- Tools and Resources
- Customer Services
- Contentious Politics and Political Violence
- Governance/Political Change
- Groups and Identities
- History and Politics
- International Political Economy
- Policy, Administration, and Bureaucracy
- Political Anthropology
- Political Behavior
- Political Communication
- Political Economy
- Political Institutions
- Political Philosophy
- Political Psychology
- Political Sociology
- Political Values, Beliefs, and Ideologies
- Politics, Law, Judiciary
- Post Modern/Critical Politics
- Public Opinion
- Qualitative Political Methodology
- Quantitative Political Methodology
- World Politics
- Share This Facebook LinkedIn Twitter
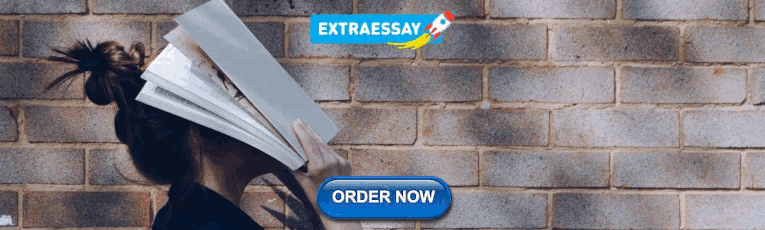
Article contents
Global migration: causes and consequences.
- Benjamin Helms Benjamin Helms Department of Politics, University of Virginia
- and David Leblang David Leblang Department of Politics, Frank Batten School of Leadership and Public Policy, University of Virginia
- https://doi.org/10.1093/acrefore/9780190228637.013.631
- Published online: 25 February 2019
International migration is a multifaceted process with distinct stages and decision points. An initial decision to leave one’s country of birth may be made by the individual or the family unit, and this decision may reflect a desire to reconnect with friends and family who have already moved abroad, a need to diversify the family’s access to financial capital, a demand to increase wages, or a belief that conditions abroad will provide social and/or political benefits not available in the homeland. Once the individual has decided to move abroad, the next decision is the choice of destination. Standard explanations of destination choice have focused on the physical costs associated with moving—moving shorter distances is often less expensive than moving to a destination farther away; these explanations have recently been modified to include other social, political, familial, and cultural dimensions as part of the transaction cost associated with migrating. Arrival in a host country does not mean that an émigré’s relationship with their homeland is over. Migrant networks are an engine of global economic integration—expatriates help expand trade and investment flows, they transmit skills and knowledge back to their homelands, and they remit financial and human capital. Aware of the value of their external populations, home countries have developed a range of policies that enable them to “harness” their diasporas.
- immigration
- international political economy
- factor flows
- gravity models
Introduction
The steady growth of international labor migration is an important, yet underappreciated, aspect of globalization. 1 In 1970 , just 78 million people, or about 2.1% of the global population, lived outside their country of birth. By 1990 , that number had nearly doubled to more than 150 million people, or about 2.8% of the global population (United Nations Population Division, 2012 ). Despite the growth of populist political parties and restrictionist movements in key destination countries, the growth in global migration shows no signs of slowing down, with nearly 250 million people living outside their country of birth as of 2015 . While 34% of all global migrants live in industrialized countries (with the United States and Germany leading the way), 38% of all global migration occurs between developing countries (World Bank, 2016 ).
Identifying the causes and consequences of international labor migration is essential to our broader understanding of globalization. Scholars across diverse academic fields, including economics, political science, sociology, law, and demography, have attempted to explain why individuals voluntarily leave their homelands. The dominant thread in the labor migration literature is influenced by microeconomics, which posits that individuals contemplating migration are rational, utility-maximizing actors who carefully weigh the potential costs and benefits of leaving their country of origin (e.g., Borjas, 1989 ; Portes & Böröcz, 1989 ; Grogger & Hanson, 2011 ). The act of migration, from this perspective, is typically conceptualized as an investment from which a migrant expects to receive some benefit, whether it be in the form of increased income, political freedom, or enhanced social ties (Schultz, 1961 ; Sjaastad, 1962 ; Collier & Hoeffler, 2014 ).
In this article we go beyond the treatment of migration as a single decision and conceive of it as a multifaceted process with distinct stages and decision points. We identify factors that are relevant at different stages in the migration process and highlight how and when certain factors interact with others during the migration process. Economic factors such as the wage differential between origin and destination countries, for example, may be the driving factor behind someone’s initial decision to migrate (Borjas, 1989 ). But when choosing a specific destination, economic factors may be conditioned by political or social conditions in that destination (Fitzgerald, Leblang, & Teets, 2014 ). Each stage or decision point has distinguishing features that are important in determining how (potential) migrants respond to the driving forces identified by scholars.
This is certainly not a theoretical innovation; migration has long been conceived of as a multi-step process, and scholars often identify the stage or decision point to which their argument best applies. However, most interdisciplinary syntheses of the literature on international labor migration do not provide a systematic treatment of this defining feature, instead organizing theoretical and empirical contributions by field of study, unit or level of analysis, or theoretical tradition (e.g., Portes & Böröcz, 1989 ; Massey et al., 1993 ; European Asylum Support Office, 2016 ). Such approaches are undoubtedly valuable in their own right. Our decision to organize this discussion by stage allows us to understand this as a process, rather than as a set of discrete events. As a result, we conceptualize international labor migration as three stages or decision points: (a) the decision to migrate or to remain at home, (b) the choice of destination, and (c) the manner by which expatriates re-engage—or choose not to re-engage—with their country of origin once abroad. We also use these decision points to highlight a number of potential new directions for future research in this still-evolving field.
Figure 1. Global migration intentions by educational attainment, 2008–2017.
Should I Stay or Should I Go, Now?
The massive growth in international labor migration in the age of globalization is remarkable, but the fact remains that over 95% of the world’s population never leave their country of origin (United Nations Population Division, 2012 ). Figure 1 shows the percentage of people who expressed an intention to move abroad between 2008 and 2017 by educational attainment, according to data from the Gallup World Poll. Over this time period, it appears that those who were highly educated expressed intent to migrate in greater numbers than those who had less than a college education, although these two groups have converged in recent years. What is most striking, however, is that a vast majority of people, regardless of educational attainment, expressed no desire to move abroad. Even though absolute flows of migrants have grown at a near-exponential rate, relative to their non-migrating counterparts, they remain a small minority. What factors are important in determining who decides to migrate and who decides to remain at home? 2
From Neoclassical Economics to the Mobility Transition
Neoclassical economic models posit that the primary driving factor behind migration is the expected difference in wages (discounted future income streams) between origin and destination countries (Sjaastad, 1962 ; Borjas, 1989 ; Clark, Hatton, & Williamson, 2007 ). All else equal, when the wage gap, minus the costs associated with moving between origin and destination, is high, these models predict large flows of labor migrants. In equilibrium, as more individuals move from origin to destination countries, the wage differential narrows, which in turn leads to zero net migration (Lewis, 1954 ; Harris & Todaro, 1970 ). Traditional models predict a negative monotonic relationship between the wage gap and the number of migrants (e.g., Sjaastad, 1962 ). However, the predictions of neoclassical models are not well supported by the empirical record. Empirical evidence shows that, at least in a cross-section, the relationship between economic development and migration is more akin to an inverted U. For countries with low levels of per capita income, we observe little migration due to a liquidity constraint: at this end of the income distribution, individuals do not have sufficient resources to cover even minor costs associated with moving abroad. Increasing income helps to decrease this constraint, and consequently we observe increased levels of emigration as incomes rise (de Haas, 2007 ). This effect, however, is not monotonic: as countries reach middle-income status, declining wage differentials lead to flattening rates of emigration, and then decreasing rates as countries enter later stages of economic development. 3
Some research explains this curvilinear relationship by focusing on the interaction between emigration incentives and constraints : for example, increased income initially makes migration more affordable (reduces constraints), but also simultaneously reduces the relative economic benefits of migrating as the wage differential narrows (as potential migrants now have the financial capacity to enhance local amenities) (Dao, Docquier, Parsons, & Peri, 2016 ). The theoretical underpinnings of this interaction, however, are not without controversy. Clemens identifies several classes of theory that attempt to explain this curvilinear relationship—a relationship that has been referred to in the literature as the mobility transition (Clemens, 2014 ). These theories include: demographic changes resulting from development that also favor emigration up to a point (Easterlin, 1961 ; Tomaske, 1971 ), the loosening of credit restraints on would-be migrants (Vanderkamp, 1971 ; Hatton & Williamson, 1994 ), a breakdown of information barriers via the building of transnational social networks (Epstein, 2008 ), structural economic changes in the development process that result in worker dislocation (Zelinsky, 1971 ; Massey, 1988 ), the dynamics of economic inequality and relative deprivation (Stark, 1984 ; Stark & Yitzhaki, 1988 ; Stark & Taylor, 1991 ), and changing immigration policies in destination countries toward increasingly wealthy countries (Clemens, 2014 ). While each of these play some role in the mobility transition curve, Dao et al. ( 2016 ) run an empirical horse race between numerous explanations and find that changing skill composition resulting from economic development is the most substantively important driver. Economic development is correlated with an increase in a country’s level of education; an increase in the level of education, in turn, is correlated with increased emigration. However, traditional explanations involving microeconomic drivers such as income, credit constraints, and economic inequality remain important factors (Dao et al., 2016 ). The diversity of explanations offered for the mobility transition curve indicates that while most research agrees the inverted-U relationship is an accurate empirical portrayal of the relationship between development and migration, little theoretical agreement exists on what drives this relationship. Complicating this disagreement is the difficulty of empirically disentangling highly correlated factors such as income, skill composition, and demographic trends in order to identify robust causal relationships.
Political Conditions at the Origin
While there is a scholarly consensus around the mobility transition and the role of economic conditions, emerging research suggests that the political environment in the origin country may also be salient. We do not refer here to forced migration, such as in the case of those who leave because they are fleeing political persecution or violent conflict. Rather, we focus on political conditions in the homeland that influence a potential migrant’s decision to emigrate voluntarily. Interpretations of how, and the extent to which, political conditions in origin countries (independent of economic conditions) influence the decision to migrate have been heavily influenced by Hirschman’s “Exit, Voice, and Loyalty” framework (Hirschman, 1970 , 1978 ). Hirschman argues that the opportunity to exit—to exit a firm, an organization, or a country—places pressure on the local authorities; voting with one’s feet forces organizations to reassess their operations.
When applied to the politics of emigration, Hirschman’s framework generates two different hypotheses. On the one hand, politicians may allow, encourage, or force the emigration of groups that oppose the regime as a political safety valve of sorts. This provides the government with a mechanism with which to manage potential political challengers by encouraging their exit. On the other hand, politicians—especially those in autocracies—may actively work to prevent exit because they fear the emigration of economic elites, the highly skilled, and others who have resources vital to the survival of the regime. 4
A small number of studies investigate how local-level, rather than national, political circumstances affect a potential migrant’s calculus. The limited empirical evidence currently available suggests that local conditions are substantively important determinants of the emigration decision. When individuals are highly satisfied with local amenities such as their own standard of living, quality of public services, and overall sense of physical security, they express far less intention to migrate compared with highly dissatisfied individuals (Dustmann & Okatenko, 2014 ). Furthermore, availability of public transport and access to better education facilities decreases the propensity to express an intention to emigrate (Cazzuffi & Modrego, 2018 ). This relationship holds across all levels of wealth and economic development, and there is some evidence that satisfaction with local amenities matters as much as, or even more than, income or wealth (Dustmann & Okatenko, 2014 ).
Political corruption, on both national and local levels, also has substantively important effects on potential migrants, especially those who are highly skilled. Broadly defined as the use of public office for political gain, political corruption operates as both a direct and an indirect factor promoting emigration. 5 Firstly, corruption may have a direct effect on the desire to emigrate in that it can decrease the political and economic power of an individual, leading to a lower standard of living and poorer quality of life in origin countries. If the reduction in life satisfaction resulting from corruption is sufficiently high—either by itself or in combination with other “push” factors—then the exit option becomes more attractive (Cooray & Schneider, 2016 ). Secondly, corruption also operates through indirect channels that influence other push factors. Given the large literature on how political corruption influences a number of development outcomes, it is conceivable that corruption affects the decision-making process of a potential migrant through its negative effect on social spending, education, and public health (Mo, 2001 ; Mauro, 1998 ; Gupta, Davoodi, & Thigonson, 2001 ).
The combination of its direct and indirect impacts means that corruption could be a significant part of a migrant’s decision-making process. At present there is limited work exploring this question, and the research does not yield a consensus. Some scholars argue that political corruption has no substantive effect on total bilateral migration, but that it does encourage migration among the highly skilled (Dimant, Krieger, & Meierrieks, 2013 ). This is the case, the argument goes, because corruption causes the greatest relative harm to the utility of those who have invested in human capital, who migrate to escape the negative effect on their fixed investment. In contrast, others find that a high level of corruption does increase emigration at the aggregate level (Poprawe, 2015 ). More nuanced arguments take into account the intensity of corruption: low to moderate levels of corruption lead to increased emigration of all groups, and especially of the highly skilled. But at high levels of corruption, emigration begins to decrease, indicating that intense corruption can act as a mobility constraint (Cooray & Schneider, 2016 ). All of these existing accounts, however, employ state-level measures of corruption by non-governmental organizations, such as those produced by Transparency International. Scholars have yet to harness micro-level survey data to explore the influence of personal corruption perception on the individual’s decision-making process.
The Land of Hopes and Dreams
Given that an individual has decided to emigrate, the next decision point is to choose a destination country. Advanced industrial democracies, such as those in the OECD, are major migrant-receiving countries, but so are Russia and several Gulf countries including Saudi Arabia, Qatar, and the United Arab Emirates (World Bank, 2016 ). A country’s constellation of political, economic, and social attributes is crucial to understanding an emigrant’s choice of destination. Potential migrants weigh all of these factors simultaneously when choosing a destination: will the destination allow political rights for the migrant and their children, is access to the labor market possible, and does the destination provide an opportunity for reunification with friends and family? In this section we focus on the non-economic factors that draw migrants to certain countries over others. In addition, we emphasize how skill level adds layers of complexity to a migrant’s calculus.
Political Environment, Both Formal and Informal
As noted earlier, traditional neoclassical models and their extensions place wage differentials and associated economic variables at the heart of a migrant’s choice. Gravity models posit that migrants choose a destination country based on their expected income—which itself is a function of the wage rate and the probability of finding employment in the destination—less the costs associated with moving (Ravenstein, 1885 ; Todaro, 1969 ; Borjas, 1989 ). A rigid focus on economic factors, however, blinds us to the empirical reality that a destination country’s political environment influences what destination a migrant chooses (Borjas, 1989 ). A country’s legal and political rights structure for migrants, as well as its level of tolerance for newcomers, is critical to migrants discriminating between an array of potential destinations. Fitzgerald, Leblang, and Teets ( 2014 ) argue, for example, that states with restrictive citizenship policies and strong radical right anti-immigrant parties will receive fewer migrants, while states with relatively liberal citizenship requirements and weak radical right political movements will receive more migrants. In the rational actor framework, migrants seek countries with hospitable political environments to maximize both their political representation in government and their access to labor market opportunities as a result of citizenship rights and social acceptance (Fitzgerald et al., 2014 ).
Using a broad sample of origin countries and 18 destination countries, they find that relative restrictiveness of citizenship policies and level of domestic support for the radical right are substantively important determinants of global migratory flows. Further, they find that these political variables condition a migrant’s choice of destination: the relative importance of economic factors such as the unemployment rate or the wage differential diminishes as a destination country’s political environment becomes more open for migrants. In other words, when migrants are choosing a destination country, political considerations may trump economic ones—a finding that is an important amendment to the primarily economics-focused calculus of the initial stage of the immigration decision.
However, prior to choosing and entering a destination country, a migrant must also navigate a country’s immigration policy—the regulation of both migrant entry and the rights and status of current migrants. While it is often assumed that a relatively more restrictive immigration policy deters entry, and vice versa, a lack of quantitative data has limited the ability of scholars to confirm this intuition cross-nationally. Money ( 1999 ) emphasizes that the policy output of immigration politics does not necessarily correlate with the outcome of international migrant flows. There are a number of unanswered questions in this field, including: is immigration policy a meaningful determinant of global flows of migration? Do certain kinds of immigration policies matter more than others? How does immigration policy interact with other political and economic factors, such as unemployment and social networks?
Only a handful of studies analyze whether or not immigration policy is a significant determinant of the size and character of migratory flows. Perhaps the most prominent answer to this question is the “gap hypothesis,” which posits that immigration rates continue to increase despite increasingly restrictive immigration policies in advanced countries (Cornelius & Tsuda, 2004 ). Some subsequent work seems to grant support to the gap hypothesis, indicating that immigration policy may not be a relevant factor and that national sovereignty as it relates to dictating migrant inflows has eroded significantly (Sassen, 1996 ; Castles, 2004 ). The gap hypothesis is not without its critics, with other scholars arguing that the existing empirical evidence actually lends it little or no support (Messina, 2007 ).
A more recent body of literature does indicate that immigration policy matters. Brücker and Schröder ( 2011 ), for example, find that immigration policies built to attract highly skilled migrants lead to higher admittance rates. They also show that diffusion processes cause neighboring countries to implement similar policy measures. Ortega and Peri ( 2013 ), in contrast to the gap hypothesis literature, find that restrictive immigration policy indeed reduces migrant inflows. But immigration policy can also have unintended effects on international migration: when entry requirements increase, migrant inflows decrease, but migrant outflows also decrease (Czaika & de Haas, 2016 ). This indicates that restrictive immigration policy may also lead to reduced circular migrant flows and encourage long-term settlement in destination countries.
Disaggregating immigration policy into its different components provides a clearer picture of how immigration policy may matter, and whether certain components matter more than others. Immigration policy is composed of both external and internal regulations. External regulations refer to policies that control migrant entry, such as eligibility requirements for migrants and additional conditions of entry. Internal regulations refer to policies that apply to migrants who have already gained status in the country, such as the security of a migrant’s legal status and the rights they are afforded. Helbling and Leblang ( 2017 ), using a comprehensive data set of bilateral migrant flows and the Immigration Policies in Comparison (IMPIC) data set, find that, in general, external regulations prove slightly more important in understanding migrant inflows (Helbling, Bjerre, Römer, & Zobel, 2017 ). This indicates that potential migrants focus more on how to cross borders, and less on the security of their status and rights once they settle. They do find, however, that both external and internal components of immigration are substantively important to international migrant flows.
The effects of policy, however, cannot be understood in isolation from other drivers of migration. Firstly, poor economic conditions and restrictive immigration policy are mutually reinforcing: when the unemployment rate is elevated, restrictive policies are more effective in deterring migrant flows. An increase in policy effectiveness in poor economic conditions suggests that states care more about deterring immigration when the economy is performing poorly. Secondly, a destination country’s restrictive immigration policy is more effective when migrants come from origin countries that have a common colonial heritage. This suggests that cultural similarities and migrant networks help to spread information about the immigration policy environment in the destination country. Social networks prove to be crucial in determining how much migrants know about the immigration policies of destination countries, regardless of other cultural factors such as colonial heritage or common language (Helbling & Leblang, 2017 ). In summary, more recent work supports the idea that immigration policy of destination countries exerts a significant influence on both the size and character of international migration flows. Much work remains to be done in terms of understanding the nuances of specific immigration policy components, the effect of policy change over time, and through what mechanisms immigration policy operates.
Transnational Social Networks
None of this should be taken to suggest that only political and economic considerations matter when a potential migrant contemplates a potential destination; perhaps one of the biggest contributions to the study of bilateral migration is the role played by transnational social networks. Migrating is a risky undertaking, and to minimize that risk, migrants are more likely to move to destinations where they can “readily tap into networks of co-ethnics” (Fitzgerald et al., 2014 , p. 410). Dense networks of co-ethnics not only help provide information about economic opportunities, but also serve as a social safety net which, in turn, helps decrease the risks associated with migration, including, but not limited to, finding housing and integrating into a new community (Massey, 1988 ; Portes & Böröcz, 1989 ; Portes, 1995 ; Massey et al., 1993 ; Faist, 2000 ; Sassen, 1995 ; Light, Bernard, & Kim, 1999 ). Having a transnational network of family members is quite important to destination choice; if a destination country has an immigration policy that emphasizes family reunification, migrants can use their familial connections to gain economically valuable permanent resident or citizenship status more easily than in other countries (Massey et al., 1993 , p. 450; Helbing & Leblang, 2017 ). When the migrant is comparing potential destinations, countries in which that migrant has a strong social network will be heavily favored in a cost–benefit analysis.
Note, however, that even outside of a strict rational actor framework with perfect information, transnational social networks still may be quite salient to destination choice. An interesting alternative hypothesis for the patterns we observe draws on theories from financial market behavior which focus on herding. Migrants choosing a destination observe the decisions of their co-ethnics who previously migrated and assume that those decisions were based on a relevant set of information, such as job opportunities or social tolerance of migrants. New migrants then choose the same destination as their co-ethnics not based on actual exchanges of valuable information, but based solely on the assumption that previous migration decisions were based on rational calculation (Epstein & Gang, 2006 ; Epstein, 2008 ). This is a classic example of herding, and the existing empirical evidence on the importance of transnational social networks cannot invalidate this alternative hypothesis. One could also explain social network effects through the lens of cumulative causation or feedback loops: the initial existence of connections in destination countries makes the act of migration less risky and attracts additional co-ethnics. This further expands migrant networks in a destination, further decreasing risk for future waves of migrants, and so on (Massey, 1990 ; Fussel & Massey, 2004 ; Fussel, 2010 ).
No matter the pathway by which social networks operate, the empirical evidence indicates that they are one of the most important determinants of destination choice. Potential migrants from Mexico, for example, who are able to tap into existing networks in the United States face lower direct, opportunity, and psychological costs of international migration (Massey & Garcia España, 1987 ). This same relationship holds in the European context; a study of Bulgarian and Italian migrants indicates that those with “social capital” in a destination community are more likely to migrate and to choose that particular destination (Haug, 2008 ). Studies that are more broadly cross-national in nature also confirm the social network hypothesis across a range of contexts and time periods (e.g., Clark et al., 2007 ; Hatton & Williamson, 2011 ; Fitzgerald et al., 2014 ).
Despite the importance of social networks, it is, again, important to qualify their role in framing the choice of destinations. It seems that the existence of co-ethnics in destination countries most strongly influences emigration when they are relatively few in number. Clark et al. ( 2007 ), in their study of migration to the United States, find that the “friends and relatives effect” falls to zero once the migrant stock in the United States reaches 8.3% of the source-country population. In addition, social networks alone cannot explain destination choice because their explanatory power is context-dependent. For instance, restrictive immigration policies limiting legal migration channels and family reunification may dampen the effectiveness of networks (Böcker, 1994 ; Collyer, 2006 ). Social networks are not an independent force, but also interact with economic and political realities to produce the global migration patterns we observe.
The Lens of Skill
For ease of presentation, we have up to now treated migrants as a relatively homogeneous group that faces similar push and pull factors throughout the decision-making process. Of course, not all migrants experience the same economic, political, and social incentives in the same way at each stage of the decision-making process. Perhaps the most salient differentiating feature of migrants is skill or education level. Generally, one can discuss a spectrum of skill and education level for current migrants, from relatively less educated (having attained a high school degree or less) to relatively more educated (having attained a college or post-graduate degree). The factors presented here that influence destination choice interact with a migrant’s skill level to produce differing destination choice patterns.
A migrant’s level of education, or human capital, often serves as a filter for the political treatment he or she anticipates in a particular destination country. For instance, the American public has a favorable view of highly educated migrants who hold higher-status jobs, while simultaneously having an opposite view of migrants who have less job training and do not hold a college degree (Hainmueller & Hiscox, 2010 ; Hainmueller & Hopkins, 2015 ). Indeed, the political discourse surrounding migration often emphasizes skill level and education as markers of migrants who “should be” admitted, across both countries and the ideological spectrum. 6 While political tolerance may be a condition of entry for migrants in the aggregate, the relatively privileged status of highly educated and skilled migrants in most destination countries may mean that this condition is not as salient.
While it is still an open question to what extent immigration policy influences international migration, it is clear that not all migrants face evenly applied migration restrictions. Most attractive destination countries have policies that explicitly favor highly skilled migrants, since these individuals often fill labor shortages in advanced industries such as high technology and applied science. Countries such as Australia, Canada, and New Zealand all employ so-called “points-based” immigration systems in which those with advanced degrees and needed skills are institutionally favored for legal entry (Papademetriou & Sumption, 2011 ). Meanwhile, the United States maintains the H-1B visa program, which is restricted by educational attainment and can only be used to fill jobs in which no native talent is available (USCIS). Even if destination countries decide to adopt more restrictive immigration policies, the move toward restriction has typically been focused on low-skilled migrants (Peters, 2017 ). In other words, even if immigration policy worldwide becomes more restrictive, this will almost certainly not occur at the expense of highly skilled migrants and will not prevent them choosing their most preferred destination.
