders involved in the development and regulation of medical AI, including government organizations, academic institutions, publishers and corporations, a multitude of reporting guidelines have arisen. The repercussion of this is a notable lack of clarity for researchers as to which guidelines to follow, uncertainty whether or not guidelines exist for their specific domain of research, and whether or not reporting standards can be expected to be enforced by publishers of mainstream academic journals. As a result, despite the abundance of reporting guidelines for healthcare, only a fraction of research items adheres to them 9 , 10 , 11 . This reflects a deficiency on the part of researchers and scholarly publishers alike.
This systematic review provides an overview of existing reporting guidelines for AI-related research in medicine that have been published by research consortia, federal institutions, or adopted by medical and medical informatics publishers. It summarizes the key elements that are near-universally considered necessary when reporting findings to ensure maximum reproducibility and clinical validity. These key elements include descriptions of the clinical rationale, the data that reported models are based on, and of the training and validation process. By highlighting guideline items that are widely agreed upon, our work aims to provide orientation to researchers, policymakers, and stakeholders in the field of medical AI and form a basis for the development of future reporting guidelines with the goal of ensuring maximum reproducibility and clinical translatability of AI-related medical research. In addition, our summary of key reporting items may provide guidance for researchers in situations where no high-quality reporting guideline currently exists for the topic of their research.
Search strategy
We report the results of this systematic review following the PRISMA 2020 statement for reporting systematic reviews 12 . To cover the breadth of published AI-related reporting guidelines in medicine, our search strategies included three sources: (i) Guidelines published as scholarly research publications listed in the database PubMed and in the EQUATOR Network’s library of reporting guidelines ( https://www.equator-network.org/library/ ), (ii) AI-related statements and requirements of international federal health agencies, and (iii) relevant journals in Medicine and Medical Informatics. The search strategy was developed by three authors with experience in medical AI research (FRK, GPV, JNK), and no preprint servers were included in the search.
PubMed was searched on June 26, 2022, without language restrictions, for literature published since database inception, on AI guidelines in the fields of preclinical, translational, and clinical medicine, using the keywords (“Artificial Intelligence” OR “Machine Learning” OR “Deep Learning”) AND (“consensus statement” OR “guideline” OR “checklist”). The EQUATOR Network’s library of reporting guidelines was searched on November 14, 2023, using the keywords “Artificial Intelligence”, “Machine Learning” and “Deep Learning”. Additionally, statements and requirements of the federal health agencies of the United States (Food and Drug Administration, FDA), the European Union (European Medicines Agency, EMA), the United Kingdom (Medicines and Healthcare Products Regulatory Agency), China (National Medical Products Association), and Japan (Pharmaceuticals and Medical Devices Agency) were reviewed with respect to further guidelines and requirements. Finally, the ten journals in Medicine and Medical Informatics with the highest journal impact factors in 2021 according to the Clarivate Journal Citation reports were screened for specific AI/ML checklist requirements for submitted articles. Studies identified as incidental findings were added independent of the aforementioned search process, thereby including studies published after the initial search on June 26, 2022.
Study selection
Duplicate studies were removed. All search results were independently screened by two physicians with experience in clinical AI research (FRK and GPV) using Rayyan 13 . Screening results were blinded until completion of each reviewer’s individual screening. The inclusion criteria were (1) the topic of the publication being AI in medicine and (2) the guideline recommendations being specific to the application of AI methods for either preclinical, translational, or clinical scenarios. Publications were excluded on the basis of (1) not providing actionable reporting guidance, (2) collecting or reassembling guideline items from existing guidelines rather than providing new guideline items or (3) reporting the intention to develop a new, as yet unpublished guideline rather than the guideline itself. Disagreements regarding guideline selection were resolved by judgment of a third reviewer (JNK).
Data extraction and analysis
Two physicians with experience in clinical AI research (FRK, GPV) reviewed all selected guidelines and extracted the year of publication, the target research phase (preclinical, translational and/or clinical research), the breadth of the guideline (general or specific to a medical subspecialty) and the consensus process as a way to assess the risk of bias. The target research phase was considered preclinical if the guideline regulates theoretical studies not involving clinical outcome data but potentially retrospectively involving patient data, translational if the guideline targets retrospective or prospective observational trials involving patient data with a potential clinical implication, and clinical if the guideline regulates interventional trials in a clinical setting. The breadth of a guideline was considered general or subject-specific depending on target research areas mentioned in the guideline. Additionally, reporting guidelines were independently graded by FRK and GPV (with arbitration by a third rater, JNK, in case of disagreement) as being either “comprehensive”, “collaborative” or “expert-led” in their consensus process. The consensus process of a guideline was classified as expert-led if the method by which it was developed did not appear to be through a consensus-based procedure, if the guideline did not involve relevant stakeholders, or if the described development procedure was not clearly outlined. Guidelines were classified as collaborative if the authors (presumably) used a formal consensus procedure involving multiple experts, but provided no details on the exact protocol or methodological structure. Comprehensive guidelines outlined a structured, consensus-based, methodical development approach involving multiple experts and relevant stakeholders with details on the exact protocol (e.g., using the Delphi procedure).
FRK and GPV extracted each guideline’s recommended items for the purpose of creating an omnibus list of all as-yet published guideline items (Supplementary Table 1 ). FRK and GPV independently evaluated each guideline for the purpose of determining which items from the omnibus list were either fully, partially, or not covered by each publication individually. Aspects that were directly described in a guideline including some details or examples were considered “fully” covered, aspects mentioned implicitly using general terms were considered “partially” covered. Disagreements were resolved by judgment of a third reviewer (JNK). Overlap of guideline content was visualized using pyCirclize 14 . Items recommended by at least 50% of all reporting guidelines or 50% of reporting guidelines with a specified systematic development process (i.e., comprehensive consensus) were considered universal recommendations for clinical AI research reporting.
Study registration
This systematic review was registered at OSF https://doi.org/10.17605/OSF.IO/YZE6J on August 25, 2023. The protocol was not amended or changed.
Reporting summary
Further information on research design is available in the Nature Portfolio Reporting Summary linked to this article.
Search results
The PubMed database search yielded 622 unique publications; another 18 guidelines were identified through other sources: 8 guidelines were identified through a search of the EQUATOR Network’s library of reporting guidelines, two guidelines were identified through review of recommendations of federal agencies; one additional guideline was included based on review of journal recommendations. Another seven additional guidelines were added as incidental findings.
After removal of duplicates, 630 publications were subjected to the screening process. Out of these, 578 records were excluded based on Title and Abstract. Of the remaining 52 full-text articles assessed for eligibility, 26 records were excluded and 26 reporting guidelines were included in the systematic review and meta-analysis (Fig. 1 ). Interrater agreement for study selection on the basis of full-text records was 71% ( n = 15 requiring third reviewer out of n = 52).
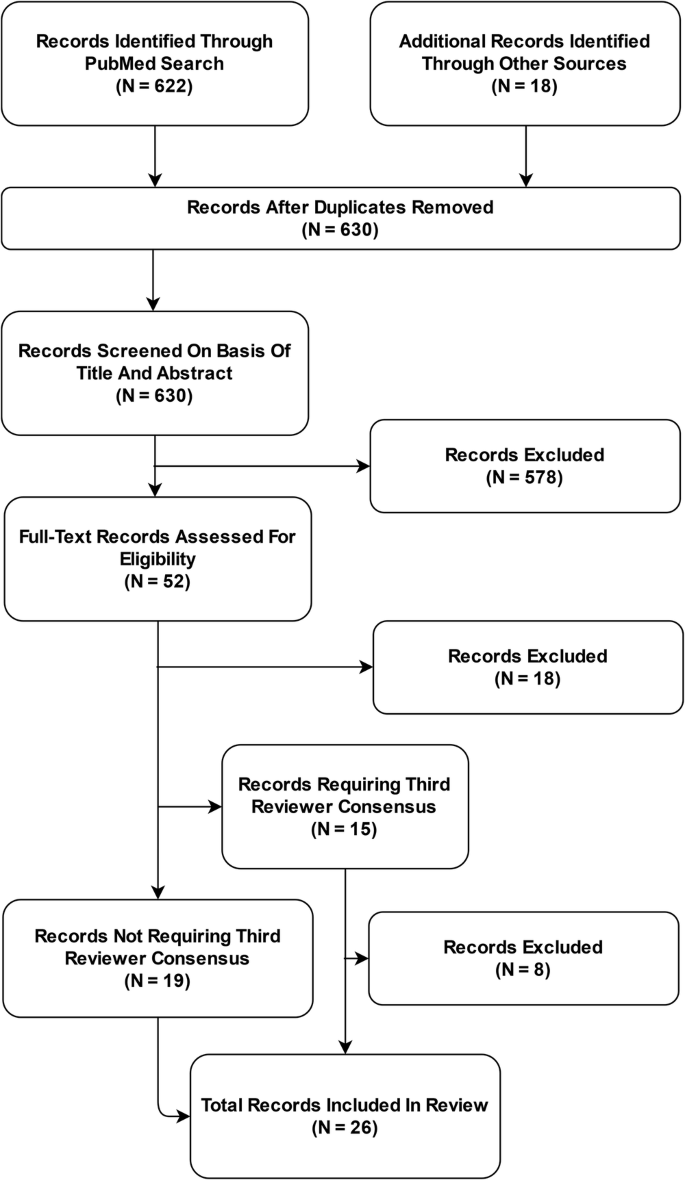
Based on a systematic review of academic publications and reporting standards demanded by international federal health institutions and leading journals in the fields of medicine and medical informatics, 26 reporting guidelines published between 2009 and 2023 were included in this analysis.
The landscape of reporting guidelines in clinical AI
A total of 26 reporting guidelines was included in this systematic review. We identified nine comprehensive, six collaborative and eleven expert-led reporting guidelines. Approximately half of all reporting guidelines ( n = 14, 54%) provided general guidelines for AI-related research in medicine. The remaining publications ( n = 12, 46%) were developed to regulate the reporting of AI-related research within a specific field of medicine. These included medical physics, dermatology, cancer diagnostics, nuclear medicine, medical imaging, cardiovascular imaging, neuroradiology, psychiatry, and dental research (Table 1 , Figs. 2 and 3 ).
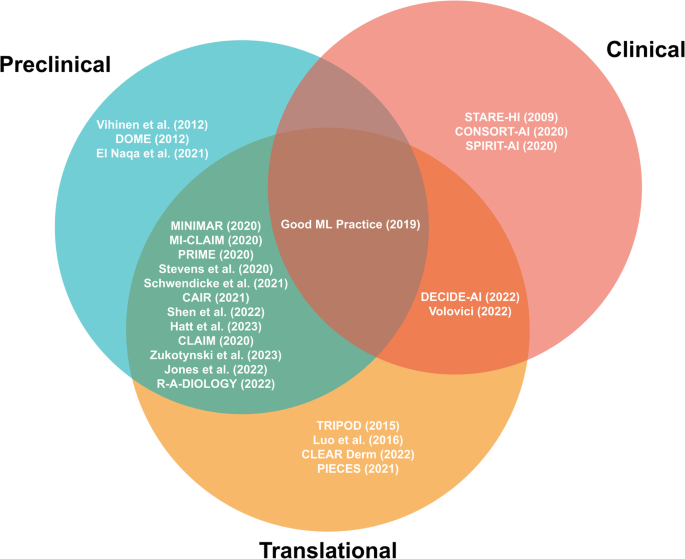
Preclinical guidelines regulate theoretical studies not involving clinical outcome data but potentially retrospectively involving patient data. Translational guidelines target retrospective or prospective observational trials involving patient data with a potential clinical implication. Clinical guidelines regulate interventional trials in a clinical setting. Reporting guidelines catering towards specific research phases are able to be more specific in their items, while those aimed at overlapping research phases tend to necessitate more general reporting items.
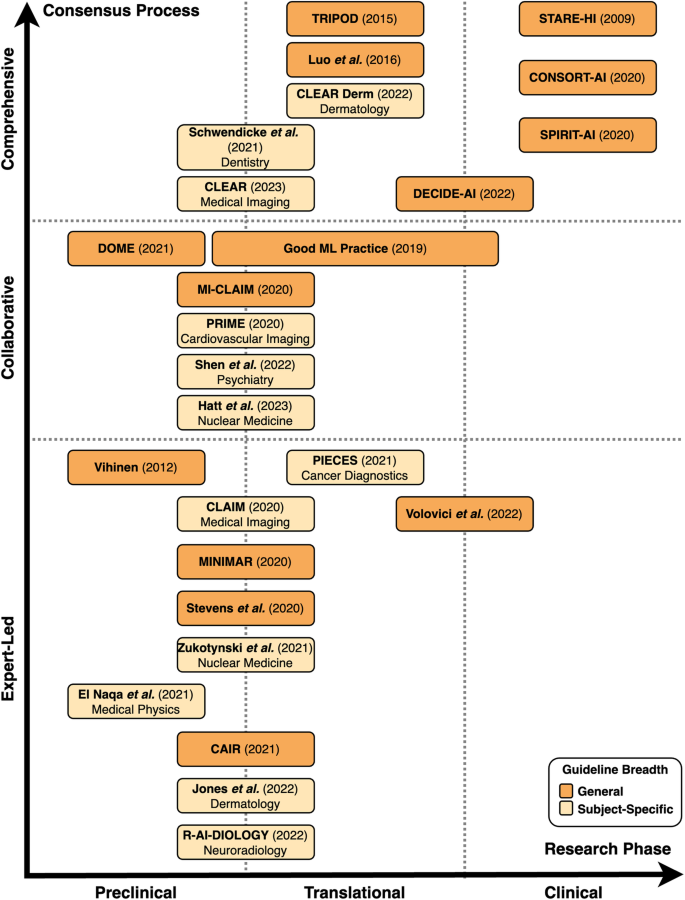
Preclinical guidelines regulate theoretical studies not involving clinical outcome data but potentially retrospectively involving patient data. Translational guidelines target retrospective or prospective observational trials involving patient data with a potential clinical implication. Clinical guidelines regulate interventional trials in a clinical setting. The breadth of guidelines is classified as general or subject-specific depending on target research areas mentioned in the guideline. In terms of the consensus process, comprehensive guidelines are based on a structured, consensus-based, methodical development approach involving multiple experts and relevant stakeholders with details on the exact protocol. Collaborative guidelines are (presumably) developed using a formal consensus procedure involving multiple experts, but provide no details on the exact protocol or methodological structure. Expert-led guidelines are not developed through a consensus-based procedure, do not involve relevant stakeholders, or do not clearly describe the development procedure.
We systematically categorized the reporting guidelines by the research phase that they were aimed at as well as the level of consensus used in their development (Fig. 2 , Fig. 3 ). The majority of guidelines ( n = 20, 77%) concern AI applications for preclinical and translational research rather than clinical trials. Of these preclinical and translational reporting guidelines, many ( n = 12) are specific for individual fields of medicine such as cardiovascular imaging, psychiatry or dermatology rather than generally applicable recommendations. In addition, these guidelines tend to more often be expert-led or collaborative ( n = 15) in nature rather than comprehensive ( n = 5). This is in contrast to the considerably fewer clinical reporting guidelines ( n = 6) that are universally general in nature and overwhelmingly comprehensive in their consensus process ( n = 4). There has been a notable increase in the publication of reporting guidelines in recent years, with 81% ( n = 21) of included guidelines having been published in or after 2020.
Consensus in guideline items
The identified guidelines were analyzed with respect to their overlap in individual guideline recommendations (Supplementary Table 1 , Fig. 4a, b ). A total of 37 unique guideline items were identified. These concerned Clinical Rationale (7 items), Data (11 items), Model Training and Validation (9 items), Critical Appraisal (3 items), and Ethics and Reproducibility (7 items). We were unable to identify a clear weighting towards certain items over others within our primary means of clustering reporting guidelines, namely the consensus procedure and whether the guideline is directed at specific research fields or provides general guidance (Fig. 4b ).
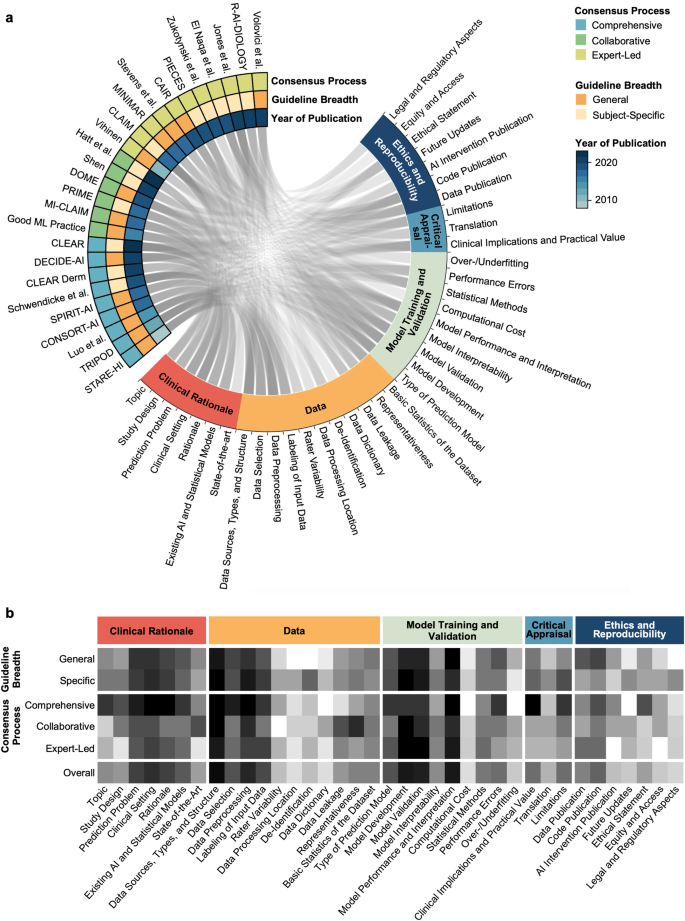
The Circos plot ( a ) displays represented content as a connecting line between guideline and guideline items. The heatmap ( b ) displays the differential representation of specific guideline aspects depending on guideline quality and breadth. Darker color represents a higher proportion of representation of the respective guideline aspect in the respective group of reporting guidelines for medical AI.
Figure 5 summarizes items that were recommended by at least 50% of all guidelines or 50% of guidelines with a specified systematic development process (comprehensive guidelines). These items are considered universal components of studies on predictive clinical AI models.
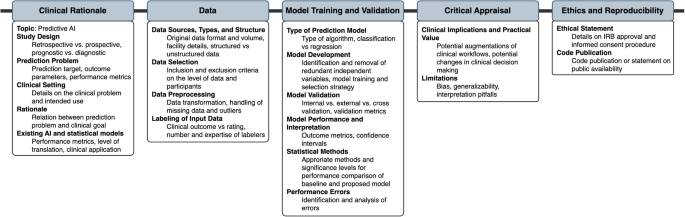
Items recommended by at least 50% of all guidelines or 50% of guidelines with a specified systematic development process were considered universal components of studies on predictive clinical AI models.
With the increasing availability of computational resources and methodological advances, the field of AI-based medical applications has experienced significant growth over the last decade. To ensure reproducibility, responsible use and clinical validity of such applications, numerous guidelines have been published, with varying development strategies, structures, application targets, content and support from research communities. We conducted a systematic review of existing guidelines for AI applications in medicine, with a focus on assessing their quality, application areas, and content.
Our analysis suggests that the majority of AI-related reporting guidelines has been conceived by individual (groups of) stakeholders without a formal consensus process and that most reporting guidelines address preclinical and translational research rather than the clinical validation of AI-based applications. Guidelines targeting specific medical fields often result from less rigorous consensus processes than broader guidelines targeting medical AI in general, resulting in some use cases for which several high-evidence guidelines are available (i.e., dermatology, medical imaging), whereas no specialty-independent guideline developed in a formal consensus process is currently available for preclinical research.
Differences in data types and tasks that AI can address in different medical specialties represent a key challenge for the development of guidelines for AI applications in medicine. Many predominantly diagnostics-based specialties such as pathology or radiology rely heavily on different types of imaging with distinct peculiarities and challenges. The need to account for such differences is stronger in preclinical and translational steps of development as compared to clinical evaluation, where AI applications are tested for validity.
Most specialty-specific guidelines address preclinical phases, and these guidelines have predominantly been conceived in less rigorous consensus processes. While individual peculiarities of specific use cases may be clearer in specific guidelines than in more general guidelines, it is conceivable that subject-specific guidelines could result in many guidelines on the same topic when use cases and guideline requirements are similar across fields. To address this issue, stratification by data type could be a potential solution to ensure that guidelines are universal yet specific enough to regulate.
Incorporation of innovations in guidelines represents another challenge, as guidelines have traditionally been distributed in the form of academic publications. In this context, the fact that AI represents a major methodological innovation has been acknowledged by regulating institutions such as the EQUATOR network, which has issued AI-specific counterparts for existing guidelines, including CONSORT(-AI) regulating randomized controlled clinical trials and SPIRIT(-AI) regulating interventional clinical trial protocols. Several other comprehensive high-quality AI-specific guideline extensions are expected to become publicly available in the near future including STARD-AI 15 , TRIPOD-AI 16 , and PRISMA-AI 17 . Ideally, guidelines should be adaptive and interactive to dynamically integrate new innovations as they emerge. Two quality assessment tools, PROBAST-AI 16 (for risk of bias and applicability assessment of prediction model studies) and QUADAS-AI 18 (for quality assessment of AI-centered diagnostic accuracy studies), will be developed alongside the anticipated AI-specific reporting guidelines.
To prevent the previously mentioned creation of multiple guidelines on the same topic, guidelines could potentially be continuously updated. However, this requires careful management to ensure that guidelines remain relevant and up-to-date without becoming overwhelming or contradictory. On a similar line, it may be worth considering whether AI-specific guidelines should repeat non-AI-specific items, such as ethics statements or Institutional Review Board (IRB) requirements. It may be useful to compare these needs with good scientific practice, to refer to existing resources, and to consider how best to balance comprehensiveness with clarity and ease of use. Whenever new guidelines are being developed, it is advisable to follow available guidance to ensure high guideline quality through methods like a structured literature review and a multi-stage Delphi process 19 , 20 .
Before entering clinical practice, medical innovations must undergo a rigorous evaluation process, and regulatory needs play a crucial role in this process. However, this can lead to undynamic processes, resulting in a gap between large amounts of preclinical research that largely do not enter steps towards clinical translation. Therefore, future guidelines should include items relevant to translational processes, such as regulatory sciences, access, updates, and assessment of feasibility for implementation into clinical practice. Less than half of the guidelines included in this review mentioned such items. By including such statements, better selection of disruptive and clinically impactful research could be made.
Despite various available guidelines, some use cases including preclinical research remain poorly regulated, and it is necessary to address gaps in existing guidelines. For such cases, it is advisable to identify the most relevant general guideline and adhere to key guideline items that are universally accepted and should be part of any AI research in the medical field. As a consequence, researchers can be guided on what to include in their research, and regulatory bodies can be more stringent in demanding adherence to guidelines. In this context, our review resulted in the finding that many high-impact medical and medical informatics journals do not demand adherence to any guidelines. While peer reviewers can encourage respective additions, more stringency in adherence to guidelines would help ensure the responsible use of AI-based medical applications.
While the content of reporting guidelines in medical AI has been critically reviewed previously 21 , 22 , this is, to our knowledge, the first systematic review on reporting guidelines used in various stages of AI-related medical research. Importantly, this review focuses on guidelines for AI applications in healthcare and intentionally does not consider guidelines for prediction models in general; this has been done elsewhere 10 .
