- Research article
- Open access
- Published: 16 March 2013
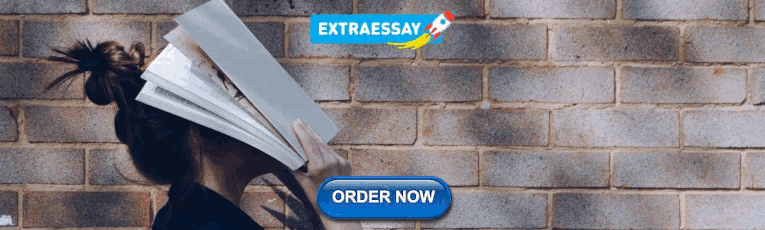
Overview of data-synthesis in systematic reviews of studies on outcome prediction models
- Tobias van den Berg 1 ,
- Martijn W Heymans 1 ,
- Stephanie S Leone 2 ,
- David Vergouw 2 ,
- Jill A Hayden 3 ,
- Arianne P Verhagen 4 &
- Henrica CW de Vet 1
BMC Medical Research Methodology volume 13 , Article number: 42 ( 2013 ) Cite this article
41k Accesses
16 Citations
1 Altmetric
Metrics details
Many prognostic models have been developed. Different types of models, i.e. prognostic factor and outcome prediction studies, serve different purposes, which should be reflected in how the results are summarized in reviews. Therefore we set out to investigate how authors of reviews synthesize and report the results of primary outcome prediction studies.
Outcome prediction reviews published in MEDLINE between October 2005 and March 2011 were eligible and 127 Systematic reviews with the aim to summarize outcome prediction studies written in English were identified for inclusion.
Characteristics of the reviews and the primary studies that were included were independently assessed by 2 review authors, using standardized forms.
After consensus meetings a total of 50 systematic reviews that met the inclusion criteria were included. The type of primary studies included (prognostic factor or outcome prediction) was unclear in two-thirds of the reviews. A minority of the reviews reported univariable or multivariable point estimates and measures of dispersion from the primary studies. Moreover, the variables considered for outcome prediction model development were often not reported, or were unclear. In most reviews there was no information about model performance. Quantitative analysis was performed in 10 reviews, and 49 reviews assessed the primary studies qualitatively. In both analyses types a range of different methods was used to present the results of the outcome prediction studies.
Conclusions
Different methods are applied to synthesize primary study results but quantitative analysis is rarely performed. The description of its objectives and of the primary studies is suboptimal and performance parameters of the outcome prediction models are rarely mentioned. The poor reporting and the wide variety of data synthesis strategies are prone to influence the conclusions of outcome prediction reviews. Therefore, there is much room for improvement in reviews of outcome prediction studies.
Peer Review reports
The methodology for prognosis research is still under development. Although there is abundant literature to help researchers perform this type of research [ 1 – 5 ], there is still no widely agreed approach to building a multivariable prediction model [ 6 ]. An important distinction in prognosis is made between prognostic factor models, also called explanatory models and outcome prediction models [ 7 , 8 ]. Prognostic factor studies investigate causal relationships, or pathways between a single (prognostic) factor and an outcome, and focus on the effect size (e.g. relative risk) of this prognostic factor which ideally is adjusted for potential confounders. Outcome prediction studies, on the other hand, combine multiple factors (e.g. clinical and non-clinical patient characteristics) in order to predict future events in individuals, and therefore focus on absolute risks, i.e. predicted probabilities in logistic regression analysis. Methods that can be used to summarize data from prognostic factor studies in a meta-analysis can easily be found in the literature [ 9 , 10 ], but this is not the case for outcome prediction studies. Therefore, in the present study we focus on how authors of published reviews have synthesized outcome prediction models. The nomenclature to indicate various types of prognosis research is not standardized. We use prognosis research as an umbrella term for all research that might explain or predict a future outcome and prognostic factor and outcome prediction as specific types of prognosis studies.
In 2006, Hayden et al. showed that in systematic reviews of prognosis studies, different methods are used to assess the quality of primary studies [ 11 ]. Moreover, when quality is assessed, integration of these quality scores in the synthesis of the review is not guaranteed. For reviews of outcome prediction models, additional characteristics are important in the synthesis of models to reflect choices made in the primary studies, such as which variables are included in statistical models and how this selection was made. These choices therefore also reflect the internal and external validity of a model and influence the predictive performance of the model. In systematic reviews the researchers synthesize results across primary outcome prediction studies which include different variables and show methodological diversity. Moreover, relevant information is not always available, due to poor reporting in the studies. For example, several researchers have found that current knowledge about the recommended number of events per variable, and the coding and selection of variables, among other features, are not always reported in primary outcome prediction research [ 12 – 14 ]. Although improvement in primary studies themselves is needed, reviews that summarize outcome prediction evidence need to consider the current diversity in methodology in primary studies.
In this meta-review we focus on reviews of outcome prediction studies, and how they summarize the characteristics of design and analysis, and the results of primary studies. As there is no guideline nor agreement how primary outcome prediction models in medical research and epidemiology should be summarized in systematic reviews, an overview of current methods helps researchers to improve and develop these methods. Moreover, current methods for outcome prediction reviews are unknown to the research community. Therefore, the aim of this review was to provide an overview on how published reviews of outcome prediction studies describe and summarize the characteristics of the analyses in primary studies, and how the data is synthesized.
Literature search and selection of studies
Systematic reviews and meta-analyses of outcome prediction models that were published between October 2005 and March 2011 were searched. We were only interested in reviews that included multivariable outcome prediction studies. In collaboration with a medical information specialist, we developed a search strategy in MEDLINE, extending on the strategy used by Hayden [ 11 ], by adding recommended other search terms for predictive and prognostic research [ 15 , 16 ]. The full search strategy is presented in Appendix 1.
Based on title and abstract, potential eligible reviews were selected by one author (TvdB), who in case of any doubt included the review. Another author (MH) checked the set of potential eligible reviews. Ineligible reviews were excluded after consensus between both authors. The full texts of the included reviews were read, and if there was any doubt on eligibility a third review author (HdV) was consulted. The inclusion criteria were met if the study design was a systematic review with or without a meta-analysis, multiple variables were studied in an outcome prediction model, and the review was written in the English language. Reviews were excluded if they were based on individual patient data only, or when the topic was genetic profiling.
Data-extraction
A data-extraction form was developed, based on important items to prognosis [ 1 , 2 , 12 , 13 , 17 ], to assess the characteristics of reviews and primary studies and is available from the first author on request. The items on this data-extraction form are shown in Appendix 2. Before the form was finalized it was pilot-tested by all review authors and minor adjustments were made after discussion about the differences in scores. One review author scored all reviews (TvdB) while other review authors (MH, AV, DV, and SL) collectively scored all reviews. Consensus meetings were held within 2 weeks after a review had been scored to solve disagreements. If consensus was not reached, a third reviewer (MH or HdV) was consulted to make a final decision.
An item was scored ‘yes’ if positive information was found about that specific methodological item, e.g. if it was clear that sensitivity analyses were conducted. If it was clear that a specific methodological requirement was not fulfilled, a ‘no’ was scored, e.g. no sensitivity analyses were conducted. In case of doubt or uncertainty, ‘unclear’ was scored. Sometimes, a methodological item could be scored as ‘not applicable’. The number of reviews within a specific answer category was reported, as well as the proportion.
Literature search and selection process
The search strategy revealed 7889 references and, based on title and abstract, 216 were selected to be read in full text (see the flowchart in Figure 1 ). Of these reviews, 89 were excluded and 127 remained. Exclusions after the full text had been read were mainly due to the focus of the research on a single variable with an outcome (prognostic factor study), analysis based on individual patient data only, or a narrative overview study design. After completing the data-extraction, the objectives and methods of 44 reviews described summaries of prognostic factor studies, and 33 reviews had an unclear approach. Therefore, a total of 50 reviews on outcome prediction studies were analyzed [ 18 – 67 ].
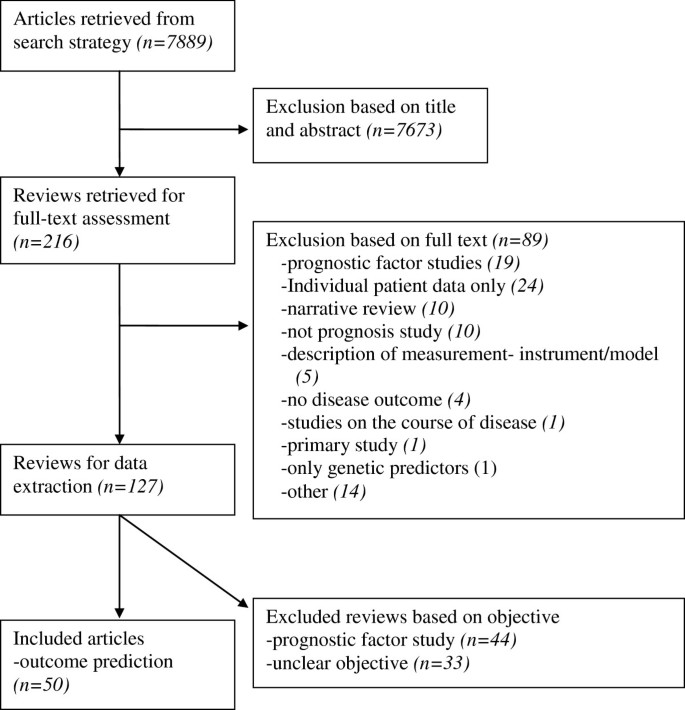
Flowchart of the search and selection process.
After completing the data-extraction form for all of the included reviews, most disagreements between review authors were found on items concerning the review objectives, the type of primary studies included, and the method of qualitative data-synthesis. Unclear reporting and, to a lesser degree, reading errors contributed to the disagreements. After consensus meetings only a small proportion of items needed to be discussed with a third reviewer.
Objective and design of the review
Table 1 , section 1 shows the items with regard to information about the reviews. Of the 50 reviews rated as summaries of outcome prediction studies, less than one third included only outcome prediction studies *[ 23 , 27 , 28 , 32 , 35 , 39 , 44 , 48 ],[ 50 , 52 , 55 , 58 , 60 , 66 ]. In about two thirds, the type of primary studies that were included was unclear, and the remaining reviews included a combination of prognostic factor and outcome prediction studies. Most reviews clearly described their outcome of interest. Also information about the assessment of the methodological quality of the primary studies, i.e. risk of bias, was provided in most reviews. In those that did, two thirds described the basic design of the primary studies in addition to a list of methodological criteria (defined in our study as a list consisting of at least four quality items). In some reviews an established criteria list was used or adapted, or a new criteria list was developed. In the reviews that assessed methodological quality, less than half actually used this information to account for differences in study quality, mainly by performing a ‘levels of evidence’ analysis, subgroup-analyses, or sensitivity analyses.
Information about the design and results of the primary studies
In Table 1 , section 2 shows information provided about the included primary studies. The outcome measures used in the included studies were reported in most of the reviews. Only 2 reviews [ 28 , 52 ] described the statistical methods that were used in the primary studies to select variables for inclusion of a final prediction model, e.g. forward or backward selection procedures, and 6 others whether and how patients were treated.
A minority of reviews [ 23 , 24 , 27 , 28 ] described for all studies the variables that were considered for inclusion in the outcome prediction model and only 5 reviews [ 36 , 37 , 39 , 48 , 55 ] reported univariable point estimates (i.e.. regression coefficients or odds ratios) and estimates of dispersion (e.g. standard errors) of all studies. Similarly, multivariable point estimates and estimates of dispersion were reported in respectively 11 and 10 of the reviews [ 21 , 26 , 27 , 31 , 33 , 37 , 44 , 52 ],[ 55 , 64 , 65 ].
With regard to the presentation of univariable and multivariable point estimates, 2 reviews presented both types of results [ 37 , 55 ], 31 did not report any estimates, and 17 reviews were unclear or reported only univariable or multivariable results [not shown in the table]. Lastly, model performance and number of events per variable were reported in 7 reviews [ 32 , 39 , 41 , 60 , 61 , 65 , 66 ] and 4 reviews [ 40 , 48 , 58 , 61 ], respectively.
Data-analysis and synthesis in the reviews
Table 1 , section 3 illustrates how the results of primary studies were summarized in the reviews. It shows that heterogeneity was described in almost all reviews by reporting differences in the study design and the characteristics of the study population. All but one review [ 57 ] summarized the results of included studies in a qualitative manner. Methods that were mainly used for that purpose were number of statistical significant results, consistency of findings, or a combination of these. Quantitative analysis, i.e. statistical pooling, was performed in 10 of the 50 reviews [ 25 , 28 , 31 , 36 , 37 , 44 , 45 , 57 – 59 ]. The quantitative methods used included random effects models and fixed effects models of regression coefficients, odds ratios or hazard ratios. Of these quantitative summaries, 40% assessed the presence of statistical heterogeneity using I 2 , Chi 2 , or the Q statistic. In two reviews [ 25 , 59 ], statistical heterogeneity was found to be present, and subgroup analysis was performed to determine the source of this heterogeneity [results not shown]. In 8 of the reviews there was a graphical presentation of the results, in which a forest plot [ 25 , 28 , 36 – 38 , 52 , 59 ], per single predictor, was the frequently used method. Other studies used a barplot [ 57 ] or a scatterplot [ 38 ]. In 6 reviews [ 25 , 26 , 32 , 43 , 46 , 58 ] a sensitivity analysis was performed to test the robustness of the choices made such as changing the cut-off value for a high or low quality primary study.
We made an overview of how systematic reviews summarize and report the results of primary outcome prediction studies. Specifically, we extracted information on how the data-synthesis was performed in reviews since outcome prediction models may consider different potential predictors, and include a dissimilar set of variables in the final prediction model, and use a variety of statistical methods to obtain an outcome prediction model.
