InVisionApp, Inc.
Inside Design
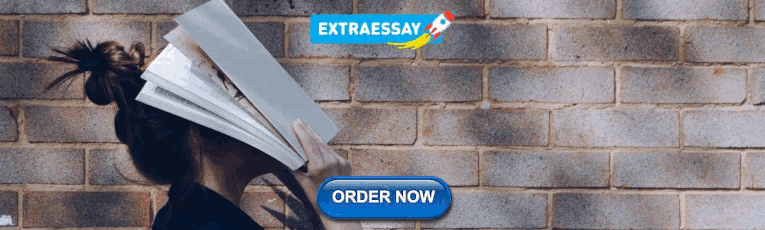
5 steps to a hypothesis-driven design process
• mar 22, 2018.
S ay you’re starting a greenfield project, or you’re redesigning a legacy app. The product owner gives you some high-level goals. Lots of ideas and questions are in your mind, and you’re not sure where to start.
Hypothesis-driven design will help you navigate through a unknown space so you can come out at the end of the process with actionable next steps.
Ready? Let’s dive in.
Step 1: Start with questions and assumptions
On the first day of the project, you’re curious about all the different aspects of your product. “How could we increase the engagement on the homepage? ” “ What features are important for our users? ”
Related: 6 ways to speed up and improve your product design process
To reduce risk, I like to take some time to write down all the unanswered questions and assumptions. So grab some sticky notes and write all your questions down on the notes (one question per note).
I recommend that you use the How Might We technique from IDEO to phrase the questions and turn your assumptions into questions. It’ll help you frame the questions in a more open-ended way to avoid building the solution into the statement prematurely. For example, you have an idea that you want to make riders feel more comfortable by showing them how many rides the driver has completed. You can rephrase the question to “ How might we ensure rider feel comfortable when taking ride, ” and leave the solution part out to the later step.
“It’s easy to come up with design ideas, but it’s hard to solve the right problem.”
It’s even more valuable to have your team members participate in the question brainstorming session. Having diverse disciplines in the room always brings fresh perspectives and leads to a more productive conversation.
Step 2: Prioritize the questions and assumptions
Now that you have all the questions on sticky notes, organize them into groups to make it easier to review them. It’s especially helpful if you can do the activity with your team so you can have more input from everybody.
When it comes to choosing which question to tackle first, think about what would impact your product the most or what would bring the most value to your users.
If you have a big group, you can Dot Vote to prioritize the questions. Here’s how it works: Everyone has three dots, and each person gets to vote on what they think is the most important question to answer in order to build a successful product. It’s a common prioritization technique that’s also used in the Sprint book by Jake Knapp —he writes, “ The prioritization process isn’t perfect, but it leads to pretty good decisions and it happens fast. ”
Related: Go inside design at Google Ventures
Step 3: Turn them into hypotheses
After the prioritization, you now have a clear question in mind. It’s time to turn the question into a hypothesis. Think about how you would answer the question.
Let’s continue the previous ride-hailing service example. The question you have is “ How might we make people feel safe and comfortable when using the service? ”
Based on this question, the solutions can be:
- Sharing the rider’s location with friends and family automatically
- Displaying more information about the driver
- Showing feedback from previous riders
Now you can combine the solution and question, and turn it into a hypothesis. Hypothesis is a framework that can help you clearly define the question and solution, and eliminate assumption.
From Lean UX
We believe that [ sharing more information about the driver’s experience and stories ] For [ the riders ] Will [ make riders feel more comfortable and connected throughout the ride ]
4. Develop an experiment and testing the hypothesis
Develop an experiment so you can test your hypothesis. Our test will follow the scientific methods, so it’s subject to collecting empirical and measurable evidence in order to obtain new knowledge. In other words, it’s crucial to have a measurable outcome for the hypothesis so we can determine whether it has succeeded or failed.
There are different ways you can create an experiment, such as interview, survey , landing page validation, usability testing, etc. It could also be something that’s built into the software to get quantitative data from users. Write down what the experiment will be, and define the outcomes that determine whether the hypothesis is valids. A well-defined experiment can validate/invalidate the hypothesis.
In our example, we could define the experiment as “ We will run X studies to show more information about a driver (number of ride, years of experience), and ask follow-up questions to identify the rider’s emotion associated with this ride (safe, fun, interesting, etc.). We will know the hypothesis is valid when we get more than 70% identify the ride as safe or comfortable. ”
After defining the experiment, it’s time to get the design done. You don’t need to have every design detail thought through. You can focus on designing what is needed to be tested.
When the design is ready, you’re ready to run the test. Recruit the users you want to target , have a time frame, and put the design in front of the users.
5. Learn and build
You just learned that the result was positive and you’re excited to roll out the feature. That’s great! If the hypothesis failed, don’t worry—you’ll be able to gain some insights from that experiment. Now you have some new evidence that you can use to run your next experiment. In each experiment, you’ll learn something new about your product and your customers.
“Design is a never-ending process.”
What other information can you show to make riders feel safe and comfortable? That can be your next hypothesis. You now have a feature that’s ready to be built, and a new hypothesis to be tested.
Principles from from The Lean Startup
We often assume that we understand our users and know what they want. It’s important to slow down and take a moment to understand the questions and assumptions we have about our product.
After testing each hypothesis, you’ll get a clearer path of what’s most important to the users and where you need to dig deeper. You’ll have a clear direction for what to do next.
by Sylvia Lai
Sylvia Lai helps startup and enterprise solve complex problems through design thinking and user-centered design methodologies at Pivotal Labs . She is the biggest advocate for the users, making sure their voices are heard is her number one priority. Outside of work, she loves mentoring other designers through one-on-one conversation. Connect with her through LinkedIn or Twitter .
Collaborate in real time on a digital whiteboard Try Freehand
Get awesome design content in your inbox each week, give it a try—it only takes a click to unsubscribe., thanks for signing up, you should have a thank you gift in your inbox now-and you’ll hear from us again soon, get started designing better. faster. together. and free forever., give it a try. nothing’s holding you back..
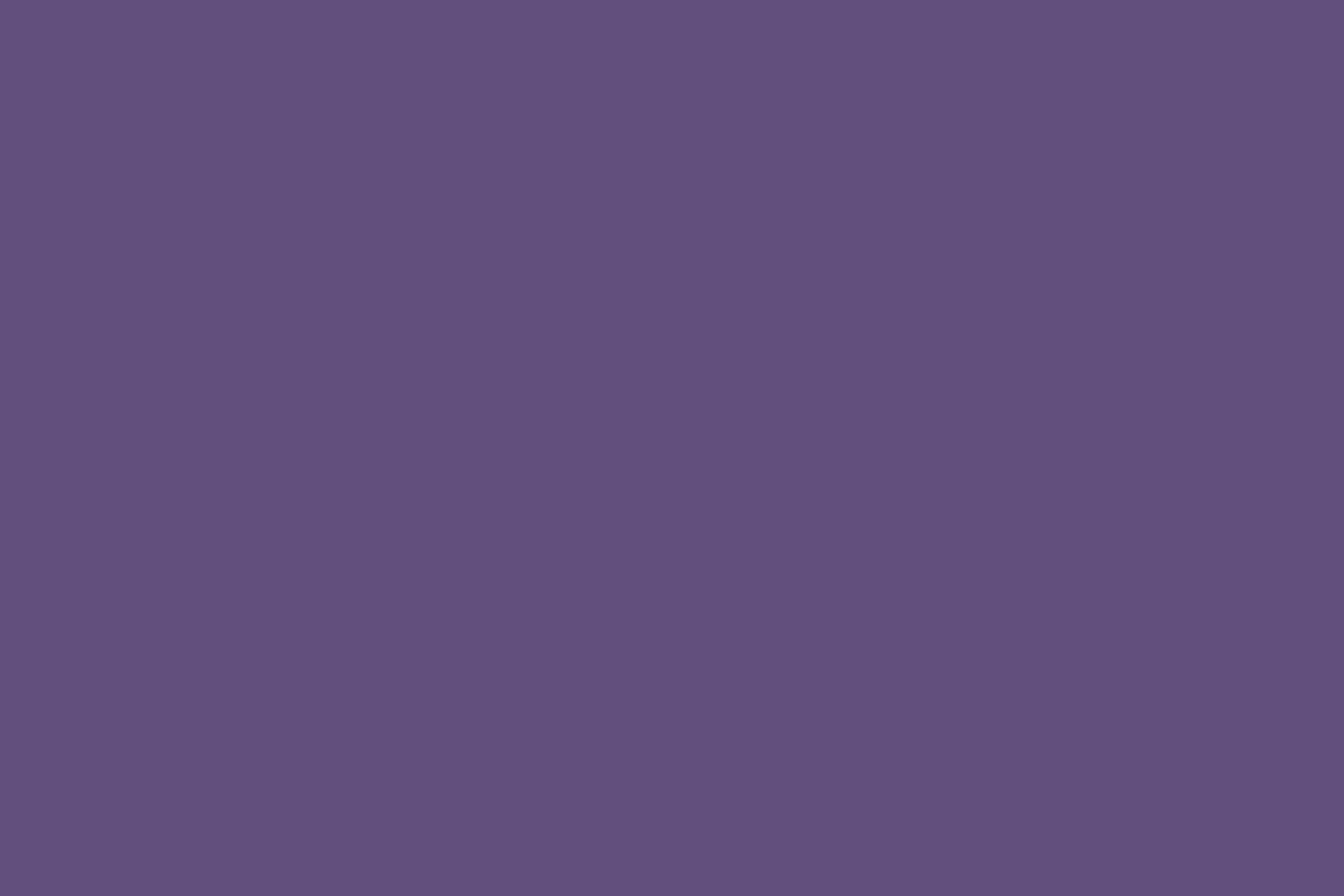
How to Implement Hypothesis-Driven Development
Remember back to the time when we were in high school science class. Our teachers had a framework for helping us learn – an experimental approach based on the best available evidence at hand. We were asked to make observations about the world around us, then attempt to form an explanation or hypothesis to explain what we had observed. We then tested this hypothesis by predicting an outcome based on our theory that would be achieved in a controlled experiment – if the outcome was achieved, we had proven our theory to be correct.
We could then apply this learning to inform and test other hypotheses by constructing more sophisticated experiments, and tuning, evolving or abandoning any hypothesis as we made further observations from the results we achieved.
Experimentation is the foundation of the scientific method, which is a systematic means of exploring the world around us. Although some experiments take place in laboratories, it is possible to perform an experiment anywhere, at any time, even in software development.
Practicing Hypothesis-Driven Development is thinking about the development of new ideas, products and services – even organizational change – as a series of experiments to determine whether an expected outcome will be achieved. The process is iterated upon until a desirable outcome is obtained or the idea is determined to be not viable.
We need to change our mindset to view our proposed solution to a problem statement as a hypothesis, especially in new product or service development – the market we are targeting, how a business model will work, how code will execute and even how the customer will use it.
We do not do projects anymore, only experiments. Customer discovery and Lean Startup strategies are designed to test assumptions about customers. Quality Assurance is testing system behavior against defined specifications. The experimental principle also applies in Test-Driven Development – we write the test first, then use the test to validate that our code is correct, and succeed if the code passes the test. Ultimately, product or service development is a process to test a hypothesis about system behaviour in the environment or market it is developed for.
The key outcome of an experimental approach is measurable evidence and learning.
Learning is the information we have gained from conducting the experiment. Did what we expect to occur actually happen? If not, what did and how does that inform what we should do next?
In order to learn we need use the scientific method for investigating phenomena, acquiring new knowledge, and correcting and integrating previous knowledge back into our thinking.
As the software development industry continues to mature, we now have an opportunity to leverage improved capabilities such as Continuous Design and Delivery to maximize our potential to learn quickly what works and what does not. By taking an experimental approach to information discovery, we can more rapidly test our solutions against the problems we have identified in the products or services we are attempting to build. With the goal to optimize our effectiveness of solving the right problems, over simply becoming a feature factory by continually building solutions.
The steps of the scientific method are to:
- Make observations
- Formulate a hypothesis
- Design an experiment to test the hypothesis
- State the indicators to evaluate if the experiment has succeeded
- Conduct the experiment
- Evaluate the results of the experiment
- Accept or reject the hypothesis
- If necessary, make and test a new hypothesis
Using an experimentation approach to software development
We need to challenge the concept of having fixed requirements for a product or service. Requirements are valuable when teams execute a well known or understood phase of an initiative, and can leverage well understood practices to achieve the outcome. However, when you are in an exploratory, complex and uncertain phase you need hypotheses.
Handing teams a set of business requirements reinforces an order-taking approach and mindset that is flawed.
Business does the thinking and ‘knows’ what is right. The purpose of the development team is to implement what they are told. But when operating in an area of uncertainty and complexity, all the members of the development team should be encouraged to think and share insights on the problem and potential solutions. A team simply taking orders from a business owner is not utilizing the full potential, experience and competency that a cross-functional multi-disciplined team offers.
Framing hypotheses
The traditional user story framework is focused on capturing requirements for what we want to build and for whom, to enable the user to receive a specific benefit from the system.
As A…. <role>
I Want… <goal/desire>
So That… <receive benefit>
Behaviour Driven Development (BDD) and Feature Injection aims to improve the original framework by supporting communication and collaboration between developers, tester and non-technical participants in a software project.
In Order To… <receive benefit>
As A… <role>
When viewing work as an experiment, the traditional story framework is insufficient. As in our high school science experiment, we need to define the steps we will take to achieve the desired outcome. We then need to state the specific indicators (or signals) we expect to observe that provide evidence that our hypothesis is valid. These need to be stated before conducting the test to reduce biased interpretations of the results.
If we observe signals that indicate our hypothesis is correct, we can be more confident that we are on the right path and can alter the user story framework to reflect this.
Therefore, a user story structure to support Hypothesis-Driven Development would be;
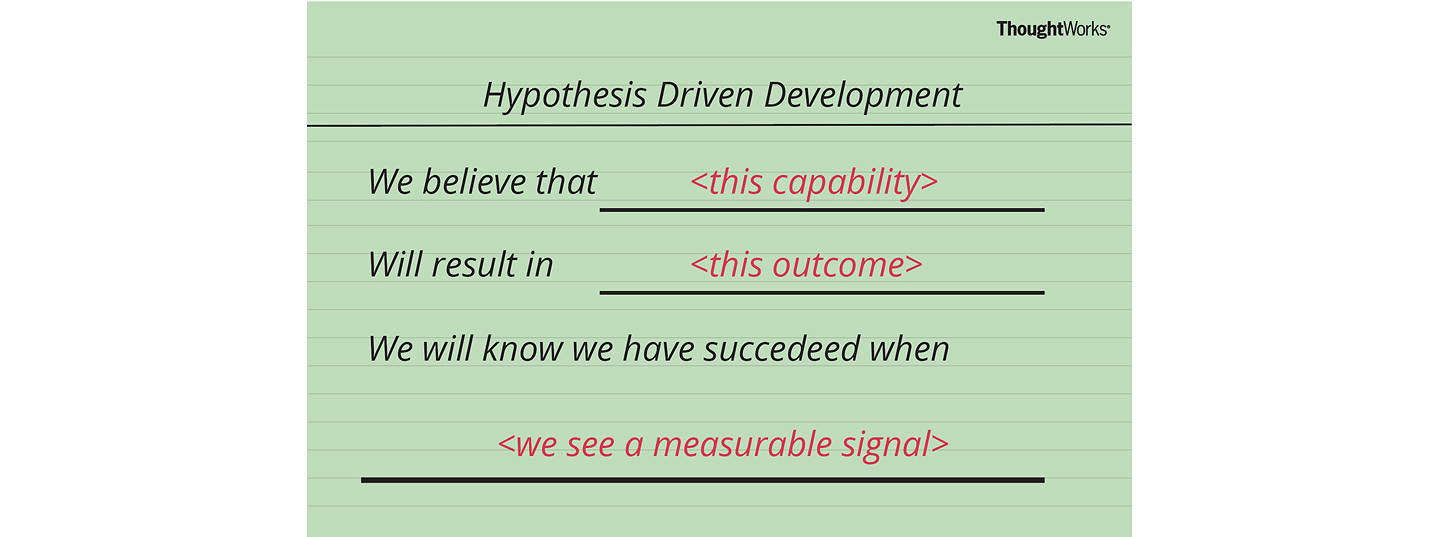
We believe < this capability >
What functionality we will develop to test our hypothesis? By defining a ‘test’ capability of the product or service that we are attempting to build, we identify the functionality and hypothesis we want to test.
Will result in < this outcome >
What is the expected outcome of our experiment? What is the specific result we expect to achieve by building the ‘test’ capability?
We will know we have succeeded when < we see a measurable signal >
What signals will indicate that the capability we have built is effective? What key metrics (qualitative or quantitative) we will measure to provide evidence that our experiment has succeeded and give us enough confidence to move to the next stage.
The threshold you use for statistically significance will depend on your understanding of the business and context you are operating within. Not every company has the user sample size of Amazon or Google to run statistically significant experiments in a short period of time. Limits and controls need to be defined by your organization to determine acceptable evidence thresholds that will allow the team to advance to the next step.
For example if you are building a rocket ship you may want your experiments to have a high threshold for statistical significance. If you are deciding between two different flows intended to help increase user sign up you may be happy to tolerate a lower significance threshold.
The final step is to clearly and visibly state any assumptions made about our hypothesis, to create a feedback loop for the team to provide further input, debate and understanding of the circumstance under which we are performing the test. Are they valid and make sense from a technical and business perspective?
Hypotheses when aligned to your MVP can provide a testing mechanism for your product or service vision. They can test the most uncertain areas of your product or service, in order to gain information and improve confidence.
Examples of Hypothesis-Driven Development user stories are;
Business story
We Believe That increasing the size of hotel images on the booking page
Will Result In improved customer engagement and conversion
We Will Know We Have Succeeded When we see a 5% increase in customers who review hotel images who then proceed to book in 48 hours.
It is imperative to have effective monitoring and evaluation tools in place when using an experimental approach to software development in order to measure the impact of our efforts and provide a feedback loop to the team. Otherwise we are essentially blind to the outcomes of our efforts.
In agile software development we define working software as the primary measure of progress.
By combining Continuous Delivery and Hypothesis-Driven Development we can now define working software and validated learning as the primary measures of progress.
Ideally we should not say we are done until we have measured the value of what is being delivered – in other words, gathered data to validate our hypothesis.
Examples of how to gather data is performing A/B Testing to test a hypothesis and measure to change in customer behaviour. Alternative testings options can be customer surveys, paper prototypes, user and/or guerrilla testing.
One example of a company we have worked with that uses Hypothesis-Driven Development is lastminute.com . The team formulated a hypothesis that customers are only willing to pay a max price for a hotel based on the time of day they book. Tom Klein, CEO and President of Sabre Holdings shared the story of how they improved conversion by 400% within a week.
Combining practices such as Hypothesis-Driven Development and Continuous Delivery accelerates experimentation and amplifies validated learning. This gives us the opportunity to accelerate the rate at which we innovate while relentlessly reducing cost, leaving our competitors in the dust. Ideally we can achieve the ideal of one piece flow: atomic changes that enable us to identify causal relationships between the changes we make to our products and services, and their impact on key metrics.
As Kent Beck said, “Test-Driven Development is a great excuse to think about the problem before you think about the solution”. Hypothesis-Driven Development is a great opportunity to test what you think the problem is, before you work on the solution.
How can you achieve faster growth?
The 6 Steps that We Use for Hypothesis-Driven Development

One of the greatest fears of product managers is to create an app that flopped because it's based on untested assumptions. After successfully launching more than 20 products, we're convinced that we've found the right approach for hypothesis-driven development.
In this guide, I'll show you how we validated the hypotheses to ensure that the apps met the users' expectations and needs.
What is hypothesis-driven development?
Hypothesis-driven development is a prototype methodology that allows product designers to develop, test, and rebuild a product until it’s acceptable by the users. It is an iterative measure that explores assumptions defined during the project and attempts to validate it with users’ feedbacks.
What you have assumed during the initial stage of development may not be valid for the users. Even if they are backed by historical data, user behaviors can be affected by specific audiences and other factors. Hypothesis-driven development removes these uncertainties as the project progresses.
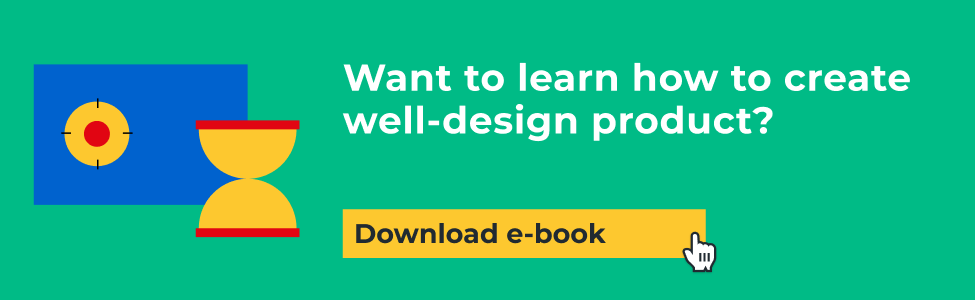
Why we use hypothesis-driven development
For us, the hypothesis-driven approach provides a structured way to consolidate ideas and build hypotheses based on objective criteria. It’s also less costly to test the prototype before production.
Using this approach has reliably allowed us to identify what, how, and in which order should the testing be done. It gives us a deep understanding of how we prioritise the features, how it’s connected to the business goals and desired user outcomes.
We’re also able to track and compare the desired and real outcomes of developing the features.
The process of Prototype Development that we use
Our success in building apps that are well-accepted by users is based on the Lean UX definition of hypothesis. We believe that the business outcome will be achieved if the user’s outcome is fulfilled for the particular feature.
Here’s the process flow:
How Might We technique → Dot voting (based on estimated/assumptive impact) → converting into a hypothesis → define testing methodology (research method + success/fail criteria) → impact effort scale for prioritizing → test, learn, repeat.
Once the hypothesis is proven right, the feature is escalated into the development track for UI design and development.
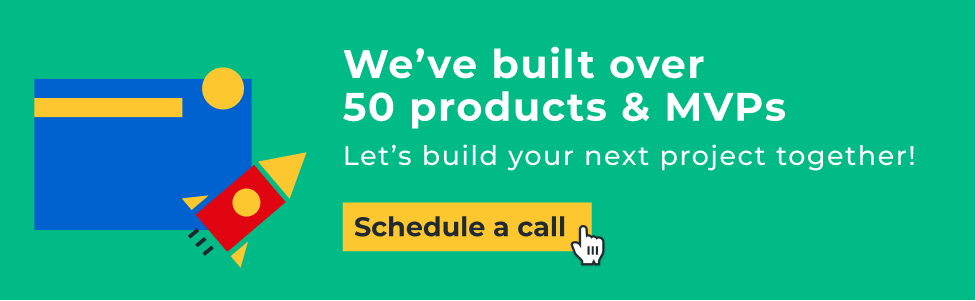
Step 1: List Down Questions And Assumptions
Whether it’s the initial stage of the project or after the launch, there are always uncertainties or ideas to further improve the existing product. In order to move forward, you’ll need to turn the ideas into structured hypotheses where they can be tested prior to production.
To start with, jot the ideas or assumptions down on paper or a sticky note.
Then, you’ll want to widen the scope of the questions and assumptions into possible solutions. The How Might We (HMW) technique is handy in rephrasing the statements into questions that facilitate brainstorming.
For example, if you have a social media app with a low number of users, asking, “How might we increase the number of users for the app?” makes brainstorming easier.
Step 2: Dot Vote to Prioritize Questions and Assumptions
Once you’ve got a list of questions, it’s time to decide which are potentially more impactful for the product. The Dot Vote method, where team members are given dots to place on the questions, helps prioritize the questions and assumptions.
Our team uses this method when we’re faced with many ideas and need to eliminate some of them. We started by grouping similar ideas and use 3-5 dots to vote. At the end of the process, we’ll have the preliminary data on the possible impact and our team’s interest in developing certain features.
This method allows us to prioritize the statements derived from the HMW technique and we’re only converting the top ones.
Step 3: Develop Hypotheses from Questions
The questions lead to a brainstorming session where the answers become hypotheses for the product. The hypothesis is meant to create a framework that allows the questions and solutions to be defined clearly for validation.
Our team followed a specific format in forming hypotheses. We structured the statement as follow:
We believe we will achieve [ business outcome],
If [ the persona],
Solve their need in [ user outcome] using [feature].
Here’s a hypothesis we’ve created:
We believe we will achieve DAU=100 if Mike (our proto persona) solve their need in recording and sharing videos instantaneously using our camera and cloud storage .
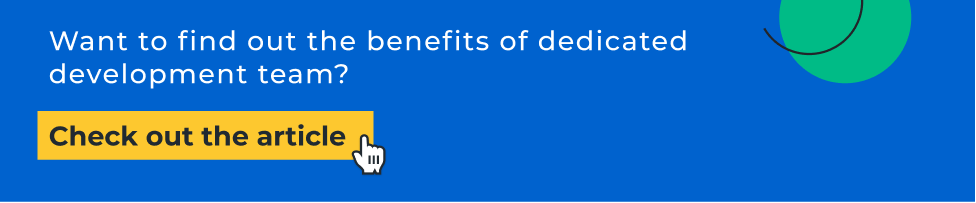
Step 4: Test the Hypothesis with an Experiment
It’s crucial to validate each of the assumptions made on the product features. Based on the hypotheses, experiments in the form of interviews, surveys, usability testing, and so forth are created to determine if the assumptions are aligned with reality.
Each of the methods provides some level of confidence. Therefore, you don’t want to be 100% reliant on a particular method as it’s based on a sample of users.
It’s important to choose a research method that allows validation to be done with minimal effort. Even though hypotheses validation provides a degree of confidence, not all assumptions can be tested and there could be a margin of error in data obtained as the test is conducted on a sample of people.
The experiments are designed in such a way that feedback can be compared with the predicted outcome. Only validated hypotheses are brought forward for development.
Testing all the hypotheses can be tedious. To be more efficient, you can use the impact effort scale. This method allows you to focus on hypotheses that are potentially high value and easy to validate.
You can also work on hypotheses that deliver high impact but require high effort. Ignore those that require high impact but low impact and keep hypotheses with low impact and effort into the backlog.
At Uptech, we assign each hypothesis with clear testing criteria. We rank the hypothesis with a binary ‘task success’ and subjective ‘effort on task’ where the latter is scored from 1 to 10.
While we’re conducting the test, we also collect qualitative data such as the users' feedback. We have a habit of segregation the feedback into pros, cons and neutral with color-coded stickers. (red - cons, green -pros, blue- neutral).
The best practice is to test each hypothesis at least on 5 users.
Step 5 Learn, Build (and Repeat)
The hypothesis-driven approach is not a single-ended process. Often, you’ll find that some of the hypotheses are proven to be false. Rather than be disheartened, you should use the data gathered to finetune the hypothesis and design a better experiment in the next phase.
Treat the entire cycle as a learning process where you’ll better understand the product and the customers.
We’ve found the process helpful when developing an MVP for Carbon Club, an environmental startup in the UK. The app allows users to donate to charity based on the carbon-footprint produced.
In order to calculate the carbon footprint, we’re weighing the options of
- Connecting the app to the users’ bank account to monitor the carbon footprint based on purchases made.
- Allowing users to take quizzes on their lifestyles.
Upon validation, we’ve found that all of the users opted for the second option as they are concerned about linking an unknown app to their banking account.
The result makes us shelves the first assumption we’ve made during pre-Sprint research. It also saves our client $50,000, and a few months of work as connecting the app to the bank account requires a huge effort.
.png)
Step 6: Implement Product and Maintain
Once you’ve got the confidence that the remaining hypotheses are validated, it’s time to develop the product. However, testing must be continued even after the product is launched.
You should be on your toes as customers’ demands, market trends, local economics, and other conditions may require some features to evolve.