Bring It on Home to Me
This article began by asserting that international labor migration is an important piece of globalization, as significant as cross-border flows of capital, goods, and services. This section argues that migrant flows enhance flows of capital and commodities. Uniquely modern conditions such as advanced telecommunications, affordable and efficient international travel, and the liberalization of financial flows mean that diasporas—populations of migrants living outside their countries of origin—and home countries often re-engage with each other (Vertovec, 2004 ; Waldinger, 2008 ). This section reviews some of the newest and most thought-provoking research on international labor migration, research that explores diaspora re-engagement and how that re-engagement alters international flows of income, portfolio and foreign direct investment (FDI), trade, and migratory flows themselves.
Remittances
As previously argued, migration is often driven by the prospect of higher wages. Rational, utility-maximizing migrants incur the cost of migration in order to earn increased income that they could not earn at home. But when migrants obtain higher wages, this additional increment to income is not always designated for individual consumption. Often, migrants use their new income to send remittances, direct transfers of money from one individual to another across national borders. Once a marginal financial flow, in 2015 remittances totaled $431 billion, far outpacing foreign aid ($135 billion) and nearly passing private debt and portfolio equity ($443 billion). More than 70% of total global remittances flow into developing countries (World Bank, 2016 ). In comparison with other financial flows such as portfolio investment and FDI, remittances are more impervious to economic crises, suggesting that they may be a countercyclical force to global downturns (Leblang, 2017 ).
Remittances represent one of the most common ways in which migrants re-engage with their homeland and alter both global income flows and distribution. Why do migrants surrender large portions of their new income, supposedly the very reason they migrated in the first place, to their families back home? New economics of labor migration (NELM) theory argues that immigration itself is motivated by a family’s need or demand for remittances—that remittances are an integral part of a family’s strategy for diversifying household financial risk (Stark & Bloom, 1985 ). Remittances “are a manifestation of informal contractual agreements between migrants and the households from which they move,” indicating that remitting is not an individual-level or purely altruistic action but rather occurs in a larger social context, that of one’s immediate or extended family (European Asylum Support Office, 2016 , p. 15).
The impact of migrant remittances on countries of origin is multifaceted yet somewhat ambiguous. Most scholarly work focuses on whether remittances positively or negatively influence existing economic conditions. A number of studies find that remittances modestly reduce poverty levels in developing countries (Adams & Page, 2005 ; Yang & Martinez, 2006 ; Acosta, Calderon, Fajnzybler, & Lopez, 2008 ; Lokshin, Bontch-Osmolovski, & Glinskaya, 2010 ). On other measures of economic well-being, such as growth, inequality, and health, the literature is quite mixed and no definitive conclusions can be drawn. For instance, some studies find that remittances encourage investment in human capital (Yang, 2008 ; Adams & Cuecuecha, 2010 ), while others find no such effect and suggest that families typically spend remittances on non-productive consumption goods (Chami, Fullenkamp, & Jahjah, 2003 ). Here we can only scratch the surface of the empirical work on remittances and economic outcomes. 7
Some of the most recent research in the field argues that remittances have a distinct political dimension, affecting regime support in developing countries and altering the conditions in which elections are held. Ahmed ( 2012 ), grouping remittances with foreign aid, argues that increased remittances allow autocratic governments to extend their tenure in office. These governments can strategically channel unearned government and household income to finance political patronage networks, which leads to a reduced likelihood of autocratic turnover, regime collapse, and mass protests against the regime. More recent research posits nearly the exact opposite: remittances are linked to a greater likelihood of democratization under autocratic regimes. Escriba-Folch, Meseguer, and Wright ( 2015 ) argue that since remittances directly increase household incomes, they reduce voter reliance on political patronage networks, undermining a key tool of autocratic stability.
Remittances may also play an important role in countries with democratic institutions, yet more research is needed to fully understand the conditions under which they matter and their substantive impact. Particularly, remittances may alter the dynamics of an election as an additional and external financial flow. There is evidence of political remittance cycles : the value of remittances spikes in the run-up to elections in developing countries. The total value of remittances to the average developing country increases by 6.6% during election years, and by 12% in elections in which no incumbent or named successor is running (O’Mahony, 2012 ). The effect is even larger in the poorest of developing countries. Finer-grained tests of this hypothesis provide additional support: using monthly and quarterly data confirms the existence of political remittance cycles, as well as using subnational rather than cross-national data (Nyblade & O’Mahony, 2014 ). However, these studies do not reveal why remittances spike, or what the effects of that spike are on electoral outcomes such as vote share, campaign financing, and political strategy.
Remittances represent a massive international financial flow that warrants more scholarly attention. While there are numerous studies on the relationship between remittances and key economic indicators, there remains much room for further work on their relationship to political outcomes in developing countries. Do remittances hasten the downfall of autocratic regimes, or do they contribute to autocratic stability? In democratic contexts, do remittances substantively influence electoral outcomes, and if so, which outcomes and how? Finally, do remittances prevent even more migration because they allow one “breadwinner from abroad” to provide for the household that remains in the homeland? While data limitations are formidable, these questions are important to the study of both international and comparative political economy.
Bilateral Trade
The argument that migrant or co-ethnic networks play an important role in international economic exchange is not novel. Greif ( 1989 , 1993 ) illustrates the role that the Maghrebi traders of the 11th century played in providing informal institutional guarantees that facilitated trade. This is but a single example. Cowen’s historical survey identifies not only the Phoenicians but also the “Spanish Jews [who] were indispensable for international commerce in the Middle Ages. The Armenians controlled the overland route between the Orient and Europe as late as the nineteenth century . Lebanese Christians developed trade between the various parts of the Ottoman empire” (Cowen, 1997 , p. 170). Rauch and Trindade ( 2002 ) provide robust empirical evidence linking the Chinese diaspora to patterns of imports and exports with their home country.
A variety of case studies document the importance of migrant networks in helping overcome problems of information asymmetries. In his study of Indian expatriates residing in the United States, Kapur ( 2014 ) documents how that community provides U.S. investors with a signal of the work ethic, labor quality, and business culture that exists in India. Likewise, Weidenbaum and Hughes ( 1996 ) chronicle the Bamboo Network—the linkages between ethnic Chinese living outside mainland China and their homeland—and how these linkages provide superior access to information and opportunities for investment.
Connections between migrant communities across countries affect cross-national investment even when these connections do not provide information about investment opportunities. In his work on the Maghrebi traders of the 11th century , Greif argues that this trading network was effective because it was able to credibly threaten collective punishment by all merchants if even one of them defected (Greif, 1989 , 1993 ). Grief shows that this co-ethnic network was able to share information regarding the past actions of actors (they could communicate a reputation)—something that was essential for the efficient functioning of markets in the absence of formal legal rules. Weidenbaum and Hughes reach a similar conclusion about the effectiveness of the Bamboo Network, remarking that “if a business owner violates an agreement, he is blacklisted. This is far worse than being sued, because the entire Chinese networks will refrain from doing business with the guilty party” (Hughes, 1996 , p. 51).
Migrants not only alter the flow of income by remitting to their countries of origin, but also influence patterns of international portfolio investment and FDI. Most existing literature on international capital allocation emphasizes monadic factors such as the importance of credible commitments and state institutional quality, failing to address explicitly dyadic phenomena that may also drive investment. Diaspora networks, in particular, facilitate cross-border investment in a number of ways. They foster a higher degree of familiarity between home and host countries, leading to a greater preference for investment in specific countries. Diaspora networks can also decrease information asymmetries in highly uncertain international capital markets in two ways. Firstly, they can provide investors with salient information about their homeland, such as consumer tastes, that can influence investment decision-making. Secondly, they can share knowledge about investment opportunities, regulation and procedures, and customs that decrease transaction costs associated with cross-border investment (Leblang, 2010 ). This place of importance for migrants suggests to the broader international political economy literature the importance of non-institutional mechanisms for channeling economic activity.
Although the hypothesized link between migrants and international investment has only recently been identified, the quantitative evidence available supports that hypothesis. Leblang ( 2010 ), using dyadic cross-sectional data, finds that diaspora networks “have both a substantively significant effect and a statistically significant effect on cross-border investment,” including international portfolio investment and FDI (p. 584). The effect of bilateral migratory flows correlates positively with the degree of information asymmetry: when informational imperfections are more pervasive in a dyad, migrants (especially the highly skilled) play a disproportionately large role in international capital allocation (Kugler, Levinthal, & Rapoport, 2017 ). Other quantitative studies find substantively similar results for FDI alone (e.g., Javorcik, Özden, Spatareanu, & Neagu, 2011 ; Aubry, Rapoport, & Reshef, 2016 ).
Many questions still remain unanswered. Firstly, does the effect of migrants on investment follow the waves of the global economy, or is it countercyclical as remittances have been shown to be? Secondly, how does this additional investment, facilitated by migrants, affect socioeconomic outcomes such as inequality, poverty, and economic development (Leblang, 2010 )? Does the participation of migrants lead to more successful FDI projects in developing countries because of their ability to break down information barriers? Within portfolio investment, do migrants lead to a preference for certain asset classes over others, and if so, what are the effects on bilateral and international capital markets? These are just a few directions in an area ripe for additional research.
Return Migration and Dual Citizenship
Besides financial flows, migrants themselves directly contribute to global flows of capital by returning to their countries of origin in large numbers. This phenomenon of return migration—or circular migration—can come in a few temporal forms, including long-term migration followed by a permanent return to a country of origin, or repeat migration in which a migrant regularly moves between destination and origin countries (Dumont & Spielvogel, 2008 ). While comparable data on return migration is scarce, some reports suggest that 20% to 50% of all immigrants leave their destination country within five years after their arrival (e.g., Borjas & Bratsberg, 1996 ; Aydemir & Robinson, 2008 ; Bratsberg, Raaum, & Sørlie, 2007 ; Dustmann & Weiss, 2007 ). An independent theoretical and empirical account of return migration does not yet exist in the literature and is beyond the scope of this paper. But in the rational actor framework, motivations to return home include a failure to realize the expected benefits of migration, changing preferences toward a migrant’s home country, achievement of a savings or other economic goal, or the opening of additional employment opportunities back home due to newly acquired experience or greater levels of economic development (Dumont & Spielvogel, 2008 ).
While most migration literature treats the country of origin as a passive actor that only provides the conditions for migration, new literature on return migration gives home country policies pride of place. Origin countries can craft policies that encourage diaspora re-engagement, incentivizing individuals to return home. Dual citizenship, for example, is an extension of extraterritorial rights, allowing migrants to retain full legal status in their home country. Dual citizenship “decreases the transaction costs associated with entering a host country’s labor market and makes it easier for migrants to return home” (Leblang, 2017 , p. 77). This leads migrants to invest their financial resources in the form of remittances back home as well as their valuable human capital. When states provide such extraterritorial rights, expatriates are 10% more likely to remit and 3% more likely to return home. Dual citizenship is also associated with a doubling of the dollar amount of remittances received by a home country (Leblang, 2017 ). These striking results suggest that in addition to the power of migrants to affect cross-border flows of money and people, countries of origin can also play a significant role.
Conclusion and Future Directions
This brief article has attempted to synthesize a broad range of literature from political science, economics, sociology, migration studies, and more to construct an account of international labor migration. To do so, the migratory process was broken down into distinct stages and decision points, focusing particularly on the decision to migrate, destination choice, and the re-engagement of migrants with their homeland. In doing so, the article also discussed the interlinkages of international migration with other fields of study in international political economy, including cross-border financial flows, trade, and investment. Through a multiplicity of approaches, we have gained a greater understanding of why people decide to move, why they decide to move to one country over another, and how and why they engage with the global economy and their homeland. Despite this intellectual progress, there remain many paths for future research at each stage of the migratory process; we highlight just a few of them here.
We know that income differentials, social ties, and local political conditions are important variables influencing the migration process. Yet the question remains: why do a small but growing number of people choose to leave while the overwhelming majority of people remain in their country of birth? Here, individual- or family-level subjective characteristics may be significant. There are a handful of observational studies that explore the relationship between subjective well-being or life satisfaction and the intention to migrate, with the nascent consensus being that life dissatisfaction increases the intention to migrate (Cai, Esipova, Oppenheimer, & Feng, 2014 ; Otrachshenko & Popova, 2014 ; Nikolova & Graham, 2015 ). But more research on intrinsic or subjective measures is needed to understand (a) their independent importance more fully and (b) how they interact with objective economic, political, and social factors. For instance, do those who are more optimistic migrate in larger numbers? Do minority individuals who feel they live in an environment in which diversity is not accepted feel a greater urge to leave home? Synthesizing these types of subjective variables and perceptions with the more prominent gravity-style models could result in a more complete picture of the international migration process.
For the “typical” migrant, one who is relatively less educated than the population in the chosen destination and does not have specialized skills, social networks are key to minimizing the risk of migrating and quickly tapping into economic opportunities in destination countries. Does this remain true for those who are highly educated? Although little empirical research exists on the topic, greater human capital and often-accompanying financial resources may operate as a substitute for the advantages offered by social networks, such as housing, overcoming linguistic barriers, and finding gainful employment. This would indicate that the “friends and family effect” is not as influential for this subset of migrants. Economic considerations, such as which destination offers the largest relative wage differential, or political considerations, such as the ease of quickly acquiring full citizenship rights, may matter more for the highly skilled. Neoclassical economic models of migration may best capture the behavior of migrants who hold human capital and who have the financial resources to independently migrate in a way that maximizes income or utility more broadly.
Since we have focused on international migration as a series of discrete decision points in this article, we have perhaps underemphasized the complexity of the physical migration process. In reality, migrants often do not pick a country and travel directly there, but travel through (perhaps several) countries of transit such as Mexico, Morocco, or Turkey along the way (Angel Castillo, 2006 ; Natter, 2013 ; Icduygu, 2005 ). There is little existing theoretical work to understand the role of transit countries in the migratory process, with much of it focusing on the potential for cooperation between destination and transit countries in managing primarily illegal immigration (Kahana & Lecker, 2005 ; Djajic & Michael, 2014 ; Djajic & Michael, 2016 ). Another related strand of the literature focuses on how wealthy destination countries are “externalizing” their immigration policy, encompassing a broader part of the migratory process than simply crossing a physically demarcated border (Duvell, 2012 ; Menjivar, 2014 ). But many questions remain, such as the following: how do we understand those who desire to enter, say, the United States, but instead relocate permanently to Mexico along the way? How do countries of transit handle the pressure of transit migrants, and how does this affect economic and political outcomes in these countries?
Finally, the focus of nearly all literature on international migration (and this article as a byproduct) implicitly views advanced economies as the only prominent destinations. However, this belies the fact that 38% of all migration stays within the “Global South” (World Bank, 2016 ). While there is certainly some literature on this phenomenon (see Ratha & Shaw, 2007 ; Gindling, 2009 ; Hujo & Piper, 2007 ), international political economy scholars have yet to sufficiently tackle this topic. The overarching research question here is: do the same push and pull factors that influence the decision to migrate and destination choice apply to those who migrate within the Global South? Do we need to construct new theories of international migration with less emphasis on factors such as wage differentials and political tolerance, or are these sufficient to understand this facet of the phenomenon? If we fail to answer these questions, we may miss explaining a significant proportion of international migration with its own consequences and policy implications.
- Abreu, A. (2012). The New Economics of Labor Migration: Beware of Neoclassicals Bearing Gifts. Forum for Social Economics , 41 (1), 46–67.
- Acosta, P. , Calderon, C. , Fajnzybler, P. , & Lopez, H. (2008). What Is the Impact of International Remittances on Poverty and Inequality in Latin America? World Development , 36 (1), 89–114.
- Adams, R., Jr. (2011). Evaluating the Economic Impact of International Remittances on Developing Countries Using Household Surveys: A Literature Review. Journal of Development Studies , 47 (6), 809–828.
- Adams, R., Jr. , & Cuecuecha, A. (2010). Remittances, Household Expenditure and Investment in Guatemala. World Development , 38 (11), 1626–1641.
- Adams, R., Jr. , & Page, J. (2005). Do International Migration and Remittances Reduce Poverty in Developing Countries? World Development , 33 (10), 1645–1669.
- Ahmed, F. Z. (2012). The Perils of Unearned Foreign Income: Aid, Remittances, and Government Survival. American Political Science Review , 106 (1), 146–165.
- Akerman, S. (1976). Theories and Methods of Migration Research. In H. Runblom & H. Norman (Eds.), From Sweden to America: A History of the Migration . Minneapolis: University of Minnesota Press.
- Angel Castillo, M. (2006). Mexico: Caught Between the United States and Central America . Migration Policy Institute.
- Aubry, A. , Rapoport, H. , & Reshef, A. (2016). Migration, FDI, and the Margins of Trade. Mimeo . Paris School of Economics.
- Aydemir, A. , & Robinson, C. (2008). Global Labour Markets, Return, and Onward Migration. Canadian Journal of Economics , 41 (4), 1285–1311.
- Böcker, A. (1994). Chain Migration over Legally Closed Borders: Settled Immigrants as Bridgeheads and Gatekeepers. Netherlands Journal of Social Sciences , 30 (2), 87–106.
- Borjas, G. J. (1989). Economic Theory and International Migration. International Migration Review , 23 (3), 457–485.
- Borjas, G. J. , & Bratsberg, B. (1996). Who Leaves? The Outmigration of the Foreign-Born. Review of Economics and Statistics , 41 (4), 610–621.
- Bratsberg, B. , Raaum, O. , & Sørlie, K. (2007). Foreign-Born Migration to and from Norway. In Ç. Özden & M. Schiff (Eds.), International Migration, Economic Development and Policy . New York: Palgrave Macmillan.
- Brücker, H. , & Schröder, P. J. H. (2011). Migration regulation contagion. European Union Politics , 12 (3), 315–335.
- Cai, R. , Esipova, N. , Oppenheimer, M. , & Feng, S. (2014). International Migration Desires Related to Subjective Well-Being. IZA Journal of Migration , 3 (8), 1–20.
- Castles, S. (2004). Why Migration Policies Fail. Ethnic and Racial Studies , 27 (2), 205–227.
- Cazzuffi, C. , & Modrego, F. (2018). Place of Origin and Internal Migration Decisions in Mexico. Spatial Economic Analysis , 13 (1), 1–19.
- Chami, R. , Fullenkamp, C. , & Jahjah, S. (2003). Are Immigrant Remittance Flows a Source of Capital for Development ? IMF Working Paper 03/189.
- Clark, X. , Hatton, T. J. , & Williamson, J. G. (2007). Explaining US Immigration, 1971–1998. Review of Economics and Statistics , 89 (2), 359–373.
- Clemens, M. A. (2014). Does Development Reduce Migration ? IZA Discussion Paper No. 8592.
- Collier, P. , & Hoeffler, A. (2014). Migration, Diasporas and Culture: An Empirical Investigation . Unpublished manuscript.
- Collyer, M. (2006). When Do Social Networks Fail to Explain Migration? Accounting for the Movement of Algerian Asylum-Seekers to the UK. Journal of Ethnic and Migration Studies , 31 (4), 699–718.
- Constant, A. , & Massey, D. S. (2002). Return Migration by German Guestworkers: Neoclassical versus New Economic Theories. International Migration , 4 0(4), 5–38.
- Cooray, A. , & Schneider, F. (2016). Does Corruption Promote Emigration? An Empirical Examination. Journal of Population Economics , 29 , 293–310.
- Cornelius, W. A. , & Tsuda, T. (2004). Controlling Immigration: The Limits of Government Intervention . Stanford, CA: Stanford University Press.
- Cowen, R. (1997). Global Diasporas: An Introduction . London: Routledge.
- Czaika, M. , & de Haas, H. (2016). The Effect of Visas on Migration Processes. International Migration Review , 51 (4), 893–926.
- Dao, T. H. , Docquier, F. , Parsons, C. , & Peri, G. (2018). Migration and Development: Dissecting the Anatomy of the Mobility Transition. Journal of Development Economics , 132 , 88–101.
- Dao, T. H. , Docquier, F. , Parsons, C. , & Peri, G. (2016). Migration and Development: Dissecting the Anatomy of the Mobility Transition . IZA Discussion Paper No. 10272.
- De Haas, H. (2007). Turning the Tide? Why Development Will Not Stop Migration. Development and Change , 38 , 819–841.
- Dimant, E. , Krieger, T. , & Meierrieks, D. (2013). The Effect of Corruption on Migration, 1985–2000. Applied Economics Letters , 20 (13), 1270–1274.
- Djajic, S. , & Michael, M. S. (2014). Controlling Illegal Immigration: On the Scope for Cooperation with a Transit Country. Review of International Economics , 22 (4), 808–824.
- Djajic, S. , & Michael, M. S. (2016). Illegal Immigration, Foreign Aid, and the Transit Countries. CESifo Economic Studies , 572–593.
- Dumont, J.-C. , & Spielvogel, G. (2008). Return Migration: A New Perspective. International Migration Outlook 2008 . OECD, 166–212.
- Dustmann, C. , & Okatenko, A. (2014). Out-Migration, Wealth Constraints, and the Quality of Local Amenities. Journal of Development Economics , 110 , 52–63.
- Dustmann, C. , & Weiss, Y. (2007). Return Migration: Theory and Empirical Evidence from the UK. British Journal of Industrial Relations , 45 (2), 236–256.
- Duvell, F. (2012). Transit Migration: A Blurred and Politicized Concept. Population, Space and Place , 18 , 415–427.
- Easterlin, R. A. (1961). Influences in European Overseas Emigration Before World War I. Economic Development and Cultural Change , 9 (3), 331–351.
- Epstein, G. (2008). Herd and Network Effects in Migration Decision-Making. Journal of Ethnic and Migration Studies , 34 (4), 567–583.
- Epstein, G. , & Gang, I. (2006). The Influence of Others on Migration Plans. Review of Development Economics , 10 (4), 652–665.
- Escriba-Folch, A. , Meseguer, C. , & Wright, J. (2015). Remittances and Democratization. International Studies Quarterly , 59 (3), 571–586.
- European Asylum Support Office . (2016). The Push and Pull Factors of Asylum-Related Migration: A Literature Review .
- Faist, T. (2000). The Volume and Dynamics of International Migration and Transnational Social Space . New York: Oxford University Press.
- Fitzgerald, J. , Leblang, D. , & Teets, J. C. (2014). Defying the Law of Gravity: The Political Economy of International Migration. World Politics , 66 (3), 406–445.
- Fussel, E. (2010). The Cumulative Causation of International Migration in Latin America. Annals of the American Academy of Political and Social Science , 630 , 162–177.
- Fussel, E. , & Massey, D. (2004). The Limits to Cumulative Causation: International Migration from Mexican Urban Areas. Demography , 41 (1), 151–171.
- Gindling, T. H. (2009). South–South Migration: The Impact of Nicaraguan Immigrants on Earnings, Inequality, and Poverty in Costa Rica. World Development , 37 (1), 116–126.
- Gould, J. D. (1979). European Inter-Continental Emigration 1815–1914: Patterns and Causes. Journal of European Economic History , 8 (3), 593–679.
- Greif, A. (1989). Reputation and Coalitions in Medieval Trade: Evidence on the Maghribi Traders. Journal of Economic History , 49 (4), 857–882.
- Greif, A. (1993). Contract Enforceability and Economic Institutions in Early Trade: The Maghribi Traders’ Coalition. American Economic Review , 83 (3), 525–548.
- Grogger, J. , & Hanson, G. H. (2011). Income Maximization and the Selection and Sorting of International Migrants. Journal of Development Economics , 95 , 42–57.
- Gupta, S. , Davoodi, H. , & Tiongson, E. (2001). Corruption and the Provision of Healthcare and Education Services. In A. Jain (Ed.), The Political Economy of Corruption . New York: Routledge.
- Hainmueller, J. , & Hiscox, M. J. (2010). Attitudes toward Highly Skilled and Low-Skilled Immigration: Evidence from a Survey Experiment. American Political Science Review , 104 (1), 61–84.
- Hainmueller, J. , & Hopkins, D. J. (2015). The Hidden Immigration Consensus: A Conjoint Analysis of Attitudes toward Immigrants. American Journal of Political Science , 59 (3), 529–548.
- Harris, J. R. , & Todaro, M. P. (1970). Migration, Unemployment and Development: A Two-Sector Analysis. American Economic Review , 60 (1), 126–142.
- Hatton, T. J. , & Williamson, J. G. (1994). What Drove the Mass Migrations from Europe in the Late Nineteenth Century? Population and Development Review , 20 (3), 533–559.
- Hatton, T. J. , & Williamson, J. G. (2011). Are Third World Emigration Forces Abating? World Development , 39 (1), 20–32.
- Haug, S. (2008). Migration Networks and Migration Decision-Making. Journal of Ethnic and Migration Studies , 34 (4), 585–605.
- Helbling, M. , Bjerre, L. , Römer, F. , & Zobel, M. (2017). Measuring Immigration Policies: The IMPIC-Database. European Political Science, 16 (1), 79–98.
- Helbling, M. , & Leblang, D. (forthcoming). Controlling Immigration? European Journal of Political Research .
- Hirschman, A. O. (1970). Exit, Voice, and Loyalty: Responses to Decline in Firms, Organizations, and Sates . Cambridge, MA: Harvard University Press.
- Hirsh, A. O. (1978). “Exit, Voice, and the State.” World Politics , 31 (1), 90–107.
- Hujo, K. , & Piper, N. (2007). South–South Migration: Challenges for Development and Social Policy. Development , 50 (4), 1–7.