Currently, in prognosis studies a distinction is made between outcome prediction models and prognostic factor models. The methodology of data synthesis in a review of the latter type of prognosis is comparable to the methodology of aetiological reviews. For that reason, in the present study we only focused on reviews of outcome prediction studies. Nonetheless, we found it difficult to distinct between both review types. Less than half of the reviews that we initially selected for data-extraction in fact seemed to serve an outcome prediction purpose. It appeared that the other reviews summarized prognostic factor studies only, or the objective was unclear. In particular, prognostic factor reviews that investigated more than one variable in addition to non-specific objectives made it difficult to clarify what the purpose of reviews was. As a consequence, we might have misclassified some of the 44 excluded reviews rated as prognostic factor. The objective of a review should also include information about the type of study that is included, that is of outcome prediction studies in this case. However, we found that in reviews aimed at outcome prediction the type of primary study was unclear for two-thirds of the reviews. An example we encountered in a review was that their purpose was “to identify preoperative predictive factors for acute post-operative pain and analgesic consumption” although the review authors included any study that identified one or more potential risk factors or predictive factors. The risk of combining both types of studies, i.e. risk factor or prognostic factor studies and predictive factor studies, is that inclusion of potential covariables in the former type are based on change in regression coefficient of the risk factor while in the latter study type all potential predictor variables are included based on their predictive ability of the outcome. This distinction may lead to: 1) biased results in a meta-analysis or other form of evidence synthesis because a risk factor is not always predictive for an outcome and 2) risk factor studies – if adjusted for potential confounders at all – have a slightly different method to obtain a multivariable model compared to outcome prediction studies which may also lead to biased regression coefficients. The distinction between prognostic factor and outcome prediction studies was already emphasized in 1983 by Copas [ 68 ]. He stated that “a method for achieving a good predictor may be quite inappropriate for other questions in regression analysis such as the interpretation of individual regression coefficients”. In other words, the methodology of outcome prediction modelling differs from that of prognostic factor modelling, and therefore combining both types of research into one review to reflect current evidence should be discouraged. Hemingway et al. [ 2 ] appealed for standard nomenclature in prognosis research, and the results of our study underline their plea. Authors of reviews and primary studies should clarify their type of research, for example by using the terms applied by Hayden et al. [ 8 ] ‘prognostic factor modelling’ and ‘outcome prediction modelling’, and give a clear description of their objective.
Studies included in outcome prediction reviews are rarely similar in design and methodology, and this is often neglected when summarizing the evidence. Differences, for instance in the variables studied and the method of analysis for variable selection might explain heterogeneity in results, and should therefore be reported and reflected on when striving to summarize evidence in the most appropriate way. There is no doubt that the methodological quality of primary studies included in reviews is related to the concept of bias [ 69 , 70 ] and it is therefore important to assess this [ 11 , 69 , 70 ]. Dissemination bias reflects if publication bias is likely to be present, how this is handled and what is done to correct for it [ 71 ]. To our knowledge, dissemination bias and especially its consequences in reviews of outcome prediction models are not studied yet. Most likely testimation bias [ 5 ], i.e. the predictors considered and the amount of predictors in relation to the effective sample size influence results more then publication bias. Therefore, we did not study dissemination bias on the review level.
With regard to the reporting of primary study characteristics in the systematic reviews, there is much room for improvement. We found that the methods of model development (e.g. the variables considered and the variable selection methods used) in the primary studies were not, or only vaguely reported in the included reviews. These methods are however important, because variable selection procedures can affect the composition of the multivariable model due to estimation bias, or may result in an increase in model uncertainty [ 72 – 74 ]. Furthermore, the predictive performance of the model can be biased by these methods [ 74 ]. We also found that only 5 of the reviews reported what kind of treatment the patients received in the primary studies. Although prescribed treatment is often not considered as a candidate predictor, it is likely to have a considerable impact on prognosis. Moreover, treatment may vary in relation to predictive variables [ 75 ], and although randomized controlled trials provide patients with similar treatment strategies, in cohort studies which are most often seen in prognosis research this is often not the case. Regardless of difficulties in defining groups that receive the same treatment, it is imperative to consider treatment in outcome prediction models. In order to ensure correct data-synthesis of the results, the primary studies not only should provide point estimates and estimates of dispersion of all the included variables, but also for non-significant findings. Whereas the results of positive or favourable findings are more often reported [ 75 – 78 ], the effects of predictive factors that do not reach statistical significance also need to be compared and summarized in a review. Imagine a variable being of statistical significance in one article, but not reported in others because of non-significance. It is likely that this one significant result is a spurious finding or that the others were underpowered. Without information about the non-significant findings in other studies, biased or even incorrect conclusions might be drawn. This means that reporting of the evidence of primary studies should be accompanied by the results of univariable and multivariable associations, regardless of their level of significance. Moreover, confidence intervals, or other estimates of dispersion are also needed in the review, and unfortunately these results were not presented in most of the reviews in our study. Some reviews considered differences in unadjusted and adjusted results, and the results of one review were sensibly stratified according to univariable and multivariable effects [ 38 ]. Other reviews merely reported multivariable results [ 31 ], or only univariable results if multivariable results were unavailable [ 58 ]. In addition to the multivariable results of a final prediction model, the predictive performance of these models is important for the assessment of clinical usefulness [ 79 ]. A prediction model in itself does not indicate how much variance in outcome is explained by the included variables. Unfortunately, in addition to the non-reporting of several primary study characteristics, the performance of the models was rarely reported in the reviews included in our overview.
Different stages can be distinguished in outcome prediction research [ 80 ]. Most outcome prediction models evaluated in the systematic reviews appeared to be in a developmental phase. Before implementation in daily practice, confirmation of the results in other studies is needed. With this type of validation studies underway, in future reviews we should acknowledge the difference between externally validated models and models from developmental studies, and analyze them separately.
In systematic reviews data can be combined quantitatively, i.e. a meta-analysis can be performed. This was done in 10 of the reviews. All of them combined point estimates (mostly odds ratios, but also a mix of odds ratios, hazard ratios and relative risks) and confidence intervals for single outcome prediction variables. This made it possible to calculate a pooled point estimate, often complemented with confidence intervals [ 81 ]. However, in outcome prediction research we are interested in the estimates of a combination of predictive factors, which makes it possible to calculate absolute risks or probabilities to predict an outcome in individuals [ 82 ]. Even if the relative risk of a variable is statistically significant, it does not provide information about the extent to which this variable is predictive for a particular outcome. The distribution of predictor values, outcome prevalence, and correlations between variables also influences the predictive value of variables within a model [ 83 ]. Effect sizes also provide no information about the amount of variation in outcomes that is explained. In summary: the current quantitative methods seem to be more of an explanatory way of summarizing the available evidence, instead of quantitatively summarizing complete outcome prediction models.
Medline was the only database that was searched for relevant reviews. Our intention was to provide an overview of recently published reviews and not to include all relevant outcome prediction reviews. Within Medline, some eligible reviews may have been missed if their titles and abstracts did not include relevant terms and information. An extensive search strategy was applied and abstracts were screened thoroughly and discussed in case of disagreement. Data-extraction was performed in pairs to prevent reading and interpretation errors. Disagreements mainly occurred when deciding on the objective of a review and the type of primary studies included, due to poor reporting in most of the reviews. This indicates a lack of clarity, explanation and reporting within reviews. Therefore, screening in pairs is a necessity, and standardized criteria should be developed and applied in future studies focusing on such reviews. Consistency in rating on the data-extraction form was enhanced by one review author rating all reviews, with one of the other review authors as second rater. Several items were scored as “no”, but we did not know whether this was a true negative (i.e. leading to bias) or that no information was reported about a particular item. For review authors it is especially difficult to summarize information about primary studies because there may be a lack of information in the studies [ 13 , 14 , 84 ].
Implications
There is still no available methodological procedure for a meta-analysis of regression coefficients of multivariable outcome prediction models. Some authors, such as Riley et al. and Altman [ 81 , 84 ], are of the opinion that it remains practically impossible, due to poor reporting, publication bias, and heterogeneity across studies. However, a considerable number of outcome prediction studies have been published, and it would be useful to integrate this body of evidence into one summary result. Moreover, there is an increase in the number of reviews that are being published. Therefore, there is a need to find the best strategy to integrate the results of primary outcome prediction studies. Consequently, until a method to quantitatively synthesize results has been developed, a sensible qualitative data-synthesis, which takes methodological differences between primary studies into account, is indicated. In summarizing the evidence, differences in methodological items and model-building strategies should be described and taken into account when assessing the overall evidence for outcome prediction. For example, univariable and multivariable results should be described separately, or subgroup analyses should be performed when they are combined. Other items that, in our opinion should be taken into consideration with regard to the data-synthesis are: study quality, variables used for model development, statistical methods used for variable selection procedures, the performance of models, and sufficient cases and non-cases to guarantee adequate study power. Regardless of whether or not these items are taken into consideration in the data-synthesis, we strongly recommend that in reviews they are described for all primary studies included so that readers can also take them into consideration.
In conclusion, poor reporting of relevant information and differences in methodology occur in primary outcome prediction research. Even the predictive ability of the models was rarely reported. This, together with our current inability to pool multivariable outcome prediction models, challenges review authors to make informative reviews of outcome prediction models.
Search strategy: 01-03-2011
Database: MEDLINE
((“systematic review”[tiab] OR “systematic reviews”[tiab] OR “Meta-Analysis as Topic”[Mesh] OR meta-analysis[tiab] OR “Meta-Analysis”[Publication Type]) AND (“2005/11/01”[EDat] : “3000”[EDat]) AND ((“Incidence”[Mesh] OR “Models, Statistical”[Mesh] OR “Mortality”[Mesh] OR “mortality ”[Subheading] OR “Follow-Up Studies”[Mesh] OR “Prognosis”[Mesh:noexp] OR “Disease-Free Survival”[Mesh] OR “Disease Progression”[Mesh:noexp] OR “Natural History”[Mesh] OR “Prospective Studies”[Mesh]) OR ((cohort*[tw] OR course*[tw] OR first episode*[tw] OR predict*[tw] OR predictor*[tw] OR prognos*[tw] OR follow-up stud*[tw] OR inciden*[tw]) NOT medline[sb]))) NOT ((“addresses”[Publication Type] OR “biography”[Publication Type] OR “case reports”[Publication Type] OR “comment”[Publication Type] OR “directory”[Publication Type] OR “editorial”[Publication Type] OR “festschrift”[Publication Type] OR “interview”[Publication Type] OR “lectures”[Publication Type] OR “legal cases”[Publication Type] OR “legislation”[Publication Type] OR “letter”[Publication Type] OR “news”[Publication Type] OR “newspaper article”[Publication Type] OR “patient education handout”[Publication Type] OR “popular works”[Publication Type] OR “congresses”[Publication Type] OR “consensus development conference”[Publication Type] OR “consensus development conference, nih”[Publication Type] OR “practice guideline”[Publication Type]) OR (“Animals”[Mesh] NOT (“Animals”[Mesh] AND “Humans”[Mesh]))).
Items used to assess the characteristics of analyses in outcome prediction primary studies and reviews:
Information about the review:
What type of studies are included?
Is(/are) the outcome(s) of interest clearly described?
Is information about the quality assessment method provided?
What method was used?
Did the review account for quality?
Information about the analysis of the primary studies:
Are the outcome measures clearly described?
Is the statistical method used for variable selection described?
Is there a description of treatments received provided?
Information about the results of the primary studies:
Are crude univariable associations and estimates of dispersion for all the variables of the primary studies presented?
Are all variables that were used for model development described?
Are the multivariable associations and estimates of dispersions presented?
Is model performance assessed and reported?
Is the number of predictors relative to the number of outcome events described?
Data-analysis and synthesis of the review:
Is the heterogeneity of primary studies described?
Is a qualitative synthesis presented?
Are methods for quantitative analysis described?
Is the statistical heterogeneity assessed?
What method is used to assess statistical heterogeneity?
If statistical heterogeneity exists, are sources of the heterogeneity investigated?
What method is used to investigate potential sources of heterogeneity?
Is a graphical presentation of the results provided?
Are sensitivity analysis performed?
On which level?
Harrell FEJ, Lee KL, Mark DB: Multivariable prognostic models: issues in developing models, evaluating assumptions and adequacy, and measuring and reducing errors. Stat Med. 1996, 15: 361-387. 10.1002/(SICI)1097-0258(19960229)15:4<361::AID-SIM168>3.0.CO;2-4.
Article PubMed Google Scholar
Hemingway H, Riley RD, Altman DG: Ten steps towards improving prognosis research. BMJ. 2009, 339: b4184-10.1136/bmj.b4184.
Moons KGM, Donders AR, Steyerberg EW, Harrell FE: Penalized maximum likelihood estimation to directly adjust diagnostic and prognostic prediction models for overoptimism: a clinical example. J Clin Epidemiol. 2004, 57: 1262-1270. 10.1016/j.jclinepi.2004.01.020.
Article CAS PubMed Google Scholar
Royston P, Altman DG, Sauerbrei W: Dichotomizing continuous predictors in multiple regression: a bad idea. Stat Med. 2006, 25: 127-141. 10.1002/sim.2331.
Steyerberg EW: Clinical Prediction Models: A Practical Approach to Development, Validation, and Updating. 2009, New York: Springer
Book Google Scholar
Royston P, Moons KGM, Altman DG, Vergouwe Y: Prognosis and prognostic research: Developing a prognostic model. BMJ. 2009, 338: b604-10.1136/bmj.b604.
Moons KGM, Royston P, Vergouwe Y, Grobbee DE, Altman DG: Prognosis and prognostic research: what, why, and how?. BMJ. 2009, 338: b375-10.1136/bmj.b375.
