Center for Teaching
Visual thinking, introduction, images as metaphors, images as stories, schematic diagrams.
- Data Visualization
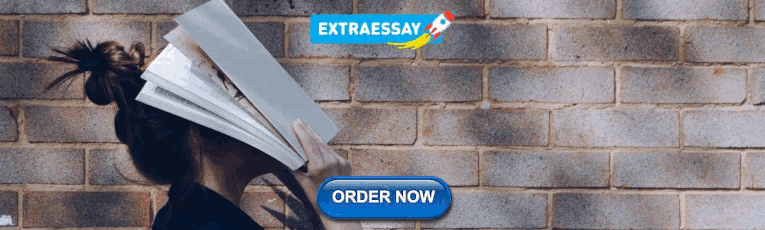
More Resources
Notes from march 2010 workshop.
Our brains are wired to rapidly make sense of and remember visual input. Visualizations in the form of diagrams, charts, drawings, pictures, and a variety of other ways can help students understand complex information. A well-designed visual image can yield a much more powerful and memorable learning experience than a mere verbal or textual description.
Below you’ll find resources for integrating visual thinking in your teaching. You’ll find ideas for enhancing your presentations and for incorporating visual thinking in your students’ in- and out-of-class activities.
On March 17, 2010, the CFT hosted a workshop on this topic. Here’s the Prezi that CFT staff Derek Bruff and Maria Ebner used to give participants in the workshop an overview of some visual thinking tools of potential use:
Visual Thinking in University Teaching on Prezi
For more notes from the workshop, as well as ideas participants generated during the workshop, see the bottom of this page.
Authoring cycle 2
Lecturing Basics
- Example: Kate O’Doherty, graduate students in psychology and human development and 2010-2011 CFT Teaching Affiliate, wrote about her use of VisualsSpeak in an educational psychology class she taught in the spring of 2010.
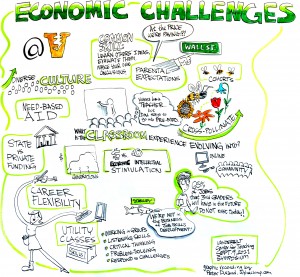
- Example: For examples of the kinds of photos you can find on Flickr, see these images found by Teaching and Learning doctoral students during an April 2011 workshop, and these images found by participants in a November 2011 conference workshop on visual thinking.
- Example: Five-Card Flickr is a storytelling exercise that involves selecting five Flickr images that tell a story. It’s based on the game Five-Card Nancy by comics expert Scott McCloud in which players make new stories out of individual panels of old Nancy comic strips.
- Example: “Digital Dossier” (embedded below) is a digital story told through a sequence of images accompanied by a soundtrack and voiceover. This type of digital story (one not involving actual video) is relatively easy to produce. See the University of Houston site for instructions. For more on “Digital Dossier,” see this Digital Natives blog post .
- Example: Dinosaur Comics is a comic strip that uses the same panels and images every day. The text, however, changes from day to day. In this blog post , Ryan North, creator of Dinosaur Comics , describes how a friend used Dinosaur Comics templates to teach English in Japan.
- Example: When Google introduced their Chrome Web browser in 2009, they had Scott McCloud create a 38-page comic book explaining how the new browser works.
- Example: Pixton is a free, online tool one can use to create comics. It allows you to create and alter images using a variety of drawing palettes, kind of like many video games allow users to create and alter their avatars.
- Example: Peter contributed to the Journal of American History article “ Ways of Seeing: Evidence and Learning in the History Classroom .” The article features essays by five historians describing ways they have included visual literacy in their courses, including a contribution by Tracy Weis of Millersville University, whose student slide was shared in the workshop.
- Example: Jason B. Jones, who teaches English at Central Connecticut State University, has his students collaboratively construct a Victorian Age timeline. Here’s a description of this assignment and a tutorial for putting together your own collaboratively built timelines.
- Example: xkcd, a “webcomic of romance, sarscasm, math, and language,” has featured movie narrative charts that show how characters in a few movies interact with each other over time.
Chaos and Fractals on Prezi
Thoughts on using Prezi as a teaching tool on Prezi
Healthcare Napkins All
- Example: For an example of one kind of visual thinking discussed in Roam’s book, see Greg Kulowiec’s post, “ Triptico Plot-It x American Revolution ,” in which the high school history teacher describes an activity where students plot events associated with the American Revolution on a coordinate plane.
- Example: VESTA (Visualization for Electronic and Structural Analysis) is a tool used by earth scientists to visualize and interact with the structure of various nanoscale objects. See the gallery for examples of what VESTA can do.
- Example: VUE is free, open-source software used to create concept maps.It has been developed by Tufts University and is available for download . The Visual Understanding Environment (VUE) “is focused on creating flexible tools for managing and integrating digital resources in support of teaching, learning and research. VUE provides a flexible visual environment for structuring, presenting, and sharing digital information.”
- Example: Debategraph is an online tool for collaboratively creating concept maps designed to help clarify debates. The interactive concept maps created by Debategraph show the relationships between arguments and evidence associated with debates and controversies. See how Christiane Amanpour uses Debategraph to add an interactive element to her CNN reporting.
- Example: Grant Casey’s Connectivism Blog explores possibilities for the use of concept maps and other online technologies to implement in his class. It is interesting to follow a group of educators experimenting with new concepts of knowledge and knowledge sharing.
Data Visualizations
- Example: Presentation Zen by Garr Reynolds provides great advice on creating simple, elegant charts and graphs that tell stories. For example, here’s a blog post by Reynolds showing the evolution of a chart from messy to elegant .
- Example: In his documentary An Inconvenient Truth , Al Gore uses a chart showing global CO2 concentrations over time to great effect. The sharp spike in CO2 levels in recent years is represented fairly dramatically in the chart. Al Gore stepping onto a scissor lift and rising through the air as the spike in the chart is revealed certainly didn’t hurt the dramatic effect!
- Example: Gapminder creates interactive infographics conveying many different kinds of data about the countries of the world. To see Gapminder in action, watch Gapminder director Hans Rosling’s TED talk below. You can also create your own Gapminder-style charts using Google Docs .
- Example: Vanderbilt Library’s Geographic Information Systems (GIS) coordinator, Lindsey Langsdon, is available to help faculty, staff, and students analyze and display geographic information. For examples of the kinds of informative maps that GIS can create, visit the Vanderbilt GIS map gallery.
- Now You See It: Simple Visualization Techniques for Quantitative Analysis by Stephen Few is a great book which focuses on the tools and techniques of visual business intelligence . See also Few’s Perceptual Edge blog .
- Envisioning Information by Edward Tufte provides strategies for visually displaying high-dimensional data. Tufte is an “analytical design” guru who is also known for his critique of PowerPoint, “ The Cognitive Style of PowerPoint .” Tufte’s Web site has further resources on visually presenting information.
- People interested in visual thinking (in and outside of academia) frequently bookmark resources on Delicious using the “vizthink” tag. See resources tagged using the Twitter hashtag #vizthink .
- Visual Thinking Strategies is a research-based education nonprofit that provides a teaching methodology, a developmentally appropriate image curriculum, and a learner-centered professional development program. Its Web site is full of useful resources, and it regularly organizes online and face-to-face workshops and events .
- Visual Language for Designers: Principles for Creating Graphics that People Understand by Conny Malamed is a large book that begins with a section on how we process visual information and then introduces principles that help you understand how to organize for perception and simplify visuals.
During the workshop, graphic facilitator Peter Durand captured the presentation and discussion on a virtual white board. Click on the images below for larger versions.
At the end the workshop, participants were asked to identify visual tools discussed in the workshop they wanted to try out in their teaching. Participants shared their ideas in words and pictures. Below you’ll find a few examples. Click on an image to see a larger version.

Teaching Guides
- Online Course Development Resources
- Principles & Frameworks
- Pedagogies & Strategies
- Reflecting & Assessing
- Challenges & Opportunities
- Populations & Contexts
Quick Links
- Services for Departments and Schools
- Examples of Online Instructional Modules
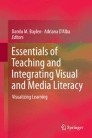
Essentials of Teaching and Integrating Visual and Media Literacy pp 49–73 Cite as
Understanding Visual Literacy: The Visual Thinking Strategies Approach
- Dabney Hailey 3 ,
- Alexa Miller 4 &
- Philip Yenawine 5
- First Online: 01 January 2015
4185 Accesses
15 Citations
This chapter makes the case for two aspects of visual literacy that the authors believe to be generally overlooked: (1) that visual literacy occurs by way of a developmental trajectory and requires instruction as well as practice, and (2) that it involves as much thought as it does visual awareness and is an integral component of the skills and beliefs related to inquiry. This chapter roots these ideas in the theory and research of cognitive psychologist Abigail Housen, coauthor of Visual Thinking Sttategies (VTS) with museum educator Philip Yenawine. Housen identified aesthetic stages that mark the development of skills helping to define visual literacy. Her research is also the basis of VTS, a method of engaging learners in deep experiences looking at art and discussing meanings with peers, a process that, this chapter posits, furthers visual literacy. This chapter presents that body of research and details the resulting VTS protocol. It reviews academic studies to date, subsequent to Housen, that document the impact of VTS interventions in various settings, and suggests beneficial areas for future research. In order to probe what development in visual literacy looks and sounds like on a granular level, two case studies of student writing from existing studies are presented and analyzed. Visual literacy skills enabled by VTS are briefly connected to broader educational concerns.
- Visual Thinking Sttategies
- Aesthetic development interview
- Viewing art
- Museum education
- Teaching strategies in museums
- Aesthetic experiences
- Visually literate
This is a preview of subscription content, log in via an institution .
Buying options
- Available as PDF
- Read on any device
- Instant download
- Own it forever
- Available as EPUB and PDF
- Compact, lightweight edition
- Dispatched in 3 to 5 business days
- Free shipping worldwide - see info
- Durable hardcover edition
Tax calculation will be finalised at checkout
Purchases are for personal use only
Adams, M., Foutz, S., & Luke, J. (2007) Thinking through art: Isabella Stewart Gardner museum school partnership program year 3 research results . Annapolis: Institute for Learning Innovation. http://www.gardnermuseum.org/microsites/tta/links/Year_3_Report.pdf .Accessed March 1, 2014
Arnheim, R. (1966). Toward a psychology of art . Berkeley: University of California Press.
Google Scholar
Arnheim, R. (1969). Visual thinking . Berkeley: University of California Press.
Baldwin, J. M. (1975). Thought and things: A study of the development and meaning of thought or generic logic (Vols. III and IV). New York: Arno.
Boudreau, J. D., Cassell, E. J., & Fuks, A. (2008). Preparing medical students to become skilled at clinical observation. Medical Teacher, 30, 857–862.
Article Google Scholar
Bruner, J. (1960). The process of education . Cambridge: Harvard University Press.
Bruner, J. (1966). Toward a theory of instruction . Cambridge: Harvard University Press.
Burchenal, M., & Grohe, M. (2007). Thinking through art: Transforming museum curriculum. Journal of Museum Education, 32 (2), 111–122. doi: http://dx.doi.org/10.1179/jme.2007.32.2.111 .
Changeux, J.-P. (2012). The good, the true, and the beautiful: A neuronal approach (trans: L. Garey). New Haven: Yale University Press.
Chapman, M., Hall, W., Colby, R., & Sisler L. A. G. (2013). How images work: An analysis of a visual intervention used to facilitate a difficult conversation and promote understanding. Qualitative Social Work, 13 (4), 456–476. doi:10.1177/1473325013496597.
Croskerry, P. (2003). The importance of cognitive errors in diagnosis and strategies to minimize them. Academic Medicine, 78 (8), 775–780.
Crouch, C. (2008). Afterword. In J. Elkins (Ed.), Visual literacy (pp. 195–204). New York: Routledge.
Curva, F., Milton, S., Wood, S., Palmer, D., & Nahmias, C. (2005). Artful citizenship report: Three-year project report . Tallahassee: The Wolfsonian-Florida International University. http://www.artfulcitizenship.org/pdf/full_report.pdf .Accessed March 1, 2014
Dallow, P. (2008). The visual complex: Mapping some interdisciplinary dimensions of visual literacy. In J. Elkins (Ed.) Visual literacy (pp. 91–104). New York: Routledge.
Debes, J. (1968). Some foundations for visual literacy. Audiovisual Instruction, 13, 961–964.
DeSantis, K., & Housen, A. (1984–2003). Selected directory of studies . New York: Visual Understanding in Education. http://vtshome.org/research/articles-other-readings .Accessed March 1, 2014
DeSantis, K., & Housen, A. (2007). Highlights of findings—San Antonio: Aesthetic development and creative and critical thinking skills study . New York: Visual Understanding in Education. http://vtshome.org/system/resources/0000/0004/SanAntonio-TX-VTS-Study.pdf .Accessed March 1, 2014
Dewey, J. (1910/1997). How we think . Boston: DC Heath & Company.
Dewey, J. (1934/1980). Art as experience . New York: Perigee Books.
Dewey, J. (1938/1997). Experience and education . New York: Touchstone Press.
Duke, L., & Housen, A. (1998). Responding to Alper: Re-presenting the MoMA studies on visual literacy and aesthetic development. Visual Arts Research, 24 (1), 92–102.
Duska, R., & Whelan, M. (1975). Moral development: A guide to Piaget and Kohlberg . New Jersey: Paulist.
Fleischer, N., Zea, A., McManama, J., & Miller, A. (March 2014). The science behind art: Teaching critical thinking through art observation . Presentation at Academic Dental Education Association conference, San Antonio, TX.
Geller, G. (2013). Tolerance for ambiguity: An ethics-based criterion for medical school selection. Academic Medicine, 88 (5), 1–4.
Grohe, M., & Egan, S. (July 2016). VTS growth over time . Presentation at the conference, VTS Summer Institute, Nelson-Atkins Museum of Art, Kansas City, MO.
Hailey, D. (2014). Visual thinking, art, and university teaching across disciplines. About Campus Magazine, 19 (4), 9–16.
Hershman, W., Miller, A., & Yadavalli, G. (2016). Fresh eyes: An arts-based workshop series for clinical faculty. In P. Brett-McLean & A. Peterkin (Eds.), Keeping reflection fresh: Top educators share their innovations in health professional education . Kent: Kent State (forthcoming).
Housen, A. (1983). The eye of the beholder: Measuring aesthetic development . Dissertation, Harvard University.
Housen, A. (1984–1991 ) . Institute of Contemporary Art Boston Audience Pilot Study (1984). Museum of Fine Arts Boston Asian Galleries Brochure Study (1990). Museum of Modern Art New York Gallery Talks Study (1991). Summary of unpublished studies. http://vtshome.org/pages/highlights-of-findings#7 .Accessed March 1, 2014
Housen, A. (1987). Three methods for understanding museum audiences. Museum Studies Journal, 2 (4), 41–49.
Housen, A. (September 1999). Eye of the beholder: Research, theory and practice . Paper presented the conference, Aesthetic and art education: A transdisciplinary approach. Calouste Gulbenkian Foundation, Lisbon, Portugal.
Housen, A. (2002). Aesthetic thought, critical thinking and transfer. Arts and Learning Journal, 18 (1), 99–132.
Housen, A. (2007). Art viewing and aesthetic development: Designing for the viewer. In P. Villeneuve (Ed.), Periphery to center: Art museum education in the 21st century (pp. 172–189). Reston: National Art Education Association.
Housen, A., & Yenawine, P. (2000–2001). VTS curriculum . New York: Visual Understanding in Education.
Jasani, S., & Saks, N. (2013). Utilizing visual art to enhance the clinical observation skills of medical students. Medical Teacher, 35, e1327–e1331. doi: http://informahealthcare.com/doi/pdf/10.3109/0142159X.2013 . 770131.
Klugman, C., Peel, J., & Beckmann-Mendez, D. (2011). Art rounds: Teaching interprofessional students visual thinking strategies at one school. Academic Medicine, 86 (10), 1266–1271.
Kohlberg, L., & Hirsch, R. H. (1977). Moral development: A review of the theory. Theory into Practice, 16 (2), 53–59.
Livingstone, M. (2002). Vision and art: The biology of seeing . New York: Abrams.
Loevinger, J. (1976). Ego development: Conceptions and theories . San Francisco: Jossey-Bass.
Loevinger, J. (1993). Measurement of personality: True or false. Psychological Inquiry: An International Journal for the Advancement of Psychological Theory, 4 (1), 1–16.
Miller, A., & Yenawine, P. (2014). Visual thinking, images, and learning in college. About Campus Magazine, 19 (4), 2–8.
Moorman, M. (2013). The meaning of visual thinking strategies for nursing students . Dissertation, University of Nevada Las Vegas.
Naghshineh, S., Hafler, J., Miller, A., Blanco, M. A., Lipsitz, S., Dubroff, R. P., Khoshbin, S., & Katz J. T. (2008). Formal art observation training improves medical students’ visual diagnostic skills. Journal of General Internal Medicine, 23 (7), 991–997.
Olson, S., & Loucks-Horsley, S. (2014). Inquiry and the National Science Education standards: A guide for teaching and learning. Committee on the Development of an Addendum to the National Science Education Standards on Scientific Inquiry; National Research Council. http://www.nap.edu/catalog.php?record_id=9596 .Accessed March 1, 2014
Parsons, M. (1987). How we understand art: A cognitive development account of aesthetic judgment . Cambridge: Cambridge University Press.
Piaget, J. (1926). The language and thought of the child . New York: Harcourt Brace.
Piaget, J. (1951). Play, dreams, and imitation in childhood . New York: W.W. Norton.
Rawlinson, K., Wood, S., Osterman, M., & Sullivan, C. (2007). Thinking critically about social issues through visual material. Journal of Museum Education, 32 (2), 155–174. doi: http://dx.doi.org/10.1179/jme.2007.32 . 2.155.
Shifrin, S. (2008). Visual literacy in North American secondary schools: Arts-centered learning, the classroom, and visual literacy. In J. Elkins (Ed.), Visual literacy (pp. 105–128). New York: Routledge.
Stafford, B. M. (2007). Echo objects: The cognitive work of images . Chicago: University of Chicago Press.
Stafford, B. M. (2008). The remaining ten percent. In J. Elkins (Ed.), Visual literacy (pp. 31–57). New York: Routledge.
Ter Horst, R., & Kruiper-Doesborgh, S. (2012). Visual thinking strategies, toegepast als therapie bij patiënten met niet-aangeboren hersenletsel. Tijdschrift voor Neuropsychologie, 7 (3), 141–150.
Vygotsky, L. (1962). Thought and language . CambridgeA: MIT Press.
Book Google Scholar
Vygotsky, L. (1978). Mind and society . Cambridge: Harvard University Press.
Yenawine, P. (2003). Jumpstarting visual literacy: Thoughts on image selection. Art Education, 56 (1), 6–12.
Yenawine, P. (2013). Visual thinking strategies: Using art to deepen thinking across school disciplines . Cambridge: Harvard Education Press.
Download references
Author information
Authors and affiliations.
Hailey Group, LLC, Cambridge, MA, USA
Dabney Hailey
Arts Practica, LLC, Guilford, CT, USA
Alexa Miller
Visual Understanding in Education, Wellfleet, MA, USA
Philip Yenawine
You can also search for this author in PubMed Google Scholar
Corresponding author
Correspondence to Dabney Hailey .
Editor information
Editors and affiliations.
Educational Technology and Foundations, University of West Georgia, Carrollton, Georgia, USA
Danilo M. Baylen
Adriana D'Alba
Abigail Housen’s stages of aesthetic development with details about stages 1 and 2 added. All quotes appearing here are taken from aesthetic development interviews Housen and associates conducted over 18 years. Aesthetic development interviews are nondirective, stream-of-consciousness-type interviews (Housen 1983 ).
Rights and permissions
Reprints and permissions
Copyright information
© 2015 Springer International Publishing Switzerland
About this chapter
Cite this chapter.
Hailey, D., Miller, A., Yenawine, P. (2015). Understanding Visual Literacy: The Visual Thinking Strategies Approach. In: Baylen, D., D'Alba, A. (eds) Essentials of Teaching and Integrating Visual and Media Literacy. Springer, Cham. https://doi.org/10.1007/978-3-319-05837-5_3
Download citation
DOI : https://doi.org/10.1007/978-3-319-05837-5_3
Published : 24 April 2015
Publisher Name : Springer, Cham
Print ISBN : 978-3-319-05836-8
Online ISBN : 978-3-319-05837-5
eBook Packages : Humanities, Social Sciences and Law Education (R0)
Share this chapter
Anyone you share the following link with will be able to read this content:
Sorry, a shareable link is not currently available for this article.
Provided by the Springer Nature SharedIt content-sharing initiative
- Publish with us
Policies and ethics
- Find a journal
- Track your research

- Education & Teaching
- Schools & Teaching

Enjoy fast, free delivery, exclusive deals, and award-winning movies & TV shows with Prime Try Prime and start saving today with fast, free delivery
Amazon Prime includes:
Fast, FREE Delivery is available to Prime members. To join, select "Try Amazon Prime and start saving today with Fast, FREE Delivery" below the Add to Cart button.
- Cardmembers earn 5% Back at Amazon.com with a Prime Credit Card.
- Unlimited Free Two-Day Delivery
- Streaming of thousands of movies and TV shows with limited ads on Prime Video.
- A Kindle book to borrow for free each month - with no due dates
- Listen to over 2 million songs and hundreds of playlists
- Unlimited photo storage with anywhere access
Important: Your credit card will NOT be charged when you start your free trial or if you cancel during the trial period. If you're happy with Amazon Prime, do nothing. At the end of the free trial, your membership will automatically upgrade to a monthly membership.
Buy new: $27.07 $27.07 FREE delivery: Friday, April 5 on orders over $35.00 shipped by Amazon. Ships from: Amazon.com Sold by: Amazon.com
Return this item for free.
Free returns are available for the shipping address you chose. You can return the item for any reason in new and unused condition: no shipping charges
- Go to your orders and start the return
- Select the return method
Buy used: $19.20
Fulfillment by Amazon (FBA) is a service we offer sellers that lets them store their products in Amazon's fulfillment centers, and we directly pack, ship, and provide customer service for these products. Something we hope you'll especially enjoy: FBA items qualify for FREE Shipping and Amazon Prime.
If you're a seller, Fulfillment by Amazon can help you grow your business. Learn more about the program.

Download the free Kindle app and start reading Kindle books instantly on your smartphone, tablet, or computer - no Kindle device required .
Read instantly on your browser with Kindle for Web.
Using your mobile phone camera - scan the code below and download the Kindle app.

Image Unavailable

- To view this video download Flash Player
Follow the author

Visual Thinking Strategies: Using Art to Deepen Learning Across School Disciplines 1st Edition
Purchase options and add-ons.
- ISBN-10 1612506097
- ISBN-13 978-1612506098
- Edition 1st
- Publisher Harvard Education Press
- Publication date October 1, 2013
- Language English
- Dimensions 6 x 0.5 x 8.9 inches
- Print length 208 pages
- See all details

Frequently bought together

Similar items that may ship from close to you

Editorial Reviews
You don’t have to convince me of the power art has to open the mind to new ways of seeing and thinking. I’m sold on Visual Thinking Strategies. This insightful book unpacks a technique that transforms classrooms into engaging, exciting laboratories for the critical thinking and communication skills our children need to master. --Daniel Pink, author of To Sell Is Human and A Whole New Mind
Practical and inspirational, this is arts integration for the twenty-first century. Distinguished museum educator Philip Yenawine presents a persuasive argument for using the art discussion approach called Visual Thinking Strategies (VTS) to develop the sophisticated thinking skills required by the Common Core. A lively blend of theory and practice, this book shows how VTS has sparked creative teaching in schools across the country. --Peggy Burchenal, Esther Stiles Eastman Curator of Education and Public Programs, Isabella Stewart Gardner Museum
From the Inside Flap
Philip Yenawine is cofounder of Visual Understanding in Education, a nonprofit research organization that trains teachers to use VTS.
From the Back Cover
About the author, product details.
- Publisher : Harvard Education Press; 1st edition (October 1, 2013)
- Language : English
- Paperback : 208 pages
- ISBN-10 : 1612506097
- ISBN-13 : 978-1612506098
- Item Weight : 10.1 ounces
- Dimensions : 6 x 0.5 x 8.9 inches
- #232 in Arts & Humanities Teaching Materials
- #254 in Elementary Education
- #314 in Common Core
About the author
Philip yenawine.
Discover more of the author’s books, see similar authors, read author blogs and more
Customer reviews
Customer Reviews, including Product Star Ratings help customers to learn more about the product and decide whether it is the right product for them.
To calculate the overall star rating and percentage breakdown by star, we don’t use a simple average. Instead, our system considers things like how recent a review is and if the reviewer bought the item on Amazon. It also analyzed reviews to verify trustworthiness.
- Sort reviews by Top reviews Most recent Top reviews
Top reviews from the United States
There was a problem filtering reviews right now. please try again later..

