- Skip to main content
- Skip to primary sidebar
- Skip to footer
- QuestionPro

- Solutions Industries Gaming Automotive Sports and events Education Government Travel & Hospitality Financial Services Healthcare Cannabis Technology Use Case NPS+ Communities Audience Contactless surveys Mobile LivePolls Member Experience GDPR Positive People Science 360 Feedback Surveys
- Resources Blog eBooks Survey Templates Case Studies Training Help center

Home Market Research
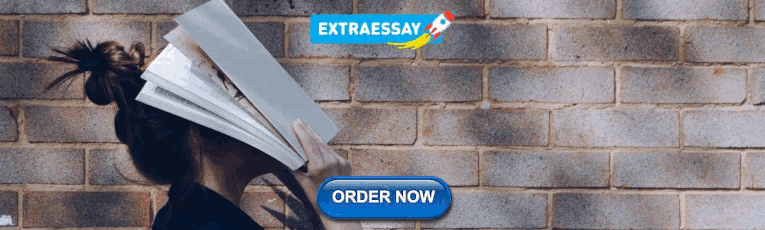
10 Quantitative Data Analysis Software for Every Data Scientist
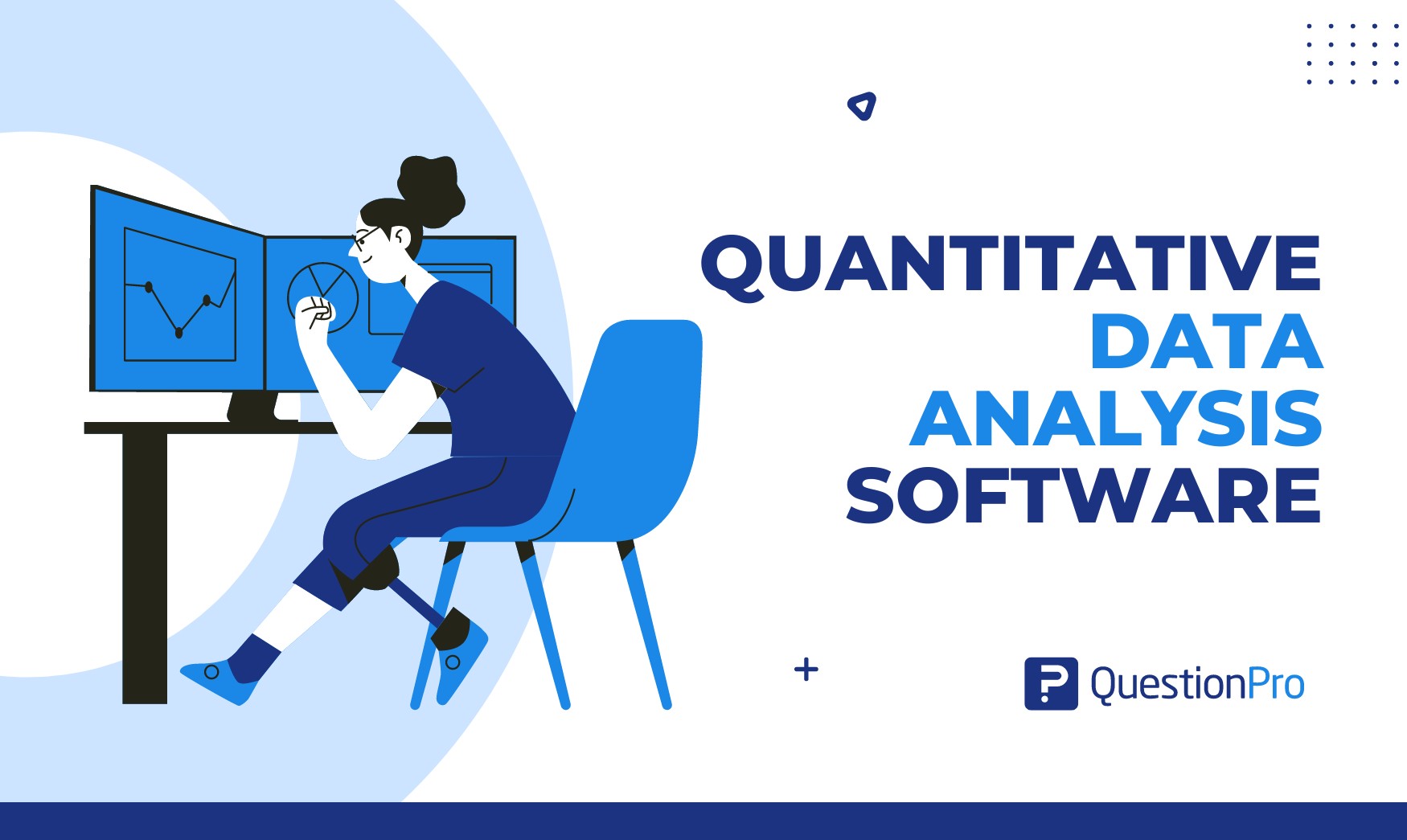
Are you curious about digging into data but not sure where to start? Don’t worry; we’ve got you covered! As a data scientist, you know that having the right tools can make all the difference in the world. When it comes to analyzing quantitative data, having the right quantitative data analysis software can help you extract insights faster and more efficiently.
From spotting trends to making smart decisions, quantitative analysis helps us unlock the secrets hidden within our data and chart a course for success.
In this blog post, we’ll introduce you to 10 quantitative data analysis software that every data scientist should know about.
What is Quantitative Data Analysis?
Quantitative data analysis refers to the process of systematically examining numerical data to uncover patterns, trends, relationships, and insights.
Unlike analyzing qualitative data, which deals with non-numeric data like text or images, quantitative research focuses on data that can be quantified, measured, and analyzed using statistical techniques.
What is Quantitative Data Analysis Software?
Quantitative data analysis software refers to specialized computer programs or tools designed to assist researchers, analysts, and professionals in analyzing numerical data.
These software applications are tailored to handle quantitative data, which consists of measurable quantities, counts, or numerical values. Quantitative data analysis software provides a range of features and functionalities to manage, analyze, visualize, and interpret numerical data effectively.
Key features commonly found in quantitative data analysis software include:
- Data Import and Management: Capability to import data from various sources such as spreadsheets, databases, text files, or online repositories.
- Descriptive Statistics: Tools for computing basic descriptive statistics such as measures of central tendency (e.g., mean, median, mode) and measures of dispersion (e.g., standard deviation, variance).
- Data Visualization: Functionality to create visual representations of data through charts, graphs, histograms, scatter plots, or heatmaps.
- Statistical Analysis: Support for conducting a wide range of statistical tests and analyses to explore relationships, test hypotheses, make predictions, or infer population characteristics from sample data.
- Advanced Analytics: Advanced analytical techniques for more complex data exploration and modeling, such as cluster analysis, principal component analysis (PCA), time series analysis, survival analysis, and structural equation modeling (SEM).
- Automation and Reproducibility: Features for automating analysis workflows, scripting repetitive tasks, and ensuring the reproducibility of results.
- Reporting and Collaboration: Tools for generating customizable reports, summaries, or presentations to communicate analysis results effectively to stakeholders.
Benefits of Quantitative Data Analysis
Quantitative data analysis offers numerous benefits across various fields and disciplines. Here are some of the key advantages:
Making Confident Decisions
Quantitative data analysis provides solid, evidence-based insights that support decision-making. By relying on data rather than intuition, you can reduce the risk of making incorrect decisions. This not only increases confidence in your choices but also fosters buy-in from stakeholders and team members.
Cost Reduction
Analyzing quantitative data helps identify areas where costs can be reduced or optimized. For instance, if certain marketing campaigns yield lower-than-average results, reallocating resources to more effective channels can lead to cost savings and improved ROI.
Personalizing User Experience
Quantitative analysis allows for the mapping of customer journeys and the identification of preferences and behaviors. By understanding these patterns, businesses can tailor their offerings, content, and communication to specific user segments, leading to enhanced user satisfaction and engagement.
Improving User Satisfaction and Delight
Quantitative data analysis highlights areas of success and areas for improvement in products or services. For instance, if a webpage shows high engagement but low conversion rates, further investigation can uncover user pain points or friction in the conversion process. Addressing these issues can lead to improved user satisfaction and increased conversion rates.
Best 10 Quantitative Data Analysis Software
1. questionpro.
Known for its robust survey and research capabilities, QuestionPro is a versatile platform that offers powerful data analysis tools tailored for market research, customer feedback, and academic studies. With features like advanced survey logic, data segmentation, and customizable reports, QuestionPro empowers users to derive actionable insights from their quantitative data.
Features of QuestionPro
- Customizable Surveys
- Advanced Question Types:
- Survey Logic and Branching
- Data Segmentation
- Real-Time Reporting
- Mobile Optimization
- Integration Options
- Multi-Language Support
- Data Export
- User-friendly interface.
- Extensive question types.
- Seamless data export capabilities.
- Limited free version.
Pricing :
Starts at $99 per month per user.
2. SPSS (Statistical Package for the Social Sciences
SPSS is a venerable software package widely used in the social sciences for statistical analysis. Its intuitive interface and comprehensive range of statistical techniques make it a favorite among researchers and analysts for hypothesis testing, regression analysis, and data visualization tasks.
- Advanced statistical analysis capabilities.
- Data management and manipulation tools.
- Customizable graphs and charts.
- Syntax-based programming for automation.
- Extensive statistical procedures.
- Flexible data handling.
- Integration with other statistical software package
- High cost for the full version.
- Steep learning curve for beginners.
Pricing:
- Starts at $99 per month.
3. Google Analytics
Primarily used for web analytics, Google Analytics provides invaluable insights into website traffic, user behavior, and conversion metrics. By tracking key performance indicators such as page views, bounce rates, and traffic sources, Google Analytics helps businesses optimize their online presence and maximize their digital marketing efforts.
- Real-time tracking of website visitors.
- Conversion tracking and goal setting.
- Customizable reports and dashboards.
- Integration with Google Ads and other Google products.
- Free version available.
- Easy to set up and use.
- Comprehensive insights into website performance.
- Limited customization options in the free version.
- Free for basic features.
Hotjar is a powerful tool for understanding user behavior on websites and digital platforms. Hotjar enables businesses to visualize how users interact with their websites, identify pain points, and optimize the user experience for better conversion rates and customer satisfaction through features like heatmaps, session recordings, and on-site surveys.
- Heatmaps to visualize user clicks, taps, and scrolling behavior.
- Session recordings for in-depth user interaction analysis.
- Feedback polls and surveys.
- Funnel and form analysis.
- Easy to install and set up.
- Comprehensive insights into user behavior.
- Affordable pricing plans.
- Limited customization options for surveys.
Starts at $39 per month.
While not a dedicated data analysis software, Python is a versatile programming language widely used for data analysis, machine learning, and scientific computing. With libraries such as NumPy, pandas, and matplotlib, Python provides a comprehensive ecosystem for data manipulation, visualization, and statistical analysis, making it a favorite among data scientists and analysts.
- The rich ecosystem of data analysis libraries.
- Flexible and scalable for large datasets.
- Integration with other tools and platforms.
- Open-source with a supportive community.
- Free and open-source.
- High performance and scalability.
- Great for automation and customization.
- Requires programming knowledge.
- It is Free for the beginners.
6. SAS (Statistical Analysis System)
SAS is a comprehensive software suite renowned for its advanced analytics, business intelligence, and data management capabilities. With a wide range of statistical techniques, predictive modeling tools, and data visualization options, SAS is trusted by organizations across industries for complex data analysis tasks and decision support.
- Wide range of statistical procedures.
- Data integration and cleansing tools.
- Advanced analytics and machine learning capabilities.
- Scalable for enterprise-level data analysis.
- Powerful statistical modeling capabilities.
- Excellent support for large datasets.
- Trusted by industries for decades.
- Expensive licensing fees.
- Steep learning curve.
- Contact sales for pricing details.
Despite its simplicity compared to specialized data analysis software, Excel remains popular for basic quantitative analysis and data visualization. With features like pivot tables, functions, and charting tools, Excel provides a familiar and accessible platform for users to perform tasks such as data cleaning, summarization, and exploratory analysis.
- Formulas and functions for calculations.
- Pivot tables and charts for data visualization.
- Data sorting and filtering capabilities.
- Integration with other Microsoft Office applications.
- Widely available and familiar interface.
- Affordable for basic analysis tasks.
- Versatile for various data formats.
- Limited statistical functions compared to specialized software.
- Not suitable for handling large datasets.
- Included in Microsoft 365 subscription plans, starts at $6.99 per month.
8. IBM SPSS Statistics
Building on the foundation of SPSS, IBM SPSS Statistics offers enhanced features and capabilities for advanced statistical analysis and predictive modeling. With modules for data preparation, regression analysis, and survival analysis, IBM SPSS Statistics is well-suited for researchers and analysts tackling complex data analysis challenges.
- Advanced statistical procedures.
- Data preparation and transformation tools.
- Automated model building and deployment.
- Integration with other IBM products.
- Extensive statistical capabilities.
- User-friendly interface for beginners.
- Enterprise-grade security and scalability.
- Limited support for open-source integration.
Minitab is a specialized software package designed for quality improvement and statistical analysis in manufacturing, engineering, and healthcare industries. With tools for experiment design, statistical process control, and reliability analysis, Minitab empowers users to optimize processes, reduce defects, and improve product quality.
- Basic and advanced statistical analysis.
- Graphical analysis tools for data visualization.
- Statistical methods improvement.
- DOE (Design of Experiments) capabilities.
- Streamlined interface for statistical analysis.
- Comprehensive quality improvement tools.
- Excellent customer support.
- Limited flexibility for customization.
Pricing:
- Starts at $29 per month.
JMP is a dynamic data visualization and statistical analysis tool developed by SAS Institute. Known for its interactive graphics and exploratory data analysis capabilities, JMP enables users to uncover patterns, trends, and relationships in their data, facilitating deeper insights and informed decision-making.
- Interactive data visualization.
- Statistical modeling and analysis.
- Predictive analytics and machine learning.
- Integration with SAS and other data sources.
- Intuitive interface for exploratory data analysis.
- Dynamic graphics for better insights.
- Integration with SAS for advanced analytics.
- Limited scripting capabilities.
- Less customizable compared to other SAS products.
QuestionPro is Your Right Quantitative Data Analysis Software?
QuestionPro offers a range of features specifically designed for quantitative data analysis, making it a suitable choice for various research, survey, and data-driven decision-making needs. Here’s why it might be the right fit for you:
Comprehensive Survey Capabilities
QuestionPro provides extensive tools for creating surveys with quantitative questions, allowing you to gather structured data from respondents. Whether you need Likert scale questions, multiple-choice questions, or numerical input fields, QuestionPro offers the flexibility to design surveys tailored to your research objectives.
Real-Time Data Analysis
With QuestionPro’s real-time data collection and analysis features, you can access and analyze survey responses as soon as they are submitted. This enables you to quickly identify trends, patterns, and insights without delay, facilitating agile decision-making based on up-to-date information.
Advanced Statistical Analysis
QuestionPro includes advanced statistical analysis tools that allow you to perform in-depth quantitative analysis of survey data. Whether you need to calculate means, medians, standard deviations, correlations, or conduct regression analysis, QuestionPro offers the functionality to derive meaningful insights from your data.
Data Visualization
Visualizing quantitative data is crucial for understanding trends and communicating findings effectively. QuestionPro offers a variety of visualization options, including charts, graphs, and dashboards, to help you visually represent your survey data and make it easier to interpret and share with stakeholders.
Segmentation and Filtering
QuestionPro enables you to segment and filter survey data based on various criteria, such as demographics, responses to specific questions, or custom variables. This segmentation capability allows you to analyze different subgroups within your dataset separately, gaining deeper insights into specific audience segments or patterns.
Cost-Effective Solutions
QuestionPro offers pricing plans tailored to different user needs and budgets, including options for individuals, businesses, and enterprise-level organizations. Whether conducting a one-time survey or needing ongoing access to advanced features, QuestionPro provides cost-effective solutions to meet your requirements.
Choosing the right quantitative data analysis software depends on your specific needs, budget, and level of expertise. Whether you’re a researcher, marketer, or business analyst, these top 10 software options offer diverse features and capabilities to help you unlock valuable insights from your data.
If you’re looking for a comprehensive, user-friendly, and cost-effective solution for quantitative data analysis, QuestionPro could be the right choice for your research, survey, or data-driven decision-making needs. With its powerful features, intuitive interface, and flexible pricing options, QuestionPro empowers users to derive valuable insights from their survey data efficiently and effectively.
So go ahead, explore QuestionPro, and empower yourself to unlock valuable insights from your data!
LEARN MORE FREE TRIAL
MORE LIKE THIS
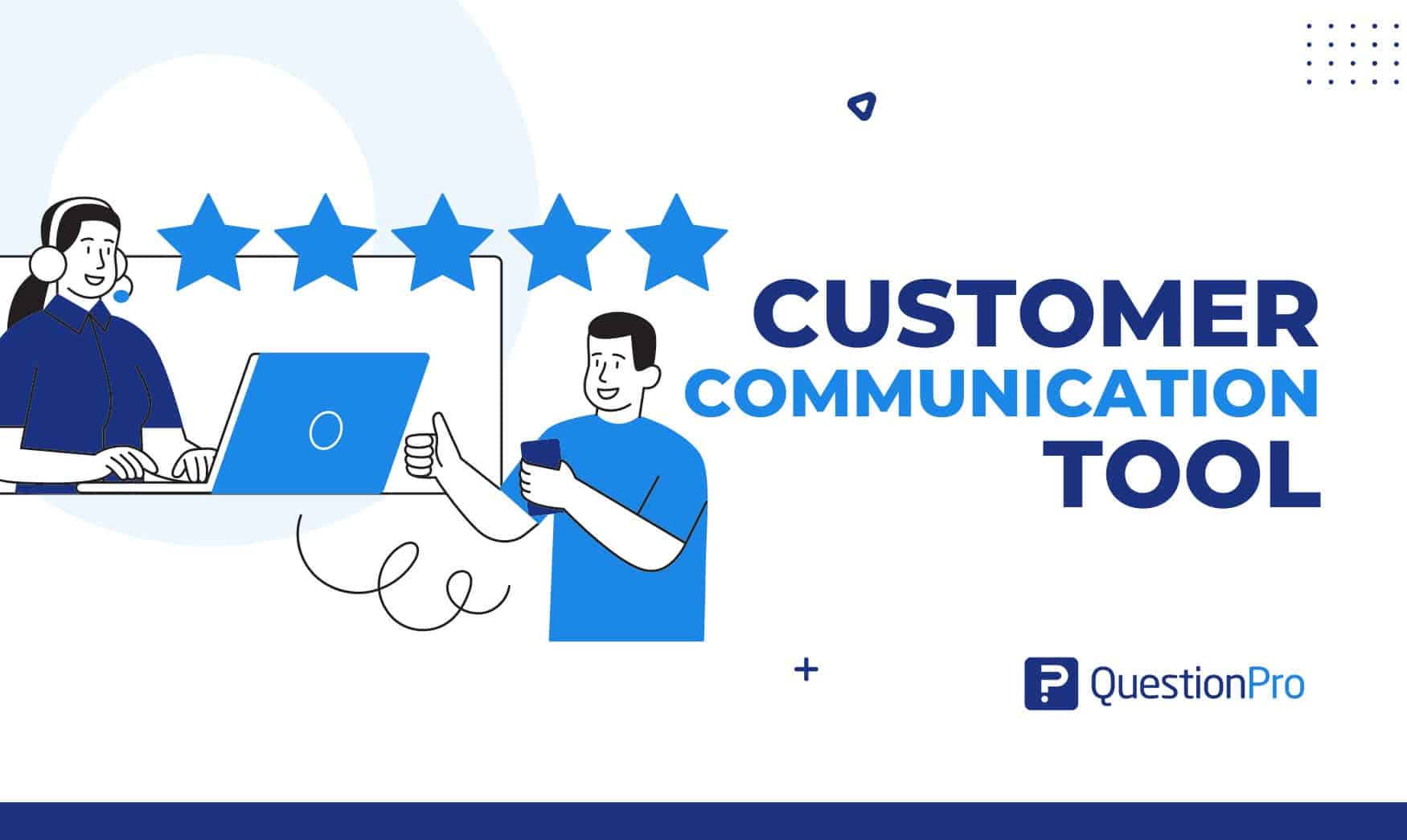
Customer Communication Tool: Types, Methods, Uses, & Tools
Apr 23, 2024
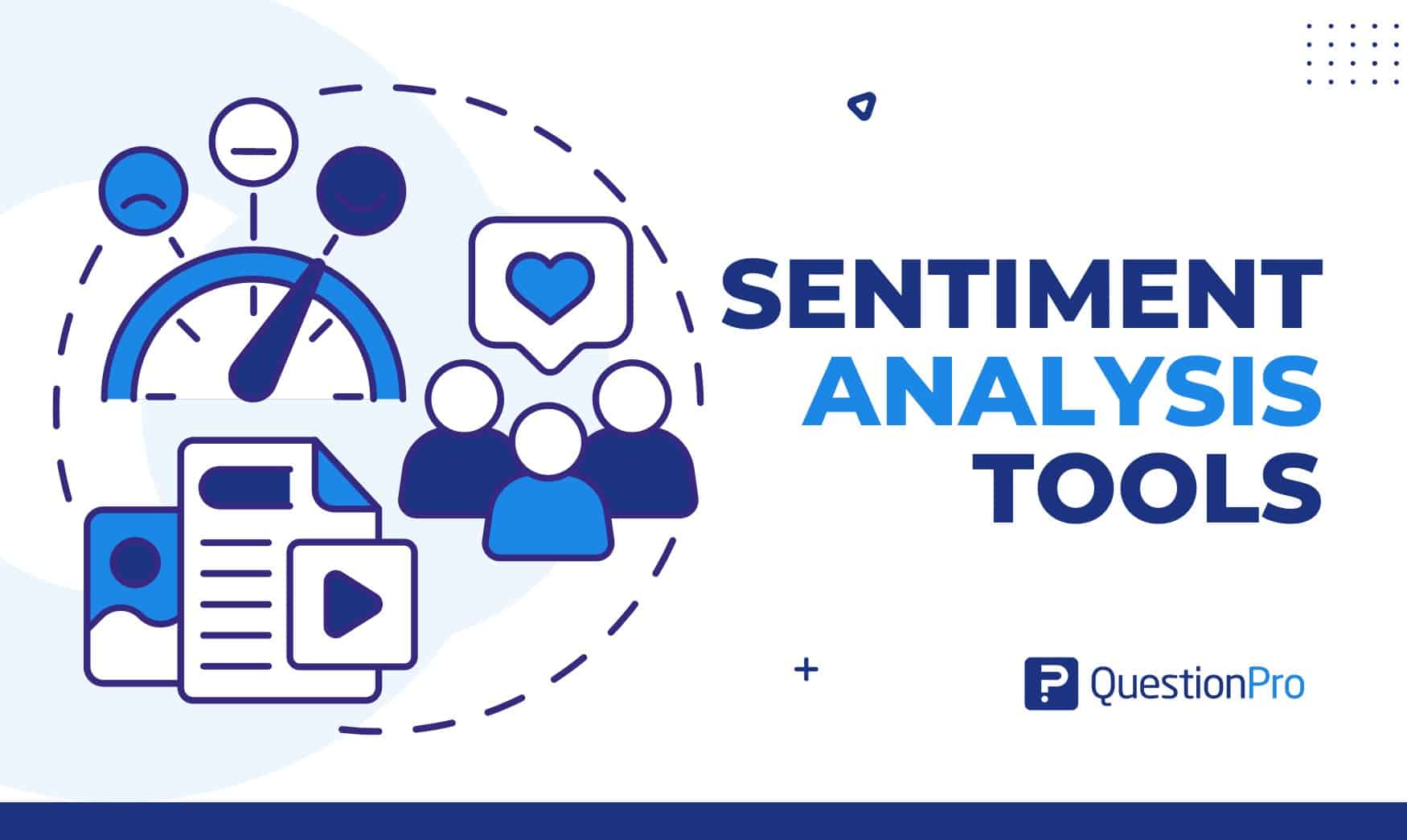
Top 12 Sentiment Analysis Tools for Understanding Emotions
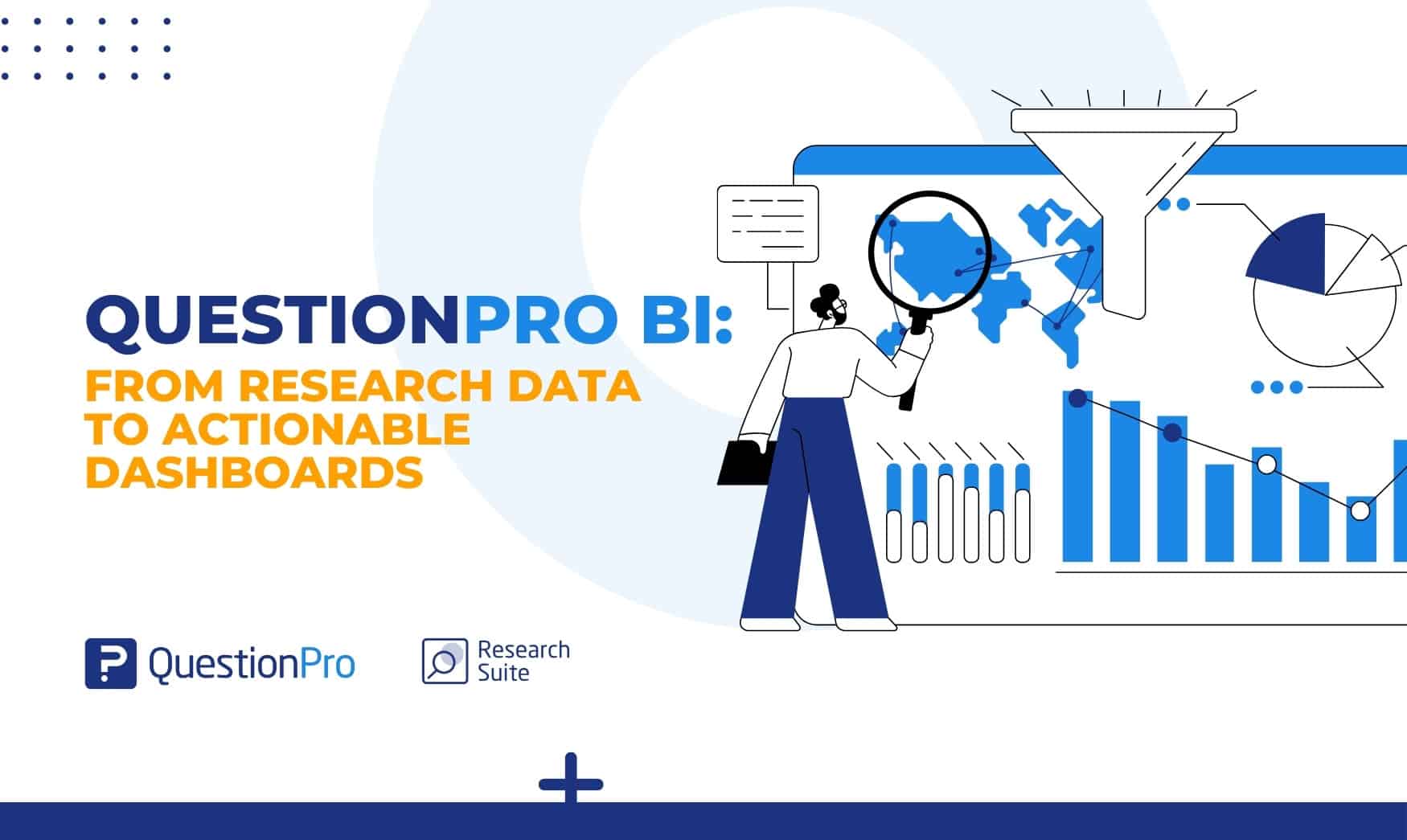
QuestionPro BI: From Research Data to Actionable Dashboards
Apr 22, 2024
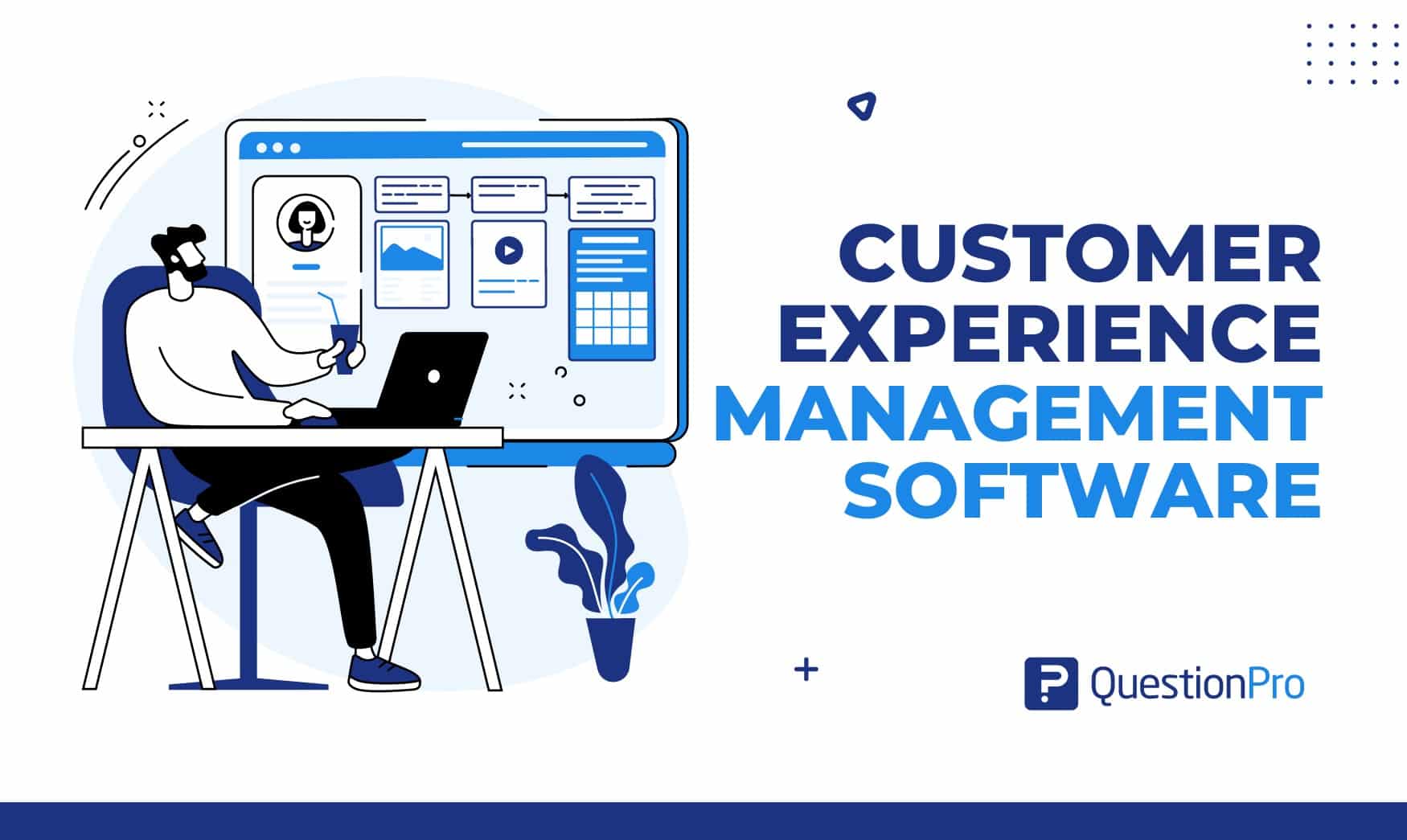
21 Best Customer Experience Management Software in 2024
Other categories.
- Academic Research
- Artificial Intelligence
- Assessments
- Brand Awareness
- Case Studies
- Communities
- Consumer Insights
- Customer effort score
- Customer Engagement
- Customer Experience
- Customer Loyalty
- Customer Research
- Customer Satisfaction
- Employee Benefits
- Employee Engagement
- Employee Retention
- Friday Five
- General Data Protection Regulation
- Insights Hub
- Life@QuestionPro
- Market Research
- Mobile diaries
- Mobile Surveys
- New Features
- Online Communities
- Question Types
- Questionnaire
- QuestionPro Products
- Release Notes
- Research Tools and Apps
- Revenue at Risk
- Survey Templates
- Training Tips
- Uncategorized
- Video Learning Series
- What’s Coming Up
- Workforce Intelligence
A Review of Software Tools for Quantitative Data Analysis
How to get started with statistical analysis
- Research, Samples, and Statistics
- Key Concepts
- Major Sociologists
- News & Issues
- Recommended Reading
- Archaeology
If you're a sociology student or budding social scientist and have started to work with quantitative (statistical) data, analytic software will be very useful.
These programs force researchers to organize and clean their data and offer pre-programmed commands that allow everything from very basic to quite advanced forms of statistical analysis .
They even offer useful visualizations that will be useful as you seek to interpret data, and that you may wish to use when presenting it to others.
There are many programs on the market that are quite expensive. The good news for students and faculty is that most universities have licenses for at least one program students and professors can use.
Also, most programs offer a free, pared-down version of the full software package which will often suffice.
Here's a review of the three main programs that quantitative social scientists use.
Statistical Package for Social Science (SPSS)
SPSS is the most popular quantitative analysis software program used by social scientists.
Made and sold by IBM, it is comprehensive, flexible, and can be used with almost any type of data file. However, it is especially useful for analyzing large-scale survey data .
It can be used to generate tabulated reports, charts, and plots of distributions and trends, as well as generate descriptive statistics such as means, medians, modes and frequencies in addition to more complex statistical analyses like regression models.
SPSS provides a user interface that makes it easy and intuitive for all levels of users. With menus and dialogue boxes, you can perform analyses without having to write command syntax, like in other programs.
It is also simple and easy to enter and edit data directly into the program.
There are a few drawbacks, however, which might not make it the best program for some researchers. For example, there is a limit on the number of cases you can analyze. It is also difficult to account for weights, strata and group effects with SPSS.
STATA is an interactive data analysis program that runs on a variety of platforms. It can be used for both simple and complex statistical analyses.
STATA uses a point-and-click interface as well as command syntax, which makes it easy to use. STATA also makes it simple to generate graphs and plots of data and results.
Analysis in STATA is centered around four windows:
- command window
- review window
- result window
- variable window
Analysis commands are entered into the command window and the review window records those commands. The variables window lists the variables that are available in the current data set along with the variable labels, and the results appear in the results window.
SAS, short for Statistical Analysis System, is also used by many businesses.
In addition to statistical analysis, it also allows programmers to perform report writing, graphics, business planning, forecasting, quality improvement, project management and more.
SAS is a great program for the intermediate and advanced user because it is very powerful; it can be used with extremely large datasets and can perform complex and advanced analyses.
SAS is good for analyses that require you to take into account weights, strata, or groups.
Unlike SPSS and STATA, SAS is run largely by programming syntax rather than point-and-click menus, so some knowledge of the programming language is required.
Other Programs
Other programs popular with sociologists include:
- R: Free to download and use. You can add your own programs to it if you are familiar with statistics and programming.
- NVio: "It helps researchers organize and analyze complex non-numerical or unstructured data, both text and multimedia," according to UCLA Library .
- MATLAB: Provides "Simulations, Multidimensional Data, Image and Signal Processing," according to NYU Libraries .
- Understanding Path Analysis
- The 7 Best Programming Languages to Learn for Beginners
- Data Cleaning for Data Analysis in Sociology
- What Is Quantitative Data?
- Pros and Cons of Secondary Data Analysis
- Cluster Analysis and How Its Used in Research
- Understanding Secondary Data and How to Use It in Research
- Qualitative Data Definition and Examples
- An Overview of Qualitative Research Methods
- 7 Graphs Commonly Used in Statistics
- Correlation Analysis in Research
- Linear Regression Analysis
- Glossary of Visual Basic Terms
- Your Comprehensive Guide to a Painless Undergrad Econometrics Project
- Benefits of the Graphical User Interface
- The Study Island Program: An In-Depth Review
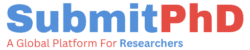
11 Best Data Analysis Software for Research [2023]
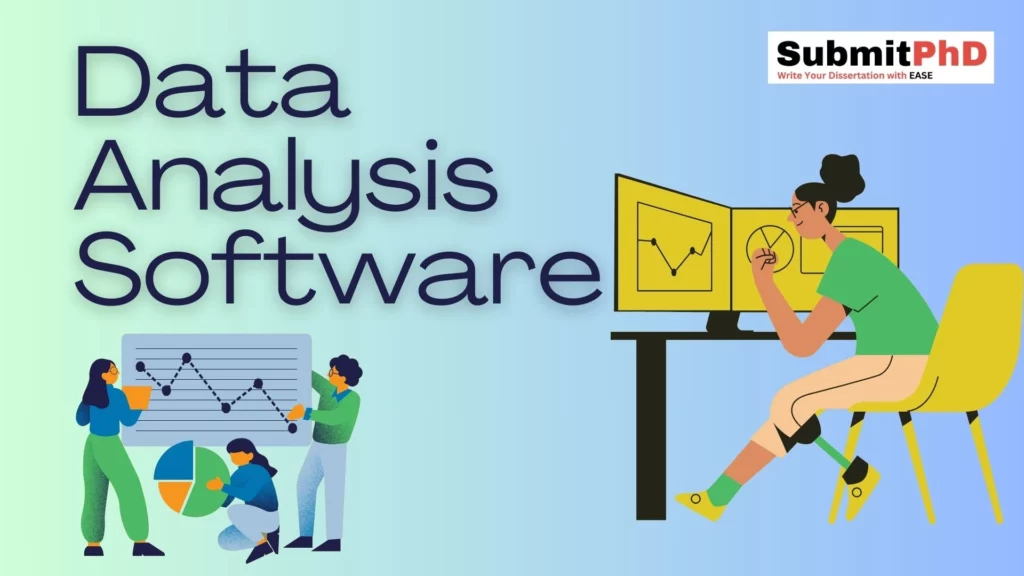
5 Best Reference Management Software for Research [FREE]
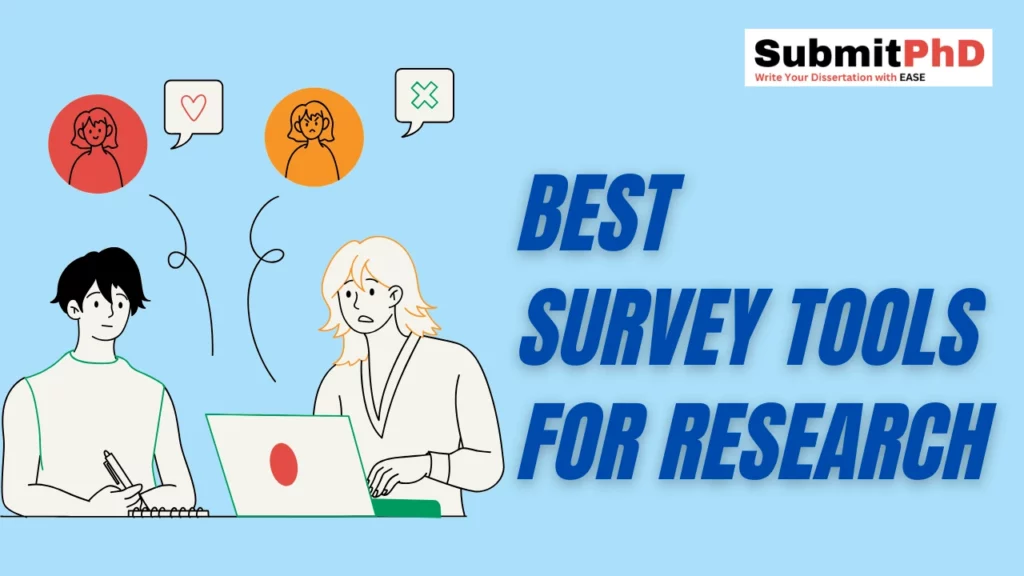
7 Best Survey Tools for Research [2023]
Leave a comment cancel reply.
Your email address will not be published. Required fields are marked *
Save my name, email, and website in this browser for the next time I comment.
Quantitative Analysis Guide: Which Statistical Software to Use?
- Finding Data
- Which Statistical Software to Use?
- Merging Data Sets
- Reshaping Data Sets
- Choose Statistical Test for 1 Dependent Variable
- Choose Statistical Test for 2 or More Dependent Variables

