Accessibility Links
- Skip to content
- Skip to search IOPscience
- Skip to Journals list
- Accessibility help
- Accessibility Help
Click here to close this panel.
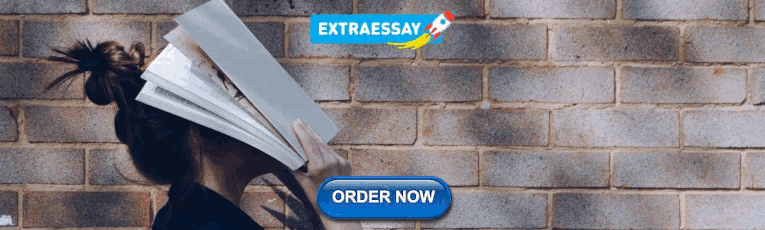
Introduction to enzymes and their applications
Saurabh Bhatia Published September 2018 • Copyright © IOP Publishing Ltd 2018 Pages 1-1 to 1-29
You need an eReader or compatible software to experience the benefits of the ePub3 file format .
Download complete PDF book , the ePub book or the Kindle book
Chapter navigation
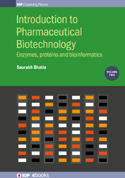
- Table of contents
- Next chapter
Export citation and abstract
Permissions.
Get permission to re-use this book
Share this chapter
Affiliations.
Amity institute of Pharmacy, Amity university. Gurgaon, Haryana, India
Published September 2018
Chapter DOI
https://doi.org/10.1088/978-0-7503-1302-5ch1
Books links
Book table of contents
About ePub3
About IOP ebooks opens in new tab
Enzyme catalysis is an area of fundamental importance in different areas. This chapter offers a concise overview to the fundamental principles and mechanisms of action, catalysis inhibition and its pharmaceutical applications. Additionally, this section also covers basics information related with enzymes such as its structure, function and different properties.
This article is available under the terms of the IOP-Standard Books License
All rights reserved. No part of this publication may be reproduced, stored in a retrieval system or transmitted in any form or by any means, electronic, mechanical, photocopying, recording or otherwise, without the prior permission of the publisher, or as expressly permitted by law or under terms agreed with the appropriate rights organization. Multiple copying is permitted in accordance with the terms of licences issued by the Copyright Licensing Agency, the Copyright Clearance Centre and other reproduction rights organisations.
Permission to make use of IOP Publishing content other than as set out above may be sought at [email protected] .
Saurabh Bhatia has asserted his right to be identified as the author of this work in accordance with sections 77 and 78 of the Copyright, Designs and Patents Act 1988.
1.1. Introduction
The cell is the structural and functional unit of life—the basic building block of living systems. Cells have the capability to effectively utilize biocatalysts, known as enzymes, which have outstanding catalytic efficiency and both substrate and reaction specificity. Enzymes have amazing catalytic power and their high level of specificity for their substrate makes them suitable for biological reactions. They are crucial for cellular metabolism. Each and every chemical reaction that takes place in plants, micro-organisms and animals proceeds at a quantifiable rate as a direct result of enzymatic catalysis. Most of the history of biochemistry is directly or indirectly related to the history of enzyme research. Catalysis in biological systems was initially reported in the early 1800s based on research into the digestion of meat. In this report the catalytic activity of secretions from the stomach, the conversion of starch into sugar by saliva, and various plant extracts were reported.
In 1837, Berzelius documented the catalytic nature of fermentation. In the 1850s Louis Pasteur reported that fermentation was a process initiated by living organisms. During this study it was reported that the fermentation of sugar into alcohol by yeast was catalyzed by ferments. He also hypothesized that these ferments are close to the structure of yeast. These ferments were later called enzymes (in yeast). The key breakthrough in the history of enzymes came in 1897 when Edward Buchner isolated, from yeast cells, the soluble active form of the set of enzymes that catalyzes the fermentation of sugar to alcohol. Emul Fischer reported the first systematic studies on enzyme specificity in the early twentieth century [ 1 ]. Later, in 1926, James Sumner extracted urease in pure crystalline form from jack beans [ 2 ]. He also recognized the protein nature of urease . In 1930, John Northrop and his co-workers crystallized pepsin and trypsin and established them as proteins [ 3 ]. In subsequent years enzymology developed rapidly (table 1.1 ). The important developments during this period are: the elucidation of major metabolic pathways, such as the glycolysis and tricarboxylic acid cycle; the detection of numerous biochemical events of digestion, coagulation, muscular contraction and endocrine function, and their roles in the maintenance, control and integration of complex metabolic processes; the kinetic backgrounds to explain the observations of enzyme action and inhibition; and the development of protocols for examining the structures of functionally sensitive proteins. There has been exhaustive research on enzyme-catalyzed reactions and enzymes involved in cell metabolism. At present, 2000 different enzymes have been recognized, each of which catalyzes a different chemical reaction. Currently, more focus is being directed towards the application of enzymes. The high efficiency of enzymes makes them commercially valuable and their specificity of action is offering diverse advantages in clinical medicine.
Table 1.1. Chronology of enzyme studies.
1.2. Properties of enzymes
Enzymes are the complex protein molecules, often called biocatalysts, which are produced by living cells. They are highly specific both in the reactions that they catalyze and in their choice of reactants, which are known as substrates. An enzyme typically catalyzes a single chemical reaction or a set of closely related reactions [ 4 ]. Side reactions resulting in the wasteful formation of by-products are rare in enzyme-catalyzed reactions, in comparison to uncatalyzed ones. Enzymes can also be defined as soluble, colloidal and organic catalysts that are produced by living cells, but are capable of acting independently of the cells [ 4 ]. Enzymes are currently being used in diverse areas in the food, feed, paper, leather, agriculture and textiles industries, resulting in major cost reductions. Simultaneously, rapid scientific progress is now encouraging the chemistry and pharmacological industries to embrace enzyme technology, a trend supported by concerns regarding energy, raw materials, health and the environment. One of the most common advantages of enzymes is their ability to function continuously even after their removal or separation from the cells. This means that even after the separation of cells from in vivo environments, they continue to work efficiently under in vitro conditions; we can conclude that these biocatalysts remain in an active state even after their isolation. Principally, enzymes are non-toxic, biodegradable and can be produced in ample amounts by micro-organisms for industrial applications. In this chapter, the isolation, production, purification, utilization and application of enzymes (in soluble and immobilized or insoluble form) are discussed in detail. Procedures such as recombinant DNA technology and protein engineering are frequently used to produce more efficient and beneficial enzymes. The industrial production and utilization of enzymes is an important part of industry. Interdisciplinary collaboration between areas such as chemistry, process engineering, microbiology and biochemistry is required to develop the best possible enzyme technology, and eventually to achieve increased production and maintain the enzyme's physico-chemical properties under in vitro environments.
For catalytic action, small quantities of an enzyme are sufficient, where this quantity of enzyme is much smaller in comparison to its substrates. The overall concentration of substrate transformed per mass of enzyme is often very large. Without exception, all enzymes are proteinaceous and exhibit all the properties of a protein. The treatment of enzymes by extreme temperature or extreme pH, or by treatment with other denaturing agents, results in the complete loss of catalytic activity. Structural configurations such as the primary, secondary, tertiary and quaternary structures of enzyme proteins are essential for their catalytic activity. The degree of catalytic activity chiefly depends on the integrity of the enzyme's structure as a protein. As per reports, enzymes have molecular weights ranging from about 12 000 to over 1 million Da. A number of enzymes consist only of polypeptides and contain no chemical groups other than amino acid residues, e.g. pancreatic ribonuclease. Numerous enzymes require a specific, heat stable, low molecular weight organic molecule, known as a co-enzyme. Moreover, a number of enzymes require both a co-enzyme and one or more metal ions for activity. A complete biochemically active compound is formed by the combination of a catalytically active enzyme (also called the protein part) with a co-enzyme or a metal ion—this is called a holoenzyme. The protein part of a holoenzyme is called an apoenzyme. In this arrangement a co-enzyme may bind covalently or noncovalently to the apoenzyme. In certain enzymes the co-enzyme or metal ion is only loosely and transiently bound to the protein. However, in others it is tightly and permanently bound, in which case it is known as a prosthetic group. A prosthetic group signifies a covalently bound co-enzyme. According to reports, co-enzymes and metal ions are stable under heating, while the protein part of an enzyme (the apoenzyme), is denatured by heat.
Prosthetic groups may be classified functionally into two major classes: co-enzymes and co-factors. Co-enzymes may be considered to be biosynthetically related to the vitamins, such as the co-enzyme nicotinamide adenine dinucleotide (NAD) which is vital for cellular energy metabolism and integrates the vitamin niacin into its chemical makeup. Moreover, a co-enzyme may be considered as a co-substrate, experiencing a chemical transformation throughout the enzyme reaction (NAD is reduced to NADH), the reversal of which requires a separate enzyme, perhaps from a different cellular site. Co-enzymes might thus travel intra-cellularly between apo-enzymes and, by transferring chemical groupings, integrate several metabolic processes. Table 1.2 shows a list of the more common co-enzymes and their functions. In contrast to co-enzymes, co-factors, such as pyridoxal phosphate or hem groups, remain with one enzyme molecule and in conjunction complete a cycle of a chemical change brought about by one enzyme turnover [ 5 ]. Other enzymes, such as carboxypeptidase, require metal ions as co-factors, the divalent cations Mg 2+ , Zn 2+ and Mn 2+ being the most common; these are often called enzyme activators [ 6 ]. Table 1.3 lists several enzymes and their respective co-factors.
Table 1.2. Several co-enzymes employed in the transfer of specific atoms or functional groups.
Table 1.3. Several enzymes and their co-factors.
1.3. Catalysis
The role of a catalyst is to increase the speed of a chemical reaction. When the rate of a chemical reaction is governed by a soluble catalyst, which may result in a further increase in the rate of chemical reaction, it is called homogeneous catalysis. In this case catalysis occurs in a solution. When the catalyst is in a separate phase from the reactants, or when catalysis occurs on a insoluble surface or an immobilized matrix, it is known as heterogeneous catalysis. Enzymes are also called biological catalysts. These biological catalysts generally have the properties of homogeneous catalysts, however, a number of enzymes present in membranes are insoluble, and thus are called heterogeneous catalysts. Enzyme specificity is the absolute specificity of protein catalysts to identify and bind to only one or a few molecules. In this process the enzyme carries a defined arrangement of atoms in their active site to bind with the substrate. This active site on the enzyme should have a shape that accurately matches the substrates. Thus specificity is achieved when an enzyme with an active site binds with the chemical reactants (the substrates) at their active sites via weak bond interactions. To undergo a chemical reaction, this active site carries certain residues that form a temporary bond with the chemical reactants, termed the binding site, whereas the catalytic site carries the residues that are responsible for catalysis. Specificity is achieved when a substrate binds to an enzyme that has a defined arrangement of atoms in the active site. An enzyme always catalyzes a single type of chemical reaction, which involves the formation and breakdown of covalent bonds. Since they are specific to one particular reaction, this feature of enzymes is called reaction specificity, also known as absolute reaction specificity, i.e. no by-products are formed.
1.4. The structure of enzymes
Enzymes always act as catalysts and small quantities compared to their substrate are required to considerably increase the rate of chemical reactions, wherein the enzymes themselves experience no overall change [ 7 , 8 ]. In contrast to all true catalysts, an enzyme does not alter the ultimate equilibrium position of a reaction, which is thermodynamically determined, thus merely the rate of completion of equilibrium of a feasible reaction is augmented. In addition to catalytic properties, enzymes exhibit the physico-chemical behavior of proteins: their solubility, electrophoretic properties, electrolytic behaviors and chemical reactivity [ 7 , 8 ]. The primary structural configuration and catalytic action of enzymes is determined by the linear chain of amino acid residues linked via peptide bonds, which constitute a protein molecule. Localized folding of the primary structure is called a secondary structure, whereas the complete folding of the molecule is known as a tertiary structure. In contrast to these structural configurations, a quaternary structure is the agglomeration of several folded chains. The structural features of enzymes are shown in figures 1.1 and 1.2 . In contrast to traditional chemical catalysts, e.g. hydrogen ions, heavy metals or metal oxides, which are most effective in organic solvents, at very high temperatures or at extreme pH values, enzymes operate most efficiently under very mild conditions. When using enzymes, there are certain issues that require attention, such as deviation from homogeneous aqueous solutions, physiological pH and temperature, which can rapidly destroy enzyme activity. However, under normal conditions the increase in reaction rate is rarely matched by their non-protein counterparts.
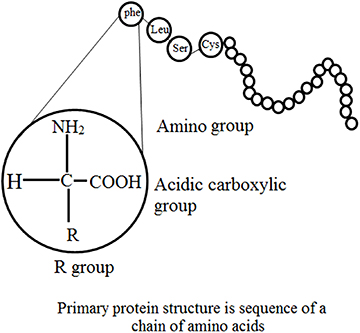
Figure 1.1. Structural features of enzyme.
Download figure:
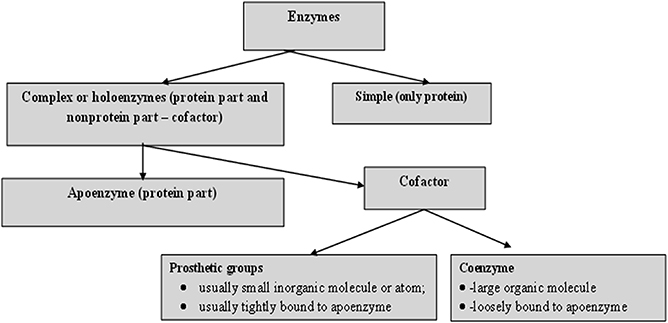
Figure 1.2. Principle components of an enzyme.
1.5. Structural features: primary and secondary structures
Three-dimensional analysis of the amino acid sequence of lysozyme of hen's egg white has demonstrated some features essential for primary structure [ 9 , 10 ]. These are:
- • Molecules derived from a similar source have a similar order of amino acid residues and appear to be random with no obvious predictability.
- • Even though numerous enzymes are intramolecularly crosslinked via disulfide bridges of cysteine, no branching occurs.
Current databases suggest that a small number of amino acids are extra and most are 'functional', i.e. the majority of them co-operatively control the higher orders of structural organization and therefore the catalytic activity. When comparing the primary structures of enzymes performing similar functions, wide structural homologies are detected in their sequence, mainly in the patterns of their nonpolar residues. For example, pancreatic juice contains five inactive precursors (zymogens), namely chymotrypsinogen A, B and C, trypsinogen and proelastase; all of these are activated to the respective proteases by proteolytic cleavage [ 11 ].
1.6. The mechanism of action of enzymes
The mechanism of action is based on a chemical reaction, in which the enzyme binds to the substrate and finally forms an enzyme–substrate complex. This reaction take place in a relatively small area of the enzyme called the active or catalytic site. In other words, the mechanism of enzyme action is based on the nature of the enzyme–substrate interaction, which accounts for the reaction specificity of the biological catalysts. The active or catalytic site of an enzyme is constituted by several amino acids, located at some distance from each other in the peptide chain. These amino acids are brought close together by the folding resulting from the secondary and tertiary structure of the enzymes. Side chains of amino acid residues at the catalytic site provide groups for binding with specific groups of the substrate. Co-factors assist the catalysis. The substrate forms bonds with amino acid residues in the substrate binding domain of the active site. The binding induces a conformational reaction in the active site. During the reaction, the enzyme forms a transition-state complex. As the products of the reaction disassociate, the enzyme returns to the original state. Two different models postulated for the mechanism of enzyme action are given below.
1.6.1. The Fisher template model (lock and key model)
This is a rigid model of the catalytic site, proposed by Emil Fischer in 1894 [ 12 ]. The model explains the interaction between a substrate and an enzyme in terms of a lock and key analogy. In this model, the catalytic site is presumed to be preshaped. The substrate fits as a key fits into a lock. The drawback of this model is the implied rigidity of the catalytic site. The model cannot explain changes in enzyme structure in the presence of allosteric modulators.
1.6.2. Induced fit model
In contrast to the above method, this model suggests a flexible mode for the catalytic site. To overcome the problems of the lock and key model owing to the rigid catalytic site, Koshland [ 13 – 15 ] suggested an induced fit model in 1963. The important feature of this procedure is the flexibility of the active site. In the induced fit model, the substrate induces a conformational change in the active site of the enzyme so that the substrate fits into the active site in the most convenient way so as to promote the chemical reaction. This method suggests competitive inhibition, allosteric modulation and inactivation of enzymes on denaturation.
The Michaelis–Menten theory of enzyme action [ 16 ] offers the basis for most current research on the mechanism of enzyme action. This concept of the enzyme–substrate complex scheme assumes the combination of the enzyme and substrate in phase one (occasionally known as the transition phase) of the enzyme activity and liberation of the enzyme and the products of the catalysis in phase two of the reaction.
1.6.3. Covalent catalysis
Covalent catalysis is evidenced in enzymes capable of forming covalent bonds between the substance and the catalytic group of the active site [ 17 ]. A number of enzymes react with their substrates to form very unstable, covalently joined enzyme–substrate complexes, which undergo further reaction to yield products much more readily than in an uncatalyzed reaction. Several of the enzymes that exhibit covalent catalytic behavior are listed in table 1.4 .
Table 1.4. Various enzymes exhibiting covalent catalytic behavior.
1.7. Catalysis via chymotrypsin
Hummel and Kalnitzky suggested an enzyme mechanism through the depiction of the sequential transition states experienced by the enzyme–substrate complex during catalysis [ 18 ]. Chymotrypsin is a digestive enzyme, responsible for proteolysis (breakdown of proteins and polypeptides) in the duodenum. Chymotrypsin favorably breaks peptide amide bonds (the carboxyl side of the amide bond is a large hydrophobic amino acid). These amino acids contain an aromatic ring in their side chain that fits into a 'hydrophobic pocket' of the enzyme. It is stimulated in the presence of trypsin. Trypsin and chymotrypsin are both serine proteases with high sequence and structural similarities, but with different substrate specificity [ 19 , 20 ].
1.7.1. Intermediary stages of chymotrypsin
As discussed above, chymotrypsin is a protease enzyme that cuts on the C-terminal phenylalanine, tryptophan and tyrosine on peptide chains [ 21 ]. Additionally, it is more specific for aromatic amino acids because of its hydrophobic pocket. Comparable to other serine proteases, chymotrypsin also catalyzes the hydrolysis of certain esters [ 22 ]. The molecular events involved in catalysis are called intermediary enzymology. Chymotrypsin, a protease, favorably accelerates breakdown of peptide bonds in which the aromatic amino acid (Phy, Try, or Trp) or bulky nonpolar R group (Met) contribute a carboxyl group. The synthetic substrate p-nitrophenyl acetate allows colorimetric analysis of chymotrypsin activity, as hydrolysis to p-nitrophenol, which is alkali, changes into the chromophore anionic forms.
1.7.2. Kinetic behavior of α -chymotrypsin
The kinetics of chymotrypsin of p-nitrophenyl acetate can be considered in a 'stop-flow' apparatus. This procedure utilizes substrate quantities of enzymes and measures the events in the first few milliseconds [ 23 ]. The use of p-nitrophenyl acetate as a substrate offers the prospect of investigating solvent effects on both the acylation of the enzyme and the hydrolysis (deacylation) of the acyl enzyme [ 23 ]. The significant features of the slow-flow kinetics of chymotrypsin are:
- - a burst phase featuring rapid liberation of an anion.
- - a subsequent 'steady-state' phase, with slower release of extra anion.
- • A 'charge relay network' acts as a proton shuttle during catalysis by chymotrypsin. The charge relay network of chymotrypsin encompasses three aminoacyl residues that are far apart in a primary structural sense, but close together in a tertiary structural sense. While most of the charged residues of chymotrypsin are present at the surface of the molecule, those of the charge relay network are hidden in the otherwise nonpolar inner side of the protein. These charges transmit residues which activate sequential proton shifts that shuttle protons in the opposite direction. An equivalent series of proton shifts is assumed to accompany the hydrolysis of the physiologic chymotrypsin substrate, e.g. a peptide.
1.7.3. Selective proteolysis in creation of the catalytic sites of enzymes
Various enzymes, hormones and other physiologically active proteins are produced as inactive precursors (zymogens) that are further transformed to the active form by selective enzymatic cleavage (limited proteolysis) of peptide bonds. The final step to activating enzymatic function is limited proteolysis, either in a single activation step or in a consecutive series (cascade). The specificity of each activation reaction is evaluated by the complementarity of the zymogen substrate and the active site of the attacking protease. The arrangement of successive activation reactions is controlled by the specificity of each enzyme, while the extent of amplification of the initial stimulus is evaluated by the effectiveness of each activating step. Zymogen activation produces a prompt and irreversible response to a physiological stimulus, and is capable of initiating new physiological functions. Classical examples are the processes of hormone production, fibrinolysis, complement activation, blood coagulation, supra-molecular assembly, metamorphosis, fertilization and digestion. The zymogens of the pancreatic serine proteases, in particular, have functioned as models for detailed studies of the nature of the molecular changes that are involved in the intense increase in enzymatic activity that results upon incomplete proteolysis of the zymogen.
Specific proteolysis is a common means of activating enzymes and other proteins in biological systems. A number of proteins are manufactured and released in the form of inactive precursor proteins called proproteins. Various enzymes attain full enzymatic activity as they suddenly fold into their characteristic three-dimensional forms. In contrast, other enzymes are produced as inactive precursors that are successively activated by breakdown of one or a few specific peptide bonds. The inactive precursor is known as a zymogen (or a pro-enzyme). In other words, when the proteins are enzymes, the proteins are called pro-ezymes or zymogens (table 1.5 ). An energy source (ATP) is not required for cleavage [ 11 ]. Thus, in comparison to reversible regulation by phosphorylation, even proteins sited outside cells can be triggered by this means. An additional noteworthy difference is that proteolytic activation, in comparison with allosteric control and reversible covalent modification, occurs just once in the life of an enzyme molecule. Transformation of a proprotein to the mature protein includes selective proteolysis. This transforms the proproteins by one or more consecutive proteolytic clips to a arrangement in which the individual activity of the mature protein (its enzymatic activity) is expressed, e.g. the hormone insulin (proinsulin), the digestive enzyme chymotrypsin (chymotrypsinogen), a number of factors for blood clotting and for the blood clot dissolution cascades, and the connective tissue protein collagen (procollagen). Chymotrypsinogen consists of 245 amino acid residues, and is practically devoid of enzymatic activity. As the reaction starts, it is converted into a fully active enzyme. This occurs when the peptide bond joining arginine 15 and isoleucine 16 is cleaved by trypsin. The subsequent active enzyme, known as π-chymotrypsin, then acts on other π -chymotrypsin molecules. Two dipeptides are eliminated to form α -chymotrypsin (the stable form of the enzyme) [ 11 ]. The three subsequent chains in α -chymotrypsin remain interconnected to each another by two interchain disulfide bonds. The outstanding feature of this process is that cleavage of a single specific peptide bond alters the protein from a catalytically inactive form into one that is fully active. The transformation of prochymotrypsin (Pro-CT), a 2,4,5-aminoacyl residue polypeptide, to the active enzyme α -chymotrypsin includes three proteolytic clips and the formation of an active intermediate called π -chymotrypsin ( π -CT) and consequently to the mature catalytically active enzyme α -chymotrypsin ( α -CT). Examples of gastric and pancreatic zymogens are listed in table 1.5 .
Table 1.5. Gastric and pancreatic zymogens.
1.7.4. Kinetic models for enzymes
Generally, enzyme kinetics is defined as the study of the rate of reactions, i.e., how the substrate concentration impacts the velocity of the reaction. Enzyme kinetics involves optimization of bio-catalytic reactions to allow process design and scaling up processes to further increase the production and minimize the overall overhead costs of various procedures. Kinetic investigations in the branch of biochemistry concerned with enzymes can be categorized into three types:
- • Transient-state kinetics : This is the stage of reaction before the steady or rapid-equilibrium state, and involves quick reactions between the enzymes and substrate. These sudden changes in the reaction mixture when the substrate and enzymes are mixed require advance equipment to monitor the reaction before it changes into the steady state. The mechanisms of the reaction are associated with the enzyme structural configuration. Basic steps are involved during an enzyme-catalyzed reaction, which allow the direct study of the intermediates and products formed during a single enzyme cycle, which may further help in direct analysis of individual reaction steps for short times. In this type of reaction a sufficient concentration of enzymes is used to witness the intermediate and product formation.
- • Steady-state kinetics : This is the phase in which the rate of formation of intermediates and the rate of decomposition remain the same, and thus the concentrations of reactive intermediates remain the same. During this reaction substrate concentration is greater than enzyme concentration. The Michaelis–Menten enzyme kinetic (figure 1.3 ) can be considered as the most often studied reaction for several enzymes. For example, chymotrypsin (protease) with a high concentration of substrate achieves maximum velocity of the reaction (called the first order of reaction) but at a certain point the substrate occupies all binding sites of the enzyme, after which further addition of substrate does not increase the rate. This is called the zeroth order of reaction (the steady state). It is the phase in which the enzyme and substrate concentrations cannot be determined using the dissociation constant. Thus steady-state enzyme kinetics is based on the theory that a catalytic reaction remains constant if the reaction is not exposed to continuous changes.
- • Rapid-equilibrium kinetics : This the phase in which both the enzyme and substrate concentrations can be determined using the dissociation constant. During this procedure total enzyme concentration remains constant during the reaction and the concentration is very small compared to the amount of substrate. In this reaction, before the rate-determining reaction, the reactions are in equilibrium with their components, thus this stage is called rapid-equilibrium kinetics.
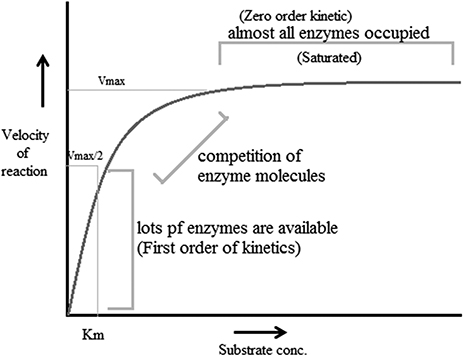
Figure 1.3. The Michaelis–Menten enzyme kinetic.
According to reports, factors that affect enzyme-catalyzed reactions also affect the velocity of a reaction. These factors are called modifiers of enzyme-catalyzed reactions. These modifiers can be divided into two classes: inorganic modifiers (enzyme activators) and organic modifiers (enzyme inhibitors). These factors can have different types of effects on the velocity of the reaction; nevertheless the most vital effect is that they offer many pathways to products, e.g. when one modifier is bound to an enzyme, it alters the rate of reaction and thus forms two rate constants. However, when two modifiers participate, there are five self-regulating equilibria, resulting in three paths for making products.