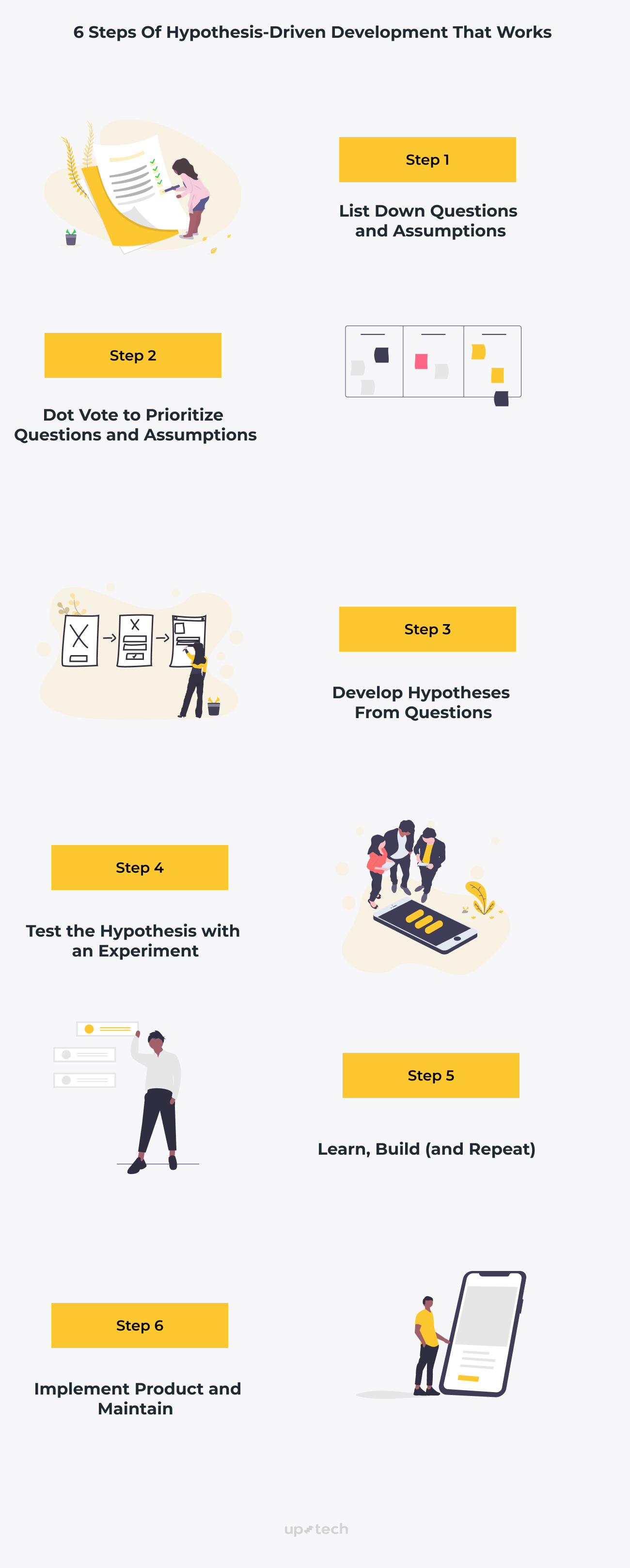
Our takeaways for hypothesis-driven development
If there’s anything that you could pick from our experience, it’s these 5 points.
1. Should every idea go straight into the backlog? No, unless they are validated with substantial evidence.
2. While it’s hard to define business outcomes with specific metrics and desired values, you should do it anyway. Try to be as specific as possible, and avoid general terms. Give your best effort and adjust as you receive new data.
3. Get all product teams involved as the best ideas are born from collaboration.
4. Start with a plan consists of 2 main parameters, i.e., criteria of success and research methods. Besides qualitative insights, you need to set objective criteria to determine if a test is successful. Use the Test Card to validate the assumptions strategically.
5. The methodology that we’ve recommended in this article works not only for products. We’ve applied it at the end of 2019 for setting the strategic goals of the company and end up with robust results, engaged and aligned team.
You'll have a better idea of which features would lead to a successful product with hypothesis-driven development. Rather than vague assumptions, the consolidated data from users will provide a clear direction for your development team.
As for the hypotheses that don't make the cut, improvise, re-test, and leverage for future upgrades.
Keep failing with product launches? I'll be happy to point you in the right direction. Drop me a message here.
Tell us about your idea. We will reach you out.
- Work together
- Product development
- Ways of working
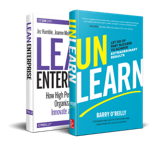
Have you read my two bestsellers, Unlearn and Lean Enterprise? If not, please do. If you have, please write a review!
- Read my story
- Get in touch
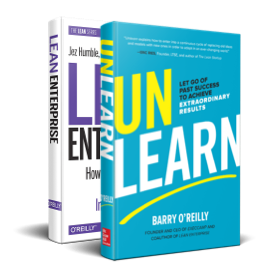
- Oval Copy 2 Blog
How to Implement Hypothesis-Driven Development
- Facebook__x28_alt_x29_ Copy
Remember back to the time when we were in high school science class. Our teachers had a framework for helping us learn – an experimental approach based on the best available evidence at hand. We were asked to make observations about the world around us, then attempt to form an explanation or hypothesis to explain what we had observed. We then tested this hypothesis by predicting an outcome based on our theory that would be achieved in a controlled experiment – if the outcome was achieved, we had proven our theory to be correct.
We could then apply this learning to inform and test other hypotheses by constructing more sophisticated experiments, and tuning, evolving, or abandoning any hypothesis as we made further observations from the results we achieved.
Experimentation is the foundation of the scientific method, which is a systematic means of exploring the world around us. Although some experiments take place in laboratories, it is possible to perform an experiment anywhere, at any time, even in software development.
Practicing Hypothesis-Driven Development [1] is thinking about the development of new ideas, products, and services – even organizational change – as a series of experiments to determine whether an expected outcome will be achieved. The process is iterated upon until a desirable outcome is obtained or the idea is determined to be not viable.
We need to change our mindset to view our proposed solution to a problem statement as a hypothesis, especially in new product or service development – the market we are targeting, how a business model will work, how code will execute and even how the customer will use it.
We do not do projects anymore, only experiments. Customer discovery and Lean Startup strategies are designed to test assumptions about customers. Quality Assurance is testing system behavior against defined specifications. The experimental principle also applies in Test-Driven Development – we write the test first, then use the test to validate that our code is correct, and succeed if the code passes the test. Ultimately, product or service development is a process to test a hypothesis about system behavior in the environment or market it is developed for.
The key outcome of an experimental approach is measurable evidence and learning. Learning is the information we have gained from conducting the experiment. Did what we expect to occur actually happen? If not, what did and how does that inform what we should do next?
In order to learn we need to use the scientific method for investigating phenomena, acquiring new knowledge, and correcting and integrating previous knowledge back into our thinking.
As the software development industry continues to mature, we now have an opportunity to leverage improved capabilities such as Continuous Design and Delivery to maximize our potential to learn quickly what works and what does not. By taking an experimental approach to information discovery, we can more rapidly test our solutions against the problems we have identified in the products or services we are attempting to build. With the goal to optimize our effectiveness of solving the right problems, over simply becoming a feature factory by continually building solutions.
The steps of the scientific method are to:
- Make observations
- Formulate a hypothesis
- Design an experiment to test the hypothesis
- State the indicators to evaluate if the experiment has succeeded
- Conduct the experiment
- Evaluate the results of the experiment
- Accept or reject the hypothesis
- If necessary, make and test a new hypothesis
Using an experimentation approach to software development
We need to challenge the concept of having fixed requirements for a product or service. Requirements are valuable when teams execute a well known or understood phase of an initiative and can leverage well-understood practices to achieve the outcome. However, when you are in an exploratory, complex and uncertain phase you need hypotheses. Handing teams a set of business requirements reinforces an order-taking approach and mindset that is flawed. Business does the thinking and ‘knows’ what is right. The purpose of the development team is to implement what they are told. But when operating in an area of uncertainty and complexity, all the members of the development team should be encouraged to think and share insights on the problem and potential solutions. A team simply taking orders from a business owner is not utilizing the full potential, experience and competency that a cross-functional multi-disciplined team offers.
Framing Hypotheses
The traditional user story framework is focused on capturing requirements for what we want to build and for whom, to enable the user to receive a specific benefit from the system.
As A…. <role>
I Want… <goal/desire>
So That… <receive benefit>
Behaviour Driven Development (BDD) and Feature Injection aims to improve the original framework by supporting communication and collaboration between developers, tester and non-technical participants in a software project.
In Order To… <receive benefit>
As A… <role>
When viewing work as an experiment, the traditional story framework is insufficient. As in our high school science experiment, we need to define the steps we will take to achieve the desired outcome. We then need to state the specific indicators (or signals) we expect to observe that provide evidence that our hypothesis is valid. These need to be stated before conducting the test to reduce the bias of interpretation of results.
If we observe signals that indicate our hypothesis is correct, we can be more confident that we are on the right path and can alter the user story framework to reflect this.
Therefore, a user story structure to support Hypothesis-Driven Development would be;
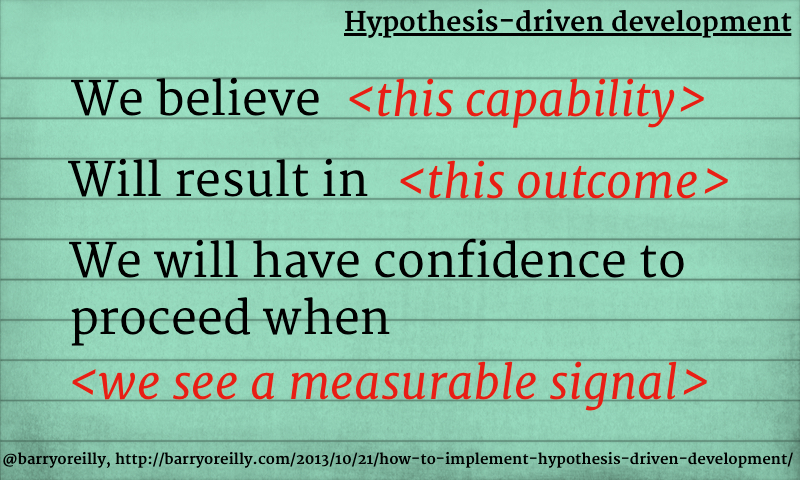
We believe < this capability >
What functionality we will develop to test our hypothesis? By defining a ‘test’ capability of the product or service that we are attempting to build, we identify the functionality and hypothesis we want to test.
Will result in < this outcome >
What is the expected outcome of our experiment? What is the specific result we expect to achieve by building the ‘test’ capability?
We will have confidence to proceed when < we see a measurable signal >
What signals will indicate that the capability we have built is effective? What key metrics (qualitative or quantitative) we will measure to provide evidence that our experiment has succeeded and give us enough confidence to move to the next stage.
The threshold you use for statistical significance will depend on your understanding of the business and context you are operating within. Not every company has the user sample size of Amazon or Google to run statistically significant experiments in a short period of time. Limits and controls need to be defined by your organization to determine acceptable evidence thresholds that will allow the team to advance to the next step.
For example, if you are building a rocket ship you may want your experiments to have a high threshold for statistical significance. If you are deciding between two different flows intended to help increase user sign up you may be happy to tolerate a lower significance threshold.
The final step is to clearly and visibly state any assumptions made about our hypothesis, to create a feedback loop for the team to provide further input, debate, and understanding of the circumstance under which we are performing the test. Are they valid and make sense from a technical and business perspective?
Hypotheses, when aligned to your MVP, can provide a testing mechanism for your product or service vision. They can test the most uncertain areas of your product or service, in order to gain information and improve confidence.
Examples of Hypothesis-Driven Development user stories are;
Business story.
We Believe That increasing the size of hotel images on the booking page Will Result In improved customer engagement and conversion We Will Have Confidence To Proceed When we see a 5% increase in customers who review hotel images who then proceed to book in 48 hours.
It is imperative to have effective monitoring and evaluation tools in place when using an experimental approach to software development in order to measure the impact of our efforts and provide a feedback loop to the team. Otherwise, we are essentially blind to the outcomes of our efforts.
In agile software development, we define working software as the primary measure of progress. By combining Continuous Delivery and Hypothesis-Driven Development we can now define working software and validated learning as the primary measures of progress.
Ideally, we should not say we are done until we have measured the value of what is being delivered – in other words, gathered data to validate our hypothesis.
Examples of how to gather data is performing A/B Testing to test a hypothesis and measure to change in customer behavior. Alternative testings options can be customer surveys, paper prototypes, user and/or guerilla testing.
One example of a company we have worked with that uses Hypothesis-Driven Development is lastminute.com . The team formulated a hypothesis that customers are only willing to pay a max price for a hotel based on the time of day they book. Tom Klein, CEO and President of Sabre Holdings shared the story of how they improved conversion by 400% within a week.
Combining practices such as Hypothesis-Driven Development and Continuous Delivery accelerates experimentation and amplifies validated learning. This gives us the opportunity to accelerate the rate at which we innovate while relentlessly reducing costs, leaving our competitors in the dust. Ideally, we can achieve the ideal of one-piece flow: atomic changes that enable us to identify causal relationships between the changes we make to our products and services, and their impact on key metrics.
As Kent Beck said, “Test-Driven Development is a great excuse to think about the problem before you think about the solution”. Hypothesis-Driven Development is a great opportunity to test what you think the problem is before you work on the solution.
We also run a workshop to help teams implement Hypothesis-Driven Development . Get in touch to run it at your company.
[1] Hypothesis-Driven Development By Jeffrey L. Taylor
More strategy insights
Say hello to venture capital 3.0, negotiation made simple with dr john lowry, how high performance organizations innovate at scale, read my newsletter.
Insights in every edition. News you can use. No spam, ever. Read the latest edition
We've just sent you your first email. Go check it out!
- Explore Insights
- Nobody Studios
- LinkedIn Learning: High Performance Organizations
Building and Using Theoretical Frameworks
- Open Access
- First Online: 03 December 2022
Cite this chapter
You have full access to this open access chapter
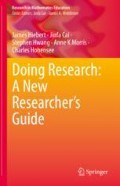
- James Hiebert 6 ,
- Jinfa Cai 7 ,
- Stephen Hwang 7 ,
- Anne K Morris 6 &
- Charles Hohensee 6
Part of the book series: Research in Mathematics Education ((RME))
14k Accesses
1 Citations
Theoretical frameworks can be confounding. They are supposed to be very important, but it is not always clear what they are or why you need them. Using ideas from Chaps. 1 and 2 , we describe them as local theories that are custom-designed for your study. Although they might use parts of larger well-known theories, they are created by individual researchers for particular studies. They are developed through the cyclic process of creating more precise and meaningful hypotheses. Building directly on constructs from the previous chapters, you can think of theoretical frameworks as equivalent to the most compelling, complete rationales you can develop for the predictions you make. Theoretical frameworks are important because they do lots of work for you. They incorporate the literature into your rationale, they explain why your study matters, they suggest how you can best test your predictions, and they help you interpret what you find. Your theoretical framework creates an essential coherence for your study and for the paper you are writing to report the study.
You have full access to this open access chapter, Download chapter PDF
Part I. What Are Theoretical Frameworks?
As the name implies, a theoretical framework is a type of theory. We will define it as the custom-made theory that focuses specifically on the hypotheses you want to test and the research questions you want to answer. It is custom-made for your study because it explains why your predictions are plausible. It does no more and no less. Building directly on Chap. 2 , as you develop more complete rationales for your predictions, you are actually building a theory to support your predictions. Our goal in this chapter is for you to become comfortable with what theoretical frameworks are, with how they relate to the general concept of theory, with what role they play in scientific inquiry, and with why and how to create one for your study.
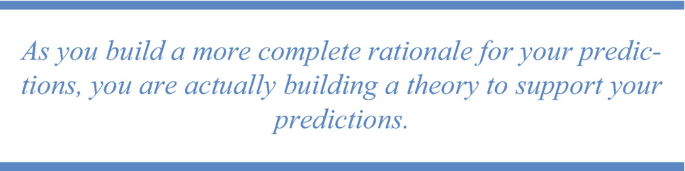
As you read this chapter, it will be helpful to remember that our definitions of terms in this book, such as theoretical framework, are based on our view of scientific inquiry as formulating, testing, and revising hypotheses. We define theoretical framework in ways that continue the coherent story we lay out across all phases of scientific inquiry and all the chapters this book. You are likely to find descriptions of theoretical frameworks in other sources that differ in some ways from our description. In addition, you are likely to see other terms that we would include as synonyms for theoretical framework, including conceptual framework. We suggest that when you encounter these special terms, make sure you understand how the authors are defining them.
Definitions of Theories
We begin by stepping back and considering how theoretical frameworks fit within the concept of theory, as usually defined. There are many definitions of theory; you can find a huge number simply by googling “theory.” Educational researchers and theorists often propose their own definitions but many of these are quite similar. Praetorius and Charalambous ( 2022 ) reviewed a number of definitions to set the stage for examining theories of teaching. Here are a few, beginning with a dictionary definition:
Lexico.com Dictionary (Oxford University Press, 2021 ): “A supposition or a system of ideas intended to explain something, especially one based on general principles independent of the thing to be explained.”
Biddle and Anderson ( 1986 ): “By scientific theory we mean the system of concepts and propositions that is used to represent, think about, and predict observable events. Within a mature science that theory is also explanatory and formalized. It does not represent ultimate ‘truth,’ however; indeed, it will be superseded by other theories presently. Instead, it represents the best explanation we have, at present, for those events we have so far observed” (p. 241).
Kerlinger ( 1964 ): “A theory is a set of interrelated constructs (concepts), definitions and propositions which presents a systematic view of phenomena by specifying relations among variables, with the purpose of explaining and predicting phenomena” (p. 11).
Colquitt and Zapata-Phelan ( 2007 ): The authors say that theories allow researchers to understand and predict outcomes of interest, describe and explain a process or sequence of events, raise consciousness about a specific set of concepts as well as prevent scholars from “being dazzled by the complexity of the empirical world by providing a linguistic tool for organizing it” (p. 1281).
For our purposes, it is important to notice two things that most definitions of theories share: They are descriptions of a connected set of facts and concepts, and they are created to predict and/or explain observed events. You can connect these ideas to Chaps. 1 and 2 by noticing that the language for the descriptors of scientific inquiry we suggested in Chap. 1 are reflected in the definitions of theories. In particular, notice in the definitions two of the descriptors: “Observing something and trying to explain why it is the way it is” and “Updating everyone’s thinking in response to more and better information.” Notice also in the definitions the emphasis on the elements of a theory similar to the elements of a rationale described in Chap. 2 : definitions, variables, and mechanisms that explain relationships.
Exercise 3.1
Before you continue reading, in your own words, write down a definition for “theoretical framework.”
Theoretical Frameworks Are Local Theories
There are strong similarities between building theories and doing scientific inquiry (formulating, testing, and revising hypotheses). In both cases, the researcher (or theorist) develops explanations for phenomena of interest. Building theories involves describing the concepts and conjectures that predict and later explain the events, and specifying the predictions by identifying the variables that will be measured. Doing scientific inquiry involves many of the same activities: formulating predictions for answers to questions about the research problem and building rationales to explain why the predictions are appropriate and reasonable.
As you move through the cycles described in Chap. 2 —cycles of asking questions, making predictions, writing out the reasons for these predictions, imagining how you would test the predictions, reading more about what scholars know and have hypothesized, revising your predictions (and maybe your questions), and so on—your theoretical rationales will become both more complete and more precise. They will become more complete as you find new arguments and new data in the literature and through talking with others, and they will become sharper as you remove parts of the rationales that originally seemed relevant but now create mostly distractions and noise. They will become increasingly customized local theories that support your predictions.
In the end, your framework should be as clean and frugal as possible without missing arguments or data that are directly relevant. In the language of mathematics, you should use an idea if and only if it makes your framework stronger, more convincing. On the one hand, including more than you need becomes a distraction and can confuse both you, as you try to conceptualize and conduct your research, and others, as they read your reports of your research. On the other hand, including less than you need means your rationale is not yet as convincing as it could be.
The set of rationales, blended together, constitute a precisely targeted custom-made theory that supports your predictions. Custom designing your rationales for your specific predictions means you probably will be drawing ideas from lots of sources and combining them in new ways. You are likely to end up with a unique local theory, a theoretical framework that has not been proposed in exactly the same way before.
A common misconception among beginning researchers is that they should borrow a theoretical framework from somewhere else, especially from well-known scholars who have theories named after them or well-known general theories of learning or teaching. You are likely to use ideas from these theories (e.g., Vygotsky’s theory of learning, Maslow’s theory of motivation, constructivist theories of learning), but you will combine specific ideas from multiple sources to create your own framework. When someone asks, “What theoretical framework are you using?” you would not say, “A Vygotskian framework.” Rather, you would say something like, “I created my framework by combining ideas from different sources so it explains why I am making these predictions.”

You should think of your theoretical framework as a potential contribution to the field, all on its own. Although it is unique to your study, there are elements of your framework that other researchers could draw from to construct theoretical frameworks for their studies, just as you drew from others’ frameworks. In rare cases, other researchers could use your framework as is. This might happen if they want to replicate your study or extend it in very specific ways. Usually, however, researchers borrow parts of frameworks or modify them in ways that better fit their own studies. And, just as you are doing with your own theoretical framework, those researchers will need to justify why borrowing or modifying parts of your framework will help them explain the predictions they are making.
Considering your theoretical framework as a contribution to the field means you should treat it as a central part of scientific inquiry, not just as a required step that must be completed before moving to the next phase. To be useful, the theoretical framework should be constructed as a critical part of conceptualizing and carrying out the research (Cai et al., 2019c ). This also means you should write out your framework as you are developing it. This will be a key part of your evolving research paper. Because your framework will be adjusted multiple times, your written document will go through many drafts.
If you are a graduate student, do not think of the potential audience for your written framework as only your advisor and committee members. Rather, consider your audience to be the larger community of education researchers. You will need to be sure all the key terms are defined and each part of your argument is clear, even to those who are not familiar with your study. This is one place where writing out your framework can benefit your study—it is easy to assume key terms are clear, but then you find out they are not so clear, even to you, when trying to communicate them. Failing to notice this lack of clarity can create lots of problems down the road.
Exercise 3.2
Researchers have used a number of different metaphors to describe theoretical frameworks. Maxwell (2005) referred to a theoretical framework as a “coat closet” that provides “places to ‘hang’ data, showing their relationship to other data,” although he cautioned that “a theory that neatly organizes some data will leave other data disheveled and lying on the floor, with no place to put them” (p. 49). Lester (2005) referred to a framework as a “scaffold” (p. 458), and others have called it a “blueprint” (Grant & Osanloo, 2014). Eisenhart (1991) described the framework as a “skeletal structure of justification” (p. 209). Spangler and Williams (2019) drew an analogy to the role that a house frame provides in preventing the house from collapsing in on itself. What aspects of a theoretical framework does each of these metaphors capture? What aspects does each fail to capture? Which metaphor do you find best fits your definition of a theoretical framework? Why? Can you think of another metaphor to describe a theoretical framework?
Part II. Why Do You Need Theoretical Frameworks?
Theoretical frameworks do lots of work for you. They have four primary purposes. They ensure (1) you have sound reasons to expect your predictions will be accurate, (2) you will craft appropriate methods to test your predictions, (3) you can interpret appropriately what you find, and (4) your interpretations will contribute to the accumulation of a knowledge base that can improve education. How do they do this?
Supporting Your Predictions
In previous chapters and earlier in this chapter, we described how theoretical frameworks are built along with your predictions. In fact, the rationales you develop for convincing others (and yourself) that your predictions are accurate are used to refine your predictions, and vice versa. So, it is not surprising that your refined framework provides a rationale that is fully aligned with your predictions. In fact, you could think of your theoretical framework as your best explanation, at any given moment during scientific inquiry, for why you will find what you think you will find.
Throughout this book, we are using “explanation” in a broad sense. As we noted earlier, an explanation for why your predictions are accurate includes all the concepts and definitions about mechanisms (Kerlinger’s, 1964 definition of “theory”) that help you describe why you think the predictions you are making are the best predictions possible. The explanation also identifies and describes all the variables that make up your predictions, variables that will be measured to test your predictions.
Crafting Appropriate Methods
Critical decisions you make to test your hypotheses form the methods for your scientific inquiry. As we have noted, imagining how you will test your hypotheses helps you decide whether the empirical observations you make can be compared with your predictions or whether you need to revise the methods (or your predictions). Remember, the theoretical framework is the coherent argument built from the rationales you develop as part of each hypothesis you formulate. Because each rationale explains why you make that prediction, it contains helpful cues for which methods would provide the fairest and most complete test of that prediction. In fact, your theoretical framework provides a logic against which you can check every aspect of the methods you imagine using.
You might find it helpful to ask yourself two questions as you think about which methods are best aligned with your theoretical framework. One is, “After reading my theoretical framework, will anyone be surprised by the methods I use?” If so, you should look back at your framework and make sure the predictions are clear and the rationales include all the reasons for your predictions. Your framework should telegraph the methods that make the most sense. The other question is, “Are there some predictions for which I can’t imagine appropriate methods?” If so, we recommend you return to your hypotheses—to your predictions and rationales (theoretical framework)—to make sure the predictions are phrased as precisely as possible and your framework is fully developed. In most cases, this will help you imagine methods that could be used. If not, you might need to revise your hypotheses.
Exercise 3.3
Kerlinger ( 1964 ) stated, “A theory is a set of interrelated constructs (concepts), definitions and propositions which presents a systematic view of phenomena by specifying relations among variables, with the purpose of explaining and predicting phenomena” (p. 11). What role do definitions play in a theoretical framework and how do they help in crafting appropriate methods?
Exercise 3.4
Sarah is in the beginning stages of developing a study. Her initial prediction is: There is a relationship between pedagogical content knowledge and ambitious teaching. She realizes that in order to craft appropriate measures, she needs to develop definitions of these constructs. Sarah’s original definitions are: Pedagogical content knowledge is knowledge about subject matter that is relevant to teaching. Ambitious teaching is teaching that is responsive to students’ thinking and develops a deep knowledge of content. Sarah recognizes that her prediction and her definitions are too broad and too general to work with. She wants to refine the definitions so they can guide the refinement of her prediction and the design of the study. Develop definitions of these two constructs that have clearer implications for the design and that would help Sarah to refine her prediction. (tip: Sarah may need to reduce the scope of her prediction by choosing to focus only on one aspect of pedagogical content knowledge and one aspect of ambitious teaching. Then, she can more precisely define those aspects.)
Guiding Interpretations of the Data
By providing rationales for your predictions, your theoretical framework explains why you think your predictions will be accurate. In education, researchers almost always find that if they make specific predictions (which they should), the predictions are not entirely accurate. This is a consequence of the fact that theoretical frameworks are never complete. Recall the definition of theories from Biddle and Anderson ( 1986 ): A theory “does not represent ultimate ‘truth,’ however; indeed, it will be superseded by other theories presently. Instead, it represents the best explanation we have, at present, for those events we have so far observed” (p. 241). If you have created your best developed and clearly stated theoretical framework that explains why you expected certain results, you can focus your interpretation on the ways in which your theoretical framework should be revised.
Focusing on realigning your theoretical framework with the data you collected produces the richest interpretation of your results. And it prevents you from making one of the most common errors of beginning researchers (and veteran researchers, as well): claiming that your results say more than they really do. Without this anchor to ground your interpretation of the data, it is easy to overgeneralize and make claims that go beyond the evidence.
In one of the definitions of theory presented earlier, Colquitt and Zapata-Phelan ( 2007 ) say that theories prevent scholars from “being dazzled by the complexity of the empirical world” (p. 1281). Theoretical frameworks keep researchers grounded by setting parameters within which the empirical world can be interpreted.
Exercise 3.5
Find two published articles that explicitly present theoretical frameworks (not all articles do). Where do you see evidence of the researchers using their theoretical frameworks to inform, shape, and connect other parts of their articles?
Showing the Contribution of Your Study
Theoretical frameworks contain the arguments that define the contribution of research studies. They do this in two ways, by showing how your study extends what is known and by setting the parameters for your contribution.
Showing How Your Study Extends What Is Known
Because your theoretical framework is built from what is already known or has been proposed, it situates your study in work that has occurred before. A clearly written framework shows readers how your study will take advantage of what is known to extend it further. It reveals what is new about what you are studying. The predictions that are generated from your framework are predictions that have never been made in quite the same way. They predict you will find something that has not been found previously in exactly this way. Your theoretical framework allows others to see the contributions that your study is likely to make even before the study has been conducted.
Setting the Parameters for Your Contribution
Earlier we noted that theoretical frameworks keep researchers grounded by setting parameters within which they should interpret their data. They do this by providing an initial explanation for why researchers expect to find particular results. The explanation is custom-built for each study. This means it uniquely explains the expected results. The results will almost surely turn out somewhat differently than predicted. Interpreting the data includes revising the initial explanation. So, you will end up with two versions of your theoretical framework, one that explains what you expected to find plus a second, updated framework that explains what you actually found.
The two frameworks—the initial version and the updated version—define the parameters of your study’s contribution. The difference between the two frameworks is what can be learned from your study. The first framework is a claim about what is known before you conduct your study about the phenomenon you are studying; the updated framework is a claim about how what is known has changed based on your results. It is the new aspects of the updated framework that capture the important contribution of your work.
Here is a brief example. Suppose you study the errors fourth graders make after receiving ordinary instruction on adding and subtracting decimal fractions. Based on empirical findings from past research, on theories of student learning, and on your own experience, you develop a rationale which predicts that a common error on “ragged” addition problems will be adding the wrong numerals. One of the reasons for this prediction is that students are likely to ignore the values of the digit positions and “line up” the numerals as they do with whole numbers. For instance, if they are asked to add 53.2 + .16, they are likely to answer either 5.48 or 54.8.