- Icduygu, A. (2005). Transit Migration in Turkey: Trends, Patterns, and Issues . Euro-Mediterranean Consortium for Applied Research on International Migration Research Report 2005/04.
- Javorcik, B. , Özden, C. , Spatareanu, M. , & Neagu, C. (2011). Migrant Networks and Foreign Direct Investment. Journal of Development Economics , 94 , 231–241.
- Kahana, N. , & Lecker, T. (2005). Competition as a Track for Preventing Illegal Immigration. Economics of Governance , 6 , 33–39.
- Kapur, D. (2014). Political Effects of International Migration. Annual Review of Political Science , 17 , 479–502.
- Kugler, M. , Levinthal, O. , & Rapoport, H. (2017). Migration and Cross-Border Financial Flows . World Bank Policy Research Working Paper 8034.
- Leblang, D. (2010). Familiarity Breeds Investment: Diaspora Networks and International Investment. American Political Science Review , 104 (3), 584–600.
- Leblang, D. (2017). Harnessing the Diaspora: Dual Citizenship, Migrant Return, and Remittances. Comparative Political Studies , 50 (1), 75–101.
- Lewis, A. W. (1954). Economic Development with Unlimited Supplies of Labor. The Manchester School , 22 (2), 139–191.
- Lichter, D. T. (1983). Socioeconomic Returns to Migration among Married Women. Social Forces , 62 (2), 487–503.
- Light, I. , Bernard, R. B. , & Kim, R. (1999). Immigrant Incorporation in the Garment Industry of Los Angeles. International Migration Review , 33 (1), 5–25.
- Lokshin, M. , Bontch-Osmolovski, M. , & Glinskaya, E. (2010). Work-Related Migration and Poverty Reduction in Nepal. Review of Development Economics , 14 (2), 323–332.
- Massey, D. S. (1988). Economic Development and International Migration in Comparative Perspective. Population and Development Review , 14 (3), 383–413.
- Massey, D. S. (1990). Social Structure, Household Strategies, and the Cumulative Causation of Migration. Population Index , 56 (1), 3–26.
- Massey, D. S. , Arango, J. , Hugo, G. , Kouaouci, A. , Pellegrino, A. , & Taylor, J. E. (1993). Theories of International Migration: A Review and Appraisal. Population and Development Review , 19 (3), 431–466.
- Massey, D. S. , & Garcia España, F. (1987). The Social Process of International Migration. Science , 237 (4816), 733–738.
- Mauro, P. (1998). Corruption and the Composition of Government Expenditure. Journal of Public Economics , 69 , 263–279.
- Menjivar, C. (2014). Immigration Law Beyond Borders: Externalizing and Internalizing Border Controls in an Era of Securitization. Annual Review of Law and Social Science , 10 , 353–369.
- Messina, A. M. (2007). The Logics and Politics of Post-WWII Migration to Western Europe . Cambridge: Cambridge University Press.
- Mincer, J. (1978). Family Migration Decisions. Journal of Political Economy , 86 (51), 749–773.
- Miller, M. K. , & Peters, M. E. (2018). Restraining the Huddled Masses: Migration Policy and Autocratic Survival . British Journal of Political Science .
- Mo, P. H. (2001). Corruption and Economic Growth. Journal of Comparative Economics , 29 , 66–79.
- Money, J. (1999). Fences and Neighbors: The Political Geography of Immigration Control . Ithaca, NY: Cornell University Press.
- Morrison, D. R. , & Lichter, D. T. (1988). Migration and Female Employment. Journal of Marriage and Family , 50 (1), 161–172.
- Natter, K. (2013). The Formation of Morocco’s Policy Towards Irregular Migration (2000–2007): Political Rationale and Policy Processes. International Migration , 52 (5), 15–28.
- Nikolova, M. , & Graham, C. (2015). Well-Being and Emigration Intentions: New Evidence from the Gallup World Poll. Unpublished manuscript.
- Nyblade, B. , & O’Mahony, A. (2014). Migrants Remittances and Home Country Elections: Cross-National and Subnational Evidence. Studies in Comparative International Development , 49 (1), 44–66.
- O’Mahony, A. (2012). Political Investment: Remittances and Elections. British Journal of Political Science , 43 (4), 799–820.
- Ortega, F. , & Peri, G. (2013). The Effect of Income and immigration Policies on International Migration. Migration Studies , 1 (1), 47–74.
- Otrachshenko, V. , & Popova, O. (2014). Life (Dis)satisfaction and the Intention to Migrate: Evidence from Central and Eastern Europe. Journal of Socio-Economics , 48 , 40–49.
- Papademetriou, D. , & Sumption, M. (2011). Rethinking Points Systems and Employer-Based Selected Immigration . Migration Policy Institute.
- Peters, M. (2017). Trading Barriers: Immigration and the Remaking of Globalization . Princeton, NJ: Princeton University Press.
- Poprawe, M. (2015). On the Relationship between Corruption and Migration: Evidence from a Gravity Model of Migration. Public Choice , 163 , 337–354.
- Portes, A. (Ed.). (1995). The Economic Sociology of Immigration . New York: Russell Sage Foundation.
- Portes, A. , & Böröcz, J. (1989). Contemporary Immigration: Theoretical Perspectives on its Determinant and Modes of Incorporation. International Migration Review , 23 (3), 606–630.
- Rapoport, H. , & Docquier, F. (2006). The Economics of Migrants’ Remittances. In S.-C. Kolm & J. M. Ythier (Eds.), Handbook on the Economics of Giving, Altruism and Reciprocity . New York: Elsevier-North Holland.
- Ratha, D. , & Shaw, W. (2007). South-South Migration and Remittances . World Bank WP 102.
- Rauch, J. E. , & Trindade, V. (2002). Ethnic Chinese Networks in International Trade. Review of Economics and Statistics , 84 (1), 116–130.
- Ravenstein, E. G. (1885). The Laws of Migration. Journal of the Royal Statistical Society of London , 48 (2), 167–235.
- Sassen, S. (1995). Immigration and Local Labour Markets. In A. Portes (Ed.), The Economic Sociology of Immigration . New York: Russell Sage Foundation.
- Sassen, S. (1996). Losing Control? Sovereignty in the Age of Globalization . New York: Columbia University Press.
- Schultz, T. W. (1961). Investment in Human Capital. American Economic Review , 51 (1), 1–17.
- Severin, T. , & Martin, M. (2018). German Parties Edge Closer to Coalition with Migration Deal . Reuters, February 2.
- Sjaastad, L. A. (1962). The Costs and Returns of Human Migration. Journal of Political Economy , 70 (5), 80–93.
- Stark, O. (1984). Rural-To-Urban Migration in LDCs: A Relative Deprivation Approach. Economic Development and Cultural Change , 32 (3), 475–486.
- Stark, O. , & Bloom, D. E. (1985). The New Economics of Labor Migration. American Economic Review , 75 (2), 173–178.
- Stark, O. , & Levhari, D. (1982). On Migration and Risk in LDCs. Economic Development and Cultural Change , 31 (1), 191–196.
- Stark, O. , & Taylor, J. E. (1991). Migration Incentives, Migration Types: The Role of Relative Deprivation. The Economic Journal , 101 (408), 1163–1178.
- Stark, O. , & Yitzhaki, S. (1988). Migration as a Response to Relative Deprivation. Journal of Population Economics , 1 (1), 57–70.
- Taylor, J. E. (1999). The New Economics of Labour Migration and the Role of Remittances in the Migration Process. International Migration , 37 (1), 63–88.
- Todaro, M. P. (1969). A Model of Labor Migration and Urban Employment in Less Developed Countries. American Economic Review , 59 (1), 138–148.
- Tomaske, J. A. (1971). The Determinants of Intercountry Differences in European Emigration: 1881–1900. Journal of Economic History , 31 (4), 840–853.
- Transparency International . (2018). What is corruption
- United Kingdom Independence Party . (2015). UKIP Launches Immigration Policy .
- United Nations Population Division . (2012). Trends in Total Migrant Stock .
- United Nations Population Division . (2013). International Migration: Age and Sex Distribution. Population Facts, September.
- United States Citizenship and Immigration Services . (2018). H-1B Fiscal Year 2018 Cap Season .
- USA Today . (2014) (20 November). Full Text: Obama’s Immigration Speech .
- Vanderkamp, J. (1971). Migration Flows, Their Determinants and the Effects of Return Migration. Journal of Political Economy , 79 (5), 1012–1031.
- Vertovec, S. (2004). Migrant Transnationalism and Modes of Transformation. International Migration Review , 38 (3), 970–1001.
- Waldinger, R. (2008). Between “Here” and “There”: Immigrant Cross-Border Activities and Loyalties. International Migration Review , 42 (Spring), 3–29.
- Weidenbaum, M. , & Hughes, S. (1996). The Bamboo Network: How Expatriate Chinese Entrepreneurs are Creating a New Economic Superpower in Asia . New York: Martin Kessler Books.
- World Bank . (2016). Migration and Remittances Factbook 2016 . 3rd ed. Washington, DC: World Bank Group.
- Yang, D. (2008). International Migration, Remittances, and Household Investment: Evidence from Philippine Migrants’ Exchange Rate Shocks. The Economic Journal , 118 (528), 591–630.
- Yang, D. , & Martinez, C. (2006). Remittances and Poverty in Migrants Home Areas: Evidence from the Philippines. In C. Ozden & M. Schiff (Eds.), International Migration, Remittances and the Brain Drain . Washington, DC: World Bank.
- Zaiceva, A. , & Zimmerman, K. (2014). Migration and the Demographic Shift. IZA Discussion Paper #8743 .
- Zelinsky, W. (1971). The Hypothesis of the Mobility Transition. Geographical Review , 61 (2), 219–249.
1. Our use of the term international labor migration follows academic and legal conventions; we use the term migration to refer to the voluntary movement of people across national borders, either in a temporary or permanent fashion. This excludes any discussion of refugees, asylum seekers, or any other groups that are forced to migrate.
2. We do not have space in this article to delve into the theoretical and empirical work unpacking the effect of demographic characteristics—age, gender, marital status, household size, and so forth on the migration decision and on subsequent flows of migrants. For comprehensive reviews, see Lichter ( 1983 ), Morrison and Lichter ( 1988 ); United Nations Population Division ( 2013 ); and Zaiceva and Zimmerman ( 2014 ).
3. Zelinsky ( 1971 ) originally identified this relationship and termed it mobility transition curve . A wealth of empirical work supports Zelinsky’s descriptive theory in a number of contexts (see Akerman, 1976 ; Gould, 1979 ; Hatton & Williamson, 1994 ; and Dao et al., 2016 ).
4. For a review of the arguments as well as some empirical tests, see Miller and Peters ( 2018 ) and Docquier, Lodigiani, Rapoport, and Schiff ( 2018 ).
5. Transparency International. “What is corruption?”
6. For example, former United Kingdom Independence Party leader Nigel Farage has called for the United Kingdom to adopt an immigration system that only allows in highly skilled migrants (“UKIP launches immigration policy”). In 2014, US President Barack Obama emphasized that he wanted to attract international students to American universities and that they “create jobs, businesses, and industries right here in America” (USA Today: “Full text: Obama’s immigration speech”). A key issue in Germany’s 2018 government formation was the creation of skill-based migration laws (Severin & Martin, 2018 ).
7. For a more comprehensive review, see Rapoport and Docquier ( 2006 ); and Adams ( 2011 ).
Related Articles
- Space, Mobility, and Legitimacy
- Immigration and Foreign Policy
Printed from Oxford Research Encyclopedias, Politics. Under the terms of the licence agreement, an individual user may print out a single article for personal use (for details see Privacy Policy and Legal Notice).
date: 27 April 2024
- Cookie Policy
- Privacy Policy
- Legal Notice
- Accessibility
- [66.249.64.20|185.148.24.167]
- 185.148.24.167
Character limit 500 /500
Global migration in the twentieth and twenty-first centuries: the unstoppable force of demography
- Original Paper
- Published: 13 January 2021
- Volume 157 , pages 417–449, ( 2021 )
Cite this article
- Thu Hien Dao 1 , 2 ,
- Frédéric Docquier 3 ,
- Mathilde Maurel 4 , 5 , 6 &
- Pierre Schaus 7
10k Accesses
17 Citations
3 Altmetric
Explore all metrics
This paper sheds light on the global migration patterns of the past 40 years, and produces migration projections for the 21st century. To do this, we build a simple model of the world economy, and we parameterize it to match the economic and socio-demographic characteristics of the world in the year 2010. We conduct backcasting and nowcasting exercises, which demonstrate that our model fits very well the past and ongoing trends in international migration, and that historical trends were mostly governed by demographic changes. Then, we describe a set of migration projections for the 21st century. In line with the backcasts, our world migration prospects are mainly governed by socio-demographic changes. Using immigration restrictions or development policies to curb these pressures requires sealing borders or triggering unprecedented economic takeoffs in migrants’ countries of origin. Increasing migration is thus a likely phenomenon for the 21st century.
Similar content being viewed by others
What Is Globalisation?
Migration and Immigrants in Europe: A Historical and Demographic Perspective
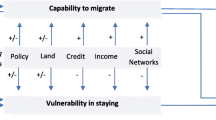
The impact of climate change on migration: a synthesis of recent empirical insights
Avoid common mistakes on your manuscript.
1 Introduction
Between 1960 and 2019, the worldwide stock of international migrants increased from 92 to 270 million, almost at the same pace as the world population. The worldwide share of migrants has fluctuated around 3% until 2010 (Ozden et al. 2011 ), with a rise since then until reaching 3.5% in 2019. In contrast, the foreign-born population increased more rapidly than the total population in high-income countries (HIC), boosting the average proportion of foreigners from 4.5 to 12.0% (+7.5 percentage points). A remarkable fact is that this change is mostly explained by the inflow of immigrants from less developed countries (LDC). The underlying root causes of this trend are known (population growth disparities between rich and poor countries, economic inequality, increased globalization, political instability, etc.). However, quantitatively speaking, little is known about their relative importance, and about the changing educational structure of past migration flows. Furthermore, the very same root causes are all projected to exert a strong influence on migration in the coming decades, while little is known about the predictability of future migration flows. This paper sheds light on these issues, addressing key questions such as: How have past income disparities, educational changes and population growth differentials shaped past migration flows? What are the pairs of countries responsible for large variations in low-skilled and high-skilled migration? How many potential migrants can be expected for the 21st century? How will future changes in education and productivity affect migration flows in general, and migration pressures to HIC in particular? Can development or visa policies be implemented to limit these flows?
To address these issues, we develop a simple and abstract model of the world economy that highlights the major mechanisms underlying migration decisions and wage inequality in the long term. It builds on a migration technology and a production technology, uses consensus specifications, and includes a limited number of parameters that can be calibrated to match the economic and socio-demographic characteristics of the world in the year 2010. Then, we conduct a set of backcasting and nowcasting experiments, which consists in simulating bilateral migration stocks retrospectively or for very recent years, and in comparing the backcasts and nowcasts with observed migration stocks. We show that our backcasts and nowcasts fit very well the observed migration data. This suggests that our model can be used to identify the main sources of variation and to predict long-run migration trends.
Analyzing counterfactual historical trends with constant distributions of income, education level or population, we highlight the key role of demography. In LDCs, the total stock of emigrants increased almost at the same pace as the total population, leading to small fluctuations of the average emigration rate between 2.6 and 3.0%. As part of this emigration process, the average propensity to emigrate from LDCs to HICs has increased by less than one percentage point over half a century. These changes have been governed by several factors (such as conflicts, rising income disparities, rising education level, or changing migration costs). They are too small to explain the threefold increase in immigration experienced in the North. Population growth differential is the main factor that reconciles stable emigration patterns in LDCs with “explosive” immigration patterns in HICs. Over the last 50 years, population growth has been systematically greater in developing countries. Footnote 1 Our numerical experiments confirm that most of the historical changes in international migration are explained by population growth differentials between countries. In particular, the world migration stocks would have virtually been constant if the population size of developing countries had not changed. Importantly, this does not mean that convergence in income and education does not matter (as apparent from our forecasting experiments). It simply means that convergence has been way to small to counteract the effect of demography, whatever the calibration of the technology and the size of human capital externalities.
We then feed our calibrated model with exogenous socio-demographic scenarios, and produce micro-founded projections of migration stocks by education level for the 21st century. We are aware that the future of migration can be influenced by many unforeseeable factors (e.g., emergence of new attractive immigration centers, economic and demographic effects of global pandemics, geopolitical cataclysms, etc.). For example, only time will tell whether the current Covid-19 pandemic will transform migration dynamics and in which direction. Hence, the objective is not to predict future migration but to identify how the traditional root drivers of migration will affect migration trends all other things being equal.
In line with the backcasting exercise, we find that the future trends in international migration are mostly governed by socio-demographic changes (i.e., changes in population size and in educational attainment). Focusing on OECD member states, we foresee a highly robust increase in their proportion of immigrants. The magnitude of the change is highly insensitive to the technological environment, and to the education scenario. In particular, a rise in schooling in developing countries increases the average propensity to emigrate but also reduces population growth rates; as far as migrant stocks are concerned, these effects are balancing each other. Changes in educational attainment strongly affect the skill composition of future migration flows but have little effect on their size. Overall, under constant immigration policies, the average share of immigrants in OECD countries increases from 12 to 25–28% during the 21st century. Given their magnitude, expected changes in immigration are henceforth referred to as migration pressures , although we do not make any value judgments about their desirability or about their welfare effects within the sending and receiving countries. This surge is mostly due to rising migration flows from sub-Saharan Africa, from the Middle East, and from a few Asian countries. Expected immigration pressures are greater in European countries (+21.2 percentage points) than in the United States (+14.3 percentage points). The greatest variations in immigration rates are observed in the United Kingdom, France, Spain; Canada is also strongly affected.
Curbing such migration pressures is difficult. Sealed borders are virtually needed to keep future migration stocks at their current level. Such drastic restrictive policies are unlikely to be implementable if the basic right to family reunification is respected. Turning our attention to development policies, we show that keeping their total emigration stock constant requires triggering unprecedented economic takeoffs.
Our paper speaks to the literature on long-term migration forces. The interdependencies between migration, population and income have rarely been accounted for in projection exercises. The demographic projections of the United Nations do not anticipate the economic and demographic forces that shape migration flows. Footnote 2 The Wittgenstein projections rely on a more complex methodology that consists of a set of probabilities to emigrate (or to immigrate) multiplied by the native population levels in the origin countries but imperfectly account for interdependencies between migration and economic variables (Lutz et al. 2017 ). Footnote 3 Hatton and Williamson ( 2003 ) examine the determinants of net emigration from Africa using a panel of 21 countries between 1977 and 1995, then subsequently use the regression estimates to predict an intensification of migration from Africa by the year 2025. From the receiving countries’ perspective, Hatton and Williamson ( 2011 ) identify the various drivers of emigration rates from developing countries to the United States from 1970 to 2004, and find abating signs of migration from Latin America and Asia to the United States while rising trend will continue in Africa. Hanson and McIntosh ( 2016 ) use empirical regressions to establish a link between changes in the demographic size/structure at origin and emigration flows. Ignoring changes in education levels and considering an exogenous economic environment, they show that the African migration pressures will mostly affect European countries until the mid-21st century.
The common feature of the present study is the use of past observations and exogenous demographic forecasts to project future migration. Our contribution is threefold. First, in terms of modeling, our paper builds on a general equilibrium framework which accounts for the interactions between migration decisions, productivity and wage disparities. Our migration projections are demographically and economically rooted. They result from a micro-founded migration technology and are compatible with the endogenous evolution of income disparities. Second, the use of a random utility specification allows allocating the world labor across multiple corridors as a function of the relative attractiveness of all destinations. Third, in terms of country coverage, our world-economy model includes the majority of countries in the world (i.e., 180 countries). The simulation results therefore offer a better overview of future global migration, although we acknowledge that migrant concentrate in a small number of corridors. Footnote 4
The remainder of the paper is organized as follows. Section 2 describes the model, defines its competitive equilibrium, and discusses its parameterization. Section 3 presents the results of the backcasting and nowcasting exercises. Forecasts are then provided in Sect. 4 . Finally, Sect. 5 concludes.
The model depicts an abstract economy with two classes of workers and J countries \((j=1,\ldots ,J)\) . The skill type s is equal to h for college graduates, and to l for the less educated. We first describe the migration technology, which determines the condition under which migration to a destination country j is profitable for type- s workers born in country i . We then describe the production technology, which determines wage disparities. The combination of endogenous migration decisions and equilibrium wages jointly determines the world distribution of income and the allocation of the world population. Finally, we discuss some important simplifying assumptions of the model and explain how we calibrate it to match migration, economic and socio-demographic data for the year 2010.
Migration technology At each period t , the number of working age natives of type s and originating from country i is denoted by \(N_{i,s,t}\) . Each native decides whether to emigrate to another country or to stay in their home country; the number of migrants from i to j is denoted by \(M_{ij,s,t}\) (hence, \(M_{ii,s,t}\) represents the number of non-migrants). After migration, the resident labor force of type s in country j is given by \(L_{j,s,t}\) .
Individual decisions to emigrate result from the comparison of discrete alternatives. To model them, we use a standard Random Utility Model (RUM) with a deterministic and a random component. The deterministic component is assumed to be logarithmic in income and to include an exogenous dyadic component. Footnote 5 At time t , the utility of a type- s individual born in country i and living in country j is given by:
where \(w_{j,s,t}\) denotes the wage rate attainable in the destination country j ; \(\widetilde{\gamma }\) is a parameter governing the marginal utility of income; \(v_{ij,s,t}\) stands for the non-wage income and amenities in country j (public goods, non-monetary amenities, and transfers minus taxes) and is netted from the legal and private costs of moving from i to j ; \(\xi _{ij,s,t}\) is the random taste component capturing heterogeneity in the preferences for alternative locations, in mobility costs, in assimilation costs, etc. This random component guarantees that individuals make heterogeneous location decisions, and cross-migration flows are possible.
The utility obtained when the same individual stays in his origin country is given by:
The random term \(\xi _{ij,s,t}\) is assumed to follow an iid extreme-value distribution of type I with scale parameter \(\mu \) . Footnote 6 Under this hypothesis, the probability that a type- s individual born in country i moving to country j is given by the following logit expression (McFadden 1984 ):
Hence, the emigration rate from i to j depends on the characteristics of all potential destinations k . The staying rates ( \(\frac{M_{ii,s,t}}{N_{i,s,t}}\) ) are governed by the same logit expression. It follows that the emigrant-to-stayer ratio is given by:
where \(\gamma \equiv \frac{\widetilde{\gamma }}{\mu }\) , the elasticity of migration choices to wage disparities, is a combination of preference and distribution parameters, and \(V_{ij,s,t}\equiv \left( \frac{v_{ij,s,t}}{v_{ii,s,t}}\right) ^{1/\mu }\) is a scale factor of the migration technology. The ratio of emigrants from i to j to stayers only depends on the characteristics of the two countries.
Production technology Income is determined based on an aggregate production function. Each country has a large number of competitive firms characterized by the same production technology and producing a homogenous good. The output in country j , \(Y_{j,t}\) , is a multiplicative function of the total quantity of labor in efficiency units, denoted by \(L_{j,T,t}\) , supplied by low-skilled and high-skilled workers by a “modified” total factor productivity (referred to as TFP henceforth), \(A_{j,t}\) . Footnote 7 Following the recent literature on labor markets, immigration and growth, Footnote 8 we assume that labor in efficiency units is a CES function of the number of college-educated and less educated workers employed. We have:
where \(\theta _{j,s,t}\) is the country and time-specific value share parameter for workers of type s (such that \(\theta _{j,h,t}+\theta _{j,l,t}=1\) ), and \(\sigma \) is the common elasticity of substitution between the two groups of workers.
Firms maximize profits and the labor market is competitive. The equilibrium wage rate for type- s workers in country j is equal to the marginal productivity of labor:
Hence, the wage ratio between college graduates and less educated workers is given by:
As long as this ratio is greater than one, a rise in human capital increases the average productivity of workers. Furthermore, greater contributions of human capital to productivity can be obtained by assuming technological externalities. Two types of technological externality are factored in. First, we consider a simple Lucas-type, aggregate externality (Lucas 1988 ) and assume that the TFP scale factor in each sector is a concave function of the skill-ratio in the resident labor force. This externality captures the fact that educated workers facilitate innovation and the adoption of advanced technologies. Its size has been the focus of many recent articles and has generated a certain level of debate. Using data from US cities (Moretti 2004 ) or US states (Acemoglu and Angrist 2000 ; Iranzo and Peri 2009 ), some instrumental-variable approaches give substantial externalities (Moretti 2004 ) while others do not (Acemoglu and Angrist 2000 ). In the empirical growth literature, there is evidence of a positive effect of schooling on innovation and technology diffusion (Benhabib and Spiegel 1994 ; Caselli et al. 2006 ; Ciccone and Papaioannou 2009 ). In parallel, another set of contributions highlights the effect of human capital on the quality of institutions (Castelló-Climent 2008 ; Bobba and Coviello 2007 ; Murtin and Wacziarg 2014 ). We write:
where \(\lambda _{t}\) captures the worldwide time variations in productivity (common to all countries), \(\overline{A}_{j}\) is the exogenous country-specific component of TFP in country j (reflecting exogenous factors such as arable land, climate, geography, etc.), and \(\epsilon \) is the elasticity of TFP to the skill ratio.