There are two mechanisms, single-substrate and multiple-substrate, that are helpful in studying the different stages of enzymatic reactions. Understanding these stages helps in understanding the properties of enzymes. Certain enzymes have single substrates (a single substrate binding site), e.g. triosephosphate isomerase, whereas certain enzymes have multiple substrates molecules (multiple binding sites), such as dihydrofolate reductase, and bind with multiple substrates. After the exploration of specific RNA sequences required for RNA replication, new biocatalysts in the form of ribozymes have emerged with the potential to catalyze specific biochemical reactions. There is a misconception about biological catalysts that all biological catalysts are made up of proteins, which is not true; some are RNA-based catalysts (ribozymes and ribosomes). Both are important for many cellular functions. A major difference between enzymes and ribozymes is that RNA-based catalysts are restricted to only a few reactions; however, their reaction mechanisms and kinetics can be studied and classified by similar procedures. Enzyme-based mutation, in particular site-directed mutagenesis, is an important approach to alter genes and investigate the functional and structural features of enzymes, e.g. mutation of the enzyme present in Coprinus cinereus peroxidase offers an understanding of its increased thermostability. Challenges involved in studying cascades of reactions catalyzed by a multi-enzyme, e.g. proteasome involved in the ubiquitin–proteasome pathway, can be overcome by establishing understanding of the complex structure and the respective biochemical reactions. This understanding allows exploration of active sites, intermediate compounds, final products and their interrelation with complex machinery, as well as biochemical reactions. It has been well understood that enzymes that accelerate complex reactions have numerous substrates and involve complex enzyme kinetic mechanisms. As discussed above, most of the biochemical reactions occurring in the body are multi-substrate reactions. In such reactions two substrates are involved and yield two products (figure 1.4 ). These types of reactions involve the transfer of a compound from one compoment to another, e.g. when glucose reacts with ATP in the presence of hexokinase it forms glucose 6-phophaste and ADP. Here, phosphate from ATP is transfered to glucose to form glucose 6 phosphate. The mechanism of catalysis involves two types of reactions: sequential and non-sequential reactions. Sequential reaction results in the formation of a ternary complex. This means that both of the substrates involved in the reaction bind with an enzyme to form the product (figure 1.4 ). Sequential reaction is further divided into two types: the random and compulsory order mechanisms. As the name suggests, in a 'random' mechanism, either substrate can bind first and any product can leave first. In contrast to the random order mechanism, in the compulsory order mechanism the order of binding of the substrate and order of release of the product is specific; this is also called the Theorell–Chance mechanism (figure 1.4 ). In a non-sequential reaction, also called the 'ping-pong' mechanism, formation of ternary complex does not take place. In these types of reactions, when the first substrate binds with enzyme its product is released, and then the second substrate binds and its product is released. Such a reaction is called a double placement reaction. Thus only a single substrate binds at a time; this may be due to the presence of a single binding site on the enzyme. Major differences between the sequential and non-sequential reactions are that the formation of a ternary complex takes place only in the sequential reaction, and that in the sequential reaction both substrates bind to the enzyme and release products, while in the non-sequential mechanism the substrates bind and release their products one after the other (figure 1.4 ).
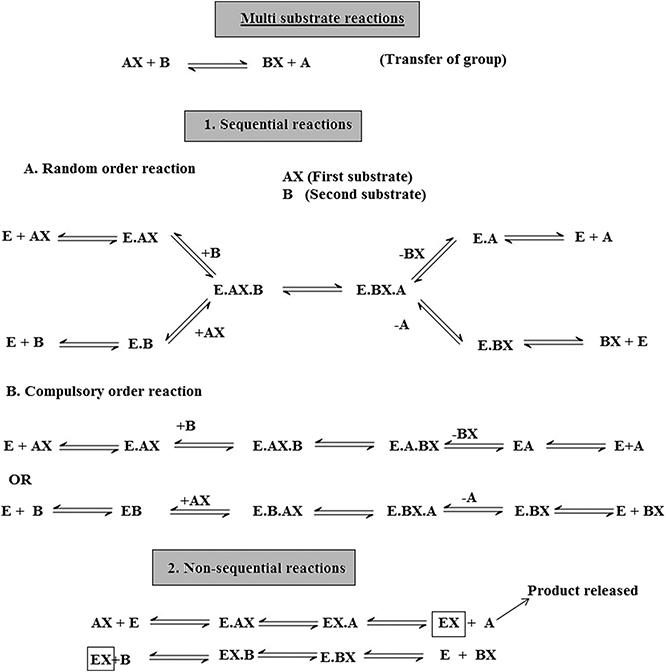
Figure 1.4. Multi-substrate reactions.
Another type of sequential mechanism is the systematic mechanism, which involves the addition of substrates and formation of products in a specific order.
1.7.5. Enzyme mediated acid–base (general) catalysis
Several protein enzymes use general acid–base catalysis as a way to increase reaction rates [ 26 ]. The amino acid histidine is optimized for this function because it has a pK(a) (where K(a) is the acid dissociation constant) near physiological pH [ 26 ].
When the substrate has been bound at the catalytic site, the charged functional groups of the side chains of neighboring aminoacyl residues may contribute in catalysis by behaving as acidic or basic catalysts. There are two extensive groups of acid–base catalysis by enzymes: general and specific (acid or base) catalysis. Specific acid or specific base catalysis are those reactions in which the reaction rates fluctuate under the influence of changes in H + or H 3 O + concentration, but are independent of the concentrations of the other acids or bases present in the solution. In contrast to specific catalysis, general acid or general base catalysis are the reactions whose rates are very reactive to all acids (proton donors) or bases (proton acceptors) present in the solution. To examine whether a given enzyme-catalyzed reaction is a general or specific acid or base catalysis, the rate of reaction is determined under two sets of circumstances:
- • at different pH values at a constant buffer concentration, and
- • at constant pH values but at different buffer concentrations. Against this background, if the degree of the reaction deviates as a function of pH at a constant buffer concentration, the reaction is specific base/acid catalyzed if the pH is above/below 7.0. If the reaction rate at a constant pH rises as the buffer concentration increases, the reaction is general base/acid catalysis, if the pH is above/below 7.0.
1.7.6. Metallozymes
Almost 25% of all enzymes include tightly bound metal ions or need them for activity. The major role of these metal ions is investigated using techniques such as x-ray crystallography, magnetic resonance imaging (MRI) and electron spin resonance (ESR). A metalloprotein is a protein that contains a metal ion co-factor. Metallozymes contain a certain amount of functional metal ion that is retained during the course of purification [ 27 ]. A metal-activated enzyme binds with metals less firmly, but needs to be activated by addition of metals. Four types of complexes are possible for the tertiary complexes of the catalytic site (Enz), a metal ion (M) and substrate (S) that exhibit 1:1:1 stoichiometry:
All of these complexes are possible for metal-activated enzymes. Metallozymes cannot form the EnzSM complex (substrate–bridge complexes), as the purified enzyme exists as Enz–M. Three generalization can be made:
- • The majority of the kinases (ATP: phosphotransferases) form substrate–bridge complexes of the type enzyme–nucleotide–M.
- • Phosphotransferases (phosphoenolpyruvate or pyruvate used as the substrate), enzymes catalyzing other reactions of phosphoenolpyruvate and carboxylases, form metal bridge complexes (Enz–M–S).
- • A particular enzyme may form one type of bridge complex with one substrate and a different type with another.
The metal ions participate in each of the four mechanisms by which the enzymes are known to accelerate the rates of chemical reaction:
- • Approximation of reactants.
- • Covalent catalysis.
- • General acid–base catalysis.
- • Induction of strain in the enzyme or substrate.
Metal ions are electrophiles (attracted to electrons) and share an electron pair forming a sigma bond. They may also be considered as super acids as they exist in neutral solutions, frequently having a positive charge which is greater than their quantity. Mn 2+ , Ca 2+ and Mg 2+ are the metal ions that are most commonly used in enzymatic catalysis. Two metal ions, iron and manganese are used in the form of haemprotein. Metal ions have the potential to accept electrons via sigma or pi bonds to successively activate electrophiles or nucleophiles. By means of donating electrons, metals can activate nucleophiles or act as nucleophiles themselves. The co-ordination sphere of a metal may bring together the enzyme and substrate or form chelate-producing distortion in either the enzyme or substrate [ 28 ]. A metal ion may also mask a nucleophile and thus avoid an otherwise probable side reaction. Metals can also function as three-dimensional templates for the co-ordination of basic groups on the enzyme or substrate.
1.8. Enzyme inhibition
Enzyme inhibition decreases the activity of an enzyme without significantly disrupting its three-dimensional macromolecular structure. Inhibition is therefore distinct from denaturation and is the result of a specific action by a reagent directed or transmitted to the active site region. When low molecular weight compounds interfere with the activity of enzymes by partially reducing or completely inhibiting the enzyme activity either reversibly or irreversibly, it is known as enzyme inhibition. The compounds responsible for such inhibition are called enzyme inhibitors. To protect the enzyme catalytic site from any change, a ligand binds with a critical side chain in the enzyme. Chemical modification can be performed to test the inhibitor for any drug value. Studies of enzymes can yield much information about the following:
- • A number of drugs useful in medicine, which seem to function because they can inhibit certain enzymes in malfunctioning cells.
- • The convenience of elucidating metabolic pathways in cells.
- • The mechanism of the catalytic activity.
- • The nature of the functional group at the active site.
- • The substrate specificity of the enzyme.
The pharmacological action of drugs is mainly based on enzyme inhibition, e.g. sulfonamides and other antibiotics. In the majority of cases the enzyme inhibited is known. The development of nerve gases, insecticides and herbicides is based on enzyme inhibition studies. There are two major types of enzyme inhibition: reversible and irreversible.
Reversible inhibitors efficiently bind to enzymes by forming weak non-covalent interactions, e.g. ionic bonds, hydrophobic interactions and hydrogen bonds. Reversible inhibitors do not form any strong chemical bonds or reactions with the enzyme, they are formed quickly and can easily be removed, in contrast to irreversible inhibitors. Reversible inhibition includes competitive inhibition, uncompetitive inhibition and noncompetitive inhibition. Irreversible inhibition includes group specific inhibition (reacts only to a certain chemical group), reactive substrate analogs (affinity label) and inhibitors that are structurally similar to the substrate and will bind to the active site, and mechanism-based inhibitors (enzymes transform the inhibitor into a reactive form within the active site).
1.9. Pharmaceutical applications
Currently, enzymes are often utilized for a broad range of applications such as: washing powders (e.g. proteases, lipases, amylases); textile manufacture (amylases and catalase to remove the starch); the leather industry (proteases to hydrolyze proteins); the paper industry; improvement of the environment; food production (enzyme-modified cheese/butter), processing (glucose oxidase for dough strengthening) and preservation; and medical applications. According to current reports, several enzymes are produced industrially and there are significant applications in the food industry (45% of use), detergent industry (35%), textiles industry (10%) and leather industry (3%). Details on the applications of individual enzymes are provided in table 1.6 .
Table 1.6. Industrially produced enzymes from plant sources and their applications.
1.9.1. Diagnostic applications of enzymes
Enzymes have been used widely in diagnostic applications varying from immunoassays to biosensors. Enzyme immunoassay methods hold great promise for application under a wide variety of conditions. Under laboratory conditions they can be as sensitive as radio-immunoassays, but they can also be adapted as simple field screening procedures [ 29 , 30 ]. The examination of enzyme quantity in the extracellular body fluids (blood plasma and serum, urine, digestive juices, amniotic fluid and cerebrospinal fluid) are vital aids to the clinical diagnosis and management of disease. Most enzyme-catalyzed reactions occur within living cells, however, when an energy imbalance occurs in the cells because of exposure to infective agents, bacterial toxins, etc, enzymes 'leak' through the membranes into the circulatory system. This causes their fluid level to be raised above the normal cell level. Estimation of the type, extent and duration of these raised enzyme activities can then furnish information on the identity of the damaged cell and indicate the extent of injury. Enzyme assays can make an important contribution to the diagnosis of diseases, as a minute change in enzyme concentration can easily be measured. Determination of the changes in enzyme level thus offers a greater degree of organ and disease differentiation in comparison to other possible clinico-chemical parameters, e.g. albumin or gamma globulin. Currently, the diagnostic specificity of enzyme tests is such that they are limited primarily to confirming diagnosis, offering data to be weighed alonside other clinical reports, owing to lack of disease specific enzymes. Table 1.7 includes a number of diagnostically important enzymes which are most often examined in clinic laboratories [ 29 , 30 ].
Table 1.7. Diagnostically significant enzymes.
1 B, brain; E, erythrocytes; H, heart muscle; Ht, hepatobiliary tract; I, intestinal mucosa; K, kidney; L, M, skeletal muscle; Pa, pancreas; P1, placenta; Pr, prostate gland; S, saliva.
1.9.1.1. Enzyme examinations in diseases of the liver and biliary
The diseases of the liver and gastrointestinal tract were among the first to which serum enzyme tests were applied. They have proved to be most effective owing to the large size of the organs and the wide range and abundance of enzymes [ 32 – 36 ]. The liver-based enzymes GOT, GPT and AP are examined to evaluate the site and nature of liver disease. LD, GGT, OCT and CHE are also examined. Several enzymes employed in the diagnosis of liver diseases along with their respective levels are listed in table 1.8 .
Table 1.8. Liver diseases and enzymes used in diagnosis [ 32 – 36 ].
1.9.1.2. Enzyme applications in heart disease
According to previous reports, no single enzyme has yet been reported to cure myocardial damage. The discovery of serum glutamine oxalacetic acid transaminase determination (GOT) in 1954 was considered a significant step forward in the diagnosis of acute myocardial infarction. A mixture of results from assays of CPK (creatine phosphokinase), HBD ( α -hydroxybutyrate dehydrogenase) and GOT (glutamine oxalacetic acid transaminase)—each of which has been shown to be elevated in more than 90% of cases—is used for diagnostic purposes [ 37 – 39 ]. The level of CPK starts rising three to four hours after the initial onset of pain, followed in order by GOT and AST (HBD) which appear after approximately eight hours. The maximum levels are reached in the same sequence, CPK after 24 h, LD 1 after 36 h and AST after about two days. The rise in enzyme levels is fairly moderate, AST and CPK increase by four to ten times their respective normal levels and LD 1 is approximately five-fold higher than normal. An enzyme known as hyaluronidase (hyaluronate hydrolysis) has been reported to cure heart attack [ 38 ]. The activity of many enzymes including aldolase, malic dehydrogenase, isomerase and ICD may increase following myocardial infarction [ 38 ].
1.9.1.3. Diagnosis of muscle disease
Skeletal muscle disorders include diseases of the muscle fibers (myopathies) or of the muscle nerves (neurogenic disorders) [ 40 ]. In myopathies CPJ, LD, ALD, GOT and GPT levels are raised. In the case of neurogenic diseases and hereditary diseases, CPK is occasionally raised (2–3 fold) [ 40 ]. Damage to the muscle may be due to extensive muscular exercise, drugs, physical trauma, inflammatory diseases, microbial infection or metabolic dysfunction, or it may be genetically predisposed. In muscular disorders the level of CPK is elevated in serum with the highest frequency and is assayed in the diagnosis of these disorders. An additional useful assayed enzyme is acetyl cholinesterase (AChE), which is significant in regulating certain nerve impulses [ 41 ]. Various pesticides affect this enzyme, so farm labors are frequently tested to be sure that they have not received accidental exposure to significant agricultural toxins. There are number of enzymes that are characteristically used in the clinical laboratory to diagnose diseases. There are highly specific markers for enzymes active in the pancreas, red blood cells, liver, heart, brain, prostate gland and many of the endocrine glands [ 41 ]. From the time when these enzymes became comparatively easy to examine using automated techniques, they have been part of the standard blood tests that veterinarians and medical doctors are likely to need in the diagnosis and treatment/management of diseases.
1.9.2. Enzymes in therapeutics
Enzymes have two significant features that differentiate them from all other types of drugs. First, enzymes frequently bind and act on their targeted sites with high affinity and specificity. Second, enzymes are catalytic and convert numerous target molecules to the desired products. These two important features make enzymes specific and potent drugs that can achieve therapeutic biochemistry in the body that small molecules cannot. These features have resulted in the development of many enzyme-based drugs for a wide range of disorders [ 42 ]. Currently, numerous enzymes are used as therapeutic agents, owing to the following features:
- • High specificity to their substrates.
- • Proficient in producing the desired effect without provoking any side effects.
- • Water soluble.
- • Extremely effective in a biological environment.
Enzymes as therapeutic agents also have some serious disadvantages which restrict their application. Their bulky structure, due to their large molecular weight, excludes them from the intracellular domain. Owing to their high proteinaceous nature they are highly antigenic and are rapidly cleared from blood plasma. Extensive purification from pyrogens and toxins is essential for parenteral enzymes, which increases the cost. Table 1.9 lists some therapeutically important enzymes.
Table 1.9. Therapeutically important enzymes.
1.9.2.1. Enzyme therapy of cancer
In traditional medicine, proteolytic enzymes derived from plant extracts have been used for a long time In addition to proteolytic enzymes from natural resources such as plants, 'modern' enzyme therapy includes pancreatic enzymes. Therapeutically, the use of proteolytic enzymes is partly based on scientific reports and is partly empirical [ 43 ]. Clinical evidence of the use of proteolytic enzymes in cancer studies has typically been obtained with an enzyme preparation comprising a combination of papain, trypsin and chymotrypsin. Earlier reports proved that enzyme therapy can reduce the adverse effects caused by radiotherapy and chemotherapy. There is also a report available that, in some types of tumors, survival may be sustained. The positive effects of systemic enzyme therapy appear to be based on its anti-inflammatory potential. Nevertheless, the exact mechanism of action of systemic enzyme therapy remains unsolved. The proportion of proteinases to antiproteinases, which is regularly used as a prognostic marker in cancer studies, is likely to be influenced by the oral administration of proteolytic enzymes, most likely via induction of the synthesis of antiproteinases. In addition, there are many alterations of cytokine composition during treatment with orally administered enzymes, which might be a sign of the efficacy of enzyme therapy [ 44 ].
Proteases and their inhibitors have long been studied in several tumor systems. However, out of numerous promising serine and metalloproteinase inhibitors, not a single one is included in oncology at present. The present exploration for active antiproteolytic agents is in contrast to the traditional approach, as evidenced by John Beard, who proposed the management of advanced cancer using fresh pancreatic extracts whose antitumor activity was based on their proteolytic potential.
The enzymatic treatment of tumors is based on the idea of denying the abnormal cells their essential metabolic precursors such as amino acids, nucleic acids and folates. A number of enzymes have been examined and evidenced as antitumor agents. l -serine dehydratase, l -arginase, carboxypeptidase G (folate depletion), l -asparaginase, l -methioninase, l -phenylalanine ammonia lyase, l -glutaminase, l -tyrosinase and xanthine oxidase have been studied for their anticancer activity. Enzyme preparations such as asparaginase (amidase), bromelain (protease) and chymotrypsin (protease) have also been studied as cancer treatments (table 1.9 ).
l -asparaginase is the most widely investigated enzyme. It has been reported in treatment against three neoplastic diseases, acute lymphoblastic leukemia, leukemic lymphosarcoma and myeloblastic leukemia. It deprives the cancerous cells of their nutritional asparagine supply. Asparagine is essential for protein synthesis, which takes place inside the cell, and decreased protein synthesis perhaps accounts for the immunosuppression and toxic effects of asparaginase-based treatment.
The prospects of enzyme-based treatment against cancer are very bright, but the difficulties of antigenicity and short circulation time remain to be overcome.
1.9.2.2. Enzymes in thrombolytic treatment
Activation of the blood clotting mechanism during inflammation is part of the body's defense mechanism which requires therapeutic intervention. Under normal physiological conditions there is an equilibrium between blood coagulation (clotting) and fibrinolysis (the process of dissolving the clotted blood) [ 47 ]. Biocatalysts such as enzymes, ribozymes, pro-enzymes, activators and pro-activators are responsible for maintaining equilibrium between clot formation and fibrinolysis. Imbalances in the concentration of these bio-activators may disturb physiology. In the biological process of fibrogenesis, clot formation takes place due to the plasma protein (soluble fibrinogen), which is ultimately converted to insoluble fibrin by the enzyme thrombin. This process is dependent on the conversion of thrombin from prothrombin. This bio-conversion takes place after the cascade of enzymatic reactions which involved certain key biological compounds called clotting factors. A blood clot dissolving enzyme known as plasmin is present in the blood as the pro-enzyme plasminogen. During clot dissolution activators convert the plasminogen to plasmin. This biological process is well regulated by certain process such as vasoconstriction, formation of a fibrin and clot platelet aggregation [ 46 ].
As the body utilizes enzymes in conserving this key balance of homeostasis, in a similar way we can utilize enzymes to repair or restore the homeostatic balance once it is lost. Several reports have shown that one of the best approaches for treating such clinical conditions is the administration of enzymes capable of converting plasminogen to plasmin (the enzyme which dissolves the clot) via intraveneous injection. This type of treatment is called therapeutic thrombolysis or thrombolytic therapy. In this treatment, pharmacological agents are used to medically induce clot breakdown [ 47 ]. Various novel thrombolytic agents have been derived from different sources for therapeutic use, such as from bacteria (streptokinase), the venom of the Malayan pit viper (Arvin), a filamentous fungus Koji mold Aspergillus oryzae (brinase), a South American snake (reptilase) and human urine (urokinase) [ 47 ].
Current advancements in thrombolytic therapy are more focused on the treatment of occlusions (blockages) of blood vessels. These types of therapy can be considered as life-saving and emergency medicine for life-threatening conditions such as myocardial infarction and massive pulmonary embolism, which are the most common reasons for cardiac arrest. This life-saving treatment is more reliable in preventing the blockages of vessels in the lungs and heart. Artery blockage conditions such as pulmonary embolism in the lungs by the formation of a clot creates tension on the right side of the heart, resulting in shortness of breath and chest pain mainly upon breathing in. Enzyme-based thrombolysis for treating massive pulmonary embolism has been considered as an effective approach to dissolving clots in these large vessels. Since surgical removal raises the chances of new blood clot formation that can cause another pulmonary embolism at the same or a different site, it is considered a dangerous practice and thrombolytic therapy is considered the more effective treatment [ 47 ]. Nevertheless, reoccurrence of clot formation or clot re-formation is very common in patients who have undergone enzyme-based thrombolytic treatment. Researchers from various organizations (1971) determined the effectiveness of streptokinase over heparin in reducing the chances of death in acute myocardial infarction patients. Significant results were obtained during this experiment. As discussed above, re-formation of the clot is one of the major concerns in fibrinolytic therapy. Most clinicians start treatment with a high dose of fibrinolytic agents, which is reduced later on. This approach may reduce disease progression for some time, but often increases the chances of clot re-formation. Even after the dissolution of the clot it is very difficult to maintain the same physiologically balanced environment (homeostasis) at the site of damaged tissues and the chance of new clot formation at that particular location is very high. Therefore, fibrinolytic based treatment is always accompanied by anticoagulants, such as heparin [ 46 ].
Major concerns associated with streptokinase therapy are fever, a tendency for bleeding, antigenicity (as with any foreign protein) and the difficulty of determining the proper dose [ 47 ]. Post-enzymatic treatment bleeding is one of the major concerns and it is also a concern when anticoagulants are used alone. According to current research, urokinase (produced in the kidneys and obtained from human urine) is considered safer than streptokinase. For the production of urokinase, 2300 l of urine is required to yield only 29 mg of purified urokinase, thus considering the expense involved in its manufacture, its clinical utilization has been restricted. Other examples are Arvin and reptilase. Utilization of these has been restricted for several reasons, but they are still considered as potential replacements for heparin as anticoagulants. Some researchers have noticed that optimum dose plays an important role and is one of the key factors in determining re-clot formation. Thorough investigation is required to overcome any shortcomings and increase the acceptance of these enzymes in therapeutic use [ 47 ].
1.9.2.3. The role of enzymes in digestive disorders and inflammations
Enzymes play an essential role in the management of various digestive disorders, such as exocrine pancreatic insufficiency [ 48 ]. Supplementation with enzymes may also be advantageous for other conditions associated with poor digestion, such as lactose intolerance. Generally, pancreatic enzymes such as porcine and bovine have been the preferred form of supplementation for exocrine pancreatic insufficiency [ 48 ]. Utilization of microbe-derived lipase has presented promise with reports showing benefits alike to pancreatic enzymes, but with a lower dosage concentration and a broader pH range. The safety and efficacy of enzymes derived from microbial species in the treatment of conditions such as malabsorption and lactose intolerance is promising. Plant-derived enzymes, e.g. bromelain from pineapple, serve as active digestive aids in the breakdown of proteins. Synergistic properties have also been reported using a combination of animal-based enzymes and microbe-derived enzymes or bromelain. Buccal administration of pancreatin (derived from an alcoholic extract of animal pancreas) enhances the enzymatic digestion of starch and proteins in patients with pancreatic cysts and pancreatitis. Pancreatin in combination with lipase is used to treat patients with fatty stools. Hydrolytic enzymes such as papain and fungal extracts ( Aspergillus niger and Aspergillus otyzae ) are used to enhance absorption from the small intestine [ 49 ]. These fungal extracts comprise amylases and proteases along with cellulases, which support the breakdown of the otherwise indigestible fibers of cabbages, etc, and thus reduce dyspepsia and flatulence [ 50 ]. Currently, micro-organisms are used at a large scale for the production of therapeutic enzymes. Among various micro-organisms Saccharomyces cerevisiae, Saccharomyces fragilis, Bacillus subtilis and two Aspergillus species are considered safe by the FDA (USA) for obtaining oral β -galactosidase (from A. oryzae ) which is often used by patients suffering from inherited intestinal disease lactose deficiency [ 51 ]. Children with this genetic disorder children are incapable of digesting milk lactose. Enzymatic preparations such as β -galactosidase catalyze the conversion of lactose to glucose and galactose, which are quickly absorbed by the intestine. Other enzymatic preparations, e.g. penicillinase (from B. subtilis ) are often used to treat hypersensitivity reactions caused by the antibiotic penicillin [ 52 ]. This enzyme catalyzes the conversion of penicillin to penicillanic acid, which is non-immunogenic. In addition, microbial and plant hydrolases are also used to decrease inflammation and edema [ 53 ]. Thrombin, trypsin, chymotrypsin, papain, streptokinase, streptodornase and sempeptidase are under clinical trial investigation. These enzymatic preparations are administered orally and have considerable proteolytic activity in the serum. Streptodornase has also displayed pain-relieving action on systemic injection [ 54 ]. Preparations have also been used to clean dirty wounds and necrotic tissue and to remove debris from second and third degree burns.