Top reviews from other countries

- Amazon Newsletter
- About Amazon
- Accessibility
- Sustainability
- Press Center
- Investor Relations
- Amazon Devices
- Amazon Science
- Start Selling with Amazon
- Sell apps on Amazon
- Supply to Amazon
- Protect & Build Your Brand
- Become an Affiliate
- Become a Delivery Driver
- Start a Package Delivery Business
- Advertise Your Products
- Self-Publish with Us
- Host an Amazon Hub
- › See More Ways to Make Money
- Amazon Visa
- Amazon Store Card
- Amazon Secured Card
- Amazon Business Card
- Shop with Points
- Credit Card Marketplace
- Reload Your Balance
- Amazon Currency Converter
- Your Account
- Your Orders
- Shipping Rates & Policies
- Amazon Prime
- Returns & Replacements
- Manage Your Content and Devices
- Recalls and Product Safety Alerts
- Conditions of Use
- Privacy Notice
- Consumer Health Data Privacy Disclosure
- Your Ads Privacy Choices
- Commitment to Diversity & Justice
- Land Acknowledgement
- PZ Doctoral Fellows
- Our First 50 Years
- Art & Aesthetics
- Assessment, Evaluation & Documentation
- Civic Engagement
- Cognition, Thinking & Understanding
- Collaboration & Group Learning
- Digital Life & Learning
- Disciplinary & Interdisciplinary Studies
- Ethics at Work
- Global & Cultural Understanding
- Humanities & Liberal Arts
- Leadership & Organizational Learning
- Learning Environments
- Making & Design
- Science Learning
- Early Childhood
- Primary / Elementary School
- Secondary / High School
- Higher Education
- Adult & Lifelong Learning
- Organizational Learning
- Agency by Design
- Aligned Programs for the 21st Century
- Artful Thinking
- Arts as Civic Commons
- Causal Learning Projects
- Center for Digital Thriving
- Citizen-Learners: A 21st Century Curriculum and Professional Development Framework
- Creando Comunidades de Indagación (Creating Communities of Inquiry)
- Creating Communities of Innovation
- Cultivating Creative & Civic Capacities
- Cultures of Thinking
- EcoLEARN Projects
- Educating with Digital Dilemmas
- Envisioning Innovation in Education
- Global Children
- Growing Up to Shape Our Place in the World
- Higher Education in the 21st Century
- Humanities and the Liberal Arts Assessment (HULA)
- Idea Into Action
- Implementation of The Good Project Lesson Plans
- Inspiring Agents of Change
- Interdisciplinary & Global Studies
- Investigating Impacts of Educational Experiences
- JusticexDesign
- Leadership Education and Playful Pedagogy (LEaPP)
- Leading Learning that Matters
- Learning Innovations Laboratory
- Learning Outside-In
- Making Ethics Central to the College Experience
- Making Learning Visible
- Multiple Intelligences
- Navigating Workplace Changes
- Next Level Lab
- Out of Eden Learn
- Pedagogy of Play
- Reimagining Digital Well-being
- Re-imagining Migration
- Signature Pedagogies in Global Education
- Talking With Artists Who Teach
- Teaching for Understanding
- The Good Project
- The Studio Thinking Project
- The World in DC
- Transformative Repair
- Visible Thinking
- Witness Tree: Ambassador for Life in a Changing Environment
- View All Projects
- At Home with PZ
- Thinking Routine Toolbox
- Zero In Newsletters
- View All Resources
- Professional Development
Search form
Project zero's thinking routine toolbox.
Welcome to Project Zero’s Thinking Routines Toolbox. This toolbox highlights thinking routines developed across a number of research projects at PZ. A thinking routine is a set of questions or a brief sequence of steps used to scaffold and support student thinking. PZ researchers designed thinking routines to deepen students’ thinking and to help make that thinking “visible.” Thinking routines help to reveal students’ thinking to the teacher and also help students themselves to notice and name particular “thinking moves,” making those moves more available and useful to them in other contexts. If you're new to thinking routines and PZ's research, please click here to explore more about thinking routines . For Tips for Using Thinking Routines Effectively, click here . For an overview of the Thinking Categories, click here . For an alphabetical list of thinking routines, click here .
- Artful Thinking ,
- Cultures of Thinking ,
- Agency by Design ,
- PZ Connect & Visible Thinking Resources ,
- and Interdisciplinary & Global Studies .
Background on PZ’s Visible Thinking
Project Zero’s broader work on Visible Thinking can be defined as a flexible and systematic research-based approach to integrating the development of students' thinking with content learning across subject matters. An extensive and adaptable collection of practices, the Visible Thinking research has a double goal: on the one hand, to cultivate students' thinking skills and dispositions, and, on the other, to deepen content learning. The PZ researchers working on the first Visible Thinking initiative, including Dave Perkins, Shari Tishman, and Ron Ritchhart, developed a number of important products, but the one that is best known over two decades later is the set of practices called Thinking Routines, which help make thinking visible. Thinking Routines loosely guide learners' thought processes. They are short, easy-to-learn mini-strategies that extend and deepen students' thinking and become part of the fabric of everyday classroom life.
Thinking routines exist in all classrooms. They are the patterns by which teachers and students operate and go about the job of learning and working together in a classroom environment. A routine can be thought of as any procedure, process, or pattern of actionthat is used repeatedly to manage and facilitate the accomplishment of specific goals or tasks. Classrooms have routines that serve to manage student behavior and interactions, to organize the work of learning, and to establish rules for communication and discourse. Classrooms also have routines that structure the way students go about the process of learning. These learning routines can be simple structures, such as reading from a text and answering the questions at the end of the chapter, or they may be designed to promote students' thinking, such as asking students what they know, what they want to know, and what they have learned as part of a unit of study.
PZ’s Visible Thinking research, both the initial project and the many projects that followed, makes extensive use of learning routines that are rich in thinking. These routines are simple structures, for example a set of questions or a short sequence of steps, that can be used across various grade levels and content areas. What makes them routines, versus mere strategies, is that they get used over and over again in the classroom so that they become part of the fabric of classroom' culture. The routines were designed by PZ researchers to become one of the regular ways students go aboutthe process of learning. Routines are patterns of action that can be integrated and used in a variety of contexts. Educators might even use more than one routine in teaching a single lesson. Routines don’t take time away from anything else educators are doing; instead, they enhance learning in the classroom.
The thinking routines included in this toolbox are organized in four ways –
- by a small set of “ Core Routines ” that target different types of thinking, are easy to get started with, and are commonly used by teachers in many disciplines and with learners of many ages,
- by the way educators use routines during a unit of study, similar to the arrangement used by Ritchhart, Church and Morrison (2011) ( Introducing and Exploring Ideas , Digging Deeper into Ideas , Synthesizing Ideas ),
- by the subject-area or topic the routines were developed to explore ( Objects & Systems , Art & Objects ), and,
- by the way educators use routines for conceptual exploration ( Possibilities and Analogies , Perspective Taking , & Perspectives, Controversies and Dilemmas ).
The Toolbox organizes the Thinking Routines into categories that describe the types of thinking the routines help to facilitate. Some routines appear in more than one category, and some routines have different versions that offer modifications for specific age groups or more specific conceptual challenges. When clicking on a routine in the Toolbox, a separate page opens with links to the downloadable PDF of the routine. All routines use a common PZ template describing the purpose of the routine, offering potential applications for the routine, and often providing suggestions for its use and tips for getting started. The PZ research project responsible for developing the routine is noted at the bottom of each page along with the copyright and licensing information and guidance about how to reference the routine. We invite and encourage educators to share their experiences using the routines! Each routine has a #hashtag listed just above the reference information. Jump in and get started!
Tips for Using Thinking Routines Effectively
- Thinking routines are designed to support particular kinds of thinking, so it’s important to choose the right tool for the specific type of thinking skill to be developed or nurtured.
- Thinking routines are also designed to be used routinely. In the same way that physical exercises need to be repeated in order to develop certain muscles, thinking routines, used repeatedly, help students to develop certain kinds of thinking. Rather than using a different thinking routine with every artifact, consider using the same thinking routine (such as See, Think, Wonder) with multiple artifacts.
- As you use the thinking routines, consider how you (or the students) will document students’ ideas and questions. Try to return to these ideas and questions at the end of the learning experience and in subsequent class sessions, so that you and the students can see how their thinking and understanding are developing.
Overview of Types of Thinking Categories
Core Thinking Routines Simple routines that are applicable across disciplines, topics, and age groups, and can be used at multiple points throughout a learning experience or unit of study. (A good place to start if you or your students are new to thinking routines.)
Introducing and Exploring Ideas Routines that help students articulate their thinking at the beginning of a learning experience and spark student curiosity and wonder, motivating further exploration.
Digging Deeper Into Ideas Routines that support students in building a deeper understanding of topics or experiences by asking them to analyze, evaluate, find complexity, and make connections.
Synthesizing and Organizing Ideas Routines that help students find coherence, draw conclusions, and distill the essence of topics or experiences.
Investigating Objects and Systems Routines that encourage students to examine everyday objects and systems, appreciate their design features, and explore their complexity.
Perspective-taking Routines that cultivate students’ capacity to look beyond their own perspective and to consider others’ experiences, thoughts, and feelings.
Considering Controversies, Dilemmas, and Perspectives Routines that promote students’ inclination to seek out and explore differences and tensions among multiple facets of complex issues.
Generating Possibilities and Analogies Routines that help students learn to formulate questions, consider alternatives, and make comparisons.
Exploring Art, Images, and Objects Routines that help develop students’ cultivate key skills of observation, interpretation, and questioning through engagement with art and objects.
Types of Thinking Categories
Clear All Filters
Core Thinking Routines
Simple routines that are applicable across disciplines, topics, and age groups, and can be used at multiple points throughout a learning experience or unit of study. (A good place to start if you or your students are new to thinking routines.)
Digging Deeper Into Ideas
Routines that support students in building a deeper understanding of topics or experiences by asking them to analyze, evaluate, find complexity, and make connections.
Introducing & Exploring Ideas
Routines that help students articulate their thinking at the beginning of a learning experience and spark student curiosity and wonder, motivating further exploration.
Investigating Objects & Systems
Routines that encourage students to examine everyday objects and systems, appreciate their design features, and explore their complexity.
Perspective-taking
Routines that cultivate students’ capacity to look beyond their own perspective and to consider others’ experiences, thoughts, and feelings.
Considering Controversies, Dilemmas, and Perspectives
Routines that promote students’ inclination to seek out and explore differences and tensions among multiple facets of complex issues.
Generating Possibilities and Analogies
Routines that help students learn to formulate questions, consider alternatives, and make comparisons.
Synthesizing & Organizing Ideas
Routines that help students find coherence, draw conclusions, and distill the essence of topics or experiences.
Exploring Art, Images, and Objects
Routines that help develop students’ cultivate key skills of observation, interpretation, and questioning through engagement with art and objects.
Global Thinking
- Privacy Policy
- Harvard Graduate School of Education
- Harvard University
- Digital Accessibility Policy
Copyright 2022 President and Fellows of Harvard College | Harvard Graduate School of Education
Subscribe to Our Mailing List
Email Address
By submitting this form, you are granting: Project Zero, 13 Appian Way, Cambridge, Massachusetts, 02138, United States, http://www.pz.gse.harvard.edu permission to email you. You may unsubscribe via the link found at the bottom of every email. (See our Email Privacy Policy for details.) Emails are serviced by Constant Contact.
- Open access
- Published: 19 March 2024
Interventions, methods and outcome measures used in teaching evidence-based practice to healthcare students: an overview of systematic reviews
- Lea D. Nielsen 1 ,
- Mette M. Løwe 2 ,
- Francisco Mansilla 3 ,
- Rene B. Jørgensen 4 ,
- Asviny Ramachandran 5 ,
- Bodil B. Noe 6 &
- Heidi K. Egebæk 7
BMC Medical Education volume 24 , Article number: 306 ( 2024 ) Cite this article
458 Accesses
Metrics details
To fully implement the internationally acknowledged requirements for teaching in evidence-based practice, and support the student’s development of core competencies in evidence-based practice, educators at professional bachelor degree programs in healthcare need a systematic overview of evidence-based teaching and learning interventions. The purpose of this overview of systematic reviews was to summarize and synthesize the current evidence from systematic reviews on educational interventions being used by educators to teach evidence-based practice to professional bachelor-degree healthcare students and to identify the evidence-based practice-related learning outcomes used.
An overview of systematic reviews. Four databases (PubMed/Medline, CINAHL, ERIC and the Cochrane library) were searched from May 2013 to January 25th, 2024. Additional sources were checked for unpublished or ongoing systematic reviews. Eligibility criteria included systematic reviews of studies among undergraduate nursing, physiotherapist, occupational therapist, midwife, nutrition and health, and biomedical laboratory science students, evaluating educational interventions aimed at teaching evidence-based practice in classroom or clinical practice setting, or a combination. Two authors independently performed initial eligibility screening of title/abstracts. Four authors independently performed full-text screening and assessed the quality of selected systematic reviews using standardized instruments. Data was extracted and synthesized using a narrative approach.
A total of 524 references were retrieved, and 6 systematic reviews (with a total of 39 primary studies) were included. Overlap between the systematic reviews was minimal. All the systematic reviews were of low methodological quality. Synthesis and analysis revealed a variety of teaching modalities and approaches. The outcomes were to some extent assessed in accordance with the Sicily group`s categories; “skills”, “attitude” and “knowledge”. Whereas “behaviors”, “reaction to educational experience”, “self-efficacy” and “benefits for the patient” were rarely used.
Conclusions
Teaching evidence-based practice is widely used in undergraduate healthcare students and a variety of interventions are used and recognized. Not all categories of outcomes suggested by the Sicily group are used to evaluate outcomes of evidence-based practice teaching. There is a need for studies measuring the effect on outcomes in all the Sicily group categories, to enhance sustainability and transition of evidence-based practice competencies to the context of healthcare practice.
Peer Review reports
Evidence-based practice (EBP) enhances the quality of healthcare, reduces the cost, improves patient outcomes, empowers clinicians, and is recognized as a problem-solving approach [ 1 ] that integrates the best available evidence with clinical expertise and patient preferences and values [ 2 ]. A recent scoping review of EBP and patient outcomes indicates that EBPs improve patient outcomes and yield a positive return of investment for hospitals and healthcare systems. The top outcomes measured were length of stay, mortality, patient compliance/adherence, readmissions, pneumonia and other infections, falls, morbidity, patient satisfaction, patient anxiety/ depression, patient complications and pain. The authors conclude that healthcare professionals have a professional and ethical responsibility to provide expert care which requires an evidence-based approach. Furthermore, educators must become competent in EBP methodology [ 3 ].
According to the Sicily statement group, teaching and practicing EBP requires a 5-step approach: 1) pose an answerable clinical question (Ask), 2) search and retrieve relevant evidence (Search), 3) critically appraise the evidence for validity and clinical importance (Appraise), 4) applicate the results in practice by integrating the evidence with clinical expertise, patient preferences and values to make a clinical decision (Integrate), and 5) evaluate the change or outcome (Evaluate /Assess) [ 4 , 5 ]. Thus, according to the World Health Organization, educators, e.g., within undergraduate healthcare education, play a vital role by “integrating evidence-based teaching and learning processes, and helping learners interpret and apply evidence in their clinical learning experiences” [ 6 ].
A scoping review by Larsen et al. of 81 studies on interventions for teaching EBP within Professional bachelor-degree healthcare programs (PBHP) (in English undergraduate/ bachelor) shows that the majority of EBP teaching interventions include the first four steps, but the fifth step “evaluate/assess” is less often applied [ 5 ]. PBHP include bachelor-degree programs characterized by combined theoretical education and clinical training within nursing, physiotherapy, occupational therapy, radiography, and biomedical laboratory students., Furthermore, an overview of systematic reviews focusing on practicing healthcare professionals EBP competencies testifies that although graduates may have moderate to high level of self-reported EBP knowledge, skills, attitudes, and beliefs, this does not translate into their subsequent EBP implementation [ 7 ]. Although this cannot be seen as direct evidence of inadequate EBP teaching during undergraduate education, it is irrefutable that insufficient EBP competencies among clinicians across healthcare disciplines impedes their efforts to attain highest care quality and improved patient outcomes in clinical practice after graduation.
Research shows that teaching about EBP includes different types of modalities. An overview of systematic reviews, published by Young et al. in 2014 [ 8 ] and updated by Bala et al. in 2021 [ 9 ], synthesizes the effects of EBP teaching interventions including under- and post graduate health care professionals, the majority being medical students. They find that multifaceted interventions with a combination of lectures, computer lab sessions, small group discussion, journal clubs, use of current clinical issues, portfolios and assignments lead to improvement in students’ EBP knowledge, skills, attitudes, and behaviors compared to single interventions or no interventions [ 8 , 9 ]. Larsen et al. find that within PBHP, collaboration with clinical practice is the second most frequently used intervention for teaching EBP and most often involves four or all five steps of the EBP teaching approach [ 5 ]. The use of clinically integrated teaching in EBP is only sparsely identified in the overviews by Young et al. and Bala et al. [ 8 , 9 ]. Therefore, the evidence obtained within Bachelor of Medicine which is a theoretical education [ 10 ], may not be directly transferable for use in PBHP which combines theoretical and mandatory clinical education [ 11 ].
Since the overview by Young et al. [ 8 ], several reviews of interventions for teaching EBP used within PBHP have been published [ 5 , 12 , 13 , 14 ].
We therefore wanted to explore the newest evidence for teaching EBP focusing on PBHP as these programs are characterized by a large proportion of clinical teaching. These healthcare professions are certified through a PBHP at a level corresponding to a University Bachelor Degree, but with strong focus on professional practice by combining theoretical studies with mandatory clinical teaching. In Denmark, almost half of PBHP take place in clinical practice. These applied science programs qualify “the students to independently analyze, evaluate and reflect on problems in order to carry out practice-based, complex, and development-oriented job functions" [ 11 ]. Thus, both the purpose of these PBHP and the amount of clinical practice included in the educations contrast with for example medicine.
Thus, this overview, identifies the newest evidence for teaching EBP specifically within PBHP and by including reviews using quantitative and/or qualitative methods.
We believe that such an overview is important knowledge for educators to be able to take the EBP teaching for healthcare professions to a higher level. Also reviewing and describing EBP-related learning outcomes, categorizing them according to the seven assessment categories developed by the Sicily group [ 2 ], will be useful knowledge to educators in healthcare professions. These seven assessment categories for EBP learning including: Reaction to the educational experience, attitudes, self-efficacy, knowledge, skills, behaviors and benefits to patients, can be linked to the five-step EBP approach. E.g., reactions to the educational experience: did the educators teaching style enhance learners’ enthusiasm for asking questions? (Ask), self-efficacy: how well do learners think they critically appraise evidence? (Appraise), skills: can learners come to a reasonable interpretation of how to apply the evidence? (Integrate) [ 2 ]. Thus, this set of categories can be seen as a basic set of EBP-related learning outcomes to classify the impact from EBP educational interventions.
Purpose and review questions
A systematic overview of which evidence-based teaching interventions and which EBP-related learning outcomes that are used will give teachers access to important knowledge on what to implement and how to evaluate EBP teaching.
Thus, the purpose of this overview is to synthesize the latest evidence from systematic reviews about EBP teaching interventions in PBHP. This overview adds to the existing evidence by focusing on systematic reviews that a) include qualitative and/ or quantitative studies regardless of design, b) are conducted among PBHP within nursing, physiotherapy, occupational therapy, midwifery, nutrition and health and biomedical laboratory science, and c) incorporate the Sicily group's 5-step approach and seven assessment categories when analyzing the EBP teaching interventions and EBP-related learning outcomes.
The questions of this overview of systematic reviews are:
Which educational interventions are described and used by educators to teach EBP to Professional Bachelor-degree healthcare students?
What EBP-related learning outcomes have been used to evaluate teaching interventions?
The study protocol was guided by the Cochrane Handbook on Overviews of Reviews [ 15 ] and the review process was reported in accordance with The Preferred Reporting Items for Systematic Reviews and Meta-analyses (PRISMA) statement [ 16 ] when this was consistent with the Cochrane Handbook.
Inclusion criteria
Eligible reviews fulfilled the inclusion criteria for publication type, population, intervention, and context (see Table 1 ). Failing a single inclusion criterion implied exclusion.
Search strategy
On January 25th 2024 a systematic search was conducted in; PubMed/Medline, CINAHL (EBSCOhost), ERIC (EBSCOhost) and the Cochrane library from May 2013 to January 25th, 2024 to identify systematic reviews published after the overview by Young et al. [ 8 ]. In collaboration with a research librarian, a search strategy of controlled vocabulary and free text terms related to systematic reviews, the student population, teaching interventions, teaching context, and evidence-based practice was developed (see Additional file 1 ). For each database, the search strategy was peer reviewed, revised, modified and subsequently pilot tested. No language restrictions were imposed.
To identify further eligible reviews, the following methods were used: Setting email alerts from the databases to provide weekly updates on new publications; backward and forward citation searching based on the included reviews by screening of reference lists and using the “cited by” and “similar results” function in PubMed and CINAHL; broad searching in Google Scholar (Advanced search), Prospero, JBI Evidence Synthesis and the OPEN Grey database; contacting experts in the field via email to first authors of included reviews, and by making queries via Twitter and Research Gate on any information on unpublished or ongoing reviews of relevance.
Selection and quality appraisal process
Database search results were merged, duplicate records were removed, and title/abstract were initially screened via Covidence [ 17 ]. The assessment process was pilot tested by four authors independently assessing eligibility and methodological quality of one potential review followed by joint discussion to reach a common understanding of the criteria used. Two authors independently screened each title/abstract for compliance with the predefined eligibility criteria. Disagreements were resolved by a third author. Four authors were paired for full text screening, and each pair assessed independently 50% of the potentially relevant reviews for eligibility and methodological quality.
For quality appraisal, two independent authors used the AMSTAR-2 (A MeaSurement Tool to Assess systematic Reviews) for reviews including intervention studies [ 18 ] and the Joanna Briggs Institute Checklist for systematic reviews and research Synthesis (JBI checklist) [ 19 ] for reviews including both quantitative and qualitative or only qualitative studies. Uncertainties in assessments were resolved by requesting clarifying information from first authors of reviews and/or discussion with co-author to the present overview.
Overall methodological quality for included reviews was assessed using the overall confidence criteria of AMSTAR 2 based on scorings in seven critical domains [ 18 ] appraised as high (none or one non-critical flaw), moderate (more than one non-critical flaw), low (one critical weakness) or critically low (more than one critical weakness) [ 18 ]. For systematic reviews of qualitative studies [ 13 , 20 , 21 ] the critical domains of the AMSTAR 2, not specified in the JBI checklist, were added.
Data extraction and synthesis process
Data were initially extracted by the first author, confirmed or rejected by the last author and finally discussed with the whole author group until consensus was reached.
Data extraction included 1) Information about the search and selection process according to the PRISMA statement [ 16 , 22 ], 2) Characteristics of the systematic reviews inspired by a standard in the Cochrane Handbook (15), 3) A citation index inspired by Young et al. [ 8 ] used to illustrate overlap of primary studies in the included systematic reviews, and to ensure that data from each primary study were extracted only once [ 15 ], 4) Data on EBP teaching interventions and EBP-related outcomes. These data were extracted, reformatted (categorized inductively into two categories: “Collaboration interventions” and “ Educational interventions ”) and presented as narrative summaries [ 15 ]. Data on outcome were categorized according to the seven assessment categories, defined by the Sicily group, to classify the impact from EBP educational interventions: Reaction to the educational experience, attitudes, self-efficacy, knowledge, skills, behaviors and benefits to patients [ 2 ]. When information under points 3 and 4 was missing, data from the abstracts of the primary study articles were reviewed.
