Help | Advanced Search
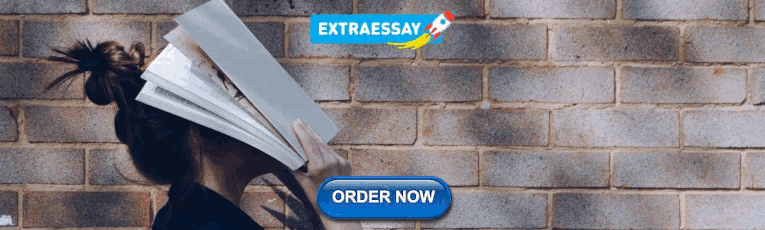
Computer Science > Computers and Society
Title: the perception of filipinos on the advent of cryptocurrency and non-fungible token (nft) games.
Abstract: This study aims to shed light on the rise of play-to-earn games in the Philippines alongside cryptocurrency. The lack of research and public understanding of its benefits and drawbacks prompted the researchers to investigate its market. As such, the study tried to look into the risks and benefits of crypto gaming if it would be regulated by the government, and how market volatility influences the churn rate of crypto games. The research used a descriptive study to determine the perception of people who are engaged in playing a crypto-game named as Axie Infinity. The results showed that most players spend their time playing Axie Infinity for about 1 to 4 hours a day. Predominantly, the return of investments for playing the game will take about 1 to 3 months. It also showed that these players agreed that there is a possible financial instability in a volatile market. With this, they have a high trust issue in terms of price manipulation, privacy and security, and its design and usability. Understanding the cryptocurrency market requires comprehending the perspective of the people who are engaged in a play-to-earn game, and their concerns are critical for any government actions aimed at regulating self-employed income earners playing (Non-fungible Tokens) NFT games in the Philippines.
Submission history
Access paper:.
- Other Formats

References & Citations
- Google Scholar
- Semantic Scholar
BibTeX formatted citation

Bibliographic and Citation Tools
Code, data and media associated with this article, recommenders and search tools.
- Institution
arXivLabs: experimental projects with community collaborators
arXivLabs is a framework that allows collaborators to develop and share new arXiv features directly on our website.
Both individuals and organizations that work with arXivLabs have embraced and accepted our values of openness, community, excellence, and user data privacy. arXiv is committed to these values and only works with partners that adhere to them.
Have an idea for a project that will add value for arXiv's community? Learn more about arXivLabs .

The Perception of Filipinos on the Advent of Cryptocurrency and Non-Fungible Token (NFT) Games
- Ryan D. Francisco College of Engineering, Technological Institute of the Philippines – Quezon City, Philippines
- Nelson C. Rodelas College of Engineering, University of the East – Caloocan, Philippines
- John Edison T. Ubaldo University of the Philippines – Diliman, Philippines
Purpose – This study aims to shed light on the rise of play-to-earn games in the Philippines alongside cryptocurrency. The lack of research and public understanding of its benefits and drawbacks prompted the researchers to investigate its market. As such, the study tried to look into the risks and benefits of crypto gaming if it would be regulated by the government, and how market volatility influences the churn rate of crypto games. Method – The research used a descriptive study to determine the perception of people who are engaged in playing “Axie Infinity”. The research also used non-probability sampling using the snowball method. The only tool used to collect data was Google Forms. Furthermore, the study used Statistical Package for Social Science (SPSS) to analyze the descriptive data. Result – The results showed that most players spend their time playing Axie Infinity for about 1 to 4 hours a day. Predominantly, the return of investments for playing the game will take about 1 to 3 months. It also showed that these players agreed that there is a possible financial instability in a volatile market. With this, they have a high trust issue in terms of price manipulation, privacy and security, and its design and usability. Conclusion – Understanding the cryptocurrency market requires comprehending the perspective of the people who are engaged in a play-to-earn game, and their concerns are critical for any government actions aimed at regulating self-employed income earners playing (Non-fungible Tokens) NFT games in the Philippines. Recommendations – It is suggested that further research must be conducted to understand how self-employed Filipino income earners comprehend NFT gaming and cryptocurrency, impacting their level of interest and participation. Research Implications – Taxing NFT games without sound regulatory frameworks may result in the disarray of this alternative income source. Therefore, the government must tread carefully to avoid a market collapse or other unfavorable outcomes.
- pdf (4051 views)
- EndNote - EndNote format (Macintosh & Windows)
- ProCite - RIS format (Macintosh & Windows)
- Reference Manager - RIS format (Windows only)

This work is licensed under a Creative Commons Attribution 4.0 International License .
This is an Open Access article distributed under the terms of the Creative Commons Attribution License (http://creativecommons.org/licenses/by/4.0), which permits unrestricted use, distribution, and reproduction in any medium, provided the original work is properly credited.
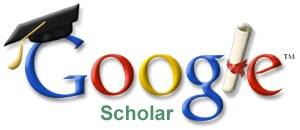
All IJCSR articles are indexed in Google Scholar. See Indexing Service and Archiving for other partners.

All articles will have a digital object identifier — ensuring wider dissemination of articles and perpetual archiving.

Open-access logo: By art designer at PLoS, modified by Wikipedia users Nina, Beao, and JakobVoss – http://www.plos.org/ , CC0, https://commons.wikimedia.org/w/index.php?curid=5069489

IJCSR is now an official member of the CrossRef community.

STEP Academic is an academic private publishing institution owned by business partners Mr. Mark Vale and Mr. John Paul Aquino. It serves as the publication partner of the University of Makati, Isabela State University, Adamson University, the Philippine Society of IT Educators (NCR and National Chapters), the Institute of Computer Engineers of the Philippines – NCR Chapter, and the Asia-Pacific Society of Volunteers Inc.
Mark Anthony Vale STEP Academic Barangay Sangandaan District VI, Quezon City Philippines (+63) 916-260-6086 (+63) 421-44-19

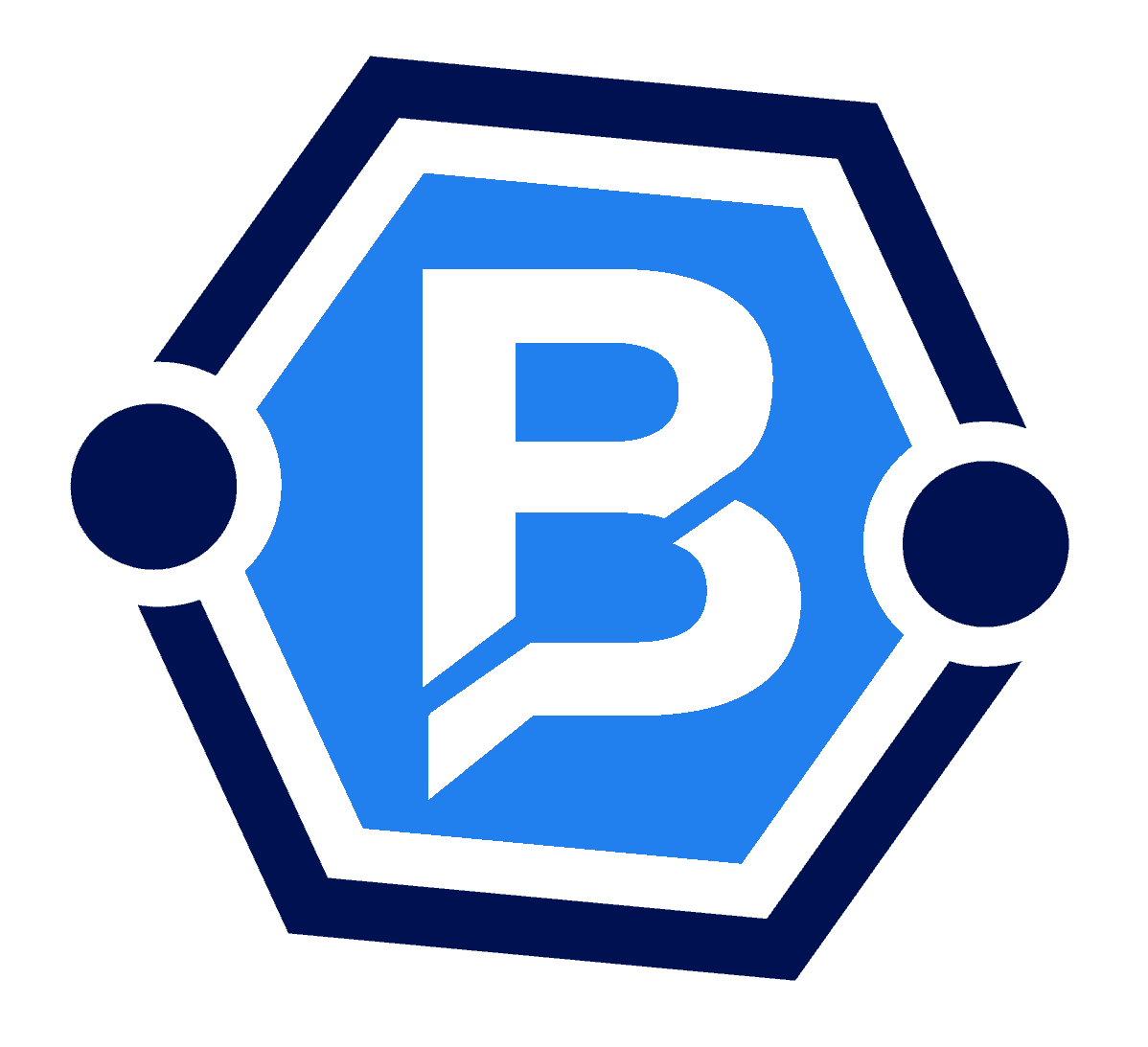
Crypto News Philippines
- Bitcoin in the Philippines: Adoption, Regulation, and Use Cases
In this article, learn about the role of Bitcoin in promoting financial services and its impact on the local economy.
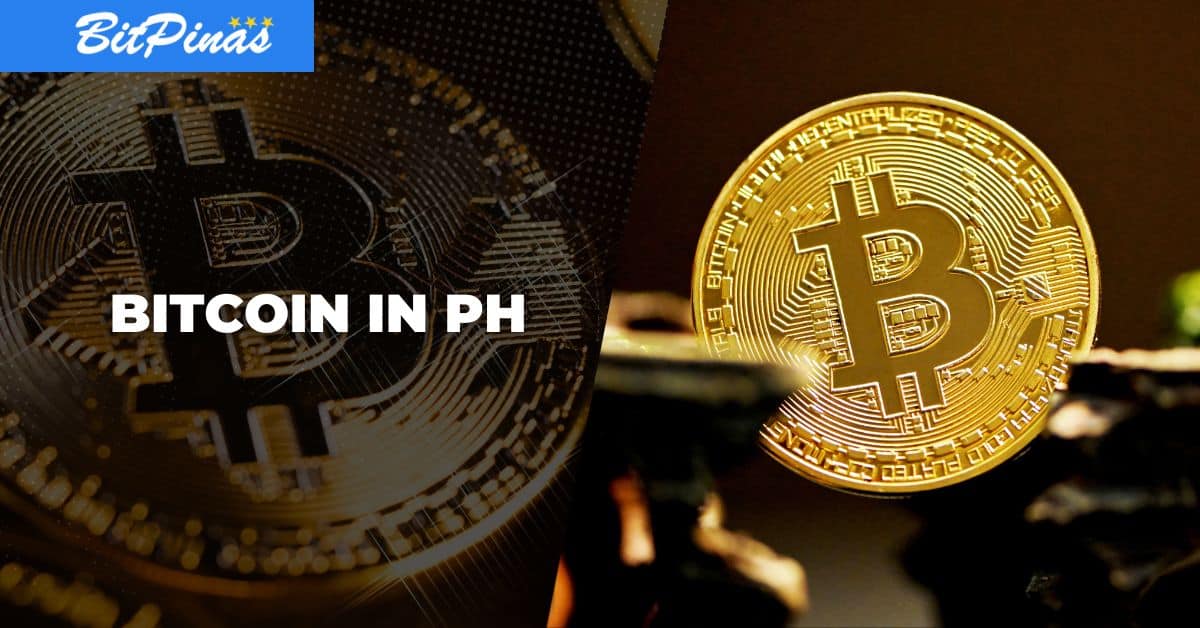
Subscribe to our newsletter!
Editing by Nathaniel Cajuday
- Bitcoin provides an alternative financial system for the unbanked and underbanked population, allowing them to participate in the global economy and access financial services.
- The adoption of Bitcoin in the country is driven by factors such as remittances, online freelancing, and investment opportunities.
- Meanwhile, the regulation of cryptocurrencies in the Philippines is still in progress, with discussions on legislation and frameworks by the Bangko Sentral ng Pilipinas (BSP) and the Securities and Exchange Commission (SEC).
Bitcoin adoption in the Philippines has been steadily growing over the years. Since the crypto bull run in 2021, the local adoption of cryptocurrency in the country has been rapid compared to the other countries surveyed by the blockchain data platform Chainalysis for its annual index report titled, “The 2022 Global Crypto Adoption Index,” where the Philippines ranked 2nd.
Welcome to the Bitcoin Month Series on BitPinas, where we discuss everything Bitcoin. Please check out the other articles:
- Bitcoin Month: Origins and Key Milestones in Bitcoin’s History
- Bitcoin Pizza Day: The Story Behind the First-Ever Real-World BTC Transaction
- How to Buy and Store Bitcoin: A Step-by-Step Guide
- Bitcoin and the Lightning Network: An Introduction to Scalability Solutions
With the emergence of Bitcoin and other cryptocurrencies, the country’s large population of tech-savvy individuals—who are unbanked or underbanked—had another way of being financially capable. Cryptocurrencies provide an alternative financial system, enabling Filipinos to participate in the global economy and access financial services.
- Remittances
Bitcoin presents a more efficient and cost-effective alternative, enabling OFWs to transfer funds to their loved ones in the Philippines with greater speed and affordability.