- Data Services Home Page
Statistical Software Comparison
- What statistical test to use?
- Data Visualization Resources
- Data Analysis Examples External (UCLA) examples of regression and power analysis
- Supported software
- Request a consultation
- Making your code reproducible
Software Access
- The first version of SPSS was developed by Norman H. Nie, Dale H. Bent and C. Hadlai Hull in and released in 1968 as the Statistical Package for Social Sciences.
- In July 2009, IBM acquired SPSS.
- Social sciences
- Health sciences
Data Format and Compatibility
- .sav file to save data
- Optional syntax files (.sps)
- Easily export .sav file from Qualtrics
- Import Excel files (.xls, .xlsx), Text files (.csv, .txt, .dat), SAS (.sas7bdat), Stata (.dta)
- Export Excel files (.xls, .xlsx), Text files (.csv, .dat), SAS (.sas7bdat), Stata (.dta)
- SPSS Chart Types
- Chart Builder: Drag and drop graphics
- Easy and intuitive user interface; menus and dialog boxes
- Similar feel to Excel
- SEMs through SPSS Amos
- Easily exclude data and handle missing data
Limitations
- Absence of robust methods (e.g...Least Absolute Deviation Regression, Quantile Regression, ...)
- Unable to perform complex many to many merge
Sample Data
- Developed by SAS
- Created in the 1980s by John Sall to take advantage of the graphical user interface introduced by Macintosh
- Orginally stood for 'John's Macintosh Program'
- Five products: JMP, JMP Pro, JMP Clinical, JMP Genomics, JMP Graph Builder App
- Engineering: Six Sigma, Quality Control, Scientific Research, Design of Experiments
- Healthcare/Pharmaceutical
- .jmp file to save data
- Optional syntax files (.jsl)
- Import Excel files (.xls, .xlsx), Text files (.csv, .txt, .dat), SAS (.sas7bdat), Stata (.dta), SPSS (.sav)
- Export Excel files (.xls, .xlsx), Text files (.csv, .dat), SAS (.sas7bdat)
- Gallery of JMP Graphs
- Drag and Drop Graph Editor will try to guess what chart is correct for your data
- Dynamic interface can be used to zoom and change view
- Ability to lasso outliers on a graph and regraph without the outliers
- Interactive Graphics
- Scripting Language (JSL)
- SAS, R and MATLAB can be executed using JSL
- Interface for using R from within and add-in for Excel
- Great interface for easily managing output
- Graphs and data tables are dynamically linked
- Great set of online resources!
- Absence of some robust methods (regression: 2SLS, LAD, Quantile)
- Stata was first released in January 1985 as a regression and data management package with 44 commands, written by Bill Gould and Sean Becketti.
- The name Stata is a syllabic abbreviation of the words statistics and data.
- The graphical user interface (menus and dialog boxes) was released in 2003.
- Political Science
- Public Health
- Data Science
- Who uses Stata?
Data Format and Compatibility
- .dta file to save dataset
- .do syntax file, where commands can be written and saved
- Import Excel files (.xls, .xlsx), Text files (.txt, .csv, .dat), SAS (.XPT), Other (.XML), and various ODBC data sources
- Export Excel files (.xls, . xlsx ), Text files (.txt, .csv, .dat), SAS (.XPT), Other (.XML), and various ODBC data sources
- Newer versions of Stata can read datasets, commands, graphs, etc., from older versions, and in doing so, reproduce results
- Older versions of Stata cannot read newer versions of Stata datasets, but newer versions can save in the format of older versions
- Stata Graph Gallery
- UCLA - Stata Graph Gallery
- Syntax mainly used, but menus are an option as well
- Some user written programs are available to install
- Offers matrix programming in Mata
- Works well with panel, survey, and time-series data
- Data management
- Can only hold one dataset in memory at a time
- The specific Stata package ( Stata/IC, Stata/SE, and Stata/MP ) limits the size of usable datasets. One may have to sacrifice the number of variables for the number of observations, or vice versa, depending on the package.
- Overall, graphs have limited flexibility. Stata schemes , however, provide some flexibility in changing the style of the graphs.
- Sample Syntax
* First enter the data manually; input str10 sex test1 test2 "Male" 86 83 "Male" 93 79 "Male" 85 81 "Male" 83 80 "Male" 91 76 "Female" 94 79 "Fem ale" 91 94 "Fem ale" 83 84 "Fem ale" 96 81 "Fem ale" 95 75 end
* Next run a paired t-test; ttest test1 == test2
* Create a scatterplot; twoway ( scatter test2 test1 if sex == "Male" ) ( scatter test2 test1 if sex == "Fem ale" ), legend (lab(1 "Male" ) lab(2 "Fem ale" ))
- The development of SAS (Statistical Analysis System) began in 1966 by Anthony Bar of North Carolina State University and later joined by James Goodnight.
- The National Institute of Health funded this project with a goal of analyzing agricultural data to improve crop yields.
- The first release of SAS was in 1972. In 2012, SAS held 36.2% of the market making it the largest market-share holder in 'advanced analytics.'
- Financial Services
- Manufacturing
- Health and Life Sciences
- Available for Windows only
- Import Excel files (.xls, .xlsx), Text files (.txt, .dat, .csv), SPSS (.sav), Stata (.dta), JMP (.jmp), Other (.xml)
- Export Excel files (.xls, . xlsx ), Text files (.txt, .dat, .csv), SPSS (.sav), Stata (.dta), JMP (.jmp), Other (.xml)
- SAS Graphics Samples Output Gallery
- Can be cumbersome at times to create perfect graphics with syntax
- ODS Graphics Designer provides a more interactive interface
- BASE SAS contains the data management facility, programming language, data analysis and reporting tools
- SAS Libraries collect the SAS datasets you create
- Multitude of additional components are available to complement Base SAS which include SAS/GRAPH, SAS/PH (Clinical Trial Analysis), SAS/ETS (Econometrics and Time Series), SAS/Insight (Data Mining) etc...
- SAS Certification exams
- Handles extremely large datasets
- Predominantly used for data management and statistical procedures
- SAS has two main types of code; DATA steps and PROC steps
- With one procedure, test results, post estimation and plots can be produced
- Size of datasets analyzed is only limited by the machine
Limitations
- Graphics can be cumbersome to manipulate
- Since SAS is a proprietary software, there may be an extensive lag time for the implementation of new methods
- Documentation and books tend to be very technical and not necessarily new user friendly
* First enter the data manually; data example; input sex $ test1 test2; datalines ; M 86 83 M 93 79 M 85 81 M 83 80 M 91 76 F 94 79 F 91 94 F 83 84 F 96 81 F 95 75 ; run ;
* Next run a paired t-test; proc ttest data = example; paired test1*test2; run ;
* Create a scatterplot; proc sgplot data = example; scatter y = test1 x = test2 / group = sex; run ;
- R first appeared in 1993 and was created by Ross Ihaka and Robert Gentleman at the University of Auckland, New Zealand.
- R is an implementation of the S programming language which was developed at Bell Labs.
- It is named partly after its first authors and partly as a play on the name of S.
- R is currently developed by the R Development Core Team.
- RStudio, an integrated development environment (IDE) was first released in 2011.
- Companies Using R
- Finance and Economics
- Bioinformatics
- Import Excel files (.xls, .xlsx), Text files (.txt, .dat, .csv), SPSS (.sav), Stata (.dta), SAS(.sas7bdat), Other (.xml, .json)
- Export Excel files (.xlsx), Text files (.txt, .csv), SPSS (.sav), Stata (.dta), Other (.json)
- ggplot2 package, grammar of graphics
- Graphs available through ggplot2
- The R Graph Gallery
- Network analysis (igraph)
- Flexible esthetics and options
- Interactive graphics with Shiny
- Many available packages to create field specific graphics
- R is a free and open source
- Over 6000 user contributed packages available through CRAN
- Large online community
- Network Analysis, Text Analysis, Data Mining, Web Scraping
- Interacts with other software such as, Python, Bioconductor, WinBUGS, JAGS etc...
- Scope of functions, flexible, versatile etc..
Limitations
- Large online help community but no 'formal' tech support
- Have to have a good understanding of different data types before real ease of use begins
- Many user written packages may be hard to sift through
# Manually enter the data into a dataframe dataset <- data.frame(sex = c("Male", "Male", "Male", "Male", "Male", "Female", "Female", "Female", "Female", "Female"), test1 = c( 86 , 93 , 85 , 83 , 91 , 94 , 91 , 83 , 96 , 95 ), test2 = c( 83 , 79 , 81 , 80 , 76 , 79 , 94 , 84 , 81 , 75 ))
# Now we will run a paired t-test t.test(dataset$test1, dataset$test2, paired = TRUE )
# Last let's simply plot these two test variables plot(dataset$test1, dataset$test2, col = c("red","blue")[dataset$sex]) legend("topright", fill = c("blue", "red"), c("Male", "Female"))
# Making the same graph using ggplot2 install.packages('ggplot2') library(ggplot2) mygraph <- ggplot(data = dataset, aes(x = test1, y = test2, color = sex)) mygraph + geom_point(size = 5) + ggtitle('Test1 versus Test2 Scores')
- Cleave Moler of the University of New Mexico began development in the late 1970s.
- With the help of Jack Little, they cofounded MathWorks and released MATLAB (matrix laboratory) in 1984.
- Education (linear algebra and numerical analysis)
- Popular among scientists involved in image processing
- Engineering
- .m Syntax file
- Import Excel files (.xls, .xlsx), Text files (.txt, .dat, .csv), Other (.xml, .json)
- Export Excel files (.xls, .xlsx), Text files (.txt, .dat, .csv), Other (.xml, .json)
- MATLAB Plot Gallery
- Customizable but not point-and-click visualization
- Optimized for data analysis, matrix manipulation in particular
- Basic unit is a matrix
- Vectorized operations are quick
- Diverse set of available toolboxes (apps) [Statistics, Optimization, Image Processing, Signal Processing, Parallel Computing etc..]
- Large online community (MATLAB Exchange)
- Image processing
- Vast number of pre-defined functions and implemented algorithms
- Lacks implementation of some advanced statistical methods
- Integrates easily with some languages such as C, but not others, such as Python
- Limited GIS capabilities
sex = { 'Male' , 'Male' , 'Male' , 'Male' , 'Male' , 'Female' , 'Female' , 'Female' , 'Female' , 'Female' }; t1 = [86,93,85,83,91,94,91,83,96,95]; t2 = [83,79,81,80,76,79,94,84,81,75];
% paired t-test [h,p,ci,stats] = ttest(t1,t2)
% independent samples t-test sex = categorical(sex); [h,p,ci,stats] = ttest2(t1(sex== 'Male' ),t1(sex== 'Female' ))
plot(t1,t2, 'o' ) g = sex== 'Male' ; plot(t1(g),t2(g), 'bx' ); hold on; plot(t1(~g),t2(~g), 'ro' )
Software Features and Capabilities
*The primary interface is bolded in the case of multiple interface types available.
Learning Curve

Further Reading
- The Popularity of Data Analysis Software
- Statistical Software Capability Table
- The SAS versus R Debate in Industry and Academia
- Why R has a Steep Learning Curve
- Comparison of Data Analysis Packages
- Comparison of Statistical Packages
- MATLAB commands in Python and R
- MATLAB and R Side by Side
- Stata and R Side by Side

- << Previous: Statistical Guidance
- Next: Merging Data Sets >>
- Last Updated: Apr 18, 2024 4:36 AM
- URL: https://guides.nyu.edu/quant
Quantitative Data Analysis: A Comprehensive Guide
By: Ofem Eteng Published: May 18, 2022
Related Articles
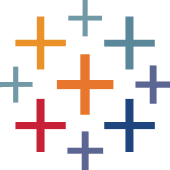
A healthcare giant successfully introduces the most effective drug dosage through rigorous statistical modeling, saving countless lives. A marketing team predicts consumer trends with uncanny accuracy, tailoring campaigns for maximum impact.
Table of Contents
These trends and dosages are not just any numbers but are a result of meticulous quantitative data analysis. Quantitative data analysis offers a robust framework for understanding complex phenomena, evaluating hypotheses, and predicting future outcomes.
In this blog, we’ll walk through the concept of quantitative data analysis, the steps required, its advantages, and the methods and techniques that are used in this analysis. Read on!
What is Quantitative Data Analysis?
Quantitative data analysis is a systematic process of examining, interpreting, and drawing meaningful conclusions from numerical data. It involves the application of statistical methods, mathematical models, and computational techniques to understand patterns, relationships, and trends within datasets.
Quantitative data analysis methods typically work with algorithms, mathematical analysis tools, and software to gain insights from the data, answering questions such as how many, how often, and how much. Data for quantitative data analysis is usually collected from close-ended surveys, questionnaires, polls, etc. The data can also be obtained from sales figures, email click-through rates, number of website visitors, and percentage revenue increase.
Quantitative Data Analysis vs Qualitative Data Analysis
When we talk about data, we directly think about the pattern, the relationship, and the connection between the datasets – analyzing the data in short. Therefore when it comes to data analysis, there are broadly two types – Quantitative Data Analysis and Qualitative Data Analysis.
Quantitative data analysis revolves around numerical data and statistics, which are suitable for functions that can be counted or measured. In contrast, qualitative data analysis includes description and subjective information – for things that can be observed but not measured.
Let us differentiate between Quantitative Data Analysis and Quantitative Data Analysis for a better understanding.
Data Preparation Steps for Quantitative Data Analysis
Quantitative data has to be gathered and cleaned before proceeding to the stage of analyzing it. Below are the steps to prepare a data before quantitative research analysis:
- Step 1: Data Collection
Before beginning the analysis process, you need data. Data can be collected through rigorous quantitative research, which includes methods such as interviews, focus groups, surveys, and questionnaires.
- Step 2: Data Cleaning
Once the data is collected, begin the data cleaning process by scanning through the entire data for duplicates, errors, and omissions. Keep a close eye for outliers (data points that are significantly different from the majority of the dataset) because they can skew your analysis results if they are not removed.
This data-cleaning process ensures data accuracy, consistency and relevancy before analysis.
- Step 3: Data Analysis and Interpretation
Now that you have collected and cleaned your data, it is now time to carry out the quantitative analysis. There are two methods of quantitative data analysis, which we will discuss in the next section.
However, if you have data from multiple sources, collecting and cleaning it can be a cumbersome task. This is where Hevo Data steps in. With Hevo, extracting, transforming, and loading data from source to destination becomes a seamless task, eliminating the need for manual coding. This not only saves valuable time but also enhances the overall efficiency of data analysis and visualization, empowering users to derive insights quickly and with precision
Hevo is the only real-time ELT No-code Data Pipeline platform that cost-effectively automates data pipelines that are flexible to your needs. With integration with 150+ Data Sources (40+ free sources), we help you not only export data from sources & load data to the destinations but also transform & enrich your data, & make it analysis-ready.
Start for free now!
Now that you are familiar with what quantitative data analysis is and how to prepare your data for analysis, the focus will shift to the purpose of this article, which is to describe the methods and techniques of quantitative data analysis.
Methods and Techniques of Quantitative Data Analysis
Quantitative data analysis employs two techniques to extract meaningful insights from datasets, broadly. The first method is descriptive statistics, which summarizes and portrays essential features of a dataset, such as mean, median, and standard deviation.
Inferential statistics, the second method, extrapolates insights and predictions from a sample dataset to make broader inferences about an entire population, such as hypothesis testing and regression analysis.
An in-depth explanation of both the methods is provided below:
- Descriptive Statistics
- Inferential Statistics
1) Descriptive Statistics
Descriptive statistics as the name implies is used to describe a dataset. It helps understand the details of your data by summarizing it and finding patterns from the specific data sample. They provide absolute numbers obtained from a sample but do not necessarily explain the rationale behind the numbers and are mostly used for analyzing single variables. The methods used in descriptive statistics include:
- Mean: This calculates the numerical average of a set of values.
- Median: This is used to get the midpoint of a set of values when the numbers are arranged in numerical order.
- Mode: This is used to find the most commonly occurring value in a dataset.
- Percentage: This is used to express how a value or group of respondents within the data relates to a larger group of respondents.
- Frequency: This indicates the number of times a value is found.
- Range: This shows the highest and lowest values in a dataset.
- Standard Deviation: This is used to indicate how dispersed a range of numbers is, meaning, it shows how close all the numbers are to the mean.
- Skewness: It indicates how symmetrical a range of numbers is, showing if they cluster into a smooth bell curve shape in the middle of the graph or if they skew towards the left or right.
2) Inferential Statistics
In quantitative analysis, the expectation is to turn raw numbers into meaningful insight using numerical values, and descriptive statistics is all about explaining details of a specific dataset using numbers, but it does not explain the motives behind the numbers; hence, a need for further analysis using inferential statistics.
Inferential statistics aim to make predictions or highlight possible outcomes from the analyzed data obtained from descriptive statistics. They are used to generalize results and make predictions between groups, show relationships that exist between multiple variables, and are used for hypothesis testing that predicts changes or differences.
There are various statistical analysis methods used within inferential statistics; a few are discussed below.
- Cross Tabulations: Cross tabulation or crosstab is used to show the relationship that exists between two variables and is often used to compare results by demographic groups. It uses a basic tabular form to draw inferences between different data sets and contains data that is mutually exclusive or has some connection with each other. Crosstabs help understand the nuances of a dataset and factors that may influence a data point.
- Regression Analysis: Regression analysis estimates the relationship between a set of variables. It shows the correlation between a dependent variable (the variable or outcome you want to measure or predict) and any number of independent variables (factors that may impact the dependent variable). Therefore, the purpose of the regression analysis is to estimate how one or more variables might affect a dependent variable to identify trends and patterns to make predictions and forecast possible future trends. There are many types of regression analysis, and the model you choose will be determined by the type of data you have for the dependent variable. The types of regression analysis include linear regression, non-linear regression, binary logistic regression, etc.
- Monte Carlo Simulation: Monte Carlo simulation, also known as the Monte Carlo method, is a computerized technique of generating models of possible outcomes and showing their probability distributions. It considers a range of possible outcomes and then tries to calculate how likely each outcome will occur. Data analysts use it to perform advanced risk analyses to help forecast future events and make decisions accordingly.
- Analysis of Variance (ANOVA): This is used to test the extent to which two or more groups differ from each other. It compares the mean of various groups and allows the analysis of multiple groups.
- Factor Analysis: A large number of variables can be reduced into a smaller number of factors using the factor analysis technique. It works on the principle that multiple separate observable variables correlate with each other because they are all associated with an underlying construct. It helps in reducing large datasets into smaller, more manageable samples.
- Cohort Analysis: Cohort analysis can be defined as a subset of behavioral analytics that operates from data taken from a given dataset. Rather than looking at all users as one unit, cohort analysis breaks down data into related groups for analysis, where these groups or cohorts usually have common characteristics or similarities within a defined period.
- MaxDiff Analysis: This is a quantitative data analysis method that is used to gauge customers’ preferences for purchase and what parameters rank higher than the others in the process.
- Cluster Analysis: Cluster analysis is a technique used to identify structures within a dataset. Cluster analysis aims to be able to sort different data points into groups that are internally similar and externally different; that is, data points within a cluster will look like each other and different from data points in other clusters.
- Time Series Analysis: This is a statistical analytic technique used to identify trends and cycles over time. It is simply the measurement of the same variables at different times, like weekly and monthly email sign-ups, to uncover trends, seasonality, and cyclic patterns. By doing this, the data analyst can forecast how variables of interest may fluctuate in the future.
- SWOT analysis: This is a quantitative data analysis method that assigns numerical values to indicate strengths, weaknesses, opportunities, and threats of an organization, product, or service to show a clearer picture of competition to foster better business strategies
How to Choose the Right Method for your Analysis?
Choosing between Descriptive Statistics or Inferential Statistics can be often confusing. You should consider the following factors before choosing the right method for your quantitative data analysis:
1. Type of Data
The first consideration in data analysis is understanding the type of data you have. Different statistical methods have specific requirements based on these data types, and using the wrong method can render results meaningless. The choice of statistical method should align with the nature and distribution of your data to ensure meaningful and accurate analysis.
2. Your Research Questions
When deciding on statistical methods, it’s crucial to align them with your specific research questions and hypotheses. The nature of your questions will influence whether descriptive statistics alone, which reveal sample attributes, are sufficient or if you need both descriptive and inferential statistics to understand group differences or relationships between variables and make population inferences.
Pros and Cons of Quantitative Data Analysis
1. Objectivity and Generalizability:
- Quantitative data analysis offers objective, numerical measurements, minimizing bias and personal interpretation.
- Results can often be generalized to larger populations, making them applicable to broader contexts.
Example: A study using quantitative data analysis to measure student test scores can objectively compare performance across different schools and demographics, leading to generalizable insights about educational strategies.
2. Precision and Efficiency:
- Statistical methods provide precise numerical results, allowing for accurate comparisons and prediction.
- Large datasets can be analyzed efficiently with the help of computer software, saving time and resources.
Example: A marketing team can use quantitative data analysis to precisely track click-through rates and conversion rates on different ad campaigns, quickly identifying the most effective strategies for maximizing customer engagement.
3. Identification of Patterns and Relationships:
- Statistical techniques reveal hidden patterns and relationships between variables that might not be apparent through observation alone.
- This can lead to new insights and understanding of complex phenomena.
Example: A medical researcher can use quantitative analysis to pinpoint correlations between lifestyle factors and disease risk, aiding in the development of prevention strategies.
1. Limited Scope:
- Quantitative analysis focuses on quantifiable aspects of a phenomenon , potentially overlooking important qualitative nuances, such as emotions, motivations, or cultural contexts.
Example: A survey measuring customer satisfaction with numerical ratings might miss key insights about the underlying reasons for their satisfaction or dissatisfaction, which could be better captured through open-ended feedback.
2. Oversimplification:
- Reducing complex phenomena to numerical data can lead to oversimplification and a loss of richness in understanding.
Example: Analyzing employee productivity solely through quantitative metrics like hours worked or tasks completed might not account for factors like creativity, collaboration, or problem-solving skills, which are crucial for overall performance.
3. Potential for Misinterpretation:
- Statistical results can be misinterpreted if not analyzed carefully and with appropriate expertise.
- The choice of statistical methods and assumptions can significantly influence results.
This blog discusses the steps, methods, and techniques of quantitative data analysis. It also gives insights into the methods of data collection, the type of data one should work with, and the pros and cons of such analysis.
Gain a better understanding of data analysis with these essential reads:
- Data Analysis and Modeling: 4 Critical Differences
- Exploratory Data Analysis Simplified 101
- 25 Best Data Analysis Tools in 2024
Carrying out successful data analysis requires prepping the data and making it analysis-ready. That is where Hevo steps in.
Want to give Hevo a try? Sign Up for a 14-day free trial and experience the feature-rich Hevo suite first hand. You may also have a look at the amazing Hevo price , which will assist you in selecting the best plan for your requirements.
Share your experience of understanding Quantitative Data Analysis in the comment section below! We would love to hear your thoughts.
Ofem is a freelance writer specializing in data-related topics, who has expertise in translating complex concepts. With a focus on data science, analytics, and emerging technologies.
No-code Data Pipeline for your Data Warehouse
- Data Analysis
- Data Warehouse
- Quantitative Data Analysis
Continue Reading
Saloni Agarwal
Enterprise Data Lake: A Simplified Guide
Riya Bothra
An Expert Guide To Enterprise Data Analysis
Gcp storage buckets list: efficient data organizing strategy, i want to read this e-book.
- Our foundation

