- Bipolar Disorder
- Therapy Center
- When To See a Therapist
- Types of Therapy
- Best Online Therapy
- Best Couples Therapy
- Best Family Therapy
- Managing Stress
- Sleep and Dreaming
- Understanding Emotions
- Self-Improvement
- Healthy Relationships
- Student Resources
- Personality Types
- Guided Meditations
- Verywell Mind Insights
- 2024 Verywell Mind 25
- Mental Health in the Classroom
- Editorial Process
- Meet Our Review Board
- Crisis Support
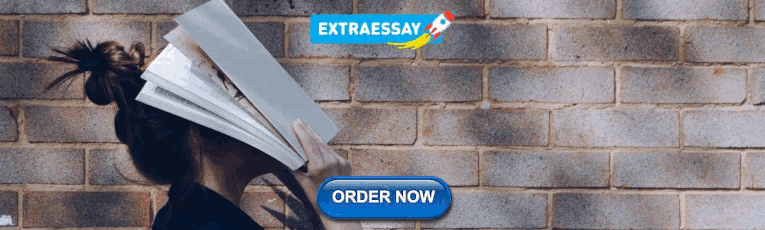
How to Write a Great Hypothesis
Hypothesis Definition, Format, Examples, and Tips
Kendra Cherry, MS, is a psychosocial rehabilitation specialist, psychology educator, and author of the "Everything Psychology Book."
:max_bytes(150000):strip_icc():format(webp)/IMG_9791-89504ab694d54b66bbd72cb84ffb860e.jpg)
Amy Morin, LCSW, is a psychotherapist and international bestselling author. Her books, including "13 Things Mentally Strong People Don't Do," have been translated into more than 40 languages. Her TEDx talk, "The Secret of Becoming Mentally Strong," is one of the most viewed talks of all time.
:max_bytes(150000):strip_icc():format(webp)/VW-MIND-Amy-2b338105f1ee493f94d7e333e410fa76.jpg)
Verywell / Alex Dos Diaz
- The Scientific Method
Hypothesis Format
Falsifiability of a hypothesis.
- Operationalization
Hypothesis Types
Hypotheses examples.
- Collecting Data
A hypothesis is a tentative statement about the relationship between two or more variables. It is a specific, testable prediction about what you expect to happen in a study. It is a preliminary answer to your question that helps guide the research process.
Consider a study designed to examine the relationship between sleep deprivation and test performance. The hypothesis might be: "This study is designed to assess the hypothesis that sleep-deprived people will perform worse on a test than individuals who are not sleep-deprived."
At a Glance
A hypothesis is crucial to scientific research because it offers a clear direction for what the researchers are looking to find. This allows them to design experiments to test their predictions and add to our scientific knowledge about the world. This article explores how a hypothesis is used in psychology research, how to write a good hypothesis, and the different types of hypotheses you might use.
The Hypothesis in the Scientific Method
In the scientific method , whether it involves research in psychology, biology, or some other area, a hypothesis represents what the researchers think will happen in an experiment. The scientific method involves the following steps:
- Forming a question
- Performing background research
- Creating a hypothesis
- Designing an experiment
- Collecting data
- Analyzing the results
- Drawing conclusions
- Communicating the results
The hypothesis is a prediction, but it involves more than a guess. Most of the time, the hypothesis begins with a question which is then explored through background research. At this point, researchers then begin to develop a testable hypothesis.
Unless you are creating an exploratory study, your hypothesis should always explain what you expect to happen.
In a study exploring the effects of a particular drug, the hypothesis might be that researchers expect the drug to have some type of effect on the symptoms of a specific illness. In psychology, the hypothesis might focus on how a certain aspect of the environment might influence a particular behavior.
Remember, a hypothesis does not have to be correct. While the hypothesis predicts what the researchers expect to see, the goal of the research is to determine whether this guess is right or wrong. When conducting an experiment, researchers might explore numerous factors to determine which ones might contribute to the ultimate outcome.
In many cases, researchers may find that the results of an experiment do not support the original hypothesis. When writing up these results, the researchers might suggest other options that should be explored in future studies.
In many cases, researchers might draw a hypothesis from a specific theory or build on previous research. For example, prior research has shown that stress can impact the immune system. So a researcher might hypothesize: "People with high-stress levels will be more likely to contract a common cold after being exposed to the virus than people who have low-stress levels."
In other instances, researchers might look at commonly held beliefs or folk wisdom. "Birds of a feather flock together" is one example of folk adage that a psychologist might try to investigate. The researcher might pose a specific hypothesis that "People tend to select romantic partners who are similar to them in interests and educational level."
Elements of a Good Hypothesis
So how do you write a good hypothesis? When trying to come up with a hypothesis for your research or experiments, ask yourself the following questions:
- Is your hypothesis based on your research on a topic?
- Can your hypothesis be tested?
- Does your hypothesis include independent and dependent variables?
Before you come up with a specific hypothesis, spend some time doing background research. Once you have completed a literature review, start thinking about potential questions you still have. Pay attention to the discussion section in the journal articles you read . Many authors will suggest questions that still need to be explored.
How to Formulate a Good Hypothesis
To form a hypothesis, you should take these steps:
- Collect as many observations about a topic or problem as you can.
- Evaluate these observations and look for possible causes of the problem.
- Create a list of possible explanations that you might want to explore.
- After you have developed some possible hypotheses, think of ways that you could confirm or disprove each hypothesis through experimentation. This is known as falsifiability.
In the scientific method , falsifiability is an important part of any valid hypothesis. In order to test a claim scientifically, it must be possible that the claim could be proven false.
Students sometimes confuse the idea of falsifiability with the idea that it means that something is false, which is not the case. What falsifiability means is that if something was false, then it is possible to demonstrate that it is false.
One of the hallmarks of pseudoscience is that it makes claims that cannot be refuted or proven false.
The Importance of Operational Definitions
A variable is a factor or element that can be changed and manipulated in ways that are observable and measurable. However, the researcher must also define how the variable will be manipulated and measured in the study.
Operational definitions are specific definitions for all relevant factors in a study. This process helps make vague or ambiguous concepts detailed and measurable.
For example, a researcher might operationally define the variable " test anxiety " as the results of a self-report measure of anxiety experienced during an exam. A "study habits" variable might be defined by the amount of studying that actually occurs as measured by time.
These precise descriptions are important because many things can be measured in various ways. Clearly defining these variables and how they are measured helps ensure that other researchers can replicate your results.
Replicability
One of the basic principles of any type of scientific research is that the results must be replicable.
Replication means repeating an experiment in the same way to produce the same results. By clearly detailing the specifics of how the variables were measured and manipulated, other researchers can better understand the results and repeat the study if needed.
Some variables are more difficult than others to define. For example, how would you operationally define a variable such as aggression ? For obvious ethical reasons, researchers cannot create a situation in which a person behaves aggressively toward others.
To measure this variable, the researcher must devise a measurement that assesses aggressive behavior without harming others. The researcher might utilize a simulated task to measure aggressiveness in this situation.
Hypothesis Checklist
- Does your hypothesis focus on something that you can actually test?
- Does your hypothesis include both an independent and dependent variable?
- Can you manipulate the variables?
- Can your hypothesis be tested without violating ethical standards?
The hypothesis you use will depend on what you are investigating and hoping to find. Some of the main types of hypotheses that you might use include:
- Simple hypothesis : This type of hypothesis suggests there is a relationship between one independent variable and one dependent variable.
- Complex hypothesis : This type suggests a relationship between three or more variables, such as two independent and dependent variables.
- Null hypothesis : This hypothesis suggests no relationship exists between two or more variables.
- Alternative hypothesis : This hypothesis states the opposite of the null hypothesis.
- Statistical hypothesis : This hypothesis uses statistical analysis to evaluate a representative population sample and then generalizes the findings to the larger group.
- Logical hypothesis : This hypothesis assumes a relationship between variables without collecting data or evidence.
A hypothesis often follows a basic format of "If {this happens} then {this will happen}." One way to structure your hypothesis is to describe what will happen to the dependent variable if you change the independent variable .
The basic format might be: "If {these changes are made to a certain independent variable}, then we will observe {a change in a specific dependent variable}."
A few examples of simple hypotheses:
- "Students who eat breakfast will perform better on a math exam than students who do not eat breakfast."
- "Students who experience test anxiety before an English exam will get lower scores than students who do not experience test anxiety."
- "Motorists who talk on the phone while driving will be more likely to make errors on a driving course than those who do not talk on the phone."
- "Children who receive a new reading intervention will have higher reading scores than students who do not receive the intervention."
Examples of a complex hypothesis include:
- "People with high-sugar diets and sedentary activity levels are more likely to develop depression."
- "Younger people who are regularly exposed to green, outdoor areas have better subjective well-being than older adults who have limited exposure to green spaces."
Examples of a null hypothesis include:
- "There is no difference in anxiety levels between people who take St. John's wort supplements and those who do not."
- "There is no difference in scores on a memory recall task between children and adults."
- "There is no difference in aggression levels between children who play first-person shooter games and those who do not."
Examples of an alternative hypothesis:
- "People who take St. John's wort supplements will have less anxiety than those who do not."
- "Adults will perform better on a memory task than children."
- "Children who play first-person shooter games will show higher levels of aggression than children who do not."
Collecting Data on Your Hypothesis
Once a researcher has formed a testable hypothesis, the next step is to select a research design and start collecting data. The research method depends largely on exactly what they are studying. There are two basic types of research methods: descriptive research and experimental research.
Descriptive Research Methods
Descriptive research such as case studies , naturalistic observations , and surveys are often used when conducting an experiment is difficult or impossible. These methods are best used to describe different aspects of a behavior or psychological phenomenon.
Once a researcher has collected data using descriptive methods, a correlational study can examine how the variables are related. This research method might be used to investigate a hypothesis that is difficult to test experimentally.
Experimental Research Methods
Experimental methods are used to demonstrate causal relationships between variables. In an experiment, the researcher systematically manipulates a variable of interest (known as the independent variable) and measures the effect on another variable (known as the dependent variable).
Unlike correlational studies, which can only be used to determine if there is a relationship between two variables, experimental methods can be used to determine the actual nature of the relationship—whether changes in one variable actually cause another to change.
The hypothesis is a critical part of any scientific exploration. It represents what researchers expect to find in a study or experiment. In situations where the hypothesis is unsupported by the research, the research still has value. Such research helps us better understand how different aspects of the natural world relate to one another. It also helps us develop new hypotheses that can then be tested in the future.
Thompson WH, Skau S. On the scope of scientific hypotheses . R Soc Open Sci . 2023;10(8):230607. doi:10.1098/rsos.230607
Taran S, Adhikari NKJ, Fan E. Falsifiability in medicine: what clinicians can learn from Karl Popper [published correction appears in Intensive Care Med. 2021 Jun 17;:]. Intensive Care Med . 2021;47(9):1054-1056. doi:10.1007/s00134-021-06432-z
Eyler AA. Research Methods for Public Health . 1st ed. Springer Publishing Company; 2020. doi:10.1891/9780826182067.0004
Nosek BA, Errington TM. What is replication ? PLoS Biol . 2020;18(3):e3000691. doi:10.1371/journal.pbio.3000691
Aggarwal R, Ranganathan P. Study designs: Part 2 - Descriptive studies . Perspect Clin Res . 2019;10(1):34-36. doi:10.4103/picr.PICR_154_18
Nevid J. Psychology: Concepts and Applications. Wadworth, 2013.
By Kendra Cherry, MSEd Kendra Cherry, MS, is a psychosocial rehabilitation specialist, psychology educator, and author of the "Everything Psychology Book."
Observational Studies
- Reference work entry
- pp 1008–1011
- Cite this reference work entry
- Slavenka Janković 2
252 Accesses
Nonexperimental studies
An observational epidemiologic study is a type of study in which the investigator observes and measures the effect of a risk factor , diagnostic test, or treatment on a particular outcome but does not intervene (in contrast with an experimental study, no attempt is made to affect the outcome).
Basic Characteristics
Appropriate use of observational studies permits the investigation of prevalence, incidence, associations, causes, and outcomes. Where there is little evidence on a subject, such studies are cost effective ways of producing and investigating hypotheses before larger and more expensive study designs are embarked upon. In addition, they are often the only realistic choice of research methodology, particularly where a randomized controlled trial would be impractical or unethical. Observational studies can be classified into descriptive studies, which are usually undertaken when little is known of the epidemiology of a disease, and...
This is a preview of subscription content, log in via an institution to check access.
Access this chapter
- Available as PDF
- Read on any device
- Instant download
- Own it forever
- Durable hardcover edition
- Dispatched in 3 to 5 business days
- Free shipping worldwide - see info
Tax calculation will be finalised at checkout
Purchases are for personal use only
Institutional subscriptions
Beaglehole R, Bonita R, Kjellström T (1993) Basic Epidemiology. WHO, Geneva
Google Scholar
Coggon D, Rose G, Barker DJP (1997) Epidemiology for Uninitiated, 4th ed. BMJ Publishing Group, London
Doll R, Peto R, Boreham J, Sutherland I (2004) Mortality in relation to smoking: 50 years' observations on male British doctors. BMJ 328:1519–1528
Article PubMed Google Scholar
Gordis L (2004) Epidemiology, 3rd ed. Elsevier-Saunders, Philadelphia
Goyder EC, Goodacre SW, Botha JL, Bodiwala GG (1997) How do individuals with diabetes use the accident and emergency department? J Accid Emerg Med 14:371–374
Janković S, Radosavljević V, Marinković J (1997) Risk factors for Graves' disease. Eur J Epidemiol 13:15–18
Sackett DL, Wennberg JE (1997) Choosing the best research design for each question. BMJ 315:1636
PubMed CAS Google Scholar
Download references
Author information
Authors and affiliations.
Institute of Epidemiology, School of Medicine, University of Belgrade, Belgrade, Serbia
Slavenka Janković
You can also search for this author in PubMed Google Scholar
Editor information
Editors and affiliations.
Network EUROlifestyle Research Association Public Health Saxony-Saxony Anhalt e.V. Medical Faculty, University of Technology, Fiedlerstr. 27, 01307, Dresden, Germany
Wilhelm Kirch ( Professor Dr. Dr. ) ( Professor Dr. Dr. )
Rights and permissions
Reprints and permissions
Copyright information
© 2008 Springer-Verlag
About this entry
Cite this entry.
Janković, S. (2008). Observational Studies . In: Kirch, W. (eds) Encyclopedia of Public Health. Springer, Dordrecht. https://doi.org/10.1007/978-1-4020-5614-7_2378
Download citation
DOI : https://doi.org/10.1007/978-1-4020-5614-7_2378
Publisher Name : Springer, Dordrecht
Print ISBN : 978-1-4020-5613-0
Online ISBN : 978-1-4020-5614-7
eBook Packages : Medicine Reference Module Medicine
Share this entry
Anyone you share the following link with will be able to read this content:
Sorry, a shareable link is not currently available for this article.
Provided by the Springer Nature SharedIt content-sharing initiative
- Publish with us
Policies and ethics
- Find a journal
- Track your research
If you're seeing this message, it means we're having trouble loading external resources on our website.
If you're behind a web filter, please make sure that the domains *.kastatic.org and *.kasandbox.org are unblocked.
To log in and use all the features of Khan Academy, please enable JavaScript in your browser.
Statistics and probability
Course: statistics and probability > unit 6.
- Types of statistical studies
- Worked example identifying experiment
- Worked example identifying observational study
- Worked example identifying sample study
Observational studies and experiments
- Appropriate statistical study example
- In an observational study, we measure or survey members of a sample without trying to affect them.
- In a controlled experiment, we assign people or things to groups and apply some treatment to one of the groups, while the other group does not receive the treatment.
Problem 1: Drinking tea before bedtime
- (Choice A) Observational study A Observational study
- (Choice B) Experiment B Experiment
Problem 2: Social media and happiness
- One group was directed to use social media sites as they usually do.
- One group was blocked from social media sites.
Want to join the conversation?
- Upvote Button navigates to signup page
- Downvote Button navigates to signup page
- Flag Button navigates to signup page

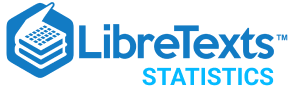
- school Campus Bookshelves
- menu_book Bookshelves
- perm_media Learning Objects
- login Login
- how_to_reg Request Instructor Account
- hub Instructor Commons
Margin Size
- Download Page (PDF)
- Download Full Book (PDF)
- Periodic Table
- Physics Constants
- Scientific Calculator
- Reference & Cite
- Tools expand_more
- Readability
selected template will load here
This action is not available.
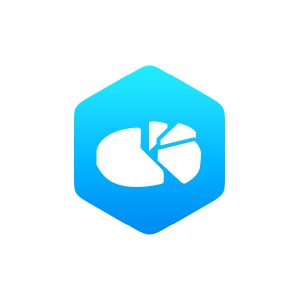
4.5: Observational study example - The Psychology of Debt
- Last updated
- Save as PDF
- Page ID 33243
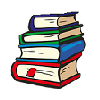
- Mark Greenwood
- Montana State University
\( \newcommand{\vecs}[1]{\overset { \scriptstyle \rightharpoonup} {\mathbf{#1}} } \)
\( \newcommand{\vecd}[1]{\overset{-\!-\!\rightharpoonup}{\vphantom{a}\smash {#1}}} \)
\( \newcommand{\id}{\mathrm{id}}\) \( \newcommand{\Span}{\mathrm{span}}\)
( \newcommand{\kernel}{\mathrm{null}\,}\) \( \newcommand{\range}{\mathrm{range}\,}\)
\( \newcommand{\RealPart}{\mathrm{Re}}\) \( \newcommand{\ImaginaryPart}{\mathrm{Im}}\)
\( \newcommand{\Argument}{\mathrm{Arg}}\) \( \newcommand{\norm}[1]{\| #1 \|}\)
\( \newcommand{\inner}[2]{\langle #1, #2 \rangle}\)
\( \newcommand{\Span}{\mathrm{span}}\)
\( \newcommand{\id}{\mathrm{id}}\)
\( \newcommand{\kernel}{\mathrm{null}\,}\)
\( \newcommand{\range}{\mathrm{range}\,}\)
\( \newcommand{\RealPart}{\mathrm{Re}}\)
\( \newcommand{\ImaginaryPart}{\mathrm{Im}}\)
\( \newcommand{\Argument}{\mathrm{Arg}}\)
\( \newcommand{\norm}[1]{\| #1 \|}\)
\( \newcommand{\Span}{\mathrm{span}}\) \( \newcommand{\AA}{\unicode[.8,0]{x212B}}\)
\( \newcommand{\vectorA}[1]{\vec{#1}} % arrow\)
\( \newcommand{\vectorAt}[1]{\vec{\text{#1}}} % arrow\)
\( \newcommand{\vectorB}[1]{\overset { \scriptstyle \rightharpoonup} {\mathbf{#1}} } \)
\( \newcommand{\vectorC}[1]{\textbf{#1}} \)
\( \newcommand{\vectorD}[1]{\overrightarrow{#1}} \)
\( \newcommand{\vectorDt}[1]{\overrightarrow{\text{#1}}} \)
\( \newcommand{\vectE}[1]{\overset{-\!-\!\rightharpoonup}{\vphantom{a}\smash{\mathbf {#1}}}} \)
In this section, the analysis of a survey of \(N = 464\) randomly sampled adults will be analyzed from a survey conducted by Lea, Webley, and Walker ( 1995 ) and available in the debt data set from the faraway package ( Faraway 2016 ) . The subjects responded to a variety of questions including whether they buy cigarettes ( cigbuy : 0 if no, 1 if yes), their housing situation ( house : 1 = rent, 2 = mortgage, and 3 = owned outright), their income group ( incomegp : 1 = lowest, 5 = highest), and their score on a continuous scale of attitudes about debt ( prodebt : 1 = least favorable, 6 = most favorable). The variable prodebt was derived as the average of a series of questions about debt with each question measured on an ordinal 1 to 6 scale, with higher values corresponding to more positive responses about \(\underline{\text{going into debt}}\) of various kinds. The ordered scale on surveys that try to elicit your opinions on topics with scales from 1 to 5, 1 to 6, 1 to 7 or even, sometimes, 1 to 10 is called a Likert scale ( Likert 1932 ) . It is not a quantitative scale and really should be handled more carefully than taking an average of a set responses as was done here. That said, it is extremely common practice in social science research to treat ordinal responses as if they are quantitative and take the average of many of them to create a more continuous response variable like the one we are using here. If you continue your statistics explorations, you will see some better techniques for analyzing ordinal responses. That said, the scale of the response is relatively easy to understand as an amount of willingness to go into debt on a scale from 1 to 6 with higher values corresponding to more willingness to be in debt.