With several online retailers and service providers now accepting Bitcoin as a payment method, customers can now use the leading crypto to make purchases, providing them with an alternative way to utilize their cryptocurrency for online transactions.
- Freelancing and Outsourcing
Bitcoin offers freelancers and service providers a convenient way to receive payments from international clients without the need for intermediaries or high transaction fees.
- Investment and Trading
Since Bitcoin is the leading cryptocurrency, many view it as a speculative investment or a long-term store of value.
- Financial Inclusion
Bitcoin plays a significant role in promoting financial inclusion in the Philippines, specifically benefiting the unbanked and underbanked population. Through cryptocurrency wallets and exchanges, individuals gain access to essential financial services, enabling them to store value, engage in transactions, and actively participate in the digital economy, even in the absence of traditional bank accounts.
- Cross-Border Transactions
Bitcoin’s decentralized nature and borderless transactions make it well-suited for cross-border payments and international transactions. It eliminates the need for intermediaries, reduces fees, and expedites the transfer process.
- Donations and Charitable Contributions
Bitcoin has been utilized for charitable purposes in the Philippines. Non-profit organizations and individuals have started accepting Bitcoin donations, enabling the transparent and efficient transfers of funds for various causes.
Read the Crypto Donations Series presented by BitPinas:
- DIGITAL BAYANIHAN: Cryptocurrency Revolutionizing Donations
- Pros and Cons of Crypto Donations
- List of NGOs, Foundations, and Charities that Accept Crypto Donations
The country has seen an increase in the use and acceptance of Bitcoin for various purposes. Its adoption in the Philippines has been driven by various factors, including remittances, online freelancing, and investment opportunities. Some of the use-cases of it that could further promote adoption in the country include:
Globally, the Philippines is known for its large population of overseas Filipino workers (OFWs) who send money back home. Remittances are also a significant economic asset, contributing 8.9% of the country’s gross domestic product (GDP). With the expensive charges of traditional remittance services, Bitcoin has emerged as a popular alternative due to its faster transactions and lower fees.
During a community meetup, Binance to the Philippines General Manager Kenneth Stern suggested that blockchain technology and cryptocurrency could revolutionize remittance services , proposing the use of cryptocurrencies and blockchain technology for their advantages of speed, direct peer-to-peer transfers, and low fees. Stern also emphasized that digital wallets and cryptocurrencies can greatly benefit the Filipino people by promoting financial inclusion and offering access to world-class financial products.
Strike, a remittance service utilizing Bitcoin’s Lightning Network, has expanded its operations to the Philippines by partnering with Bitcoin payments firm Pouch.ph to convert BTC into local fiat and facilitate transfers to recipients’ bank or mobile money accounts. The company aims to offer faster and cheaper international payments compared to traditional methods by leveraging the Lightning Network on the Bitcoin blockchain.
Bitcoin has gained popularity as an investment asset in the Philippines. Many Filipinos view Bitcoin as a store of value and a hedge against inflation. Local cryptocurrency exchanges provide platforms for buying and selling Bitcoin, allowing individuals to invest in the cryptocurrency market.
- Online Freelancing
Especially during the pandemic, the Philippines had a thriving freelance industry, with many Filipinos working remotely for international clients. Aside from e-payments, Bitcoin has become a preferred method of payment for freelancers. This mode of payment has been especially essential for those receiving payments from clients outside the country, as it offers faster and cheaper cross-border transactions compared to traditional payment methods.
With the large population of the country still unbanked due to limited access to traditional banking services, Bitcoin has played a role in expanding financial inclusion in the Philippines. Cryptocurrencies provide an alternative means for individuals to store value, make transactions, and access financial services without relying on traditional banks.
Boracay Island, one of the top tourist spots in the Philippines, is currently dubbed as a “ Bitcoin Island ” through the efforts of Pouch.ph, which has been actively promoting the use of Bitcoin on the island. (Read more: I Got a Haircut in Boracay and Paid With Bitcoin )
- Blockchain and Cryptocurrency Startups
The Philippines has shown interest in promoting blockchain technology and fostering a supportive environment for cryptocurrency startups. Initiatives like the “Crypto Valley of Asia” in the Cagayan Economic Zone have aimed to attract blockchain and cryptocurrency businesses, contributing to the growth of the industry in the country.
Last March, the first-ever Bitcoin Island Retreat commenced at the Henann Regency Resort and Spa in Boracay Island, Aklan, Philippines. It was attended by speakers across multiple industries and crypto-business sectors, including the former Solicitor General and Current Dean of Silliman University College of Law, Florin Hilbay.
Recently, mobile money and crypto wallet Maya has expanded its services by allowing users to convert specific cryptocurrencies to Bitcoin directly within the app.
Student Pays Meal with Maya, Wins 1M worth of Bitcoin
- Awareness and Education
Bitcoin awareness and education efforts have increased in the Philippines. Local communities, organizations, and online platforms offer resources and educational events to help individuals understand Bitcoin, blockchain technology, and cryptocurrency investing. (Read more: List of Web3 Educational Platforms to Help Filipinos Get Started in Crypto and Blockchain )
In his lecture at the University of the Philippines, Hilbay encouraged understanding Bitcoin’s complexity, highlighting its immunity to inflation and potential as a global reserve asset. He also differentiated between the fiat system and the Bitcoin system, describing the latter as a permissionless, separate monetary system.
Hilbay also expressed his bullish stance on Bitcoin, calling it “the only real money for the 21st century” during the Bitcoin Island Retreat. Prior to these, he launched a book on The Bitcoin Monetary Network .
Cryptocurrency in general is still not properly regulated in the country, as there are still no definite laws addressing this industry.
Recently, Atty. Rafael Padilla, the co-founder and trustee of BlockDevs Asia, professor of law at San Beda Alabang, and author of Fintech: Law and First Principles, published a paper in BitPinas , discussing how private ordering can lead to the organic integration of environmental, social, and governance (ESG) principles as a uniform commercial practice or lex mercatoria among bitcoin miners.
As early as 2021, the Bangko Sentral ng Pilipinas (BSP) and Securities and Exchange Commission (SEC) expressed that regulation of cryptocurrencies is different from encouraging people to adapt.
- Securities and Exchange Commission
Last March, SEC Commissioner Kelvin Lee revealed that the Commission had already created a regulatory framework for cryptocurrencies in order to offer more protection and security to investors in the Philippines; however, it was postponed due to the FTX collapse. Currently, the draft has not been released to the public.
On January 19, the SEC-PhiliFintech Innovation Office announced its partnership with the UP Law Center (UPLC) through the UP Legal Center Research Program through a memorandum of understanding.
- Bangko Sentral ng Pilipinas
Last year, the central bank urged the Senate to draft legislation that specifically addresses digital assets, including cryptocurrencies and non-fungible tokens (NFTs), in order to safeguard consumers. During a meeting with the Senate Committee on Banks, Financial Institutions, and Currencies and representatives from FintechAlliance.Ph, Binance, and the Cagayan Economic Zone Authority (CEZA), the BSP discussed the Digital Assets Act (Senate Bill 184) and Senate Resolution 126 concerning cryptocurrencies and digital assets.
Currently, the BSP keeps tabs on virtual asset service providers (VASPs) to ensure that users are only dealing with licensed and regulated entities. (Read more: List of Philippine Financial Institutions Both VASP and EMI Licenses )
This article is published on BitPinas: Bitcoin in the Philippines: Adoption, Regulation, and Use Cases
Disclaimer: BitPinas articles and its external content are not financial advice. The team serves to deliver independent, unbiased news to provide information for Philippine-crypto and beyond.
Shiela, a senior reporter at BitPinas, has been a part of the team since 2021. She specializes in local web3 news, all while crafting feature articles and delivering global crypto industry updates. Shiela holds a degree in broadcasting from the Polytechnic University of the Philippines.
Similar Posts
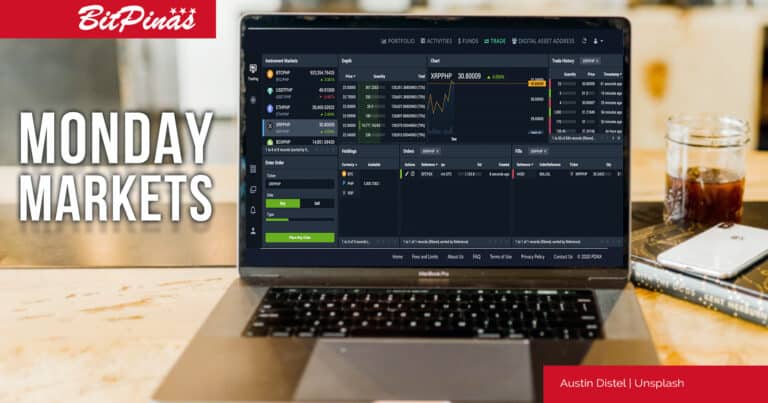
Wednesday Watch: Should Corporate Treasurers Consider Bitcoin?
I can imagine a stream of more bullish news in the coming days.
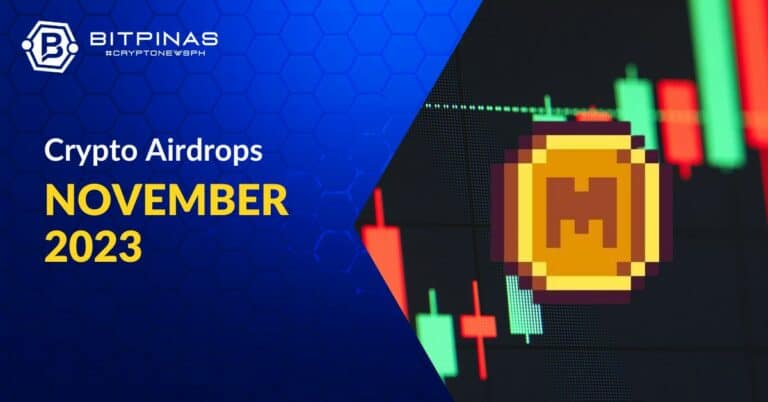
Top 5 Must-Participate Crypto Airdrops This November 2023
Discover the top 5 crypto airdrops this month and learn how to participate in these opportunities in a few clicks.
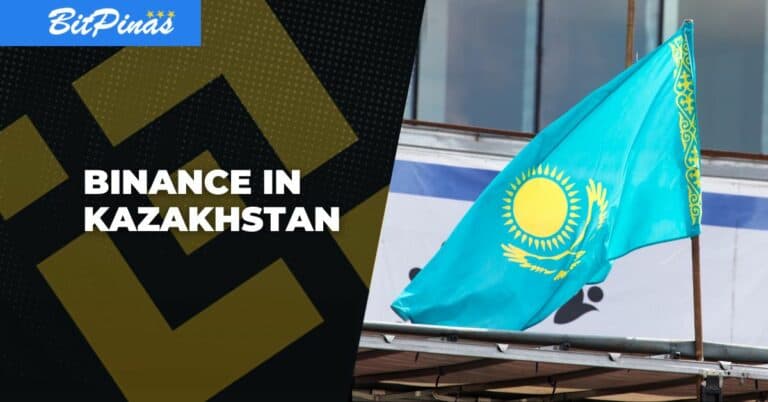
Binance Launches a Regulated Platform in Kazakhstan
Binance announces the launch of its digital asset platform in Kazakhstan, providing users with crypto exchange conversion.
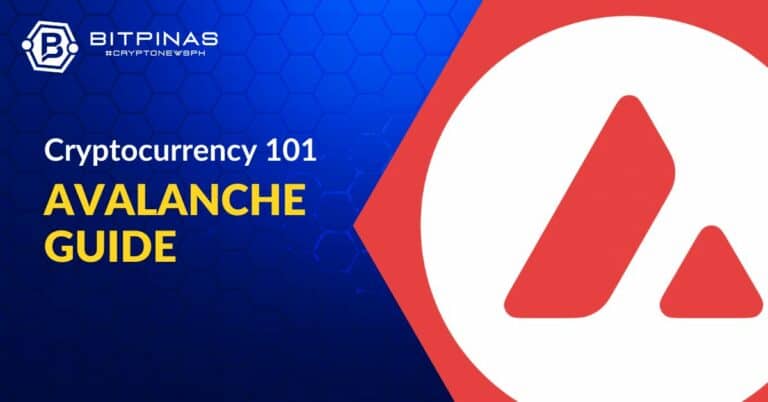
Avalanche | How to Buy AVAX in the Philippines
Avalanche’s network has subnets composed of three chains: C-chain, X-chain and P-chain. These chains stand for contract, exchange and platform. Later, these subnets will be discussed under use cases or utilities.
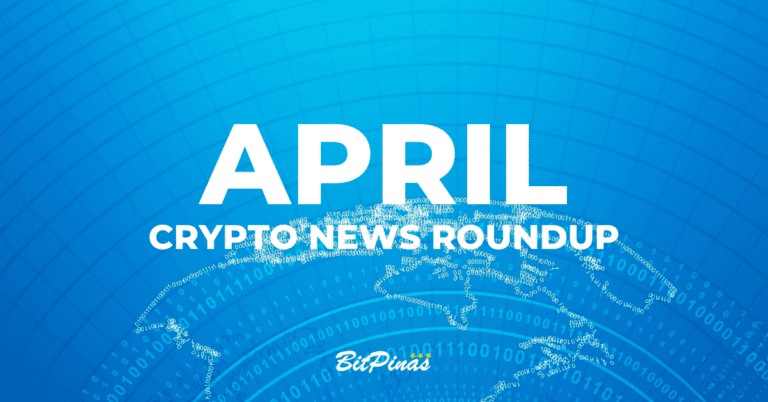
Philippines Crypto & Blockchain News Roundup: April 2023
Get the latest updates in Philippine crypto and blockchain news with our comprehensive roundup for April 2023!