Best Software for Quantitative Data Analysis: The Definitive Guide
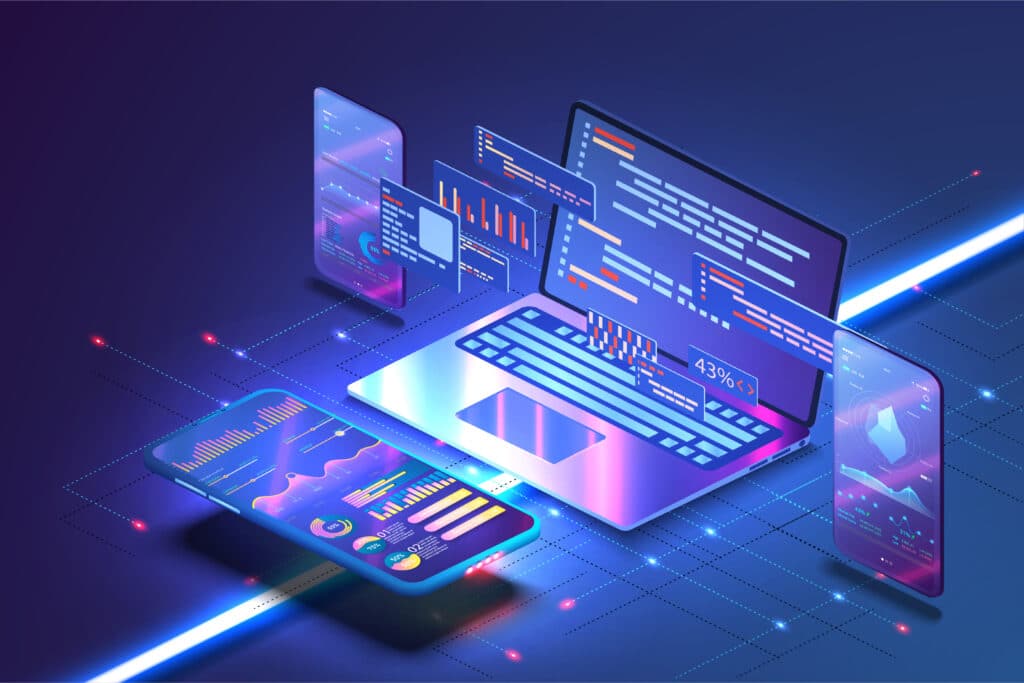
Data can be a scary thing to navigate. Even with the explosion of information that we have at our fingertips, it can still be challenging to make sense of it all. There are multiple ways in which quantitative data can be analysed and interpreted, but there are also many pitfalls to avoid along the way. It’s not just about analyzing raw numbers and figures; data analysis should be strategic, smart, and actionable. In this blog post, we’ll discuss the best software for quantitative data analysis so you can find the most suitable option for your needs. Since there are several options available on the market, we’ve outlined some key considerations before making recommendations based on specific use cases and price points. Read on to learn more!
What Is Quantitative Data Analysis?
Quantitative data analysis is the process of collecting and interpreting numerical or statistical data to make decisions or solve problems. It’s an important component of data science, as it includes the collection, cleaning and organizing of data as well as basic visualizations and analyses. It’s crucial to effectively analyze large amounts of data because it allows you to understand what’s happening in your business and use it to inform your decision-making. The analysis can be anything from a few simple calculations to running complex algorithms on a large dataset. The goal is to gain insights that can help you to make better decisions based on the data you have.
Why is Quantitative Data Analysis Important?
Quantitative data analysis is important for a variety of reasons, but its primary value is that it allows you to make more accurate and informed decisions. When you’re using data to inform your decision-making, you’re being more strategic and less reactive. You’re able to base your decisions on facts rather than gut feelings or emotions. This is critical for all areas of business, but it’s especially useful in the following areas:
– Marketing: This is one of the most popular areas where quantitative analysis is used. Data allows you to make better decisions about your marketing campaigns, such as what kind of ads to run, which audience to target, etc.
– Sales: If you’re analyzing sales data, you can better understand what’s happening with your sales funnel. This allows you to make changes to increase conversion rates and close more sales.
– Product and operations: If you’re analyzing product or operational data, you can make better decisions about what to prioritize and where to focus your efforts.
– Finance: This is one of the oldest uses for quantitative analysis, but it’s still incredibly important. Data analysis allows you to make better decisions about managing your finances, such as what interest rate to offer on a loan.
Best Tools for Basic Data Analysis
Basic data analysis is the most basic form of data analysis. It covers the bare minimum of what data analysis is and does. Basic data analysis is designed to give you a high-level overview of the data and let you know if there’s anything wrong with it. If you’re dealing with small amounts of data, basic data analysis can be done in Excel. However, it’s not recommended to analyze large amounts of data in Excel. If the data is large, you’re better off using a dedicated data analysis tool. There are many great tools for basic data analysis, including these top picks:
– Google Sheets: Google Sheets is an excellent option for basic data analysis. It’s a free tool that allows you to analyze small to medium-sized datasets. It’s ideal for businesses that are still growing, as it allows you to make sense of your data without having to invest in more expensive tools. – Microsoft Excel: While Excel isn’t the best option for large datasets, it’s a great tool for basic data analysis for smaller datasets. It allows you to perform calculations, create visualizations, and more.
Best Tools for Advanced Data Analysis
Advanced data analysis goes beyond basic data analysis and allows you to go deeper into your data. It allows you to discover insights that weren’t visible in your data during the basic analysis process. There are many tools out there that allow you to do advanced data analysis. However, many of these tools are only useful if you have a large amount of data. If you don’t have a large dataset, it may be challenging to do advanced data analysis with these tools. So, if you don’t have a large amount of data, you might want to use one of the tools for basic data analysis. If you have a large amount of data, there are many tools for advanced data analysis that you can use. Some of the best software for quantitative data analysis for advanced data analysis are available here in cmnty with all the available modules.
Quantitative data analysis can be challenging, but it’s also incredibly important. It allows you to make better decisions and understand your data more clearly. It also helps you to identify any issues with your data so they can be corrected. The best quantitative data analysis software depends on a variety of factors, such as the type of data you’re analyzing, the volume of data, the complexity of the data, etc. From basic to advanced data analysis, the best tools are designed to make the process as easy as possible.
We care about your privacy
We and third parties use cookies on our website for statistical, preference, and marketing purposes. Google Analytics cookies are anonymized. You can change your preference by clicking on 'Configure'. By clicking on 'Accept', you accept the use of all cookies as described in our privacy statement .
Choose your privacy preferences
Through the cookie statement on our website, you can change or withdraw your consent at any time. In our privacy policy, you can find more information about who we are, how you can contact us, and how we process personal data.
Preferences
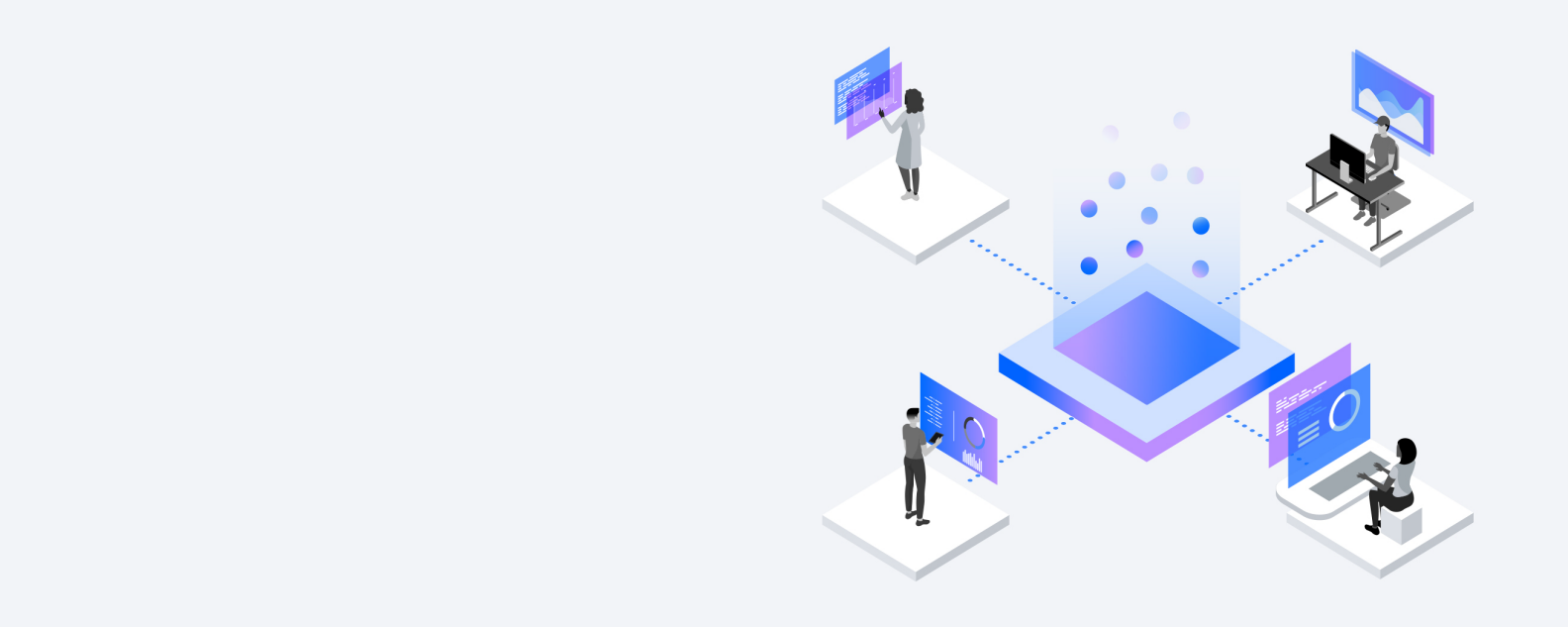
The IBM® SPSS® software platform offers advanced statistical analysis, a vast library of machine learning algorithms, text analysis, open-source extensibility, integration with big data and seamless deployment into applications.
Its ease of use, flexibility and scalability make SPSS accessible to users of all skill levels. What’s more, it’s suitable for projects of all sizes and levels of complexity, and can help you find new opportunities, improve efficiency and minimize risk.
Within the SPSS software family of products, IBM SPSS Statistics supports a top-down, hypothesis testing approach to your data, while IBM SPSS Modeler exposes patterns and models hidden in data through a bottom-up, hypothesis generation approach.
The AI studio that brings together traditional machine learning along with the new generative AI capabilities powered by foundation models.
SPSS Statistics for Students
Prepare and analyze data with an easy-to-use interface without having to write code.
Choose from purchase options including subscription and traditional licenses.
Empower coders, noncoders and analysts with visual data science tools.
IBM SPSS Modeler helps you tap into data assets and modern applications, with algorithms and models that are ready for immediate use.
IBM SPSS Modeler is available on IBM Cloud Pak for Data. Take advantage of IBM SPSS Modeler on the public cloud.
Manage analytical assets, automate processes and share results more efficiently and securely.
Get descriptive and predictive analytics, data preparation and real-time scoring.
Use structural equation modeling (SEM) to test hypotheses and gain new insights from data.
Create a platform that can make predictive analytics easier for big data.
Find support resources for SPSS Statistics.
Get technical tips and insights from other SPSS users.
Gain new perspective through expert guidance.
Find support resources for IBM SPSS Modeler.
Learn how to use linear regression analysis to predict the value of a variable based on the value of another variable.
Learn how logistic regression estimates the probability of an event occurring, based on a dataset of independent variables.
Learn about new statistical procedures, data visualization tools and other improvements in SPSS Statistics 29.
Discover how you can uncover data insights that solve business and research problems.
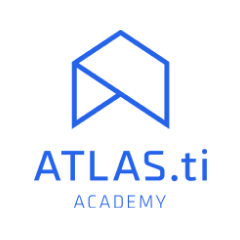
Quantitative research
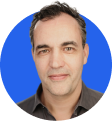
Objectives and applications
Quantitative research methods, choosing a quantitative research design, software for quantitative research.
Quantitative and qualitative research are commonly considered differing fundamentally. Yet, their objectives, as well as their applications, overlap in numerous ways. Quantitative Research is considered to have as its main purpose the quantification of data. This allows generalizations of results from a sample to an entire population of interest and the measurement of the incidence of various views and opinions in a given sample.
Yet, quantitative research is not infrequently followed by qualitative research, which aims to explore select findings further. Qualitative research is considered particularly suitable for gaining an in-depth understanding of underlying reasons and motivations. It provides insights into the setting of a problem. At the same time, it frequently generates ideas and hypotheses for later quantitative research.
Quantitative research measures the frequency or intensity of a phenomenon or its distribution, hypotheses can be tested, and insights inferred. At the beginning of the research process, theories about the facts under investigation have already been proposed, from which hypotheses are derived. The actual data are then collected by quantitative methods. In the social sciences, often, these are surveys using questionnaires or experiments. Statistical methods are used to dissect and evaluate the data, often using control groups. The research process results are then, in turn, related to the previously established theories and interpreted.
The advantages of quantitative research are high reliability, fast processing of large amounts of data, and high comparability. There are several methods of quantitative research:
- standardized surveys
- standardized observations
- experiments and trials
- quantitative content analysis
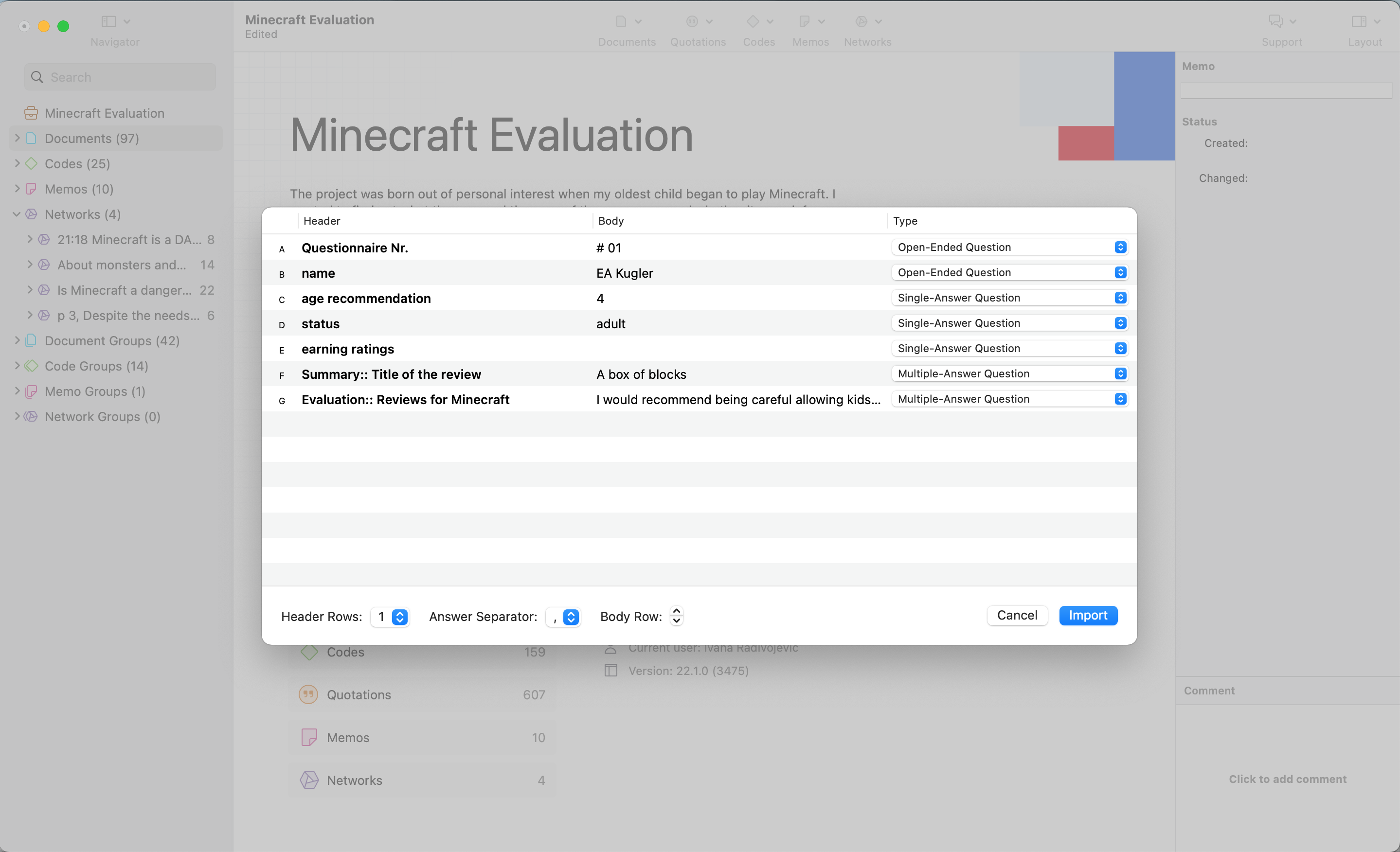
Analysis of ideas, actions, and values made possible with ATLAS.ti.
Turn your data into key insights with our powerful tools. Download a free trial today.
The research design is composed of:
- Type of research
- Data collection
- Data description
- Method of analysis
Which method of data collection and analysis is suitable depends on the research questions.
A distinction can be made between dependent and independent variables in quantitative research. Independent variables are considered to have an effect on other variables in the research context. They influence the dependent variable(s). Regression analysis can be run to determine whether an independent variable has an effect. For example, one can examine the bathing time (dependent variable) of swimming pool guests as a function of the water temperature (independent variable).
Correlational analysis can be used to determine whether two variables are related, but no cause and effect relationship can be established. For example, it has been observed that more children are born in places where many storks live. This however does not mean that storks deliver babies. The simple explanation for this observation is that birth rates are higher in the countryside, and storks also prefer to live in this environment.
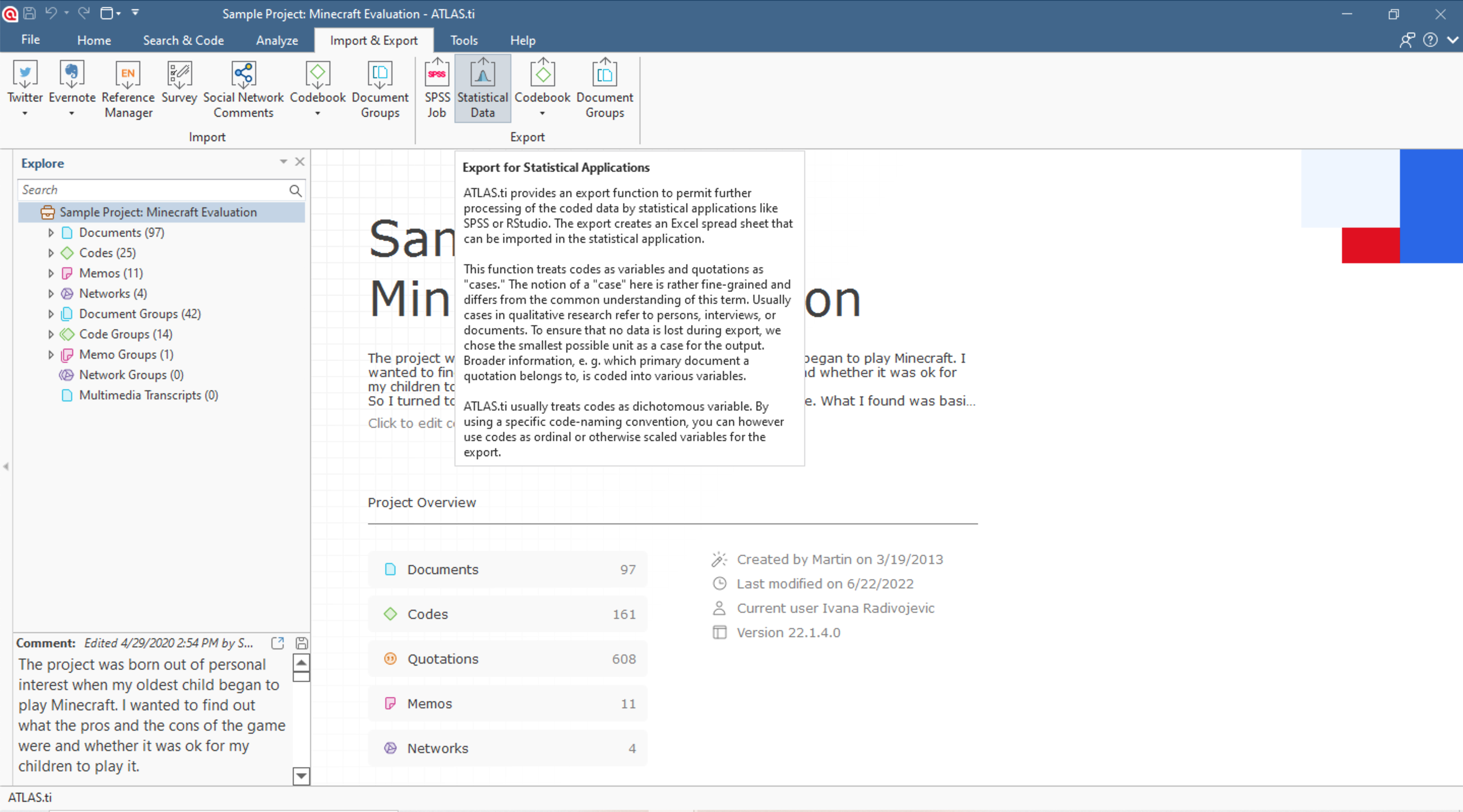
Quantitative research, predominantly statistical analysis, is common in the social sciences. Many software programs designed for use with quantitative data are available today. The main requirements for such packages are that they are comprehensive and flexible. A useful statistical software tool can generate tabulated reports, charts, and plots of distributions and trends and generate descriptive statistics and more complex statistical analyses. Lastly, a user interface that makes it very easy and intuitive for all levels of users is a must.
Examples of statistical analysis software are SPSS, Excel, SAS, or R. The presentation of results of studies usually takes place in the form of tables or graphs.
Suppose you have used ATLAS.ti for analyzing qualitative data. If your sample is sufficiently large, and you want to confirm results via statistical procedures, you can export your ATLAS.ti data for use in SPSS, Excel, SAS, or R. ATLAS.it offers two output options - an SPSS syntax file or a generic Excel file for input in any statistical software. Each coded data segment becomes a case, and each code and code group a variable.
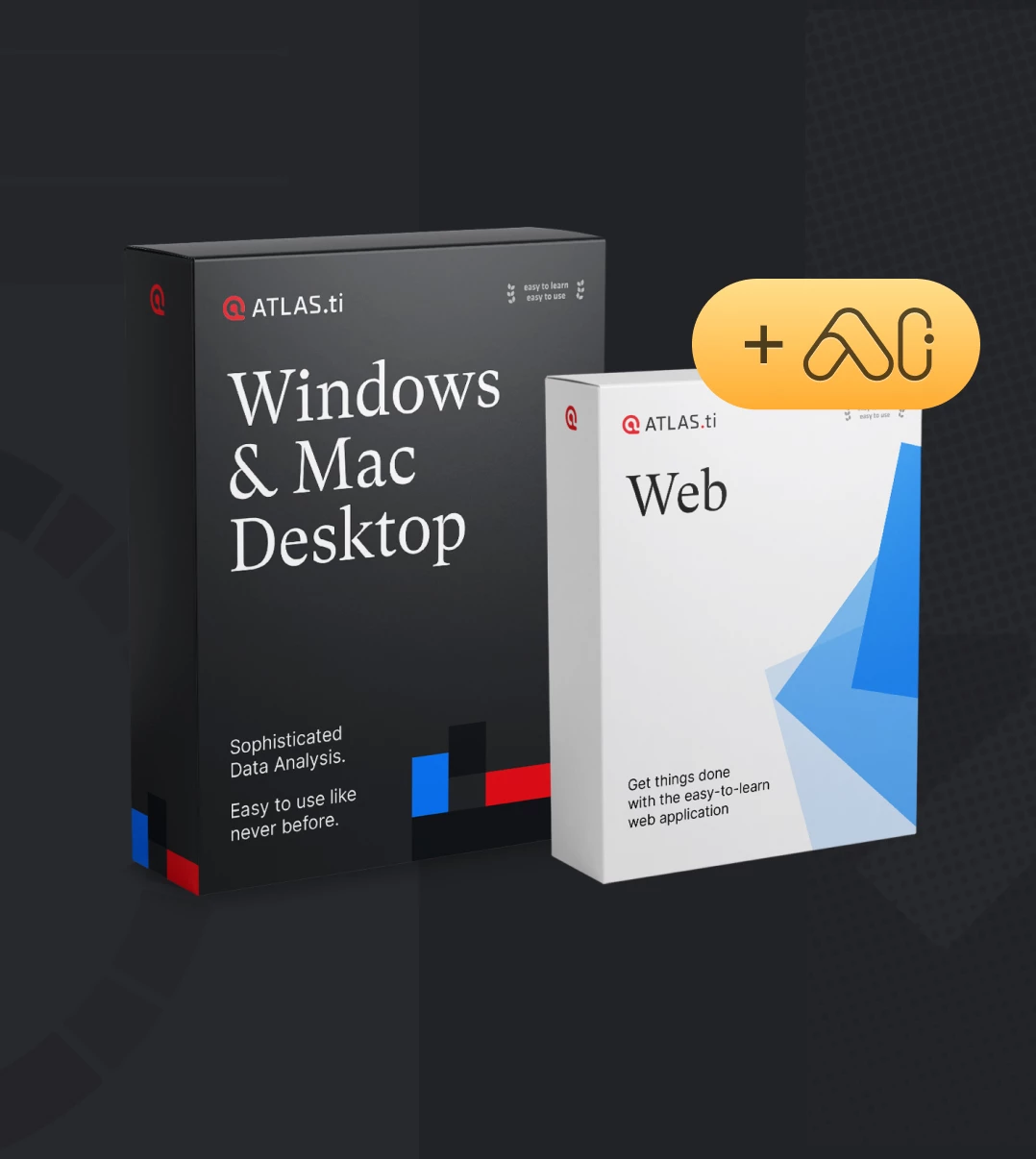
Analyze transcripts, notes, and more with ATLAS.ti
Intuitive tools to help you with your research. Check them out with a free trial of ATLAS.ti.
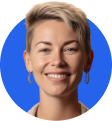
Libraries | Research Guides
Software for data analysis.
- Quantitative Tools
- Qualitative Tools
Meet one-on-one with a research data specialist from the library or NUIT.
Request a Consult
Statistical Software Guides and Tutorials

- Sage Research Methods Core Collection This link opens in a new window A collection of e-books and other resources covering research methods in the social and behavioral sciences. It contains the popular Little Green Book series as well as other titles on quantitative analysis
- NUIT Research Data Services: Training and Learning NUIT offers data analysis training through workshops and online learning.
- LinkedIn Learning Northwestern provides faculty, staff, and students with access to this suite of online courses.
Online Courses

- IBM's SPSS User Guide
- SPSS Tutorials
Online Tutorials

- Stata Documentation The official user guide, along with manuals and examples for using specific statistical methods in Stata.
- Stata Learning Modules Beginner-friendly guide to Stata from UCLA's Advanced Research Computing.
- SAS Learning Modules Beginner-friendly guide to SAS from UCLA's Advanced Research Computing.
- Google's Python Class Unlike R, Python is a general-purpose programming language. This site offers a more general introduction to Python, which you may want for background knowledge before moving on to using Python for data analysis.
Accessing Software
Open-source software.
Both R and Python are free and open source. NUIT's Research Data Services offers installation guidelines:
- I nstalling R and RStudio
- Installing Python and Jupyter
Proprietary Software
Northwestern provides access to licensed software in the library computer labs and on NUWorkspace , a virtual desktop. NUIT also makes free or discounted software licenses available. In addition to these campus-wide resources, your department may have software licenses you can access.
Sarah Thorngate

- << Previous: Home
- Next: Qualitative Tools >>
- Last Updated: Feb 23, 2024 11:33 AM
- URL: https://libguides.northwestern.edu/data2