When you conduct your study, you present problems, handwritten, in both horizontal and vertical form. The horizontal form presents the numbers using the format shown above. The vertical form places one numeral over the other but not carefully aligned:
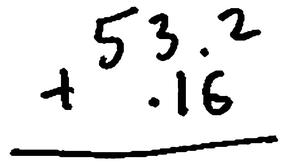
You find the predicted error occurs, but only for problems written in vertical form. To interpret these data, you look back at your theoretical framework and realize that students might ignore the value of the digits if the format reminded them of the way they lined up digits for whole number addition but might consider the value of the digits if they are forced to align the digits themselves, either by rewriting the problem or by just adding in their heads. A measure of what you (and others) learned from this study is the change in possible explanations (your theoretical frameworks). This does not mean your updated theoretical framework is “correct” or will make perfectly accurate predictions next time. But, it does mean that you are very likely moving toward more accurate predictions and toward a deeper understanding of how students think about adding decimal fractions.
Anchoring the Coherence of Your Study (and Your Evolving Research Paper)
Your theoretical framework serves as the anchor or center point around which all other aspects of your study should be aligned. This does not mean it is created first or that all other aspects are changed to align with the framework after it is created. The framework also changes as other aspects are considered. However, it is useful to always check alignment by beginning with the framework and asking whether other aspects are aligned and, if not, adjusting one or the other. This process of checking alignment is equally true when writing your evolving research paper as when planning and conducting your study.
Part III. How Do You Construct a Theoretical Framework for Your Study?
How do you start the process? Because constructing a theoretical framework is a natural extension of constructing rationales for your predictions, you already started as soon as you began formulating hypotheses: making predictions for what you will find and writing down reasons for why you are making these predictions. In Chap. 2 , we talked about beginning this process. In this section, we will explore how you can continue building out your rationales into a full-fledged theoretical framework.
Building a Theoretical Framework in Phases
Building your framework will occur in phases and proceed through cycles of clarifying your questions, making more precise and explicit your predictions, articulating reasons for making these predictions, and imagining ways of testing the predictions. The major source for ideas that will shape the framework is the research literature. That said, conversations with colleagues and other experts can help clarify your predictions and the rationales you develop to justify the predictions.
As you read relevant literature, you can ask: What have researchers found that help me predict what I will find? How have they explained their findings, and how might those explanations help me develop reasons for my predictions? Are there new ways to interpret past results so they better inform my predictions? Are there ways to look across previous results (and claims) and see new patterns that I can use to refine my predictions and enrich my rationales? How can theories that have credibility in the research community help me understand what I might find and help me explain why this is the case? As we have said, this process will go back and forth between clarifying your predictions, adjusting your rationales, reading, clarifying more, adjusting, reading, and so on.
One Researcher’s Experience Constructing a Theoretical Framework: The Continuing Case of Martha
In Chap. 2 , we followed Martha, a doctoral student in mathematics education, as she was working out the topic for her study, asking questions she wanted to answer, predicting the answers, and developing rationales for these predictions. Our story concluded with a research question, a sample set of predictions, and some reasons for Martha’s predictions. The question was: “Under what conditions do middle school teachers who lack conceptual knowledge of linear functions benefit from five 2-hour learning opportunity (LO) sessions that engage them in conceptual learning of linear functions as assessed by changes in their teaching toward a more conceptual emphasis of linear functions?” Her predictions focused on particular conditions that would affect the outcomes in particular ways. She was beginning to build rationales for these predictions by returning to the literature and identifying previous research and theory that were relevant. We continue the story here.
Imagine Martha continuing to read as she develops her theoretical framework—the rationales for her predictions. She tweaks some of her predictions based on what other researchers have already found. As she continues reading, she comes across some related literature on learning opportunities for teachers. A number of articles describe the potential of another form of LOs that might help teachers teach mathematics more conceptually—analyzing videos of mathematics lessons.
The data suggested that teachers can improve their teaching by analyzing videos of other teachers’ lessons as well as their own. However, the results were mixed so researchers did not seem to know exactly what makes the difference. Martha also read that teachers who already can analyze videos of lessons and spontaneously describe the mathematics that students are struggling with and offer useful suggestions for how to improve learning opportunities for students teach toward more conceptual learning goals, and their students learn more (Kersting et al., 2010 , 2012 ). These findings caught Martha’s attention because it is unusual to find correlates with conceptual teaching and better achievement. What is not known, realized Martha, is whether teachers who learn to analyze videos in this way, through specially designed LOs, would look like the teachers who already could analyze them. Would teachers who learned to analyze videos teach more conceptually?
It occurred to Martha she could bring these lines of research together by extending what is known along both lines. Recall our earlier suggestion of looking across the literature and noticing new patterns that can inform your work. Martha thought about studying how, exactly, these two skills are related: analyzing videos in particular ways and teaching conceptually. Would the relationships reported in the literature hold up for teachers who learn to describe the mathematics students are struggling with and make useful suggestions for improving students’ LOs?
Martha was now conflicted. She was well on her way to developing a testable hypothesis about the effects of learning about linear functions, but she was really intrigued by the work on analyzing videos of teaching. In addition, she saw several advantages of switching to this new topic:
The research question could be formulated quite easily. It would be something like: “What are the relationships between learning to analyze videos of mathematics teaching in particular ways (specified from prior research) and teaching for conceptual understanding?”
She could imagine predicting the answers to this question based directly on previous research. She would predict connections between particular kinds of analysis skills and levels of conceptual teaching of mathematics in ways that employed these skills.
The level of conceptual teaching, a challenging construct to define with her previous topic (the effects of professional development on the teaching of linear functions), was already defined in the work on analyzing videos of mathematics teaching, so that would solve a big problem. The definition foregrounded particular sets of behaviors and skills such as identifying key learning moments in a lesson and focusing on students’ thinking about the key mathematical idea during these moments. In other words, Martha saw ways to adapt a definition that had already been used and tested.
The issue of transfer—another challenging issue in her original hypothesis—was addressed more directly in this setting because the learning environment—analyzing videos of classroom teaching—is quite close to the classroom environment in which participants’ conceptual teaching would be observed.
Finally, the nature of learning opportunities, an aspect of her original idea she still needed to work through, had been explored in previous studies on this new topic, and connections were found between studying videos and changes in teaching.
Given all these advantages, Martha decided to change her topic and her research question. We applaud this decision for two major reasons. First, Martha’s interest grew as she explored this new topic. She became excited about conducting a study that might answer the research question she posed. It is always good to be passionate about what you study. Second, Martha was more likely to contribute important new insights if she could extend what is already known rather than explore a new area. Exploring something quite new requires lots of effort defining terms, creating measures, making new predictions, developing reasons for the predictions, and so on. Sometimes, exploring a new area has payoffs. But, as a beginning researcher, we suggest you take advantage of work that has already been done and extend it in creative ways.
Although Martha’s idea of extending previous work came with real advantages, she still faced a number of challenges. A first, major challenge was to decide whether she could build a rationale that would predict learning to analyze videos caused more conceptual teaching. Or, could she only build a rationale that would predict that there was a relationship between changes in analyzing videos and level of conceptual teaching? Perhaps a cause-effect relationship existed but in the opposite direction: If teachers learned to teach more conceptually, their analysis of teaching videos would improve. Although most of the literature described learning to analyze videos as the potential cause of teaching conceptually, Martha did not believe there was sufficient evidence to build a rationale for this prediction. Instead, she decided to first determine if a relationship existed and, if so, to understand the relationship. Then, if warranted, she could develop and test a hypothesis of causation in a future study. In fact, the direction of the causation might become clearer when she understood the relationship more clearly.
A second major challenge was whether to study the relationship as it existed or as one (or both) of the constructs was changing. Past research had explored the relationship as it existed, without inducing changes in either analyzing videos or teaching conceptually. So, Martha decided she could learn more about the relationship if one of the constructs was changing in a planned way. Because researchers had argued that teachers’ analysis of video could be changed with appropriate LOs, and because changing teachers’ teaching practices has resisted simple interventions, Martha decided to study the relationship as she facilitated changes in teachers’ analysis of videos. This would require gathering data on the relationship at more than one point in time.
Even after resolving these thorny issues, Martha faced many additional challenges. Should she predict a closer relationship between learning to analyze video and teaching for conceptual understanding before teachers began learning to analyze videos or after? Perhaps the relationship increases over time because conceptual teaching often changes slowly. Should she predict a closer relationship if the content of the videos teachers analyzed was the same as the content they would be teaching? Should she predict the relationship will be similar across pairs of similar topics? Should she predict that some analysis skills will show closer relationships to levels of conceptual teaching than others? These questions and others occurred to Martha as she was formulating her predictions, developing justifications for her predictions, and considering how she would test the predictions.
Based on her reading and discussions with colleagues, Martha phrased her initial predictions as follows:
There will be a significant positive correlation between teachers’ performance on analysis of videos and the extent to which they create conceptual learning opportunities for their students both before and after proposed learning experiences.
The relationship will be stronger:
Before the proposed opportunities to learn to analyze videos of teaching;
When the videos and the instruction are about similar mathematical topics; and,
When the videos analyzed display conceptual misunderstandings among students.
Of the video analysis skills that will be assessed, the two that will show the strongest relationship are spontaneously describing (1) the mathematics that students are struggling with and (2) useful suggestions for how to improve the conceptual learning opportunities for students.
Martha’s rationales for these predictions—her theoretical framework—evolved along with her predictions. We will not detail the framework here, but we will note that the rationale for the first prediction was based on findings from past research. In particular, the prediction is generated by reasoning that if there has been no special intervention, the tendency to analyze videos in particular ways and to teach conceptually develop together. This might explain Kersting’s findings described earlier. The second and third predictions were based on the literature on teachers’ learning, especially their learning from analyzing videos of teaching.
Before leaving Martha at this point in her journey, we want to make an important point about the change she made to her research topic. Changes like this occur quite often as researchers do the hard intellectual work of developing testable hypotheses that guide research studies. When this happens to you, it can feel like you have lost ground. You might feel like you wasted your time on the original topic. In Chap. 1 , we described inevitable “failure” when engaged in scientific inquiry. Failure is often associated with realizing the data you collected do not come close to supporting your predictions. But a common kind of failure occurs when researchers realize the direction they have been pursuing should change before they collect data. This happened in Martha’s case because she came across a topic that was more intriguing to her and because it helped solve some problems she was facing with the previous topic. This is an example of “failing productively” (see Chap. 1 ). Martha did not succeed in pursuing her original idea, but while she was recognizing the problems, she was also seeing new possibilities.
Constantly Improving Your Framework
We will use Martha’s experience to be more specific about the back-and-forth process in which you will engage as you flesh out your framework. We mentioned earlier your review of the literature as a major source of ideas and evidence that will affect your framework.
Reviewing Published Empirical Evidence
One of the best sources for helping you specify your predictions are studies that have been conducted on related topics. The closer to your topic, the more helpful will be the evidence for anticipating what you will find. Many beginning researchers worry they will locate a study just like the one they are planning. This (almost) never happens. Your study will be different in some ways, and a study that is very similar to yours can be extraordinarily helpful in specifying your predictions. Be excited instead of terrified when you come across a study with a title similar to yours.
Try to locate all the published research that has been conducted on your topic. What does “on your topic” mean? How widely should you cast your net? There are no rules here; you will need to use your professional judgment. However, here is a general guide: If the study does not help you clarify your predictions, change your confidence in them, or strengthen your rationale, then it falls outside your net.
In addition to helping specify your predictions, prior research studies can be a goldmine for developing and strengthening your theoretical framework. How did researchers justify their predictions or explain why they found what they did? How can you use these ideas to support (or change) your own predictions?
By reading research on similar topics, you might also imagine ways of testing your predictions. Maybe you learn of ways you could design your study, measures you could use to collect data, or strategies you could use to analyze your data. As you find helpful ideas, you will want to keep track of where you found these ideas so you can cite the appropriate sources as you write drafts of your evolving research paper.
Examining Theories
You will read a wide range of theories that provide insights into why things might work like they do. When the phenomena addressed by the theory are similar to those you will study, the associated theories can help you think through your own predictions and why you are making them. Returning to Martha’s situation, she could benefit from reading theories on adult learning, especially teacher learning, on transferring knowledge from one setting to another, on professional development for teachers, on the role of videos in learning, on the knowledge needed to teach conceptually, and so on.
Focusing on Variables and Mechanisms
As you review the literature and search for evidence and ideas that could strengthen your predictions and rationales, it is useful to keep your eyes on two components: the variables you will attend to and the mechanisms that might explain the relationships between the variables. Predictions could be considered statements about expected behaviors of the variables. The theoretical framework could be thought of as a description of all the variables that will be deliberately attended to plus the mechanisms conjectured to account for these relationships.
In Martha’s case, the most obvious variables are the responses teachers give to questions about their analysis of the videos and the features observed in their teaching practices. The mechanism of primary interest is the (mental and social) process that transforms the skills, knowledge, and attention involved in analyzing videos into particular kinds of teaching practices—or vice versa. The definition of conceptual teaching she adopted from previous studies gave her a clue about the mechanisms—about how and why learning to analyze videos might affect classroom teaching. The definition included attending to key learning moments in a lesson and tracking students’ thinking during these moments. Martha predicted that if teachers learned to attend to these aspects of teaching when viewing videos, they might attend to them when planning and implementing their own teaching.
As Martha reviewed the literature, she identified a number of variables that might affect the likelihood and extent of this translation. Here are some examples: how well teachers understand the mathematics in the videos and the mathematics they will teach; the nature of the videos themselves; the number of opportunities teachers have to analyze videos and the ways in which these opportunities are structured; teachers’ analysis of videos and their teaching practices before the learning opportunities begin; and how much time they have to apply what they learn to their own teaching.
Martha identified these additional variables because she learned they might have a direct influence on the mechanisms that could explain the relationship between analyzing videos and teaching. Some variables might support these mechanisms, and some might interfere. Martha’s task at this point in her work is to identify and describe all the variables that could play a meaningful role in the outcome of her study. This means to identify each variable for which it is possible to establish a clear and direct connection between the variable and the relationship she planned to investigate. Using the outcome of this task, Martha then needs to update her description of the mechanisms that could account for the relationships she expects to see and review her predictions and theoretical framework with these variables and mechanisms in mind.
Exercise 3.6
Review the predictions that Martha made and identify the variables that play a role in these predictions. Even though you might not be immersed in this literature, think about the alignment between the variables included in the predictions and those that could impact the relationships in which Martha is interested. Are there other missing variables that should be included in her predictions?
How Do You Know When You Have Finished Building Your Theoretical Framework?
The question of when your theoretical framework is finished could be answered in several ways. First, it is never really finished. As you continue to write your evolving research paper, you will continue strengthening your framework. You might even refine the framework as you write the final draft of your paper, after you have collected and analyzed your data. Furthermore, if you do follow-up studies, you will continue to build your framework.
A second answer is that you should invest the time and effort to build a theoretical framework that is as finished as possible at each point in the research process. As you write each draft of your evolving research paper, you should feel as if you have the strongest, most robust rationale you can have for your current predictions. In other words, you should feel that with each succeeding draft you have finished building your framework, even though you are quite sure you have not.
A third answer addresses a common, related question: “How do I know when I have included enough ideas and borrowed from enough sources? Would including another idea or citing another source be useful?” The answer is that you should include only those ideas that contribute to building a stronger framework. When you wonder whether you should include another idea or reference, ask yourself whether doing so would make your framework stronger in all the ways we described earlier.
Exercise 3.7
In 2–3 pages (single spaced), write out the plan for your study. The plan should include your research questions, your predictions of the answers, your rationale for the predictions (i.e., your theoretical framework), and your imagined plan for testing the predictions. Be as explicit and precise as you can. Be sure you have identified the critical variables and described the mechanism(s) that could explain the phenomena, the relationships, and/or the changes you predict. Look back to see if the logic connecting the parts is obvious. Ask yourself whether the tests you plan are what anyone familiar with your framework would expect (i.e., there should be no surprises).
Part IV. Refining a Theoretical Framework: A Scholarly Dialogue
As we noted above, conversations with colleagues and other experts can help you refine your theoretical framework by clarifying your predictions and digging into the details of the rationales you develop to justify those predictions. This is as true for experienced researchers as it is for beginning researchers. The dialogue below is an example of how two colleagues, Adrian (A) and Corin (C), work together to gradually formulate a testable hypothesis. Some of their conversation will look familiar as they refine their prediction through multiple steps of discussion:
Narrowing the focus of their prediction.
Making their prediction more testable.
Being more specific about what they want to study.
Engaging their prediction in cycles of refinements.
Determining the appropriate level/grainsize of their prediction (zoom in, zoom out).
Adding more predictions.
Thinking about underlying mechanisms (i.e., what explains the relationships between their variables).
Putting their predictions on a continuum (going from black and white to grey).
In addition, they construct their theoretical framework to match their hypotheses through multiple steps:
Defining and rationalizing their variables.
Re-evaluating their initial rationales in response to changes in their initial predictions.
Asking themselves “why” questions about predictions and rationales.
Finding empirical evidence and theory that better supports their evolving predictions.
Keeping in mind what they are going to be measuring.
Making sure their rationales support each link in their chain of reasoning.
Identifying underlying mechanisms.
Making sure that statements are included in their rationale if and only if they directly support their predictions and are essential to the argument.
They begin with the following hypothesis:
Prediction: Students will exhibit more persistence in mathematical course taking in high school if they work in groups.
Brief Description of Rationale: When people work in groups, they feel more competent and learn better (Cohen & Lotan, 2014 ; Jansen, 2012). When people feel more competent, they persist in additional mathematical course taking (Bandura & Schunk, 1981 ; Dweck, 1986 ).
So, do we think this hypothesis is testable?
Well actually, who these students are is probably something we need to be more specific about.
Good point, and also, since Algebra 2 is the bridge to additional course taking (i.e., the first course students don’t have to take), perhaps we should target Algebra 2. How about if we change our prediction to the following: Algebra 2 students will exhibit more mathematical persistence in mathematical course taking in high school if they work in groups in Algebra 2.
Okay, but another problem is that it would take a long time to collect data that would inform a prediction about the courses students take, and over that amount of time I’m not sure we could even tell if groupwork was responsible. What if we limited our prediction to: Algebra 2 students will exhibit more mathematical persistence in Algebra 2 if they work in groups.
Good idea! But when we talk about persistence, do we mean students don’t quit, or that they don’t drop the course, or productively struggle during class, or turn in their homework, or is it something else we mean? To me, what would be testable about mathematical persistence would be persistence at the problem level, such as when students get stuck on a problem, but they don’t give up.
I agree. So, let’s predict the following: Algebra 2 students will exhibit more mathematical persistence in Algebra 2 when they get stuck on problems if they work in groups. That’s something I think we could test.
Yes, but I think we need to be even more specific about what we mean by mathematical persistence when students get stuck on problems.
Hmm, what if we focused specifically on mathematical persistence that involves staying engaged in trying to solve a problem for the duration of a problem-solving session or until the problem gets solved? But that also makes me wonder if we want to be focusing on persistence at the individual level or at the group level?
Umm, I think we should focus on persistence at the individual level, because that’s more consistent with our original interest in persistence in course taking, which is about individual students, not about groups.
Okay, that makes sense. So then how about this for a prediction: If Algebra 2 students work in groups, they will be more likely to stay engaged in trying to solve problems for the duration of a problem-solving session or until they solve the problem.
To this point in the dialogue, Adrian and Corin are developing a theoretical framework by sharpening what they mean by their prediction and making sure their prediction is testable. In the next part, they return to their original idea to make sure they have not strayed too far by making their prediction more precise. The dialogue illustrates how making predictions should support the goal of understanding the relationship between variables and the mechanisms for change.
Yes, I’m liking the way this prediction is evolving. However, I also feel like our prediction is now so focused that we’ve lost a bit of our initial idea of competence and learning, which is what we were initially interested in. Could we do something to bring those ideas back? Perhaps we could create more predictions to get at more of those ideas?
Great idea! Okay, so to help us see what we are missing now, let’s look back at the initial links in our chain of reasoning. We initially said that Working in Groups leads to Feeling Competent & Learning Better leads to Persistence in Math Course Taking. But our chain of reasoning has changed. I think it’s more like this: Working in Groups on Problems leads to Staying Engaged in Problem Solving leads to Greater Sense of Competence and Learning Better leads to More Persistence in Course Taking.
Okay, so if that’s the case, it looks like our new prediction just tests the first link in this chain, the link between Working in Groups on Problems and Staying Engaged in Problem Solving. It looks like there are three other potential predictions we could make; we could make a prediction about the relationship between Staying Engaged in Problem Solving and having a Greater Sense of Competence, between Staying Engaged in Problem Solving and Learning Better, and between having a Greater Sense of Competence/Learning Better and More Persistence in Course Taking.
Clearly that’s too many predictions for us to tackle in one study and actually I am aware of several studies that already address the third prediction. So, we can use those studies as part of our rationale and don’t need to study that link.
I agree. Let’s just add one prediction, one about the link between Staying Engaged and Sense of Competence. In our initial prediction, we just had a vague connection between Working in Groups and Sense of Competence. But in our new prediction, we were more specific that working in groups helps students stay engaged until the end of a problem-solving session. So, I guess we could say for a second prediction then that When Algebra 2 students stay engaged in problem solving until the end of a problem-solving session, they develop a greater sense of competence.
Okay so we will have two predictions to examine with our study: Prediction 1 is: If Algebra 2 students work in groups, they will be more likely to stay engaged in trying to solve problems for the duration of a problem-solving session or until they solve the problem. This prediction deals with the first link in our chain of reasoning. And then Prediction 2 is: If Algebra 2 students try to solve problems for the duration of a problem-solving session or until they solve the problem, they will be more likely to develop a sense of competence. Oh, as soon as I finished stating that prediction, the thought just came to me, “sense of competence about what?”
How about if we focused on sense of competence in being able to solve similar problems in the future? Actually, maybe that’s too limited. Maybe we should expand our prediction a bit more so we include a sense of competence that’s at least somewhat closer to more course taking? Something like sense of competence that involves feeling capable of understanding future Algebra 2 concepts. That’s at least bigger than sense of competence at solving similar problems. If students feel they’re capable of understanding future Algebra 2 concepts, then they will probably be more likely to persist in course taking too.
Okay, that makes sense. So, then our Prediction 2 could be: If Algebra 2 students try to solve problems for the duration of a problem-solving session or until they solve the problem, they will be more likely to feel they will be capable of understanding future Algebra 2 concepts.
Oh, I just had an additional idea! What if we changed the two predictions one more time to allow for more or less of the variables? For example, Prediction 1 could be: The more Algebra 2 students work in groups, the more likely they will stay engaged in trying to solve problems for the duration of a problem-solving session or until they solve the problem.
Yes, great. So, that would mean Prediction 2 could be: The more Algebra 2 students try to solve problems for the duration of a problem-solving session or until they solve the problem, the more likely they will feel they are capable of understanding future Algebra 2 concepts.
So, I think we’re happy with our predictions for now, but I think we need to work on our rationales for those predictions because they no longer apply very well.
Okay, to recap, our original chain of reasoning was Working in Groups leads to Feeling Competent & Learning Better leads to Persistence in Math Course Taking. Our initial rationales were the following: For the link between working in groups and feeling competent, we based that link on Cohen and Lotan’s ( 2014 ) book on Designing Groupwork, in which they explain why and how all students can feel competent through their engagement in groupwork. We also based this link on that 2012 Jansen study that found that groupwork helped students enact their competence in math. Then, for the link between competence and persistence, we based that link on the Bandura and Schunk ( 1981 ) study and on the work by Carol Dweck ( 1986 ) that show that children who feel more competent in arithmetic, tend to persist more.
Corin and Adrian have looked back at their initial research idea. In doing so, they illustrated how developing a theoretical framework involves developing and refining a chain of reasoning. They continue by working on developing rationales for their predictions.
Okay, so let’s think if any of our previous rationales still work. How about Elizabeth Cohen’s work? I still think her work applies because it shows that groupwork can affect engagement. But now that I think about it, another part of her work indicates that groupwork needs particular norms in order to be effective. So maybe we should tighten up our predictions to focus just on groupwork that has particular norms?
But, on the other hand, what about Jo Boaler’s ( 1998 ) “Open and Closed Mathematics” article? In that study, students at the Phoenix Park School did not have much structure, and in spite of that, groupwork worked quite well for those students, better than individual work did for students at the Amber Hill School who had highly structured instruction.
That’s a good point. So maybe we should leave our predictions about groupwork as is (i.e., not focus on particular norms). Also, the ideas in the Boaler article would be good to add to our theoretical framework because it deals with secondary students, which aligns better with the ages of the Algebra 2 students we are planning on studying.
Okay, so we’re adding the ideas in the Boaler article. I also think we need to find literature that specifies the kind of engagement we want to focus on. Looking at the engagement literature would sharpen our thinking about the engagement we are most interested in. We should consider Brigid Barron’s ( 2003 ) study, “When Smart Groups Fail.” In her study, students produced better products if they engaged with each other and with the content. But that makes me think that we are mostly just focused on the latter, namely on how individuals engage with the content.
I agree we’re focused on individuals’ engagement with the content. Come to think of it, the fact that we’re focused on how individuals engage with content rather than how groups engage further justifies why we’re not looking at groupwork norms. But let me ask a question we need to answer. Why are we focusing on how individuals engage with content? It’s not just a preference. It’s because we think individual engagement with content is related to feeling capable. So, our decision to focus on individual engagement aligns with our predictions. And even though we’re not including Barron’s work in our framework, considering her work helped sharpen our thinking about what we’re focusing on.
You know, we are kind of in a weird space because we’re focusing on individual engagement with content at the same time as we are predicting that groupwork leads to more engagement. In other words, we are and aren’t taking a social perspective. But what this reminds me of is how, from the perspective of the theory of constructivism, even though individuals have to make sense of things for themselves, social interactions are what drives sense making. In fact, here’s a quote from von Glasersfeld ( 1995 ): “Piaget has stressed many times that the most frequent cause of accommodation is the interaction” (p. 66). So, I think we can use constructivism as a theoretical justification for predicting that the social activity of groupwork is what is related to individual engagement with content.
Interesting! Yes, makes sense. When you were describing that, I had another insight from constructivism. You know how when someone experiences a perturbation, it also creates a need in them to resolve the perturbation, right? So maybe perturbations are the mechanism explaining why groupwork leads to more individual engagement with content. Groupwork potentially generates perturbations, meaning the person engages more to try to resolve those perturbations.
Okay, now that we have brought in the idea of perturbations as potentially being the mechanism that drives how working in groups leads to staying more engaged, perhaps we need to reconsider what we will be measuring in our study. Will it be perturbations, or will it be staying engaged that we should be measuring?
I think what we are saying is that the need to resolve perturbations is part of the underlying mechanism, but measuring the need to resolve perturbations would be difficult if not impossible. So, instead, I think we should focus on measuring the variable staying engaged , a variable we can measure. And then if we find that more working in groups leads to more staying engaged, that also gives us more evidence that our theoretical framework with perturbations as a mechanism is viable. In other words, mechanisms are part of our framework and by testing our prediction, we are testing our theoretical framework (i.e., our rationales) too.
This final part of the dialogue illustrates that the rationale for a study continues to develop as the predictions continue to be refined and testability continues to be considered. In other words, the development of the predictions and rationale (i.e., the theoretical framework) should be iterative and ongoing.
Through their discussion, Adrian and Corin have refined both their predictions and their rationales. In the process, the key ideas they have drawn on contributed to their rationales and thus to constructing their theoretical framework.
Part V. Distinctions Between Rationales, Theoretical Frameworks, and Literature Reviews
We have introduced a number of terms that play critical roles in the scientific inquiry process. Because they refer to related and sometimes overlapping ideas, keeping straight their meanings and uses can be challenging. It might be helpful to revisit each of them briefly to describe how they are similar to, and different from, each other.
To distinguish between rationales, theoretical frameworks, and literature reviews, it is useful to consider the roles they play as you plan and conduct a study compared to the roles they play when you write the report of your study.
Thinking Through a Study
The chronology of the thinking process often moves through many cycles of identifying a research problem or asking a question, and then reading the literature to learn more about the problem, and then refining and narrowing the scope of a question that would add to or extend what is known, and then predicting (guessing) an answer to the question and asking yourself why you predicted this answer and writing a first draft of your rationale, and then reading the literature to improve your rationale, and then realizing you can refine the question further along with specifying a clearer and more targeted prediction, and then reading the literature to further improve your rationale, and then realizing you can refine the question further along with a clearer and more targeted prediction, and so on.
The primary activity that generates more specific and clearer hypotheses is searching and reviewing literature . You can return to the literature as often as you need to build your rationales . As your rationales develop, they morph into your theoretical framework . The theoretical framework is a coherent argument that threads together the individual rationales and explains why your predictions are the best predictions the field can make at this time.
If you have one research question and one prediction you will have one rationale. In this case, your rationale is essentially the same as your theoretical framework. If you have more than one research question, you will have multiple predictions and multiple rationales. As you develop rationales for each prediction, you might find lots of overlap. Maybe the literatures you read to refine each prediction and develop each rationale overlap, and maybe the arguments you piece together include many of the same elements. Your theoretical framework emerges from weaving the rationales together into one coherent argument. Although this process is more complicated than the thinking process for one prediction, it is more common. If you find few connections among the rationales for each prediction, we recommend stepping back and asking whether you are conducting more than one study. It might make more sense to sort the questions into two or more studies because the rationales for the predicted answers are drawing from different literatures.