The limitations of this systematic review are primarily related to its methodology: First, our search strategy was developed by three of the authors (FRK, GPV, JNK), without any external review of the search strategy 23 and without input from a librarian. Similarly, our systematic search was limited to the publication database PubMed, the EQUATOR Network’s library of reporting guidelines ( https://www.equator-network.org/library ), journal guidelines and guidelines of major federal institutions. An involvement of internal peer reviewers with journalogical experience in the development of the search strategy and an inclusion of preprint servers in the search may have revealed additional guidelines to include in this systematic review. Second, our systematic review included only a basic assessment of the risk of bias, differentiating between expert-led, collaborative and comprehensive guidelines by analyzing the rigor of the consensus process. While risk of bias assessment tools developed for systematic reviews of observational or interventional trials 24 , 25 would not be appropriate for a methodological review, an in-depth analysis with a custom, methods-centered tool 26 could have provided more insights on the specific shortcomings of the included guidelines. Third, we acknowledge the potential limitation of the context-agnostic nature of our summary of consensus items. While we intentionally adopted a generalized approach to create broadly applicable findings, we recognize that this lack of nuance may result in our findings being of varying applicability depending on the specific subject domain. Fourth, this systematic review has limitations related to guideline selection and classification and limited generalizability. To allow for focused comparison of guideline content, only those reporting guidelines offering actionable items were included. Three high-quality reporting guidelines were excluded given that they do not specifically address AI in medicine: STARD 27 , STROBE 28 , and SPIRIT 29 , 30 . While these guidelines are clearly out of the scope of this systematic review and some of these guidelines have dedicated AI-specific guidelines in development (e.g. STARD-AI), indicating that the creators of the guidelines themselves may have seen deficiencies regarding computational medical research, they could still have provided valuable insights. Similarly, some publications were considered out of scope for reviewing very specific areas of AI such as surrogate metrics 31 without demanding actionable items. In addition, future guideline updates could result in changes in the landscape of AI reporting guidelines, which this systematic review cannot represent. Nevertheless, this review contributes to the scientific landscape in two ways: First, it provides a resource for scientists as to what guideline to adhere to. Second, it highlights potential areas for improvement that policymakers, scientific institutions and journal editors can reinforce.
In conclusion, this systematic review provides a comprehensive overview of existing guidelines for AI applications in medicine. While the guidelines reviewed vary in quality and scope, they generally provide valuable guidance for developing and evaluating AI-based models. However, the lack of standardization across guidelines, particularly regarding the ethical, legal, and social implications of AI in healthcare, highlights the need for further research and collaboration in this area. Furthermore, as AI-based models become more prevalent in clinical practice, it will be essential to update guidelines regularly to reflect the latest developments in the field and ensure their continued relevance. Good scientific practice needs to be reinforced by every individual scientist and every scientific institution. It is the same with reporting guidelines. No guideline in itself can guarantee quality and reproducibility of research. A guideline only unfolds its power when interpreted by responsible scientists.
Data availability
All included guidelines are publicly available. The list of guideline items included in published guidelines regulating medical AI research that was generated in this systematic review is published along with this work (Supplementary Table 1 ).
Esteva, A. et al. Dermatologist-level classification of skin cancer with deep neural networks. Nature. 542 , 115–118 (2017).
Article CAS PubMed PubMed Central Google Scholar
Lauritsen, S. M. et al. Early detection of sepsis utilizing deep learning on electronic health record event sequences. Artif. Intell. Med. 104 , 101820 (2020).
Article PubMed Google Scholar
Wu, X., Li, R., He, Z., Yu, T. & Cheng, C. A value-based deep reinforcement learning model with human expertise in optimal treatment of sepsis. NPJ Digit. Med. 6 , 15 (2023).
Article PubMed PubMed Central Google Scholar
Kather, J. N. et al. Pan-cancer image-based detection of clinically actionable genetic alterations. Nat. Cancer. 1 , 789–799 (2020).
Jayakumar, S. et al. Quality assessment standards in artificial intelligence diagnostic accuracy systematic reviews: a meta-research study. NPJ Digit. Med. 5 , 11 (2022).
Simera, I., Moher, D., Hoey, J., Schulz, K. F. & Altman, D. G. The EQUATOR Network and reporting guidelines: Helping to achieve high standards in reporting health research studies. Maturitas. 63 , 4–6 (2009).
Simera, I. et al. Transparent and accurate reporting increases reliability, utility, and impact of your research: reporting guidelines and the EQUATOR Network. BMC Med. 8 , 24 (2010).
Rayens, M. K. & Hahn, E. J. Building Consensus Using the Policy Delphi Method. Policy Polit. Nurs. Pract. 1 , 308–315 (2000).
Article Google Scholar
Samaan, Z. et al. A systematic scoping review of adherence to reporting guidelines in health care literature. J. Multidiscip. Healthc. 6 , 169–188 (2013).
PubMed PubMed Central Google Scholar
Lu, J. H. et al. Assessment of Adherence to Reporting Guidelines by Commonly Used Clinical Prediction Models From a Single Vendor: A Systematic Review. JAMA Netw. Open. 5 , e2227779 (2022).
Yusuf, M. et al. Reporting quality of studies using machine learning models for medical diagnosis: a systematic review. BMJ Open. 10 , e034568 (2020).
Page, M. J. et al. The PRISMA 2020 statement: An updated guideline for reporting systematic reviews. J. Clin. Epidemiol. 134 , 178–189 (2021).
Ouzzani, M., Hammady, H., Fedorowicz, Z. & Elmagarmid, A. Rayyan-a web and mobile app for systematic reviews. Syst. Rev. 5 , 210 (2016).
Shimoyama Y. Circular visualization in Python (Circos Plot, Chord Diagram) - pyCirclize. Github; Available: https://github.com/moshi4/pyCirclize (accessed: April 1, 2024).
Sounderajah, V. et al. Developing a reporting guideline for artificial intelligence-centred diagnostic test accuracy studies: the STARD-AI protocol. BMJ Open. 11 , e047709 (2021).
Collins, G. S. et al. Protocol for development of a reporting guideline (TRIPOD-AI) and risk of bias tool (PROBAST-AI) for diagnostic and prognostic prediction model studies based on artificial intelligence. BMJ Open. 11 , e048008 (2021).
Cacciamani, G. E. et al. PRISMA AI reporting guidelines for systematic reviews and meta-analyses on AI in healthcare. Nat. Med. 29 , 14–15 (2023).
Article CAS PubMed Google Scholar
Sounderajah, V. et al. A quality assessment tool for artificial intelligence-centered diagnostic test accuracy studies: QUADAS-AI. Nat. Med. 27 , 1663–1665 (2021).
Moher, D., Schulz, K. F., Simera, I. & Altman, D. G. Guidance for developers of health research reporting guidelines. PLoS Med. 7 , e1000217 (2010).
Schlussel, M. M. et al. Reporting guidelines used varying methodology to develop recommendations. J. Clin. Epidemiol. 159 , 246–256 (2023).
Ibrahim, H., Liu, X. & Denniston, A. K. Reporting guidelines for artificial intelligence in healthcare research. Clin. Experiment. Ophthalmol. 49 , 470–476 (2021).
Shelmerdine, S. C., Arthurs, O. J., Denniston, A. & Sebire N. J. Review of study reporting guidelines for clinical studies using artificial intelligence in healthcare. BMJ Health Care Inform. 28 , https://doi.org/10.1136/bmjhci-2021-100385 (2021).
McGowan, J. et al. PRESS Peer Review of Electronic Search Strategies: 2015 Guideline Statement. J. Clin. Epidemiol. 75 , 40–46 (2016).
Sterne, J. A. C. et al. RoB 2: a revised tool for assessing risk of bias in randomised trials. BMJ. 366 , l4898 (2019).
Higgins, J. P. T. et al. The Cochrane Collaboration’s tool for assessing risk of bias in randomised trials. BMJ. 343 , d5928 (2011).
Cukier, S. et al. Checklists to detect potential predatory biomedical journals: a systematic review. BMC Med. 18 , 104 (2020).
Bossuyt, P.M. et al. Towards complete and accurate reporting of studies of diagnostic accuracy: The STARD Initiative. Radiology. 226 , 24–28 (2003).
Elm von, E. et al. Strengthening the Reporting of Observational Studies in Epidemiology (STROBE) statement: guidelines for reporting observational studies. BMJ 335 , 806–808 (2007).
Chan, A.-W. et al. SPIRIT 2013 statement: defining standard protocol items for clinical trials. Ann. Intern. Med. 158 , 200–207 (2013).
Chan, A.-W. et al. SPIRIT 2013 explanation and elaboration: guidance for protocols of clinical trials. BMJ. 346 , e7586 (2013).
Reinke, A. et al. Common Limitations of Image Processing Metrics: A Picture Story. arXiv. https://doi.org/10.48550/arxiv.2104.05642 (2021).
Talmon, J. et al. STARE-HI-Statement on reporting of evaluation studies in Health Informatics. Int. J. Med. Inform. 78 , 1–9 (2009).
Vihinen, M. How to evaluate performance of prediction methods? Measures and their interpretation in variation effect analysis. BMC Genomics. 13 , S2 (2012).
Collins, G. S., Reitsma, J. B., Altman, D. G., Moons, K. G. M. Transparent reporting of a multivariable prediction model for individual prognosis or diagnosis (TRIPOD): The TRIPOD statement. BMJ . 350 , https://doi.org/10.1136/bmj.g7594 (2015).
Luo, W. et al. Guidelines for Developing and Reporting Machine Learning Predictive Models in Biomedical Research: A Multidisciplinary View. J. Med. Internet Res. 18 , e323 (2016).
Center for Devices, Radiological Health. Good Machine Learning Practice for Medical Device Development: Guiding Principles . U.S. Food and Drug Administration, FDA, 2023. Available: https://www.fda.gov/medical-devices/software-medical-device-samd/good-machine-learning-practice-medical-device-development-guiding-principles .
Mongan, J., Moy, L. & Kahn, C. E. Jr. Checklist for Artificial Intelligence in Medical Imaging (CLAIM): A Guide for Authors and Reviewers. Radiol. Artif. Intell. 2 , e200029 (2020).
Liu, X., Rivera, S. C., Moher, D., Calvert, M. J. & Denniston, A. K. SPIRIT-AI and CONSORT-AI Working Group Reporting guidelines for clinical trial reports for interventions involving artificial intelligence: the CONSORT-AI Extension. BMJ. 370 , m3164 (2020).
Norgeot, B. et al. Minimum information about clinical artificial intelligence modeling: the MI-CLAIM checklist. Nat. Med. 26 , 1320–1324 (2020).
Sengupta, P. P. et al. Proposed requirements for cardiovascular imaging-related machine learning evaluation (PRIME): A checklist. JACC Cardiovasc. Imaging. 13 , 2017–2035 (2020).
Cruz Rivera, S., Liu, X., Chan, A.-W., Denniston, A. K. & Calvert, M. J. SPIRIT-AI and CONSORT-AI Working Group Guidelines for clinical trial protocols for interventions involving artificial intelligence: the SPIRIT-AI extension. Lancet Digit Health. 2 , e549–e560 (2020).
Hernandez-Boussard, T., Bozkurt, S., Ioannidis, J. P. A. & Shah, N. H. MINIMAR (MINimum Information for Medical AI Reporting): Developing reporting standards for artificial intelligence in health care. J. Am. Med. Inform. Assoc. 27 , 2011–2015 (2020).
Stevens, L. M., Mortazavi, B. J., Deo, R. C., Curtis, L. & Kao, D. P. Recommendations for Reporting Machine Learning Analyses in Clinical Research. Circ. Cardiovasc. Qual. Outcomes. 13 , e006556 (2020).
Walsh, I., Fishman, D., Garcia-Gasulla, D., Titma, T. & Pollastri, G. ELIXIR Machine Learning Focus Group, et al. DOME: recommendations for supervised machine learning validation in biology. Nat. Methods. 18 , 1122–1127 (2021).
Olczak, J. et al. Presenting artificial intelligence, deep learning, and machine learning studies to clinicians and healthcare stakeholders: an introductory reference with a guideline and a Clinical AI Research (CAIR) checklist proposal. Acta Orthop. 92 , 513–525 (2021).
Kleppe, A. et al. Designing deep learning studies in cancer diagnostics. Nat. Rev. Cancer. 21 , 199–211 (2021).
El Naqa, I. et al. AI in medical physics: guidelines for publication. Med. Phys. 48 , 4711–4714 (2021).
Zukotynski, K. et al. Machine Learning in Nuclear Medicine: Part 2—Neural Networks and Clinical Aspects. J. Nucl. Med. 62 , 22–29 (2021).
Schwendicke, F. et al. Artificial intelligence in dental research: Checklist for authors, reviewers, readers. J. Dent. 107 , 103610 (2021).
Daneshjou, R. et al. Checklist for Evaluation of Image-Based Artificial Intelligence Reports in Dermatology: CLEAR Derm Consensus Guidelines From the International Skin Imaging Collaboration Artificial Intelligence Working Group. JAMA Dermatol. 158 , 90–96 (2022).
Vasey, B. et al. Reporting guideline for the early-stage clinical evaluation of decision support systems driven by artificial intelligence: DECIDE-AI. Nat. Med. 28 , 924–933 (2022).
Jones, O. T. et al. Artificial intelligence and machine learning algorithms for early detection of skin cancer in community and primary care settings: a systematic review. Lancet Digit Health. 4 , e466–e476 (2022).
Haller, S., Van Cauter, S., Federau, C., Hedderich, D. M. & Edjlali, M. The R-AI-DIOLOGY checklist: a practical checklist for evaluation of artificial intelligence tools in clinical neuroradiology. Neuroradiology. 64 , 851–864 (2022).
Shen, F. X. et al. An Ethics Checklist for Digital Health Research in psychiatry: Viewpoint. J. Med. Internet Res. 24 , e31146 (2022).
Volovici, V., Syn, N. L., Ercole, A., Zhao, J. J. & Liu, N. Steps to avoid overuse and misuse of machine learning in clinical research. Nat. Med. 28 , 1996–1999 (2022).
Hatt, M. et al. Joint EANM/SNMMI guideline on radiomics in nuclear medicine: Jointly supported by the EANM Physics Committee and the SNMMI Physics, Instrumentation and Data Sciences Council. Eur. J. Nucl. Med. Mol. Imaging. 50 , 352–375 (2023).
Kocak, B. et al. CheckList for EvaluAtion of Radiomics research (CLEAR): a step-by-step reporting guideline for authors and reviewers endorsed by ESR and EuSoMII. Insights Imaging. 14 , 75 (2023).
Download references
Acknowledgements
F.R.K. is supported by the German Cancer Research Center (CoBot 2.0), the Joachim Herz Foundation (Add-On Fellowship for Interdisciplinary Life Science) and the German Research Foundation (Deutsche Forschungsgemeinschaft, DFG) as part of Germany’s Excellence Strategy (EXC 2050/1, Project ID 390696704) within the Cluster of Excellence”Centre for Tactile Internet with Human-in-the-Loop” (CeTI) of Dresden University of Technology. Furthermore, F.R.K. receives support from the Indiana Clinical and Translational Sciences Institute funded, in part, by Grant Number UM1TR004402 from the National Institutes of Health, National Center for Advancing Translational Sciences, Clinical and Translational Sciences Award. G.P.V. is partly supported by BMBF (Federal Ministry of Education and Research) in DAAD project 57616814 (SECAI, School of Embedded Composite AI, https://secai.org/ ) as part of the program Konrad Zuse Schools of Excellence in Artificial Intelligence. J.N.K. is supported by the German Federal Ministry of Health (DEEP LIVER, ZMVI1-2520DAT111) and the Max-Eder-Programme of the German Cancer Aid (grant #70113864), the German Federal Ministry of Education and Research (PEARL, 01KD2104C; CAMINO, 01EO2101; SWAG, 01KD2215A; TRANSFORM LIVER, 031L0312A), the German Academic Exchange Service (SECAI, 57616814), the German Federal Joint Committee (Transplant.KI, 01VSF21048) the European Union (ODELIA, 101057091; GENIAL, 101096312) and the National Institute for Health and Care Research (NIHR, NIHR213331) Leeds Biomedical Research Centre. The views expressed are those of the author(s) and not necessarily those of the National Institutes of Health, the NHS, the NIHR or the Department of Health and Social Care.
Open Access funding enabled and organized by Projekt DEAL.
Author information
These authors contributed equally: Fiona R. Kolbinger, Gregory P. Veldhuizen.
Authors and Affiliations
Else Kroener Fresenius Center for Digital Health, TUD Dresden University of Technology, Dresden, Germany
Fiona R. Kolbinger, Gregory P. Veldhuizen, Jiefu Zhu & Jakob Nikolas Kather
Department of Visceral, Thoracic and Vascular Surgery, University Hospital and Faculty of Medicine Carl Gustav Carus, TUD Dresden University of Technology, Dresden, Germany
Fiona R. Kolbinger
Weldon School of Biomedical Engineering, Purdue University, West Lafayette, IN, USA
Regenstrief Center for Healthcare Engineering, Purdue University, West Lafayette, IN, USA
Department of Biostatistics and Health Data Science, Richard M. Fairbanks School of Public Health, Indiana University, Indianapolis, IN, USA
Indiana University Simon Comprehensive Cancer Center, Indiana University School of Medicine, Indianapolis, IN, USA
Department of Diagnostic and Interventional Radiology, University Hospital Aachen, Aachen, Germany
Daniel Truhn
Department of Medicine III, University Hospital RWTH Aachen, Aachen, Germany
Jakob Nikolas Kather
Department of Medicine I, University Hospital Dresden, Dresden, Germany
Medical Oncology, National Center for Tumor Diseases (NCT), University Hospital Heidelberg, Heidelberg, Germany
You can also search for this author in PubMed Google Scholar
Contributions
F.R.K., G.P.V. and J.N.K. conceptualized the study, developed the search strategy, conducted the review, curated, analyzed, and interpreted the data. F.R.K., G.P.V. and J.Z. prepared visualizations. D.T. and J.N.K. provided oversight, mentorship, and funding. F.R.K. and G.P.V. wrote the original draft of the manuscript. All authors reviewed and approved the final version of the manuscript.
Corresponding author
Correspondence to Jakob Nikolas Kather .
Ethics declarations
Competing interests.
D.T. holds shares in StratifAI GmbH and has received honoraria for lectures from Bayer. J.N.K. declares consulting services for Owkin, France, DoMore Diagnostics, Norway, Panakeia, UK, Scailyte, Switzerland, Cancilico, Germany, Mindpeak, Germany, MultiplexDx, Slovakia, and Histofy, UK; furthermore, he holds shares in StratifAI GmbH, Germany, has received a research grant by GSK, and has received honoraria by AstraZeneca, Bayer, Eisai, Janssen, MSD, BMS, Roche, Pfizer and Fresenius. All other authors declare no conflicts of interest.
Peer review
Peer review information.
Communications Medicine thanks Weijie Chen, David Moher and the other, anonymous, reviewer(s) for their contribution to the peer review of this work. A peer review file is available.
Additional information
Publisher’s note Springer Nature remains neutral with regard to jurisdictional claims in published maps and institutional affiliations.
Supplementary information
Peer review file, supplementary information, reporting summary, rights and permissions.
Open Access This article is licensed under a Creative Commons Attribution 4.0 International License, which permits use, sharing, adaptation, distribution and reproduction in any medium or format, as long as you give appropriate credit to the original author(s) and the source, provide a link to the Creative Commons licence, and indicate if changes were made. The images or other third party material in this article are included in the article’s Creative Commons licence, unless indicated otherwise in a credit line to the material. If material is not included in the article’s Creative Commons licence and your intended use is not permitted by statutory regulation or exceeds the permitted use, you will need to obtain permission directly from the copyright holder. To view a copy of this licence, visit http://creativecommons.org/licenses/by/4.0/ .
Reprints and permissions
About this article
Cite this article.
Kolbinger, F.R., Veldhuizen, G.P., Zhu, J. et al. Reporting guidelines in medical artificial intelligence: a systematic review and meta-analysis. Commun Med 4 , 71 (2024). https://doi.org/10.1038/s43856-024-00492-0
Download citation
Received : 18 August 2023
Accepted : 27 March 2024
Published : 11 April 2024
DOI : https://doi.org/10.1038/s43856-024-00492-0
Share this article
Anyone you share the following link with will be able to read this content:
Sorry, a shareable link is not currently available for this article.
Provided by the Springer Nature SharedIt content-sharing initiative
Quick links
- Explore articles by subject
- Guide to authors
- Editorial policies
Sign up for the Nature Briefing newsletter — what matters in science, free to your inbox daily.