Second, we assume skill-biased technical change. As technology improves, the relative productivity of high-skilled workers increases (Acemoglu 2002 ; Restuccia and Vandenbroucke 2013 ). For example, Autor et al. ( 2003 ) show that computerization is associated with a declining relative demand in industry for routine manual and cognitive tasks, and increased relative demand for non-routine cognitive tasks. The observed relative demand shift favors college versus non-college labor. We write:
where \(\overline{Q}_{j}\) is the exogenous country-specific component of the skill bias in productivity in country j , and \(\kappa \) is the elasticity of the skill bias to the skill ratio.
Competitive equilibrium The link between the native and resident population is tautological:
The dynamics of the world economy is governed by a succession of temporary equilibria defined as:
Definition – For a set \(\left\{ \gamma ,\sigma ,\epsilon ,\kappa ,\lambda _{t}\right\} \) of common parameters, a set \(\left\{ \overline{A}_{j},\overline{Q}_{j}\right\} _{\forall j}\) of country-specific parameters, a set \(\left\{ V_{ij,s,t}\right\} _{\forall i,j,s}\) of bilateral (net) migration costs, and for given distribution of the native population \(\left\{ N_{j,s,t}\right\} _{\forall j,s}\) , a temporary competitive equilibrium for period t is an allocation of labor \(\left\{ M_{ij,s,t}\right\} _{\forall i,j,s}\) and a vector of wages \(\left\{ w_{j,s,t}\right\} _{\forall j,s}\) satisfying (i) utility maximization conditions, Eq. ( 4 ), (ii) profit maximization conditions, Eq. ( 6 ), (iii) technological constraints, Eqs. ( 8 ) and ( 9 ), and (iv) the aggregation constraints, Eq. ( 10 ).
A temporary equilibrium allocation of labor is characterized by a system of \(2\times J\times (J+1)\) i.e., \(2\times J\times (J-1)\) bilateral ratio of migrants to stayers, \(2\times J\) wage rates, and \(2\times J\) aggregation constraints. In the next sub-sections, we use data for 180 countries (developed and developing independent territories) and explain how we parameterize our system of 65,160 simultaneous equations per period. Once properly calibrated, this model can be used to conduct a large variety of numerical experiments.
Parameterization for the year 2010 The year 2010 is the most recent year for which skill-specific matrices of bilateral migration stocks are available. The model can be parameterized to match the economic and socio-demographic characteristics of 180 countries as in the year 2010.
Regarding the production technology, we collect data on GDP in PPP values ( \(Y_{j,2010}\) ) from the Maddison’s project described in Bolt and Van Zanden ( 2014 ), and combine them with data on the size and structure of the labor force from the Wittgenstein Centre for Demography and Global Human Capital ( \(L_{j,s,2010}\) ), and data on the wage ratio between college graduates and less educated workers, \(\frac{w_{j,h,2010}}{w_{j,l,2010}}\) , from Hendricks ( 2004 ). Footnote 9 The labor force is proxied by population aged 25 to 64. Using these data, we proceed in three steps to calibrate the production technology. First, in line with the labor market literature (Ottaviano and Peri 2012 ; Angrist 1995 ), we assume that the elasticity of substitution between college-educated and less educated workers, \(\sigma \) , is equal to 2 or 3. Second, for a given \(\sigma \) , we calibrate the ratio of value shares, \(\frac{\theta _{j,h,2010}}{\theta _{j,l,2010}}\) , as a residual from Eq. ( 7 ) to match the observed wage ratio. Since \(\theta _{j,h}+\theta _{j,l}=1\) , this determines both \(\theta _{j,h,2010}\) and \(\theta _{j,l,2010}\) as well as the quantity of labor per efficiency unit, \(L_{j,T,2010}\) , defined in Eq. ( 5 ). Third, we use Eq. ( 5 ) and calibrate the TFP level, \(A_{j,2010}\) , to match the observed GDP and we normalize \(\lambda _{2010}\) to unity (without loss of generality). When simulating the model backward or forward (see below), we consider several variants for \(\epsilon \) and for \(\kappa \) , allowing the ratio of value shares and the TFP level vary with the skill ratio. When all technological parameters are calibrated, we use Eq. ( 6 ) to proxy the wage rates for each skill group.
As for the migration technology, we use the DIOC-E database of the OECD. DIOC-E builds on the Database on Immigrants in OECD countries (DIOC) described in Arslan et al. ( 2015 ) where migrants are defined as foreign-born population. The data are collected by country of destination and are mainly based on population censuses or administrative registers. The DIOC database provides detailed information on the country of origin, demographic characteristics and level of education of the population of 34 OECD member states. DIOC-E extends the latter by characterizing the structure of the population of 86 non-OECD destination countries. Focusing on the populations aged 25 to 64, we thus end up with matrices of bilateral migration from 180 origin countries to 120 destination countries (34 OECD + 86 non-OECD countries) by education level, as well as proxies for the native population ( \(N_{i,s,2010}\) ). We assume that immigration stocks in the 60 missing countries are zero, which allows us to compute comprehensive migration matrices. Footnote 10
Regarding the elasticity of bilateral migration to the wage ratio, \(\gamma \) , Bertoli and Moraga Fernandez-Huertas ( 2013 ) find a value between 0.6 and 0.7. We use 0.7 as a benchmark but other values (0.5 or 1.0) are considered in our robustness checks. Finally, we calibrate \(V_{ij,s,2010}\) as a residual of Eq. ( 4 ) to match the observed ratio of bilateral migrants to stayers. In Appendix A, we show that the calibrated scale factors are negatively correlated with standard determinants of migration costs such as geographic distance, migration policy, etc.
In sum, the migration and technology parameters are such that our model perfectly matches the world distribution of income, the world population allocation and skill structure as well as bilateral migration stocks as of the year 2010.
Caveats Our model relies on simplifying assumptions that may, at first, seem unrealistic but do not, arguably, invalidate our results. Firstly, although one period is meant to represent 10 years, we assume a “drawing-with-replacement” migration process. This means that we ignore path dependency in migration decisions (i.e., having migrated to country j at time t influences the individual location at time \(t+1\) ), as if all migrants returned to their home country at the end of each period t , and made new migration decisions at the beginning of period \(t+1\) . This simplification is compatible with the existence of both temporary and permanent migrants although it disregards differences between them. This “drawing-with-replacement” migration hypothesis allows us to model the trends in migration stocks as a simple function of concomitant population trends and without keeping track of the entire log of past migration flows. Footnote 11 This is why we can compute the competitive equilibrium as a succession of temporary equilibria.
Secondly, the migration technology is calibrated using migration stock data, which are assumed to reflect the long-run migration equilibrium. This implies that the calibrated values for \(V_{ij,s,t}\) implicitly account for net amenity differentials, private and visa costs of migration, and network effects (i.e., the effect of past migration stocks on migration flows). Migration costs are expressed as a disutility. They include monetary moving costs (e.g., passport and travel costs) a well as utility-loss equivalents of migration quotas (similar to tariff equivalent of non-tariff barriers in trade). These costs are treated as exogenous. Footnote 12
Thirdly, the model is calibrated to match labor force and migration stock data by education level, assuming that these stocks are proxied by the number of individuals aged 25–64. We thus consider individuals aged 25–64 as a homogenous group and abstract from the heterogeneity in the propensity to migrate across age groups. We are aware that individuals aged 20–34 are more migratory than older age groups (Hatton and Williamson 1998 ; UNDESA 2013 ) due to higher present values of migration in intertemporal utility function (Hatton and Williamson 2011 ; Djajic et al. 2016 ). However, in Appendix A, we show that the past variations in the population aged 20–34 are highly correlated with past variations in the population aged 25–64. The correlation equals 0.77 over the period 1970–2010 and the slope of the regression line is close to unity. Similarly, when using the medium variant of the UN population projections, this correlation equals 0.70 over the long period of 2010–2100. Hence, variations in the population aged 25 to 64 capture well the migration pressures related to demographic factors.
Fourthly, our model without physical capital features a globalized economy with a common international interest rate. This hypothesis is in line with Kennan ( 2013 ) or Klein and Ventura ( 2009 ) who assume that capital “chases” labor. Footnote 13 Eq. ( 5 ) can be seen as the reduced form of a system of two equations, a first-stage production function with capital and labor (e.g., in the Cobb-Douglas case, \(Y_{j,t}= \tilde{A}_{j,t} K_{j,t}^{\alpha } L_{j,T,t}^{1-\alpha }\) where \(\tilde{A}_{j,t}\) is the actual TFP level of country j at time t ) and an arbitrage condition implying that the interest rates are equalized across countries ( \(R_{t}= \tilde{A}_{j,t} \alpha K_{j,t}^{\alpha -1} L_{j,T,t}^{1-\alpha }\) ). Substituting the arbitrage equation into the first-stage production function gives Eq. ( 5 ), in which the “modified” TFP level is defined as \(A_{j,t} = \tilde{A}_{j,t}^{1/(1-\alpha )}(\alpha /R_{t})^{\alpha /(1-\alpha )}\) . Clearly, changes in the international interest rate \(R_{t}\) or multiplicative changes in TFP level (due to exogenous technological progress, \(\lambda _{t}\) ) have no effect on the ratio of “modified” TFP and on the ratio of wage rates between two countries. From Eq. ( 4 ), they have no effect on migration behaviors.
3 Backcasting and nowcasting
Our first objective is to gauge the ability of our model to replicate aggregate historical and contemporaneous migration data as well as to backcast the educational structure of past migration stocks. In our backcasting exercises, we use the model to simulate retrospectively bilateral migration stocks by education level, and compare the results with proxies of observed migration stocks for the years 1970, 1980, 1990 and 2000. Footnote 14 Similarly, we test the performance of our nowcasts by comparing the data predicted by our model for the year of 2020 with the most recent migration data of 2019 obtained from the United Nations Global migration database. To do so, we feed the model with two types of inputs, namely skill-specific wage rates and levels of the native (pre-migration) population for all countries. In the nowcasting simulation, we use the population estimates for 2020 from Lutz et al. ( 2017 ), as in the forecasting section. Footnote 15 These backasting and nowcasting exercises shed light on the relevance our parameterization strategy (i.e. what value for \(\gamma \) , \(\sigma \) and \(\kappa \) should be favored?) and on the role of socio-demographic and technological changes in explaining the aggregate variations in past migration.
Worldwide migration stocks Figure 1 illustrates the backcasting and nowcasting results obtained for the worldwide stock of working-age migrants in our benchmark scenario with \(\gamma = 0.7\) , \(\sigma = 2\) , and \(\kappa = 0.214\) . In particular, Fig. 1 a compares the evolution of actual and predicted worldwide migration stocks by decade for the year 1970 to 2020. For the \(180\times 120\) corridors, the (rescaled) data gives a stock of 55 million migrants aged 25 to 64 in 1970, 120 million migrants in 2010, and 141 million migrants in 2020. Our benchmark model almost exactly matches this evolution. By construction, the model perfectly matches the 2010 data. Interestingly, it almost perfectly matches the data for the years 1970 and 2020, Footnote 16 while it slightly overestimates the stock in 1980, 1990 and 2000.
We see this result as evidencing the realism of the model. In Appendix C.2, we check whether the model’s aggregate performance depends on the parameter set. We produce backcasts under 18 scenarios, considering three possible values for \(\gamma \) (0.5, 0.7 and 1.0), two possible values for \(\sigma \) (2.0 and 3.0) and three possible values for \(\kappa \) (0, half the estimated elasticity of the value shares to the skill ratio, and 100% of the estimated elasticity). Figure C.1 shows that the model with \(\gamma = 0.7\) gives the best fit. Assuming \(\gamma = 1.0\) leads to an overestimation of past migrant stocks, while \(\gamma = 0.5\) leads to an underestimation. By contrast, our backcasts are fairly robust to the choice of \(\sigma \) , the elasticity of substitution between workers, and of \(\kappa \) , the skill-biased externality. Although technological variants drastically affect within-country income disparities (in particular, the wage ratio between college graduates and the less educated), they have negligible effects on aggregate migration stocks. This is due to the fact that income disparities are mostly governed by between-country inequality (i.e., by the TFP levels, which are calibrated under each scenario to match the average levels of income per worker), and that the worldwide proportion of college graduates is so small that changes in their migration propensity have negligible effects on the aggregate.
Coming back to the benchmark scenario with \(\gamma =0.7\) , \(\sigma =2\) and \(\kappa =0.214\) , Fig. 1 b compares our backcasts for the years 1970 to 2000 with counterfactual retrospective simulations. The first counterfactual neutralizes demographic changes that occurred between 1970 and 2010; it assumes that the size of the working age population is kept constant at the 2010 level in all countries. The second counterfactual neutralizes the changes in education; it assumes that the share of college graduates is kept constant in all countries. The third counterfactual neutralizes the changes in income disparities; it assumes constant wage rates in all countries.
On the one hand, the simulations reveal that past changes/rises in education marginally increased the worldwide migration stock, while the past changes/decreases in income inequality marginally reduced it. These effects are quantitatively small, which does not mean that cross-country disparities in education and income have no influence on migration trends. It is because past changes in human capital has been limited in poor countries, and income disparities have been stable for the last fifty years (with the exception of emerging countries). Hence, the contribution of these two factors has been limited.
On the other hand, Fig. 1 b shows that demographic changes explains a large amount of the variability in migration stocks. The number of worldwide migrants in 1970 would have almost been equal to the current stocks (in fact, it would have been 2% smaller only) if the population size of each country had been identical to the current level. This confirms that past changes in aggregate migrant stocks were predominantly governed by population growth in LDCs and demographic imbalances. Indeed, the population ratio between developing and high-income countries increased from 3.5 in 1970 to 5.5 in 2010.
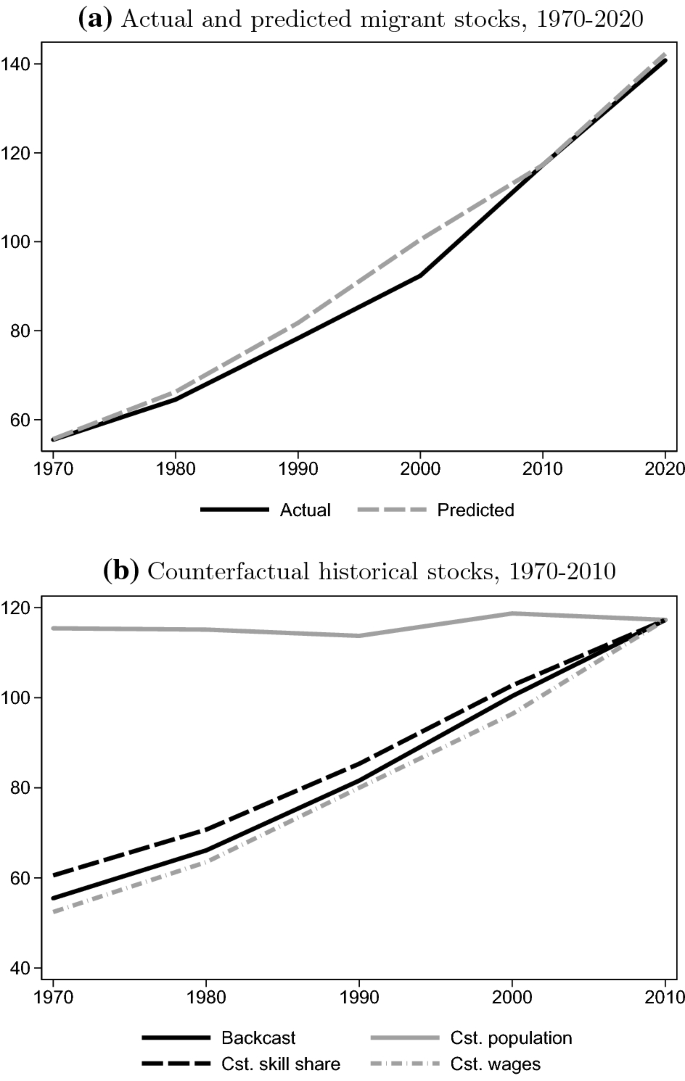
Actual and predicted migrant stocks (in million). a Actual and predicted migrant stocks, 1970–2020. b Counterfactual historical stocks, 1970–2010. Note : Fig. a compares actual and predicted stocks of working-aged migrants. To obtain the actual working-age migrant stocks, we use the share of working-aged migrants in 2010 to rescale the total migrant stocks from Ozden et al. ( 2011 ) for the years 1960–2000 and from the UNPOP database for the year 2020. Predicted migrants stocks are computed from the scenario in which \(\gamma = 0.7\) , \(\sigma = 2\) , and \(\kappa = 0.214\) . In Fig. b , the first counterfactual keeps the size of the working-age population constant at the 2010 level in all countries. The second counterfactual keeps the share of college graduates constant at the 2010 level in all countries. The third counterfactual keeps wage rates constant at the 2010 level in all countries
Bilateral migration backcasts We now investigate the capacity of the model to match the decadal distributions of immigrant stocks by destination, and the decadal distributions of emigrant stocks by origin. Figure 2 provides a graphical visualization of the goodness of fit by comparing the observed and simulated bilateral stocks of immigrants and emigrants for each decade. Footnote 17
By construction, as the observed past immigration stocks of all ages are scaled to match the working-age ones in 2010, the predicted immigrant stocks are perfectly matched in that year. For previous years, the correlation is unsurprisingly smaller; it decreases with the distance from the year 2010. This is because our model does neither identify past variations in migration policies (e.g. the Schengen agreement in the European Union, changes in the H1B visa policy in the US, the points-system schemes in Canada, Australia, New Zealand, guest worker programs in the Persian Gulf, etc.) nor past changes in net amenities and non-pecuniary push/pull factors (e.g., conflicts, political unrest, etc.). The biggest gaps between the observed and predicted migration stocks recorded in our data come from the non-consideration of the collapse of the Soviet Union, the end of the French-Algerian war and of the Vietnam war, the conflict between Cuba and the US. In addition, the model imperfectly predicts the evolution of intra-EU migration, the evolution of labor mobility to Persian Gulf countries, the evolution of migrant stocks from developing countries to the US, Canada and Australia, and the evolution of immigration to Israel (especially the flows of Russian Jews after the late 1980—the so-called Post-Soviet aliyah).
Yet, the scatterplots on Fig. 2 show high correlations between the observed and predicted bilateral migration volumes throughout all decades. The lowest reported R-squared are 0.76 for immigrant stocks and 0.69 for emigrant stocks in 1970. These numbers reach 0.93 and 0.90, respectively, for the years 2000 and 2020. This demonstrates that the constant \(V_{ij}\) hypothesis does a good job on average despite big changes in immigration policies in the past whose restrictiveness was either increasing or decreasing. Footnote 18 In the former case, it may be that stricter entry policies have been balanced by increasing network effects.
As far as the technological variants are concerned, Table C.1 in Appendix C.2 confirms that they play a negligible role. The correlation between variants is always around 0.99. The variant with \(\sigma =2\) and no skill-biased externality marginally outperforms the others in replicating immigrant stocks; the one with \(\sigma =3\) and with skill biased externalities does a slightly better job in matching emigrant stocks. Hence, the backcasting exercise shows that our model does an excellent job in explaining the long term evolution of migration stocks; however, it does not help eliminate irrelevant technological scenarios. Regressing the log of actual immigration stocks on their predicted levels per decade, we obtain regression coefficients that are not significantly different from unity, with standard errors around 0.004 for the years 2000 and 2020, around 0.006 for 1990, 0.008 for 1980 and 0.010 for 1970. Roughly speaking, this means that the 95% confidence interval surrounding our predictions increases by about 0.4% per decade departing from 2010. For 1970 (4 decades before the calibration year), there is a 95% probability that the observed migration stocks are comprised between 98 and 102% of our predicted levels.
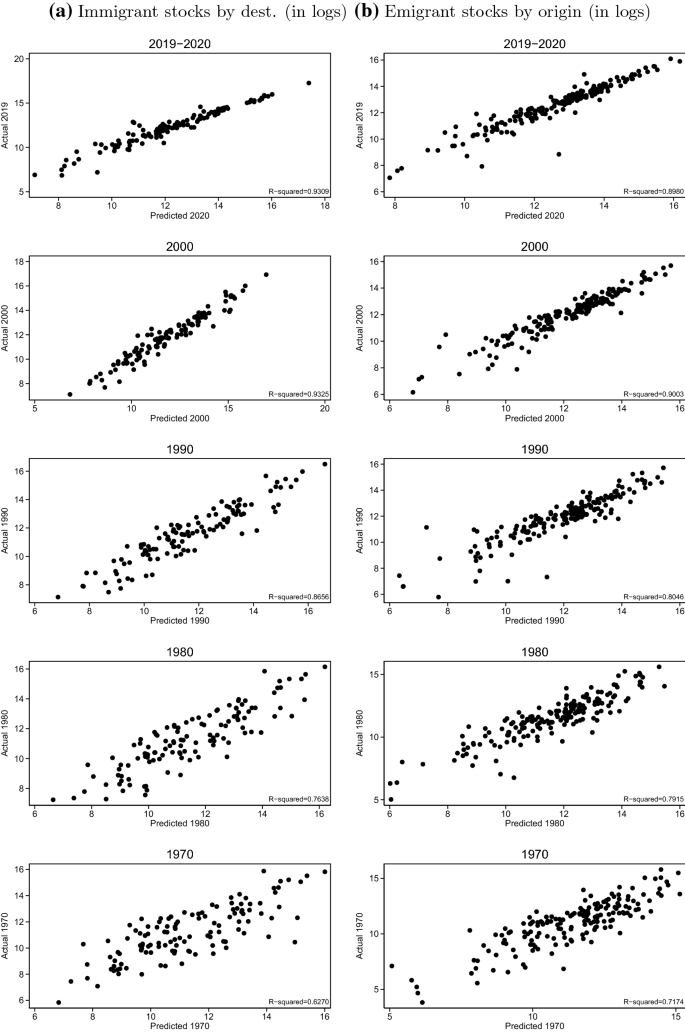
Comparison between actual and predicted migrant stocks, 1970–2020 a Immigrant stocks by dest. (in logs). b Emigrant stocks by origin (in logs). Note : Authors’ computations based on the variant with \(\gamma =0.7\) , \(\sigma =2\) and \(\kappa = 0.214\)
Backcasts by skill group Data on migration stocks by educational level are available for a few decades only. The DIOC database of Arslan et al. ( 2015 ) provides homogeneous data for the census rounds 2000 and 2010. In Appendix C.2, we show that our model replicates well the changes in the educational structure of migration stocks observed between two census rounds. As migration data by skill group do not exist for earlier periods, we use our model to backcast the global net flows of college-educated and less educated workers between regions. We use the scenario with \(\gamma =0.7\) , \(\sigma =2\) and with full skill-biased externalities. Footnote 19 For each pair of countries, we compute the net flow as the difference between the stock of migrants in 2010 and that of 1970, \(\Delta M_{ij,s}\equiv M_{ij,s,2010}-M_{ij,s,1970}\) . These net flows form the matrix \({\mathcal {M}}\) . On Fig. 3 , we group countries into eight regions and use circular ideograms following Krzywinski et al. ( 2009 ) to highlight the major components of \({\mathcal {M}}\) . Net flows are colored according to their origin, and their width is proportional to their size. The direction of the flow is captured by the colors of the outside (i.e., country of origin) and inside (i.e., country of destination) borders of the circle.
We also characterize the clusters of origins and destinations that caused the greatest variations in global migration between 1970 and 2010. Using the same matrix of migration net flows as above (denoted by \({\mathcal {M}}\) and including the \(J\times J\) net flows between 1970 and 2010, \(\Delta M_{ij,s}\) ). We use the Max-Sum Submatrix algorithm defined in Appendix B, a standard tool in applied mathematics which is used to identify the sub-matrix with a fixed dimension \(o\times d\) that maximizes the total migration net flows (i.e., that captures the greatest fraction of the worldwide variations in migration stocks).
Figure 3 a focuses on the net flows of less educated workers. The net flow of low-skilled immigrants equals 35.2 million over the 1970–2010 period. The ten main regional corridors account for 79% of the total, and industrialized regions appear 6 times as a main destination. By decreasing the order of magnitude, they include Latin America to North America (27.6%), migration within the South and East Asian region (13%), from MENA to Europe (6.8%), migration between former Soviet countries (5.2%), migration within sub-Saharan Africa (5.1%), intra-European movements (4.5%), Latin America to Europe (4.4%), South and East Asia to Western offshoots (4.2%), Others to Europe (4.0%), and migration between Latin American countries (4.0%). It is worth noting the low-skilled mobility from sub-Saharan Africa to Europe is not part of the top ten: it only represents 3.8% of the total (the 11th largest regional corridor). Applying the Max-Sum submatrix problem to the net flows of low-skilled migrants, we can identify the 25 origins and the 25 destinations (625 entries) that account for 64% of the worldwide net flows of low-skilled migrants between 1970 and 2010.
The 25 main destinations (in alphabetical order) are: Australia, Austria, Belarus, Belgium, Canada, Dominican Republic, France, Germany, Greece, Hong Kong, India, Israel, Italy, Kazakhstan, Malaysia, Nepal, the Netherlands, Oman, Russia, Saudi Arabia, Spain, Thailand, the United Kingdom, the United States, and Venezuela.