These data are typical of survey data where respondents were not required to answer all questions and there are some missing responses. We could clean out any individuals that failed to respond to all questions (called “complete cases”) using the drop_na function, which will return responses only for subjects that responded to every question in the data set, debt . The change in sample size is available by running the dim function on the two data sets – there were \(464\) observations (rows) initially along with \(13\) variables (columns) and once observations with any missing values were dropped there are \(N = 304\) for us to analyze. Losing 35% of the observations is a pretty noticeable loss.
Using drop_na() with a list a variable names, we can focus on the three variables we are using in this model and whether the responses are missing on them, only cleaning out rows that are missing on incomegp , cigbuy , and/or prodebt 92 . The missingness is less dramatic, retaining \(N = 388\) observations in debtRc for our analysis using these three variables.
The second approach seems better as it drops fewer observations so we will use that below. But suppose that people did not want to provide their income levels if they were in the lowest or highest income groups and that is why they are missing. Then we would be missing responses systematically and conclusions could be biased because of ignoring particular types of subjects. We don’t have particular statistical tools to easily handle this problem but every researcher should worry about non-response when selected subjects do not respond at all or fail to answer some questions. When the missing values are systematic in some fashion and not just missing randomly (missing randomly might be thought of as caused by “demonic intrusion” ( Hurlbert 1984 ) that can’t be easily explained or related to the types of responses), then we worry about non-response bias that is systematically biasing our results because of the missing responses. This also ties back into our discussion of who was sampled. We need to think carefully about who was part of the sample but refused to participate and how that might impact our inferences. And whether we can even address the research question of interest based on what was measured given those that refused/failed to respond. For example, suppose we are studying river flows and are interested in the height of a river. Missingness in these responses could arise because a battery fails or the data logger “crashes” (not related to the responses and so not definitely problematic) or because of something about the measurements to be taken that causes the missingness (suppose the gage can only can measure between one and three feet deep and the river is running at four feet deep during a flood or below 1 foot during a drought). The first machine failures are very different from the height-based missing responses; the height-based missingness clearly leads to bias in estimating mean river height because of what can not be observed. In Chapter 5, we introduce the tableplot as another tool to visualize data that can also show missing data patterns to help you think about these sorts of issues further 93 . If you delete observations and the missing data are not random/non-systematic, your scope of inference is restricted to just those subjects that provided responses and were analyzed. If the missingness is random and not related to aspects of the measurements taken, then some missingness can be tolerated and still retain some comfort that inferences can be extended to the population a random sample of subjects was taken from.
Ignoring this potential for bias in the results for the moment, we are first interested in whether buying cigarettes/not and income groups interact in their explanation of the respondent’s mean opinions on being in debt. The interaction plot (Figure 4.15) may suggest an interaction between cigbuy and incomegp where the lines cross, switching which of the cigbuy levels is higher (income levels 2, 3, and 5) or even almost not different (income levels 1 and 4). But it is not as clear as the previous examples, especially with how large the SEs are relative the variation in the means. The interaction \(F\) -test helps us objectively assess evidence against the null hypothesis of no interaction. Based on the plot, there do not appear to be differences based on cigarette purchasing but there might be some differences between the income groups if we drop the interaction from the model. If we drop the interaction, then this suggests that we might be in Scenario 2 or 3 where a single main effect of interest is present.
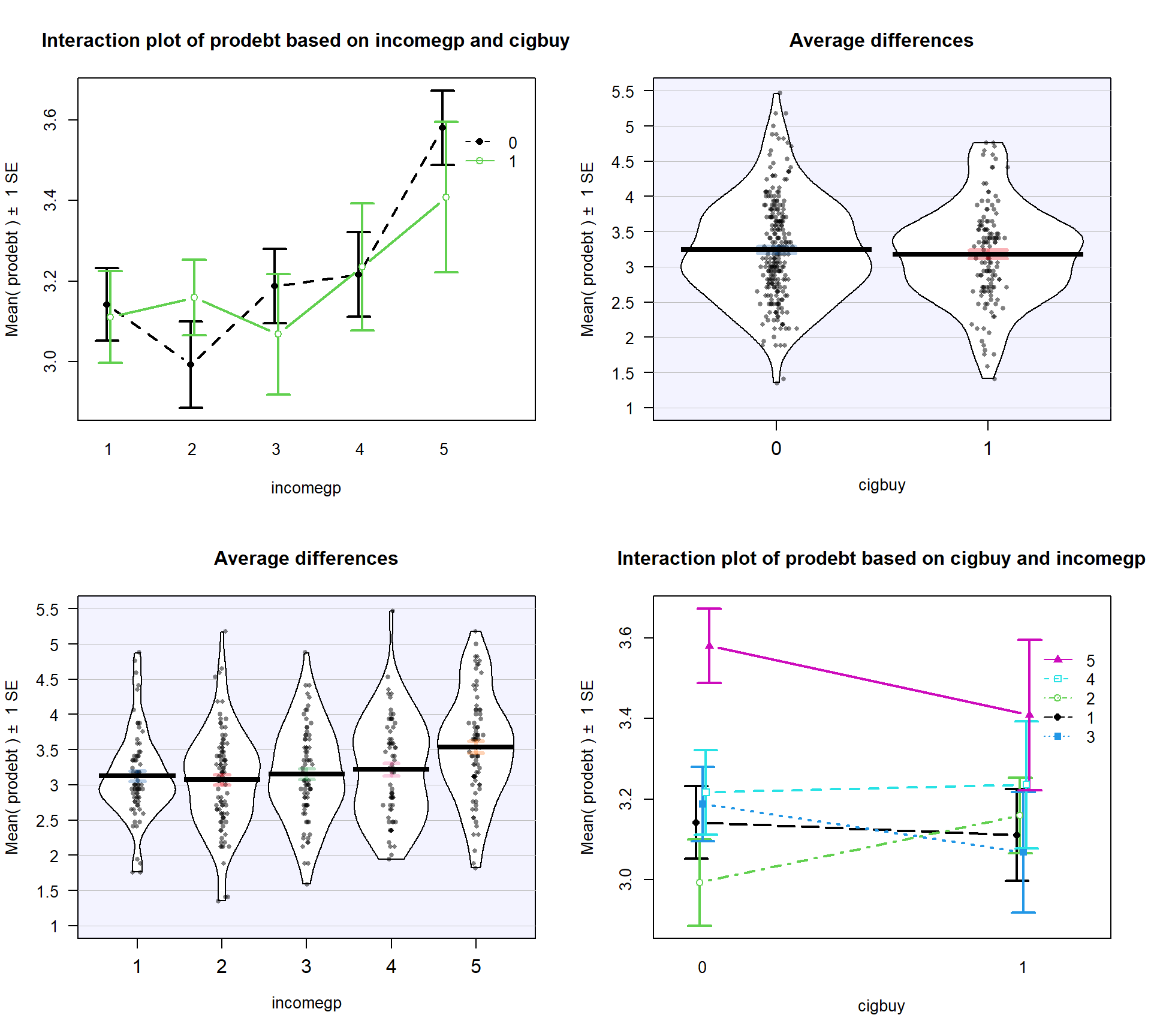
As in other situations, and especially with observational studies where a single large sample is collected and then the levels of the factor variables are observed, it is important to check for balance – whether all the combinations of the two predictor variables are similarly represented. Even more critically, we need to check whether all the combinations of levels of factors are measured. If a combination is not measured, then we lose the ability to estimate the mean for that combination and the ability to test for an interaction. A solution to that problem would be to collapse the categories of one of the variables, changing the definitions of the levels but if you fail to obtain information for all combinations, you can’t work with the interaction model. In this situation, we barely have enough information to proceed (the smallest \(n_{jk}\) is 13 for income group 4 that buys cigarettes). We have a very unbalanced design with counts between 13 and 60 in the different combinations, so lose some resistance to violation of assumptions but can proceed to explore the model with a critical eye on how the diagnostic plots look.
The test for the interaction is always how we start our modeling in Two-Way ANOVA situations. The ANOVA table suggests that there is little evidence against the null hypothesis of no interaction between the income level and buying cigarettes on the opinions of the respondents towards debt ( \(F(4,378) = 0.686\) , p-value = 0.6022), so we would conclude that there is likely not an interaction present here and we can drop the interaction from the model. This suggests that the initial assessment that the interaction wasn’t too prominent was correct. We should move to the additive model here but first need to check the assumptions to make sure we can trust this initial test.

The diagnostic plots (Figure 4.16) seem to be pretty well-behaved with no apparent violations of the normality assumption and no clear evidence of a violation of the constant variance assumption. There is no indication of a problem with the independence assumption because there is no indication of structure to the measurements of the survey respondents that might create dependencies. In observational studies, violations of the independence assumption might come from repeated measures of the same person over time or multiple measurements within the same family/household or samples that are clustered geographically, none of which are part of the survey information we have. The random sampling from a population should allow inferences to a larger population except for that issue of removing partially missing responses so we can’t safely generalize results beyond the complete observations we are using without worry that the missing subjects are systematically different from those we are able to analyze. We also don’t have much information on the exact population sampled, so will just leave this vague here but know that there would be a population these conclusions apply since it was random sample (at least those that would answer the questions). All of this suggests proceeding to fitting and exploring the additive model is reasonable here. No causal inferences are possible because this is an observational study.
- After ruling out the interaction of income and cigarette status on opinions about debt, we can focus on the additive model.
\(\Leftrightarrow\) All \(\tau_j\text{'s} = 0\) in additive model.
\(\Leftrightarrow\) Not all \(\tau_j\text{'s} = 0\) in additive model.
\(\Leftrightarrow\) All \(\gamma_k\text{'s} = 0\) in additive model.
\(\Leftrightarrow\) Not all \(\gamma_k\text{'s} = 0\) in additive model.
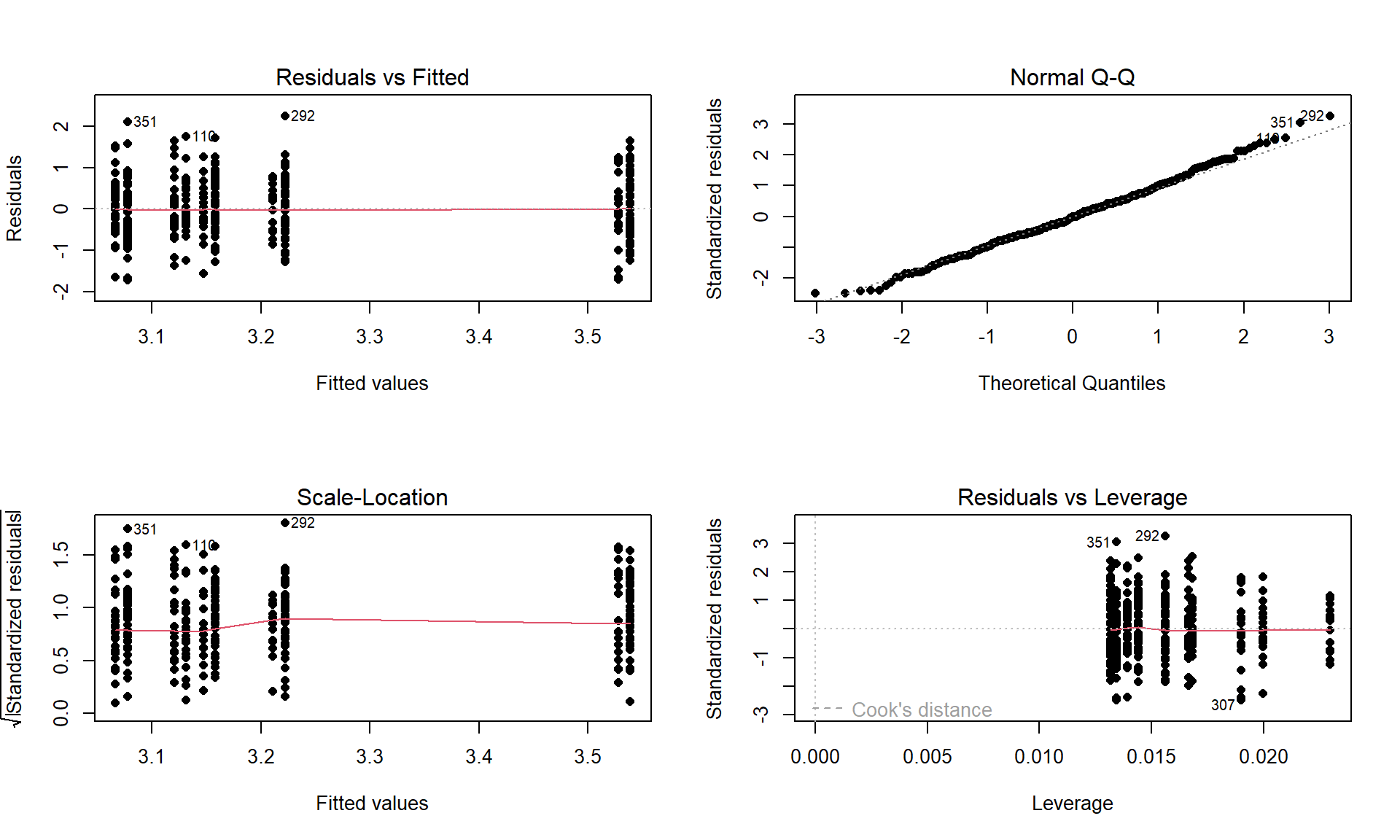
- In the Residuals vs Fitted and the Scale-Location plots in Figure 4.17, the differences in variability among groups is minor and nothing suggests a violation. If you change models, you should always revisit the diagnostic plots to make sure you didn’t create problems that were not present in more complicated models.
- We can also explore the partial residuals here as provided in Figure 4.18. The variability in the partial residuals appears to be similar across the different levels of each predictor, controlled for the other variable, and so does suggest any issues that were missed by just looking at the overall residuals versus fitted values in our regular diagnostic plots. Note how hard it is to see differences in the mean for levels of cigbuy in this plot relative to the variability in the partial residuals but that the differences in the means in incomegp are at least somewhat obvious.
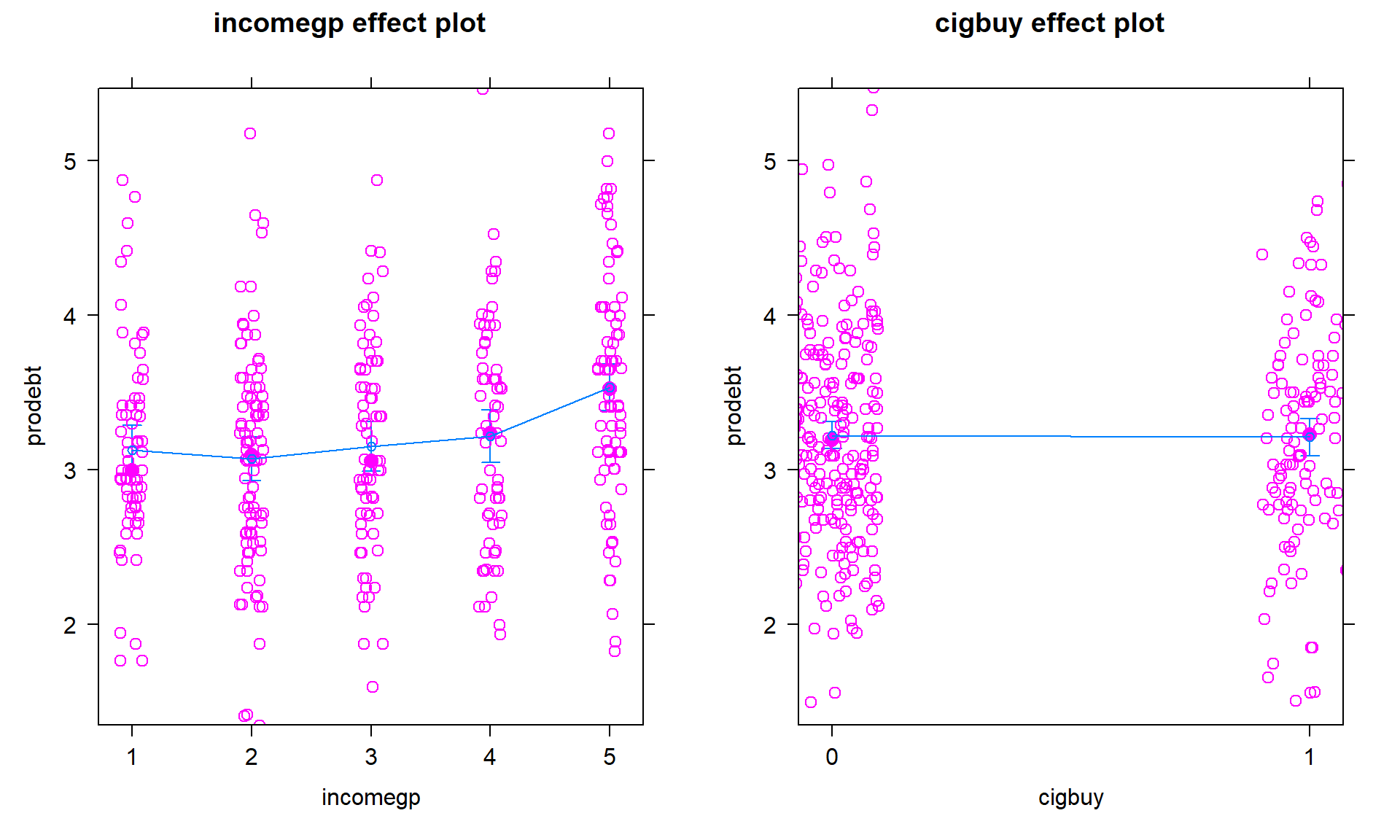
- The QQ-Plot in Figure 4.17 does not suggest a problem with this assumption.