1.10. Plants and algae enzyme systems
Plant based foods are usually consumed in their raw form [ 68 ]. This eases the main concern with animal-based enzymes by preserving the integrity of the enzymes themselves. Moreover, plant-based digestive enzymes are effective over a broad scope of pH levels. This range is usually between 3.0 and 9.0, which is highly well-matched with the human gastrointestinal environment [ 55 – 72 ]. Thus plant-based enzymes are compatible for supporting comprehensive digestive health. Protease, amylase, lipase and cellulose are the important enzymes and are present in plants. Protease breaks down protein that can be present in meat, fish, poultry, eggs, cheese and nuts. Amylase assists your body with the breakdown and subsequent absorption of carbohydrates and starches. Lipase aids the digestion of fat. When your diet includes lipase-rich foods, it eases the production burden on the gall bladder, liver and pancreas. Cellulase is present in many fruits and vegetables, and it breaks down food fibers, which increases their nutritional value to our bodies. The presence of cellulase in plant-based sources is important, because it is not naturally present in the human body. Fruits and vegetables are an ideal source for enzymes. They are enzyme-rich and easily consumed without needing to be cooked or processed, ultimately preserving the full functionality of the enzymes. By using plant biotechnology several enzymes can be produced from plants as well algal resources [ 56 – 72 ].
During algal photosynthesis various proteins and enzymes are produced which can be utilized in economic development and environment management, such as in wastewater treatment, production of fine chemicals, and biodiesel production [ 56 – 72 ]. Due to their potential to capture and fix carbon dioxide using solar energy, photosynthetic marine algae are considered as potential models for the production of proteins. It has been recently observed that algal chloroplasts can be transformed for the production recombinant proteins [ 55 ]. Five different classes of recombinant enzymes; xylanase, α-galactosidase, phytase, phosphate anhydrolase, and β-mannanase, D. tertiolecta or C. reinhardtii were in the plastids of D. tertiolecta or C. reinhardtii. Similar strategies should allow for recombinant protein production in many species of marine algae [ 55 ].
- Go to reference in chapter
Export references: BibTeX RIS
B, brain; E, erythrocytes; H, heart muscle; Ht, hepatobiliary tract; I, intestinal mucosa; K, kidney; L, M, skeletal muscle; Pa, pancreas; P1, placenta; Pr, prostate gland; S, saliva.
Class 3 Hydrolases
EC 3.1-3.4.21
- © 2009
- Antje Chang 0
Technical University Braunschweig Bioinformatics & Systems Biology, Braunschweig, Germany
You can also search for this editor in PubMed Google Scholar
- Offers concise and complete description of about 5,000 enzymes sufficiently well characterized as well as their application in analytical, synthetic and biotechnology processes, in food industry, and for medicinal treatments
- This new, second edition reflects considerable progress in enzymology: many of the enzymes have either been newly classified, or re-classified
- Content in this new edition has doubled: now consists of 39 volumes as well as a synonym index
- Starting in 2009 all newly classified enzymes are treated in the Supplement Volumes
- Available in print as well as online
Part of the book series: Springer Handbook of Enzymes (HDBKENZYMES, volume S5)
42k Accesses
This is a preview of subscription content, log in via an institution to check access.
Access this book
- Available as PDF
- Read on any device
- Instant download
- Own it forever
- Compact, lightweight edition
- Dispatched in 3 to 5 business days
- Free shipping worldwide - see info
- Durable hardcover edition
Tax calculation will be finalised at checkout
Other ways to access
Licence this eBook for your library
Institutional subscriptions
Table of contents (60 chapters)
Front matter, polyneuridine-aldehyde esterase, hormone-sensitive lipase, acetylajmaline esterase, quorum-quenching n-acyl-homoserine lactonase, pheophorbidase, phenylacetyl-coa hydrolase, bile-acid-coa hydrolase, choloyl-coa hydrolase, mannosyl-3-phosphoglycerate phosphatase, 2-phosphosulfolactate phosphatase, α-ribazole phosphatase, pyridoxal phosphatase, phosphoethanolamine/phosphocholine phosphatase, lipid-phosphate phosphatase, acireductone synthase, cyclic-guanylate-specific phosphodiesterase, ribonuclease d.
- Applied Microbiology
- Biochemistry
- Biotransformation
- Food Science
- Molecular Medicine
- biotechnology
About this book
Editors and affiliations, technical university braunschweig bioinformatics & systems biology, braunschweig, germany.
Antje Chang
Bibliographic Information
Book Title : Class 3 Hydrolases
Book Subtitle : EC 3.1-3.4.21
Editors : Antje Chang
Series Title : Springer Handbook of Enzymes
DOI : https://doi.org/10.1007/978-3-540-85703-7
Publisher : Springer Berlin, Heidelberg
eBook Packages : Biomedical and Life Sciences , Biomedical and Life Sciences (R0)
Copyright Information : Springer-Verlag Berlin Heidelberg 2009
Hardcover ISBN : 978-3-540-85702-0 Published: 19 May 2009
Softcover ISBN : 978-3-662-51884-7 Published: 23 August 2016
eBook ISBN : 978-3-540-85703-7 Published: 31 July 2009
Edition Number : 2
Number of Pages : XX, 462
Topics : Enzymology , Human Physiology , Pharmacology/Toxicology , Biochemistry, general , Molecular Medicine , Food Science
- Publish with us
Policies and ethics
- Find a journal
- Track your research
- Open access
- Published: 12 January 2006
Enzymes: An integrated view of structure, dynamics and function
- Pratul K Agarwal 1
Microbial Cell Factories volume 5 , Article number: 2 ( 2006 ) Cite this article
89k Accesses
116 Citations
5 Altmetric
Metrics details
Microbes utilize enzymes to perform a variety of functions. Enzymes are biocatalysts working as highly efficient machines at the molecular level. In the past, enzymes have been viewed as static entities and their function has been explained on the basis of direct structural interactions between the enzyme and the substrate. A variety of experimental and computational techniques, however, continue to reveal that proteins are dynamically active machines, with various parts exhibiting internal motions at a wide range of time-scales. Increasing evidence also indicates that these internal protein motions play a role in promoting protein function such as enzyme catalysis. Moreover, the thermodynamical fluctuations of the solvent, surrounding the protein, have an impact on internal protein motions and, therefore, on enzyme function. In this review, we describe recent biochemical and theoretical investigations of internal protein dynamics linked to enzyme catalysis. In the enzyme cyclophilin A, investigations have lead to the discovery of a network of protein vibrations promoting catalysis. Cyclophilin A catalyzes peptidyl-prolyl cis/trans isomerization in a variety of peptide and protein substrates. Recent studies of cyclophilin A are discussed in detail and other enzymes (dihydrofolate reductase and liver alcohol dehydrogenase) where similar discoveries have been reported are also briefly discussed. The detailed characterization of the discovered networks indicates that protein dynamics plays a role in rate-enhancement achieved by enzymes. An integrated view of enzyme structure, dynamics and function have wide implications in understanding allosteric and co-operative effects, as well as protein engineering of more efficient enzymes and novel drug design.
Introduction
Microbial cell factories operate as a collection of efficient molecular machines. The success of these factories depends on the efficiency of a particular class of biomolecules – protein enzymes. Enzymes are responsible for catalyzing reactions in a variety of biological processes in all living cells. It is well known that enzymes are highly efficient catalysts as they can accelerate reactions by as many as 17 orders of magnitude [ 1 , 2 ]. The factors that enable enzymes to provide the large enhancement of reaction rates; however, still remain a matter of discussion [ 3 , 4 ]. For more than a century, the activity of enzymes has been related to their structure; the "lock-and-key" and "induced-fit" hypotheses have suggested that the structural interactions between enzymes and the substrates play a role in enzyme catalysis [ 5 , 6 ]. Such a view is incomplete as it fails to explain allosteric and cooperative effects, as well as the detailed mechanism of the large rate-enhancement achieved by enzymes. Enzymes catalyze reactions on a wide range of time-scales, which are similar to the time-scales for various events of internal protein dynamics, raising the question whether dynamics and enzyme catalysis are interrelated or not (see Figure 1 ) [ 7 – 12 ]. It is known that protein dynamics plays a role in many aspects of enzyme function, including substrate/cofactor binding or release. Its connection to the substrate turnover step, however, has been challenging to ascertain.
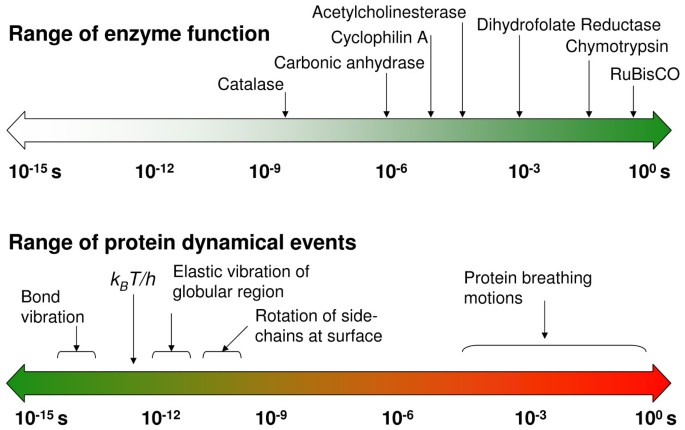
The range of time-scales involved in substrate turnover step of enzyme catalyzed reactions and internal protein dynamics are similar. Note the universal frequency factor (k B T/h), which is commonly used in transition state theory; k B is the Boltzmann's constant, T represents the ambient temperature and h is the Planck's constant.
An integrated view of protein structure, dynamics, and function is emerging, where proteins are considered as dynamically active machines and internal protein motions are closely linked to function such as enzyme catalysis. Currently there is wide interest, both from experimental and computational groups, in investigating this interconnection. A number of investigations have provided fascinating details about the movement of protein parts and their involvement in enzyme function. Techniques such as X-ray crystallography and small-angle scattering [ 13 , 14 ], NMR studies [ 15 – 17 ], hydrogen-deuterium exchange [ 18 ], neutron scattering [ 19 ], biochemical and mutational analysis [ 7 , 20 , 21 ] have provided vital clues at individual time-scales; however, the detailed understanding of protein dynamics requires information over a broad range of time-scales. Moreover, the hydration-shell and bulk solvent fluctuations have been suggested to impact protein dynamics, and therefore, protein function [ 22 , 23 ]. Theoretical studies and computational modeling are playing a vital role in investigating the link between protein dynamics, solvent fluctuations and enzyme catalysis at multiple time-scales [ 8 , 10 – 12 ].
In this review, we describe recent biochemical and theoretical/computational studies that have investigated the link between protein dynamics and enzyme catalysis. In particular, we describe the recent investigations of the peptidyl-prolyl cis/trans isomerization activity of the enzyme cyclophilin A, followed by a discussion on similar evidence from other enzyme reactions, namely the hydride transfer reactions catalyzed by dihydrofolate reductase and by liver alcohol dehydrogenase. There are wide implications of understanding the interconnection between protein structure, dynamics and function such as enzyme catalysis. It is known that enzymes catalyzing the same reactions belong to a protein "fold" family, where the overall characteristic shape of the protein is similar. Also, enzymes catalyzing mechanistically similar reactions often belong to the same super-family of protein fold. The benefits of better understanding of enzyme "folds" and dynamics include the possibility of improving the efficiency of microbial factories by engineering of enzymes, as well as designing new enzymes with novel functionalities. Further, there are medical implications of allosteric and cooperative effects for enzyme activity in novel drug design.
Cyclophilin A
The peptidyl-prolyl cis/trans isomerase (PPIase) activity of cyclophilin A (CypA) has been investigated in detail for the link between protein dynamics and enzymatic catalysis, both by biochemical experiments and theoretical methods [ 10 – 12 , 15 , 16 ]. CypA is a ubiquitously expressed cytosolic protein, which was discovered as the major intracellular receptor protein for the immunosuppressive drug cyclosporin A [ 24 , 25 ]. CypA belongs to the cyclophilin class of enzymes, which are involved in many biological reactions including protein folding, intracellular protein transport and signaling [ 26 , 27 ]. CypA acts as a PPIase, catalyzing the isomerization of peptidyl-prolyl amide bonds that are N-terminal to proline residues in a wide variety of peptides and protein substrates (see Figure 2 ) [ 26 , 28 ]. Human CypA is a single peptide chain with 165 amino acids. Its molecular architecture consists of an eight-stranded anti-parallel β-barrel with hydrophobic residues forming a core at the center and the active-site located on one face of the molecule (see Figure 3 ) [ 29 – 31 ]. In addition to the β-strands and α-helices, there are several flexible surface loop regions as indicated by large temperature factors from X-ray crystallographic studies.
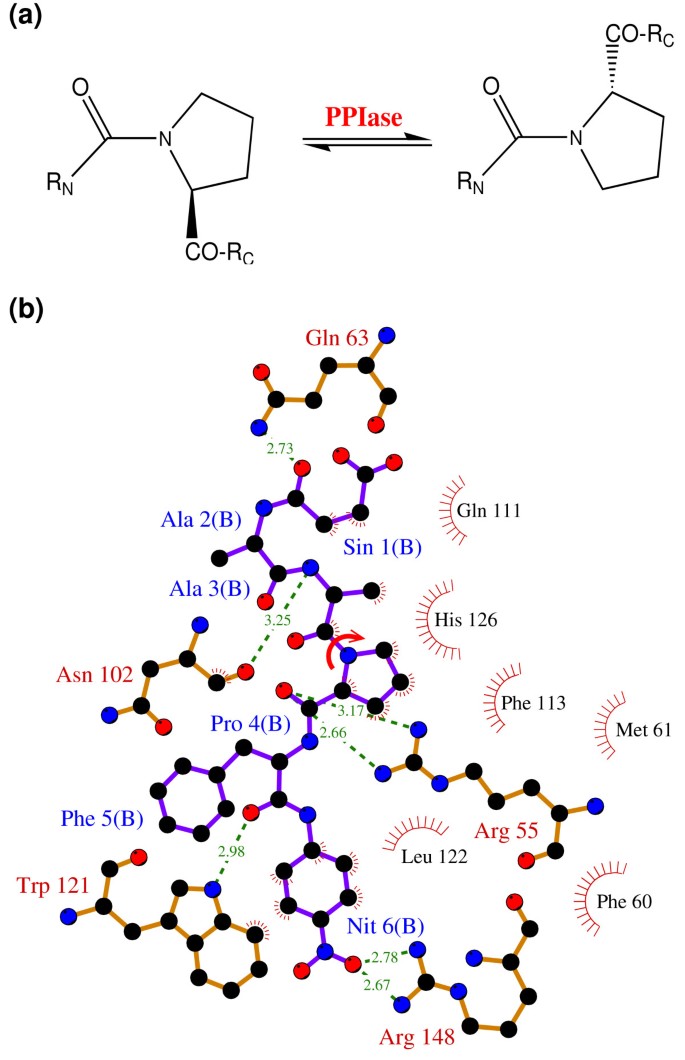
The reaction catalyzed by CypA (a) CypA is a member of a family of enzymes known as PPIase, which catalyze the cis/trans isomerization of peptide bonds N-terminal to proline residues in peptides and proteins (b) The active-site of CypA with a peptide substrate. The shown substrate has the sequence succinyl(Sin)-Ala-Ala-Pro-Phe- p -nitroanilide(Nit) and is labeled as chain B. The red arrow indicates the catalyzed isomerization. Several residues are conserved for their role in enzyme reaction. The dynamical motion of these hydrophobic and hydrophilic residues is linked to the substrate turnover step [10–12]. The green lines indicate hydrogen bonds between substrate and enzyme, while the hydrophobic interactions are depicted by small red radiating lines.
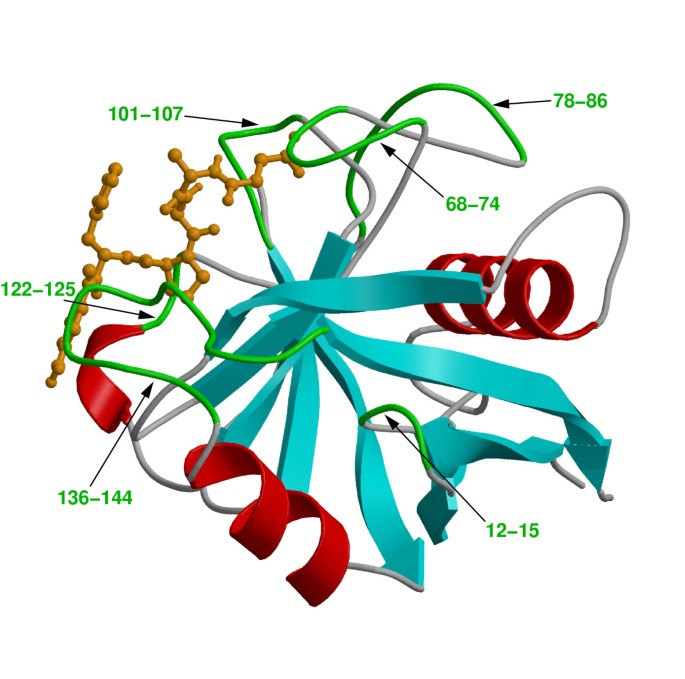
Three-dimensional structure of CypA. Protein secondary structure is represented with cyan arrows (β-sheets) and red helices (α-helices) based on crystal structure from Zhao and Ke (PDB code: 1RMH) [29]. The green labeled regions are flexible surface loops, showing large displacements in X-ray structures (large temperature factors) and NMR relaxation studies. A peptide substrate is shown as orange ball-and-stick model.
A number of factors make CypA an attractive system for investigating the link between internal protein dynamics and enzymatic activity; it is a small protein and does not require metal ions or cofactors for PPIase activity and it catalyzes peptide bond isomerization in a wide variety of substrates. Further, there is also biomedical interest in CypA; cyclophilins are of interest as drug targets because of their likely involvement in the broad spectrum, anti-infective activities of cyclosporin A and non-immunosuppressive derivatives thereof [ 32 , 33 ]. In addition, Gag-encoded capsid protein (CA) from human immunodeficiency virus type 1 (HIV-1), is a naturally occurring biologically relevant substrate for CypA [ 16 ]. The protein-protein complex between CypA and CA has been the subject of many experimental studies [ 16 , 34 – 37 ]. There is medical interest in CypA-CA complex, as incorporation of CypA is required for infectious activity of HIV-1 [ 38 , 39 ].
Genomic analysis based on multiple sequence alignment has identified conserved residues in the CypA active-site and also distal to the active-site. This analysis was based on aligning 50 PPIase sequences from 25 diverse organisms, ranging from bacteria to human [ 10 ]. The results from this analysis are depicted in Figure 4 . Detailed structural insights have indicated that the active-site of CypA shows conserved residues forming crucial hydrophobic and hydrophilic interactions with the substrate residues [see Figure 2(b) ]. In addition, there are several conserved and semi-conserved residues that are more than 12 Å from the active-site. Until recently, the role of these distal residues in the enzyme function was not very well understood. As described below the dynamical motions of some of these residues have been found to play a role in catalysis.
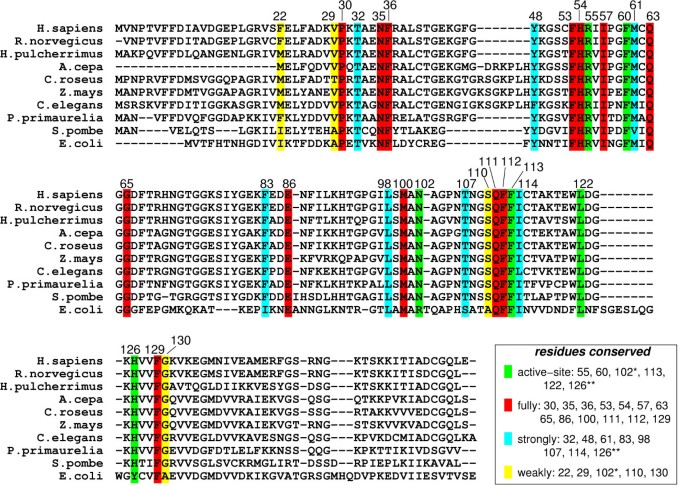
Genomic analysis for sequence conservation of CypA. Full analysis was performed on 50 sequences from species ranging from bacteria to human, results from 10 representative sequences are listed above. 17 of 165 amino acid residues in the human CypA sequence were found to be conserved in all 50 PPIases sequences examined: Pro30; Asn35; Phe36; Phe53; His54; Arg55; Ile57; Phe60; Gln63; Gly65; Glu86; Met100; Gln111; Phe112; Phe113; Leu122; and Phe129. Eight additional residues were found to be strongly conserved: Thr32; Tyr48; Met61; Phe83; Leu98; Thr107; Ile114 and His126. Five other residues were found to be weakly conserved: Phe22; Val29; Asn102; Ser110 and Gly130. Conserved active-site residues (fully conserved Arg55, Phe60, Leu122; strongly conserved His126**; and weakly conserved Asn102*) are shown with green background. Red background shows fully conserved residues distal to the active-site; residues with cyan background are strongly conserved; and residues with yellow background are weakly conserved.
NMR studies of CypA performed by Kern and coworkers, have suggested a link between internal protein dynamics and substrate isomerization step [ 15 , 16 ]. The studies were based on 15 N spin relaxation investigations of small peptide substrate as well as two-dimensional (2D) 1 H- 15 N heteronuclear exchange studies of the N-terminal of capsid protein (CA N ) from HIV-1. In these studies, conformational fluctuations within the active-site of CypA were detected on the time-scale of the reaction (hundreds of microseconds) and the rates of conformational dynamics were found to be strongly correlated with the substrate isomerization step. Several active-site and surface loop regions showed motions only in the presence of substrate, these regions included the residues: Arg55, Lys82, Leu98, Ser99, Ala101, Asn102, Ala103, and Gly109. Based on these studies, the authors proposed a reaction mechanism for PPIase activity of CypA, where the isomerization step takes place with a rate constant of about 9000 s -1 , and motions of the protein coincide with the rate of substrate turnover step. CypA residue Arg55 is a major contributor to catalysis [ 40 ], for which the observed changes in backbone conformation are likely to be coupled with motions of the catalytically essential side chain.
Theoretical and computational modeling of the PPIase activity of CypA has provided novel insights into understanding the relationship between dynamical events in proteins and enzyme catalysis, including the mechanism of rate-enhancement achieved by enzymes [ 10 – 12 ]. Note, the time-scale of CypA reaction is hundreds of microseconds, which is beyond the reach of present day molecular dynamics simulations. Molecular dynamics, the commonly used computational technique, is limited to nanosecond time-scale (up to 100 nanoseconds at best) due to the limitation of available computing power. A different theoretical framework was, therefore, used for detailed computational modeling of the entire reaction pathway. This framework is described briefly below, more details are available in other reviews [ 9 , 41 ]. The theoretical investigations of enzyme catalysis are based on description of the reaction using transition state theory (TST) and generation of a free energy profile as a function of suitable reaction coordinate. In the TST framework, protein dynamics has been suggested to impact the reaction rate in two ways. Enzymes can either decrease the activation energy barrier (ΔG ‡ ) for the reaction or alter the active-site conditions such that more reactive trajectories are converted to product successfully. Figure 5 shows the behavior of two trajectories, the first trajectory crosses the transition state (TS) barrier but is unsuccessful and returns to the reactant side. The second trajectory crosses the barrier several times before becoming productive. Transmission coefficient (κ) is a corrective pre-factor corresponding to the fraction of reactive trajectories that successfully cross the TS barrier and become productive. For CypA, the free energy profiles were generated using the amide bond dihedral angle of the peptide bond as reaction coordinate. Note, in the computational studies described here the unit of reaction coordinate is degrees (°). The free energy profiles were generated for isomerization of 3 small peptide substrates as well as the biologically relevant substrate CA N . The procedure for generation of these profiles requires multiple simulations of small sections along the reaction path by using molecular dynamics and umbrella sampling [ 42 ], and combining them to provide information regarding the time-scale of the reaction [ 43 ]. More details about the computational methods can be found in refs. [ 10 ] and [ 11 ]. In addition to obtaining the free energy profile this modeling procedure also sampled the enzyme-substrate conformations along the entire reaction pathway. These conformations have been used for detailed analysis of structural and dynamical changes during the enzyme reaction mechanism.
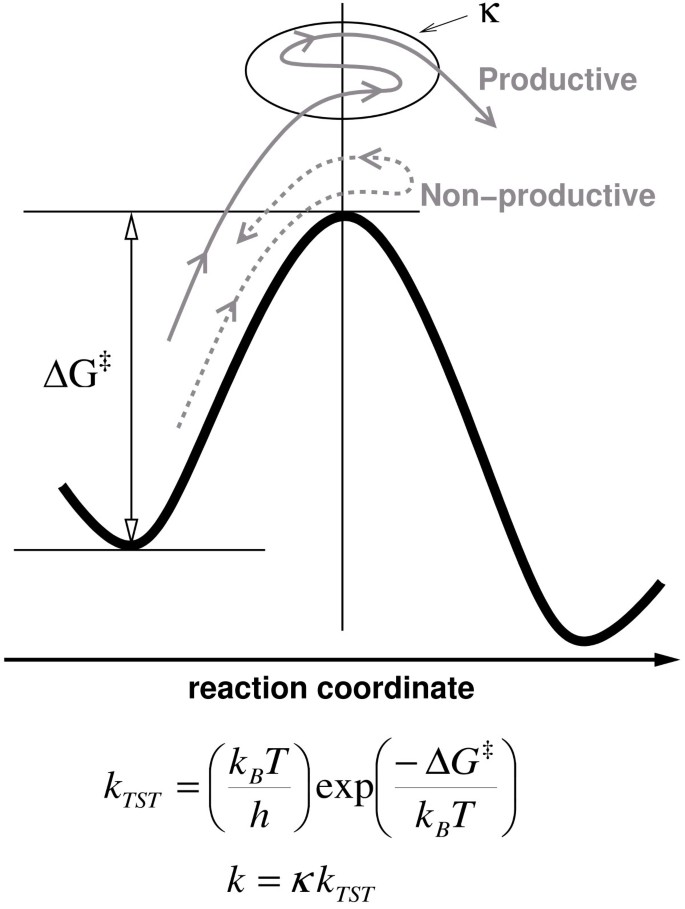
Schematic illustration of free energy profile for an enzymatic reaction. Protein dynamics can influence reaction rates in two possible ways; by altering height of the activation free energy barrier (ΔG ‡ ) and transmission coefficient (κ). k B is the Boltzmann's constant, T is the temperature, h is the Planck's constant and k TST represents the transition state theory reaction rate.
Structural analysis of the active-site along the course of reaction indicates the role of important hydrophilic (Arg55 and Asn102) and hydrophobic (Phe60, Phe113, Leu122 and His126) residues of CypA in stabilization of the substrate peptide. The target proline from substrate remains essentially fixed in the hydrophobic pocket formed by CypA residues, while the carbonyl oxygen atom of the preceding substrate residue rotates 180°. Quantum mechanical modeling of the active-site indicates single bond character for the peptide bond near the TS. The results from theoretical modeling were found to be in agreement with the reaction mechanism proposed on the basis of crystallographic studies [ 37 ]. This mechanism requires minimum deviation from the ground state crystal structure and displays single bond character for the peptide bond near TS. Dynamical fluctuations of the enzyme backbone in certain regions (CypA 101–104) were found to impact the nature of interactions between the enzyme and substrate, therefore, alter the nature of peptide bond during the course of reaction mechanism.