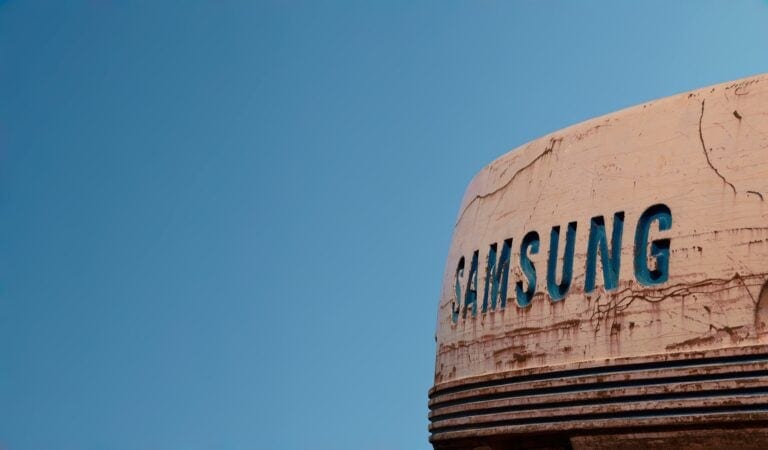
Samsung Blockchain Keystore Adds Bitcoin Support
Blockchain Keystore SDK is confirmed available for Galaxy S10e, S10+, S10 5G, Note10, and the Note10+ within specific regions.
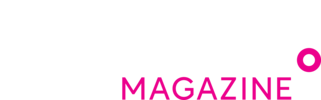
Cryptocurrency to become mainstream in the Philippines
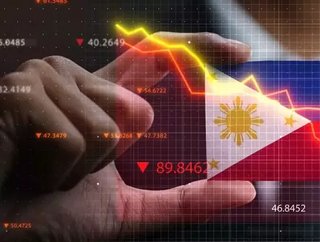
The Philippines is one of the world’s fastest adopters of cryptocurrency, new reports show. According to data, the pandemic has fuelled the trend, with Filipinos releasing the value of crypto as an investment and income generating currency.
A rise in crypto-friendly technology in the country has also facilitated it’s uptake, which means the Philippines is now on a par with Vietnam and Nigeria.
Reports by consensus experts from the Bangko Sentral ng Pilipinas (BSP) earlier this month show that gaming rewards, BTC, ATM networks and rural banking integration are ‘reliable early indicators’ for the upsurge that makes crypto growth in the Philippines the world’s third-fastest growing sector.
The The Fintech Philippines Association and European Chamber of Commerce of the Philippines have reported similar findings.
Bitcoin first became popular in the Philippines 2017, when its value skyrocketed from $1,000 to over $19,000 in the space of a few months. Since then, BSP has installed a reliable platform that protects crypto customers and also encourages bitcoin uptake, which is still in a nascent stage.
As of December 2020, BSP has also opened up channels with 17 virtual currency exchanges that are industry compliant.
Crypto currency adoption
Speaking about the swift adoption of cryptocurrency, Nichel Gaba , CEO and f ounder of Philippine Assets and Digital Exchange, said, “Between December 2020 and today, something happened to put bitcoin and crypto into a sace where even traditional portfolio managers need to consider them. We are living in interesting times in crypto,” he added.
Gaba pointed out the fintech industry in the Philippines was still at an evolving stage, and said that although crypto-supporting platforms were effective, the industry in general required more support from government and regulatory institutions.
He added that the mainstream position of cryptocurrency was close to fruition in the Philippines due to the swiftly evolving technological landscape which has seen PDAX enjoy a 70-fold increase in transactions and a 15-fold customer increase in less than a year.
BSP also recently launched a new security framework that protects cryptocurrency from being used in money laundering. Speaking about the changes, Melchor Plabasan , Technology Ris k and Innovation Supervision Director of BSP, pointed out that the use of crypto has revolutionised remittances and payments as well as opening up new fundraising opportunities.
He said, “Regulations are designed to protect the investing public. We have expanded their scope and strengthened the rules on consumer protection and cybersecurity controls.”
- Bitcoin Hits US$60,000: Is it set for an all Time High? Crypto
- How Significant is the BSVA’s Testing of Teranode? Crypto
- Bitcoin Reclaims US$1tn Valuation; the Bull Market is Here Crypto
- How Significant is SEC’s Approval of Spot Bitcoin ETFs? Crypto
Featured Articles
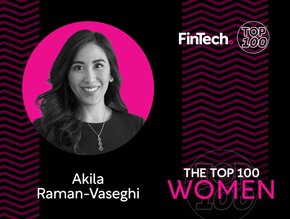
Top 100 Women 2024: Akila Raman-Vaseghi, Goldman Sachs No. 9
FinTech Magazine’s Top 100 Women in FinTech honours Goldman Sachs’s Akila Raman-Vaseghi at Number 9 for 2024 …
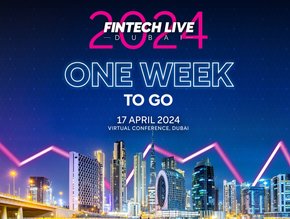
FinTech LIVE Dubai: 1 Week to Go!
Find out everything you need to know about FinTech LIVE: Dubai, the must-attend virtual event for fintech leaders in the Middle East and Africa …
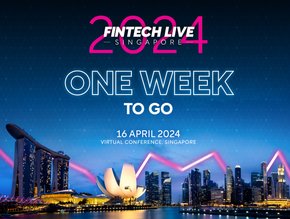
FinTech LIVE Singapore: 1 Week to Go!
With just one more week to go, find out everything you need to know about the must-attend virtual event for fintech leaders in Asia …
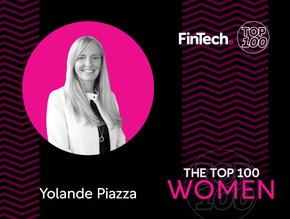
Top 100 Women 2024: Yolande Piazza, Google – No. 8
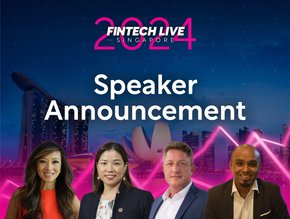
FinTech LIVE Singapore Announces Four New Speakers
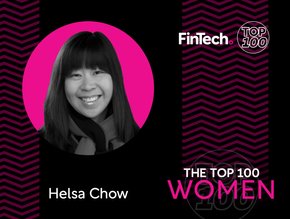
Top 100 Women 2024: Helsa Chow, SVP, Citi – No. 6
- Top 100 Women 2024: Kim Mason, Royal Bank of Canada – No. 5
- Top 100 Women 2024: Supreet Warna, HSBC – No. 4
- SUBMISSIONS OPEN: The Global FinTech Awards 2024
- Top 100 Women 2024: Lori Beer, JPMorgan Chase & Co. – No. 3
- Advent set to Acquire Nuvei in US$6.3bn Deal
Thank you for visiting nature.com. You are using a browser version with limited support for CSS. To obtain the best experience, we recommend you use a more up to date browser (or turn off compatibility mode in Internet Explorer). In the meantime, to ensure continued support, we are displaying the site without styles and JavaScript.
- View all journals
- My Account Login
- Explore content
- About the journal
- Publish with us
- Sign up for alerts
- Open access
- Published: 13 April 2024
Psycholinguistic and emotion analysis of cryptocurrency discourse on X platform
- Moein Shahiki Tash 1 ,
- Olga Kolesnikova nAff1 ,
- Zahra Ahani 1 &
- Grigori Sidorov 1
Scientific Reports volume 14 , Article number: 8585 ( 2024 ) Cite this article
Metrics details
- Computational science
- Computer science
- Human behaviour
- Scientific data
This paper provides an extensive examination of a sizable dataset of English tweets focusing on nine widely recognized cryptocurrencies, specifically Cardano, Binance, Bitcoin, Dogecoin, Ethereum, Fantom, Matic, Shiba, and Ripple. Our goal was to conduct a psycholinguistic and emotional analysis of social media content associated with these cryptocurrencies. Such analysis can enable researchers and experts dealing with cryptocurrencies to make more informed decisions. Our work involved comparing linguistic characteristics across the diverse digital coins, shedding light on the distinctive linguistic patterns emerging in each coin’s community. To achieve this, we utilized advanced text analysis techniques. Additionally, this work unveiled an understanding of the interplay between these digital assets. By examining which coin pairs are mentioned together most frequently in the dataset, we established co-mentions among different cryptocurrencies. To ensure the reliability of our findings, we initially gathered a total of 832,559 tweets from X. These tweets underwent a rigorous preprocessing stage, resulting in a refined dataset of 115,899 tweets that were used for our analysis. Overall, our research offers valuable perception into the linguistic nuances of various digital coins’ online communities and provides a deeper understanding of their interactions in the cryptocurrency space.
Introduction
How people employ words daily can reveal a wealth of information about their beliefs, fears, thought processes, social connections, and personal characteristics 1 . Nowadays, online social media platforms significantly affect human life, and people freely pen their thoughts on social networks 2 . Furthermore, the extensive use of social media platforms has been instrumental in spreading awareness about groundbreaking projects. The proliferation of digital technologies has been facilitated by the process of globalization 3 . Owing to cryptocurrencies’ digital character, wide-ranging conversations occur in online forums and on social media platforms, including X (formerly known as Twitter) and Facebook. These platforms serve as significant determinants of the prevailing sentiment among the general public regarding cryptocurrencies and to a certain extent, influence their market valuations 4 , 5 . Notably, these networks are home to a huge user base, encompassing billions of individuals and a vast, intricate web of interconnected relationships among them 6 .
On January 29, 2021, Elon Musk, the world’s wealthiest individual at that time 7 , took a surprising step by adding the hashtag #bitcoin to his X bio. This unexpected move triggered an outpouring of excitement and prompted a surge in cryptocurrency enthusiasts rushing to buy Bitcoin. Remarkably, this seemingly minor action had a significant impact, quickly driving the price of Bitcoin from around $32,000 to over $38,000, ultimately leading to a remarkable increase of $111 billion in the cryptocurrency’s market capitalization 8 .
The majority of data produced on social networks is unstructured, making it challenging to quantify. As a result, it is typically analyzed using various characteristic features 9 . In the above instance, we observed a significant role that social media plays in shaping the cryptocurrency market.
Prior research in the field of cryptocurrency and blockchain technology has explored a wide range of subjects and methodologies. For instance, scholars have utilized natural language processing (NLP) techniques to analyze various aspects such as miner extractable value (MEV) in social media discussions 10 . Similarly, others developed strategies for maximizing wealth through Initial Coin Offerings (ICOs) in blockchain ventures 11 . Additionally, there have been endeavors to predict cryptocurrency prices and investigate the societal ramifications of these emerging technologies in contemporary business environments 12 . However, very few studies have addressed the psycholinguistics and emotions associated with the discourse on cryptocurrencies in social media. To contribute to these existing avenues of inquiry, our study aims to bridge a significant gap in the literature. Specifically, we intend to conduct psycholinguistic and emotion analyses, alongside with assessing the readability of cryptocurrency comments on social platforms, with NLP methods. By adopting this innovative approach, we seek to get more knowledge of the psychological and emotional dimensions in cryptocurrency discourse, which have thus far received limited attention in scholarly research. Our objective in this endeavor is twofold: to enhance awareness among newcomers in digital marketing to prevent misguided investments, and to offer support to traders who rely on metrics such as the fear and greed index in their trading strategies.
We analyzed nine distinct digital coins using psycholinguistic methods to assist cryptocurrency enthusiasts. The cryptocurrencies examined in this paper encompass Bitcoin 13 , Ethereum 14 , Ripple 15 , Binance 16 , Dogecoin 17 , Shiba 18 , Fantom 19 , Matic 20 , and Cardano 21 . Psycholinguistics is the examination of how linguistic elements and psychological aspects are interconnected. It is important to emphasize that we did not consider user-specific characteristics; our primary focus was solely on textual data. To clarify, we utilized psycholinguistic attributes that often convey the underlying meaning communicated by text. The text analysis we conducted comprises the following categories:
1. LIWC (Linguistic Inquiry and Word Count) 22
2. Sentiment analysis 23
3. Emotion analysis 24
4. Assessment of readability 25 , 26 , 27 , 28
Concerning the features we used for our computerized text analysis, first, we employed subcategories of LIWC 29 . We utilized only a selection of such subcategories, including Analytical Thinking, Clout, Drives, Affect, Money, Hope, Attention, Netspeak, and Filler. This internal dictionary encompasses an extensive compilation of more than 12,000 words, word stems, phrases, and specific emoticons. Each dictionary entry is associated with one or more categories, or subdictionaries, strategically designed to evaluate a wide range of psychosocial constructs 22 .
Investors typically initiate an assessment of public sentiment surrounding a particular cryptocurrency before making investment decisions 30 . Consequently, sentiment and emotion analysis in cryptocurrency markets has gained significant prominence 31 . Research indicates that tweets expressing positive sentiments can exert a substantial influence on cryptocurrency demand, and conversely, negative sentiments can have a similar effect 32 , 33 .
Readability refers to the level of ease with which a piece of writing can be understood or comprehended, primarily influenced by its writing style and presentation 34 . Readability not only relates to how easily a text can be understood with respect to its writing style but also takes into account how well readers comprehend it, read it at an appropriate speed, and find it engaging 35 .
Moreover, we went a step further by investigating the “reasons and significance” aspect. In simpler terms, we sought to determine which characteristics among the aforementioned four hold more importance for novice investors. To accomplish this, we explored the following research questions.
RQ1: Do psycholinguistic characteristics vary among digital coins?
RQ2: What are the dominant feelings expressed by X users regarding the cryptocurrencies under study?
RQ3: Does the readability level of tweets exhibit uniformity across all selected digital currencies?
RQ4: Is there any co-mention among different cryptocurrencies?
To address these research inquiries, we analyzed tweets related to nine distinct cryptocurrencies. We conducted psycholinguistic investigations and emotion analysis to respond to RQ1, RQ2, and RQ3 and extracted the above categories of features from the dataset, including LIWC, Readability, Sentiment, and Emotions analysis. To answer RQ4, we established a co-mention among different cryptocurrency coins, identifying which two coins tend to be mentioned together more frequently.