Results of the search
The database search yielded 691 references after duplicates were removed. Title and abstract screening deemed 525 references irrelevant. Searching via other methods yielded two additional references. Out of 28 study reports assessed for eligibility 22 were excluded, leaving a total of six systematic reviews. Screening resulted in 100% agreement among the authors. Figure 1 details the search and selection process. Reviews that might seem relevant but did not meet the eligibility criteria [ 15 ], are listed in Additional file 2 . One protocol for a potentially relevant review was identified as ongoing [ 23 ].
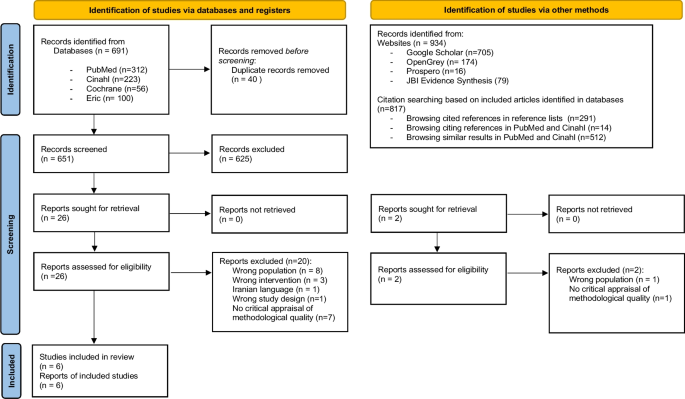
PRISMA flow diagram on search and selection of systematic reviews
Characteristics of included systematic reviews and overlap between them
The six systematic reviews originated from the Middle East, Asia, North America, Europe, Scandinavia, and Australia. Two out of six reviews did not identify themselves as systematic reviews but did fulfill this eligibility criteria [ 12 , 20 ]. All six represented a total of 64 primary studies and a total population of 6649 students (see Table 2 ). However, five of the six systematic reviews contained a total of 17 primary studies not eligible to our overview focus (e.g., postgraduate students) (see Additional file 3 ). Results from these primary studies were not extracted. Of the remaining primary studies, six were included in two, and one was included in three systematic reviews. Data from these studies were extracted only once to avoid double-counting. Thus, the six systematic reviews represented a total of 39 primary studies and a total population of 3394 students. Nursing students represented 3280 of these. One sample of 58 nutrition and health students and one sample of 56 mixed nursing and midwife students were included but none from physiotherapy, occupational therapy, or biomedical laboratory scientists. The majority ( n = 28) of the 39 primary studies had a quantitative design whereof 18 were quasi-experimental (see Additional file 4 ).
Quality of systematic review
All the included systematic reviews were assessed as having critically low quality with 100% concordance between the two designed authors (see Fig. 2 ) [ 18 ]. The main reasons for the low quality of the reviews were a) not demonstrating a registered protocol prior to the review [ 13 , 20 , 24 , 25 ], b) not providing a list of excluded studies with justification for exclusion [ 12 , 13 , 21 , 24 , 25 ] and c) not accounting for the quality of the individual studies when interpreting the result of the review [ 12 , 20 , 21 , 25 ].
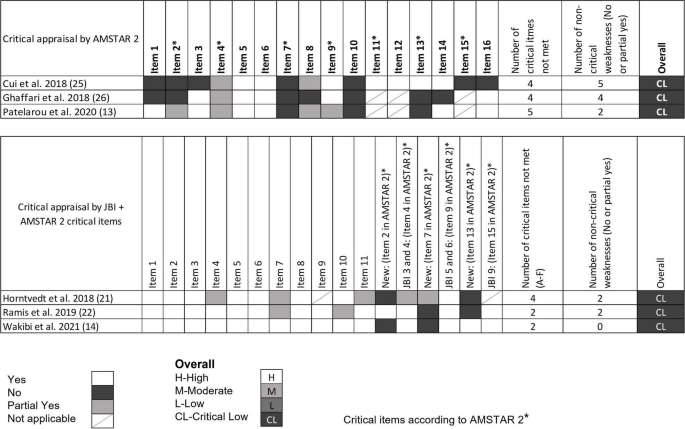
Overall methodological quality assessment for systematic reviews. Quantitative studies [ 12 , 24 , 25 ] were assessed following the AMSTAR 2 critical domain guidelines. Qualitative studies [ 13 , 20 , 21 ] were assessed following the JBI checklist. For overall classification, qualitative studies were also assessed with the following critical AMSTAR 2 domains not specified in the JBI checklist (item 2. is the protocol registered before commencement of the review, item 7. justification for excluding individual studies and item 13. consideration of risk of bias when interpreting the results of the review)
Missing reporting of sources of funding for primary studies and not describing the included studies in adequate detail were, most often, the two non-critical items of the AMSTAR 2 and the JBI checklist, not met.
Most of the included reviews did report research questions including components of PICO, performed study selection and data extraction in duplicate, used appropriate methods for combining studies and used satisfactory techniques for assessing risk of bias (see Fig. 2 ).
Main findings from the systematic reviews
As illustrated in Table 2 , this overview synthesizes evidence on a variety of approaches to promote EBP teaching in both classroom and clinical settings. The systematic reviews describe various interventions used for teaching in EBP, which can be summarized into two themes: Collaboration Interventions and Educational Interventions.
Collaboration interventions to teach EBP
In general, the reviews point that interdisciplinary collaboration among health professionals and/or others e.g., librarian and professionals within information technologies is relevant when planning and teaching in EBP [ 13 , 20 ].
Interdisciplinary collaboration was described as relevant when planning teaching in EBP [ 13 , 20 ]. Specifically, regarding literature search Wakibi et al. found that collaboration between librarians, computer laboratory technicians and nurse educators enhanced students’ skills [ 13 ]. Also, in terms of creating transfer between EBP teaching and clinical practice, collaboration between faculty, library, clinical institutions, and teaching institutions was used [ 13 , 20 ].
Regarding collaboration with clinical practice, Ghaffari et al. found that teaching EBP integrated in clinical education could promote students’ knowledge and skills [ 25 ]. Horntvedt et al. found that during a six-week course in clinical practice, students obtained better skills in reading research articles and orally presenting the findings to staff and fellow students [ 20 ]. Participation in clinical research projects combined with instructions in analyzing and discussing research findings also “led to a positive approach and EBP knowledge” [ 20 ]. Moreover, reading research articles during the clinical practice period enhances the students critical thinking skills. Furthermore, Horntvedt et al. mention, that students found it meaningful to conduct a “mini” – research project in clinical settings, as the identified evidence became relevant [ 20 ].
Educational interventions
Educational interventions can be described as “Framing Interventions” understood as different ways to set up a framework for teaching EBP, and “ Teaching methods ” understood as specific methods used when teaching EBP.
Various educational interventions were described in most reviews [ 12 , 13 , 20 , 21 ]. According to Patelarou et al., no specific educational intervention regardless of framing and methods was in favor to “ increase knowledge, skills and competency as well as improve the beliefs, attitudes and behaviors of nursing students” [ 12 ].
Framing interventions
The approaches used to set up a framework for teaching EBP were labelled in different ways: programs, interactive teaching strategies, educational programs, courses etc. Approaches of various durations from hours to months were described as well as stepwise interventions [ 12 , 13 , 20 , 21 , 24 , 25 ].
Some frameworks [ 13 , 20 , 21 , 24 ] were based on the assessments categories described by the Sicily group [ 2 ] or based on theory [ 21 ] or as mentioned above clinically integrated [ 20 ]. Wakibi et al. identified interventions used to foster a spirit of inquiry and EBP culture reflecting the “5-step approach” of the Sicily group [ 4 ], asking PICOT questions, searching for best evidence, critical appraisal, integrating evidence with clinical expertise and patient preferences to make clinical decisions, evaluating outcomes of EBP practice, and disseminating outcomes useful [ 13 ]. Ramis et al. found that teaching interventions based on theory like Banduras self-efficacy or Roger’s theory of diffusion led to positive effects on students EBP knowledge and attitudes [ 21 ].
Teaching methods
A variety of teaching methods were used such as, lectures [ 12 , 13 , 20 ], problem-based learning [ 12 , 20 , 25 ], group work, discussions [ 12 , 13 ], and presentations [ 20 ] (see Table 2 ). The most effective method to achieve the skills required to practice EBP as described in the “5-step approach” by the Sicely group is a combination of different teaching methods like lectures, assignments, discussions, group works, and exams/tests.
Four systematic reviews identified such combinations or multifaceted approaches [ 12 , 13 , 20 , 21 ]. Patelarou et al. states that “EBP education approaches should be blended” [ 12 ]. Thus, combining the use of video, voice-over, PowerPoint, problem-based learning, lectures, team-based learning, projects, and small groups were found in different studies. This combination had shown “to be effective” [ 12 ]. Similarly, Horntvedt et al. found that nursing students reported that various teaching methods improved their EBP knowledge and skills [ 20 ].
According to Ghaffari et al., including problem-based learning in teaching plans “improved the clinical care and performance of the students”, while the problem-solving approach “promoted student knowledge” [ 25 ]. Other teaching methods identified, e.g., flipped classroom [ 20 ] and virtual simulation [ 12 , 20 ] were also characterized as useful interactive teaching interventions. Furthermore, face-to-face approaches seem “more effective” than online teaching interventions to enhance students’ research and appraisal skills and journal clubs enhance the students critically appraisal-skills [ 12 ].
As the reviews included in this overview primarily are based on qualitative, mixed methods as well as quasi-experimental studies and to a minor extent on randomized controlled trials (see Table 2 ) it is not possible to conclude of the most effective methods. However, a combination of methods and an innovative collaboration between librarians, information technology professionals and healthcare professionals seem the most effective approach to achieve EBP required skills.
EBP-related outcomes
Most of the systematic reviews presented a wide array of outcome assessments applied in EBP research (See Table 3 ). Analyzing the outcomes according to the Sicily group’s assessment categories revealed that assessing “knowledge” (used in 19 out of 39 primary studies), “skills” (used in 18 out of 39 primary studies) and “attitude” (used in 17 out of 39) were by far the most frequently used assessment categories, whereas outcomes within the category of “behaviors” (used in eight studies) “reaction to educational experience” (in five studies), “self-efficacy” (in two studies), and “benefits for the patient” (in one study), were used to a far lesser extent. Additionally, outcomes, that we were not able to categorize within the seven assessment categories, were “future use” and “Global EBP competence”.
The purpose of this overview of systematic reviews was to collect and summarize evidence of the diversity of EBP teaching interventions and outcomes measured among professional bachelor- degree healthcare students.
Our results give an overview of “the state of the art” of using and measuring EBP in PBHP education. However, the quality of included systematic reviews was rated critically low. Thus, the result cannot support guidelines of best practice.
The analysis of the interventions and outcomes described in the 39 primary studies included in this overview, reveals a wide variety of teaching methods and interventions being used and described in the scientific literature on EBP teaching of PBHP students. The results show some evidence of the five step EBP approach in accordance with the inclusion criteria “interventions aimed at teaching one or more of the five EBP steps; Ask, Search, Appraise, Integrate, Assess/evaluate”. Most authors state, that the students´ EBP skills, attitudes and knowledge improved by almost any of the described methods and interventions. However, descriptions of how the improvements were measured were less frequent.
We evaluated the described outcome measures and assessments according to the seven categories proposed by the Sicily group and found that most assessments were on “attitudes”, “skills” and “knowledge”, sometimes on “behaviors” and very seldom on” reaction to educational experience”, “self-efficacy” and “benefits to the patients”. To our knowledge no systematic review or overview has made this evaluation on outcome categories before, but Bala et al. [ 9 ] also stated that knowledge, skills, and attitudes are the most common evaluated effects.
Comparing the outcomes measured between mainly medical [ 9 ] and nursing students, the most prevalent outcomes in both groups are knowledge, skills and attitudes around EBP. In contrast, measuring on the students´ patient care or on the impact of the EBP teaching on benefits for the patients is less prevalent. In contrast Wu et al.’s systematic review shows that among clinical nurses, educational interventions supporting implementation of EBP projects can change patient outcomes positively. However, they also conclude that direct causal evidence of the educational interventions is difficult to measure because of the diversity of EBP projects implemented [ 26 ]. Regarding EBP behavior the Sicily group recommend this category to be assessed by monitoring the frequency of the five step EBP approach, e.g., ASK questions about patients, APPRAISE evidence related to patient care, EVALUATE their EBP behavior and identified areas for improvement [ 2 ]. The results also showed evidence of student-clinician transition. “Future use” was identified in two systematic reviews [ 12 , 13 ] and categorized as “others”. This outcome is not included in the seven Sicily categories. However, a systematic review of predictive modelling studies shows, that future use or the intention to use EBP after graduation are influenced by the students EBP familiarity, EBP capability beliefs, EBP attitudes and academic and clinical support [ 27 ].
Teaching and evaluating EBP needs to move beyond aiming at changes in knowledge, skills, and attitudes, but also start focusing on changing and assessing behavior, self-efficacy and benefit to the patients. We recommend doing this using validated tools for the assessment of outcomes and in prospective studies with longer follow-up periods, preferably evaluating the adoption of EBP in clinical settings bearing in mind, that best teaching practice happens across sectors and settings supported and supervised by multiple professions.
Based on a systematic review and international Delphi survey, a set of interprofessional EBP core competencies that details the competence content of each of the five steps has been published to inform curriculum development and benchmark EBP standards [ 28 ]. This consensus statement may be used by educators as a reference for both learning objectives and EBP content descriptions in future intervention research. The collaboration with clinical institutions and integration of EBP teaching components such as EBP assignments or participating in clinical research projects are important results. Specifically, in the light of the dialectic between theoretical and clinical education as a core characteristic of Professional bachelor-degree healthcare educations.
Our study has some limitations that need consideration when interpreting the results. A search in the EMBASE and Scopus databases was not added in the search strategy, although it might have been able to bring additional sources. Most of the 22 excluded reviews included primary studies among other levels/ healthcare groups of students or had not critically appraised their primary studies. This constitutes insufficient adherence to methodological guidelines for systematic reviews and limits the completeness of the reviews identified. Often, the result sections of the included reviews were poorly reported and made it necessary to extract some, but not always sufficient, information from the primary study abstracts. As the present study is an overview and not a new systematic review, we did not extract information from the result section in the primary studies. Thus, the comprehensiveness and applicability of the results of this overview are limited by the methodological limitations in the six included systematic reviews.
The existing evidence is based on different types of study designs. This heterogeneity is seen in all the included reviews. Thus, the present overview only conveys trends around the comparative effectiveness of the different ways to frame, or the methods used for teaching EBP. This can be seen as a weakness for the clarity and applicability of the overview results. Also, our protocol is unpublished, which may weaken the transparency of the overview approach, however our search strategies are available as additional material (see Additional file 1 ). In addition, the validity of data extraction can be discussed. We extracted data consecutively by the first and last author and if needed consensus was reached by discussion with the entire research group. This method might have been strengthened by using two blinded reviewers to extract data and present data with supporting kappa values.
The generalizability of the results of this overview is limited to undergraduate nursing students. Although, we consider it a strength that the results represent a broad international perspective on framing EBP teaching, as well as teaching methods and outcomes used among educators in EBP. Primary studies exist among occupational therapy and physiotherapy students [ 5 , 29 ] but have not been systematically synthesized. However, the evidence is almost non-existent among midwife, nutrition and health and biomedical laboratory science students. This has implications for further research efforts because evidence from within these student populations is paramount for future proofing the quality assurance of clinical evidence-based healthcare practice.
Another implication is the need to compare how to frame the EBP teaching, and the methods used both inter-and mono professionally among these professional bachelor-degree students. Lastly, we support the recommendations of Bala et al. of using validated tools to increase the focus on measuring behavior change in clinical practice and patient outcomes, and to report in accordance with the GREET guidelines for educational intervention studies [ 9 ].
This overview demonstrates a variety of approaches to promote EBP teaching among professional bachelor-degree healthcare students. Teaching EBP is based on collaboration with clinical practice and the use of different approaches to frame the teaching as well as different teaching methods. Furthermore, this overview has elucidated, that interventions often are evaluated according to changes in the student’s skills, knowledge and attitudes towards EBP, but very rarely on self-efficacy, behaviors, benefits to the patients or reaction to the educational experience as suggested by the Sicily group. This might indicate that educators need to move on to measure the effect of EBP on outcomes comprising all categories, which are important to enhance sustainable behavior and transition of knowledge into the context of practices where better healthcare education should have an impact. In our perspective these gaps in the EBP teaching are best met by focusing on more collaboration with clinical practice which is the context where the final endpoint of teaching EBP should be anchored and evaluated.
Availability of data and materials
The datasets used an/or analyzed during the current study are available from the corresponding author on reasonable request.
Abbreviations
Evidence-Based Practice
Professional bachelor-degree healthcare programs
Mazurek Melnyk B, Fineout-Overholt E. Making the Case for Evidence-Based Practice and Cultivalting a Spirit of Inquiry. I: Mazurek Melnyk B, Fineout-Overholt E, redaktører. Evidence-Based Practice in Nursing and Healthcare A Guide to Best Practice. 4. ed. Wolters Kluwer; 2019. p. 7–32.
Tilson JK, Kaplan SL, Harris JL, Hutchinson A, Ilic D, Niederman R, et al. Sicily statement on classification and development of evidence-based practice learning assessment tools. BMC Med Educ. 2011;11(78):1–10.
Google Scholar
Connor L, Dean J, McNett M, Tydings DM, Shrout A, Gorsuch PF, et al. Evidence-based practice improves patient outcomes and healthcare system return on investment: Findings from a scoping review. Worldviews Evid Based Nurs. 2023;20(1):6–15.
Article PubMed Google Scholar
Dawes M, Summerskill W, Glasziou P, Cartabellotta N, Martin J, Hopayian K, et al. Sicily statement on evidence-based practice. BMC Med Educ. 2005;5(1):1–7.
Article PubMed PubMed Central Google Scholar
Larsen CM, Terkelsen AS, Carlsen AF, Kristensen HK. Methods for teaching evidence-based practice: a scoping review. BMC Med Educ. 2019;19(1):1–33.
Article CAS Google Scholar
World Health Organization. Nurse educator core competencies. 2016 https://apps.who.int/iris/handle/10665/258713 Accessed 21 Mar 2023.
Saunders H, Gallagher-Ford L, Kvist T, Vehviläinen-Julkunen K. Practicing healthcare professionals’ evidence-based practice competencies: an overview of systematic reviews. Worldviews Evid Based Nurs. 2019;16(3):176–85.
Young T, Rohwer A, Volmink J, Clarke M. What Are the Effects of Teaching Evidence-Based Health Care (EBHC)? Overview of Systematic Reviews PLoS ONE. 2014;9(1):1–13.
Bala MM, Poklepović Peričić T, Zajac J, Rohwer A, Klugarova J, Välimäki M, et al. What are the effects of teaching Evidence-Based Health Care (EBHC) at different levels of health professions education? An updated overview of systematic reviews. PLoS ONE. 2021;16(7):1–28.
Article Google Scholar
Copenhagen University. Bachelor in medicine. 2024 https://studier.ku.dk/bachelor/medicin/undervisning-og-opbygning/ Accessed 31 Jan 2024.
Ministery of Higher Education and Science. Professional bachelor programmes. 2022 https://ufm.dk/en/education/higher-education/university-colleges/university-college-educations Accessed 31 Jan 2024.
Patelarou AE, Mechili EA, Ruzafa-Martinez M, Dolezel J, Gotlib J, Skela-Savič B, et al. Educational Interventions for Teaching Evidence-Based Practice to Undergraduate Nursing Students: A Scoping Review. Int J Env Res Public Health. 2020;17(17):1–24.
Wakibi S, Ferguson L, Berry L, Leidl D, Belton S. Teaching evidence-based nursing practice: a systematic review and convergent qualitative synthesis. J Prof Nurs. 2021;37(1):135–48.
Fiset VJ, Graham ID, Davies BL. Evidence-Based Practice in Clinical Nursing Education: A Scoping Review. J Nurs Educ. 2017;56(9):534–41.
Pollock M, Fernandes R, Becker L, Pieper D, Hartling L. Chapter V: Overviews of Reviews. I: Higgins J, Thomas J, Chandler J, Cumpston M, Li T, Page M, et al., editors. Cochrane Handbook for Systematic Reviews of Interventions version 62. 2021 https://training.cochrane.org/handbook Accessed 31 Jan 2024.
Page MJ, McKenzie JE, Bossuyt PM, Boutron I, Hoffmann TC, Mulrow CD, m.fl. The PRISMA 2020 statement: an updated guideline for reporting systematic reviews. BMJ. 2021;372:1-9
Covidence. Covidence - Better systematic review management. https://www.covidence.org/ Accessed 31 Jan 2024.
Shea BJ, Reeves BC, Wells G, Thuku M, Hamel C, Moran J, et al. AMSTAR 2: a critical appraisal tool for systematic reviews that include randomised or non-randomised studies of healthcare interventions, or both. BMJ. 2017;21(358):1–9.
Joanna Briggs Institute. Critical Appraisal Tools. https://jbi.global/critical-appraisal-tools Accessed 31 Jan 2024.
Horntvedt MT, Nordsteien A, Fermann T, Severinsson E. Strategies for teaching evidence-based practice in nursing education: a thematic literature review. BMC Med Educ. 2018;18(1):1–11.
Ramis M-A, Chang A, Conway A, Lim D, Munday J, Nissen L. Theory-based strategies for teaching evidence-based practice to undergraduate health students: a systematic review. BMC Med Educ. 2019;19(1):1–13.
Rethlefsen ML, Kirtley S, Waffenschmidt S, Ayala AP, Moher D, Page MJ, et al. PRISMA-S: an extension to the PRISMA Statement for Reporting Literature Searches in Systematic Reviews. Syst Rev. 2021;10(1):1–19.
Song CE, Jang A. Simulation design for improvement of undergraduate nursing students’ experience of evidence-based practice: a scoping-review protocol. PLoS ONE. 2021;16(11):1–6.
Cui C, Li Y, Geng D, Zhang H, Jin C. The effectiveness of evidence-based nursing on development of nursing students’ critical thinking: A meta-analysis. Nurse Educ Today. 2018;65:46–53.
Ghaffari R, Shapoori S, Binazir MB, Heidari F, Behshid M. Effectiveness of teaching evidence-based nursing to undergraduate nursing students in Iran: a systematic review. Res Dev Med Educ. 2018;7(1):8–13.
Wu Y, Brettle A, Zhou C, Ou J, Wang Y, Wang S. Do educational interventions aimed at nurses to support the implementation of evidence-based practice improve patient outcomes? A systematic review. Nurse Educ Today. 2018;70:109–14.
Ramis MA, Chang A, Nissen L. Undergraduate health students’ intention to use evidence-based practice after graduation: a systematic review of predictive modeling studies. Worldviews Evid Based Nurs. 2018;15(2):140–8.
Albarqouni L, Hoffmann T, Straus S, Olsen NR, Young T, Ilic D, et al. Core competencies in evidence-based practice for health professionals: consensus statement based on a systematic review and Delphi survey. JAMA Netw Open. 2018;1(2):1–12.
Hitch D, Nicola-Richmond K. Instructional practices for evidence-based practice with pre-registration allied health students: a review of recent research and developments. Adv Health Sci Educ Theory Pr. 2017;22(4):1031–45.
Download references
Acknowledgements
The authors would like to acknowledge research librarian Rasmus Sand for competent support in the development of literature search strategies.
This work was supported by the University College of South Denmark, which was not involved in the conduct of this study.
Author information
Authors and affiliations.
Nursing Education & Department for Applied Health Science, University College South Denmark, Degnevej 17, 6705, Esbjerg Ø, Denmark
Lea D. Nielsen
Department of Oncology, Hospital of Lillebaelt, Beriderbakken 4, 7100, Vejle, Denmark
Mette M. Løwe
Biomedical Laboratory Science & Department for Applied Health Science, University College South Denmark, Degnevej 17, 6705, Esbjerg Ø, Denmark
Francisco Mansilla
Physiotherapy Education & Department for Applied Health Science, University College South Denmark, Degnevej 17, 6705, Esbjerg Ø, Denmark
Rene B. Jørgensen
Occupational Therapy Education & Department for Applied Health Science, University College South Denmark, Degnevej 17, 6705, Esbjerg Ø, Denmark
Asviny Ramachandran
Department for Applied Health Science, University College South Denmark, Degnevej 17, 6705, Esbjerg Ø, Denmark
Bodil B. Noe
Centre for Clinical Research and Prevention, Section for Health Promotion and Prevention, Bispebjerg and Frederiksberg Hospital, Nordre Fasanvej 57, 2000, Frederiksberg, Denmark
Heidi K. Egebæk
You can also search for this author in PubMed Google Scholar
Contributions
All authors have made substantial contributions to the conception and design of the study, acquisition of data, analysis, and interpretation of data, writing the main manuscript, preparing figures and tables and revising the manuscripts.
Corresponding author
Correspondence to Lea D. Nielsen .
Ethics declarations
Ethics approval and consent to participate.
Not applicable.
Consent for publication
Competing interests.
The authors declare no competing interests.
Additional information
Publisher’s note.
Springer Nature remains neutral with regard to jurisdictional claims in published maps and institutional affiliations.
Supplementary Information
Supplementary material 1., supplementary material 2., supplementary material 3., supplementary material 4., rights and permissions.
Open Access This article is licensed under a Creative Commons Attribution 4.0 International License, which permits use, sharing, adaptation, distribution and reproduction in any medium or format, as long as you give appropriate credit to the original author(s) and the source, provide a link to the Creative Commons licence, and indicate if changes were made. The images or other third party material in this article are included in the article's Creative Commons licence, unless indicated otherwise in a credit line to the material. If material is not included in the article's Creative Commons licence and your intended use is not permitted by statutory regulation or exceeds the permitted use, you will need to obtain permission directly from the copyright holder. To view a copy of this licence, visit http://creativecommons.org/licenses/by/4.0/ . The Creative Commons Public Domain Dedication waiver ( http://creativecommons.org/publicdomain/zero/1.0/ ) applies to the data made available in this article, unless otherwise stated in a credit line to the data.