Hayden JA, Dunn KM, van der Windt DA, Shaw WS: What is the prognosis of back pain?. Best Pract Res Clin Rheumatol. 2010, 24: 167-179. 10.1016/j.berh.2009.12.005.
Hayden JA, Chou R, Hogg-Johnson S, Bombardier C: Systematic reviews of low back pain prognosis had variable methods and results: guidance for future prognosis reviews. J Clin Epidemiol. 2009, 62: 781-796. 10.1016/j.jclinepi.2008.09.004.
Krasopoulos G, Brister SJ, Beattie WS, Buchanan MR: Aspirin “resistance” and risk of cardiovascular morbidity: systematic review and meta-analysis. BMJ. 2008, 336: 195-198. 10.1136/bmj.39430.529549.BE.
Article PubMed PubMed Central Google Scholar
Hayden JA, Cote P, Bombardier C: Evaluation of the quality of prognosis studies in systematic reviews. Ann Intern Med. 2006, 144: 427-437. 10.7326/0003-4819-144-6-200603210-00010.
Mallett S, Timmer A, Sauerbrei W, Altman DG: Reporting of prognostic studies of tumour markers: a review of published articles in relation to REMARK guidelines. Br J Cancer. 2010, 102: 173-180. 10.1038/sj.bjc.6605462.
Mallett S, Royston P, Waters R, Dutton S, Altman DG: Reporting performance of prognostic models in cancer: a review. BMC Med. 2010, 8: 21-10.1186/1741-7015-8-21.
Mallett S, Royston P, Dutton S, Waters R, Altman DG: Reporting methods in studies developing prognostic models in cancer: a review. BMC Med. 2010, 8: 20-10.1186/1741-7015-8-20.
Ingui BJ, Rogers MA: Searching for clinical prediction rules in MEDLINE. J Am Med Inform Assoc. 2001, 8: 391-397. 10.1136/jamia.2001.0080391.
Article CAS PubMed PubMed Central Google Scholar
Wilczynski NL: Natural History and Prognosis. PDQ, Evidence-Based Principles and Practice. Edited by: McKibbon KA, Wilczynski NL, Eady A, Marks S. 2009, Shelton, Connecticut: People’s Medical Publishing House
Google Scholar
Austin PC, Tu JV: Automated variable selection methods for logistic regression produced unstable models for predicting acute myocardial infarction mortality. J Clin Epidemiol. 2004, 57: 1138-1146. 10.1016/j.jclinepi.2004.04.003.
Lee M, Chodosh J: Dementia and life expectancy: what do we know?. J Am Med Dir Assoc. 2009, 10: 466-471. 10.1016/j.jamda.2009.03.014.
Gravante G, Garcea G, Ong S: Prediction of Mortality in Acute Pancreatitis: A Systematic Review of the Published Evidence. Pancreatology. 2009, 9: 601-614. 10.1159/000212097.
Celestin J, Edwards RR, Jamison RN: Pretreatment psychosocial variables as predictors of outcomes following lumbar surgery and spinal cord stimulation: a systematic review and literature synthesis. Pain Med. 2009, 10: 639-653. 10.1111/j.1526-4637.2009.00632.x.
Wright AA, Cook C, Abbott JH: Variables associated with the progression of hip osteoarthritis: a systematic review. Arthritis Rheum. 2009, 61: 925-936. 10.1002/art.24641.
Heitz C, Hilfiker R, Bachmann L: Comparison of risk factors predicting return to work between patients with subacute and chronic non-specific low back pain: systematic review. Eur Spine J. 2009, 18: 1829-35. 10.1007/s00586-009-1083-9.
Sansam K, Neumann V, O’Connor R, Bhakta B: Predicting walking ability following lower limb amputation: a systematic review of the literature. J Rehabil Med. 2009, 41: 593-603. 10.2340/16501977-0393.
Detaille SI, Heerkens YF, Engels JA, van der Gulden JWJ, van Dijk FJH: Common prognostic factors of work disability among employees with a chronic somatic disease: a systematic review of cohort studies. Scand J Work Environ Health. 2009, 35: 261-281. 10.5271/sjweh.1337.
Walton DM, Pretty J, MacDermid JC, Teasell RW: Risk factors for persistent problems following whiplash injury: results of a systematic review and meta-analysis. J Orthop Sports Phys Ther. 2009, 39: 334-350.
van Velzen JM, van Bennekom CAM, Edelaar MJA, Sluiter JK, Frings-Dresen MHW: Prognostic factors of return to work after acquired brain injury: a systematic review. Brain Inj. 2009, 23: 385-395. 10.1080/02699050902838165.
Borghuis MS, Lucassen PLBJ, van de Laar FA, Speckens AE, van Weel C, olde Hartman TC: Medically unexplained symptoms, somatisation disorder and hypochondriasis: course and prognosis. A systematic review. J Psychosom Res. 2009, 66: 363-377. 10.1016/j.jpsychores.2008.09.018.
Bramer JAM, van Linge JH, Grimer RJ, Scholten RJPM: Prognostic factors in localized extremity osteosarcoma: a systematic review. Eur J Surg Oncol. 2009, 35: 1030-1036. 10.1016/j.ejso.2009.01.011.
Tandon P, Garcia-Tsao G: Prognostic indicators in hepatocellular carcinoma: a systematic review of 72 studies. Liver Int. 2009, 29: 502-510. 10.1111/j.1478-3231.2008.01957.x.
Santaguida PL, Hawker GA, Hudak PL: Patient characteristics affecting the prognosis of total hip and knee joint arthroplasty: a systematic review. Can J Surg. 2008, 51: 428-436.
PubMed PubMed Central Google Scholar
Elmunzer BJ, Young SD, Inadomi JM, Schoenfeld P, Laine L: Systematic review of the predictors of recurrent hemorrhage after endoscopic hemostatic therapy for bleeding peptic ulcers. Am J Gastroenterol. 2008, 103: 2625-2632. 10.1111/j.1572-0241.2008.02070.x.
Adamson SJ, Sellman JD, Frampton CMA: Patient predictors of alcohol treatment outcome: a systematic review. J Subst Abuse Treat. 2009, 36: 75-86. 10.1016/j.jsat.2008.05.007.
Paez JIG, Costa SF: Risk factors associated with mortality of infections caused by Stenotrophomonas maltophilia: a systematic review. J Hosp Infect. 2008, 70: 101-108. 10.1016/j.jhin.2008.05.020.
Johnson SR, Swiston JR, Granton JT: Prognostic factors for survival in scleroderma associated pulmonary arterial hypertension. J Rheumatol. 2008, 35: 1584-1590.
PubMed Google Scholar
Clarke SA, Eiser C, Skinner R: Health-related quality of life in survivors of BMT for paediatric malignancy: a systematic review of the literature. Bone Marrow Transplant. 2008, 42: 73-82. 10.1038/bmt.2008.156.
Kok M, Cnossen J, Gravendeel L, van der Post J, Opmeer B, Mol BW: Clinical factors to predict the outcome of external cephalic version: a metaanalysis. Am J Obstet Gynecol. 2008, 199: 630-637.
Stuart-Harris R, Caldas C, Pinder SE, Pharoah P: Proliferation markers and survival in early breast cancer: a systematic review and meta-analysis of 85 studies in 32,825 patients. Breast. 2008, 17: 323-334. 10.1016/j.breast.2008.02.002.
Kamper SJ, Rebbeck TJ, Maher CG, McAuley JH, Sterling M: Course and prognostic factors of whiplash: a systematic review and meta-analysis. Pain. 2008, 138: 617-629. 10.1016/j.pain.2008.02.019.
Nijrolder I, van der Horst H, van der Windt D: Prognosis of fatigue. A systematic review. J Psychosom Res. 2008, 64: 335-349. 10.1016/j.jpsychores.2007.11.001.
Williams M, Williamson E, Gates S, Lamb S, Cooke M: A systematic literature review of physical prognostic factors for the development of Late Whiplash Syndrome. Spine (Phila Pa 1976). 2007, 32: E764-E780. 10.1097/BRS.0b013e31815b6565.
Article Google Scholar
Willemse-van Son AHP, Ribbers GM, Verhagen AP, Stam HJ: Prognostic factors of long-term functioning and productivity after traumatic brain injury: a systematic review of prospective cohort studies. Clin Rehabil. 2007, 21: 1024-1037. 10.1177/0269215507077603.
Alvarez J, Wilkinson J, Lipshultz S: Outcome Predictors for Pediatric Dilated Cardiomyopathy: A Systematic Review. Prog Pediatr Cardiol. 2007, 23: 25-32. 10.1016/j.ppedcard.2007.05.009.
Mallen CD, Peat G, Thomas E, Dunn KM, Croft PR: Prognostic factors for musculoskeletal pain in primary care: a systematic review. Br J Gen Pract. 2007, 57: 655-661.
Stroke Risk in Atrial Fibrillation Working Group: Independent predictors of stroke in patients with atrial fibrillation: a systematic review. Neurology. 2007, 69: 546-554.
Kent PM, Keating JL: Can we predict poor recovery from recent-onset nonspecific low back pain? A systematic review. Man Ther. 2008, 13: 12-28. 10.1016/j.math.2007.05.009.
Tjang YS, van Hees Y, Korfer R, Grobbee DE, van der Heijden GJMG: Predictors of mortality after aortic valve replacement. Eur J Cardiothorac Surg. 2007, 32: 469-474. 10.1016/j.ejcts.2007.06.012.
Pfannschmidt J, Dienemann H, Hoffmann H: Surgical resection of pulmonary metastases from colorectal cancer: a systematic review of published series. Ann Thorac Surg. 2007, 84: 324-338. 10.1016/j.athoracsur.2007.02.093.
Williamson E, Williams M, Gates S, Lamb SE: A systematic literature review of psychological factors and the development of late whiplash syndrome. Pain. 2008, 135: 20-30. 10.1016/j.pain.2007.04.035.
Tas U, Verhagen AP, Bierma-Zeinstra SMA, Odding E, Koes BW: Prognostic factors of disability in older people: a systematic review. Br J Gen Pract. 2007, 57: 319-323.
Rassi AJ, Rassi A, Rassi SG: Predictors of mortality in chronic Chagas disease: a systematic review of observational studies. Circulation. 2007, 115: 1101-1108. 10.1161/CIRCULATIONAHA.106.627265.
Belo JN, Berger MY, Reijman M, Koes BW, Bierma-Zeinstra SMA: Prognostic factors of progression of osteoarthritis of the knee: a systematic review of observational studies. Arthritis Rheum. 2007, 57: 13-26. 10.1002/art.22475.
Langer-Gould A, Popat RA, Huang SM: Clinical and demographic predictors of long-term disability in patients with relapsing-remitting multiple sclerosis: a systematic review. Arch Neurol. 2006, 63: 1686-1691. 10.1001/archneur.63.12.1686.
Lamme B, Mahler CW, van Ruler O, Gouma DJ, Reitsma JB, Boermeester MA: Clinical predictors of ongoing infection in secondary peritonitis: systematic review. World J Surg. 2006, 30: 2170-2181. 10.1007/s00268-005-0333-1.
van Dijk GM, Dekker J, Veenhof C, van den Ende CHM: Course of functional status and pain in osteoarthritis of the hip or knee: a systematic review of the literature. Arthritis Rheum. 2006, 55: 779-785. 10.1002/art.22244.
Aalto TJ, Malmivaara A, Kovacs F: Preoperative predictors for postoperative clinical outcome in lumbar spinal stenosis: systematic review. Spine (Phila Pa 1976). 2006, 31: E648-E663. 10.1097/01.brs.0000231727.88477.da.
Hauser CA, Stockler MR, Tattersall MHN: Prognostic factors in patients with recently diagnosed incurable cancer: a systematic review. Support Care Cancer. 2006, 14: 999-1011. 10.1007/s00520-006-0079-9.
Bollen CW, Uiterwaal CSPM, van Vught AJ: Systematic review of determinants of mortality in high frequency oscillatory ventilation in acute respiratory distress syndrome. Crit Care. 2006, 10: R34-10.1186/cc4824.
Steenstra IA, Verbeek JH, Heymans MW, Bongers PM: Prognostic factors for duration of sick leave in patients sick listed with acute low back pain: a systematic review of the literature. Occup Environ Med. 2005, 62: 851-860. 10.1136/oem.2004.015842.
Bai M, Qi X, Yang Z: Predictors of hepatic encephalopathy after transjugular intrahepatic portosystemic shunt in cirrhotic patients: a systematic review. J Gastroenterol Hepatol. 2011, 26: 943-51. 10.1111/j.1440-1746.2011.06663.x.
Monteiro-Soares M, Boyko E, Ribeiro J, Ribeiro I, Dinis-Ribeiro M: Risk stratification systems for diabetic foot ulcers: a systematic review. Diabetologia. 2011, 54: 1190-1199. 10.1007/s00125-010-2030-3.
Lichtman JH, Leifheit-Limson EC, Jones SB: Predictors of hospital readmission after stroke: a systematic review. Stroke. 2010, 41: 2525-2533. 10.1161/STROKEAHA.110.599159.
Ronden RA, Houben AJ, Kessels AG, Stehouwer CD, de Leeuw PW, Kroon AA: Predictors of clinical outcome after stent placement in atherosclerotic renal artery stenosis: a systematic review and meta-analysis of prospective studies. J Hypertens. 2010, 28: 2370-2377.
de Jonge RCJ, van Furth AM, Wassenaar M, Gemke RJBJ, Terwee CB: Predicting sequelae and death after bacterial meningitis in childhood: a systematic review of prognostic studies. BMC Infect Dis. 2010, 10: 232-10.1186/1471-2334-10-232.
Colohan SM: Predicting prognosis in thermal burns with associated inhalational injury: a systematic review of prognostic factors in adult burn victims. J Burn Care Res. 2010, 31: 529-539. 10.1097/BCR.0b013e3181e4d680.