Writing the Evolving Research Paper
We recommend that you write drafts of the research report as you think through your study and make decisions about how to proceed. Although your thinking will be fluid and evolving, we recommend that you follow the conventions of academic writing as you write drafts. For example, we recommend that you structure the paper using the five typical major sections of a journal article: introduction, theoretical framework, methods, results, and discussion. Each of these sections will go through multiple drafts as you plan your study, collect the data, analyze the data, and interpret the results.
In the introduction, you will present the research problem you are studying. This includes describing the problem, explaining why it is significant, defining the special terms you use, and often presenting the research questions you will address along with the answers you predict. Sometimes the questions and predictions are part of the next section—the theoretical framework.
In the theoretical framework, you will present your best arguments for expecting the predicted answers to the research questions. You will not trace the many cycles in which you engaged to get to the best versions of your arguments but rather present the latest and best version. The report of a study does not describe the chronology of the back-and-forth messiness always involved in thinking through all aspects of the study. What you learned from reviewing the literature will be an integral part of your arguments. In other words, the review of research will be included in the presentation of your theoretical framework rather than in a separate section.
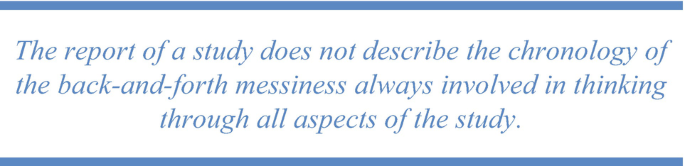
The literature you choose to include to present your theoretical framework is not all the literature you reviewed for conducting your study. Rather, the literature cited in your paper should be the literature that contributed to building your theoretical framework, and only that literature. In other words, the theoretical framework places the boundaries on what you should review in the paper.
Beginning researchers are often tempted to review much of what they read. Researchers put lots of time into reading, and leaving lots of it out when writing the paper can make all that reading feel like a waste of time. It is not a waste of time; it is always part of the research process. But, reviewing more than you need in the paper becomes a distraction and diverts the reader from the main points.
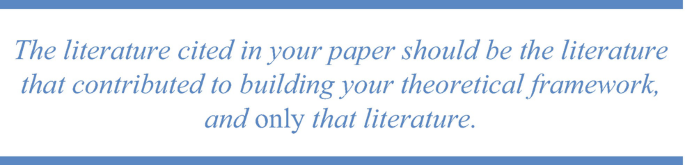
What should you do if the editor of the journal requires, or recommends, a section titled “review of research”? We recommend you create a somewhat more elaborated review for this section and then show exactly how you used the literature to build your rationale in the theoretical framework section.
Reviewers notice when the theoretical framework and the literature reviewed do not provide sufficient justification for the research questions (or the hypotheses). We found that about 13% of JRME reviews noted an especially important gap—the research questions in a paper were not sufficiently motivated. We expect the same would be true for other research journals. Reviewers also note when manuscripts either do not have an explicit theoretical framework or when they seem to be juggling more than one theoretical framework.
Part VI. Moving to Methods
A significant benefit of building rich and precise theoretical frameworks is the guidance they provide for selecting and creating the methods you will use to test your hypotheses. The next phase in the process of scientific inquiry is crafting your methods: choosing your research design, selecting your sample, developing your measures, deciding on your data analysis strategies, and so on. In Chap. 4 , we discuss how you can do this in ways that keep your story coherent.
Bandura, A., & Schunk, D. H. (1981). Cultivating competence, self-efficacy, and intrinsic interest through proximal self-motivation. Journal of Personality and Social Psychology, 41 , 586–598. https://doi.org/10.1037/0022-3514.41.3.586
Article Google Scholar
Barron, B. (2003). When smart groups fail. Journal of the Learning Sciences, 12 (3), 307–359. https://doi.org/10.1207/S15327809JLS1203_1
Biddle, B. J., & Anderson, D. S. (1986). Theory, methods, knowledge, and research on teaching. In M. C. Wittrock (Ed.), Handbook of research on teaching: A project of the American Educational Research Association . Macmillan.
Google Scholar
Boaler, J. (1998). Open and closed mathematics: Student experiences and understandings. Journal for Research in Mathematics Education, 29 , 41–62.
Cai, J., Morris, A., Hohensee, C., Hwang, S., Robison, V., Cirillo, M., Kramer, S. L., & Hiebert, J. (2019c). Theoretical framing as justifying. Journal for Research in Mathematics Education, 50 (3), 218–224. https://doi.org/10.5951/jresematheduc.50.3.0218
Cohen, E. G., & Lotan, R. A. (2014). Designing groupwork: Strategies for the heterogenous classroom (3rd ed.). Teachers College Press.
Colquitt, J. A., & Zapata-Phelan, C. P. (2007). Trends in theory building and theory testing: A five-decade study of the academy of management journal. The Academy of Management Journal, 50 (6), 1281–1303.
Dweck, C. S. (1986). Motivational processes affecting learning. American Psychologist, 41 , 1040–1048. https://doi.org/10.1037/0003-066X.41.10.1040
Kerlinger, F. N. (1964). Foundations of behavioral research: Educational and psychological inquiry . Holt, Rinehardt, & Winston.
Kersting, N. B., Givvin, K., Sotelo, F., & Stigler, J. W. (2010). Teacher’s analysis of classroom video predicts student learning of mathematics: Further explorations of a novel measure of teacher knowledge. Journal of Teacher Education , 61(1–2), 172–181. https://doi.org/10.1177/0022487109347875 , 172.
Kersting, N. B., Givvin, K. B., Thompson, B., Santagata, R., & Stigler, J. (2012). Developing measures of usable knowledge: Teachers’ analyses of mathematics classroom videos predict teaching quality and student learning. American Educational Research Journal, 49 (3), 568–590. https://doi.org/10.3102/0002831212437853
Oxford University Press. (2021). Theory. In Lexico.com dictionary . Lexico.com . https://www.lexico.com/en/definition/theory
Praetorius, A.-K., & Charalambous, C. (2022). Moving the field forward with respect to theorizing teaching: An introduction. In A.-K. Praetorius & C. Charalmabous (Eds.), Expert perspectives on theorizing teaching: Current status, problematic issues, and aspirations . Springer.
von Glasersfeld, E. (1995). Radical constructivism: A way of knowing and learning . Falmer Press.
Download references
Author information
Authors and affiliations.
School of Education, University of Delaware, Newark, DE, USA
James Hiebert, Anne K Morris & Charles Hohensee
Department of Mathematical Sciences, University of Delaware, Newark, DE, USA
Jinfa Cai & Stephen Hwang
You can also search for this author in PubMed Google Scholar
Rights and permissions
Open Access This chapter is licensed under the terms of the Creative Commons Attribution 4.0 International License ( http://creativecommons.org/licenses/by/4.0/ ), which permits use, sharing, adaptation, distribution and reproduction in any medium or format, as long as you give appropriate credit to the original author(s) and the source, provide a link to the Creative Commons license and indicate if changes were made.
The images or other third party material in this chapter are included in the chapter's Creative Commons license, unless indicated otherwise in a credit line to the material. If material is not included in the chapter's Creative Commons license and your intended use is not permitted by statutory regulation or exceeds the permitted use, you will need to obtain permission directly from the copyright holder.
Reprints and permissions
Copyright information
© 2023 The Author(s)
About this chapter
Hiebert, J., Cai, J., Hwang, S., Morris, A.K., Hohensee, C. (2023). Building and Using Theoretical Frameworks. In: Doing Research: A New Researcher’s Guide. Research in Mathematics Education. Springer, Cham. https://doi.org/10.1007/978-3-031-19078-0_3
Download citation
DOI : https://doi.org/10.1007/978-3-031-19078-0_3
Published : 03 December 2022
Publisher Name : Springer, Cham
Print ISBN : 978-3-031-19077-3
Online ISBN : 978-3-031-19078-0
eBook Packages : Education Education (R0)
Share this chapter
Anyone you share the following link with will be able to read this content:
Sorry, a shareable link is not currently available for this article.
Provided by the Springer Nature SharedIt content-sharing initiative
- Publish with us
Policies and ethics
- Find a journal
- Track your research
- Bipolar Disorder
- Therapy Center
- When To See a Therapist
- Types of Therapy
- Best Online Therapy
- Best Couples Therapy
- Best Family Therapy
- Managing Stress
- Sleep and Dreaming
- Understanding Emotions
- Self-Improvement
- Healthy Relationships
- Student Resources
- Personality Types
- Guided Meditations
- Verywell Mind Insights
- 2023 Verywell Mind 25
- Mental Health in the Classroom
- Editorial Process
- Meet Our Review Board
- Crisis Support
How to Write a Great Hypothesis
Hypothesis Format, Examples, and Tips
Kendra Cherry, MS, is a psychosocial rehabilitation specialist, psychology educator, and author of the "Everything Psychology Book."
:max_bytes(150000):strip_icc():format(webp)/IMG_9791-89504ab694d54b66bbd72cb84ffb860e.jpg)
Amy Morin, LCSW, is a psychotherapist and international bestselling author. Her books, including "13 Things Mentally Strong People Don't Do," have been translated into more than 40 languages. Her TEDx talk, "The Secret of Becoming Mentally Strong," is one of the most viewed talks of all time.
:max_bytes(150000):strip_icc():format(webp)/VW-MIND-Amy-2b338105f1ee493f94d7e333e410fa76.jpg)
Verywell / Alex Dos Diaz
- The Scientific Method
Hypothesis Format
Falsifiability of a hypothesis, operational definitions, types of hypotheses, hypotheses examples.
- Collecting Data
Frequently Asked Questions
A hypothesis is a tentative statement about the relationship between two or more variables. It is a specific, testable prediction about what you expect to happen in a study.
One hypothesis example would be a study designed to look at the relationship between sleep deprivation and test performance might have a hypothesis that states: "This study is designed to assess the hypothesis that sleep-deprived people will perform worse on a test than individuals who are not sleep-deprived."
This article explores how a hypothesis is used in psychology research, how to write a good hypothesis, and the different types of hypotheses you might use.
The Hypothesis in the Scientific Method
In the scientific method , whether it involves research in psychology, biology, or some other area, a hypothesis represents what the researchers think will happen in an experiment. The scientific method involves the following steps:
- Forming a question
- Performing background research
- Creating a hypothesis
- Designing an experiment
- Collecting data
- Analyzing the results
- Drawing conclusions
- Communicating the results
The hypothesis is a prediction, but it involves more than a guess. Most of the time, the hypothesis begins with a question which is then explored through background research. It is only at this point that researchers begin to develop a testable hypothesis. Unless you are creating an exploratory study, your hypothesis should always explain what you expect to happen.
In a study exploring the effects of a particular drug, the hypothesis might be that researchers expect the drug to have some type of effect on the symptoms of a specific illness. In psychology, the hypothesis might focus on how a certain aspect of the environment might influence a particular behavior.
Remember, a hypothesis does not have to be correct. While the hypothesis predicts what the researchers expect to see, the goal of the research is to determine whether this guess is right or wrong. When conducting an experiment, researchers might explore a number of factors to determine which ones might contribute to the ultimate outcome.
In many cases, researchers may find that the results of an experiment do not support the original hypothesis. When writing up these results, the researchers might suggest other options that should be explored in future studies.
In many cases, researchers might draw a hypothesis from a specific theory or build on previous research. For example, prior research has shown that stress can impact the immune system. So a researcher might hypothesize: "People with high-stress levels will be more likely to contract a common cold after being exposed to the virus than people who have low-stress levels."
In other instances, researchers might look at commonly held beliefs or folk wisdom. "Birds of a feather flock together" is one example of folk wisdom that a psychologist might try to investigate. The researcher might pose a specific hypothesis that "People tend to select romantic partners who are similar to them in interests and educational level."
Elements of a Good Hypothesis
So how do you write a good hypothesis? When trying to come up with a hypothesis for your research or experiments, ask yourself the following questions:
- Is your hypothesis based on your research on a topic?
- Can your hypothesis be tested?
- Does your hypothesis include independent and dependent variables?
Before you come up with a specific hypothesis, spend some time doing background research. Once you have completed a literature review, start thinking about potential questions you still have. Pay attention to the discussion section in the journal articles you read . Many authors will suggest questions that still need to be explored.
To form a hypothesis, you should take these steps:
- Collect as many observations about a topic or problem as you can.
- Evaluate these observations and look for possible causes of the problem.
- Create a list of possible explanations that you might want to explore.
- After you have developed some possible hypotheses, think of ways that you could confirm or disprove each hypothesis through experimentation. This is known as falsifiability.
In the scientific method , falsifiability is an important part of any valid hypothesis. In order to test a claim scientifically, it must be possible that the claim could be proven false.
Students sometimes confuse the idea of falsifiability with the idea that it means that something is false, which is not the case. What falsifiability means is that if something was false, then it is possible to demonstrate that it is false.
One of the hallmarks of pseudoscience is that it makes claims that cannot be refuted or proven false.
A variable is a factor or element that can be changed and manipulated in ways that are observable and measurable. However, the researcher must also define how the variable will be manipulated and measured in the study.
For example, a researcher might operationally define the variable " test anxiety " as the results of a self-report measure of anxiety experienced during an exam. A "study habits" variable might be defined by the amount of studying that actually occurs as measured by time.
These precise descriptions are important because many things can be measured in a number of different ways. One of the basic principles of any type of scientific research is that the results must be replicable. By clearly detailing the specifics of how the variables were measured and manipulated, other researchers can better understand the results and repeat the study if needed.
Some variables are more difficult than others to define. How would you operationally define a variable such as aggression ? For obvious ethical reasons, researchers cannot create a situation in which a person behaves aggressively toward others.
In order to measure this variable, the researcher must devise a measurement that assesses aggressive behavior without harming other people. In this situation, the researcher might utilize a simulated task to measure aggressiveness.
Hypothesis Checklist
- Does your hypothesis focus on something that you can actually test?
- Does your hypothesis include both an independent and dependent variable?
- Can you manipulate the variables?
- Can your hypothesis be tested without violating ethical standards?
The hypothesis you use will depend on what you are investigating and hoping to find. Some of the main types of hypotheses that you might use include:
- Simple hypothesis : This type of hypothesis suggests that there is a relationship between one independent variable and one dependent variable.
- Complex hypothesis : This type of hypothesis suggests a relationship between three or more variables, such as two independent variables and a dependent variable.
- Null hypothesis : This hypothesis suggests no relationship exists between two or more variables.
- Alternative hypothesis : This hypothesis states the opposite of the null hypothesis.
- Statistical hypothesis : This hypothesis uses statistical analysis to evaluate a representative sample of the population and then generalizes the findings to the larger group.
- Logical hypothesis : This hypothesis assumes a relationship between variables without collecting data or evidence.
A hypothesis often follows a basic format of "If {this happens} then {this will happen}." One way to structure your hypothesis is to describe what will happen to the dependent variable if you change the independent variable .
The basic format might be: "If {these changes are made to a certain independent variable}, then we will observe {a change in a specific dependent variable}."
A few examples of simple hypotheses:
- "Students who eat breakfast will perform better on a math exam than students who do not eat breakfast."
- Complex hypothesis: "Students who experience test anxiety before an English exam will get lower scores than students who do not experience test anxiety."
- "Motorists who talk on the phone while driving will be more likely to make errors on a driving course than those who do not talk on the phone."
Examples of a complex hypothesis include:
- "People with high-sugar diets and sedentary activity levels are more likely to develop depression."
- "Younger people who are regularly exposed to green, outdoor areas have better subjective well-being than older adults who have limited exposure to green spaces."
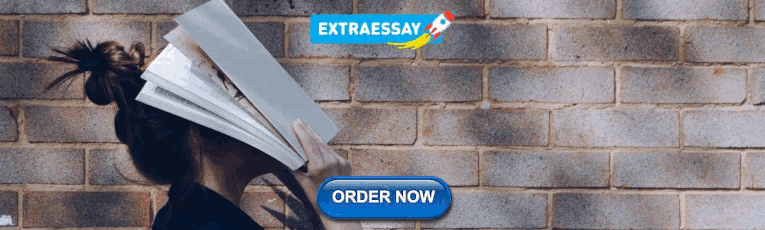
Examples of a null hypothesis include:
- "Children who receive a new reading intervention will have scores different than students who do not receive the intervention."
- "There will be no difference in scores on a memory recall task between children and adults."
Examples of an alternative hypothesis:
- "Children who receive a new reading intervention will perform better than students who did not receive the intervention."
- "Adults will perform better on a memory task than children."
Collecting Data on Your Hypothesis
Once a researcher has formed a testable hypothesis, the next step is to select a research design and start collecting data. The research method depends largely on exactly what they are studying. There are two basic types of research methods: descriptive research and experimental research.
Descriptive Research Methods
Descriptive research such as case studies , naturalistic observations , and surveys are often used when it would be impossible or difficult to conduct an experiment . These methods are best used to describe different aspects of a behavior or psychological phenomenon.
Once a researcher has collected data using descriptive methods, a correlational study can then be used to look at how the variables are related. This type of research method might be used to investigate a hypothesis that is difficult to test experimentally.
Experimental Research Methods
Experimental methods are used to demonstrate causal relationships between variables. In an experiment, the researcher systematically manipulates a variable of interest (known as the independent variable) and measures the effect on another variable (known as the dependent variable).
Unlike correlational studies, which can only be used to determine if there is a relationship between two variables, experimental methods can be used to determine the actual nature of the relationship—whether changes in one variable actually cause another to change.
A Word From Verywell
The hypothesis is a critical part of any scientific exploration. It represents what researchers expect to find in a study or experiment. In situations where the hypothesis is unsupported by the research, the research still has value. Such research helps us better understand how different aspects of the natural world relate to one another. It also helps us develop new hypotheses that can then be tested in the future.
Some examples of how to write a hypothesis include:
- "Staying up late will lead to worse test performance the next day."
- "People who consume one apple each day will visit the doctor fewer times each year."
- "Breaking study sessions up into three 20-minute sessions will lead to better test results than a single 60-minute study session."
The four parts of a hypothesis are:
- The research question
- The independent variable (IV)
- The dependent variable (DV)
- The proposed relationship between the IV and DV
Castillo M. The scientific method: a need for something better? . AJNR Am J Neuroradiol. 2013;34(9):1669-71. doi:10.3174/ajnr.A3401
Nevid J. Psychology: Concepts and Applications. Wadworth, 2013.
By Kendra Cherry, MSEd Kendra Cherry, MS, is a psychosocial rehabilitation specialist, psychology educator, and author of the "Everything Psychology Book."
Have a language expert improve your writing
Run a free plagiarism check in 10 minutes, generate accurate citations for free.
- Knowledge Base
Hypothesis Testing | A Step-by-Step Guide with Easy Examples
Published on November 8, 2019 by Rebecca Bevans . Revised on June 22, 2023.
Hypothesis testing is a formal procedure for investigating our ideas about the world using statistics . It is most often used by scientists to test specific predictions, called hypotheses, that arise from theories.
There are 5 main steps in hypothesis testing:
- State your research hypothesis as a null hypothesis and alternate hypothesis (H o ) and (H a or H 1 ).
- Collect data in a way designed to test the hypothesis.
- Perform an appropriate statistical test .
- Decide whether to reject or fail to reject your null hypothesis.
- Present the findings in your results and discussion section.
Though the specific details might vary, the procedure you will use when testing a hypothesis will always follow some version of these steps.
Table of contents
Step 1: state your null and alternate hypothesis, step 2: collect data, step 3: perform a statistical test, step 4: decide whether to reject or fail to reject your null hypothesis, step 5: present your findings, other interesting articles, frequently asked questions about hypothesis testing.
After developing your initial research hypothesis (the prediction that you want to investigate), it is important to restate it as a null (H o ) and alternate (H a ) hypothesis so that you can test it mathematically.
The alternate hypothesis is usually your initial hypothesis that predicts a relationship between variables. The null hypothesis is a prediction of no relationship between the variables you are interested in.
- H 0 : Men are, on average, not taller than women. H a : Men are, on average, taller than women.
Receive feedback on language, structure, and formatting
Professional editors proofread and edit your paper by focusing on:
- Academic style
- Vague sentences
- Style consistency
See an example
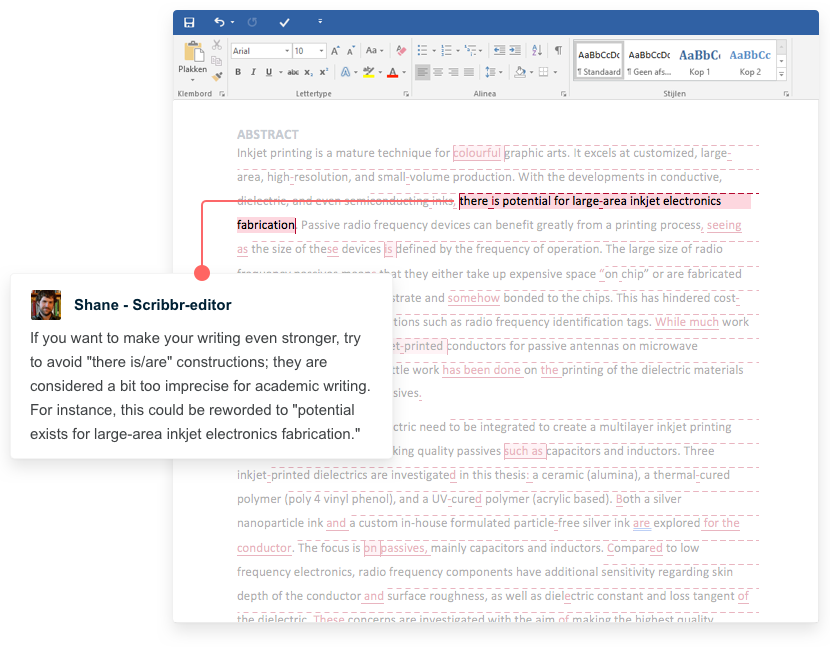
For a statistical test to be valid , it is important to perform sampling and collect data in a way that is designed to test your hypothesis. If your data are not representative, then you cannot make statistical inferences about the population you are interested in.
There are a variety of statistical tests available, but they are all based on the comparison of within-group variance (how spread out the data is within a category) versus between-group variance (how different the categories are from one another).
If the between-group variance is large enough that there is little or no overlap between groups, then your statistical test will reflect that by showing a low p -value . This means it is unlikely that the differences between these groups came about by chance.
Alternatively, if there is high within-group variance and low between-group variance, then your statistical test will reflect that with a high p -value. This means it is likely that any difference you measure between groups is due to chance.
Your choice of statistical test will be based on the type of variables and the level of measurement of your collected data .
- an estimate of the difference in average height between the two groups.
- a p -value showing how likely you are to see this difference if the null hypothesis of no difference is true.
Based on the outcome of your statistical test, you will have to decide whether to reject or fail to reject your null hypothesis.
In most cases you will use the p -value generated by your statistical test to guide your decision. And in most cases, your predetermined level of significance for rejecting the null hypothesis will be 0.05 – that is, when there is a less than 5% chance that you would see these results if the null hypothesis were true.
In some cases, researchers choose a more conservative level of significance, such as 0.01 (1%). This minimizes the risk of incorrectly rejecting the null hypothesis ( Type I error ).
Prevent plagiarism. Run a free check.
The results of hypothesis testing will be presented in the results and discussion sections of your research paper , dissertation or thesis .
In the results section you should give a brief summary of the data and a summary of the results of your statistical test (for example, the estimated difference between group means and associated p -value). In the discussion , you can discuss whether your initial hypothesis was supported by your results or not.
In the formal language of hypothesis testing, we talk about rejecting or failing to reject the null hypothesis. You will probably be asked to do this in your statistics assignments.
However, when presenting research results in academic papers we rarely talk this way. Instead, we go back to our alternate hypothesis (in this case, the hypothesis that men are on average taller than women) and state whether the result of our test did or did not support the alternate hypothesis.
If your null hypothesis was rejected, this result is interpreted as “supported the alternate hypothesis.”
These are superficial differences; you can see that they mean the same thing.
You might notice that we don’t say that we reject or fail to reject the alternate hypothesis . This is because hypothesis testing is not designed to prove or disprove anything. It is only designed to test whether a pattern we measure could have arisen spuriously, or by chance.
If we reject the null hypothesis based on our research (i.e., we find that it is unlikely that the pattern arose by chance), then we can say our test lends support to our hypothesis . But if the pattern does not pass our decision rule, meaning that it could have arisen by chance, then we say the test is inconsistent with our hypothesis .
If you want to know more about statistics , methodology , or research bias , make sure to check out some of our other articles with explanations and examples.
- Normal distribution
- Descriptive statistics
- Measures of central tendency
- Correlation coefficient
Methodology
- Cluster sampling
- Stratified sampling
- Types of interviews
- Cohort study
- Thematic analysis
Research bias
- Implicit bias
- Cognitive bias
- Survivorship bias
- Availability heuristic
- Nonresponse bias
- Regression to the mean
Hypothesis testing is a formal procedure for investigating our ideas about the world using statistics. It is used by scientists to test specific predictions, called hypotheses , by calculating how likely it is that a pattern or relationship between variables could have arisen by chance.
A hypothesis states your predictions about what your research will find. It is a tentative answer to your research question that has not yet been tested. For some research projects, you might have to write several hypotheses that address different aspects of your research question.
A hypothesis is not just a guess — it should be based on existing theories and knowledge. It also has to be testable, which means you can support or refute it through scientific research methods (such as experiments, observations and statistical analysis of data).
Null and alternative hypotheses are used in statistical hypothesis testing . The null hypothesis of a test always predicts no effect or no relationship between variables, while the alternative hypothesis states your research prediction of an effect or relationship.
Cite this Scribbr article
If you want to cite this source, you can copy and paste the citation or click the “Cite this Scribbr article” button to automatically add the citation to our free Citation Generator.
Bevans, R. (2023, June 22). Hypothesis Testing | A Step-by-Step Guide with Easy Examples. Scribbr. Retrieved April 3, 2024, from https://www.scribbr.com/statistics/hypothesis-testing/
Is this article helpful?
Rebecca Bevans
Other students also liked, choosing the right statistical test | types & examples, understanding p values | definition and examples, what is your plagiarism score.
Hypothesis Testing Framework
Now that we've seen an example and explored some of the themes for hypothesis testing, let's specify the procedure that we will follow.
Hypothesis Testing Steps
The formal framework and steps for hypothesis testing are as follows:
- Identify and define the parameter of interest
- Define the competing hypotheses to test
- Set the evidence threshold, formally called the significance level
- Generate or use theory to specify the sampling distribution and check conditions
- Calculate the test statistic and p-value
- Evaluate your results and write a conclusion in the context of the problem.
We'll discuss each of these steps below.
Identify Parameter of Interest
First, I like to specify and define the parameter of interest. What is the population that we are interested in? What characteristic are we measuring?
By defining our population of interest, we can confirm that we are truly using sample data. If we find that we actually have population data, our inference procedures are not needed. We could proceed by summarizing our population data.
By identifying and defining the parameter of interest, we can confirm that we use appropriate methods to summarize our variable of interest. We can also focus on the specific process needed for our parameter of interest.
In our example from the last page, the parameter of interest would be the population mean time that a host has been on Airbnb for the population of all Chicago listings on Airbnb in March 2023. We could represent this parameter with the symbol $\mu$. It is best practice to fully define $\mu$ both with words and symbol.
Define the Hypotheses
For hypothesis testing, we need to decide between two competing theories. These theories must be statements about the parameter. Although we won't have the population data to definitively select the correct theory, we will use our sample data to determine how reasonable our "skeptic's theory" is.
The first hypothesis is called the null hypothesis, $H_0$. This can be thought of as the "status quo", the "skeptic's theory", or that nothing is happening.
Examples of null hypotheses include that the population proportion is equal to 0.5 ($p = 0.5$), the population median is equal to 12 ($M = 12$), or the population mean is equal to 14.5 ($\mu = 14.5$).
The second hypothesis is called the alternative hypothesis, $H_a$ or $H_1$. This can be thought of as the "researcher's hypothesis" or that something is happening. This is what we'd like to convince the skeptic to believe. In most cases, the desired outcome of the researcher is to conclude that the alternative hypothesis is reasonable to use moving forward.
Examples of alternative hypotheses include that the population proportion is greater than 0.5 ($p > 0.5$), the population median is less than 12 ($M < 12$), or the population mean is not equal to 14.5 ($\mu \neq 14.5$).
There are a few requirements for the hypotheses:
- the hypotheses must be about the same population parameter,
- the hypotheses must have the same null value (provided number to compare to),
- the null hypothesis must have the equality (the equals sign must be in the null hypothesis),
- the alternative hypothesis must not have the equality (the equals sign cannot be in the alternative hypothesis),
- there must be no overlap between the null and alternative hypothesis.
You may have previously seen null hypotheses that include more than an equality (e.g. $p \le 0.5$). As long as there is an equality in the null hypothesis, this is allowed. For our purposes, we will simplify this statement to ($p = 0.5$).