Computational modeling has identified a variety of internal protein dynamical events linked to CypA enzyme activity, ranging from femtosecond (10 -15 s) to microsecond and longer (> 10 -6 s) time-scales. On one side of this range there are fast motions, occurring at femtosecond-nanosecond time-scales, consisting of harmonic movements of bonds, angles and a few atoms. These motions are commonly referred to as vibrations. On the other side of this range there are concerted conformational fluctuations occurring on the microsecond (and longer) time-scale. These slower motions or conformational fluctuations, which have been previously referred to as breathing motions , span a large part of the protein. Normal mode analysis is a computational technique that has been commonly used to obtain information regarding the dynamical motions in proteins. This technique provides information about dynamics at several time-scales for a particular protein conformation (present at a local minimum). Normal mode analysis is not suitable for obtaining the slow protein motions occurring at the time-scale of the reaction due to the large changes in protein conformations involved. Another computational technique, known as quasi-harmonic analysis, can be used to calculate vibrational modes from a collection of conformations or system snapshots [ 44 ]. Quasi-harmonic analysis of CypA-substrate conformations along the entire reaction pathway provided protein vibrational modes representing conformational fluctuations at the time-scale of the reaction (microsecond-millisecond time-scale). These computed slow protein vibrational modes show concerted motions over a large region of the protein, the backbone in several regions of the protein and side-chains of the many residues (especially on the surface) show large displacements. In CypA, a subset of these modes was found to be coupled to the reaction; 3 protein vibrational modes with the largest coupling to the catalytic step show displacements in several conserved residues in the active-site as well as in other parts of the enzyme structure. Note, these conserved vibrational modes are different from random thermal fluctuations observed in the biomolecules.
The detailed characterization of internal protein dynamics events linked to enzyme catalysis in CypA has lead to the discovery of a network of protein vibrations (see Figure 6 ). This network plays an important role in promoting the isomerization reaction [ 10 – 12 ]. The discovery of this network is based on identification of 3 protein vibrations on the time-scale of the reaction, investigation of the dynamical flexibility of the CypA backbone, monitoring the conserved residues and interactions over the course of enzyme reaction. Dynamical cross-correlation analysis of enzyme parts indicated that several surface loops (distant to each other in sequence) show highly correlated motions during the course of the reaction. These correlated motions form the network of vibrations through a series of interactions, as shown by yellow arrows in Figure 6 . Note that this network extends from the surface regions of the enzyme all the way to the active-site, through interconnection of conserved residues and interactions. The vibrations in this network are transmitted to the active-site, where dynamical motions alter the crucial hydrophobic and hydrophilic interactions between enzyme and substrate. As noted above these interactions play a critical role in the reaction mechanism by controlling the nature of the peptide bond, as well as in rotation of the carbonyl oxygen atom from the residue preceding the target proline of the substrate. Evidence for the existence of this network comes from previous NMR studies, where motions have been detected in network residues only during the substrate turnover [ 15 ]. Further, the flexibility of the network residues is confirmed by observation of large temperature factors in X-ray studies [ 29 – 31 , 37 ]. More recently, new investigations performed by Kern and coworkers, using NMR studies have confirmed the presence of this network in CypA [ 45 ]. Moreover, NMR investigations conducted by Blackledge and coworkers have also observed the role of protein motions in transfer of information between β-strands [ 46 ]. These recent findings have illustrated the role of coupled networks in propagation of local changes over large distances in protein structure.
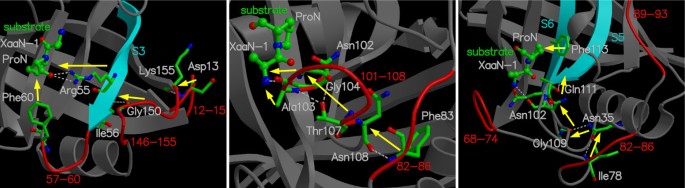
A network of coupled protein vibration promoting catalysis in cyclophilin A [10]. Alternate pathways by which protein dynamics impacts the enzyme reaction are depicted based on 3 protein vibrational modes coupled to the reaction. Loops colored in red and residues indicated by ball-and-stick show largest displacements in vibrational modes coupled to the substrate turnover step. The yellow arrows represent the network pathway from outside of the enzyme to the active-site. Reprinted with permission from Agarwal et al., Biochemistry (2004) 43 , 10605–10618 . Copyright 2004 American Chemical Society.
The discovered network of protein vibrations has a promoting effect on the CypA enzyme activity, and is therefore, a factor contributing to rate-enhancement. Certain protein vibrational modes alter the reaction by changing the active-site environment such that more reaction trajectories cross to the product side. A new theoretical technique has been designed and was used to investigate impact of reaction coupled vibrational modes on the reaction mechanism [ 12 ]. This technique allows addition of kinetic energy to a selective vibrational mode and observing the dynamical behavior of the trajectory (see Figure 7 ). Figure 7(a) shows the change in behavior of trajectories with increasing amount of kinetic energy present in a protein vibrational mode. The result from further investigations show that the presence of energy in certain reaction coupled promoting modes causes the reaction trajectories to overcome the activation energy barrier quickly and more effectively [see Figure 7(b)–(c) ]. Note that not all modes promote the reaction, as indicated by a non-promoting mode [that is a mode which is not coupled to the reaction; see Figure 7(d) ]. Also, the theoretical investigations were performed by adding varying amounts of energy to see the effect of these vibrations in a short simulation (picosecond time-scale). The trend indicates that smaller amounts of kinetic energy present in these modes, which is expected to be present in real system, promotes the reaction at longer time-scales (hundreds of microseconds). The biophysical role of the discovered network in the enzyme reaction can be understood by observing changes that are introduced in the active-site by reaction promoting vibrations. Detailed analysis indicates that the dynamical behavior of reaction trajectories is correlated with the fluctuations in the enzyme-substrate interactions as a result of increased energy in the protein vibrational mode. Rate-enhancing modes impact the key active-site interactions to make the reaction proceed from reactant side to the product side. An interesting observation, from this analysis, is that the maximum enzyme stabilization occurs close to the TS (consistent with the TS stabilization theory for enzyme catalysis). The role of the reaction promoting vibrations could, therefore, be interpreted as internal protein dynamical events that facilitate in the stabilization of the TS.
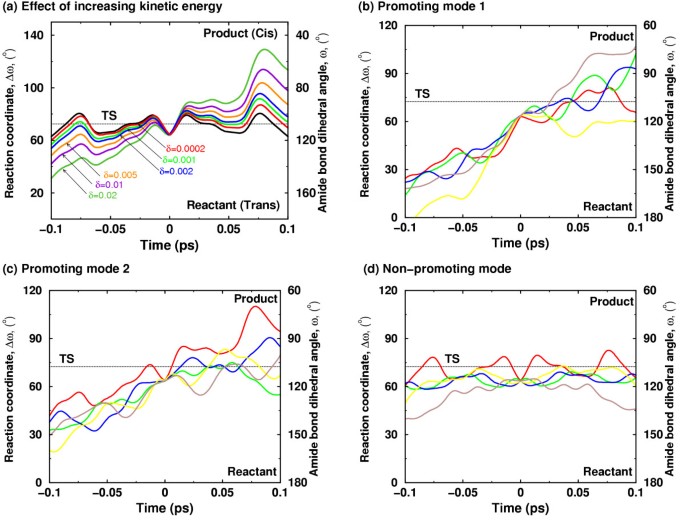
Effect of additional kinetic energy in selective protein vibrational modes (a) Increased amount of kinetic energy in a mode coupled to the enzyme reaction allows the trajectory to cross the barrier successfully from the reactant side to the product side. The solid black curve represents the native trajectory (no additional kinetic energy), and δ indicates the fraction of system kinetic energy added to the protein vibrational mode. (b)-(d) representative trajectories from simulations with increased kinetic energy in network protein vibrational modes and a non promoting mode. 2% of system kinetic energy was added to protein vibrations mode. Five representative trajectories from each mode are shown in different colors. Not all protein vibrational modes show increased barrier recrossing; much less effect on the barrier crossing is seen in a mode not coupled to the reaction [12]. Reprinted with permission from Agarwal et al., J. Am. Chem. Soc. (2005) 127 , 15248–15256 . Copyright (2005) American Chemical Society.
Solvent surrounding the enzyme also plays a role in the enzyme reaction. In many enzyme reactions, hydrolysis of small molecules provides the energy for overcoming the activation energy barrier; however, in other cases the required energy is provided by the thermodynamical fluctuations of the solvent. The fluctuations in the hydration-shell and bulk-solvent surrounding the enzyme are correlated with the internal protein motions. Detailed characterization of the flexible surface loop regions indicates that the side-chains of several surface residues extend into the solvent and the motion of these residues is coupled to the motion of surrounding solvent molecules. CypA investigations indicated the presence of vibrations (on picosecond) time-scale in several crucial surface residues, which are present in the loop regions showing large displacements in reaction promoting vibrational modes. Previous theoretical investigations have also shown that the transition in internal motion of proteins can be driven by the temperature of the solvent [ 47 , 48 ]. In CypA, computational modeling has shown transfer of energy from solvent to the external regions of the enzyme. This energy transfer changes the behavior of reaction trajectories, through the network of protein vibrations, to promote catalysis (see Figure 8 ).
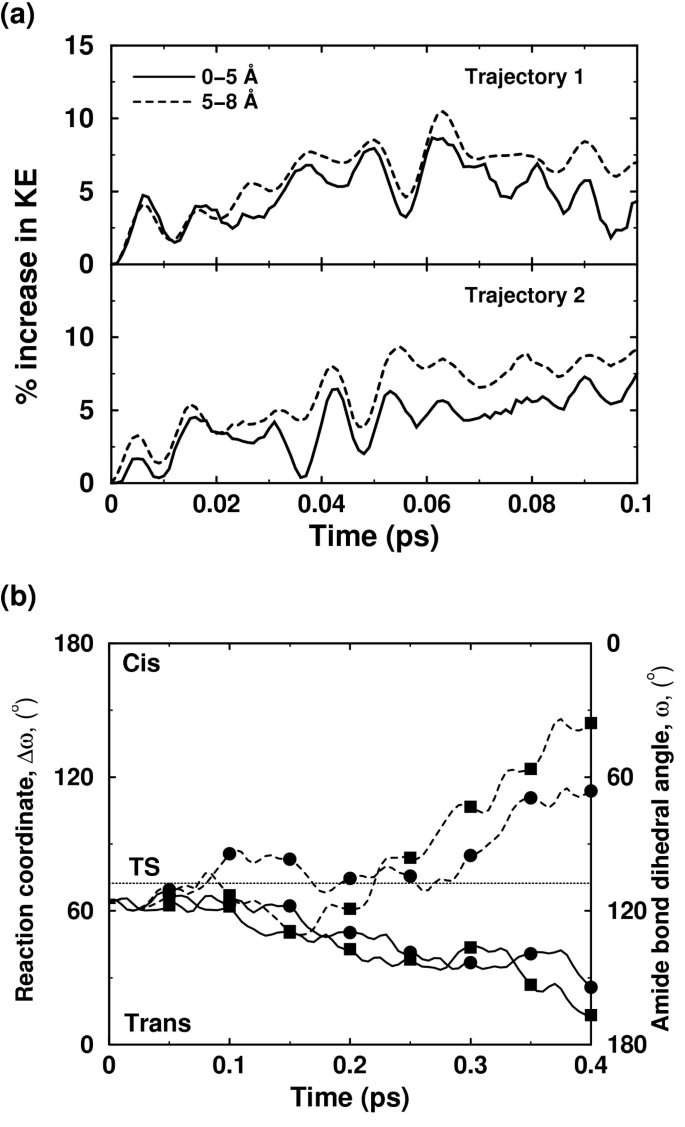
Effect of additional kinetic energy in first solvation shell of an enzyme. (a) Kinetic energy is transferred from the solvent to the protein residues, as indicated by increasing energy in the protein regions (up to 5 Å from protein surface, and between 5 Å and 8 Å from the surface) (b) Two otherwise non-productive regular trajectories (solid lines) become productive (broken lines) due to transfer of energy from the solvent to residues forming parts of the protein vibrations network. The corresponding trajectories are indicated by squares and circles [12]. Reprinted with permission from Agarwal et al., J. Am. Chem. Soc. (2005) 127 , 15248–15256 . Copyright (2005) American Chemical Society.
An interesting outcome of detailed characterization of the network of protein vibrations in CypA is the insight into the conservation of protein residues. The genomic analysis for sequence conservation reveals that there are several residues which are conserved due to their dynamical role in catalysis. Active-site residues, which are key players in the catalytic step, are often conserved in different species. In addition to the active-site residues (Arg55, Phe60, Asn102 and Ala103), which directly participate in the catalytic step, there are several distal residues (including Pro30, Asn35, Phe36, Phe83, Glu86), which are also conserved. Note, that some of these residues are more than 17 Å from the active-site. Results from detailed structural analysis (summarized in Table 1 ) indicate that these and other distal residues form crucial points and hydrogen bonds in the discovered network and, therefore, are conserved in species ranging from bacteria to human. This finding also has interesting implications on the understanding of the secondary and tertiary protein structure. The discovered network promoting catalysis may provide some insights into the conservation of protein "folds"; enzymes catalyzing similar reactions often belong to the same fold family, and enzymes catalyzing mechanistically similar reactions belong the same protein super-family [ 49 , 50 ].
Other enzymes: dihydrofolate reductase and liver alcohol dehydrogenase
Experimental and computational investigations have revealed the impact of protein dynamics on catalysis in other enzyme systems. Experimental and computational studies of the enzyme dihydrofolate reductase (DHFR) have indicated a link between protein dynamical events and the substrate turnover step of hydride transfer. X-ray crystallography has demonstrated changes in orientation of surface loops along different sub-states along the reaction pathway [ 51 ]. Similarly, the surface loop conformations have been linked to the catalytic step by NMR studies [ 20 ]. Theoretical and computational studies using hybrid quantum-mechanical and molecular mechanics (QM/MM) methodology have discovered a network of coupled promoting motions [ 8 , 52 , 53 ]. Similar to the network of protein vibrations in CypA described above, the network in DHFR is also formed by interconnection of residues and crucial interactions ranging from surface regions all the way to active-site. Changes in hydrogen-bonds and crucial interactions along the reaction profile have been observed similar to those present during catalysis by CypA. An important discovery by the computational methods was the identification of the residue Ile14 as a dynamical contributor to catalysis. Recently, the importance of this residue in the catalytic step has been confirmed by NMR studies [ 54 ]. The presence of this DHFR network has been confirmed by investigations from several research groups [ 55 , 56 ]. Investigations of DHFR have provided evidence that changing the enzyme structure leads to changed dynamics and, therefore, change in function [ 21 , 53 , 57 ]. Mutation of a single surface residue, more than 12 Å away from active site, changes the dynamics and leads to a rate reduction by a factor of 163.
Liver alcohol dehydrogenase (LADH) is another enzyme where dynamical motions of the protein residues have been linked to the catalytic step. Detailed biochemical and computational studies have identified conserved active-site residue Val203, whose motion are a key player in altering the active-site chemical environment to promote the reaction [ 58 – 63 ].
In this review, we have presented recent developments that continue to support an emerging integrated view of protein structure, dynamics and function such as enzyme catalysis. The success of microbial cell factories depends on optimal performance of molecular machines inside the cell. Enzymes perform their function with remarkable efficiency, as they increase the reaction rate by many orders of magnitude. Until recently, enzymes (and proteins in general) were considered static assemblies; however, recent investigations continue to provide evidence which indicate that enzymes are dynamically active assemblies. Detailed experimental and theoretical/computational investigations of enzyme CypA suggest that the internal protein motions are a designed part of the protein structure and contribute to its function of catalyzing peptidyl-prolyl cis/trans isomerization. Supporting evidence from other systems (DHFR and LADH) indicates that the interconnection between structure, dynamics and function is present in other enzymes as well.
The overall emerging picture of protein dynamics, solvent fluctuations and enzyme function based on recent insights is depicted in Figure 9 . Along with structural interactions, internal motions at fast time-scales control the chemical environment of the active-site favoring the catalytic step to proceed to the product state. The thermodynamical fluctuations of the hydration-shell and bulk solvent provide energy to overcome the activation energy barrier (in cases where no other source of energy is available). The flexible surface loop regions of the enzyme show dynamical coupling with the solvent. This dynamical coupling allows the transfer of energy from the solvent to the surface regions of the enzyme. This energy is eventually transferred to the active-site through networks of motions or vibrations. The slower conformational fluctuations in the networks (at time-scale of the reaction) alter the enzyme-substrate interactions such that more reaction trajectories cross TS barrier to reach the product state successfully.
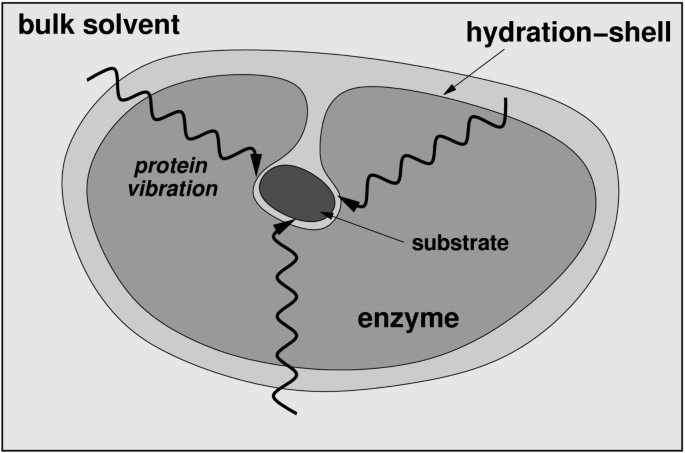
A schematic representation of the integrated view of enzyme structure, dynamics and function . Enzyme structure and internal protein dynamics events play a role in the catalytic step. Conserved residues from the surface to the active-site participate in network of protein motions or vibrations that promotes catalysis. The surface residues are coupled to the thermo-dynamical fluctuations of the solvent, and possibly play a role in transfer of energy from solvent to the protein.
The integrated view is supported by evidence from investigations of many other proteins and enzymes as well [ 64 – 66 ]. Sequence analysis with thermodynamic mapping have indicated long range energetic coupling in proteins [ 67 ]; slow conformational fluctuations could possibly be the mechanism of energy transfer over long ranges in protein structure and, therefore, provide insights into understanding allosteric effects. Simulations have already revealed that energy can be transferred between specific vibrational modes in a protein [ 68 , 69 ]. It is also interesting to note that designing active-site mimics of the enzymes is difficult and change in enzyme structure far away from the active-site leads to slow or inactive enzymes. The integrated view offers a possible explanation, as the distal regions of the enzyme contribute to catalysis through dynamical coupling with the solvent and by transferring the required energy to the active-site. Therefore, this integrated view has wide implications in enzyme chemistry, protein engineering and drug design. Manipulation of enzyme catalyzed reactions may be possible; for example, laser pulse has already been used to initiate an enzyme reaction involving thermally excited protein dynamics (molecular motions on picosecond time-scale) [ 70 ]. On the basis of better understanding of enzyme structure, dynamics and function it may be possible to design more efficient enzymes or enzymes with novel functionalities. Further, the understanding of allosteric and cooperative effects could help in designing better and novel drugs.
Abbreviations
transmission coefficient
activation energy barrier (energy difference between reactant and the activated state)
capsid protein from HIV-1
N-terminal of capsid protein
cyclophilin A
dihydrofolate reductase
human immunodeficiency virus type 1
liver alcohol dehydrogenase
nuclear magnetic resonance
peptidyl-prolyl cis/trans isomerase
transition state
transition state theory
Neet KE: Enzyme catalytic power minireview series. J Biol Chem. 1998, 273: 25527-25528. 10.1074/jbc.273.40.25527.
Article CAS Google Scholar
Radzicka A, Wolfenden R: A Proficient Enzyme. Science. 1995, 267: 90-93.
Kraut J: How Do Enzymes Work. Science. 1988, 242: 533-540.
Knowles JR: Enzyme Catalysis - Not Different, Just Better. Nature. 1991, 350: 121-124. 10.1038/350121a0.
Fischer E: Ber Dtsch Chem Ges. 1894, 27: 3189-
Haldane JBS: Enzymes. 1930, London, Longmans, Green
Google Scholar
Cannon WR, Benkovic SJ: Solvation, reorganization energy, and biological catalysis. J Biol Chem. 1998, 273: 26257-26260. 10.1074/jbc.273.41.26257.
Agarwal PK, Billeter SR, Rajagopalan PTR, Benkovic SJ, Hammes-Schiffer S: Network of coupled promoting motions in enzyme catalysis. Proc Natl Acad Sci U S A. 2002, 99: 2794-2799. 10.1073/pnas.052005999.
Benkovic SJ, Hammes-Schiffer S: A perspective on enzyme catalysis. Science. 2003, 301: 1196-1202. 10.1126/science.1085515.
Agarwal PK, Geist A, Gorin A: Protein dynamics and enzymatic catalysis: investigating the peptidyl-prolyl cis-trans isomerization activity of cyclophilin A. Biochemistry. 2004, 43: 10605-10618. 10.1021/bi0495228.
Agarwal PK: Cis/trans isomerization in HIV-1 capsid protein catalyzed by cyclophilin A: insights from computational and theoretical studies. Proteins: Struct Func Bioinform. 2004, 56: 449-463. 10.1002/prot.20135.
Agarwal PK: Role of protein dynamics in reaction rate enhancement by enzymes. J Am Chem Soc. 2005, 127: 15248-15256. 10.1021/ja055251s.
Schramm VL, Shi WX: Atomic motion in enzymatic reaction coordinates. Curr Opin Struct Biol. 2001, 11: 657-665. 10.1016/S0959-440X(01)00269-X.
Heller WT: Influence of multiple well defined conformations on small-angle scattering of proteins in solution. Acta Crystallogr D. 2005, 61: 33-44. 10.1107/S0907444904025855.
Article Google Scholar
Eisenmesser EZ, Bosco DA, Akke M, Kern D: Enzyme dynamics during catalysis. Science. 2002, 295: 1520-1523. 10.1126/science.1066176.
Bosco DA, Eisenmesser EZ, Pochapsky S, Sundquist WI, Kern D: Catalysis of cis/trans isomerization in native HIV-1 capsid by human cyclophilin A. Proc Natl Acad Sci U S A. 2002, 99: 5247-5252. 10.1073/pnas.082100499.
Wand AJ: Dynamic activation of protein function: A view emerging from NMR spectroscopy. Nat Struct Biol. 2001, 8: 926-931. 10.1038/nsb1101-926.
Zavodszky P, Kardos J, Svingor A, Petsko GA: Adjustment of conformational flexibility is a key event in the thermal adaptation of proteins. Proc Natl Acad Sci U S A. 1998, 95: 7406-7411. 10.1073/pnas.95.13.7406.
Zaccai G: Biochemistry - How soft is a protein? A protein dynamics force constant measured by neutron scattering. Science. 2000, 288: 1604-1607. 10.1126/science.288.5471.1604.
Osborne MJ, Schnell J, Benkovic SJ, Dyson HJ, Wright PE: Backbone dynamics in dihydrofolate reductase complexes: Role of loop flexibility in the catalytic mechanism. Biochemistry. 2001, 40: 9846-9859. 10.1021/bi010621k.
Cameron CE, Benkovic SJ: Evidence for a functional role of the dynamics of glycine-121 of Escherichia coli dihydrofolate reductase obtained from kinetic analysis of a site-directed mutant. Biochemistry. 1997, 36: 15792-15800. 10.1021/bi9716231.
Fenimore PW, Frauenfelder H, McMahon BH, Young RD: Bulk Solvent and hydration-shell fluctuations, similar to a- and b-fluctuations in glasses, control protein motions and functions. P Natl Acad Sci USA. 2004, 101: 14408-14413. 10.1073/pnas.0405573101.
Frauenfelder H, Fenimore PW, McMahon BH: Hydration, slaving and protein function. Biophys Chem. 2002, 98: 35-48. 10.1016/S0301-4622(02)00083-2.
Handschumacher RE, Harding MW, Rice J, Drugge RJ: Cyclophilin - a Specific Cytosolic Binding-Protein for Cyclosporin-A. Science. 1984, 226: 544-547.
Takahashi N, Hayano T, Suzuki M: Peptidyl-Prolyl Cis-Trans Isomerase Is the Cyclosporin-a-Binding Protein Cyclophilin. Nature. 1989, 337: 473-475. 10.1038/337473a0.
Gothel SF, Marahiel MA: Peptidyl-prolyl cis-trans isomerases, a superfamily of ubiquitous folding catalysts. Cell Mol Life Sci. 1999, 55: 423-436. 10.1007/s000180050299.
Rovira P, Mascarell L, Truffa-Bachi P: The impact of immunosuppressive drugs on the analysis of T-cell activation. Curr Med Chem. 2000, 7: 673-692.
Fischer G: Chemical aspects of peptide bond isomerisation. Chem Soc Rev. 2000, 29: 119-127. 10.1039/a803742f.
Zhao YD, Ke HM: Crystal structure implies that cyclophilin predominantly catalyzes the trans to cis isomerization. Biochemistry. 1996, 35: 7356-7361. 10.1021/bi9602775.
Zhao YD, Ke HM: Mechanistic implication of crystal structures of the cyclophilin-dipeptide complexes. Biochemistry. 1996, 35: 7362-7368. 10.1021/bi960278x.
Vajdos FE, Yoo SH, Houseweart M, Sundquist WI, Hill CP: Crystal structure of cyclophilin A complexed with a binding site peptide from the HIV-1 capsid protein. Protein Sci. 1997, 6: 2297-2307.
Page AP, Kumar S, Carlow CKS: Parasite Cyclophilins and Antiparasite Activity of Cyclosporine-A. Parasitology Today. 1995, 11: 385-388. 10.1016/0169-4758(95)80007-7.
Chappell LH, Wastling JM: Cyclosporine-a - Antiparasite Drug, Modulator of the Host-Parasite Relationship and Immunosuppressant. Parasitology. 1992, 105: S25-S40.
Gamble TR, Vajdos FF, Yoo SH, Worthylake DK, Houseweart M, Sundquist WI, Hill CP: Crystal structure of human cyclophilin A bound to the amino- terminal domain of HIV-1 capsid. Cell. 1996, 87: 1285-1294. 10.1016/S0092-8674(00)81823-1.
Yoo SH, Myszka DG, Yeh CY, McMurray M, Hill CP, Sundquist WI: Molecular recognition in the HIV-1 capsid/cyclophilin a complex. J Mol Biol. 1997, 269: 780-795. 10.1006/jmbi.1997.1051.