Clay FJ, Newstead SV, McClure RJ: A systematic review of early prognostic factors for return to work following acute orthopaedic trauma. Injury. 2010, 41: 787-803. 10.1016/j.injury.2010.04.005.
Brabrand M, Folkestad L, Clausen NG, Knudsen T, Hallas J: Risk scoring systems for adults admitted to the emergency department: a systematic review. Scand J Trauma Resusc Emerg Med. 2010, 18: 8-10.1186/1757-7241-18-8.
Montazeri A: Quality of life data as prognostic indicators of survival in cancer patients: an overview of the literature from 1982 to 2008. Health Qual Life Outcomes. 2009, 7: 102-10.1186/1477-7525-7-102.
Copas JB: Prediction and Shrinkage. J R Stat Soc Ser B (methodological). 1983, 45: 311-354.
Atkins D, Best D, Briss PA: Grading quality of evidence and strength of recommendations. BMJ. 2004, 328: 1490-
Deeks JJ, Dinnes J, D’Amico R: Evaluating non-randomised intervention studies. Health Technol Assess. 2003, 7: iii-173-
Parekh-Bhurke S, Kwok CS, Pang C: Uptake of methods to deal with publication bias in systematic reviews has increased over time, but there is still much scope for improvement. J Clin Epidemiol. 2011, 64: 349-57. 10.1016/j.jclinepi.2010.04.022.
Steyerberg EW: Selection of main effects. Clinicical Prediction Models: A Practical Approach to Development, Validation, and Updating. 2009, New York: Springer
Chatfield C: Model Uncertainty, Data Mining and Statistical Inference. J R Stat Soc Ser A. 1995, 158: 419-466. 10.2307/2983440.
Steyerberg EW, Eijkemans MJ, Habbema JD: Stepwise selection in small data sets: a simulation study of bias in logistic regression analysis. J Clin Epidemiol. 1999, 52: 935-942. 10.1016/S0895-4356(99)00103-1.
Altman DG: Systematic reviews of evaluations of prognostic variables. BMJ. 2001, 323: 224-228. 10.1136/bmj.323.7306.224.
Kyzas PA, Ioannidis JPA, axa-Kyza D: Quality of reporting of cancer prognostic marker studies: association with reported prognostic effect. J Natl Cancer Inst. 2007, 99: 236-243. 10.1093/jnci/djk032.
Kyzas PA, Ioannidis JPA, axa-Kyza D: Almost all articles on cancer prognostic markers report statistically significant results. Eur J Cancer. 2007, 43: 2559-2579. 10.1016/j.ejca.2007.08.030.
Rifai N, Altman DG, Bossuyt PM: Reporting bias in diagnostic and prognostic studies: time for action. Clin Chem. 2008, 54: 1101-1103. 10.1373/clinchem.2008.108993.
Vergouwe Y, Steyerberg EW, Eijkemans MJC, Habbema JD: Validity of prognostic models: when is a model clinically useful?. Semin Urol Oncol. 2002, 20: 96-107. 10.1053/suro.2002.32521.
Altman DG, Vergouwe Y, Royston P, Moons KGM: Prognosis and prognostic research: validating a prognostic model. BMJ. 2009, 338: b605-10.1136/bmj.b605.
Altman DG: Systematic reviews of evaluations of prognostic variables. Systematic Reviews in Health Care. Edited by: Egger M, Smith GD, Altman DG. 2001, London: BMJ Publishing Group, 228-47.
Chapter Google Scholar
Ware JH: The limitations of risk factors as prognostic tools. N Engl J Med. 2006, 355: 2615-2617. 10.1056/NEJMp068249.
Harrell FE: Multivariable modeling strategies. Regression modeling strategies with applications to linear models, logistic regression, and survival analysis. 2001, New York: Springer,
Riley RD, Abrams KR, Sutton AJ: Reporting of prognostic markers: current problems and development of guidelines for evidence-based practice in the future. Br J Cancer. 2003, 88: 1191-1198. 10.1038/sj.bjc.6600886.
Pre-publication history
The pre-publication history for this paper can be accessed here: http://www.biomedcentral.com/1471-2288/13/42/prepub
Download references
Acknowledgment
We thank Ilse Jansma, MSc, for her contributions as a medical information specialist regarding the Medline search strategy. No compensation was received for her contribution.
No external funding was received for this study.
Author information
Authors and affiliations.
Department of Epidemiology and Biostatistics and the EMGO Institute for Health and Care Research, VU University Medical Centre, Amsterdam, The Netherlands
Tobias van den Berg, Martijn W Heymans & Henrica CW de Vet
Department of General Practice and the EMGO Institute for Health and Care Research, VU University Medical Centre, Amsterdam, The Netherlands
Stephanie S Leone & David Vergouw
Department of Community Health and Epidemiology, Dalhousie University, Halifax, Nova Scotia, Canada
Jill A Hayden
Department of General Practice, Erasmus Medical Centre, Rotterdam, The Netherlands
Arianne P Verhagen
You can also search for this author in PubMed Google Scholar
Corresponding author
Correspondence to Tobias van den Berg .
Additional information
Competing interests.
All authors report no conflicts of interests.
Authors’ contributions
TvdB, had full access to all of the data in the study and takes responsibility for the integrity of the data and the accuracy of the data analysis. Study concept and design: TvdB, MH, JH, AV, HdV. Acquisition of data: TvdB, MH, SL, DV, AV, HdV Analysis and interpretation of data: TvdB, MH, HdV. Drafting of the manuscript: TvdB, MH, HdV. Critical revision of the manuscript for important intellectual content: TvdB, MH, SL, DV, JH, AV, HdV. Statistical analysis: TvdB Study supervision: MH, HdV. All authors read and approved the final manuscript.
Authors’ original submitted files for images
Below are the links to the authors’ original submitted files for images.
Authors’ original file for figure 1
Authors’ original file for figure 2, rights and permissions.
This article is published under license to BioMed Central Ltd. This is an Open Access article distributed under the terms of the Creative Commons Attribution License ( http://creativecommons.org/licenses/by/2.0 ), which permits unrestricted use, distribution, and reproduction in any medium, provided the original work is properly cited.
Reprints and permissions
About this article
Cite this article.
van den Berg, T., Heymans, M.W., Leone, S.S. et al. Overview of data-synthesis in systematic reviews of studies on outcome prediction models. BMC Med Res Methodol 13 , 42 (2013). https://doi.org/10.1186/1471-2288-13-42
Download citation
Received : 26 September 2012
Accepted : 04 March 2013
Published : 16 March 2013
DOI : https://doi.org/10.1186/1471-2288-13-42
Share this article
Anyone you share the following link with will be able to read this content:
Sorry, a shareable link is not currently available for this article.
Provided by the Springer Nature SharedIt content-sharing initiative
- Meta-analysis
- Forecasting
BMC Medical Research Methodology
ISSN: 1471-2288
- General enquiries: [email protected]
Should I do a synthesis (i.e. literature review)?
- Questions & Quandaries
- Published: 18 April 2024
Cite this article
- H. Carrie Chen 1 ,
- Ayelet Kuper 2 , 3 , 4 ,
- Jennifer Cleland 5 &
- Patricia O’Sullivan 6
257 Accesses
1 Altmetric
Explore all metrics
This column is intended to address the kinds of knotty problems and dilemmas with which many scholars grapple in studying health professions education. In this article, the authors address the question of whether one should conduct a literature review or knowledge synthesis, considering the why, when, and how, as well as its potential pitfalls. The goal is to guide supervisors and students who are considering whether to embark on a literature review in education research.
Avoid common mistakes on your manuscript.
Two junior colleagues come to you to ask your advice about carrying out a literature review on a particular topic. “Should they?” immediately pops into your mind, followed closely by, if yes, then what kind of literature review is appropriate? Our experience is that colleagues often come to suggest a literature review to “kick start” their research (in fact, some academic programs require them as part of degree requirements), without a full understanding of the work involved, the different types of literature review, and what type of literature review might be most suitable for their research question. In this Questions and Quandaries, we address the question of literature reviews in education research, considering the why, when, and how, as well as potential pitfalls.
First, what is meant by literature review? The term literature review has been used to refer to both a review of the literature and a knowledge synthesis (Maggio et al., 2018 ; Siddaway et al., 2019 ). For our purposes, we employ the term as commonly used to refer to a knowledge synthesis , which is a formal comprehensive review of the existing body of literature on a topic. It is a research approach that critically integrates and synthesizes available evidence from multiple studies to provide insight and allow the drawing of conclusions. It is an example of Boyer’s scholarship of integration (Boyer, 1990 ). In contrast, a review of the literature is a relatively casual and expedient method for attaining a general overview of the state of knowledge on a given topic to make the argument that a new study is needed. In this interpretation, a literature review serves as a key starting point for anyone conducting research by identifying gaps in the literature, informing the study question, and situating one’s study in the field.
Whether a formal knowledge synthesis should be done depends on if a review is needed and what the rationale is for the review. The first question to consider is whether a literature review already exists. If no, is there enough literature published on the topic to warrant a review? If yes, does the previous review need updating? How long has it been since the last review and has the literature expanded so much or are there important new studies that need integrating to justify an updated review? Or were there flaws in the previous review that one intends to address with a new review? Or does one intend to address a different question than the focus of the previous review?
If the knowledge synthesis is to be done, it should be driven by a research question. What is the research question? Can it be answered by a review? What is the purpose of the synthesis? There are two main purposes for knowledge synthesis– knowledge support and decision support. Knowledge support summarizes the evidence while decision support takes additional analytical steps to allow for decision-making in particular contexts (Mays et al., 2005 ).
If the purpose is to provide knowledge support, then the question is how or what will the knowledge synthesis add to the literature? Will it establish the state of knowledge in an area, identify gaps in the literature/knowledge base, and/or map opportunities for future research? Cornett et al., performed a scoping review of the literature on professional identity, focusing on how professional identity is described, why the studies where done, and what constructs of identity were used. Their findings advanced understanding of the state of knowledge by indicating that professional identity studies were driven primarily by the desire to examine the impact of political, social and healthcare reforms and advances, and that the various constructs of professional identity across the literature could be categorized into five themes (Cornett et al., 2023 ).
If, on the other hand, the purpose of the knowledge synthesis is to provide decision support, for whom will the synthesis be relevant and how will it improve practice? Will the synthesis result in tools such as guidelines or recommendations for practitioners and policymakers? An example of a knowledge synthesis for decision support is a systematic review conducted by Spencer and colleagues to examine the validity evidence for use of the Ottawa Surgical Competency Operating Room Evaluation (OSCORE) assessment tool. The authors summarized their findings with recommendations for educational practice– namely supporting the use of the OSCORE for in-the-moment entrustment decisions by frontline supervisors in surgical fields but cautioning about the limited evidence for support of its use in summative promotions decisions or non-surgical contexts (Spencer et al., 2022 ).
If a knowledge synthesis is indeed appropriate, its methodology should be informed by its research question and purpose. We do not have the space to discuss the various types of knowledge synthesis except to say that several types have been described in the literature. The five most common types in health professions education are narrative reviews, systematic reviews, umbrella reviews (meta-syntheses), scoping reviews, and realist reviews (Maggio et al., 2018 ). These represent different epistemologies, serve different review purposes, use different methods, and result in different review outcomes (Gordon, 2016 ).
Each type of review lends itself best to answering a certain type of research question. For instance, narrative reviews generally describe what is known about a topic without necessarily answering a specific empirical question (Maggio et al., 2018 ). A recent example of a narrative review focused on schoolwide wellbeing programs, describing what is known about the key characteristics and mediating factors that influence student support and identifying critical tensions around confidentiality that could make or break programs (Tan et al., 2023 ). Umbrella reviews, on the other hand, synthesize evidence from multiple reviews or meta-analyses and can illuminate agreement, inconsistencies, or evolution of evidence on a topic. For example, an umbrella review on problem-based learning highlighted the shift in research focus over time from does it work, to how does it work, to how does it work in different contexts, and pointed to directions for new research (Hung et al., 2019 ).
Practical questions for those considering a literature review include whether one has the time required and an appropriate team to conduct a high-quality knowledge synthesis. Regardless of the type of knowledge synthesis and use of quantitative or qualitative methods, all require rigorous and clear methods that allow for reproducibility. This can take time, up to 12–18 months. A high-quality knowledge synthesis also requires a team whose members have expertise not only in the content matter, but also in knowledge synthesis methodology and in literature searches (i.e. a librarian). A team with multiple reviewers with a variety of perspectives can also help manage the volume of large reviews, minimize potential biases, and strengthen the critical analysis.
Finally, a pitfall one should be careful to avoid is merely summarizing everything in the literature without critical evaluation and integration of the information. A knowledge synthesis that merely bean counts or presents a collection of unconnected information that has not been reflected upon or critically analyzed does not truly advance knowledge or decision-making. Rather, it leads us back to our original question of whether it should have been done in the first place.
Boyer, E. L. (1990). Scholarship reconsidered: Priorities of the professoriate (pp. 18–21). Princeton University Press.
Cornett, M., Palermo, C., & Ash, S. (2023). Professional identity research in the health professions—a scoping review. Advances in Health Sciences Education , 28 (2), 589–642.
Article Google Scholar
Gordon, M. (2016). Are we talking the same paradigm? Considering methodological choices in health education systematic review. Medical Teacher , 38 (7), 746–750.
Hung, W., Dolmans, D. H. J. M., & van Merrienboer, J. J. G. (2019). A review to identify key perspectives in PBL meta-analyses and reviews: Trends, gaps and future research directions. Advances in Health Sciences Education , 24 , 943–957.
Maggio, L. A., Thomas, A., & Durning, S. J. (2018). Knowledge synthesis. In T. Swanwick, K. Forrest, & B. C. O’Brien (Eds.), Understanding Medical Education: Evidence, theory, and practice (pp. 457–469). Wiley.