- The test statistics are \(F(4,382) = 5.54\) and \(F(1,382) = 0.0201\) with p-values of 0.00024 and 0.887.
- There was initially little to no evidence against the null hypothesis of no interaction between income group and cigarette buying on pro-debt feelings ( \(F(4,378) = 0.686\) , p-value = \(0.6022\) ) so we would conclude that there is likely not an interaction in the population and the interaction was dropped from the model. There is strong evidence against the null hypothesis of no difference in the mean pro-debt feelings in the population across the income groups, after adjusting for cigarette buying ( \(F(4,382) = 5.54\) , p-value = \(0.00024\) ), so we would conclude that there is some difference in them. There is little evidence against the null hypothesis of no difference in the mean pro-debt feelings in the population based on cigarette buying/not, after adjusting for income group ( \(F(1,382) = 0.0201\) , p-value = \(0.887\) ), so we would conclude that there is probably not a difference across cigarette buying/not and could consider dropping this term from the model.
So we learned that the additive model was more appropriate for these responses and that the results resemble Scenario 2 or 3 with only one main effect being important. In the additive model, the coefficients can be interpreted as shifts from the baseline after controlling for the other variable in the model.
- Figure 4.19 shows the increasing average comfort with being in debt as the income groups go up except between groups 1 and 2 where 1 is a little higher than two. Being a cigarette buyer was related to a lower comfort level with debt but is really no different from those that did not report buying cigarettes. It would be possible to consider follow-up tests akin to the Tukey’s HSD comparisons for the levels of incomegp here but that is a bit beyond the scope of this course – focus on the estimated mean for the 5 th income group being over 3.5 and none of the others over 3.2. That seems like an interesting although modest difference in mean responses across income groups after controlling for cigarette purchasing or not.
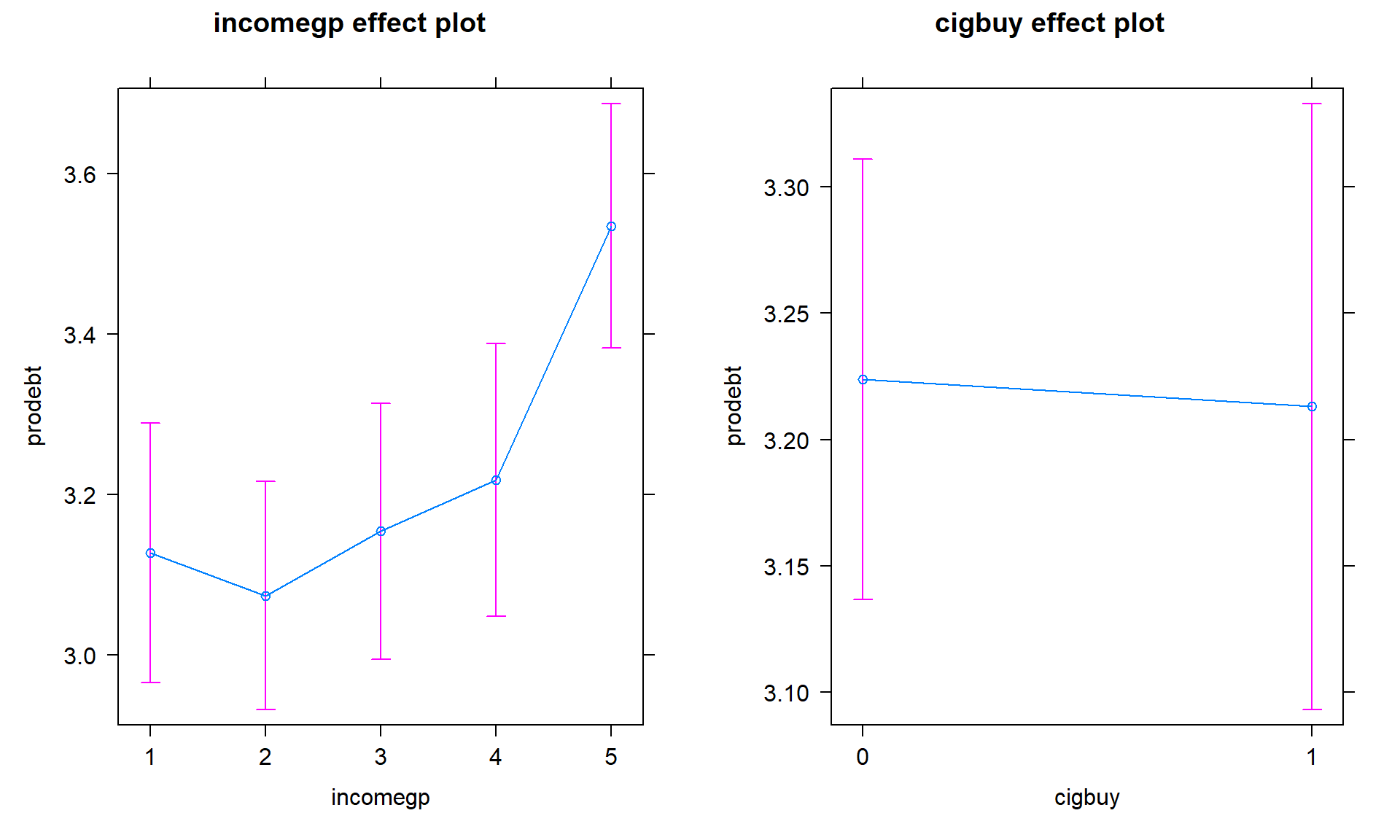
- Because the income group and cigarette purchasing were not (and really could not) be randomly assigned, causal inference is not possible here. The data set came from a random sample but from an unspecified population and then there were missing observations. At best we can make inferences to those in that population that would answer these questions and it would be nice to know more about the population to really understand who this actually applies to. There would certainly be concerns about non-response bias in doing inference to the entire population that these data were sampled from.
The estimated coefficients can also be interesting to interpret for the additive model. Here are the model summary coefficients:
In the model, the baseline group is for non-cigarette buyers ( cigbuy = 0 ) and income group 1 with \(\widehat{\alpha} = 3.131\) points. Regardless of the cigbuy level, the difference between income groups 2 and 1 is estimated to be \(\widehat{\tau}_2 = -0.054\) , an decrease in the mean score of 0.054 points. The difference between income groups 3 and 1 is \(\widehat{\tau}_3 = 0.027\) points, regardless of cigarette smoking status. The estimated difference between cigarette buyers and non-buyers was estimated as \(\widehat{\gamma}_2 = -0.011\) points for any income group, remember that this variable had a large p-value in this model. The additive model-based estimates for all six combinations can be found in Table 4.3.
One final plot of the fitted values from this additive model in Figure 4.20 hopefully crystallizes the implications of an additive model and reinforces that this model creates and assumes that the differences across levels of one variable are the same regardless of the level of the other variable and that this creates parallel lines. The difference between cigbuy levels across all income groups is a drop in -0.011 points. The income groups have the same differences regardless of cigarette buying or not, with income group 5 much higher than the other four groups. The minor differences in cigarette purchasing and large p-value for it controlled for income group suggest that we could also refine the model further and drop the cigbuy additive term and just focus on the income groups as a predictor – and this takes us right back to a One-Way ANOVA model so is not repeated here.

In general, we proceed through the following steps in any 2-WAY ANOVA situation:
- Make a pirate-plot and an interaction plot.
- Fit the interaction model; examine the test for the interaction.
- If there is a problem with normality or equal variance, consider a “transformation” of the response as discussed in Chapter 7. This can help make the responses have similar variances or responses (and the model residuals) to be more normal, but sometimes not both.
- If the interaction test has a small p-value, that is your main result. Focus on the term-plot and the interaction plot from (1) to fully understand the results, adding Tukey’s HSD results to intplot to see which means of the combinations of levels are detected as being different. Discuss the sizes of differences and the pattern of the estimated interaction.
- Focus on the results for each explanatory variable, using Type II tests especially if the design is not balanced. Possibly consider further model refinement to only retain one of the two variables (the one with the smaller p-value) if a p-value is large. Follow One-Way ANOVA recommendations from this point on.
- Report the initial interaction test results and the results for the test for each variable from the model that is re-fit without the interaction.
- Model coefficients in the additive model are interesting as they are shifts from baseline for each level of each variable, controlling for the other variable – interpret those differences if the number of levels is not too great.
Whether you end up favoring an additive or interaction model or do further model refinement, all steps of the hypothesis testing protocol should be engaged and a story based on the final results should be compiled, supported by the graphical displays such as the term-plots and interaction plots.

An official website of the United States government
The .gov means it’s official. Federal government websites often end in .gov or .mil. Before sharing sensitive information, make sure you’re on a federal government site.
The site is secure. The https:// ensures that you are connecting to the official website and that any information you provide is encrypted and transmitted securely.
- Publications
- Account settings
Preview improvements coming to the PMC website in October 2024. Learn More or Try it out now .
- Advanced Search
- Journal List
- v.90(8); 2004 Aug

Interpretation of observational studies
1 Department of Clinical Epidemiology, Aarhus University Hospital, Aarhus, Denmark
S P Johnsen
M w gillman.
2 Department of Ambulatory Care and Prevention, Harvard Medical School and Harvard Pilgrim Health Care, Boston, Massachusetts, USA
H T Sørensen
The aim of this paper is to review the most important methodological strengths and limitations of observational studies of humans, as opposed to experimental studies. The names “observational” and “experimental” go a long way in describing the differences. In an experimental study—that is, a randomised controlled trial (RCT)—the investigator experiments with the effect of the exposure by assigning exposure to a random sample of the study subjects. In an observational study, on the other hand, the investigator can only observe the effect of the exposure on the study subjects; he or she plays no role in assigning exposure to the study subjects. This makes observational studies much more vulnerable to methodological problems, so it is only reasonable that RCTs are considered the best way of proving causality. 1
One might ask why all studies are not experimental. First, not all research questions are suitable for an experimental design. Studies of diagnostics tests and treatment are highly suited, but studies of drug effects in pregnant women, or of the prognostic impact of diseases, are among the research questions that cannot be studied in an experimental design. Second, RCTs are often very expensive, in terms of both time and money, and an RCT may be conducted only after observational studies have failed to provide a clear answer to the research question. Observational studies give an idea about the incidence, prevalence, and prognosis of the disease that is studied, and this information is necessary for proper planning of the RCT. Often, the RCT will confirm what has been found in the preceding observational studies, 2, 3 but occasionally the findings differ, or are even in the opposite direction, as in recent studies of the effect of hormone replacement therapy on cardiovascular risk. 4– 6
Consequently, most research questions will be addressed in one or more observational studies, so it is highly relevant for all physicians to be able to critically interpret findings from such studies.
ASSOCIATIONS
In clinical epidemiology, the two basic components of any study are exposure and outcome. The exposure can be a risk factor, a prognostic factor, a diagnostic test, or a treatment, and the outcome is usually death or disease. In an observational study, the frequency of an outcome—or an exposure, depending on the study design—is measured, estimated, or visualised. Risks, rates, prevalences, and odds are common measures of the frequency of an outcome, and comparing them between groups will yield relative frequency measures—that is, relative risks, rate ratios, prevalence ratios, and odds ratios. These describe the association between exposure and outcome and provide the basis for the study’s conclusions.
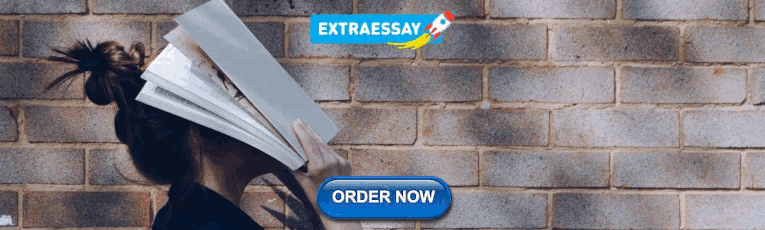
Surrogate measures
If the outcome of interest is disease, the actual study outcome is sometimes a surrogate measure for the disease. Surrogate measures are often used when the disease is so rare or so far in the future that it would take an unreasonably long follow up period to obtain a sufficient number of outcomes.
- Example —In the Los Angeles atherosclerosis study, Nordstrom and colleagues examined the three year progression of intima–media thickness of the carotids in a group of 40 to 60 year olds. Exposure groups were defined by the degree of physical activity. The authors found that intima–media thickness, a surrogate measure for cardiovascular morbidity, was inversely related to the degree of physical activity. 7
Hypertension and hyperlipidaemia are among the most frequently used surrogate measures for cardiovascular disease, but even though the association between the surrogate measure and the true outcome may be biologically plausible, using the surrogate measure may produce misleading results if the association with the true outcome is not based on empirical evidence. 8, 9
OBSERVATIONAL STUDY DESIGNS
Three main study designs are used in observational studies: cohort (follow up), case–control, and cross sectional designs (fig 1 1 ). 10

The three main study designs used in observational studies: cohort (follow up), case–control, and cross sectional.
Cohort design
In a cohort study, patients with different levels of exposure are followed forward in time to determine the incidence of the outcome in question in each exposure group. With this design, the investigator can study several outcomes within the same study, and the most common frequency measures are relative risks, incidence rate ratios, and excess risks. If the outcome of interest is rare, a very large study population must be followed to observe a number of outcomes that is sufficient to demonstrate a precise association between the exposure and the outcome—that is, that will rule out chance as an explanation for the observed findings. 10
Case–control design
In a case–control design, the first step is to identify those with the outcome of interest—the cases. That makes it a good design for studying rare outcomes, which would require a huge sample size in a cohort design, and this means that case–control studies are generally cheaper, too. 10 Having identified the cases, the investigator selects the controls from the source population. There are a number of methods of doing this, but, regardless of the method, the level of exposure is compared between cases and controls. The relative frequency measure is the odds ratio, which is an estimate of the relative risk. The estimate is better if the disease is rare, 8 but still the odds ratio will be biased away from the null—that is, it will be further from 1.0 than the relative risk, in studies of a dichotomous outcome. Using a nested case–control design and the incidence density sampling technique, however, allows the investigator to assume that the odds ratio is an unbiased estimate of the incidence rate ratio. A nested case–control study is actually a case–control study set within a cohort study, and the point of incidence density sampling is that controls are selected so that they have the same time at risk as cases.
Cross sectional design
Studies with a cross sectional design are also called prevalence studies. 11 With this design, exposure and outcome are measured simultaneously. Prevalence rates can be compared between groups, but the terms “exposure” and “outcome” are treacherous because the sequencing of the two is impossible to assess. 10 Therefore, a cross sectional study is generally used to provide the basis for a subsequent cohort study, case–control study, or RCT.
REASONS FOR ASSOCIATIONS
There are four principal reasons for associations in an epidemiologic study: bias, confounding, chance, and cause. An essential aim of the design and analysis phases is to prevent, reduce, and assess bias, confounding, and chance, so that a causal unbiased association between exposure and outcome is estimated. 10
Bias means that a measure of association between exposure and outcome is systematically wrong. Evaluation of bias is a two step process: first, the investigator must determine whether bias is present; and then, second, consider its magnitude and direction. Usually, epidemiologists talk about bias away from the null and bias towards the null, meaning that the reported measure of association is systematically overestimated or underestimated, respectively. The two main types of bias are selection bias and information bias.
Selection bias
Selection bias relates to the design phase of an observational study, and it is more common in case–control studies than in cohort studies. In a case–control study, the investigator selects cases and controls that represent a source population, and the only intended difference between the two groups is the outcome. Selection bias occurs if the selection process introduces another, unintended systematic difference between the groups, and this systematic difference is associated with the exposure. In other words, the apparent association between exposure and outcome in a biased case–control study is in fact a combination of the association between exposure and outcome and the association between being selected as a case or control and the exposure. 12 Selection bias can be either towards the null or away from the null.
- Example —Using a case–control design, von Eyben examined smoking as a risk factor for myocardial infarction. Cases were patients with a myocardial infarction between 1983 and 1997, and controls were selected among patients admitted with inguinal hernia and acute appendicitis in 1990–1996. In 1997, both groups were asked in a questionnaire about their smoking habits, among other things, and the authors reported that smoking increased the risk of myocardial infarction. However, 27 of 77 patients with acute myocardial infarction had died at the time of the questionnaire, and, as the authors pointed out, that might have introduced selection bias; the possibility of being available at the time of the questionnaire is an unintended systematic difference between cases and controls, and it is also related to smoking. In other words, as above, the apparent association between smoking and myocardial infarction is a combination of the association between smoking and myocardial infarction and the association between smoking and being available at the time of the questionnaire. The resulting bias is presumably towards the null because it is likely that those who smoked less had a higher possibility of being available at the time of the questionnaire than those who smoked more. 13
In a cohort study, selection bias occurs if the investigator’s selection of exposed and reference groups introduces a systematic difference, other than the exposure, between the groups, and this systematic difference is associated with the outcome.
Information bias
In a cohort design, an error in measuring exposure or outcome may cause information bias. Non-differential misclassification is seen when the errors in classification of exposure or outcome are random. An example of non-differential misclassification is the misclassification caused by coding errors due to accidental mistyping. In contrast, differential misclassification in a cohort study is when the degree or direction of misclassification depends on exposure status, or other variables. 11 In RCTs, this is avoided by standardised outcome assessment and blinding to exposure status. Non-differential misclassification will usually lead to bias towards the null, whereas differential misclassification can lead to bias in either direction. 12
In a case–control study, cases and controls may have different degrees or directions of misclassification of exposure—that is, differential misclassification. This is of particular concern when exposure status is self reported, in which case a bias is called recall bias .
- Example —Tzourio and colleagues used a case–control study to examine whether migraine is a risk factor for ischaemic stroke in young women. Cases were women under 45 years of age with ischaemic stroke, and controls were randomly selected women with orthopaedic or rheumatological illnesses. Cases and controls were interviewed about headache, and other factors, and the authors found an odds ratio of 6.2 for ischaemic stroke among women with migraine with aura. Perhaps this was due to recall bias; cases may have been more aware of signs and symptoms that might explain their stroke. 14
CONFOUNDING
Confounding is about the characteristics of the study subjects; patients with certain characteristics tend to have certain exposures. The aim of an observational study is to examine the effect of the exposure, but sometimes the apparent effect of the exposure is actually the effect of another characteristic which is associated with the exposure and with the outcome. This other characteristic is a confounder, provided that it is not an intermediate step between the exposure and the outcome. 8 Therefore, a high cholesterol value should not be treated as a confounder in a study of the risk of coronary heart disease (outcome) in patients with severe obesity (exposed group) and patients with normal weight (reference group); although a high cholesterol value is associated with both obesity and coronary heart disease, it is an intermediate step because it may be caused by obesity.