Reprints and permissions
About this article
Cite this article.
Nielsen, L.D., Løwe, M.M., Mansilla, F. et al. Interventions, methods and outcome measures used in teaching evidence-based practice to healthcare students: an overview of systematic reviews. BMC Med Educ 24 , 306 (2024). https://doi.org/10.1186/s12909-024-05259-8
Download citation
Received : 29 May 2023
Accepted : 04 March 2024
Published : 19 March 2024
DOI : https://doi.org/10.1186/s12909-024-05259-8
Share this article
Anyone you share the following link with will be able to read this content:
Sorry, a shareable link is not currently available for this article.
Provided by the Springer Nature SharedIt content-sharing initiative
- MH "Students, Health occupations+"
- MH "Students, occupational therapy"
- MH "Students, physical therapy"
- MH "Students, Midwifery"
- “Students, Nursing"[Mesh]
- “Teaching"[Mesh]
- MH "Teaching methods+"
- "Evidence-based practice"[Mesh]
BMC Medical Education
ISSN: 1472-6920
- Submission enquiries: [email protected]
- General enquiries: [email protected]
- Our Mission
Developing Early Literacy Skills With Hexagonal Thinking Strategies
Hexagonal thinking can enhance literacy instruction by encouraging students to make connections between sounds, words, and meanings.
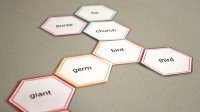
Hexagonal thinking is a highly effective, versatile learning strategy that helps students see connections between various concepts. It was created by Betsy Potash as a critical thinking activity that is frequently used in social studies, science, and language arts.
During the activity, students place hexagons next to each other to visually show a connection. For each connection, they must also be able to share a rationale. This strategy therefore allows teachers to gain insight into students’ thinking and to potentially correct misconceptions in real time.
Students can work individually to complete the activity, but its real power manifests when they work in pairs or groups. It’s a “low floor/high ceiling” activity, meaning it’s accessible and has a high yield on learning.
As a special education teacher working with fourth-grade students of varying abilities, I wondered how I could utilize this strategy when teaching reading. Learning how to read requires, at minimum, two major abilities: decoding and language comprehension—the end result of which is reading comprehension.
When students struggle either with their ability to decode or with their language comprehension, the consequence is that they also have difficulty comprehending what they read. Using hexagonal thinking in my classroom allows me to engage students while differentiating instruction to meet various student needs. It allows me to provide scaffolding and feedback in the moment. And students love completing a learning activity that feels like a puzzle.
I’ve used this approach to teach relationships between phonemes (sounds), orthography (spelling), syntax (parts of speech), and morphology (meaning).
One of the earliest and most essential skills that children learn is to rhyme. They learn that hat and bat end the same way, with the -at ending. As they progress, they learn about the individual sounds that make up the word hat : /h/-/a/-/t/. They also begin to isolate sounds like the /a/ in the middle.
Hexagonal thinking can support and promote this skill. For novice learners, you can use a forced-choice approach, asking students to identify all of the words, for example, that have the same middle sound: hat , cat , rat , bat .
The students can tell you what all of these words have in common. For striving learners, you can prompt them to tell you the differences: Hat starts with /h/, for example, while bat starts with /b/. For less scaffolding, you can provide cards with multiple middle sounds and multiple beginning and/or ending sounds, therefore challenging your learners.
Orthography
Spelling is a more challenging skill for students to acquire. Hexagonal thinking requires students to slow down and look for orthographic patterns in words. For example, the words twice and ice both have a soft c sound because the c is followed by an e.
You can further challenge your students by sharing two words with the same sounds but different spellings. They can place the words cow and loud next to each other and work to discover and provide an explanation that the two words have the same sound, but one uses -ow to spell that sound, while the other uses -ou .
Another use for hexagonal thinking is making connections between the functions of words. Using patterns that students have already learned, you can create hexagons that also fall into the categories of noun, verb, or adjective.
Here, again, you can provide maximum scaffolding by color-coding or using words from one category, or you can have your students determine what categories the words represent. This approach would be ideal for categories like concrete and abstract nouns, various forms of the verb “to be,” action verbs, and common or proper nouns.
Morphology and Vocabulary
Morphemes are the smallest unit of meaning in language. Morphemes include prefixes ( re- , un- , dis- ), suffixes (plural -s and -es ), base word (like run in the word running ), and root words (like -ject in the word interject ).
You can give younger and novice learners words that share a prefix, such as unkind , undo , and unable , and then ask them to explain what those words have in common and how the prefix changes each base word. With students who are ready for an extra challenge, consider giving them words that have a shared root word, and ask them to explain how the words are related—for example, phone , megaphone , phonograph , and symphony .
Vocabulary takes it a step further and allows students to work with synonyms, antonyms, and even shades of meaning. I had students create a hive of words that share an essential meaning but vary in degree of similarity, as in this set of words: stroll , jog , run , and sprint . This activity offers students the opportunity to engage in rich discourse, not only about the concrete meaning of each word, but in the nuances of each word as well.
Deepening Literacy
Some children will naturally see connections between words and literacy concepts; however, most students will need multiple exposures to words through deliberate practice in order to achieve mastery. Deliberate practice includes a specific goal, a high level of engagement, and coaching or feedback throughout the process. Hexagonal thinking meets that goal.
For educators catering to students with diverse learning needs, hexagonal thinking presents a dynamic approach to teaching reading skills. Its structured format allows for scaffolding and personalized feedback, catering to individual student abilities, and facilitating a deeper understanding of fundamental reading components such as phonemes, orthography, syntax, and morphology.
By encouraging students to analyze relationships between words and their meanings, hexagonal thinking promotes vocabulary development and cultivates comprehension skills essential for proficient reading.
Thank you for visiting nature.com. You are using a browser version with limited support for CSS. To obtain the best experience, we recommend you use a more up to date browser (or turn off compatibility mode in Internet Explorer). In the meantime, to ensure continued support, we are displaying the site without styles and JavaScript.
- View all journals
- My Account Login
- Explore content
- About the journal
- Publish with us
- Sign up for alerts
- Open access
- Published: 27 January 2024
An actor-model framework for visual sensory encoding
- Franklin Leong ORCID: orcid.org/0009-0000-5510-5975 1 ,
- Babak Rahmani 2 nAff5 ,
- Demetri Psaltis ORCID: orcid.org/0000-0003-4684-8800 3 ,
- Christophe Moser ORCID: orcid.org/0000-0002-2078-0273 2 &
- Diego Ghezzi ORCID: orcid.org/0000-0002-0554-7510 1 , 4
Nature Communications volume 15 , Article number: 808 ( 2024 ) Cite this article
3164 Accesses
113 Altmetric
Metrics details
- Biomedical engineering
- Computational science
- Electrical and electronic engineering
- Information technology
- Learning algorithms
A fundamental challenge in neuroengineering is determining a proper artificial input to a sensory system that yields the desired perception. In neuroprosthetics, this process is known as artificial sensory encoding, and it holds a crucial role in prosthetic devices restoring sensory perception in individuals with disabilities. For example, in visual prostheses, one key aspect of artificial image encoding is to downsample images captured by a camera to a size matching the number of inputs and resolution of the prosthesis. Here, we show that downsampling an image using the inherent computation of the retinal network yields better performance compared to learning-free downsampling methods. We have validated a learning-based approach (actor-model framework) that exploits the signal transformation from photoreceptors to retinal ganglion cells measured in explanted mouse retinas. The actor-model framework generates downsampled images eliciting a neuronal response in-silico and ex-vivo with higher neuronal reliability than the one produced by a learning-free approach. During the learning process, the actor network learns to optimize contrast and the kernel’s weights. This methodological approach might guide future artificial image encoding strategies for visual prostheses. Ultimately, this framework could be applicable for encoding strategies in other sensory prostheses such as cochlear or limb.
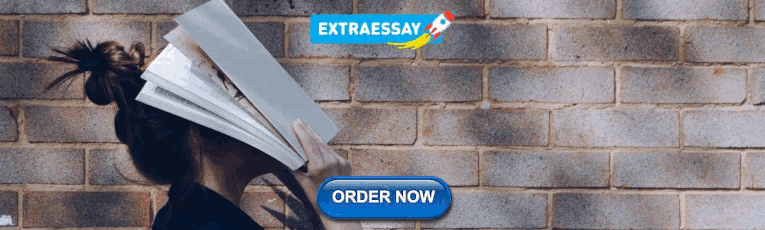
Similar content being viewed by others
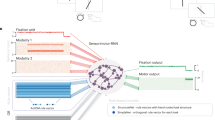
Natural language instructions induce compositional generalization in networks of neurons
Reidar Riveland & Alexandre Pouget
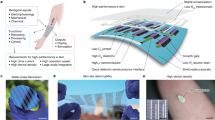
High-speed and large-scale intrinsically stretchable integrated circuits
Donglai Zhong, Can Wu, … Zhenan Bao
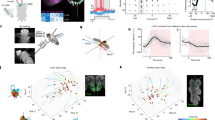
Motor neurons generate pose-targeted movements via proprioceptive sculpting
Benjamin Gorko, Igor Siwanowicz, … Stephen J. Huston
Introduction
Sensory organs capture information from the environment and convert it into neuronal signals that are interpreted by the brain during cognition. This transformation is known as sensory encoding.
Similarly, a sensory prosthesis converts information from sensors into artificial stimulation parameters to replace natural functions (artificial sensory encoding). However, prosthetic devices typically have an input range much smaller than biological systems. For example, the number of electrodes in neural implants is usually several orders of magnitude lesser than the number of sensory neurons 1 , 2 . Therefore, artificial sensory encoding is a form of dimensionality reduction. High-dimensional information from sensors is reduced to a few stimulation parameters while trying to maximally preserve the information, so that few electrodes can write information in a format that the brain can read and understand. A notable example is auditory encoding in cochlear implants, where sound is converted into electrical stimulation of a few frequency regions within the auditory nerve 3 , 4 , 5 . This process allows deaf individuals to hear sound. Likewise, limb prostheses provide amputees with tactile feedback to enhance manual dexterity, increase prosthesis embodiment, and improve their quality of life 6 , 7 , 8 .
Artificial sensory encoding plays a huge role also in visual prostheses. Artificial visual encoding converts high resolution images captured by an external camera into a spatiotemporal pattern of artificial stimuli delivered by a retinal 9 , 10 , 11 , 12 , optic nerve 13 , 14 , or cortical prosthesis 15 , 16 . Artificial visual encoding is critical to improve the patient’s perception, but it is not straightforward. In the retina, information flows from approximately 120 million photoreceptors to roughly 1.2 million retinal ganglion cells (RGCs) divided into several classes, which project to several brain nuclei, including the lateral geniculate nucleus and then to the visual areas where further image processing occurs 17 , 18 , 19 , 20 . The complexity of the visual information process requires advanced encoding strategies to ensure effective stimulation of the visual neurons leading to a useful artificial vision.
To date, there have been several visual prostheses implanted in patients 16 , 21 , 22 , 23 , 24 , 25 , 26 , but most devices were tested to recognize only letters and shapes using simple image encoding techniques (e.g. pixel averaging). In the Argus® II, the most implanted device so far, pixel averaging was used in conjunction with video filters to downsample the camera image to the resolution of the implanted array (6 × 10 pixels). Recently, there has been considerable research dedicated to the development of better image encoding algorithms. Some approaches include object detection, edge detection, and content-aware retargeting method 27 , 28 . In general, such methods aim to reduce the complexity of the image and highlight interesting content and features. For example, edge detection may identify the discontinuity of brightness in an image to locate the outline of an object. By reducing the amount of information, the user could better perceive the environment. However, these algorithms do not consider the retinal information processing from photoreceptors to RGCs. Therefore, their encoding potential might be limited.
Finding the proper artificial input to a sensory system that elicits the desired perception is an ill-posed problem: there are multiple inputs that could possibly yield the same output. In a linear system, the ability to produce a desired output can be determined by measuring the system’s response to a series of arbitrary inputs (forward pathway) and then computing its inverse (backward pathway). Determining the forward pathway would entail measuring the responses of the whole system, which is practically impossible in a biological system given the large number of cells and the low number and resolution of measuring electrodes. Yet, even if the system’s forward pathway is fully characterized, for large scale systems involving many variables, obtaining its inverse (backward pathway) is computationally intensive. Moreover, neuronal processing is non-linear, and it is probed only with partial measurements, thus further complicating this problem.
There had been significant efforts to generate in-silico retina models (forward pathway) that potentially could be used for efficient artificial visual encoding 29 , 30 , 31 , 32 . Retinal information processing is complex 33 , 34 , and finding a high-performing in-silico retinal model is critical since it will directly impact the outcome of the image encoding algorithm. In recent years, convolutional neural networks (CNNs) have been very successful at modeling the retina and outperformed conventional approaches such as linear-nonlinear models or generalized linear models 35 , 36 , which are less effective in capturing the retinal dynamics when white noise and natural scenes are presented 35 , 36 . Hence, using CNNs to model the retina presents a great potential in improving artificial visual encoding.
Still, a computational method to estimate the backward pathway, given the limited set of measures obtained in the forward pathway, is necessary for artificial visual encoding. Again, neural networks perform well in solving ill-posed inverse problems 37 . Therefore, we propose an end-to-end neural network-based approach for both retina modeling and image encoding which considers the retinal information processing. We validate an actor-model framework designed to learn non-linear downsampling patterns through a learning-based approach 37 , 38 . Performance is assessed through the measurement of neuronal reliability 35 . By integrating the measured retinal information processing into the framework, we demonstrated, in-silico and ex-vivo, that the generated downsampled images elicit a neuronal response with higher neuronal reliability (+4.9% in-silico and +2.9% ex-vivo, median percent increase) compared to a learning-free approach (i.e. pixel averaging). During the process, the actor network learned to optimize contrast and the kernel’s weights evolved towards a Mexican hat shape which resembles the receptive field (RF) of RGCs. These properties work in conjunction to enable effective downsampling.
The actor-model framework used in this study is general and could be exploited for other image encoding processes or even in other fields of artificial sensory encoding, such as auditory and tactile. Albeit belonging to different sensory pathways, auditory and tactile sensations share similar properties which allow us to postulate the potential effectiveness in other sensory encoding systems 34 , 39 . This learning-based approach could serve as a template for future encoding strategies accounting for the natural transformation process occurring in the sensory organ. A more effective encoding method entails that the brain could better interpret the encoded information, leading to improved perception of a prosthesis user.
The actor-model framework in retinal processing
The actor-model framework is built following a 3-step approach (Fig. 1 ). In step 1, we projected a set of high-resolution images \({{{{{\bf{X}}}}}}\in {{\mathbb{R}}}^{128x128}\) (128 × 128 pixels) to mouse retinas explanted over a transparent multielectrode array (MEA) used to detect neural spikes from RGCs in response to image projection. For each identified RGC, we built a response vector by summing up the average number of spikes in response to image projection (Fig. 1 , inset). Then, we combined the response vectors from each recorded RGC across multiple retinas into a neural response matrix forming the ground truth response \({{{{{\bf{Y}}}}}}\in {{\mathbb{R}}}^{n{{{{{\rm{x}}}}}}m}\) where n is the number of RGCs ( n = 60 RGCs from N = 10 retinas) and m is the number of images projected ( m = 1200). We refer to the explanted retinas as the biological system ( \({{{{{\mathscr{F}}}}}}:{{{{{\bf{X}}}}}}\to {{{{{\bf{Y}}}}}}\) ). In step 2, we trained a CNN to act as a digital twin of the retina. We refer to it as the forward model \((\hat{{{{{{\mathscr{F}}}}}}}:{{{{{\bf{X}}}}}}\to \hat{{{{{{\bf{Y}}}}}}})\) . We sent the same high-resolution images to the forward model, generating a predicted response matrix ( \(\hat{{{{{{\bf{Y}}}}}}}\) ). We calculated the Poisson loss against the prediction and ground truth to update the forward model along with the regularization terms ( \(\theta \ast={{\mbox{arg}}}{\min }_{\theta }[\ell (\widehat{{ {\mathcal F} }_{\theta }}({{{{{\bf{X}}}}}}),\, {\mathcal F} ({{{{{\bf{X}}}}}}))+{{{{{\bf{R}}}}}}]\) ) where \({{{{{{\rm{\theta }}}}}}}^{*}\) is the optimized parameters of \(\hat{{{{{{\mathscr{F}}}}}}}\) , l is the loss function, and R is the regularization term. Then, we conducted hyperparameter optimization with a random search. Once the forward model is trained, we fix its weights. In step 3, we prepended another CNN, the actor network \(A:{{{{{\bf{X}}}}}}\to {{{{{{\bf{X}}}}}}}_{{{{{{\bf{down}}}}}}}\) where \({{{{{{\bf{X}}}}}}}_{{{{{{\bf{down}}}}}}}\in {{\mathbb{R}}}^{32x32}\) , which learns to downsample images. Again, we sent the same high-resolution image set into the actor network, which reduces them to lower-resolution images (32 × 32 pixels, four-fold downsampling). The low-resolution images are sent through the fixed forward model, generating a predicted response matrix \({\hat{{{{{{\bf{Y}}}}}}}}_{{{{{{\bf{Actor}}}}}}}=\hat{{{{{{\mathscr{F}}}}}}}(A({{{{{\bf{X}}}}}}))\) . Similar to step 2, we compared the predicted response of the lower-resolution images against the ground truth by calculating the Poisson loss. The loss is then used to update the actor network. The forward model remained fixed. As the actor network is updated, it learns to distill pertinent features to downsample images while generating a neuronal response similar to high-resolution images.
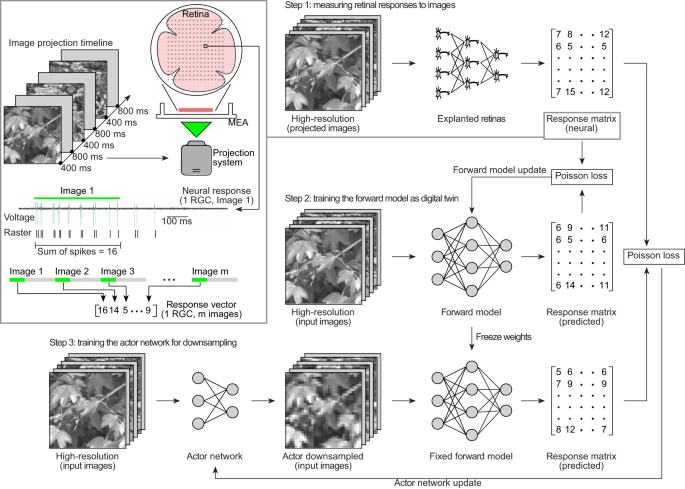
In step 1, the neuronal response of RGCs is measured by projecting high-resolution images onto explanted mouse retinas. Then, it is converted into a neural response matrix. The inset sketch shows how a response vector is built. Images are projected for 400 ms (green bars) separated by a 800-ms long gray frame (gray bars). RGCs are isolated after spike detection and clustering. A response vector is built for each RGC by summing up the number of spikes occurring during image projection (400-ms window). The image sequence was repeated 10 times to account for trial-to-trial variability, and responses to the same images were averaged (not shown in the sketch). Hence, numbers in the response vector are not necessarily integers. Response vectors are arranged into the response matrix. In step 2, the neuronal response matrix serves as ground truth for the training of the forward model. Given the same input, the predicted response of the model is compared against the ground truth by calculating the Poisson loss, then used to update the forward model. After training, the forward model is fixed. In step 3, the actor network is prepended to the fixed forward model. High-resolution images are passed into the actor network which downsample and feed them into the fixed forward model. The predicted response is compared against the ground truth by calculating the Poisson loss, then used to update the actor network. High-resolution image reproduced from the Open Access van Hateren Natural Image Dataset available at https://github.com/hunse/vanhateren (MIT License).
We determined the dimension of high-resolution image set by performing an ex-vivo experiment equivalent to the one described step 1, but with an image set containing 240 original images replicated in 4 different sizes (256 × 256, 128 × 128, 64 × 64, and 32 × 32 pixels; downsampling by pixel averaging), each projected 10 times. For each dimension, the image set was split into odd and even groups (5 repetitions each) to allow comparisons within the same image dimension. To compare between different dimensions, we always used the odd group. We recorded neural responses from identified RGCs ( n = 12 from N = 3 retinas) and built corresponding response vectors. Then, we used neuronal reliability as a quantitative measure to compare the resolutions in pairs. Briefly, the ex-vivo neuronal reliability of each RGC is measured as the R 2 value between the neuronal responses to two paired images (Fig. 2a ). Paired images are the same image presented at either the same (e.g. 256 vs 256) or different (e.g. 256 vs 128) resolutions. Pooling all the RGCs together (Fig. 2b, c ), we did not find any statistically significant difference in neuronal reliability when images of 256 × 256 pixels are downsampled to 128 x 128 pixels (two-tailed paired Wilcoxon test, p = 0.30). For all other comparisons, we found statistically significant differences (two-tailed paired Wilcoxon tests: 256−64, p = 0.0122; 256−32, p = 0.0005; 128 − 64, p = 0.0200; 128 − 32, p = 0.0005; 64 − 32, p = 0.0009).
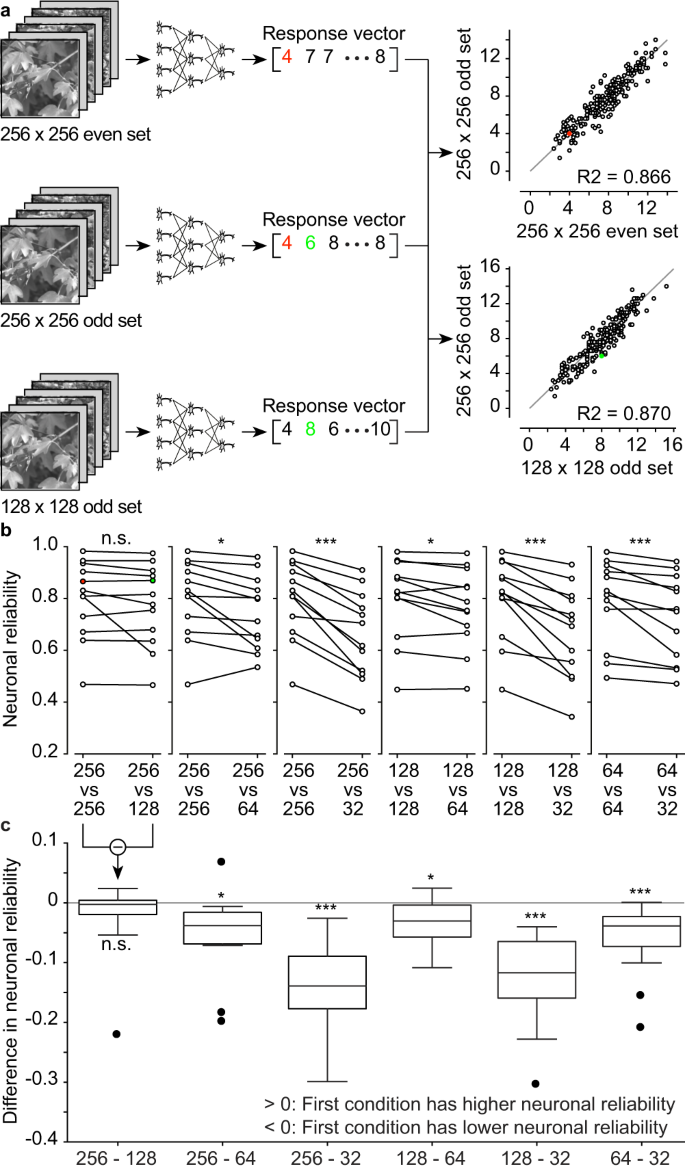
a Neuronal reliability of a RGC to the same image dimension (256 ×256 pixels) or to different image dimensions (256 x 256 pixels vs 128 ×128 pixels). The scatter plots are built from the response vectors. The red and green dots correspond to the red and green numbers in the response vectors. The gray lines are the identity lines. b Plots of the paired neuronal reliabilities for all the recorded RGCs ( n = 12 RGCs from N = 3 retinas). c , Each boxplot is the distribution of the pairwise difference in neuronal reliability from panel b . The box spans from 25 th to the 75 th percentiles, the line is the median, and the whiskers are 1.5 times the interquartile range. The black dots indicate outliers. Two-tailed paired Wilcoxon tests: 256 − 128 ( p = 0.30, reported as n.s.), 256 − 64 ( p = 0.0122, reported as *), 256 − 32 ( p = 0.0005, reported as ***), 128 − 64 ( p = 0.0200, reported as *), 128 − 32 ( p = 0.0005, reported as ***), 64 − 32 ( p = 0.0009, reported as ***). Statistical analysis applies to both panels b and c . High-resolution image reproduced from the Open Access van Hateren Natural Image Dataset available at https://github.com/hunse/vanhateren (MIT License).
On the one hand, we aimed to minimize the high-resolution image dimension so as to reduce the number of parameters of the forward model. More parameters will result in greater computational complexity and larger risk of overfitting. Since we did not find a statistically significant difference while downsampling from 256 × 256 to 128 × 128 pixels, we rejected the 256 × 256 pixel size. On the other hand, we wanted to maximize the high-resolution size so that it can give a statistically significant difference in neuronal reliability compared to the downsampled images, hence we chose 128 x 128 pixels for the high-resolution images. The choice of four-fold downsampling is also derived from this experiment. A reduction in neuronal reliability is required between high-resolution images and images downsampled by pixel averaging to fully leverage on the potential of the actor-model framework to downsample images with higher neuronal reliability. Four-fold downsampling (128 vs. 32) exhibited the greatest reduction in neuronal reliability (one-tailed paired Wilcoxon test, p = 0.0002).
Actor-downsampled images elicit correlating responses to high-resolution images in-silico
With the forward model and actor network trained, we conducted a comparison of the performance in-silico between the actor-model framework and the pixel averaging method for four-fold downsampling (Fig. 3 ). Here, the forward model functions as the digital twin of an explanted mouse retina. We used different types of images as inputs (200 unique images for each group), including high-resolution images (high-resolution), images downsampled by the actor network (actor downsampled), and images downsampled using the pixel averaging method (average downsampled). Lastly, we computed the in-silico neuronal reliability (Fig. 3a ). Briefly, the neuronal reliability of each modeled neuron ( n = 60) is measured as the R 2 between the neuronal responses of explanted retinas to high-resolution images (ground truth; step 1 in Fig. 1 ; n = 60 RGCs from N = 10 retinas) and the predicted responses of the forward model to the same high-resolution images (Fig. 3a , magenta), average downsampled images (Fig. 3a , yellow) or actor downsampled images (Fig. 3a , cyan). The comparison between predicted response of high-resolution images and ground truth defines the baseline reliability of a modeled neuron (Fig. 3a , magenta). The ground truth compared to predicted response of downsampled images (Fig. 3a , actor in cyan and average in yellow) is the in-silico neuronal reliability for downsampled images, which is how similar the responses to downsampled images are to those from high-resolution images in-silico.
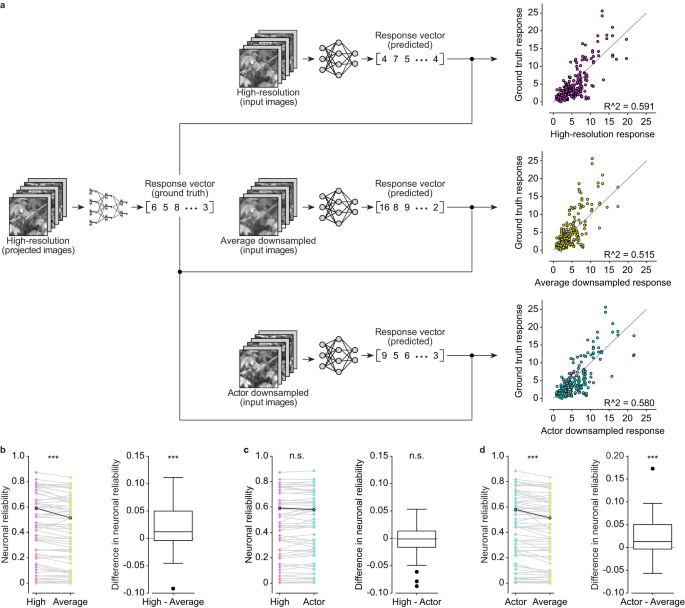
a High-resolution, actor downsampled and average downsample images are passed as input to the forward model to obtain a predicted response in-silico. On the right there are the scatter plots of the same representative neuron from which the R 2 2 value between the predicted responses and the ground truth response is computed. The gray line is the identity line. b – d The left plots are the paired distribution of neuronal reliabilities ( n = 60 modeled neurons). Highlighted points correspond to the representative neuron in a . The boxplots show the distribution of the pairwise difference in neuronal reliability. The box spans from the 25 th to the 75 th percentiles, the line is the median, and the whiskers are 1.5 times the interquartile range. The black dots indicate outliers. Two-tailed paired Wilcoxon tests: High - Average ( p = 0.0001, reported as ***), High - Actor ( p = 0.56, reported as n.s.), Actor - Average ( p = 0.0001, reported as ***). High-resolution image reproduced from the Open Access van Hateren Natural Image Dataset available at https://github.com/hunse/vanhateren (MIT License).