Mays, N., Pope, C., & Popay, J. (2005). Systematically reviewing qualitative and quantitative evidence to inform management and policy-making in the health field. Journal of Health Services Research & Policy , 10 (1_suppl), 6–20.
Siddaway, A. P., Wood, A. M., & Hedges, L. V. (2019). How to do a systematic review: A best practice guide for conducting and reporting narrative reviews, meta-analyses, and meta-syntheses. Annual Review of Psychology , 70 , 747–770.
Spencer, M., Sherbino, J., & Hatala, R. (2022). Examining the validity argument for the Ottawa Surgical Competency operating room evaluation (OSCORE): A systematic review and narrative synthesis. Advances in Health Sciences Education , 27 , 659–689.
Tan, E., Frambach, J., Driessen, E., & Cleland, J. (2023). Opening the black box of school-wide student wellbeing programmes: A critical narrative review informed by activity theory. Advances in Health Sciences Education . https://doi.org/10.1007/s10459-023-10261-8 . Epub ahead of print 02 July 2023.
Download references
Author information
Authors and affiliations.
Georgetown University School of Medicine, Washington, DC, USA
H. Carrie Chen
Wilson Centre for Research in Education, University Health Network, University of Toronto, Toronto, Canada
Ayelet Kuper
Division of General Internal Medicine, Sunnybrook Health Sciences Center, Toronto, Canada
Department of Medicine, University of Toronto, Toronto, Canada
Lee Kong Chian School of Medicine, Nanyang Technological University Singapore, Singapore, Singapore
Jennifer Cleland
University of California San Francisco School of Medicine, San Francisco, CA, USA
Patricia O’Sullivan
You can also search for this author in PubMed Google Scholar
Corresponding author
Correspondence to H. Carrie Chen .
Additional information
Publisher’s note.
Springer Nature remains neutral with regard to jurisdictional claims in published maps and institutional affiliations.
Rights and permissions
Reprints and permissions
About this article
Chen, H.C., Kuper, A., Cleland, J. et al. Should I do a synthesis (i.e. literature review)?. Adv in Health Sci Educ (2024). https://doi.org/10.1007/s10459-024-10335-1
Download citation
Published : 18 April 2024
DOI : https://doi.org/10.1007/s10459-024-10335-1
Share this article
Anyone you share the following link with will be able to read this content:
Sorry, a shareable link is not currently available for this article.
Provided by the Springer Nature SharedIt content-sharing initiative
- Find a journal
- Publish with us
- Track your research

About Systematic Reviews
Strategy for Data Synthesis in Systematic Review
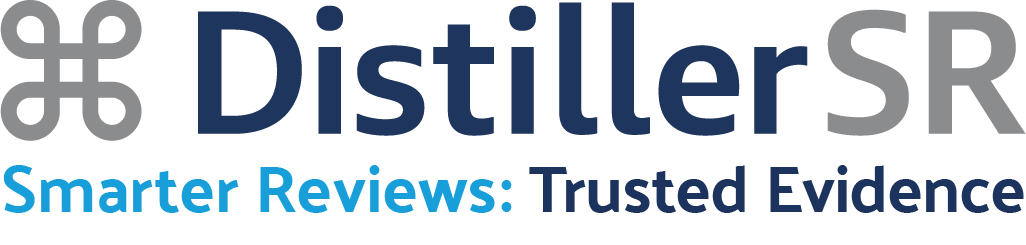
Automate every stage of your literature review to produce evidence-based research faster and more accurately.
The purpose of a data extraction table within a systematic review becomes apparent during synthesis, where reviewers collate and evaluate the meaning of the data gathered. Synthesis means that reviewers use the information from their data extraction template for systematic review to create coherent bodies of data that can be analyzed to gain a deeper understanding of the information conveyed.
Reviewers should have a clear strategy showing how they will approach data synthesis to expedite and verify outcomes, such as whether or not their specific review subject requires a meta-analysis or a quantitative synthesis.
The Importance of a Data Synthesis Strategy
Numerous synthesis methodologies are available, making it important to have a defined data extraction process systematic review relevant that describes how a reviewer will categorize and interpret data and use that evaluation to reach conclusions.
Appropriate research approaches can adopt broad categories, such as emerging, qualitative, quantitative, and conventional syntheses. However, each has varying characteristics, context, assumptions, analysis units, strengths, and restrictions that determine which potential technique is most suited to the systematic review in question.
The right data extraction process for systematic review will depend on these variables and the anticipated outcomes and theories that the study seeks to uphold or disprove.
Alternative Data Synthesis Approaches
Below, we examine the four primary subsections of data synthesis used in systematic reviews to demonstrate how each applies depending on the data types available.
Conventional Synthesis
This is used to produce charts, diagrams, maps, and tables, demonstrating conceptual frameworks or theories. This type of data synthesis examines data types such as quantitative studies, literature, policy documentation, and qualitative research.
Some downsides include a reduced element of critique, and systematic evaluation, making it more suitable for reassessing existing topics or preliminary conceptualization for new pieces of research.
Qualitative Synthesis
Our next data synthesis approach involves collating or integrating multiple data sets comprising qualitative research findings and theoretical literature. Outcomes involve conceptual frameworks or maps, definitions, and narrative summaries of the subject matter.
Quantitative Synthesis
This category of systematic review is similar to qualitative synthesis, although it uses quantitative studies to produce generalizable statements, narrative summaries, and mathematical scoring evaluations.
Emerging Synthesis
Finally, approaching data synthesis with an emerging strategy takes a newer approach, incorporating literature and metrics from a broad spectrum of data types, including diverse subject groups.
Selected data sources might include quantitative and qualitative studies, editorials, policies, evaluations, commentaries, and theoretical work. A systematic review adopting an emerging data synthesis approach can produce conceptual maps, decision-making reports, and statistics such as charts, graphs, diagrams, and scoring.
Learn More About DistillerSR
(Article continues below)
Why Does Systematic Review Require a Data Synthesis Strategy?
Synthesized data represents the results derived from studies and analyzed in relevance to the question or theory the systematic review attempts to answer. Because the synthesis technique used dictates the data used and the possible outcomes of the review, reviewers must take the right approach, evaluating the strengths and drawbacks of each and how synthesis adds value to the exercise.
The right strategy can make a considerable difference to the integrity of the outcomes and effects found and the value and credence of the quality of the information provided as a final conclusion.
3 Reasons to Connect
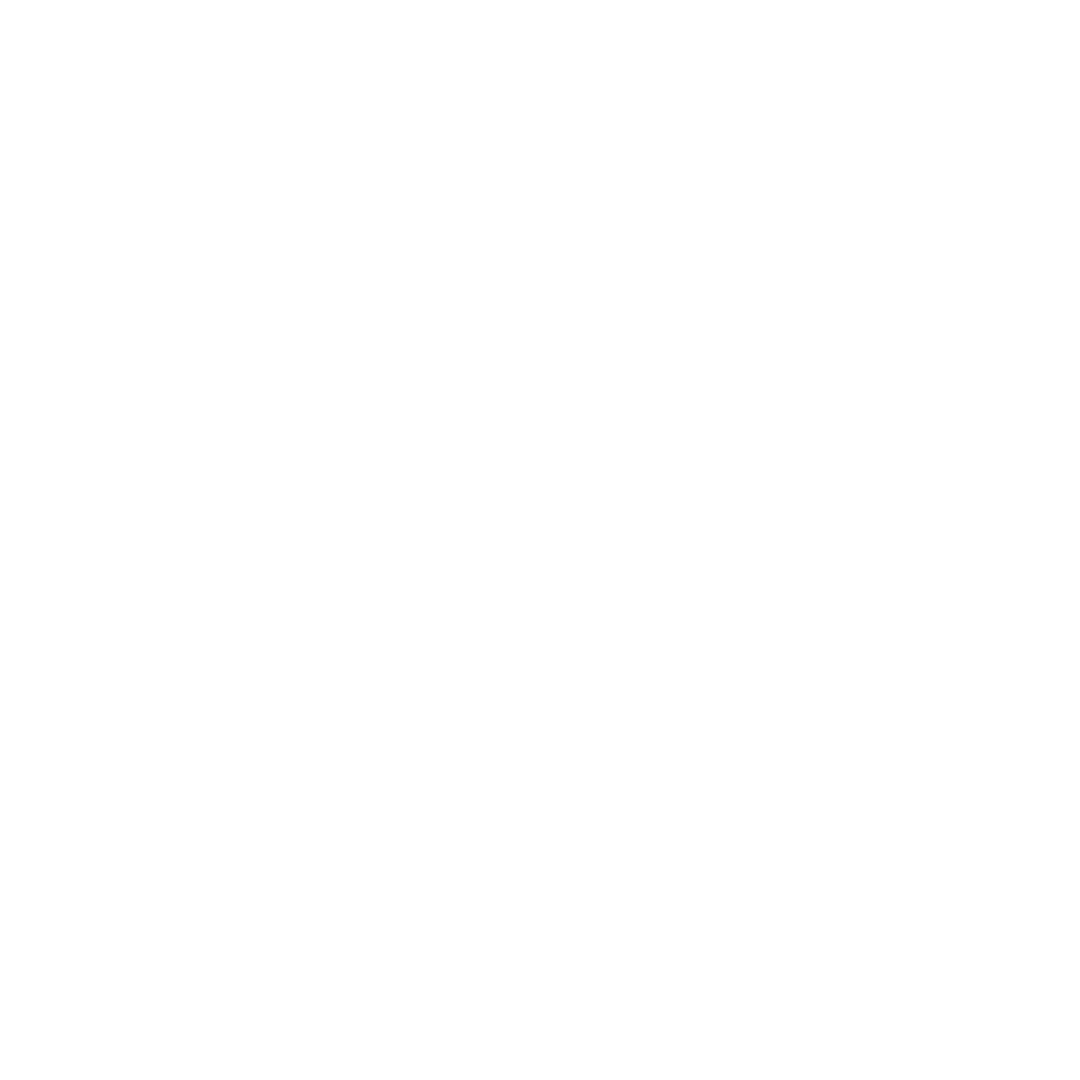

Good review practice: a researcher guide to systematic review methodology in the sciences of food and health
- About this guide
- Part A: Systematic review method
- What are Good Practice points?
- Part C: The core steps of the SR process
- 1.1 Setting eligibility criteria
- 1.2 Identifying search terms
- 1.3 Protocol development
- 2. Searching for studies
- 3. Screening the results
- 4. Evaluation of included studies: quality assessment
- 5. Data extraction
- 6. Data synthesis and summary
- 7. Presenting results
- Links to current versions of the reference guidelines
- Download templates
- Food science databases
- Process management tools
- Screening tools
- Reference management tools
- Grey literature sources
- Links for access to protocol repository and platforms for registration
- Links for access to PRISMA frameworks
- Links for access to 'Risk of Bias' assessment tools for quantitative and qualitative studies
- Links for access to grading checklists
- Links for access to reporting checklists
- What questions are suitable for the systematic review methodology?
- How to assess feasibility of using the method?
- What is a scoping study and how to construct one?
- How to construct a systematic review protocol?
- How to construct a comprehensive search?
- Study designs and levels of evidence
- Download a pdf version This link opens in a new window
Data synthesis and summary
Data synthesis and summary .
Data synthesis includes synthesising the findings of primary studies and when possible or appropriate some forms of statistical analysis of numerical data. Synthesis methods vary depending on the nature of the evidence (e.g., quantitative, qualitative, or mixed), the aim of the review and the study types and designs. Reviewers have to decide and preselect a method of analysis based on the review question at the protocol development stage.
Synthesis Methods
Narrative summary : is a summary of the review results when meta-analysis is not possible. Narrative summaries describe the results of the review, but some can take a more interpretive approach in summarising the results . [8] These are known as " evidence statements " and can include the results of quality appraisal and weighting processes and provide the ratings of the studies.
Meta-analysis : is a quantitative synthesis of the results from included studies using statistical analysis methods that are extensions to those used in primary studies. [9] Meta-analysis can provide a more precise estimate of the outcomes by measuring and counting for uncertainty of outcomes from individual studies by means of statistical methods. However, it is not always feasible to conduct statistical analyses due to several reasons including inadequate data, heterogeneous data, poor quality of included studies and the level of complexity. [10]
Qualitative Data Synthesis (QDS) : is a method of identifying common themes across qualitative studies to create a great degree of conceptual development compared with narrative reviews. The key concepts are identified through a process that begins with interpretations of the primary findings reported to researchers which will then be interpreted to their views of the meaning in a second-order and finally interpreted by reviewers into explanations and generating hypotheses. [11]
Mixed methods synthesis: is an advanced method of data synthesis developed by EPPI-Centre to better understand the meanings of quantitative studies by conducting a parallel review of user evaluations to traditional systematic reviews and combining the findings of the syntheses to identify and provide clear directions in practice. [11]
- << Previous: 5. Data extraction
- Next: 7. Presenting results >>
- Last Updated: Sep 18, 2023 1:16 PM
- URL: https://ifis.libguides.com/systematic_reviews

- University of Texas Libraries
- UT Libraries
Systematic Reviews & Evidence Synthesis Methods
- Types of Reviews
- Formulate Question
- Find Existing Reviews & Protocols
- Register a Protocol
- Searching Systematically
- Supplementary Searching
- Managing Results
- Deduplication
- Critical Appraisal
- Glossary of terms
- Librarian Support
- Video tutorials This link opens in a new window
- Systematic Review & Evidence Synthesis Boot Camp
Once you have completed your analysis, you will want to both summarize and synthesize those results. You may have a qualitative synthesis, a quantitative synthesis, or both.
Qualitative Synthesis
In a qualitative synthesis, you describe for readers how the pieces of your work fit together. You will summarize, compare, and contrast the characteristics and findings, exploring the relationships between them. Further, you will discuss the relevance and applicability of the evidence to your research question. You will also analyze the strengths and weaknesses of the body of evidence. Focus on where the gaps are in the evidence and provide recommendations for further research.