Saphire ACS, Bobardt MD, Gallay PA: trans-complementation rescue of cyclophilin A-deficient viruses reveals that the requirement for cyclophilin A in human immunodeficiency virus type 1 replication is independent of its isomerase activity. J Virol. 2002, 76: 2255-2262. 10.1128/jvi.76.5.2255-2262.2002.
Howard BR, Vajdos FF, Li S, Sundquist WI, Hill CP: Structural insights into the catalytic mechanism of cyclophilin A. Nat Struct Biol. 2003, 10: 475-481. 10.1038/nsb927.
Braaten D, Franke EK, Luban J: Cyclophilin A is required for an early step in the life cycle of human immunodeficiency virus type 1 before the initiation of reverse transcription. J Virol. 1996, 70: 3551-3560.
CAS Google Scholar
Wiegers K, Krausslich HG: Differential dependence of the infectivity of HIV-1 group O isolates on the cellular protein cyclophilin A. Virology. 2002, 294: 289-295. 10.1006/viro.2001.1347.
Li G, Cui Q: What is so special about Arg 55 in the catalysis of cyclophilin A? insights from hybrid QM/MM simulations. J Am Chem Soc. 2003, 125: 15028-15038. 10.1021/ja0367851.
Garcia-Viloca M, Gao J, Karplus M, Truhlar DG: How enzymes work: Analysis by modern rate theory and computer simulations. Science. 2004, 303: 186-195. 10.1126/science.1088172.
Torrie GM, Valleau JP: Non-Physical Sampling Distributions in Monte-Carlo Free-Energy Estimation - Umbrella Sampling. J Comput Phys. 1977, 23: 187-199. 10.1016/0021-9991(77)90121-8.
Kumar S, Bouzida D, Swendsen RH, Kollman PA, Rosenberg JM: The Weighted Histogram Analysis Method for Free-Energy Calculations on Biomolecules .1. The Method. J Comput Chem. 1992, 13: 1011-1021. 10.1002/jcc.540130812.
Levy RM, Karplus M, Kushick J, Perahia D: Evaluation of the Configurational Entropy for Proteins - Application to Molecular-Dynamics Simulations of an Alpha-Helix. Macromolecules. 1984, 17: 1370-1374. 10.1021/ma00137a013.
Eisenmesser EZ, Millet O, Labeikovsky W, Korzhnev DM, Wolf-Watz M, Bosco DA, Skalicky JJ, Kay LE, Kern D: Intrinsic dynamics of an enzyme underlies catalysis. Nature. 2005, 438: 117-121. 10.1038/nature04105.
Bouvignies G, Bernado P, Meier S, Cho K, Grzesiek S, Bruschweiler R, Blackledge M: Identification of slow correlated motions in proteins using residual dipolar and hydrogen-bond scalar couplings. P Natl Acad Sci USA. 2005, 102: 13885-13890. 10.1073/pnas.0505129102.
Tournier AL, Xu JC, Smith JC: Translational hydration water dynamics drives the protein glass transition. Biophys J. 2003, 85: 1871-1875.
Tournier AL, Xu JC, Smith JC: Solvent caging of internal motions in myoglobin at low temperatures. Physchemcomm. 2003, 6-8. 10.1039/b209839c.
Gerlt JA, Babbitt PC: Mechanistically diverse enzyme superfamilies: the importance of chemistry in the evolution of catalysis. Curr Opin Chem Biol. 1998, 2: 607-612. 10.1016/S1367-5931(98)80091-4.
Babbitt PC, Gerlt JA: Understanding enzyme superfamilies - Chemistry as the fundamental determinant in the evolution of new catalytic activities. J Biol Chem. 1997, 272: 30591-30594. 10.1074/jbc.272.49.30591.
Sawaya MR, Kraut J: Loop and subdomain movements in the mechanism of Escherichia coli dihydrofolate reductase: Crystallographic evidence. Biochemistry. 1997, 36: 586-603. 10.1021/bi962337c.
Agarwal PK, Billeter SR, Hammes-Schiffer S: Nuclear quantum effects and enzyme dynamics in dihydrofolate reductase catalysis. J Phys Chem B. 2002, 106: 3283-3293. 10.1021/jp020190v.
Watney JB, Agarwal PK, Hammes-Schiffer S: Effect of mutation on enzyme motion in dihydrofolate reductase. J Am Chem Soc. 2003, 125: 3745-3750. 10.1021/ja028487u.
Schnell JR, Dyson HJ, Wright PE: Effect of cofactor binding and loop conformation on side chain methyl dynamics in dihydrofolate reductase. Biochemistry. 2004, 43: 374-383. 10.1021/bi035464z.
Garcia-Viloca M, Truhlar DG, Gao JL: Reaction-path energetics and kinetics of the hydride transfer reaction catalyzed by dihydrofolate reductase. Biochemistry. 2003, 42: 13558-13575. 10.1021/bi034824f.
Thorpe IF, Brooks CL: The coupling of structural fluctuations to hydride transfer in dihydrofolate reductase. Proteins: Struct Func Bioform. 2004, 57: 444-457. 10.1002/prot.20219.
Rajagopalan PTR, Lutz S, Benkovic SJ: Coupling interactions of distal residues enhance dihydrofolate reductase catalysis: Mutational effects on hydride transfer rates. Biochemistry. 2002, 41: 12618-12628. 10.1021/bi026369d.
Bahnson BJ, Colby TD, Chin JK, Goldstein BM, Klinman JP: A link between protein structure and enzyme catalyzed hydrogen tunneling. Proc Natl Acad Sci U S A. 1997, 94: 12797-12802. 10.1073/pnas.94.24.12797.
Colby TD, Bahnson BJ, Chin JK, Klinman JP, Goldstein BM: Active site modifications in a double mutant of liver alcohol dehydrogenase: Structural studies of two enzyme-ligand complexes. Biochemistry. 1998, 37: 9295-9304. 10.1021/bi973184b.
Agarwal PK, Webb SP, Hammes-Schiffer S: Computational studies of the mechanism for proton and hydride transfer in liver alcohol dehydrogenase. J Am Chem Soc. 2000, 122: 4803-4812. 10.1021/ja994456w.
Webb SP, Agarwal PK, Hammes-Schiffer S: Combining electronic structure methods with the calculation of hydrogen vibrational wavefunctions: Application to hydride transfer in liver alcohol dehydrogenase. J Phys Chem B. 2000, 104: 8884-8894. 10.1021/jp001635n.
Billeter SR, Webb SP, Agarwal PK, Iordanov T, Hammes-Schiffer S: Hydride transfer in liver alcohol dehydrogenase: Quantum dynamics, kinetic isotope effects, and role of enzyme motion. J Am Chem Soc. 2001, 123: 11262-11272. 10.1021/ja011384b.
Billeter SR, Webb SP, Iordanov T, Agarwal PK, Hammes-Schiffer S: Hybrid approach for including electronic and nuclear quantum effects in molecular dynamics simulations of hydrogen transfer reactions in enzymes. J Chem Phys. 2001, 114: 6925-6936. 10.1063/1.1356441.
Hammes GG: Multiple conformational changes in enzyme catalysis. Biochemistry. 2002, 41: 8221-8228. 10.1021/bi0260839.
Kohen A: Kinetic isotope effects as probes for hydrogen tunneling, coupled motion and dynamics contributions to enzyme catalysis. Prog React Kinet Mec. 2003, 28: 119-156.
Tousignant A, Pelletier JN: Protein motions promote catalysis. Chem Biol. 2004, 11: 1037-1042. 10.1016/j.chembiol.2004.06.007.
Lockless SW, Ranganathan R: Evolutionarily conserved pathways of energetic connectivity in protein families. Science. 1999, 286: 295-299. 10.1126/science.286.5438.295.
Moritsugu K, Miyashita O, Kidera A: Vibrational energy transfer in a protein molecule. Phys Rev Lett. 2000, 85: 3970-3973. 10.1103/PhysRevLett.85.3970.
Moritsugu K, Miyashita O, Kidera A: Temperature dependence of vibrational energy transfer in a protein molecule. J Phys Chem B. 2003, 107: 3309-3317. 10.1021/jp027823q.
Heyes DJ, Hunter CN, van Stokkum IHM, van Grondelle R, Groot ML: Ultrafast enzymatic reaction dynamics in protochlorophyllide oxidoreductase. Nat Struct Biol. 2003, 10: 491-492. 10.1038/nsb929.
Download references
Acknowledgements
PKA would like to thank Dr. Brahma Ghosh for feedback on the manuscript.
Author information
Authors and affiliations.
Computational Biology Institute, and Computer Science and Mathematics Division, Oak Ridge National Laboratory, Oak Ridge, Tennessee, 37831, USA
Pratul K Agarwal
You can also search for this author in PubMed Google Scholar
Corresponding author
Correspondence to Pratul K Agarwal .
Additional information
Authors' contributions.
PKA drafted and revised the manuscript.
Authors’ original submitted files for images
Below are the links to the authors’ original submitted files for images.
Authors’ original file for figure 1
Authors’ original file for figure 2, authors’ original file for figure 3, authors’ original file for figure 4, authors’ original file for figure 5, authors’ original file for figure 6, authors’ original file for figure 7, authors’ original file for figure 8, authors’ original file for figure 9, rights and permissions.
Open Access This article is published under license to BioMed Central Ltd. This is an Open Access article is distributed under the terms of the Creative Commons Attribution License ( https://creativecommons.org/licenses/by/2.0 ), which permits unrestricted use, distribution, and reproduction in any medium, provided the original work is properly cited.
Reprints and permissions
About this article
Cite this article.
Agarwal, P.K. Enzymes: An integrated view of structure, dynamics and function. Microb Cell Fact 5 , 2 (2006). https://doi.org/10.1186/1475-2859-5-2
Download citation
Received : 08 November 2005
Accepted : 12 January 2006
Published : 12 January 2006
DOI : https://doi.org/10.1186/1475-2859-5-2
Share this article
Anyone you share the following link with will be able to read this content:
Sorry, a shareable link is not currently available for this article.
Provided by the Springer Nature SharedIt content-sharing initiative
- Enzyme Catalysis
- Protein Dynamic
- Activation Energy Barrier
- Internal Protein
- Transition State Theory
Microbial Cell Factories
ISSN: 1475-2859
- Submission enquiries: [email protected]
Thank you for visiting nature.com. You are using a browser version with limited support for CSS. To obtain the best experience, we recommend you use a more up to date browser (or turn off compatibility mode in Internet Explorer). In the meantime, to ensure continued support, we are displaying the site without styles and JavaScript.
- View all journals
- My Account Login
- Explore content
- About the journal
- Publish with us
- Sign up for alerts
- Open access
- Published: 05 December 2017
Beyond the Michaelis-Menten equation: Accurate and efficient estimation of enzyme kinetic parameters
- Boseung Choi 1 ,
- Grzegorz A. Rempala 2 &
- Jae Kyoung Kim ORCID: orcid.org/0000-0001-7842-2172 3
Scientific Reports volume 7 , Article number: 17018 ( 2017 ) Cite this article
43k Accesses
78 Citations
4 Altmetric
Metrics details
- Computational biology and bioinformatics
Examining enzyme kinetics is critical for understanding cellular systems and for using enzymes in industry. The Michaelis-Menten equation has been widely used for over a century to estimate the enzyme kinetic parameters from reaction progress curves of substrates, which is known as the progress curve assay. However, this canonical approach works in limited conditions, such as when there is a large excess of substrate over enzyme. Even when this condition is satisfied, the identifiability of parameters is not always guaranteed, and often not verifiable in practice. To overcome such limitations of the canonical approach for the progress curve assay, here we propose a Bayesian approach based on an equation derived with the total quasi-steady-state approximation. In contrast to the canonical approach, estimates obtained with this proposed approach exhibit little bias for any combination of enzyme and substrate concentrations. Importantly, unlike the canonical approach, an optimal experiment to identify parameters with certainty can be easily designed without any prior information. Indeed, with this proposed design, the kinetic parameters of diverse enzymes with disparate catalytic efficiencies, such as chymotrypsin, fumarase, and urease, can be accurately and precisely estimated from a minimal amount of timecourse data. A publicly accessible computational package performing such accurate and efficient Bayesian inference for enzyme kinetics is provided.
Similar content being viewed by others
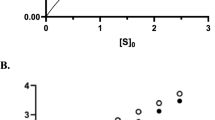
The measurement of true initial rates is not always absolutely necessary to estimate enzyme kinetic parameters
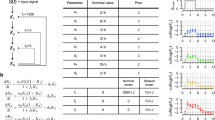
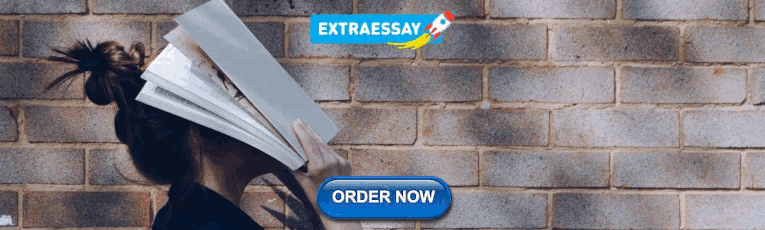
Predictive power of non-identifiable models
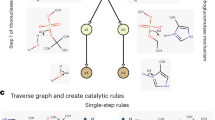
EzMechanism: an automated tool to propose catalytic mechanisms of enzyme reactions
Introduction.
Because enzymes can modulate biochemical reaction rates by selectively catalyzing specific substrates 1 , they play fundamental roles in metabolism, signal transduction, and cell regulation, and their malfunction can cause serious diseases 2 , 3 . Furthermore, enzymes have been used as extremely specific catalysts in diverse industrial fields such as drug development, biofuel production, and food processing 4 . A canonical approach used to understand enzyme kinetics for a century has been based on the Michaelis-Menten equation (MM equation), which was developed by Michaelis and Menten 5 and then was more rigorously derived by Briggs and Haldane 6 using the standard quasi-steady-state approximation (sQSSA) 7 . The equation describes the dependence of enzyme-catalyzed reaction rates on the concentration of substrate by using two parameters, the catalytic constant, k cat and the Michaelis-Menten constant, K M (see below for details). The k cat determines the maximum rate of the reaction at saturating substrate concentrations, V max = k cat E T , where E T is total enzyme concentration, and the K M is the substrate concentration at which the reaction rate is half of V max .
There are two major assays to estimate k cat and K M from a measured accumulation of product over time (i.e. progress curve): the initial velocity assay (initial rate analysis) and the reaction progress curve assay (progress curve analysis) 8 , 9 , 10 , 11 , 12 . For the initial velocity assay, initial rates of the reaction are measured for a range of substrate concentrations. Then, by using a linear transform of these data, such as Lineweaver-Burk plots, the two parameters can be easily estimated without use of any computational tools 8 , 9 . Recent advances in computational tools have led to an alternative approach: the reaction progress curve assay. In this assay, the entire timecourse (i.e. progress curve) is fitted to the solution of a differential equation or integrated rate equation, and thus the data is used more efficiently than in the initial velocity assay 10 , 11 , 13 . Albeit more technically challenging, the progress curve assay requires less data to estimate parameters than the initial velocity assay does.
Since both assays are based on the MM equation, they should be performed only when the MM equation is valid, that is, when the enzyme concentration is a much lower than the sum of the substrate concentration and the K M 7 , 14 (see below for more details). Because the value of K M is usually not known a priori, to ensure the validity of the MM equation, in vitro experiments are typically performed with a much lower enzyme concentration than substrate concentration 15 . However, such conditions cannot be guaranteed in vivo , because endogenous enzyme concentrations are much higher than those used in a typical in vitro assay 16 , 17 . It is therefore risky to use the MM equation to analyze in vivo data and to predict in vivo enzyme activity by using parameters estimated from an in vitro assay 15 , 18 . Furthermore, even when the MM equation is valid, precise estimation is not guaranteed, because of the highly correlated structure and unidentifiability of the parameters 19 , 20 , 21 , 22 , 23 . That is, even though estimated parameters can fit the data accurately, the estimates can differ greatly from the actual values of k cat and K M . Because of the identifiability issue, experimental designs to infer the maximum possible information about the parameters have been investigated 12 , 13 , 20 , 21 , 22 , 23 , 24 . For instance, to ensure that the parameters can be identified from the initial velocity assay, the initial concentration of substrate needs to be increased from a low level to a higher level until the reaction velocity is saturated. For the saturation, generally the initial substrate concentration needs to be larger than 10 K M , but often such high concentrations cannot be achieved 24 . For the progress curve assay, the initial substrate concentration is recommended to be at a similar level to K M 23 , 25 . Note that both assays require prior knowledge of K M , which gives rise to the conundrum that, in order to estimate K M , the approximate value of K M needs to be known.
To overcome such limits on the inference using the model based on the MM equation, which is referred to as the sQ (standard QSSA) model, here we propose an alternative approach. In our approach, we use a different approximate model that is derived with the total QSSA and is referred to as the tQ (total QSSA) model 26 , 27 , 28 , 29 . By applying the Bayesian inference based on either the sQ or the tQ model to the product progress curve, we found that the estimates obtained with the sQ model were considerably biased when the enzyme concentration was not low. On the other hand, the estimates obtained with the tQ model were not biased for any combination of enzyme and substrate concentrations. Thus, with the tQ model, the experimental data from various conditions can be pooled without any restrictions to improve the accuracy and precision of the estimation. For instance, when two sets of timecourse data obtained under low and high enzyme concentrations are used together, the tQ model, but not the sQ model, leads to accurate and precise estimation. Another advantage of our approach is that, by analyzing the scatter plots of current estimates, the next optimal experiment to ensure the parameter identifiability can be easily designed without requiring any prior knowledge of the k cat and K M values. The proposed optimized design yields accurate and precise estimation from a minimal amount of data simulated based on the kinetics of various enzymes: chymotrypsin, fumarase and urease, which have disparate catalytic efficiencies ( k cat / K M ). We provide a publicly accessible computational package that performs the Bayesian inference based on the tQ model, thus leading to accurate and efficient estimation of enzyme kinetics.
Two types of models describing enzyme kinetics: The sQ and tQ models
A fundamental enzyme reaction consists of a single enzyme and a single substrate, where the free enzyme (E) reversibly binds with the substrate (S) to form the complex (C), and the complex irreversibly dissociates into the product (P) and the free enzyme:
where the total enzyme concentration ( E T ≡ C + E ) and the total substrate and product concentration ( S T ≡ S + C + P ) are conserved. A popular model describing the accumulation of the product over time is based on the MM equation, as follows (see Supplementary Method for detailed derivation):
where K M = ( k b + k cat )/ k f is the Michaelis-Menten constant and k cat is the catalytic constant. This sQ model derived with the standard QSSA has been widely used to estimate the kinetic parameters, K M and k cat from the progress curve of the product 8 , 9 , 10 , 11 , 23 , 25 . Another model describing the accumulation of the product is derived with the total QSSA; it was developed later than the sQ model and thus has received less attention for parameter estimation 26 , 27 , 28 , 29 :
Although this tQ model is more complicated than the sQ model, it is accurate over wider ranges than the sQ model. Specifically, the sQ model is accurate when
which requires a low enzyme concentration 7 , 14 . On the other hand, the tQ model is accurate when
where K = k b / k f is the dissociation constant 27 , 28 , 29 . Importantly, this condition is generally valid and thus the tQ model, unlike the sQ model, is accurate even when the enzyme is in excess. See 14 , 30 for more details.
Next, we investigated the accuracy of the stochastic simulations performed with both models. Specifically, we compared the stochastic simulations using the Gillespie algorithm based on the propensity functions from either the original full model (described in Table S1 ), the sQ model (Table S2 ), or the tQ model (Table S3 ) for 9 different conditions 31 , 32 , 33 , 34 , 35 , 36 : E T is either lower than, similar to, or higher than K M , and S T is also either lower than, similar to, or higher than K M (Fig. 1 ). The stochastic simulations of the sQ model fail to approximate those of the original full model when E T is not low (i.e., E T is lower than neither S T nor K M ). On the other hand, stochastic simulations using the tQ model are accurate for all conditions (Fig. 1 ), as is consistent with a recent study showing that stochastic simulations with the sQ and the tQ models are accurate when their deterministic validity conditions hold (Eqs ( 3 ) and ( 4 )) 37 , 38 . Taken together, the tQ model is valid for a wider range of conditions than the sQ model is in both the deterministic and the stochastic sense.
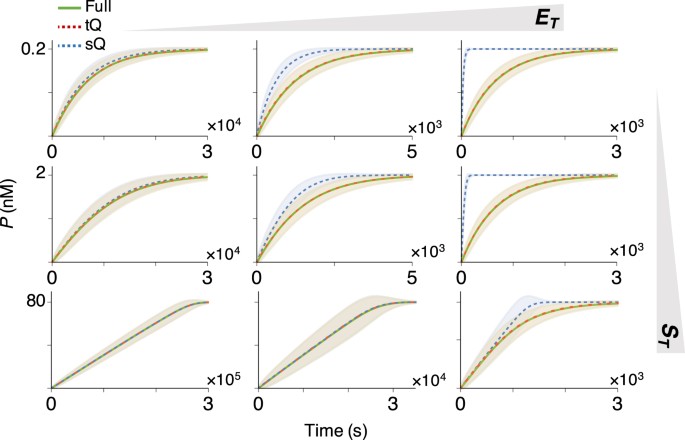
Whereas the sQ model fails to approximate the original full model as E T increases, the tQ model is accurate regardless of E T . Stochastic simulations of the original full model (Table S1 ), the sQ model (Table S2 ), and the tQ model (Table S3 ) were performed with S T = 0.2, 2, or 80 nM, and E T = 0.2, 2, or 40 nM. Note that these concentrations are either lower than, similar to, or higher than K M ≈ 2 nM. Here, the lines and colored ranges represent a mean trajectory and fluctuation range (±2 σ from the mean) of 10 4 stochastic simulations.
Estimation with the tQ model is unbiased for any combination of enzyme and substrate concentrations
Because the tQ model is accurate for a wider range of conditions than the sQ model is (Fig. 1 ), we hypothesized that the parameter estimation based on the tQ model is also accurate for more general conditions. To investigate this hypothesis, we first generated 10 2 noisy progress curves of P from the stochastic simulations of the original full model (Fig. S1 ). Then, we inferred parameters ( k cat and K M ) from these simulated data sets by applying the Bayesian inference with the likelihood functions based on either the sQ or the tQ model, under weakly informative gamma priors (Fig. S2 ) (see Methods for details). Note that throughout this study, we have used the simulated product progress curves (e.g. Fig. S1 ) because we need to know the true values of parameters for the accurate comparison of the estimations based on the sQ model and the tQ model.
We first focused on the estimation of the k cat under the assumption that the value of K M is known. When E T is low, so that both the sQ and the tQ models are accurate (Fig. 1 left), posterior samples obtained with both models are similar and successfully capture the true value of k cat (Fig. 2a left). The posterior samples obtained with the two models are similar because, when E T is low and thus \({E}_{T}\ll {S}_{T}+{K}_{M}\) , both models (Eqs 1 and 2 ) are approximately equivalent as follows:
where the first approximation comes from the Taylor expansion in terms of \({E}_{T}({S}_{T}-P)/({E}_{T}+{K}_{M}+{S}_{T}-P)\ll 1\) (see 27 , 28 , 29 for details). Therefore, when \({E}_{T}\ll {S}_{T}+{K}_{M}\) and thus the sQ model is accurate, estimations with the sQ and the tQ models should be similar. On the other hand, when E T is high, they show clear differences (Fig. 2a right): the posterior samples obtained with the sQ model show large errors, while those obtained with the tQ model accurately capture the true value of k cat .
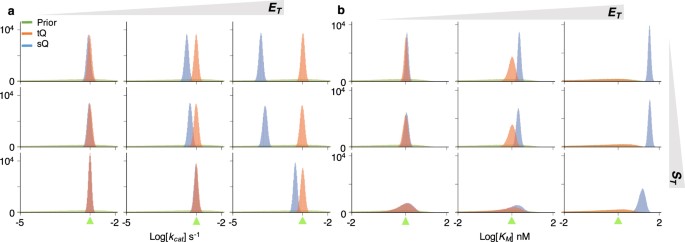
The estimation of a single parameter ( k cat or K M ) with either the sQ or the tQ model. For each condition ( S T = 0.2, 2, or 80 nM, and E T = 0.2, 2, or 40 nM), 10 5 posterior samples of either k cat ( a ) or K M ( b ) were obtained by applying the Bayesian inference to 10 2 noisy data sets (Fig. S1 ) (see Methods for details). When the k cat is sampled, the K M is fixed at its true value ( a ) and vice versa ( b ). Here, green triangles indicate the true values of the parameters. Whereas the estimates of k cat and K M obtained with the sQ model are biased as E T increases, those obtained with the tQ model have negligible bias regardless of conditions (See Fig. S3 for box plots of estimates). As E T or S T increases, the posterior variance of K M increases when the tQ model is used.
Similar results are also observed in the box plots of posterior means and posterior coefficient of variations (CVs) (Fig. S3a,b ). Whereas posterior means obtained with the sQ model are biased when E T is high, those obtained with the tQ model are accurate for all conditions (Fig. S3a ). In particular, narrow distributions of posterior means indicate that the estimation of k cat with the tQ model is robust aginst the noise in the data (Fig. S1 ). Furthermore, posterior CVs are much smaller than prior CVs (Fig. S3b ), indicating precise estimation of k cat with the tQ model.
Next, K M was estimated under the assumption that the value of k cat is known (Fig. 2b ). Posterior samples of the K M obtained with the sQ model again show errors that grow with increasing E T . Note that the estimates of the K M are biased upward, which implies that using the posterior estimates of K M to validate the MM equation ( \({K}_{M}\gg {E}_{T}\) ) can be misleading. On the other hand, the estimates of K M obtained with the tQ model are little biased for all conditions. However, unlike the narrow posterior distributions of k cat (Fig. 2a ), those of K M obtained with the tQ model become wider; so precision decreases as E T or S T increases (Fig. 2b ). These patterns are also observed in the box plots of posterior means and posterior CVs (Fig. S3c,d ). The identifiability problem arises because, when \({E}_{T}\gg {K}_{M}\) or \({S}_{T}\gg {K}_{M}\) and thus \({E}_{T}+{S}_{T}\gg {K}_{M}\) , the K M is negligible in the tQ model (Eq. 2 ), as follows:
Specifically, when K M is too low, the value of K M has little effect on the dynamics of the tQ model and thus the K M is structurally unidentifiable. Taken together, the estimations of K M with both the sQ and the tQ models are not satisfactory, although for different reasons: estimations with the sQ model can be biased and those with the tQ model can be structurally unidentifiable (Fig. 2b ). Similar patterns were also observed when a more informative prior was given (Fig. S4 ). In particular, even with the informative prior, estimates obtained with the sQ model still show considerable error as E T increases.