The 25 main origins (in alphabetical order) are: Albania, Algeria, Bangladesh, Colombia, the Dominican Republic, Ecuador, Guatemala, Haiti, India, Indonesia, Jamaica, Kazakhstan, Mexico, Morocco, Myanmar, Pakistan, the Philippines, Poland, Romania, Russia, Slovenia, Turkey, Ukraine, Uzbekistan, and Vietnam.
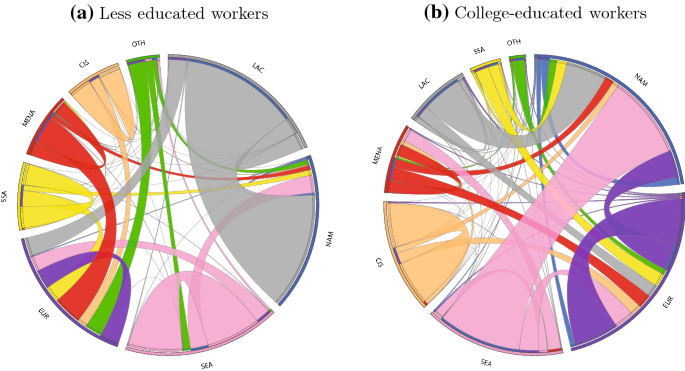
Global migration net flows, 1970–2010. a Less educated workers. b College-educated workers. Regions: Europe (in dark blue), Western offshoots (NAM in light blue)(These include the United States, Canada, Australia and New Zealand),the Middle East and Northern Africa (MENA in red), sub-Saharan Africa (SSA in yellow), South and East Asia including South and South-East Asia (SEA in pink), the former Soviet countries (CIS in orange), Latin America and the Caribbean (LAC in grey), and Others (OTH in green). Net flows are colored according to their origin, and their width is proportional to their size. The direction of the flow is captured by the colors of the outside (i.e., country of origin) and inside (i.e., country of destination) borders of the circle (color figure online)
Figure 3 b represents the net flows of college graduates. The net flow of high-skilled immigrants equals 27.6 million over the 1970–2010 period. The ten main regional corridors account for 74% of the total. A major difference with the low-skilled is that industrialized regions appear 9 times as a main destination, at least if we treat the Persian Gulf countries (as part of the MENA region) as industrialized. By decreasing order of magnitude, the top-10 includes South and East Asia to Western offshoots (19.8% of the total), intra-European movements (10.7%), migration between former Soviet countries (10.5%), Latin America to Western offshoots (9.7%), Europe to Western offshoots (6.5%), South and East Asia to Europe (4.6%), MENA to Europe (3.3%), sub-Saharan Africa to Europe (3.2%), South and East Asia to the MENA (3.1%), and Latin America to Europe (2.9%). Applying the Max-Sum submatrix problem to the net flows of low-skilled migrants, the set of main destinations mostly includes high-income countries. The 625 entries of the Max-sum submatrix account for 55% of the worldwide net flow of college-educated migrants between 1970 and 2010.
The 25 main destinations (in alphabetical order) are: Australia, Austria, Belarus, Canada, France, Germany, India, Ireland, Israel, Italy, Japan, Kazakhstan, the Netherlands, New Zealand, Oman, Russia, Saudi Arabia, Spain, Sweden, Switzerland, Thailand, Ukraine, the United Arab Emirates, the United Kingdom, and the United States.
The 25 main origins (in alphabetical order) are: Algeria, Bangladesh, Canada, China, Colombia, Egypt, France, Germany, India, Iran, Japan, Kazakhstan, Mexico, Morocco, Pakistan, the Philippines, Poland, Romania, Russia, South Korea, Ukraine, the United Kingdom, the United States, Uzbekistan, and Vietnam.
Overall, as shown in the left panel of Table 2 below, the model predicts that the proportion of college graduates in the total immigrant stocks of the OECD countries rose from 13.3 to 34.0% between 1970 and 2010. It increased from 10.8 to 28.4% in EU15, from 13.9 to 34.4% in the United States. Larger changes were observed in Canada, Australia or the United Kingdom.
4 Forecasting
We now use the parameterized model to produce projections of migration stocks and income disparities for the 21st century. Availability of population projections until the end of the century allows us to systematically predict migration for that entire period, though the longer the distance from 2010, the more uncertain are our projections. Extrapolating insights from our backcasting exercice, there would be a 95% probability that actual migration stocks will be comprised between 98 and 102% of our predicted levels 40 years after the calibration year (i.e., in 2050), and between 95 and 105% of our predicted stock around the year 2100 (as the confidence interval increases by 0.4% per decade). This is at least what we obtain when the spatial and socio-demographic structure of the world population is observable. Hence, when turning our attention to forecasts, an additional source of uncertainty relates to the evolution of other socio-demographic variables. Below, we first describe our two main projection scenarios. We then discuss the global trends in international migration and income inequality generated by these two scenarios, with a special focus on migration flows to OECD countries, before discussing the policy options than can be used to curb future migration pressures.
Projection scenarios We feed our model with two socio-demographic scenarios obtained from the Wittgenstein Centre for Demography and Global Human Capital (Lutz et al. 2017 ). Footnote 20 The so-called Shared Socioeconomic Pathways (SSPs) have been designed to capture future trends with respect to education, fertility, mortality and socioeconomic challenges (e.g., climate change mitigation and adaptation strategies). We use two scenarios, SSP2 and SSP3, that involve a strong negative association between the level of (female) education and both fertility and (child) mortality rates. The empowerment of women through education is key: it translates into significant changes in attitudes and behaviors. In high fertility countries it brings down birth rates and improves the survival of children. However, the effect of this better education on population growth is delayed because of two factors. First, if more girls get educated today, the effect on fertility will occur some 15 years later, when they will be in their child-bearing years. Second, the decrease in fertility rates does not translate immediately into falling absolute numbers of births because of the large age-structural momentum of population growth. Hence, differences between the two scenarios become visible only after a few decades (after 2050). SSP2 and SSP3 are defined below (Lutz et al. 2017 ):
Scenario SSP2 (Continuation/Medium Population Scenario) is “the middle-of-the-road scenario in which trends typical of recent decades continue, with some progress toward achieving development goals [...]. Development of low income countries is uneven, with some countries making good progress, while others make less.” It is assumed that each country will follow the average path of school expansion that advanced countries have experienced.
Scenario SSP3 (Fragmentation/Stalled Social Development) “portrays a world separated into regions characterized by extreme poverty, pockets of moderate wealth, and many countries struggling to maintain living standards for rapidly growing populations.” SSP3 is based upon the assumption that everywhere the most recently observed rates of school enrolment are frozen at their current levels. As compared with SSP2, SSP3 is characterized by an higher increase in the world population together with a lower educational level, and delayed demographic transition (higher fertility and mortality rates).
In the benchmark simulations, we also assume constant migration costs and amenity differentials \(V_{ij,s,2010}\) —which appears to be a reasonable working hypothesis in the backcasting exercise—and a constant technological progress of 1.5% per year. The latter hypothesis has no incidence on migration decisions as it affects all countries in a symmetric way. The hypothesis of constant migration costs will be relaxed in the end of the section. Our forecasts do not account for future conflict, climate shocks or natural disasters. Footnote 21 Future efforts should incorporate those elements. Finally, we acknowledge the reverse impacts of migration on population growth in sending countries due to change in demographic structure and transfer of reproductive norms. They are however not accounted for in this paper, which takes socio-demographic scenarios as given in order to analyze their effects on income and migration.
Global implications We first highlight the implications of these two socio-demographic scenarios for income growth, global inequality and migration pressures. The global income and migration forecasts are depicted on Fig. 4 , which combines the data for the period 1970–2010, and the model forecasts for the subsequent years. Two scenarios of SSP2 and SSP3 are presented in this Fig. 4 , both with \(\sigma = 2.0\) , \(\gamma = 0.7\) , and full technological externalities, i.e. \(\kappa = 0.214\) and \(\epsilon = 0.207\) . Footnote 22
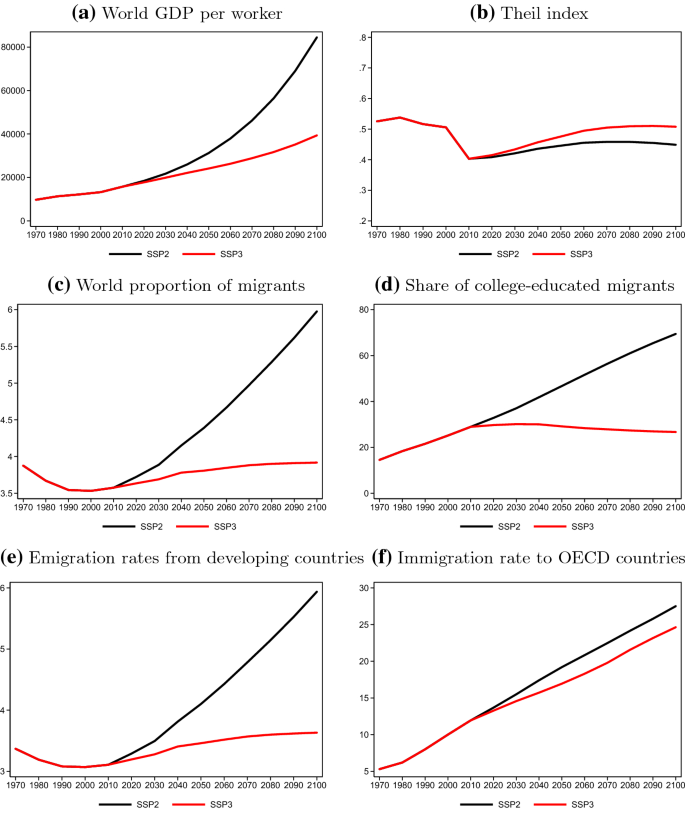
Global income and migration forecasts, 1970–2100. a World GDP per worker. b Theil index. c World proportion of migrants. d Share of college-educated migrants. e Emigration rates from developing countries. f Immigration rate to OECD countries. Note : Authors’ computations based on the variant with \(\gamma =0.7\) , \(\sigma =2\) and \(\kappa =0.214\)
Let us first focus on income projections. Figure 4 a shows the evolution of the worldwide level of GDP per worker. Under SSP3, the average GDP/worker in 2050 is 1.5 times higher than the level of 2010 (and 2.4 times greater in 2100). Under SSP2 and due to the rise in the level of schooling, the average GDP/worker in 2050 is twice as high as the level of 2010 (and 3.5 times greater in 2100). Footnote 23 Figure 4 b describes the evolution of the Theil index between 1970 and 2100. We combine our backcasts, nowcasts and forecasts, and account for between-country inequality and within-country inequality (between the college-educated and less educated representative workers, only). Globally, we show that the Theil index decreases from 1970 to 2010, a phenomenon that can be due to convergence in the productivity scale factors between high-income and emerging countries. Our projections do not account for convergence forces that are not driven by human capital. Under SSP2, the model predicts that the Theil index is constant over time, or is increasing slightly when externalities are included. Under SSP3, we predict an increase in the Theil index.
Figure 4 c, d depict the evolution of the worldwide proportion of international migrants and of the skill structure of migration. Under SSP3, the proportion of migrants (ranging from 3.6 and 3.9%) and the share of college-educated (around 30%) are fairly stable. By contrast, under SSP2, progress in education makes people more mobile. Under constant migration policies, the proportion of migrants increases from 3.6% in 2010 to 4.5% in 2050 and to 6.0% in 2100, and the share of college graduates increases from 29% in 2010 to 34% in 2050 and to 70% in 2100. It is worth noticing that the important gap between the worldwide proportions of migrants in SSP2 and SSP3 does not result from a big difference in terms of worldwide migrant volume. The global stock of migrants amounts to 117 million in 2010. Under SSP2, it reaches 205 million in 2050 and 257 million in 2100. Under SSP3, it equals 180 million in 2050 and 245 million in 2100. This is because SSP2 and SSP3 involves drastically different demographic trends in the developing world. In 2010, the working-age population is estimated at 3.28 billion. Under SSP2, it will reach 4.67 billion by 2050 and 4.29 billion by 2100. Under SSP3, it will reach 4.74 billion by 2050, and 6.26 billion by 2100.
As for the proportion of the high skill population, it should be recalled that our backcasts reveal that past changes in educational attainment were small in developing countries; they hardly affected the trajectory of global migration (see Fig. 1 b). Figure 4 d illustrates the marked effect of human capital trends. SPP2 predicts large educational changes in the coming decades, with strong implications for the skill structure of global migration. Footnote 24
We now focus on emigration and immigration rates, separately. Figure 4 e depicts the evolution of emigration rates, defined as the ratio of emigrants to natives originating from developing countries. The average emigration rate equals 3.1% in 2010. Under SSP2, it is predicted to reach 4.1% in 2050 and to be twice as large in the year 2100; under SSP3, it reaches 3.6% only by the end of the century. As explained above, the emigration rate is governed by the change in the average level of education in the developing world. Under SSP2 progress in education makes people more mobile (remember college graduates migrate more than the less educated). Under SSP3 emigration rates remain fairly stable over time given the slower progress in education. Similar patterns emerge from Fig. 4 c,e, suggesting that the world proportion of migrants is shaped by emigration rates from developing countries.
Finally, Fig. 4 f depicts the evolution of the average fraction of immigrants in OECD member states, defined as the proportion of foreign-born in the total population. This proportion equals 12% in the year 2010 and it is expected to increase drastically over the 21st century. Nevertheless, Figure D.2 in the Appendix D.2 points to a remarkable result that the magnitude of the change is highly insensitive to socio-demographic and technological scenarios. Under SSP3, emigration rates from developing countries vary little, but population growth is large. Under the SSP2 scenario, the rise in emigration rates is larger, but it is partly offset by the fall in the population growth rates of developing countries. Under SSP2, the share of immigrants to OECD countries reaches 19.2% by 2050, and 27.5% by 2100. Under SSP3, this number reaches 16.9% by 2050, and 24.6% by 2100.
Implications for HI countries Table 1 provides projections of immigration rates for the main high-income, destination countries under constant immigration policies. Remember this hypothesis performed well when producing backcasting results. Results obtained under the SSP2 socio-demographic scenario are presented in the top panel; results obtained under SSP3 are presented in the bottom panel. In both cases, we consider the variant with \(\sigma =2\) and full technological externalities, the scenario that is the most compatible with future educational changes. Footnote 25 Under SSP2, from 2010 to 2050, the proportion of immigrants increases from 14.5% to 24.7% (i.e. 10.2 percentage points) in the EU15 and from 17.7% to 26.7% (i.e. 9 percentage points) in the United States. Under SSP2 and over the 21st century, the proportion of immigrants increases by 21.2 percentage points in the EU15 and by 14.3 percentage points in the United States. The greatest variations are obtained for the United Kingdom and for Canada. Under SSP3, the average population growth rates are larger in developing countries, with the exception of Asia. The proportion of immigrants increases by 8.3 percentage points by 2050 and 24.3 percentage points by 2100 in the EU15, and by 7.6 percentage points by 2050 and 22.4 percentage points by the end of the century in the United States. The greatest variations are obtained for Spain, the United Kingdom and for Canada. Projections for the coming 50 years are farily robust to the socio-demographic scenario, and extremely robust to the technological scenario.
In line with Hanson and McIntosh ( 2016 ) or Docquier and Machado ( 2017 ), future migration pressures mainly affect European countries, and are mostly due to rising migration flows from developing countries. To illustrate this, we use the same Max-Sum Submatrix algorithm as in the previous section, and apply it to the matrix of total migration net flows from developing countries to the 27 members of the European Union between 2010 and 2060; projections for subsequent years are more uncertain and scenario-sensitive. For each socio-demographic scenario, we identify the sub-matrix with a fixed dimension of \(25\times 10\) that maximizes the total migration net flows.
Under the SSP2 scenario, we obtain the following results (in alphabetical order):
Main destination countries: Belgium, France, Germany , Ireland, Italy, Netherlands, Portugal, Spain, Sweden, and the United Kingdom .
Main countries of origin: Afghanistan, Algeria, Angola, Bangladesh , Cameroon, Dem. Rep. of Congo, Cote d’Ivoire, Ghana, India , Iran, Iraq, Kenya, Madagascar, Mali, Morocco , Mozambique, Nigeria, Pakistan, Philippines, Senegal, Somalia , Tanzania, Turkey, Uganda , and Zimbabwe.
And under the SSP3 scenario, we have (by alphabetical order):
Main destination countries: Austria, Belgium, France, Germany, Italy, Netherlands, Portugal, Spain, Sweden, and the United Kingdom .
Main countries of origin: Afghanistan, Algeria, Angola, Bangladesh , Bolivia, Colombia, Dem. Rep. of Congo, Cote d’Ivoire , Ecuador, Ghana, India, Iraq , Kazakhstan, Kenya, Madagascar, Mali, Morocco, Nigeria, Pakistan , Peru, Philippines, Senegal, Somalia, Turkey, and Uganda .
Under SSP2, migration flows from sub-Saharan Africa and from the MENA play a key role, as well as the flows from a few Asian countries with large populations. As the majority of African migrants go to Europe, the EU15 experience greater migration pressures. Under SSP3, this change is mostly due to immigration from Africa, although the magnitude of this phenomenon is smaller than under SSP2. However, migration pressures from Asia, from MENA, and from some Latin American countries are stronger. Clearly, there is a large intersection of 9 destination countries (see countries in italics above) that are all member states of the EU15, and for which future migration pressures are expected to be strong, whatever the socio-demographic scenario for the coming half century. And there is large intersection of 20 developing countries (in italics above) that are responsible for such migration pressures, including sub-Saharan African countries, the MENA countries, and a few Asian countries.
Remarkably, the predicted rise in immigration to the main destination countries can be accompanied by a major change in its skill structure. Table 2 shows that the share of the highly skilled in the total number of immigrants to the main destination countries has always been increasing since 1970 and will continue to rise over the 21st century in the SSP2 scenario. By 2050, the share of high skilled will reach 49.7% in the EU15, 56.4% in the United States, 90.1% in Canada, and 68.5% in Australia. By the end of the century, this number will rise drastically to reach 75.1% in the EU15, 79.4% in the United States, 91.9% in Canada, and 85.2% in Australia. In other words, under SSP2 the number of immigrants to the main destinations will increase but they will also be more and more skilled. The situation differs with SSP3 where those numbers will sluggish and only increase by 1 or 2 percentage points compared to the current levels of 2010.
The case for migration restrictions We finally turn our attention to the inescapable nature of these migration trends. To illustrate the difficulty of curbing future migration pressure with immigration restrictions, we computed the relative change in \(V_{ij}\) required to keep the dyadic and skill-specific migration stocks at their level of 2010 in the years 2020, 2030, 2040 and 2050. The scale factor \(V_{ij,s}\) , while not directly interpretable in monetary unit, is proportional to migration costs which are affected by many factors, among which the size of the dyadic migration stocks is one of its most important determinants identified in the literature. As the elasticity of \(V_{ij,s}\) to the network size is equal to 0.725 (see Table A.1 in the Appendix A), dividing the relative change in \(V_{ij,s}\) by 0.725 gives the relative change in the network size that is required to limit people’s incentives to emigrated and keep the stock of migrants constant. The average result for income groups and for the main migration corridors are depicted in Fig. 5 under the SSP2 scenario.
As SSP2 predicts a rise in human capital, future migration pressures are stronger among college graduates than among the less educated. Hence, keeping skill-specific migration stocks at their level of 2010 requires a drastic change in \(V_{ij,h}\) , and smaller changes in \(V_{ij,l}\) . Looking at broad income groups, changes in \(V_{ij,h}\) are equivalent to reducing the network size by 13 to 21%. However, looking at the largest migration corridors (Mexico to US and sub-Saharan African countries to the EU15), the fall in \(V_{ij,h}\) is usually close to 100% (and sometimes even higher) while the fall in \(V_{ij,l}\) varies between 19% in the case of Mexican migrants to the US, and 86% in case of African migrants to Germany. Under SSP3, similare changes in \(V_{ij,l}\) and \(V_{ij,l}\) are required to keep migration stocks constant. While it is difficult to translate these measures into migration policy variables, our results suggest that sealed borders are virtually needed to control future migration pressures, as it is the case during the current Covid-19 crisis. The target is likely unattainable if the basic right to family reunification is respected.
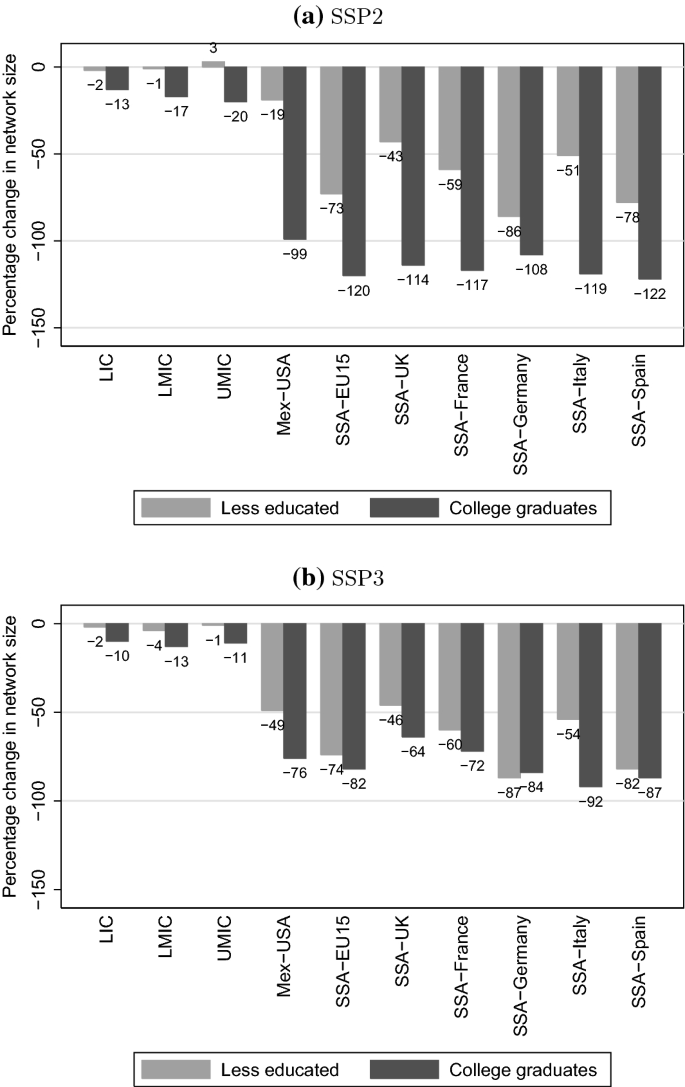
Migration policies to limit migration pressures (Expressed as equivalent variations in the dyadic network size required to keep skill-specific migration stocks constant) a SSP2. b SSP3. Note: We computed the relative change in the scale factor of the migration technology, \(\frac{\Delta V_{ij}}{V_{ij}}\) , required to keep bilateral migration stocks constant over the period 2010–2050. We express this change as an equivalent decrease in the network size using \(\frac{\Delta V_{ij}}{V_{ij}}=0.725 \times \frac{\Delta Netw_{ij}}{Netw_{ij}}\) . Results for college graduates (in dark gray) and the the less educated (in light gray) are thus expressed in terms of \(\frac{\Delta Netw_{ij}}{Netw_{ij}}\)
The case for development policies Under the European Migration Compacts, Footnote 26 an investment plan has also been proposed to stimulate employment opportunities and income in Africa, in the hope of reducing migration pressures. The effectiveness of these Migration Compacts depends on the resources allocated to their implementation (in comparison to the development targets to be reached), and on the effectiveness of the measures undertaken. To illustrate the difficulty curbing future migration pressure with development policies, we consider the intersection of 20 developing countries emerging from our Max-Sum Submatrix problem (referred to as Compact 1 ), Footnote 27 or the combined region of sub-Saharan African and the MENA countries (referred to as Compact 2 ). We consider these sets of countries as potential partners of a Migration Compact, and we quantify the homothetic change in TFP (above normal trend) and derive the consequent GDP annual growth rates required to keep their total emigration stocks to Europe at their levels of 2010. Our simulations account for all general equilibrium effects.
Table 3 provides the results of these policy experiments, taking the population size and structure as given. Our discussion mainly focuses on net migration flows in the next two decades, the period for which socio-demographic variations between SPP2 and SPP3 are smaller and less likely to be affected by the TFP changes. In other words, this consideration partially mitigates the issue that fertility and human capital are endogenously affected by income, which our model does not account for. Indeed, if TFP and GDP start increasing from 2010 onwards, population growth rates and the skill composition of the labor force will be gradually impacted. Results obtained for 2030 and after (essentially beyond one generation) are likely to overestimate the requested changes and should be treated with more caution.