To summarize from above, possible hypotheses statements are:
$H_0: p = 0.5$ vs. $H_a: p > 0.5$
$H_0: M = 12$ vs. $H_a: M < 12$
$H_0: \mu = 14.5$ vs. $H_a: \mu \neq 14.5$
In our second example about Airbnb hosts, our hypotheses would be:
$H_0: \mu = 2100$ vs. $H_a: \mu > 2100$.
Set Threshold (Significance Level)
There is one more step to complete before looking at the data. This is to set the threshold needed to convince the skeptic. This threshold is defined as an $\alpha$ significance level. We'll define exactly what the $\alpha$ significance level means later. For now, smaller $\alpha$s correspond to more evidence being required to convince the skeptic.
A few common $\alpha$ levels include 0.1, 0.05, and 0.01.
For our Airbnb hosts example, we'll set the threshold as 0.02.
Determine the Sampling Distribution of the Sample Statistic
The first step (as outlined above) is the identify the parameter of interest. What is the best estimate of the parameter of interest? Typically, it will be the sample statistic that corresponds to the parameter. This sample statistic, along with other features of the distribution will prove especially helpful as we continue the hypothesis testing procedure.
However, we do have a decision at this step. We can choose to use simulations with a resampling approach or we can choose to rely on theory if we are using proportions or means. We then also need to confirm that our results and conclusions will be valid based on the available data.
Required Condition
The one required assumption, regardless of approach (resampling or theory), is that the sample is random and representative of the population of interest. In other words, we need our sample to be a reasonable sample of data from the population.
Using Simulations and Resampling
If we'd like to use a resampling approach, we have no (or minimal) additional assumptions to check. This is because we are relying on the available data instead of assumptions.
We do need to adjust our data to be consistent with the null hypothesis (or skeptic's claim). We can then rely on our resampling approach to estimate a plausible sampling distribution for our sample statistic.
Recall that we took this approach on the last page. Before simulating our estimated sampling distribution, we adjusted the mean of the data so that it matched with our skeptic's claim, shown in the code below.
We'll see a few more examples on the next page.
Using Theory
On the other hand, we could rely on theory in order to estimate the sampling distribution of our desired statistic. Recall that we had a few different options to rely on:
- the CLT for the sampling distribution of a sample mean
- the binomial distribution for the sampling distribution of a proportion (or count)
- the Normal approximation of a binomial distribution (using the CLT) for the sampling distribution of a proportion
If relying on the CLT to specify the underlying sampling distribution, you also need to confirm:
- having a random sample and
- having a sample size that is less than 10% of the population size if the sampling is done without replacement
- having a Normally distributed population for a quantitative variable OR
- having a large enough sample size (usually at least 25) for a quantitative variable
- having a large enough sample size for a categorical variable (defined by $np$ and $n(1-p)$ being at least 10)
If relying on the binomial distribution to specify the underlying sampling distribution, you need to confirm:
- having a set number of trials, $n$
- having the same probability of success, $p$ for each observation
After determining the appropriate theory to use, we should check our conditions and then specify the sampling distribution for our statistic.
For the Airbnb hosts example, we have what we've assumed to be a random sample. It is not taken with replacement, so we also need to assume that our sample size (700) is less than 10% of our population size. In other words, we need to assume that the population of Chicago Airbnbs in March 2023 was at least 7000. Since we do have our (presumed) population data available, we can confirm that there were at least 7000 Chicago Airbnbs in the population in 2023.
Additionally, we can confirm that normality of the sampling distribution applies for the CLT to apply. Our sample size is more than 25 and the parameter of interest is a mean, so this meets our necessary criteria for the normality condition to be valid.
With the conditions now met, we can estimate our sampling distribution. From the CLT, we know that the distribution for the sample mean should be $\bar{X} \sim N(\mu, \frac{\sigma}{\sqrt{n}})$.
Now, we face our next challenge -- what to plug in as the mean and standard error for this distribution. Since we are adopting the skeptic's point of view for the purpose of this approach, we can plug in the value of $\mu_0 = 2100$. We also know that the sample size $n$ is 700. But what should we plug in for the population standard deviation $\sigma$?
When we don't know the value of a parameter, we will generally plug in our best estimate for the parameter. In this case, that corresponds to plugging in $\hat{\sigma}$, or our sample standard deviation.
Now, our estimated sampling distribution based on the CLT is: $\bar{X} \sim N(2100, 41.4045)$.
If we compare to our corresponding skeptic's sampling distribution on the last page, we can confirm that the theoretical sampling distribution is similar to the simulated sampling distribution based on resampling.
Assumptions not met
What do we do if the necessary conditions aren't met for the sampling distribution? Because the simulation-based resampling approach has minimal assumptions, we should be able to use this approach to produce valid results as long as the provided data is representative of the population.
The theory-based approach has more conditions, and we may not be able to meet all of the necessary conditions. For example, if our parameter is something other than a mean or proportion, we may not have appropriate theory. Additionally, we may not have a large enough sample size.
- First, we could consider changing approaches to the simulation-based one.
- Second, we might look at how we could meet the necessary conditions better. In some cases, we may be able to redefine groups or make adjustments so that the setup of the test is closer to what is needed.
- As a last resort, we may be able to continue following the hypothesis testing steps. In this case, your calculations may not be valid or exact; however, you might be able to use them as an estimate or an approximation. It would be crucial to specify the violation and approximation in any conclusions or discussion of the test.
Calculate the evidence with statistics and p-values
Now, it's time to calculate how much evidence the sample contains to convince the skeptic to change their mind. As we saw above, we can convince the skeptic to change their mind by demonstrating that our sample is unlikely to occur if their theory is correct.
How do we do this? We do this by calculating a probability associated with our observed value for the statistic.
For example, for our situation, we want to convince the skeptic that the population mean is actually greater than 2100 days. We do that by calculating the probability that a sample mean would be as large or larger than what we observed in our actual sample, which was 2188 days. Why do we need the larger portion? We use the larger portion because a sample mean of 2200 days also provides evidence that the population mean is larger than 2100 days; it isn't limited to exactly what we observed in our sample. We call this specific probability the p-value.
That is, the p-value is the probability of observing a test statistic as extreme or more extreme (as determined by the alternative hypothesis), assuming the null hypothesis is true.
Our observed p-value for the Airbnb host example demonstrates that the probability of getting a sample mean host time of 2188 days (the value from our sample) or more is 1.46%, assuming that the true population mean is 2100 days.
Test statistic
Notice that the formal definition of a p-value mentions a test statistic . In most cases, this word can be replaced with "statistic" or "sample" for an equivalent statement.
Oftentimes, we'll see that our sample statistic can be used directly as the test statistic, as it was above. We could equivalently adjust our statistic to calculate a test statistic. This test statistic is often calculated as:
$\text{test statistic} = \frac{\text{estimate} - \text{hypothesized value}}{\text{standard error of estimate}}$
P-value Calculation Options
Note also that the p-value definition includes a probability associated with a test statistic being as extreme or more extreme (as determined by the alternative hypothesis . How do we determine the area that we consider when calculating the probability. This decision is determined by the inequality in the alternative hypothesis.
For example, when we were trying to convince the skeptic that the population mean is greater than 2100 days, we only considered those sample means that we at least as large as what we observed -- 2188 days or more.
If instead we were trying to convince the skeptic that the population mean is less than 2100 days ($H_a: \mu < 2100$), we would consider all sample means that were at most what we observed - 2188 days or less. In this case, our p-value would be quite large; it would be around 99.5%. This large p-value demonstrates that our sample does not support the alternative hypothesis. In fact, our sample would encourage us to choose the null hypothesis instead of the alternative hypothesis of $\mu < 2100$, as our sample directly contradicts the statement in the alternative hypothesis.
If we wanted to convince the skeptic that they were wrong and that the population mean is anything other than 2100 days ($H_a: \mu \neq 2100$), then we would want to calculate the probability that a sample mean is at least 88 days away from 2100 days. That is, we would calculate the probability corresponding to 2188 days or more or 2012 days or less. In this case, our p-value would be roughly twice the previously calculated p-value.
We could calculate all of those probabilities using our sampling distributions, either simulated or theoretical, that we generated in the previous step. If we chose to calculate a test statistic as defined in the previous section, we could also rely on standard normal distributions to calculate our p-value.
Evaluate your results and write conclusion in context of problem
Once you've gathered your evidence, it's now time to make your final conclusions and determine how you might proceed.
In traditional hypothesis testing, you often make a decision. Recall that you have your threshold (significance level $\alpha$) and your level of evidence (p-value). We can compare the two to determine if your p-value is less than or equal to your threshold. If it is, you have enough evidence to persuade your skeptic to change their mind. If it is larger than the threshold, you don't have quite enough evidence to convince the skeptic.
Common formal conclusions (if given in context) would be:
- I have enough evidence to reject the null hypothesis (the skeptic's claim), and I have sufficient evidence to suggest that the alternative hypothesis is instead true.
- I do not have enough evidence to reject the null hypothesis (the skeptic's claim), and so I do not have sufficient evidence to suggest the alternative hypothesis is true.
The only decision that we can make is to either reject or fail to reject the null hypothesis (we cannot "accept" the null hypothesis). Because we aren't actively evaluating the alternative hypothesis, we don't want to make definitive decisions based on that hypothesis. However, when it comes to making our conclusion for what to use going forward, we frame this on whether we could successfully convince someone of the alternative hypothesis.
A less formal conclusion might look something like:
Based on our sample of Chicago Airbnb listings, it seems as if the mean time since a host has been on Airbnb (for all Chicago Airbnb listings) is more than 5.75 years.
Significance Level Interpretation
We've now seen how the significance level $\alpha$ is used as a threshold for hypothesis testing. What exactly is the significance level?
The significance level $\alpha$ has two primary definitions. One is that the significance level is the maximum probability required to reject the null hypothesis; this is based on how the significance level functions within the hypothesis testing framework. The second definition is that this is the probability of rejecting the null hypothesis when the null hypothesis is true; in other words, this is the probability of making a specific type of error called a Type I error.
Why do we have to be comfortable making a Type I error? There is always a chance that the skeptic was originally correct and we obtained a very unusual sample. We don't want to the skeptic to be so convinced of their theory that no evidence can convince them. In this case, we need the skeptic to be convinced as long as the evidence is strong enough . Typically, the probability threshold will be low, to reduce the number of errors made. This also means that a decent amount of evidence will be needed to convince the skeptic to abandon their position in favor of the alternative theory.
p-value Limitations and Misconceptions
In comparison to the $\alpha$ significance level, we also need to calculate the evidence against the null hypothesis with the p-value.
The p-value is the probability of getting a test statistic as extreme or more extreme (in the direction of the alternative hypothesis), assuming the null hypothesis is true.
Recently, p-values have gotten some bad press in terms of how they are used. However, that doesn't mean that p-values should be abandoned, as they still provide some helpful information. Below, we'll describe what p-values don't mean, and how they should or shouldn't be used to make decisions.
Factors that affect a p-value
What features affect the size of a p-value?
- the null value, or the value assumed under the null hypothesis
- the effect size (the difference between the null value under the null hypothesis and the true value of the parameter)
- the sample size
More evidence against the null hypothesis will be obtained if the effect size is larger and if the sample size is larger.
Misconceptions
We gave a definition for p-values above. What are some examples that p-values don't mean?
- A p-value is not the probability that the null hypothesis is correct
- A p-value is not the probability that the null hypothesis is incorrect
- A p-value is not the probability of getting your specific sample
- A p-value is not the probability that the alternative hypothesis is correct
- A p-value is not the probability that the alternative hypothesis is incorrect
- A p-value does not indicate the size of the effect
Our p-value is a way of measuring the evidence that your sample provides against the null hypothesis, assuming the null hypothesis is in fact correct.
Using the p-value to make a decision
Why is there bad press for a p-value? You may have heard about the standard $\alpha$ level of 0.05. That is, we would be comfortable with rejecting the null hypothesis once in 20 attempts when the null hypothesis is really true. Recall that we reject the null hypothesis when the p-value is less than or equal to the significance level.
Consider what would happen if you have two different p-values: 0.049 and 0.051.
In essence, these two p-values represent two very similar probabilities (4.9% vs. 5.1%) and very similar levels of evidence against the null hypothesis. However, when we make our decision based on our threshold, we would make two different decisions (reject and fail to reject, respectively). Should this decision really be so simplistic? I would argue that the difference shouldn't be so severe when the sample statistics are likely very similar. For this reason, I (and many other experts) strongly recommend using the p-value as a measure of evidence and including it with your conclusion.
Putting too much emphasis on the decision (and having a significant result) has created a culture of misusing p-values. For this reason, understanding your p-value itself is crucial.
Searching for p-values
The other concern with setting a definitive threshold of 0.05 is that some researchers will begin performing multiple tests until finding a p-value that is small enough. However, with a p-value of 0.05, we know that we will have a p-value less than 0.05 1 time out of every 20 times, even when the null hypothesis is true.
This means that if researchers start hunting for p-values that are small (sometimes called p-hacking), then they are likely to identify a small p-value every once in a while by chance alone. Researchers might then publish that result, even though the result is actually not informative. For this reason, it is recommended that researchers write a definitive analysis plan to prevent performing multiple tests in search of a result that occurs by chance alone.
Best Practices
With all of this in mind, what should we do when we have our p-value? How can we prevent or reduce misuse of a p-value?
- Report the p-value along with the conclusion
- Specify the effect size (the value of the statistic)
- Define an analysis plan before looking at the data
- Interpret the p-value clearly to specify what it indicates
- Consider using an alternate statistical approach, the confidence interval, discussed next, when appropriate

An official website of the United States government
The .gov means it’s official. Federal government websites often end in .gov or .mil. Before sharing sensitive information, make sure you’re on a federal government site.
The site is secure. The https:// ensures that you are connecting to the official website and that any information you provide is encrypted and transmitted securely.
- Publications
- Account settings
Preview improvements coming to the PMC website in October 2024. Learn More or Try it out now .
- Advanced Search
- Journal List
- R Soc Open Sci
- v.10(8); 2023 Aug
- PMC10465209
On the scope of scientific hypotheses
William hedley thompson.
1 Department of Applied Information Technology, University of Gothenburg, Gothenburg, Sweden
2 Institute of Neuroscience and Physiology, Sahlgrenska Academy, University of Gothenburg, Gothenburg, Sweden
3 Department of Pedagogical, Curricular and Professional Studies, Faculty of Education, University of Gothenburg, Gothenburg, Sweden
4 Department of Clinical Neuroscience, Karolinska Institutet, Stockholm, Sweden
Associated Data
This article has no additional data.
Hypotheses are frequently the starting point when undertaking the empirical portion of the scientific process. They state something that the scientific process will attempt to evaluate, corroborate, verify or falsify. Their purpose is to guide the types of data we collect, analyses we conduct, and inferences we would like to make. Over the last decade, metascience has advocated for hypotheses being in preregistrations or registered reports, but how to formulate these hypotheses has received less attention. Here, we argue that hypotheses can vary in specificity along at least three independent dimensions: the relationship, the variables, and the pipeline. Together, these dimensions form the scope of the hypothesis. We demonstrate how narrowing the scope of a hypothesis in any of these three ways reduces the hypothesis space and that this reduction is a type of novelty. Finally, we discuss how this formulation of hypotheses can guide researchers to formulate the appropriate scope for their hypotheses and should aim for neither too broad nor too narrow a scope. This framework can guide hypothesis-makers when formulating their hypotheses by helping clarify what is being tested, chaining results to previous known findings, and demarcating what is explicitly tested in the hypothesis.
1. Introduction
Hypotheses are an important part of the scientific process. However, surprisingly little attention is given to hypothesis-making compared to other skills in the scientist's skillset within current discussions aimed at improving scientific practice. Perhaps this lack of emphasis is because the formulation of the hypothesis is often considered less relevant, as it is ultimately the scientific process that will eventually decide the veracity of the hypothesis. However, there are more hypotheses than scientific studies as selection occurs at various stages: from funder selection and researcher's interests. So which hypotheses are worthwhile to pursue? Which hypotheses are the most effective or pragmatic for extending or enhancing our collective knowledge? We consider the answer to these questions by discussing how broad or narrow a hypothesis can or should be (i.e. its scope).
We begin by considering that the two statements below are both hypotheses and vary in scope:
- H 1 : For every 1 mg decrease of x , y will increase by, on average, 2.5 points.
- H 2 : Changes in x 1 or x 2 correlate with y levels in some way.
Clearly, the specificity of the two hypotheses is very different. H 1 states a precise relationship between two variables ( x and y ), while H 2 specifies a vaguer relationship and does not specify which variables will show the relationship. However, they are both still hypotheses about how x and y relate to each other. This claim of various degrees of the broadness of hypotheses is, in and of itself, not novel. In Epistemetrics, Rescher [ 1 ], while drawing upon the physicist Duhem's work, develops what he calls Duhem's Law. This law considers a trade-off between certainty or precision in statements about physics when evaluating them. Duhem's Law states that narrower hypotheses, such as H 1 above, are more precise but less likely to be evaluated as true than broader ones, such as H 2 above. Similarly, Popper, when discussing theories, describes the reverse relationship between content and probability of a theory being true, i.e. with increased content, there is a decrease in probability and vice versa [ 2 ]. Here we will argue that it is important that both H 1 and H 2 are still valid scientific hypotheses, and their appropriateness depends on certain scientific questions.
The question of hypothesis scope is relevant since there are multiple recent prescriptions to improve science, ranging from topics about preregistrations [ 3 ], registered reports [ 4 ], open science [ 5 ], standardization [ 6 ], generalizability [ 7 ], multiverse analyses [ 8 ], dataset reuse [ 9 ] and general questionable research practices [ 10 ]. Within each of these issues, there are arguments to demarcate between confirmatory and exploratory research or normative prescriptions about how science should be done (e.g. science is ‘bad’ or ‘worse’ if code/data are not open). Despite all these discussions and improvements, much can still be done to improve hypothesis-making. A recent evaluation of preregistered studies in psychology found that over half excluded the preregistered hypotheses [ 11 ]. Further, evaluations of hypotheses in ecology showed that most hypotheses are not explicitly stated [ 12 , 13 ]. Other research has shown that obfuscated hypotheses are more prevalent in retracted research [ 14 ]. There have been recommendations for simpler hypotheses in psychology to avoid misinterpretations and misspecifications [ 15 ]. Finally, several evaluations of preregistration practices have found that a significant proportion of articles do not abide by their stated hypothesis or add additional hypotheses [ 11 , 16 – 18 ]. In sum, while multiple efforts exist to improve scientific practice, our hypothesis-making could improve.
One of our intentions is to provide hypothesis-makers with tools to assist them when making hypotheses. We consider this useful and timely as, with preregistrations becoming more frequent, the hypothesis-making process is now open and explicit . However, preregistrations are difficult to write [ 19 ], and preregistered articles can change or omit hypotheses [ 11 ] or they are vague and certain degrees of freedom hard to control for [ 16 – 18 ]. One suggestion has been to do less confirmatory research [ 7 , 20 ]. While we agree that all research does not need to be confirmatory, we also believe that not all preregistrations of confirmatory work must test narrow hypotheses. We think there is a possible point of confusion that the specificity in preregistrations, where researcher degrees of freedom should be stated, necessitates the requirement that the hypothesis be narrow. Our belief that this confusion is occurring is supported by the study Akker et al . [ 11 ] where they found that 18% of published psychology studies changed their preregistered hypothesis (e.g. its direction), and 60% of studies selectively reported hypotheses in some way. It is along these lines that we feel the framework below can be useful to help formulate appropriate hypotheses to mitigate these identified issues.
We consider this article to be a discussion of the researcher's different choices when formulating hypotheses and to help link hypotheses over time. Here we aim to deconstruct what aspects there are in the hypothesis about their specificity. Throughout this article, we intend to be neutral to many different philosophies of science relating to the scientific method (i.e. how one determines the veracity of a hypothesis). Our idea of neutrality here is that whether a researcher adheres to falsification, verification, pragmatism, or some other philosophy of science, then this framework can be used when formulating hypotheses. 1
The framework this article advocates for is that there are (at least) three dimensions that hypotheses vary along regarding their narrowness and broadness: the selection of relationships, variables, and pipelines. We believe this discussion is fruitful for the current debate regarding normative practices as some positions make, sometimes implicit, commitments about which set of hypotheses the scientific community ought to consider good or permissible. We proceed by outlining a working definition of ‘scientific hypothesis' and then discuss how it relates to theory. Then, we justify how hypotheses can vary along the three dimensions. Using this framework, we then discuss the scopes in relation to appropriate hypothesis-making and an argument about what constitutes a scientifically novel hypothesis. We end the article with practical advice for researchers who wish to use this framework.
2. The scientific hypothesis
In this section, we will describe a functional and descriptive role regarding how scientists use hypotheses. Jeong & Kwon [ 21 ] investigated and summarized the different uses the concept of ‘hypothesis’ had in philosophical and scientific texts. They identified five meanings: assumption, tentative explanation, tentative cause, tentative law, and prediction. Jeong & Kwon [ 21 ] further found that researchers in science and philosophy used all the different definitions of hypotheses, although there was some variance in frequency between fields. Here we see, descriptively , that the way researchers use the word ‘hypothesis’ is diverse and has a wide range in specificity and function. However, whichever meaning a hypothesis has, it aims to be true, adequate, accurate or useful in some way.
Not all hypotheses are ‘scientific hypotheses'. For example, consider the detective trying to solve a crime and hypothesizing about the perpetrator. Such a hypothesis still aims to be true and is a tentative explanation but differs from the scientific hypothesis. The difference is that the researcher, unlike the detective, evaluates the hypothesis with the scientific method and submits the work for evaluation by the scientific community. Thus a scientific hypothesis entails a commitment to evaluate the statement with the scientific process . 2 Additionally, other types of hypotheses can exist. As discussed in more detail below, scientific theories generate not only scientific hypotheses but also contain auxiliary hypotheses. The latter refers to additional assumptions considered to be true and not explicitly evaluated. 3
Next, the scientific hypothesis is generally made antecedent to the evaluation. This does not necessitate that the event (e.g. in archaeology) or the data collection (e.g. with open data reuse) must be collected before the hypothesis is made, but that the evaluation of the hypothesis cannot happen before its formulation. This claim state does deny the utility of exploratory hypothesis testing of post hoc hypotheses (see [ 25 ]). However, previous results and exploration can generate new hypotheses (e.g. via abduction [ 22 , 26 – 28 ], which is the process of creating hypotheses from evidence), which is an important part of science [ 29 – 32 ], but crucially, while these hypotheses are important and can be the conclusion of exploratory work, they have yet to be evaluated (by whichever method of choice). Hence, they still conform to the antecedency requirement. A further way to justify the antecedency is seen in the practice of formulating a post hoc hypothesis, and considering it to have been evaluated is seen as a questionable research practice (known as ‘hypotheses after results are known’ or HARKing [ 33 ]). 4
While there is a varying range of specificity, is the hypothesis a critical part of all scientific work, or is it reserved for some subset of investigations? There are different opinions regarding this. Glass and Hall, for example, argue that the term only refers to falsifiable research, and model-based research uses verification [ 36 ]. However, this opinion does not appear to be the consensus. Osimo and Rumiati argue that any model based on or using data is never wholly free from hypotheses, as hypotheses can, even implicitly, infiltrate the data collection [ 37 ]. For our definition, we will consider hypotheses that can be involved in different forms of scientific evaluation (i.e. not just falsification), but we do not exclude the possibility of hypothesis-free scientific work.
Finally, there is a debate about whether theories or hypotheses should be linguistic or formal [ 38 – 40 ]. Neither side in this debate argues that verbal or formal hypotheses are not possible, but instead, they discuss normative practices. Thus, for our definition, both linguistic and formal hypotheses are considered viable.
Considering the above discussion, let us summarize the scientific process and the scientific hypothesis: a hypothesis guides what type of data are sampled and what analysis will be done. With the new observations, evidence is analysed or quantified in some way (often using inferential statistics) to judge the hypothesis's truth value, utility, credibility, or likelihood. The following working definition captures the above:
- Scientific hypothesis : an implicit or explicit statement that can be verbal or formal. The hypothesis makes a statement about some natural phenomena (via an assumption, explanation, cause, law or prediction). The scientific hypothesis is made antecedent to performing a scientific process where there is a commitment to evaluate it.
For simplicity, we will only use the term ‘hypothesis’ for ‘scientific hypothesis' to refer to the above definition for the rest of the article except when it is necessary to distinguish between other types of hypotheses. Finally, this definition could further be restrained in multiple ways (e.g. only explicit hypotheses are allowed, or assumptions are never hypotheses). However, if the definition is more (or less) restrictive, it has little implication for the argument below.
3. The hypothesis, theory and auxiliary assumptions
While we have a definition of the scientific hypothesis, we have yet to link it with how it relates to scientific theory, where there is frequently some interconnection (i.e. a hypothesis tests a scientific theory). Generally, for this paper, we believe our argument applies regardless of how scientific theory is defined. Further, some research lacks theory, sometimes called convenience or atheoretical studies [ 41 ]. Here a hypothesis can be made without a wider theory—and our framework fits here too. However, since many consider hypotheses to be defined or deducible from scientific theory, there is an important connection between the two. Therefore, we will briefly clarify how hypotheses relate to common formulations of scientific theory.
A scientific theory is generally a set of axioms or statements about some objects, properties and their relations relating to some phenomena. Hypotheses can often be deduced from the theory. Additionally, a theory has boundary conditions. The boundary conditions specify the domain of the theory stating under what conditions it applies (e.g. all things with a central neural system, humans, women, university teachers) [ 42 ]. Boundary conditions of a theory will consequently limit all hypotheses deduced from the theory. For example, with a boundary condition ‘applies to all humans’, then the subsequent hypotheses deduced from the theory are limited to being about humans. While this limitation of the hypothesis by the theory's boundary condition exists, all the considerations about a hypothesis scope detailed below still apply within the boundary conditions. Finally, it is also possible (depending on the definition of scientific theory) for a hypothesis to test the same theory under different boundary conditions. 5
The final consideration relating scientific theory to scientific hypotheses is auxiliary hypotheses. These hypotheses are theories or assumptions that are considered true simultaneously with the theory. Most philosophies of science from Popper's background knowledge [ 24 ], Kuhn's paradigms during normal science [ 44 ], and Laktos' protective belt [ 45 ] all have their own versions of this auxiliary or background information that is required for the hypothesis to test the theory. For example, Meelh [ 46 ] auxiliary theories/assumptions are needed to go from theoretical terms to empirical terms (e.g. neural activity can be inferred from blood oxygenation in fMRI research or reaction time to an indicator of cognition) and auxiliary theories about instruments (e.g. the experimental apparatus works as intended) and more (see also Other approaches to categorizing hypotheses below). As noted in the previous section, there is a difference between these auxiliary hypotheses, regardless of their definition, and the scientific hypothesis defined above. Recall that our definition of the scientific hypothesis included a commitment to evaluate it. There are no such commitments with auxiliary hypotheses, but rather they are assumed to be correct to test the theory adequately. This distinction proves to be important as auxiliary hypotheses are still part of testing a theory but are separate from the hypothesis to be evaluated (discussed in more detail below).
4. The scope of hypotheses
In the scientific hypothesis section, we defined the hypothesis and discussed how it relates back to the theory. In this section, we want to defend two claims about hypotheses:
- (A1) Hypotheses can have different scopes . Some hypotheses are narrower in their formulation, and some are broader.
- (A2) The scope of hypotheses can vary along three dimensions relating to relationship selection , variable selection , and pipeline selection .
A1 may seem obvious, but it is important to establish what is meant by narrower and broader scope. When a hypothesis is very narrow, it is specific. For example, it might be specific about the type of relationship between some variables. In figure 1 , we make four different statements regarding the relationship between x and y . The narrowest hypothesis here states ‘there is a positive linear relationship with a magnitude of 0.5 between x and y ’ ( figure 1 a ), and the broadest hypothesis states ‘there is a relationship between x and y ’ ( figure 1 d ). Note that many other hypotheses are possible that are not included in this example (such as there being no relationship).

Examples of narrow and broad hypotheses between x and y . Circles indicate a set of possible relationships with varying slopes that can pivot or bend.
We see that the narrowest of these hypotheses claims a type of relationship (linear), a direction of the relationship (positive) and a magnitude of the relationship (0.5). As the hypothesis becomes broader, the specific magnitude disappears ( figure 1 b ), the relationship has additional options than just being linear ( figure 1 c ), and finally, the direction of the relationship disappears. Crucially, all the examples in figure 1 can meet the above definition of scientific hypotheses. They are all statements that can be evaluated with the same scientific method. There is a difference between these statements, though— they differ in the scope of the hypothesis . Here we have justified A1.