Quantitative Synthesis
Whether or not your Systematic Review includes a full meta-analysis, there is typically some element of data analysis. The quantitative synthesis combines and analyzes the evidence using statistical techniques. This includes comparing methodological similarities and differences and potentially the quality of the studies conducted.
Summarizing vs. Synthesizing
In a systematic review, researchers do more than summarize findings from identified articles. You will synthesize the information you want to include.
While a summary is a way of concisely relating important themes and elements from a larger work or works in a condensed form, a synthesis takes the information from a variety of works and combines them together to create something new.
Synthesis :
"The goal of a systematic synthesis of qualitative research is to integrate or compare the results across studies in order to increase understanding of a particular phenomenon, not to add studies together. Typically the aim is to identify broader themes or new theories – qualitative syntheses usually result in a narrative summary of cross-cutting or emerging themes or constructs, and/or conceptual models."
Denner, J., Marsh, E. & Campe, S. (2017). Approaches to reviewing research in education. In D. Wyse, N. Selwyn, & E. Smith (Eds.), The BERA/SAGE Handbook of educational research (Vol. 2, pp. 143-164). doi: 10.4135/9781473983953.n7
- Approaches to Reviewing Research in Education from Sage Knowledge
Data synthesis (Collaboration for Environmental Evidence Guidebook)
Interpreting findings and and reporting conduct (Collaboration for Environmental Evidence Guidebook)
Interpreting results and drawing conclusions (Cochrane Handbook, Chapter 15)
Guidance on the conduct of narrative synthesis in systematic reviews (ESRC Methods Programme)
- Last Updated: Apr 9, 2024 8:57 PM
- URL: https://guides.lib.utexas.edu/systematicreviews
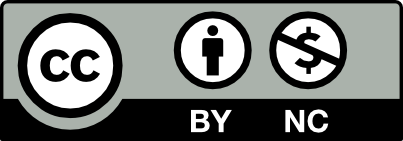
Library buildings are open for UniSA staff and students via UniSA ID swipe cards. Please contact us on Ask the Library for any assistance. Find out about other changes to Library services .
- Overview of systematic reviews
- Other review types
- Glossary of terms
- Define question
- Top tools and techniques
- How to search
- Where to search
- Subject headings
- Search filters
- Review your search
- Run your search on other databases
- Search the grey literature
- Report search results
- Updating a search
- How to screen
- Critical appraisal
Data synthesis
Data synthesis overview.
Now that you have extracted your data , the next step is to synthesise the data.
Move through the slide deck below to learn about data synthesis. Alternatively, download the PDF document at the bottom of this box.
- Data synthesis This document is a printable version of the slide deck above.
Forest plot example
If you have conducted a meta-analysis, you can present a summary of each study and your overall findings in a forest plot.
Select the i icons in the image below to learn about each component of a forest plot.
Test your knowledge
Test your knowledge about meta-analysis and forest plots in the activity below.
Guidelines and standards
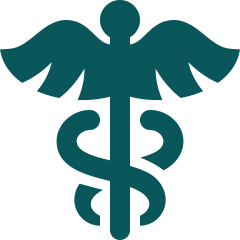
- Preferred Reporting Items for Systematic Reviews and Meta-Analyses (PRISMA) website
Items 13a - 13f of the PRISMA 2020 Checklist address synthesis methods and are described further in the Explanation and Elaboration document.
Other standards
- Overview of systematic reviews See the overview page (of this guide) for additional guidelines and standards.
- Interpreting and understanding meta-analysis graphs: a practical guide (2006) Provides a practical guide for appraising systematic reviews for relevance to clinical practice and interpreting meta-analysis graphs .
- What is a meta-analysis? (CEBI, University of Oxford) Provides addition information about meta-analyses.
- How to read a forest plot (CEBI, University of Oxford) Additional detailed information about how to read a forest plot.
Additional readings:
- Modern meta-analysis review and update of methodologies (2017) Comprehensive details about conducting meta-analyses.
- Meta-synthesis and evidence-based health care - a method for systematic review (2012) This article describes the process of systematic review of qualitative studies.
- Lessons from comparing narrative synthesis and meta-analysis in a systematic review (2015). Investigates the contribution and implications of narrative synthesis and meta-analysis in a systematic review.
- Speech-language pathologist interventions for communication in moderate-severe dementia: a systematic review (2018) An example of a systematic review without a meta-analysis.
Next topic →
- << Previous: Extraction
- Next: Write >>
- Last Updated: Apr 22, 2024 9:44 AM
- URL: https://guides.library.unisa.edu.au/SystematicReviews
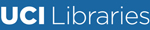
- Langson Library
- Science Library
- Grunigen Medical Library
- Law Library
- Connect From Off-Campus
- Accessibility
- Gateway Study Center
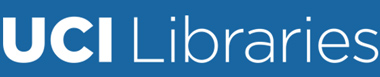
Email this link
Systematic reviews & evidence synthesis methods.
- Schedule a Consultation / Meet our Team
- What is Evidence Synthesis?
- Types of Evidence Synthesis
- Evidence Synthesis Across Disciplines
- Finding and Appraising Existing Systematic Reviews
- 1. Develop a Protocol
- 2. Draft your Research Question
- 3. Select Databases
- 4. Select Grey Literature Sources
- 5. Write a Search Strategy
- 6. Register a Protocol
- 7. Translate Search Strategies
- 8. Citation Management
- 9. Article Screening
- 10. Risk of Bias Assessment
- 11. Data Extraction
- 12. Synthesize, Map, or Describe the Results
- Open Access Evidence Synthesis Resources
Data Extraction
Whether you plan to perform a meta-analysis or not, you will need to establish a regimented approach to extracting data. Researchers often use a form or table to capture the data they will then summarize or analyze. The amount and types of data you collect, as well as the number of collaborators who will be extracting it, will dictate which extraction tools are best for your project. Programs like Excel or Google Spreadsheets may be the best option for smaller or more straightforward projects, while systematic review software platforms can provide more robust support for larger or more complicated data.
It is recommended that you pilot your data extraction tool, especially if you will code your data, to determine if fields should be added or clarified, or if the review team needs guidance in collecting and coding data.
Data Extraction Tools
- Excel Excel is the most basic tool for the management of the screening and data extraction stages of the systematic review process. Customized workbooks and spreadsheets can be designed for the review process.
- Covidence Covidence is a software platform built specifically for managing each step of a systematic review project, including data extraction. Read more about how Covidence can help you customize extraction tables and export your extracted data.
- RevMan RevMan is free software used to manage Cochrane reviews. For more information on RevMan, including an explanation of how it may be used to extract and analyze data, watch Introduction to RevMan - a guided tour .
- SRDR SRDR (Systematic Review Data Repository) is a Web-based tool for the extraction and management of data for systematic review or meta-analysis. It is also an open and searchable archive of systematic reviews and their data. Access the help page for more information.
- DistillerSR DistillerSR is a systematic review management software program, similar to Covidence. It guides reviewers in creating project-specific forms, extracting, and analyzing data.
- Sumari JBI Sumari (the Joanna Briggs Institute System for the United Management, Assessment and Review of Information) is a systematic review software platform geared toward fields such as health, social sciences, and humanities. Among the other steps of a review project, it facilitates data extraction and data synthesis. View their short introductions to data extraction and analysis for more information.
- The Systematic Review Toolbox The SR Toolbox is a community-driven, searchable, web-based catalogue of tools that support the systematic review process across multiple domains. Use the advanced search option to restrict to tools specific to data extraction.
Additional Information
These resources offer additional information and examples of data extraction forms:
Brown, S. A., Upchurch, S. L., & Acton, G. J. (2003). A framework for developing a coding scheme for meta-analysis. Western Journal of Nursing Research , 25 (2), 205–222. https://doi.org/10.1177/0193945902250038
Elamin, M. B., Flynn, D. N., Bassler, D., Briel, M., Alonso-Coello, P., Karanicolas, P. J., … Montori, V. M. (2009). Choice of data extraction tools for systematic reviews depends on resources and review complexity. Journal of Clinical Epidemiology , 62 (5), 506–510. https://doi.org/10.1016/j.jclinepi.2008.10.016
Higgins, J.P.T., & Thomas, J. (Eds.) (2022). Cochrane handbook for systematic reviews of interventions Version 6.3. The Cochrane Collaboration. Available from https://training.cochrane.org/handbook/current (see Part 2: Core Methods, Chapters 4, 5)
Research guide from the George Washington University Himmelfarb Health Sciences Library.
- << Previous: 10. Risk of Bias Assessment
- Next: 12. Synthesize, Map, or Describe the Results >>
- Last Updated: Apr 22, 2024 7:04 PM
- URL: https://guides.lib.uci.edu/evidence-synthesis
Off-campus? Please use the Software VPN and choose the group UCIFull to access licensed content. For more information, please Click here
Software VPN is not available for guests, so they may not have access to some content when connecting from off-campus.
Log in using your username and password
- Search More Search for this keyword Advanced search
- Latest content
- Current issue
- BMJ Journals More You are viewing from: Google Indexer
You are here
- Volume 26, Issue 3
- Summarising good practice guidelines for data extraction for systematic reviews and meta-analysis
- Article Text
- Article info
- Citation Tools
- Rapid Responses
- Article metrics

- http://orcid.org/0000-0001-6589-5456 Kathryn S Taylor 1 ,
- http://orcid.org/0000-0002-7791-8552 Kamal R Mahtani 1 ,
- http://orcid.org/0000-0003-1139-655X Jeffrey K Aronson 2
- 1 Nuffield Department of Primary Care Health Sciences , University of Oxford , Oxford , UK
- 2 Centre for Evidence Based Medicine , University of Oxford , Oxford , UK
- Correspondence to Dr Kathryn S Taylor, Nuffield Department of Primary Care Health Sciences, University of Oxford, Oxford OX2 6GG, UK; kathryn.taylor{at}phc.ox.ac.uk
https://doi.org/10.1136/bmjebm-2020-111651
Statistics from Altmetric.com
Request permissions.
If you wish to reuse any or all of this article please use the link below which will take you to the Copyright Clearance Center’s RightsLink service. You will be able to get a quick price and instant permission to reuse the content in many different ways.
Data extraction is the process of a systematic review that occurs between identifying eligible studies and analysing the data, whether it can be a qualitative synthesis or a quantitative synthesis involving the pooling of data in a meta-analysis. The aims of data extraction are to obtain information about the included studies in terms of the characteristics of each study and its population and, for quantitative synthesis, to collect the necessary data to carry out meta-analysis. In systematic reviews, information about the included studies will also be required to conduct risk of bias assessments, but these data are not the focus of this article.
Following good practice when extracting data will help make the process efficient and reduce the risk of errors and bias. Failure to follow good practice risks basing the analysis on poor quality data, and therefore providing poor quality inputs, which will result in poor quality outputs, with unreliable conclusions and invalid study findings. In computer science, this is known as ‘garbage in, garbage out’ or ‘rubbish in, rubbish out’. Furthermore, providing insufficient information about the included studies for readers to be able to assess the generalisability of the findings from a systematic review will undermine the value of the pooled analysis. Such failures will cause your systematic review and meta-analysis to be less useful than it ought to be.
Some guidelines for data extraction are formal, including those described in the Cochrane Handbook for Systematic Reviews of Interventions, 1 the Cochrane Handbook for Diagnostic Test Accuracy Reviews, 2 3 the Preferred Reporting Items for Systematic Reviews and Meta-Analyses (PRISMA) reporting guidelines for systematic reviews and their protocols 4–7 and other sources, 8 9 , formal guidelines are complemented with informal advice in the form of examples and videos on how to avoid possible pitfalls and guidance on how to carry out data extraction more efficiently. 10–12
Guidelines for data extraction involve recommendations for:
Duplication.
Anticipation.
Organisation.
Documentation.
Duplication
Ideally, at least two reviewers should extract data independently, 1 2 9–12 particularly outcome data, 1 as data extraction by only one person can generate errors. 1 13 Data will be extracted from the same sources into identical data extraction forms. If time or resources prevent independent dual extraction, one reviewer should extract the full data and another should independently check the extracted data for both accuracy and completeness. 8 In rapid or restricted reviews, an acceptable level of verification of the data extraction by the first reviewer may be achieved by a second reviewer extracting a random sample of data. 14 Then before comparing the extracted data and seeking a consensus, the extent to which coded (categorical) data extracted by two different reviewers is consistent and may be measured using kappa statistics, 1 2 12 15 or Fleis kappa statistics, when more than two people have extracted the data. 16 Formal comparisons are not routine in Cochrane Reviews, and the Cochrane Handbook recommends that if agreement is to be formally assessed, it should focus only on key outcomes or risk of bias assessments. 1
Anticipation
Disagreement between reviewers when extracting data . Some differences in extracted data are simply due to human error, and such conflicts can be easily resolved. Conflicts and questions about clinical issues, about which data to extract, or whether the relevant data have been reported can be addressed by involving both clinicians and methodologists in data extraction. 3 12 The protocol should set out the strategy for resolving disagreements between reviewers, using consensus and, if necessary, arbitration by another reviewer. If arbitration fails, the study authors should be contacted for clarification. If that is unsuccessful, the disagreement should be documented and reported. 1 6 7
Outcome data being reported in different ways, which are not necessarily suitable for meta-analysis . Many resources are available for helping with data extraction, involving various methods and equations to transform reported data or make estimates. 1 2 10 The protocol may acknowledge this by stating that any estimates made and their justification will be documented and reported.
Including estimates and alternative data . It is also important to anticipate the roles that extracted data will play in the analysis. Studies should be highlighted when multiple sets of outcome data are reported or when estimates have been made in extracting outcome data. 9 Clearly identifying these studies during the data extraction phase will ensure that the studies can be quickly identified later, during the data analysis phase.