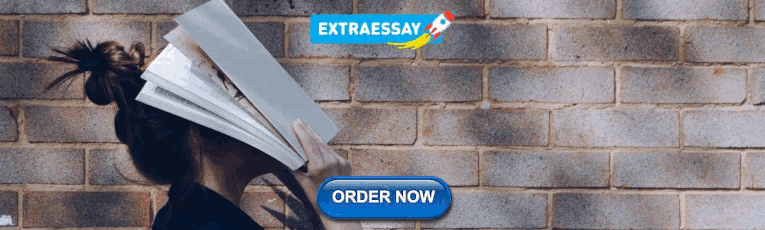
Related work
A cryptocurrency is a form of digital currency designed for use as a means of exchange. It relies on robust cryptographic techniques to secure financial transactions, regulate the creation of additional units, and validate asset transfers 36 . Because of their substantial market values, cryptocurrencies have gained considerable interest, with some individuals regarding them as legitimate currencies and others as attractive investment prospects 33 .
Sentiment and emotion analysis
Aslam et al. 37 focused on sentiment analysis and emotion detection in cryptocurrency-related tweets collected using specific hashtags such as ’#cryptocurrency’, ’#cryptomarket’, and ’#Bitcoin’, amassing a total of 40,000 tweets. The authors employed traditional feature extraction methods like Bag-of-Words (BoW), TF-IDF, and Word2Vec, along with machine learning models including Random Forest (RF), Decision Tree (DT), k-Nearest Neighbors (KNN), Support Vector Machine (SVM), Gaussian Naive Bayes (GNB), and Logistic Regression (LR). Additionally, they leveraged advanced deep learning techniques, specifically a combination of Convolutional Neural Networks (CNN) and Long Short-Term Memory (LSTM) networks, to classify tweet sentiments as positive, negative, or neutral. Notably, they introduced an ensemble model that merges LSTM and Gated Recurrent Unit (GRU) 38 , 39 , achieving remarkable accuracy scores of 99% for sentiment analysis and 92% for emotion prediction.
Research in Ibrahim et al. 40 centered on predicting early market movements of Bitcoin by harnessing sentiment analysis of X data 41 , 42 . The primary objective of their work was to introduce a Composite Ensemble Prediction Model (CEPM) built upon sentiment analysis. They employed a combination of data mining techniques, machine learning algorithms, and natural language processing to decipher public sentiment and mood states pertaining to cryptocurrencies. The research evaluated various models, such as Logistic Regression, Binary Classified Vector Prediction, Support Vector Machine, Naïve Bayes, and a single XGBoost 43 for sentiment analysis. The remarkable point to be highlighted is the CEPM’s outperformance of other approaches, demonstrating its effectiveness in forecasting early Bitcoin market movements via the analysis of sentiment in X data.
Shahzad et al. 44 presented a framework for performing sentiment analysis on X data with the aim of predicting the future price of Bitcoin. They highlighted the significance of NLP in bridging the gap between human communication and digital data and emphasized the growing importance of sentiment analysis in the field. The authors utilized three artificial intelligence tools, namely, LR, LSTM, and Deep Neural Network (DNN) Regressor to evaluate their performance in predicting Bitcoin prices. The best performance was demonstrated by the LSTM model.
Rahman et al. 45 explored the usage of various natural language processing models for sentiment analysis in the context of cryptocurrency and financial market prediction. They used a dataset of approximately 100,000 news items, including tweets and Reddit posts, gathered from 77 public X timelines and Reddit subreddits over a six-month period from July to December 2021. The study also examined the creation of ensemble models, encompassing all 22 selected models as well as a subset of the top three models labeled as “ensemble (all) “ and “ensemble (top 3) “, which included Aigents+, Aigents, and FinBERT. The “ensemble (top 3)” method exhibited a higher degree of correlation with other models compared to the rest.
Huang et al. 46 collected a substantial dataset comprising 24,000 cryptocurrency-related tweets and 70,000 comments from Sina-Weibo using specific keywords. The study adopted a methodology that utilized a training dataset consisting of posts from the top 100 crypto investor’s accounts on Sina-Weibo over the most recent seven days, while the subsequent day’s posts served as testing data. Remarkably, their sentiment analysis approach based on LSTM surpassed the time series auto-regression (AR) method by 87.0% in precision and 92.5% in recall.
The authors of 47 aimed to detect sentiment and emotion in X posts and utilized this information for recommendations. They used a dataset containing tweets and user data and manually annotated 7,246 tweets and replies. Their approach involved text preprocessing and applying a Naïve Bayes classifier with cross-validation. The findings demonstrated that analyzing the entire text provided superior accuracy compared to focusing on specific words (NAVA). Moreover, as the number of cross-validation folds increased, the accuracy showed improvement. Specifically, in the realm of emotion analysis, the Naïve Bayes classifier achieved an accuracy of 47.34%. Furthermore, in sentiment analysis, Naïve Bayes outperformed other classifiers significantly, attaining an accuracy of 66.86%.
The researchers in 48 utilized the AIT-2018 dataset 49 to construct a model for detecting emotions expressed in tweets. The dataset of tweets was acquired through the X API by extracting tweets containing emotion-related hashtags such as ’#angry’, ’#annoyed’, ’#panic’, ’#happy’, ’#love’, and ’#surprised’. The proposed model integrated lexical-based approaches, employing emotion lexicons like WordNet-Affect and EmoSenticNet, along with supervised classifiers to autonomously classify multi-class emotions from the dataset. The authors conducted experiments employing three machine learning classifiers: Naïve Bayes, DT, and SVM. Their findings demonstrated that when filtering tweets using EmoSenticNet words, the precision in detecting emotions significantly improved. Specifically, the SVM classifier achieved a high precision rate of 89.28% in the Anger class, surpassing previous results obtained using logistic regression.
Psycholinguistic analysis
Psycholinguistics utilizes various methods to comprehend language in the context of psychological processes. These methods encompass observational research, analysis, experimental studies, and the application of neuroimaging techniques 50 . Researchers also make use of text analysis models to interpret findings related to the language system. This section explores the methodologies employed by researchers in this field. Butt et al. 29 presented a comprehensive analysis of the psycholinguistic aspects of rumors on online social media (OSM). Using the PHEME dataset 51 , which encompasses nine breaking news events, the researchers examined source tweets (rumor and non-rumor) and response tweets. They integrated various psycholinguistic features, including LIWC, SenticNet 52 , readability indices, and emotions to uncover user behavior patterns. Rumor source tweets were found to be characterized by language related to the past, prepositions, and motivations associated with reward, risk, and power. In contrast, non-rumor source tweets exhibited affective and cognitive processes, present-oriented language, and motivations linked to affiliation and achievement. Emotional analysis revealed that non-rumor tweets tended towards neutrality, while rumor-source tweets evoked fear and grief, subsequently prompting anger and fear in reactions.
Narman et al. 53 reported an analysis of Reddit comments employing seven readability techniques to discern the education levels of users interested in eight cryptocurrencies. The data collection process involved gathering comments data from subreddits of eight cryptocurrencies by selecting ten to seventy top posts for each coin to collect distinct usernames. For education level information, they used Reddit.com to gather and categorize the collected comments data. The analysis was performed using seven text readability techniques. Interestingly, the results indicate that a majority, approximately 60%, possess an education level equivalent to middle school, with 30% at the high school level, while the remaining 10% span other educational levels.
The researches in 54 aimed to assess the readability of tweets for English language learners. Their task involved collecting a dataset of 14,659 tweets and obtaining readability judgments from participants representing different language groups. For methodology, they analyzed various linguistic and content-related factors in the tweets, including emojis, hashtags, mentions, and links, as well as traditional readability measures like Flesch Reading Ease and Dale-Chall scores. The results revealed that demographic factors, such as language proficiency and education, were stronger predictors of tweet readability than any other single feature.
The proposal in 55 included a framework to analyze linguistic features and cultural distinctions in climate-related tweets from the UK and Nigeria. A dataset of 81,507 English-language tweets was collected, comprising 44,071 from the UK and 37,436 from Nigeria. The study combined transformer networks with linguistic feature analysis, including the application of the (LIWC-22) software 56 ,version 15.0, to address small dataset limitations and identify cultural differences 22 . Findings reveal that Nigerians tend to use more leadership language and informal words in climate change discussions on X, emphasizing the urgency of the issue. In contrast, UK discourse on climate change is characterized by more formality, logic, and longer words per sentence. The study also confirmed the geographical attribution of tweets using DistilBERT 55 , achieving an 83% accuracy rate.
This section provides a detailed overview of the data acquisition processes we employed. We clarify the exact steps undertaken during preprocessing and explore the complexities of conducting co-mention analyses among various cryptocurrency coins.
Data collection
The data collection process commenced with the acquisition of X data pertaining to nine popular cryptocurrencies: Cardano, Bitcoin, Binance, Dogecoin, Ethereum, Fantom, Matic, Shiba, and Ripple 57 . These specific cryptocurrencies were selected for inclusion in the dataset due to their widespread usage across various research studies conducted by different scholars 18 , 19 , 20 , 58 , 59 . This endeavor yielded a substantial dataset comprising 832,559 tweets spanning from September 2021 to March 2023. After undergoing essential preprocessing steps, the dataset available for analysis was refined, resulting in a curated dataset consisting of 115,899 tweets. Table 1 presents dataset statistics both before and after preprocessing. Additionally, it lists the names of the coins and their respective symbols, which we utilized as keywords for extracting tweets from X. This extraction process was conducted separately for each coin, using both the name and the symbol as search criteria.
Data preprocessing
The utilization of the Tweepy 60 API was instrumental in our tweet data collection procedure, as it empowered us to filter tweets according to diverse criteria, including date, location, language, and various tweet attributes, for example, the number of retweets. In the final phase, we focused exclusively on English-language tweets, excluding unnecessary fields such as ’username’, ’id’, ’date’, ’likeCount’, and ’retweetCount’ retaining only the actual tweet content. After obtaining the dataset, we conducted a multi-step data preprocessing procedure to refine and enhance the data. This procedure involved the following key steps:
URL Removal: We applied a regular expression pattern to identify and subsequently remove any URLs. Text Cleaning: This step included the removal of special characters, such as punctuation marks, with the assistance of a designated dictionary of special characters. Additionally, we excluded words that had a length less than or equal to two characters. The result was a cleaned version of the text data.
Data labeling
In the process of data labeling, we examined each tweet systematically, with the primary objective of identifying any references to the selected cryptocurrencies. Notably, the search encompassed both “Bitcoin” and “Btc” in a case-insensitive manner, with any discovery leading to the classification of the tweet as Btc. This procedure was iteratively applied to all cryptocurrencies listed in Table 1 , encompassing both their complete nomenclature and associated abbreviations.
Further, we encountered instances where tweets discussed multiple cryptocurrencies simultaneously which was uncovered as co-mention among these cryptocurrencies. The results of this co-occurrence analysis are considered in Section 3.4. To tackle this challenge effectively, a comprehensive set encompassing the names of all pertinent cryptocurrencies was devised. For instance, to annotate tweets as of Bitcoin, tweets mentioning any other cryptocurrency present in this predefined set were systematically excluded. The set itself comprised a roster of cryptocurrency names, notably including “Cardano”, “Ada”, “Fantom”, “Ftm”, “Matic”, “Shiba”, “Shib”, “Dogecoin”, “Doge”, “Ripple”,“Xrp”, “Ethereum”, “Eth”, “Binance” and, “Bnb“.
Subsequently, the inclusion of both Bitcoin and Btc into this enumerated list facilitated the resolution of similar issues encountered with other cryptocurrencies, with the same process being replicated across each cryptocurrency to ensure comprehensive data labeling. As an example, in the dataset, a tweet was identified as featuring the keyword Matic. The content of the tweet is provided below: APompliano Good day sir, I have 100$ to invest in a coin right, small but what I can afford for now. So, Im thinking It I should rather go for $ada $matic, $doge What do you suggest fam.
This tweet was acquired using the keyword Matic, and the keywords to be examined for the Matic coin included:’Cardano’, ’Ada’, ’Fantom’, ’Ftm’, ’Bitcoin’, ’Btc’, ’Shiba’, ’Shib’, ’Dogecoin’, ’Doge’, ’Ripple’, ’Xrp’, ’Ethereum’, ’Eth’, ’Binance’ and, ’Bnb’. The exclusion criterion described above ensured that if any of these keywords were present in a tweet, except for the keywords related to the specific coin for which we used keywords to extract tweets, that tweet should be removed. In our example, it’s evident that the tweet contains both ’ada’ and ’doge’ keywords, indicating that it should be removed.
Figure 1 illustrates the processing steps for a tweet.
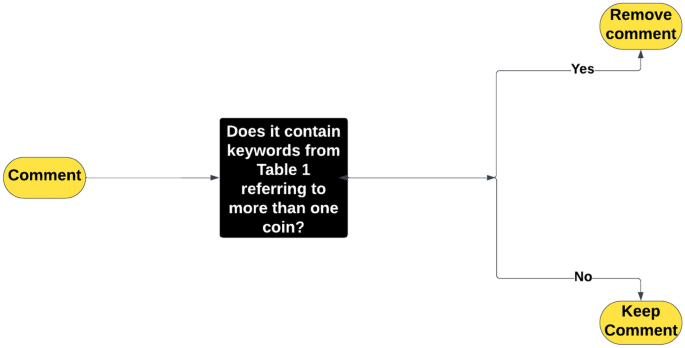
Example tweet processing.
Cryptocurrencies co-mention
During the labeling process, we examined co-mention and co-occurrence among various cryptocurrencies in tweets. Such analysis resulted in an interesting observation: multiple cryptocurrencies often co-occurred in the same tweets, indicating a significant level of co-mention, which led us to reconsider our labeling model, as previously detailed.