Under the SSP2 socio-demographic scenario, keeping the stock of the 20 main origin countries ( Compact 1 ) at its level of 2010 requires TFP to increase by 58% in 2020 and by 128% in 2030, compared to the baseline. Under the SSP3 scenario, the required TFP changes amount to 49% in 2020 and by 99% in 2030. Overall, this means multiplying GDP per capita by 2 above the normal trend over the next two decades. Equivalently, this requires a TFP growth rate of 5% per year under SPP2 (instead of 1.5% a year in the baseline), and a TFP growth rate of 4.2% a year under SPP3. In terms of GDP growth, the required levels are on average twice as high as the baseline levels; in all variants, the requested annual GDP growth rate is close to 10%. Implementing Migration Compacts with all sub-Saharan African and MENA countries ( Compact 2 ) requires similar changes in TFP and gives rise to similar effects. Footnote 28
Takeoffs of this nature have rarely been observed in the course of history. Footnote 29 They basically require all SSA and MENA countries to enter the “modern growth club” during the 21st century. Based on facts from the 19th and 20th centuries, Bénétrix et al. ( 2015 ) estimate that joining the club requires an annual GDP growth rate above 5% over a period of ten years; Jones and Romer ( 2010 ) argue that higher threshold growth rates are needed in the current period. Still, “explosive-growth” episodes were indeed recently observed in emerging countries. Taiwan multiplied its income per capita by 5 between 1980 and 2000, and South Korea multiplied it by 7.5 over the same period; China has increased its income level tenfold since 1990 with an average GDP growth rate of 8% per year. Similar takeoffs have not been observed in sub-Saharan Africa. However, Rwanda, which is usually seen as one of the fastest growing economies in Africa, has increased its income per capita threefold in the post-genocide period.
Sustaining TFP growth rates of 4 to 5% or real GDP growth rates of 8 to 10% per year on the spatial scale of a continent and over several decades is unprecedented. So far, development policies have not triggered such resounding and generalized economic booms (Hausmann et al. 2005 ). Hence, dramatic changes in the effectiveness of aid are needed if policymakers want to use development tools to reduce migration pressures (Berthélemy et al. 2009 ; Berthélemy and Maurel 2010 ; Gary and Maurel 2015 ). In addition, generating these booms in SSA and MENA would only attenuate migration pressures to Europe, but would not eliminate them since migration pressures from other countries and regions would still be observed. Table 3 shows that the EU15 immigration rate in 2060 would be around 20% in all scenarios, compared to 14.6% in 2010. Reinforcing immigration restrictions is another complementary policy avenue. However, it is a priori unclear whether changes in laws and policies can significantly affect the size of immigration flows. Past restrictions on migration have not prevented third-country nationals from moving in past decades (it may be recalled that our backcasts with constant \(V_{ij,s,t}\) fit well past migration flows), and have caused displacements and increasing flows of irregular migrants. Over the 21st century, increasing migration seems to be an inevitable phenomenon, which raises important challenges in terms of policy coherence for most industrialized countries.
5 Conclusion
The number of asylum applications lodged in 2015 in EU Member States exceeded 1.3 million, putting migration policy in the forefront of the global policy debate. While the proximate cause of the current crisis is the conflict and political unrest in the Middle East and Africa, the recent trends and forecasts for the world economy strongly suggest that there may be further episodes of large-scale migration in the near future, in Europe and in other OECD countries. Specifically, the underlying root causes of increased migration (demographic growth differentials, economic inequality, increased globalization, political instability, climatic changes) are all projected to exert a stronger influence on migration in the coming decades.
Relying on socio-demographic and technological scenarios, this paper produces integrated backcasts, nowcasts and forecasts of income and bilateral migration stocks for all pairs of countries. Our model fits very well the trends in international migration of the last 40 years, and demonstrates that historical trends were mostly governed by demographic changes. Turning to the migration prospects for the 21st century, we also find that world migration prospects are mainly governed by socio-demographic changes; they are virtually insensitive to the technological environment. We predict a highly robust increase in immigration pressures in general, and in European immigration in particular. These migration pressures are mostly explained by the demographic changes in sub-Saharan Africa and in the MENA countries. Curbing them with immigration restrictions or with development policies requires sealing borders or triggering unprecedented economic booms in many developing countries. More than ever, improving the management of migration flows and the coherence between development and migration policies will represent major challenges for European countries in the 21st century. In particular, helping developing countries to increase human capital for all and for women in particular could drastically transform the skill structure of global migration and make destination countries less hostile to immigrants.
The population ratio between LDCs and HICs increased from 3.1 in 1960 to 5.5 in 2010. This explains why a 0.9% increase in emigration rate from LDCs translated into a 6.5% increase in the share of immigrants to HICs.
In the medium variant, they assume long-run convergence towards low fertility and high life expectancy across countries, and constant immigration flows.
Future migration flows reflect expert opinion about future socio-political and economic trends that could affect migration. From 2060 onwards, it is assumed that net migration flows converge to zero (attained in the 2095–2100 period), implying that migration stocks also converge to zero.
A similar approach is used in Docquier and Machado ( 2017 ) who focus on 34 destination countries and consider non-official socio-demographic projections. Identifying assumptions are refined here thanks to the backcasting experiments, and richer technology and policy variants are considered.
Although Grogger and Hanson ( 2011 ) find that a linear utility specification fits the patterns of positive selection and sorting in the migration data well, most studies rely on a logarithmic utility function (Bertoli and Moraga Fernandez-Huertas 2013 ; Beine and Parsons 2015 ; Beine et al. 2019 ; Ortega and Peri 2013 ).
Bertoli and Moraga Fernandez-Huertas ( 2015 ), Bertoli and Moraga Fernandez-Huertas ( 2013 ) or Ortega and Peri ( 2013 ) used more general distributions, allowing for a positive correlation in the application of shocks across similar countries.
In fact, there is a slight abuse of terms here as \(A_{j,t}\) implicitly includes capital in supplement to the usual TFP, which is by definition the residual that explains a country’s output level apart from capital and labor. This is why we define \(A_{j,t}\) as a “modified TFP.”
See Katz and Murphy ( 1992 ), Card and Lemieux ( 2001 ), Caselli et al. ( 2006 ), Borjas ( 2003 ), Borjas ( 2013 ), Card ( 2009 ), Ottaviano and Peri ( 2012 ), Docquier et al. ( 2015 ) among others.
When missing, the latter are supplemented using the estimates of Docquier et al. ( 2015 ).
The 60 destinations that do not have any migrant recorded in our dataset are: Afghanistan, Algeria, Angola, Azerbaijan, Bahamas, Bangladesh, Barbados, Bhutan, Bosnia and Herzegovina, Brunei, Burundi, Cape Verde, Central African Republic, Chad, Congo D.R., Congo R, Djibouti, East Timor, Equatorial Guinea, Eritrea, Ethiopia, Gabon, Guinea-Bissau, Guyana, Haiti, Jordan, Korea, Lebanon, Lesotho, Libya, Macedonia, Madagascar, Maldives, Mauritania, Micronesia, Moldova, Morocco, Myanmar, Nigeria, Occupied Palestinian Territory, Pakistan, Papua New Guinea, St. Lucia, St. Vincent and the Grenadines, Samoa, Sao Tome and Principe, Singapore, Solomon Islands, Somalia, Suriname, Swaziland, Syria, Tonga, Tunisia, Turkmenistan, Uzbekistan, Vanuatu, Vietnam, Yemen, Zimbabwe. None of these destinations is an important immigration country.
Which is implicitly captured by the level of dyadic net migration costs, \(V_{ij,t,s}\) .
In practice, visa restrictions are likely to depend on the intensity of immigration pressures as well as on origin and/or destination countries’ characteristics.
Interestingly, Ortega and Peri ( 2009 ) find that capital adjustments are rapid in open economies: an inflow of immigrants increases one-for-one employment and capital stocks in the short term (i.e. within one year), leaving the capital/labor ratio unchanged.
Appendix C.2 provides details on the computation of these proxies.
Note that the pre-migration population levels are not directly observable but they can be identified as outcomes of our model, as explained in Appendix C.1.
Table C.2 in the Appendix C.3 shows that the actual total number of migrants of 2019 is 141 million. All of our predicted numbers for the year 2020 are only slightly higher. In the benchmark scenario where we consider the demographic scenario SSP2, \(\gamma =0.7\) , \(\sigma =2\) and full technological externalities, our predicted number of world migrants reaches 142 million in 2020. The correlation between the actual and predicted immigrants by destination and emigrants by origin is also very high (more than 0.94).
Table C.1 in the in the Appendix C.2 provides the coefficient of correlation between our backcasts for 1970–2000 and the actual observations aggregate at country level for each decade and for different parameter sets. It evidences the robustness of the model to different technological scenarios.
In the late 20th century from 1970 to 2000, we document both forms of tighter and loosened immigration policies in major receiving countries. In Western Europe, the Guest Worker program came to an end following the 1973–4’s oil crisis. While in the US, a series of immigration acts were introduced allowing more entry of family immigrants (the 1990 Immigration Act), legalization of illegal immigrants (the 1986 Reform and Control Act) (see Clark et al. ( 2007 ) for an overview) before immigration policies became restrictive again after the September 11 attacks in 2001. The third wave of immigration to the Gulf region also took place during this period after 1971—year of official independence of GCC countries from the United Kingdom—where mass industrialization and modernization have led to large importation of foreign workers.
Assuming \(\kappa \) is large, we may overestimate the causal effect of the skill ratio on the ratio of value shares. However, disregarding causation issues, this technological scenario is the most compatible with the cross-country correlation between human capital and the wage structure: it fits the cross-country correlation between the skill bias and the skill ratio in the year 2010.
More details about these scenarios are provided in Appendix D.
For instance, Missirian and Schlenker ( 2014 ) show that asylum applications increased when global temperatures rose, Desmet et al. ( 2018 ) predict how local sea-level changes affect human displacement. Burzynski et al. ( 2019 ) predict that 210 to 320 million people will be forced or incentivized to move over the 21st century. However, under constant migration policies, climate migrants will move first from rural to urban areas, within their own countries, before moving across borders (Maurel and Tuccio 2015 ). Existing literature suggests that massive international flows of climate refugees are unlikely, except under generalized and persistent conflicts over resources (Rigaud et al. 2018 ).
Additional results of different technological scenarios are presented in the Figure D.2 in the Appendix D.2. Under SSP3, worldwide changes in human capital are negligible; eliminating technological externalities hardly modifies the results. Under SSP2, technological scenarios play a more important role after 2050 but have little influence on global trends.
In Figure D.2 of the Appendix D.2, productivity growth is boosted when technological externalities are factored in. By contrast, assuming a higher level for \(\sigma \) generates very similar income projections.
Figure D.2 in the Appendix D.2 shows another remarkable result is that the global trends in international migration are virtually unaffected by the technological environment; they are totally governed by socio-demographic changes.
Very similar results are obtained when technological externalities are zero, as shown in Appendix D.2.
In line with the Sustainable Development Goals and the New York Declaration for Refugees and Migrants (UN 2016 ), the European Commission has outlined a general line of action to address the global challenge of future migration (see the European Agenda on Migration and the new Partnership Framework on Migration). Migration Compacts include a set of measures to be implemented in the home country, targeting the reinforcement of border controls, the readmission of migrants who have been denied entry, or a higher level of economic development.
These include Afghanistan, Algeria, Angola, Bangladesh, Dem. Rep. of Congo, Cote d’Ivoire, Ghana, India, Iraq, Kenya, Madagascar, Mali, Morocco, Nigeria, Pakistan, Philippines, Senegal, Somalia, Turkey, and Uganda.
We have also conducted another set of simulations ( Compact 3 ) keeping constant the total emigration stocks of sub-Saharan African countries only. The resulting required TFP growth rates are higher than the ones in Compact 2 where growth is fostered in both the MENA and SSA regions. This shows the capacity of the MENA countries to absorb migrants from SSA. Thus smaller but simultaneous investment in both regions is recommended to curb migration pressures to Europe.
This was even the case during the Industrial Revolution. Between 1820 and 1900, GDP per capita rose 2.5 times in Western Europe, and 3.3 fold in the United States (Maddison 2007 ). In other words, growth rates were 1.2 and 1.5% a year, respectively.
Acemoglu, D. (2002). Technical change, inequality, and the labor market. Journal of Economic Literature , 40 (1), 7–72.
Article Google Scholar
Acemoglu, D., & Angrist, J. (2000). How large are human-capital externalities? Evidence from compulsory schooling laws. NBER Macroeconomics Annual , 15 , 9–59.
Angrist, J. (1995). The economic returns to schooling in the West Bank and Gaza strip. American Economic Review , 6 (1), 1065–1087.
Google Scholar
Arslan, C., Dumont, J., Kone, Z., Moullan, Y., Ozden, C., Parsons, C., & Xenogiani, T. (2015). A new profile of migrants in the aftermath of the recent economic crisis. ( OECD Social, Employment and Migration Working Papers ), 160. https://www.oecd.org/els/mig/wp160.pdf
Autor, D., Levy, F., & Murnane, R. (2003). The skill content of recent technological change: An empirical exploration. Quarterly Journal of Economics , 118 (4), 1279–1333.
Beine, A., Bourgeon, P., & Bricongne, J. (2019). Aggregate fluctuations and international migration. Scandinavian Journal of Economics , 121 (1), 117–152.
Beine, M., & Parsons, C. (2015). Climatic factors as determinants of international migration. Scandinavian Journal of Economics , 117 (2), 723–767.
Bénétrix, A., O-Rourke, K., & Williamson, J. (2015). The spread of manufacturing to the poor periphery 1870–2007. Open Economies Review , 26 (1), 1–37.
Benhabib, J., & Spiegel, M. (1994). The role of human capital in economic development evidence from aggregate cross-country data. Journal of Monetary Economics , 34 (2), 143–173.
Berthélemy, J., Beuran, M., & Maurel, M. (2009). Aid and migration: Substitutes or complements? World Development , 37 (10), 1589–1599.
Berthélemy, J. & Maurel, M. (2010). The consistency of EU foreign policies towards new member states . In: Keereman F., Szekely I. (eds) Five Years of an Enlarged EU. Springer, Berlin, Heidelberg.
Bertoli, S., & Moraga Fernandez-Huertas, J. (2013). Multilateral resistance to migration. Journal of Development Economics , 102 , 79–100.
Bertoli, S., & Moraga Fernandez-Huertas, J. (2015). The size of the cliff at the border. Regional Science and Urban Economics , 51 (C), 1–6.
Bobba, M., & Coviello, D. (2007). Weak instruments and weak identification, in estimating the effects of education, on democracy. Economic Letters , 96 (3), 301–306.
Bolt, J., & Van Zanden, J. (2014). The maddison project: Collaborative research on historical national accounts. Economic History Review , 67 (3), 627–651.
Borjas, G. (2003). The labor demand curve is downward sloping: Reexamining the impact of immigration on the labor market. Quarterly Journal of Economics , 118 (4), 1335–1374.
Borjas, G. (2013). The analytics of the wage effect of immigration. IZA Journal of Migration , 2 (1), 22.
Burzynski, M., Deuster, C., Docquier, F., & de Melo, J. (2019). Climate change, inequality and human migration. ( CEPR Discussion Paper ), No. 13997, CEPR: London.
Card, D. (2009). Immigration and inequality. American Economic Review , 99 (2), 1–21.
Card, D., & Lemieux, T. (2001). Can falling supply explain the rising return to college for younger men? A cohort-based analysis. Quarterly Journal of Economics , 116 (2), 705–746.
Caselli, F., Coleman, I., & John, W. (2006). The world technology frontier. American Economic Review , 96 (3), 499–522.
Castelló-Climent, A. (2008). On the distribution of education and democracy. Journal of Development Economics , 87 (2), 179–190.
Ciccone, A., & Papaioannou, E. (2009). Human capital, the structure of production, and growth. Review of Economics and Statistics , 91 (1), 66–82.
Clark, X., Hatton, T., & Williamson, J. (2007). Explaining us immigration, 1971–1998. Review of Economics and Statistics , 89 (2), 359–373.
Desmet, K., Kopp, R., Kulp, S., Nagy, D., Oppenheimer, M., Rossi-Hansberg, E., & Strauss, B. (2018). Evaluating the economic cost of coastal flooding. ( NBER Working Paper ), No. 24918, NBER: Cambridge, MA.
Djajic, S., Kirdar, M., & Vinogradova, A. (2016). Source-country earnings and emigration. Journal of International Economics , 99 , 46–67.
Docquier, F., & Machado, J. (2017). Income disparities, population and migration flows over the twenty first century. Italian Economic Journal , 3 (2), 125–149.
Docquier, F., Machado, J., & Sekkat, K. (2015). Efficiency gains from liberalizing labor mobility. Scandinavian Journal of Economics , 117 (2), 303–346.
Gary, A., & Maurel, M. (2015). Donors’ policy consistency and economic growth. Kyklos , 68 (4), 511–551.
Grogger, J., & Hanson, G. (2011). Income maximization and the selection and sorting of international migrants. Journal of Development Economics , 95 (1), 42–57.
Hanson, G., & McIntosh, C. (2016). Is the mediterranean the new Rio Grande? US and EU immigration pressures in the long run. Journal of Economic Perspectives , 30 (4), 57–82.
Hatton, T., & Williamson, J. (1998). The age of mass migration: Causes and economic impact . Oxford: Oxford University Press.
Hatton, T., & Williamson, J. (2003). Demographic and economic pressure on emigration out of Africa. Scandinavian Journal of Economics , 105 (3), 465–486.
Hatton, T., & Williamson, J. (2011). Are third world emigration forces abating? World Development , 39 (1), 20–32.
Hausmann, R., Pritchett, L., & Rodrik, D. (2005). Growth accelerations. Journal of Economic Growth , 10 (4), 303–329.
Hendricks, L. (2004). A database of mincerian earnings regressions. Available online at ,. www.lhendircks.org/Mincer.htm .
Iranzo, S., & Peri, G. (2009). “Schooling externalities, technology, and productivity: Theory and evidence from U.S. states. Review of Economics and Statistics , 91 (2), 420–431.
Jones, C., & Romer, P. (2010). The new Kaldor facts: ideas, institutions, population, and human capital. American Economic Journal: Macroeconomics , 2 (1), 224–245.
Katz, L., & Murphy, K. (1992). Changes in relative wages, 1963–1987: Supply and demand factors. Quarterly Journal of Economics , 107 (1), 35–78.
Kennan, J. (2013). Open borders. Review of Economic Dynamics , 16 (2L), 1–13.
Klein, P., & Ventura, G. (2009). Productivity differences and the dynamic effects of labor movements. Journal of Monetary Economics , 56 (8), 1059–1073.
Krzywinski, M., Schein, J., Birol, I., Connors, J., Gascoyne, R., Horsman, D., et al. (2009). Genome Research , 19 , 1639–1645.
Lucas, J. R. (1988). On the mechanics of economic development. Journal of Monetary Economics , 22 (1), 3–42.
Lutz, W., Butz, W., & Samir, K. (2017). World Population and Human Capital in the Twenty-First Century: An Overview . Oxford: Oxford University Press.
Book Google Scholar
Maddison, A. (2007). Contours of the world economy 1–2030 AD: Essays in macro-economic history . Oxford: Oxford University Press.
Maurel, M., & Tuccio, M. (2015). Climate instability, urbanization and international migration. Journal of Development Studies , 52 (5), 735–752.
McFadden, D. (1984). Econometric analysis of qualitative response models. Handbook of Econometrics , 2 , 1395–1457.
Missirian, A., & Schlenker, W. (2014). Asylum applications respond to temperature fluctuations. Science , 358 (6370), 1610–1614.
Moretti, E. (2004). Workers’ education, spillovers, and productivity: Evidence from plant-level production functions. American Economic Review , 94 (3), 656–690.
Murtin, F., & Wacziarg, R. (2014). The democratic transition. Journal of Economic Growth , 19 (2), 141–181.
Ortega, F., & Peri, G. (2009). The causes and effects of international migrations: Evidence from OECD countries 1980-2005. ( NBER Working Paper ), No. 14833, NBER: Cambridge, MA.
Ortega, F., & Peri, G. (2013). The role of income and immigration policies in attracting international migrants. Migration Studies , 1 (1), 1–28.
Ottaviano, G., & Peri, G. (2012). Rethinking the effect of immigration on wages. Journal of the European Economic Association , 10 (1), 152–197.
Ozden, C., Parsons, C., Schiff, M., & Walmsley, T. (2011). Where on earth is everybody? The evolution of global bilateral migration 1960–2000. World Bank Economic Review , 25 (1), 12–56.
Restuccia, D., & Vandenbroucke, G. (2013). The evolution of education: A macroeconomic analysis. International Economic Review , 54 (3), 915–936.
Rigaud, K. K., de Sherbinin, A., Jones, B., Bergmann, J., Clement, V., Ober, K., Schewe, J., Adamo, S., McCusker, B., Heuser, S., et al. (2018). Groundswell - preparing for internal climate migration.
UN, G. A. (2016). New York declaration for refugees and migrants . United Nations, Doc. A/71/L, 1, 13, United Nations: New York.
UNDESA (2013). World Youth Report: Youth and Migration . United Nations: New York.
Download references
Author information
Authors and affiliations.
RES/LIDAM, Université catholique de Louvain , Louvain-la-Neuve, Belgium
Thu Hien Dao
Department of Economics, University of Bielefeld, Bielefeld, Germany
LISER, Esch-sur-Alzette, Luxembourg
Frédéric Docquier
Centre d’Économie de la Sorbonne (CES - UMR8174), Université Paris 1 Panthéon-Sorbonne, Paris, France
Mathilde Maurel
CNRS, Paris, France
FERDI, Clermont-Ferrand, France
Department of Computer Science & Engineering, Université catholique de Louvain, Louvain-la-Neuve, Belgium
Pierre Schaus
You can also search for this author in PubMed Google Scholar
Corresponding author
Correspondence to Thu Hien Dao .
Additional information
Publisher's note.
Springer Nature remains neutral with regard to jurisdictional claims in published maps and institutional affiliations.
We thank three anonymous referees for their helpful comments and suggestions. We also benefited from discussions with Christiane Clemens, Giuseppe de Arcangelis, Timothy Hatton, Vincent Vanderberghe, and Gerald Willmann. This paper was presented at the conference on “Demographic Challenges in Africa” jointly organized by the French Agency for Development and the University of Paris 1 Panthéon-Sorbonne in February 2017, at the 8th International Conference on “Economics of Global Interactions: New Perspectives on Trade, Factor Mobility and Development” at the University of Bari Aldo Moro in September 2017, and at the Workshop on “The drivers and impacts of migration and labour mobility in origins and destinations: Building the evidence base for policies that promote safe, orderly and regular people’s and labour mobility for poverty reduction and sustainable development” at FAO Headquarters in Rome in December 2017. The authors are grateful to the participants for valuable comments. Thu Hien Dao acknowledges financial support from the European Commission in the framework of the European Doctorate in Economics Erasmus Mundus (EDEEM). Frédéric Docquier and Pierre Schaus acknowledge financial support from the ARC convention on “New approaches to understanding and modelling global migration trends” (convention 18/23-091).
Electronic supplementary material
Below is the link to the electronic supplementary material.
Supplementary material 1 (pdf 5116 KB)
About this article.
Dao, T.H., Docquier, F., Maurel, M. et al. Global migration in the twentieth and twenty-first centuries: the unstoppable force of demography. Rev World Econ 157 , 417–449 (2021). https://doi.org/10.1007/s10290-020-00402-1
Download citation
Accepted : 29 October 2020
Published : 13 January 2021
Issue Date : May 2021
DOI : https://doi.org/10.1007/s10290-020-00402-1
Share this article
Anyone you share the following link with will be able to read this content:
Sorry, a shareable link is not currently available for this article.
Provided by the Springer Nature SharedIt content-sharing initiative
- International migration
- Migration prospects
- World economy
JEL classification
- Find a journal
- Publish with us
- Track your research
Cookie settings
These necessary cookies are required to activate the core functionality of the website. An opt-out from these technologies is not available.
In order to further improve our offer and our website, we collect anonymous data for statistics and analyses. With the help of these cookies we can, for example, determine the number of visitors and the effect of certain pages on our website and optimize our content.
Selecting your areas of interest helps us to better understand our audience.
- Subject areas
Demographic and economic determinants of migration
Demographic and economic determinants of migration Updated
Push and pull factors drive the decision to stay or move.
Colgate University, USA, and IZA, Germany
Elevator pitch
There are a myriad of economic and non-economic forces behind the decision to migrate. Migrants can be “pushed” out of their home countries due to deteriorating economic conditions or political unrest. Conversely, migrants are often “pulled” into destinations that offer high wages, good health care, strong educational systems, or linguistic proximity. In making their decision, individuals compare the net benefits of migration to the costs. By better understanding what forces affect specific migrant flows (e.g. demographic characteristics, migrant networks, and economic conditions), policymakers can set policy to target (or reduce) certain types of migrants.
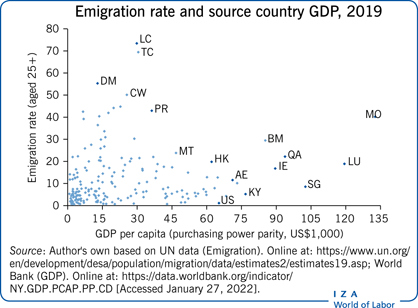
Key findings
Increases in income differentials across countries often lead to increases in migrant flows.
Strong migrant networks have historically played a large role in enhancing migrant flows.
Tax and government transfer policies (e.g. welfare, health care, and educational systems) can attract migrants.
Migration serves as a form of insurance for households and the desire to remit earnings from abroad may form part of the household decision to migrate.