Risk of double counting patients . Some studies involve multiple reports, but the study should be the unit of interest. 1 Tracking down multiple reports and ensuring that patients are not double-counted may require good detective skills.
Risk of human error, inconsistency and subjectivity when extracting data . The protocol should state whether data extraction was independent and carried out in duplicate, if a standardised data extraction form was used, and whether it was piloted. The protocol should also state any special instruction, for example, only extracting prespecified eligibility criteria. 1 2 6–9 11 12
Ambiguous or incomplete data . Authors should be contacted to seek clarification about data and make enquiries about the availability of unreported data. 1 2 9 The process of confirming and obtaining data from authors should be prespecified 6 7 including the number of attempts that will be made to make contact, who will be contacted (eg, the first author), and what form the data request will take. Asking for data that are likely to be readily available will reduce the risk of authors offering data with preconditions.
Extracting the right amount of data . Time and resources are wasted extracting data that will not be analysed, such as the language of the publication and the journal name when other extracted data (first author, title and year) adequately identify the publication. The aim of the systematic review will determine which study characteristics are extracted. 16 For example, if the prevalence of a disease is important and is known to vary across cities, the country and city should be extracted. Any assumptions and simplifications should be listed in the protocol. 6 7 The protocol should allow some flexibility for alternative analyses by not over-aggregating data, for example, collecting data on smoking status in categories ‘smoker/ex-smoker/never smoked’ instead of ‘smoker/non-smoker’. 11
Organisation
Guidelines recommend that the process of extracting data should be well organised. This involves having a clear plan, which should feature in the protocol, stating who will extract the data, the actual data that will be extracted, details about the use, development, piloting of a standardised data extraction form 1 6–9 and having good data management procedures, 10 including backing up files frequently. 11 Standardised data extraction forms can provide consistency in a systematic review, while at the same time reducing biases and improving validity and reliability. It may be possible to reuse a form from another review. 12 It is recommended that the data extraction form is piloted and that reviewers receive training in advance 1 2 12 and instructions should be given with extraction forms (eg, about codes and definitions used in the form) to reduce subjectivity and to ensure consistency. 1 2 12 It is recommended that instructions be integrated into the extraction form, so that they are seen each time data are extracted, rather than having instructions in a separate instruction document, which may be ignored or forgotten. 2 Data extraction forms may be paper based or electronic or involve sophisticated data systems. Each approach will have advantages and disadvantages. 1 11 17 For example, using a paper-based form does not require internet access or software skills, but using an electronic extraction form facilitates data analysis. Data systems, while costly, can provide online data storage and automated comparisons between data that have been independently extracted.
Documentation
Data extraction procedures and preanalysis calculations should be well documented 9 10 and based on ‘good bookkeeping’. 5 10 Having good documentation supports accurate reporting, transparency and the ability to scrutinise and replicate the analysis. Reporting guidelines for systematic reviews are provided by PRISMA, 4 5 and these correspond to the set of PRISMA guidelines for protocols of systematic reviews. 6 7 In cases where data are derived from multiple reports, documenting the source of each data item will facilitate the process of resolving disagreements with other reviewers, by enabling the source of conflict to be quickly identified. 10
Data extraction is both time consuming and error-prone, and automation of data extraction is still in its infancy. 1 18 Following both formal and informal guidelines for good practice in data extraction ( table 1 ) will make the process efficient and reduce the risk of errors and bias when extracting data. This will contribute towards ensuring that systematic reviews and meta-analyses are carried out to a high standard.
- View inline
Summarising guidelines for extracting data for systematic reviews meta-analysis
- Higgins JPT ,
- Leeflang MM ,
- Davenport CF ,
- Takwoini Y ,
- Davenport CF
- Liberati A ,
- Tetzlaff J , et al
- Altman DG ,
- Shamseer L ,
- Clarke M , et al
- Centre for Reviews and Dissemination, University of York
- Collaboration for Environmental Evidence
- Dalhousie University
- Buscemi N ,
- Hartling L ,
- Vandermeer B , et al
- Plüddemann A ,
- Aronson JK ,
- Onakpoya I , et al
- Vedula SS ,
- Hadar N , et al
- Jonnalagadda SR ,
Twitter @dataextips
Contributors KST and KRM conceived the idea of the series of which this is one part. KST wrote the first draft of the manuscript. All authors revised the manuscript and agreed the final version.
Funding This research was supported by the National Institute for Health Research Applied Research Collaboration Oxford and Thames Valley at Oxford Health NHS Foundation Trust.
Disclaimer The views expressed in this publication are those of the authors and not necessarily those of the NIHR or the Department of Health and Social Care.
Competing interests KRM and JKA were associate editors of BMJ Evidence Medicine at the time of submission.
Provenance and peer review Commissioned; internally peer reviewed.
Read the full text or download the PDF:
A Guide to Evidence Synthesis: What is Evidence Synthesis?
- Meet Our Team
- Our Published Reviews and Protocols
- What is Evidence Synthesis?
- Types of Evidence Synthesis
- Evidence Synthesis Across Disciplines
- Finding and Appraising Existing Systematic Reviews
- 0. Develop a Protocol
- 1. Draft your Research Question
- 2. Select Databases
- 3. Select Grey Literature Sources
- 4. Write a Search Strategy
- 5. Register a Protocol
- 6. Translate Search Strategies
- 7. Citation Management
- 8. Article Screening
- 9. Risk of Bias Assessment
- 10. Data Extraction
- 11. Synthesize, Map, or Describe the Results
- Evidence Synthesis Institute for Librarians
- Open Access Evidence Synthesis Resources
What are Evidence Syntheses?
What are evidence syntheses.
According to the Royal Society, 'evidence synthesis' refers to the process of bringing together information from a range of sources and disciplines to inform debates and decisions on specific issues. They generally include a methodical and comprehensive literature synthesis focused on a well-formulated research question. Their aim is to identify and synthesize all of the scholarly research on a particular topic, including both published and unpublished studies. Evidence syntheses are conducted in an unbiased, reproducible way to provide evidence for practice and policy-making, as well as to identify gaps in the research. Evidence syntheses may also include a meta-analysis, a more quantitative process of synthesizing and visualizing data retrieved from various studies.
Evidence syntheses are much more time-intensive than traditional literature reviews and require a multi-person research team. See this PredicTER tool to get a sense of a systematic review timeline (one type of evidence synthesis). Before embarking on an evidence synthesis, it's important to clearly identify your reasons for conducting one. For a list of types of evidence synthesis projects, see the next tab.
How Does a Traditional Literature Review Differ From an Evidence Synthesis?
How does a systematic review differ from a traditional literature review.
One commonly used form of evidence synthesis is a systematic review. This table compares a traditional literature review with a systematic review.
Video: Reproducibility and transparent methods (Video 3:25)
Reporting Standards
There are some reporting standards for evidence syntheses. These can serve as guidelines for protocol and manuscript preparation and journals may require that these standards are followed for the review type that is being employed (e.g. systematic review, scoping review, etc).
- PRISMA checklist Preferred Reporting Items for Systematic Reviews and Meta-Analyses (PRISMA) is an evidence-based minimum set of items for reporting in systematic reviews and meta-analyses.
- PRISMA-P Standards An updated version of the original PRISMA standards for protocol development.
- PRISMA - ScR Reporting guidelines for scoping reviews and evidence maps
- PRISMA-IPD Standards Extension of the original PRISMA standards for systematic reviews and meta-analyses of individual participant data.
- EQUATOR Network The EQUATOR (Enhancing the QUAlity and Transparency Of health Research) Network is an international initiative that seeks to improve the reliability and value of published health research literature by promoting transparent and accurate reporting and wider use of robust reporting guidelines. They provide a list of various standards for reporting in systematic reviews.
Video: Guidelines and reporting standards
PRISMA Flow Diagram
The PRISMA flow diagram depicts the flow of information through the different phases of an evidence synthesis. It maps the search (number of records identified), screening (number of records included and excluded), and selection (reasons for exclusion). Many evidence syntheses include a PRISMA flow diagram in the published manuscript.
See below for resources to help you generate your own PRISMA flow diagram.
- PRISMA Flow Diagram Tool
- PRISMA Flow Diagram Word Template
- << Previous: Our Published Reviews and Protocols
- Next: Types of Evidence Synthesis >>
- Last Updated: Apr 24, 2024 2:56 PM
- URL: https://guides.library.cornell.edu/evidence-synthesis

An official website of the United States government
The .gov means it’s official. Federal government websites often end in .gov or .mil. Before sharing sensitive information, make sure you’re on a federal government site.
The site is secure. The https:// ensures that you are connecting to the official website and that any information you provide is encrypted and transmitted securely.
- Publications
- Account settings
Preview improvements coming to the PMC website in October 2024. Learn More or Try it out now .
- Advanced Search
- Journal List
- BMC Med Res Methodol

Overview of data-synthesis in systematic reviews of studies on outcome prediction models
Tobias van den berg.
1 Department of Epidemiology and Biostatistics and the EMGO Institute for Health and Care Research, VU University Medical Centre, Amsterdam, The Netherlands
Martijn W Heymans
Stephanie s leone.
2 Department of General Practice and the EMGO Institute for Health and Care Research, VU University Medical Centre, Amsterdam, The Netherlands
David Vergouw
Jill a hayden.
3 Department of Community Health and Epidemiology, Dalhousie University, Halifax, Nova Scotia, Canada
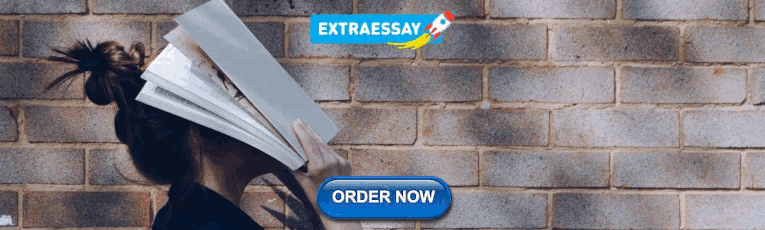
Arianne P Verhagen
4 Department of General Practice, Erasmus Medical Centre, Rotterdam, The Netherlands
Henrica CW de Vet
Many prognostic models have been developed. Different types of models, i.e. prognostic factor and outcome prediction studies, serve different purposes, which should be reflected in how the results are summarized in reviews. Therefore we set out to investigate how authors of reviews synthesize and report the results of primary outcome prediction studies.
Outcome prediction reviews published in MEDLINE between October 2005 and March 2011 were eligible and 127 Systematic reviews with the aim to summarize outcome prediction studies written in English were identified for inclusion.
Characteristics of the reviews and the primary studies that were included were independently assessed by 2 review authors, using standardized forms.
After consensus meetings a total of 50 systematic reviews that met the inclusion criteria were included. The type of primary studies included (prognostic factor or outcome prediction) was unclear in two-thirds of the reviews. A minority of the reviews reported univariable or multivariable point estimates and measures of dispersion from the primary studies. Moreover, the variables considered for outcome prediction model development were often not reported, or were unclear. In most reviews there was no information about model performance. Quantitative analysis was performed in 10 reviews, and 49 reviews assessed the primary studies qualitatively. In both analyses types a range of different methods was used to present the results of the outcome prediction studies.
Conclusions
Different methods are applied to synthesize primary study results but quantitative analysis is rarely performed. The description of its objectives and of the primary studies is suboptimal and performance parameters of the outcome prediction models are rarely mentioned. The poor reporting and the wide variety of data synthesis strategies are prone to influence the conclusions of outcome prediction reviews. Therefore, there is much room for improvement in reviews of outcome prediction studies.
The methodology for prognosis research is still under development. Although there is abundant literature to help researchers perform this type of research [ 1 - 5 ], there is still no widely agreed approach to building a multivariable prediction model [ 6 ]. An important distinction in prognosis is made between prognostic factor models, also called explanatory models and outcome prediction models [ 7 , 8 ]. Prognostic factor studies investigate causal relationships, or pathways between a single (prognostic) factor and an outcome, and focus on the effect size (e.g. relative risk) of this prognostic factor which ideally is adjusted for potential confounders. Outcome prediction studies, on the other hand, combine multiple factors (e.g. clinical and non-clinical patient characteristics) in order to predict future events in individuals, and therefore focus on absolute risks, i.e. predicted probabilities in logistic regression analysis. Methods that can be used to summarize data from prognostic factor studies in a meta-analysis can easily be found in the literature [ 9 , 10 ], but this is not the case for outcome prediction studies. Therefore, in the present study we focus on how authors of published reviews have synthesized outcome prediction models. The nomenclature to indicate various types of prognosis research is not standardized. We use prognosis research as an umbrella term for all research that might explain or predict a future outcome and prognostic factor and outcome prediction as specific types of prognosis studies.
In 2006, Hayden et al. showed that in systematic reviews of prognosis studies, different methods are used to assess the quality of primary studies [ 11 ]. Moreover, when quality is assessed, integration of these quality scores in the synthesis of the review is not guaranteed. For reviews of outcome prediction models, additional characteristics are important in the synthesis of models to reflect choices made in the primary studies, such as which variables are included in statistical models and how this selection was made. These choices therefore also reflect the internal and external validity of a model and influence the predictive performance of the model. In systematic reviews the researchers synthesize results across primary outcome prediction studies which include different variables and show methodological diversity. Moreover, relevant information is not always available, due to poor reporting in the studies. For example, several researchers have found that current knowledge about the recommended number of events per variable, and the coding and selection of variables, among other features, are not always reported in primary outcome prediction research [ 12 - 14 ]. Although improvement in primary studies themselves is needed, reviews that summarize outcome prediction evidence need to consider the current diversity in methodology in primary studies.