In this section, we explore specific, noteworthy co-mentions among cryptocurrency coins. These co-mentions provide valuable information for our investigation, enhancing our understanding of the relationships and trends emerging in the cryptocurrency ecosystem as reflected in social media discourse. The co-mention matrix provided in Table 2 serves as a tool for assessing the relationships between different cryptocurrencies, particularly concerning their trends and market dynamics, as opposed to a sole focus on price movements. An illustrative example lies in the substantial positive co-mention of Bitcoin (Btc) and Ethereum (Eth) in 53.52% of tweets. This significant co-occurrence indicates that when Bitcoin undergoes an upward trend, or garners increased market attention, Ethereum frequently follows suit. This co-occurrence can be attributed to the prominent positions both cryptocurrencies occupy in the market, as well as to their substantial influence on overall market sentiment.
In contrast, co-mention values nearing 0% in Table 2 signify a lack of substantial co-occurrence among cryptocurrencies. This absence of mention underscores the potential for diversification strategies for designing a cryptocurrency portfolio. Cryptocurrencies exhibiting low or negative co-mention can be strategically employed to diversify a portfolio, potentially resulting in reduced overall portfolio risk. Conversely, cryptocurrencies demonstrating high positive co-mention may offer limited diversification benefits as they tend to move in sync with one another.
Inside the domain of cryptocurrency portfolio management and risk mitigation, these co-mention observations underscore the critical importance of accurate asset selection and allocation, especially in light of the observed co-mention among cryptocurrencies. Such strategic decision-making becomes paramount in achieving diversified and risk-optimized cryptocurrency portfolios.
In this research, we examined cryptocurrency data, concentrating on a specific group of cryptocurrencies. Our choice of these particular coins was driven by their significant popularity among users, as well as the limited availability of substantial data for other coins. To interpret the data, we applied four analytical methods explained in section " Introduction ". Here we present the outcomes of our analysis for each of the aforementioned cryptocurrencies. The selection of features was made considering their past influence 29 , 61 . In the analysis conducted, LIWC assessments were applied to nine cryptocurrencies, resulting in an extensive collection of nine distinct analyses. We selected values that were highly informative for extracting linguistic interpretations relevant to cryptocurrencies. Our choice was made to capture key aspects of sentiment, linguistic style, and thematic content pertinent to discussions around cryptocurrencies. By narrowing down our focus to these particular features, we aimed to mine information from the psychological and linguistic dimensions of cryptocurrency discourse, thus aligning analysis with our goals. these categories encompass analytical thinking (metric of logical, formal thinking), clout (language of leadership), drives (related to personal motivations and psychological desires), affect (linguistic expressions associated with emotional and affective states expressed by a given text), money (refers to a set of linguistic cues or indicators related to financial terms, wealth, and economic aspects, Want (a human ability that allows individuals to envision future events with flexibility), attention (crucial subset of the “Perception” category), netspeak (represents a subset of the conversational category) and filler (non-essential sounds, words, or phrases, commonly used in speech to fill in pauses and maintain the flow of conversation without altering its meaning). In the drives and affect categories, additional features will be elaborated upon in the following discussion. Our examination indicated that Fantom attracts a larger number of tweets centered on technical aspects and holds a higher level of trust in comparison to other cryptocurrencies. For Binance, our observations revealed that the tweets predominantly revolve around themes of affiliation, achievements, and the pursuit of power and wealth. This pattern in discussions on Binance suggests a focus on notable accomplishments and financial success, indicative of a unique narrative and sentiment surrounding the coin. For Matic, the tweets primarily center around emotional impact compared to other cryptocurrencies. This emphasis on affective responses suggests that the coin is particularly influenced by emotional novelty. This distinctive characteristic could be considered a contributing factor to the fluctuations in the coin’s price, as emotional sentiment plays a significant role in shaping market dynamics and investor behavior. Our analysis revealed that Dogecoin exhibits a higher prevalence of netspeak, the informal language commonly used on the internet, compared to other cryptocurrencies. Conversely, Ethereum appears to attract more attention relative to other coins. This distinction suggests that Dogecoin is characterized by a more casual and internet-centric communication style, while Ethereum stands out for its ability to capture increased Attention and interest. A deeper understanding of the communication dynamics and community sentiment surrounding different coins may aid investors in making more informed choices, aligning their investment strategies with the unique qualities and trends associated with each cryptocurrency. From an emotional perspective, most cryptocurrencies exhibit a generally moderate and harmonious emotional profile. Notably, there is a distinct focus on the emotional category of Anticipation, with Dogecoin taking the forefront in this aspect. In this context, Anticipation likely signifies the expectation or excitement surrounding the future prospects, developments, or events associated with these cryptocurrencies.The outcomes of our analysis are presented in Table 5 . In terms of readability, the analysis revealed that Dogecoin’s tweets are relatively more challenging to read and comprehend, as indicated by lower scores on the Flesch Reading Ease measure. The Flesch-Kincaid and Dale-Chall Measures suggest an average reading difficulty level akin to content tailored for college graduates. Conversely, Ethereum’s tweets, as per the Gunning Fog Index, demand a higher level of reading proficiency, indicating a more complex and advanced readability suitable for individuals with a college-level education and vocabulary. To explore additional results, refer to Figs. 5 and 6 s, as well as Table 6 .
The LIWC model revolutionized psychological research by making the analysis of language data more robust, accessible, and scientifically rigorous than ever before. LIWC-22 examines over 100 textual dimensions, all of which have undergone validation by esteemed research institutions globally. With over 20,000 scientific publications utilizing LIWC, it has become a widely recognized and trusted tool in the field 62 giving way to novel approaches in analysis 63 , 64 . Although LIWC provides several benefits, it has its limitations. One drawback is its dependence on predefined linguistic categories, which might not encompass nuances and variations present in natural language. Furthermore, LIWC may encounter challenges in accurately deciphering sarcasm, irony, and other subtle forms of language usage, potentially resulting in text misinterpretation.
To effectively convey the outcomes of our analysis, average values among all the tweets were computed for each of LIWC categories. Averages can help identify broadscale sentiment trends over time. By tracking changes in average scores across key linguistic categories, such as sentiment, emotion, or cognitive processes, one can observe shifts in user sentiment and attitudes towards cryptocurrencies, market developments, or external events. Therefore, the average was calculated by summing up the scores of all comments related to each coin for each LIWC feature and then dividing by the total number of comments for that coin. These computed averages provide information along the linguistic and psychological dimensions intertwined with the selected digital currencies. A comprehensive presentation of these average values for each category can be found in Table 3 .
Analytical thinking and clout
Analytical Thinking, when showing high numerical values, signifies a formal, logical, and hierarchical thought process. Conversely, lower numbers suggest a more informal, personal, present-focused, and narrative style of thinking 65 . The values of this category computed for tweets related to cryptocurrency, reach their highest average score of 67.76 in texts mentioning Fantom. This fact indicates that, on average, discussions in this domain exhibit a relatively high level of logical and formal thinking. Conversely, the lowest average score of 52.00 was found for Ripple, which might suggest that discussions concerning this particular cryptocurrency place slightly less emphasis on logical and analytical thinking compared to the cryptocurrency domain’s average.
Clout is one of the four summary variables in LIWC designed to assess the degree of confidence and certainty conveyed in the text 66 , 67 . Our analysis revealed that the cryptocurrency Fantom exhibits a relatively high Clout score, with an average result of 70.91. This suggests that discussions and conversations related to Fantom often convey a strong sense of confidence and certainty. This high Clout score may also indicate a substantial degree of assurance in Fantom stability. In contrast, the cryptocurrency Ripple demonstrates a comparatively lower Clout score with an average result of 43.39. Figure 2 presents a comparative evaluation of Analytical Thinking and Clout scores across different cryptocurrencies. This suggests that discussions related to Ripple may not consistently display the same level of confidence and certainty found in the Fantom discussions. In essence, when Fantom demonstrates higher Clout values, it signifies that the users who composed the tweets are expressing increased confidence. This, in turn, leads us to infer a heightened level of knowledge on their part. In both analyses, we observed that Fantom consistently had the highest scores, indicating a higher level of analytical thinking and confidence in discussions related to it. Conversely, Ripple consistently had the lowest scores in both categories, suggesting a relatively lower emphasis on analytical thinking and a lower degree of expressed confidence in discussions related to it. While these observations suggest a correlation between analytical thinking and confidence in these specific cryptocurrency discussions, it’s important to note that correlation does not imply causation. Other factors, such as market conditions, community sentiment, and news events, can also influence these results. For example, when we examined Binance, we foound that it ranks as the second-highest in terms of Analytical Thinking scores among the various cryptocurrencies. However, when we assess it as the position in the Clout category, Binance ranks fifth. The results of Analytical Thinking and Clout analysis related to digital currencies can be viewed in Table 3 .
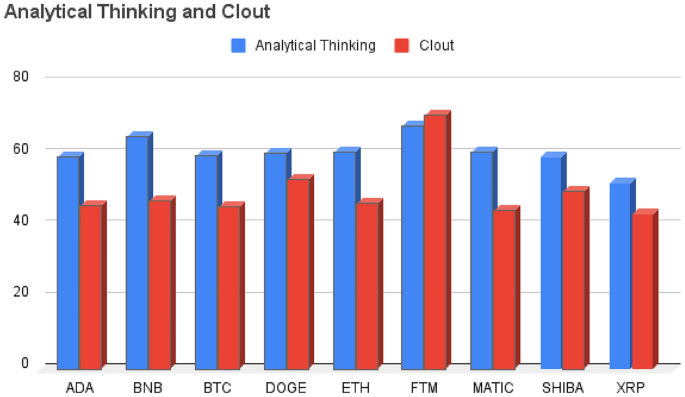
Comparative evaluation of analytical thinking and clout scores across different cryptocurrencies.
Drives and affect
Drives is a comprehensive dimension that encapsulates various needs and motives 65 . In our LIWC analysis, we concentrated on the Drives, particularly examining the aspects of Affiliation, Achievement, and Power. We observed that the presence of Affiliation-related language (such as “us” and “help“) is comparatively lower in discussions related to Cardano, while it appears more frequently in conversations about Dogecoin. Similarly, in terms of Achievement-related language (including “work”, “better”, and “best“), Dogecoin tends to have fewer instances compared to Matic. Furthermore, when examining Power-related language (like “allow” and “power“), we found that Dogecoin exhibits a lower frequency, while Bitcoin discussions tend to feature a greater occurrence of such language. These patterns highlight variations in linguistic expressions across different cryptocurrencies, shedding light on the distinctive characteristics of discussions over different digital coins. Upon closer examination, it became evident that tweets originating from Binance sources tended to include a higher frequency of words associated with Drives, whereas Fantom source tweets had a notably lower occurrence of Drives-related words. Additional details can be found in Fig. 3 .
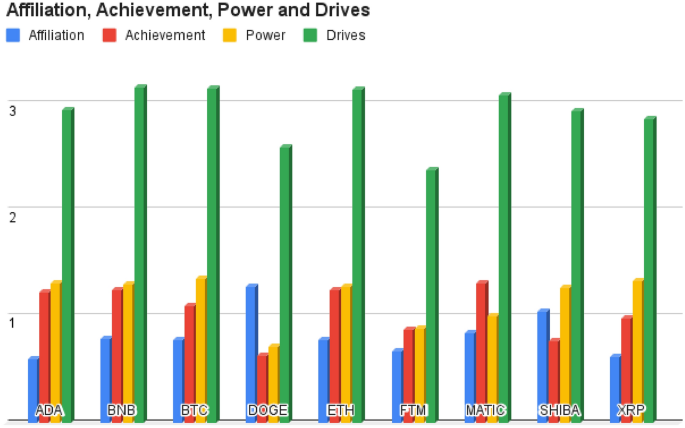
Frequency of language associated with affiliation, achievement, power, and drives across different cryptocurrency discussions.
In the Affect 1 subset, our analysis encompassed various emotional dimensions, including Positive Emotion, Negative Emotion, Anxiety, Anger, Sadness, and Swear Words. In the upcoming Emotion section, we delve deeper into affective analysis. However, in this preliminary report, we provide an overview of the affective processes observed in the LIWC analysis. It can be observed in Table 3 that there is a variation in affective (good, well, new, love) content among different cryptocurrencies. Notably, Matic coin exhibits a higher level of affective language, while Ada appears to have a lower level. This distinction becomes clearer when we explore the affective subcategories including Positive tone (new, love), Negative tone (bad, wrong, too much, hate), Emotion (good, love, happy, hope), and Swear words (shit, fuckin*, fuck, damn), as depicted in Fig. 4 . It becomes evident that Matic coin scores higher in Positive tone and Emotion, while Bitcoin registers a higher Negative tone. Additionally, Ripple stands out with a higher score in Swear words, indicating potential user dissatisfaction. When we further break down the Emotion category into its subsets, which encompass Anxiety (worry, fear, afraid, nervous), Anger (hate, mad, angry, frustr), and Sadness (sad, disappoint, cry), we notice that Dogecoin exhibits a higher score in Anxiety, Ripple in Anger, while most of the nine analyzed coins show similar values for Sadness. These observations contribute to our analysis and highlight the varying affective language usage across different cryptocurrencies, which we explore in greater detail in the subsequent Emotion section.
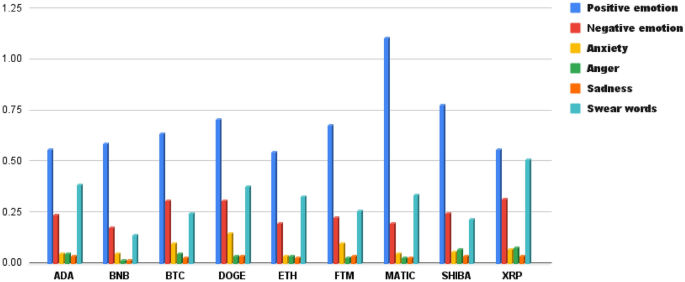
Comparative analysis of affective language dimensions-positive tone, negative tone, emotion, and swear words-across different cryptocurrencies.