There are two principal ways to reduce confounding in observational studies: (1) prevention in the design phase by restriction or matching; and (2) adjustment in the statistical analyses by either stratification or multivariable techniques. These methods require that the confounding variables are known and measured. In an RCT, on the other hand, the randomisation process allows the investigator to assume that not only known, but also unknown, potential confounders are distributed evenly among the exposed and the unexposed. Therefore, they are not associated with the exposure, hence they cannot be confounders.
Restriction
Confounding can be reduced by restricting the study population to those with a specific value of the confounding variable. 12 This method, also known as specification, 10 makes examinations of the association between the confounder and the outcome invalid, and the findings cannot be generalised to those who were left out by the restriction.
- Example —Ayanian and colleagues examined the effect of specialty of ambulatory care physicians on mortality after myocardial infarction in elderly patients. They found that patients who saw a cardiologist had lower mortality than patients who saw an internist or a family practitioner. They excluded patients who died within three months, patients with metastatic cancer or a do-not-resuscitate order, patients who enrolled in a health maintenance organisation, patients residing in nursing homes, patients who lacked Medicare part B coverage, and patients with no ambulatory visits. These restrictions could serve to reduce confounding from the degree of morbidity, as the moribund and the, presumably, perfectly well patients, with no need for ambulatory visits, were excluded. 15
Matching constrains subjects in different exposure groups to have the same value of potential confounders, often age and sex. 10 However, with increasing numbers of matching variables, the identification of matched subjects becomes progressively demanding, and matching does not reduce confounding by factors other than the matching variables. Matching is most commonly used in case–control studies, but it can be used in cohort studies as well.
- Example —In a study of predictors of peripheral arterial disease, Ridker and colleagues nested a case–control design in the Physicians’ Health Study cohort, consisting exclusively of men. They identified 140 cohort members who developed peripheral arterial disease (cases). Controls were selected with the incidence density sampling technique and matched on age and smoking status to reduce confounding by these variables. The matching variables, the 11 candidate predictors, and remaining confounders (hypertension, body mass index, family history of premature atherosclerosis, diabetes, and exercise frequency) were then included in a multivariate model. The authors concluded that the total cholesterol/high density lipoprotein cholesterol (TC/HDL-C) ratio and C reactive protein (CRP) were the strongest lipid and non-lipid predictors of peripheral arterial disease. 16
Stratified analysis
Stratification means that the study population is divided into a number of strata (subsets), so that subjects within a stratum share a characteristic, and each stratum is analysed separately. If the study population is to be divided into more than a few strata, it has to be large to begin with to yield conclusive results. Stratified analyses are the best way to evaluate effect modification (see below), but they are also a way of examining, or adjusting for, confounding. Confounding can be adjusted for if the strata are recombined with the Mantel-Haenszel method or a similar method. 8
- Example —Ridker and colleagues studied whether CRP would improve prediction of risk of myocardial infarction. They used data from the Physicians’ Health Study and included 245 cases with myocardial infarction and 372 controls. Baseline exposure data were obtained from blood samples drawn at the time of inclusion in the Physicians’ Health Study. Patients were stratified according to baseline cholesterol value, and separate logistic regression analyses were presented for each stratum. Baseline concentrations of CRP were associated with increased risk of myocardial infarction in all strata. 17
Multivariate modelling
Multivariate analyses are methods that simultaneously adjust (control) for several variables to estimate the independent effect of each one. Usually, one of the variables in the model describes whether a patient is exposed, another describes whether the outcome is observed, and the remaining variables describe the values of potential confounders. A multivariate model will then estimate the effect of the exposure on the outcome, given that exposed patients and reference patients are similar with respect to the confounders in the model. The most commonly used multivariate methods are the Cox proportional hazards model, the logistic regression model, and the linear regression model.
Although multivariate models have proven to be useful and have gained an enormous popularity, they may also be treacherous since there is no limit to the amount of data that can be included in the analyses and condensed into very few numbers. As a consequence, a multivariate model can be like a black box, and if nothing but the adjusted estimates is presented, readers have no chance of understanding why the estimates turned out as they did. It is therefore essential that the construction of multivariate models is carefully documented and presented, and that the models are biologically plausible.
- Example —Based on data from the Nurses’ Health Study, Solomon and colleagues found that those with rheumatoid arthritis had a higher risk of myocardial infarction and stroke than those without rheumatoid arthritis. This was based on two pooled logistic regression models. The first included only rheumatoid arthritis (exposure), myocardial infarction or stroke (outcomes), and age (potential confounder). The second model included more potential confounders: hypertension, diabetes, high cholesterol, parental history of myocardial infarction before age 60 years, body mass index, cigarette use, physical activity, alcohol use, aspirin use, menopausal status, hormone replacement therapy use, oral glucocorticoid use, non-steroidal anti-inflammatory drug use, folate intake, omega-3 fatty acid intake, and vitamin E supplement intake. The authors showed that many of the potential confounders were associated with rheumatoid arthritis, and they stated that potential confounders were known or suspected risk factors for cardiovascular disease. Nonetheless, the two models yielded similar results, suggesting that there was no confounding by the additional potential confounders. This was not discussed. 18
CONFOUNDING BY INDICATION
Unmeasured confounding cannot be adjusted for, and confounding by indication is a type of confounding that is usually unmeasured. It occurs in many observational studies, and it means that the patient characteristics that have made a doctor prescribe a particular drug to (or choose to operate on, or perform a diagnostic test on, etc) a particular patient is a confounder. 19 There may be a number of measurable patient characteristics that could be part of the indication (age, sex, diseases, cholesterol value, blood pressure, etc). Nevertheless, even among 74 year old women with diabetes, recent myocardial infarction, a total cholesterol of 6.2 mmol/l, and a blood pressure of 160/90 mm Hg, doctors have a reason for prescribing a particular drug to a particular patient and for not prescribing it to another. Although confounding by indication can be prevented completely only in an RCT with proper randomisation, 19 methods for handling it in observational studies are also available. 20
- Example —In a case–control design, Lewis and colleagues found a reduced risk of myocardial infarction among users of third generation oral contraceptives versus users of second generation oral contraceptives. Users of second generation oral contraceptives, however, were almost three times more likely to be hospitalised than users of third generation oral contraceptives. Is it possible that the generally healthy patients are prescribed third generation oral contraceptives, whereas the less healthy are prescribed second generation oral contraceptives? 21
EFFECT MODIFICATION
Effect modification means that the effect of the exposure depends on the level of another variable, and effect modification is often confused with confounding, although it is something altogether different. Confounding is to be avoided, whereas effect modification shows a phenomenon that may have biological, clinical or public health relevance.
- Example —In a recent study, Olshan and colleagues found that heavy smoking modified the effect of expression of glutathione S-transferase theta (GSTT1) as a risk factor for atherosclerosis. Consequently, two risk estimates for atherosclerosis in patients with GSTT1 expression were presented: one for “ever smokers” and one for “heavy smokers”. Presenting only one risk estimate would have concealed this information. 22
The precision of an estimate of the association between exposure and outcome is usually expressed as a confidence interval (usually a 95% confidence interval). The confidence interval can be interpreted as the interval which, with a 95% certainty, holds the true value of the association if the study is unbiased. Consequently, the wider the confidence interval, the less certain we are that we have precisely estimated the strength of the association. The width of the confidence interval is determined by the number of subjects with the outcome of interest, which in turn is determined by the sample size.
A p value is closely related to a confidence interval, but is interpreted as the probability that the findings would be as observed, or even more extreme, if the null hypothesis, which usually states that the exposure has no effect on the outcome, were true. 11 A p value above 0.05 translates to a 95% confidence interval that includes the null value of one, and both mean that the null hypothesis of no effect is retained. However, p values and confidence intervals are often misused to dichotomise findings into “association” (p value below < 0.05 or confidence interval excluding 1) and “no association”, but this is an overly simplistic way of describing a biologic mechanism. Common sense and clinical experience are necessary to separate a meaningful, although not statistically significant, association from a statistically significant association that has no meaning. Any association will eventually have a statistically significant point estimate if the investigator keeps adding to the sample size.
Observational studies are here to stay. Their primary strength is that they are the only possible way of studying a number of important research questions, but they are also cheaper and faster than RCTs. The negative side is their lower validity, and readers must carefully assess all the four possible explanations of an association: bias, confounding, chance, cause.
- Open access
- Published: 15 May 2024
The association between ultra-processed food and common pregnancy adverse outcomes: a dose-response systematic review and meta-analysis
- Sepide Talebi 1 , 2 ,
- Sanaz Mehrabani 3 ,
- Seyed Mojtaba Ghoreishy 4 , 5 ,
- Alexei Wong 6 ,
- Aliasghar Moghaddam 7 ,
- Peyman Rahimi Feyli 7 ,
- Parsa Amirian 8 ,
- Mahsa Zarpoosh 8 ,
- Mohammad Ali Hojjati Kermani 9 &
- Sajjad Moradi 10
BMC Pregnancy and Childbirth volume 24 , Article number: 369 ( 2024 ) Cite this article
Metrics details
Given the increasing incidence of negative outcomes during pregnancy, our research team conducted a dose-response systematic review and meta-analysis to investigate the relationship between ultra-processed foods (UPFs) consumption and common adverse pregnancy outcomes including gestational diabetes mellitus (GDM), preeclampsia (PE), preterm birth (PTB), low birth weight (LBW), and small for gestational age (SGA) infants. UPFs are described as formulations of food substances often modified by chemical processes and then assembled into ready-to-consume hyper-palatable food and drink products using flavors, colors, emulsifiers, and other cosmetic additives. Examples include savory snacks, reconstituted meat products, frozen meals that have already been made, and soft drinks.
A comprehensive search was performed using the Scopus, PubMed, and Web of Science databases up to December 2023. We pooled relative risk (RR) and 95% confidence intervals (CI) using a random-effects model.
Our analysis (encompassing 54 studies with 552,686 individuals) revealed a significant association between UPFs intake and increased risks of GDM (RR = 1.19; 95% CI: 1.10, 1.27; I 2 = 77.5%; p < 0.001; studies = 44; number of participants = 180,824), PE (RR = 1.28; 95% CI: 1.03, 1.59; I 2 = 80.0%; p = 0.025; studies = 12; number of participants = 54,955), while no significant relationships were found for PTB, LBW and SGA infants. Importantly, a 100 g increment in UPFs intake was related to a 27% increase in GDM risk (RR = 1.27; 95% CI: 1.07, 1.51; I 2 = 81.0%; p = 0.007; studies = 9; number of participants = 39,812). The non-linear dose-response analysis further indicated a positive, non-linear relationship between UPFs intake and GDM risk P nonlinearity = 0.034, P dose-response = 0.034), although no such relationship was observed for PE (P nonlinearity = 0.696, P dose-response = 0.812).
In summary, both prior to and during pregnancy, chronic and excessive intake of UPFs is associated with an increased risk of GDM and PE. However, further observational studies, particularly among diverse ethnic groups with precise UPFs consumption measurement tools, are imperative for a more comprehensive understanding.
Peer Review reports
Introduction
The Centers for Disease Control and Prevention’s (CDC’s) 2022 National Center for Health Statistics report alarmingly indicates a persistent rise in pregnancy-related mortality in the US across three decades, highlighting significant disparities in “race” and maternal age [ 1 ]. This trend underscores the pivotal role of addressing common pregnancy adverse outcomes as a critical component of maternal morbidity and mortality prevention strategies [ 2 ].
Promoting healthy dietary habits during pregnancy is imperative to meet the increased physiological needs of expectant mothers. The phenomenon of “nutritional transition”, characterized by a shift towards high-calorie, low-micronutrient foods, culminates in malnutrition and obesity [ 3 ]. The significance of maternal nutrition in prenatal care is heavily emphasized by researchers as a preventive measure against adverse pregnancy outcomes [ 4 ]. The consumption of diets rich in refined carbohydrates, fats, and sweets is linked to an increased risk of gestational diabetes mellitus (GDM) and preterm birth (PTB) [ 5 ]. Moreover, such dietary patterns adversely affect women’s health by exacerbating hypertensive disorders and contributing to conditions like preeclampsia (PE), low birth weight (LBW), and small-for-gestational-age (SGA) infants [ 6 ]. Recognizing the detrimental impact of these unhealthy dietary patterns, it becomes crucial to consider the role of food processing in the maternal diet.
The NOVA classification, a framework for grouping edible substances, categorizes foods into four groups based on the extent and purpose of food processing applied, ranging from unprocessed or minimally processed foods to ultra-processed foods (UPFs) [ 7 , 8 ]. UPFs are characterized by their high content of additives such as preservatives, artificial flavors, colors, and sweeteners, and are typically devoid of whole or minimally processed ingredients [ 9 ]. The consumption of UPFs has been associated with higher risks of obesity, hypertension, cancer, and other chronic diseases [ 8 , 10 , 11 , 12 ]. These foods are implicated in disrupting insulin signaling, promoting excessive energy intake, weight gain, and increased urinary concentrations of phthalate metabolites, which act as endocrine disruptors [ 13 , 14 ]. In the context of adverse pregnancy outcomes, recent meta-analytic work highlighted a heightened risk of GDM (odds ratio (OR): 1.48; 95% confidence interval (CI): 1.17, 1.87) and PE (OR: 1.28; 95% CI: 1.15, 1.42) among high UPFs consumers, with no significant associations observed in LBW, PTB, and Large for Gestational Age (LGA) [ 15 ]. However, the previous meta-analysis did not encompass a comprehensive set of extant studies for each adverse outcome (as evidenced by the inclusion of only 10 studies for GDM in contrast to the 44 studies incorporated in our current investigation), thereby underscoring the challenge posed by the unutilized data in previous analyses. Additionally, recent studies of relevance have emerged [ 16 , 17 , 18 ] and the preceding meta-analytic work did not include a dose-response analysis [ 15 ]. The integration of dose-response analysis offers benefits such as facilitating the formulation of public health directives, augmenting precision, and quantifying the dose-response relationship. Consequently, we decided to conduct an updated dose-response systematic review and meta-analysis to rigorously evaluate the association between UPFs consumption and common adverse pregnancy outcomes, including GDM, SGA, LBW, PTB, and PE.
This systematic review and meta-analysis was conducted according to the guidelines specified in the 2020 Preferred Reporting Items for Systematic Reviews and Meta-Analyses (PRISMA) [ 19 ]. The study protocol was registered with the International Prospective Register of Systematic Reviews Database (PROSPERO) under the registration number CRD42023486135.
Literature search and selection
A systematic literature search was done employing PubMed/MEDLINE, ISI Web of Science and Scopus, with no date restrictions, up to December 6, 2023. The search keywords and strategy are reported in Supplementary Table 1 . Data from grey literature sources such as notes, conference abstracts, reviews, case reports, letters, short surveys, and reports were obtained from a manual search of references mentioned in original research articles published in one of these databases. To augment the breadth of research identified, references within reviews and pertinent studies that met eligibility criteria were further subjected to manual examination.
Inclusion and exclusion criteria
Inclusion criteria were defined as follows: a) observational studies (cohort, case-control, or cross-sectional,) in adult subjects (≥18 years) reporting data on the association between UPFs intake and the risk of adverse pregnancy outcomes (including GDM PE, PTB, LBW, and SGA infants), and reporting effect estimates in the form of hazard ratio (HR), relative risk (RR), or odds ratios (OR), each with at least 95% confidence interval (95% CI). Exclusion criteria included: a) studies done in children and adolescents (< 18 years), b) studies without sufficient data (for instance, those failing to report effect sizes or 95% CIs, instead reporting beta coefficients), and c) those with no relevant exposure. Study titles and abstracts, as well as full-text reviews from database searches meeting the inclusion criteria, were assessed by two reviewers (ST and SM). Any disagreements regarding study inclusion/exclusion criteria were resolved by consensus following discussion. The PICOS tool for each article was reported in Supplementary Table 2 .
Data extraction
Two investigators (FJ and SM) extracted the following data, based on a standardized extraction form, from the studies that met the inclusion criteria: a) first author’s name, year of publication, and country of origin; b) study characteristics (design, follow-up period, and source of data on health status); c) participant characteristics (number of participants/cases, age and sex); d) methods of evaluating UPFs intake; e) the risk of adverse pregnancy outcomes; f) main study results (outcomes), and g) covariates utilized for adjustments in multivariate analyses. Any disagreement regarding data extraction characteristics was decided by consensus following the discussion.
Quality assessment
Applying the Newcastle-Ottawa Scale (NOS) [ 20 ], two investigators assessed the quality of each shortlisted study. The NOS was specifically chosen due to its comprehensive framework designed to evaluate the quality of non-randomized studies. This scale excels in its design, content, and user-friendliness, making it particularly suitable for integrating quality assessments into the interpretation of meta-analytic results. The NOS scale for systematic reviews or meta-analyses, allocating up to 9 points across three domains: study group selection (four points), study group comparability (two points), and exposure and outcomes ascertainment for case-control or cohort studies (three points). Studies scoring 7–9 are deemed high quality/low risk of bias, whereas a score of 0–3 indicates a high risk of bias. Table 1 shows the consensus from this assessment.
Statistical analyses and data synthesis
Statistical analyses were performed applying STATA version 14.0 (StataCorp, College Station, TX, USA) and SPSS version 25.0 (IBM, Armonk, NY, USA). The RR and 95% CI were established as overall effect sizes in this work, similar to effect estimates reported in observational articles meeting the inclusion criteria for this meta-analysis [ 21 ]. The synthesized effect estimates were reported as pooled RR with 95% CI. Due to anticipated heterogeneity between studies, effect estimates were calculated using the DerSimonian-Laird weighted random-effects model [ 22 ]. A pairwise meta-analysis combined the effect size results for the highest and lowest categories of UPFs intake. Heterogeneity among the articles was examined by the Cochran Q and I-squared (I 2 ) statistics, with the I 2 value estimated from [(Q-df)/Q × 100%]; where Q is the χ 2 value and df the corresponding degrees of freedom. Between-study heterogeneity was considered significant when the Cochran Q statistic was significant ( p < 0.05) or if I 2 > 50%; specifically, low, moderate, high, and extreme heterogeneity was defined based on the I 2 statistics cut-offs of < 25%, 25–50%, 50–75%, and >75%, respectively.
Furthermore, subgroup analyses were conducted to evaluate any potential effects due to the study design (cross-sectional, case-control, or cohort), UPFs classification method (NOVA food classification, Western-type diet pattern, fast-food, or sweets consumption), the study region of origin (North America, South America, Asia, Europe, and Australia), pre-pregnancy body mass index (< 25 kg/m 2 and ≥ 25 kg/m 2 ) [ 23 , 24 ], age (< 30 years and ≥ 30 years) [ 24 ], number of cases (< 100 or ≥ 100), number of participants (< 1000 or ≥ 1000), dietary assessment method (food frequency questionnaires [FFQ], 24 h recall, or food records), dietary assessment period (pre-pregnancy, early pregnancy, mid-pregnancy), and other covariate adjustments. Sensitivity analysis was conducted by omitting each study and evaluating the remaining pooled effect estimates. Publication bias was evaluated by visual inspection of funnel plots, and formal testing using Egger’s regression asymmetry and Begg’s rank correlation tests [ 25 , 26 ], with outcomes considered as significant at p < 0.05.