An official website of the United States government
The .gov means it’s official. Federal government websites often end in .gov or .mil. Before sharing sensitive information, make sure you’re on a federal government site.
The site is secure. The https:// ensures that you are connecting to the official website and that any information you provide is encrypted and transmitted securely.
- Publications
- Account settings
Preview improvements coming to the PMC website in October 2024. Learn More or Try it out now .
- Advanced Search
- Journal List
- HHS Author Manuscripts

Guidelines for Reporting of Statistics for Clinical Research in Urology
Melissa assel.
a Memorial Sloan Kettering Cancer Center, New York, NY, USA
Daniel Sjoberg
Andrew elders.
b Glasgow Caledonian University, Glasgow, UK
Xuemei Wang
c The University of Texas, MD Anderson Cancer Center, Houston, TX, USA
Dezheng Huo
d The University of Chicago, Chicago, IL, USA
Albert Botchway
e Southern Illinois University School of Medicine, Springfield, IL, USA
Kristin Delfino
f University of Minnesota, Minneapolis, MN, USA
Zhiguo Zhao
g Cleveland Clinic, Cleveland, OH, USA
Tatsuki Koyama
h Vanderbilt University Medical Center, Nashville, TN, USA
Brent Hollenbeck
i University of Michigan, Ann Arbor, MI, USA
j Janssen Research & Development, NJ, USA
Whitney Zahnd
k University of South Carolina, Columbia, SC, USA
Emily C. Zabor
Michael w. kattan, andrew j. vickers.
Author contributions : Andrew J. Vickers had full access to all the data in the study and takes responsibility for the integrity of the data and the accuracy of the data analysis.
Acquisition of data : None.
Analysis and interpretation of data : None.
Drafting of the manuscript : Vickers, Assel, Sjoberg, Kattan.
Critical revision of the manuscript for important intellectual content : All authors.
Statistical analysis : None.
Obtaining funding : None.
Administrative, technical, or material support : None.
Supervision : None.
Other : None.
In an effort to improve the quality of statistics in the clinical urology literature, statisticians at European Urology, The Journal of Urology, Urology , and BJUI came together to develop a set of guidelines to address common errors of statistical analysis, reporting, and interpretation. Authors should “break any of the guidelines if it makes scientific sense to do so” but would need to provide a clear justification. Adoption of the guidelines will, in our view, not only increase the quality of published papers in our journals, but also improve statistical knowledge in our field in general.
It is widely acknowledged that the quality of statistics in the clinical research literature is poor. This is true for urology just as it is for other medical specialties. In 2005, Scales et al [ 1 ] published a systematic evaluation of the statistics in papers appearing in a single month in one of the four leading urology medical journals: European Urology , The Journal of Urology , Urology , and BJUI . They reported widespread errors, including 71% of papers with comparative statistics having at least one statistical flaw. These findings mirror many others in the literature; see, for instance, the review given by Lang and Altman [ 2 ]. The quality of statistical reporting in urology journals has no doubt improved since 2005, but remains unsatisfactory.
The four urology journals in the Scales et al’s [ 1 ] review have come together to publish a shared set of statistical guidelines, adapted from those in use at one of the journals, European Urology , since 2014 [ 3 ]. The guidelines will also be adopted by European Urology Focus and European Urology Oncology . Statistical reviewers at the four journals will systematically assess submitted manuscripts using the guidelines to improve statistical analysis, reporting, and interpretation. Adoption of the guidelines will, in our view, not only increase the quality of published papers in our journals, but also improve statistical knowledge in our field in general. Asking an author to follow a guideline about, say, the fallacy of accepting the null hypothesis would no doubt result in a better paper, but we hope that it would also enhance the author’s understanding of hypothesis tests.
The guidelines are didactic, based on the consensus of the statistical consultants to the journals. We avoided, where possible, making specific analytic recommendations and focused instead on analyses or methods of reporting statistics that should be avoided. We intend to update the guidelines over time and hence encourage readers who question the value or rationale of a guideline to write to the authors.
1. The golden rule
1.1. break any of the guidelines if it makes scientific sense to do so.
Science varies too much to allow methodologic or reporting guidelines to apply universally.
2. Reporting of design and statistical analysis
2.1. follow existing reporting guidelines for the type of study you are reporting, such as consort for randomized trials, remark for marker studies, tripod for prediction models, strobe for observational studies, or amstar for systematic reviews.
Statisticians and methodologists have contributed extensively to a large number of reporting guidelines. The first is widely recognized to be the Consolidated Standards of Reporting Trials (CONSORT) statement on reporting of randomized trials, but there are now many other guidelines, covering a wide range of different types of study. Reporting guidelines can be downloaded from the Equator website ( http://www.equator-network.org ).
2.2. Describe cohort selection fully
It is insufficient to state, for instance, that “the study cohort consisted of 1144 patients treated for benign prostatic hyperplasia at our institution.” The cohort needs to be defined in terms of dates (eg, “presenting March 2013 to December 2017”), inclusion criteria (eg, “IPSS > 12”), and whether patients were selected to be included (eg, for a research study) versus being a consecutive series. Exclusions should be described one by one, with the number of patients omitted for each exclusion criterion to give the final cohort size (eg, “patients with prior surgery [ n = 43], allergies to 5-ARIs [ n = 12], and missing data on baseline prostate volume [ n = 86] were excluded to give a final cohort for analysis of 1003 patients”). Note that the inclusion criteria can be omitted if obvious from the context (eg, no need to state “undergoing radical prostatectomy for histologically proven prostate cancer”); on the contrary, dates may need to be explained if their rationale could be questioned (eg, “March 2013, when our specialist voiding clinic was established, to December 2017”).
2.3. Describe the practical steps of randomization in randomized trials
Although this reporting guideline is part of the CONSORT statement, it is so critical and so widely misunderstood that it bears repeating. The purpose of randomization is to prevent selection bias. This can be achieved only if the consenting patients cannot guess their treatment allocation before registration in the trial or change it afterward. This safeguard is known as allocation concealment . Stating merely that “a randomization list was created by a statistician” or that “envelope randomization was used” does not ensure allocation concealment: a list could have been posted in the nurse’s station for all to see; envelopes can be opened and resealed. Investigators need to specify the exact logistic steps taken to ensure allocation concealment. The best method is to use a password-protected computer database.
2.4. The statistical methods should describe the study questions and the statistical approaches used to address each question
Many statistical methods sections state only something like “Mann-Whitney was used for comparisons of continuous variables and Fisher’s exact for comparisons of binary variables.” This says little more than “the inference tests used were not grossly erroneous for the type of data.” Instead, statistical methods sections should lay out each primary study question separately: carefully detail the analysis associated with each and describe the rationale for the analytic approach, if this is not obvious or there are reasonable alternatives. Special attention and description should be provided for rarely used statistical techniques.
2.5. The statistical methods should be described in sufficient detail to allow replication by an independent statistician given the same data set
Vague reference to “adjusting for confounders” or “nonlinear approaches” is insufficiently specific to allow replication, a cornerstone of the scientific method. All statistical analyses should be specified in the Methods section, including details such as the covariates included in a multivariable model. All variables should be clearly defined where there is room for ambiguity. For instance, avoid saying that “Gleason grade was included in the model”; state instead “Gleason grade group was included in four categories 1, 2, 3, and 4 or 5.”
3. Inference and p values
3.1. do not accept the null hypothesis.
In a court case, defendants are declared guilty or not guilty; there is no verdict of “innocent.” Similarly, in a statistical test, the null hypothesis is rejected or not rejected. If the p value is 0.05 or higher, investigators should avoid conclusions such as “the drug was ineffective,” “there was no difference between groups,” or “response rates were unaffected.” Instead, authors should use phrases such as “we did not see evidence of a drug effect,” “we were unable to demonstrate a difference between groups,” or simply “there was no statistically significant difference in response rates.”
3.2. P values just above 5% are not a trend, and they are not moving
Avoid saying that a p value such as 0.07 shows a “trend” (which is meaningless) or “approaches statistical significance” (because the p value is not moving). Alternative language might be that “although we saw some evidence of improved response rates in patients receiving the novel procedure, differences between groups did not meet conventional levels of statistical significance.”
3.3. The p values and 95% confidence intervals do not quantify the probability of a hypothesis
A p value of, say, 0.03 does not mean that there is 3% probability that the findings are due to chance. Additionally, a 95% confidence interval (CI) should not be interpreted as a 95% certainty that the true parameter value is in the range of the 95% CI. The correct interpretation of a p value is the probability of finding the observed or more extreme results when the null hypothesis is true; the 95% CI will contain the true parameter value 95% of the time were a study to be repeated many times using different samples.
3.4. Do not use confidence intervals to test hypotheses
Investigators often interpret confidence intervals in terms of hypotheses. For instance, investigators might claim that there is a statistically significant difference between groups because the 95% CI for the odds ratio excludes 1. Such claims are problematic because confidence intervals are concerned with estimation, and not inference. Moreover, the mathematical method to calculate confidence intervals may be different from those used to calculate p values. It is perfectly possible to have a 95% CI that includes no difference between groups even though the p value is <0.05 or vice versa. For instance, in a study of 100 patients in two equal groups, with event rates of 70% and 50%, the p value from Fisher’s exact test is 0.066 but the 95% CI for the odds ratio is 1.03–5.26. The 95% CI for the risk difference and risk ratio also exclude no difference between groups.
3.5. Take care to interpret results when reporting multiple p values
The more questions you ask, the more likely you are to get a spurious answer to at least one of them. For example, if you report p values for five independent true null hypotheses, the probability that you will falsely reject at least one is not 5%, but >20%. Although formal adjustment of p values is appropriate in some specific cases, such as genomic studies, a more common approach is to simply interpret p values in the context of multiple testing. For instance, if an investigator examines the association of 10 variables with three different endpoints, thereby testing 30 separate hypotheses, a p value of 0.04 should not be interpreted in the same way as if the study tested only a single hypothesis with a p value of 0.04.
3.6. Do not report separate p values for each of two different groups in order to address the question of whether there is a difference between groups
One scientific question means one statistical hypothesis tested by one p value. To illustrate the error of using two p values to address one question, take the case of a randomized trial of drug versus placebo to reduce voiding symptoms, with 30 patients in each group. The authors might report that symptom scores improved by 6 (standard deviation 14) points in the drug group ( p = 0.03 by one-sample t test) and by 5 (standard deviation 15) points in the placebo group ( p = 0.08). However, the study hypothesis concerns the difference between drug and placebo. To test a single hypothesis, a single p value is needed. A two-sample t test for these data gives a p value of 0.8—unsurprising, given that the scores in each group were virtually the same—confirming that it would be unsound to conclude that the drug was effective based on the finding that the change was significant in the drug group but not in placebo controls.
3.7. Use interaction terms in place of subgroup analyses
A similar error to the use of separate tests for a single hypothesis is when an intervention is shown to have a statistically significant effect in one group of patients but not in another. A more appropriate approach is to use what is known as an interaction term in a statistical model. For instance, to determine whether a drug reduced pain scores more in women than in men, the model might be as follows:
It is sometimes appropriate to report estimates and confidence intervals within subgroups of interest, but p values should be avoided.
3.8. Tests for change over time are generally uninteresting
A common analysis is to conduct a paired t test comparing, say, erectile function in older men at baseline with erectile function after 5 yr of follow-up. The null hypothesis here is that “erectile function does not change over time,” which is known to be false. Investigators are encouraged to focus on estimation rather than on inference, reporting, for example, the mean change over time along with a 95% CI.
3.9. Avoid using statistical tests to determine the type of analysis to be conducted
Numerous statistical tests are available that can be used to determine how a hypothesis test should be conducted. For instance, investigators might conduct a Shapiro-Wilk test for normality to determine whether to use a t test or a Mann-Whitney test, and Cochran’s Q to decide whether to use a fixed-effect or a random-effect approach in a meta-analysis or to use a t test for between-group differences in a covariate to determine whether that covariate should be included a multivariable model. The problem with these sorts of approaches is that they are often testing a null hypothesis that is known to be false. For instance, no data set perfectly follows a normal distribution. Moreover, it is often questionable that changing the statistical approach in the light of the test is actually of benefit. Statisticians are far from unanimous as to whether Mann-Whitney is always superior to t test when data are nonnormal, or that fixed effects are invalid under study heterogeneity, or that the criterion of adjusting for a variable should be whether it is significantly different between groups. Investigators should generally follow a prespecified analytic plan, only altering the analysis if the data unambiguously point to a better alternative.
3.10. When reporting p values, be clear about the hypothesis tested and ensure that the hypothesis is a sensible one
The p values test very specific hypotheses. When reporting a p value in the Results section, state the hypothesis being tested unless this is completely clear. Take, for instance, the statement “pain scores were higher in group 1 and similar in groups 2 and 3 ( p = 0.02).” It is ambiguous whether the p value of 0.02 is testing group 1 versus groups 2 and 3 combined or the hypothesis that pain score is same in all three groups. Clarity about the hypotheses being tested can help avoid the testing of inappropriate hypotheses. For instance, p values for differences between groups at baseline in a randomized trial is testing a null hypothesis that is known to be true (informally, that any observed differences between groups are due to chance).
4. Reporting of study estimates
4.1. use appropriate levels of precision.
Reporting a p value of 0.7345 suggests that there is an appreciable difference between p values of 0.7344 and 0.7346. Reporting that 16.9% of 83 patients responded entails a precision (to the nearest 0.1%) that is nearly 200 times greater than the width of the confidence interval (10–27%). Reporting in a clinical study that the mean calorie consumption was 2069.9 suggest that calorie consumption can be measured extremely precisely by a food questionnaire. Some might argue that being overly precise is irrelevant, because the extra numbers can always be ignored. The counterargument is that investigators should think very hard about every number they report, rather than just carelessly cutting and pasting numbers from the statistical software printout. The specific guidelines for precision are as follows:
- Report p values to a single significant figure unless the p value is close to 0.05, in which case, report two significant figures. Do not report “not significant” for p values of 0.05 or higher. Very low p values can be reported as p < 0.001 or similar. A p value can indeed be 1, although some investigators prefer to report this as >0.9. For instance, the following p values are reported to appropriate precision: <0.001, 0.004, 0.045, 0.13, 0.3, 1.
- Report percentages, rates, and probabilities to two significant figures, for example, 75%, 3.4%, 0.13%.
- Do not report p values of 0, as any experimental result has a nonzero probability.
- Do not give decimal places if a probability or proportion is 1 (eg, a p value of 1.00 or a percentage of 100.00%). The decimal places suggest that it is possible to have, say, a p value of 1.05. There is a similar consideration for data that can take only integer values. It makes sense to state that, for instance, the mean number of pregnancies was 2.4, but not that 29% of women reported 1.0 pregnancy.
- There is generally no need to report estimates to more than three significant figures.
- Hazard and odds ratios are normally reported to two decimal places, although this can be avoided for high odds ratios (eg, 18.2 rather than 18.17).
4.2. Avoid redundant statistics in cohort descriptions
Authors should be selective about the descriptive statistics reported, and ensure that each and every number provides unique information. Authors should avoid reporting descriptive statistics that can readily be derived from the data that have already been provided. For instance, there is no need to state that in a cohort, 40% were men and 60% were women; choose one or the other. Another common error is to include a column of descriptive statistics for two groups separately and then combine the whole cohort. If, say, the median age is 60 in group 1 and 62 in group 2, we do not need to be told that the median age in the cohort as a whole is close to 61.
4.3. For descriptive statistics, median and quartiles are preferred over means and standard deviations (or standard errors); range should be avoided
The median and quartiles provide all sorts of useful information; for instance, 50% of patients had values above the median or between the quartiles. The range gives the values of just two patients and so is generally uninformative of the data distribution.
4.4. Report estimates for the main study questions
A clinical study typically focuses on a limited number of scientific questions. Authors should generally provide an estimate for each of these questions. In a study comparing two groups, for instance, authors should give an estimate of the difference between groups, and avoid giving only data on each group separately or simply saying that the difference was or was not significant. In a study of a prognostic factor, authors should give an estimate of the strength of the prognostic factor, such as an odds ratio or a hazard ratio, as well as reporting a p value testing the null hypothesis of no association between the prognostic factor and outcome.
4.5. Report confidence intervals for the main estimates of interest
Authors should generally report a 95% CI around the estimates relating to the key research questions, but not other estimates given in a paper. For instance, in a study comparing two surgical techniques, the authors might report adverse event rates of 10% and 15%; however, the key estimate in this case is the difference between groups, so this estimate, 5%, should be reported along with a 95% CI (eg, 1–9%). Confidence intervals should not be reported for the estimates within each group (eg, adverse event rate in group A of 10%, 95% CI 7–13%). Similarly, confidence intervals should not be given for statistics such as mean age or gender ratio.
4.6. Do not treat categorical variables as continuous
Variables such as Gleason grade groups are scored 1–5, but it is not true that the difference between groups 3 and 4 is half as great as the difference between groups 2 and 4. Variables such as Gleason grade groups should be reported as categories (eg, 40% grade group 1, 20% group 2, 20% group 3, 20% groups 4 and 5) rather than as a continuous variable (eg, mean Gleason score of 2.4). Similarly, categorical variables such as Gleason should be entered into regression models not as a single variable (eg, a hazard ratio of 1.5 per 1-point increase in Gleason grade group) but as multiple categories (eg, a hazard ratio of 1.6 comparing Gleason grade group 2 with group 1 and a hazard ratio of 3.9 comparing group 3 to group 1).
4.7. Avoid categorization of continuous variables unless there is a convincing rationale
A common approach to a variable such as age is to define patients as either old (aged ≥60 yr) or young (aged <60 yr) and then enter age into analyses as a categorical variable, reporting, for example, that “patients aged 60 and over had twice the risk of an operative complication than patients aged less than 60”. In epidemiologic and marker studies, a common approach is to divide a variable into quartiles and report a statistic such as a hazard ratio for each quartile compared with the lowest (“reference”) quartile. This is problematic because it assumes that all values of a variable within a category are the same. For instance, it is likely not the case that a patient aged 65 yr has the same risk as a patient aged 90 yr, but a very different risk from that of a patient aged 64 yr. It is generally preferable to leave variables in a continuous form, reporting, for instance, how risk changes with a 10-yr increase in age. Nonlinear terms can also be used, to avoid the assumption that the association between age and risk follows a straight line.
4.8. Do not use statistical methods to obtain cut-points for clinical practice
Various statistical methods are available to dichotomize a continuous variable. For instance, outcomes can be compared on either side of several different cut-points and the optimal cut-point chosen as the one associated with the smallest p value. Alternatively, investigators might choose a cut-point that leads to the highest value of sensitivity + specificity, that is, the point closest to the top left-hand corner of a receiver operating curve (ROC). Such methods are inappropriate for determining clinical cut-points because they do not consider clinical consequences. The ROC approach, for instance, assumes that sensitivity and specificity are of equal value, whereas it is generally worse to miss disease than to treat unnecessarily. The smallest p value approach tests strength of evidence against the null hypothesis, which has little to do with the relative benefits and harms of a treatment or further diagnostic workup.
4.9. The association between a continuous predictor and outcome can be demonstrated graphically, particularly by using nonlinear modeling
In high-school mathematics, we often thought about the relationship between y and x by plotting a line on a graph, with a scatterplot added in some cases. This also holds true for many scientific studies. In the case of a study of age and complication rates, for instance, an investigator could plot age on the x axis against the risk of a complication on the y axis and show a regression line, perhaps with a 95% CI. Nonlinear modeling is often useful because it avoids assuming a linear relationship and allows the investigator to determine questions such as whether risk starts to increase disproportionately beyond a given age.
4.10. Do not ignore significant heterogeneity in meta-analyses
Informally speaking, heterogeneity statistics test whether variations between the results of different studies in a meta-analysis are consistent with chance or whether such variation reflects, at least in part, true differences between studies. If heterogeneity is present, authors need to do more than merely report the p value and focus on the random-effect estimate. Authors should investigate the sources of heterogeneity and try to determine the factors that lead to differences in study results, for example, by identifying common features of studies with similar findings or idiosyncratic aspects of studies with outlying results.
4.11. For time-to-event variables, report the number of events but not the proportion
Take the case of a study that reported the following: “of 60 patients accrued, 10 (17%) died.” Although it is important to report the number of events, patients entered the study at different times and were followed for different periods; hence, the reported proportion of 17% is meaningless. The standard statistical approach to time-to-event variables is to calculate probabilities, such as the risk of death being 60% by 5 yr or the median survival—the time at which the probability of survival first drops below 50%—being 52 mo.
4.12. For time-to-event analyses, report median follow-up for patients without the event or the number followed without an event at a given follow-up time
It is often useful to describe how long a cohort has been followed. To illustrate the appropriate methods of doing so, take the case of a cohort of 1000 pediatric cancer patients treated in 1970 and followed to 2010. If the cure rate was only 40%, median follow-up for all patients might only be a few years; however, the median follow-up for patients who survived was 40 yr. This latter statistic gives a much better impression of how long the cohort had been followed. Now assume that in 2009, a second cohort of 2000 patients was added to the study. The median follow-up for survivors will now be around a year, which is again misleading. An alternative would be to report a statistic such as “312 patients have been followed without an event for at least 35 years.”
4.13. For time-to-event analyses, describe when follow-up starts and when and how patients are censored
A common error is that investigators use a censoring date that leads to an overestimate of survival. For example, when assessing the metastasis-free survival, a patient without a record of metastasis should be censored on the date of the last time the patient was known to be free of metastasis (eg, negative bone scan, undetectable prostate-specific antigen [PSA]), and not at the date of last patient contact (which may not have involved assessment of metastasis). For overall survival, the date of last patient contact would be an acceptable censoring date because the patient was indeed known to be event free at that time. When assessing cause-specific endpoints, special consideration should be given to the cause of death. The endpoints “disease-specific survival” and “disease-free survival” have specific definitions, and require careful attention to methods. With disease-specific survival, authors need to consider carefully how to handle death due to other causes. One approach is to censor patients at the time of death, but this can lead to a bias in certain circumstances, such as when the predictor of interest is associated with other-cause death and the probability of other-cause death is moderate or high. A competing risk analysis is appropriate in these situations. With disease-free survival, both evidence of disease (eg, disease recurrence) and death from any cause are counted as events, and so censoring at the time of other-cause death is inappropriate. If investigators are specifically interested only in the former and wish to censor deaths from other causes, they should define their endpoint as “freedom from progression.”