Want words signify the author’s desires or preferences. Typically, wants are philosophically differentiated from needs by conceptualizing needs as innate and essential for survival, while wants are learned and generally linked to additional satisfaction beyond basic necessities 68 . What is important for cryptocurrency analysis in this category is the aspect of hope (want, hope, wanted, wish) as Want, or Hope, is a remarkable human ability that allows individuals to envision future events and their potential outcomes with flexibility 69 . Many users have high hopes for the future of cryptocurrency, anticipating greater benefits from their investments. From Table 3 , it becomes evident that Shiba is the cryptocurrency that garners most hope among users. The range of hope scores falls between 0.19 and 0.41, with the lowest level of hope associated with Fantom. This data suggests that Shiba is particularly promising in the eyes of cryptocurrency enthusiasts, while Fantom elicits comparatively less optimism.
Another important LIWC category is Money (business, pay, price, and market) 22 . The range of Money scores, from 2.46 for Shiba to 10.51 for Binance, indicates varying degrees of discussion or emphasis on cryptocurrency financial aspects. Notably, Binance stands out with the highest score, suggesting a significant emphasis on business and financial aspects in discussions related to this coin. Conversely, Shiba has the lowest score, indicating relatively less emphasis on these financial terms in conversations related to it. These findings offer a glimpse into the importance placed on financial and business-related aspects and potentially shed light on the perception and use of the cryptocurrencies in the broader context of market and economy.
At the dawn of experimental psychology, William James wrote that everyone knows what attention is. It is the taking possession by the mind, in a clear and vivid manner 70 . When users include the term Attention in their tweets, it signifies their intention to draw focus to a significant event or topic. Upon reviewing Table 3 , it becomes evident that Ethereum tweets receive more attention than tweets about the other cryptocurrencies, indicating a heightened interest or emphasis on Ethereum-related matters. Conversely, tweets concerning Dogecoin appear to attract less attention when compared to tweets about the other coins, suggesting a relatively lower level of interest or engagement in discussions related to it. For Shiba, our observations indicate a prevalent sense of hope and an increased use of filler words compared to the other cryptocurrencies. This heightened expression of hope suggests a more optimistic sentiment surrounding Shiba when contrasted with the other coins. Additionally, the frequent use of filler words, including expressions like “wow”, “sooo”, and “youknow” signifies a more conversational and engaged discourse. This linguistic pattern may reflect a greater level of enthusiasm and interaction among Shiba enthusiasts.
Netspeak and filler
This analysis includes words commonly used in social media and text messaging, such as “bae”, “lol” and basic punctuation-based emoticons like “:)” and “;)” 65 , 71 . This mode of communication is widely employed by netizens during computer-mediated communication (CMC). In the context of cryptocurrency discussions, which predominantly transpire on online forums, social media platforms, and chat groups, it is customary for participants to incorporate netspeak into their interactions. Through the analysis of netspeak, researchers can understand more the degree of user engagement and interaction. Notably, the adoption of terms such as “HODL” (a deliberate misspelling of “hold”, indicating a long-term investment strategy) or “moon” (indicating an expectation of significant price increases) serves as meaningful pointers to user sentiment and active participation in discussions. In the obtained results, Matic stands out prominently with a notably high netspeak score, signaling the prevalence of internet-specific expressions and informal language related to it. The results can be found in Table 3 . Fillers (wow, sooo, youknow) are non-essential sounds, words, or phrases, such as “well”, “erm” or “hmm” commonly used in speech to occupy pauses and maintain the flow of conversation without altering its meaning 65 , 72 , 73 . The filler analysis results highlight that Shiba and Dogecoin exhibit higher scores in this category compared to the other cryptocurrencies, with scores ranging between 0.02 and 0.04 for the remaining coins, as depicted in Table 3 . In the sentiment analysis, it’s clear that Fantom distinguishes itself with a notably elevated positive score in comparison to the other cryptocurrencies. A consistently positive sentiment can enhance investor confidence, attract new stakeholders, and contribute to a more favorable market perception. Table 3 presents the remaining outcomes for the other cryptocurrencies.
Sentiment and emotions analysis
Table 4 provides a detailed sentiment analysis, encompassing positive, neutral, and negative percentages for various digital coins. In the world of cryptocurrency investments, it’s common for investors to assess public sentiment before making their decisions, as highlighted in prior research 30 . Consequently, sentiment analysis has gained substantial importance on cryptocurrency markets 74 . Studies have shown that tweets expressing positive emotions wield substantial influence over cryptocurrency demand, while negative sentiments can have the opposite effect 32 , 33 .
Analyzing the data in Table 4 , it becomes apparent that Fantom distinguishes itself by displaying a notably higher positive sentiment percentage in comparison to its digital counterparts, which strongly suggests an elevated degree of interest and enthusiasm among investors towards this digital coin.
Examining opinions involves another aspect known as emotion detection. In contrast to sentiment, which can be positive, negative, or neutral, emotions offer richer categorization over personality traits by revealing experiences of joy, anger, and more. Automated methods for emotion detection have been developed to enhance the analysis of individual sentiments. The primary goal of emotion analysis is to identify the specific words or sentences conveying emotions 75 . To achieve such analysis, we employed the NRCLex library to extract and categorize emotions from text 24 . NRCLex is a Python library designed for natural language processing and sentiment analysis. The acronym stands for “Natural Resources Canada Lexicon”, and it is particularly focused on assessing sentiment in text based on word associations. NRCLex is built upon a lexicon that assigns sentiment scores to words, allowing users to analyze the sentiment of individual words, sentences, or entire documents 76 . Table 5 provides the outcomes of our emotion analysis, revealing a narrow range of results for various emotions: Anger (0.02-0.04), Surprise (0.01-0.02), Sadness (0.01-0.03), Disgust (0.01-0.02), and Joy (0.02-0.04). These consistent findings suggest that most of the coins evoke similar emotional responses, highlighting their emotional proximity.
In contrast, when it comes to emotions such as Fear and Trust, there are more noticeable differences between the coins. For instance, when examining the sentiment of Cardano, the fear score is 0.0324, while the trust score is higher at 0.1252. Similarly, for Ripple, the fear score is 0.0416, with a trust score of 0.1172. The scores provide a difference in the emotional tones associated with these cryptocurrencies, indicating the levels of fear and trust expressed in the analyzed content.
Furthermore, the emotion of Anticipation stands out with higher scores in tweets, indicating that many users are keen on anticipating the future of these coins. Notably, Dogecoin (0.3752) and Shiba (0.3467) generate more anticipation among users when compared to the other coins.
Readability
In this section, we pay attention to the readability of data, utilizing metrics such as the Flesch Reading Ease 25 , Flesch-Kincaid Grade Level 26 , Gunning Fog Index 27 , and Dale-Chall Readability Score 28 . Assessing readability helps distinguish between text that is straightforward to grasp and text that is complex and demands a high level of education or intelligence to comprehend. Numerous readability metrics exist for text evaluation, and we have chosen to employ the above four measures as the most widely recognized tests to assess tweets.
Table 6 presents the significant differences in readability scores across tweets related to nine different digital coins.
The Flesch Reading Ease score provides an indication of how easily a text can be understood, with higher scores indicating greater readability. Flesch Reading Ease score can be observed in Fig. 5 . The Flesch-Kincaid Grade Level is a metric that estimates the educational grade level required to understand a piece of text based on factors like sentence length and word complexity. Analyzing the readability scores for the tweets related to each digital coin shows the linguistic complexity employed in discussions surrounding these coins. The presence of significant differences in readability scores suggests variations in the accessibility and comprehension levels required to engage with these tweets. Negative scores in some readability metrics, such as the Flesch Reading Ease and Flesch-Kincaid Grade Level, indicate higher levels of complexity, while positive scores indicate greater ease of comprehension. Refer to Fig. 6 for the necessary details to assess the readability levels of the specified analyses (Flesch-Kincaid Grade Level, Gunning Fog Index, Dale-Chall Readability Score). Table 6 provides evidence on the fact that Dogecoin possesses a notably lower score in Flesch Reading Ease compared to the other cryptocurrencies, which suggests that the communication pertaining to Dogecoin might present hurdles in accessibility and comprehension for the typical reader. Getting rid of such readability obstacles have the potential to amplify the effectiveness of communication, expand audience involvement, and cultivate heightened comprehension and acceptance of cryptocurrencies among varied stakeholders. This observation aligns with Fig. 5 77 , where we notice a pronounced level of complexity in comprehending tweets related to Dogecoin. To gain a better understanding of the varied readability levels, it’s essential to consider both Fig. 5 78 , 79 and Table 6 . When examining the Flesch-Kincaid Grade Level and Dale-Chall Readability in Table 6 , Dogecoin emerges with higher values compared to the other cryptocurrencies, signifying an average grade level and a college reading level, respectively. Furthermore, an examination of the results pertaining to the Gunning Fog Index, as depicted in Table 6 and Fig. 6 , reveals that Ethereum stands out with a higher score. This observation implies that understanding tweets related to Ethereum requires a reading comprehension level equivalent to a college education.
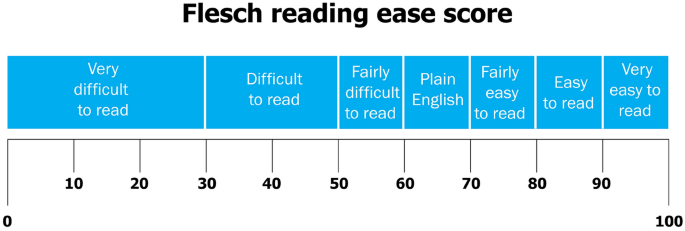
Flesch reading ease score.
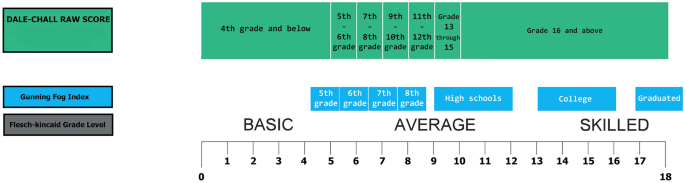
Dale-Chall Readability Score, Gunning Fog Index, Flesch-Kincaid Grade Level.
In the process of labeling our data, we identified a notable co-mention among various cryptocurrencies. We resolved this issue by excluding tweets that mentioned more than one coin or used abbreviations for coins not relevant to our research. Consequently, we focused our analysis on the specific set of cryptocurrencies pertinent to our study.
During the utilization of the LIWC software in our analysis, it became apparent that not all its components contributed substantively to the objectives of our research. In light of this, we exercised judicious discernment in the curation of our selection, focusing exclusively on those specific LIWC analyses that bore direct relevance to the concerns and interests of researchers, investors, and individuals engaged in the realm of digital marketing. Additionally, we imposed constraints on our scrutiny of emotional aspects, as certain LIWC framework components exhibited redundancy with one another.
When comparing the Analytical Thinking and Clout aspects to other LIWC features, we could see that these two scores are higher across all coins. This suggests that the tweets generally lean toward logical and formal thought. Notably, among all the coins, Fantom has the highest scores in these aspects, indicating that discussions regarding it are particularly characterized by logical and formal thinking compared to the other cryptocurrencies. After conducting a LIWC analysis, it became apparent that the highest scores were associated with two features that we considered above with concepts such as money, personal drives, and emotional affect. Furthermore, concerning the category of money and personal drives, Binance displayed notably higher scores compared to the other cryptocurrencies. Notably, Matic exhibited significantly higher levels of emotional affect when compared to the other cryptocurrencies. In contrast, other features such as Hope, Attention, Netspeak, and Filler exhibited remarkably low scores, nearly hovering around one percent, when compared to the features we previously discussed. This suggests that the tweets of users are primarily centered on analytical thinking, clout, personal drives, emotional affect, and financial matters. In the sentiment analysis, Fantom stands out with a higher positive score when compared to the other cryptocurrencies. On the contrary, Ripple registers a significantly elevated negative score. This information suggests that Fantom is generating a higher level of positive sentiment, possibly due to positive news, community sentiment, or price performance, while Ripple is experiencing more negative sentiment, which could be linked to negative news or market sentiment surrounding the coin. Emotionally, the majority of cryptocurrencies displayed a relatively modest and well-balanced emotional profile. Interestingly, there was an emphasis on the emotional category of Anticipation, and in this aspect, Dogecoin took the lead. Anticipation in this context likely refers to the expectation or excitement surrounding the future prospects, developments, or events related to these cryptocurrencies. The reason could be upcoming upgrades, partnerships, or any other factors that create a sense of anticipation among the cryptocurrency community. Regarding readability as assessed by Flesch Reading Ease, Dogecoin’s tweets scored lower on this measure. This implies that the content related to Dogecoin is relatively more difficult to read and comprehend, as its language and sentence structure are complex. Concerning Flesch-Kincaid and Dale-Chall measures, Dogecoin’s tweets received higher scores on these measures, indicating that the reading difficulty is at an average level, similar to what one might expect from a college graduate. While the Flesch-Kincaid measure estimates the U.S. grade level needed to understand the text, the Dale-Chall measure also assesses reading difficulty and is often used as a more accurate indicator for texts aimed at older audiences. Speaking of the Gunning Fog Index, Ethereum’s content, on the other hand, registered higher scores on this measure, implying a need for college-level reading proficiency. This means that content related to Ethereum is more challenging to read and understand, requiring a higher level of education and vocabulary.
Limitations
One significant challenge encountered during the data collection phase revolved around sentences containing references to multiple cryptocurrencies. Deciphering the intended cryptocurrency from such sentences posed a considerable complexity, leading to inaccurate analysis for each coin. Therefore, these data were excluded for a more precise analysis of psycholinguistics and emotions for each coin. Additionally, the sheer volume of data presented logistical hurdles, rendering manual labeling impractical in terms of both time and financial resources.
Moreover, the dynamic nature of the cryptocurrency landscape poses another limitation, as sudden events or developments can influence user comments and sentiments, leading to shifts in behavior and sentiment. For instance, specific events like the collapse of Terra Luna or Celsius in 2022 80 , 81 , led to significant market price decreases. Despite efforts to mitigate these impacts through regular monitoring and updates, the inherent volatility of the cryptocurrency market presents challenges in maintaining the consistency and relevance of the dataset over time.