A dose-response meta-analysis was completed to estimate the RRs per 100 g increment in UPFs intake, based on the method introduced by Greenland and colleagues [ 27 , 28 ]. For this process, studies needed to report the number of cases (i.e., participants with incidence) and non-cases (i.e., participants without incidence) or person-years (i.e., the number of people in the study and the duration of their participation) as well as the median point of UPFs intake across more than three categories of intake. Ultimately, a one-stage linear mixed-effects meta-analysis was undertaken to model the dose-response associations, estimating and combining study-specific slope lines to obtain an average slope in a single stage. This linear mixed-effects meta-analysis includes studies with two categories of exposures (at least two effect sizes) in the dose-response analysis.
Quality of evidence
The quality of evidence across articles was ranked employing the Grading of Recommendations Assessment, Development, and Evaluation (GRADE) working group guidelines. The GRADE criteria categorize evidence quality into high, moderate, low, or very low levels [ 29 ].
Study characteristics
Our systematic search and examination of reference lists yielded a total of 3433 records. After omitting duplicates, 2787 articles remained for assessment (Fig. 1 ). A title and abstract review led to the removal of 2707 articles. Subsequent full-text assessment of the 80 remaining studies resulted in the exclusion of a further 26 articles for the following reasons: five articles reported outcomes not relevant to our research scope, six lacked sufficient data, and 15 did not focus on relevant exposure (Supplemental Table 3 ). Consequently, 54 studies met our inclusion criteria and were selected in the present meta-analysis [ 16 , 17 , 18 , 30 , 31 , 32 , 33 , 34 , 35 , 36 , 37 , 38 , 39 , 40 , 41 , 42 , 43 , 44 , 45 , 46 , 47 , 48 , 49 , 50 , 51 , 52 , 53 , 54 , 55 , 56 , 57 , 58 , 59 , 60 , 61 , 62 , 63 , 64 , 65 , 66 , 67 , 68 , 69 , 70 , 71 , 72 , 73 , 74 , 75 , 76 , 77 , 78 , 79 ].
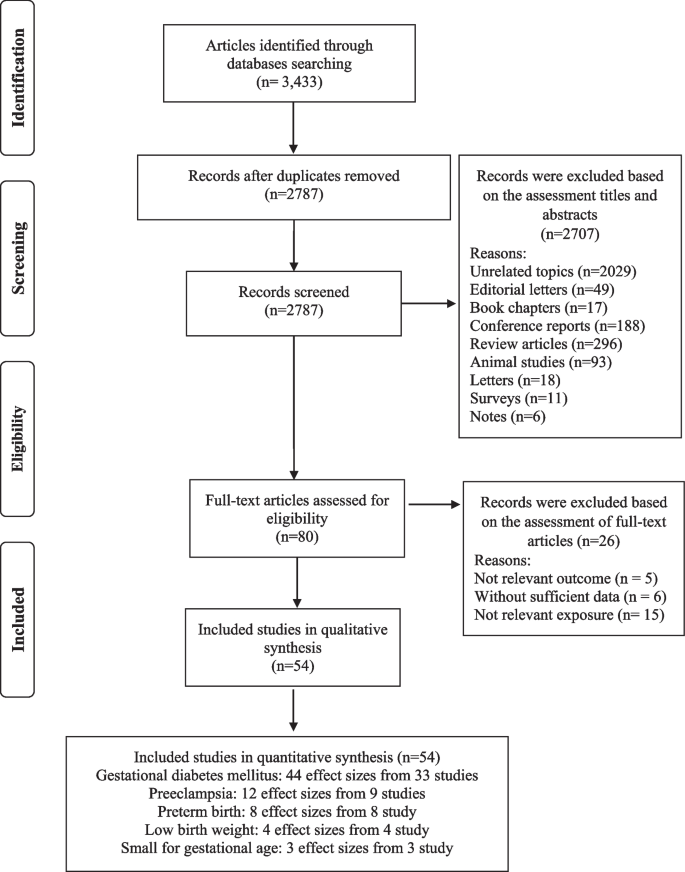
Flow chart of the process of the study selection
The selected studies (detailed in Supplemental Table 4 ) encompass 38 cohort studies [ 16 , 31 , 33 , 34 , 35 , 36 , 37 , 39 , 40 , 42 , 43 , 44 , 45 , 46 , 47 , 48 , 49 , 51 , 52 , 54 , 55 , 57 , 58 , 59 , 60 , 61 , 62 , 65 , 66 , 67 , 69 , 70 , 71 , 73 , 74 , 75 , 78 ], 11 case-control studies [ 17 , 18 , 30 , 32 , 34 , 50 , 63 , 66 , 68 , 72 , 77 ], and five cross-sectional studies [ 38 , 46 , 64 , 76 , 79 ]. These articles, conducted between 1988 and 2023, originated from different countries including the USA [ 33 , 36 , 53 , 58 , 60 , 62 , 69 , 74 , 78 ], the UK [ 16 ], China [ 43 , 49 , 51 , 71 , 73 ], Brazil [ 31 , 59 , 63 , 64 , 68 , 79 ], Spain [ 39 , 40 , 42 , 55 , 57 ], Iran [ 17 , 18 , 30 , 32 , 48 , 54 , 66 , 76 , 77 ], Malaysia [ 75 ], Palestine [ 72 ], Australia [ 45 , 46 , 65 ], Singapore [ 37 , 38 ], Norway [ 35 , 44 , 47 ], Japan [ 41 , 67 ], Czech Republic [ 34 ], Iceland [ 70 ] and Denmark [ 61 ]. The study-specific, maximally adjusted RR was reported for 552,686 individuals across the included articles and was pooled for meta-analysis to assess the association between UPFs and the risk GDM [ 16 , 32 , 33 , 34 , 36 , 38 , 39 , 40 , 41 , 43 , 48 , 49 , 50 , 51 , 53 , 54 , 55 , 56 , 59 , 60 , 64 , 65 , 66 , 70 , 71 , 72 , 73 , 74 , 75 , 77 , 78 , 79 ], PE [ 17 , 18 , 30 , 35 , 48 , 52 , 62 , 69 , 74 , 76 ], PTB [ 31 , 37 , 44 , 45 , 46 , 48 , 52 , 58 , 61 , 67 ], LBW [ 45 , 63 , 67 ] and SGA infants [ 46 , 67 , 68 ]. The Newcastle-Ottawa grade (used for quality assessment) categorized 27 studies as high quality [ 17 , 33 , 35 , 36 , 37 , 39 , 40 , 41 , 42 , 43 , 44 , 45 , 47 , 51 , 53 , 55 , 57 , 58 , 60 , 62 , 65 , 67 , 69 , 74 , 75 , 78 ] and 27 as medium quality [ 16 , 18 , 30 , 31 , 32 , 34 , 38 , 46 , 48 , 49 , 50 , 52 , 54 , 56 , 59 , 61 , 63 , 64 , 66 , 68 , 70 , 71 , 72 , 73 , 76 , 77 , 79 ]. Moreover, the outcomes revealed that the level of agreement between investigators for data collection as well as for quality assessment was appropriate (Kappa = 0.897).
Ultra-processed food and common adverse pregnancy outcomes
Our results suggested a significant relationship between higher UPF intake and an increased risk of GDM (RR = 1.19; 95% CI: 1.10, 1.27; I 2 = 77.5%; p < 0.001; n = 44), PE (RR = 1.28; 95% CI: 1.03, 1.59; I 2 = 80.0%; p = 0.025; n = 12), but not PTB (RR = 1.06; 95% CI: 0.97, 1.17; I 2 = 34.2%; p = 0.231; n = 8), LBW (RR = 1.01; 95% CI: 0.91, 1.12; I 2 = 52.2%; p = 0.905; n = 4) and SGA infants (RR = 1.11; 95% CI: 0.81, 1.52; I 2 = 66.3%; p = 0.532; n = 3), (Refer to Table 1 , Supplementary Fig. 1 ).
In the context of GDM, subgroup analysis showed that a greater UPFs intake was significantly associated with an enhanced risk in cohort studies (vs. cross-sectional) (RR = 1.18; 95% CI: 1.09, 1.27; I 2 = 79.3%; p < 0.001; n = 31) and case-control studies (RR = 2.06; 95% CI: 1.31, 3.35; I 2 = 77.7%; p = 0.002; n = 10), particularly in studies assessed western dietary pattern (RR = 1.34; 95% CI: 1.01, 1.76; I 2 = 43.0%; p = 0.040; n = 7) or fast-foods (RR = 1.32; 95% CI: 1.15, 1.51; I 2 = 79.3%; p < 0.001; n = 22), (vs. NOVA classification or sweets consumption), in North America (vs. Europe, South America, Asia and Australia) (RR = 1.43; 95% CI: 1.27, 1.53; I 2 = 45.4%; p < 0.001; n = 10), and across studies with > 100 number of case (RR = 1.38; 95% CI: 1.21, 1.58; I 2 = 74.8%; p < 0.001; n = 12)(vs. < 100 number of case), in studies with > 1000 number of participants (RR = 1.33; 95% CI: 1.15, 1.54; I 2 = 76.9%; p < 0.001; n = 21)(vs. < 1000 number of participants), in studies used FFQ for dietary assessment (RR = 1.27; 95% CI: 1.14, 1.43; I 2 = 78.6%; p < 0.001; n = 34) (vs. 24 h recall or food record), particularly in studies where the period of dietary assessment was at early pregnancy (RR = 1.26; 95% CI: 1.09, 1.46; I 2 = 80.5%; p = 0.002; n = 19) (vs. pre-pregnancy or mid-pregnancy). Moreover, subgroup analysis for covariates adjustment showed that BMI and physical activity may influence the association between UPF intake and the risk of GDM (Table 2 ).
For PE, the subgroup analysis also highlighted that greater UPFs intake was significantly associated with an enhanced risk in studies assessed western dietary pattern (RR = 2.51; 95% CI: 1.13, 5.57; I 2 = 91.1%; p = 0.023; n = 3) or NOVA classification (RR = 1.22; 95% CI: 1.04, 1.42; I 2 = 0.0%; p = 0.013; n = 3), (vs. sweets consumption), in Asia (vs. Europe or US areas) (RR = 1.65; 95% CI: 1.07, 2.55; I 2 = 86.1%; p < 0.001; n = 6), and across studies with > 100 number of case (RR = 1.57; 95% CI: 1.03, 2.40; I 2 = 93.2%; p < 0.001; n = 4)(vs. < 100 number of case), in studies with number of < 1000 participants (RR = 1.65; 95% CI: 1.07, 2.55; I 2 = 86.1%; p = 0.023; n = 6)(vs. > 1000 number of participants), in participants aged ≥30 years (RR = 1.28; 95% CI: 1.07, 1.54; I 2 = 50.4%; p = 0.089; n = 5)(vs. participants aged < 30 years), in participants with pre-pregnancy-BMI > 25 (RR = 1.52; 95% CI: 1.07, 2.15; I 2 = 84.7%; p = 0.021; n = 1)(vs. participants with pre-pregnancy-BMI ≤ 25), in studies used FFQ for dietary assessment (RR = 1.38; 95% CI: 1.10, 1.72; I 2 = 82.6%; p = 0.005; n = 10) (vs. questions), and particularly in studies where the period of dietary assessment was at mid-pregnancy (RR = 1.23; 95% CI: 1.05, 1.43; I 2 = 38.8%; p = 0.009; n = 3) (vs. early pregnancy). Furthermore, subgroup analysis for covariates adjustment showed that BMI and physical activity may influence the association between UPF intake and the risk of PE (Table 3 ).
Linear and non-linear dose-response analysis
The linear dose-response analysis (refer to Table 1 and Fig. 2 ) indicates a 27% increase in GDM risk per 100 g increment in UPF intake RR = 1.27; 95% CI: 1.07, 1.51; I 2 = 81.0%; p = 0.007; n = 9). However, the linear dose-response analysis for other outcomes was not undertaken due to the limited number of studies available.
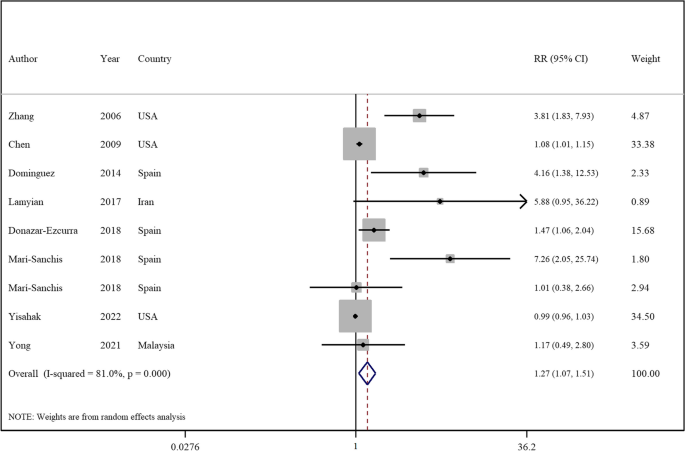
Forest plots showing the linear dose-response meta-analysis of mortality risk for 100 g change in ultra-processed food consumption in daily intake and risk of gestational diabetes mellitus
The non-linear dose-response analysis revealed a positive non-linear relationship between UPFs intake and GDM risk (P nonlinearity = 0.034, P dose-response = 0.034, Fig. 3 ), but not for PE (P nonlinearity = 0.696, P dose-response = 0.812, Fig. 4 ). The non-linear dose-response analysis was not conducted for other outcomes due to insufficient studies.
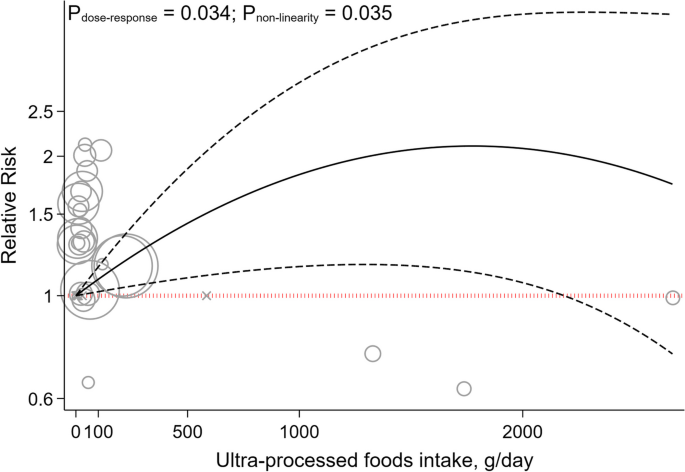
Non-linear dose-response indicated associations between UPF intake and the risk of gestational diabetes mellitus
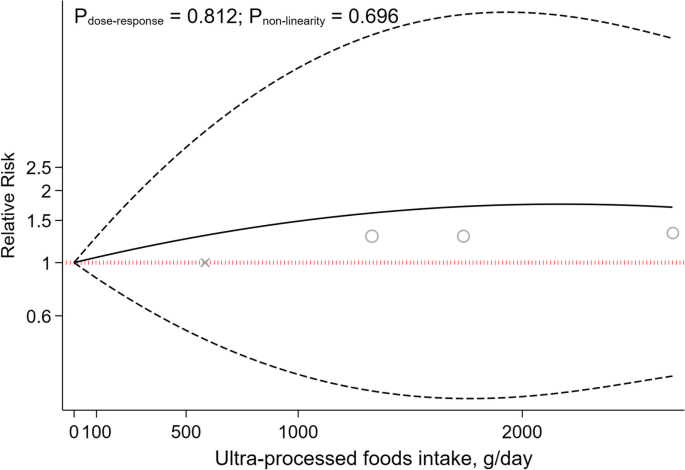
Non-linear dose-response indicated associations between UPF intake and the risk of preeclampsia
Sensitivity analyses and publication bias
Sensitivity analysis across the highest to the lowest meta-analysis for GDM, PE, PTB, LBW and SGA infants showed no significant influence of any single study (Supplemental Fig. 2 ).
No evidence of publication bias was found in articles related to the association with an increased risk of PE ( p = 0.529, Egger’s test; p = 0.891, Begg’s), PTB ( p = 0.458, Egger’s test; p = 0.473, Begg’s), LBW ( p = 0.905, Egger’s test; p = 1.00, Begg’s test), and SGA infants ( p = 0.348, Egger’s test; p = 1.00, Begg’s test). Although, for GDM, Egger’s test indicated potential publication bias ( p < 0.001), not corroborated by Begg’s test ( p = 0.241). As illustrated in Supplemental Fig. 3 , the funnel plot was symmetrical for the association between the UPFs intake and all outcomes, except for studies that reported the risk of GDM disease.
Utilizing the GRADE scale for quality evaluation, we detected the evidence for associations between UPFs intake and risk of GDM, PE, PTB, LBW and SGA infants was classified as moderate (Refer to Table 1 ).
In the realm of maternal and fetal health, the quality of dietary intake during pregnancy is of paramount significance. Accumulating evidence suggests a correlation between the consumption of UPFs and the deterioration of diet quality, potentially elevating the risk of various health complications [ 80 , 81 , 82 ]. This systematic review and meta-analysis aimed to elucidate the relationship between UPFs intake and adverse pregnancy outcomes including GDM, SGA, LBW, PTB, and PE, through an integrative analysis of existing studies. Our outcomes indicate a significant association between UPFs consumption and increased risks of PE, and GDM either prior to or during pregnancy. However, no significant association was found between UPFs intake and the risks of LBW, SGA, and PTB. Importantly, a 27% increment in the incidence of GDM was linked to a 100 g increase in UPF intake. Furthermore, a positive, non-linear relationship between UPF intake and GDM risk was identified through non-linear dose-response analysis, albeit no analogous association was found for PE.
The results of the current work showed a positive association between UPFs consumption and the risk of PE. In addition, subgroup analysis revealed this relationship to be more pronounced in studies using the NOVA-food classification and a Western dietary pattern for UPFs intake assessment, compared to those focusing on sweet intake. The NOVA classification categorizes foods based on the extent of processing, encompassing various UPFs. Moreover, the association between UPFs consumption and the risk of PE was significant in studies conducted in Asia (vs other regions). Prevalence of PE varies globally, ranging from 0.2–6.7% in Asia, 2.8–9.2% in Oceania, 2.8–5.2% in Europe, 2.6–4.0% in North America, and 1.8–7.7% in South America and the Caribbean [ 83 ]. However, the high heterogeneity in Asian studies should be noted when interpreting this result. Furthermore, a significant association was observed in studies involving women aged 30 years or older, aligning with the increased PE risk associated with advanced maternal age [ 84 ]. Additionally, a significant association was identified between PE risk and UPFs intake in women with pre-pregnancy BMI higher than 25 kg/m 2 (vs BMI ≤25). This aligns with previous findings linking excessive weight gain in expectant mothers to an elevated PE risk, with overweight and obese mothers facing substantially higher risks [ 85 ].