4.14. For time-to-event analyses, avoid reporting mean follow-up or survival time, or estimates of survival in those who had the event
All three estimates are problematic in the context of censored data.
4.15. For time-to-event analyses, make sure that all predictors are known at time zero or consider alternative approaches such as a landmark analysis or time-dependent covariates
In many cases, variables of interest vary over time. As a simple example, imagine that we were interested in whether PSA velocity predicted time to progression in prostate cancer patients on active surveillance. The problem is that PSA is measured at various time points after diagnosis. Unless they were being careful, investigators might use time from diagnosis in a Kaplan-Meier or Cox regression, but use PSA velocity calculated on PSA values measured at 1- and 2-yr follow-up. As another example, investigators might determine whether response to chemotherapy predicts cancer survival, but measure survival from the time of the first dose, before response is known. It is obviously invalid to use information known only “after the clock starts.” There are two main approaches to this problem. A “landmark analysis” is often used when the variable of interest is generally known within a short and well-defined period of time, such as adjuvant therapy or chemotherapy response. In brief, the investigators start the clock at a fixed “landmark” (eg, 6 mo after surgery). Patients are eligible only if they are still at risk at the landmark (eg, patients who recur before 6 mo are excluded) and the status of the variable is fixed at that time (eg, a patient who receives chemotherapy at 7 mo is defined as being in the no adjuvant group). Alternatively, investigators can use a time-dependent variable approach. In brief, this “resets the clock” each time new information is available about a variable. This would be the approach most typically used for the PSA velocity and progression example.
4.16. When presenting Kaplan-Meier figures, present the number at risk and truncate follow-up when numbers are low
Giving the number of risk is useful for helping to understand when patients were censored. When presenting Kaplan-Meier figures, a good rule of thumb is to truncate follow-up when the number at risk in any group falls below 5 (or even 10) as the tail of a Kaplan-Meier distribution is very unstable.
5. Multivariable models and diagnostic tests
5.1. multivariable, propensity, and instrumental variable analyses are not a magic wand.
Some investigators assume that multivariable adjustment “removes confounding,” “makes groups similar,” or “mimics a randomized trial.” There are two problems with such claims. First, the value of a variable recorded in a data set is often approximate and so may mask differences between groups. For instance, clinical stage might be used as a covariate in a study comparing treatments for localized prostate cancer. However, stage T2c might constitute a small nodule on each prostate lobe or, alternatively, most of the prostate consisting of a large, hard mass. The key point is that if one group has more T2c disease than the other, it is also likely that those with T2c disease in that group will fall toward the more aggressive end of the spectrum. Multivariable adjustment has the effect of making the rates of T2c in each group the same, but does not ensure that the type of T2c is identical. Second, a model adjusts for only a small number of measured covariates, which does not exclude the possibility of important differences in unmeasured (or even unmeasurable) covariates. A common assumption is that propensity methods somehow provide better adjustment for confounding than traditional multivariable methods. Except in certain rare circumstances, such as when the number of covariates is large relative to the number of events, propensity methods give extremely similar results to multivariable regression. Similarly, instrumental variables analyses depend on the availability of a good instrument, which is less common than is often assumed. In many cases, the instrument is not strongly associated with the intervention, leading to a large increase in the 95% CI or, in some cases, an underestimate of treatment effects.
5.2. Avoid stepwise selection
Investigators commonly choose which variables to include in a multivariable model by first determining which variables are statistically significant on univariable analysis; alternatively, they may include all variables in a single model and then remove those that are not significant. This type of data-dependent variable selection in regression models has several undesirable properties, increasing the risk of overfit and making many statistics, such as the 95% CI, highly questionable. The use of stepwise selection should be restricted to a limited number of circumstances, such as during the initial stages of developing a model, if there is poor knowledge of what variables might be predictive.
5.3. Avoid reporting estimates such as odds or hazard ratios for covariates when examining the effects of interventions
In a typical observational study, an investigator might explore the effects of two different approaches to radical prostatectomy on recurrence while adjusting for covariates such as stage, grade, and PSA. It is rarely worth reporting estimates such as odds or hazard ratios for the covariates. For instance, it is well known that a high Gleason score is strongly associated with recurrence: reporting a hazard ratio of, say, 4.23 is not helpful and is a distraction from the key finding—the hazard ratio between the two types of surgery.
5.4. Rescale predictors to obtain interpretable estimates
Predictors sometimes have a moderate association with outcome and can take a large range of values. This can lead to uninterpretable estimates. For instance, the odds ratio for cancer per year of age might be given as 1.02 (95% CI 1.01, 1.02; p < 0.0001). It is not helpful to have the upper bound of a confidence interval be equivalent to the central estimate; a better alternative would be to report an odds ratio per 10 yr of age. This is simply achieved by creating a new variable equal to age divided by 10 to obtain an odds ratio of 1.16 (95% CI 1.10, 1.22; p < 0.0001) per 10-yr difference in age.
5.5. Avoid reporting both univariate and multivariable analyses unless there is a good reason
Comparison of univariate and multivariable models can be of interest when trying to understand mechanisms. For instance, if race is a predictor of outcome on univariate analysis, but not after adjustment for income and access to care, one might conclude that poor outcome in African Americans is explained by socioeconomic factors. However, the routine reporting of estimates from both univariate and multivariable analysis is discouraged.
5.6. Avoid ranking predictors in terms of strength
It is tempting for authors to rank predictors in a model, claiming, for instance, that “the novel marker was the strongest predictor of recurrence.” Most commonly, this type of claim is based on comparisons of odds or hazard ratios. Such rankings are not meaningful since, among other reasons, it depends on how variables are coded. For instance, the odds ratio for hK2, and hence whether or not it is an apparently “stronger” predictor than PSA, will depend on whether it is entered in nanograms or picograms per milliliter. Further, it is unclear how one should compare model coefficients when both categorical and continuous variables are included. Finally, the prevalence of a categorical predictor also matters: a predictor with an odds ratio of 3.5 but a prevalence of 0.1% is less important than one with a prevalence of 50% and an odds ratio of 2.0.
5.7. Discrimination is a property not of a multivariable model but rather of the predictors and the data set
Although model building is generally seen as a process of fitting coefficients, discrimination is largely a property of which predictors are available. For instance, we have excellent models for prostate cancer outcome primarily because Gleason score is very strongly associated with malignant potential. In addition, discrimination is highly dependent on how much a predictor varies in the data set. As an example, a model to predict erectile dysfunction that includes age will have much higher discrimination for a population sample of adult men than for a group of older men presenting at a urology clinic because there is a greater variation in age in the population sample. Authors need to consider these points when drawing conclusions about the discrimination of models. This is also why authors should be cautious about comparing the discrimination of different multivariable models where these were assessed in different data sets.
5.8. Correction for overfit is strongly recommended for internal validation
In the same way that it is easy to predict last week’s weather, a prediction model generally has very good properties when evaluated on the same data set used to create the model. This problem is generally described as overfit. Various methods are available to correct for overfit, including cross validation and bootstrap resampling. Note that such methods should include all steps of model building. For instance, if an investigator uses stepwise methods to choose which predictors should go into the model and then fits the coefficients, a typical cross-validation approach would be to (1) split the data into 10 groups, (2) use stepwise methods to select predictors using the first nine groups, (3) fit coefficients using the first nine groups, (4) apply the model to the 10th group to obtain predicted probabilities, and (5) repeat steps 2–4 until all patients in the data set have a predicted probability derived from a model fitted to a data set that did not include that patient’s data. Statistics such as the area under the curve are then calculated using the predicted probabilities directly.
5.9. Calibration should be reported and interpreted correctly
Calibration is a critical component of a statistical model: the main concern for any patient is whether the risk given by a model is close to his or her true risk. It is rarely worth reporting calibration for a model created and tested on the same data set, even if techniques such as cross validation are used. This is because calibration is nearly always excellent on internal validation. Where a prespecified model is tested on an independent data set, calibration should be displayed graphically in a calibration plot. The Hosmer-Lemeshow test addresses an inappropriate null hypothesis and should be avoided. Note also that calibration depends on both the model coefficients and the data set being examined. A model cannot be inherently “well calibrated.” All that can be said is that predicted and observed risks are close in a specific data set, representative of a given population.
5.10. Avoid reporting sensitivity and specificity for continuous predictors or a model
Investigators often report sensitivity and specificity at a given cut-point for a continuous predictor (such as a PSA value of 10 ng/ml), or report specificity at a given sensitivity (such as 90%). Reporting sensitivity and specificity is not of value because it is unclear how high sensitivity or specificity would have to be in order to be high enough to justify clinical use. Similarly, it is very difficult to determine which of two tests, one with higher sensitivity and the other with higher specificity, is preferable because clinical value depends on the prevalence of disease and the relative harms of a false-positive result compared with a false-negative result. In the case of reporting specificities at fixed sensitivity, or vice versa, it is all but impossible to choose the specific sensitivity rationally. For instance, a team of investigators may state that they want to know specificity at 80% sensitivity, because they want to ensure that they catch 80% of cases. However, 80% might be too low if prevalence is high or too high if prevalence is low.
5.11. Report the clinical consequences of using a test or a model
In place of statistical abstractions such as sensitivity and specificity, or an ROC, authors are encouraged to choose illustrative cut-points and then report results in terms of clinical consequences. As an example, consider a study in which a marker is measured in a group of patients undergoing biopsy. Authors could report that if a given level of the marker had been used to determine biopsy, then a certain number of biopsies would have been conducted and a certain number of cancers found and missed.
5.12. Interpret decision curves with careful reference to threshold probabilities
It is insufficient merely to report that, for instance, “the marker model had highest net benefit for threshold probabilities of 35–65%.” Authors need to consider whether those threshold probabilities are rational. If the study reporting benefit between 35% and 65% concerned detection of high-grade prostate cancer, few, if any, urologists would demand that a patient have at least a one-in-three chance of high-grade disease before recommending biopsy. The authors would therefore need to conclude that the model was not of benefit.
6. Conclusions and interpretation
6.1. draw a conclusion, do not just repeat the results.
Conclusion sections are often simply a restatement of the results. For instance, “a statistically significant relationship was found between body mass index (BMI) and disease outcome” is not a conclusion. Authors instead need to state implications for research and/or clinical practice. For instance, a conclusion section might call for research to determine whether the association between BMI is causal or make a recommendation for more aggressive treatment of patients with a higher BMI.
6.2. Avoid using words such as “may” or “might”
A conclusion that a novel treatment “may” be of benefit would be untrue only if it had been proved that the treatment was ineffective. Indeed, that the treatment may help would have been the rationale for the study in the first place. Using words such as may in the conclusion is equivalent to stating, “we know no more at the end of this study than we knew at the beginning”—reason enough to reject a paper for publication.
6.3. A statistically significant p value does not imply clinical significance
A small p value means that only the null hypothesis has been rejected. This may or may not have implications for clinical practice. For instance, that a marker is a statistically significant predictor of outcome does not imply that treatment decisions should be made on the basis of that marker. Similarly, a statistically significant difference between two treatments does not necessarily mean that the former should be preferred to the latter. Authors need to justify any clinical recommendations by carefully analyzing the clinical implications of their findings.
6.4. Avoid pseudolimitations such as “small sample size” and “retrospective analysis”; consider instead sources of potential bias and the mechanism for their effect on findings
Authors commonly describe study limitations in a rather superficial way, such as “small sample size and retrospective analysis are limitations.” However, a small sample size may be immaterial if the results of the study are clear. For instance, if a treatment or predictor is associated with a very large odds ratio, a large sample size might be unnecessary. Similarly, a retrospective design might be entirely appropriate, as in the case of a marker study with very long-term follow-up, and have no discernible disadvantages compared with a prospective study. Discussion of limitations should include both the likelihood and the effect size of possible bias.
6.5. Consider the impact of missing data and patient selection
It is rare that complete data are obtained from all patients in a study. A typical paper might report, for instance, that of 200 patients, eight had data missing on important baseline variables and 34 did not complete the end-of-study questionnaire, leading to a final data set of 158. Similarly, many studies include a relatively narrow subset of patients, such as 50 patients referred for imaging before surgery out of the 500 treated surgically during that time frame. In both cases, it is worth considering analyses to investigate whether patients with missing data or who were not selected for treatment were different in some way from those who were included in the analyses. Although statistical adjustment for missing data is complex and warranted only in a limited set of circumstances, basic analyses to understand the characteristics of patients with missing data are relatively straightforward and are often helpful.
6.6. Consider the possibility and impact of ascertainment bias
Ascertainment bias occurs when an outcome depends on a test, and the propensity for a patient to be tested is associated with the predictor. PSA screening provides a classic example: prostate cancer is found by biopsy, but the main reason why men are biopsied is an elevated PSA. A study in a population subject to PSA screening will, therefore, overestimate the association between PSA and prostate cancer. Ascertainment bias can also be caused by the timing of assessments. For instance, the frequency of biopsy in prostate cancer active surveillance will depend on prior biopsy results and PSA level, and this induces an association between those predictors and time to progression.
6.7. Do not confuse outcome with response among subgroups of patients undergoing the same treatment: patients with poorer outcomes may still be good candidates for that treatment
Investigators often compare outcomes in different subgroups of patients, all receiving the same treatment. A common error is to conclude that patients with poor outcome are not good candidates for that treatment and should receive an alternative approach. This conclusion confuses differences between patients for differences between treatments. As a simple example, patients with large tumors are more likely to recur after surgery than patients with small tumors, but that cannot be taken to suggest that resection is not indicated for patients with tumors greater than a certain size. Indeed, surgery is generally more strongly indicated for patients with aggressive (but localized) disease, and such patients are unlikely to do well on surveillance.
6.8. Be cautious about causal attribution: correlation does not imply causation
It is well known that “correlation does not imply causation,” but authors often slip into this error in making conclusions. The Introduction and Methods sections might insist that the purpose of the study is merely to determine whether there is an association between, say, treatment frequency and treatment response, but the conclusions may imply that, for instance, more frequent treatment would improve response rates.
7. Use and interpretation of p values
It is apparent from even the most cursory reading of the medical literature that p values are widely misused and misunderstood. One of the most common errors is accepting the null hypothesis, for instance, concluding from a p value of 0.07 that a drug is ineffective or that two surgical techniques are equivalent. This particular error is described in detail in guideline 3.1. The more general problem, which we address here, is that p values are often given excessive weight in the interpretation of a study. Indeed, studies are often classed by investigators into “positive” or “negative” based on statistical significance. Gross misuse of p values has led some to advocate banning the use of p values completely [ 4 ].
We follow the American Statistical Association statement on p values and encourage all researchers to read either the full statement [ 5 ] or the summary [ 6 ]. In particular, we emphasize that p value is just one statistic that helps interpret a study; it does not determine our interpretations. Drawing conclusions for research or clinical practice from a clinical research study requires evaluation of the strengths and weaknesses of study methodology, results of other pertinent data published in the literature, biological plausibility, and effect size. Sound and nuanced scientific judgment cannot be replaced by just checking whether one of the many statistics in a paper is or is not <0.05.
8. Concluding remarks
These guidelines are not intended to cover all medical statistics but rather the statistical approaches most commonly used in clinical research papers in urology. It is quite possible for a paper to follow all the guidelines and yet be statistically flawed, or to break numerous guidelines and still be statistically sound. On balance, however, the analysis, reporting, and interpretation of clinical urologic research will be improved by adherence to these guidelines.
Acknowledgments
Funding/Support and role of the sponsor : This work was supported in part by the Sidney Kimmel Center for Prostate and Urologic Cancers, P50-CA92629 SPORE grant from the National Cancer Institute to Dr. H. Scher, and the P30-CA008748 NIH/NCI Cancer Center Support Grant to Memorial Sloan-Kettering Cancer Center.
Financial disclosures: Andrew J. Vickers certifies that all conflicts of interest, including specific financial interests and relationships and affiliations relevant to the subject matter or materials discussed in the manuscript (eg, employment/affiliation, grants or funding, consultancies, honoraria, stock ownership or options, expert testimony, royalties, or patents filed, received, or pending), are the following: None.
Publisher's Disclaimer: This is a PDF file of an unedited manuscript that has been accepted for publication. As a service to our customers we are providing this early version of the manuscript. The manuscript will undergo copyediting, typesetting, and review of the resulting proof before it is published in its final citable form. Please note that during the production process errors may be discovered which could affect the content, and all legal disclaimers that apply to the journal pertain.
- Alzheimer's disease & dementia
- Arthritis & Rheumatism
- Attention deficit disorders
- Autism spectrum disorders
- Biomedical technology
- Diseases, Conditions, Syndromes
- Endocrinology & Metabolism
- Gastroenterology
- Gerontology & Geriatrics
- Health informatics
- Inflammatory disorders
- Medical economics
- Medical research
- Medications
- Neuroscience
- Obstetrics & gynaecology
- Oncology & Cancer
- Ophthalmology
- Overweight & Obesity
- Parkinson's & Movement disorders
- Psychology & Psychiatry
- Radiology & Imaging
- Sleep disorders
- Sports medicine & Kinesiology
- Vaccination
- Breast cancer
- Cardiovascular disease
- Chronic obstructive pulmonary disease
- Colon cancer
- Coronary artery disease
- Heart attack
- Heart disease
- High blood pressure
- Kidney disease
- Lung cancer
- Multiple sclerosis
- Myocardial infarction
- Ovarian cancer
- Post traumatic stress disorder
- Rheumatoid arthritis
- Schizophrenia
- Skin cancer
- Type 2 diabetes
- Full List »
share this!
April 16, 2024
This article has been reviewed according to Science X's editorial process and policies . Editors have highlighted the following attributes while ensuring the content's credibility:
fact-checked
peer-reviewed publication
trusted source
New guidelines reflect growing use of AI in health care research
by NDORMS, University of Oxford
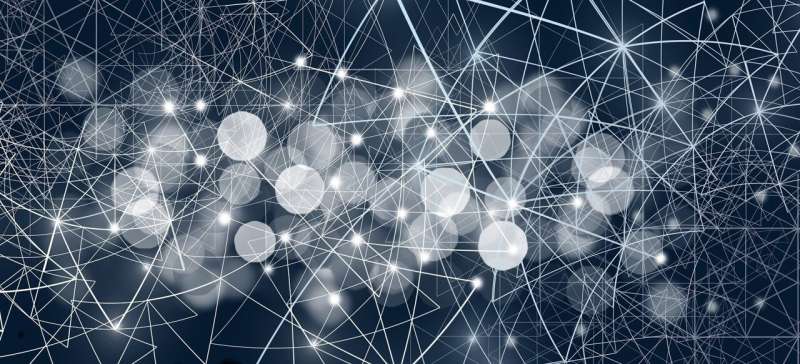
The widespread use of artificial intelligence (AI) in medical decision-making tools has led to an update of the TRIPOD guidelines for reporting clinical prediction models. The new TRIPOD+AI guidelines are launched in the BMJ today.
The TRIPOD guidelines (which stands for Transparent Reporting of a Multivariable Prediction Model for Individual Prognosis Or Diagnosis) were developed in 2015 to improve tools to aid diagnosis and prognosis that are used by doctors. Widely used, their uptake by medical practitioners to estimate the probability that a specific condition is present or may occur in the future, has helped improve transparency and accuracy of decision-making and significantly improve patient care.
But research methods have moved on since 2015, and we are witnessing an acceleration of studies that are developing prediction models using AI, specifically machine learning methods. Transparency is one of the six core principles underpinning the WHO guidance on ethics and governance of artificial intelligence for health. TRIPOD+AI has therefore been developed to provide a framework and set of reporting standards to boost reporting of studies developing and evaluating AI prediction models regardless of the modeling approach.
The TRIPOD+AI guidelines were developed by a consortium of international investigators, led by researchers from the University of Oxford alongside researchers from other leading institutions across the world, health care professionals , industry, regulators, and journal editors. The development of the new guidance was informed by research highlighting poor and incomplete reporting of AI studies, a Delphi survey, and an online consensus meeting.
Gary Collins, Professor of Medical Statistics at the Nuffield Department of Orthopaedics, Rheumatology and Musculoskeletal Sciences (NDORMS), University of Oxford, and lead researcher in TRIPOD, says, "There is enormous potential for artificial intelligence to improve health care from earlier diagnosis of patients with lung cancer to identifying people at increased risk of heart attacks. We're only just starting to see how this technology can be used to improve patient outcomes.
"Deciding whether to adopt these tools is predicated on transparent reporting. Transparency enables errors to be identified, facilitates appraisal of methods and ensures effective oversight and regulation. Transparency can also create more trust and influence patient and public acceptability of the use of prediction models in health care."
The TRIPOD+AI statement consists of a 27-item checklist that supersedes TRIPOD 2015. The checklist details reporting recommendations for each item and is designed to help researchers, peer reviewers, editors, policymakers and patients understand and evaluate the quality of the study methods and findings of AI-driven research.
A key change in TRIPOD+AI has been an increased emphasis on trustworthiness and fairness. Prof. Carl Moons, UMC Utrecht said, "While these are not new concepts in prediction modeling, AI has drawn more attention to these as reporting issues. A reason for this is that many AI algorithms are developed on very specific data sets that are sometimes not even from studies or could simply be drawn from the internet.
"We also don't know which groups or subgroups were included. So to ensure that studies do not discriminate against any particular group or create inequalities in health care provision, and to ensure decision-makers can trust the source of the data, these factors become more important."
Dr. Xiaoxuan Liu and Prof Alastair Denniston, Directors of the NIHR Incubator for Regulatory Science in AI & Digital Health care are co-authors of TRIPOD+AI explained, "Many of the most important applications of AI in medicine are based on prediction models. We were delighted to support the development of TRIPOD+AI which is designed to improve the quality of evidence in this important area of AI research."
TRIPOD 2015 helped change the landscape of clinical research reporting bringing minimum reporting standards to prediction models. The original guidelines have been cited over 7500 times, featured in multiple journal instructions to authors, and been included in WHO and NICE briefing documents.
"I hope the TRIPOD+AI will lead to a marked improvement in reporting, reduce waste from incompletely reported research and enable stakeholders to arrive at an informed judgment based on full details on the potential of the AI technology to improve patient care and outcomes that cut through the hype in AI-driven health care innovations," concluded Gary.
Explore further
Feedback to editors