Within this framework, when we discuss whether a hypothesis is narrower or broader in scope, this is a relation between two hypotheses where one is a subset of the other. This means that if H 1 is narrower than H 2 , and if H 1 is true, then H 2 is also true. This can be seen in figure 1 a–d . Suppose figure 1 a , the narrowest of all the hypotheses, is true. In that case, all the other broader statements are also true (i.e. a linear correlation of 0.5 necessarily entails that there is also a positive linear correlation, a linear correlation, and some relationship). While this property may appear trivial, it entails that it is only possible to directly compare the hypothesis scope between two hypotheses (i.e. their broadness or narrowness) where one is the subset of the other. 6
4.1. Sets, disjunctions and conjunctions of elements
The above restraint defines the scope as relations between sets. This property helps formalize the framework of this article. Below, when we discuss the different dimensions that can impact the scope, these become represented as a set. Each set contains elements. Each element is a permissible situation that allows the hypothesis to be accepted. We denote elements as lower case with italics (e.g. e 1 , e 2 , e 3 ) and sets as bold upper case (e.g. S ). Each of the three different dimensions discussed below will be formalized as sets, while the total number of elements specifies their scope.
Let us reconsider the above restraint about comparing hypotheses as narrower or broader. This can be formally shown if:
- e 1 , e 2 , e 3 are elements of S 1 ; and
- e 1 and e 2 are elements of S 2 ,
then S 2 is narrower than S 1 .
Each element represents specific propositions that, if corroborated, would support the hypothesis. Returning to figure 1 a , b , the following statements apply to both:
- ‘There is a positive linear relationship between x and y with a slope of 0.5’.
Whereas the following two apply to figure 1 b but not figure 1 a :
- ‘There is a positive linear relationship between x and y with a slope of 0.4’ ( figure 1 b ).
- ‘There is a positive linear relationship between x and y with a slope of 0.3’ ( figure 1 b ).
Figure 1 b allows for a considerably larger number of permissible situations (which is obvious as it allows for any positive linear relationship). When formulating the hypothesis in figure 1 b , we do not need to specify every single one of these permissible relationships. We can simply specify all possible positive slopes, which entails the set of permissible elements it includes.
That broader hypotheses have more elements in their sets entails some important properties. When we say S contains the elements e 1 , e 2 , and e 3 , the hypothesis is corroborated if e 1 or e 2 or e 3 is the case. This means that the set requires only one of the elements to be corroborated for the hypothesis to be considered correct (i.e. the positive linear relationship needs to be 0.3 or 0.4 or 0.5). Contrastingly, we will later see cases when conjunctions of elements occur (i.e. both e 1 and e 2 are the case). When a conjunction occurs, in this formulation, the conjunction itself becomes an element in the set (i.e. ‘ e 1 and e 2 ’ is a single element). Figure 2 illustrates how ‘ e 1 and e 2 ’ is narrower than ‘ e 1 ’, and ‘ e 1 ’ is narrower than ‘ e 1 or e 2 ’. 7 This property relating to the conjunction being narrower than individual elements is explained in more detail in the pipeline selection section below.

Scope as sets. Left : four different sets (grey, red, blue and purple) showing different elements which they contain. Right : a list of each colour explaining which set is a subset of the other (thereby being ‘narrower’).
4.2. Relationship selection
We move to A2, which is to show the different dimensions that a hypothesis scope can vary along. We have already seen an example of the first dimension of a hypothesis in figure 1 , the relationship selection . Let R denote the set of all possible configurations of relationships that are permissible for the hypothesis to be considered true. For example, in the narrowest formulation above, there was one allowed relationship for the hypothesis to be true. Consequently, the size of R (denoted | R |) is one. As discussed above, in the second narrowest formulation ( figure 1 b ), R has more possible relationships where it can still be considered true:
- r 1 = ‘a positive linear relationship of 0.1’
- r 2 = ‘a positive linear relationship of 0.2’
- r 3 = ‘a positive linear relationship of 0.3’.
Additionally, even broader hypotheses will be compatible with more types of relationships. In figure 1 c , d , nonlinear and negative relationships are also possible relationships included in R . For this broader statement to be affirmed, more elements are possible to be true. Thus if | R | is greater (i.e. contains more possible configurations for the hypothesis to be true), then the hypothesis is broader. Thus, the scope of relating to the relationship selection is specified by | R |. Finally, if |R H1 | > |R H2 | , then H 1 is broader than H 2 regarding the relationship selection.
Figure 1 is an example of the relationship narrowing. That the relationship became linear is only an example and does not necessitate a linear relationship or that this scope refers only to correlations. An alternative example of a relationship scope is a broad hypothesis where there is no knowledge about the distribution of some data. In such situations, one may assume a uniform relationship or a Cauchy distribution centred at zero. Over time the specific distribution can be hypothesized. Thereafter, the various parameters of the distribution can be hypothesized. At each step, the hypothesis of the distribution gets further specified to narrower formulations where a smaller set of possible relationships are included (see [ 47 , 48 ] for a more in-depth discussion about how specific priors relate to more narrow tests). Finally, while figure 1 was used to illustrate the point of increasingly narrow relationship hypotheses, it is more likely to expect the narrowest relationship, within fields such as psychology, to have considerable uncertainty and be formulated with confidence or credible intervals (i.e. we will rarely reach point estimates).
4.3. Variable selection
We have demonstrated that relationship selection can affect the scope of a hypothesis. Additionally, at least two other dimensions can affect the scope of a hypothesis: variable selection and pipeline selection . The variable selection in figure 1 was a single bivariate relationship (e.g. x 's relationship with y ). However, it is not always the case that we know which variables will be involved. For example, in neuroimaging, we can be confident that one or more brain regions will be processing some information following a stimulus. Still, we might not be sure which brain region(s) this will be. Consequently, our hypothesis becomes broader because we have selected more variables. The relationship selection may be identical for each chosen variable, but the variable selection becomes broader. We can consider the following three hypotheses to be increasing in their scope:
- H 1 : x relates to y with relationship R .
- H 2 : x 1 or x 2 relates to y with relationship R .
- H 3 : x 1 or x 2 or x 3 relates to y with relationship R .
For H 1 –H 3 above, we assume that R is the same. Further, we assume that there is no interaction between these variables.
In the above examples, we have multiple x ( x 1 , x 2 , x 3 , … , x n ). Again, we can symbolize the variable selection as a non-empty set XY , containing either a single variable or many variables. Our motivation for designating it XY is that the variable selection can include multiple possibilities for both the independent variable ( x ) and the dependent variable ( y ). Like with relationship selection, we can quantify the broadness between two hypotheses with the size of the set XY . Consequently, | XY | denotes the total scope concerning variable selection. Thus, in the examples above | XY H1 | < | XY H2 | < | XY H3 |. Like with relationship selection, hypotheses that vary in | XY | still meet the definition of a hypothesis. 8
An obvious concern for many is that a broader XY is much easier to evaluate as correct. Generally, when | XY 1 | > | XY 2 |, there is a greater chance of spurious correlations when evaluating XY 1 . This concern is an issue relating to the evaluation of hypotheses (e.g. applying statistics to the evaluation), which will require additional assumptions relating to how to evaluate the hypotheses. Strategies to deal with this apply some correction or penalization for multiple statistical testing [ 49 ] or partial pooling and regularizing priors [ 50 , 51 ]. These strategies aim to evaluate a broader variable selection ( x 1 or x 2 ) on equal or similar terms to a narrow variable selection ( x 1 ).
4.4. Pipeline selection
Scientific studies require decisions about how to perform the analysis. This scope considers transformations applied to the raw data ( XY raw ) to achieve some derivative ( XY ). These decisions can also involve selection procedures that drop observations deemed unreliable, standardizing, correcting confounding variables, or different philosophies. We can call the array of decisions and transformations used as the pipeline . A hypothesis varies in the number of pipelines:
- H 1 : XY has a relationship(s) R with pipeline p 1 .
- H 2 : XY has a relationship(s) R with pipeline p 1 or pipeline p 2 .
- H 3 : XY has a relationship(s) R with pipeline p 1 or pipeline p 2 , or pipeline p 3 .
Importantly, the pipeline here considers decisions regarding how the hypothesis shapes the data collection and transformation. We do not consider this to include decisions made regarding the assumptions relating to the statistical inference as those relate to operationalizing the evaluation of the hypothesis and not part of the hypothesis being evaluated (these assumptions are like auxiliary hypotheses, which are assumed to be true but not explicitly evaluated).
Like with variable selection ( XY ) and relationship selection ( R ), we can see that pipelines impact the scope of hypotheses. Again, we can symbolize the pipeline selection with a set P . As previously, | P | will denote the dimension of the pipeline selection. In the case of pipeline selection, we are testing the same variables, looking for the same relationship, but processing the variables or relationships with different pipelines to evaluate the relationship. Consequently, | P H1 | < | P H2 | < | P H3 |.
These issues regarding pipelines have received attention as the ‘garden of forking paths' [ 52 ]. Here, there are calls for researchers to ensure that their entire pipeline has been specified. Additionally, recent work has highlighted the diversity of results based on multiple analytical pipelines [ 53 , 54 ]. These results are often considered a concern, leading to calls that results should be pipeline resistant.
The wish for pipeline-resistant methods entails that hypotheses, in their narrowest form, are possible for all pipelines. Consequently, a narrower formulation will entail that this should not impact the hypothesis regardless of which pipeline is chosen. Thus the conjunction of pipelines is narrower than single pipelines. Consider the following three scenarios:
- H 3 : XY has a relationship(s) R with pipeline p 1 and pipeline p 2 .
In this instance, since H 1 is always true if H 3 is true, thus H 3 is a narrower formulation than H 1 . Consequently, | P H3 | < | P H1 | < | P H2 |. Decreasing the scope of the pipeline dimension also entails the increase in conjunction of pipelines (i.e. creating pipeline-resistant methods) rather than just the reduction of disjunctional statements.
4.5. Combining the dimensions
In summary, we then have three different dimensions that independently affect the scope of the hypothesis. We have demonstrated the following general claim regarding hypotheses:
- The variables XY have a relationship R with pipeline P .
And that the broadness and narrowness of a hypothesis depend on how large the three sets XY , R and P are. With this formulation, we can conclude that hypotheses have a scope that can be determined with a 3-tuple argument of (| R |, | XY |, | P |).
While hypotheses can be formulated along these three dimensions and generally aim to be reduced, it does not entail that these dimensions behave identically. For example, the relationship dimensions aim to reduce the number of elements as far as possible (e.g. to an interval). Contrastingly, for both variables and pipeline, the narrower hypothesis can reduce to single variables/pipelines or become narrower still and become conjunctions where all variables/pipelines need to corroborate the hypothesis (i.e. regardless of which method one follows, the hypothesis is correct).
5. Additional possible dimensions
No commitment is being made about the exhaustive nature of there only being three dimensions that specify the hypothesis scope. Other dimensions may exist that specify the scope of a hypothesis. For example, one might consider the pipeline dimension as two different dimensions. The first would consider the experimental pipeline dimension regarding all variables relating to the experimental setup to collect data, and the latter would be the analytical pipeline dimension regarding the data analysis of any given data snapshot. Another possible dimension is adding the number of situations or contexts under which the hypothesis is valid. For example, any restraint such as ‘in a vacuum’, ‘under the speed of light’, or ‘in healthy human adults' could be considered an additional dimension of the hypothesis. There is no objection to whether these should be additional dimensions of the hypothesis. However, as stated above, these usually follow from the boundary conditions of the theory.
6. Specifying the scope versus assumptions
We envision that this framework can help hypothesis-makers formulate hypotheses (in research plans, registered reports, preregistrations etc.). Further, using this framework while formulating hypotheses can help distinguish between auxiliary hypotheses and parts of the scientific hypothesis being tested. When writing preregistrations, it can frequently occur that some step in the method has two alternatives (e.g. a preprocessing step), and there is not yet reason to choose one over the other, and the researcher needs to make a decision. These following scenarios are possible:
- 1. Narrow pipeline scope . The researcher evaluates the hypothesis with both pipeline variables (i.e. H holds for both p 1 and p 2 where p 1 and p 2 can be substituted with each other in the pipeline).
- 2. Broad pipeline scope. The researcher evaluates the hypothesis with both pipeline variables, and only one needs to be correct (i.e. H holds for either p 1 or p 2 where p 1 and p 2 can be substituted with each other in the pipeline). The result of this experiment may help motivate choosing either p 1 or p 2 in future studies.
- 3. Auxiliary hypothesis. Based on some reason (e.g. convention), the researcher assumes p 1 and evaluates H assuming p 1 is true.
Here we see that the same pipeline step can be part of either the auxiliary hypotheses or the pipeline scope. This distinction is important because if (3) is chosen, the decision becomes an assumption that is not explicitly tested by the hypothesis. Consequently, a researcher confident in the hypothesis may state that the auxiliary hypothesis p 1 was incorrect, and they should retest their hypothesis using different assumptions. In the cases where this decision is part of the pipeline scope, the hypothesis is intertwined with this decision, removing the eventual wiggle-room to reject auxiliary hypotheses that were assumed. Furthermore, starting with broader pipeline hypotheses that gradually narrow down can lead to a more well-motivated protocol for approaching the problem. Thus, this framework can help researchers while writing their hypotheses in, for example, preregistrations because they can consider when they are committing to a decision, assuming it, or when they should perhaps test a broader hypothesis with multiple possible options (discussed in more detail in §11 below).
7. The reduction of scope in hypothesis space
Having established that different scopes of a hypothesis are possible, we now consider how the hypotheses change over time. In this section, we consider how the scope of the hypothesis develops ideally within science.
Consider a new research question. A large number of hypotheses are possible. Let us call this set of all possible hypotheses the hypothesis space . Hypotheses formulated within this space can be narrower or broader based on the dimensions discussed previously ( figure 3 ).

Example of hypothesis space. The hypothesis scope is expressed as cuboids in three dimensions (relationship ( R ), variable ( XY ), pipeline ( P )). The hypothesis space is the entire possible space within the three dimensions. Three hypotheses are shown in the hypothesis space (H 1 , H 2 , H 3 ). H 2 and H 3 are subsets of H 1 .
After the evaluation of the hypothesis with the scientific process, the hypothesis will be accepted or rejected. 9 The evaluation could be done through falsification or via verification, depending on the philosophy of science commitments. Thereafter, other narrower formulations of the hypothesis can be formulated by reducing the relationship, variable or pipeline scope. If a narrower hypothesis is accepted, more specific details about the subject matter are known, or a theory has been refined in greater detail. A narrower hypothesis will entail a more specific relationship, variable or pipeline detailed in the hypothesis. Consequently, hypotheses linked to each other in this way will become narrower over time along one or more dimensions. Importantly, considering that the conjunction of elements is narrower than single elements for pipelines and variables, this process of narrower hypotheses will lead to more general hypotheses (i.e. they have to be applied in all conditions and yield less flexibility when they do not apply). 10
Considering that the scopes of hypotheses were defined as sets above, some properties can be deduced from this framework about how narrower hypotheses relate to broader hypotheses. Let us consider three hypotheses (H 1 , H 2 , and H 3 ; figure 3 ). H 2 and H 3 are non-overlapping subsets of H 1 . Thus H 2 and H 3 are both narrower in scope than H 1 . Thus the following is correct:
- P1: If H 1 is false, then H 2 is false, and H 2 does not need to be evaluated.
- P2: If H 2 is true, then the broader H 1 is true, and H 1 does not need to be evaluated.
- P3: If H 1 is true and H 2 is false, some other hypothesis H 3 of similar scope to H 2 is possible.
For example, suppose H 1 is ‘there is a relationship between x and y ’, H 2 is ‘there is a positive relationship between x and y ’, and H 3 is ‘a negative relationship between x and y ’. In that case, it becomes apparent how each of these follows. 11 Logically, many deductions from set theory are possible but will not be explored here. Instead, we will discuss two additional consequences of hypothesis scopes: scientific novelty and applications for the researcher who formulates a hypothesis.
P1–P3 have been formulated as hypotheses being true or false. In practice, hypotheses are likely evaluated probabilistically (e.g. ‘H 1 is likely’ or ‘there is evidence in support of H 1 ’). In these cases, P1–P3 can be rephrased to account for this by substituting true/false with statements relating to evidence. For example, P2 could read: ‘If there is evidence in support of H 2 , then there is evidence in support of H 1 , and H 1 does not need to be evaluated’.
8. Scientific novelty as the reduction of scope
Novelty is a key concept that repeatedly occurs in multiple aspects of the scientific enterprise, from funding to publishing [ 55 ]. Generally, scientific progress establishes novel results based on some new hypothesis. Consequently, the new hypothesis for the novel results must be narrower than previously established knowledge (i.e. the size of the scopes is reduced). Otherwise, the result is trivial and already known (see P2 above). Thus, scientific work is novel if the scientific process produces a result based on hypotheses with either a smaller | R |, | XY |, or | P | compared to previous work.
This framework of dimensions of the scope of a hypothesis helps to demarcate when a hypothesis and the subsequent result are novel. If previous studies have established evidence for R 1 (e.g. there is a positive relationship between x and y ), a hypothesis will be novel if and only if it is narrower than R 1 . Thus, if R 2 is narrower in scope than R 1 (i.e. | R 2 | < | R 1 |), R 2 is a novel hypothesis.
Consider the following example. Study 1 hypothesizes, ‘There is a positive relationship between x and y ’. It identifies a linear relationship of 0.6. Next, Study 2 hypothesizes, ‘There is a specific linear relationship between x and y that is 0.6’. Study 2 also identifies the relationship of 0.6. Since this was a narrower hypothesis, Study 2 is novel despite the same result. Frequently, researchers claim that they are the first to demonstrate a relationship. Being the first to demonstrate a relationship is not the final measure of novelty. Having a narrower hypothesis than previous researchers is a sign of novelty as it further reduces the hypothesis space.
Finally, it should be noted that novelty is not the only objective of scientific work. Other attributes, such as improving the certainty of a current hypothesis (e.g. through replications), should not be overlooked. Additional scientific explanations and improved theories are other aspects. Additionally, this definition of novelty relating to hypothesis scope does not exclude other types of novelty (e.g. new theories or paradigms).
9. How broad should a hypothesis be?
Given the previous section, it is elusive to conclude that the hypothesis should be as narrow as possible as it entails maximal knowledge gain and scientific novelty when formulating hypotheses. Indeed, many who advocate for daring or risky tests seem to hold this opinion. For example, Meehl [ 46 ] argues that we should evaluate theories based on point (or interval) prediction, which would be compatible with very narrow versions of relationships. We do not necessarily think that this is the most fruitful approach. In this section, we argue that hypotheses should aim to be narrower than current knowledge , but too narrow may be problematic .
Let us consider the idea of confirmatory analyses. These studies will frequently keep the previous hypothesis scopes regarding P and XY but aim to become more specific regarding R (i.e. using the same method and the same variables to detect a more specific relationship). A very daring or narrow hypothesis is to minimize R to include the fewest possible relationships. However, it becomes apparent that simply pursuing specificness or daringness is insufficient for selecting relevant hypotheses. Consider a hypothetical scenario where a researcher believes virtual reality use leads people to overestimate the amount of exercise they have done. If unaware of previous studies on this project, an apt hypothesis is perhaps ‘increased virtual reality usage correlates with a less accuracy of reported exercise performed’ (i.e. R is broad). However, a more specific and more daring hypothesis would be to specify the relationship further. Thus, despite not knowing if there is a relationship at all, a more daring hypothesis could be: ‘for every 1 h of virtual reality usage, there will be, on average, a 0.5% decrease in the accuracy of reported exercise performed’ (i.e. R is narrow). We believe it would be better to establish the broader hypothesis in any scenario here for the first experiment. Otherwise, if we fail to confirm the more specific formulation, we could reformulate another equally narrow relative to the broader hypothesis. This process of tweaking a daring hypothesis could be pursued ad infinitum . Such a situation will neither quickly identify the true hypothesis nor effectively use limited research resources.
By first discounting a broader hypothesis that there is no relationship, it will automatically discard all more specific formulations of that relationship in the hypothesis space. Returning to figure 3 , it will be better to establish H 1 before attempting H 2 or H 3 to ensure the correct area in the hypothesis space is being investigated. To provide an analogy: when looking for a needle among hay, first identify which farm it is at, then which barn, then which haystack, then which part of the haystack it is at before we start picking up individual pieces of hay. Thus, it is preferable for both pragmatic and cost-of-resource reasons to formulate sufficiently broad hypotheses to navigate the hypothesis space effectively.
Conversely, formulating too broad a relationship scope in a hypothesis when we already have evidence for narrower scope would be superfluous research (unless the evidence has been called into question by, for example, not being replicated). If multiple studies have supported the hypothesis ‘there is a 20-fold decrease in mortality after taking some medication M’, it would be unnecessary to ask, ‘Does M have any effect?’.
Our conclusion is that the appropriate scope of a hypothesis, and its three dimensions, follow a Goldilocks-like principle where too broad is superfluous and not novel, while too narrow is unnecessary or wasteful. Considering the scope of one's hypothesis and how it relates to previous hypotheses' scopes ensures one is asking appropriate questions.
Finally, there has been a recent trend in psychology that hypotheses should be formal [ 38 , 56 – 60 ]. Formal theories are precise since they are mathematical formulations entailing that their interpretations are clear (non-ambiguous) compared to linguistic theories. However, this literature on formal theories often refers to ‘precise predictions’ and ‘risky testing’ while frequently referencing Meehl, who advocates for narrow hypotheses (e.g. [ 38 , 56 , 59 ]). While perhaps not intended by any of the proponents, one interpretation of some of these positions is that hypotheses derived from formal theories will be narrow hypotheses (i.e. the quality of being ‘precise’ can mean narrow hypotheses with risky tests and non-ambiguous interpretations simultaneously). However, the benefit from the clarity (non-ambiguity) that formal theories/hypotheses bring also applies to broad formal hypotheses as well. They can include explicit but formalized versions of uncertain relationships, multiple possible pipelines, and large sets of variables. For example, a broad formal hypothesis can contain a hyperparameter that controls which distribution the data fit (broad relationship scope), or a variable could represent a set of formalized explicit pipelines (broad pipeline scope) that will be tested. In each of these instances, it is possible to formalize non-ambiguous broad hypotheses from broad formal theories that do not yet have any justification for being overly narrow. In sum, our argumentation here stating that hypotheses should not be too narrow is not an argument against formal theories but rather that hypotheses (derived from formal theories) do not necessarily have to be narrow.
10. Other approaches to categorizing hypotheses
The framework we present here is a way of categorizing hypotheses into (at least) three dimensions regarding the hypothesis scope, which we believe is accessible to researchers and help link scientific work over time while also trying to remain neutral with regard to a specific philosophy of science. Our proposal does not aim to be antagonistic or necessarily contradict other categorizing schemes—but we believe that our framework provides benefits.
One recent categorization scheme is the Theoretical (T), Auxiliary (A), Statistical (S) and Inferential (I) assumption model (together becoming the TASI model) [ 61 , 62 ]. Briefly, this model considers theory to generate theoretical hypotheses. To translate from theoretical unobservable terms (e.g. personality, anxiety, mass), auxiliary assumptions are needed to generate an empirical hypothesis. Statistical assumptions are often needed to test the empirical hypothesis (e.g. what is the distribution, is it skewed or not) [ 61 , 62 ]. Finally, additional inferential assumptions are needed to generalize to a larger population (e.g. was there a random and independent sampling from defined populations). The TASI model is insightful and helpful in highlighting the distance between a theory and the observation that would corroborate/contradict it. Part of its utility is to bring auxiliary hypotheses into the foreground, to improve comparisons between studies and improve theory-based interventions [ 63 , 64 ].
We do agree with the importance of being aware of or stating the auxiliary hypotheses, but there are some differences between the frameworks. First, the number of auxiliary assumptions in TASI can be several hundred [ 62 ], whereas our framework will consider some of them as part of the pipeline dimension. Consider the following four assumptions: ‘the inter-stimulus interval is between 2000 ms and 3000 ms', ‘the data will be z-transformed’, ‘subjects will perform correctly’, and ‘the measurements were valid’. According to the TASI model, all these will be classified similarly as auxiliary assumptions. Contrarily, within our framework, it is possible to consider the first two as part of the pipeline dimension and the latter two as auxiliary assumptions, and consequently, the first two become integrated as part of the hypothesis being tested and the latter two auxiliary assumptions. A second difference between the frameworks relates to non-theoretical studies (convenience, applied or atheoretical). Our framework allows for the possibility that the hypothesis space generated by theoretical and convenience studies can interact and inform each other within the same framework . Contrarily, in TASI, the theory assumptions no longer apply, and a different type of hypothesis model is needed; these assumptions must be replaced by another group of assumptions (where ‘substantive application assumptions' replace the T and the A, becoming SSI) [ 61 ]. Finally, part of our rationale for our framework is to be able to link and track hypotheses and hypothesis development together over time, so our classification scheme has different utility.
Another approach which has some similar utility to this framework is theory construction methodology (TCM) [ 57 ]. The similarity here is that TCM aims to be a practical guide to improve theory-making in psychology. It is an iterative process which relates theory, phenomena and data. Here hypotheses are not an explicit part of the model. However, what is designated as ‘proto theory’ could be considered a hypothesis in our framework as they are a product of abduction, shaping the theory space. Alternatively, what is deduced to evaluate the theory can also be considered a hypothesis. We consider both possible and that our framework can integrate with these two steps, especially since TCM does not have clear guidelines for how to do each step.
11. From theory to practice: implementing this framework
We believe that many practising researchers can relate to many aspects of this framework. But, how can a researcher translate the above theoretical framework to their work? The utility of this framework lies in bringing these three scopes of a hypothesis together and explaining how each can be reduced. We believe researchers can use this framework to describe their current practices more clearly. Here we discuss how it can be helpful for researchers when formulating, planning, preregistering, and discussing the evaluation of their scientific hypotheses. These practical implications are brief, and future work can expand on the connection between the full interaction between hypothesis space and scope. Furthermore, both authors have the most experience in cognitive neuroscience, and some of the practical implications may revolve around this type of research and may not apply equally to other fields.
11.1. Helping to form hypotheses
Abduction, according to Peirce, is a hypothesis-making exercise [ 22 , 26 – 28 ]. Given some observations, a general testable explanation of the phenomena is formed. However, when making the hypothesis, this statement will have a scope (either explicitly or implicitly). Using our framework, the scope can become explicit. The hypothesis-maker can start with ‘The variables XY have a relationship R with pipeline P ’ as a scaffold to form the hypothesis. From here, the hypothesis-maker can ‘fill in the blanks’, explicitly adding each of the scopes. Thus, when making a hypothesis via abduction and using our framework, the hypothesis will have an explicit scope when it is made. By doing this, there is less chance that a formulated hypothesis is unclear, ambiguous, and needs amending at a later stage.
11.2. Assisting to clearly state hypotheses
A hypothesis is not just formulated but also communicated. Hypotheses are stated in funding applications, preregistrations, registered reports, and academic articles. Further, preregistered hypotheses are often omitted or changed in the final article [ 11 ], and hypotheses are not always explicitly stated in articles [ 12 ]. How can this framework help to make better hypotheses? Similar to the previous point, filling in the details of ‘The variables XY have a relationship R with pipeline P ’ is an explicit way to communicate the hypothesis. Thinking about each of these dimensions should entail an appropriate explicit scope and, hopefully, less variation between preregistered and reported hypotheses. The hypothesis does not need to be a single sentence, and details of XY and P will often be developed in the methods section of the text. However, using this template as a starting point can help ensure the hypothesis is stated, and the scope of all three dimensions has been communicated.
11.3. Helping to promote explicit and broad hypotheses instead of vague hypotheses
There is an important distinction between vague hypotheses and broad hypotheses, and this framework can help demarcate between them. A vague statement would be: ‘We will quantify depression in patients after treatment’. Here there is uncertainty relating to how the researcher will go about doing the experiment (i.e. how will depression be quantified?). However, a broad statement can be uncertain, but the uncertainty is part of the hypothesis: ‘Two different mood scales (S 1 or S 2 ) will be given to patients and test if only one (or both) changed after treatment’. This latter statement is transparently saying ‘S 1 or S 2 ’ is part of a broad hypothesis—the uncertainty is whether the two different scales are quantifying the same construct. We keep this uncertainty within the broad hypothesis, which will get evaluated, whereas a vague hypothesis has uncertainty as part of the interpretation of the hypothesis. This framework can be used when formulating hypotheses to help be broad (where needed) but not vague.
11.4. Which hypothesis should be chosen?
When considering the appropriate scope above, we argued for a Goldilocks-like principle of determining the hypothesis that is not too broad or too narrow. However, when writing, for example, a preregistration, how does one identify this sweet spot? There is no easy or definite universal answer to this question. However, one possible way is first to identify the XY , R , and P of previous hypotheses. From here, identify what a non-trivial step is to improve our knowledge of the research area. So, for example, could you be more specific about the exact nature of the relationship between the variables? Does the pipeline correspond to today's scientific standards, or were some suboptimal decisions made? Is there another population that you think the previous result also applies to? Do you think that maybe a more specific construct or subpopulation might explain the previous result? Could slightly different constructs (perhaps easier to quantify) be used to obtain a similar relationship? Are there even more constructs to which this relationship should apply simultaneously? Are you certain of the direction of the relationship? Answering affirmatively to any of these questions will likely make a hypothesis narrower and connect to previous research while being clear and explicit. Moreover, depending on the research question, answering any of these may be sufficiently narrow to be a non-trivial innovation. However, there are many other ways to make a hypothesis narrower than these guiding questions.