The association between UPFs intake and PE can be elucidated through several mechanisms. The risk factors for PE, including GDM, maternal obesity, and advanced maternal age, are extensively documented in the literature [ 84 , 85 , 86 ]. It has been established that adopting healthy lifestyle habits (including dietary patterns) can mitigate these risk factors [ 87 ]. A higher intake of UPFs is associated with a diminished dietary quality, marked by an increased consumption of sugars and fats, alongside a decrease in fiber, protein, vitamins, and minerals [ 88 , 89 ]. UPFs are known to contain elevated levels of pro-inflammatory agents such as refined sugars, salt, and trans fats. The ingestion of these inflammatory components can precipitate oxidative stress and systemic inflammation [ 90 , 91 , 92 ], which are implicated in the pathogenesis of preeclampsia [ 93 , 94 ]. Additionally, the presence of trans fatty acids, added phosphates, and a high salt content in UPFs may impair endothelial function [ 95 , 96 , 97 ], a critical factor in the pathophysiology of hypertension observed in preeclampsia [ 98 ]. Furthermore, the intake of UPFs can alter the composition and diversity of the gut microbiota [ 99 ]. Studies have shown that food additives commonly found in UPFs, such as emulsifiers, sweeteners, and colorants, adversely affect the gut flora [ 100 ]. The interplay between the gut microbiota and the placenta, referred to as the “gut–placenta” axis, is crucial for understanding the etiology of PE. Dysbiosis of the gut microbiota and bacterial products like lipopolysaccharide (LPS) have been identified as promotive of PE [ 101 , 102 ]. According to Kell et al., microbial infection, particularly through bacterial products such as LPS (also known as endotoxin), which is highly inflammatory, can initiate an innate immune response that exacerbates inflammation [ 103 ]. Hence, it is hypothesized that dysbiosis induced by UPFs consumption may play a significant role in the promotion of preeclampsia. Moreover, UPFs intake is positively associated with a risk of obesity [ 104 ], a condition marked by insulin resistance and hyperinsulinemia, crucial factors in PE development [ 105 ]. Pregnant women with obesity and PE exhibit higher leptin levels, correlating with increased Tumor Necrosis Factor-Alfa (TNF-α), Interleukin 6 (IL-6), and C-reactive protein concentrations [ 106 , 107 ]. Additionally, excessive adipose tissue near the reproductive tract is the source of increased complement components and fragments in preeclamptic pregnancies. These complement proteins may promote an imbalance in angiogenic factors (characterized by increased production of antiangiogenic factors and a decrease in proangiogenic factors). This imbalance leads to placental injury, resulting in decreased blood flow to the tissue, and is accompanied by changes in cytokines levels (decreased IL-10 and increased TNF-α) before the onset of PE [ 108 ].
Our pooled analysis also revealed that higher UPFs intake was related to an increased risk of GDM. This association was significant in studies employing cohort and case-control designs (as opposed to those with cross-sectional methodologies). The inherent recall bias in cross-sectional studies that rely on self-reporting, is a notable limitation affecting the reliability of outcomes [ 109 ]. Moreover, this association was more pronounced in studies that used Western dietary patterns and fast-food consumption for the assessment of UPFs intake (vs those employing NOVA food classification and sweet consumption metrics). The concept of a dietary pattern, which represents the aggregate of eating and drinking habits, is critical as it exerts a greater impact on health and chronic diseases than any individual food item [ 110 ]. Additionally, the application of the NOVA food classification in existing studies is less frequent, suggesting the need for further research utilizing this methodology to derive more meaningful results. Geographical variations were also evident, with significant associations observed in studies conducted in America, compared to those in Asia and Europe. This is in context with the differing regional prevalences of GDM: 7.1% in North America and the Caribbean, 7.8% in Europe and 20.8% in South-East Asia [ 111 ]. Despite the higher prevalence of GDM in Asian populations, the greater intake of UPFs in American and European cohorts may have influenced the study outcomes [ 112 , 113 , 114 , 115 ]. Additionally, a positive association between UPFs intake and GDM risk was observed in studies focusing on women with a pre-pregnancy BMI > 25. Previous research indicates that being overweight or obese before and during pregnancy is a significant risk factor for GDM [ 116 , 117 , 118 ]. However, the scarcity of studies in women with pre-pregnancy BMI > 25 kg/m 2 kg/m suggests the need for more research in this demographic for robust conclusions.
Our outcomes also indicated that a 100 g increase in UPF intake was associated with a 27% increase in the risk of GDM. Moreover, the non-linear dose-response analysis similarly showed a positive, non-linear association between the consumption of UPFs and the risk of GDM. These findings underscore the significant impact that UPF consumption can have on GDM risk. The evidence points towards a robust and worrying correlation where even moderate increases in UPF intake can precipitate a marked rise in GDM risk, highlighting the critical need for dietary awareness and intervention among pregnant women. This aligns with broader nutritional science, emphasizing the importance of minimizing UPF consumption to mitigate not only GDM risk but potentially other metabolic disorders as well, given the multitude of adverse mechanisms through which UPFs affect glucose metabolism and insulin sensitivity.
Pathophysiologically, UPFs intake may increase GDM risk through several mechanisms. In pregnant women with GDM, pre-pregnancy reduced insulin sensitivity and β-cell dysfunction lead to hyperglycemia [ 119 , 120 ]. The hypothesis that excessive sugar intake may augment body mass, thereby indirectly precipitating insulin resistance and subsequent diabetes, is widely recognized. Moreover, the liver’s capacity to assimilate and metabolize refined sugars prevalent in UPFs (such as fructose and sucrose) may be compromised, leading to augmented fat deposition and deteriorated insulin sensitivity [ 121 ]. Furthermore, insulin resistance may be induced by cosmetic ingredients present in UPFs. For example, dietary additives like carrageenan, employed as a thickening and stabilizing agent, may interfere with insulin signaling and thus foster insulin resistance [ 122 ]. Additionally, UPFs intake correlates with increased production of reactive oxygen species and inflammatory biomarkers [ 123 ], inducing insulin resistance through molecular pathways such as β-cell and mitochondrial dysfunction, decreased GLUT4 expression, impaired insulin signaling and heightened inflammatory responses [ 124 ]. Furthermore, UPFs often contain packaging materials like phthalates and bisphenol A, known to have endocrine disruption properties that may contribute to insulin resistance and diabetes development [ 125 , 126 ]. The ingestion of substantial quantities of UPFs also elevates inflammation, a pivotal factor in the genesis of insulin resistance, culminating in hyperglycemia and the development of GDM [ 127 ]. A diet replete with saturated fats, trans fats, sugars, and salt, characteristic of high UPFs consumption, may contribute to chronic inflammation [ 128 ]. Furthermore, excessive UPFs consumption may supplant essential components of a balanced and nutritious diet. For instance, fruits and vegetables are associated with an anti-inflammatory effect [ 129 ]. In addition, the leaching of chemicals from food packaging into UPFs could introduce non-nutritional elements such as phthalates or bisphenol A, potentially eliciting an inflammatory response [ 130 ].
The present study did not establish a significant association between UPFs consumption and the risk of LBW. This result may be attributable to several factors. Firstly, a limited number of studies have evaluated the association between UPFs intake and LBW risk. Additionally, the intake of high-sugar foods (such as sugar-sweetened beverages) has been correlated with an increased risk of LBW in non-GDM subjects [ 34 , 40 ]. This could be attributed to impaired fetal nutrition due to reduced vascular function, potentially induced by oxidative stress, inflammation, and endothelial dysfunction associated with high sugar consumption [ 131 ]. However, in GDM subjects this association may not be found due to the higher glucose loads in the fetus [ 47 ]. Therefore, additional research is warranted in both GDM and non-GDM populations to elucidate these relationships comprehensively.
Moreover, SGA was not associated with the intake of UPFs according to the pooled analysis of conducted studies. Although additional studies are necessary to explore this relationship further, existing evidence suggests that a fast-food dietary pattern may lead to increased fat intake and a reduced intake of essential micronutrients crucial for fetal development [ 132 ]. Maternal UPFs intake is linked to lower protein intake, reduced overall nutrition quality, and higher intake of trans fats, carbohydrates and saturated fats, which may increase the risk of neonatal adiposity [ 133 , 134 , 135 ]. Furthermore, higher fast-food intake during pregnancy has been linked with an elevated risk of maternal obesity, which in turn, may increase the likelihood of LGA babies [ 132 , 136 ].
Regarding PTB, the current study found no association with UPFs consumption. Previous research has indicated that dietary patterns rich in fruits and vegetables are associated with a lower risk of PTB [ 37 , 45 ]. Inadequate nutrition before and during pregnancy can lead to health issues for both the mother and fetus, increasing the risk of preterm delivery and intrauterine growth retardation [ 137 ]. The absence of an association between UPFs consumption and preterm delivery in this study could be due to various factors, including the need for a higher UPFs consumption threshold during pregnancy to manifest negative impacts on preterm birth. Additionally, the varied diet of pregnant women, typically including beneficial foods such as fruits, vegetables and nuts, may mitigate the adverse effects of UPFs.
The current investigation has several crucial strengths that make its findings highly significant. Firstly, by pooling all available observational data on the topic, the study provides a comprehensive and robust analysis of the relationship between UPFs intake and adverse pregnancy outcomes. Secondly, the study’s use of a dose-response analysis adds further weight to its conclusions and bolsters our understanding of the link between these two factors. However, there are limitations to consider. These include potential information and recall biases due to the self-reported nature of dietary intake assessments (such as the FFQ) and the absence of specific dietary tools for assessing UPFs consumption. Additionally, this meta-analysis included studies that did not use NOVA’s specialized dietary assessments. Moreover, dietary changes following pregnancy discovery could affect results, and the observational nature of the included studies precludes causal inference. Despite the inclusion of numerous confounding variables, several factors must be cautiously considered in the interpretation of the research findings. For instance, the socio-economic status of participants influences their dietary habits, while race and ethnicity may affect pregnancy outcomes. Furthermore, disparities in access to healthcare services can impact dietary choices and pregnancy outcomes. Other health statuses, such as mental health conditions among pregnant subjects, also influence dietary selections and pregnancy results [ 138 , 139 ]. Finally, the availability of data on broader categories such as diabetes in pregnancy and hypertensive disorders was limited, hindering our ability to conduct a comprehensive analysis on these broader categories.
Our outcomes indicate that prior to or during pregnancy, UPFs intake is associated with a higher risk of GDM and PE. However, no significant link tying UPFs intake to SGA, LWB and PTB was established. Importantly, a 100 g increment in UPFs intake was related to a 27% increase in GDM risk. This study aligns with global trends, where a rise in adverse pregnancy outcomes seems to align with the escalation of industrialization and the corresponding surge in UPFs production and consumption. Investigating the potential linkage between UPFs intake and the rise of adverse pregnancy outcomes may help in the development of nutrition-centric policies for expecting mothers and promote more health-conscious decision-making. To further substantiate these findings, extensive empirical research is required. Future studies should encompass observational research across diverse ethnic groups. Moreover, the adoption of more precise tools for measuring UPFs consumption is imperative. In observational research, it may be challenging to ascertain whether the consumption of UPFs directly contributes to adverse pregnancy outcomes or if it serves as an indicator of other underlying factors. Components of UPFs may escalate the risk of negative pregnancy outcomes. Moreover, UPF consumption could be part of a complex interplay affecting other variables that directly result in adverse outcomes. For instance, UPF intake could influence gestational weight gain, potentially leading to insulin resistance, which is known to correlate with unfavorable pregnancy outcomes, including GDM. Additionally, the consumption of UPFs may not only diminish dietary quality but also be linked with various lifestyle and dietary factors, such as poor diet quality, thereby increasing the risk of adverse pregnancy outcomes [ 140 ]. The bidirectional correlation between UPF consumption and unfavorable pregnancy outcomes also merits consideration. For example, individuals experiencing depression or other health conditions might alter their dietary patterns to include a higher intake of UPFs [ 138 ]. Evaluating changes in UPF consumption over time, utilizing precise questionnaires that assess food items classified as UPFs according to the NOVA food classification system, and their association with other health outcomes affecting pregnancy, such as obesity, could offer insights into this relationship. Considering these approaches is essential to enhance the depth and accuracy of investigations into the potential association between UPFs intake and the incidence of adverse pregnancy outcomes.
Availability of data and materials
The datasets generated and/or analyzed during the current study are not publicly available due but are available from the corresponding author on reasonable request.
Hoyert DL, Miniño AM. Maternal Mortality in the United States: Changes in Coding, Publication, and Data Release, 2018. Natl Vital Stat Rep. 2020;69(2):1–18.
PubMed Google Scholar
Parikh NI, Gonzalez JM, Anderson CA, Judd SE, Rexrode KM, Hlatky MA, et al. Adverse pregnancy outcomes and cardiovascular disease risk: unique opportunities for cardiovascular disease prevention in women: a scientific statement from the American Heart Association. Circulation. 2021;143(18):e902–e16.
Article CAS PubMed Google Scholar
Hashmi AH, Solomon N, Lee SJ, Min AM, Gilder ME, Wiladphaingern J, et al. Nutrition in transition: historical cohort analysis summarising trends in under-and over-nutrition among pregnant women in a marginalised population along the Thailand–Myanmar border from 1986 to 2016. Br J Nutr. 2019;121(12):1413–23.
Lind JM, Hennessy A, McLean M. Cardiovascular disease in women: the significance of hypertension and gestational diabetes during pregnancy. Curr Opin Cardiol. 2014;29(5):447–53.
Article PubMed Google Scholar
Englund-Ögge L, Birgisdottir BE, Sengpiel V, Brantsæter AL, Haugen M, Myhre R, et al. Meal frequency patterns and glycemic properties of maternal diet in relation to preterm delivery: results from a large prospective cohort study. PLoS One. 2017;12(3):e0172896.
Article PubMed PubMed Central Google Scholar
Mekie M, Mekonnen W, Assegid M. Cohabitation duration, obstetric, behavioral and nutritional factors predict preeclampsia among nulliparous women in West Amhara zones of Ethiopia: age matched case control study. PLoS One. 2020;15(1):e0228127.
Article CAS PubMed PubMed Central Google Scholar
Monteiro CA, Cannon G, Levy R, Moubarac J-C, Jaime P, Martins AP, et al. NOVA The star shines bright. World Nutrition. 2016;7(1–3):28–38.
Google Scholar
da Costa Louzada ML, Baraldi LG, Steele EM, Martins APB, Canella DS, Moubarac J-C, et al. Consumption of ultra-processed foods and obesity in Brazilian adolescents and adults. Prev Med. 2015;81:9–15.
Article Google Scholar
Monteiro CA, Levy RB, Claro RM, Castro IRR, Cannon G. A new classification of foods based on the extent and purpose of their processing. Cadernos de saude publica. 2010;26:2039–49.
Mendonça RD, Pimenta AM, Gea A, de la Fuente-Arrillaga C, Martinez-Gonzalez MA, Lopes ACS, et al. Ultraprocessed food consumption and risk of overweight and obesity: the University of Navarra Follow-up (SUN) cohort study. Am J Clin Nutr. 2016;104(5):1433–40.
Mendonça RD, Lopes ACS, Pimenta AM, Gea A, Martinez-Gonzalez MA, Bes-Rastrollo M. Ultra-processed food consumption and the incidence of hypertension in a Mediterranean cohort: the Seguimiento Universidad de Navarra project. Am J Hypertens. 2017;30(4):358–66.
Fiolet T, Srour B, Sellem L, Kesse-Guyot E, Allès B, Méjean C, Deschasaux M, Fassier P, Latino-Martel P, Beslay M, Hercberg S, Lavalette C, Monteiro CA, Julia C, Touvier M. Consumption of ultra-processed foods and cancer risk: results from NutriNet-Santé prospective cohort. BMJ. 2018;360:k322. https://doi.org/10.1136/bmj.k322 .
Bhattacharyya S, Feferman L, Tobacman JK. Carrageenan inhibits insulin signaling through GRB10-mediated decrease in Tyr (P)-IRS1 and through inflammation-induced increase in Ser (P) 307-IRS1. J Biol Chem. 2015;290(17):10764–74.
Buckley JP, Kim H, Wong E, Rebholz CM. Ultra-processed food consumption and exposure to phthalates and bisphenols in the US National Health and nutrition examination survey, 2013–2014. Environ Int. 2019;131:105057.
Paula WO, Patriota ES, Gonçalves VS, Pizato N. Maternal consumption of ultra-processed foods-rich diet and perinatal outcomes: a systematic review and meta-analysis. Nutrients. 2022;14(15):3242.
Mahendra A, Kehoe SH, Kumaran K, Krishnaveni GV, Arun N, Pidaparthy P, et al. Periconceptional diet and the risk of gestational diabetes in south Indian women: findings from the BAngalore nutrition gestational diabetes LiFEstyle study (BANGLES). Lancet Glob Health. 2023;11:S4.
Kooshki A, Sovizi B, Mahmoudi R, Ghezi S, Foroumandi E. The association between food groups and preeclampsia: a case-control study. Hyperten Pregnancy. 2022;41(1):64–9.
Article CAS Google Scholar
Moradi M, Niazi A, Selajgeh F, Mazloumi E. Comparing dietary patterns during pregnancy in women with preeclampsia and healthy women: a case-control study. J Midwife Reproduct Health. 2022;10(1)
Page MJ, McKenzie JE, Bossuyt PM, Boutron I, Hoffmann TC, Mulrow CD, et al. The PRISMA 2020 statement: an updated guideline for reporting systematic reviews. BMJ. 2021;372:n71.
Wells GA, Shea B, O’Connell D, Peterson J, Welch V, Losos M, et al. The Newcastle-Ottawa scale (NOS) for assessing the quality of nonrandomised studies in meta-analyses. Oxford: Ottawa Hospital Research Institute; 2000.
Symons M, Moore D. Hazard rate ratio and prospective epidemiological studies. J Clin Epidemiol. 2002;55(9):893–9.
DerSimonian R, Laird N. Meta-analysis in clinical trials. Control Clin Trials. 1986;7(3):177–88.
Jayedi A, Soltani S, Zargar MS, Khan TA, Shab-Bidar SJB. Central fatness and risk of all cause mortality: systematic review and dose-response meta-analysis of 72 prospective cohort studies. 2020;370.
Song C, Lyu Y, Li C, Liu P, Li J, Ma R, et al. Long-term risk of diabetes in women at varying durations after gestational diabetes: a systematic review and meta-analysis with more than 2 million women 2018;19(3):421–9.
Begg CB, Mazumdar M. Operating characteristics of a rank correlation test for publication bias. Biometrics. 1994;50(4):1088–101.