Macroeconomic conditions at home and abroad can affect the flow of migrants.
Migration costs are central to the migration decision, they take many forms (e.g. transportation, job search, visa fees, smuggler fees), and often vary with distance.
Strict migration policy acts as a constraint in the migration decision.
Demographic factors such as age, education, marital status, and language impact a person’s willingness to migrate.
Worsening political and environmental conditions abroad and/or improving conditions at home can lead to less migration.
Author's main message
Many factors affect the net benefits and costs of migration and thus impact the decision to migrate. Studies indicate that the include the availability of migrant networks, differences in income across countries, and demographic factors, but many other factors also affect the migration decision. Constraints created by tighter migration policy represent the most significant hurdle to migration. Prior to making significant changes in migration policy, policymakers should make sure they sort out which factors are driving each migrant flow so they are better prepared to welcome their new migrants.
Consider the forces that would motivate people and their families to leave their home country and move to another to start a new life. Some of those forces are economic: a higher standard of living, the chance to find a better job, or a more comfortable safety net. However, other forces are at play as well, such as superior amenities (e.g. air quality), a democratic political system, and access to a better education system. Between 2010 and 2020, the growth in the migrant stock worldwide increased by 5.1 million each year [1] . Many more would like to migrate if given the opportunity. Understanding the key determinants of migration patterns is essential when considering the implications of immigration policy. Setting immigration policy without thinking through the various mechanisms that are driving migrant flows is misguided and could lead to unintended consequences.
It should be noted that this article focuses on international migration, not internal migration. However, many of the determinants discussed in this article would also apply to internal migration.
Discussion of pros and cons
Factors enhancing migration, income differentials and income inequality.
The net benefits to migration include the increase in income (or “utility”) gained by migrating, or the difference between origin country (where migrants are moving from) and host country (where migrants are moving to) income. Workers in poorer countries are often looking for higher wages and better employment opportunities, which can usually be found in richer countries. As a result, nearly every economic model of migration incorporates income differentials and finds them to be an important factor when trying to explain migrant flows in a given period. Income can serve as a “push” factor: low income levels can push migrants out of their home country, as shown in the Illustration . Conversely, high income levels “pull” migrants into a specific country or region ( Figure 1 ) [2] . In this context, push factors are conditions that propel people to leave the origin country, while pull factors are conditions that entice people to enter a destination country.
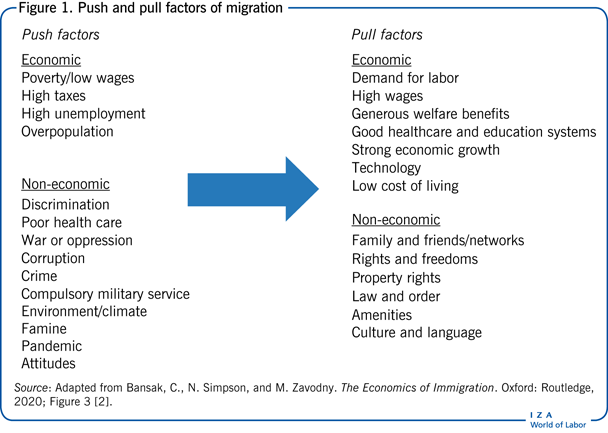
In many cases, both push and pull forces are at play but income tends to have larger pull effects than push effects [3] . Over time, income differentials between countries may change, which can affect migrant flows; as income differentials increase (either by origin country income decreasing and/or host country income increasing), there is a larger incentive to migrate. The reverse holds true when income differentials decrease. In this case, the net gain to migration falls, so fewer workers find it attractive to migrate abroad. In addition, the level of income in the home country is relevant. For very low levels of income, people may not be able to afford migration. As income increases, more can afford to migrate, but the gains to migration fall. Income levels and differences in income, by affecting the net benefits of migration, are typically considered among the most important determinants in the decision to migrate.
Income variability can also affect the migration decision. If income is highly volatile, workers may be incentivized to search for more stable income streams, especially in countries where credit markets are nonexistent or incomplete. (Economic theory indicates that individuals can save or borrow to smooth out their income streams, but in some countries access to credit markets is limited.) Thus, less income volatility in the host country may increase the net benefits of migration and/or decrease migration costs. In addition, countries with high amounts of income inequality often have large gaps between wages for high-skilled workers and low-skilled workers. Similar to income levels, the distribution of income can work as a push or pull factor. “There is a higher incentive to migrate if one is poor among rich than if one is poor among poor,” suggesting that income inequality is a push factor [4] , p. 5. At the same time, income inequality may act as a pull factor: potential migrants with high levels of education may be pulled to countries where the returns to skills are high.
Networks: Family and friends
Nearly every contemporary study of the determinants of migration considers the importance of migrant networks in the host country. The most typical way to measure networks is to control for the stock of migrants from a given migrant's origin country in the host country (or region). Since migrant stocks are captured in most national censuses, network effects are easily measured. Research consistently finds a strong, positive effect of migrant networks on predicting new migrant flows. That is, migrants are often attracted to host countries with large populations of migrants (diasporas) from the same country of origin. Having a community of people who speak the same language and share the same culture leads to lower psychological costs associated with migration. Networks may also reduce the economic costs of migration by helping migrants find employment and housing, as well as helping the migrant assimilate to their new location. Over time, as migrants assimilate to their new country, their reliance on the migrant community may decrease as they learn the host country's culture and language, thereby reducing the net benefits created by the local migrant community.
Estimates suggest that if the stock of migrants from a specific country increases by 1,000 people, then the flow of migrants will increase by 4.7 people per year, although the effect dampens as the migrant stock gets bigger, suggesting that migrant networks have the largest effect when migrant stocks are small [5] . In the majority of studies decomposing the various determinants of migration, migrant networks are usually the most important predictor of migrant flows. For example, migrant stocks explain more than 70% of the observed variation in migrant flows to OECD countries [6] . In addition, migrant networks tend to matter most for migrants coming from poor origin countries [7] .
Taxes, transfers, and the government safety net
Taxes and government transfers (e.g. unemployment insurance, welfare) often serve as pull factors rather than push factors (but in theory they could be both). Income taxes, for example, reduce the return to working and hence lower the net benefits of migration. Countries with progressive income taxes (where higher incomes are taxed more heavily) may deter the flow of high-skilled migrants since lower after-tax wages will reduce the incentive to migrate. However, low-skilled migrants will be taxed very little (if at all) in a country with progressive taxes, so they may have larger incentives to migrate. Migrant flows are found to be lower to countries with higher tax burdens [8] . Other types of taxes will similarly distort the migration decision. For example, countries with high consumption (or sales) taxes have higher costs of living (especially for low-income migrants). Similarly, capital gains taxes may discourage migrants who seek investment opportunities abroad.
The availability of social insurance programs appears to affect whether or not people migrate. Receiving access to strong health care and educational systems in the host country may increase the benefit of migration and serve as a strong pull factor. In fact, families often migrate abroad primarily for educational reasons; they want their children to have better educational opportunities than what are offered back home.
Countries with generous government transfers may attract migrants; these locales are often considered “welfare magnets.” The welfare magnet hypothesis suggests that migrants choose their destination based on the generosity of public assistance programs. Welfare payments, for example, can be viewed as a substitute for earnings during the time spent searching for a job. Government transfers may thus attract certain types of migrants that would be eligible for these types of benefits. Increasingly, governments are making it more difficult for migrants to receive government transfers (at least in the period immediately following migration). That is, migrants often have to work in the host country for a few years before becoming eligible for government benefits. Most of the empirical evidence on the subject finds that countries with more generous public assistance benefits, as measured by social expenditures as a percentage of GDP, do not necessarily attract more migrants [7] . However, migrants from the poorest countries of origin appear to be more likely to migrate to countries with relatively generous social programs.
Insurance and remittances
For many, the decision to migrate is a household-level decision. The family unit as a whole must decide whether the entire family will migrate or if it will send only one member of the household abroad. In this way, migration may be a form of insurance against uncertainty in the form of household- and macro-level shocks. Remittances are the primary insurance mechanism for migrants; they allow family members who migrate abroad to send a portion of their income back home. In addition, families often help to relax the tight borrowing constraints that potential migrants face, especially in helping to finance the move. Households may be motivated by the insurance that migration provides, either by smoothing out macroeconomic cycles over time and across countries, or through cash or in-kind transfers received from family members abroad. Overall, using migration as a form of household-level insurance against individual or aggregate shocks can help reduce volatility in household incomes and increase the net benefit to migration over time. For some families (especially in relatively poor countries of origin), remittances can represent a significant fraction of household income, compounding these effects.
Macroeconomics factors: Exchange rates, business cycles, unemployment
Besides income (as measured by per capita GDP), many other macroeconomic factors impact the migration decision. Accordingly, exchange rates directly affect relative prices abroad and, hence, purchasing power. A strong foreign currency (relative to the currency in the country of origin) will allow the money earned abroad to buy more back home, increasing the net benefit to migration. Conversely, a strong home currency will reduce the value of earnings abroad. Exchange rates operate through both wages and remittances in affecting the migration decision.
As mentioned above, income volatility driven by business cycles (e.g. macroeconomic fluctuations) or changes in employment status can push migrants out of countries of origin or pull them into host countries. High unemployment in a country of origin may act as a push factor. In addition, migrants typically move to regions with low unemployment. Conversely, in times of economic recession, high unemployment rates in a host country deter migration, as evident in the 2007−2009 global financial crisis and the Covid pandemic in 2020.
Well-being, ability, optimism, aspirations, and risk tolerance
Many other non-economic factors may influence the decision to migrate as well. Measures of well-being (or happiness) are related to aggregate well-being and migrant flows. For example, fewer people move out of relatively unhappy countries as aggregate happiness levels increase. Optimism may also affect the migration decision: the net benefit of migration may seem larger for an optimistic person than for a pessimistic person. Researchers have found some evidence indicating that migrants tend to be more optimistic than non-migrants.
Moreover, people's innate ability, ambitions, or risk aversion likely form part of the migration decision. It could be argued that ambitious people are more likely to migrate, while people who are risk averse may not be willing to gamble by migrating to a new country (the costs of migration may seem insurmountable to them). However, many of these traits are unobservable in the data as they can be difficult to quantify.
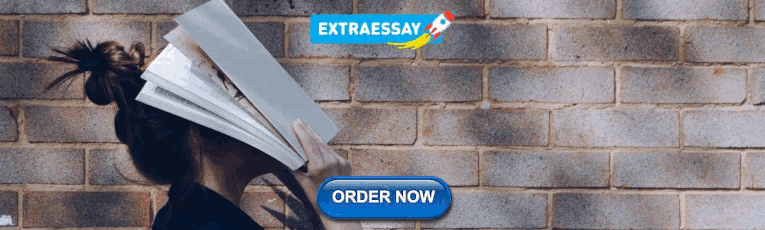
Hurdles to migration and other factors
There are several factors that make migration more difficult; some of these factors reduce the net benefits of migration, while most directly increase the costs, thereby reducing the net present value (NPV) of migration and the incentives to migrate.
Migration costs
Obviously, the direct economic costs of migration include the travel costs associated with the move. These generally depend on the distance traveled as airfare and other travel-related costs typically increase with distance. The so-called gravity model of migration explicitly considers the role of distance in estimating migrant flows. In nearly every study where the gravity model is employed, distance is significantly and negatively correlated with migrant flows. In addition, people often have to pay significant fees for visas and resident permits to gain legal entry. Visa fees and costs for permits vary significantly across both origin and host countries. In addition to direct costs, there are indirect costs of migration. For example, the psychological costs of leaving family and friends back home likely reduce the likelihood of migration.
In addition, migration is an uncertain endeavor, so people seek information about the potential host country. These information costs (e.g. time) can be high but are constantly decreasing due to technological progress. A potential migrant can now acquire better information about the availability of housing, jobs, and government benefits, for example, in advance of making the decision to migrate. Technological improvements thereby lower migration costs, which, in turn, increase migrant flows.
Immigration policy
Immigration policy plays a significant role in limiting the flow of international migrants. Some countries make it difficult for people to leave; however, more common is to restrict the number of people entering a country each year. When estimating the determinants of migration, it is important to control for host country immigration policy. Immigration policy can vary over time, so actual and/or expected changes in immigration policy impact the NPV of migration and alter the flow of migrants. The extent to which a country protects its borders and imposes its immigration policy is important; countries which are known to be relatively easy to enter may be more attractive to potential migrants. In contrast, when countries tighten their immigration policies, the costs of migrating may become sufficiently high to discourage migration. For example, when the US puts an emphasis on border enforcement and deportation, it is more difficult to migrate to the US. Immigration policies may also alter the effect of push and pull factors; for example, policies that are more restrictive may prevent some people from migrating, even if income differentials are significant.
In most theoretical models of migration, immigration policy serves as a proxy for migration costs. Countries with relaxed immigration policy will be less costly to move to, while countries with tight policy (i.e. well-enforced) will be more costly. Consider the extreme case in which no people are allowed to migrate—the costs of migration always exceed the benefits. For all other cases, a positive flow of migrants suggests that the net benefits of migration exceed the costs for some migrants.
Demographic characteristics
The characteristics of potential migrants have long been a central part of explaining who migrates and who does not. It is important to note that those who migrate for work purposes can be quite different from those who migrate for family reunification or refugee/asylum status. Moreover, each migrant flow is unique in terms of the demographic characteristics, including age, gender, marital status, educational attainment, and so on. Labor migrants are often young, since they will have more time to realize the expected benefits of migration. Young migrants from neighboring countries are often attracted to destination countries with older populations indicating that demographic differences are important in explaining migration patterns [9] . Historically, labor migrants were typically working-age men, but, increasingly, migrant flows consist of women and families in search of employment abroad (and in some cases joining families already in the host country). Marital status is also important, with married people typically being less likely to migrate on their own and more likely to return home sooner.
The reasons driving the migration decision may be very different depending on the skill or education level of the migrant. In fact, there are important differences in the response of recent migrant flows on the basis of health and language. For example, studies typically find that migrants are healthier than non-migrants in the origin country and natives in the host country, and migration rates are higher among people who speak the same language as the host country. In fact, emigration rates are 14% to 20% higher among countries whose languages are linguistically similar compared to those that are linguistically distant [10] . Speaking the same language and being healthier may lower migration costs since the transitions into the culture and work are easier.
Overall, nearly every study of migration finds a significant role for demographic characteristics in explaining migrant populations. The set of characteristics that matters most varies significantly over time and across specific migrant flows.
Politics, attitudes, climate, and environmental conditions
When countries are different from one another in terms of regulations and political situations, the costs of migrating between those countries could be quite high and may reduce the NPV of migration. Moreover, worsening political conditions abroad and/or improving conditions at home can reduce the incentive to migrate. Significant differences in the political conditions and human rights between countries may partially explain why people do not migrate between them. For example, women in countries with very few rights for women may not be able to leave without the permission of their husband or father. Women in these countries experience bad labor market outcomes so may not be able to afford to migrate, even if allowed. As women's rights improve, female migration often increases.
Environmental conditions may push migrants out of their country of origin, especially in the short term. Natural disasters can lead to a dramatic uptick in migration as households experience a large sudden (and often uninsurable) shock. Famines, much like natural disasters, can also push people out of their home country. Temperature changes may lead to out-migration but only in agriculture-dependent countries [11] . Improvements in the quality of life in the country of origin may also reduce the incentive to migrate. The consensus emerging is that climate change does not lead to large-scale international migration; however, climate can “trap” populations by tightening financial constraints, reducing the ability to migrate [12] .
The Covid-19 pandemic led to a sudden closure of international borders and a halt to worldwide migration. Migration restrictions were tightened abruptly, but will take years to fully unwind. While there are competing factors at play, the pandemic has likely reduced the incentives to migrate abroad due to increased worldwide uncertainty. Thus, economic and noneconomic factors will continue to drive international migration but some people may be less likely to migrate; decision-making processes may look very different in the future. There may be periods of unpredictable migration flows. In addition, countries may keep their Covid-era immigration restrictions in place, further complicating the process of measuring the responsiveness of worldwide migrant flows to various factors.
Other considerations
While political and environmental factors can act as hurdles to migration, they can also serve as push and pull factors. Complicated political circumstances that create uncertainty in the origin country can serve as a push factor, while countries that are well-functioning democratic societies may attract new migrants, thereby increasing the benefits of migration. Push factors related to a country's political situation include war, oppression, corruption, crime, or discrimination, while pull factors consist of established property rights, law and order, and freedom. Measures of political freedom produced by the Heritage Foundation, for example, are often included in empirical analyses and are found to be important predictors of migrant flows [13] .
War has been among the leading causes of large migrant flows historically, as recently evident in Syria and Ukraine. Repressive political regimes often drive people to leave their home country for fear of persecution, increasing the NPV of migration. Corruption often increases the cost of doing business, so many people who do not want to participate in bribery are pushed out of their (corrupt) origin country and pulled into a country with well-established property rights and rule of law. In addition, civil wars, upheavals, and human rights violations are well-documented push factors.
Push and pull factors affect the NPV calculation for all migrants, whether they are legal or illegal, low skilled or high skilled, young or old. It is important to note that some factors play bigger roles for certain migrant groups because of immigration policy. For example, if a country admits migrants based primarily on family preferences, the pull factor of family may matter a lot for legal migrants. Meanwhile, economic conditions may matter more for illegal migrants but are less important for refugees and asylum seekers. In many countries, illegal migrants enter mainly to work, while legal migrants enter for a wider variety of reasons, including family ties. Regardless of their legal status, low-skilled and high-skilled workers alike are affected by the push and pull of relative economic conditions. However, high-skilled workers may have more resources to cover the costs associated with migration since they are likely to have higher household income.
In addition, the timing of migration is important. In thinking through the net benefits of migration, an individual or household may decide to move abroad and have plans to return home at a certain point in time once they have maximized the benefits of living abroad, especially if they left family behind in the country of origin.
Limitations and gaps
As with most studies of migration, finding reliable cross-country data over time is nontrivial. Most countries keep track of people coming into their countries (immigrants), while very few countries accurately measure people moving out of their countries (emigrants). In addition, accurately measuring the flow of illegal migrants into a country is complicated (although not impossible).
In an ideal world, countries would keep track of people coming into and leaving their countries over time. Importantly, detailed data on each migrant would be available to researchers, including a complete set of demographic information, their migration history, country of origin, reasons for migration, and migrant status (e.g. the type of visa they entered under). Increasingly, countries are doing a better job of collecting some of this information, though detailed demographic information is not always included. Ethnographic surveys provide many demographic details but are often not representative of migrant flows as a whole. In addition, given the political sensitivities around setting migration policies, countries are often unwilling to share their comprehensive data sets with researchers. To complicate matters, relatively poor countries cannot invest in systems that can handle this level of data collection.
In order to better understand the various reasons behind complex migration decisions, governments will have to collect better data and then be willing to share them with researchers. The EU, for example, has been the most willing to both collect the relevant data and share them publicly, which has generated a sizable increase in the research done on EU migrant flows. International organizations such as the International Organization for Migration and the United Nations Population Division are helping overcome the hurdles by providing comparable migration data across countries and time. Ideally, linking migration data to administrative records will provide comprehensive data sets that can disentangle the various determinants of migration; Scandinavian countries are the leaders in this regard. Still, more countries, rich and poor alike, need to cooperate.
The Covid-19 pandemic likely altered how migration decisions are made. As a result, migrant flows may be unpredictable for the near future. Researches are just beginning the task of sorting out the various factors that influenced migration decisions during the pandemic. The post-Covid immigration policy responses need to be analyzed.
Summary and policy advice
Researchers have analyzed a variety of migrant flows using a multitude of empirical approaches to be able to tease out the key drivers of migration. Income differentials, migrant networks, and demographic factors are found to be robust predictors of migrant flows. Many other factors identified in this article have also been found to be correlated with migrant flows; however, their ability to predict flows is lower.
Policymakers must carefully consider the key set of determinants for the particular flow of migrants entering their country. It would be difficult to set effective policy without first understanding both the push and pull factors. For example, if the goal is to reduce the number of migrants entering a country in cases when network effects are most relevant, then policy should be changed to be centered less on family preferences. Conversely, if income differentials are driving flows, perhaps migrants could be more heavily taxed (via entry or visa fees) to reduce flows.
Having reliable estimates about how migrant flows respond to fluctuations in GDP or unemployment rates, for example, could be particularly helpful for governments who want to set policy to slow the outflow or inflow of migrants. Likewise, suppose policymakers had a better understanding of the extent to which households in a certain country use migration as an insurance mechanism to buffer against fluctuations in household income: governments could work with non-governmental organizations to develop affordable household insurance schemes as a way of reducing the incentive to migrate. Or suppose host countries had a better understanding of the demographic characteristics of their specific migrant populations so that services could be targeted specifically toward their needs to ensure better integration into the host country. Perhaps most importantly, if a government could quantify (with relative certainty) the change in migrant flows as a result of a change in migrant policy (i.e. increasing visa application fees or granting amnesty to undocumented migrants), governments could make well-informed policy decisions.
Acknowledgments
The author thanks anonymous referees and the IZA World of Labor editors for many helpful suggestions on earlier drafts. Previous work of the author contains a larger number of background references for the material presented here and has been used intensively in parts of this article [2] . Version 2 of the article updates the figures, includes information on migration after the Covid-19 pandemic, and adds new “Further reading” and “Key references” [1] , [3] , [9] , [10] , [11] , [12] .
Competing interests
The IZA World of Labor project is committed to the IZA Code of Content . The author declares to have observed the principles outlined in the code.
© Nicole B. Simpson
evidence map
- show one-pager
Full citation
Data source(s), data type(s).
How can we improve IZA World of Labor? Click here to take our 5-minute survey and enter a prize draw.
2021 Theses Doctoral
Three Essays on International Migration
Huang, Xiaoning
Today, there are about 250 million international migrants globally, and the number is increasing each year. Immigrants have contributed to the global economy, bridged cultural and business exchanges between host and home countries, and increased ethnic, racial, social, and cultural diversity in the host societies. Immigrants have also been overgeneralized about, misunderstood, scapegoated, and discriminated against. Understanding what drives international migration, who migrate, and how immigrants fare in destination has valuable theoretical, practical, and policy implications. This dissertation consists of three essays on international immigration. The first paper aims to test a series of immigration theories by studying immigrant skill-selection into South Africa and the United States. Most of the research on the determinants of immigrant skill selection has been focusing on immigrants in the United States and other developed destination countries. However, migration has been growing much faster in recent years between developing countries. This case study offers insights into the similarities and differences of immigration theories within the contexts of international migration into South Africa and the US. This project is funded by the Hamilton Research Fellowship of Columbia School of Social Work. The second paper narrows down the focus onto Asian immigrants in the United States, studying how the skill-selection of Asian immigrants from different regions has evolved over the past four decades. Asian sending countries have experienced tremendous growth in their economy and educational infrastructure. The rapid development provides an excellent opportunity to test the theories on the associations between emigrants’ skill-selection and sending countries’ income, inequality, and education level. On the other hand, during the study period, the United States has had massive expansion employment-based immigration system, followed by cutbacks in immigration policies. I study the association between immigration patterns and these policies to draw inferences on how the changes in immigration policies have affected the skill selection of Asian immigrants. This research is funded by Columbia University Weatherhead East Asia Institute’s Dorothy Borg Research Program Dissertation Research Fellowship. The third paper centers on the less-educated immigrant groups in the US and investigates the gap in welfare use between less-educated immigrant and native households during 1995-2018, spanning periods of economic recessions and recoveries, changes in welfare policy regimes, and policies towards immigrants. I use “decomposition analysis” to study to what extend demographic factors, macroeconomic trends, and welfare and immigration policy could explain the disparities in welfare participation between immigrants and natives. This paper is co-authored with Dr. Neeraj Kaushal from Columbia School of Social Work and Dr. Julia Shu-Huah Wang from the University of Hong Kong. The work has been published in Population Research and Policy Review (doi.org/10.1007/s11113-020-09621-8).