Multidisciplinary research team creates computational models to predict heart valve leakage in children
3 minutes ago

Research uncovers new reasons to target neutrophils for tuberculosis therapy
24 minutes ago

New treatment method using plasma irradiation promotes faster bone healing

Good blood pressure control could prevent fibroids

Study suggests the brain's reward system works to make others happy, not just ourselves
2 hours ago

Researchers discover cause of rare congenital lung malformations

An effective drug delivery system for next-generation treatments to hitch a ride in cancer cells

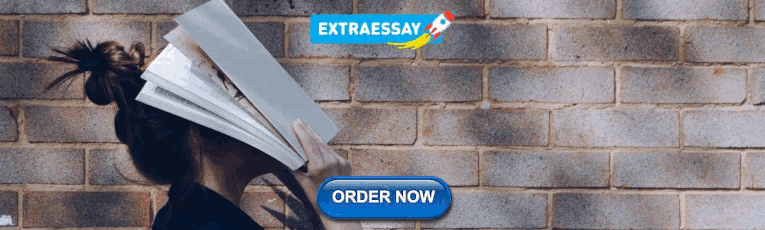
Study on rats shows a junk food diet can cause long-term damage to adolescent brains

Mouse study finds small extracellular vesicles from young blood extend lifespan and restore physiological functions

From opioid overdose to treatment initiation: Outcomes associated with peer support in emergency departments
3 hours ago
Related Stories

Experts establish checklist detailing key consensus reporting items for primary care studies
Nov 28, 2023

A new standard for reporting epidemic prediction research
Oct 19, 2021

Urology treatment studies show increased reporting of harmful effects
Dec 21, 2023

New reporting guidelines developed to improve AI in health care settings
May 19, 2022

New guidelines to improve reporting standards of studies that investigate causal mechanisms
Sep 21, 2021

New guidelines for reporting clinical trials of biofield therapies released
Feb 8, 2024
Recommended for you

How AI improves physician and nurse collaboration to boost patient care
5 hours ago

GPT-4 matches radiologists in detecting errors in radiology reports

Study reveals AI enhances physician-patient communication
23 hours ago

Study shows AI improves accuracy of skin cancer diagnoses
Apr 12, 2024

New AI method captures uncertainty in medical images
Apr 11, 2024

Decoding spontaneous thoughts from the brain via machine learning
Let us know if there is a problem with our content.
Use this form if you have come across a typo, inaccuracy or would like to send an edit request for the content on this page. For general inquiries, please use our contact form . For general feedback, use the public comments section below (please adhere to guidelines ).
Please select the most appropriate category to facilitate processing of your request
Thank you for taking time to provide your feedback to the editors.
Your feedback is important to us. However, we do not guarantee individual replies due to the high volume of messages.
E-mail the story
Your email address is used only to let the recipient know who sent the email. Neither your address nor the recipient's address will be used for any other purpose. The information you enter will appear in your e-mail message and is not retained by Medical Xpress in any form.
Newsletter sign up
Get weekly and/or daily updates delivered to your inbox. You can unsubscribe at any time and we'll never share your details to third parties.
More information Privacy policy
Donate and enjoy an ad-free experience
We keep our content available to everyone. Consider supporting Science X's mission by getting a premium account.
E-mail newsletter
Europe PMC requires Javascript to function effectively.
Either your web browser doesn't support Javascript or it is currently turned off. In the latter case, please turn on Javascript support in your web browser and reload this page.
Search life-sciences literature (43,920,221 articles, preprints and more)
- Free full text
- Citations & impact
- Similar Articles
Guidelines for reporting of statistics for clinical research in urology.
Author information, affiliations.
- Sjoberg D 1
- Vickers AJ 1
- Botchway A 5
- Delfino K 5
ORCIDs linked to this article
- Zahnd W | 0000-0001-5174-8666
- Vickers AJ | 0000-0003-1525-6503
- | 0000-0003-4144-8314
- Elders A | 0000-0003-4172-4702
BJU International , 20 Jan 2019 , 123(3): 401-410 https://doi.org/10.1111/bju.14640 PMID: 30537407 PMCID: PMC6397060
Free full text in Europe PMC
Abstract
Free full text .