Egger M, Smith GD, Schneider M, Minder C. Bias in meta-analysis detected by a simple, graphical test. BMJ (Clin res ed). 1997;315(7109):629–34.
Berlin JA, Longnecker MP, Greenland S. Meta-analysis of epidemiologic dose-response data. Epidemiology. 1993;4(3):218–28. https://doi.org/10.1097/00001648-199305000-00005 .
Orsini N, Bellocco R, Greenland SJTsj. Generalized least squares for trend estimation of summarized dose–response data 2006;6(1):40–57.
Guyatt GH, Oxman AD, Vist GE, Kunz R, Falck-Ytter Y, Alonso-Coello P, et al. GRADE: an emerging consensus on rating quality of evidence and strength of recommendations. Bmj. 2008;336(7650):924–6.
Abbasi R, Bakhshimoghaddam F, Alizadeh M. Major dietary patterns in relation to preeclampsia among Iranian pregnant women: a case–control study. J Matern Fetal Neonatal Med. 2021;34(21):3529–36.
Alves-Santos NH, Cocate PG, Benaim C, Farias DR, Emmett PM, Kac G. Prepregnancy dietary patterns and their association with perinatal outcomes: a prospective cohort study. J Acad Nutr Diet. 2019;119(9):1439–51.
Asadi M, Shahzeidi M, Nadjarzadeh A, Hashemi Yusefabad H, Mansoori A. The relationship between pre-pregnancy dietary patterns adherence and risk of gestational diabetes mellitus in Iran: a case–control study. Nutr Diet. 2019;76(5):597–603.
Bao W, Tobias DK, Olsen SF, Zhang C. Pre-pregnancy fried food consumption and the risk of gestational diabetes mellitus: a prospective cohort study. Diabetol. 2014;57(12):2485–91.
Bartáková V, Kuricová K, Zlámal F, Bělobrádková J, Kaňková K. Differences in food intake and genetic variability in taste receptors between Czech pregnant women with and without gestational diabetes mellitus. Eur J Nutr. 2018;57(2):513–21.
Brantsæter AL, Haugen M, Samuelsen SO, Torjusen H, Trogstad L, Alexander J, et al. A dietary pattern characterized by high intake of vegetables, fruits, and vegetable oils is associated with reduced risk of preeclampsia in nulliparous pregnant Norwegian women. J Nutr. 2009;139(6):1162–8.
Chen LW, Hu FB, Yeung E, Willett W, Zhang CL. Prospective study of pre-gravid sugar-sweetened beverage consumption and the risk of gestational diabetes mellitus. Diabetes Care. 2009;32(12):2236–41.
Chia AR, de Seymour JV, Colega M, Chen LW, Chan YH, Aris IM, et al. A vegetable, fruit, and white rice dietary pattern during pregnancy is associated with a lower risk of preterm birth and larger birth size in a multiethnic Asian cohort: the growing up in Singapore towards healthy outcomes (GUSTO) cohort study. Am J Clin Nutr. 2016;104(5):1416–23.
de Seymour J, Chia AR, Colega M, Jones B, McKenzie E, Cai SR, et al. Maternal dietary patterns and gestational diabetes mellitus in a multi-ethnic Asian cohort: the GUSTO study. Nutrients. 2016;8(9)
Dominguez LJ, Martinez-Gonzalez MA, Basterra-Gortari FJ, Gea A, Barbagallo M, Bes-Rastrollo M. Fast food consumption and gestational diabetes incidence in the SUN project. PLoS One. 2014;9(9):e106627.
Donazar-Ezcurra M, Lopez-del Burgo C, Martinez-Gonzalez MA, Basterra-Gortari FJ, de Irala J, Bes-Rastrollo M. Soft drink consumption and gestational diabetes risk in the SUN project. Clin Nutr. 2018;37(2):638–45.
Dong JY, Kimura T, Ikehara S, Cui M, Kawanishi Y, Yamagishi K, et al. Chocolate consumption and risk of gestational diabetes mellitus: the Japan environment and Children's study. Br J Nutr. 2019;122(8):936–41.
Drozd-Dąbrowska M, Trusewicz R, Ganczak M. Selected risk factors of developmental delay in polish infants: a case-control study. Int J Environ Res Public Health. 2018;15(12)
Du HY, Jiang H, Karmin O, Bo C, Xu LJ, Liu SP, et al. Association of dietary pattern during pregnancy and gestational diabetes mellitus: a prospective cohort study in northern China. Biomed Environ Sci. 2017;30(12):887–97.
CAS PubMed Google Scholar
Englund-Ögge L, Brantsæter AL, Sengpiel V, Haugen M, Birgisdottir BE, Myhre R, et al. Maternal dietary patterns and preterm delivery: Results from large prospective cohort study. BMJ (Online). 2014;348
Gete DG, Waller M, Mishra GD. Prepregnancy dietary patterns and risk of preterm birth and low birth weight: findings from the Australian longitudinal study on Women's health. Am J Clin Nutr. 2020;111(5):1048–58.
Grieger JA, Grzeskowiak LE, Clifton VL. Preconception dietary patterns in human pregnancies are associated with preterm delivery. J Nutr. 2014;144(7):1075–80.
Grundt JH, Eide GE, Brantsæter AL, Haugen M, Markestad T. Is consumption of sugar-sweetened soft drinks during pregnancy associated with birth weight? Mater Child Nutrit. 2017;13(4):e12405.
Hajianfar H, Esmaillzadeh A, Feizi A, Shahshahan Z, Azadbakht L. The association between major dietary patterns and pregnancy-related complications. Archiv Iran Med. 2018;21(10):443–51.
Hehua Z, Yang X, Qing C, Shanyan G, Yuhong Z. Dietary patterns and associations between air pollution and gestational diabetes mellitus. Environ Int. 2021;147
Hezaveh ZS, Feizy Z, Dehghani F, Sarbakhsh P, Moini A, Vafa M. The association between maternal dietary protein intake and risk of gestational diabetes mellitus. International journal of. Prev Med. 2019;10(1)
Hu JJ, Oken E, Aris IM, Lin PID, Ma YA, Ding N, et al. Dietary patterns during pregnancy are associated with the risk of gestational diabetes mellitus: evidence from a Chinese prospective birth cohort study. Nutrients. 2019;11(2)
Ikem E, Halldorsson TI, Birgisdóttir BE, Rasmussen MA, Olsen SF, Maslova E. Dietary patterns and the risk of pregnancy-associated hypertension in the Danish National Birth Cohort: a prospective longitudinal study. BJOG: An Int J Obstetr Gynaecol. 2019;126(5):663–73.
Kahr MK, Suter MA, Ballas J, Ramin SM, Monga M, Lee W, et al. Geospatial analysis of food environment demonstrates associations with gestational diabetes. Am J Obstet Gynecol. 2016;214(1):110.e1-.e9.
Lamyian M, Hosseinpour-Niazi S, Mirmiran P, Banaem LM, Goshtasebi A, Azizi F. Pre-pregnancy fast food consumption is associated with gestational diabetes mellitus among tehranian women. Nutrients. 2017;9(3)
Leone A, Martínez-González M, Craig W, Fresán U, Gómez-Donoso C, Bes-Rastrollo M. Pre-gestational consumption of ultra-processed foods and risk of gestational diabetes in a Mediterranean cohort. The SUN project. Nutrients. 2021;13(7)
Lotfi MH, Fallahzadeh H, Rahmanian M, Hosseinzadeh M, Lashkardoost H, Doaei S, et al. Association of food groups intake and physical activity with gestational diabetes mellitus in Iranian women. J Mater -Fetal Neonatal Med. 2020;33(21):3559–64.
Marí-Sanchis A, Díaz-Jurado G, Basterra-Gortari FJ, de la Fuente-Arrillaga C, Martínez-González MA, Bes-Rastrollo M. Association between pre-pregnancy consumption of meat, iron intake, and the risk of gestational diabetes: the SUN project. Eur J Nutr. 2018;57(3):939–49.
Martin CL, Sotres-Alvarez D, Siega-Riz AM. Maternal dietary patterns during the second trimester are associated with preterm birth. J Nutr. 2015;145(8):1857–64.
Nascimento GR, Alves LV, Fonseca CL, Figueiroa JN, Alves JG. Dietary patterns and gestational diabetes mellitus in a low income pregnant women population in Brazil - a cohort study. Archivos Latinoamericanos de Nutricion. 2016;66(4):301–8.
Osorio-Yáñez C, Gelaye B, Qiu C, Bao W, Cardenas A, Enquobahrie DA, et al. Maternal intake of fried foods and risk of gestational diabetes mellitus. Ann Epidemiol. 2017;27(6):384–90.e1.
Rasmussen MA, Maslova E, Halldorsson TI, Olsen SF. Characterization of dietary patterns in the Danish National Birth Cohort in relation to preterm birth. PLoS One. 2014;9(4)
Saftlas AF, Triche EW, Beydoun H, Bracken MB. Does chocolate intake during pregnancy reduce the risks of preeclampsia and gestational hypertension? Ann Epidemiol. 2010;20(8):584–91.
Santos IS, Victora CG, Huttly S, Carvalhal JB. Caffeine intake and low birth weight: a population-based case-control study. Am J Epidemiol. 1998;147(7):620–7.
Sartorelli DS, Crivellenti LC, Zuccolotto DCC, Franco LJ. Relationship between minimally and ultra-processed food intake during pregnancy with obesity and gestational diabetes mellitus. Cadernos de Saude Publica. 2019;35(4)
Schoenaker DA, Soedamah-Muthu SS, Callaway LK, Mishra GD. Pre-pregnancy dietary patterns and risk of gestational diabetes mellitus: results from an Australian population-based prospective cohort study. Diabetol. 2015;58(12):2726–35. https://doi.org/10.1007/s00125-015-3742-1 . Epub 2015 Sep 10.
Sedaghat F, Akhoondan M, Ehteshami M, Aghamohammadi V, Ghanei N, Mirmiran P, Rashidkhani B. Maternal Dietary Patterns and Gestational Diabetes Risk: A Case-Control Study. J Diabetes Res. 2017;2017:5173926. https://doi.org/10.1155/2017/5173926 . Epub 2017 Dec 6.
Tamada H, Ebara T, Matsuki T, Kato S, Sato H, Ito Y, et al. Ready-meal consumption during pregnancy is a risk factor for stillbirth: the Japan environment and Children’s study (JECS). Japan environment and Children’s study, Ready-Meal Consumption During Pregnancy is a Risk Factor for Stillbirth: The Japan Environment and Children’s Study (JECS).
Teixeira JA, Hoffman DJ, Castro TG, Saldiva S, Francisco RPV, Vieira SE, et al. Pre-pregnancy dietary pattern is associated with newborn size: results from ProcriAr study. Br J Nutr. 2021;126(6):903–12.
Triche EW, Grosso LM, Belanger K, Darefsky AS, Benowitz NL, Bracken MB. Chocolate consumption in pregnancy and reduced likelihood of preeclampsia. Epidemiol. 2008;19(3):459–64.
Tryggvadottir EA, Medek H, Birgisdottir BE, Geirsson RT, Gunnarsdottir I. Association between healthy maternal dietary pattern and risk for gestational diabetes mellitus. Eur J Clin Nutr. 2016;70(2):237–42.
Tsoi KY, Chan RSM, Tam CH, Li LS, Tam WH, Ma RCW. Dietary patterns of Chinese pregnant women in Hong Kong. Asia Pac J Clin Nutr. 2022;31(3):378–93.
Wahedy K, El Bilbeisi AHH, Bakry MJ. DIETARY PATTERNS and THEIR ASSOCIATION with GLYCEMIC CONTROL and RISK of GESTATIONAL DIABETES MELLITUS in GAZA STRIP, PALESTINE: a CASE-CONTROL STUDY. Bullet Pharmaceut Sci Assiut. 2021;44(2):537–49.
Wen L, Ge H, Qiao J, Zhang L, Chen X, Kilby MD, et al. Maternal dietary patterns and risk of gestational diabetes mellitus in twin pregnancies: a longitudinal twin pregnancies birth cohort study. Nutr J. 2020;19(1)
Yisahak SF, Hinkle SN, Mumford SL, Gleason JL, Grantz KL, Zhang C, et al. Periconceptional and first trimester Ultraprocessed food intake and maternal Cardiometabolic outcomes. Diabetes Care. 2022;45(9):2028–36.
Yong HY, Mohd Shariff Z, Mohd Yusof BN, Rejali Z, Tee YYS, Bindels J, van der Beek EM. Beverage Intake and the Risk of Gestational Diabetes Mellitus: The SECOST. Nutrients. 2021;13(7):2208. https://doi.org/10.3390/nu13072208 .
Zareei S, Homayounfar R, Naghizadeh MM, Ehrampoush E, Amiri Z, Rahimi M, et al. Dietary pattern in patients with preeclampsia in Fasa. Iran Shiraz E Med J. 2019;20(11)
Zareei S, Homayounfar R, Naghizadeh MM, Ehrampoush E, Rahimi M. Dietary pattern in pregnancy and risk of gestational diabetes mellitus (GDM). Diabet Metabol Syndrome: Clin Res Rev. 2018;12(3):399–404.
Zhang C, Schulze MB, Solomon CG, Hu FB. A prospective study of dietary patterns, meat intake and the risk of gestational diabetes mellitus. Diabetologia. 2006;49(11):2604-13. https://doi.org/10.1007/s00125-006-0422-1 . Epub 2006 Sep 7.
Zuccolotto DCC, Crivellenti LC, Franco LJ, Sarotelli DS. Dietary patterns of pregnant women, maternal excessive body weight and gestational diabetes. Revista de Saude Publica. 2019;53.
Moradi S, Hojjati Kermani MA, Bagheri R, Mohammadi H, Jayedi A, Lane MM, et al. Ultra-processed food consumption and adult diabetes risk: a systematic review and dose-response Meta-analysis. Nutrients. 2021;13(12)
Suksatan W, Moradi S, Naeini F, Bagheri R, Mohammadi H, Talebi S, Mehrabani S, Hojjati Kermani MA, Suzuki K. Ultra-Processed Food Consumption and Adult Mortality Risk: A Systematic Review and Dose-Response Meta-Analysis of 207,291 Participants. Nutrients. 2021;14(1):174. https://doi.org/10.3390/nu14010174 .
Babaei A, Pourmotabbed A, Talebi S, Mehrabani S, Bagheri R, Ghoreishy SM, et al. The association of ultra-processed food consumption with adult inflammatory bowel disease risk: a systematic review and dose-response meta-analysis of 4 035 694 participants. Nutr Rev. 2023;
Umesawa M, Kobashi G. Epidemiology of hypertensive disorders in pregnancy: prevalence, risk factors, predictors and prognosis. Hyperten res: official j Japan Soc Hypert. 2017;40(3):213–20.
Sutan R, Aminuddin NA, Mahdy ZA. Prevalence, maternal characteristics, and birth outcomes of preeclampsia: a cross-sectional study in a single tertiary healthcare center in greater Kuala Lumpur Malaysia. Front Public Health. 2022;10:973271.
Bodnar LM, Ness RB, Markovic N, Roberts JM. The risk of preeclampsia rises with increasing prepregnancy body mass index. Ann Epidemiol. 2005;15(7):475–82.
Catalano PM, McIntyre HD, Cruickshank JK, McCance DR, Dyer AR, Metzger BE, et al. The hyperglycemia and adverse pregnancy outcome study: associations of GDM and obesity with pregnancy outcomes. Diabetes Care. 2012;35(4):780–6.
Perry A, Stephanou A, Rayman MP. Dietary factors that affect the risk of pre-eclampsia. BMJ nutrit prevent health. 2022;5(1):118–33.
Martini D, Godos J, Bonaccio M, Vitaglione P, Grosso G. Ultra-processed foods and nutritional dietary profile: a Meta-analysis of nationally representative samples. Nutrients. 2021;13(10)
Maldonado-Pereira L, Barnaba C, de Los CG, Medina-Meza IG. Evaluation of the nutritional quality of ultra-processed foods (ready to eat + fast food): fatty acids, sugar, and sodium. J Food Sci. 2022;87(8):3659–76.
Monguchi T, Hara T, Hasokawa M, Nakajima H, Mori K, Toh R, et al. Excessive intake of trans fatty acid accelerates atherosclerosis through promoting inflammation and oxidative stress in a mouse model of hyperlipidemia. J Cardiol. 2017;70(2):121–7.
Ma X, Nan F, Liang H, Shu P, Fan X, Song X, et al. Excessive intake of sugar: an accomplice of inflammation. Front Immunol. 2022;13:988481.
Wojcik M, Krawczyk M, Zieleniak A, Mac Marcjanek K, Wozniak LA. Chapter 14 - associations of high blood sugar with oxidative stress and inflammation in patients with type 2 diabetes. In: Preuss HG, Bagchi D, editors. Dietary Sugar Salt and Fat in Human Health. Academic Press; 2020. p. 305–23.
Chapter Google Scholar
Chiarello DI, Abad C, Rojas D, Toledo F, Vázquez CM, Mate A, et al. Oxidative stress: Normal pregnancy versus preeclampsia. Biochim Biophys Acta (BBA)-Mol Basis Dis. 2020;1866(2):165354.
Harmon AC, Cornelius DC, Amaral LM, Faulkner JL, Cunningham MW, Jr., Wallace K, et al. The role of inflammation in the pathology of preeclampsia. Clinical science (London, England : 1979). 2016;130(6):409–19.
Patik JC, Lennon SL, Farquhar WB, Edwards DG. Mechanisms of dietary sodium-induced impairments in Endothelial function and potential countermeasures. Nutrients. 2021;13(1)
Shuto E, Taketani Y, Tanaka R, Harada N, Isshiki M, Sato M, et al. Dietary phosphorus acutely impairs endothelial function. J Am Soc Nephrol: JASN. 2009;20(7):1504–12.
Lopez-Garcia E, Schulze MB, Meigs JB, Manson JE, Rifai N, Stampfer MJ, et al. Consumption of trans fatty acids is related to plasma biomarkers of inflammation and Endothelial Dysfunction1. J Nutr. 2005;135(3):562–6.
Lamarca B. Endothelial dysfunction. An important mediator in the pathophysiology of hypertension during pre-eclampsia. Minerva Ginecol 2012;64(4):309–20.
Fernandes AE, Rosa PWL, Melo ME, Martins RCR, Santin FGO, Moura AMSH, et al. Differences in the gut microbiota of women according to ultra-processed food consumption. Nutr Metab Cardiovasc Dis. 2023;33(1):84–9. https://doi.org/10.1016/j.numecd.2022.09.025 . Epub 2022 Oct 11.
Whelan K, Bancil AS, Lindsay JO, Chassaing B. Ultra-processed foods and food additives in gut health and disease. Nat Rev Gastroenterol Hepatol. 2024;22:1–22.
Fan M, Li X, Gao X, Dong L, Xin G, Chen L, et al. LPS induces preeclampsia-like phenotype in rats and HTR8/SVneo cells dysfunction through TLR4/p38 MAPK pathway. Front Physiol. 2019;10:1030.