11.5. The confirmatory–exploratory continuum
Research is often dichotomized into confirmatory (testing a hypothesis) or exploratory (without a priori hypotheses). With this framework, researchers can consider how their research acts on some hypothesis space. Confirmatory and exploratory work has been defined in terms of how each interacts with the researcher's degrees of freedom (where confirmatory aims to reduce while exploratory utilizes them [ 30 ]). Both broad confirmatory and narrow exploratory research are possible using this definition and possible within this framework. How research interacts with the hypothesis space helps demarcate it. For example, if a hypothesis reduces the scope, it becomes more confirmatory, and trying to understand data given the current scope would be more exploratory work. This further could help demarcate when exploration is useful. Future theoretical work can detail how different types of research impact the hypothesis space in more detail.
11.6. Understanding when multiverse analyses are needed
Researchers writing a preregistration may face many degrees of freedom they have to choose from, and different researchers may motivate different choices. If, when writing such a preregistration, there appears to be little evidential support for certain degrees of freedom over others, the researcher is left with the option to either make more auxiliary assumptions or identify when an investigation into the pipeline scope is necessary by conducting a multiverse analysis that tests the impact of the different degrees of freedom on the result (see [ 8 ]). Thus, when applying this framework to explicitly state what pipeline variables are part of the hypothesis or an auxiliary assumption, the researcher can identify when it might be appropriate to conduct a multiverse analysis because they are having difficulty formulating hypotheses.
11.7. Describing novelty
Academic journals and research funders often ask for novelty, but the term ‘novelty’ can be vague and open to various interpretations [ 55 ]. This framework can be used to help justify the novelty of research. For example, consider a scenario where a previous study has established a psychological construct (e.g. well-being) that correlates with a certain outcome measure (e.g. long-term positive health outcomes). This framework can be used to explicitly justify novelty by (i) providing a more precise understanding of the relationship (e.g. linear or linear–plateau) or (ii) identifying more specific variables related to well-being or health outcomes. Stating how some research is novel is clearer than merely stating that the work is novel. This practice might even help journals and funders identify what type of novelty they would like to reward. In sum, this framework can help identify and articulate how research is novel.
11.8. Help to identify when standardization of pipelines is beneficial or problematic to a field
Many consider standardization in a field to be important for ensuring the comparability of results. Standardization of methods and tools entails that the pipeline P is identical (or at least very similar) across studies. However, in such cases, the standardized pipeline becomes an auxiliary assumption representing all possible pipelines. Therefore, while standardized pipelines have their benefits, this assumption becomes broader without validating (e.g. via multiverse analysis) which pipelines a standardized P represents. In summary, because this framework helps distinguish between auxiliary assumptions and explicit parts of the hypothesis and identifies when a multiverse analysis is needed, it can help determine when standardizations of pipelines are representative (narrower hypotheses) or assumptive (broader hypotheses).
12. Conclusion
Here, we have argued that the scope of a hypothesis is made up of three dimensions: the relationship ( R ), variable ( XY ) and pipeline ( P ) selection. Along each of these dimensions, the scope can vary. Different types of scientific enterprises will often have hypotheses that vary the size of the scopes. We have argued that this focus on the scope of the hypothesis along these dimensions helps the hypothesis-maker formulate their hypotheses for preregistrations while also helping demarcate auxiliary hypotheses (assumed to be true) from the hypothesis (those being evaluated during the scientific process).
Hypotheses are an essential part of the scientific process. Considering what type of hypothesis is sufficient or relevant is an essential job of the researcher that we think has been overlooked. We hope this work promotes an understanding of what a hypothesis is and how its formulation and reduction in scope is an integral part of scientific progress. We hope it also helps clarify how broad hypotheses need not be vague or inappropriate.
Finally, we applied this idea of scopes to scientific progress and considered how to formulate an appropriate hypothesis. We have also listed several ways researchers can practically implement this framework today. However, there are other practicalities of this framework that future work should explore. For example, it could be used to differentiate and demarcate different scientific contributions (e.g. confirmatory studies, exploration studies, validation studies) with how their hypotheses interact with the different dimensions of the hypothesis space. Further, linking hypotheses over time within this framework can be a foundation for open hypothesis-making by promoting explicit links to previous work and detailing the reduction of the hypothesis space. This framework helps quantify the contribution to the hypothesis space of different studies and helps clarify what aspects of hypotheses can be relevant at different times.
Acknowledgements
We thank Filip Gedin, Kristoffer Sundberg, Jens Fust, and James Steele for valuable feedback on earlier versions of this article. We also thank Mark Rubin and an unnamed reviewer for valuable comments that have improved the article.
1 While this is our intention, we cannot claim that every theory has been accommodated.
2 Similar requirements of science being able to evaluate the hypothesis can be found in pragmatism [ 22 ], logical positivism [ 23 ] and falsification [ 24 ].
3 Although when making inferences about a failed evaluation of a scientific hypothesis it is possible, due to underdetermination, to reject the auxiliary hypothesis instead of rejecting the hypothesis. However, that rejection occurs at a later inference stage. The evaluation using the scientific method aims to test the scientific hypothesis, not the auxiliary assumptions.
4 Although some have argued that this practice is not as problematic or questionable (see [ 34 , 35 ]).
5 Alternatively, theories sometimes expand their boundary conditions. A theory that was previously about ‘humans' can be used with a more inclusive boundary condition. Thus it is possible for the hypothesis-maker to use a theory about humans (decision making) and expand it to fruit flies or plants (see [ 43 ]).
6 A similarity exists here with Popper, where he uses set theory in a similar way to compare theories (not hypotheses). Popper also discusses how theories with overlapping sets but neither is a subset are also comparable (see [ 24 , §§32–34]). We do not exclude this possibility but can require additional assumptions.
7 When this could be unclear, we place the element within quotation marks.
8 Here, we have assumed that there is no interaction between these variables in variable selection. If an interaction between x 1 and x 2 is hypothesized, this should be viewed as a different variable compared to ‘ x 1 or x 2 ’. The motivation behind this is because the hypothesis ‘ x 1 or x 2 ’ is not a superset of the interaction (i.e. ‘ x 1 or x 2 ’ is not necessarily true when the interaction is true). The interaction should, in this case, be considered a third variable (e.g. I( x 1 , x 2 )) and the hypothesis ‘ x 1 or x 2 or I( x 1 , x 2 )’ is broader than ‘ x 1 or x 2 ’.
9 Or possibly ambiguous or inconclusive.
10 This formulation of scope is compatible with different frameworks from the philosophy of science. For example, by narrowing the scope would in a Popperian terminology mean prohibiting more basic statements (thus a narrower hypothesis has a higher degree of falsifiability). The reduction of scope in the relational dimension would in Popperian terminology mean increase in precision (e.g. a circle is more precise than an ellipse since circles are a subset of possible ellipses), whereas reduction in variable selection and pipeline dimension would mean increase universality (e.g. ‘all heavenly bodies' is more universal than just ‘planets') [ 24 ]. For Meehl the reduction of the relationship dimension would amount to decreasing the relative tolerance of a theory to the Spielraum [ 46 ] .
11 If there is no relationship between x and y , we do not need to test if there is a positive relationship. If we know there is a positive relationship between x and y , we do not need to test if there is a relationship. If we know there is a relationship but there is not a positive relationship, then it is possible that they have a negative relationship.
Data accessibility
Declaration of ai use.
We have not used AI-assisted technologies in creating this article.
Authors' contributions
W.H.T.: conceptualization, investigation, writing—original draft, writing—review and editing; S.S.: investigation, writing—original draft, writing—review and editing.
Both authors gave final approval for publication and agreed to be held accountable for the work performed therein.
Conflict of interest declaration
We declare we have no competing interests.
We received no funding for this study.
Click through the PLOS taxonomy to find articles in your field.
For more information about PLOS Subject Areas, click here .
Loading metrics
Open Access
Peer-reviewed
Research Article
Capturing farm diversity with hypothesis-based typologies: An innovative methodological framework for farming system typology development
Roles Conceptualization, Data curation, Formal analysis, Methodology, Validation, Visualization, Writing – original draft, Writing – review & editing
Affiliation Farming Systems Ecology, Wageningen University & Research, Wageningen, The Netherlands
Roles Conceptualization, Formal analysis, Investigation, Methodology, Validation, Writing – original draft, Writing – review & editing
Roles Conceptualization, Formal analysis, Investigation, Validation, Writing – original draft, Writing – review & editing
Roles Conceptualization, Formal analysis, Investigation, Validation, Visualization, Writing – original draft
Affiliations Farming Systems Ecology, Wageningen University & Research, Wageningen, The Netherlands, Plant Production Systems, Wageningen University & Research, Wageningen, The Netherlands
Roles Conceptualization, Supervision, Writing – original draft, Writing – review & editing
Affiliation Plant Production Systems, Wageningen University & Research, Wageningen, The Netherlands
Roles Conceptualization, Funding acquisition, Investigation, Methodology, Supervision, Writing – original draft
Roles Conceptualization, Formal analysis, Methodology, Validation, Writing – original draft, Writing – review & editing
Affiliation CIMMYT-Southern Africa, Harare, Zimbabwe
Roles Conceptualization, Funding acquisition, Project administration, Supervision, Writing – original draft, Writing – review & editing
* E-mail: [email protected]
- Stéphanie Alvarez,
- Carl J. Timler,
- Mirja Michalscheck,
- Wim Paas,
- Katrien Descheemaeker,
- Pablo Tittonell,
- Jens A. Andersson,
- Jeroen C. J. Groot
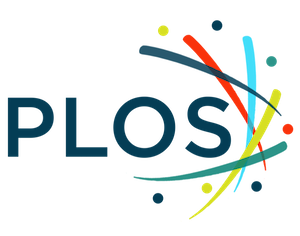
- Published: May 15, 2018
- https://doi.org/10.1371/journal.pone.0194757
- Reader Comments
Creating typologies is a way to summarize the large heterogeneity of smallholder farming systems into a few farm types. Various methods exist, commonly using statistical analysis, to create these typologies. We demonstrate that the methodological decisions on data collection, variable selection, data-reduction and clustering techniques can bear a large impact on the typology results. We illustrate the effects of analysing the diversity from different angles, using different typology objectives and different hypotheses, on typology creation by using an example from Zambia’s Eastern Province. Five separate typologies were created with principal component analysis (PCA) and hierarchical clustering analysis (HCA), based on three different expert-informed hypotheses. The greatest overlap between typologies was observed for the larger, wealthier farm types but for the remainder of the farms there were no clear overlaps between typologies. Based on these results, we argue that the typology development should be guided by a hypothesis on the local agriculture features and the drivers and mechanisms of differentiation among farming systems, such as biophysical and socio-economic conditions. That hypothesis is based both on the typology objective and on prior expert knowledge and theories of the farm diversity in the study area. We present a methodological framework that aims to integrate participatory and statistical methods for hypothesis-based typology construction. This is an iterative process whereby the results of the statistical analysis are compared with the reality of the target population as hypothesized by the local experts. Using a well-defined hypothesis and the presented methodological framework, which consolidates the hypothesis through local expert knowledge for the creation of typologies, warrants development of less subjective and more contextualized quantitative farm typologies.
Citation: Alvarez S, Timler CJ, Michalscheck M, Paas W, Descheemaeker K, Tittonell P, et al. (2018) Capturing farm diversity with hypothesis-based typologies: An innovative methodological framework for farming system typology development. PLoS ONE 13(5): e0194757. https://doi.org/10.1371/journal.pone.0194757
Editor: Iratxe Puebla, Public Library of Science, UNITED KINGDOM
Received: September 22, 2016; Accepted: March 10, 2018; Published: May 15, 2018
Copyright: © 2018 Alvarez et al. This is an open access article distributed under the terms of the Creative Commons Attribution License , which permits unrestricted use, distribution, and reproduction in any medium, provided the original author and source are credited.
Data Availability: Data are available in the manuscript and supporting information file. Additional data are from the Africa RISING/SIMLEZA project whose Project Coordinator and Chief Scientist for Africa RISING East and Southern Africa, respectively Irmgard Hoeschle-Zeledon (IITA) and Mateete Bekunda (IITA), may be contacted at [email protected] and [email protected] . Other contacts are available at https://africa-rising.net/contacts/ . The authors confirm that others have the same access to the data as the authors.
Funding: The fieldwork of this study was conducted within the Africa RISING/SIMLEZA research-for-development program in Zambia that is led by the International Institute of Tropical Agriculture (IITA). The research was partly funded by the United States Agency for International Development (USAID; https://www.usaid.gov/ ) as part of the US Government’s Feed the Future Initiative. The contents are the responsibility of the producing organizations and do not necessarily reflect the opinion of USAID or the U.S. Government. The CGIAR Research program Humidtropics and all the donors supported this research through their contributions to the CGIAR Fund. The funders had no role in study design, data collection and analysis, decision to publish, or preparation of the manuscript. For a list of Fund donors please see: https://www.cgiar.org/funders/ .
Competing interests: The authors have declared that no competing interests exist.
Introduction
Smallholder farming systems are highly heterogeneous in many characteristics such as individual farming households’ land access, soil fertility, cropping, livestock assets, off-farm activities, labour and cash availability, socio-cultural traits, farm development trajectories and livelihood orientations, e.g. [ 1 , 2 ]. Farm typologies can help to summarize this diversity among farming systems. Typology construction has been defined as a process of classification, description, comparison and interpretation or explanation of a set of elements on the basis of selected criteria, allowing reduction and simplification of a multiplicity of elements into a few basic/elementary types ([ 3 ] cited by [ 4 ]). As a result, farm typologies are a tool to comprehend the complexity of farming systems by providing a simplified representation of the diversity within the farming system by organizing farms into quite homogenous groups, the farm types. These identified farm types are defined as a specific combination of multiple features [ 5 – 7 ].
Capturing farming system heterogeneity through typologies is considered as a useful first step in the analysis of farm performance and rural livelihoods [ 8 – 9 ]. Farm typologies can be used for many purposes, for instance i) the selection of representative farms or prototype farms as case study objects, e.g. [ 10 – 12 ]; ii) the targeting or fine-tuning of interventions, for example by identifying opportunities and appropriate interventions per farm type, e.g. [ 13 – 18 ]; iii) for the extension of technologies, policies or ex-ante impact assessments to larger spatial or organizational scales (up-scaling and/or out-scaling), e.g. [ 19 – 22 ]; and iv) to support the identification of farm development trajectories and evolution patterns, e.g. [ 23 – 28 ].
Various approaches can be used to develop farm typologies [ 29 ]. The identification of criteria defining a farm type can be based on the knowledge of local stakeholders, such as extension workers and/or farmers, or derived from the analysis of data collected using farm household surveys which provide a large set of quantitative and qualitative variables to describe the farm household system [ 30 ]. Perrot et al. [ 26 ] proposed to define "aggregation poles" with local experts, i.e. virtual farms summarising the discriminating characteristics of a farm type, which can then be used as reference for the aggregation (manually or with statistical techniques) of actual farming households into specific farm types. Based on farm surveys and interviews, Capillon [ 6 ] used a (manual) step-by-step comparison of farm functioning to distinguish different types; this analysis focused on the tactical and strategic choices of farmers and on the overall objective of the household. Based on this approach, farm types were created using statistical techniques to first group farms according their structure, then within each of these structural groups, define individual farm types on the basis of their strategic choices and orientation [ 31 ]. Landais et al. [ 32 ] favoured the comparison of farming practices for the identification of farm types. Kostrowicki and Tyszkiewicz [ 33 ] proposed the identification of types based on the inherent farm characteristics in terms of social, organizational and technical, or economic criteria, and then representing these multiple dimensions in a typogram, i.e. a multi-axis graphic divided into quadrants, similar to a radar chart. Nowadays, statistical techniques have largely replaced the manual analysis of the survey data and the manual farm aggregation/comparison. Statistical techniques using multivariate analysis are one of the most commonly applied approaches to construct farm typologies, e.g. [ 34 – 41 ]. These approaches apply data-reduction techniques, i.e. combining multiple variables into a smaller number of ‘factors’ or ‘principal components’, and clustering algorithms on large databases.
Typologies are generally conditioned by their objective, the nature of the available data, and the farm sample [ 42 ]. Thus, the methodological decisions on data collection, variable selection, data-reduction and clustering have a large impact on the resulting typology. Furthermore, typologies tend to remain a research tool that is not often used by local stakeholders [ 42 ]. In order to make typologies more meaningful and used, we argue that typology development should involve local stakeholders (iteratively) and be guided by a hypothesis on the local agricultural features and the criteria for differentiating farm household systems. This hypothesis can be based on perceptions of, and theories on farm household functioning, constraints and opportunities within the local context, and the drivers and mechanisms of differentiation [ 43 – 44 ]. Drivers of differentiation can include biophysical conditions, and the variation therein, as well as socio-economic and institutional conditions such as policies, markets and farm household integration in value chains.
The objective of this article is to present a methodological approach for typology construction on the basis of an explicit hypothesis. Building on a case study of Zambia, we investigate how typology users’—here, two development projects—objectives and initial hypothesis regarding farm household diversity, impacts typology construction and consequently, its results. Based on this we propose a methodological framework for typology construction that utilizes a combination of expert knowledge, participatory approaches and multivariate statistical methods. We further discuss how an iterative process of hypothesis-refinement and typology development can inform participatory learning and dissemination processes, thus fostering specific adoption in addition to the fine-tuning and effective out-scaling of innovations.
Materials and methods
Typology construction in the eastern province, zambia.
We use a sample of smallholder farms in the Eastern Province of Zambia to illustrate the importance of hypothesis formulation in the first stages of the typology development. This will be done by showing the effects of using different hypotheses on the typology construction process and its results, while using the same dataset. Our experience with typology construction with stakeholders in Zambia made clear that i) the initial typology objective and hypotheses were not clearly defined nor made explicit at the beginning of the typology development, and ii) iterative feedbacks with local experts are needed to confirm the validity of the typology results.
The typology construction work in the Eastern Province of Zambia ( Fig 1 ) was performed for a collaboration between SIMLEZA (Sustainable Intensification of Maize-Legume Systems for the Eastern Province of Zambia) and Africa RISING (Africa Research in Sustainable Intensification for the Next Generation; https://africa-rising.net/ ); two research for development projects operating in the area. Africa RISING is led by IITA (International Institute of Tropical Agriculture; http://www.iita.org/ ) and aims to create opportunities for smallholder farm households to move out of hunger and poverty through sustainably intensified farming systems that improve food, nutrition, and income security, particularly for women and children, and conserve or enhance the natural resource base. SIMLEZA is a research project led by CIMMYT (International Maize and Wheat Improvement Center; http://www.cimmyt.org/ ) which, amongst other objectives, seeks to facilitate the adoption and adaptation of productive, resilient and sustainable agronomic practices for maize-legume cropping systems in Zambia’s Eastern Province. The baseline survey data that was used was collected by the SIMLEZA project in 2010/2011. The survey dataset ( S1 Dataset ) was used to develop three typologies using three different objectives, to investigate the effects that different hypotheses have on typology results.
- PPT PowerPoint slide
- PNG larger image
- TIFF original image
https://doi.org/10.1371/journal.pone.0194757.g001
Zambia’s Eastern Province is located on a plateau with flat to gently rolling landscapes at altitudes between 900 to 1200 m above sea level. The growing season lasts from November to April, with most of the annual rainfall of about 1000 mm falling between December and March [ 45 ]. Known for its high crop production potential, Eastern Zambia is considered the country’s ‘maize basket’ [ 46 ]. However, despite its high agricultural potential ( Table 1 ), the Eastern Province is one of the poorest regions of Zambia, with the majority of its population living below the US$1.25/day poverty line [ 47 ].
https://doi.org/10.1371/journal.pone.0194757.t001
The SIMLEZA baseline survey captured household data of about 800 households in three districts, Lundazi, Chipata and Katete ( Fig 1 ). Although smallholder farmers in these districts grow similar crops, including maize, cotton, tobacco, and legumes (such as cowpeas and soy beans), the relative importance of these crops, the livestock herd size and composition, and their market-orientation differ substantially, both between and within districts. The densely populated Chipata and Katete districts (respectively, 67.6 and 60.4 persons/km 2 ) [ 48 ] located along the main road connecting the Malawian and Zambian capital cities are characterised by highly intensive land use, relatively small land holdings and relatively small livestock numbers. The Lundazi district, by contrast, has rather extensive land-use and a low population density (22.4 persons/km 2 ) [ 48 ], and is characterised by large patches of unused and fallow lands, which are reminiscent of land-extensive slash and burn agriculture.
Alternative typology objectives and hypotheses
Iterative consultations with some of the SIMLEZA-project members in Zambia, informed the subsequent construction of three farm household typologies, all based on different objectives. The objective of the first typology (T1) was to classify the surveyed smallholder farms on the basis of the most distinguishing features of the farm structure (including crop and livestock components). The first hypothesis was that farm households could be grouped by farm structure, captured predominantly in terms of wealth indicators such as farm and herd size. When the resulting typology was not deemed useful by the local project members (because it did not focus enough on the cropping activities targeted by the project), a second typology was constructed with a new objective and hypothesis. The objective of the second typology (T2) was to differentiate farm households in terms of their farming resources (land and labour) and their integration of grain legumes (GL). The second hypothesis was that farming systems could be grouped according to their land and labour resources and their use of legumes, highlighting the labour and land resources (or constraints) of the groups integrating the most legumes. But again the resulting typology did not satisfy the local project members; they expected to see clear differences in the typology results across the three districts (Lundazi, Chipata and Katete), as the districts represented rather different farming contexts. Thus for the third typology (T3), the local partners hypothesized that the farm types and the possibilities for more GL integration would be strongly divergent for the three districts, due to differences in biophysical and socio-economic conditions ( Table 1 ). The hypothesis used was that the farm households could be grouped according to their land and labour resources and their use of legumes and that the resulting types would differ between the three districts. Therefore, the objective of the third typology focused on GL integration as for T2, but for the three districts separately (T3-Lundazi, T3-Chipata and T3-Katete).
Multivariate analysis on different datasets
On the basis of the household survey dataset, five sub-databases were extracted which corresponded to the three subsets of variables chosen to address the different typology objectives ( Table 2 ). The first two sub-databases included all three districts (T1 and T2) and the last three sub-databases corresponded to the subdivision of the data per district (T3). In each sub-database, some surveyed farms were identified as outliers and others had missing values; these farms were excluded from the multivariate analysis. A Principal Component Analysis (PCA) was conducted to reduce each dataset into a few synthetic variables, i.e. the first principal components (PCs). This was followed by an Agglomerative Hierarchical Clustering using the Ward’s minimum-variance method, which was applied on the outcomes of the PCA (PCs’ scores) to identify clusters. The Ward’s method minimizes within-cluster variation by comparing two clusters using the sum of squares between the two clusters, summed over all variables [ 49 ]. The number of clusters (i.e. farm types) was defined using the dendrogram shape, in particular the decrease of the dissimilarity index (“Height”) according to the increase of the number of clusters. The resulting types were interpreted by the means of the PCA results and put into perspective with the knowledge of the local reality. All the statistical analyses were executed in R (version 3.1.0, ade4 package; [ 50 ]).
https://doi.org/10.1371/journal.pone.0194757.t002
Results and discussion on the contrasting typologies
Of the five PCAs, the first four principal components explained between 55% and 64% of the variability in the five sub-databases (64, 55, 55, 57 and 62% for respectively T1, T2, and T3-Lundazi, T3-Chipata and T3-Katete). The four PCs are most strongly correlated to variables related to farm structure, labour use and income. The variables most correlated with PC1 were the size of the farmed land ( oparea ; five PCAs), the number of tropical livestock units ( tlu ; four PCAs), the cost of the hired labour ( hirecost ; four PCAs) and total income or income generated by cropping activities ( totincome or cropincome ; five PCAs) (Figs 2 , 3 and 4 ).
The red colour variables are the most explanatory of the horizontal axis (PC1); those in blue are the most explanatory variables of vertical axes (PC2, PC3 and PC4), thus defining the gradients.
https://doi.org/10.1371/journal.pone.0194757.g002
https://doi.org/10.1371/journal.pone.0194757.g003
The red coloured variables are the most explanatory of the horizontal axis (PC1); those in blue are the most explanatory variables of vertical axes (PC2) and those in violet are variables correlated with both PC1 and PC2.
https://doi.org/10.1371/journal.pone.0194757.g004
The following discriminant dimensions were more related to the specific objective of each typology. For the typology T1, PC1, PC2, PC3 and PC4 were related to the most important livestock activity (i.e. contribution of each livestock type to the total tropical livestock units (TLU) represented by cattleratio , chickenratio , pigratio and smallrumratio respectively), thus distinguishing the farms by their dominant livestock type ( Fig 2 ). The six resulting farm types are organized along a land and TLU gradient, from type 1 (larger farms) to type 6 (smaller farms). In addition to land and TLU, the farm types differed according their herd composition: large cattle herds for type 1 and type 2, mixed herds of cattle and small ruminants or pig for type 3, mostly pigs for type 4, small ruminant herds for type 5 and finally, mostly poultry for type 6 ( Fig 2 ).
For the typology T2, the labour constraints for land preparation ( preplabrat ) and weeding ( weedlabrat ) determined the second discriminant dimension (PC2), while the legume features (experience, legume evaluation and cropped legume proportion represented by legexp , legscore and legratio respectively) only appeared correlated to PC3 or PC4. However, these two last dimensions were not useful to discriminate the surveyed farms, since the farm types tended to overlap in PC3 and PC4 ( Fig 3 ). Therefore, while these were variables of interest (i.e. targeted in the T2-typology objective), no clear difference or trend across farm types was identified for the legume features in the multivariate results ( Fig 3 ). The five resulting farm types were also organized along a land and TLU gradient, which was correlated with the income generated per year from cropping activities ( cropincome ) and the hired labour ( hiredcost ), ranging from type 1 (higher resource-endowed farms employing a large amount of external labour) to type 5 (resource-constrained farms, using almost only family labour). Furthermore, type 4 and type 5 were characterized by their most time-consuming cropping activity, weeding and soil preparation respectively ( Fig 3 ).
For the typology T3, Lundazi, Chipata and Katete farms tended to primarily be distinguished according to a farm size, labour and income gradients ( Fig 4 ). The number of the livestock units ( tlu ) remained an important discriminant dimension that was correlated to either PC1 or PC2 in the three districts ( Fig 4 ). Although the selection of the variables was made to differentiate the farmers according to their legume practices ( legratio ), this dimension appeared only in PC3 or PC5, explaining less than 12% of the variability surveyed. Moreover, similarly to T2, the farm types identified were not clearly distinguishable on these dimensions. Thus, besides the clear differences among farms in terms of their land size, labour and income (PC1), farms were primarily segregated by their source of income, i.e. cropping activities ( cropincratio ) vs. animal activities ( anlincratio ) ( Fig 4 ). In T3-Lundazi, T3-Chipata and T3-Katete, the resulting farm types were also organized along a resource-endowment gradient, from type 1 (higher resource-endowed farms) to type 6 (resource-constrained farms). Additionally, they were distinguished by their main source of income: i) for T3-Lundazi, large livestock sales for type 2, mostly crop products sales (low livestock sales) for types 1, 3, 4, and 6, and off-farm activities for type 5; ii) for T3-Chipata, crop revenues for type 3, livestock sales for type 2 and mixed revenues from crop sales and off-farm activities for type 1, 4 and 5; iii) for T3-Katete, crop revenues for types 3 and 5, mixed revenues from crop sales and off-farm activities for type 1, 2 and 4, and mixed revenues from livestock sales and off-farm activities for type 6 ( Fig 4 ).
The overlap of the typologies is presented in Figs 5 and 6 . A strong overlap is indicated by a high percentage (and darker shading) in only one cell per row and column (Figs 5b and 6 ). The overlap between the presented typologies was not clear (Figs 5 and 6 ) despite the importance of farm size, labour and income in the first principle component (PC1) in all typologies. The best overlap was observed between the typology T2 and the typology T3 for the Chipata district (T3-Chipata). Moreover, the types 1 (i.e. farms with larger farm area, higher income and more labour used) overlapped between typologies: 69% of type 1 from T2 belonged to type 1 from T1 ( Fig 5 ) and, 100 and 89% of the types 1 from Lundazi and Katete, respectively, belonged to type 1 from T2 ( Fig 6 ). The majority of the unclassified farms (i.e. farms present in T1 but detected as outliers in T2 and T3) were related to the ‘wealthier’ types, type 1 and type 2 (Figs 5 and 6 ).