Simultaneous estimation of k cat and K M suffers from the lack of identifiability
Next, we considered simultaneous estimation of two parameters, k cat and K M , which is the typical goal of enzyme kinetics. For the same gamma priors used in the single-parameter estimation (Fig. 2 ), the distributions of posterior samples obtained with both models became wider overall (Fig. 3 ). To find the reason for such imprecise estimation, we analysed the scatter plots of posterior k cat and K M samples (Fig. 4 ). When \({S}_{T}\ll {K}_{M}\) (Fig. 4a–c ), the posterior samples of k cat and K M obtained with the sQ model exhibited a strong correlation, because the dynamics of the sQ model depend only on the ratio k cat / K M , as seen in the following approximation:
where \({K}_{M}\gg {S}_{T}\ge {S}_{T}-P\) is used. On the other hand, when \({S}_{T}\gg {K}_{M}\) (Fig. 4g–i ), the scatter plot of the sQ model becomes horizontal, indicating the structure unidentifiability of the K M . Indeed, the value of K M has nearly no effect on the dynamics of the sQ model, as seen in the following approximation:
where K M + S T ≈ S T is used as \({S}_{T}\gg {K}_{M}\) . Such lack of parameter identifiability when \({S}_{T}\ll {K}_{M}\) or \({S}_{T}\gg {K}_{M}\) is consistent with previous studies, which recommend using S T ≈ K M for more precise estimation 22 , 23 . However, even when S T ≈ K M , estimates are still imprecise (Fig. 3a and b middle). Furthermore, as E T increases, the estimates obtained with the sQ model are biased (Fig. 3 ) like in the single-parameter estimation (Fig. 2 ). Based on this analysis it appears that the simultaneous estimation of k cat and K M with the sQ model is challenging because of both identifiability and bias problems.
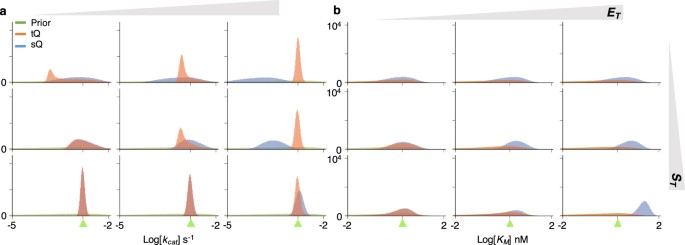
Simultaneous estimation of two parameters ( k cat and K M ) with either the sQ or the tQ model. From the same 10 2 data sets (Fig. S1 ) used in the single-parameter estimation (Fig. 2 ), 10 5 posterior samples of the k cat ( a ) and the K M ( b ) were obtained together. Although the same prior is given, the posterior distributions become wider than the single-parameter estimation (Fig. 2 ). Here, green triangles indicate the true values of k cat or K M .
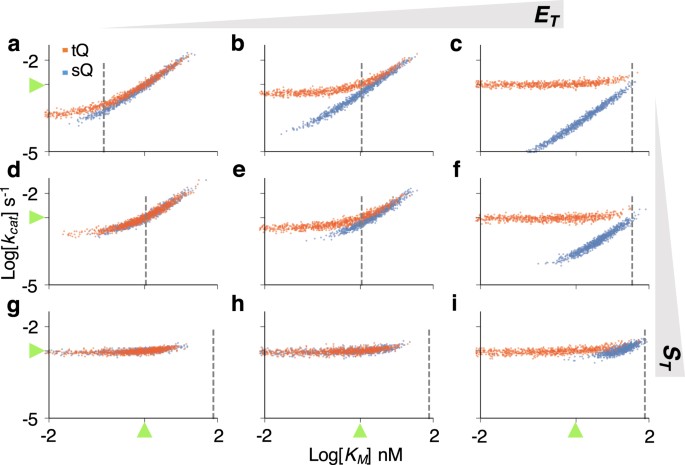
The scatter plots of posterior samples obtained with the two-parameter estimation (Fig. 3 ). The scatter plots imply two types of structure unidentifiability: strong correlation between k cat and K M , and unidentifiability of K M , which is represented as a horizontal plot. Positively correlated scatter plots of the tQ model are changed to horizontal ones when the sampled K M is much lower than S T + E T (dashed gray lines). Here, green triangles represent the true values of parameters.
When \({E}_{T}\gg {K}_{M}\) or \({S}_{T}\gg {K}_{M}\) , the K M has a negligible effect on the dynamics of the tQ model (Eq. 6 ), and thus only k cat was identifiable in the single-parameter estimation (Fig. 2a and b right or bottom). Similarly, when both k cat and K M are inferred simultaneously with the tQ model, estimation of only k cat is accurate and precise (Fig. 3a and b right or bottom), as is shown by the horizontal scatter plots along the true value of k cat (Fig. 4c,f,g–i ). In other cases (when neither \({E}_{T}\gg {K}_{M}\) nor \({S}_{T}\gg {K}_{M}\) ), posterior variance of both parameters dramatically increases compared to the single-parameter estimation (Figs 2 and 3 left and top). Such imprecise estimation stems from two sources, according to the scatter plots (Fig. 4a,b,d,e ). When k cat and K M decrease together, the behavior of the tQ model changes little as the SQ model (Eq. 5 ), which leads to the strong correlation between posterior samples of k cat and K M . As the estimates of K M keep decreasing together with those of k cat , so that they become much less than E T + S T (dashed vertical line of Fig. 4 ), the tQ model no longer depends on the value of K M , as shown in Eq. 6 , and thus the scatter plots become horizontal.
Combined data from different experiments allow accurate and precise estimation with the tQ model
As shown above, the estimation of both k cat and K M using a single progress curve suffers from considerable bias and lack of identifiability (Figs 3 and 4 ), which is consistent with previous studies reporting that a progress curve obtained from a single experiment is not enough to identify both parameters simultaneously 19 . Thus, here, we investigate whether using multiple timecourse data sets obtained under different experimental conditions can improve the estimation.
In typical in vitro assays, progress curves are measured with either a fixed S T and varied E T or a fixed E T and varied S T 8 , 9 , 10 , 11 , 39 . We first consider the case when progress curves are measured with a fixed S T and a varied E T . Specifically, progress curves from both low and high E T are used to estimate parameters for a fixed S T at different levels (Fig. S1 top and bottom). In this case, posterior samples obtained with the sQ model show considerable errors as the data from high E T is used (Figs 5a and S5 ). On the other hand, the posterior samples obtained with the tQ model accurately capture the true values of both k cat and K M with low variance (Figs 5a and S5 ). Such improvement stems from the fact that data obtained under the low and high E T provide different types of information for parameter estimation. Specifically, from the high E T data, although the K M is not identifiable, the k cat can be accurately estimated with the tQ model (Fig. 4c,f,i ). Such accurate estimation of k cat from the high E T data can prevent the correlation between the k cat and the K M when they are estimated from the low E T data (Fig. 4a,d ). Indeed, the narrow scatter plots of the tQ model (Fig. 5b left and middle) are the intersection of two scatter plots, a horizontal one obtained with the high E T data (Fig. 4c,f ) and a nonhorizontal one obtained with the low E T data (Fig. 4a,d ). However, when S T is high, the scatter plot from the low E T also becomes horizontal (Fig. 4c ), and thus the synergistic effect of using combined data decreases (Fig. 5a,b right). Taken together, the tQ model can accurately estimate both parameters from the combination of low E T and high E T data when S T is not much larger than K M . Note that such low S T is preferred for in vitro experiments 24 , 39 , 40 , 41 and is the case for most physiological conditions 24 .
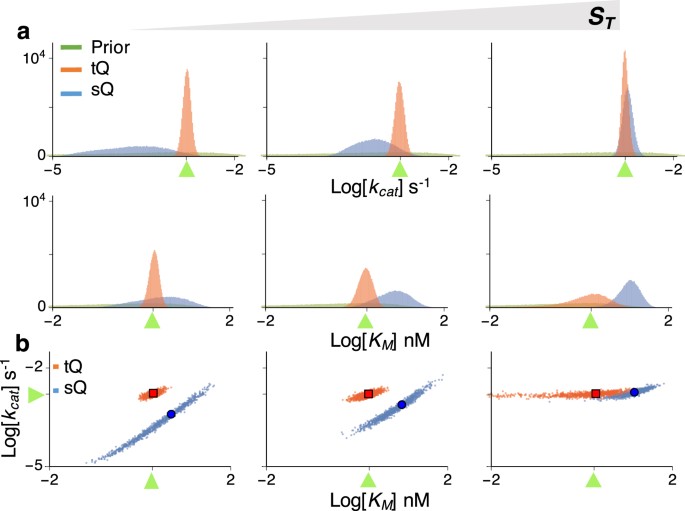
When data obtained under low E T and high E T are used together, the accuracy and precision of estimaties obtained with the tQ model, but not with the sQ model, are enhanced. ( a ) Posterior samples are inferred using data sets from E T = 0.2 nM (Fig. S1 top) and E T = 40 nM (Fig. S1 bottom) together for either S T = 0.2, 2, or 80 nM. The posterior variance of the tQ model dramatically decreases to the level of the single-parameter estimation (Fig. 2 ). However, the estimates of the sQ model show considerable bias. Here, green triangles represent the true values of k cat or K M . ( b ) The scatter plots of the posterior samples. Here green triangles, blue circles, and red squares represent true values, posterior means of the sQ model, and those of the tQ model, respectively.
Next, we consider the case when progress curves are measured with a fixed E T and a varied S T . Specifically, the combination of two progress curves from low and high S T is used to infer parameters for a fixed E T at different levels (Fig. S1 left and right). When E T is low, and thus the sQ and the tQ models behave similarly (Eq. 5 ), posterior samples obtained with both models accurately capture the true values of k cat and K M (Figs 6a left and S6 ). Again, the narrow scatter plot (Fig. 6b left) is obtained as the intersection of a nonhorizontal scatter plot of low S T (Fig. 4a ) and a horizontal scatter plot of high S T (Fig. 4g ). However, as E T increases, and thus the sQ model becomes less accurate, those obtained with the sQ model are biased, as expected (Figs 6a right and S6 ). Whereas such biases are not observed in those obtained with the tQ model, the precision of K M estimates decreases as E T increases, as in the single-parameter estimation (Fig. 2 and Eq. 6 ).
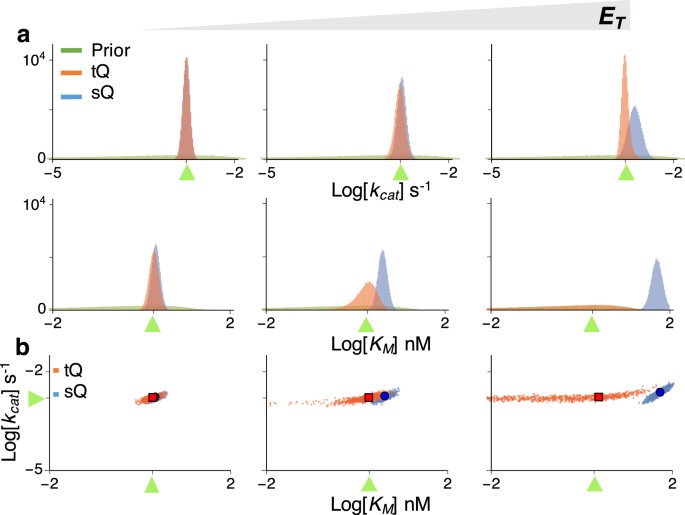
Estimation using the data obtained under low S T and high S T together. ( a ) Posterior samples are inferred using data sets from S T = 0.2 nM (Fig. S1 left) and S T = 80 nM (Fig. S1 right) together for either E T = 0.2, 2, or 40 nM. When E T is low, both the sQ and the tQ models allow accurate and precise estimation. As E T increases, the estimates obtained with the sQ model become inaccurate, and the estimates of K M obtained with the tQ model become less precise, similar to the single-parameter estimation (Fig. 2 ). Here, green triangles represent the true values of k cat or K M . ( b ) The scatter plots of the posterior samples. Here green triangles, blue circles, and red squares represent true values, posterior means of the sQ model, and those of the tQ model, respectively.
Optimal design of experiments for accurate and efficient estimation with the tQ model
When a progress curve obtained from a single experiment is used, the posterior scatter plots of the tQ model can be categorized as a correlated type (Fig. 4a,b,d,e ) and a horizontal type (Fig. 4c,f,g–i ). The intersections of these two different types of scatter plots tend to be narrowly distributed near the true value (Figs 5b and 6b ). Thus, combining two such data sets allows accurate estimation of both k cat and K M (Figs 5a and 6a ). Specifically, a progress curve measured under \({E}_{T}\ll {K}_{M}\) and \({S}_{T}\ll {K}_{M}\) (Fig. 4a,b,d,e ) and one measured under \({E}_{T}\gg {K}_{M}\) or \({S}_{T}\gg {K}_{M}\) (Fig. 4c,f,g–i ) provide different types of information for parameter estimation; so using both data sets leads to successful estimation. However, it is hard to compare the values of S T , E T , and K M in practice, because the value of K M is usually unknown a priori. This problem can be easily resolved by using the scatter plot. That is, if the posterior scatter plot obtained from the first experiment is horizontal, then both E T and S T should be decreased for the next experiment, so that the nonhorizontal scatter plot can be obtained (Fig. 7a ). On the other hand, if the scatter plot from the first experiment shows a strong correlation between K M and k cat , then either S T or E T should be increased in the next experiment (Fig. 7b ). Basically, without any prior information of the value of K M and k cat , the shape of the scatter plots of the current estimates determines the next optimal experimental design, which ensures accurate and precise estimation. However, this approach cannot be used with the sQ model, because estimation with the sQ model can be biased, depending on the relationship between E T or S T and K M , which is unknown a priori. That is, unlike the tQ model, precise estimation does not always guarantee accurate estimation with the sQ model, as seen above (e.g. Fig. 5a right).
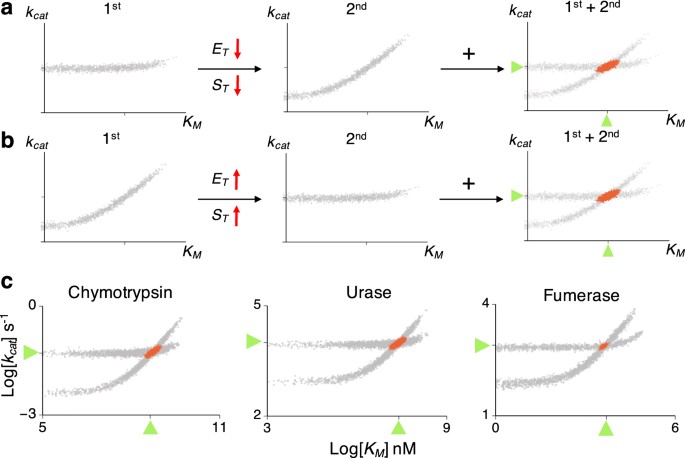
The optimal experimental design for accurate and precise estimation with the tQ model. ( a ) When the scatter plot of posterior samples from the first experiment is horizontal, E T and S T need to be decreased to obtain the nonhorizontal scatter plot in the next experiment. Then, using the combination of the two experiments leads to accurate and precise estimation (red scatter plots). ( b ) When the scatter plot from the first experiment is nonhorizontal, E T or S T need to be increased in the next experiment to obtain a horizontal scatter plot. ( c ) Inference with a single progress curve from the low E T (0.1 K M ) and the high E T (10 K M ) leads to nonhorizontal and horizontal scatter plots, respectively, for chymotrypsin, urease, and fumarase (gray scatter plots). When both data sets were used together, accurate estimates were obtained for all enzymes (red scatter plots). Here, low S T (0.1 K M ) is used. Here, green triangles represent the true values of the parameters.
We test whether the proposed approach with the tQ model can accurately estimate k cat and K M for catalysis of the N-acetylglycine ethyl ester, fumarate, and urea by the enzymes the chymotrypsin, urease, and fumarase, respectively (Fig. 7c ). These three enzymes were chosen because they have disparate catalytic efficiencies ( k cat / K M ) 1 : 0.12, 4 · 10 5 , and 1.6 · 10 8 s −1 M −1 , respectively. For each enzyme, 10 2 noisy timecourse data sets were generated using stochastic simulations based on known enzyme kinetic parameters 1 . When progress curves obtained with low E T and low S T are used, as expected, nonhorizontal scatter plots of posterior samples were obtained for all three enzymes (Fig. 7c ). This indicates that either E T or S T should be increased in the next experiment to obtain a horizontal scatter plot. Indeed, when a progress curve with a 100-fold increase of E T was used, horizontal scatter plots were obtained for all enzymes (Fig. 7c ). Therefore, when these two progress curves are used together, both k cat and K M can be accurately estimated (Fig. 7c red dots). These results support that such two-step optimized experimental design (Fig. 7a,b ) to get two different types of scatter plots allows accurate and efficient estimation of enzyme kinetics with the tQ model. The computational package performing such estimation is provided (see Method for the details).
The standard approach for estimating enzyme kinetic parameters even today continues to be based on the 100-year old MM equation (Eq. 1 ) 5 , 6 . However, when enzyme concentration is high, this approach can lead to biased estimation (Fig. 2 ). Even when enzyme concentration is relatively low, it may not be possible to identify kinetic parameters (Figs 3 and 4 ). To overcome the limitations of the canonical approach, we proposed an estimation method based on an alternative to the MM equation: the tQ model (Eq. 2 ), which is derived with the total QSSA 26 , 27 , 28 , 29 . Because the estimation procedure with the tQ model is not biased regardless of enzyme or substrate concentrations (Fig. 2 ), more accurate and precise estimations can be made when pooled data from different experimental conditions are used, unlike the canonical approach (Figs 5 and 6 ). It appears thus that the tQ model is especially appropriate for creating a consistent Bayesian inferential framework, which becomes more accurate as more data is used.
The canonical enzyme kinetic assay based on the MM equation generally requires a large excess of substrate over enzyme 42 . However, such conditions impose experimental limitations and cannot be always guaranteed and verified 15 . For instance, it is hard to generate a high concentration of barely soluble substrate 24 , and a low concentration of substrate is required for sensitive kinetic analysis, e.g., in the case of QD-FRET-based probes 39 , 40 , 41 . Importantly, to analyze in vivo enzyme kinetics, where enzyme concentration is often high 16 , 17 , 18 , our approach, but not the canonical approach, can be used. For example, one needs to estimate the kinetic parameters underlying drug metabolism by CYP enzymes in the liver in order to predict the effects of drugs, as is essential for drug development 43 . Because of dosing requirements for potent drugs, the amount of CYP enzyme can greatly exceed the drug amount in the liver 44 , 45 . Another large area where our estimation method can be applied is in the development of nanobiosensors, which measure in vivo activity of a specific enzyme for precise diagnostics, because such enzymes are often in large excess over biosensors 46 , 47 .
KinTek Explorer has been widely used to estimate enzyme kinetic parameters from the progress curves 48 , 49 , 50 . This software provides the confidence contours, which reveal the relationships between the estimated parameters. This approach recommends using multiple data sets to narrow down the confidence contours and thus improve precision of estimates and resolve the unidentifiability issue. Our finding (Fig. 7a,b ) can provide the specific type of data sets required for the identifiability of k cat and K M , so that the KinTek Explore could perform parameter estimation more efficiently for the Michales-Menten type of enzyme reactions.
Since the initial velocity estimation with the MM equation is not accurate when enzyme concentration is high (Fig. 1 ), the standard initial velocity based on the MM equation would also be inaccurate 15 . On the other hand, the tQ model accurately captures the initial velocity for all conditions, and thus the modified initial velocity assay based on the tQ model is likely to be accurate over a wider range of conditions. To simplify such estimation procedures, an interesting future study could derive an analogous Lineweaver-Burk plot or the Hanes-Woolf plot 8 , 9 , 15 , 42 for the tQ model.
Even with relatively large noise in the data (Fig. S1 ), our proposed method leads to accurate estimation (Figs 5 , 6 and 7 ), indicating its robustness against experimental noise and some minor inaccuracy of the tQ model in certain ranges of parameter observed in 14 , 30 . Furthermore, if there are departures from simple non-inhibitory enzyme kinetics (e.g. inhibition of enzyme by product) 51 , 52 , our method can be easily adjusted by modifying the tQ model (see 53 , 54 for the tQ model for other enzyme kinetics). Our work can also be used to improve the estimation of the kinetics underlying diverse biological functions, such as gene regulation 55 , 56 , cellular rhythms 57 , 58 , 59 , quorum sensing 60 , 61 , signal cascade 62 , 63 and membrane transport 64 , 65 , where the MM equation has been widely used.
Simulated Data
To obtain timecourse data (Fig. S1 ) for Bayesian inference, stochastic simulations of the original full model (Table S1 ) were performed with the Gillespie algorithm 66 . E (0) = E T , S (0) = S T , C (0) = 0, and P (0) = 0 are used as initial conditions following the typical in vitro enzyme kinetics protocol.
Description of the Bayesian inference approach
The Bayesian inference approach is used to estimate the catalytic constant k cat and the Michaelis-Menten constant K M , based on the hazard function, with respective rates described in Eq. 1 for the sQ model and in Eq. 2 for the tQ model (Fig. S2 ). The likelihood functions are constructed based on an approximation to the underlying Markov model 66 as follows.
where λ i is given by
where P i is the scaled number of product molecules observed at time point t i over [0, T ] = [ t 0 , t m ] and n i = P i − P i −1 is an observed increment of P i . With these likelihood functions, the usual independent gamma priors 67 are assigned to k cat and K M in order to get their posterior distributions with the help of the Markov Chain Monte Carlo (MCMC) method. Weakly informative gamma priors are used for both k cat and K M : their prior means are the same as their true values, and their prior variance is 10 times larger than the prior mean, which covers orders of magnitude (e.g. Fig. 2 ). The estimation of a single parameter, i.e., either k cat or K M is done conditionally on the other parameter. For estimating the two parameters simultaneously, the Gibbs sampler method is used. In order to draw the sample for K M , we also use the Metropolis-Hastings algorithm within the Gibbs sampler step. See Supplementary material for further details.
Computational code
The R package that performs the Bayesian inference based on the tQ model is available on the CRAN repository ( https://cran.r-project.org/web/packages/EKMCMC ).
Chang, R. Physical chemistry for the chemical and biological sciences (University Science Books, 2000).
Cooper, G. The Cell : A Molecular approach (USA: Sinauer Associates, 2000).
Griffiths, A. J. Modern genetic analysis : integrating genes and genomes (Macmillan, 2002).
Kirk, O., Borchert, T. V. & Fuglsang, C. C. Industrial enzyme applications. Curr. Opin. Biotechnol. 13 , 345–351 (2002).
Article CAS PubMed Google Scholar
Michaelis, L. & Menten, M. L. Die kinetik der invertinwirkung. Biochem. z 49 , 352 (1913).
Google Scholar
Briggs, G. E. & Haldane, J. B. S. A note on the kinetics of enzyme action. Biochem. J. 19 , 338 (1925).
Article CAS PubMed PubMed Central Google Scholar
Segel, L. A. & Slemrod, M. The quasi-steady-state assumption - a case-study in perturbation. SIAM Rev. 31 , 446–477 (1989).
Article MathSciNet MATH Google Scholar
Tummler, K., Lubitz, T., Schelker, M. & Klipp, E. New types of experimental data shape the use of enzyme kinetics for dynamic network modeling. FEBS J. 281 , 549–571 (2014).
Johnson, K. A. A century of enzyme kinetic analysis, 1913 to 2013. FEBS Lett 587 , 2753–2766 (2013).
Duggleby, R. G. Analysis of enzyme progress curves by nonlinear regression. Methods Enzymol 249 , 61–90 (1995).
Duggleby, R. G. & Wood, C. Analysis of progress curves for enzyme-catalysed reactions. automatic construction of computer programs for fitting integrated rate equations. Biochem. J. 258 , 397–402 (1989).
Chen, W. W., Niepel, M. & Sorger, P. K. Classic and contemporary approaches to modeling biochemical reactions. Genes & Dev 24 , 1861–1875 (2010).
Article CAS Google Scholar
Varón, R. et al . An alternative analysis of enzyme systems based on the whole reaction time: evaluation of the kinetic parameters and initial enzyme concentration. J. Math. Chem. 42 , 789–813 (2007).
Schnell, S. & Maini, P. K. A century of enzyme kinetics. should we believe in the km and vmax estimates? Comments Theor. Biol. 8 , 169–187 (2003).
Article Google Scholar
Pinto, M. F. et al . Enzyme kinetics: the whole picture reveals hidden meanings. The FEBS J. 282 , 2309–2316 (2015).
Albe, K. R., Butler, M. H. & Wright, B. E. Cellular concentrations of enzymes and their substrates. J. Theor. Biol. 143 , 163–195 (1990).
Srere, P. A. Enzyme concentrations in tissues. Science. 158 , 936–937 (1967).
Eunen, K. V. & Bakker, B. M. The importance and challenges of in vivo -like enzyme kinetics. Perspectives Sci. 1 , 126–130 (2014).
Nikolova, N., Tenekedjiev, K. & Kolev, K. Uses and misuses of progress curve analysis in enzyme kinetics. Cent. Eur. J. Biol 3 , 345–350 (2008).
CAS PubMed PubMed Central Google Scholar
Cornish-Bowden, A. One hundred years of michaelis–menten kinetics. Perspectives Sci. 4 , 3–9 (2015).
Yang, X., Long, G., Jiang, H., Liao, P. & Liao, F. Integration of kinetic analysis of reaction curve with a proper classical approach for enzymatic analysis. The Sci . World J . 2012 (2012).
Duggleby, R. G. Experimental designs for estimating the kinetic parameters for enzyme-catalysed reactions. J. Theor. Biol. 81 , 671–684 (1979).
Stroberg, W. & Schnell, S. On the estimation errors of k m and v from time-course experiments using the michaelis–menten equation. Biophys. Chem. 219 , 17–27 (2016).
Bisswanger, H. Enzyme assays. Perspectives Sci. 1 , 41–55 (2014).
Duggleby, R. G. & Clarke, R. B. Experimental designs for estimating the parameters of the michaelis-menten equation from progress curves of enzyme-catalyzed reactions. Biochimica et Biophys. Acta (BBA)-Protein Struct. Mol. Enzymol. 1080 , 231–236 (1991).
Cha, S. Kinetic behavior at high enzyme concentrations magnitude of errors of michaelis-menten and other approximations. J. Biol. Chem. 245 , 4814–4818 (1970).
CAS PubMed Google Scholar
Tzafriri, A. R. Michaelis-menten kinetics at high enzyme concentrations. Bull. Math. Biol. 65 , 1111–1129 (2003).
Article CAS PubMed MATH Google Scholar
Bersani, A. M., Bersani, E., DellAcqua, G. & Pedersen, M. G. New trends and perspectives in nonlinear intracellular dynamics: one century from michaelis–menten paper. Continuum Mech. Thermodyn. 27 , 659–684 (2015).