Quantitative Data Analysis 101
The lingo, methods and techniques, explained simply.
By: Derek Jansen (MBA) and Kerryn Warren (PhD) | December 2020
Quantitative data analysis is one of those things that often strikes fear in students. It’s totally understandable – quantitative analysis is a complex topic, full of daunting lingo , like medians, modes, correlation and regression. Suddenly we’re all wishing we’d paid a little more attention in math class…
The good news is that while quantitative data analysis is a mammoth topic, gaining a working understanding of the basics isn’t that hard , even for those of us who avoid numbers and math . In this post, we’ll break quantitative analysis down into simple , bite-sized chunks so you can approach your research with confidence.
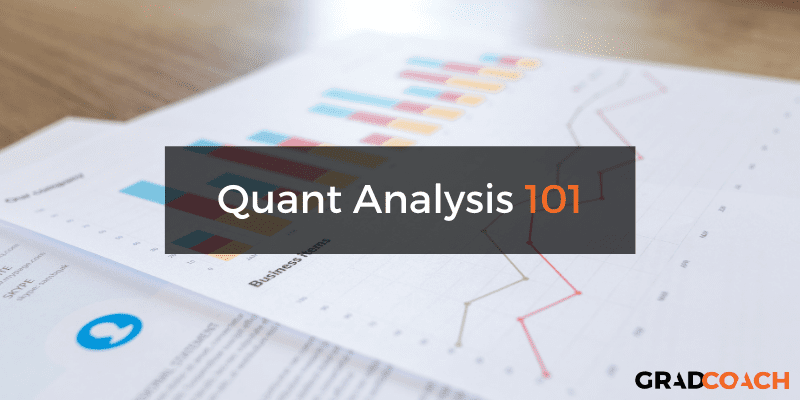
Overview: Quantitative Data Analysis 101
- What (exactly) is quantitative data analysis?
- When to use quantitative analysis
- How quantitative analysis works
The two “branches” of quantitative analysis
- Descriptive statistics 101
- Inferential statistics 101
- How to choose the right quantitative methods
- Recap & summary
What is quantitative data analysis?
Despite being a mouthful, quantitative data analysis simply means analysing data that is numbers-based – or data that can be easily “converted” into numbers without losing any meaning.
For example, category-based variables like gender, ethnicity, or native language could all be “converted” into numbers without losing meaning – for example, English could equal 1, French 2, etc.
This contrasts against qualitative data analysis, where the focus is on words, phrases and expressions that can’t be reduced to numbers. If you’re interested in learning about qualitative analysis, check out our post and video here .
What is quantitative analysis used for?
Quantitative analysis is generally used for three purposes.
- Firstly, it’s used to measure differences between groups . For example, the popularity of different clothing colours or brands.
- Secondly, it’s used to assess relationships between variables . For example, the relationship between weather temperature and voter turnout.
- And third, it’s used to test hypotheses in a scientifically rigorous way. For example, a hypothesis about the impact of a certain vaccine.
Again, this contrasts with qualitative analysis , which can be used to analyse people’s perceptions and feelings about an event or situation. In other words, things that can’t be reduced to numbers.
How does quantitative analysis work?
Well, since quantitative data analysis is all about analysing numbers , it’s no surprise that it involves statistics . Statistical analysis methods form the engine that powers quantitative analysis, and these methods can vary from pretty basic calculations (for example, averages and medians) to more sophisticated analyses (for example, correlations and regressions).
Sounds like gibberish? Don’t worry. We’ll explain all of that in this post. Importantly, you don’t need to be a statistician or math wiz to pull off a good quantitative analysis. We’ll break down all the technical mumbo jumbo in this post.
Need a helping hand?
As I mentioned, quantitative analysis is powered by statistical analysis methods . There are two main “branches” of statistical methods that are used – descriptive statistics and inferential statistics . In your research, you might only use descriptive statistics, or you might use a mix of both , depending on what you’re trying to figure out. In other words, depending on your research questions, aims and objectives . I’ll explain how to choose your methods later.
So, what are descriptive and inferential statistics?
Well, before I can explain that, we need to take a quick detour to explain some lingo. To understand the difference between these two branches of statistics, you need to understand two important words. These words are population and sample .
First up, population . In statistics, the population is the entire group of people (or animals or organisations or whatever) that you’re interested in researching. For example, if you were interested in researching Tesla owners in the US, then the population would be all Tesla owners in the US.
However, it’s extremely unlikely that you’re going to be able to interview or survey every single Tesla owner in the US. Realistically, you’ll likely only get access to a few hundred, or maybe a few thousand owners using an online survey. This smaller group of accessible people whose data you actually collect is called your sample .
So, to recap – the population is the entire group of people you’re interested in, and the sample is the subset of the population that you can actually get access to. In other words, the population is the full chocolate cake , whereas the sample is a slice of that cake.
So, why is this sample-population thing important?
Well, descriptive statistics focus on describing the sample , while inferential statistics aim to make predictions about the population, based on the findings within the sample. In other words, we use one group of statistical methods – descriptive statistics – to investigate the slice of cake, and another group of methods – inferential statistics – to draw conclusions about the entire cake. There I go with the cake analogy again…
With that out the way, let’s take a closer look at each of these branches in more detail.
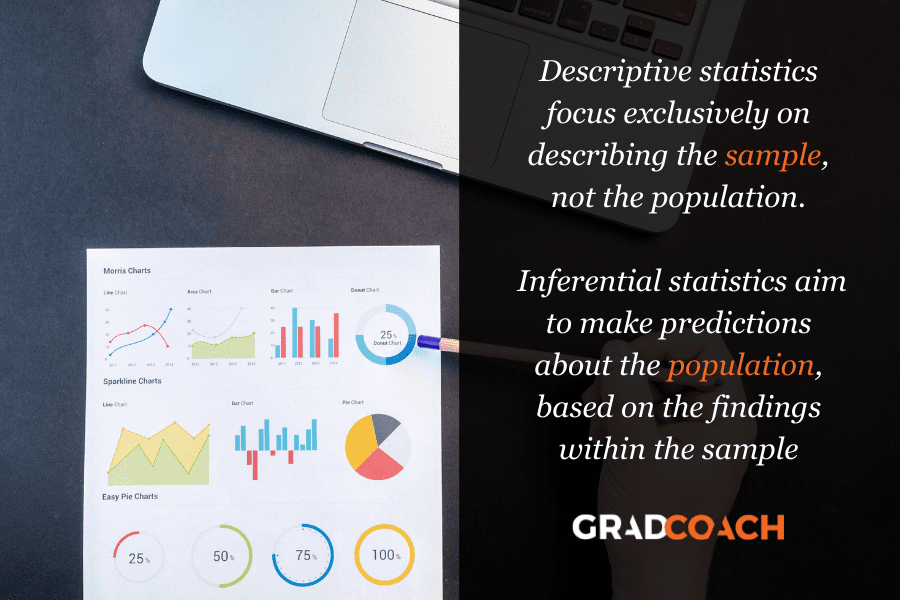
Branch 1: Descriptive Statistics
Descriptive statistics serve a simple but critically important role in your research – to describe your data set – hence the name. In other words, they help you understand the details of your sample . Unlike inferential statistics (which we’ll get to soon), descriptive statistics don’t aim to make inferences or predictions about the entire population – they’re purely interested in the details of your specific sample .
When you’re writing up your analysis, descriptive statistics are the first set of stats you’ll cover, before moving on to inferential statistics. But, that said, depending on your research objectives and research questions , they may be the only type of statistics you use. We’ll explore that a little later.
So, what kind of statistics are usually covered in this section?
Some common statistical tests used in this branch include the following:
- Mean – this is simply the mathematical average of a range of numbers.
- Median – this is the midpoint in a range of numbers when the numbers are arranged in numerical order. If the data set makes up an odd number, then the median is the number right in the middle of the set. If the data set makes up an even number, then the median is the midpoint between the two middle numbers.
- Mode – this is simply the most commonly occurring number in the data set.
- In cases where most of the numbers are quite close to the average, the standard deviation will be relatively low.
- Conversely, in cases where the numbers are scattered all over the place, the standard deviation will be relatively high.
- Skewness . As the name suggests, skewness indicates how symmetrical a range of numbers is. In other words, do they tend to cluster into a smooth bell curve shape in the middle of the graph, or do they skew to the left or right?
Feeling a bit confused? Let’s look at a practical example using a small data set.
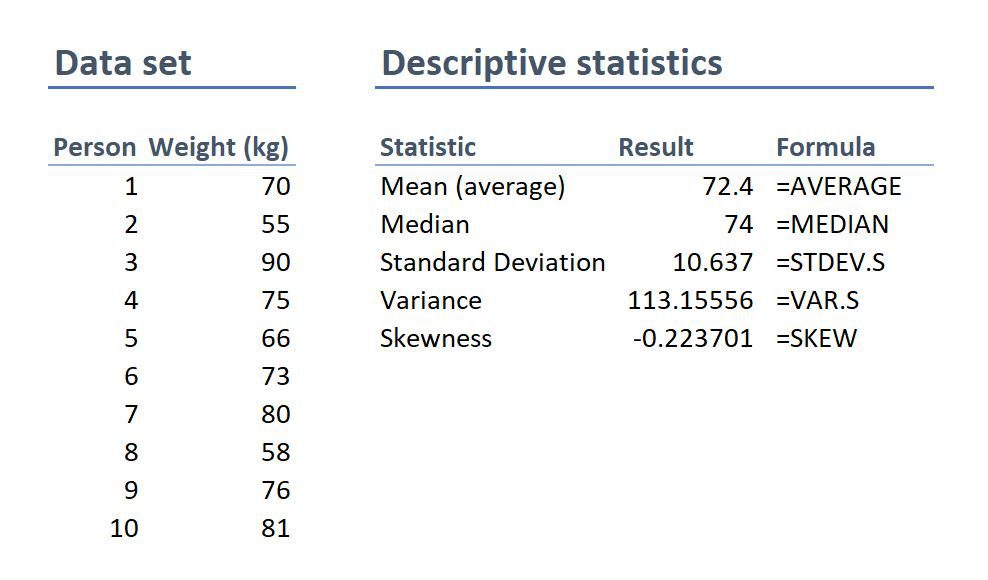
On the left-hand side is the data set. This details the bodyweight of a sample of 10 people. On the right-hand side, we have the descriptive statistics. Let’s take a look at each of them.
First, we can see that the mean weight is 72.4 kilograms. In other words, the average weight across the sample is 72.4 kilograms. Straightforward.
Next, we can see that the median is very similar to the mean (the average). This suggests that this data set has a reasonably symmetrical distribution (in other words, a relatively smooth, centred distribution of weights, clustered towards the centre).
In terms of the mode , there is no mode in this data set. This is because each number is present only once and so there cannot be a “most common number”. If there were two people who were both 65 kilograms, for example, then the mode would be 65.
Next up is the standard deviation . 10.6 indicates that there’s quite a wide spread of numbers. We can see this quite easily by looking at the numbers themselves, which range from 55 to 90, which is quite a stretch from the mean of 72.4.
And lastly, the skewness of -0.2 tells us that the data is very slightly negatively skewed. This makes sense since the mean and the median are slightly different.
As you can see, these descriptive statistics give us some useful insight into the data set. Of course, this is a very small data set (only 10 records), so we can’t read into these statistics too much. Also, keep in mind that this is not a list of all possible descriptive statistics – just the most common ones.
But why do all of these numbers matter?
While these descriptive statistics are all fairly basic, they’re important for a few reasons:
- Firstly, they help you get both a macro and micro-level view of your data. In other words, they help you understand both the big picture and the finer details.
- Secondly, they help you spot potential errors in the data – for example, if an average is way higher than you’d expect, or responses to a question are highly varied, this can act as a warning sign that you need to double-check the data.
- And lastly, these descriptive statistics help inform which inferential statistical techniques you can use, as those techniques depend on the skewness (in other words, the symmetry and normality) of the data.
Simply put, descriptive statistics are really important , even though the statistical techniques used are fairly basic. All too often at Grad Coach, we see students skimming over the descriptives in their eagerness to get to the more exciting inferential methods, and then landing up with some very flawed results.
Don’t be a sucker – give your descriptive statistics the love and attention they deserve!
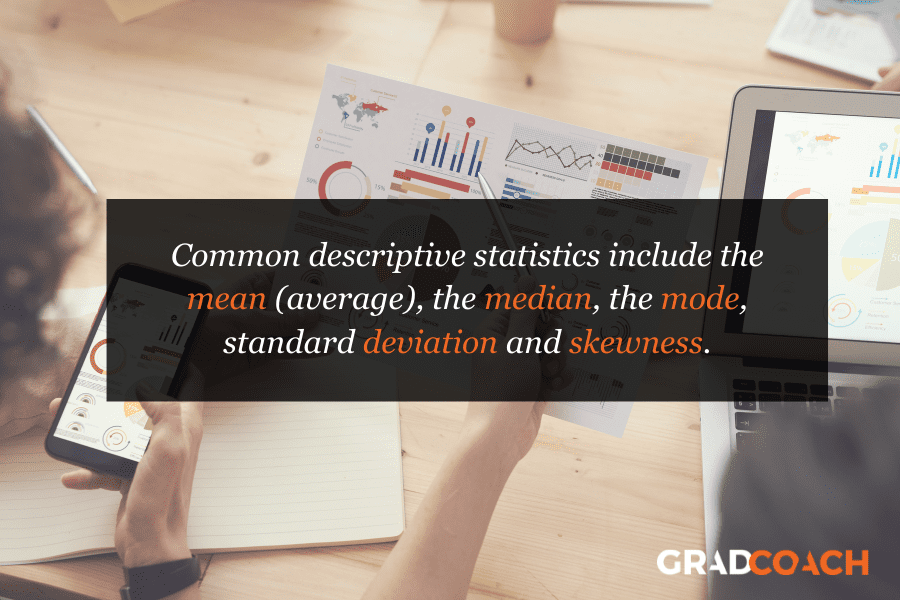
Branch 2: Inferential Statistics
As I mentioned, while descriptive statistics are all about the details of your specific data set – your sample – inferential statistics aim to make inferences about the population . In other words, you’ll use inferential statistics to make predictions about what you’d expect to find in the full population.
What kind of predictions, you ask? Well, there are two common types of predictions that researchers try to make using inferential stats:
- Firstly, predictions about differences between groups – for example, height differences between children grouped by their favourite meal or gender.
- And secondly, relationships between variables – for example, the relationship between body weight and the number of hours a week a person does yoga.
In other words, inferential statistics (when done correctly), allow you to connect the dots and make predictions about what you expect to see in the real world population, based on what you observe in your sample data. For this reason, inferential statistics are used for hypothesis testing – in other words, to test hypotheses that predict changes or differences.
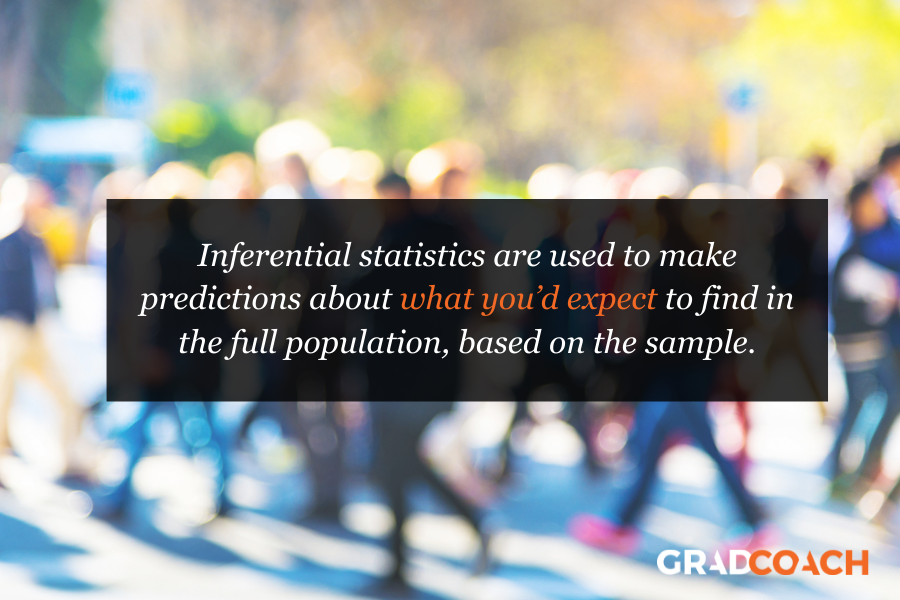
Of course, when you’re working with inferential statistics, the composition of your sample is really important. In other words, if your sample doesn’t accurately represent the population you’re researching, then your findings won’t necessarily be very useful.
For example, if your population of interest is a mix of 50% male and 50% female , but your sample is 80% male , you can’t make inferences about the population based on your sample, since it’s not representative. This area of statistics is called sampling, but we won’t go down that rabbit hole here (it’s a deep one!) – we’ll save that for another post .
What statistics are usually used in this branch?
There are many, many different statistical analysis methods within the inferential branch and it’d be impossible for us to discuss them all here. So we’ll just take a look at some of the most common inferential statistical methods so that you have a solid starting point.
First up are T-Tests . T-tests compare the means (the averages) of two groups of data to assess whether they’re statistically significantly different. In other words, do they have significantly different means, standard deviations and skewness.
This type of testing is very useful for understanding just how similar or different two groups of data are. For example, you might want to compare the mean blood pressure between two groups of people – one that has taken a new medication and one that hasn’t – to assess whether they are significantly different.
Kicking things up a level, we have ANOVA, which stands for “analysis of variance”. This test is similar to a T-test in that it compares the means of various groups, but ANOVA allows you to analyse multiple groups , not just two groups So it’s basically a t-test on steroids…
Next, we have correlation analysis . This type of analysis assesses the relationship between two variables. In other words, if one variable increases, does the other variable also increase, decrease or stay the same. For example, if the average temperature goes up, do average ice creams sales increase too? We’d expect some sort of relationship between these two variables intuitively , but correlation analysis allows us to measure that relationship scientifically .
Lastly, we have regression analysis – this is quite similar to correlation in that it assesses the relationship between variables, but it goes a step further to understand cause and effect between variables, not just whether they move together. In other words, does the one variable actually cause the other one to move, or do they just happen to move together naturally thanks to another force? Just because two variables correlate doesn’t necessarily mean that one causes the other.
Stats overload…
I hear you. To make this all a little more tangible, let’s take a look at an example of a correlation in action.
Here’s a scatter plot demonstrating the correlation (relationship) between weight and height. Intuitively, we’d expect there to be some relationship between these two variables, which is what we see in this scatter plot. In other words, the results tend to cluster together in a diagonal line from bottom left to top right.
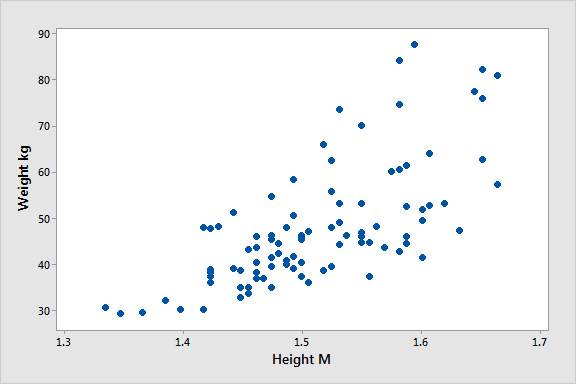
As I mentioned, these are are just a handful of inferential techniques – there are many, many more. Importantly, each statistical method has its own assumptions and limitations.
For example, some methods only work with normally distributed (parametric) data, while other methods are designed specifically for non-parametric data. And that’s exactly why descriptive statistics are so important – they’re the first step to knowing which inferential techniques you can and can’t use.
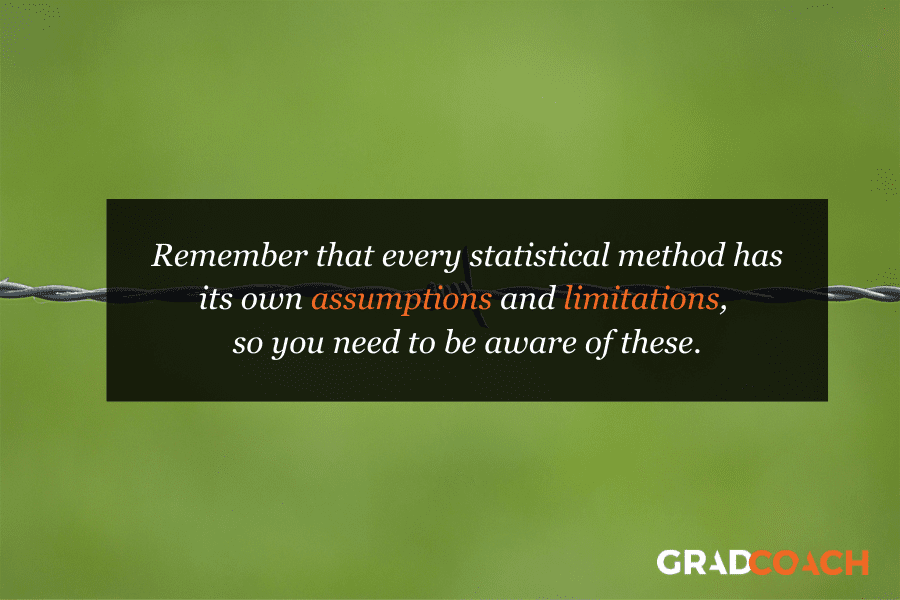
How to choose the right analysis method
To choose the right statistical methods, you need to think about two important factors :
- The type of quantitative data you have (specifically, level of measurement and the shape of the data). And,
- Your research questions and hypotheses
Let’s take a closer look at each of these.
Factor 1 – Data type
The first thing you need to consider is the type of data you’ve collected (or the type of data you will collect). By data types, I’m referring to the four levels of measurement – namely, nominal, ordinal, interval and ratio. If you’re not familiar with this lingo, check out the video below.
Why does this matter?
Well, because different statistical methods and techniques require different types of data. This is one of the “assumptions” I mentioned earlier – every method has its assumptions regarding the type of data.
For example, some techniques work with categorical data (for example, yes/no type questions, or gender or ethnicity), while others work with continuous numerical data (for example, age, weight or income) – and, of course, some work with multiple data types.
If you try to use a statistical method that doesn’t support the data type you have, your results will be largely meaningless . So, make sure that you have a clear understanding of what types of data you’ve collected (or will collect). Once you have this, you can then check which statistical methods would support your data types here .
If you haven’t collected your data yet, you can work in reverse and look at which statistical method would give you the most useful insights, and then design your data collection strategy to collect the correct data types.
Another important factor to consider is the shape of your data . Specifically, does it have a normal distribution (in other words, is it a bell-shaped curve, centred in the middle) or is it very skewed to the left or the right? Again, different statistical techniques work for different shapes of data – some are designed for symmetrical data while others are designed for skewed data.
This is another reminder of why descriptive statistics are so important – they tell you all about the shape of your data.
Factor 2: Your research questions
The next thing you need to consider is your specific research questions, as well as your hypotheses (if you have some). The nature of your research questions and research hypotheses will heavily influence which statistical methods and techniques you should use.
If you’re just interested in understanding the attributes of your sample (as opposed to the entire population), then descriptive statistics are probably all you need. For example, if you just want to assess the means (averages) and medians (centre points) of variables in a group of people.
On the other hand, if you aim to understand differences between groups or relationships between variables and to infer or predict outcomes in the population, then you’ll likely need both descriptive statistics and inferential statistics.
So, it’s really important to get very clear about your research aims and research questions, as well your hypotheses – before you start looking at which statistical techniques to use.
Never shoehorn a specific statistical technique into your research just because you like it or have some experience with it. Your choice of methods must align with all the factors we’ve covered here.
Time to recap…
You’re still with me? That’s impressive. We’ve covered a lot of ground here, so let’s recap on the key points:
- Quantitative data analysis is all about analysing number-based data (which includes categorical and numerical data) using various statistical techniques.
- The two main branches of statistics are descriptive statistics and inferential statistics . Descriptives describe your sample, whereas inferentials make predictions about what you’ll find in the population.
- Common descriptive statistical methods include mean (average), median , standard deviation and skewness .
- Common inferential statistical methods include t-tests , ANOVA , correlation and regression analysis.
- To choose the right statistical methods and techniques, you need to consider the type of data you’re working with , as well as your research questions and hypotheses.
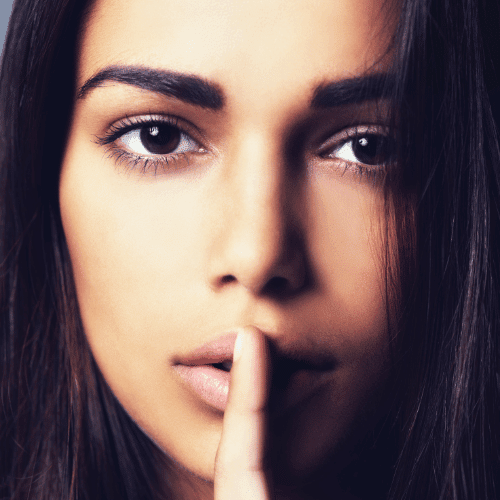
Psst... there’s more!
This post was based on one of our popular Research Bootcamps . If you're working on a research project, you'll definitely want to check this out ...
You Might Also Like:
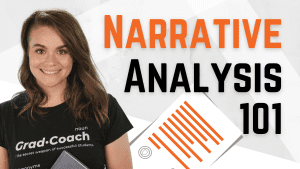
74 Comments
Hi, I have read your article. Such a brilliant post you have created.
Thank you for the feedback. Good luck with your quantitative analysis.
Thank you so much.
Thank you so much. I learnt much well. I love your summaries of the concepts. I had love you to explain how to input data using SPSS
Amazing and simple way of breaking down quantitative methods.
This is beautiful….especially for non-statisticians. I have skimmed through but I wish to read again. and please include me in other articles of the same nature when you do post. I am interested. I am sure, I could easily learn from you and get off the fear that I have had in the past. Thank you sincerely.
Send me every new information you might have.
i need every new information
Thank you for the blog. It is quite informative. Dr Peter Nemaenzhe PhD
It is wonderful. l’ve understood some of the concepts in a more compréhensive manner
Your article is so good! However, I am still a bit lost. I am doing a secondary research on Gun control in the US and increase in crime rates and I am not sure which analysis method I should use?
Based on the given learning points, this is inferential analysis, thus, use ‘t-tests, ANOVA, correlation and regression analysis’
Well explained notes. Am an MPH student and currently working on my thesis proposal, this has really helped me understand some of the things I didn’t know.
I like your page..helpful
wonderful i got my concept crystal clear. thankyou!!
This is really helpful , thank you
Thank you so much this helped
Wonderfully explained
thank u so much, it was so informative
THANKYOU, this was very informative and very helpful
This is great GRADACOACH I am not a statistician but I require more of this in my thesis
Include me in your posts.
This is so great and fully useful. I would like to thank you again and again.
Glad to read this article. I’ve read lot of articles but this article is clear on all concepts. Thanks for sharing.
Thank you so much. This is a very good foundation and intro into quantitative data analysis. Appreciate!
You have a very impressive, simple but concise explanation of data analysis for Quantitative Research here. This is a God-send link for me to appreciate research more. Thank you so much!
Avery good presentation followed by the write up. yes you simplified statistics to make sense even to a layman like me. Thank so much keep it up. The presenter did ell too. i would like more of this for Qualitative and exhaust more of the test example like the Anova.
This is a very helpful article, couldn’t have been clearer. Thank you.
Awesome and phenomenal information.Well done
The video with the accompanying article is super helpful to demystify this topic. Very well done. Thank you so much.
thank you so much, your presentation helped me a lot
I don’t know how should I express that ur article is saviour for me 🥺😍
It is well defined information and thanks for sharing. It helps me a lot in understanding the statistical data.
I gain a lot and thanks for sharing brilliant ideas, so wish to be linked on your email update.
Very helpful and clear .Thank you Gradcoach.
Thank for sharing this article, well organized and information presented are very clear.
VERY INTERESTING AND SUPPORTIVE TO NEW RESEARCHERS LIKE ME. AT LEAST SOME BASICS ABOUT QUANTITATIVE.
An outstanding, well explained and helpful article. This will help me so much with my data analysis for my research project. Thank you!
wow this has just simplified everything i was scared of how i am gonna analyse my data but thanks to you i will be able to do so
simple and constant direction to research. thanks
This is helpful
Great writing!! Comprehensive and very helpful.
Do you provide any assistance for other steps of research methodology like making research problem testing hypothesis report and thesis writing?
Thank you so much for such useful article!
Amazing article. So nicely explained. Wow
Very insightfull. Thanks
I am doing a quality improvement project to determine if the implementation of a protocol will change prescribing habits. Would this be a t-test?
The is a very helpful blog, however, I’m still not sure how to analyze my data collected. I’m doing a research on “Free Education at the University of Guyana”
tnx. fruitful blog!
So I am writing exams and would like to know how do establish which method of data analysis to use from the below research questions: I am a bit lost as to how I determine the data analysis method from the research questions.
Do female employees report higher job satisfaction than male employees with similar job descriptions across the South African telecommunications sector? – I though that maybe Chi Square could be used here. – Is there a gender difference in talented employees’ actual turnover decisions across the South African telecommunications sector? T-tests or Correlation in this one. – Is there a gender difference in the cost of actual turnover decisions across the South African telecommunications sector? T-tests or Correlation in this one. – What practical recommendations can be made to the management of South African telecommunications companies on leveraging gender to mitigate employee turnover decisions?
Your assistance will be appreciated if I could get a response as early as possible tomorrow
This was quite helpful. Thank you so much.
wow I got a lot from this article, thank you very much, keep it up