Guidelines for reporting of statistics for clinical research in urology
Melissa assel.
1 Memorial Sloan Kettering Cancer Center
Daniel Sjoberg
Mr. andrew elders.
2 Glasgow Caledonian University
Xuemei Wang
3 The University of Texas, MD Anderson Cancer Center
Dezheng Huo
4 The University of Chicago
Albert Botchway
5 Southern Illinois University School of Medicine
Kristin Delfino
6 University of Minnesota
Zhiguo Zhao
7 Cleveland Clinic
Tatsuki Koyama
8 Vanderbilt University Medical Center
Brent Hollenbeck
9 University of Michigan
10 Janssen Research & Development
Whitney Zahnd
11 University of South Carolina
Emily C. Zabor
Michael w. kattan, dr. andrew j. vickers.
In an effort to improve the quality of statistics in the clinical urology literature, statisticians at European Urology, The Journal of Urology, Urology and BJUI came together to develop a set of guidelines to address common errors of statistical analysis, reporting and interpretation. Authors should “break any of the guidelines if it makes scientific sense to do so” but would need to provide a clear justification. Adoption of the guidelines will in our view not only increase the quality of published papers in our journals but improve statistical knowledge in our field in general.
It is widely acknowledged that the quality of statistics in the clinical research literature is poor. This is true for urology just as it is for other medical specialties. In 2005, Scales et al. published a systematic evaluation of the statistics in papers appearing in a single month in one of the four leading urology medical journals: European Urology, The Journal of Urology, Urology and BJUI. They reported widespread errors, including 71% of papers with comparative statistics having at least one statistical flaw[ 1 ]. These findings mirror many others in the literature, see, for instance, the review given by Lang and Altman[ 2 ]. The quality of statistical reporting in urology journals has no doubt improved since 2005, but remains unsatisfactory.
The four urology journals in the Scales et al. review have come together to publish a shared set of statistical guidelines, adapted from those in use at one of the journals, European Urology, since 2014[ 3 ]. The guidelines will also be adopted by European Urology Focus and European Urology Oncology. Statistical reviewers at the four journals will systematically assess submitted manuscripts using the guidelines to improve statistical analysis, reporting and interpretation. Adoption of the guidelines will, in our view, not only increase the quality of published papers in our journals but improve statistical knowledge in our field in general. Asking an author to follow a guideline about, say, the fallacy of accepting the null hypothesis, would no doubt result in a better paper, but we hope that it would also enhance the author’s understanding of hypothesis tests.
The guidelines are didactic, based on the consensus of the statistical consultants to the journals. We avoided, where possible, making specific analytic recommendations and focused instead on analyses or methods of reporting statistics that should be avoided. We intend to update the guidelines over time and hence encourage readers who question the value or rationale of a guideline to write to the authors.
- 1. The golden rule: Break any of the guidelines if it makes scientific sense to do so.
Science varies too much to allow methodologic or reporting guidelines to apply universally.
- 2. Reporting of design and statistical analysis
2.1. Follow existing reporting guidelines for the type of study you are reporting, such as CONSORT for randomized trials, ReMARK for marker studies, TRIPOD for prediction models, STROBE for observational studies, or AMSTAR for systematic reviews.
Statisticians and methodologists have contributed extensively to a large number of reporting guidelines. The first is widely recognized to be the Consolidated Standards of Reporting Trials (CONSORT) statement on the reporting of randomized trials, but there are now many other guidelines, covering a wide range of different types of study. Reporting guidelines can be downloaded from the Equator Web site ( http://www.equator-network.org ).
2.2. Describe cohort selection fully.
It is insufficient to state, for instance, “the study cohort consisted of 1144 patients treated for benign prostatic hyperplasia at our institution”. The cohort needs to be defined in terms of dates (e.g. “presenting March 2013 to December 2017”), inclusion criteria (e.g. “IPSS > 12”) and whether patients were selected to be included (e.g. for a research study) vs. being a consecutive series. Exclusions should be described one by one, with the number of patients omitted for each exclusion criterion to give the final cohort size (e.g. “patients with prior surgery (n=43), allergies to 5-ARIs (n=12) and missing data on baseline prostate volume (n=86) were excluded to give a final cohort for analysis of 1003 patients”). Note that inclusion criteria can be omitted if obvious from context (e.g. no need to state “undergoing radical prostatectomy for histologically proven prostate cancer”); on the other hand, dates may need to be explained if their rationale could be questioned (e.g. “March 2013, when our specialist voiding clinic was established to December 2017”).
2.3. Describe the practical steps of randomization in randomized trials.
Although this reporting guideline is part of the CONSORT statement, it is so critical and so widely misunderstood that it bears repeating. The purpose of randomization is to prevent selection bias. This can be achieved only if those consenting patients cannot guess a patient’s treatment allocation before registration in the trial or change it afterward. This safeguard is known as allocation concealment . Stating merely that “a randomization list was created by a statistician” or that “envelope randomization was used” does not ensure allocation concealment: a list could have been posted in the nurse’s station for all to see; envelopes can be opened and resealed. Investigators need to specify the exact logistic steps taken to ensure allocation concealment. The best method is to use a password-protected computer database.
2.4. The statistical methods should describe the study questions and the statistical approaches used to address each question.
Many statistical methods sections state only something like “Mann-Whitney was used for comparisons of continuous variables and Fisher’s exact for comparisons of binary variables”. This says little more than “the inference tests used were not grossly erroneous for the type of data”. Instead, statistical methods sections should lay out each primary study question separately: carefully detail the analysis associated with each and describe the rationale for the analytic approach, where this is not obvious or if there are reasonable alternatives. Special attention and description should be provided for rarely used statistical techniques.
2.5. The statistical methods should be described in sufficient detail to allow replication by an independent statistician given the same data set.
Vague reference to “adjusting for confounders” or “non-linear approaches” is insufficiently specific to allow replication, a cornerstone of the scientific method. All statistical analyses should be specified in the Methods section, including details such as the covariates included in a multivariable model. All variables should be clearly defined where there is room for ambiguity. For instance, avoid saying that “Gleason grade was included in the model”; state instead “Gleason grade group was included in four categories 1, 2, 3 and 4 or 5”.
- 3. Inference and p-values (see also “Use and interpretation of p-values” below)
3.1. Don’t accept the null hypothesis.
In a court case, defendants are declared guilty or not guilty, there is no verdict of “innocent”. Similarly, in a statistical test, the null hypothesis is rejected or not rejected. If the p-value is 0.05 or more, investigators should avoid conclusions such as “the drug was ineffective”, “there was no difference between groups” or “response rates were unaffected”. Instead, authors should use phrases such as “we did not see evidence of a drug effect”, “we were unable to demonstrate a difference between groups” or simply “there was no statistically significant difference in response rates”.
3.2. P-values just above 5% are not a trend, and they are not moving.
Avoid saying that a p-value such as 0.07 shows a “trend” (which is meaningless) or “approaches statistical significance” (because the p-value isn’t moving). Alternative language might be: “although we saw some evidence of improved response rates in patients receiving the novel procedure, differences between groups did not meet conventional levels of statistical significance”.
3.3. P-values and 95% confidence intervals do not quantify the probability of a hypothesis.
A p-value of, say, 0.03 does not mean that there is 3% probability that the findings are due to chance. Additionally, a 95% confidence interval should not be interpreted as a 95% certainty the true parameter value is in the range of the 95% confidence interval. The correct interpretation of a p-value is the probability of finding the observed or more extreme results when the null hypothesis is true; the 95% confidence interval will contain the true parameter value 95% of the time were a study to be repeated many times using different samples.
3.4. Don’t use confidence intervals to test hypotheses.
Investigators often interpret confidence intervals in terms of hypotheses. For instance, investigators might claim that there is a statistically significant difference between groups because the 95% confidence interval for the odds ratio excludes 1. Such claims are problematic because confidence intervals are concerned with estimation, not inference. Moreover, the mathematical method to calculate confidence intervals may be different from those used to calculate p-values. It is perfectly possible to have a 95% confidence interval that includes no difference between groups even though the p-value is less than 0.05 or vice versa . For instance, in a study of 100 patients in two equal groups, with event rates of 70% and 50%, the p-value from Fisher’s exact test is 0.066 but the 95% C.I. for the odds ratio is 1.03 to 5.26. The 95% C.I. for the risk difference and risk ratio also exclude no difference between groups.
3.5. Take care interpreting results when reporting multiple p-values.
The more questions you ask, the more likely you are to get a spurious answer to at least one of them. For example, if you report p-values for five independent true null hypotheses, the probability that you will falsely reject at least one is not 5%, but >20%. Although formal adjustment of p-values is appropriate in some specific cases, such as genomic studies, a more common approach is simply to interpret p-values in the context of multiple testing. For instance, if an investigator examines the association of 10 variables with three different endpoints, thereby testing 30 separate hypotheses, a p-value of 0.04 should not be interpreted in the same way as if study tested only a single hypothesis with a p-value of 0.04.
3.6. Do not report separate p-values for each of two different groups in order to address the question of whether there is a difference between groups.
One scientific question means one statistical hypothesis tested by one p-value. To illustrate the error of using two p-values to address one question, take the case of a randomized trial of drug versus placebo to reduce voiding symptoms, with 30 patients in each group. The authors might report that symptom scores improved by 6 (standard deviation 14) points in the drug group (p=0.03 by one-sample t-test) and 5 (standard deviation 15) points in the placebo group (p=0.08). However, the study hypothesis concerns the difference between drug and placebo. To test a single hypothesis, a single p-value is needed. A two-sample t-test for these data gives a p-value for 0.8 – unsurprising, given that the scores in each group were virtually the same - confirming that it would be unsound to conclude that the drug was effective based on the finding that change was significant in the drug group but not in placebo controls.
3.7. Use interaction terms in place of subgroup analyses.
A similar error to the use of separate tests for a single hypothesis is when an intervention is shown to have a statistically significant effect in one group of patients but not another. One approach that is more appropriate is to use what is known as an interaction term in a statistical model. For instance, to determine whether a drug reduced pain scores more in women than men, the model might be as follows:
It is sometimes appropriate to report estimates and confidence intervals within subgroups of interest, but p-values should be avoided.
3.8. Tests for change over time are generally uninteresting.
A common analysis is to conduct a paired t-test comparing, say, erectile function in older men at baseline with erectile function after 5 years of follow-up. The null hypothesis here is that “erectile function does not change over time”, which is known to be false. Investigators are encouraged to focus on estimation rather than inference, reporting, for example, the mean change over time along with a 95% confidence interval.
3.9. Avoid using statistical tests to determine the type of analysis to be conducted.
Numerous statistical tests are available that can be used to determine how a hypothesis test should be conducted. For instance, investigators might conduct a Shapiro-Wilk test for normality to determine whether to use a t-test or Mann-Whitney, Cochran’s Q to decide whether to use a fixed- or random-effects approach in a meta-analysis or use a t-test for between-group differences in a covariate to determine whether that covariate should be included a multivariable model. The problem with these sorts of approaches is that they are often testing a null hypothesis that is known to be false. For instance, no data set perfectly follows a normal distribution. Moreover, it is often questionable that changing the statistical approach in the light of the test is actually of benefit. Statisticians are far from unanimous as to whether Mann-Whitney is always superior to t-test when data are non-normal, or that fixed effects are invalid under study heterogeneity, or that the criterion of adjusting for a variable should be whether it is significantly different between groups. Investigators should generally follow a prespecified analytic plan, only altering the analysis if the data unambiguously point to a better alternative.
3.10. When reporting p-values, be clear about the hypothesis tested and ensure that the hypothesis is a sensible one.
P-values test very specific hypotheses. When reporting a p-value in the results section, state the hypothesis being tested unless this is completely clear. Take, for instance, the statement “Pain scores were higher in group 1 and similar in groups 2 and 3 (p=0.02)”. It is ambiguous whether the p-value of 0.02 is testing group 1 vs. groups 2 and 3 combined or the hypothesis that pain score is the same in all three groups. Clarity about the hypotheses being tested can help avoid the testing of inappropriate hypotheses. For instance, p-values for differences between groups at baseline in a randomized trial is testing a null hypothesis that is known to be true (informally, that any observed differences between groups are due to chance).
- 4. Reporting of study estimates
4.1. Use appropriate levels of precision.
Reporting a p-value of 0.7345 suggests that there is an appreciable difference between p-values of 0.7344 and 0.7346. Reporting that 16.9% of 83 patients responded entails a precision (to the nearest 0.1%) that is nearly 200 times greater than the width of the confidence interval (10% to 27%). Reporting in a clinical study that the mean calorie consumption was 2069.9 suggests that calorie consumption can be measured extremely precisely by a food questionnaire. Some might argue that being overly precise is irrelevant, because the extra numbers can always be ignored. The counter-argument is that investigators should think very hard about every number they report, rather than just carelessly cutting and pasting numbers from the statistical software printout. The specific guidelines for precision are as follows:
Report p-values to a single significant figure unless the p is close to 0.05, in which case, report two significant figures. Do not report “NS” for p-values of 0.05 or above. Very low p-values can be reported as p<0.001 or similar. A p-value can indeed be 1, although some investigators prefer to report this as >0.9. For instance, the following p-values are reported to appropriate precision: <0.001, 0.004, 0.045, 0.13, 0.3, 1.
Report percentages, rates and probabilities to 2 significant figures, e.g. 75%, 3.4%, 0.13%.
Do not report p-values of zero, as any experimental result has a non-zero probability.
Do not give decimal places if a probability or proportion is 1 (e.g. a p-value of 1.00 or a percentage of 100.00%). The decimal places suggest it is possible to have, say, a p-value 1.05. There is a similar consideration for data that can only take integer values. It makes sense to state that, for instance, the mean number of pregnancies was 2.4, but not that 29% of women reported 1.0 pregnancies.
There is generally no need to report estimates to more than three significant figures.
Hazard and odds ratios are normally reported to two decimal places, although this can be avoided for high odds ratios (e.g. 18.2 rather than 18.17).
4.2. Avoid redundant statistics in cohort descriptions.
Authors should be selective about the descriptive statistics reported and ensure that each and every number provides unique information. Authors should avoid reporting descriptive statistics that can be readily derived from data that have already been provided. For instance, there is no need to state 40% of a cohort were men and 60% were women, choose one or the other. Another common error is to include a column of descriptive statistics for two groups separately and then the whole cohort combined. If, say, the median age is 60 in group 1 and 62 in group 2, we do not need to be told that the median age in the cohort as a whole is close to 61.
4.3. For descriptive statistics, median and quartiles are preferred over means and standard deviations (or standard errors); range should be avoided.
The median and quartiles provide all sorts of useful information, for instance, that 50% of patients had values above the median or between the quartiles. The range gives the values of just two patients and so is generally uninformative of the data distribution.
4.4. Report estimates for the main study questions.
A clinical study typically focuses on a limited number of scientific questions. Authors should generally provide an estimate for each of these questions. In a study comparing two groups, for instance, authors should give an estimate of the difference between groups, and avoid giving only data on each group separately or, simply saying that the difference was or was not significant. In a study of a prognostic factor, authors should give an estimate of the strength of the prognostic factor, such as an odds ratio or hazard ratio, as well as reporting a p-value testing the null hypothesis of no association between the prognostic factor and outcome.
4.5. Report confidence intervals for the main estimates of interest.
Authors should generally report a 95% confidence interval around the estimates relating to the key research questions, but not other estimates given in a paper. For instance, in a study comparing two surgical techniques, the authors might report adverse event rates of 10% and 15%; however, the key estimate in this case is the difference between groups, so this estimate, 5%, should be reported along with a 95% confidence interval (e.g. 1% to 9%). Confidence intervals should not be reported for the estimates within each group (e.g. adverse event rate in group A of 10%, 95% CI 7% to 13%). Similarly, confidence intervals should not be given for statistics such as mean age or gender ratio.
4.6. Do not treat categorical variables as continuous.
A variable such as Gleason grade groups are scored 1 – 5, but it is not true that the difference between group 3 and 4 is half as great as the difference between group 2 and 4. Variables such as Gleason grade group should be reported as categories (e.g. 40% grade group 1, 20% group 2, 20% group 3, 20% group 4 and 5) rather than as a continuous variable (e.g. mean Gleason score of 2.4). Similarly, categorical variables such as Gleason should be entered into regression models not as a single variable (e.g. a hazard ratio of 1.5 per 1-point increase in Gleason grade group) but as multiple categories (e.g. hazard ratio of 1.6 comparing Gleason grade group 2 to group 1 and hazard ratio of 3.9 comparing group 3 to group 1).
4.7. Avoid categorization of continuous variables unless there is a convincing rationale.
A common approach to a variable such as age is to define patients as either old (≥ 60) or young (<60) and then enter age into analyses as a categorical variable, reporting, for example, that “patients aged 60 and over had twice the risk of an operative complication than patients aged less than 60”. In epidemiologic and marker studies, a common approach is to divide a variable into quartiles and report a statistic such as a hazard ratio for each quartile compared to the lowest (“reference”) quartile. This is problematic because it assumes that all values of a variable within a category are the same. For instance, it is likely not the case that a patient aged 65 has the same risk as a patient aged 90, but a very different risk to a patient aged 64. It is generally preferable to leave variables in a continuous form, reporting, for instance, how risk changes with a 10-year increase in age. Non-linear terms can also be used, to avoid the assumption that the association between age and risk follows a straight line.
4.8. Do not use statistical methods to obtain cut-points for clinical practice.
There are various statistical methods available to dichotomize a continuous variable. For instance, outcomes can be compared either side of several different cut-points, and the optimal cut-point chosen as the one associated with the smallest p-value. Alternatively, investigators might choose a cut-point that leads to the highest value of sensitivity + specificity, that is, the point closest to the top left-hand corner of a Receiver Operating Curve (ROC). Such methods are inappropriate for determining clinical cut-points because they do not consider clinical consequences. The ROC curve approach, for instance, assumes that sensitivity and specificity are of equal value, whereas it is generally worse to miss disease than to treat unnecessarily. The smallest p-value approach tests strength of evidence against the null hypothesis, which has little to do with the relative benefits and harms of a treatment or further diagnostic work up.
4.9. The association between a continuous predictor and outcome can be demonstrated graphically, particularly by using non-linear modeling.
In high-school math we often thought about the relationship between y and x by plotting a line on a graph, with a scatterplot added in some cases. This also holds true for many scientific studies. In the case of a study of age and complication rates, for instance, an investigator could plot age on the x axis against risk of a complication on the y axis and show a regression line, perhaps with a 95% confidence interval. Non-linear modeling is often useful because it avoids assuming a linear relationship and allows the investigator to determine questions such as whether risk starts to increase disproportionately beyond a given age.
4.10. Do not ignore significant heterogeneity in meta-analyses.
Informally speaking, heterogeneity statistics test whether variations between the results of different studies in a meta-analysis are consistent with chance, or whether such variation reflects, at least in part, true differences between studies. If heterogeneity is present, authors need to do more than merely report the p-value and focus on the random-effects estimate. Authors should investigate the sources of heterogeneity and try to determine the factors that lead to differences in study results, for example, by identifying common features of studies with similar findings or idiosyncratic aspects of studies with outlying results.