As expected, we observed a statistically significant reduction in neuronal reliability of the average downsampled images compared to the high-resolution images (Fig. 3b ; two-tailed paired Wilcoxon test, p = 0.0001; one-tailed paired Wilcoxon test, p < 0.0001). However, we did not observe any statistically significant difference in neuronal reliability between high-resolution images and actor downsampled images (Fig. 3c ; two-tailed paired Wilcoxon test, p = 0.56). Furthermore, the neuronal reliability of the actor downsampled images was significantly higher than the one of average downsampled images (Fig. 3d ; two-tailed paired Wilcoxon test, p = 0.0001; one-tailed paired Wilcoxon test, p < 0.0001). It appears that some neurons exhibit low in-silico neuronal reliability, this effect could be attributed to the learning of the model. The parameters for some of the neurons could be overfitted, and since we do not model each neuron separately, it is difficult to ensure that every neuron is optimally modeled. Overall, we found a 4.9% median percent increase in neuronal reliability for actor downsampled images compared to downsampled images by pixel averaging. It is worth noticing that the performance increase is not specific to the type (ON or OFF) of the modeled neuron (Supplementary Fig. 1a ; two-tailed Mann-Whitney U test, p = 0.3845) or to its RF size (Supplementary Fig. 1b ; Pearson correlation coefficient r = 0.0713, p = 0.5885).
Based on these in-silico results, the actor network has found a way that can elicit a neuronal response more similar to the ground truth compared to downsampling by pixel averaging.
Among the various learning-free approaches we choose pixel averaging as the benchmark for evaluation, since it is the conventional visual encoding approach used in prosthetic devices. Nevertheless, we investigated the performance of the actor-model framework relative to other downsampling methods common in image processing. Specifically, we evaluated bilinear interpolation (Bil), nearest neighbor interpolation (Near), lanczos kernel with radius 3 (Lan3), lanczos kernel with radius 5 (Lan5), cubic interpolation (Cub), gaussian kernel (Gau), area interpolation with anti-aliased resampling (Area), Mitchell-Netravali cubic non-interpolating filter (Mit). The actor downsampled images consistently elicited higher in-silico neuronal reliability compared to the other learning-free methods (Fig. 4 ; two-tailed paired Wilcoxon tests: p = 0.0001 for Actor - Average and Actor - Area, p < 0.0001 for other comparisons; one-tailed paired Wilcoxon tests, p < 0.0001 for all). This result further accentuates the effectiveness and the necessity of having a learning-based framework tailored for the retina.
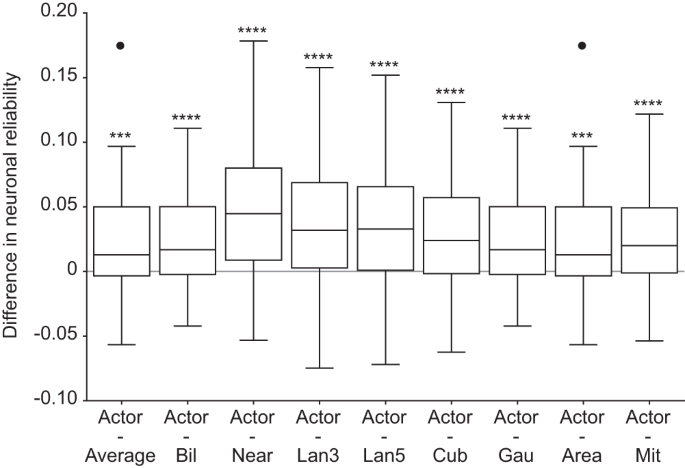
Each boxplot is the distribution of the pairwise difference in neuronal reliability between actor downsampled images and downsampled images by a learning-free method ( n = 60 modeled neurons). The first boxplot is the same reported in Fig. 3d ). The box shows the 25 th and the 75 th percentiles respectively, the line is the median, and the whiskers are 1.5 times the interquartile range. The black dots indicate outliers. Two-tailed paired Wilcoxon tests: Actor - Average and Actor - Area ( p = 0.0001, reported as ***), other comparisons ( p < 0.0001, reported as ****).
Since we did not find another downsampling method that outperforms the actor-network framework, we continued using pixel averaging as a reference method. The next logical step is to validate these in-silico findings ex-vivo in explanted retinas.
Actor-downsampled images elicit correlating responses to high-resolution images ex-vivo
Finally, we validated the actor-model framework ex-vivo. We measured the neuronal responses of mouse retinas when presented with high-resolution images, actor downsampled images, and average downsampled images ( n = 21 RGCs from N = 8 retinas; 200 unique images, 10 repeats per image). High-resolution images were presented twice: first to determine a new ground truth, and then to compute neuronal reliability of high-resolution images. We evaluated the performance of the actor-model framework by calculating its ex-vivo neuronal reliability when compared to the new ground truth (Fig. 5a ). Qualitatively, results ex-vivo match in-silico data except for a statistically significant reduction in neuronal reliability between high-resolution images and actor downsampled images (Fig. 5c ; two-tailed paired Wilcoxon test, p < 0.0001; one-tailed paired Wilcoxon test, p < 0.0001). This result was expected as information loss would occur during downsampling. The neuronal reliability of average downsampled images is still significantly lower than the one of high-resolution images (Fig. 5b ; two-tailed paired Wilcoxon test, p < 0.0001; one-tailed paired Wilcoxon test, p < 0.0001). Importantly, the neuronal reliability of actor downsampled images is significantly higher than the neuronal reliability of average downsampled images (Fig. 5d ; two-tailed paired Wilcoxon test, p = 0.0012; one-tailed paired Wilcoxon test, p = 0.0006). The actor downsampling method performs 2.9% better than the average downsampling method (median percentage increase). Similar to in-silico results, the performance increase is not specific to types (ON or OFF) of RGC (Supplementary Fig. 1c ; two-tailed Mann-Whitney U test, p = 0.4003) or to its RF (Supplementary Fig. 1d ; Pearson correlation coefficient r = −0.0838, p = 0.7181).
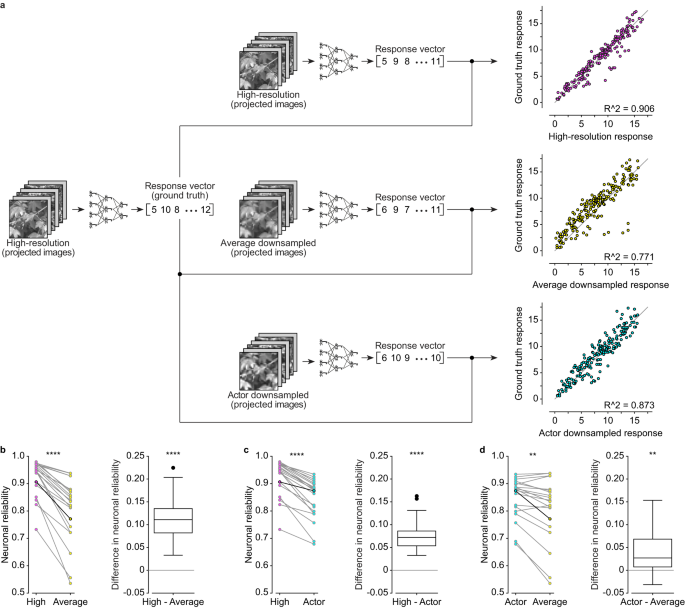
a High-resolution, actor downsampled and average downsample images are projected onto retinas explanted over a MEA. On the right there are the scatter plots of the same RGC from which the R 2 value between the obtained responses and the ground truth response is computed. The gray line is the identity line. b – d The left plots are the paired distribution of neuronal reliabilities for all the RGCs ( n = 21 RGCs from N = 8 retinas). Highlighted points correspond to the RGC in a . The boxplots show the distribution of the pairwise difference in neuronal reliability. The box spans from the 25 th to the 75 th percentiles, the line is the median, and the whiskers are 1.5 times the interquartile range. The black dots indicate outliers. Two-tailed paired Wilcoxon tests: High vs Average ( p < 0.0001, reported as ****), High vs Actor ( p < 0.0001, reported as ****), Actor vs Average ( p = 0.0012, reported as **). High-resolution image reproduced from the Open Access van Hateren Natural Image Dataset available at https://github.com/hunse/vanhateren (MIT License).
It is worth reporting that we found a statistically significant difference in the average response of RGCs to high-resolution, actor downsampled and average downsampled images (Supplementary Fig. 2 ; Friedman test, p < 0.0001). In particular, the mean response to average downsampled images is significantly lower than the average response to both high-resolution and actor downsampled images (Nemenyi post-hoc test, p = 0.001 for both comparisons). On the contrary, the mean response of RGCs to actor downsampled images was not significantly different from the response to high-resolution images (Nemenyi post-hoc test, p = 0.5398). This result confirms that actor downsampled images elicited neural responses more similar to high-resolution images.
Actor network develops features for optimal downsampling
Then, we delved deeper into the pertinent attributes of effective downsampling by comparing high-resolution images to their respective downsampled version.
Upon visual examination, it is evident that actor downsampled images better resemble the original images (Fig. 6a ). Furthermore, the quantification of the local contrast difference shows that actor downsampled images exhibit a significantly higher local contrast compared to average downsampled images (Fig. 6b ; two-tailed paired Wilcoxon test, p < 0.0001; one-tailed paired Wilcoxon test, p < 0.0001). This result implies that the actor network strives to increase the image contrast, highlighting its significance in generating neuronal responses akin to those of high-resolution images.
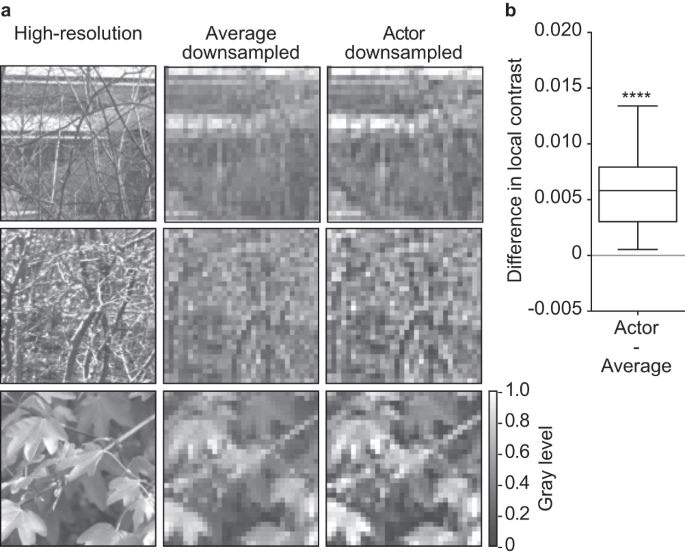
a On the left, there are three high-resolution images. The same images after four-fold average downsampling (middle) and four-fold actor downsampling (right). b Distribution of the pairwise difference in local contrast between actor and average downsampled images ( n = 200 unique images; Two-tailed paired Wilcoxon test, p < 0.0001, reported as ****). The box spans from the 25 th to the 75 th percentiles, the line is the median, and the whiskers are 1.5 times the interquartile range. High-resolution images reproduced from the Open Access van Hateren Natural Image Dataset available at https://github.com/hunse/vanhateren (MIT License).
The next step is understanding how contrast affects neuronal reliability. First, we looked at the difference in local contrast of downsampled images by the other learning-free approaches (Fig. 7a ; two-tailed paired Wilcoxon tests: p = 0.8433 for Actor – Mit, p < 0.0001 for all other comparisons). Most learning-free downsampling approaches preserve the local contrast better than pixel averaging, and some even better than the actor network (e.g. Near, Lan3, Lan5 and Cub; median difference in local contrast <0). This result is counterintuitive since the neuronal reliability of these methods was not higher than the actor network (Fig. 4 ) .
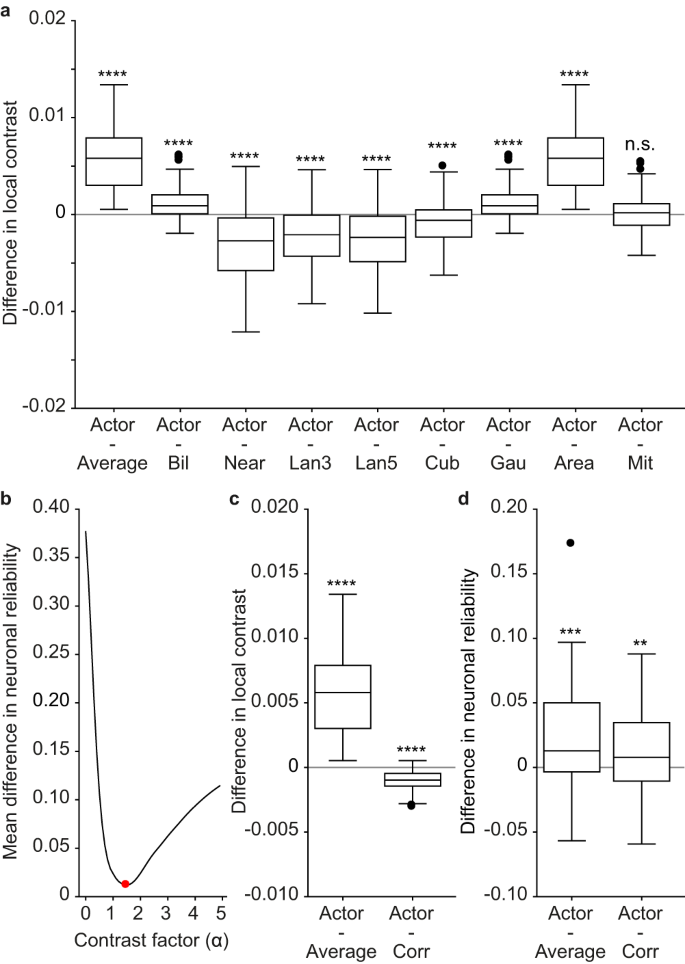
a Each boxplot is the distribution of the pairwise difference in local contrast between actor downsampled images and downsampled images by a learning-free method ( n = 200 unique images). Two-tailed paired Wilcoxon tests: Actor - Mit ( p = 0.8433, reported as n.s.), other comparisons ( p < 0.0001, reported as ****). The first boxplot is the difference between actor downsampled and downsampled images by pixel averaging (as in Fig. 6b ). b Mean difference in neuronal reliability between actor downsampled images and downsampled images by pixel averaging with corrected contrast as a function of the contrast factor α (average of n = 60 modeled neurons). The red circle indicates the minimum of the curve for α = 1.5. c Distribution of the pairwise differences in local contrast ( n = 200 unique images) between actor downsampled images and images downsampled by pixel averaging (left, as in Fig. 6b ) and images downsampled by pixel averaging with optimized contrast at α = 1.5 (right). Two-tailed paired Wilcoxon tests: Actor - Average ( p < 0.0001, reported as ****), Actor - Corr ( p < 0.0001, reported as ****). d Distribution of the pairwise d ifferences in neuronal reliability ( n = 60 modeled neurons) between actor downsampled images and images downsampled by pixel averaging (left, as in Fig. 4 ) and images downsampled by pixel averaging with optimized contrast at α = 1.5 (right). Two-tailed paired Wilcoxon tests: Actor - Average ( p = 0.0001, reported as ***), Actor - Corr ( p = 0.0082, reported as **). The boxes span from the 25 th to the 75 th percentiles, the line is the median, and the whiskers are 1.5 times the interquartile range.
To further investigate contrast, we modified the pixel averaging approach by either reducing or increasing the contrast of downsampled images before feeding them to the forward model. The gap in neuronal reliability between actor downsampled and average downsampled images widened when the contrast of average downsampled images is artificially reduced (Fig. 7b , contrast factor α < 1). Conversely, when the contrast of average downsampled images is artificially increased (α > 1), the gap in neuronal reliability decreases and minimizes for α = 1.5. Moreover, when the contrast was increased further (α > 1.5), the gap widened again. When the gap in neuronal reliability is minimized, the local contrast of the corrected average downsampled images is closer to the actor downsampled images although the difference is still statistically significant (Fig. 7c ; two-tailed paired Wilcoxon test, p < 0.0001). This result indicates that there is an optimal level of contrast preservation which is reached by the actor network. Hence, other learning-free models fail to perform even though contrast was better preserved. Moreover, although increasing the contrast of the average downsampled images resulted in higher neuronal reliability, the actor network still performed better (Fig. 7d ; two-tailed paired Wilcoxon test, p = 0.0082; one-tailed paired Wilcoxon test, p = 0.0041).
Hence, we hypothesize that contrast might not be the only factor learned by the actor network. Indeed, the six kernels learned by the actor network closely resemble a Mexican hat shape (Laplacian of a Gaussian) which is a common function in algorithms for edge detection 40 (Fig. 8 ). This function, or its fast approximation difference of Gaussians, is also traditionally used to model the center-surround organization of the RGC RF 31 . This result is unexpected since the actor network was not specifically trained to mimic center-surround properties, yet it emerges when optimized based on neuronal responses. The actor-model framework could integrate edge detection into the downsampling process by convoluting with a Mexican hat function. Hence, we hypothesize that this component also contributed to a more effective downsampling of the images in conjunction with optimizing the contrasts, leading to higher neuronal reliability.
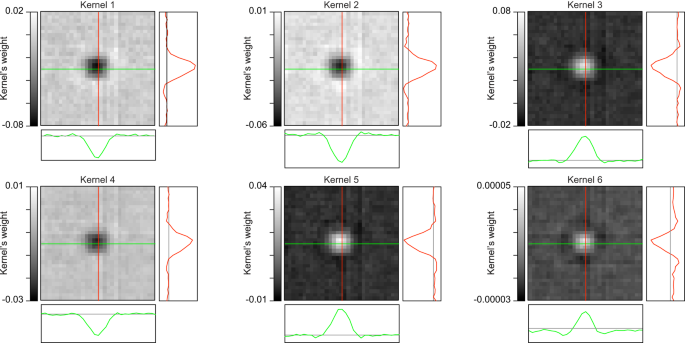
Visualization of the six kernels learned by the actor network. For each plot, the green profile corresponds to the line plot of the kernel across the horizontal midline (in green). The red profile corresponds to the line plot of the kernel across the vertical midline (in red). The kernels are categorized into two distinct groups: those with predominantly positive weights and those with predominantly negative weights, resembling center ON and center OFF properties of RGCs.
In-silico prediction of x-fold downsampling
In the previous sections, we explored and validated the effectiveness of the actor-model framework during four-fold downsampling, where images were reduced from 128 x 128 pixels to 32 x 32 pixels. The actor-model framework elicited a higher neuronal reliability (4.9% in-silico and 2.9% ex-vivo) compared to a learning-free approach (pixel averaging). Therefore, we decided to vary the folds of downsampling in-silico to understand the extent of aggregation before the information loss cannot be recovered by the framework (Fig. 9 ). We trained multiple actor networks as described in the preceding sections (960 unique images) to downsample the high-resolution images, each learning a different downsampling fold: 2-fold (64 x 64 pixels), 4-fold (32 x 32 pixels), 8-fold (16 x 16 pixels), 16-fold (8 x 8 pixels), and 32-fold (4 x 4 pixels). We observe statistically significant differences in neuronal reliability between actor downsampled images and average downsampled images up to 8-fold downsampling ( n = 60; two-tailed paired Wilcoxon tests: 2-fold, p = 0.0178; 4-fold, p = 0.0004; 8-fold, p = 0.0006). However, from 16-fold onwards, this difference was not observed ( n = 60; two-tailed paired Wilcoxon tests: 16-fold, p = 0.8024; 32-fold, p = 0.4483;). This was expected as 16-fold downsampling corresponds to reducing the original image from 128 x 128 pixels to 8 x 8 pixels. Therefore, each pixel has a size of 400 x 400 µm 2 , exceeding the RF size of most RGC in the mouse retina 41 (Supplementary Fig. 1 ). Hence, the amount of information loss during downsampling might be too much to be recovered.
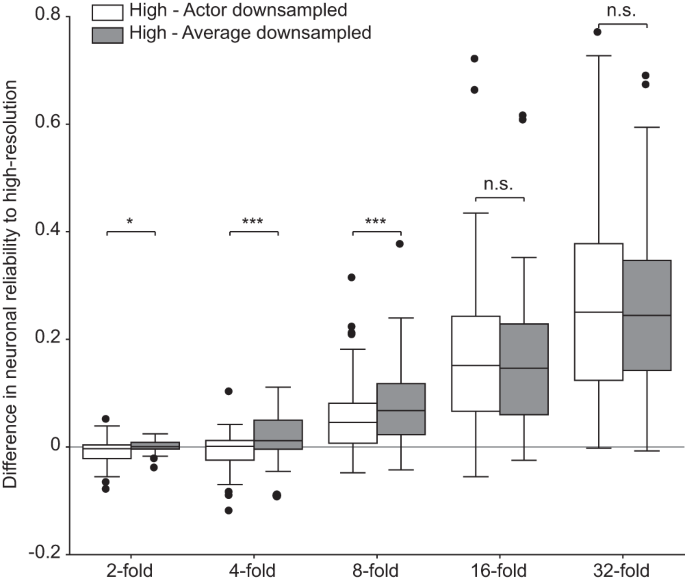
Each boxplot is the distribution of the pairwise difference in in-silico neuronal reliability between the downsampled images (actor in white and average in gray) and high-resolution images for different downsampling folds ( n = 60 modeled neurons). The box spans from the 25th to the 75th percentiles, the line is the median, and the whiskers are 1.5 times the interquartile range. The black dots indicate outliers. Two-tailed paired Wilcoxon tests: 2-fold ( p = 0.0178, reported as *), 4-fold ( p = 0.0004, reported as ***), 8-fold ( p = 0.0006, reported as ***), 16-fold ( p = 0.8024, reported as n.s.), 32-fold ( p = 0.4483, reported as n.s.).
Although we observed a significant difference between the neuronal reliability of actor downsampled images and average downsampled images for 2-fold downsampling, a significant difference was not observed between neuronal reliability of average downsampled images to high-resolution images (two-tailed paired Wilcoxon test, p = 0.1925), while actor downsampled images has slightly different neuronal reliability than high-resolution images (two-tailed paired Wilcoxon test, p = 0.0277; one-tailed paired Wilcoxon test, p = 0.0139). This result suggests that the forward model is robust to downsampling. This is not out of expectation as we used a CNN to model the retina, which could possess a certain level of robustness to downsampling.
In this study, we have illustrated the efficacy of an actor-model framework to learn a downsampling method that outperforms common learning-free techniques. Furthermore, we substantiated the effectiveness of our approach by analyzing the neuronal responses of RGCs, and we identified contrast as a crucial feature for effective downsampling. Additionally, we observed the emergence of distinct patterns in the learned kernels of the actor network, mimicking the center-surround properties of RGCs. In this section, we assess our approach against the state-of-the-art in the field, we delve into the implications of our findings, and propose potential avenues for advancing image encoding research.
Previously, the actor-model framework was employed within a physical system, where it successfully learned the necessary input to yield any desired output when transmitted through a multimode fiber. The performance achieved in that study was comparable to gold-standard methods 37 . Here, we sought to harness the potential of the actor-model framework within a biological system, which is often characterized by complexity and high variability. In contrast to a physical system, we encountered a distinct set of challenges during the experimentation process. Firstly, like in many biological ex-vivo experiments, the difficulty lay in the sample viability, which constrained the duration of data collection. Given that neural networks typically require vast amounts of data to be effective, this limitation poses a significant obstacle to training the neural network efficiently. Secondly, the number of neurons recorded could fluctuate depending on the quality of the dissected tissue and other factors. Although we used 256 electrodes, not every electrode could successfully capture the electrical activity of RGCs. These issues imposed conducting experiments on multiple retinas and consolidating the recorded RGCs into a single dataset. As such, the framework must learn and generalize across different mice to capture inter-sample variability, which could add another layer of difficulty. Lastly, intrinsic variability within the retina presented another hurdle. The response could vary from trial to trial when the same image was projected twice, with this variability being more pronounced than in the physical systems used in the previous work. Consequently, our actor-model framework required greater robustness to be effective. Nevertheless, the actor-model framework successfully learned efficient downsampling. This achievement underscores the robustness of the actor-model framework and its capacity to discover solutions irrespective of whether it is applied to a physical or biological system. We contend that the potential of the actor-model framework is not limited to image encoding in the retina, and we look forward to future experiments that could capitalize on this versatile framework. For example, in the context of tactile feedback, Eldeeb and Akcakaya demonstrated the potential of using electroencephalography (EEG) to guide the electrical stimulation parameters 42 . The actor-model framework could also be applied in this situation, where the actor network could determine the electrical stimulation required to elicit the desired EEG response.
In the actor-model framework, artificial image encoding depends on two components: the forward model (digital twin of the retina) and the actor network (artificial image encoding). Much research has been previously dedicated to deriving a forward model of the retina 32 , 35 , 36 , 43 , 44 , 45 , 46 , 47 . Selecting a suitable forward model plays a crucial role in this study. Conventional models such as linear-nonlinear model and generalized model have been shown to model the retina. However, such conventional models generally fail to represent retinal responses to natural scenes 35 . On the other hand, CNNs have been shown in recent years to be significantly better in modeling the retina for both white noise and natural scenes 35 , 36 , suggesting a level of complexity hidden within its seemingly simple structure. Hence, we constructed a digital twin of the retina using a CNN forward model which accounted for the inherent computation of the retinal network. In this study, the innovation lies in the combination of a forward model with a CNN encoder (actor network), while the architecture of the forward model is derived from the state-of-the-art 35 . Since the actor network optimized the image encoding process based on the output of the forward model, the positive results observed in this study also indicate the robustness of the forward model per se. Hence, our results highlight the suitability of CNNs as a forward model of the retina. However, as the field progresses and more forward models are derived, it will be possible to substitute the forward model used in this study with a more accurate one 48 .
As visual technology progresses and electrode resolution increases, image encoding methods become increasingly crucial. This study demonstrates that a learning-based approach, which accounts for the biological retinal process, yields superior results. We foresee this learning-based method serving as a foundation for future research, potentially leading to the identification of the most effective image encoding technique. This study shows the benefits of using CNNs for image encoding (actor network) which, to the best of our knowledge, their use for efficient image encoding has not been reported despite their great potential. CNNs are efficient in capturing the relevant features of the image. This model highlighted the importance of contrast during downsampling, confirming also its importance for a CNN forward model of the retina 35 . In general, employing a learning-based approach enables further analysis of the learned features, providing insights into the underlying dynamics of the system. Also, the weight-sharing properties of CNNs allow for generalization within each retina and across different retinas. Weight-sharing property in CNNs refers to how each learned kernel is applied across the entire image, thus reducing the number of parameters to be learned. A significant challenge in biological experiments involves conducting multiple trials with different samples. RGCs are recorded from multiple sessions and combined into a single dataset. However, even with such methods, it is not guaranteed that every area of the image is captured by the neuronal activity. Nevertheless, since weights are shared within a CNN, the actor network can extract pertinent features based solely on the subset of RGCs measured and subsequently apply the learned kernels through the whole visual space. These distilled features can then generalize to other retinas and to other areas of the images not captured by neurons, as demonstrated by the results obtained in the ex-vivo experiments for validation. Although we could not guarantee that the RGCs recorded in the ex-vivo validation experiment (Fig. 5 ) would co-locate with the RGCs used for the forward model and actor network training (Fig. 1 ), the actor network generalization capability highlights the robustness and invariance towards the location of the RGCs recorded. Additionally, we show that the actor network produces images that are neither biased towards the type of RGCs nor to their RF size (Supplementary Fig. 1 ).
Several visual encoding approaches have been proposed so far. In computer vision, a conventional approach is based on saliency detection (e.g. edge detection, object detection or content-aware retargeting). These approaches generally aim at reducing the complexity of the image, highlighting interesting content and features while removing less interesting information 27 , 28 , 49 , 50 . The actor-model framework is compatible with saliency-based detection. First, one would apply saliency detection algorithms to extract important information, and then pass the processed images through the actor-model framework for more effective downsampling. In visual prostheses, image encoding can consist of two phases: image processing (e.g. downsampling) followed by stimulus optimization. Most of the image encoding strategies focus on the stimulus optimization rather than on the image processing phase, which is typically based on pixel averaging when present. A ‘naive’ approach for stimulus optimization sets the stimulation strength of each electrode based on the corresponding pixel value of the downsampled images 51 .
At the biophysical level, Ghaffari et al. utilized a feedforward neural network to optimize the stimulation parameters to achieve a more localized RGC activation but validated it only in-silico 52 . Similarly, Spencer et al. tried to approximate and invert a biophysical forward model that converts the stimulation strength to retinal activation 53 . Both studies aim to produce a more focused retinal activation and thus could potentially be integrated with the actor-model framework for the next phase which involves the conversion of the more effective actor downsampled images to stimulation parameters.
At the perceptual level, Fauvel et al. presented a preferential Bayesian optimization algorithm to optimize a visual encoder based on patients’ feedback 54 . However, the encoder was not proposed, and the optimization was based on a prosthetic vision simulator which generates patterns of phosphenes (phosphene model) mimicking the visual experience of patients implanted with the Argus II® device 29 . Shah et al. proposed a greedy iteration to select electrical stimuli that minimizes the error between the expected visual perception and the target image 55 . The model for the expected perception was obtained by projecting continuous white noise and measuring the RGC responses. Then, a linear filter is fitted for each RGC to find the reconstruction matrix. They perform a closed loop optimization of the electrical stimuli. First, they provided electrical stimulation and measured the RGC response within a temporal window. Then, using the reconstruction matrix, they predicted the perception and used it as the loss function for the optimization. One of the limitations of this approach is the reliance on the expected perception. Since the expected perception is obtained by the reconstruction matrix, it is unclear how it could be derived for a blind patient. In our study, we faced a similar issue since both the actor and the model networks are optimized to a set of RGCs. However, we showed in the validation experiments that the actor-model framework can generalize across retinas.
A few other studies have tried to optimize stimulation parameters using neural networks 56 , 57 , 58 . Van Steveninck et al. proposed an end-to-end optimization strategy in conjunction with a fixed phosphene model 56 . They suggested mapping the image to stimulation parameters using a CNN (encoder). However, they also included a secondary CNN (decoder) to post-process the output of the phosphene model, reconstructing the image. Throughout this process, both the encoder and the decoder are trained concurrently by minimizing the loss function between the reconstructed and the original image. Thus, it is unclear if the encoder had genuinely learned the optimal stimulation parameters, or the decoder is simply adept at reconstructing the image. Relic et al. addressed this issue by using a neural network surrogate of the phosphene model and removing the secondary decoder 57 . In this case, since the phosphene model is non-differentiable, a surrogate model which consists of a neural network is needed as replacement so that the loss value could propagate backwards to train the encoder model to produce the optimal stimulation parameters. Granley et al. refined this pipeline by deriving a differentiable phosphene model, removing the need of a surrogate model 58 .