Thanks for yhe guidance. Can you send me this guidance on my email? To enable offline reading?
Thank you very much, this service is very helpful.
Every novice researcher needs to read this article as it puts things so clear and easy to follow. Its been very helpful.
Wonderful!!!! you explained everything in a way that anyone can learn. Thank you!!
I really enjoyed reading though this. Very easy to follow. Thank you
Many thanks for your useful lecture, I would be really appreciated if you could possibly share with me the PPT of presentation related to Data type?
Thank you very much for sharing, I got much from this article
This is a very informative write-up. Kindly include me in your latest posts.
Very interesting mostly for social scientists
Thank you so much, very helpfull
You’re welcome 🙂
woow, its great, its very informative and well understood because of your way of writing like teaching in front of me in simple languages.
I have been struggling to understand a lot of these concepts. Thank you for the informative piece which is written with outstanding clarity.
very informative article. Easy to understand
Beautiful read, much needed.
Always greet intro and summary. I learn so much from GradCoach
Quite informative. Simple and clear summary.
I thoroughly enjoyed reading your informative and inspiring piece. Your profound insights into this topic truly provide a better understanding of its complexity. I agree with the points you raised, especially when you delved into the specifics of the article. In my opinion, that aspect is often overlooked and deserves further attention.
Absolutely!!! Thank you
Thank you very much for this post. It made me to understand how to do my data analysis.
Submit a Comment Cancel reply
Your email address will not be published. Required fields are marked *
Save my name, email, and website in this browser for the next time I comment.
- Print Friendly
Learn / Guides / Quantitative data analysis guide
Back to guides
8 quantitative data analysis methods to turn numbers into insights
Setting up a few new customer surveys or creating a fresh Google Analytics dashboard feels exciting…until the numbers start rolling in. You want to turn responses into a plan to present to your team and leaders—but which quantitative data analysis method do you use to make sense of the facts and figures?
Last updated
Reading time.
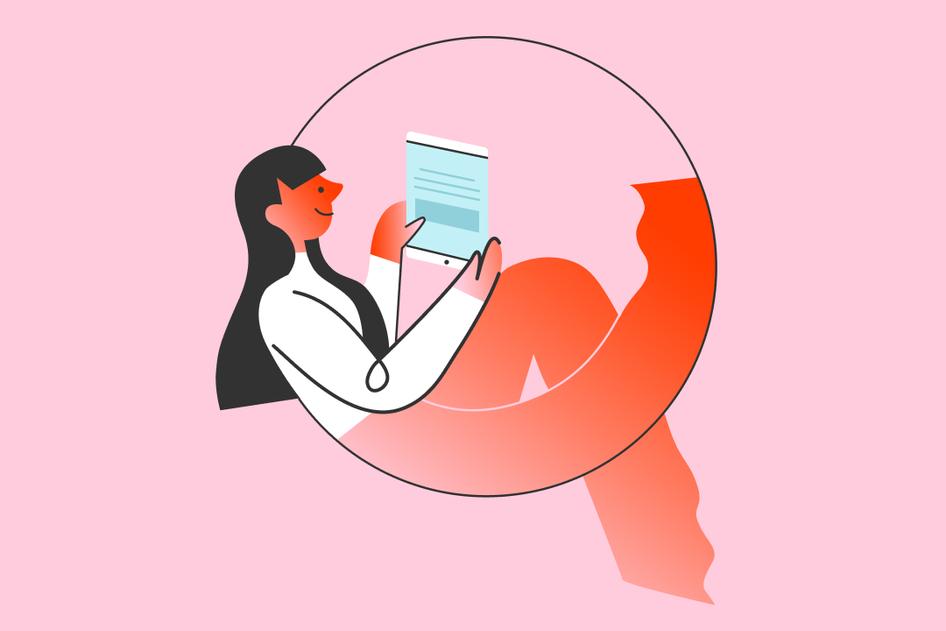
This guide lists eight quantitative research data analysis techniques to help you turn numeric feedback into actionable insights to share with your team and make customer-centric decisions.
To pick the right technique that helps you bridge the gap between data and decision-making, you first need to collect quantitative data from sources like:
Google Analytics
Survey results
On-page feedback scores
Fuel your quantitative analysis with real-time data
Use Hotjar’s tools to collect quantitative data that helps you stay close to customers.
Then, choose an analysis method based on the type of data and how you want to use it.
Descriptive data analysis summarizes results—like measuring website traffic—that help you learn about a problem or opportunity. The descriptive analysis methods we’ll review are:
Multiple choice response rates
Response volume over time
Net Promoter Score®
Inferential data analyzes the relationship between data—like which customer segment has the highest average order value—to help you make hypotheses about product decisions. Inferential analysis methods include:
Cross-tabulation
Weighted customer feedback
You don’t need to worry too much about these specific terms since each quantitative data analysis method listed below explains when and how to use them. Let’s dive in!
1. Compare multiple-choice response rates
The simplest way to analyze survey data is by comparing the percentage of your users who chose each response, which summarizes opinions within your audience.
To do this, divide the number of people who chose a specific response by the total respondents for your multiple-choice survey. Imagine 100 customers respond to a survey about what product category they want to see. If 25 people said ‘snacks’, 25% of your audience favors that category, so you know that adding a snacks category to your list of filters or drop-down menu will make the purchasing process easier for them.
💡Pro tip: ask open-ended survey questions to dig deeper into customer motivations.
A multiple-choice survey measures your audience’s opinions, but numbers don’t tell you why they think the way they do—you need to combine quantitative and qualitative data to learn that.
One research method to learn about customer motivations is through an open-ended survey question. Giving customers space to express their thoughts in their own words—unrestricted by your pre-written multiple-choice questions—prevents you from making assumptions.
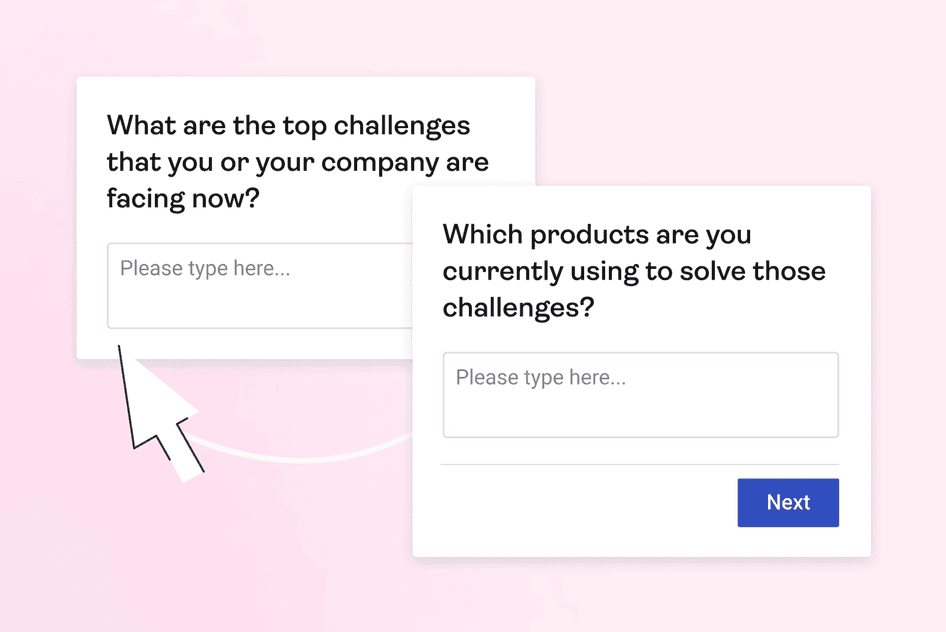
Hotjar’s open-ended surveys have a text box for customers to type a response
2. Cross-tabulate to compare responses between groups
To understand how responses and behavior vary within your audience, compare your quantitative data by group. Use raw numbers, like the number of website visitors, or percentages, like questionnaire responses, across categories like traffic sources or customer segments.
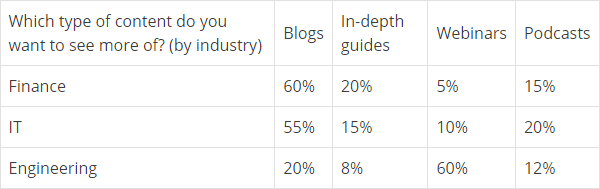
Let’s say you ask your audience what their most-used feature is because you want to know what to highlight on your pricing page. Comparing the most common response for free trial users vs. established customers lets you strategically introduce features at the right point in the customer journey .
💡Pro tip: get some face-to-face time to discover nuances in customer feedback.
Rather than treating your customers as a monolith, use Hotjar to conduct interviews to learn about individuals and subgroups. If you aren’t sure what to ask, start with your quantitative data results. If you notice competing trends between customer segments, have a few conversations with individuals from each group to dig into their unique motivations.
Hotjar Engage lets you identify specific customer segments you want to talk to
Mode is the most common answer in a data set, which means you use it to discover the most popular response for questions with numeric answer options. Mode and median (that's next on the list) are useful to compare to the average in case responses on extreme ends of the scale (outliers) skew the outcome.
Let’s say you want to know how most customers feel about your website, so you use an on-page feedback widget to collect ratings on a scale of one to five.
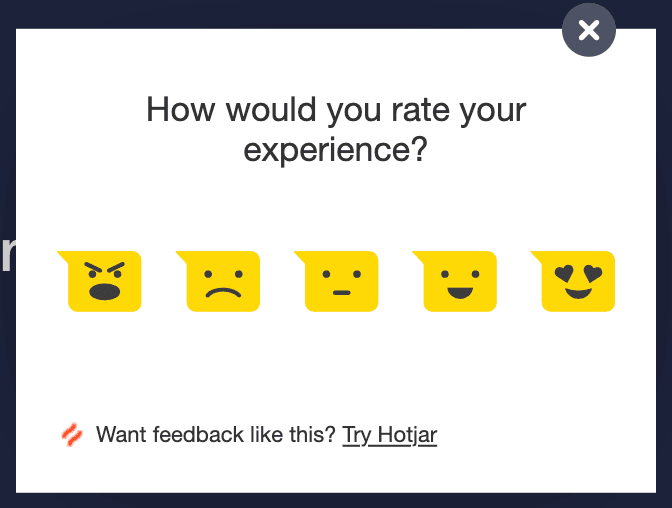
If the mode, or most common response, is a three, you can assume most people feel somewhat positive. But suppose the second-most common response is a one (which would bring the average down). In that case, you need to investigate why so many customers are unhappy.
💡Pro tip: watch recordings to understand how customers interact with your website.
So you used on-page feedback to learn how customers feel about your website, and the mode was two out of five. Ouch. Use Hotjar Recordings to see how customers move around on and interact with your pages to find the source of frustration.
Hotjar Recordings lets you watch individual visitors interact with your site, like how they scroll, hover, and click
Median reveals the middle of the road of your quantitative data by lining up all numeric values in ascending order and then looking at the data point in the middle. Use the median method when you notice a few outliers that bring the average up or down and compare the analysis outcomes.
For example, if your price sensitivity survey has outlandish responses and you want to identify a reasonable middle ground of what customers are willing to pay—calculate the median.
💡Pro-tip: review and clean your data before analysis.
Take a few minutes to familiarize yourself with quantitative data results before you push them through analysis methods. Inaccurate or missing information can complicate your calculations, and it’s less frustrating to resolve issues at the start instead of problem-solving later.
Here are a few data-cleaning tips to keep in mind:
Remove or separate irrelevant data, like responses from a customer segment or time frame you aren’t reviewing right now
Standardize data from multiple sources, like a survey that let customers indicate they use your product ‘daily’ vs. on-page feedback that used the phrasing ‘more than once a week’
Acknowledge missing data, like some customers not answering every question. Just note that your totals between research questions might not match.
Ensure you have enough responses to have a statistically significant result
Decide if you want to keep or remove outlying data. For example, maybe there’s evidence to support a high-price tier, and you shouldn’t dismiss less price-sensitive respondents. Other times, you might want to get rid of obviously trolling responses.
5. Mean (AKA average)
Finding the average of a dataset is an essential quantitative data analysis method and an easy task. First, add all your quantitative data points, like numeric survey responses or daily sales revenue. Then, divide the sum of your data points by the number of responses to get a single number representing the entire dataset.
Use the average of your quant data when you want a summary, like the average order value of your transactions between different sales pages. Then, use your average to benchmark performance, compare over time, or uncover winners across segments—like which sales page design produces the most value.
💡Pro tip: use heatmaps to find attention-catching details numbers can’t give you.
Calculating the average of your quant data set reveals the outcome of customer interactions. However, you need qualitative data like a heatmap to learn about everything that led to that moment. A heatmap uses colors to illustrate where most customers look and click on a page to reveal what drives (or drops) momentum.
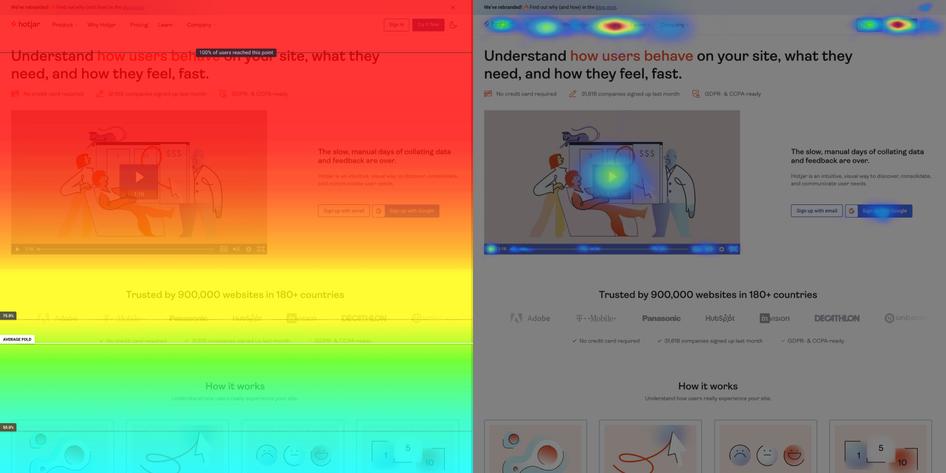
Hotjar Heatmaps uses color to visualize what most visitors see, ignore, and click on
6. Measure the volume of responses over time
Some quantitative data analysis methods are an ongoing project, like comparing top website referral sources by month to gauge the effectiveness of new channels. Analyzing the same metric at regular intervals lets you compare trends and changes.
Look at quantitative survey results, website sessions, sales, cart abandons, or clicks regularly to spot trouble early or monitor the impact of a new initiative.
Here are a few areas you can measure over time (and how to use qualitative research methods listed above to add context to your results):
7. Net Promoter Score®
Net Promoter Score® ( NPS ®) is a popular customer loyalty and satisfaction measurement that also serves as a quantitative data analysis method.
NPS surveys ask customers to rate how likely they are to recommend you on a scale of zero to ten. Calculate it by subtracting the percentage of customers who answer the NPS question with a six or lower (known as ‘detractors’) from those who respond with a nine or ten (known as ‘promoters’). Your NPS score will fall between -100 and 100, and you want a positive number indicating more promoters than detractors.
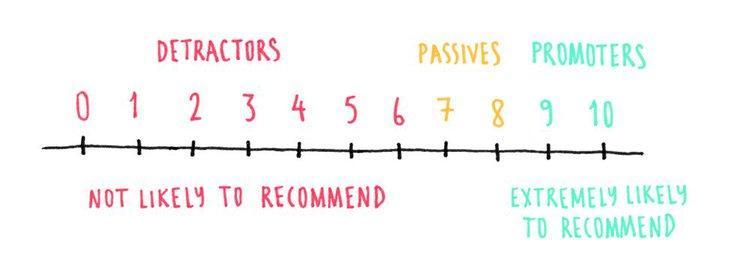
💡Pro tip : like other quantitative data analysis methods, you can review NPS scores over time as a satisfaction benchmark. You can also use it to understand which customer segment is most satisfied or which customers may be willing to share their stories for promotional materials.
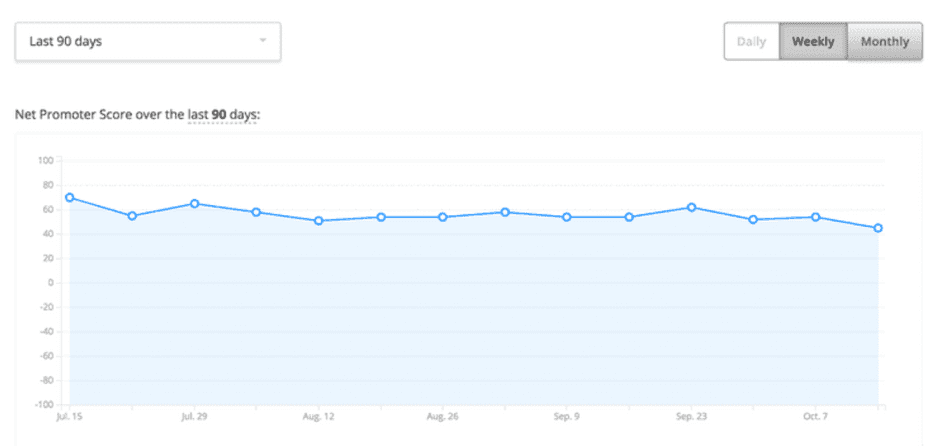
Review NPS score trends with Hotjar to spot any sudden spikes and benchmark performance over time
8. Weight customer feedback
So far, the quantitative data analysis methods on this list have leveraged numeric data only. However, there are ways to turn qualitative data into quantifiable feedback and to mix and match data sources. For example, you might need to analyze user feedback from multiple surveys.
To leverage multiple data points, create a prioritization matrix that assigns ‘weight’ to customer feedback data and company priorities and then multiply them to reveal the highest-scoring option.
Let’s say you identify the top four responses to your churn survey . Rate the most common issue as a four and work down the list until one—these are your customer priorities. Then, rate the ease of fixing each problem with a maximum score of four for the easy wins down to one for difficult tasks—these are your company priorities. Finally, multiply the score of each customer priority with its coordinating company priority scores and lead with the highest scoring idea.
💡Pro-tip: use a product prioritization framework to make decisions.
Try a product prioritization framework when the pressure is on to make high-impact decisions with limited time and budget. These repeatable decision-making tools take the guesswork out of balancing goals, customer priorities, and team resources. Four popular frameworks are:
RICE: weighs four factors—reach, impact, confidence, and effort—to weigh initiatives differently
MoSCoW: considers stakeholder opinions on 'must-have', 'should-have', 'could-have', and 'won't-have' criteria
Kano: ranks ideas based on how likely they are to satisfy customer needs
Cost of delay analysis: determines potential revenue loss by not working on a product or initiative
Share what you learn with data visuals
Data visualization through charts and graphs gives you a new perspective on your results. Plus, removing the clutter of the analysis process helps you and stakeholders focus on the insight over the method.
Data visualization helps you:
Get buy-in with impactful charts that summarize your results
Increase customer empathy and awareness across your company with digestible insights
Use these four data visualization types to illustrate what you learned from your quantitative data analysis:
Bar charts reveal response distribution across multiple options
Line graphs compare data points over time
Scatter plots showcase how two variables interact
Matrices contrast data between categories like customer segments, product types, or traffic source
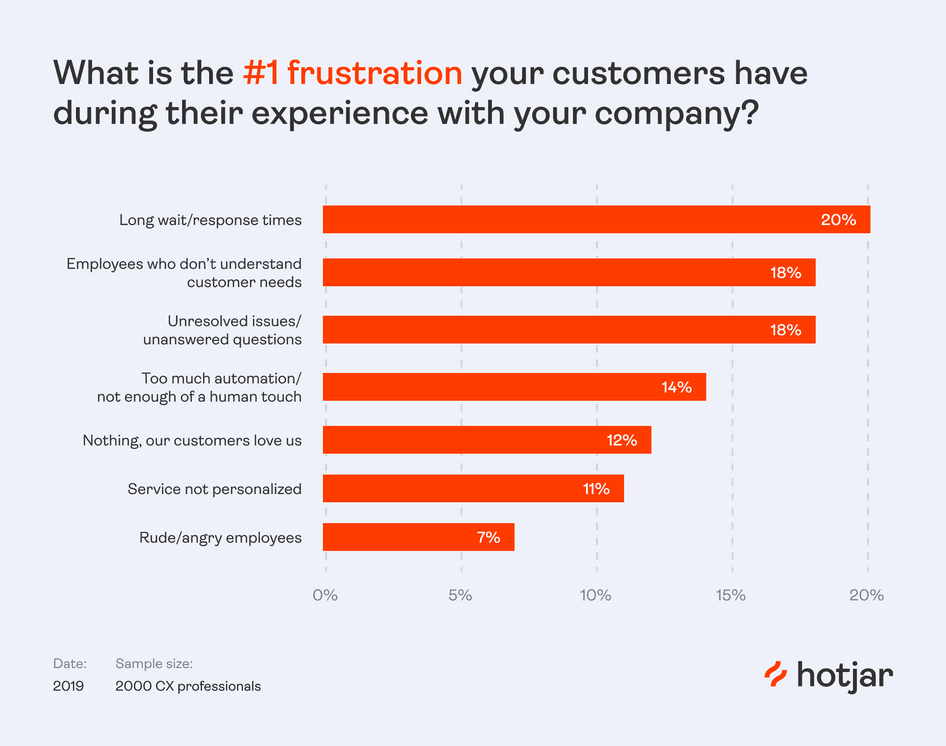
Use a variety of customer feedback types to get the whole picture
Quantitative data analysis pulls the story out of raw numbers—but you shouldn’t take a single result from your data collection and run with it. Instead, combine numbers-based quantitative data with descriptive qualitative research to learn the what, why, and how of customer experiences.
Looking at an opportunity from multiple angles helps you make more customer-centric decisions with less guesswork.
Stay close to customers with Hotjar
Hotjar’s tools offer quantitative and qualitative insights you can use to make customer-centric decisions, get buy-in, and highlight your team’s impact.
Frequently asked questions about quantitative data analysis
What is quantitative data.
Quantitative data is numeric feedback and information that you can count and measure. For example, you can calculate multiple-choice response rates, but you can’t tally a customer’s open-ended product feedback response. You have to use qualitative data analysis methods for non-numeric feedback.
What are quantitative data analysis methods?
Quantitative data analysis either summarizes or finds connections between numerical data feedback. Here are eight ways to analyze your online business’s quantitative data:
Compare multiple-choice response rates
Cross-tabulate to compare responses between groups
Measure the volume of response over time
Net Promoter Score
Weight customer feedback
How do you visualize quantitative data?
Data visualization makes it easier to spot trends and share your analysis with stakeholders. Bar charts, line graphs, scatter plots, and matrices are ways to visualize quantitative data.
What are the two types of statistical analysis for online businesses?
Quantitative data analysis is broken down into two analysis technique types:
Descriptive statistics summarize your collected data, like the number of website visitors this month
Inferential statistics compare relationships between multiple types of quantitative data, like survey responses between different customer segments
Quantitative data analysis process
Previous chapter
Quantitative data analysis software
Next chapter
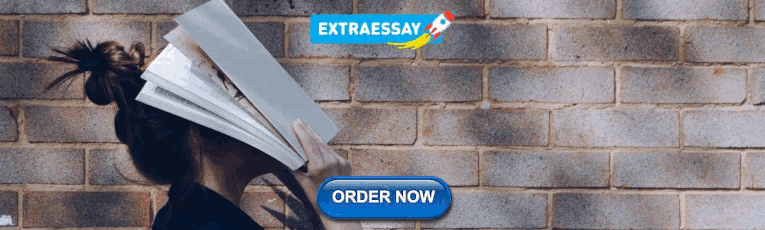
Data Analysis in Quantitative Research
- Reference work entry
- First Online: 13 January 2019
- Cite this reference work entry
- Yong Moon Jung 2
1753 Accesses
1 Citations
Quantitative data analysis serves as part of an essential process of evidence-making in health and social sciences. It is adopted for any types of research question and design whether it is descriptive, explanatory, or causal. However, compared with qualitative counterpart, quantitative data analysis has less flexibility. Conducting quantitative data analysis requires a prerequisite understanding of the statistical knowledge and skills. It also requires rigor in the choice of appropriate analysis model and the interpretation of the analysis outcomes. Basically, the choice of appropriate analysis techniques is determined by the type of research question and the nature of the data. In addition, different analysis techniques require different assumptions of data. This chapter provides introductory guides for readers to assist them with their informed decision-making in choosing the correct analysis models. To this end, it begins with discussion of the levels of measure: nominal, ordinal, and scale. Some commonly used analysis techniques in univariate, bivariate, and multivariate data analysis are presented for practical examples. Example analysis outcomes are produced by the use of SPSS (Statistical Package for Social Sciences).
This is a preview of subscription content, log in via an institution to check access.
Access this chapter
- Available as PDF
- Read on any device
- Instant download
- Own it forever
- Available as EPUB and PDF
- Durable hardcover edition
- Dispatched in 3 to 5 business days
- Free shipping worldwide - see info
Tax calculation will be finalised at checkout
Purchases are for personal use only
Institutional subscriptions
Armstrong JS. Significance tests harm progress in forecasting. Int J Forecast. 2007;23(2):321–7.
Article Google Scholar
Babbie E. The practice of social research. 14th ed. Belmont: Cengage Learning; 2016.
Google Scholar
Brockopp DY, Hastings-Tolsma MT. Fundamentals of nursing research. Boston: Jones & Bartlett; 2003.
Creswell JW. Research design: qualitative, quantitative, and mixed methods approaches. Thousand Oaks: Sage; 2014.
Fawcett J. The relationship of theory and research. Philadelphia: F. A. Davis; 1999.
Field A. Discovering statistics using IBM SPSS statistics. London: Sage; 2013.
Grove SK, Gray JR, Burns N. Understanding nursing research: building an evidence-based practice. 6th ed. St. Louis: Elsevier Saunders; 2015.
Hair JF, Black WC, Babin BJ, Anderson RE, Tatham RD. Multivariate data analysis. Upper Saddle River: Pearson Prentice Hall; 2006.
Katz MH. Multivariable analysis: a practical guide for clinicians. Cambridge: Cambridge University Press; 2006.
Book Google Scholar
McHugh ML. Scientific inquiry. J Specialists Pediatr Nurs. 2007; 8 (1):35–7. Volume 8, Issue 1, Version of Record online: 22 FEB 2007
Pallant J. SPSS survival manual: a step by step guide to data analysis using IBM SPSS. Sydney: Allen & Unwin; 2016.
Polit DF, Beck CT. Nursing research: principles and methods. Philadelphia: Lippincott Williams & Wilkins; 2004.
Trochim WMK, Donnelly JP. Research methods knowledge base. 3rd ed. Mason: Thomson Custom Publishing; 2007.
Tabachnick, B. G., & Fidell, L. S. (2013). Using multivariate statistics. Boston: Pearson Education.
Wells CS, Hin JM. Dealing with assumptions underlying statistical tests. Psychol Sch. 2007;44(5):495–502.
Download references
Author information
Authors and affiliations.
Centre for Business and Social Innovation, University of Technology Sydney, Ultimo, NSW, Australia
Yong Moon Jung
You can also search for this author in PubMed Google Scholar
Corresponding author
Correspondence to Yong Moon Jung .
Editor information
Editors and affiliations.
School of Science and Health, Western Sydney University, Penrith, NSW, Australia
Pranee Liamputtong
Rights and permissions
Reprints and permissions
Copyright information
© 2019 Springer Nature Singapore Pte Ltd.
About this entry
Cite this entry.
Jung, Y.M. (2019). Data Analysis in Quantitative Research. In: Liamputtong, P. (eds) Handbook of Research Methods in Health Social Sciences. Springer, Singapore. https://doi.org/10.1007/978-981-10-5251-4_109
Download citation
DOI : https://doi.org/10.1007/978-981-10-5251-4_109
Published : 13 January 2019
Publisher Name : Springer, Singapore
Print ISBN : 978-981-10-5250-7
Online ISBN : 978-981-10-5251-4
eBook Packages : Social Sciences Reference Module Humanities and Social Sciences Reference Module Business, Economics and Social Sciences
Share this entry
Anyone you share the following link with will be able to read this content:
Sorry, a shareable link is not currently available for this article.
Provided by the Springer Nature SharedIt content-sharing initiative
- Publish with us
Policies and ethics
- Find a journal
- Track your research
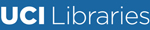
- Langson Library
- Science Library
- Grunigen Medical Library
- Law Library
- Connect From Off-Campus
- Accessibility
- Gateway Study Center
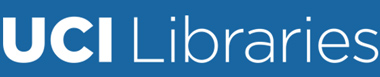
Email this link
Software for data analysis.
- Qualitative Data Analysis Software (Free)
- Python Libraries
- StoryMaps This link opens in a new window
- Other Helpful Tools - StatTransfer and OpenRefine
- DSS Programming Workshops
Software Comparison
*The primary interface is bolded in the case of multiple interface types available
- << Previous: Qualitative Data Analysis Software (Free)
- Next: Excel >>
- Last Updated: Feb 15, 2024 1:40 PM
- URL: https://guides.lib.uci.edu/dataanalysis
Off-campus? Please use the Software VPN and choose the group UCIFull to access licensed content. For more information, please Click here
Software VPN is not available for guests, so they may not have access to some content when connecting from off-campus.
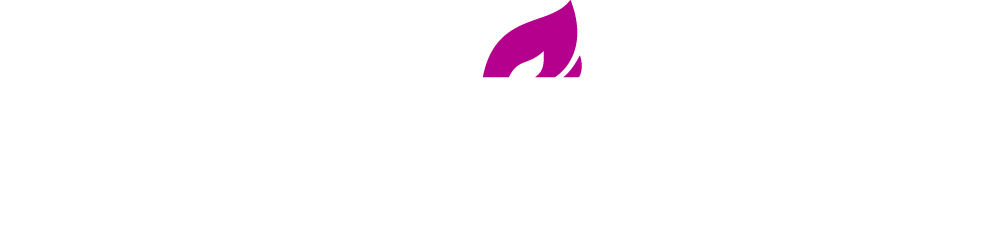
No products in the cart.
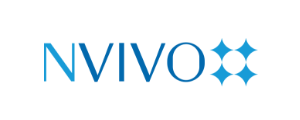
#1 QUALITATIVE DATA ANALYSIS SOFTWARE FOR 30 YEARS
Leading Qualitative Data Analysis Software with AI Solution
Enhance your use of nvivo 14.
Harness the Power of AI in NVivo
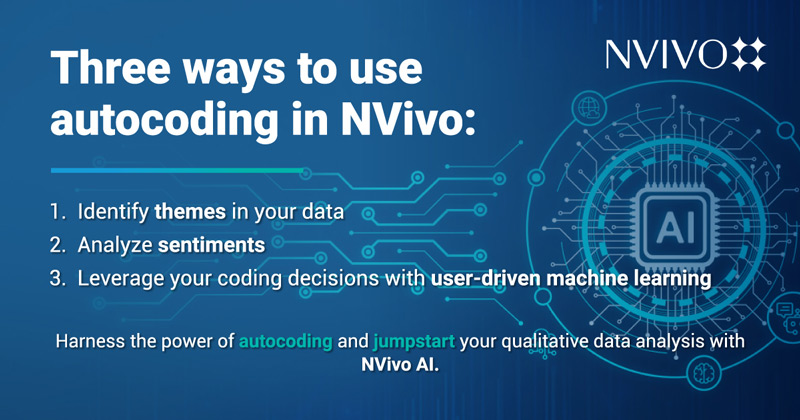
The NVivo Getting Started Bundle includes all the essentials you need for your research.
An NVivo license: The most cited and powerful software for qualitative data analysis . Choose a Windows or Mac individual license.
NVivo Core Skills Online Course: Includes videos, live coaching and a Q&A forum to get you up and running fast.
Access the entire bundle for just the normal price of NVivo. That’s a saving of $279.99 USD! Available for a limited time only, don’t miss out.
Click more with your research team, less with your mouse, collaborate, discover all the ways nvivo 14 works for you, enhance team research, boost productivity, collaborate easily, uncover richer insights, make robust conclusions, deliver comprehensive findings, enjoy a more streamlined user experience, looking to upgrade.
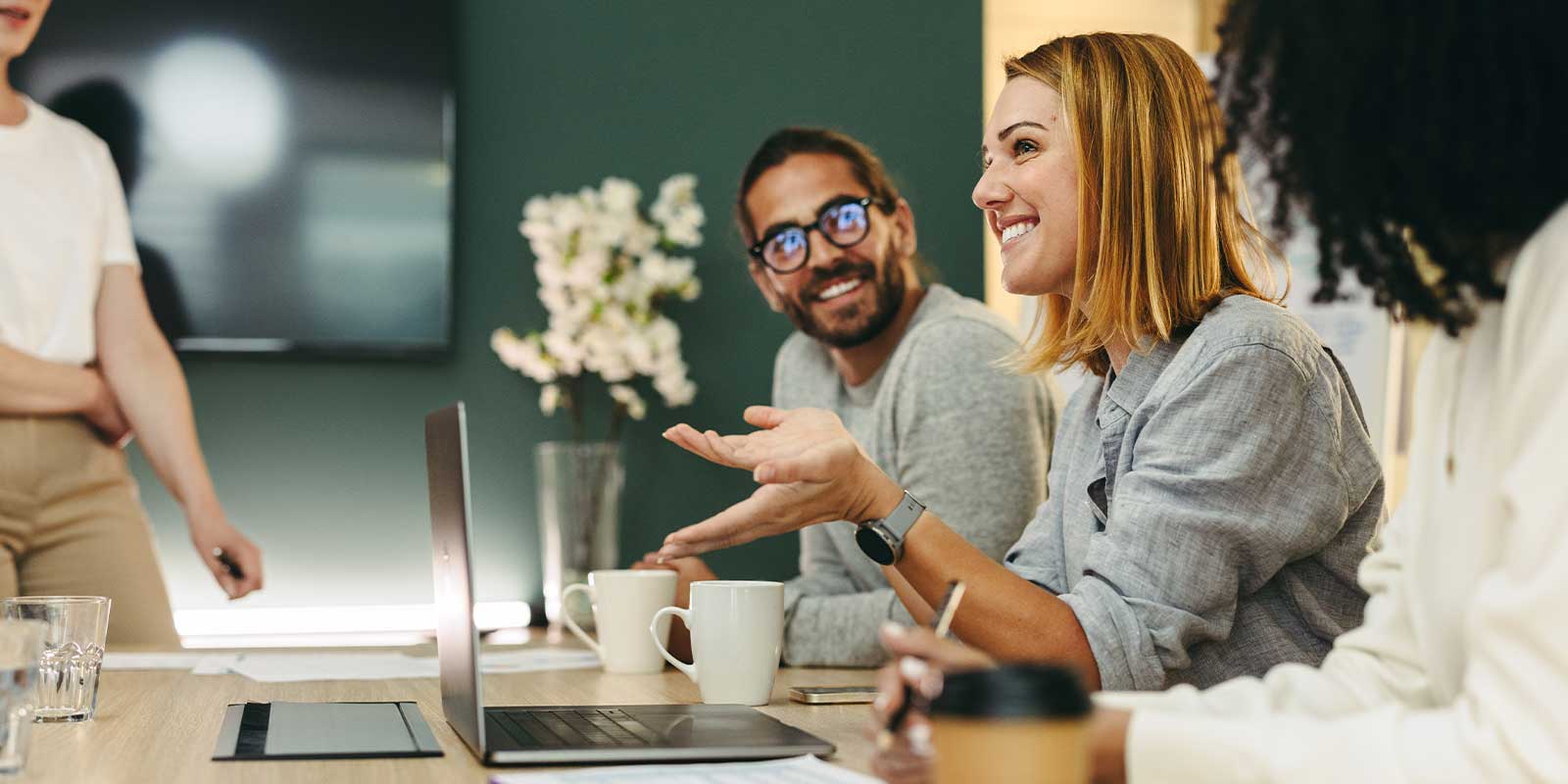
Collaboration Cloud
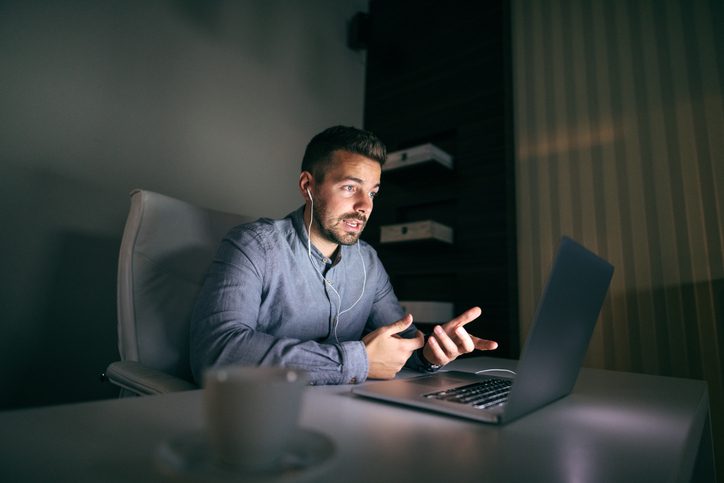
Collaboration Server
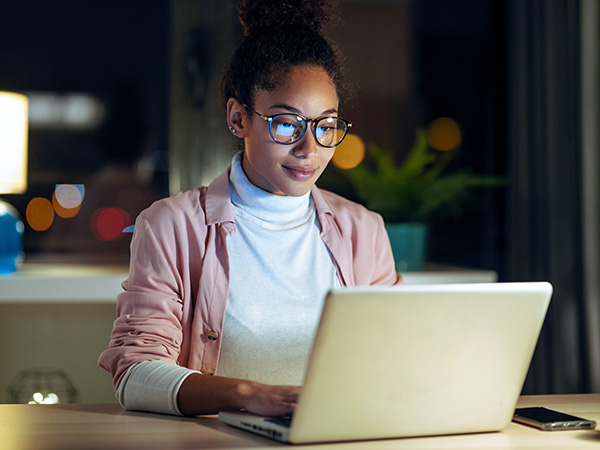
Transcription
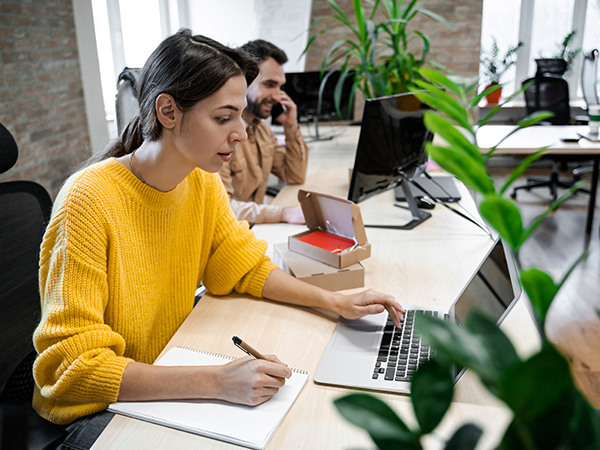
NVivo Academy
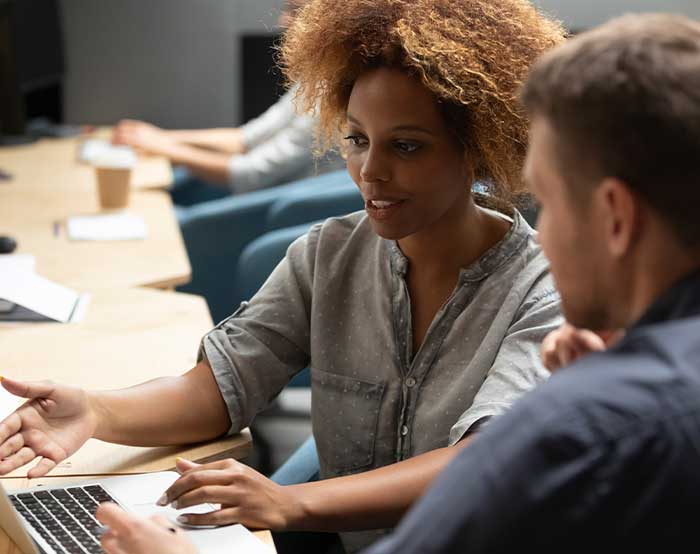
NVivo 14 Licenses
Student Licenses provide access to all the features of NVivo, limited for 12 months.
Individual and small group licenses (up to nine) can be bought online.
Organization licenses are available. If you want to purchase ten or more licenses, or enter an entreprise agreement, contact our sales team.
Enterprise Licensing: Better Research, Insights, and Outcomes for All
Lumivero’s team-based solutions allow you to:, need to know more about nvivo, what is nvivo, what can i do with nvivo, who is nvivo for, how much does nvivo cost.
It's easy to buy student, individual and small group licenses (student license limited to one per account, individual and small group licenses up to nine) online.
To purchase ten or more NVivo licenses for your team or organization, Contact Us to reach our sales team or one of our international NVivo partners.
How do I upgrade NVivo?
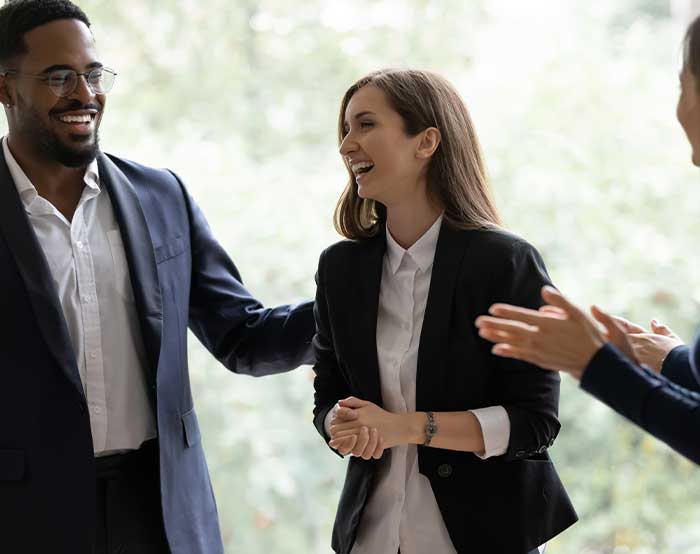
Get Started with NVivo Today
Begin your journey towards deeper insights and more robust results. NVivo provides better research collaboration, deeper integration, and is easier to use than ever.
Request a demo