4.11. For time-to-event variables, report the number of events but not the proportion.
Take the case of a study that reported: “of 60 patients accrued, 10 (17%) died”. While it is important to report the number of events, patients entered the study at different times and were followed for different periods, so the reported proportion of 17% is meaningless. The standard statistical approach to time-to-event variables is to calculate probabilities, such as the risk of death being 60% by five years or the median survival – the time at which the probability of survival first drops below 50% - being 52 months.
4.12. For time-to-event analyses, report median follow-up for patients without the event or the number followed without an event at a given follow-up time.
It is often useful to describe how long a cohort has been followed. To illustrate the appropriate methods of doing so, take the case of a cohort of 1,000 pediatric cancer patients treated in 1970 and followed to 2010. If the cure rate was only 40%, median follow-up for all patients might only be a few years, whilst the median follow-up for patients who survived was 40 years. This latter statistic gives a much better impression of how long the cohort had been followed. Now assume that in 2009, a second cohort of 2000 patients was added to the study. The median follow-up for survivors will now be around a year, which is again misleading. An alternative would be to report a statistic such as “312 patients have been followed without an event for at least 35 years”.
4.13. For time-to-event analyses, describe when follow-up starts and when and how patients are censored.
A common error is that investigators use a censoring date which leads to an overestimate of survival. For example, when assessing the metastasis-free survival a patient without a record of metastasis should be censored on the date of the last time the patient was known to be free of metastasis (e.g. negative bone scan, undetectable PSA), not at the date of last patient contact (which may not have involved assessment of metastasis). For overall survival, date of last patient contact would be an acceptable censoring date because the patient was indeed known to be event-free at that time. When assessing cause-specific endpoints, special consideration should be given the cause of death. The endpoints “disease-specific survival” and “disease-free survival” have specific definitions and require careful attention to methods. With disease-specific survival, authors need to consider carefully how to handle death due to other causes. One approach is to censor patients at the time of death, but this can lead to bias in certain circumstances, such as when the predictor of interest is associated with other cause death and the probability of other cause death is moderate or high. Competing risk analysis is appropriate in these situations. With disease-free survival, both evidence of disease (e.g. disease recurrence) and death from any cause are counted as events, and so censoring at the time of other cause death is inappropriate. If investigators are specifically interested only in the former, and wish to censor deaths from other causes, they should define their endpoint as “freedom from progression”.
4.14. For time-to-event analyses, avoid reporting mean follow-up or survival time, or estimates of survival in those who had the event.
All three estimates are problematic in the context of censored data.
4.15. For time-to-event analyses, make sure that all predictors are known at time zero or consider alternative approaches such as a landmark analysis or time-dependent covariates.
In many cases, variables of interest vary over time. As a simple example, imagine we were interested in whether PSA velocity predicted time to progression in prostate cancer patients on active surveillance. The problem is that PSA is measured at various times after diagnosis. Unless they were being careful, investigators might use time from diagnosis in a Kaplan-Meier or Cox regression but use PSA velocity calculated on PSAs measured at one and two-year follow-up. As another example, investigators might determine whether response to chemotherapy predicts cancer survival, but measure survival from the time of the first dose, before response is known. It is obviously invalid to use information only known “after the clock starts”. There are two main approaches to this problem. A “landmark analysis” is often used when the variable of interest is generally known within a short and well-defined period of time, such as adjuvant therapy or chemotherapy response. In brief, the investigators start the clock at a fixed “landmark” (e.g. 6 months after surgery). Patients are only eligible if they are still at risk at the landmark (e.g. patients who recur before six months are excluded) and the status of the variable is fixed at that time (e.g. a patient who gets chemotherapy at 7 months is defined as being in the no adjuvant group). Alternatively, investigators can use a time-dependent variable approach. In brief, this “resets the clock” each time new information is available about a variable. This would be the approach most typically used for the PSA velocity and progression example.
4.16. When presenting Kaplan-Meier figures, present the number at risk and truncate follow-up when numbers are low.
Giving the number of risk is useful for helping to understand when patients were censored. When presenting Kaplan-Meier figures a good rule of thumb is to truncate follow-up when the number at risk in any group falls below 5 (or even 10) as the tail of a Kaplan-Meier distribution is very unstable.
- 5. Multivariable models and diagnostic tests
5.1. Multivariable, propensity and instrumental variable analyses are not a magic wand.
Some investigators assume that multivariable adjustment “removes confounding”, “makes groups similar” or “mimics a randomized trial”. There are two problems with such claims. First, the value of a variable recorded in a data set is often approximate and so may mask differences between groups. For instance, clinical stage might be used as a covariate in a study comparing treatments for localized prostate cancer. But stage T2c might constitute a small nodule on each prostate lobe or, alternatively, most of the prostate consisting of a large, hard mass. The key point is that if one group has more T2c disease than the other, it is also likely that the T2c’s in that group will fall towards the more aggressive end of the spectrum. Multivariable adjustment has the effect of making the rates of T2c in each group the same, but does not ensure that the type of T2c is identical. Second, a model only adjusts for a small number of measured covariates. That does not exclude the possibility of important differences in unmeasured (or even unmeasurable) covariates. A common assumption is that propensity methods somehow provide better adjustment for confounding than traditional multivariable methods. Except in certain rare circumstances, such as when the number of covariates is large relative to the number of events, propensity methods give extremely similar results to multivariable regression. Similarly, instrumental variables analyses depend on the availability of a good instrument, which is less common than is often assumed. In many cases, the instrument is not strongly associated with the intervention, leading to a large increase in the 95% confidence interval or, in some cases, an underestimate of treatment effects.
5.2. Avoid stepwise selection.
Investigators commonly choose which variables to include in a multivariable model by first determining which variables are statistically significant on univariable analysis; alternatively, they may include all variables in a single model but then remove any that are not significant. This type of data-dependent variable selection in regression models has several undesirable properties, increasing the risk of overfit and making many statistics, such as the 95% confidence interval, highly questionable. Use of stepwise selection should be restricted to a limited number of circumstances, such as during the initial stages of developing a model, if there is poor knowledge of what variables might be predictive.
5.3. Avoid reporting estimates such as odds or hazard ratios for covariates when examining the effects of interventions.
In a typical observational study, an investigator might explore the effects of two different approaches to radical prostatectomy on recurrence while adjusting for covariates such as stage, grade and PSA. It is rarely worth reporting estimates such as odds or hazard ratios for the covariates. For instance, it is well known that a high Gleason score is strongly associated with recurrence: reporting a hazard ratio of say, 4.23, is not helpful and a distraction from the key finding, the hazard ratio between the two types of surgery.
5.4. Rescale predictors to obtain interpretable estimates.
Predictors sometimes have a moderate association with outcome and can take a large range of values. This can lead to uninterpretable estimates. For instance, the odds ratio for cancer per year of age might be given as 1.02 (95% CI 1.01, 1.02; p<0.0001). It is not helpful to have the upper bound of a confidence interval be equivalent to the central estimate; a better alternative would be to report an odds ratio per ten years of age. This is simply achieved by creating a new variable equal to age divided by ten to obtain an odds ratio of 1.16 (95% CI 1.10, 1.22; p<0.0001) per 10-year difference in age.
5.5. Avoid reporting both univariate and multivariable analyses unless there is a good reason.
Comparison of univariate and multivariable models can be of interest when trying to understand mechanisms. For instance, if race is a predictor of outcome on univariate analysis, but not after adjustment for income and access to care, one might conclude that poor outcome in African-Americans is explained by socioeconomic factors. However, the routine reporting of estimates from both univariate and multivariable analysis is discouraged.
5.6. Avoid ranking predictors in terms of strength.
It is tempting for authors to rank predictors in a model, claiming, for instance, “the novel marker was the strongest predictor of recurrence”. Most commonly, this type of claim is based on comparisons of odds or hazard ratios. Such rankings are not meaningful since, among other reasons, it depends on how variables are coded. For instance, the odds ratio for hK2, and hence whether or not it is an apparently “stronger” predictor than PSA, will depend on whether it is entered in nanograms or picograms per ml. Further, it is unclear how one should compare model coefficients when both categorical and continuous variables are included. Finally, the prevalence of a categorical predictor also matters: a predictor with an odds ratio is 3.5 but a prevalence if 0.1% is less important that one with a 50% prevalence and an odds ratio of 2.0.
5.7. Discrimination is a property not of a multivariable model but rather of the predictors and the data set.
Although model building is generally seen as a process of fitting coefficients, discrimination is largely a property of what predictors are available. For instance, we have excellent models for prostate cancer outcome primarily because Gleason score is very strongly associated with malignant potential. In addition, discrimination is highly dependent on how much a predictor varies in the data set. As an example, a model to predict erectile dysfunction that includes age will have much higher discrimination for a population sample of adult men than for a group of older men presenting at a urology clinic, because there is a greater variation in age in the population sample. Authors need to consider these points when drawing conclusions about the discrimination of models. This is also why authors should be cautious about comparing the discrimination of different multivariable models where these were assessed in different datasets.
5.8. Correction for overfit is strongly recommended for internal validation.
In the same way that it is easy to predict last week’s weather, a prediction model generally has very good properties when evaluated on the same data set used to create the model. This problem is generally described as overfit. Various methods are available to correct for overfit, including crossvalidation and bootstrap resampling. Note that such methods should include all steps of model building. For instance, if an investigator uses stepwise methods to choose which predictors should go into the model and then fits the coefficients, a typical crossvalidation approach would be to: (1) split the data into ten groups, (2) use stepwise methods to select predictors using the first nine groups, (3) fit coefficients using the first nine groups, (4) apply the model to the 10 th group to obtain predicted probabilities, and (5) repeat steps 2–4 until all patients in the data set have a predicted probability derived from a model fitted to a data set that did not include that patient’s data. Statistics such as the AUC are then calculated using the predicted probabilities directly.
5.9. Calibration should be reported and interpreted correctly.
Calibration is a critical component of a statistical model: the main concern for any patient is whether the risk given by a model is close to his or her true risk. It is rarely worth reporting calibration for a model created and tested on the same data set, even if techniques such as crossvalidation are used. This is because calibration is nearly always excellent on internal validation. Where a pre-specified model is tested on an independent data set, calibration should be displayed graphically in a calibration plot. The Hosmer-Lemeshow test addresses an inappropriate null hypothesis and should be avoided. Note also that calibration depends upon both the model coefficients and the dataset being examined. A model cannot be inherently “well calibrated.” All that can be said is that predicted and observed risk are close in a specific data set, representative of a given population.
5.10. Avoid reporting sensitivity and specificity for continuous predictors or a model.
Investigators often report sensitivity and specificity at a given cut-point for a continuous predictor (such as a PSA of 10 ng /mL), or report specificity at a given sensitivity (such as 90%). Reporting sensitivity and specificity is not of value because it is unclear how high sensitivity or specificity would have to be so as to be high enough to justify clinical use. Similarly, it is very difficult to determine which of two tests, one with a higher sensitivity and the other with a higher specificity, is preferable because clinical value depends on the prevalence of disease and the relative harms of a false-positive compared with a false-negative result. In the case of reporting specificities at fixed sensitivity, or vice versa, it is all but impossible to choose the specific sensitivity rationally. For instance, a team of investigators may state that they want to know specificity at 80% sensitivity, because they want to ensure they catch 80% of cases. But 80% might be too low if prevalence is high, or too high if prevalence is low.
5.11. Report the clinical consequences of using a test or a model.
In place of statistical abstractions such as sensitivity and specificity, or an ROC curve, authors are encouraged to choose illustrative cut-points and then report results in terms of clinical consequences. As an example, consider a study in which a marker is measured in a group of patients undergoing biopsy. Authors could report that if a given level of the marker had been used to determine biopsy, then a certain number of biopsies would have been conducted and a certain number of cancers found and missed.
5.12. Interpret decision curves with careful reference to threshold probabilities.
It is insufficient merely to report that, for instance, “the marker model had highest net benefit for threshold probabilities of 35 – 65%”. Authors need to consider whether those threshold probabilities are rational. If the study reporting benefit between 35 – 65% concerned detection of high-grade prostate cancer, few if any urologists would demand that a patient have at least a one-in-three chance of high-grade disease before recommending biopsy. The authors would therefore need to conclude that the model was not of benefit.
- 6. Conclusions and interpretation
6.1. Draw a conclusion, don’t just repeat the results.
Conclusion sections are often simply a restatement of the results. For instance, “a statistically significant relationship was found between body mass index (BMI) and disease outcome” is not a conclusion. Authors instead need to state implications for research and / or clinical practice. For instance, a conclusion section might call for research to determine whether the association between BMI is causal or make a recommendation for more aggressive treatment of patients with higher BMI.
6.2. Avoid using words such as “may” or “might”.
A conclusion such as that a novel treatment “may” be of benefit would only be untrue if it had been proven that the treatment was ineffective. Indeed, that the treatment may help would have been the rationale for the study in the first place. Using words such as may in the conclusion is equivalent to stating, “we know no more at the end of this study than we knew at the beginning”, reason enough to reject a paper for publication.
6.3. A statistically significant p-value does not imply clinical significance.
A small p-value means only that the null hypothesis has been rejected. This may or may not have implications for clinical practice. For instance, that a marker is a statistically significant predictor of outcome does not imply that treatment decisions should be made on the basis of that marker. Similarly, a statistically significant difference between two treatments does not necessarily mean that the former should be preferred to the latter. Authors need to justify any clinical recommendations by carefully analyzing the clinical implications of their findings.
6.4. Avoid pseudo-limitations such as “small sample size” and “retrospective analysis”, consider instead sources of potential bias and the mechanism for their effect on findings.
Authors commonly describe study limitations in a rather superficial way, such as, “small sample size and retrospective analysis are limitations”. But a small sample size may be immaterial if the results of the study are clear. For instance, if a treatment or predictor is associated with a very large odds ratio, a large sample size might be unnecessary. Similarly, a retrospective design might be entirely appropriate, as in the case of a marker study with very long-term follow-up, and have no discernible disadvantages compared to a prospective study. Discussion of limitations should include both the likelihood and effect size of possible bias.
6.5. Consider the impact of missing data and patient selection.
It is rare that complete data is obtained from all patients in a study. A typical paper might report, for instance, that of 200 patients, 8 had data missing on important baseline variables and 34 did not complete the end of study questionnaire, leading to a final data set of 158. Similarly, many studies include a relatively narrow subset of patients, such as 50 patients referred for imaging before surgery, out of the 500 treated surgically during that timeframe. In both cases, it is worth considering analyses to investigate whether patients with missing data or who were not selected for treatment were different in some way from those who were included in the analyses. Although statistical adjustment for missing data is complex and is warranted only in a limited set of circumstances, basic analyses to understand the characteristics of patients with missing data are relatively straightforward and are often helpful.
6.6. Consider the possibility and impact of ascertainment bias
Ascertainment bias occurs when an outcome depends on a test, and the propensity for a patient to be tested is associated with the predictor. PSA screening provides a classic example: prostate cancer is found by biopsy, but the main reason why men are biopsied is because of an elevated PSA. A study in a population subject to PSA screening will therefore overestimate the association between PSA and prostate cancer. Ascertainment bias can also be caused by the timing of assessments. For instance, frequency of biopsy in prostate cancer active surveillance will depend on prior biopsy results and PSA level, and this induces an association between those predictors and time to progression.
6.7. Do not confuse outcome with response among subgroups of patients undergoing the same treatment: patients with poorer outcomes may still be good candidates for that treatment.
Investigators often compare outcomes in different subgroups of patients all receiving the same treatment. A common error is to conclude that patients with poor outcome are not good candidates for that treatment and should receive an alternative approach. This is to confuse differences between patients for differences between treatments. As a simple example, patients with large tumors are more likely to recur after surgery than patients with small tumors, but that cannot be taken to suggest that resection is not indicated for patients with tumors greater than a certain size. Indeed, surgery is generally more strongly indicated for patients with aggressive (but localized) disease and such patients are unlikely to do well on surveillance.
6.8. Be cautious about causal attribution: correlation does not imply causation.
It is well-known that “correlation does not imply causation” but authors often slip into this error in making conclusions. The introduction and methods section might insist that the purpose of the study is merely to determine whether there is an association between, say, treatment frequency and treatment response, but the conclusions may imply that, for instance, more frequent treatment would improve response rates.
- Use and interpretation of p-values
That p-values are widely misused and misunderstood is apparent from even the most cursory reading of the medical literature. One of the most common errors is accepting the null hypothesis, for instance, concluding from a p-value of 0.07 that a drug is ineffective or that two surgical techniques are equivalent. This particular error is described in detail in guideline 3.1. The more general problem, which we address here, is that p-values are often given excessive weight in the interpretation of a study. Indeed, studies are often classed by investigators into “positive” or “negative” based on statistical significance. Gross misuse of p-values has led some to advocate banning the use of p-values completely[ 4 ].
We follow the American Statistical Association statement on p-values and encourage all researchers to read either the full statement[ 5 ] or the summary[ 6 ]. In particular, we emphasize that the p-value is just one statistic that helps interpret a study, it does not determine our interpretations. Drawing conclusions for research or clinical practice from a clinical research study requires evaluation of the strengths and weakness of study methodology, the results of other pertinent data published in the literature, biological plausibility and effect size. Sound and nuanced scientific judgment cannot be replaced by just checking whether one of the many statistics in a paper is or is not less than 0.05.
- Concluding remarks
These guidelines are not intended to cover all medical statistics but rather the statistical approaches most commonly used in clinical research papers in urology. It is quite possible for a paper to follow all of the guidelines yet be statistically flawed or to break numerous guidelines and still be statistically sound. On balance, however, the analysis, reporting and interpretation of clinical urologic research will be improved by adherence to these guidelines.
- Acknowledgments
Funding support : Supported in part by the Sidney Kimmel Center for Prostate and Urologic Cancers, P50-CA92629 SPORE grant from the National Cancer Institute to Dr. H. Scher, and the P30-CA008748 NIH/NCI Cancer Center Support Grant to Memorial Sloan-Kettering Cancer Center.
Conflicts of interest : The authors have nothing to disclose.
Full text links
Read article at publisher's site: https://doi.org/10.1111/bju.14640
Citations & impact
Impact metrics, citations of article over time, alternative metrics.