Article ADS MathSciNet MATH Google Scholar
Borghans, J. M., De Boer, R. J. & Segel, L. A. Extending the quasi-steady state approximation by changing variables. Bull. Math. Biol. 58 , 43–63 (1996).
Schnell, S. & Maini, P. Enzyme kinetics far from the standard quasi-steady-state and equilibrium approximations. Math. Comput. Model. 35 , 137–144 (2002).
Rao, C. V. & Arkin, A. P. Stochastic chemical kinetics and the quasi-steady-state assumption: Application to the Gillespie algorithm. J. Chem. Phys. 118 , 4999–5010 (2003).
Article ADS CAS Google Scholar
Barik, D., Paul, M. R., Baumann, W. T., Cao, Y. & Tyson, J. J. Stochastic simulation of enzyme-catalyzed reactions with disparate timescales. Biophys. J. 95 , 3563–3574 (2008).
Article ADS CAS PubMed PubMed Central Google Scholar
Thomas, P., Straube, A. V. & Grima, R. The slow-scale linear noise approximation: an accurate, reduced stochastic description of biochemical networks under timescale separation conditions. BMC Syst . Biol . 6 (2012).
Kim, J. K., Josić, K. & Bennett, M. R. The validity of quasi-steady-state approximations in discrete stochastic simulations. Biophys. J. 107 , 783–793 (2014).
Kim, J. K. & Sontag, E. D. Reduction of multiscale stochastic biochemical reaction networks using exact moment derivation. PLoS Comput. Biol. 13 , e1005571 (2017).
Article ADS PubMed PubMed Central Google Scholar
Kim, J. K., Rempala, G. A. & Kang, H.-W. Reduction for stochastic biochemical reaction networks with multiscale conservations. Multiscale Modeling & Simulation, 15 (4), 1376–1403 (2017).
Sanft, K. R., Gillespie, D. T. & Petzold, L. R. Legitimacy of the stochastic michaelis-menten approximation. IET Syst. Biol. 5 , 58–69 (2011).
Kim, J. K., Josić, K. & Bennett, M. R. The relationship between stochastic and deterministic quasi-steady state approximations. BMC Syst. Biol. 9 , 87 (2015).
Article PubMed PubMed Central Google Scholar
Sapsford, K. E. et al . Monitoring of enzymatic proteolysis on a electroluminescent-ccd microchip platform using quantum dot-peptide substrates. Sensors Actuators B: Chem. 139 , 13–21 (2009).
Algar, W. R. et al . Proteolytic activity at quantum dot-conjugates: Kinetic analysis reveals enhanced enzyme activity and localized interfacial hopping. Nano Lett. 12 , 3793–3802 (2012).
Article ADS CAS PubMed Google Scholar
Algar, W. R. et al . Multiplexed tracking of protease activity using a single color of quantum dot vector and a time-gated forster resonance energy transfer relay. Anal. Chem. 84 , 10136–10146 (2012).
Singh, N. et al . A safe lithium mimetic for bipolar disorder. Nat. Commun. 4 , 1332 (2013).
Obach, R. S. & Reed-Hagen, A. E. Measurement of michaelis constants for cytochrome p450-mediated biotransformation reactions using a substrate depletion approach. Drug Metab. Dispos. 30 , 831–837 (2002).
Wienkers, L. C. & Heath, T. G. Predicting in vivo drug interactions from in vitro drug discovery data. Nat. Rev. Drug Discov. 4 , 825–833 (2005).
Houston, J. B. & Kenworthy, K. E. In vitro - in vivo scaling of cyp kinetic data not consistent with the classical michaelis-menten model. Drug Metab. Dispos. 28 , 246–254 (2000).
Turk, B. E., Huang, L. L., Piro, E. T. & Cantley, L. C. Determination of protease cleavage site motifs using mixture-based oriented peptide libraries. Nat. Biotechnol. 19 , 661–667 (2001).
Kwong, G. A. et al . Mathematical framework for activity-based cancer biomarkers. Proc. Natl. Acad. Sci. 112 , 12627–12632 (2015).
Johnson, K. A. Fitting enzyme kinetic data with kintek global kinetic explorer. Methods Enzymol. 467 , 601–626 (2009).
Johnson, K. A., Simpson, Z. B. & Blom, T. Global kinetic explorer: a new computer program for dynamic simulation and fitting of kinetic data. Anal. Biochem. 387 , 20–29 (2009).
Johnson, K. A., Simpson, Z. B. & Blom, T. Fitspace explorer: an algorithm to evaluate multidimensional parameter space in fitting kinetic data. Anal. Biochem. 387 , 30–41 (2009).
Cao, W. & Enrique, M. Quantitative full time course analysis of nonlinear enzyme cycling kinetics. Sci. Reports 3 , 2658 (2013).
Duggleby, R. G. Quantitative analysis of the time courses of enzyme-catalyzed reactions. Methods 24 , 168–174 (2001).
Pedersen, M. G., Bersani, A. M., Bersani, E. & Cortese, G. The total quasi-steady-state approximation for complex enzyme reactions. Math. Comput. Simul. 79 , 1010–1019 (2008).
Ciliberto, A., Capuani, F. & Tyson, J. J. Modeling networks of coupled enzymatic reactions using the total quasi-steady state approximation. PLoS Comput. Biol 3 , e45 (2007).
Article ADS MathSciNet PubMed PubMed Central Google Scholar
Ronen, M., Rosenberg, R., Shraiman, B. I. & Alon, U. Assigning numbers to the arrows: parameterizing a gene regulation network by using accurate expression kinetics. Proc. Natl. Acad. Sci. 99 , 10555–10560 (2002).
Del Vecchio, D., Abdallah, H., Qian, Y. & Collins, J. J. A blueprint for a synthetic genetic feedback controller to reprogram cell fate. Cell Syst . (2017).
Pigolotti, S., Krishna, S. & Jensen, M. H. Oscillation patterns in negative feedback loops. Proc. Natl. Acad. Sci. 104 , 6533–6537 (2007).
Article ADS MathSciNet CAS PubMed PubMed Central MATH Google Scholar
Kim, J. K. Protein sequestration versus hill-type repression in circadian clock models. IET Syst . Biol . 10 , 125–135(10) (2016).
Gotoh, T. et al . Model-driven experimental approach reveals the complex regulatory distribution of p53 by the circadian factor period 2. Proc. Natl. Acad. Sci. 113 , 13516–13521 (2016).
Dockery, J. D. & Keener, J. P. A mathematical model for quorum sensing in pseudomonas aeruginosa. Bull. Math. Biol. 63 , 95–116 (2001).
Chen, Y., Kim, J. K., Hirning, A. J., Josić, K. & Bennett, M. R. Emergent genetic oscillations in a synthetic microbial consortium. Sci. 349 , 986–989 (2015).
Ossareh, H. R., Ventura, A. C., Merajver, S. D. & Del Vecchio, D. Long signaling cascades tend to attenuate retroactivity. Biophys. J. 100 , 1617–1626 (2011).
Chen, M., Wang, L., Liu, C. C. & Nie, Q. Noise attenuation in the on and off states of biological switches. ACS Synth. Biol. 2 , 587–593 (2013).
Knight, M. J., Senior, L., Nancolas, B., Ratcliffe, S. & Curnow, P. Direct evidence of the molecular basis for biological silicon transport. Nat . Commun . 7 (2016).
Lawson, M. J., Drawert, B., Khammash, M., Petzold, L. & Yi, T.-M. Spatial stochastic dynamics enable robust cell polarization. PLoS Comput. Biol 9 , e1003139 (2013).
Gillespie, D. T. Exact stochastic simulation of coupled chemical reactions. The J . Phys . Chem . 2340–2361 (1977).
Choi, B. & Rempala, G. A. Inference for discretely observed stochastic kinetic networks with applications to epidemic modeling. Biostat. 13 , 153–165 (2012).
Article MATH Google Scholar
Download references
Acknowledgements
The research was initiated when all authors were visiting The Mathematical Biosciences Institute at the Ohio State University. This work was supported by the National Research Foundation of Korea grant NRF-2017R1D1A3B03031008 (B.C.), US National Science Foundation (NSF) Grant DMS-1440386 (G.A.R.) and DMS-1318886 (G.A.R.), the National Research Foundation of Korea grant N01160447 (J.K.K.), and the TJ Park Science Fellowship of POSCO TJ Park Foundation (J.K.K.).
Author information
Authors and affiliations.
Korea University Sejong campus, Division of Economics and Statistics, Department of National Statistics, Sejong, 30019, Korea
Boseung Choi
The Ohio State University, Division of Biostatistics and Mathematical Biosciences Institute, Columbus, OH, 43210, USA
Grzegorz A. Rempala
Korea Advanced Institute of Science and Technology, Department of Mathematical Sciences, Daejeon, 34141, Korea
Jae Kyoung Kim
You can also search for this author in PubMed Google Scholar
Contributions
J.K.K. designed the research. B.C. and J.K.K. performed simulations and analysis. All authors discussed the results and wrote the manuscript.
Corresponding author
Correspondence to Jae Kyoung Kim .
Ethics declarations
Competing interests.
The authors declare that they have no competing interests.
Additional information
Publisher's note: Springer Nature remains neutral with regard to jurisdictional claims in published maps and institutional affiliations.
Electronic supplementary material
Supplementary information, rights and permissions.
Open Access This article is licensed under a Creative Commons Attribution 4.0 International License, which permits use, sharing, adaptation, distribution and reproduction in any medium or format, as long as you give appropriate credit to the original author(s) and the source, provide a link to the Creative Commons license, and indicate if changes were made. The images or other third party material in this article are included in the article’s Creative Commons license, unless indicated otherwise in a credit line to the material. If material is not included in the article’s Creative Commons license and your intended use is not permitted by statutory regulation or exceeds the permitted use, you will need to obtain permission directly from the copyright holder. To view a copy of this license, visit http://creativecommons.org/licenses/by/4.0/ .
Reprints and permissions
About this article
Cite this article.
Choi, B., Rempala, G.A. & Kim, J.K. Beyond the Michaelis-Menten equation: Accurate and efficient estimation of enzyme kinetic parameters. Sci Rep 7 , 17018 (2017). https://doi.org/10.1038/s41598-017-17072-z
Download citation
Received : 05 May 2017
Accepted : 22 November 2017
Published : 05 December 2017
DOI : https://doi.org/10.1038/s41598-017-17072-z
Share this article
Anyone you share the following link with will be able to read this content:
Sorry, a shareable link is not currently available for this article.
Provided by the Springer Nature SharedIt content-sharing initiative
This article is cited by
Construction and optimization of a biocatalytic route for the synthesis of neomenthylamine from menthone.
- Hui-Jue Zhu
Bioresources and Bioprocessing (2023)
Impact of the Error Structure on the Design and Analysis of Enzyme Kinetic Models
- Elham Yousefi
- Werner G. Müller
Statistics in Biosciences (2023)
Natural Parameter Conditions for Singular Perturbations of Chemical and Biochemical Reaction Networks
- Justin Eilertsen
- Santiago Schnell
- Sebastian Walcher
Bulletin of Mathematical Biology (2023)
Comparison of in vitro Antifungal Activity Methods Using Extract of Chitinase-producing Aeromonas sp. BHC02
- Bilge Hilal Cadirci
- Gulesme Yilmaz
The Protein Journal (2023)
A self-triggered radioligand therapy agent for fluorescence imaging of the treatment response in prostate cancer
- Hongchuang Xu
European Journal of Nuclear Medicine and Molecular Imaging (2022)
By submitting a comment you agree to abide by our Terms and Community Guidelines . If you find something abusive or that does not comply with our terms or guidelines please flag it as inappropriate.
Quick links
- Explore articles by subject
- Guide to authors
- Editorial policies
Sign up for the Nature Briefing newsletter — what matters in science, free to your inbox daily.


An official website of the United States government
The .gov means it’s official. Federal government websites often end in .gov or .mil. Before sharing sensitive information, make sure you’re on a federal government site.
The site is secure. The https:// ensures that you are connecting to the official website and that any information you provide is encrypted and transmitted securely.
- Publications
- Account settings
Preview improvements coming to the PMC website in October 2024. Learn More or Try it out now .
- Advanced Search
- Journal List
- ScientificWorldJournal
- v.2022; 2022

Pectinase from Microorganisms and Its Industrial Applications
Setegn haile.
Department of Biotechnology, College of Biological and Chemical Engineering, Addis Ababa Science and Technology University, 16417 Addis Ababa, Ethiopia
Abate Ayele
Associated data.
No data were used to support this study.
The utilization of microbial pectinase in different industries has been increased in its world demand. The major sources of pectinase are microorganisms mainly bacteria, fungi and yeast. The utilization of low-cost agro-industrial wastes as substrates has been preferable in pectinase production. Pectinase production faced various parameters optimization constraints such as temperature, pH and production times which are the main factors in pectinase production. The pectinase enzyme is getting attention due to its several advantages; hence, it needs to be explored further to take its maximum advantage in different industries. This review discusses the pectin substance structure, substrate for pectinase production, factors influencing pectinase production, the industrial application of microbial pectinase and also discusses challenges and future opportunities of applying microbial pectinase in industry.
1. Introduction
Enzymes are substances present in the cells of living organisms in small amounts which are capable of speeding up chemical reactions without themselves being altered after the reaction. As compared to chemical catalysts, enzymes have many advantages such as high specificity, a high catalytic efficiency, and an adjustable activity, which greatly promote the use of enzymes in pharmaceutical, chemical, and food industries [ 1 , 2 ]. Due to these desirable features, the demand for industrial enzymes has catapulted to new heights which call for constant research and development, to optimize their production and minimize resource costs [ 3 ]. The discovery of enzymes was started in the middle of the nineteenth century and they were first introduced in the industrial application using fungal enzymes. However, after twenty years Boidin and Effront introduced the bacterial enzymes in the industry [ 4 ].
Most of the industrial demand for enzymes is originated from microorganisms. Due to their high growing capability, short life span, and easiness of genetic manipulation, microorganisms are preferred in industry for enzyme production. Microbial enzymes are supplied, well-standardized, and marketed by a few competing companies. Among these industrially important enzymes, pectinases have a special significance due to their multiple uses in important sectors such as food, textile, beverages, pulp and paper, and biofuel industries [ 5 ]. The microbial pectinases account for twenty-five percent of the worldwide food and industrial enzyme scale and market increase from time to time [ 6 ].
The commercial applications of pectinases were observed in the 1930s [ 7 , 8 ]. Pectic enzyme is another name of pectinases that belongs to the polysaccharides family [ 9 ]. They assist plants in their cell wall extension, tissues softening at the time of maturation and storage and also ecologically maintain the plant's waste materials decomposition and recycling [ 10 ]. Pectinase enzymes are extensively used in the food industry particularly in fruit juice extraction and clarification [ 11 ].
The aim of this review begins with an overview of pectinases, their structure, and pectic material classification. Factors affecting pectinase production, pectinase substrate, pectinase microorganisms, and pectinolytic substrate are also addressed, as well as numerous industrial applications of microbial pectinases and challenges and future opportunities for using microbial pectinase in industry.
2. Pectic Substance and Its Structure
The compound hydrolyzed by pectinase has generic names called pectic substances. Pectin is also defined as a polysaccharide that is rich in very important sugars, galacturonic acid and methanol as main components and has a high molecular weight heterogeneous and acidic structural polysaccharide which is one of the major ingredients of cereals, vegetables, fruits and fibers [ 12 ]. Pectin constituent of the middle lamella and primary cell wall in the plant cell wall and within the wall forms a matrix in which a network of cellulose and hemicelluloses. According to Hassan and Ali [ 13 ]; the pectic substances are complicated colloidal acid polysaccharides with a long galacturonic acid pillar chain and interconnected together by glycoside bonds in Figure 1 . This is the basic unit of pectic substances which is also known as homogalacturonan.

Structure of pectin and its functional groups [ 14 ].
Pectin is one of the most complex bio-macromolecules in nature and it can be composed of 17 different monosaccharides and at least seven different polysaccharides as reported by [ 11 ]. Nighojkar et al. [ 15 ] reported that other sugars, such as D-glucuronic acid, L-fucose, D-glucose, D-mannose, and D-xylose are found in the side chains of pectin substances. Based on the American chemical society pectic substances that are used as a substrate in the pectinase productions are classified as pectic acid, pectin acid, pectin and protopectin. The main important criteria to classify those pectic substances were based on the solubility of these pectic substances by water in Table 1 [ 16 ].
Type of pectic substances and its description.
Pectin is considered one of the most complex substrates (heteropolysaccharides) found in the cell wall and middle lamella of fruits and vegetables [ 17 , 18 ]. Pectins have been detected in fruits and vegetables such as apple, citrus and beet in Table 2 .
Percentage of pectin in some vegetables and fruit [ 19 ].
2.1. Pectinase and Its Classification
Pectinases are defined as mixed enzymes that hydrolyze pectic substances, mostly present in microorganisms and higher plants [ 11 ]. Pectinase is the collection of enzymes that catalyze the pectin-containing substances produced by plants and microbes. The majority of commercial enzymes are obtained by employing fungal cultures [ 20 ]. Pectinase is involved in the metabolism of the cell wall as well as in the growth of the cell, senescence, ripening of fruits, pathogenesis and abscission process. Pectinases have been commercially prepared from the microorganisms especially from the fungi since the 1970s′, [ 21 ]. According to the enzyme commission and the international union of biochemistry, pectinase enzymes are classified under the hydrolase group [ 22 ].
2.1.1. Protopectinase
As explained in the previous Table 1 , the insoluble part of pectic substances is called protopectin and it needs other solvents to degrade pectin. Protopectinase is one classification of pectinase that degrade insoluble protopectin which is present in unripe fruits and gives rise to highly polymerized soluble pectin. The other name of this enzyme called pectinosinase [ 23 ]. Hassan and Ali [ 13 ] reported that protopectinase breaks down the insoluble protopectin into highly polymerized soluble pectin and its activity is can be analyzed by carbazole sulphuric acid method with the help of which the amount of pectin related material released from protopectin can be determined.
2.1.2. Pectin Methylesterases
One of the main functional groups in the pectin structure is methoxyl ester as shown in Figure 1 . Pectin methylesterases are responsible for the removal of this methoxyl group from pectic substances, and this removal process finally produces pectic acid and methanol. Pectin methylesterase is also known as pectin pectylhydrolase, pectinesterase, pectin demethoxylase, pectase, and pectolipase, is a carboxylic acid esterase and belongs to the hydrolase group of enzymes [ 24 ].
Pectin methylesterase which is extracted by fungi species act as a multichain system in the removal of a methyl group in randomly action and if pectin methylesterase produced by plants is acting either at a non-reducing end or next to a free carboxyl group and continue along the molecule by a single mechanism [ 25 ]. Pectin methylesterases de-esterification of methyl ester bond at α 1–4 D galacturonosylsubunit with the addition of water and produces Pectate polymer which is negatively charged and methanol in Figure 2 [ 26 ]. As Oumer [ 14 ] reported, the action of pectin methylesterase in pectic substances finally produced pectate.

Action of Pectin methylesterase on polygalacturonic acid chain [ 13 ].
2.1.3. Polygalacturonase
Polygalacturonase one of the classification pectinase and is also called depolymerase because it acts in the depolymerization process. Polygalacturonases are the pectinolytic enzymes that catalyze the hydrolytic cleavage of the polygalacturonic acid chain in the presence of water [ 26 , 27 ]. Therefore, the function of this enzyme is splitting the alpha 1–4 glycoside bond between galacturonic monomers. Patidar et al. [ 28 ] investigated that polygalacturonases are categorized into two parts. Those are endo-polygalacturonase and exo-polygalacturonase. Endopolygalacturonase hydrolyses polygalacturonic acids and liberates oligogalacturonic acids. Exo-polygalacturonase hydrolyzes pectic acids and liberates mono-galacturonate. Polygalacturonases are the pectinolytic-depolymerase enzyme that hydrolyses polygalacturonic acid chain at the point of 1⟶4 α glycosidic linkage with the induction of water molecule in Figure 3 . Polygalacturonasesare most widely studied in the family of pectinase enzymes that have functional, technical and biological involvement in the industries processing food and in the interaction between plants and fungus [ 21 ].

The action of polygalacturonases on polygalacturonic acid chain [ 13 ].
2.2. Substrate for Pectinases Production
Phutela et al. [ 29 ] reported that the natural substrates like malt sprout, wheat bran, rice bran, pomegranate, lemon, banana and orange, maximum pectinase activity of 589.0 ± 0.36 were observed in wheat bran by Aspergillus fumigatus . Bayoumi et al. [ 30 ] reported that maximum polygalacturonase productivity of 437.5 U/ml was obtained in the presence of 1.25 g/25 ml on Solanum tuberosum peels when compared to other agro-industrial wastes ( Solanum melanogena, Eichhornia crassipes and citrus peel) by Bacillus firmus at 37°C for 92 hours. Deepak et al. [ 31 ] utilized fruit wastes of cashew, banana, pineapple and grape for pectinase production by Aspergillus foetidus . Amongst the waste material, pectinase activity was found to be at a maximum level of 0.35 U/ml in grape waste followed by pineapple waste (30.0 U/ml) at a temperature of 40°C. Palaniyappan et al. [ 32 ] investigated the production of pectinase by Aspergillus Niger. The results of the study revealed that the pectinase activity was found to be at the highest level of 5.17 U/ml in 1% wheat flour as a substrate. Suresh and Viruthagiri [ 33 ] studied the pectinase production using wheat bran and sugarcane bagasse as substrates by Aspergillus Niger and observed maximum pectinase activity of 164.15 U/ml in M2 medium with mixed substrates of 90% of wheat bran and 10% sugarcane bagasse at 96 hrs of incubation.
Anuradha et al. [ 34 ] reported that the pectin rich raw substrates like orange peel, Jack fruit rind, carrot peel and beet-root peel, the maximum pectinase production was found in Jack fruit rind (38 U/ml) followed by carrot peel (36 U/ml) bee-root peel (24 U/ml) and orange peel (16.8 U/ml) by Aspergillus awamori . Bhardwaj and Garg [ 35 ] evaluated the selection of the substrate for the process of enzyme biosynthesis based on the following factors. (1) They should be the cheapest agro-industrial waste with availability at any time of the year. (2) Their storage should represent no problem in comparison with other substrates and should resist any drastic effect due to exposure to other environmental conditions e.g., temperature, variation in the weather from season to season and from day tonight. The family of citrus fruits consists of oranges, kinnow, khatta, lime, lemon (Galgal), Malta, Mausami, sweet orange, etc. and they all are known to contain an appreciable amount of pectin. Besides these, other fruits like mango ( Mangifera indica ), avocado pear ( Persea americana ), guava ( Psidium guajava ), banana ( Musa sapientum ), papaya ( Carica papaya ), cashew apple ( Anacardium occidentale ), garden-egg ( Solanum nigrum Linn.), star apple ( Crysophylum albidium ), and tomato ( Lycopersicum esculentum ) in Table 3 also contain substantial amounts of pectin having a high gelling grade.
Agro-industrial waste as substrates for pectinase production by using microorganisms.
2.3. Microorganisms for Pectinase Production
Initially, microorganisms such as bacteria, fungi and yeast as well as actinomycetes were extracted from the stomach of calves and baby goats. Enzymes are now produced. Micro-organism enzymes are better than animal or plant enzymes [ 48 ]. Different microorganisms are involved in the production of pectinase by using pectin as a carbon source. Pectins are degraded by several microorganisms that produce a variety of compounds and enzymes which are involved in several industrial applications. Many important bacteria, fungi and yeasts are skillful at degrading pectins substances to produce pectinases [ 41 ].
2.3.1. Pectinolytic Fungi
Several fungal species can degrade pectic substances by producing pectinolytic enzymes. The most popular and more efficient fungi in the pectinase production are Aspergillus Niger, Aspergillus awamori , Penicillium restrictum, Trichoderma viride , Mucor piriformis and Yarrowia lipolytica have a great role in both submerged as well as solid-state fermentation for the production of various industrially important products. Aspergillus Niger , Aspergillus oryzae , and Penicillium expansum are the types of fungi that are generally considered safe by the United States Food and Drugs Administration are put to use in the food industry [ 16 ]. Kumari et al. [ 49 ] isolate pectinase-producing strain Penicillium janthinellum from the soil and has been found to produce significant amounts of an extracellular pectinase subsequently characterized as exo-polygalacturonase. The different fungal strains from vegetable wastes and screened them for their pectinolytic activity. Among them, Tetracoccosporium species was found to be good producers of pectinase and it showed a clearance zone of 20 mm pectinolytic activity around the colonies [ 50 ]. Khairnar et al. [ 51 ] studied the pectinase production of different strains of Aspergillus Niger . They observed the highest zone of clearance of pectin hydrolysis in Aspergillus Niger is 4.5 mm. Ten fungal isolates were isolated from municipal solid waste. Among them, a maximum zone of clearance of above 3.0 mm for pectinolytic activity was exhibited by Penicillium chrysogenum and Aspergillus Niger [ 52 ]. Different researchers have been identified very important fungi to the production of pectinase in various temperatures as well as the duration of incubation time as shown below ( Table 4 ).
Optimization of important microorganisms in pectinase production.
2.3.2. Pectinolytic Yeasts
Kavuthodi and Sebastian [ 78 ] reported that Saccharomyces fragilis , Saccharomyces thermantitonum, Torulopsis kefyr, Candida pseudotropicalis var , lactosa, and Candida pseudotropicalis are types of yeast that can degrade pectin substances in the pectinase production processes. The other report also indicates additional yeast species for pectinase production, these species include Saccharomyces species , Cryptococcus species , Aureobasidium pullulans, Rhodotorula dairenensis, Kluyveromyces marxianus, Geotrichum klebahnii , and Wickerhanomyces anomalus , [ 13 , 79 ].
Wickerhamomyces anomalous one of the classification of species Pichia anomala produced pectinolytic enzymes in liquid medium containing glucose and citrus pectin as carbon and energy sources. In the current studies, enzymes made by this wild yeast strain were characterized, and physicochemical properties of polygalacturonase were determined by the study of the influence of temperature and pH on its activity and stability to evaluate the application of the supernatant in the maceration of potato tissues [ 80 ].
The different investigations identified different yeast species and characterized in molecular method to the production of pectinolytic enzymes from grapes peel. Based on that identification, several species have a good potential to degrade pectin substance these species include: Hanseniaspora species, Saccharomyces cerevisiae, Rhodotorula dairenensis, Candida zemplinina, Metschnikowia species, Aureobasidium pullulans, and Cryptococcus saitoi [ 81 ].