- University of Oregon Libraries
- Research Guides
How to Choose Data Analysis Software
- Open Source Software
- Qualitative Analysis Software
- UO Available Software
- Data Resources
Librarian for Research Data Management and Reproducibility

Quantitative Analysis Software- Open Source
- R & R-Studio
Julia is an open source quantitative analysis software. Typical users include scientists, mathematicians, and engineers. Free download available .
Import and Export File Capabilities
Import: Text files (.csv, .tsv, .wsv, .txt)
Export: Text files (.csv, .dat)

OpenRefine (previously known as Google Refine) is an open source pre-analysis software, built for cleaning and transforming messy data. Typical users include the social sciences, humanities, and profit/nonprofit corporations. Free download available .
Import: Excel files (.xls, .xlsx), Text files (.csv, .tsv), Web-based files (.xml, .html, .rdf) & additional formats (.json, .tar, .tar gz, Google Spreadsheets, Google Fusion Tables)
Export: Excel files (.xls, .xlsx), Text files (.csv, tsv), Web-based files (.html) & additional formats (.json, .tar, .tar gz)
View this OpenRefine video tutorial for more information on its use as a mix-methods evaluation and analysis tool.
Python is an open source quantitative analysis software for both first-time and experienced coders. Typical users include the social sciences, arts, engineers, government agencies, academia, and profit/nonprofit corporations. Free download available .
Import and Export File Capability
Import: Excel files (.xls, .xlsx), Text files (.txt, .csv) & additional formats (.sql, HDF5)
Export: Excel files (.xls, .xlsx), Text files ).txt, .csv) & additional formats (HDF5)
View this Python video tutorial for first-time Python users to learn the basics of the software.
R & R-Studio
R and R-Studio are open source quantitative analysis software specifically for network and text analysis, data mining, and web scraping. Typical users include scientists, economists, bioinformatics, sociologists, and marketing researchers. Free download available for R and R-Studio . For a walk through on how to install both programs, see this How-To Guide .
Import and Export File Capabilities
Import: Excel files (.xls, .xlsx), Text files (.txt, .dat, .csv), Web-based files (.xml) & additional formats (.sav, .dta, .sas7bdat, .json)
Export: Excel files (.xlsx), Text files (.txt, .csv) & additional formats (.sav, .dta, .json)
- Quarto Cheat Sheet
Tableau Public
Tableau Public is an proprietary quantitative analysis software with high graphics and an intuitive user interface. Typical users include profit/nonprofit corporations. Free download available .
Import: Excel files (.xls, .xlsx), Text files (.txt, .csv) & Access files (.mdb, .accdb)
Export: Text files (.pdf) & Web-based files (embedded web links)
View this Tableau Public video tutorial for a basic training overview of Tableau's visual analytic techniques.
WEKA is an open sources quantitative analysis software developed in New Zealand. Typical users include academia and profit/nonprofit corporations. Free download available .
Import: Text files (.txt, .csv), Web-based files & additional formats (.arff, .libsvm)
View this WEKA video tutorial for first-time users, including how to get started.
Quantitative Analysis Resources
- << Previous: UO Available Software
- Next: Data Resources >>
- Last Updated: Apr 5, 2024 1:24 PM
- URL: https://researchguides.uoregon.edu/How-to-Choose-Data-Analysis-Software
Contact Us Library Accessibility UO Libraries Privacy Notices and Procedures
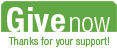
1501 Kincaid Street Eugene, OR 97403 P: 541-346-3053 F: 541-346-3485
- Visit us on Facebook
- Visit us on Twitter
- Visit us on Youtube
- Visit us on Instagram
- Report a Concern
- Nondiscrimination and Title IX
- Accessibility
- Privacy Policy
- Find People

*RESEARCH DATA SERVICES (RDS) @ Georgia State University Library: WORKSHOPs ~ Data Analysis Tools ~ Quantitative (Stata, SPSS, SAS, R, Python) & Qualitative (NVivo)
- RDS@GSU ~ iCollege/Syllabus Language
- RDS@GSU ~ Scope of Services
- WORKSHOPs ~ Calendar (Live - Online or In-Person) This link opens in a new window
- WORKSHOPs ~ Recordings
- WORKSHOPs ~ Data Analysis Tools ~ Quantitative (Stata, SPSS, SAS, R, Python) & Qualitative (NVivo)
- WORKSHOPs ~ Methods for Data Analysis & Collection ~ Survey Design, Qualitative Methods, Data Discovery
- WORKSHOPs ~ Mapping & Data Visualization (Tableau, ArcGIS, Power BI, Social Explorer)
- WORKSHOPs ~ Etiquette & Policies
- WORKSHOPs ~ College-To-Career Competencies & Skills
- GSU Data Ready! Badges
- TEACHING with Data & Statistics
- Data Events This link opens in a new window
- PIDLit Public Interest Data Literacy This link opens in a new window
- CAREERS in Data Services
WORKSHOPs ~ DATA ANALYSIS TOOLS
Coding: r | python | python for machine learning | text data analysis w/ r & python, software: spss | sas | stata | nvivo | nvivo for team coding , r workshop series.
Get GSU Data Ready! Badge Micro-Credentials for completing these workshops to show others your commitment to learning data skills! Learn more at lib.gsu.edu/data-read y

- LIVE workshops currently not offered.
- For RECORDED workshops: Click here .
- For ONLINE GUIDE: Click here .
The R workshop series will introduce participants to the fundamentals of using the R programming language and associated tools for the purposes of performing common data analysis tasks. The R programming language is 100% free to use and is extremely popular amongst researchers in both academia, business, and non-profits. It is especially useful for conducting statistical analysis.
This series consists of four workshops. For individuals who are new to R, coding, or data analysis, it is highly recommended that the workshops be attended in sequential order. Additionally, while these workshops are taught exclusively using code (i.e. there are no point-and-click methods), attendees do not need to have any prior experience with programming, coding, or scripting. All are welcome.
Skill Requirements: None.
Software Requirements for Hands-on Participation:
For participants wishing to follow along with the “hands-on” portion of the workshop, please see the directions at the following url: https://research.library.gsu.edu/R/workshop
R 1: Getting Started with R and RStudio
Workshop Topics:
- Using RStudio to work with R
- R syntax, commands, functions, and packages
- Opening, viewing, and exploring data
- Generating basic descriptive statistics from data
R 2: Tidyverse and Manipulating Data
- Introduction to Tidyverse packages (emphasis on dplyr)
- Transforming and generating variables
- Handling data with missing values
- “Piping” data and data processes
R 3: Data Visualization and Mapping
- Creating statistical plots using ggplot2
- Customizing plot colors, themes, labels, etc…
- Working with GIS data to create maps
- Modifying maps with overlays, custom aesthetics, and additional data
R 4: Statistical Modelling
- Basic analysis, descriptive statistics, t-tests
- Creating linear models (multiple linear regression & logistic regression)
- Evaluating linear models and generating predictions
- Creating simple machine learning models (Time permitting)
Python & Data Workshop Series
Get a GSU Data Ready! Badge Micro-Credential for completing these workshops to show others your commitment to learning data skills! Learn more at lib.gsu.edu/data-read y

The Python & Data workshop series will introduce participants to the fundamentals of using the Python programming language and associated tools for the purposes of performing common data analysis tasks. Python is an extremely popular programming language used by analysts, researchers, and scientists in many different disciplines.
This series consists of three workshops. For individuals who are new to Python, coding, or data analysis, it is highly recommended that the workshops be attended in sequential order. Additionally, while these workshops are taught exclusively using code (i.e. there are no point-and-click methods), attendees do not need to have any prior experience with programming, coding, or scripting. All are welcome.
- Participants will need a Google / Gmail account in order to access Google Colab
- No software installation is required.
Python & Data 0: Google Colab
This video-only recorded workshop provides a short, high-level overview of Google Colab and how it relates to the other Python workshops. There is no live version of this workshop; it is solely available as a recorded version on our recorded workshops page linked above.
- Brief overview of Google Colab
- Uploading, managing, and saving data in Google Colab environment
Python & Data 1: Getting Started with Python
- Using Google Colab and Jupyter to work with Python
- Python syntax, commands, functions, and packages/modules
Python & Data 2: Manipulating & Transforming Data
- Selecting, sub-setting, and manipulating data
- Generating crosstabs / contingency tables
Python & Data 3: Visualizing Data & Creating Models
- Plotting and visualizing data using Matplotlib and Seaborn
- Defining statistical models using both formulas and matrices
- Fitting and inspecting statistical models (e.g. anova, linear regression)
Python for Machine Learning (ML) Workshop Series
This applied Machine Learning (ML) series introduces participants to the fundamentals of supervised learning and provides experience in applying several ML algorithms in Python. Participants will gain experience in regression modeling; assessing model adequacy, prediction precision, and computational performance; and learn several tools for visualizing each step of the process.
This series consists of three (3) workshops. For individuals who are new to Python and/or Google Colab, it is highly recommended that you first complete the prerequisite Python & Data Workshop Series 0-3 workshops . For those who are new to Machine Learning, it is highly recommended that the workshops in this series be attended in sequential order. While these workshops are taught exclusively using code (i.e., there are no point-and-click methods), attendees do not need to have any prior experience with programming, coding, or scripting. All are welcome.
For participants wishing to follow along with the “hands-on” portion of the workshop, please see the directions here .
Python for Machine Learning (ML) 1: Univariate Linear Regression
Fundamentals of supervised learning in Python; applying a rudimentary ML model using univariate linear regression (i.e., one feature).
- Overview: “What is Machine Learning?”
- Univariate Linear Regression Model
- Mean-Squared Error Cost Function
- Gradient Descent Algorithm for Linear Regression
Prerequisites: Python & Data Workshop Series 0-3: https://lib.gsu.edu/rds-recordings
Python for Machine Learning (ML) 2: Multivariate Linear Regression
Fundamentals of supervised learning in Python; applying an ML model using multivariate regression (i.e., multiple features).
- Multivariate Regression Model
- Vectorization
- Feature Scaling
- Feature Engineering
Prerequisites: Python & Data Workshop Series 0-3: https://lib.gsu.edu/rds-recordings and Python for Machine Learning (ML) 1: Univariate Linear Regression Workshop.
Python for Machine Learning (ML) 3: Logistic Regression
Fundamentals of supervised learning in Python; applying an ML model using logistic regression (e.g., classification prediction).
- Logistic Regression Model
- Cost Function for Logistic Regression
- Gradient Descent for Logistic Regression
- Overfitting & Model Adequacy
Prerequisites: Python & Data Workshop Series 0-3: https://lib.gsu.edu/rds-recordings , Python for Machine Learning (ML) 1: Univariate Linear Regression Workshop, and Python for Machine Learning (ML) 2: Multivariate Linear Regression Workshop.
Text Data Analysis Workshop

Text Data: Basics of Text Processing and Regular Expressions
The size and volume of textual data available to academic researchers is absolutely immense. Consequently, for some researchers, having the skills to process, transform, and analyze text data using computational tools is increasingly necessary for certain types of research. This workshop will introduce the fundamentals of working with and manipulating text data using scripting languages (e.g. Python, R). This includes loading, processing, and preparing text data for use with quantitative models. Although advanced natural language processing (NLP) models are not included in this workshop, some possible applications may be demonstrated if time permits. No special background knowledge or skills are required to attend. All are welcome.
Skill Requirements: Basic familiarity with Python, R, or any scripting language preferred.
- Common text and string operations
- Tokenization, transformations, and processing
- Regular Expressions
- N-grams and term frequencies
SPSS Workshop Series
Spss workshop series.

- For LIVE workshops dates/times: Click here .
The SPSS 1 and SPSS 2 workshops in this two-part series focus on using the point-and-click method for using SPSS; the syntax/code method is introduced briefly.
SPSS 1: Getting Started
This workshop is the first of a two-part series on SPSS , a statistical software package that is widely used by scientists throughout the social sciences for analysis of quantitative data.
Please note: This workshop focuses on using the point-and-click method for using SPSS; the syntax/code method is introduced briefly.
Workshop Topics
- Navigating SPSS
- Entering and importing data from different formats (such as text and Excel files)
- Defining variables (defining and labeling codes, selecting appropriate levels of measurement)
- Manipulating and transforming data (selecting cases and splitting files; recoding and computing variables)
- Running descriptive statistics
- Generating simple graphs
Prerequisites: None.
SPSS 2: Analyzing Data
This workshop is the second of a two-part series on SPSS , a statistical software package that is widely used by scientists throughout the social sciences for analysis of quantitative data.
- Cross-tabulation and Chi-Square tests
- Analysis of Variance (ANOVA)
- Correlation analysis
- Multiple regression analysis
Prerequisites: Attendance at SPSS 1 preferred, or completion of Parts 1-6 of the Lynda.com "SPSS Statistics Essential Training" tutorial .
SAS Workshop Series
Sas workshop series.

This workshop series completes all analysis using code. No previous knowledge of coding is required. This series is for the Windows version of SAS.
SAS 1: SAS Basics
This is the first SAS workshop in a two-part series. This interactive workshop will introduce users to the SAS system. Applied, hands on, examples using real data will be used. NOTE: This workshop is aimed at people who do not have experience using the SAS system. Those who have used SAS in the past may find this workshop too foundational and are encouraged to attend our forthcoming advanced SAS sessions.
- Reading data into SAS
- Conducting basic data cleaning and recoding
- Using basic SAS procedures (e.g., PROC CONTENTS, PROC PRINT, PROC FREQ) to view and understand data.
- PROC FREQ, PROC UNIVARIATE, and PROC MEANS will be demonstrated to complete basic descriptive statistics.
- An introduction to bivariate statistics in SAS.
Prerequisites: No prior experience with SAS is required. Basic understanding of univariate and bivariate statistics is helpful but not required.
SAS 2: Data Analysis
This is the second SAS workshop in a two-part series. In this interactive workshop, SAS users will go beyond the basics to develop comfort with more advanced statistical analyses using the SAS system. Applied, hands on, examples using real data will be used. NOTE: Basic knowledge of the SAS system will be helpful for those who want to participate in the applied portion of the workshop.
- Conducting bivariate and multivariable analyses using SAS procedures like PROC FREQ, PROC TTEST, PROC ANOVA, PROC GLM, PROC LOGISTIC, and PROC REG.
- Best practices for checking statistical assumptions, selecting appropriate statistical procedures, and reporting and visualizing results will be discussed.
Prerequisites: Basic knowledge of the SAS system will be helpful for those who want to participate in the applied portion of the workshop. Basic understanding of bivariate and multivariable statistics is helpful.
Stata Workshop Series
Stata workshop series.

- For RECORDED workshops: Click here .
This workshop series completes all analysis using code. No previous knowledge of coding is required. This series is for the Windows version of Stata. See the Stata research guide here.
Stata 1: Introduction to Stata
This workshop is the first of a three-part series on Stata . Stata is a statistical software package. Stata is widely used by scientists throughout the social sciences for analysis of quantitative data ranging from simple descriptive analysis to complex statistical modeling.
Please note: This workshop completes all analysis using code. No previous knowledge of coding is required.
- Opening data
- Generating variables (basic)
- Frequency distributions
- Analysis: summary statistics
Prerequisites: None.
Stata 2: Basic Data Analysis
This workshop is the second in a three-part series on Stata . Stata is a statistical software package. Stata is widely used by scientists throughout the social sciences for analysis of quantitative data ranging from simple descriptive analysis to complex statistical modeling.
- Generating variables (advanced)
- Analysis: Chi-square, ANOVA, regression
- Navigating help features
Prerequisites: Stata 1 or basic knowledge of Stata.
Stata 3: Advanced Data Analysis
This workshop is the third in a three-part series on Stata . Stata is a statistical software package. Stata is widely used by scientists throughout the social sciences for analysis of quantitative data ranging from simple descriptive analysis to complex statistical modeling.
- Troubleshooting code
- Generating scales
- 3, 4, and 5 way cross tabulations
Prerequisites: Stata 1 and Stata 2 or moderate knowledge of Stata.
NVivo Workshop Series
Nvivo workshop series.

This workshop is for the *WINDOWS* version of NVivo. The Mac version differs significantly from the Windows; consequently, attending the Windows workshop if you will be using the Mac version is not recommended. We do not offer workshops on the Mac version due to having no Mac labs to do so. If you need one-on-one training for the Mac version of NVivo, please directly contact Mandy Swygart-Hobaugh, Ph.D. See the NVivo research guide here.
NVivo 1 for Windows: Getting Started
This is the first workshop in a two-part series on NVivo qualitative data analysis software.
- Getting to know the NVivo workspace
- Exploring different types of data types/files that can be analyzed
- Basic coding of text-based files
- Using Queries and Automated features to explore and code your data
- Recording comments and ideas
Prerequisites: Basic understanding of qualitative research methods is suggested, but not required. Watching this short tutorial on coding qualitative data before attending the workshop is recommended.
NVivo 2 for Windows: Exploring Your Data
This is the second workshop in a two-part series on NVivo qualitative data analysis software.
- Creating Classifications with Attribute Values to facilitate comparative analyses
- Crosstab and Matrix Coding queries
- Data visualizations
- Sharing findings with Reports and exporting Codebooks
Prerequisites: NVivo 1
NVivo for Team Coding Workshop
Nvivo for team coding.
Get a GSU Data Ready! Badge Micro-Credential for completing this workshop plus the NVivo 1 workshop to show others your commitment to learning data skills! Learn more at lib.gsu.edu/data-read y
This workshop is on using NVivo qualitative data analysis software to do team work / team coding in NVivo involving 2 or more people . It will cover various strategies for tracking team members’ work in NVivo and comparing coding, including generating inter-rater reliability measures.
- Creating and organizing NVivo project files for independent coding
- Merging / importing individual team member's project files into a master copy file.
- Comparing coding between team members, including generating inter-rater reliability measures
- Challenges of having team members working across Windows and Mac versions
Prerequisites: Attendance at minimally the NVivo 1 workshop (live or recorded) and optionally the NVivo 2 workshop (live or recorded).
- << Previous: WORKSHOPs ~ Recordings
- Next: WORKSHOPs ~ Methods for Data Analysis & Collection ~ Survey Design, Qualitative Methods, Data Discovery >>
- Last Updated: Apr 24, 2024 4:40 PM
- URL: https://research.library.gsu.edu/dataservices

- Privacy Policy

Home » Quantitative Data – Types, Methods and Examples
Quantitative Data – Types, Methods and Examples
Table of Contents