These studies methodologically relate to our study since CNNs were used. However, one key difference lies in the scope. Our actor-model framework optimizes the image encoding process by learning the most efficient downsampling pipeline while their method optimizes the electrical stimulation parameters directly. As a result, the actor-model framework could easily translate clinically as it improves on the conventional method which involves downsampling the images, and the features pertinent to effective downsampling found in this study can generalize well to different patients. On the other hand, the studies of Relic et al. and Granley et al. were performed in-silico only and the phosphene model used to predict the perceived perception had to be fitted to each individual patient. In both studies, the network architecture can be decomposed into encoder and decoder, where the encoder learns to generate the optimal electrical stimulation parameters while the decoder is either a differentiable or surrogate phosphene model aiming at predicting the perceived phosphenes. This is analogous to our actor network which learns to optimally downsample the images and our model network which acts as the digital twin of the retina. While these studies bear resemblances to our work, a shared theme across them is the application of phosphene models for in-silico performance measures without validation. On the contrary, the actor-model framework showed better performance in both in-silico and ex-vivo environments using neuronal reliability as performance index.
As a step toward a learning-based approach for image encoding, there remains ample room for improvement and exploration. In this study, we projected static images and summed the number of spikes within a specified window. Constrained by the hardware employed, we were unable to present images in a continuous format (i.e., movie format), which prevented us from verifying whether our proposed methods would be applicable to more dynamic natural scenes. A logical next step would be to validate these results using continuous projections of natural scenes. In addition to exploring continuous format, the same approach could be evaluated on reducing the bit size of the depth of the images concurrently while downsampling. Reducing from 256 levels of grayscale to 8 levels would also be useful for visual prostheses to better calibrate the strength of electrical stimulation.
Another avenue is investigating the generalization of our approach across species. The actor-model framework was trained using data collected from mouse retinas. We also discovered that the results derived from these trained models could be generalized across different mouse retinas, as the validation experiments were conducted with new retinas. However, mouse retinas have anatomical and functional differences from human retinas. It would be interesting to explore whether projecting the various downsampled images onto retinas of other species would yield similar improvements (e.g. non-human primates). If successful, this would imply that the features learned by the actor network may possess the capacity to generalize even across different species, highlighting the potential for broader applicability of this method, in particular towards visual prostheses in humans.
In conclusion, this study presents a neural network-based approach for optimizing image distillation in the context of visual prostheses. The proposed actor-model framework learns to downsample images while accounting for the biological processes of the retina, resulting in more effective downsampling patterns. This research not only contributes to the advancement of image encoding techniques for visual prostheses but also highlights the importance of incorporating natural biological transformations. In general, integrating neural networks into sensory encoding could hold the key to better perception of the visual prosthesis users. Future research could build upon this learning-based approach to develop even more accurate and effective image encoding methods, enhancing the quality of life for individuals relying on such devices.
Electrophysiological recordings
Animal experiments were authorized by the Direction Générale de la Santé de la République et Canton de Genève in Switzerland (authorization number GE31/20). C57BL/6J mice ( n = 21; age 75.3 ± 26.4 days, mean ± SD) were dark-adapted for 1 hr prior to euthanasia. Euthanasia was carried out via intraperitoneal injection of sodium pentobarbital (150 mg kg −1 ). Dissection and recording of retinas were performed in carboxygenated (95% O 2 and 5% CO 2 ) Ames medium (USBiological, A1372-25) under dim red light. Retinas were maintained at 25 °C throughout the experiment. Explanted retinas were positioned on a poly-lysine coated membrane (Sigma, P8920; Repligen 132544), with the RGCs side facing a 256-channel MEA (256MEA200/30iR-ITO, Multichannel systems) with 30-µm electrodes spaced 200 µm. The data sampling rate was set at 25 kHz. Data were collected using a custom python code.
Visual stimulation
Images were projected using a custom-built setup with a Digital Mirror Device (V-7000 Hi‑Speed V‑Modules, ViALUX) coupled to a white LED (MWWHF2, Thorlabs). The stimulus was focused on the photoreceptors via standard optics, with an average power of 13 nW. The projected area covered 3.2 x 3.2 mm 2 . The image set is the Open Access van Hateren Natural Image Dataset (available at https://github.com/hunse/vanhateren , MIT License), comprising 4212 monochromatic and calibrated images captured in a variety of natural environments 59 , further processed to maintain a linear relationship between scene luminance and pixel values 35 . This processing step was described as crucial to prevent the retinal system from having to adapt to varying light intensity levels found in different environments 35 . The final image set used in this experiment (3190 images) was obtained from Goldin et al. 35 , which was then sub-sampled for the different experiments conducted. Each image is projected for 400 ms, and an 800-ms long gray frame is inserted between images to return the firing rate to baseline value.
Spike detection and sorting
The SpyKING CIRCUS algorithm (version 1.1.0) was used for spike sorting 60 . Manual inspection was performed using Phy software (version 2.0b5) 61 , including verification of gaussian distribution in the amplitudes, waveforms present in multiple channels, presence of a dip in autocorrelogram, and merging/separating clusters as necessary. To assess the reliability of the recorded neurons and account for experimental drift, random binary checkerboard stimuli was presented at the start of the experiment, and then redisplayed roughly every half an hour 43 . The check size was 50 µm, the refresh rate was 33 Hz and the presentation time was 5 min. The correlation coefficient of a cell’s average response to the same stimulus across different blocks of trials was calculated. Only neurons with a correlation exceeding 0.3 were selected for further analysis 36 . Consequently, many neurons that were not responsive over the entire experimental period (approximately 5 hrs) were discarded. To filter out poor-quality clusters, we presented a random binary checkerboard at the end of the experiment for 1 h to characterize the RF size of each spike-sorted RGC by spike-triggered averaging (STA) analysis. STA is given by \({STA}=\frac{1}{{n}_{{sp}}}{\sum }_{i=1}^{T}{y}_{i}{{{{{{\bf{X}}}}}}}_{i}\) where \({n}_{{sp}}={\sum }_{i=1}^{T}{y}_{i}\) is the total number of spikes, \({y}_{i}\) is the spike count in the bin and \({{{{{{\bf{X}}}}}}}_{i}\) is the binary checkerboard at i time bin. Clusters not displaying a recognizable RF were excluded from further analysis.
Image analysis
Image analysis was performed in Python (version 3.10.6) with the OpenCV library (version 4.6.0.66). Local contrast of every downsampled image was quantified by averaging the luminance variance of a sliding window of size 7 pixels x 7 pixels. The RF size of RGCs was measured after STA analysis. The background noise was smoothed by convoluting a median filter and the contrast was enhanced to better separate the RF from the background. Last, a 2D Gaussian was fitted to measure the RF area. The average model with enhanced/reduced contrast (corrected) was obtained by the TensorFlow method accordion to \({X}_{{corr}}=\left[{{{{{{\bf{X}}}}}}}_{{{{{{\rm{down}}}}}}}-{\bar{{{{{{\bf{X}}}}}}}}_{{{{{{\rm{down}}}}}}}\right]*\alpha+{\bar{{{{{{\bf{X}}}}}}}}_{{{{{{\rm{down}}}}}}}\) where \({{{{{{\bf{X}}}}}}}_{{{{{{\rm{down}}}}}}}\) are the downsampled images, \({\bar{{{{{{\bf{X}}}}}}}}_{{{{{{\rm{down}}}}}}}\) is the average downsampled image and α is the contrast factor.
Model architecture
The forward model architecture adheres to the current state of the art 35 , 62 , 63 . It consists of two layers, the first layer of the CNN, \({{{{{{\boldsymbol{k}}}}}}}_{{rsk}}\) , convolutes the input image. The output of the first convolution layer passes through a pointwise nonlinear function, \({f}_{{{{{{{\rm{\theta }}}}}}}_{k}[1]}\) , to obtain non-negative activation values. For each neuron n, a readout weight \({{{{{{\boldsymbol{w}}}}}}}_{{ijkn}}\) , which factorizes as \({{{{{{\boldsymbol{w}}}}}}}_{{ijkn}}={{{{{{\boldsymbol{u}}}}}}}_{{ijn}}{{{{{{\boldsymbol{v}}}}}}}_{{kn}}\) , is applied. Here, i and j index space, with \({{{{{{\boldsymbol{u}}}}}}}_{{ijn}}\) representing spatial weights and \({{{{{{\boldsymbol{v}}}}}}}_{{kn}}\) denoting feature weights. Another nonlinear activation function, \({f}_{{{{{{{\rm{\theta }}}}}}}_{k}[2]}\) , is performed, followed by the utilization of a Poisson noise model during training. Softplus was chosen as the activation function for \({f}_{{{{{{{\rm{\theta }}}}}}}_{k}[1]}\) and \({f}_{{{{{{{\rm{\theta }}}}}}}_{k}[2]}\) . Essentially, the k th neuron of the first layer is represented as \({A}_{k}={f}_{{{{{{{\rm{\theta }}}}}}}_{k}[1]}({{{{{{\bf{K}}}}}}}_{k}*{{{{{\bf{X}}}}}})\) . The spiking rate \({r}_{n}\) of the n th neuron given an input image X was \({r}_{n}({{{{{\bf{X}}}}}})={f}_{{{{{{{\rm{\theta }}}}}}}_{n}[2]}(\mathop{\sum}\limits_{k}\mathop{\sum}\limits_{{ij}}{u}_{{ijn}}{v}_{{kn}}{A}_{{ijk}})\) . Additionally, batch normalization was applied to the outputs of the first layer. Laplacian regularization was applied to the convolutional kernels of the first layer. For the feature and spatial weights of the second layer, we used L1 regularization such that: \({L}_{\varDelta }=\frac{\mathop{\sum}\limits_{k}\mathop{\sum}\limits_{{rs}}({{{{{{\bf{K}}}}}}}_{{rsk}}*\varDelta ){{2}}_{{rs}}}{\varepsilon+\mathop{\sum}\limits_{{rsk}}{({{{{{{\bf{K}}}}}}}_{{rsk}})}^{2}}\) and \({L}_{l1}={\lambda }_{{sp}}\mathop{\sum}\limits_{{ijn}}|{u}_{{ijn}}|+{\lambda }_{f}\mathop{\sum}\limits_{{kn}}|{v}_{{kn}}|\) with \(\varepsilon=1{0}^{-8}\) . The actor network consists of a convolution kernel that was prepended to the forward model (Supplementary Fig. 3 ). Poisson loss was used for the optimization of the actor network.
Forward model and actor network training
Given m image-response pairs \(({{{{{{\bf{X}}}}}}}_{1},\, {{{{{{\bf{y}}}}}}}_{1}),...,({{{{{{\bf{X}}}}}}}_{m},\, {{{{{{\bf{y}}}}}}}_{m})\) , the loss function of the forward model is provided by \(L=\left(\frac{1}{m}{\sum }_{k=1}^{m}r({{{{{{\bf{X}}}}}}}_{k})-{y}_{k}{log} \,{r}({{{{{{\bf{X}}}}}}}_{k})\right)+{L}_{l1}{\lambda }_{l1}+{\lambda }_{\varDelta }{L}_{\varDelta }\) . The first term corresponds to the Poisson loss, while the second and third terms represent the regularization terms. The model was fitted with the Adam optimizer on the training set (1200 images, 0.8:0.1:0.1 train:validation:test split). The responses were obtained by summing up the number of events elicited within a 400-ms window from the image onset. The training of the actor network was similar except we only used L2 regularization instead of the second and third term used in the training of the forward model. We maintained a constant batch size of 32 during training for both the actor network and the forward model. For the learning rate, we started with 0.001 for forward model and 0.002 for actor network. To avoid overfitting, we employed both early stopping and the decay mechanism with maximum 1000 epochs. The hyperparameters for the regularization term were optimized by performing a random search for the forward model, while a grid search was employed for the actor network. The optimal hyperparameter values were the ones whose model produced the lowest loss value without regularization terms on the validation dataset. The hyperparameters for the forward model were 0.0033 for smoothing factor of convolution kernels, 0.00278 for spatial sparsity factor and 1.34 −6 for feature sparsity factor. For the actor network, the best run had 6 convolution kernels of size 31 x 31 with 0.1 L2 regularization.
Neuronal reliability
As a quantitative measurement of performance for both in-silico and ex-vivo experiments, we calculated the R 2 value. For each neuron, we calculated the R 2 value for each input image and found the average across the images and neurons, such that: \(R=\frac{\sum \left({{{{{{\bf{x}}}}}}}_{i}-\bar{{{{{{\bf{x}}}}}}}\right)\left({{{{{{\bf{y}}}}}}}_{i}-\bar{{{{{{\bf{y}}}}}}}\right)}{\sqrt{\sum {\left({{{{{{\bf{x}}}}}}}_{i}-\bar{{{{{{\bf{x}}}}}}}\right)}^{2}{\left({{{{{{\bf{y}}}}}}}_{i}-\bar{{{{{{\bf{y}}}}}}}\right)}^{2}}}\) . Where x and y can be the predicted response or actual neuronal response to different sets of images.
Statistical analysis
Statistical analyses were conducted with the Python Scipy library (python version 3.10.6, scipy version 1.9.1). The Shapiro-Wilk normality test was performed to justify the use of non-parametric tests.
Reporting summary
Further information on research design is available in the Nature Portfolio Reporting Summary linked to this article.
Data availability
The authors declare that the data supporting the findings of this study are available in the paper. The dataset to replicate the study is available at https://doi.org/10.5281/zenodo.1051530 . The image set used in the study was obtained by Dr. Olivier Marre ( https://doi.org/10.1038/s41467-022-33242-8 ). The original image set is the Open Access van Hateren Natural Image Dataset available at https://github.com/hunse/vanhateren (MIT license). Any additional requests for information can be directed to, and will be fulfilled by, the corresponding author. Source data are provided with this paper.
Code availability
The code used in this study is available at https://doi.org/10.5281/zenodo.10519578 .
Wang, B.-Y. et al. Electronic photoreceptors enable prosthetic visual acuity matching the natural resolution in rats. Nat. Commun. 13 , 6627 (2022).
Article ADS PubMed PubMed Central CAS Google Scholar
Borda, E. & Ghezzi, D. Advances in visual prostheses: engineering and biological challenges. Prog Biomed. Eng. 4 , 032003 (2022).
Article Google Scholar
Wilson, B. S. & Dorman, M. F. Cochlear implants: A remarkable past and a brilliant future. Hear. Res. 242 , 3–21 (2008).
Article PubMed PubMed Central Google Scholar
Wilson, B. S. et al. Better speech recognition with cochlear implants. Nature 352 , 236–238 (1991).
Article ADS PubMed CAS Google Scholar
Zeng, F.-G., Rebscher, S., Harrison, W., Sun, X. & Feng, H. Cochlear implants: system design, integration, and evaluation. IEEE Rev. Biomed. Eng. 1 , 115–142 (2008).
Valle, G. et al. Biomimetic intraneural sensory feedback enhances sensation naturalness, tactile sensitivity, and manual dexterity in a bidirectional prosthesis. Neuron 100 , 37–45.e7 (2018).
Article PubMed CAS Google Scholar
Bensmaia, S. J., Tyler, D. J. & Micera, S. Restoration of sensory information via bionic hands. Nat. Biomed. Eng. 7 , 443–455 (2023).
Article PubMed Google Scholar
Losanno, E., Mender, M., Chestek, C., Shokur, S. & Micera, S. Neurotechnologies to restore hand functions. Nat. Rev. Bioeng. 1 , 390–407 (2023).
Vagni, P. et al. POLYRETINA restores light responses in vivo in blind Göttingen minipigs. Nat. Commun. 13 , 3678 (2022).
Chenais, N. A. L., Leccardi, M. J. I. A. & Ghezzi, D. Photovoltaic retinal prosthesis restores high-resolution responses to single-pixel stimulation in blind retinas. Commun. Mater. 2 , 28 (2021).
Article CAS Google Scholar
Ferlauto, L. et al. Design and validation of a foldable and photovoltaic wide-field epiretinal prosthesis. Nat. Commun. 9 , 992 (2018).
Article ADS PubMed PubMed Central Google Scholar
Leccardi, M. J. I. A. et al. Photovoltaic organic interface for neuronal stimulation in the near-infrared. Commun. Mater. 1 , 21 (2020).
Gaillet, V. et al. Spatially selective activation of the visual cortex via intraneural stimulation of the optic nerve. Nat. Biomed. Eng. 4 , 181–194 (2020).
Gaillet, V., Borda, E., Zollinger, E. G. & Ghezzi, D. A machine-learning algorithm correctly classifies cortical evoked potentials from both visual stimulation and electrical stimulation of the optic nerve. J. Neural Eng. 18 , 046031 (2021).
Article ADS Google Scholar
Chen, X., Wang, F., Fernandez, E. & Roelfsema, P. R. Shape perception via a high-channel-count neuroprosthesis in monkey visual cortex. Science 370 , 1191–1196 (2020).
Fernández, E. et al. Visual percepts evoked with an Intracortical 96-channel microelectrode array inserted in human occipital cortex. J. Clin. Invest. 131 , e151331 (2021).
Jeon, C.-J., Strettoi, E. & Masland, R. H. The major cell populations of the mouse retina. J. Neurosci. 18 , 8936–8946 (1998).
Article PubMed PubMed Central CAS Google Scholar
Masland, R. H. The neuronal organization of the retina. Neuron 76 , 266–280 (2012).
Masland, R. H. Accurate maps of visual circuitry. Nature 500 , 154–155 (2013).
Masland, R. H. Neuronal diversity in the retina. Curr. Opin. Neurobiol. 11 , 431–436 (2001).
Luo, Y. H.-L. & Cruz, Lda The Argus® II retinal prosthesis system. Prog. Retin. Eye Res. 50 , 89–107 (2016).
Edwards, T. L. et al. Assessment of the electronic retinal implant Alpha AMS in Restoring Vision to Blind Patients with End-Stage Retinitis Pigmentosa. Ophthalmology 125 , 432–443 (2018).
Palanker, D., Mer, Y. L., Mohand-Said, S. & Sahel, J. A. Simultaneous perception of prosthetic and natural vision in AMD patients. Nat. Commun. 13 , 513 (2022).
Muqit, M. M. K. et al. Six-month safety and efficacy of the intelligent retinal implant System II device in retinitis pigmentosa. Ophthalmology 126 , 637–639 (2019).
Petoe, M. A. et al. A Second-Generation (44-Channel) Suprachoroidal Retinal prosthesis: interim clinical trial results. Transl. Vis. Sci. Technol. 10 , 12 (2021).
Veraart, C., Wanet‐Defalque, M., Gérard, B., Vanlierde, A. & Delbeke, J. Pattern recognition with the optic nerve visual prosthesis. Artif. Organs 27 , 996–1004 (2003).
Wang, J. et al. The application of computer vision to visual prosthesis. Artif. Organs 45 , 1141–1154 (2021).
Sanchez-Garcia, M., Martinez-Cantin, R. & Guerrero, J. J. Semantic and structural image segmentation for prosthetic vision. PLoS ONE 15 , e0227677 (2020).
Beyeler, M., Boynton, G., Fine, I. & Rokem, A. pulse2percept: A Python-based simulation framework for bionic vision. Proc. 16th Python Sci. Conf . 81–88. https://doi.org/10.25080/shinma-7f4c6e7-00c (2017).
Lozano, A. et al. Neurolight: A deep learning neural interface for cortical visual prostheses. Int. J. Neural Syst. 30 , 2050045 (2020).
Wienbar, S. & Schwartz, G. W. The dynamic receptive fields of retinal ganglion cells. Prog. Retin. Eye Res. 67 , 102–117 (2018).
Lozano, A., Garrigós, J., Martínez, J. J., Ferrández, J. M. & Fernández, E. Towards a Deep Learning Model of Retina: Retinal Neural Encoding of Color Flash Patterns. in 464–472 (Springer International Publishing, 2017). https://doi.org/10.1007/978-3-319-59740-9_46 .
Field, G. D. & Chichilnisky, E. J. Information processing in the primate retina: circuitry and coding. Annu. Rev. Neurosci. 30 , 1–30 (2007).
Saal, H. P., Harvey, M. A. & Bensmaia, S. J. Rate and timing of cortical responses driven by separate sensory channels. eLife 4 , e10450 (2015).
Goldin, M. A. et al. Context-dependent selectivity to natural images in the retina. Nat. Commun. 13 , 5556 (2022).
McIntosh, L. T., Maheswaranathan, N., Nayebi, A., Ganguli, S. & Baccus, S. A. Deep learning models of the retinal response to natural scenes. Adv. Neural Inf. Process. Syst. 29 , 1369–1377 (2016).
PubMed PubMed Central Google Scholar
Rahmani, B. et al. Actor neural networks for the robust control of partially measured nonlinear systems showcased for image propagation through diffuse media. Nat. Mach. Intell. 2 , 403–410 (2020).
Rahmani, B., Psaltis, D. & Moser, C. Variational framework for partially-measured physical system control: examples of vision neuroscience and optical random media. arXiv . https://doi.org/10.48550/arxiv.2110.13228 (2021).
Pei, Y. C., Hsiao, S. S. & Bensmaia, S. J. The tactile integration of local motion cues is analogous to its visual counterpart. Proc. Natl. Acad. Sci. 105 , 8130–8135 (2008).
Jin, F. & Feng, D. Image Registration Algorithm using Mexican Hat function-based operator and grouped feature matching strategy. PLoS ONE 9 , e95576 (2014).
Ran, Y. et al. Type-specific dendritic integration in mouse retinal ganglion cells. Nat. Commun. 11 , 2101 (2020).
Eldeeb, S. & Akcakaya, M. EEG guided electrical stimulation parameters generation from texture force profiles. J. Neural Eng. 19 , 066042 (2022).
Chichilnisky, E. J. A simple white noise analysis of neuronal light responses. Netw. Comput. Neural Syst . 12 , 199–213 (2001).
Pillow, J. W. et al. Spatio-temporal correlations and visual signalling in a complete neuronal population. Nature 454 , 995–999 (2008).
Gollisch, T. & Meister, M. Modeling convergent ON and OFF pathways in the early visual system. Biol. Cybern. 99 , 263–278 (2008).
Gollisch, T. & Meister, M. Rapid neural coding in the retina with relative spike latencies. Science 319 , 1108–1111 (2008).
Demb, J. B., Zaghloul, K., Haarsma, L. & Sterling, P. Bipolar cells contribute to nonlinear spatial summation in the Brisk-Transient (Y) Ganglion cell in mammalian retina. J. Neurosci. 21 , 7447–7454 (2001).
Rahmani, B., Psaltis, D. & Moser, C. Natural image synthesis for the retina with variational information bottleneck representation. Adv. Neural Inf. Process. Syst. (2022). https://openreview.net/forum?id=FkPZGtTxXx6 .
Al-Atabany, W., McGovern, B., Mehran, K., Berlinguer-Palmini, R. & Degenaar, P. A processing platform for optoelectronic/optogenetic retinal prosthesis. IEEE Trans. Biomed. Eng. 60 , 781–791 (2013).
Kasowski, J., Wu, N. & Beyeler, M. Towards immersive virtual reality simulations of bionic vision. Augment. Hum. Conf. 2021 313–315. https://doi.org/10.1145/3458709.3459003 (2021).
Cruz et al. The Argus II epiretinal prosthesis system allows letter and word reading and long-term function in patients with profound vision loss. Brit. J. Ophthalmol . 97 , 632 (2013).
Ghaffari, D. H., Chang, Y.-C., Mirzakhalili, E. & Weiland, J. D. Closed-loop optimization of retinal Ganglion cell responses to epiretinal stimulation: a computational study. 2021 10th Int. IEEEEMBS Conf. Neural Eng. (NER) 00 , 597–600 (2021).
Spencer, M. J., Kameneva, T., Grayden, D. B., Meffin, H. & Burkitt, A. N. Global activity shaping strategies for a retinal implant. J. Neural Eng. 16 , 026008 (2018).
Fauvel, T. & Chalk, M. Human-in-the-loop optimization of visual prosthetic stimulation. J. Neural Eng. 19 , 036038 (2022).
Shah, N. P. et al. Optimization of electrical stimulation for a high-fidelity artificial retina. 2019 9th Int. IEEEEMBS Conf. Neural Eng. (NER) 00 , 714–718 (2019).
Steveninck, J. et al. End-to-end optimization of prosthetic vision. J Vision 22 , 20 (2022).
Relic, L., Zhang, B., Tuan, Y.-L. & Beyeler, M. Deep learning-based perceptual stimulus encoder for bionic vision. arXiv https://doi.org/10.48550/arxiv.2203.05604 (2022).
Granley, J., Relic, L. & Beyeler, M. Hybrid neural autoencoders for stimulus encoding in visual and other sensory neuroprostheses. arXiv. https://doi.org/10.48550/arxiv.2205.13623 (2022).
Hateren, J. Hvan & Schaaf, Avander Independent component filters of natural images compared with simple cells in primary visual cortex. Proc. R. Soc. Lond. Ser. B: Biol. Sci. 265 , 359–366 (1998).
Yger, P. et al. A spike sorting toolbox for up to thousands of electrodes validated with ground truth recordings in vitro and in vivo. eLife 7 , e34518 (2018).
Rossant, C. et al. Spike sorting for large, dense electrode arrays. Nat. Neurosci. 19 , 634–641 (2016).
Klindt, D. A., Ecker, A. S., Euler, T. & Bethge, M. Neural system identification for large populations separating “what” and “where.” arXiv. https://doi.org/10.48550/arxiv.1711.02653 (2017).
Cadena, S. A. et al. Deep convolutional models improve predictions of macaque V1 responses to natural images. PLoS Comput. Biol. 15 , e1006897 (2019).
Download references
Acknowledgements
We would like to thank Prof. Silvestro Micera (EPFL, Switzerland) for reading the manuscript and providing valuable feedback. We would also like to thank the team of Dr. Olivier Marre (Institut de la Vision, France) for providing the code and preprocessed images used in this manuscript and the team of Dr. Serge Picaud (Institut de la Vision, France), for their advice to improve the setup for retinal recording. The project was supported by the EPFL STI e-seed fund.
Author information
Babak Rahmani
Present address: Microsoft Research, Cambridge, UK
Authors and Affiliations
Medtronic Chair in Neuroengineering, Center for Neuroprosthetics and Institute of Bioengineering, School of Engineering, École Polytechnique Fédérale de Lausanne, Geneva, Switzerland
Franklin Leong & Diego Ghezzi
Laboratory of Applied Photonics Devices, Institute of Electrical and Micro Engineering, School of Engineering, École Polytechnique Fédérale de Lausanne, Lausanne, Switzerland
Babak Rahmani & Christophe Moser
Optics Laboratory, Institute of Electrical and Micro Engineering, School of Engineering, École Polytechnique Fédérale de Lausanne, Lausanne, Switzerland
Demetri Psaltis
Ophthalmic and Neural Technologies Laboratory, Department of Ophthalmology, University of Lausanne, Hôpital ophtalmique Jules-Gonin, Fondation Asile des Aveugles, Lausanne, Switzerland
Diego Ghezzi
You can also search for this author in PubMed Google Scholar
Contributions
F.L. performed experiments, modeled and analyzed data and wrote the manuscript. B.R. performed experiments. D.P. and C.M. designed the study. D.G. designed and led the study and wrote the manuscript. All the authors read and edited the manuscript.
Corresponding author
Correspondence to Diego Ghezzi .
Ethics declarations
Competing interests.
The authors declare no competing interests.
Peer review
Peer review information.
: Nature Communications thanks Maxwell Turner, Al-Azawi Mohammad and the other anonymous reviewers for their contribution to the peer review of this work. A peer review file is available.
Additional information
Publisher’s note Springer Nature remains neutral with regard to jurisdictional claims in published maps and institutional affiliations.
Supplementary information
Supplementary information, peer review file, reporting summary, source data, source data, rights and permissions.
Open Access This article is licensed under a Creative Commons Attribution 4.0 International License, which permits use, sharing, adaptation, distribution and reproduction in any medium or format, as long as you give appropriate credit to the original author(s) and the source, provide a link to the Creative Commons license, and indicate if changes were made. The images or other third party material in this article are included in the article’s Creative Commons license, unless indicated otherwise in a credit line to the material. If material is not included in the article’s Creative Commons license and your intended use is not permitted by statutory regulation or exceeds the permitted use, you will need to obtain permission directly from the copyright holder. To view a copy of this license, visit http://creativecommons.org/licenses/by/4.0/ .
Reprints and permissions
About this article
Cite this article.
Leong, F., Rahmani, B., Psaltis, D. et al. An actor-model framework for visual sensory encoding. Nat Commun 15 , 808 (2024). https://doi.org/10.1038/s41467-024-45105-5
Download citation
Received : 17 August 2023
Accepted : 15 January 2024
Published : 27 January 2024
DOI : https://doi.org/10.1038/s41467-024-45105-5
Share this article
Anyone you share the following link with will be able to read this content:
Sorry, a shareable link is not currently available for this article.
Provided by the Springer Nature SharedIt content-sharing initiative
By submitting a comment you agree to abide by our Terms and Community Guidelines . If you find something abusive or that does not comply with our terms or guidelines please flag it as inappropriate.
Quick links
- Explore articles by subject
- Guide to authors
- Editorial policies
Sign up for the Nature Briefing newsletter — what matters in science, free to your inbox daily.