The ‘unclassified’ farms are farms that were included in T1 but were detected as outliers for T2. Fig 6a illustrates the overlapping between T1 and T2, comparing the individual position each farm in the two dendrogram of the two typologies, while Fig 6b quantifies the percentage of overlap between the two typologies.
https://doi.org/10.1371/journal.pone.0194757.g005
The intensity of the red colouring indicates the percentage of overlap.
https://doi.org/10.1371/journal.pone.0194757.g006
For the all the typologies (T1, T2, T3-Lundazi, T3-Chipata and T3-Katete), the main discriminating dimension was related to resource endowment: farm structure in terms of land area and/or animal numbers, labour use and income, which has been observed in many typology studies. In this case, the change in typology objective and the corresponding inclusion of variables from the dataset on legume integration (e.g. legratio ) did not result in a clearer separation among farm types in T2 when compared to T1. The importance of farm structure variables in explaining the datasets’ variability (Figs 2 , 3 and 4 ) resulted in overlap among typologies regarding the larger, more well-endowed farms, that comprised ca. 10% of the farms, but for types representing medium- and resource-constrained farms the overlap between typologies was limited (Figs 5 and 6 ).
The difference between typologies T2 and T3 relates to a scale change, i.e. from province to district scale. Zooming in on a smaller scale allows amplification of the local diversity. Indeed, the range of variation could be different at provincial level (i.e. here three districts were merged) when compared to the district level ( Table 1 ). Thus narrowing the study scale makes intra-district variability more visible, and potentially reveals new types leading to a segregation/splitting of one province-level type into several district-level types ( Fig 7 ). The differences between typologies that arise from scale differences highlight the importance of scale definition when investigating out-scaling and up-scaling of target interventions.
Distribution of observations of a quantitative variable (e.g. farm area) at the province level (level 1) and at the district level (level 2). The different colours are associated with different values classes within the variable. Zooming in from scale 1 to scale 2, magnifies the variation within the district, potentially revealing new classes.
https://doi.org/10.1371/journal.pone.0194757.g007
Methodological framework for typology construction
The proposed methodological framework ( Fig 8 ) aims to integrate statistical and participatory methods for hypothesis-based typology construction using quantitative data, to create a typology that is not only statistically sound and reproducible but is also firmly embedded in the local socio-cultural, economic and biophysical context. From a heterogeneous population of farms to the grouping into coherent farm types, the step-wise structure of this typology construction framework comprises the following steps: i) precisely state the objective of the typology; ii) formulate a hypothesis on farming systems diversity; iii) design a sampling method for data collection; iv) select the variables characterizing the farm households; v) cluster the farm households using multivariate statistics; and vi) verify and validate the typology result with the hypothesis and discuss the usability of the typology with (potential) typology users. This step-wise process can be repeated if the multivariate analysis results do not match the diversity of the targeted population as perceived by the validation panel and typology users ( Fig 8 ).
https://doi.org/10.1371/journal.pone.0194757.g008
Typology objectives, target population and expert panel
A farm typology is dependent on the project goals and the related research, innovation or development question [ 39 ], which determine the typology objective. This will affect the delineation of the system under study, i.e. the target population size, in socio-institutional and geographical dimensions. The socio-institutional aspects that affect the size of the target population include criteria such as the type of entities involved (e.g., farms, rural households or individual farmers) and some initial cut-off criteria. These cut-off criteria can help in reducing the population size, such as a minimum or maximum structural size or the production orientation (e.g., food production, commercial and/or export-oriented; conventional or organic). The geographical dimension will affect the size of the target population by determining the spatial scale of the study, which in turn can be influenced by natural or administrative boundaries or by biophysical conditions such as suitability for farming. The scale at which the study is conducted can amplify or reduce the diversity that is encountered ( Fig 7 ).
Stakeholders (including farmers) with a good knowledge of the local conditions and the target population and its dynamics can inform the various steps of the typology development, forming an expert panel for consultation throughout the typology construction process. The composition of the panel can be related to the objective of the typology. Existing stakeholder selection techniques, e.g. [ 51 – 52 ] can be used for the identification and selection of panel experts. The group of experts can be split into a ‘design panel’ that is involved in the construction of the typology, and a ‘validation panel’ for independent validation of the result (cf. Section ‘Hypothesis verification and typology validation’). Finally, involving local stakeholders who are embedded in the target population may trigger a broader local involvement in the research process, facilitating data collection and generating more feedback and acceptance and usability of the results [ 43 ].
Hypothesis on typology structure
A multiplicity of typologies could describe the same faming environment depending on the typology objective and thus the selected criteria for typology development [ 43 ]. In the proposed framework ( Fig 8 ), the typology development is based on the formulation of a hypothesis on the diversity of the target population by the local experts, the design panel, in order to guide the selection of variables to be used in the multivariate statistical analysis. The hypothesis relates to the main features of local agriculture, stakeholder assumptions and theories on farm functioning and livelihood strategies in the local context, and on their interpretation of the relevant external forces and mechanisms that can differentiate farm households. Heterogeneity can emerge in response to very diverse socio-cultural, economic and biophysical drivers that can vary in significance within the studied region. In addition to the primary discriminatory features, the hypothesis can also make the following features explicit; the most prominent types of farms that are expected, their relative proportions, the most crucial differences between the farm types, the gradients along which the farms may be organized and possible relationships or correlations between specific farm characteristics. These perceptions and theories about the local diversity in rural livelihoods and farm enterprises are often present but are not always made explicit; the hypothesis formulation by the design panel is meant to make these explicit and intelligible to the external researchers. Hence, the design panel is expected to reflect on the drivers and features of the farm diversity encountered in the targeted population and reach a consensus on the main differentiating criteria and, ideally, have a preliminary inventory of the expected farm types.
An example of a hypothesis formulated by local experts could be that farms are distinguished by the size of the livestock herd, their reliance on external feeds and their proximity to livestock sale-yards; thus, there may be a gradient from large livestock herds, very reliant on external feeds, and close the sale-yards, to small herds, less reliant on external feeds further away from sale-yards. The discussions of the design panel are guided by the general typology objective. The hypothesis can further be informed by other participatory methods, previous studies in the area or by field observations. This allows for a wide range of information to be used for the hypothesis consolidation. Most of the information compiled in the formulated hypothesis is qualitative, but can also be informed by maps and spatial data in geographical information systems. The statistical analysis that follows will use quantitative features and boundaries of the farm entities in the study region.
Data collection, sampling and key variables selection
The creation of a database on the target population is an essential step in the typology construction based on quantitative methods. The farm sampling needs to capture the diversity of the target population [ 41 ]. The size of the sample and the sampling method [ 53 ] affect the proportion of farms belonging to each resulting farm type; for instance a very small farm type is likely to be absent in a reduced sample. Thus the sampling process, notably the choice of sample size, should be guided by the initial hypothesis.
The survey questionnaire needs to reflect the hypothesis formulated in the previous step, i.e. containing at least the main features and differentiation criteria listed by the design panel. However, the survey can be designed to capture the entire farming system [ 1 , 8 ], collecting information related to all its components (i.e. household/family, cropping system, livestock system), their interactions, and the interactions with the biophysical environment in which the farming system is located (e.g. environmental context, economic context, socio-cultural context). The anticipated analytical methods to be applied, especially the multivariate techniques, also guide decisions about the nature of data (e.g. categorical or continuous data) to collect.
Finally, the selection of key variables for the multivariate analysis is adapted to the typology objective following the previous step of exchanges with the expert panel and hypothesis formulation. Together researchers and the expert design panel select the key variables that correspond to the formulated hypothesis. These selected key variables constitute a sub-database of the collected data, which will be used for the multivariate analysis. Kostrowicki [ 54 ] advised to favour integrative variables (i.e. combining several attributes) rather than elementary variables. The number of surveyed entities has to be larger than the number of key variables; a factor five is often advised [ 49 ].
Multivariate statistics
Multivariate statistical analysis techniques are useful to identify explanatory variables (discriminating variables) and to group farms into homogeneous groups that represent farm types. A standard approach is to apply a data-reduction method on the selected set of variables (key variables) to derive a smaller set of non-correlated components or factors. Then clustering techniques are applied to the coordinates of the farms on these new axes. Candidate data-reduction techniques include: i) Principal Component Analysis for quantitative (continuous or discrete) variables, e.g. [ 1 , 36 , 55 ]; ii) Multiple Correspondence Analysis for categorical variables, e.g. [ 33 ]; iii) Multiple Factorial Analysis for categorical variables organized in multi-table and multi-block data sets, e.g. [ 34 ]; iv) Hill and Smith Analysis for mixed quantitative and qualitative variables, e.g. [ 27 ]; v) Multidimensional scaling to build a classification configuration in a specific dimension, e.g. [ 41 , 56 ]; or vi) variable clustering to reduce qualitative and quantitative variables into a small set of (quantitative) “synthetic variables” used as input for the farm clustering, e.g. [ 57 ]. Although the number of key variables is reduced, the variability of the dataset is largely preserved. However, as a result of the multivariate analysis, not all the key variables selected will necessarily be retained as discriminating variables.
Subsequently, a classification method or clustering analysis (CA) can be applied on these components or factors to identify clusters that minimize variability within clusters and maximize differences between clusters. There are two methods of CA commonly used: i) Non-hierarchical clustering, i.e. a separation of observations/farms space into disjoint groups/types where the number of groups (k) is fixed; and ii) Hierarchical clustering, i.e. a stepwise aggregation of observations/farms space into disjoint groups/types (first each farm is a group all by itself, and then at each step, the two most similar groups are merged until only one group with all farms remains). The Agglomerative Hierarchical Clustering algorithm is often used in the typology construction process, e.g. [ 24 , 34 , 35 , 41 , 55 ]. The two clustering methods can be used together to combine the strengths of the two approaches, e.g. [ 15 , 58 , 59 ]. When used in combination, hierarchical clustering is used to estimate the number of clusters, while non-hierarchical clustering is used to calculate the cluster centres. Some statistical techniques exist to support the choice of the number of clusters and to test the robustness of the cluster results, such as clustergrams, slip-samples or bootstrapping techniques [ 49 , 60 , 61 ]. The “practical significance” of the cluster result has to be verified [ 49 ]. In practice, a limited number of farm types is often preferred, e.g. three to five for Giller et al. [ 8 ], and six to fifteen for Perrot and Landais [ 42 ].
Hypothesis verification and typology validation
The resulting farm types have to be conceptually meaningful, representative of and easily identifiable within the target population [ 62 ]. The farm types resulting from the multivariate and cluster analysis are thus compared with the initial hypothesis (cf. Section ‘Hypothesis on typology structure’; Fig 8 ), by comparing the number of types defined, their characteristics and their relative proportions in the target population. The correlations among variables that have emerged from the multivariate analysis can also be checked with local experts. This has to be part of an iterative process where the results of the statistical analysis are compared with the reality of the target population in discussion with the expert panels ( Fig 8 ). When involved in this process, local stakeholders can help in understanding the differences between the hypothesis and the results of the statistical analysis. In the case of results that deviate from the hypothesis, the multivariate and cluster analysis may need to be repeated using a different selection of variables, by examining outliers or the distributions of the selected variables. The discussion and feedback sessions with local stakeholders (‘design panel’ of experts) may need to be re-initiated until no new information emerges from the feedback sessions. Later, the driving effects of external conditions (such as biophysical and socio-economic features) on farming systems differentiation can be tested statistically analysing the relationships between the resulting farm types and external features variables.
Finally, when the design panel recognizes the farm types identified with the statistics analysis, an independent validation of the typology results and its usability by potential users is desired ( Fig 8 ). Preferably, to allow an independent verification of the constructed typology, a ‘validation panel’ should be independent of the design panel that formulated the hypothesis. The resulting typology is presented to the validation panel whose members are asked to compare it with their own knowledge on the local farming systems diversity. The objective of this last step is to, in hindsight, demonstrate that the simplified representation reflected in the typology is a reasonable representation of the target population and that the typology satisfies the project goals. Some criteria were proposed to support the validation process of the typology by the validation panel ([ 3 ] cited by [ 4 ]): i) Clarity –farm types should be clearly defined and thus understandable by the local stakeholders (including the validation panel); ii) Coherence –examples of existing farms should be identifiable by the local experts for each farm type, and, any gradient highlighted during the hypothesis formulation should be recognizable in the typology results; iii) Exhaustiveness –most of the target population should be included in the resulting farm types; iv) Economy –the typology should include only the necessary number of farm types to represent most of the target population diversity; and, v) Utility and acceptability –the typology should be accepted and judged as useful by the stakeholders (especially by the validation panel), for instance by providing diagnostics on the target population like the production constraints per identified farm type.
Thus, eventually the typology construction has gone through two triangulation processes: expert triangulation (by design panel and validation panel) and methodological triangulation (using statistical analysis and participatory methods).
General discussion
Importance of the learning process.
The hypothesis-based typology construction process constitutes a learning process for the stakeholders involved such as local experts, local policy makers and research for development (R4D) project leaders, and for the research team that develops the typology. For the local stakeholders, the process could lead to a more explicit articulation of the perceived (or theorised) diversity within the farming population and use of the constructed typology. The process involves an exchange of ideas and notions, and provides incentives to find consensus among different perspectives. Obviously, the resulting typology itself allows for reflection on the actual differences between farming households and on opportunities for farm development. By recognizing different farm types and the associated distributions of characteristics, typologies could also help farmers to identify development pathways through a comparison of their own farm household system with others ( Where am I ?), identifying successful tactics and strategies of other farm types ( What can I change ?) and their performances ( What improvement can I expect ?).
The research team not only gains a quantitative insight into the diversity and its distribution from the developed typology, but also obtains a detailed qualitative view on the target population, particularly if selected farms representing the identified farm types are studied in more detail. Indeed, the interactions with local experts and discussions about the interpretation of the typology could also provide insights into, for instance, socio-cultural dynamics and power relations within the farming population and local institutions, as well as other aspects not necessarily collected during the survey. For example, social mechanisms can become more visible to the researcher when the relationships between farm types are described during the discussions with the expert panels.
Farm/household dynamics
Farms are moving targets [ 8 ], while typologies based on one-time measurements or data collection surveys provide only a snapshot of farm situations at a certain period of time [ 54 ]. Due to farm dynamics, these typologies could become obsolete and hence it is preferable to regularly update typologies [ 28 , 29 ].
However, it has been argued that typologies based on participatory approaches tend to be more stable in time [ 29 ], because they are more qualitative and therefore could also integrate the local background and accumulated experience from the local participants. Consequently, the resulting qualitative types change less over time, although individual farms may change from one farm type to another [ 26 , 34 ]. Thus, the framework presented here would allow combining the longer-term (and more qualitative) vision of the local diversity from the local stakeholders including the general observed trend into the hypothesis formulation, and the shorter-term situation of individual households.
Typologies as social constructs
It is important to recognize that typology construction is a social process, and therefore that typologies are social constructs. The perspectives and biases of the various stakeholders in the typology construction process, including methodological decision-making by the research team (such as the selection of the key variables, selection of principal components and clusters, and their interpretation, etc.) shape the resulting typologies, and subsequently their usability in research and policy making. Consequently, participatory typology construction may be considered as an outcome of negotiation processes between different stakeholders aiming to reach consensus on the interpretation of heterogeneity within the smallholder farming population [ 63 ]. The consensus-oriented hypothesis formulation described here is also a way to mitigate the dominance of particular stakeholders in shaping the typology constructing process. Multiple consultations, feedbacks to the local stakeholders and the typology validation by the independent assessors (the validation panel) further limit the dominant influence of more powerful stakeholders.
Typology versus simpler farm classification
Taking into account multiple features of the farm household systems, typologies facilitate the comparison of these complex systems within a multi–dimensional space [ 7 ]. However, with multivariate analysis, the underlying structure of the data defines the ranking of dimensions in terms of their power to explain variability. Therefore, as shown previously (cf. Section ‘ Results and discussion on the contrasting typologies ’), there is no guarantee that the multivariate analysis will highlight one specific dimension targeted by the researcher or the intervention project. Thus, if the goal is simply to classify farms based on one or two dimensions, a simpler classification based only on one or two variables may suffice to define useful farm classes for the intervention project. For example, an intervention project focused on supporting new legume growers, could classify farm(er)s on their legume cultivated area and their years of experience with legume cultivation only. In that case, we would not use the term farm typology but rather farm classification.
Farm types and individual farmers
Farm typologies are groupings based on some selected criteria and the farm types tend to be homogeneous in these criteria, with some intra-group variability. Thus, typologies are useful for gathering farmers for discussion such that one would have groups of farmers who manage their farms similarly, have similar general strategies, or face similar constraints and have comparable opportunities. This is how typologies can be especially helpful in targeting interventions to specific farm types. However, individual farm differences remain; criteria that were not included in the typology and also individual farmer characteristics, such as values, culture, background or personal goals and projects can account for the observed individual farm differences. Thus, when interacting with individual farmers, much more farm-specific, social (household and community) and personal features can arise, for example their risk aversion or other hidden (non-surveyed) issues that would influence their adoption of novel interventions. This highlights the intra-type heterogeneity and also exposes the potential pitfalls when targeting interventions to be adopted by farmers.
Agricultural research and development projects that evaluate or promote specific agricultural practices and technologies usually provide a particular set of interventions, for instance oriented towards soil conservation, improvement of cropping systems or animal husbandry. The focus and aims of such projects shape also the differentiation of the project’s target population into farm types that are often used for targeting interventions. In addition, a project’s specific impact and out-scaling objectives influence the number of farmers targeted and the spatial scale at which the interventions need to be disseminated, thus influencing the farmer selection strategy. Constructing farm typologies can help to get a better handle on the existing heterogeneity within a targeted farming population. However, the methodological decisions on data collection, variable selection, data-reduction and clustering can bear a large impact on the typology construction process and its results. We argue that the typology construction should therefore be guided by a hypothesis on the diversity and distribution of the targeted population based both on the demands of the project and on prior knowledge of the study area. This will affect the farming household selection strategy, the data that will be collected and the statistical methods applied.
We combined hypothesis-based research, context specificities and methodological issues into a new framework for typology construction. This framework incorporates different triangulation processes to enhance the quality of typology results. First, a methodological triangulation process supports the fusion of i) ‘snapshot’ information from household surveys with ii) long-term qualitative knowledge derived from the accumulated experience of experts. This fusion results in the construction of a contextualized quantitative typology, which provides ample opportunities for exchange of knowledge between experts (including farmers) and researchers. Second, an expert triangulation process involving the ‘design panel’ and the ‘validation panel’, results in the reduced influence of individual subjectivity. As shown in the Zambian illustration, the typology results were highly sensitive to the typology objective and the corresponding selection of key variables, and scale of the study. Changing from one set of variables to another or, from one scale to another, resulted in the surveyed farms shifting between types (Figs 5 and 6 ). We have thus highlighted the importance of having a well-defined (and imbedded in local knowledge) typology objective and hypothesis at the beginning of the process. Taking into account both triangulation processes in the presented framework, we conclude that the framework facilitates a solid typology construction that provides a good basis for further evaluation of entry points for system innovation, exploration of tradeoffs and synergies between multiple (farmer) objectives and to inform decisions on improvements in farm performance.
Supporting information
S1 dataset. data used for the typology construction..
https://doi.org/10.1371/journal.pone.0194757.s001
Acknowledgments
The fieldwork of this study was conducted within the Africa RISING/SIMLEZA research-for-development program in Zambia that is led by the International Institute of Tropical Agriculture (IITA). The research was partly funded by the United States Agency for International Development (USAID; https://www.usaid.gov/ ) as part of the US Government’s Feed the Future Initiative. The contents are the responsibility of the producing organizations and do not necessarily reflect the opinion of USAID or the U.S. Government.
In addition, we would like to thank the CGIAR Research program Humidtropics and all donors who supported this research through their contributions to the CGIAR Fund. For a list of Fund donors please see: https://www.cgiar.org/funders/ .
- View Article
- Google Scholar
- 3. Legendre R. Dictionnaire actuel de l'éducation. Montréal, Guérin; 2005. French.
- 4. Larouche C. La validation d’une typologie des conceptions des universités en vue d’évaluer leur performance. Doctoral dissertation, Université Laval, Québec. 2011. www.theses.ulaval.ca/2011/27956/27956.pdf . French.
- 6. Capillon A. Typologie des exploitations agricoles: contribution à l’étude régionale des problèmes techniques. Tomes I et II. Doctoral dissertation, AgroParisTech (Institut National Agronomique Paris-Grignon). 1993. French.
- 18. Timler C, Michalscheck M, Alvarez S, Descheemaeker K, Groot JCJ. Exploring options for sustainable intensification through legume integration in different farm types in Eastern Zambia. In: Ӧborn I, Vanlauwe B, Phillips M, Thomas R, Atta-Krah K. Sustainable Intensification in Smallholder Agriculture: An Integrated Systems Research Approach. Routledge, Taylor & Francis Group 2016.
- PubMed/NCBI
- 23. Albaladejo C, Duvernoy I. La durabilité des exploitations agricoles de fronts pionniers vue comme une capacité d'évolution. In: Journées du Programme Environnement-Vie-Société ‘les Temps de l’Environnement’. Toulouse, France, 5–7 November 1997; 203–210. French.
- 33. Kostrowicki J, Tyszkiewicz W, editors. Agricultural Typology: Selected Methodological Materials. Instytut Geografii Polskiej Akademii Nauk. 1970.
- 40. Mbetid-Bessane E, Havard M, Nana PD, Djonnewa A, Djondang K, Leroy J. Typologies des exploitations agricoles dans les savanes d’Afrique centrale: un regard sur les méthodes utilisées et leur utilité pour la recherche et le développement. In: Savanes africaines: des espaces en mutation, des acteurs face à de nouveaux défis. Actes du colloque, Garoua, Cameroun, Cirad-Prasac. 2003. French.
- 42. Perrot C, Landais E. Exploitations agricoles: pourquoi poursuivre la recherche sur les méthodes typologiques?. Cahiers de la Recherche Développement. 1993; 33. French.
- 46. Aregheore EM. Country Pasture/Forage Resource Profiles Zambia. FAO. 2014. http://www.fao.org/ag/agp/AGPC/doc/Counprof/zambia/zambia.htm . Accessed: 15 September 2016.
- 47. Malapit HJ, Sproule K, Kovarik C, Meinzen-Dick RS, Quisumbing AR, Ramzan F, et al. Measuring progress toward empowerment: Women’s empowerment in agriculture index: Baseline report. International Food Policy Research Institute. 2014.
- 48. Central Statistics Office. 2011. http://www.zamstats.gov.zm . Accessed: 15 September 2015.
- 49. Hair JF, Black WC, Babin BJ, Anderson RE. Multivariate Data Analysis: A Global Perspective, Seventh Edition, Pearson. 2010.
- 53. Kumar R. Research methodology: a step-by-step guide for beginners, Fourth Edition. Sage, Los Angeles. 2014. http://www.uk.sagepub.com/kumar4e/
- 60. Mucha HJ. Assessment of Stability in Partitional Clustering Using Resampling Techniques. Archives of Data Science (Online First) 1. 2014.
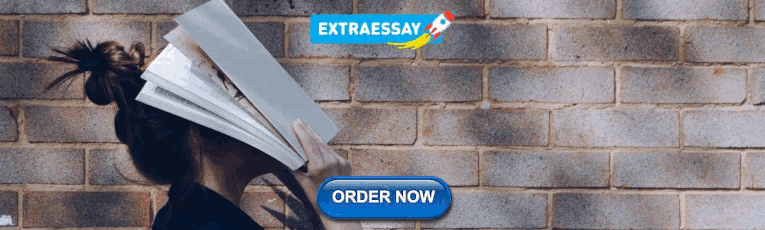
IMAGES
VIDEO
COMMENTS
McKinsey consultants follow three steps in this cycle: Form a hypothesis about the problem and determine the data needed to test the hypothesis. Gather and analyze the necessary data, comparing ...
And, being hypothesis-driven was required to have any success at McKinsey. A hypothesis is an idea or theory, often based on limited data, which is typically the beginning of a thread of further investigation to prove, disprove or improve the hypothesis through facts and empirical data. The first step in being hypothesis-driven is to focus on ...
5. Phrase your hypothesis in three ways. To identify the variables, you can write a simple prediction in if…then form. The first part of the sentence states the independent variable and the second part states the dependent variable. If a first-year student starts attending more lectures, then their exam scores will improve.
The Hypothesis Progression Framework. ... So, for example, let's imagine that, based on some early conversations and market research, we have a hypothesis that office administrators of small ...
Recruit the users you want to target, have a time frame, and put the design in front of the users. 5. Learn and build. You just learned that the result was positive and you're excited to roll out the feature. That's great! If the hypothesis failed, don't worry—you'll be able to gain some insights from that experiment.
Practicing Hypothesis-Driven Development is thinking about the development of new ideas, products and services - even organizational change - as a series of experiments to determine whether an expected outcome will be achieved. ... Our teachers had a framework for helping us learn - an experimental approach based on the best available ...
Here's the process flow: How Might We technique → Dot voting (based on estimated/assumptive impact) → converting into a hypothesis → define testing methodology (research method + success/fail criteria) → impact effort scale for prioritizing → test, learn, repeat. Once the hypothesis is proven right, the feature is escalated into the ...
Make observations. Formulate a hypothesis. Design an experiment to test the hypothesis. State the indicators to evaluate if the experiment has succeeded. Conduct the experiment. Evaluate the results of the experiment. Accept or reject the hypothesis. If necessary, make and test a new hypothesis.
Developing a conceptual framework in research. A conceptual framework is a representation of the relationship you expect to see between your variables, or the characteristics or properties that you want to study. Conceptual frameworks can be written or visual and are generally developed based on a literature review of existing studies about ...
Exercise 3.2. Researchers have used a number of different metaphors to describe theoretical frameworks. Maxwell (2005) referred to a theoretical framework as a "coat closet" that provides "places to 'hang' data, showing their relationship to other data," although he cautioned that "a theory that neatly organizes some data will leave other data disheveled and lying on the floor ...
A theoretical framework guides the research process like a roadmap for the study, so you need to get this right. Theoretical framework 1,2 is the structure that supports and describes a theory. A theory is a set of interrelated concepts and definitions that present a systematic view of phenomena by describing the relationship among the variables for explaining these phenomena.
4. Formulate your hypothesis. After collecting background information and making a prediction based on your question, plan a statement that lays out your variables, subjects and predicted outcome. Whether you write it as an "if/then" or declarative statement, your hypothesis should include the prediction to be tested.
What is a hypothesis and how can you write a great one for your research? A hypothesis is a tentative statement about the relationship between two or more variables that can be tested empirically. Find out how to formulate a clear, specific, and testable hypothesis with examples and tips from Verywell Mind, a trusted source of psychology and mental health information.
Hypothesis testing example. You want to test whether there is a relationship between gender and height. Based on your knowledge of human physiology, you formulate a hypothesis that men are, on average, taller than women. To test this hypothesis, you restate it as: H 0: Men are, on average, not taller than women. H a: Men are, on average, taller ...
Hypothesis-Driven Hunting with PEAK. With our trusty hypothesis in hand and having applied the ABLE framework, we're ready to proceed through the three phases of the hunt: Prepare, Execute, and Act. Each phase plays a crucial role in unraveling the mysteries hidden in the depths of your network, guiding you from the initial planning stages to the final act of sharing your hard-earned findings.
The formal framework and steps for hypothesis testing are as follows: Identify and define the parameter of interest; Define the competing hypotheses to test ... One is that the significance level is the maximum probability required to reject the null hypothesis; this is based on how the significance level functions within the hypothesis testing ...
8 Steps to Problem-Solving from McKinsey. Solve at the first meeting with a hypothesis. Intuition is as important as facts. Do your research but don't reinvent the wheel. Tell the story behind ...
Hypothesis testing framework We start with a null hypothesis (H 0) that represents the status quo. We develop an alternative hypothesis (H A) that represents our research question (what we're testing for). We conduct a hypothesis test under the assumption that the null hypothesis is true, either via simulation or theoretical methods.
Specifically, we propose a novel software cross-modal retrieval framework named Deep Hypothesis Testing (DeepHT). In DeepHT, to capture the unique semantics of the code's control flow structure, all control flow paths (CFPs) in the control flow graph are mapped to a CFP sample set in the sample space.
Definition: Hypothesis is an educated guess or proposed explanation for a phenomenon, based on some initial observations or data. It is a tentative statement that can be tested and potentially proven or disproven through further investigation and experimentation. Hypothesis is often used in scientific research to guide the design of experiments ...
We will next explore how the Bayesian framework unifies our understanding of perception, human cognition, and decision-making. ... (hypothesis | data). It reflects the degree of belief in a hypothesis based on the information provided by the observed data and is the result of each Bayesian computation. Likelihood: the probability of the data ...
Auxiliary hypothesis. Based on some reason ... Thus, when making a hypothesis via abduction and using our framework, the hypothesis will have an explicit scope when it is made. By doing this, there is less chance that a formulated hypothesis is unclear, ambiguous, and needs amending at a later stage. 11.2. Assisting to clearly state hypotheses
That hypothesis is based both on the typology objective and on prior expert knowledge and theories of the farm diversity in the study area. We present a methodological framework that aims to integrate participatory and statistical methods for hypothesis-based typology construction. ... Using a well-defined hypothesis and the presented ...