Quantitative Data
Definition:
Quantitative data refers to numerical data that can be measured or counted. This type of data is often used in scientific research and is typically collected through methods such as surveys, experiments, and statistical analysis.
Quantitative Data Types
There are two main types of quantitative data: discrete and continuous.
- Discrete data: Discrete data refers to numerical values that can only take on specific, distinct values. This type of data is typically represented as whole numbers and cannot be broken down into smaller units. Examples of discrete data include the number of students in a class, the number of cars in a parking lot, and the number of children in a family.
- Continuous data: Continuous data refers to numerical values that can take on any value within a certain range or interval. This type of data is typically represented as decimal or fractional values and can be broken down into smaller units. Examples of continuous data include measurements of height, weight, temperature, and time.
Quantitative Data Collection Methods
There are several common methods for collecting quantitative data. Some of these methods include:
- Surveys : Surveys involve asking a set of standardized questions to a large number of people. Surveys can be conducted in person, over the phone, via email or online, and can be used to collect data on a wide range of topics.
- Experiments : Experiments involve manipulating one or more variables and observing the effects on a specific outcome. Experiments can be conducted in a controlled laboratory setting or in the real world.
- Observational studies : Observational studies involve observing and collecting data on a specific phenomenon without intervening or manipulating any variables. Observational studies can be conducted in a natural setting or in a laboratory.
- Secondary data analysis : Secondary data analysis involves using existing data that was collected for a different purpose to answer a new research question. This method can be cost-effective and efficient, but it is important to ensure that the data is appropriate for the research question being studied.
- Physiological measures: Physiological measures involve collecting data on biological or physiological processes, such as heart rate, blood pressure, or brain activity.
- Computerized tracking: Computerized tracking involves collecting data automatically from electronic sources, such as social media, online purchases, or website analytics.
Quantitative Data Analysis Methods
There are several methods for analyzing quantitative data, including:
- Descriptive statistics: Descriptive statistics are used to summarize and describe the basic features of the data, such as the mean, median, mode, standard deviation, and range.
- Inferential statistics : Inferential statistics are used to make generalizations about a population based on a sample of data. These methods include hypothesis testing, confidence intervals, and regression analysis.
- Data visualization: Data visualization involves creating charts, graphs, and other visual representations of the data to help identify patterns and trends. Common types of data visualization include histograms, scatterplots, and bar charts.
- Time series analysis: Time series analysis involves analyzing data that is collected over time to identify patterns and trends in the data.
- Multivariate analysis : Multivariate analysis involves analyzing data with multiple variables to identify relationships between the variables.
- Factor analysis : Factor analysis involves identifying underlying factors or dimensions that explain the variation in the data.
- Cluster analysis: Cluster analysis involves identifying groups or clusters of observations that are similar to each other based on multiple variables.
Quantitative Data Formats
Quantitative data can be represented in different formats, depending on the nature of the data and the purpose of the analysis. Here are some common formats:
- Tables : Tables are a common way to present quantitative data, particularly when the data involves multiple variables. Tables can be used to show the frequency or percentage of data in different categories or to display summary statistics.
- Charts and graphs: Charts and graphs are useful for visualizing quantitative data and can be used to highlight patterns and trends in the data. Some common types of charts and graphs include line charts, bar charts, scatterplots, and pie charts.
- Databases : Quantitative data can be stored in databases, which allow for easy sorting, filtering, and analysis of large amounts of data.
- Spreadsheets : Spreadsheets can be used to organize and analyze quantitative data, particularly when the data is relatively small in size. Spreadsheets allow for calculations and data manipulation, as well as the creation of charts and graphs.
- Statistical software : Statistical software, such as SPSS, R, and SAS, can be used to analyze quantitative data. These programs allow for more advanced statistical analyses and data modeling, as well as the creation of charts and graphs.
Quantitative Data Gathering Guide
Here is a basic guide for gathering quantitative data:
- Define the research question: The first step in gathering quantitative data is to clearly define the research question. This will help determine the type of data to be collected, the sample size, and the methods of data analysis.
- Choose the data collection method: Select the appropriate method for collecting data based on the research question and available resources. This could include surveys, experiments, observational studies, or other methods.
- Determine the sample size: Determine the appropriate sample size for the research question. This will depend on the level of precision needed and the variability of the population being studied.
- Develop the data collection instrument: Develop a questionnaire or survey instrument that will be used to collect the data. The instrument should be designed to gather the specific information needed to answer the research question.
- Pilot test the data collection instrument : Before collecting data from the entire sample, pilot test the instrument on a small group to identify any potential problems or issues.
- Collect the data: Collect the data from the selected sample using the chosen data collection method.
- Clean and organize the data : Organize the data into a format that can be easily analyzed. This may involve checking for missing data, outliers, or errors.
- Analyze the data: Analyze the data using appropriate statistical methods. This may involve descriptive statistics, inferential statistics, or other types of analysis.
- Interpret the results: Interpret the results of the analysis in the context of the research question. Identify any patterns, trends, or relationships in the data and draw conclusions based on the findings.
- Communicate the findings: Communicate the findings of the analysis in a clear and concise manner, using appropriate tables, graphs, and other visual aids as necessary. The results should be presented in a way that is accessible to the intended audience.
Examples of Quantitative Data
Here are some examples of quantitative data:
- Height of a person (measured in inches or centimeters)
- Weight of a person (measured in pounds or kilograms)
- Temperature (measured in Fahrenheit or Celsius)
- Age of a person (measured in years)
- Number of cars sold in a month
- Amount of rainfall in a specific area (measured in inches or millimeters)
- Number of hours worked in a week
- GPA (grade point average) of a student
- Sales figures for a product
- Time taken to complete a task.
- Distance traveled (measured in miles or kilometers)
- Speed of an object (measured in miles per hour or kilometers per hour)
- Number of people attending an event
- Price of a product (measured in dollars or other currency)
- Blood pressure (measured in millimeters of mercury)
- Amount of sugar in a food item (measured in grams)
- Test scores (measured on a numerical scale)
- Number of website visitors per day
- Stock prices (measured in dollars)
- Crime rates (measured by the number of crimes per 100,000 people)
Applications of Quantitative Data
Quantitative data has a wide range of applications across various fields, including:
- Scientific research: Quantitative data is used extensively in scientific research to test hypotheses and draw conclusions. For example, in biology, researchers might use quantitative data to measure the growth rate of cells or the effectiveness of a drug treatment.
- Business and economics: Quantitative data is used to analyze business and economic trends, forecast future performance, and make data-driven decisions. For example, a company might use quantitative data to analyze sales figures and customer demographics to determine which products are most popular among which segments of their customer base.
- Education: Quantitative data is used in education to measure student performance, evaluate teaching methods, and identify areas where improvement is needed. For example, a teacher might use quantitative data to track the progress of their students over the course of a semester and adjust their teaching methods accordingly.
- Public policy: Quantitative data is used in public policy to evaluate the effectiveness of policies and programs, identify areas where improvement is needed, and develop evidence-based solutions. For example, a government agency might use quantitative data to evaluate the impact of a social welfare program on poverty rates.
- Healthcare : Quantitative data is used in healthcare to evaluate the effectiveness of medical treatments, track the spread of diseases, and identify risk factors for various health conditions. For example, a doctor might use quantitative data to monitor the blood pressure levels of their patients over time and adjust their treatment plan accordingly.
Purpose of Quantitative Data
The purpose of quantitative data is to provide a numerical representation of a phenomenon or observation. Quantitative data is used to measure and describe the characteristics of a population or sample, and to test hypotheses and draw conclusions based on statistical analysis. Some of the key purposes of quantitative data include:
- Measuring and describing : Quantitative data is used to measure and describe the characteristics of a population or sample, such as age, income, or education level. This allows researchers to better understand the population they are studying.
- Testing hypotheses: Quantitative data is often used to test hypotheses and theories by collecting numerical data and analyzing it using statistical methods. This can help researchers determine whether there is a statistically significant relationship between variables or whether there is support for a particular theory.
- Making predictions : Quantitative data can be used to make predictions about future events or trends based on past data. This is often done through statistical modeling or time series analysis.
- Evaluating programs and policies: Quantitative data is often used to evaluate the effectiveness of programs and policies. This can help policymakers and program managers identify areas where improvements can be made and make evidence-based decisions about future programs and policies.
When to use Quantitative Data
Quantitative data is appropriate to use when you want to collect and analyze numerical data that can be measured and analyzed using statistical methods. Here are some situations where quantitative data is typically used:
- When you want to measure a characteristic or behavior : If you want to measure something like the height or weight of a population or the number of people who smoke, you would use quantitative data to collect this information.
- When you want to compare groups: If you want to compare two or more groups, such as comparing the effectiveness of two different medical treatments, you would use quantitative data to collect and analyze the data.
- When you want to test a hypothesis : If you have a hypothesis or theory that you want to test, you would use quantitative data to collect data that can be analyzed statistically to determine whether your hypothesis is supported by the data.
- When you want to make predictions: If you want to make predictions about future trends or events, such as predicting sales for a new product, you would use quantitative data to collect and analyze data from past trends to make your prediction.
- When you want to evaluate a program or policy : If you want to evaluate the effectiveness of a program or policy, you would use quantitative data to collect data about the program or policy and analyze it statistically to determine whether it has had the intended effect.
Characteristics of Quantitative Data
Quantitative data is characterized by several key features, including:
- Numerical values : Quantitative data consists of numerical values that can be measured and counted. These values are often expressed in terms of units, such as dollars, centimeters, or kilograms.
- Continuous or discrete : Quantitative data can be either continuous or discrete. Continuous data can take on any value within a certain range, while discrete data can only take on certain values.
- Objective: Quantitative data is objective, meaning that it is not influenced by personal biases or opinions. It is based on empirical evidence that can be measured and analyzed using statistical methods.
- Large sample size: Quantitative data is often collected from a large sample size in order to ensure that the results are statistically significant and representative of the population being studied.
- Statistical analysis: Quantitative data is typically analyzed using statistical methods to determine patterns, relationships, and other characteristics of the data. This allows researchers to make more objective conclusions based on empirical evidence.
- Precision : Quantitative data is often very precise, with measurements taken to multiple decimal points or significant figures. This precision allows for more accurate analysis and interpretation of the data.
Advantages of Quantitative Data
Some advantages of quantitative data are:
- Objectivity : Quantitative data is usually objective because it is based on measurable and observable variables. This means that different people who collect the same data will generally get the same results.
- Precision : Quantitative data provides precise measurements of variables. This means that it is easier to make comparisons and draw conclusions from quantitative data.
- Replicability : Since quantitative data is based on objective measurements, it is often easier to replicate research studies using the same or similar data.
- Generalizability : Quantitative data allows researchers to generalize findings to a larger population. This is because quantitative data is often collected using random sampling methods, which help to ensure that the data is representative of the population being studied.
- Statistical analysis : Quantitative data can be analyzed using statistical methods, which allows researchers to test hypotheses and draw conclusions about the relationships between variables.
- Efficiency : Quantitative data can often be collected quickly and efficiently using surveys or other standardized instruments, which makes it a cost-effective way to gather large amounts of data.
Limitations of Quantitative Data
Some Limitations of Quantitative Data are as follows:
- Limited context: Quantitative data does not provide information about the context in which the data was collected. This can make it difficult to understand the meaning behind the numbers.
- Limited depth: Quantitative data is often limited to predetermined variables and questions, which may not capture the complexity of the phenomenon being studied.
- Difficulty in capturing qualitative aspects: Quantitative data is unable to capture the subjective experiences and qualitative aspects of human behavior, such as emotions, attitudes, and motivations.
- Possibility of bias: The collection and interpretation of quantitative data can be influenced by biases, such as sampling bias, measurement bias, or researcher bias.
- Simplification of complex phenomena: Quantitative data may oversimplify complex phenomena by reducing them to numerical measurements and statistical analyses.
- Lack of flexibility: Quantitative data collection methods may not allow for changes or adaptations in the research process, which can limit the ability to respond to unexpected findings or new insights.
About the author
Muhammad Hassan
Researcher, Academic Writer, Web developer
You may also like

Primary Data – Types, Methods and Examples

Qualitative Data – Types, Methods and Examples

Research Data – Types Methods and Examples

Secondary Data – Types, Methods and Examples

Information in Research – Types and Examples
TechRepublic
Account information.
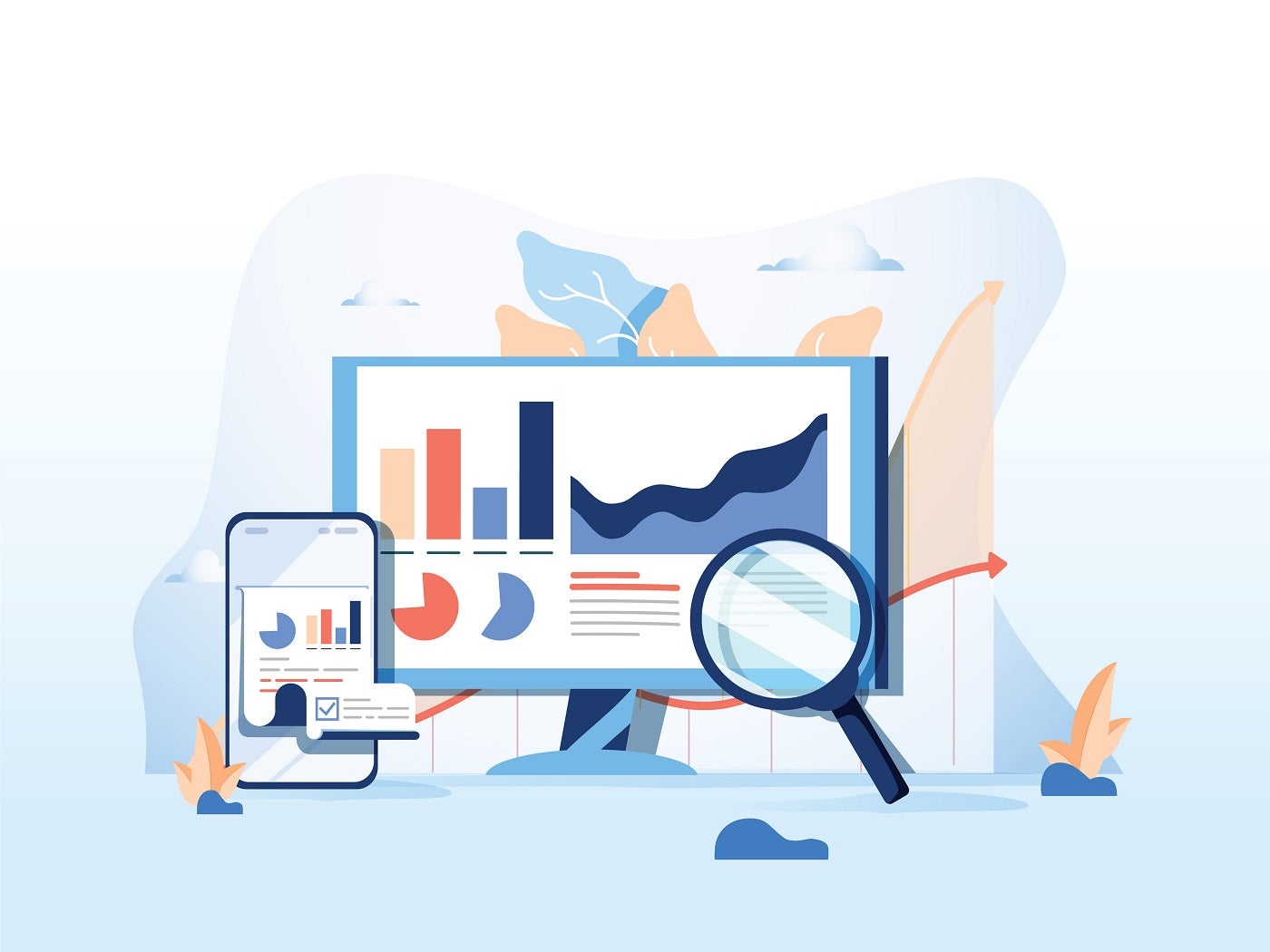
Share with Your Friends
10 Best Reporting Tools and Software of 2024
Your email has been sent
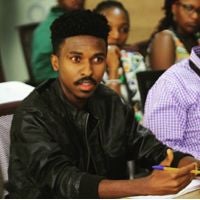
- Best for comprehensive data integration: Zoho Analytics
- Best for task-based reporting: Asana
- Best for high-level project reporting: Hive
- Best for data-driven decision-making: Google Looker
- Best for customizable project reporting: Wrike
- Best for visual project tracking: monday.com
- Best for all-in-one project management: ClickUp
- Best for agile project management: Jira Software
- Best for data visualization: Tableau
- Best for Microsoft ecosystem integration: Power BI
Reporting tools and software are crucial to teams, especially in terms of project management as they provide a structured way to track progress, identify risks and make informed decisions. They offer a sweeping view of project health that helps managers to not only pinpoint areas of concern but also identify successes. With effective reporting, an organization gets transparency and ensures its stakeholders are aligned, which plays a part in making projects successful since everyone involved has access to the same information and insights. We’ve analyzed 10 top reporting tools and software worth your consideration.
Top reporting software: Comparison table
Zoho analytics: best for comprehensive data integration.

Zoho Analytics is a reporting tool that excels at aggregating data from a wide array of sources as it connects with over 250 data sources, including files, feeds, databases and cloud services. Its comprehensive suite of reporting options includes charts, pivot tables, summary views, tabular views and more. Zoho Analytics also offers an intuitive drag-and-drop interface to further simplify the report creation process and make it accessible for users of varying skill levels.
Zoho Analytics offers plans starting at $22 per month for the Basic plan, while the Standard, Premium and Enterprise plans cost $45, $112 and $445 per month, respectively, when billed annually. There’s also a Custom plan for prospective users to share their requirements.
- Extensive data integration from over 250 sources.
- Data preparation and management tools for accurate analysis.
- A wide array of visualization options for insightful reporting ( Figure A ).
- AI and ML-powered augmented analytics for guided insights.
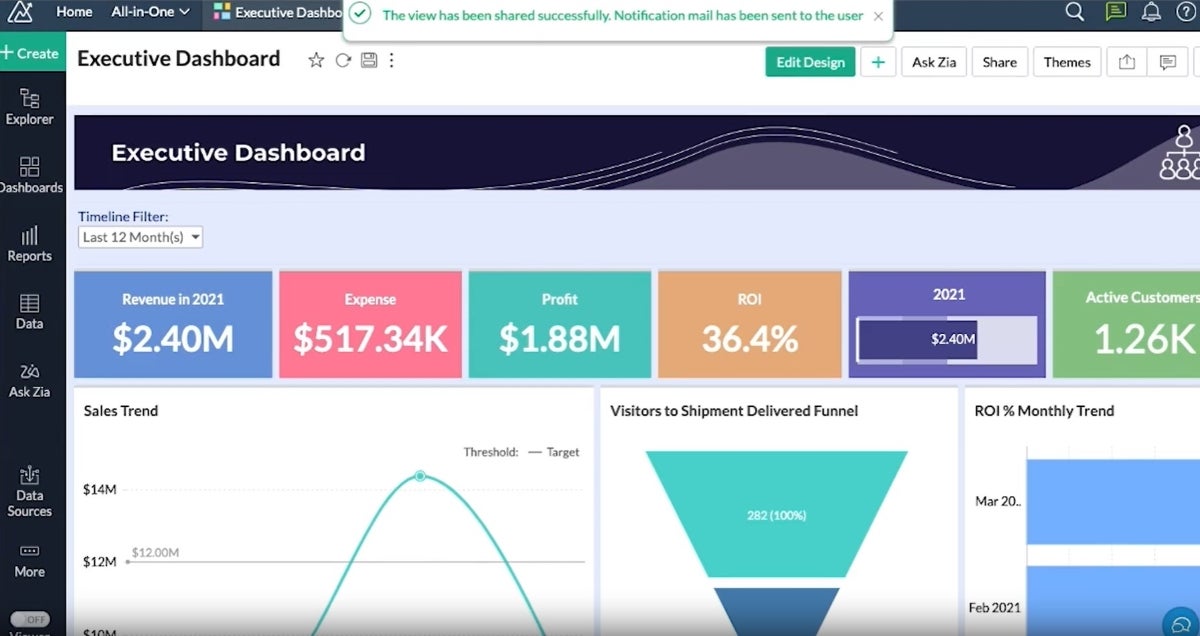
Integrations
Zoho Analytics’s integrations include Zoho CRM, Salesforce CRM, Microsoft Dynamics CRM, HubSpot CRM and Zoho Bigin.
- Comprehensive data integration capabilities.
- Wide range of visualization tools.
- Advanced augmented analytics features.
- May be complex for beginners.
- Customization can require a learning curve.
Why we chose Zoho Analytics
We selected Zoho Analytics for its broad range of reporting capabilities and user-friendly design. Its ability to present data in various visual formats makes analysis flexible and insightful and caters to diverse reporting needs as well as a wide variety of users.
Learn more about other Zoho products, like Zoho Projects and Zoho Vault .
Asana: Best for task-based reporting

Asana simplifies project management with its Universal Reporting feature, which provides teams with a clear overview of task progress and project health. Its visual reporting format is designed for easy interpretation, meaning that users at all levels within an organization can easily access and use Asana.
Asana’s paid plans include the Premium plan at $10.99 per user per month, billed annually, and the Business plan at $24.99 per user per month. Its Enterprise plan’s pricing hasn’t been listed publicly.
- Visual and intuitive reporting tools for task and project tracking ( Figure B ).
- Goal tracking to align daily tasks with strategic objectives.
- Real-time updates to keep teams informed on project progress.
- A variety of highly customizable charts.
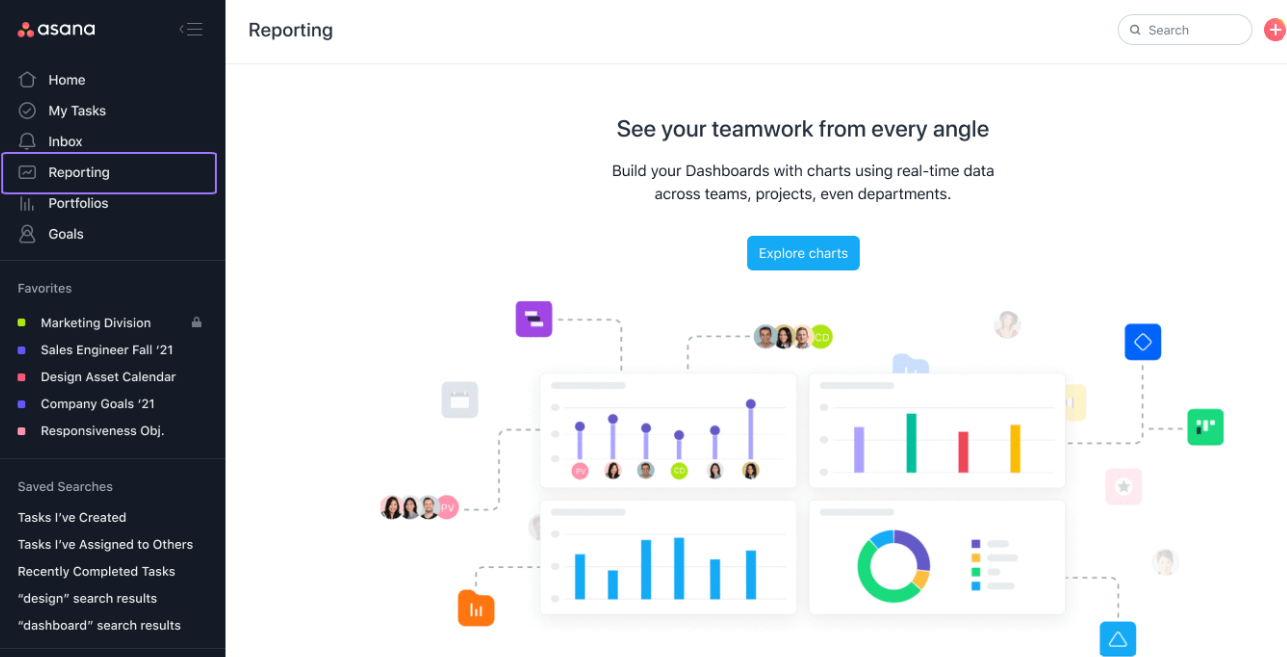
Asana’s top integrations include Microsoft Teams, Slack, the Asana for Gmail add-on, Asana for Adobe Creative Cloud and Google Calendar.
- User-friendly reporting and task management.
- Effective goal alignment features.
- Wide range of integrations.
- Limited depth in analytical features.
- Real-time analytics are somewhat restricted.
Why we chose Asana
We simply selected Asana for its user-friendly approach to task-based reporting. Asana is also highly effective when it comes to aligning tasks with organizational goals.
For more information, check out our full Asana review .
Hive: Best for high-level project reporting
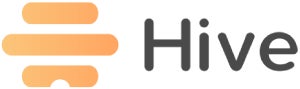
Hive is recognized for its high-level reporting capabilities, offering a suite of options for a variety of project management use cases. With features like goals, analytics dashboards and timesheet reporting, Hive provides a comprehensive tool for gaining visibility and gathering insights into projects.
Hive has two premium plans atop a free plan. Teams at $12 per user per month when billed annually and $18 when billed monthly, and Enterprise, whose prices aren’t publicly listed.
- Goals for setting, tracking and monitoring goals across teams.
- Analytics dashboards to showcase project status, project breakdowns and more.
- Timesheets reporting to analyze data across timesheets.
- Multiple views like Portfolio, Summary, Table, Kanban and more ( Figure C ).
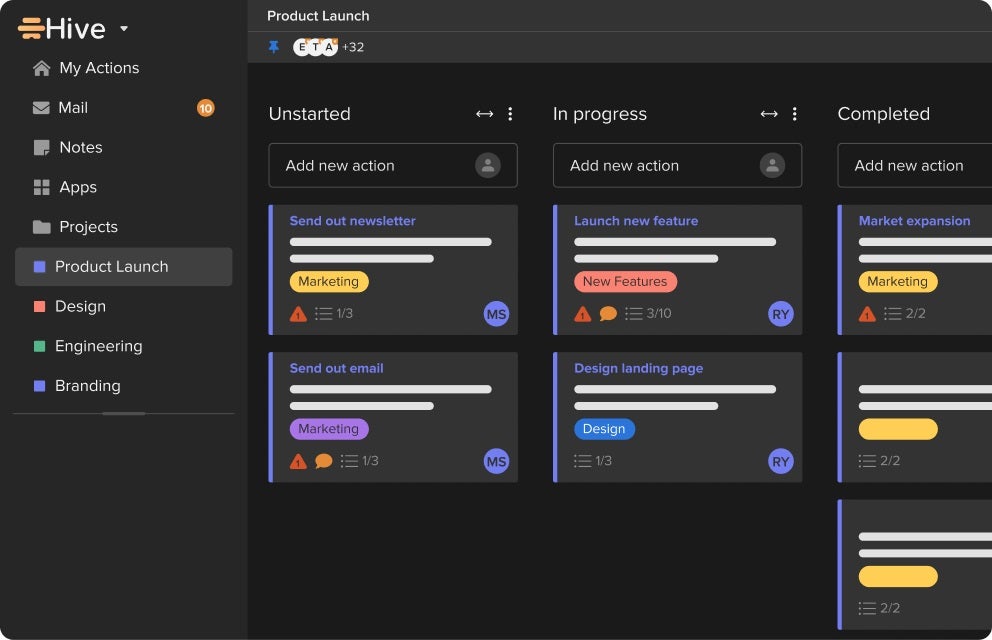
Hive’s top integrations include Google Calendar, Gmail, Google Sheets, Google Drive and Slack.
- Customizable high-level reporting options.
- Variety of views for different reporting needs.
- Efficient project and action management features.
- May require initial setup time to customize views.
- Some advanced features might be available only on higher-tier plans.
Why we chose Hive
We selected Hive for its versatile high-level reporting options and customizable views. They bring a flexible and comprehensive overview to projects.
For more information, check out our full Hive review .
Google Looker: Best for data-driven decision-making
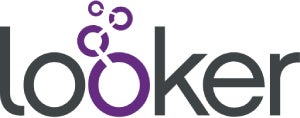
A rather different entry from most tools on this list, Google Looker stands as a unified business intelligence platform that excels at turning data into actionable insights. It offers self-service BI that allows users to access, analyze and act on up-to-date, trusted data. As a reporting tool, Looker offers reliable data experiences at scale and empowers users with real-time insights.
Looker has a 30-day free trial, and its Standard plan costs $5,000 per month. For an annual quote, as well as quotes for the Enterprise and Embed plans, contact Google sales.
- Embedded analytics and applications for enhanced data experiences.
- Data modeling to unify business metrics across teams and applications.
- Real-time insights to empower users with up-to-date information.
- An extensive template gallery for templates on many of Google’s applications ( Figure D ).
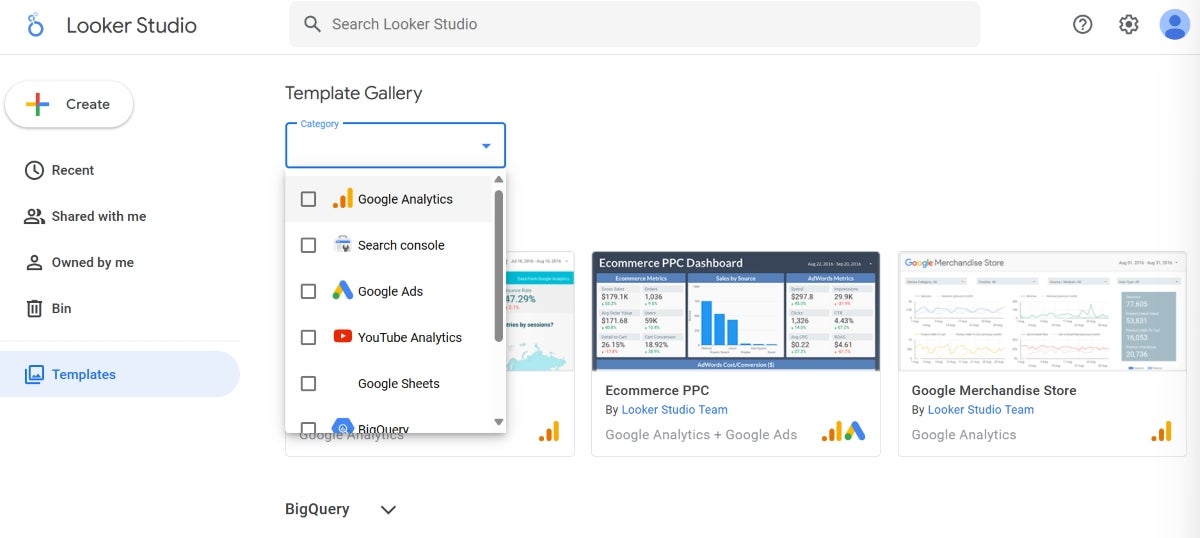
Looker offers extensive integration capabilities, including BigQuery, Spanner, Cloud SQL and Cloud Storage.
- Unified platform for all BI needs.
- Real-time insights for up-to-date decision-making.
- Extensive integration capabilities with data sources.
- Pricing transparency could be improved.
- May require a learning curve to fully utilize advanced features.
Why we chose Google Looker
Google Looker’s reporting capabilities can be seen particularly through its embedded analytics and real-time insights. It easily unifies business metrics across teams and applications. It’s also a great tool for users predominantly using applications in the Google ecosystem.
Wrike: Best for customizable project reporting
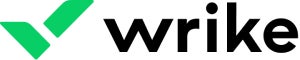
Wrike stands out for its highly customizable reporting features. This flexibility, combined with Wrike’s thorough resource management and advanced analytics, makes Wrike competent enough to provide detailed insights into project performance and resource allocation and flexible enough to adapt to various workflows.
Wrike has five plans: the ones with prices listed are the Free plan, Team plan at $9.80 per user per month and Business plan at $24.80 per user per month. The Enterprise and Pinnacle plans’ pricing plans aren’t publicly listed.
- Customizable reports for tailored project insights ( Figure E ).
- Resource management to monitor progress and identify risks.
- Advanced analytics for deep visibility into project performance.
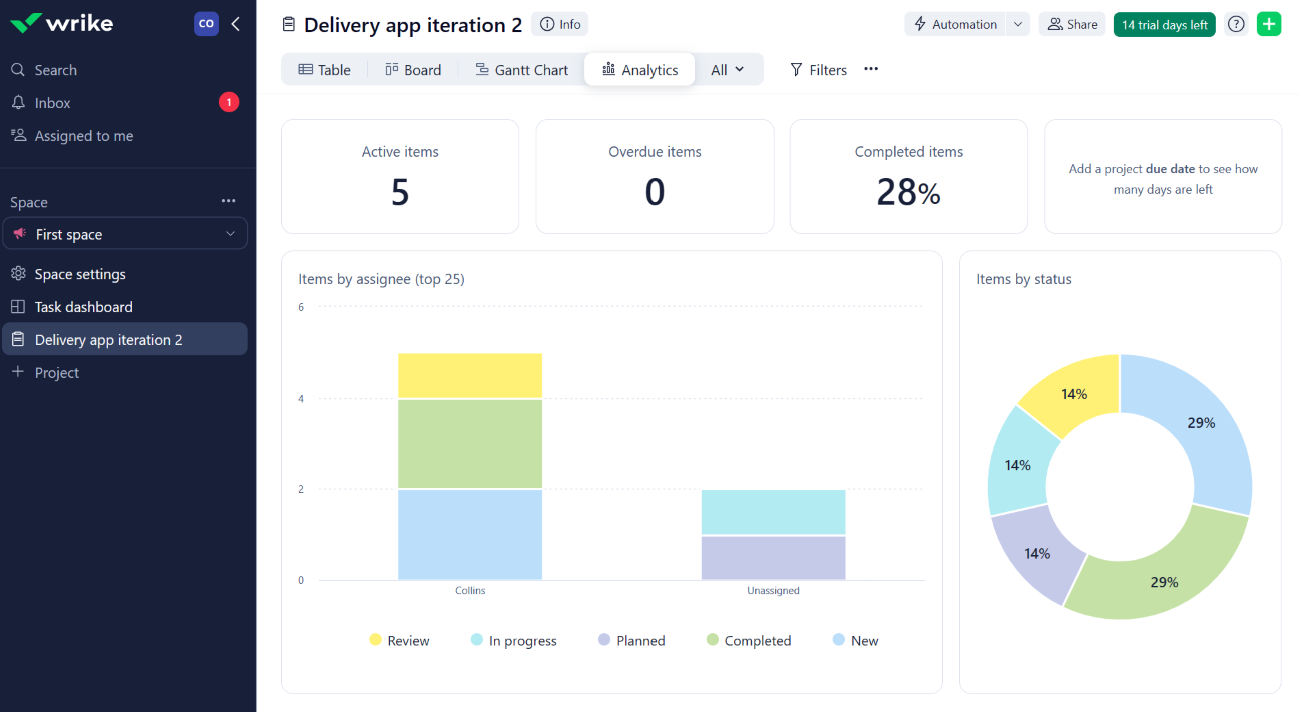
Wrike’s top integrations include Jira, GitHub, Google Sheets, Azure DevOps and HubSpot.
- Highly customizable reporting options.
- Comprehensive project and resource monitoring.
- Advanced analytics capabilities.
- Customization options may require time to master.
- Extensive features can be overwhelming for newcomers.
Why we chose Wrike
Wrike has robust reporting capabilities and customizable features, which give users the flexibility and depth needed to gain extensive insights into their projects and resources.
For more information, check out our full Wrike review .
monday.com: Best for visual project tracking