Jin J, Gao L, Zou X, Zhang Y, Zheng Z, Zhang X, et al. Gut Dysbiosis promotes preeclampsia by regulating macrophages and trophoblasts. Circ Res. 2022;131(6):492–506.
Kell DB, Kenny LC. A dormant microbial component in the development of preeclampsia. Front Med. 2016;3:60.
Rauber F, Chang K, Vamos EP, da Costa Louzada ML, Monteiro CA, Millett C, et al. Ultra-processed food consumption and risk of obesity: a prospective cohort study of UK biobank. Eur J Nutr. 2021;60(4):2169–80.
Wondmkun YT. Obesity, insulin resistance, and type 2 diabetes: associations and therapeutic implications. Diabet metab syndrome obes: targets therapy. 2020;13:3611–6.
Teran E, Escudero C, Moya W, Flores M, Vallance P, Lopez-Jaramillo P. Elevated C-reactive protein and pro-inflammatory cytokines in Andean women with pre-eclampsia. Int J Gynecol Obstet. 2001;75(3):243–9.
Palei AC, Spradley FT, Granger JP. Chronic hyperleptinemia results in the development of hypertension in pregnant rats. Am J Phys Regul Integr Comp Phys. 2015;308(10):R855–R61.
CAS Google Scholar
Olson KN, Redman LM, Sones JL. Obesity "complements" preeclampsia. Physiol Genom. 2019;51(3):73–6.
Wang X, Cheng Z. Cross-sectional studies: strengths, weaknesses, and recommendations. Chest. 2020;158(1 Supplement):S65–71.
Mozaffarian D, Ludwig DS. Dietary guidelines in the 21st century—a time for food. Jama. 2010;304(6):681–2.
Wang H, Li N, Chivese T, Werfalli M, Sun H, Yuen L, et al. IDF diabetes atlas: estimation of global and regional gestational diabetes mellitus prevalence for 2021 by International Association of Diabetes in pregnancy study Group's criteria. Diabetes Res Clin Pract. 2022;183:109050.
Kityo A, Lee SA. The intake of ultra-processed foods and prevalence of chronic kidney disease: the health examinees study. Nutrients. 2022;14(17)
Nardocci M, Leclerc B-S, Louzada M-L, Monteiro CA, Batal M, Moubarac J-C. Consumption of ultra-processed foods and obesity in Canada. Can J Public Health. 2019;110:4–14.
Rauber F, Louzada MLC, Steele EM, Millett C, Monteiro CA, Levy RB. Ultra-processed food consumption and chronic non-communicable diseases-related dietary nutrient profile in the UK (2008–2014). Nutrients. 2018;10(5):587.
Lavigne-Robichaud M, Moubarac J-C, Lantagne-Lopez S, Johnson-Down L, Batal M, Sidi EAL, et al. Diet quality indices in relation to metabolic syndrome in an indigenous Cree (Eeyouch) population in northern Québec. Canada Pub health nutrit. 2018;21(1):172–80.
Sun Y, Shen Z, Zhan Y, Wang Y, Ma S, Zhang S, et al. Effects of pre-pregnancy body mass index and gestational weight gain on maternal and infant complications. BMC pregnan childbirth. 2020;20(1):1–13.
Linder T, Eder A, Monod C, Rosicky I, Eppel D, Redling K, et al. Impact of prepregnancy overweight and obesity on treatment modality and pregnancy outcome in women with gestational diabetes mellitus. Front Endocrinol. 2022;13:799625.
Najafi F, Hasani J, Izadi N, Hashemi-Nazari S-S, Namvar Z, Shamsi H, et al. Risk of gestational diabetes mellitus by pre-pregnancy body mass index: a systematic review and meta-analysis. Diabet Metab Syndrome: Clin Res Rev. 2021;15(4):102181.
McIntyre HD, Catalano P, Zhang C, Desoye G, Mathiesen ER, Damm P. Gestational diabetes mellitus. Nat Rev Dis Primers. 2019;5(1):47.
Plows JF, Stanley JL, Baker PN, Reynolds CM, Vickers MH. The pathophysiology of gestational diabetes mellitus. Int J Mol Sci. 2018;19(11):3342.
Stanhope KL. Sugar consumption, metabolic disease and obesity: the state of the controversy. Crit Rev Clin Lab Sci. 2016;53(1):52–67.
Bhattacharyya S, I OS, Katyal S, Unterman T, Tobacman JK. Exposure to the common food additive carrageenan leads to glucose intolerance, insulin resistance and inhibition of insulin signalling in HepG2 cells and C57BL/6J mice. Diabetologia. 2012;55(1):194–203.
Quetglas-Llabrés MM, Monserrat-Mesquida M, Bouzas C, Mateos D, Ugarriza L, Gómez C, et al. Oxidative stress and inflammatory biomarkers are related to high intake of ultra-processed food in old adults with metabolic syndrome. Antioxidants (Basel, Switzerland). 2023;12(8).
Yaribeygi H, Sathyapalan T, Atkin SL, Sahebkar A. Molecular mechanisms linking oxidative stress and diabetes mellitus. Oxidative Med Cell Longev. 2020;2020:8609213.
Bertoli S, Leone A, Battezzati A. Human bisphenol a exposure and the “diabesity phenotype”. Dose-Response. 2015;13(3):1559325815599173.
Rolfo A, Nuzzo AM, De Amicis R, Moretti L, Bertoli S, Leone A. Fetal–maternal exposure to endocrine disruptors: correlation with diet intake and pregnancy outcomes. Nutrients. 2020;12(6):1744.
Lorenzo PI, Martín-Montalvo A, Cobo Vuilleumier N, Gauthier BR. Molecular modelling of islet β-cell adaptation to inflammation in pregnancy and gestational diabetes mellitus. Int J Mol Sci. 2019;20(24)
Christ A, Lauterbach M, Latz E. Western diet and the immune system: an inflammatory connection. Immunity. 2019;51(5):794–811.
Neale E, Batterham M, Tapsell LC. Consumption of a healthy dietary pattern results in significant reductions in C-reactive protein levels in adults: a meta-analysis. Nutr Res. 2016;36(5):391–401.
Liu Z, Lu Y, Zhong K, Wang C, Xu X. The associations between endocrine disrupting chemicals and markers of inflammation and immune responses: a systematic review and meta-analysis. Ecotoxicol Environ Saf. 2022;234:113382.
Node K, Inoue T. Postprandial hyperglycemia as an etiological factor in vascular failure. Cardiovasc Diabetol. 2009;8:23.
Hu Z, Tylavsky FA, Kocak M, Fowke JH, Han JC, Davis RL, et al. Effects of maternal dietary patterns during pregnancy on early childhood growth trajectories and obesity risk: the CANDLE study. Nutrients. 2020;12(2)
Wang Y, Wang K, Du M, Khandpur N, Rossato SL, Lo C-H, et al. Maternal consumption of ultra-processed foods and subsequent risk of offspring overweight or obesity: results from three prospective cohort studies. Bmj. 2022:379.
Paula WO, Gonçalves VSS, Patriota ESO, Franceschini SCC, Pizato N. Impact of ultra-processed food consumption on quality of diet among Brazilian pregnant women assisted in primary health care. Int J Environ Res Public Health. 2023;20(2)
Crume TL, Brinton JT, Shapiro A, Kaar J, Glueck DH, Siega-Riz AM, et al. Maternal dietary intake during pregnancy and offspring body composition: the healthy start study. Am J Obstet Gynecol. 2016;215(5):609–e1-e8.
Article PubMed Central Google Scholar
Wan Y, Chen Y, Wu X, Yin A, Tian F, Zhang H, et al. Mediation effect of maternal triglyceride and fasting glucose level on the relationship between maternal overweight/ obesity and fetal growth: a prospective cohort study. BMC Pregnancy and Childbirth. 2023;23(1):449.
Akbari Z, Mansourian M, Kelishadi R. Relationship of the intake of different food groups by pregnant mothers with the birth weight and gestational age: need for public and individual educational programs. J educ health promot. 2015;4:23.
Fischer S, Morales-Suárez-Varela M. The bidirectional relationship between gestational diabetes and depression in pregnant women: a systematic search and review. Healthcare (Basel, Switzerland). 2023;11(3).
Khan R, Waqas A, Bilal A, Mustehsan ZH, Omar J, Rahman A. Association of Maternal depression with diet: a systematic review. Asian J Psychiatr. 2020;52:102098.
Ancira-Moreno M, O'Neill MS, Rivera-Dommarco J, Batis C, Rodríguez Ramírez S, Sánchez BN, et al. Dietary patterns and diet quality during pregnancy and low birthweight: the PRINCESA cohort. Matern Child Nutr. 2020;16(3):e12972.
Download references
Acknowledgments
Author information, authors and affiliations.
Students’ Scientific Research Center (SSRC), Tehran University of Medical Sciences, Tehran, Iran
Sepide Talebi
Department of Clinical Nutrition, School of Nutritional Sciences and Dietetics, Tehran University of Medical Sciences, Tehran, Iran
Food Security Research Center, Isfahan University of Medical Sciences, Isfahan, Iran
Sanaz Mehrabani
Department of Nutrition, School of Public Health, Iran University of Medical Sciences, Tehran, Iran
Seyed Mojtaba Ghoreishy
Student research committee, School of Public Health, Iran University of Medical Sciences, Tehran, Iran
Department of Health and Human Performance, Marymount University, Arlington, VA, USA
Alexei Wong
Department of Clinical Sciences, Faculty of Veterinary Medicine, Razi University, Kermanshah, Iran
Aliasghar Moghaddam & Peyman Rahimi Feyli
General Practitioner, Kermanshah University of Medical Sciences (KUMS), Kermanshah, Iran
Parsa Amirian & Mahsa Zarpoosh
Clinical Tuberculosis and Epidemiology Research Center, National Research Institute of Tuberculosis and Lung Diseases (NRITLD), Masih Daneshvari Hospital, Shahid Beheshti University of Medical Sciences, Tehran, Iran
Mohammad Ali Hojjati Kermani
Department of Nutrition and Food Sciences, Research Center for Evidence-Based Health Management, Maragheh University of Medical Sciences, Maragheh, Iran
Sajjad Moradi
You can also search for this author in PubMed Google Scholar
Contributions
SM and ST designed this study. SM, MZ and PA contributed to the conduct of the search. SM and ST performed the statistical analysis and interpreted the results. SM, SM-GH, Sanaz Merabani, and MA-HK wrote the initial manuscript. AW, AM and PR_F critically revised the manuscript and contributed to the subsequent drafts of the manuscript. All authors approved the final version of the manuscript.
Corresponding author
Correspondence to Sajjad Moradi .
Ethics declarations
Ethics approval and consent to participate.
This article does not contain any studies with human participants or animals performed by any of the authors.
Consent for publication
Not Applicable.
Competing interests
The authors declare no competing interests.
Additional information
Publisher’s note.
Springer Nature remains neutral with regard to jurisdictional claims in published maps and institutional affiliations.
Supplementary Information
Supplementary material 1., supplementary material 2., supplementary material 3., supplementary material 4., supplementary material 5., supplementary material 6., supplementary material 7., supplementary material 8., rights and permissions.
Open Access This article is licensed under a Creative Commons Attribution 4.0 International License, which permits use, sharing, adaptation, distribution and reproduction in any medium or format, as long as you give appropriate credit to the original author(s) and the source, provide a link to the Creative Commons licence, and indicate if changes were made. The images or other third party material in this article are included in the article's Creative Commons licence, unless indicated otherwise in a credit line to the material. If material is not included in the article's Creative Commons licence and your intended use is not permitted by statutory regulation or exceeds the permitted use, you will need to obtain permission directly from the copyright holder. To view a copy of this licence, visit http://creativecommons.org/licenses/by/4.0/ . The Creative Commons Public Domain Dedication waiver ( http://creativecommons.org/publicdomain/zero/1.0/ ) applies to the data made available in this article, unless otherwise stated in a credit line to the data.
Reprints and permissions
About this article
Cite this article.
Talebi, S., Mehrabani, S., Ghoreishy, S.M. et al. The association between ultra-processed food and common pregnancy adverse outcomes: a dose-response systematic review and meta-analysis. BMC Pregnancy Childbirth 24 , 369 (2024). https://doi.org/10.1186/s12884-024-06489-w
Download citation
Received : 18 January 2024
Accepted : 07 April 2024
Published : 15 May 2024
DOI : https://doi.org/10.1186/s12884-024-06489-w
Share this article
Anyone you share the following link with will be able to read this content:
Sorry, a shareable link is not currently available for this article.
Provided by the Springer Nature SharedIt content-sharing initiative
- Ultra-processed foods
- Gestational diabetes mellitus
- Preeclampsia
- Preterm birth
BMC Pregnancy and Childbirth
ISSN: 1471-2393
- Submission enquiries: [email protected]
- General enquiries: [email protected]
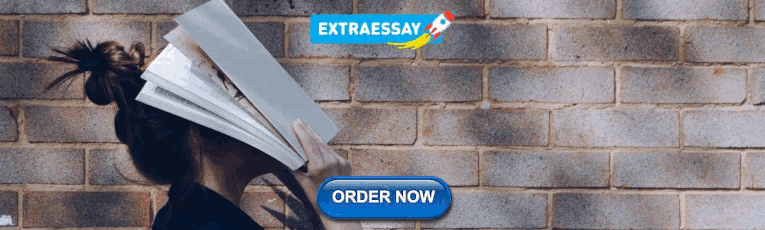
COMMENTS
Observational study example. Observational studies are usually quite straightforward to design and conduct. Sometimes all you need is a notebook and pen! As you design your study, you can follow these steps. Step 1: Identify your research topic and objectives. The first step is to determine what you're interested in observing and why.
Observational studies, including online surveys, describe the existing phenomena at a larger scale, often involving various control groups. Observational studies include variable-scale epidemiological investigations at different time points. ... An example of this is the hygiene hypothesis proposing an inverse relationship between infections in ...
Observational Study Definition. In an observational study, the researchers only observe the subjects and do not interfere or try to influence the outcomes. In other words, the researchers do not control the treatments or assign subjects to experimental groups. Instead, they observe and measure variables of interest and look for relationships ...
A hypothesis is a tentative statement about the relationship between two or more variables. It is a specific, testable prediction about what you expect to happen in a study. It is a preliminary answer to your question that helps guide the research process. Consider a study designed to examine the relationship between sleep deprivation and test ...
The observational design is subdivided into descriptive, including cross-sectional, case report or case series, and correlational, and analytic which includes cross-section, case-control, and cohort studies. Each research design has its uses and points of strength and limitations. The aim of this article to provide a simplified approach for the ...
3. Simple hypothesis. A simple hypothesis is a statement made to reflect the relation between exactly two variables. One independent and one dependent. Consider the example, "Smoking is a prominent cause of lung cancer." The dependent variable, lung cancer, is dependent on the independent variable, smoking. 4.
Introduction. Observational studies involve the study of participants without any forced change to their circumstances, that is, without any intervention.1 Although the participants' behaviour may change under observation, the intent of observational studies is to investigate the 'natural' state of risk factors, diseases or outcomes. For drug therapy, a group of people taking the drug ...
r reporting and interpretation of observational studies. We review statistical principles that are fundamental to understanding what observational data can offer. The concepts include the relationship between a study sample and the target population, and the two primary forms of statistical analysis: estimation and hypothesis testing. The concept of bias, and confounding in particular, is ...
Observational studies can be classified into descriptive studies, which are usually undertaken when little is known of the epidemiology of a disease, and analytic studies, which are carried out when leads about etiology are already available. ... Analytic Studies. Analytic studies (hypothesis testing studies) are designed specifically to test ...
A research hypothesis is a statement that proposes a possible explanation for an observable phenomenon or pattern. It guides the direction of a study and predicts the outcome of the investigation. A research hypothesis is testable, i.e., it can be supported or disproven through experimentation or observation. Characteristics of a good hypothesis
Study Protocol. Randomized Experiment: Before the experiment starts, a protocol describes the design, outcomes, type of analysis, etc. Better Observational Study: Before the analysis of the data starts, a protocol describes the design, outcomes, type of analysis, etc. Poorer Observational Study: If we run many regressions, something publishable ...
Actually, the term is "Sample Survey" and you may search online for it. I think the difference lies in the aim of the three types of studies, sample surveys want to get data for a parameter while observational studies and experiments want to convert some data into information, i.e., correlation and causation respectively.
One common observational study is about the possible effect of a treatment on subjects, where the assignment of subjects into a treated group versus a control group is outside the control of the investigator. [1] [2] This is in contrast with experiments, such as randomized controlled trials, where each subject is randomly assigned to a treated ...
There are seven types of observational studies. Researchers might choose to use one type of observational study or combine any of these multiple observational study approaches: 1. Cross-sectional studies. Cross-sectional studies happen when researchers observe their chosen subject at one particular point in time.
For example, studying the long-term effects of smoking requires an observational approach because we can't ethically assign people to smoke or abstain from smoking. Cost-Effective: Observational studies are generally less expensive and time-consuming than experiments. Longitudinal Research: They are well-suited for long-term studies or those ...
Cohort studies and case-control studies are two primary types of observational studies that aid in evaluating associations between diseases and exposures. In this review article, we describe these study designs, methodological issues, and provide examples from the plastic surgery literature. Keywords: observational studies, case-control study ...
The scientific method does include observation. The steps of the scientific method include: Observation. Question. Hypothesis. Experiment. Conclusion. These steps can be modified to include other ...
Learn the observational study definition in statistics and understand how an observational study takes place. See observational study examples to learn how it works. Updated: 11/21/2023
Figure 4.15: Interaction plot array of prodebt by income group (1 to 5) and whether they buy cigarettes (0 = no, 1 = yes).. As in other situations, and especially with observational studies where a single large sample is collected and then the levels of the factor variables are observed, it is important to check for balance - whether all the combinations of the two predictor variables are ...
Examine experimental study's definition and see examples of observational studies vs experiments. Updated: 11/21/2023 ... Hypothesis Testing. Ch 11. Studying for Statistics 101.
Interpretation of observational studies. The aim of this paper is to review the most important methodological strengths and limitations of observational studies of humans, as opposed to experimental studies. The names "observational" and "experimental" go a long way in describing the differences. In an experimental study—that is, a ...
Objectives Given the increasing incidence of negative outcomes during pregnancy, our research team conducted a dose-response systematic review and meta-analysis to investigate the relationship between ultra-processed foods (UPFs) consumption and common adverse pregnancy outcomes including gestational diabetes mellitus (GDM), preeclampsia (PE), preterm birth (PTB), low birth weight (LBW), and ...
Explore the details of a Observational Research Senior Manager career in Washington D.C.. Live. Win. Thrive. Search and apply for a rewarding new career at Amgen. ... For example, build a study to evaluate the safety and effectiveness of an Amgen medicine to inform a regulatory decision (e.g., label expansion/change) and fulfill a global or ...