These limitations underscore the necessity for a cautious interpretation of the study’s findings. Future research endeavors in this domain should strive to address such methodological challenges through enhanced data collection techniques and strategies tailored to the dynamic nature of cryptocurrency discourse.
Conclusions and future work
This paper presents a substantial dataset of English tweets related to cryptocurrencies, which were labeled using cryptocurrency keywords and abbreviations (e.g., ADA for Cardano, Ftm for Fantom, Matic for Matic, Btc for Bitcoin, Shib for Shiba, Dogecoin for Doge, Xrp for Ripple, Eth for Ethereum, and Bnb for Binance). Initially, we collected 832,559 tweets, which were reduced to 115,899 tweets after preprocessing. These tweets span from September 2021 to March 2023 and pertain to nine digital coins, namely Cardano, Bitcoin, Binance, Dogecoin, Ethereum, Fantom, Matic, Shiba, and Ripple.
This study conducted psycholinguistic and sentiment analyses on this dataset, utilizing tools such as LIWC, Emotion, Sentiment, and Readability analysis. To avoid LIWC framework redundancy, constraints were applied to the examination of emotional aspects. Our analysis revealed distinct linguistic characteristics and sentiment patterns associated with various cryptocurrencies.
Our investigation into the psycholinguistic characteristics of digital coins showed notable variations among different cryptocurrencies. Through detailed analysis of tweets related to nine distinct digital currencies, we discerned prevalent sentiments expressed by users, assessed the consistency of readability levels across various coins, and identified co-mention between different cryptocurrencies. Leveraging techniques such as psycholinguistic investigations, emotion analysis, and co-mention studies, we obtained valuable estimation of users’ perceptions and interactions.
In a broader context, our study revealed significant psycholinguistic differences among cryptocurrency data. We observed variations in sentiment and emotion analyses, as well as disparities in the readability levels associated with different cryptocurrencies. In future research, we aim to diversify our analysis techniques to delve deeper into the psychological aspects of cryptocurrency discourse. Specifically, we plan to explore sentiments of hope 69 , 82 and regret 83 in textual data using various Natural Language Processing (NLP) methodologies. Additionally, we intend to leverage Large Language Models (LLMs) to conduct psycholinguistic analyses, with an expectation to a deeper analysis of underlying linguistic patterns and emotional tones present in cryptocurrency discussions. Furthermore, our future work will involve classification algorithms with diverse machine learning approaches to distinguish bullish and bearish sentiments in comments, utilizing manual labeling for training data.
Data availability
The datasets generated and/or analysed during the current study are available in the GitHub repository, https://github.com/moeintash72/cryptocurrency-data- .
Pennebaker, J. W., Boyd, R. L., Jordan, K. & Blackburn, K. The development and psychometric properties of liwc2015 (Tech Rep, 2015).
Balouchzahi, F., Sidorov, G. & Shashirekha, H. L. Fake news spreaders profiling using n-grams of various types and shap-based feature selection. J. Intell. Fuzzy Syst. 42 , 4437–4448 (2022).
Article Google Scholar
Blanco-González-Tejero, C., Cano-Marin, E., Ulrich, K. & Giralt-Escobar, S. Leveraging blockchain for industry funding: A social media analysis. Sustain. Technol. Entrep. 3 , 100071 (2024).
Google Scholar
Barber, S., Boyen, X., Shi, E. & Uzun, E. Bitter to better-how to make bitcoin a better currency. In Financial Cryptography and Data Security: 16th International Conference, FC 2012, Kralendijk, Bonaire, Februray 27–March 2, 2012, Revised Selected Papers 16 , 399–414 (Springer, 2012).
Reid, F. & Harrigan, M. An Analysis of Anonymity in the Bitcoin System (Springer, 2013).
Book Google Scholar
Mulahuwaish, A., Loucks, M., Qolomany, B. & Al-Fuqaha, A. Topic modeling based on two-step flow theory: Application to tweets about bitcoin. IT Prof. 25 , 52–63. https://doi.org/10.1109/MITP.2023.3253103 (2023).
Klebnikov, S. Elon musk is the richest person in the world-again [www document]. Forbes. https://www.forbes.com/sites/sergeiklebnikov/2021/01/14/elon-musk-is-the-richestperson-in-the-world-again/ . Aaccessed 31 Jan 2021 (2021).
Musk, E. Am considering taking tesla private at \$420. funding secured. Retrieved June 1, 2019 (2018).
Cano-Marin, E., Mora-Cantallops, M. & Sánchez-Alonso, S. Twitter as a predictive system: A systematic literature review. J. Bus. Res. 157 , 113561 (2023).
Fu, Y., Zhuang, Z. & Zhang, L. Ai ethics on blockchain: Topic analysis on twitter data for blockchain security. In Science and Information Conference , 82–100 (Springer, 2023).
Choi, Y., Kim, B. & Lee, S. Blockchain ventures and initial coin offerings. Int. J. Technoentrep. 4 , 32–46 (2020).
Park, J. & Seo, Y.-S. Twitter sentiment analysis-based adjustment of cryptocurrency action recommendation model for profit maximization. In IEEE Access (2023).
Nakamoto, S. Bitcoin: A peer-to-peer electronic cash system. Decent. Bus. Rev. 20 , 20 (2008).
Wood, G. et al. Ethereum: A secure decentralised generalised transaction ledger. Ethereum Project Yellow Pap. 151 , 1–32 (2014).
Schwartz, D. et al. The ripple protocol consensus algorithm. Ripple Labs Inc White Pap. 5 , 151 (2014).
Disli, M., Abd Rabbo, F., Leneeuw, T. & Nagayev, R. Cryptocurrency comovements and crypto exchange movement: The relocation of binance. Financ. Res. Lett. 48 , 102989 (2022).
Nani, A. The doge worth 88 billion dollars: A case study of dogecoin. Convergence 28 , 1719–1736 (2022).
Pagariya, P., Shinde, S., Shivpure, R., Patil, S. & Jarali, A. Cryptocurrency analysis and forecasting. In 2022 2nd Asian Conference on Innovation in Technology (ASIANCON) , 1–6. https://doi.org/10.1109/ASIANCON55314.2022.9909168 (2022).
David, H. Investing In Fantom (FTM)—everything you need to know (2024). https://www.securities.io/investing-in-fantom/ .
Cointelegraph. Polygon blockchain explained: A beginner’s guide to MATIC (2024). https://cointelegraph.com/learn/polygon-blockchain-explained-a-beginners-guide-to-matic .
Stilt. What is Cardano? (2024). https://www.stilt.com/blog/2021/10/what-is-cardano/ .
Boyd, R. L., Ashokkumar, A., Seraj, S. & Pennebaker, J. W. The Development and Psychometric Properties of liwc-22 1–47 (University of Texas at Austin, 2022).
TextBlob. TextBlob: Simplified text processing (2024). https://textblob.readthedocs.io/en/dev/ .
Mohammad, S. M. & Turney, P. D. Nrc emotion lexicon. Natl. Res. Council Can. 2 , 234 (2013).
Farr, J. N., Jenkins, J. J. & Paterson, D. G. Simplification of flesch reading ease formula. J. Appl. Psychol. 35 , 333 (1951).
Kincaid, J., Fishburne, R., Rogers, R. & Chissom, B. Derivation of New Readability Formula for Navy Enlisted Personnel (Navy Research Branch, 1975).
Gunning, R. The fog index after twenty years. J. Bus. Commun. 6 , 3–13 (1969).
Flesch, R. A new readability yardstick. J. Appl. Psychol. 32 , 221 (1948).
Article CAS PubMed Google Scholar
Butt, S., Sharma, S., Sharma, R., Sidorov, G. & Gelbukh, A. What goes on inside rumour and non-rumour tweets and their reactions: A psycholinguistic analyses. Comput. Hum. Behav. 135 , 107345 (2022).
Inamdar, A., Bhagtani, A., Bhatt, S. & Shetty, P. M. Predicting cryptocurrency value using sentiment analysis. In 2019 International Conference on Intelligent Computing and Control Systems (ICCS) , 932–934 (IEEE, 2019).
Chuen, D., Guo, L. & Wang, Y. Cryptocurrency: A new investment opportunity?. SSRN Electron. J. https://doi.org/10.2139/ssrn.2994097 (2017).
Wołk, K. Advanced social media sentiment analysis for short-term cryptocurrency price prediction. Expert. Syst. 37 , e12493 (2020).
Lamon, C., Nielsen, E. & Redondo, E. Cryptocurrency price prediction using news and social media sentiment. SMU Data Sci. Rev 1 , 1–22 (2017).
Klare, G. R. The measurement of readability. (No Title) (1963).
Dale, E. & Chall, J. S. The concept of readability. Elem. Engl. 26 , 19–26 (1949).
Mohapatra, S., Ahmed, N. & Alencar, P. Kryptooracle: A real-time cryptocurrency price prediction platform using twitter sentiments. In 2019 IEEE International Conference on Big Data (Big Data) , 5544–5551 (IEEE, 2019).
Aslam, N., Rustam, F., Lee, E., Washington, P. B. & Ashraf, I. Sentiment analysis and emotion detection on cryptocurrency related tweets using ensemble lstm-gru model. IEEE Access 10 , 39313–39324 (2022).
Jamil, R. et al. Detecting sarcasm in multi-domain datasets using convolutional neural networks and long short term memory network model. PeerJ Comput. Sci. 7 , e645 (2021).
Article PubMed PubMed Central Google Scholar
Mujahid, M. et al. Sentiment analysis and topic modeling on tweets about online education during covid-19. Appl. Sci. 11 , 8438 (2021).
Article CAS Google Scholar
Ibrahim, A. Forecasting the early market movement in bitcoin using twitter’s sentiment analysis: An ensemble-based prediction model. In 2021 IEEE International IOT, Electronics and Mechatronics Conference (IEMTRONICS) , 1–5 (IEEE, 2021).
Pano, T. & Kashef, R. A corpus of btc tweets in the era of covid-19. In 2020 IEEE International IOT, Electronics and Mechatronics Conference (IEMTRONICS) , 1–4 (IEEE, 2020).
Pano, T. & Kashef, R. A complete vader-based sentiment analysis of bitcoin (btc) tweets during the era of covid-19. Big Data Cogn. Comput. 4 , 33 (2020).
Wang, L., Wang, X., Chen, A., Jin, X. & Che, H. Prediction of type 2 diabetes risk and its effect evaluation based on the xgboost model. In Healthcare , vol. 8, 247 (MDPI, 2020).
Shahzad, M. K. et al. Bpte: Bitcoin price prediction and trend examination using twitter sentiment analysis. In 2021 International Conference on Information and Communication Technology Convergence (ICTC) , 119–122 (IEEE, 2021).
Raheman, A., Kolonin, A., Fridkins, I., Ansari, I. & Vishwas, M. Social media sentiment analysis for cryptocurrency market prediction. arXiv:2204.10185 (arXiv preprint) (2022).
Huang, X. et al. Lstm based sentiment analysis for cryptocurrency prediction. In Database Systems for Advanced Applications: 26th International Conference, DASFAA 2021, Taipei, Taiwan, April 11–14, 2021, Proceedings, Part III 26 , 617–621 (Springer, 2021).
Sailunaz, K. & Alhajj, R. Emotion and sentiment analysis from twitter text. J. Comput. Sci. 36 , 101003 (2019).
Shah, F. M., Reyadh, A. S., Shaafi, A. I., Ahmed, S. & Sithil, F. T. Emotion detection from tweets using ait-2018 dataset. In 2019 5th International Conference on Advances in Electrical Engineering (ICAEE) , 575–580 (IEEE, 2019).
Mohammad, S., Bravo-Marquez, F., Salameh, M. & Kiritchenko, S. Semeval-2018 task 1: Affect in tweets. In Proceedings of the 12th International Workshop on Semantic Evaluation , 1–17 (2018).
BCcampus. Accessibility Toolkit-2nd Edition-Open Textbook (2024). https://opentextbc.ca/accessibilitytoolkit/ .
Kochkina, E., Liakata, M. & Zubiaga, A. All-in-one: Multi-task learning for rumour verification. arXiv:1806.03713 (arXiv preprint) (2018).
Cambria, E., Gelbukh, A., Poria, S. & Kwok, K. Sentic api: A common-sense based api for concept-level sentiment analysis. In CEUR Workshop Proceedings , vol. 1141, 19–24 (CEUR-WS, 2014).
Narman, H. S., Uulu, A. D. & Liu, J. Profile analysis for cryptocurrency in social media. In 2018 IEEE International Symposium on Signal Processing and Information Technology (ISSPIT) , 229–234. https://doi.org/10.1109/ISSPIT.2018.8642634 (2018).
Jacob, P. & Uitdenbogerd, A. L. Readability of twitter tweets for second language learners. In Proceedings of the The 17th Annual Workshop of the Australasian Language Technology Association , 19–27 (2019).
Wuraola, I., Dethlefs, N. & Marciniak, D. Linguistic pattern analysis in the climate change-related tweets from UK and Nigeria. In Proceedings of the 2023 CLASP Conference on Learning with Small Data (LSD) , 90–97 (2023).
LIWC. LIWC : A linguistic inquiry and word count standard (2024). https://www.liwc.app/download (2024).
James, R. 12 most popular types of cryptocurrency (2024). https://finance.yahoo.com/news/12-most-popular-types-cryptocurrency-221243578.html .
Singh, P. K., Pandey, A. K. & Bose, S. A new grey system approach to forecast closing price of bitcoin, bionic, cardano, dogecoin, ethereum, xrp cryptocurrencies. Qual. Quantity 57 , 2429–2446 (2023).
Vidal-Tomás, D., Briola, A. & Aste, T. Ftx’s downfall and Binance’s consolidation: The fragility of centralised digital finance. Phys. A 625 , 129044 (2023).