monday.com is a favorite among teams that love visual task management and prioritize ease of use as it offers a visually intuitive platform for project tracking. Its advanced reporting features, such as stacked charts and workload views, provide a thorough overview of project progress and team capacity. monday.com’s dashboard customization is very flexible; this enables teams to mold their reporting to meet their project needs.
monday has a free plan and a handful of premium plans, namely, Basic at $9 per seat per month, billed annually, or $12 per seat billed monthly; Standard at $12 per seat per month, billed annually, or $14 per seat billed monthly; Pro at $19 per seat per month, billed annually, or $24 per seat billed monthly; and Enterprise, which offers customized pricing.
- Stacked charts for multi-dimensional data analysis.
- Workload views for balanced resource allocation.
- Pivot tables for detailed data breakdowns.
- Customizable dashboards for tailored project insights ( Figure F ).
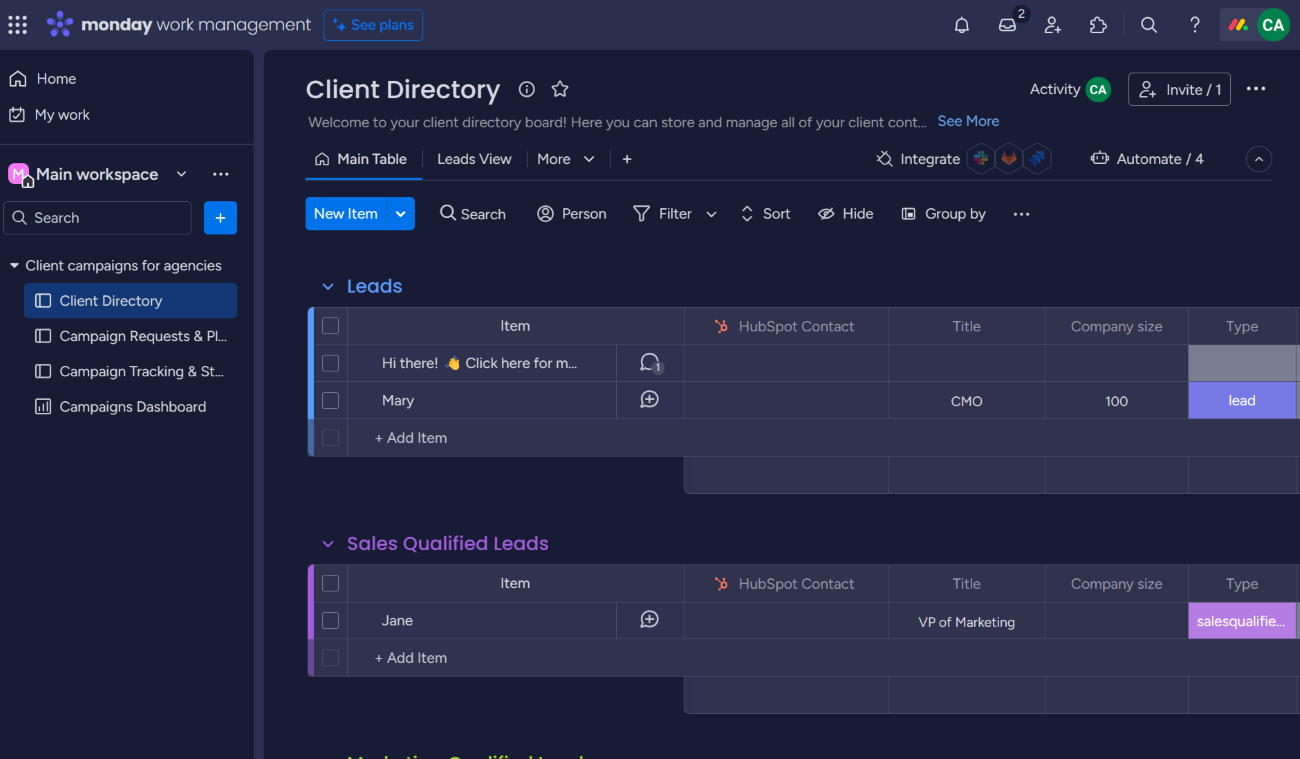
Some of the best monday.com integrations include GitLab, OneDrive, Todoist, Slack and Microsoft Teams.
- Highly visual and intuitive interface.
- Advanced reporting for comprehensive project insights.
- Flexible dashboard customization.
- Can be overwhelming for new users due to numerous features.
- Some advanced features require higher-tier plans.
Why we chose monday.com
monday.com is a visually intuitive platform and has advanced reporting capabilities. It delivers a balance between visual project tracking and in-depth reporting.
For more information, check out our full monday.com review .
ClickUp: Best for all-in-one project management
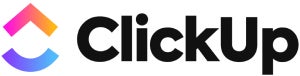
ClickUp is recognized for its all-in-one approach to project management, offering a wide range of features from task management to time tracking and goal setting. Its reporting features are designed to provide teams with insights into productivity and project progress, supporting data-driven decision-making. ClickUp’s customizable dashboards and reporting tools allow teams to monitor key metrics and track performance effectively.
ClickUp offers a generous free forever plan alongside three premium tiers: Unlimited at $7 per user per month when billed annually, or $10 per user per month when billed monthly; Business at $12 per user per month when billed annually, or $19 per user per month when billed monthly; and Enterprise that needs prospective users to contact ClickUp for a custom quote.
- Comprehensive dashboards for project overview ( Figure G ).
- Customizable reporting for tailored insights.
- Goal tracking to align efforts with objectives.
- Time tracking to monitor task durations and productivity.
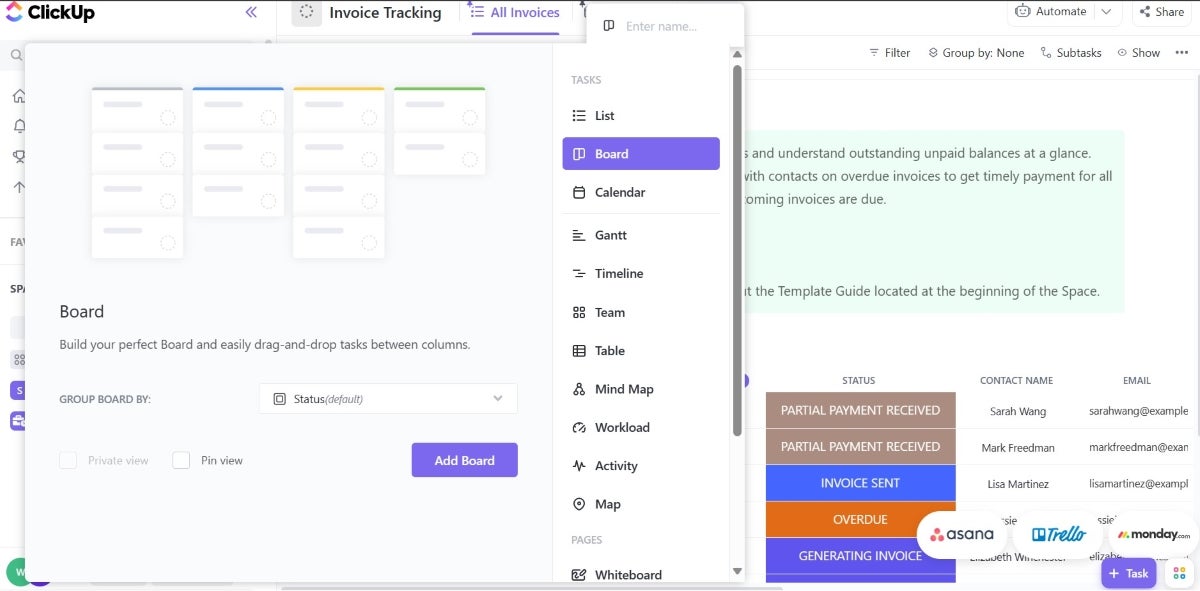
Some of ClickUp’s top integrations include Gmail, Zoom, HubSpot, Make and Google Calendar.
- Versatile all-in-one project management solution.
- Extensive customization options for dashboards and reporting.
- Generous free plan with substantial features.
- Steep learning curve due to feature richness.
- Customization can be time-consuming.
Why we chose ClickUp
We included ClickUp because of its comprehensive feature set and flexibility, offering teams an all-in-one solution for project management and reporting. It proves suitable for a wide range of project types and sizes.
For more information, check out our full ClickUp review .
Jira Software: Best for agile project management

Jira Software is tailored for agile project management with specialized reporting features like sprint reports, burndown charts and velocity charts. These agile-centric reports give teams critical insights into their agile processes to help them optimize workflows and improve sprint planning. It’s worth considering for software development teams and those that follow scrum or kanban frameworks.
Jira offers a free plan for 10 users max. Its premium plans are the Standard plan at about $8.15 per user per month and the Premium plan at about $16 per user per month. It also offers an Enterprise plan that’s billed annually. However, you need to contact Jira for a quote.
- Sprint reports for tracking sprint progress ( Figure H ).
- Burndown charts for visualizing task completion.
- Velocity charts for assessing team performance over sprints.
- Cumulative flow diagrams for Kanban teams.
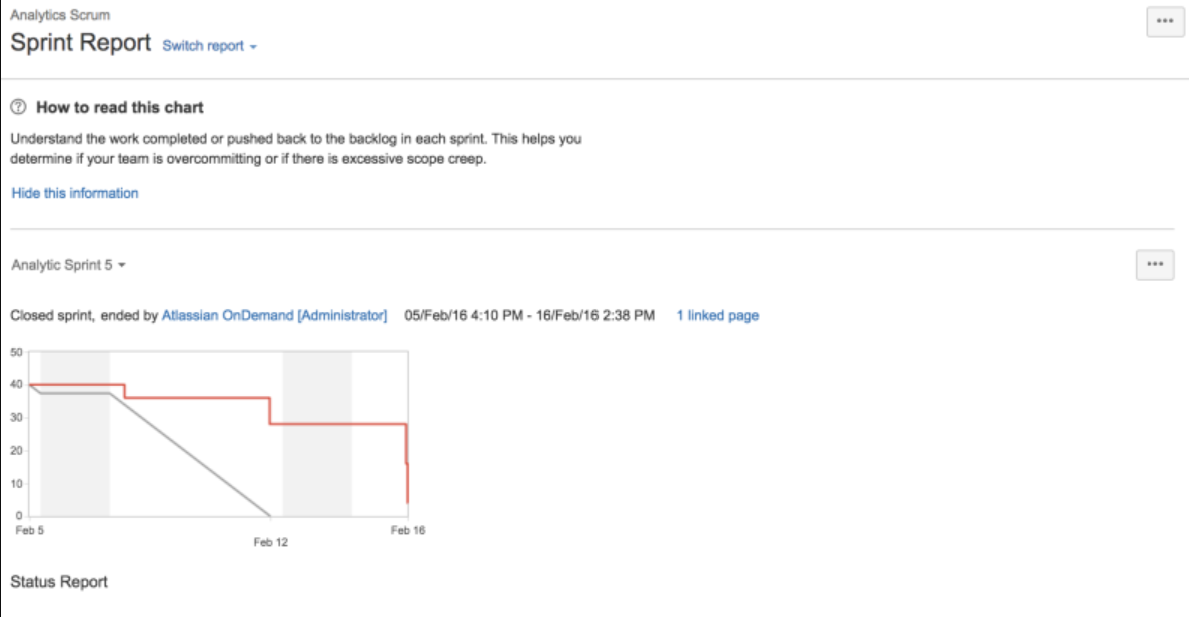
Jira has extensive integrations with development tools like Bitbucket, Confluence, GitHub, Opsgenie, Jenkins and Dynatrace.
- Tailored for agile project management.
- Comprehensive reporting for scrum and kanban teams.
- Wide range of integrations with development tools.
- Primarily focused on software development teams.
- Can be complex for non-technical users.
Why we chose Jira Software
Jira Software has robust agile reporting features and is capable of providing deep insights into agile project management processes, especially for teams practicing scrum or kanban methodologies.
For more information, check out our full Jira Software review .
Tableau: Best for data visualization
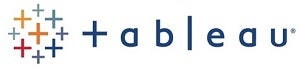
Tableau sets the standard for data visualization, offering a wide range of chart types and interactive dashboards that make complex data understandable at a glance. As reporting software, it offers a user-friendly interface and powerful data handling capabilities for users to create detailed and insightful visual reports.
Tableau’s pricing starts at $15 per user per month, with its highest tier costing $75 per user per month, both billed annually.
- Wide range of visualization options.
- User-friendly interface for non-technical users ( Figure I ).
- Powerful data handling and processing capabilities.
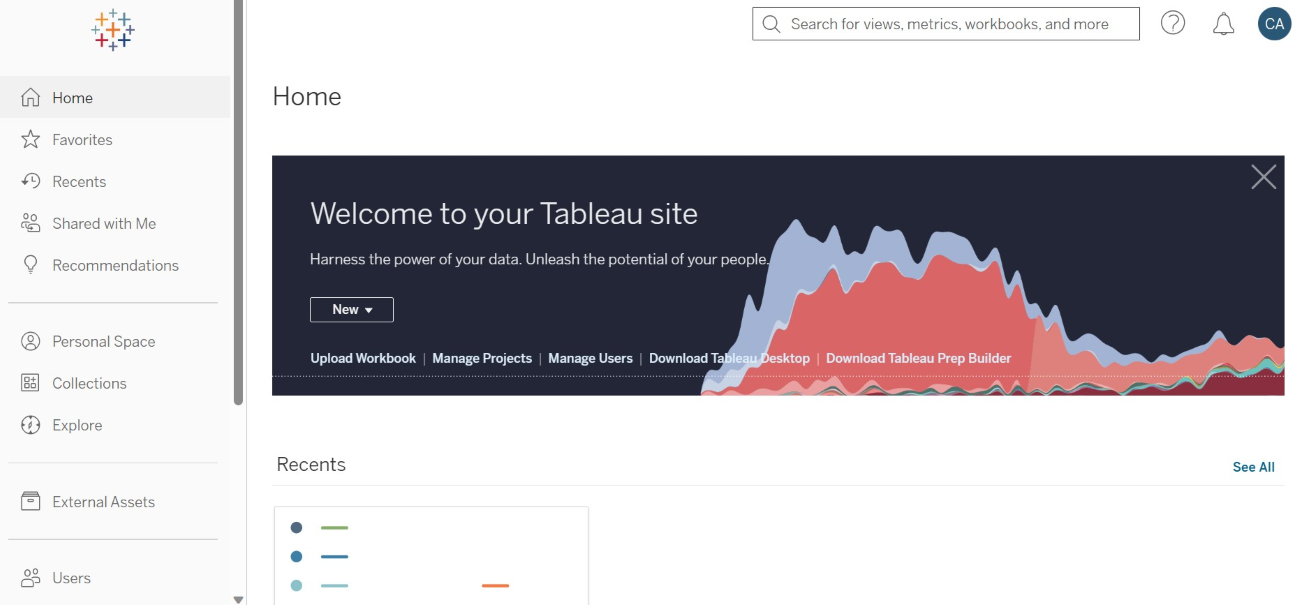
Tableau’s top integrations include Salesforce, Google Analytics, Microsoft Excel, Amazon Redshift and Snowflake.
- Leading data visualization capabilities.
- Intuitive interface for easy use.
- Strong data connectivity options.
- Higher price point compared to some competitors.
- Can require significant resources for large datasets.
Why we chose Tableau
We considered Tableau because of its unparalleled data visualization capabilities and user-friendly interface. It should make it to your shortlist if your teams value both data accessibility and detailed reporting.
For more information, check out our full Tableau review .
Power BI: Best for Microsoft ecosystem integration
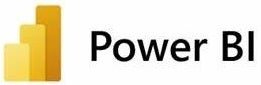
Power BI is a key player in the reporting and analytics space, especially for those deeply embedded in the Microsoft ecosystem. Its seamless integration with other Microsoft products, like Excel and Azure, makes it a no-brainer for teams that want compatibility and ease of use with their reporting tools. What makes it a great reporting and analytics tool is its ability to handle large datasets and provide advanced analytics, including AI capabilities and custom visualizations.
Power BI offers a free version, with premium plans starting at $10 per user per month for the Pro plan and $20 per user per month for the Premium plan.
- Seamless integration with Microsoft products.
- Advanced analytics with AI capabilities.
- Custom visualizations for personalized reporting ( Figure J ).
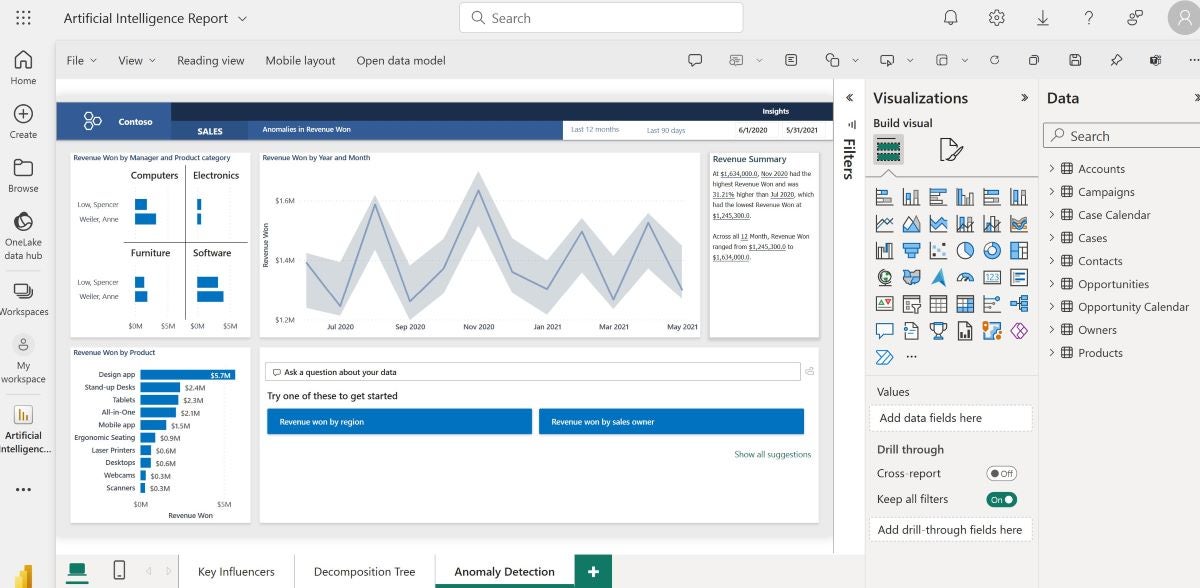
Aside from a variety of tools in the Microsoft ecosystem like Microsoft Office 365, Power BI’s top integrations include Asana, HubSpot, Google Sheets and Salesforce Pardot.
- Strong Microsoft integration.
- Comprehensive analytics and AI features.
- Flexible pricing with a robust free version.
- Can be complex for new users.
- Limited integration outside the Microsoft ecosystem.
Why we chose Power BI
We chose Power BI due to its strong analytics capabilities combined with its seamless integration with tools in the Microsoft ecosystem. It’s a particularly fitting choice for teams that already use Microsoft products.
For more information, check out our full Power BI review .
Key features of reporting software
Real-time analytics.
Real-time analytics allows users to view, assess and analyze data as it flows into the business, which can be displayed on dashboards or reports. With this, users get to make decisions faster since they get instant, descriptive insights from the most current data.
Custom reports
Custom reports save time as they automate the data gathering and report generation processes. After the initial setup, reporting processes can be entirely streamlined, with live data feeds ensuring that any additional requests can be quickly addressed by making changes to existing reports.
Dashboard customization
Dashboard customization is crucial in reporting software as it allows users to set up their reporting environment based on their needs. Custom dashboards can provide in-depth data on various aspects of business operations, illustrating potential revenue and areas where improvements are needed. Businesses can mix and match data sources for a comprehensive view of their digital environment.
Automated reporting
This kind of reporting streamlines the process of generating regular reports and reduces the manual effort required while making sure that stakeholders receive timely updates. Users can schedule report generation and ensure that reports are always current and reflect the latest data.
Data visualization
Data visualization transforms complex datasets into graphical representations, making it easier to understand trends, patterns and outliers. This feature helps to make data more accessible and actionable, which enables users to quickly grasp the insights presented in the data.
How do I choose the best reporting software for my business?
First things first, when it comes to choosing the best reporting software for you, you must match a tool’s capabilities to your needs. For small to medium-sized businesses, tools like Zoho Analytics and ClickUp offer a vast feature set at a more accessible price point, which makes them great options when seeking value without compromising on functionality. Larger enterprises or those with more complex reporting and data analysis needs might lean towards Power BI or Tableau, known for their advanced analytics and integration within larger ecosystems.
Consider the types of reports you need, the data you’re working with and who will be using the tool. For teams that prioritize real-time data and collaboration, monday.com and Asana provide user-friendly interfaces and seamless integration with other productivity tools. On the other hand, if your focus is on in-depth data analysis and visualization, Tableau’s extensive customization options and Power BI’s deep Microsoft integration stand out.
In essence, the best reporting tool is one that not only fits your budget and technical requirements but also grows with your business, adapting to changing needs and helping you make informed decisions based on accurate, up-to-date data.
Methodology
Our approach to identifying the top reporting tools for 2024 involved a detailed examination of each tool’s core features, ease of use, use cases and pricing. This allowed us to provide popular tools that cut across industries, use cases and team sizes. Additionally, we tested the tools where possible to understand how they approached reporting and compared our findings to verified reviews by real users. From this, we got to understand the pros and cons of each tool.
Subscribe to the Project Management Insider Newsletter
Subscribe to Project Management Insider for best practices, reviews and resources. From project scheduling software to project planning apps, stay up to date with the latest in project management tools. Delivered Wednesdays
- The Best Project Management Software and Tools for 2024
- The Best Simple Project Management Software of 2024
- The Best Project Management Certifications in 2024
- Telephone Interview Cheat Sheet: Project Manager
Create a TechRepublic Account
Get the web's best business technology news, tutorials, reviews, trends, and analysis—in your inbox. Let's start with the basics.
* - indicates required fields
Sign in to TechRepublic
Lost your password? Request a new password
Reset Password
Please enter your email adress. You will receive an email message with instructions on how to reset your password.
Check your email for a password reset link. If you didn't receive an email don't forgot to check your spam folder, otherwise contact support .
Welcome. Tell us a little bit about you.
This will help us provide you with customized content.
Want to receive more TechRepublic news?
You're all set.
Thanks for signing up! Keep an eye out for a confirmation email from our team. To ensure any newsletters you subscribed to hit your inbox, make sure to add [email protected] to your contacts list.
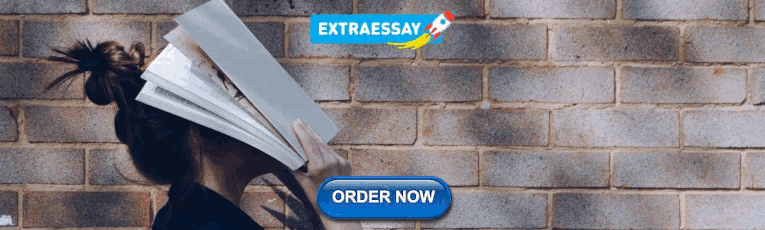
IMAGES
VIDEO
COMMENTS
Best 10 Quantitative Data Analysis Software. 1. QuestionPro. Known for its robust survey and research capabilities, QuestionPro is a versatile platform that offers powerful data analysis tools tailored for market research, customer feedback, and academic studies.
6. Kissmetrics. Kissmetrics is a software for quantitative data analysis that focuses on customer analytics and helps businesses understand user behavior and customer journeys. Kissmetrics lets you track user actions, create funnels to analyze conversion rates, segment your user base, and measure customer lifetime value.
Learn about the three main programs that quantitative social scientists use for statistical analysis: SPSS, STATA, and SAS. Compare their features, advantages, and disadvantages, and find out how to get started with each one.
1. Microsoft Excel. Microsoft Excel is a widely available spreadsheet software often used for basic data analysis and visualization. It is user-friendly and suitable for researchers working with small datasets. Excel is readily accessible and frequently used for preliminary data exploration and simple calculations.
History. The first version of SPSS was developed by Norman H. Nie, Dale H. Bent and C. Hadlai Hull in and released in 1968 as the Statistical Package for Social Sciences.; In July 2009, IBM acquired SPSS. Users. Social sciences; Health sciences; Marketing; Academia Data Format and Compatibility
Quantitative data has to be gathered and cleaned before proceeding to the stage of analyzing it. Below are the steps to prepare a data before quantitative research analysis: Step 1: Data Collection. Before beginning the analysis process, you need data. Data can be collected through rigorous quantitative research, which includes methods such as ...
IBM® SPSS® Statistics is a powerful statistical software platform. It offers a user-friendly interface and a robust set of features that lets your organization quickly extract actionable insights from your data. Advanced statistical procedures help ensure high accuracy and quality decision making. All facets of the analytics lifecycle are ...
The best quantitative data analysis software depends on a variety of factors, such as the type of data you're analyzing, the volume of data, the complexity of the data, etc. From basic to advanced data analysis, the best tools are designed to make the process as easy as possible.
The IBM® SPSS® software platform offers advanced statistical analysis, a vast library of machine learning algorithms, text analysis, open-source extensibility, integration with big data and seamless deployment into applications. Its ease of use, flexibility and scalability make SPSS accessible to users of all skill levels.
The University of Oregon provides access to a number of quantitative analysis software for current students and faculty. Some software may be limited by department. ... Analyzing Quantitative Data is an excellent book for social sciences courses on data analysis and research methods at the upper-undergraduate and graduate levels. It also serves ...
Learn the basics of quantitative research, a positivist research approach that focuses on the quantification of data to test hypotheses and theories. Find out how to choose a research design, software, and methods for data analysis. Compare ATLAS.ti with other software packages and see examples of exporting your data to SPSS, Excel, SAS, or R.
A collection of e-books and other resources covering research methods in the social and behavioral sciences. It contains the popular Little Green Book series as well as other titles on quantitative analysis. NUIT Research Data Services: Training and Learning. NUIT offers data analysis training through workshops and online learning.
Quantitative data analysis is one of those things that often strikes fear in students. It's totally understandable - quantitative analysis is a complex topic, full of daunting lingo, like medians, modes, correlation and regression.Suddenly we're all wishing we'd paid a little more attention in math class…. The good news is that while quantitative data analysis is a mammoth topic ...
Here's how to make sense of your company's numbers in just four steps: 1. Collect data. Before you can actually start the analysis process, you need data to analyze. This involves conducting quantitative research and collecting numerical data from various sources, including: Interviews or focus groups.
8. Weight customer feedback. So far, the quantitative data analysis methods on this list have leveraged numeric data only. However, there are ways to turn qualitative data into quantifiable feedback and to mix and match data sources. For example, you might need to analyze user feedback from multiple surveys.
Abstract. Quantitative data analysis serves as part of an essential process of evidence-making in health and social sciences. It is adopted for any types of research question and design whether it is descriptive, explanatory, or causal. However, compared with qualitative counterpart, quantitative data analysis has less flexibility.
Research Guides: Software for Data Analysis: Quantitative Data Analysis Software
NVivo qualitative data analysis software helps to discover richer insights from your qualitative & mixed methods research. Organize, store, and analyze data today! The NVivo 14 Bundle is Back - Save $280! Free Trial. Community. ... quantitative, or mixed methods data analysis, we can help your whole team work better together — collaborating ...
MATLAB is a quantitative analysis software optimized for solving scientific and engineering problems. Typical users include education-based (specifically linear algebra and numerical analysis), scientists, and engineers. ... Analyzing Quantitative Data is an excellent book for social sciences courses on data analysis and research methods at the ...
Introduction to Quantitative Data Analysis in the Behavioral and Social Sciences also features coverage of the following: * The overall methodology and research mind-set for how to approach quantitative data analysis and how to use statistics tests as part of research data analysis * A comprehensive understanding of the data, its connection to ...
nominal. It is important to know w hat kind of data you are planning to collect or analyse as this w ill. affect your analysis method. A 12 step approach to quantitative data analysis. Step 1 ...
Stata 3: Advanced Data Analysis. This workshop is the third in a three-part series on Stata. Stata is a statistical software package. Stata is widely used by scientists throughout the social sciences for analysis of quantitative data ranging from simple descriptive analysis to complex statistical modeling.
The purpose of quantitative data is to provide a numerical representation of a phenomenon or observation. Quantitative data is used to measure and describe the characteristics of a population or sample, and to test hypotheses and draw conclusions based on statistical analysis. Some of the key purposes of quantitative data include:
Best for task-based reporting: Asana. Best for high-level project reporting: Hive. Best for data-driven decision-making: Google Looker. Best for customizable project reporting: Wrike. Best for ...