Visual culture and the Moscow Metro
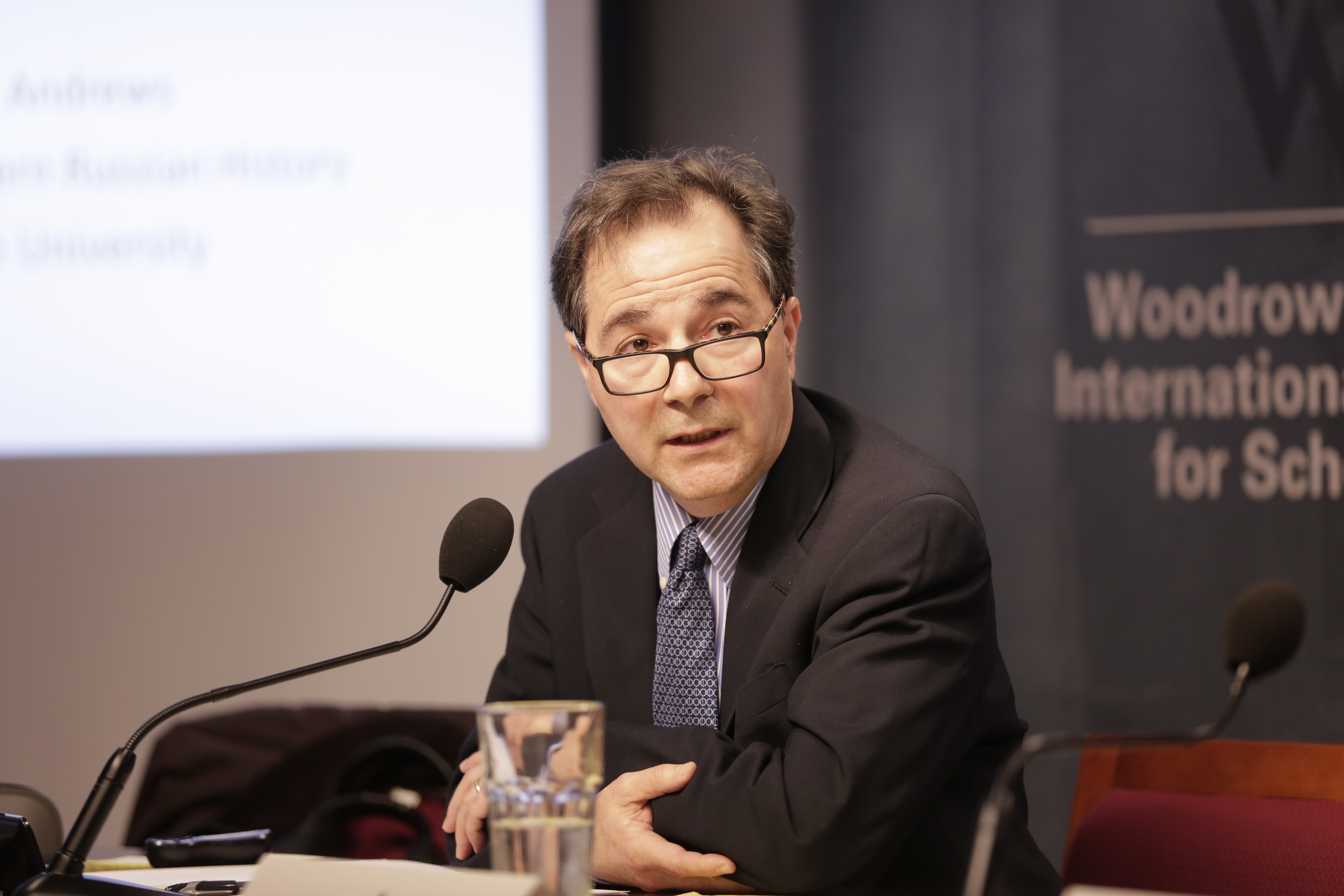
James Andrews first rode the Moscow Metro in the 1980s as an undergraduate student studying in the former Soviet Union.
Now after 35 years, the metro is taking him on a new journey: documenting how its iconic public spaces reveal the story of Moscow itself as the city evolved under Joseph Stalin and his Soviet and post-Soviet successors.
This May, Andrews, professor of history, gave a public lecture on his new research project at the Kennan Institute in Washington, D.C. The Kennan Institute is part of the Woodrow Wilson Center, where Andrews was a former senior resident fellow.
The talk, which has been made available on YouTube, was so well-received that Andrews immediately received an additional invitation to present at the Jordan Center for the Advanced Study of Russia at New York University.
What makes the Moscow Metro a fascinating subject for a historian? More people ride the Moscow Metro each day than the New York City Subway and London Tube combined, yet its history is relatively unknown, Andrews said.
“Everyone has a story about the metro, but there has never been a book in English about its history,” Andrews said. “Many of my generation spent an endless amount of time on the metro. Before people had cell phones, you would always say, ‘Well, we’ll meet on the platform at this station.’ Everyone spent time traveling through this cavernous system. It’s a labyrinth really, but at the same time people don’t know much about its history in detail.”
Andrews’ previous books focus on the history of Russian science, technology and the Soviet space program. His new book project on the metro is another opportunity to study iconic Soviet technology, as well as visual culture.
The original metro stations, built with deep shaft tunneling methods beginning in 1932, had airy open ceilings with gorgeous bas-relief architecture—a canvas that Stalin used to create a public art monument to socialism.
“Socialist countries had a tendency to produce technologies that they could adorn with stories,” Andrews said. “Their metro was started in the 1930s under Stalin, and they invested a lot of money in it. It was important for two reasons: there was a utilitarian nature. The crowds were incredible in Moscow. They needed better public transportation. People had been flooding in from the countryside during collectivization. The other thing was to come up with a monument to socialism. That’s why they hired all these architects to decorate, and they decorated each station thematically.”
In the years after Stalin’s death, Nikita Khrushchev issued an edict rejecting the metro’s flamboyant architectural style in favor of a pre-fabricated, practical style, Andrews said. At the same time all over the city, de-Stalinization was happening, and street signs, busts of Stalin and station names were changed or removed.
“The irony is Stalin funded it,” Andrews said. “It’s a Stalin-era display technology project, but by the Khrushchev era they were erasing Stalin’s name from it.”
Andrews has had unlimited access to Moscow’s architectural archives, including many original photographic negatives, and will return to Russia next summer to research political archives.
“The Bolsheviks liked to document everything, but I was shocked at how many photographs they had taken of all these projects, documenting every stage and how well they were preserved,” he said.
Throughout his research, he has uncovered more stories hidden within familiar metro spaces.
One Russian architectural archivist told Andrews about being born in the metro in 1942, during a time when its underground stations housed makeshift hospitals, military meetings and even cultural events such as film nights.
Public spaces bear new stories over time, and Andrews is closely following the story told by newly constructed metro stations. New stations are bringing back marble-laden aesthetics and cultural themes that highlight famous Russian figures like chemist Dmitri Mendeleev or writer Fyodor Dostoyevsky, he said.
“I think the story tells us now that during Putin’s era you see a resurgence of Russian nationalism and Russian themes,” he said. “Not every station can be imploded into this reductive narrative, but it points again to how the narrative of the metro stations changes as the politics of Russian change to some extent and the city itself.”
And it shows how the Moscow Metro has a seemingly endless amount of track for a historian to travel.
“What I love about the project is there is a political history to it, a technological history, a social history, an engineering element, and an artistic element,” Andrews said. “It’s a multi-disciplinary study, which is both fascinating to me and challenging.”