Tweepy. API-tweepy 4.14.0 documentation (2024). https://docs.tweepy.org/en/stable/api.html .
Lee, C. J. & Chua, H. N. Using linguistics and psycholinguistics features in machine learning for fake news classification through twitter. In Proceedings of International Conference on Data Science and Applications: ICDSA 2021, Volume 1 , 717–730 (Springer, 2022).
LIWC. Linguistic Inquiry and Word Count (LIWC, 2024). https://www.liwc.app/ .
Lyu, S., Ren, X., Du, Y. & Zhao, N. Detecting depression of Chinese microblog users via text analysis: Combining linguistic inquiry word count (liwc) with culture and suicide related lexicons. Front. Psych. 14 , 1121583 (2023).
Bojić, L. M. The patterns of influence: Liwc analysis of leading news portals’ impact and communication accommodation theory on twitter. Ethnoanthropol. Probl. 18 , 589–612 (2023).
Pennebaker, J. W., Francis, M. E. & Booth, R. J. Linguistic Inquiry and Word Count: Liwc 2001 Vol. 71 (Lawrence Erlbaum Associates, 2001).
Kacewicz, E., Pennebaker, J. W., Davis, M., Jeon, M. & Graesser, A. C. Pronoun use reflects standings in social hierarchies. J. Lang. Soc. Psychol. 33 , 125–143 (2014).
Pennebaker, J. W., Booth, R. J., Boyd, R. L. & Francis, M. E. Linguistic Inquiry and Word Count: LIWC2015 Operator’s Manual (2015).
Oliver, R. L. Satisfaction: A Behavioral Perspective on the Consumer (Routledge, 2014).
Balouchzahi, F., Sidorov, G. & Gelbukh, A. Polyhope: Two-level hope speech detection from tweets. Expert Syst. Appl. 225 , 120078 (2023).
Chun, M. M., Golomb, J. D. & Turk-Browne, N. B. A taxonomy of external and internal attention. Annu. Rev. Psychol. 62 , 73–101 (2011).
Article PubMed Google Scholar
Liu, W. & Liu, W. Analysis on the word-formation of English netspeak neologism. J. Arts Human. 3 , 22–30 (2014).
Baalen, I. V. Male and female language: Growing together. Retrieved on September 25, 2018 (2001).
Santos, N. M. B., Alarcón, M. M. H. & Pablo, I. M. Fillers and the development of oral strategic competence in foreign language learning. Porta Linguarum 191 , 201 (2016).
Chuen, D. L. K., Guo, L. & Wang, Y. Cryptocurrency: A new investment opportunity?. J. Altern. Invest. 20 , 16–40 (2017).
Chen, Y.-L., Chang, C.-L. & Yeh, C.-S. Emotion classification of youtube videos. Decis. Support Syst. 101 , 40–50 (2017).
TutorialsPoint. Emotion classification using NRC Lexicon in Python (2024). https://www.tutorialspoint.com/emotion-classification-using-nrc-lexicon-in-python .
Rock Content. Flesch Reading Ease Score: Is It Still Relevant in 2024? (2024). https://rockcontent.com/blog/flesch-reading-ease-score/ .
Zamanian, M. & Heydari, P. Readability of texts: State of the art. Theory Pract. Lang. Stud. 2 , 43–53 (2012).
Readable. Flesch reading ease and the Flesch Kincaid grade level (2024). https://readable.com/readability/flesch-reading-ease-flesch-kincaid-grade-level/ .
Briola, A., Vidal-Tomás, D., Wang, Y. & Aste, T. Anatomy of a stablecoin’s failure: The terra-luna case. Financ. Res. Lett. 51 , 103358 (2023).
Anthony Clarke. 7 biggest crypto collapses of 2022 the industry would like to forget (2022). https://cointelegraph.com/news/7-biggest-crypto-collapses-of-2022-the-industry-would-like-to-forget (2022).
Sidorov, G., Balouchzahi, F., Butt, S. & Gelbukh, A. Regret and hope on transformers: An analysis of transformers on regret and hope speech detection datasets. Appl. Sci. 13 , 3983 (2023).
Balouchzahi, F., Butt, S., Sidorov, G. & Gelbukh, A. Reddit: Regret detection and domain identification from text. Expert Syst. Appl. 225 , 120099 (2023).
Download references
Acknowledgements
The work was done with partial support from the Mexican Government through the grant A1-S-47854 of CONACYT, Mexico, grants 20241816, 20241819, and 20240951 of the Secretaría de Investigación y Posgrado of the Instituto Politécnico Nacional, Mexico. The authors thank the CONACYT for the computing resources brought to them through the Plataforma de Aprendizaje Profundo para Tecnologas del Lenguaje of the Laboratorio de Supercómputo of the INAOE, Mexico and acknowledge the support of Microsoft through the Microsoft Latin America PhD Award.
Author information
Olga Kolesnikova
Present address: Instituto Politécnico Nacional (IPN), Centro de Investigación en Computación (CIC), Mexico, Mexico
Authors and Affiliations
Instituto Politécnico Nacional (IPN), Centro de Investigación en Computación (CIC), Mexico, Mexico
Moein Shahiki Tash, Zahra Ahani & Grigori Sidorov
You can also search for this author in PubMed Google Scholar
Contributions
M.S.T., O.K., Z.A., and G.S. contributed to this manuscript as follows: M.S.T. conceived and designed the study, collected and analyzed the Twitter data, performed the psycholinguistic and emotion analysis, and drafted the manuscript. O.K. contributed to the data analysis, provided expertise in statistical modeling, and critically revised the manuscript for important intellectual content. Z.A. assisted in data collection, conducted literature review, and contributed to the interpretation of results. G.S. provided guidance on computational methodologies, supervised the research process, and contributed to manuscript revisions.
Corresponding authors
Correspondence to Moein Shahiki Tash or Olga Kolesnikova .
Ethics declarations
Competing interests.
The authors declare no competing interests.
Additional information
Publisher's note.
Springer Nature remains neutral with regard to jurisdictional claims in published maps and institutional affiliations.
Rights and permissions
Open Access This article is licensed under a Creative Commons Attribution 4.0 International License, which permits use, sharing, adaptation, distribution and reproduction in any medium or format, as long as you give appropriate credit to the original author(s) and the source, provide a link to the Creative Commons licence, and indicate if changes were made. The images or other third party material in this article are included in the article’s Creative Commons licence, unless indicated otherwise in a credit line to the material. If material is not included in the article’s Creative Commons licence and your intended use is not permitted by statutory regulation or exceeds the permitted use, you will need to obtain permission directly from the copyright holder. To view a copy of this licence, visit http://creativecommons.org/licenses/by/4.0/ .
Reprints and permissions
About this article
Cite this article.
Tash, M.S., Kolesnikova, O., Ahani, Z. et al. Psycholinguistic and emotion analysis of cryptocurrency discourse on X platform. Sci Rep 14 , 8585 (2024). https://doi.org/10.1038/s41598-024-58929-4
Download citation
Received : 21 November 2023
Accepted : 04 April 2024
Published : 13 April 2024
DOI : https://doi.org/10.1038/s41598-024-58929-4
Share this article
Anyone you share the following link with will be able to read this content:
Sorry, a shareable link is not currently available for this article.
Provided by the Springer Nature SharedIt content-sharing initiative
- Cryptocurrency
- Psycholinguistic
- Digital coins
- Reliability
By submitting a comment you agree to abide by our Terms and Community Guidelines . If you find something abusive or that does not comply with our terms or guidelines please flag it as inappropriate.
Quick links
- Explore articles by subject
- Guide to authors
- Editorial policies
Sign up for the Nature Briefing newsletter — what matters in science, free to your inbox daily.

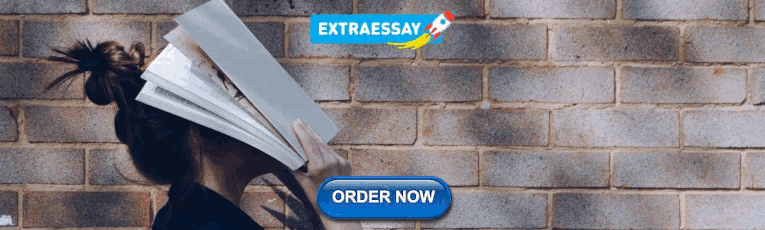
IMAGES
VIDEO
COMMENTS
Cryptocurrency is a form of virtual money that is focused on cryptography and electronic communication concepts. There have been hundreds of cryptocurrencies launched in recent years, with Bitcoin being the most common. Cryptocurrencies have gained a lot of coverage in recent years [8].
In determining the demographic profile of cryptocurrency users from the Web3 Philippines Facebook group in this study, the most common age group were 18 to 27 years old, with 261 frequencies covering 64.6%. The majority of cryptocurrency users, 307 or 76.0%, were males. The most prevalent civil status was single with 331 frequencies.
Akbari Darshak. Pipaliya. A cryptocurrency is a digital asset designed to work as a medium of exchange that uses cryptography to secure its transaction to control the creation of additional units ...
The Philippines has the second-lowest cryptocurrency adoption rate in the world, at 2.9 percent, after only Singapore at 3.3 percent. Furthermore, by the end of 2015, knowledge concerning ...
Abstract. This study tackles cryptocurrency adoption, wherein it evaluates the acceptability and use of cryptocurrency as a payment method in the Philippines with an emphasis on identifying the factors that influence behavioral intentions of individuals.
City, Philippines), and Engr. Ana Antoniette C. Illahi, PCpE (De La Salle University, Manila, Philippines). Abstract Purpose - This study aims to shed light on the rise of play-to-earn games in the Philippines alongside cryptocurrency. The lack of research and public understanding of its benefits
In the Philippines and Southeast Asia, economic analysis has been conducted on how cryptocurrency investment could hedge portfolios, or taken an institutional view to assess macro-economic impacts ...
:- This analysis of the literature aims to shed light on the Philippines decision to follow the alternative currency scheme. Limited knowledge of cryptocurrencies, as well as knowledge of where to find required block chain wallets on the internet, is now a challenge. Philippines has legalized the used of cryptocurrency approved by the Bangko Sentral ng Pilipinas (Central Bank of the ...
Abstract. On February 6, 2017, the Bangko Sentral ng Pilipinas ("BSP") issued the Guidelines for Virtual Currency Exchanges (BSP Circular No. 944, or "Circular"), providing the rules and regulations governing operations of Virtual Currency ("VC") Exchanges in the Philippines. The Circular is incorporated as Section 4512N of the ...
NTRC Tax Research Journal Vol. XXXIV.6 November-December 2022 2 Basic Information and Regulation of Cryptocurrency in the Philippines and Other ASEAN Member States cryptographic2 proof is used to maintain the integrity of the network instead of relying on third-party banks and other institutions.
This study aims to shed light on the rise of play-to-earn games in the Philippines alongside cryptocurrency. The lack of research and public understanding of its benefits and drawbacks prompted the researchers to investigate its market. As such, the study tried to look into the risks and benefits of crypto gaming if it would be regulated by the government, and how market volatility influences ...
4. Future research directions. In this section, we explain a few key areas that future research within cryptocurrencies should study in order to progress our understanding and appreciation of cryptocurrencies. Firstly, and related to the final paper in this special issue, stablecoins.
the cryptocurrency bubbles in 2018 and domestic currency. In this research, there are at least six different geographi-cal portfolios that lead to cryptocurrencies' effects on risk-adjusted returns and hedging abilities. Therefore, there are three major purposes of this research: to analyze whether
According to Exton and Doidge (2018), the share of respondents owning cryptocurrencies ranges from 5% in Belgium to 12% in Romania and 18% in Turkey. Deutsche Postbank (2018) estimates the share of German citizens owning cryptocurrency at 3%, and at 4% among digital natives. Global Web Index (2018) conducted a worldwide survey among 111,899 ...
Purpose - This study aims to shed light on the rise of play-to-earn games in the Philippines alongside cryptocurrency. The lack of research and public understanding of its benefits and drawbacks prompted the researchers to investigate its market. As such, the study tried to look into the risks and benefits of crypto gaming if it would be regulated by the government, and how market volatility ...
This paper intends to present an update of the various corporate governance reforms in the Philippines since the adoption of the 2002 Code of Corporate Governance issued by the Philippine ...
Bitcoin adoption in the Philippines has been steadily growing over the years. Since the crypto bull run in 2021, the local adoption of cryptocurrency in the country has been rapid compared to the other countries surveyed by the blockchain data platform Chainalysis for its annual index report titled, "The 2022 Global Crypto Adoption Index," where the Philippines ranked 2nd.
A recent survey has revealed that the Philippines ranks 10th in cryptocurrency adoption, with over 11.6 million Filipinos owning digital assets. This is also evidenced in the fact that according ...
The The Fintech Philippines Association and European Chamber of Commerce of the Philippines have reported similar findings. Bitcoin first became popular in the Philippines 2017, when its value skyrocketed from $1,000 to over $19,000 in the space of a few months. Since then, BSP has installed a reliable platform that protects crypto customers ...
the relative novelty of cryptocurrency. 3. Methods The paper is based on a descriptive research design. The study used a researcher-made questionnaire of three parts. The first part determined the demographic profile of the respondents in terms of age and gender. The first part also
Request PDF | On Nov 1, 2019, Miriam F. Bongo and others published Blockchain technology in the Philippines: Status, trends, and ways forward | Find, read and cite all the research you need on ...
The Philippines has also exhibited increasing optimism about crypto. Banko Sentral ng Pilipinas (BSP) data shows that the volume of cryptocurrency transactions grew 362% year on year to nearly 20 ...
This paper provides an extensive examination of a sizable dataset of English tweets focusing on nine widely recognized cryptocurrencies, specifically Cardano, Binance, Bitcoin, Dogecoin, Ethereum ...
Based on the results of the R-Square test, perceptions of benefits, perceptions of convenience, and investment risk affect interest in cryptocurrency investment by 21.9%, while the remaining 78.1% ...