Article citations
To assess the contributing factors of nutritional and health status amongst elderlies residing in the nursing homes of fars province, iran: a cross sectional study..
Joulaei H , Keshani P , Kashfinejad SM , Foroozanfar Z , Mohsenpour MA , Fararouei M
Health Sci Rep , 7(3):e1940, 07 Mar 2024
Cited by: 0 articles | PMID: 38455646 | PMCID: PMC10918975
Prevalence of metabolically healthy obesity and healthy overweight and the associated factors in southern Iran: A population-based cross-sectional study.
Taherifard E , Taherifard E , Jeddi M , Ahmadkhani A , Kelishadi R , Poustchi H , Gandomkar A , Malekzadeh F , Mohammadi Z , Molavi Vardanjani H
Health Sci Rep , 7(2):e1909, 15 Feb 2024
Cited by: 0 articles | PMID: 38361808 | PMCID: PMC10867705
Maternal serum angiopoietins levels in pre-eclampsia and pregnancy outcomes.
Bayor F , Adu-Bonsaffoh K , Antwi-Boasiako C
Health Sci Rep , 7(1):e1806, 15 Jan 2024
Cited by: 0 articles | PMID: 38226360 | PMCID: PMC10788767
The effects of low calorie, high protein diet on body composition, duration and sleep quality on obese adults: A randomized clinical trial.
Javaheri FSH , Ostadrahimi AR , Nematy M , Arabi SM , Amini M
Health Sci Rep , 6(11):e1699, 16 Nov 2023
Cited by: 0 articles | PMID: 38028703 | PMCID: PMC10652319
Exploring therapeutic potential of Woodfordia fruticosa (L.) Kurz leaf and bark focusing on antioxidant, antithrombotic, antimicrobial, anti-inflammatory, analgesic, and antidiarrheal properties.
Rahman MM , Soma MA , Sultana N , Hossain MJ , Sufian MA , Rahman MO , Rashid MA
Health Sci Rep , 6(10):e1654, 25 Oct 2023
Cited by: 1 article | PMID: 37885464 | PMCID: PMC10599101
Similar Articles
To arrive at the top five similar articles we use a word-weighted algorithm to compare words from the Title and Abstract of each citation.
Guidelines for Reporting of Statistics for Clinical Research in Urology.
Assel M , Sjoberg D , Elders A , Wang X , Huo D , Botchway A , Delfino K , Fan Y , Zhao Z , Koyama T , Hollenbeck B , Qin R , Zahnd W , Zabor EC , Kattan MW , Vickers AJ
Eur Urol , 75(3):358-367, 21 Dec 2018
Cited by: 43 articles | PMID: 30580902 | PMCID: PMC6391870
Guidelines for reporting of statistics in European Urology.
Vickers AJ , Sjoberg DD , European Urology
Eur Urol , 67(2):181-187, 15 Jul 2014
Cited by: 39 articles | PMID: 25037638
Do journals publishing in the field of urology endorse reporting guidelines? A survey of author instructions.
Kunath F , Grobe HR , Rücker G , Engehausen D , Antes G , Wullich B , Meerpohl JJ
Urol Int , 88(1):54-59, 19 Nov 2011
Cited by: 21 articles | PMID: 22104723
Hematology journals do not sufficiently adhere to reporting guidelines: a systematic review.
Wayant C , Smith C , Sims M , Vassar M
J Thromb Haemost , 15(4):608-617, 27 Feb 2017
Cited by: 11 articles | PMID: 28122156
A systematic survey of the quality of research reporting in general orthopaedic journals.
Parsons NR , Hiskens R , Price CL , Achten J , Costa ML
J Bone Joint Surg Br , 93(9):1154-1159, 01 Sep 2011
Cited by: 37 articles | PMID: 21911523
Funding
Funders who supported this work.
NCI NIH HHS (3)
Grant ID: P30 CA008748
23503 publication s
Grant ID: P50 CA092629
634 publication s
Grant ID: P30 CA016672
13424 publication s
National Cancer Institute (1)
Grant ID: P50‐CA92629
3 publication s
National Institutes of Health (1)
Grant ID: P30‐CA008748
10 publication s
Europe PMC is part of the ELIXIR infrastructure
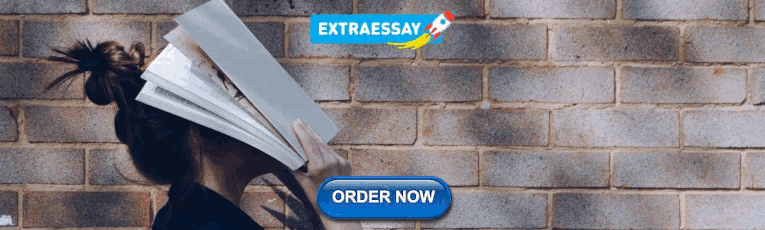
IMAGES
VIDEO
COMMENTS
In an effort to improve the quality of statistics in the clinical urology literature, statisticians at European Urology, The Journal of Urology, Urology and BJU International came together to develop a set of guidelines to address common errors of statistical analysis, reporting, and interpretation. Authors should 'break any of the guidelines ...
In an effort to improve the quality of statistics in the clinical urology literature statisticians at European Urology, The Journal of Urology®, Urology and BJUI developed a set of guidelines to address common errors of statistical analysis, reporting and interpretation. Authors should break any of the guidelines if it makes scientific sense to do so but would need to provide clear justification.
: Guidelines for reporting of statistics for clinical research in urology. J Urol 2019; 201: 595. Link, Google Scholar; 2. . Statistical methods in anesthesia articles: an evaluation of two American journals during two six-month periods. Anesth Analg 1985; 64: 607. Google Scholar; 3. : Writing up clinical research: a statistician's view.
Vickers A, Assel M, Sjoberg D, Qin R, Zhao Z, Koyama T, Botchway A, Wang X, Huo D, Kattan M, Zabor E and Harrell F (2020) Guidelines for Reporting of Figures and Tables for Clinical Research in Urology Journal of Urology, VOL. 204, NO. 1, (121-133), Online publication date: 1-Jul-2020.
P30 CA016672/CA/NCI NIH HHS/United States. Investigators submitting clinical research to European Urology are encouraged to follow guidelines for the reporting of statistics. Adoption of the guidelines will not only increase the quality of published papers, but also improve statistical knowledge in urology in general.
Skip to Article Content; Skip to Article Information; In an effort to improve the quality of statistics in the clinical urology literature, statisticians at European Urology, The Journal of Urology, Urology and BJU International came together to devel...
Abstract. In an effort to improve the presentation of and information within tables and figures in clinical urology research, we propose a set of appropriate guidelines. We introduce 6 principles (1) include graphs only if they improve the reader's ability to understand the study findings; (2) think through how a graph might best convey ...
The specific guidelines for precision are listed below. Report p-values to a single significant figure unless the p is close to 0.05 (for example, 0.01 to 0.2), in which case, report 2 significant figures. Do not report "NS" for p-values of 0.05 or above. Low p-values can be reported as p <0.001 or similar.
quality of statistical reporting in urology journals has no doubt improved since 2005, but remains unsatisfactory. The four urology journals in the Scales et al's [1] review have come together to publish a shared set of statistical guidelines, adapted from those in use at one of the journals, European Urology, since 2014 [3]. The guidelines
European Urology has established three principles for improving the quality of statistics in papers published in our journal: (1) systematic guidance for authors based on common statistical errors ...
Guidelines for reporting of statistics for clinical research in urology Melissa Assel1, Daniel Sjoberg1, Andrew Elders2, Xuemei Wang3, Dezheng Huo4, Albert Botchway5, Kristin Delfino5, Yunhua Fan6, Zhiguo Zhao8, Tatsuki Koyama8, Brent Hollenbeck9, Rui Qin10, Whitney Zahnd11, Emily C. Zabor1, Michael W. Kattan7 and Andrew J. Vickers1 1Memorial Sloan Kettering Cancer Center, New York, NY, USA ...
This is followed by guidelines on tables. Note that in the following sections, references to the "statistical reporting guidelines" refer to the "Guidelines for Reporting of Statistics for Clinical Research in Urology" that were copublished by the four major urology journals and which have been adopted more widely since.
In an effort to improve the quality of statistics in the clinical urology literature, statisticians at European Urology, The Journal of Urology, Urology and BJUI came together to develop a set of guidelines to address common errors of statistical analysis, reporting and interpretation. Authors should "break any of the guidelines if it makes scientific sense to do so" but would need to ...
DOI: 10.1016/j.eururo.2018.12.014 Corpus ID: 58571422; Guidelines for Reporting of Statistics for Clinical Research in Urology. @article{Assel2019GuidelinesFR, title={Guidelines for Reporting of Statistics for Clinical Research in Urology.}, author={Melissa J. Assel and Daniel D. Sjoberg and Andrew Elders and Xuemei Wang and Dezheng Huo and Albert Botchway and Kristin R. Delfino and Yunhua Fan ...
Abstract. In an effort to improve the presentation of and information within tables and figures in clinical urology research, we propose a set of appropriate guidelines. We introduce six principles: (1) include graphs only if they improve the reader's ability to understand the study findings; (2) think through how a graph might best convey ...
Kolbinger, Veldhuizen et al. systematically review reporting guidelines for artificial intelligence (AI) methods in clinical research. They identify several essential, commonly recommended items ...
In an effort to improve the quality of statistics in the clinical urology literature, statisticians at European Urology, The Journal of Urology, Urology, and BJUI came together to develop a set of guidelines to address common errors of statistical analysis, reporting, and interpretation. Authors should "break any of the guidelines if it makes scientific sense to do so" but would need to ...
In an effort to improve the quality of statistics in the clinical urology literature, statisticians at European Urology, The Journal of Urology, Urology and BJU International came together to develop a set of guidelines to address common errors of statistical analysis, reporting, and interpretation. Authors should 'break any of the guidelines if it makes scientific sense to do so', but ...
The widespread use of artificial intelligence (AI) in medical decision-making tools has led to an update of the TRIPOD guidelines for reporting clinical prediction models. The new TRIPOD+AI ...
A set of guidelines to address common errors of statistical analysis, reporting, and interpretation is developed to improve the quality of statistics in the clinical urology literature. In an effort to improve the quality of statistics in the clinical urology literature, statisticians at European Urology, The Journal of Urology, Urology and BJU International came together to develop a set of ...
A set of guidelines to address common errors of statistical analysis, reporting and interpretation is developed to improve the quality of statistics in the clinical urology literature. In an effort to improve the quality of statistics in the clinical urology literature, statisticians at European Urology, The Journal of Urology, Urology and BJUI came together to develop a set of guidelines to ...
2 Abstract In an effort to improve the quality of statistics in the clinical urology literature, statisticians at European Urology, The Journal of Urology, Urology and BJUI came together to develop a set of guidelines to address common errors of statistical analysis, reporting and interpretation.
https://orcid.org. Europe PMC. Menu. About. About Europe PMC; Preprints in Europe PMC