Turn Your Curiosity Into Discovery
Latest facts.
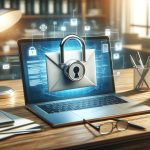
Tips and Tricks to Help You Create a HIPAA Compliant Email
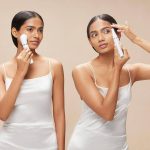
How to Stop Facial Hair Growth in Females Naturally
40 facts about elektrostal.
Written by Lanette Mayes
Modified & Updated: 02 Mar 2024

Reviewed by Jessica Corbett
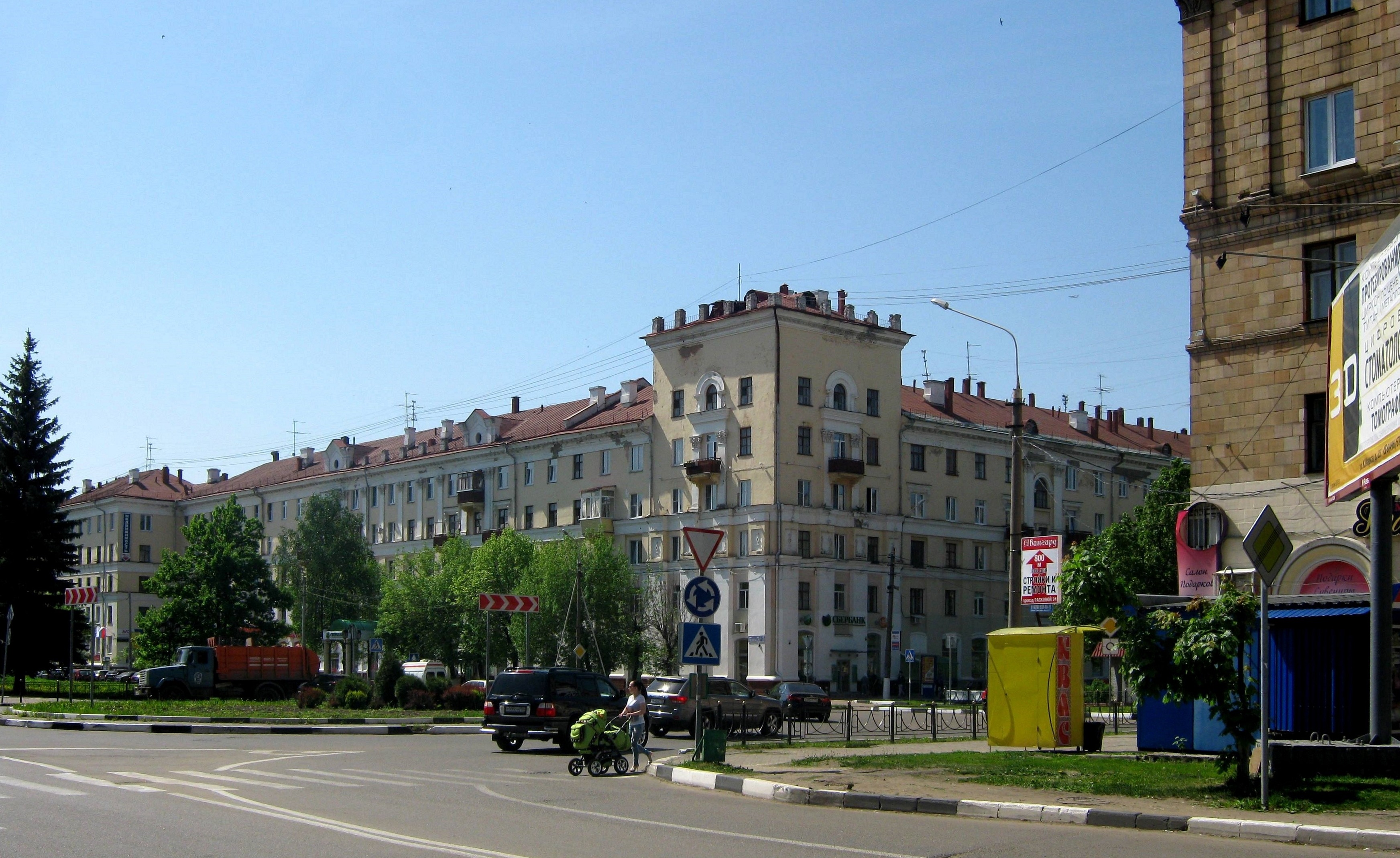
Elektrostal is a vibrant city located in the Moscow Oblast region of Russia. With a rich history, stunning architecture, and a thriving community, Elektrostal is a city that has much to offer. Whether you are a history buff, nature enthusiast, or simply curious about different cultures, Elektrostal is sure to captivate you.
This article will provide you with 40 fascinating facts about Elektrostal, giving you a better understanding of why this city is worth exploring. From its origins as an industrial hub to its modern-day charm, we will delve into the various aspects that make Elektrostal a unique and must-visit destination.
So, join us as we uncover the hidden treasures of Elektrostal and discover what makes this city a true gem in the heart of Russia.
Key Takeaways:
- Elektrostal, known as the “Motor City of Russia,” is a vibrant and growing city with a rich industrial history, offering diverse cultural experiences and a strong commitment to environmental sustainability.
- With its convenient location near Moscow, Elektrostal provides a picturesque landscape, vibrant nightlife, and a range of recreational activities, making it an ideal destination for residents and visitors alike.
Known as the “Motor City of Russia.”
Elektrostal, a city located in the Moscow Oblast region of Russia, earned the nickname “Motor City” due to its significant involvement in the automotive industry.
Home to the Elektrostal Metallurgical Plant.
Elektrostal is renowned for its metallurgical plant, which has been producing high-quality steel and alloys since its establishment in 1916.
Boasts a rich industrial heritage.
Elektrostal has a long history of industrial development, contributing to the growth and progress of the region.
Founded in 1916.
The city of Elektrostal was founded in 1916 as a result of the construction of the Elektrostal Metallurgical Plant.
Located approximately 50 kilometers east of Moscow.
Elektrostal is situated in close proximity to the Russian capital, making it easily accessible for both residents and visitors.
Known for its vibrant cultural scene.
Elektrostal is home to several cultural institutions, including museums, theaters, and art galleries that showcase the city’s rich artistic heritage.
A popular destination for nature lovers.
Surrounded by picturesque landscapes and forests, Elektrostal offers ample opportunities for outdoor activities such as hiking, camping, and birdwatching.
Hosts the annual Elektrostal City Day celebrations.
Every year, Elektrostal organizes festive events and activities to celebrate its founding, bringing together residents and visitors in a spirit of unity and joy.
Has a population of approximately 160,000 people.
Elektrostal is home to a diverse and vibrant community of around 160,000 residents, contributing to its dynamic atmosphere.
Boasts excellent education facilities.
The city is known for its well-established educational institutions, providing quality education to students of all ages.
A center for scientific research and innovation.
Elektrostal serves as an important hub for scientific research, particularly in the fields of metallurgy, materials science, and engineering.
Surrounded by picturesque lakes.
The city is blessed with numerous beautiful lakes, offering scenic views and recreational opportunities for locals and visitors alike.
Well-connected transportation system.
Elektrostal benefits from an efficient transportation network, including highways, railways, and public transportation options, ensuring convenient travel within and beyond the city.
Famous for its traditional Russian cuisine.
Food enthusiasts can indulge in authentic Russian dishes at numerous restaurants and cafes scattered throughout Elektrostal.
Home to notable architectural landmarks.
Elektrostal boasts impressive architecture, including the Church of the Transfiguration of the Lord and the Elektrostal Palace of Culture.
Offers a wide range of recreational facilities.
Residents and visitors can enjoy various recreational activities, such as sports complexes, swimming pools, and fitness centers, enhancing the overall quality of life.
Provides a high standard of healthcare.
Elektrostal is equipped with modern medical facilities, ensuring residents have access to quality healthcare services.
Home to the Elektrostal History Museum.
The Elektrostal History Museum showcases the city’s fascinating past through exhibitions and displays.
A hub for sports enthusiasts.
Elektrostal is passionate about sports, with numerous stadiums, arenas, and sports clubs offering opportunities for athletes and spectators.
Celebrates diverse cultural festivals.
Throughout the year, Elektrostal hosts a variety of cultural festivals, celebrating different ethnicities, traditions, and art forms.
Electric power played a significant role in its early development.
Elektrostal owes its name and initial growth to the establishment of electric power stations and the utilization of electricity in the industrial sector.
Boasts a thriving economy.
The city’s strong industrial base, coupled with its strategic location near Moscow, has contributed to Elektrostal’s prosperous economic status.
Houses the Elektrostal Drama Theater.
The Elektrostal Drama Theater is a cultural centerpiece, attracting theater enthusiasts from far and wide.
Popular destination for winter sports.
Elektrostal’s proximity to ski resorts and winter sport facilities makes it a favorite destination for skiing, snowboarding, and other winter activities.
Promotes environmental sustainability.
Elektrostal prioritizes environmental protection and sustainability, implementing initiatives to reduce pollution and preserve natural resources.
Home to renowned educational institutions.
Elektrostal is known for its prestigious schools and universities, offering a wide range of academic programs to students.
Committed to cultural preservation.
The city values its cultural heritage and takes active steps to preserve and promote traditional customs, crafts, and arts.
Hosts an annual International Film Festival.
The Elektrostal International Film Festival attracts filmmakers and cinema enthusiasts from around the world, showcasing a diverse range of films.
Encourages entrepreneurship and innovation.
Elektrostal supports aspiring entrepreneurs and fosters a culture of innovation, providing opportunities for startups and business development.
Offers a range of housing options.
Elektrostal provides diverse housing options, including apartments, houses, and residential complexes, catering to different lifestyles and budgets.
Home to notable sports teams.
Elektrostal is proud of its sports legacy, with several successful sports teams competing at regional and national levels.
Boasts a vibrant nightlife scene.
Residents and visitors can enjoy a lively nightlife in Elektrostal, with numerous bars, clubs, and entertainment venues.
Promotes cultural exchange and international relations.
Elektrostal actively engages in international partnerships, cultural exchanges, and diplomatic collaborations to foster global connections.
Surrounded by beautiful nature reserves.
Nearby nature reserves, such as the Barybino Forest and Luchinskoye Lake, offer opportunities for nature enthusiasts to explore and appreciate the region’s biodiversity.
Commemorates historical events.
The city pays tribute to significant historical events through memorials, monuments, and exhibitions, ensuring the preservation of collective memory.
Promotes sports and youth development.
Elektrostal invests in sports infrastructure and programs to encourage youth participation, health, and physical fitness.
Hosts annual cultural and artistic festivals.
Throughout the year, Elektrostal celebrates its cultural diversity through festivals dedicated to music, dance, art, and theater.
Provides a picturesque landscape for photography enthusiasts.
The city’s scenic beauty, architectural landmarks, and natural surroundings make it a paradise for photographers.
Connects to Moscow via a direct train line.
The convenient train connection between Elektrostal and Moscow makes commuting between the two cities effortless.
A city with a bright future.
Elektrostal continues to grow and develop, aiming to become a model city in terms of infrastructure, sustainability, and quality of life for its residents.
In conclusion, Elektrostal is a fascinating city with a rich history and a vibrant present. From its origins as a center of steel production to its modern-day status as a hub for education and industry, Elektrostal has plenty to offer both residents and visitors. With its beautiful parks, cultural attractions, and proximity to Moscow, there is no shortage of things to see and do in this dynamic city. Whether you’re interested in exploring its historical landmarks, enjoying outdoor activities, or immersing yourself in the local culture, Elektrostal has something for everyone. So, next time you find yourself in the Moscow region, don’t miss the opportunity to discover the hidden gems of Elektrostal.
Q: What is the population of Elektrostal?
A: As of the latest data, the population of Elektrostal is approximately XXXX.
Q: How far is Elektrostal from Moscow?
A: Elektrostal is located approximately XX kilometers away from Moscow.
Q: Are there any famous landmarks in Elektrostal?
A: Yes, Elektrostal is home to several notable landmarks, including XXXX and XXXX.
Q: What industries are prominent in Elektrostal?
A: Elektrostal is known for its steel production industry and is also a center for engineering and manufacturing.
Q: Are there any universities or educational institutions in Elektrostal?
A: Yes, Elektrostal is home to XXXX University and several other educational institutions.
Q: What are some popular outdoor activities in Elektrostal?
A: Elektrostal offers several outdoor activities, such as hiking, cycling, and picnicking in its beautiful parks.
Q: Is Elektrostal well-connected in terms of transportation?
A: Yes, Elektrostal has good transportation links, including trains and buses, making it easily accessible from nearby cities.
Q: Are there any annual events or festivals in Elektrostal?
A: Yes, Elektrostal hosts various events and festivals throughout the year, including XXXX and XXXX.
Was this page helpful?
Our commitment to delivering trustworthy and engaging content is at the heart of what we do. Each fact on our site is contributed by real users like you, bringing a wealth of diverse insights and information. To ensure the highest standards of accuracy and reliability, our dedicated editors meticulously review each submission. This process guarantees that the facts we share are not only fascinating but also credible. Trust in our commitment to quality and authenticity as you explore and learn with us.
Share this Fact:
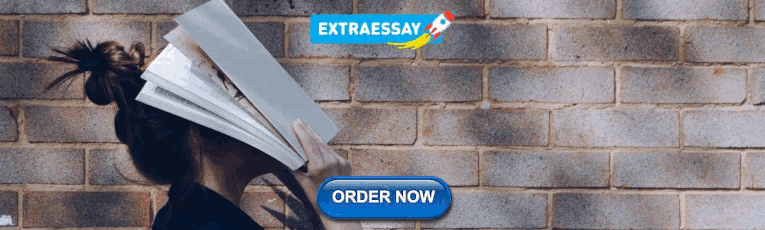
IMAGES
VIDEO
COMMENTS
Visual Thinking Strategies (VTS) is an educational non-profit that trains educators in schools, museums, and institutions of higher education to use a student-centered facilitation method to create inclusive discussions. Our intensive professional development programs provide individuals with the tools to become skilled facilitators of complex ...
Visual Thinking Strategies is a popular teaching method initiated by teacher-discussions of art images, and is commonly documented for having positive effects of teachers and students. Today, VTS is used in museums, schools, universities and health institutions around the world, and as an organization, provides workshop training for educators.
A well-designed visual image can yield a much more powerful and memorable learning experience than a mere verbal or textual description. Below you'll find resources for integrating visual thinking in your teaching. You'll find ideas for enhancing your presentations and for incorporating visual thinking in your students' in- and out-of ...
Keep the three questions simple so that instead the visual parts of the brain can focus on the picture. Integrating argument writing: Keep the engaging vibe going by asking students to write what they said or what they heard during the session. You can display the three VTS questions as a guide. Ask students to first write what they think is ...
Philip Yenawine, former education director of New York's Museum of Modern Art and co-creator of the curriculum outlined in Visual Thinking Strategies (Harvard Education Press, 2013), writes engagingly about his years of experience with young students in the classroom. He reveals how the VTS curriculum was developed,
The Thinking Eye works with Visual Thinking Strategies (VTS) as a method to both study and develop visual thinking skills. Facilitated VTS discussions about visual works of art or complex imagery ideally take place in a group and are always structured by the same basic 3 questions:
This chapter aims to deepen comprehension of visual literacy and how it can be developed through teaching a specific methodology, Visual Thinking Strategies (VTS). The authors' perspective is shaped by long-standing practice as educators using VTS and regularly engaging in close study of VTS interventions.
Visual Thinking Strategies (VTS) is a collaborative, student-centered teaching method that improves critical thinking skills and fosters inclusive community-building dialogue through facilitated discussions of visual images.. You do not need any special art training to use this strategy. The goal of VTS is not to teach the history of a work of art but, rather, to encourage students to observe ...
Visual Thinking Strategies (VTS) is an inquiry-based teaching technique grounded in teacher-student discussions, initially, of art images. First developed in the United States during the 1990s, it was created in its original inception to improve a participant's ability to describe, analyse, and interpret imagery and do this through active ...
Background Arts-based pedagogical tools have been increasingly incorporated into medical education. Visual Thinking Strategies (VTS) is a research-based, constructivist teaching methodology that aims to improve visual literacy, critical thinking, and communication skills through the process of investigating works of art. Harvard Medical School pioneered the application of VTS within medical ...
Visible Thinking is a flexible and systematic research-based conceptual framework, which aims to integrate the development of students' thinking with content learning across subject matters. Visible Thinking began as an initiative to develop a research-based approach to teaching thinking dispositions. The approach emphasized three core ...
Teaching. Visual thinking is extremely beneficial in the classroom, and teaching is one of the most important applications of visual thinking strategies. Teachers can use visual thinking to help explain complicated concepts so students can develop a deep relationship between ideas and can also help teach new information to establish strong ...
education; Visual Thinking Strategies ... DOWNLOAD BOOK DETAILS. Visual Thinking Strategies Using Art to Deepen Learning Across School Disciplines. by Philip Yenawine. 208 Pages, 6.00 x 9.00 in. Paperback; 9781612506098; Published: October 2013; $30.00. Buy . eBook; 9781612506111; Published: October 2013; $28.00. Buy Digital Book ...
The Visual Thinking Strategies (VTS) teaching method has been successfully implemented in schools, districts, and cultural institutions nationwide, including bilingual schools in California, West Orange Public Schools in New Jersey, and the San Francisco Museum of Modern Art.
This toolbox highlights thinking routines developed across a number of research projects at PZ. A thinking routine is a set of questions or a brief sequence of steps used to scaffold and support student thinking. PZ researchers designed thinking routines to deepen students' thinking and to help make that thinking "visible.".
Visual Thinking Strategies offers training in facilitation, coaching and aesthetic development. Whether you're new to facilitation or a seasoned practitioner, VTS offers professional development to support growth in all aspects of VTS methodology. Since 1995, VTS has welcomed over 12,000 educators and practitioners into classrooms, museums ...
To fully implement the internationally acknowledged requirements for teaching in evidence-based practice, and support the student's development of core competencies in evidence-based practice, educators at professional bachelor degree programs in healthcare need a systematic overview of evidence-based teaching and learning interventions. The purpose of this overview of systematic reviews was ...
For educators catering to students with diverse learning needs, hexagonal thinking presents a dynamic approach to teaching reading skills. Its structured format allows for scaffolding and personalized feedback, catering to individual student abilities, and facilitating a deeper understanding of fundamental reading components such as phonemes ...
In the changed conditions, it is of interest to study the most adaptive coping strategies that contribute to the preservation of psychological well-being. The study involved 88 girls in Russia ...
Among the various learning-free approaches we choose pixel averaging as the benchmark for evaluation, since it is the conventional visual encoding approach used in prosthetic devices.
James Andrews presents "Narrating the Soviet Metropolis: Visual Culture in the Moscow Metro" at the Kennan Institute in Washington, D.C., on May 17, 2016. The talk, which has been made available on YouTube, was so well-received that Andrews immediately received an additional invitation to present at the Jordan Center for the Advanced Study of ...
Abigail Housen is the co-founder of Visual Understanding in Education and the co-author of the VTS curriculum. Housen received her Ed.D from Harvard in 1983 and has pioneered aesthetic development research for over 30 years. ... His most recent book, Visual Thinking Strategies: Using Art to Deepen Learning Across School Disciplines, was ...
Lanette Mayes. Elektrostal is a vibrant city located in the Moscow Oblast region of Russia. With a rich history, stunning architecture, and a thriving community, Elektrostal is a city that has much to offer. Whether you are a history buff, nature enthusiast, or simply curious about different cultures, Elektrostal is sure to captivate you.
Bachelors in Mechanical Engineering are interdisciplinary degrees that teach students how to design or improve mechanical and thermal devices and systems using analysis and computer-aided design. Engineering schools teach students how to find solutions to develop new processes and products, ranging from small component designs to extremely ...