2.3.3. Pectinolytic Bacteria
Erwinia species, Pseudomonas fluorescens, Bacillus , Pseudomonas , and Micrococcus have a good potential to degrade pectin in the production of pectinase [ 20 , 56 , 78 ]. Other such as S treptomyces bacteria also has pectinolytic properties as reported by Ramirez-Tapias et al. [ 82 ]. Bacillus licheniformis has been reported as pectinolytic bacteria that were isolated from the rotten vegetable. The efficiency of Bacillus licheniformis to pectinase production was determined by the primary and secondary screening methods. The primary screening was carried out by the potassium–iodide flooding method and the secondary screening was carried out by fermentation. The efficiency of Bacillus licheniformis on the pectinase activity was recorded as 341 U/ml [ 83 ]. A newly isolated Brevibacillus borstelensis reported good pectinase (pectin lyase) production and characterization. The enzyme activities of Brevibacillus borstelensis were reported as 5.25 U/ml [ 84 ]. Soil is collected from different villages of Guntur District (Duggirala and Burripalem) from a depth of 1–15 inches to isolate desired pectinase-producing bacteria. This was used as the inoculum for the isolation of the organisms from the peel. Bacillus pumilus a potential pectinase-producing strain was isolated from this soil [ 85 ]. The pectinase-producing bacteria were investigated from orchard soil, at different locations of Kurukshetra, Haryana, India, using pectin agar medium at pH 7.2. Out of 109 bacterial pectinolytic isolates, isolate NV53 identified as Bacillus species MBRL576 produced a maximum zone of clearance after the addition of 1% cetyl trimethyl ammonium bromide and also exhibited the highest pectinase production [ 35 ]. Nine bacterial strains isolated from fruit and vegetable waste dump soil of two market areas, decayed banana, tomato and garden soil and reported that the maximum zone of clearance was observed in the bacterial isolate Streptococcus species 5 cm followed using Staphylococcus aureus anaerobius 5 cm [ 86 ]. Two bacterial strains isolated from rotten oranges and reported that the largest pectinolytic zone of 25 mm was observed by two bacterial strains Staphylococcus aureus and Bacillus cereus [ 87 ]. Different research works show that, among different bacterial isolates screened for pectinolytic properties Bacillus strains were selected as the most potent enzyme producers [ 56 , 88 ].
2.4. Factor Affecting Pectinase Production
Various Factors affect microbial pectinase production.
2.4.1. Effect of pH on Pectinase Production
The effects of pH on pectinase production have been reported by various researchers. Torimiro and Okonji [ 54 ] investigated the pectinase production by Bacillus species. Their report tried to the optimized effect of pH on the production of pectinase, the range of optimized pH was 4–10. But the maximum amount of pectinase was recorded at pH 7. The production and optimization of pectinase were carried out by Bacillus species MFW7 using Cassava as substrate, the optimization of this pectinase production was included various ranges of pH 3.5, 4.5, 5.5, 6.5, 7.5, 8.5 and 9.5, among that wide range of pH, the maximum pectinase activities were observed at pH of 6.5 as reported by Kumar et al. [ 89 ]. Another investigation showed pectinase produced from Chryseobacterium indologenes strain SD. This production was optimized with different ranges of pH 5–9 in 0.5 intervals, but the highest production of pectinase was obtained at pH 7.5 as investigated by Roy et al. [ 90 ]. The production of polygalacturonase was optimized by different ranges of pH by using Bacillus sphaericus. The ranges of pH were 4.4, 5, 5.6, 6.2, 6.8 and 7.4 in 0.6 intervals. From that pH variation, the maximum activities of polygalacturonase were obtained at 6.8 as reported by Jayani et al. [ 91 ]. The effect of pH on pectinase production was also optimized by using Bacillus species FW5 and Erwinia species FW2. The ranges of pH were 5–9. Among those various ranges of pH, the maximum production of pectinase was obtained at 7 by both Bacillus species FW5 and Erwinia species FW2 as reported by Mehta et al. [ 61 ].
2.4.2. The Effect of Temperature
Different researchers obtained maximum pectinase production by various bacterial species in different temperature ranges. The polygalacturonase was produced at different temperatures starting from 25–50°C in five-degree Celsius intervals. From this temperature, the maximum polygalacturonase was produced at 30°C by Bacillus sphaericus [ 91 ]. The maximum amount of production of polygalacturonase was produced by Enterobacter tabaci NR1466677. This study was carried out starting from 20–45°C. The optimum temperature of this enzyme production was observed at 35°C [ 92 ]. The maximum pectinase produced by Erwinia species FW2 within various temperature ranges. This temperature range was 20–65°C. Among those temperatures, the maximum pectinase production was observed at 37°C as reported by Mehta et al. [ 61 ]. The alkaline pectin lyase is produced by a newly isolated Brevibacillus borstelensis (P35). This alkaline pectinase was produced in the temperature range of 20–100°C. The maximum amount of this pectin lyase was observed at 60°C as reported by Demir et al. [ 84 ]. An extracellular Pectinase was also produced by newly isolated Bacillus subtilis strain and the maximum total activity of pectinase from Bacillus subtilis grown in medium including pectin as a carbon source at 37°C as reported by Mercimek takci and Turkmen [ 93 ]. Polygalacturonase is one part of pectinase produced by pectinolytic bacteria Bacillus licheniformis strain GD2. The production of polygalacturonase was produced in the three temperature ranges of 45–65°C and among these temperatures, the maximum amount of polygalacturonase activities appeared at 45°C [ 94 ]. The various Bacillus species have been reported as they can produce pectinase within various temperature ranges. The maximum activities of pectinase were recorded by Bacillus firmus and Bacillus endophyticus at the temperature of 37°C. The Bacillus coagulant and Bacillus vietnamisis were produced the maximum amount of pectinase activities at the temperature of 30°C as reported by Khan and Barate [ 95 ]. A different study shows that Erwinia species can produce polygalacturonase. The highest amount of polygalacturonase activities has been reported that were investigated in different temperature ranges starting from 20–45°C. But the maximum polygalacturonase activities were recorded at 35°C by Erwinia carotovora MTCC1428 as reported by Kothari and Baig, [ 58 ].
2.4.3. The Effect of Fermentation Times
The maximum production of pectinase from different microorganisms varies from time to time. The Bacillus species MFW7 produced a significant amount of pectinase after 96 hours of incubation in fermentation medium reported by Kalaichelva [ 89 ] and Erwinia carotovora MTCC1428 produced the maximum amount of polygalacturonase activity at the end of 72 hours fermentation time in liquid state fermentation condition [ 58 ]. The highest activities of polygalacturonase were observed after 120 hours of fermentation time by using Bacillus species [ 96 ]. The other bacterial isolate K6 was identified as Chryseobacterium indologenes strain. This isolate produces maximum extracellular pectinase at the end of 72 hours of incubation time [ 90 ]. The alkaline pectinase is also produced by the Cocci species. This species produced the maximum amount of pectinase after 72 hours fermentation times [ 97 ]. The maximum pectinase was produced using Erwinia species FW2 and Bacillus species FW5. Those two different species produced the maximum amount of pectinase after the end of 96 hours of fermentation time as reported by Mehta et al. [ 61 ].
2.4.4. The Effect of Substrate Concentration
As the concentration of pectin varies, it affected the production of pectinase. The highest amount of pectinase activities were observed at 0.8% of pectin concentration. This maximum activity of pectinase was carried out by taking various concentration ranges (0.1–1%) in 0.1% intervals of pectin concentration as investigated by Khan and Barate, [ 95 ]. Other studies indicated that as the concentration of pectin increased, the activity of pectinase increased up to optimum concentration and after the optimum concentration, the activity of pectinase was decreased. Among the various pectin concentrations ranges 0.1%, 0.2%, 0.5%, 1%, and 1.5%, the maximum amount of pectinase activities were recorded at 0.5% of pectin concentration and decreased after this concentration as reported by Mehta et al. [ 61 ]. Polygalacturonase is one part of pectinase that shows a good activity by different citrus pectin concentration ranges 0.25%, 0.5%, 0.75%, 1%, 1.25%, and 1.5%. The highest amount of polygalacturonase activities was recorded at 1.25% of citrus pectin concentration and the activities of polygalacturonase were decreased after that optimum pectin concentration as reported by Jayani et al. [ 91 ]. Polygalacturonase can produce from glucose, sucrose, galactose and soluble starch as a carbon source by Enterobacter aerogenes NBO2. This enzyme has been produced with different concentration of carbon sources (0.5%, 1%, 1.5%, 2%, 2.5% and 3% w/v). From those various concentrations of carbon source, the maximum amount of polygalacturonase was recorded at 1% of each carbon source as investigated by Darah et al. [ 98 ]. The highest Exo and endo pectinase activity of 0.79 U/ml and 0.01 U/ml was reported in sugar beet as substrate using Aspergillus Niger [ 99 ]. Okafor et al. [ 100 ] during their investigation on two pectinase-producing fungal isolates, Aspergillus Niger and Penicillium chrysogenum using the different agro-wastes, including pineapple peel, orange peels, sawdust, sugarcane pulps and wheat bran, as the sole carbon source reported the highest pectinase activity of 350.28 and 478.25 Uml-1 protein using Aspergillus Niger and Penicillium chrysogenum, respectively, in wheat bran as sole carbon source. From various agricultural waste and agro-industrial byproducts (banana peel, wheat bran, sugar cane bagasse, and orange bagasse), the best substrate for PG production by Penicillium species was found to be in orange bagasse with enhanced enzyme production of 64.5 U/mg followed by wheat bran 53.6 U/mg [ 101 ].
3. Industrial Applications of Microbial Pectinases
Applications studies with pectinases are ongoing in global research fields to obtain maximum fastened activity with enzymes. The wide application of pectinase is attributed to its increasing global demand. The application of pectinolytic enzyme is varied according to the availability of physical conditions. Pectinases have been used in several conventional industrial processes, such as textile, plant fiber processing, tea, coffee, and oil extraction and treatment of industrial wastewater, containing pectinaceous material in Figure 4 .

Various applications of pectinases [ 21 , 26 , 102 ].
3.1. Textile Processing
Pectinase, in combination with other enzymes such as amylase, lipase, cellulase, and hemicellulase, has been used in the textile industry to remove sizing agents from cotton, substituting the usage of harsh chemicals [ 103 ]. Different combinations of enzymes, such as cellulose with pectinase and cellulose with pectinase and protease, have been utilized for the bioscouring of cotton to achieve effective whiteness and absorbency of the textile fabric [ 104 ]. The use of enzymes such as pectinases in conjunction with amylases, lipases, cellulases and other hemicellulolytic enzymes to remove sizing agents has decreased the use of harsh chemicals in the textile industry, resulting in a lower discharge of waste chemicals to the environment, improving both the safety of working conditions for textile workers and the quality of the fabric [ 105 ].
3.2. Fruits and Vegetable Processing
Pulp treatment, fruit juice extraction, and clarity are all factors in the use of microbial pectinases in the fruit and vegetable industry. Pectinases contribute to the reduction of viscosity, the clarity of juice, and the maceration of vegetables, as well as the reduction of fermentation time [ 104 , 106 , 107 ]. In the fruit and vegetable juice industry, pectinase is widely used. These industries commercially produce a variety of juices, including sparkling clear juices, cloudy juices, and unicellular products, to selectively hydrolyze middle lamella polysaccharides to safeguard plant cell integrity [ 103 ]. Since the pectinolytic enzyme is one of the upcoming enzymes, most fruit industries are used in fruit juice processing. Due to the presence of pectin polysaccharide in fruit juice, the fruit juice is naturally cloud [ 108 ]. By nature; any fruits have a high concentration of pectin. This high concentration of pectin leads to the colloid formation in the juice, which leads to creating problems in the processing of clear fruit juices. The appearances of cloudiness in fruit juices also lead to a problem in the market. The traditional processes of extracting fruit juice are also not attractive and consume huge energy. Due to this reason, pectinase has a great role in the production, extraction and extraction of fruit juices [ 90 ]. The use of the enzymatic solution for the treatment of fruits and vegetable mash afforded a high juice extraction and a pulp with good pressing characteristics [ 96 ]. Pectinase is used in the fruit juice industry before clarifying to avoid pectin-protein flocculation and reduce viscosity. To improve permeation flux in microfiltration, ultrafiltration, and reverse osmosis, the early treatments of fruit juice with the addition of pectinase have been researched in large quantities [ 55 ]. According to the report of Ajayi et al. [ 109 ]; pectinase enzymes that obtained from deteriorated fruits as substrate was used in the clarification of apple juice from various apple fruits with different volume of pectinase to compare with the corresponding volume of water and commercial pectinase where applied in the juice clarification process. Pectinase lowers the viscosity of fruit juice during the clarifying process by degrading the pectin material in the juice and improving the pressing ability of the pulp, while simultaneously breaking down the jelly structure and increasing the yields of fruit juice. The refinement of vegetable fibers during the starch manufacturing process, such as the curing of coffee, cocoa, and tobacco, canning of orange segments, and extracting sugar from date fruits, is another important feature of pectinase enzymes in industrial processes [ 48 ]. Pectinases improve fruit juice production by reducing blurred vision and breaking pectic structures [ 110 ]. The use of pectinases in the preparation of fruit juice encourages the liberation of phenolic compounds from the fruit skin [ 111 ].
3.3. Wine Processing
Pectinolytic enzymes' primary roles in the winemaking process are to aid in extraction, maximize juice yield, facilitate filtration, and intensify flavor and color [ 106 ]. The use of pectinases in winemaking accelerates maceration, enhances juice extraction yield, speeds up filtration, and improves flavor and color. Before inoculating the alcoholic fermentation, the fruits were macerated with pectinases. This technique improves the wine's quality [ 111 ]. The addition of pectic enzymes to the crushing of fruits during the winemaking process enhances the volume of free-flow juice and reduces pressing time. It also aids in the filtration and clarity of juice as well as also improves the chromaticity and stability of red wines [ 112 ].
3.4. Coffee and Tea Fermentation
In the process of tea fermentation, instant tea powder have a great role to make drinking tea. This instant tea powder has a huge concentration of pectin because it is made from leaves. The preparation of tea by using this powder leads appearance of foam formation on tea due to the high concentration of pectin. Pectinase such as Polygalacturonase is used in the tea process to destroy the foam-forming property of instant tea powders by destroying pectins, increasing the quality of tea, color changes and highly valuable in the market [ 13 , 113 ]. Pectinase is also used in the coffee fermentation process. The coffee bean has had covers that surrounded its internal structures these hardcovers of coffee beans are called mucilage. The mucilage also has viscous and gelatinous properties that are not comfortable to make drinkable coffee. During the process of the alkaline pectinase is used to remove mucilage coat from the coffee bean before using the coffee bean [ 113 ]. Coffee is fermented with pectinolytic microbes to remove the mucilage coat from the beans and to improve tea fermentation and froth-producing properties [ 21 ]. Alkaline pectinases have been employed in tea fermentation to degrade pectins and remove the mucilaginous layer from coffee beans [ 17 ] in Figure 5 , preventing the foaming of instant tea granules [ 114 ].

Pectinase for mucilage removal of coffee beans [ 17 ].
3.5. Oil Extraction
Pectinase and other cell wall degrading enzymes (CWDE) have been widely explored for oil extraction from various sources such as flaxseed, olives, dates, and so on [ 104 ]. Citrus oils, such as lemon oil, can be extracted with pectinases because these enzymes disrupt pectin's emulsifying properties, which prevent oils from being removed from citrus peel extracts [ 14 , 105 ].
3.6. Paper and Pulp Industries
The use of chlorine-containing bleaching compounds in the paper and pulp industry produces toxic, mutagenic, and bioaccumulating organochlorine byproducts. These are the source of significant disruption in the ecosystem. The use of pectinase in this case is to avoid the toxicity of chlorinated compounds in the ecosystem [ 107 , 111 ]. Pectinases are used in the papermaking process to depolymerize galacturonic acid polymers, reducing the cationic requirement of pectin solutions [ 14 , 114 ]. In the paper and pulp industry, sheet formation is a critical process that is affected by the presence of pectins in the pulp, which causes yellowing of the paper [ 111 , 112 ].
3.7. Recycling of Waste Paper
Environmental risks are created by chemical deinking; however, enzymatic deinking minimizes pollution risks, energy consumption, disposal problems, and enhances performance. During the deinking process, a group of enzymes (pectinases, hemicellulases, cellulases, and ligninolytic enzymes) is used. Enzymes alter the bonding properties of ink and fiber, resulting in the removal of ink from the surface of the fibers during washing [ 111 ].
3.8. Wastewater Treatment
For treatment of wastewater from citrus processing industries, various processes have been investigated, which include: physical dewatering, spray irrigation, chemical coagulation, direct activated sludge treatment and chemical hydrolysis followed by methane fermentation. These processes have low efficiency due to chemical resistance of the pectic substances, high treatment cost, long treatment periods and complexity of the process farces [ 12 ]. Vegetable food processing industries release wastewater as by-products. These wastes have pectic substances because naturally, those vegetables are rich in pectic substances. Pretreatment of these wastewaters with pectinolytic enzymes facilitates removal of pectinaceous material and renders it suitable for decomposition by activated sludge treatment, [ 113 ]. Pectin is released by the vegetable food processing industry as a by-product, which contains wastewaters. The addition of pectinolytic enzymes to these wastewaters enhances the removal of pectinaceous material and makes them amenable for decomposition by activated sludge treatment [ 21 ]. Pectinases are enzymes that are used to remove pectin from wastewaters before they are treated. The use of pectinolytic organisms during the treatment of activated sludge is an environmentally beneficial, cost-effective, and time-saving process [ 111 ].
3.9. Prebiotics/Functional Foods
A prebiotic is a fermented food that allows for specific changes in the gut microbiome's makeup and/or activity to enhance the host immune system [ 107 , 115 ]. Pectin and pectin-derived oligosaccharides (PDO) are emerging as excellent candidates in new generation prebiotics. It has been observed that intestinal bacteria ferment methylated pectin to form short-chain fatty acids (SCFA) such as acetate, propionate, and butyrate, which are beneficial to health [ 107 ]. Pectinase is used to make functional food components and nutraceuticals, as well as to boost food's antioxidant potential [ 116 ].
4. Challenges and Future Opportunities of Applying Microbial Pectinase in Industry
Enzymes have a major impact on practically every industrial area (for example, food, feed, pharmaceuticals), and as a result, the market for industrial enzymes is rapidly expanding to meet the ever-increasing demand of consumers [ 117 ]. However, the stability of enzymes and the cost of enzymes lead to a delay in their advancement in industrial sectors. For commercialization, the enzyme's stability against severe temperatures, adverse pH environments, and organic solvents is critical. Enzymes' limited resistance to intense industrial conditions limits their application in commercial processes [ 103 ]. Several approaches can be used to optimize pectinase production; however, because enzymes are unstable, the cost of broad applicability is higher [ 104 , 117 ]. Thermophilic enzymes are gaining attention in research because temperature control during large-scale fermentation processes is challenging and expensive [ 107 ]. The cost viability of producing pectinase from selective microorganisms and implementing environmental conditions is one of the most important factors [ 118 ]. Pectinases can be used in a variety of industrial processes to improve the quality and quantity of final products. In this approach, it's critical to look into the production process and physicochemical properties of novel enzymes [ 119 ]. To further reduce the overall cost, research should be conducted in the area of immobilization of pectinase enzyme for reusing purposes [ 120 ]. Genetic engineering is a far more efficient option because the changes are completely controlled. This process involves taking the relevant gene from the microorganism that naturally produces a particular enzyme (donor) and inserting it into another microorganism that will produce the enzyme more efficiently (host) [ 22 ]. More study is needed to find strains that produce pectinase in combination with other enzymes, and the exact mix is needed for each application. This will reduce the cost of production for a specific application significantly. The emphasis of future pectinolytic research should be on elucidating the molecular mechanisms that regulate enzyme secretion as well as the mechanisms of action of distinct pectinolytic against various agro-industrial pectic substrates. In this way, well-designed studies can provide important tools for manipulating microbes to produce high quantities of efficient and cost-effective enzymes. Enzymology, molecular biology, and screening techniques have advanced, allowing the textile industry to develop new enzyme-based technologies that are more environmentally friendly. Pectinases appear to be able to perform all processes in the future. Pectinases have great attention in industrial applications such as the textile industry and fruit processing industries, oil extraction, coffee and tea fermentation. Finally, it is concluded that the role of pectinases in various industrial processes has been discovered to be curiously recognizable, with promising outcomes. From the extensive research, it is clear that pectinolytic enzymes have been considered as an imperative for the significant development or improvement of enzymes to industrial applications. Thus, the successful completion of this approach for the use of microbial pectinase, research should focus on protein engineering to obtain more robust and versatile pectic enzymes, as well as the optimization of production processes using new strains.
Acknowledgments
The authors wish to acknowledge all who had been instrumental in the creation of this review article.
Data Availability
Conflicts of interest.
The authors declare that they have no conflicts of interest.
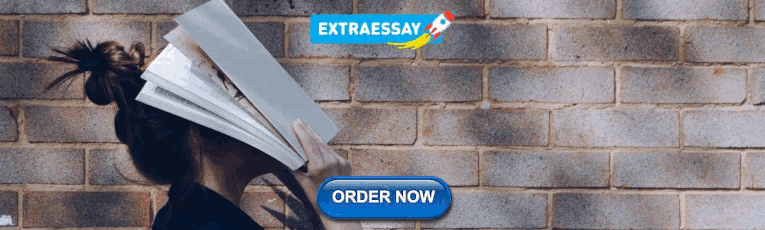
IMAGES
VIDEO
COMMENTS
isolated, from yeast cells, the soluble active form of the set of enzymes that catalyzes. the fermentation of sugar to alcohol. Emul Fischer reported the first systematic. studies on enzyme speci ...
Enzymes are potent catalysts. The enormous catalytic activity of enzymes can perhaps best be expressed by a constant, k cat, that is variously referred to as the turnover rate, turnover frequency or turnover number.This constant represents the number of substrate molecules that can be converted to product by a single enzyme molecule per unit time (usually per minute or per second).
Much of what an enzyme does is to bring the reacting species together in a geometry that favors reac-tion. This is so important that in some cases, reactivity (7). Finally, enzymes provide extra stabilizing inter-actions for the transition state (or unstable inter-mediates) in the reaction mechanism.
1.1. Introduction. The cell is the structural and functional unit of life—the basic building block of living systems. Cells have the capability to effectively utilize biocatalysts, known as enzymes, which have outstanding catalytic efficiency and both substrate and reaction specificity. Enzymes have amazing catalytic power and their high ...
Biotechnology and Applied Biochemistry publishes original full-length articles, short communications, and reviews of biotechnology. The journal is dedicated to rapid publication of high quality, cutting-edge research at the interface between life sciences and their technological exploitation. The Editors will consider papers for publication based on their novelty, their immediate or future ...
out by enzymes, and because of the fascinating aspects of the research related to it, enzymology has become one of the most rapidly expanding sciences. The primary purpose of this book is to discuss the role of enzymes in anesthesiology. To be able to accomplish this, it is necessary to consider first some basic aspects of enzymology.
Printed on acid-free paper 2/3141m-5 43210. Preface Today, as the full information about the genome is becoming available for a ... 1987 at the German National Research Centre for Biotechnology in Braun-schweig (GBF), continued at the University of Cologne from 1996 to 2007, and ... The enzymes in this Handbook are arranged according to the ...
2. Early enzymology‐demystifying life. In 1833, diastase (a mixture of amylases) was the first enzyme to be discovered, [2] quickly followed by other hydrolytic enzymes such as pepsin and invertase, [3] but the term enzyme was only coined in 1877 by Wilhelm Kühne. [4] The concept of catalysts, chemicals facilitating a reaction without undergoing any change themselves, was introduced in 1836 ...
Microbes utilize enzymes to perform a variety of functions. Enzymes are biocatalysts working as highly efficient machines at the molecular level. In the past, enzymes have been viewed as static entities and their function has been explained on the basis of direct structural interactions between the enzyme and the substrate. A variety of experimental and computational techniques, however ...
Enzymes are very speci c, and it was suggested by the Nobel laureate Emil Fischer in that this was because both the enzyme and the substrate possess speci c complementary geometricshapesthat texactlyintooneanother[ ]. isis o enreferredtoas thelockandkey model.However,while this model explains enzyme speci city, it fails to explain the
The molecular function of enzymes is defined as their ability to catalyze biochemical reactions; it is manually classified by the Enzyme Commission and robust approaches to quantitatively compare catalytic reactions are just beginning to appear. Here, we present an overview of studies at the interface of the evolution and function of enzymes.
Examining enzyme kinetics is critical for understanding cellular systems and for using enzymes in industry. The Michaelis-Menten equation has been widely used for over a century to estimate the ...
enzymes, and supplements published annually have now extended this number to over 5 000. Within this system, all enzymes are described by a four-part Enzyme Commission (EC) number. For example, the enzyme with the trivial name lactate dehydrogenase has the EC number 1.1.1.27, and is more correctly called l-lactate: NAD+ oxidoreductase.
2 Early enzymology-demystifying life. In 1833, diastase (a mixture of amylases) was the first enzyme to be discovered, 2 quickly followed by other hydrolytic enzymes such as pepsin and invertase, 3 but the term enzyme was only coined in 1877 by Wilhelm Kühne. 4 The concept of catalysts, chemicals facilitating a reaction without undergoing any change themselves, was introduced in 1836 5 by ...
The potential of enzyme-based drugs can be improved in regard to specific factors. First, the in vivo half-life of the molecules should be improved; second, the targeted action is not always accurate; and third, valid methods are necessary to control the patient's immune system response during treatments based on enzymes [].In this context, novel approaches to monitor the immune response ...
Gene editing has the potential to solve fundamental challenges in agriculture, biotechnology, and human health. CRISPR-based gene editors derived from microbes, while powerful, often show significant functional tradeoffs when ported into non-native environments, such as human cells. Artificial intelligence (AI) enabled design provides a powerful alternative with potential to bypass ...
The surprising power of small language models. Microsoft Research Blog, 2023. [JCWZ17]Mandar Joshi, Eunsol Choi, Daniel S. Weld, and Luke Zettlemoyer. Triviaqa: A large scale distantly supervised challenge dataset for reading comprehension, 2017. [JLD+23]Jiaming Ji, Mickel Liu, Juntao Dai, Xuehai Pan, Chi Zhang, Ce Bian, Chi Zhang, Ruiyang
Digestive enzymes are produced and secreted by the gastrointestinal system to degrade fats, proteins, and carbohydrates, to accomplish the digestion and, afterwards, the absorption of nutrients. Their supplementation, when indicated, may provide a reliable help as an adjuvant treatment of several disorders characterized by an impairment of ...
growing set of papers that use the toolkit of modern econometrics to evaluate industrial policy. We do not provide a comprehensive overview of current empirical research. 12 Rather, our goal is to evaluate where recent papers have made progress on empirical challenges and discuss where the largest gaps in our knowledge remain.
Microbial enzymes are supplied, well-standardized, and marketed by a few competing companies. Among these industrially important enzymes, pectinases have a special significance due to their multiple uses in important sectors such as food, textile, beverages, pulp and paper, and biofuel industries .