Geographic Areas
- South Africa
- United States
- Social service
- Immigrants--Economic aspects
- Immigrants--Social conditions
- Race discrimination
- Immigrants--Education
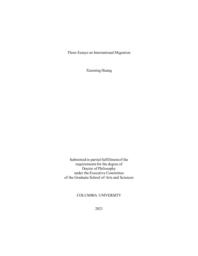
More About This Work
- DOI Copy DOI to clipboard
Numbers, Facts and Trends Shaping Your World
Read our research on:
Full Topic List
Regions & Countries
- Publications
- Our Methods
- Short Reads
- Tools & Resources
Read Our Research On:
- Global Migration and Demography
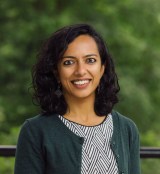
Sahana Mukherjee
Associate Director, Research
Global Migration and Demography Race and Ethnicity
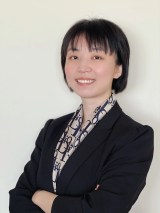
Research Associate
Demographics and Social Trends Global Migration and Demography Race and Ethnicity
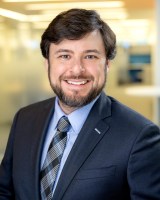
Jens Manuel Krogstad
Senior Writer and Editor
Global Migration and Demography Hispanic Trends Race and Ethnicity
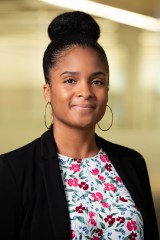
Khadijah Edwards
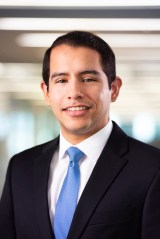
Luis Noe-Bustamante
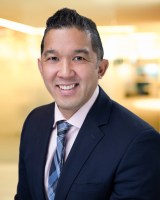
Neil G. Ruiz
Head of New Research Initiatives
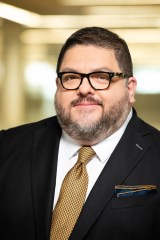
Mark Hugo Lopez
Director, Race and Ethnicity Research
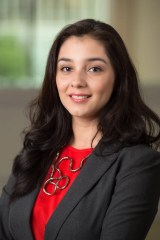
Ana Gonzalez-Barrera
Former Senior Researcher
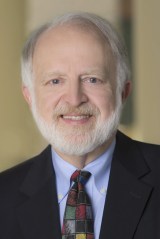
Jeffrey S. Passel
Senior Demographer
Global Migration and Demography Hispanic Trends
Filter By Expertise
- Communications Strategy
- Demographics and Social Trends
- Digital Strategy
- Global Attitudes and Trends
- Hispanic Trends
- Internet and Technology
- News and Information
- Race and Ethnicity
- Religion and Public Life
- Research Methodology
- Science and Society
- U.S. Politics and Policy
1615 L St. NW, Suite 800 Washington, DC 20036 USA (+1) 202-419-4300 | Main (+1) 202-857-8562 | Fax (+1) 202-419-4372 | Media Inquiries
Research Topics
- Age & Generations
- Coronavirus (COVID-19)
- Economy & Work
- Family & Relationships
- Gender & LGBTQ
- Immigration & Migration
- International Affairs
- Internet & Technology
- Methodological Research
- News Habits & Media
- Non-U.S. Governments
- Other Topics
- Politics & Policy
- Race & Ethnicity
- Email Newsletters
ABOUT PEW RESEARCH CENTER Pew Research Center is a nonpartisan fact tank that informs the public about the issues, attitudes and trends shaping the world. It conducts public opinion polling, demographic research, media content analysis and other empirical social science research. Pew Research Center does not take policy positions. It is a subsidiary of The Pew Charitable Trusts .
Copyright 2024 Pew Research Center
Terms & Conditions
Privacy Policy
Cookie Settings
Reprints, Permissions & Use Policy
Global migration’s impact and opportunity
Migration is a key feature of our increasingly interconnected world . It has also become a flashpoint for debate in many countries, which underscores the importance of understanding the patterns of global migration and the economic impact that is created when people move across the world’s borders. A new report from the McKinsey Global Institute (MGI), People on the move: Global migration’s impact and opportunity , aims to fill this need.
Refugees might be the face of migration in the media, but 90 percent of the world’s 247 million migrants have moved across borders voluntarily, usually for economic reasons. Voluntary migration flows are typically gradual, placing less stress on logistics and on the social fabric of destination countries than refugee flows. Most voluntary migrants are working-age adults, a characteristic that helps raise the share of the population that is economically active in destination countries.
By contrast, the remaining 10 percent are refugees and asylum seekers who have fled to another country to escape conflict and persecution. Roughly half of the world’s 24 million refugees are in the Middle East and North Africa, reflecting the dominant pattern of flight to a neighboring country. But the recent surge of arrivals in Europe has focused the developed world’s attention on this issue. A companion report, Europe’s new refugees: A road map for better integration outcomes , examines the challenges and opportunities confronting individual countries.
While some migrants travel long distances from their origin countries, most migration still involves people moving to neighboring countries or to countries in the same part of the world (exhibit). About half of all migrants globally have moved from developing to developed countries—indeed, this is the fastest-growing type of movement. Almost two-thirds of the world’s migrants reside in developed countries, where they often fill key occupational shortages . From 2000 to 2014, immigrants contributed 40 to 80 percent of labor-force growth in major destination countries.

Moving more labor to higher-productivity settings boosts global GDP. Migrants of all skill levels contribute to this effect, whether through innovation and entrepreneurship or through freeing up natives for higher-value work. In fact, migrants make up just 3.4 percent of the world’s population, but MGI’s research finds that they contribute nearly 10 percent of global GDP. They contributed roughly $6.7 trillion to global GDP in 2015—some $3 trillion more than they would have produced in their origin countries. Developed nations realize more than 90 percent of this effect.
Would you like to learn more about the McKinsey Global Institute ?
Employment rates are slightly lower for immigrants than for native workers in top destinations, but this varies by skill level and by region of origin. Extensive academic evidence shows that immigration does not harm native employment or wages, although there can be short-term negative effects if there is a large inflow of migrants to a small region, if migrants are close substitutes for native workers, or if the destination economy is experiencing a downturn.
Realizing the benefits of immigration hinges on how well new arrivals are integrated into their destination country’s labor market and into society. Today immigrants tend to earn 20 to 30 percent less than native-born workers. But if countries narrow that wage gap to just 5 to 10 percent by integrating immigrants more effectively across various aspects of education, housing, health, and community engagement, they could generate an additional boost of $800 billion to $1 trillion to worldwide economic output annually. This is a relatively conservative goal, but it can nevertheless produce broader positive effects, including lower poverty rates and higher overall productivity in destination economies.
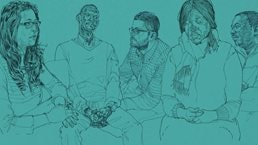
People on the move: Migrant voices
A series of portraits tells migrants’ stories—part of the 'i am a migrant' campaign.
The economic, social, and civic dimensions of integration need to be addressed holistically. MGI looked at how the leading destinations perform on 18 indicators and found that no country has achieved strong integration outcomes across all of these dimensions, though some do better than others. But in destinations around the world, many stakeholders are trying new approaches. We identify more than 180 promising interventions that offer useful models for improving integration. The private sector has a central role to play in this effort—and incentives to do so. When companies participate, they stand to gain access to new markets and pools of new talent.
The stakes are high. The success or failure of integration can reverberate for many years, influencing whether second-generation immigrants become fully participating citizens who reach their full productive potential or remain in a poverty trap.
Lola Woetzel , Jacques Bughin , and James Manyika are directors of the McKinsey Global Institute, where Anu Madgavkar is a partner and Ashwin Hasyagar is a fellow; Khaled Rifai is a partner in McKinsey’s New York office, Frank Mattern is a senior partner in the Frankfurt office, and Tarek Elmasry and Amadeo Di Lodovico are senior partners in the Dubai office.
Explore a career with us
Related articles.
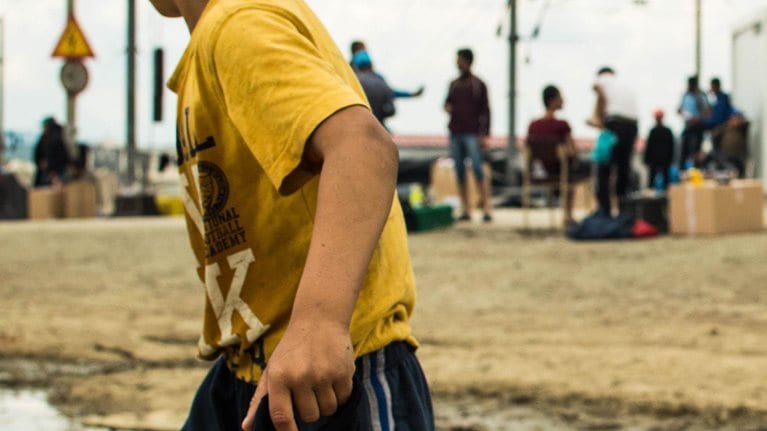
A road map for integrating Europe’s refugees

Creating a global framework for immigration
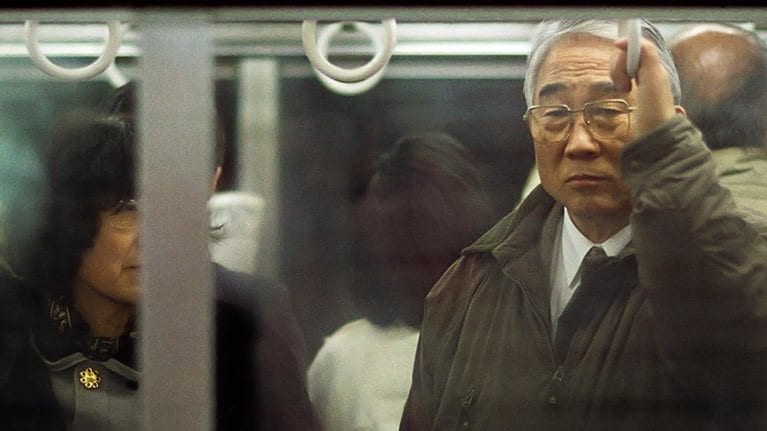
Urban world: Meeting the demographic challenge in cities
Utility Links
- University of Pennsylvania
- Office of the Provost
- Penn Global
Secondary Nav Perry World House
Primary nav perry world house, drawer menu perry world house.
- Back to main menu
- Meet Our Staff
- Announcements
- Join Our Mailing List
- Our Building
- All Experts 2023-24
- Distinguished Global Leaders
- Non-Resident Senior Advisor
- Visiting Fellows and Visiting Scholars Programs
- Apply to Become or Nominate a Visiting Fellow or Visiting Scholar
- All Distinguished Visiting Fellows
- All Visiting Scholars
- Distinguished Scholars-in-Residence
- All Lightning Scholars
- All Postdoctoral Fellows
- Borders and Boundaries Postdoctoral Fellowships
- Global Innovation Program Postdoctoral Fellowships
- Penn Identity & Conflict Lab Postdoctoral Fellowship
- Faculty Fellows 2023-24
- Report: Responses Against China's Coercion in the Indo-Pacific: Developing a Toolkit from the Philippines and Taiwan
- 2023 Global Order Colloquium | A New Age of Nuclearity? Great Powers and Greater Consequences
- Workshop | The Future of Nuclear Weapons, Statecraft, and Deterrence After Ukraine: April 2023
- Workshop | Economic Security and the Future of the Global Order in the Indo-Pacific: February 2023
- A Fracturing World: The Future of Globalization | Report and Thought Pieces
- 2022 Global Order Colloquium | A Fracturing World: The Future of Globalization: September 13-14, 2022
- Workshop | The Global Order After Russia's Invasion of Ukraine: April 2022
- Workshop | Challenges and Opportunities at the Dawn of the New Space Age: March 2022
- 2021 Global Order Colloquium Report and Thought Pieces
- Keeping Score: A New Approach to Geopolitical Forecasting
- 2023 Global Shifts Colloquium | Living with Extreme Heat: Our Shared Future
- Global Climate Finance Workshop Report and Thought Pieces | October 3, 2022
- Islands on the Climate Front Line: Risk and Resilience | Report and Thought Pieces
- Borders and Boundaries Project Personnel
- Call for Papers: 2022 Conference on International Borders in a Globalizing World
Global Climate Security Atlas
- Cities, Geopolitics, and the International Legal Order Report and Thought Pieces
- Global Justice and Human Rights
- Past Seminar Series
- Publications
- The World Today
- Global Lens
- Critical Conversations
- Event Policies
- Event Inventory
- IO COVID-19 Online Supplemental Issue
- Penn on the World after COVID-19
- Penn Pandemic Diary
- 'The Global Cable' Podcast
- Apply to Become a Faculty Affiliate
- Faculty Fellows 2022-23
- Lightning Scholars
- Global Career Week 2022
- Meet our 2023-24 World House Student Fellows
- Past World House Student Fellows
- World House Student Fellows Policy Projects
- World House Student Fellows Summer Awards
- Past Graduate Associates
- Graduate Associates Policy Papers
- House Committee
- Undergraduate Essay Prize
- Graduate Essay Prize
- Summer Internship Program
- Attend an Event
- Global Policy Course Enrichment Grants
- International Visitors Grant Program
- Perry World House-Foreign Affairs Emerging Scholars Policy Prize
Global Shifts: Urbanization, Migration, and Climate Change
Basic page sidebar menu perry world house.
Ambassador Thilmeeza Hussain, permanent representative of Maldives to the UN, speaks at the 2022 Global Shifts Colloquium | Photo by Eddy Marenco
Today, tens of millions of people move and are regularly displaced around the world through a combination of forces – environmental, societal, political, and security. Human movement and adaptation in response to ever changing catalysts has always been a salient feature of the global landscape.
Whether due to protracted conflict or political unrest, climate change or demographic shifts, human movement is often essential for communities to survive and thrive. Simultaneously, rapid urbanization has made cities home to 70 percent of the world’s population, resulting in a trend toward cities playing increasingly important roles on the world stage and being in the vanguard for global solutions.
At a moment when the world is seeing the greatest level of displacement since World War II, and the climate emergency grows more acute by the day, these complex and intersecting global phenomena – urbanization, migration, and climate change – demand sophisticated and coordinated action from researchers and policymakers working in concert.
The Global Shifts research theme examines these phenomena, highlights the specific challenges their intersections produce, and charts a path that allows for the best global policy responses to emerge.

Global Shifts Colloquium: Living with Extreme Heat: Our Shared Future | March 21-22, 2023
Perry World House’s 2023 Global Shifts Colloquium will explore how to manage the growing global threat of extreme heat, as climate change causes temperatures to spike around the world. It will feature two public events: one focused on building urban resilience to extreme heat, featuring Chief Heat Officers from several major cities; and one on the dangers of extreme heat, featuring bestselling author and journalist Jeff Goodell.
Workshop: Global Climate Finance | October 3, 2022
Together with the Climate Center of the Environmental, Social and Governance Initiative at the Wharton School, Perry World House convened this workshop to examine the role of finance in addressing the climate emergency. It explored how to increase finance to meet adaptation needs globally, especially for those countries most vulnerable to climate impacts, as well as how to assure that financial resources were made more accessible and impactful. The convening also explored the international financial architecture needed to meet the most ambitious of global climate goals and spur a just transition to a post-carbon world.
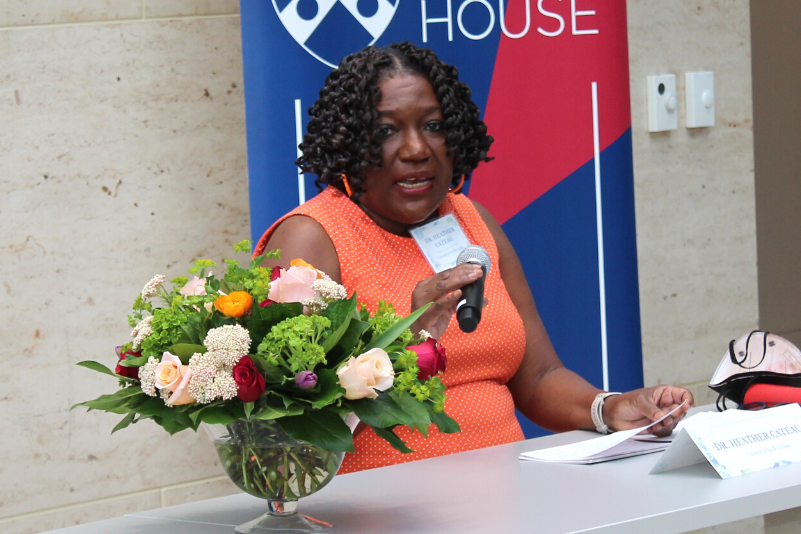
Global Climate Finance Workshop Report and Thought Pieces
This report and short series of thought pieces explore\ financial policy solutions to the climate crisis, the role the private sector should play, and how existing international systems can be changed to better support climate adaptation and mitigation.
2022 Global Order Colloquium Report and Thought Pieces
This report looks back at Perry World House’s 2022 Global Shifts Colloquium, "Islands on the Climate Front Line: Risk and Resilience." This event explored how small island developing states, or SIDS – which are among the most climate vulnerable countries in the world – are responding to the climate emergency. Alongside the report, we released a series of thought pieces from experts who attended the convening, examining how to
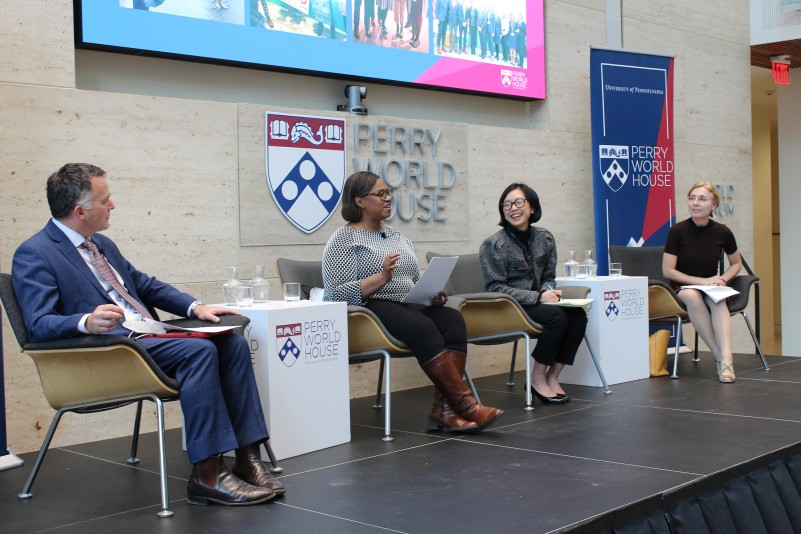
Borders and Boundaries Project
Do international political borders matter in the modern world, and, if so, in what ways? The Borders and Boundaries Project at Perry World House researches how political life both affects and is affected by international borders and border security policies. This interdisciplinary, multi-method effort is directed by Professor Beth Simmons and is composed of research teams studying border politics across a variety of different research areas.
Perry World House's Global Climate Security Atlas is an online climate data visualization project. Bringing over 200 geospatial climate-related datasets together into one place, the website layers these datasets over a map of the world. Users can use this map to compare physical climate projections, environmental and ecological data, and information on social and political systems.
Great Powers and Urbanization Project
Over the course of the early twenty-first century, cities have increasingly moved into the international arena, taking on a growing role in global issues. Great power politics and urbanization are not separate phenomena. Peace, power, and prosperity in the twenty-first century will require urban expertise, as will solving global problems around climate change, migration, and equitable development. The Great Powers and Urbanization Project, or GPUP, is a collaboration of global leaders in international and urban affairs: the University of Pennsylvania’s Perry World House, the University of Melbourne’s Connected Cities Lab , the Chicago Council on Global Affairs , and the Argentine Council for International Relations ( Consejo Argentino para las Relaciones Internacionales ) .
3803 Locust Walk
Philadelphia, PA 19104
T. 215-573-5730
F. 215-573-9371
©2024 University of Pennsylvania, Philadelphia, PA 19104
Footer Menu
- Report Accessibility Issues and Get Help
- Privacy Policy
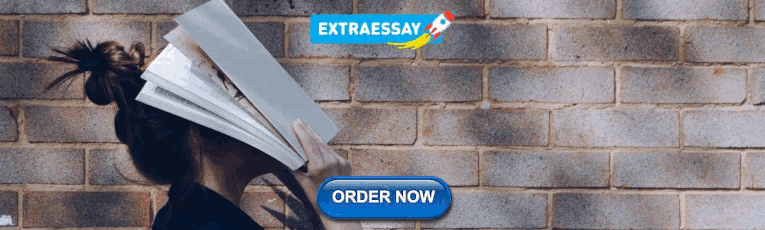
IMAGES
VIDEO
COMMENTS
Demographic projections can be quite reliable, but huge uncertainties - in the realms of health, changes in human life span, scientific advances, migration, global warming and wars - make overall predictions extremely uncertain. . David E Bloom is Professor of Economics and Demography at the Harvard School of Public Health
Shifts in global population distribution have long been rooted in a "slow demography paradigm" (Billari 2022) whereby gradual shifts in population size and structure occur in response to differentials in fertility and mortality.In contrast, migration has the potential to generate rapid shifts in population size, composition, and distribution, particularly at the local level.
Introduction. The steady growth of international labor migration is an important, yet underappreciated, aspect of globalization. 1 In 1970, just 78 million people, or about 2.1% of the global population, lived outside their country of birth.By 1990, that number had nearly doubled to more than 150 million people, or about 2.8% of the global population (United Nations Population Division, 2012).
By 2011, Japan had reached a GNP per capita at Purchasing Power Parity (PPP) of US$ 32,195 compared with the Republic of Korea at US$ 28,230 (UNDP, 2011). The internal migration in the largest ...
Introduction. It is commonly assumed that international migration has accelerated over the past fifty years, that migrants travel over increasingly long distances, and that migration has become much more diverse in terms of origins and destinations of migrants (Arango, 2000: 291).In this context, Vertovec coined the term "super-diversity" to indicate the unprecedented degree of immigrant ...
Figure 6. Annual domestic migration rate, United States of America, 1947-1948 to 2008-2009. Figure 7. Demographic profile of Ghana, 2000-2050. However, massive and increasing migration throughout ...
Abstract. In the past 50 years, the world accelerated its transition out of long-term demographic stability. As infant and child mortality rates fell, populations began to soar. In most countries, this growth led to falling fertility rates. Although fertility has fallen, the population continues to increase because of population momentum; it ...
This paper sheds light on the global migration patterns of the past 40 years, and produces migration projections for the 21st century. To do this, we build a simple model of the world economy, and we parameterize it to match the economic and socio-demographic characteristics of the world in the year 2010. We conduct backcasting and nowcasting exercises, which demonstrate that our model fits ...
The fact that global migration is highly concentrated in a relatively low number of migration corridors (around 20 percent of world migration is within 15 bilateral corridors), partly reflects such colonial and other historical ties between states (Czaika and de Haas 2014; Flahaux and de Haas 2016; Vezzoli and Flahaux 2017; Natter 2014; Vezzoli ...
In the past 50 years, the world accelerated its transition out of long-term demographic stability. As infant and child mortality rates fell, populations began to soar. In most countries, this growth led to falling fertility rates. Although fertility has fallen, the population continues to increase because of population momentum; it will eventually level off. In the meantime, demographic change ...
Strict migration policy acts as a constraint in the migration decision. Demographic factors such as age, education, marital status, and language impact a person's willingness to migrate. Worsening political and environmental conditions abroad and/or improving conditions at home can lead to less migration.
and expected global demographic transition. Section III reviews the recent literature that has explored the macroeconomic implications of demographic change and describes the model that we employ. Section IV presents our baseline results estimating the impact of demographic change from 2005 until 2080, and Section V examines how sensitive these are
The demographic crisis and global migration…. Changes in birth rate (which has been falling gradually since the 1950s) and death. rate have resulted in a decline in the growth rate of the world ...
Three Essays on International Migration. Today, there are about 250 million international migrants globally, and the number is increasing each year. Immigrants have contributed to the global economy, bridged cultural and business exchanges between host and home countries, and increased ethnic, racial, social, and cultural diversity in the host ...
How environmental change affects human populations. While population dynamics influence the environment and the global climate, at the same time human beings are affected by changes in the environmental and climate systems as shown in Figure 1.The consequences of global warming on human health and well-being are already being felt (Watts et al. Citation 2021).
Neil G. Ruiz. Head of New Research Initiatives. Demographics and Social Trends, Global Migration and Demography, Race and Ethnicity.
In fact, migrants make up just 3.4 percent of the world's population, but MGI's research finds that they contribute nearly 10 percent of global GDP. They contributed roughly $6.7 trillion to global GDP in 2015—some $3 trillion more than they would have produced in their origin countries. Developed nations realize more than 90 percent of ...
The demographic regimes of modern affluent societies are long life, below-replacement fertility (sometimes, well below large and increasing proportions of elderly, and appreciable. poorer areas. Their main population problems, gauged by. demic scrutiny and public debate, are concerned with the last aging and immigration.
The recent books Exodus: How Migration is Changing Our World by Paul Collier and The Price of Rights: Regulating International Labor Migration by Martin Ruhs raise a number of questions about the underlying economic model. The essay shows how these concerns can greatly attenu-ate the predicted gains. ( JEL F22, F66, J11, J18, J61) 1. Introduction.
Perry World House's 2023 Global Shifts Colloquium will explore how to manage the growing global threat of extreme heat, as climate change causes temperatures to spike around the world. It will feature two public events: one focused on building urban resilience to extreme heat, featuring Chief Heat Officers from several major cities; and one ...
DEMOGRAPHIC TRANSITION MODEL Grover 2014 explained that: -population demographics continued to evolve as a result of the relationship between birth and death rates within a country. -DMT uses death rates and birth rates -the observation and the documentation of this global phenomenon produced a model. Lesthaege (2010) -The declines in fertility ...
Global Demography Global demography. is the study of population globally based on factors such as: age, race, sex, and a study how birth rates and death rates change. It encompasses the study of the size, structure, and distribution of these populations and temporal changes in them in response to birth, aging, death, or incidence of disease.
2. Global Demographic Trends and Patterns. The global population, which stood at just over 2 billion in 1950, is 6.5 billion today. The world is currently gaining new inhabitants at a rate of 76 million people a year (representing the difference, in 2005, between 134 million births and 58 million deaths).