In this meta-review we focus on reviews of outcome prediction studies, and how they summarize the characteristics of design and analysis, and the results of primary studies. As there is no guideline nor agreement how primary outcome prediction models in medical research and epidemiology should be summarized in systematic reviews, an overview of current methods helps researchers to improve and develop these methods. Moreover, current methods for outcome prediction reviews are unknown to the research community. Therefore, the aim of this review was to provide an overview on how published reviews of outcome prediction studies describe and summarize the characteristics of the analyses in primary studies, and how the data is synthesized.
Literature search and selection of studies
Systematic reviews and meta-analyses of outcome prediction models that were published between October 2005 and March 2011 were searched. We were only interested in reviews that included multivariable outcome prediction studies. In collaboration with a medical information specialist, we developed a search strategy in MEDLINE, extending on the strategy used by Hayden [ 11 ], by adding recommended other search terms for predictive and prognostic research [ 15 , 16 ]. The full search strategy is presented in Appendix 1.
Based on title and abstract, potential eligible reviews were selected by one author (TvdB), who in case of any doubt included the review. Another author (MH) checked the set of potential eligible reviews. Ineligible reviews were excluded after consensus between both authors. The full texts of the included reviews were read, and if there was any doubt on eligibility a third review author (HdV) was consulted. The inclusion criteria were met if the study design was a systematic review with or without a meta-analysis, multiple variables were studied in an outcome prediction model, and the review was written in the English language. Reviews were excluded if they were based on individual patient data only, or when the topic was genetic profiling.
Data-extraction
A data-extraction form was developed, based on important items to prognosis [ 1 , 2 , 12 , 13 , 17 ], to assess the characteristics of reviews and primary studies and is available from the first author on request. The items on this data-extraction form are shown in Appendix 2. Before the form was finalized it was pilot-tested by all review authors and minor adjustments were made after discussion about the differences in scores. One review author scored all reviews (TvdB) while other review authors (MH, AV, DV, and SL) collectively scored all reviews. Consensus meetings were held within 2 weeks after a review had been scored to solve disagreements. If consensus was not reached, a third reviewer (MH or HdV) was consulted to make a final decision.
An item was scored ‘yes’ if positive information was found about that specific methodological item, e.g. if it was clear that sensitivity analyses were conducted. If it was clear that a specific methodological requirement was not fulfilled, a ‘no’ was scored, e.g. no sensitivity analyses were conducted. In case of doubt or uncertainty, ‘unclear’ was scored. Sometimes, a methodological item could be scored as ‘not applicable’. The number of reviews within a specific answer category was reported, as well as the proportion.
Literature search and selection process
The search strategy revealed 7889 references and, based on title and abstract, 216 were selected to be read in full text (see the flowchart in Figure 1 ). Of these reviews, 89 were excluded and 127 remained. Exclusions after the full text had been read were mainly due to the focus of the research on a single variable with an outcome (prognostic factor study), analysis based on individual patient data only, or a narrative overview study design. After completing the data-extraction, the objectives and methods of 44 reviews described summaries of prognostic factor studies, and 33 reviews had an unclear approach. Therefore, a total of 50 reviews on outcome prediction studies were analyzed [ 18 - 67 ].

Flowchart of the search and selection process.
After completing the data-extraction form for all of the included reviews, most disagreements between review authors were found on items concerning the review objectives, the type of primary studies included, and the method of qualitative data-synthesis. Unclear reporting and, to a lesser degree, reading errors contributed to the disagreements. After consensus meetings only a small proportion of items needed to be discussed with a third reviewer.
Objective and design of the review
Table 1 , section 1 shows the items with regard to information about the reviews. Of the 50 reviews rated as summaries of outcome prediction studies, less than one third included only outcome prediction studies *[ 23 , 27 , 28 , 32 , 35 , 39 , 44 , 48 ],[ 50 , 52 , 55 , 58 , 60 , 66 ]. In about two thirds, the type of primary studies that were included was unclear, and the remaining reviews included a combination of prognostic factor and outcome prediction studies. Most reviews clearly described their outcome of interest. Also information about the assessment of the methodological quality of the primary studies, i.e. risk of bias, was provided in most reviews. In those that did, two thirds described the basic design of the primary studies in addition to a list of methodological criteria (defined in our study as a list consisting of at least four quality items). In some reviews an established criteria list was used or adapted, or a new criteria list was developed. In the reviews that assessed methodological quality, less than half actually used this information to account for differences in study quality, mainly by performing a ‘levels of evidence’ analysis, subgroup-analyses, or sensitivity analyses.
Characteristics of the reviews and provided information about the included primary studies
* includes ‘yes’ and ‘unclear’ categories.
# numbers and percentages may add up to more than 23 and 100%, due to multiple methods in some reviews.
Information about the design and results of the primary studies
In Table 1 , section 2 shows information provided about the included primary studies. The outcome measures used in the included studies were reported in most of the reviews. Only 2 reviews [ 28 , 52 ] described the statistical methods that were used in the primary studies to select variables for inclusion of a final prediction model, e.g. forward or backward selection procedures, and 6 others whether and how patients were treated.
A minority of reviews [ 23 , 24 , 27 , 28 ] described for all studies the variables that were considered for inclusion in the outcome prediction model and only 5 reviews [ 36 , 37 , 39 , 48 , 55 ] reported univariable point estimates (i.e.. regression coefficients or odds ratios) and estimates of dispersion (e.g. standard errors) of all studies. Similarly, multivariable point estimates and estimates of dispersion were reported in respectively 11 and 10 of the reviews [ 21 , 26 , 27 , 31 , 33 , 37 , 44 , 52 ],[ 55 , 64 , 65 ].
With regard to the presentation of univariable and multivariable point estimates, 2 reviews presented both types of results [ 37 , 55 ], 31 did not report any estimates, and 17 reviews were unclear or reported only univariable or multivariable results [not shown in the table]. Lastly, model performance and number of events per variable were reported in 7 reviews [ 32 , 39 , 41 , 60 , 61 , 65 , 66 ] and 4 reviews [ 40 , 48 , 58 , 61 ], respectively.
Data-analysis and synthesis in the reviews
Table 1 , section 3 illustrates how the results of primary studies were summarized in the reviews. It shows that heterogeneity was described in almost all reviews by reporting differences in the study design and the characteristics of the study population. All but one review [ 57 ] summarized the results of included studies in a qualitative manner. Methods that were mainly used for that purpose were number of statistical significant results, consistency of findings, or a combination of these. Quantitative analysis, i.e. statistical pooling, was performed in 10 of the 50 reviews [ 25 , 28 , 31 , 36 , 37 , 44 , 45 , 57 - 59 ]. The quantitative methods used included random effects models and fixed effects models of regression coefficients, odds ratios or hazard ratios. Of these quantitative summaries, 40% assessed the presence of statistical heterogeneity using I 2 , Chi 2 , or the Q statistic. In two reviews [ 25 , 59 ], statistical heterogeneity was found to be present, and subgroup analysis was performed to determine the source of this heterogeneity [results not shown]. In 8 of the reviews there was a graphical presentation of the results, in which a forest plot [ 25 , 28 , 36 - 38 , 52 , 59 ], per single predictor, was the frequently used method. Other studies used a barplot [ 57 ] or a scatterplot [ 38 ]. In 6 reviews [ 25 , 26 , 32 , 43 , 46 , 58 ] a sensitivity analysis was performed to test the robustness of the choices made such as changing the cut-off value for a high or low quality primary study.
We made an overview of how systematic reviews summarize and report the results of primary outcome prediction studies. Specifically, we extracted information on how the data-synthesis was performed in reviews since outcome prediction models may consider different potential predictors, and include a dissimilar set of variables in the final prediction model, and use a variety of statistical methods to obtain an outcome prediction model.
Currently, in prognosis studies a distinction is made between outcome prediction models and prognostic factor models. The methodology of data synthesis in a review of the latter type of prognosis is comparable to the methodology of aetiological reviews. For that reason, in the present study we only focused on reviews of outcome prediction studies. Nonetheless, we found it difficult to distinct between both review types. Less than half of the reviews that we initially selected for data-extraction in fact seemed to serve an outcome prediction purpose. It appeared that the other reviews summarized prognostic factor studies only, or the objective was unclear. In particular, prognostic factor reviews that investigated more than one variable in addition to non-specific objectives made it difficult to clarify what the purpose of reviews was. As a consequence, we might have misclassified some of the 44 excluded reviews rated as prognostic factor. The objective of a review should also include information about the type of study that is included, that is of outcome prediction studies in this case. However, we found that in reviews aimed at outcome prediction the type of primary study was unclear for two-thirds of the reviews. An example we encountered in a review was that their purpose was “to identify preoperative predictive factors for acute post-operative pain and analgesic consumption” although the review authors included any study that identified one or more potential risk factors or predictive factors. The risk of combining both types of studies, i.e. risk factor or prognostic factor studies and predictive factor studies, is that inclusion of potential covariables in the former type are based on change in regression coefficient of the risk factor while in the latter study type all potential predictor variables are included based on their predictive ability of the outcome. This distinction may lead to: 1) biased results in a meta-analysis or other form of evidence synthesis because a risk factor is not always predictive for an outcome and 2) risk factor studies – if adjusted for potential confounders at all – have a slightly different method to obtain a multivariable model compared to outcome prediction studies which may also lead to biased regression coefficients. The distinction between prognostic factor and outcome prediction studies was already emphasized in 1983 by Copas [ 68 ]. He stated that “a method for achieving a good predictor may be quite inappropriate for other questions in regression analysis such as the interpretation of individual regression coefficients”. In other words, the methodology of outcome prediction modelling differs from that of prognostic factor modelling, and therefore combining both types of research into one review to reflect current evidence should be discouraged. Hemingway et al. [ 2 ] appealed for standard nomenclature in prognosis research, and the results of our study underline their plea. Authors of reviews and primary studies should clarify their type of research, for example by using the terms applied by Hayden et al. [ 8 ] ‘prognostic factor modelling’ and ‘outcome prediction modelling’, and give a clear description of their objective.
Studies included in outcome prediction reviews are rarely similar in design and methodology, and this is often neglected when summarizing the evidence. Differences, for instance in the variables studied and the method of analysis for variable selection might explain heterogeneity in results, and should therefore be reported and reflected on when striving to summarize evidence in the most appropriate way. There is no doubt that the methodological quality of primary studies included in reviews is related to the concept of bias [ 69 , 70 ] and it is therefore important to assess this [ 11 , 69 , 70 ]. Dissemination bias reflects if publication bias is likely to be present, how this is handled and what is done to correct for it [ 71 ]. To our knowledge, dissemination bias and especially its consequences in reviews of outcome prediction models are not studied yet. Most likely testimation bias [ 5 ], i.e. the predictors considered and the amount of predictors in relation to the effective sample size influence results more then publication bias. Therefore, we did not study dissemination bias on the review level.
With regard to the reporting of primary study characteristics in the systematic reviews, there is much room for improvement. We found that the methods of model development (e.g. the variables considered and the variable selection methods used) in the primary studies were not, or only vaguely reported in the included reviews. These methods are however important, because variable selection procedures can affect the composition of the multivariable model due to estimation bias, or may result in an increase in model uncertainty [ 72 - 74 ]. Furthermore, the predictive performance of the model can be biased by these methods [ 74 ]. We also found that only 5 of the reviews reported what kind of treatment the patients received in the primary studies. Although prescribed treatment is often not considered as a candidate predictor, it is likely to have a considerable impact on prognosis. Moreover, treatment may vary in relation to predictive variables [ 75 ], and although randomized controlled trials provide patients with similar treatment strategies, in cohort studies which are most often seen in prognosis research this is often not the case. Regardless of difficulties in defining groups that receive the same treatment, it is imperative to consider treatment in outcome prediction models. In order to ensure correct data-synthesis of the results, the primary studies not only should provide point estimates and estimates of dispersion of all the included variables, but also for non-significant findings. Whereas the results of positive or favourable findings are more often reported [ 75 - 78 ], the effects of predictive factors that do not reach statistical significance also need to be compared and summarized in a review. Imagine a variable being of statistical significance in one article, but not reported in others because of non-significance. It is likely that this one significant result is a spurious finding or that the others were underpowered. Without information about the non-significant findings in other studies, biased or even incorrect conclusions might be drawn. This means that reporting of the evidence of primary studies should be accompanied by the results of univariable and multivariable associations, regardless of their level of significance. Moreover, confidence intervals, or other estimates of dispersion are also needed in the review, and unfortunately these results were not presented in most of the reviews in our study. Some reviews considered differences in unadjusted and adjusted results, and the results of one review were sensibly stratified according to univariable and multivariable effects [ 38 ]. Other reviews merely reported multivariable results [ 31 ], or only univariable results if multivariable results were unavailable [ 58 ]. In addition to the multivariable results of a final prediction model, the predictive performance of these models is important for the assessment of clinical usefulness [ 79 ]. A prediction model in itself does not indicate how much variance in outcome is explained by the included variables. Unfortunately, in addition to the non-reporting of several primary study characteristics, the performance of the models was rarely reported in the reviews included in our overview.
Different stages can be distinguished in outcome prediction research [ 80 ]. Most outcome prediction models evaluated in the systematic reviews appeared to be in a developmental phase. Before implementation in daily practice, confirmation of the results in other studies is needed. With this type of validation studies underway, in future reviews we should acknowledge the difference between externally validated models and models from developmental studies, and analyze them separately.
In systematic reviews data can be combined quantitatively, i.e. a meta-analysis can be performed. This was done in 10 of the reviews. All of them combined point estimates (mostly odds ratios, but also a mix of odds ratios, hazard ratios and relative risks) and confidence intervals for single outcome prediction variables. This made it possible to calculate a pooled point estimate, often complemented with confidence intervals [ 81 ]. However, in outcome prediction research we are interested in the estimates of a combination of predictive factors, which makes it possible to calculate absolute risks or probabilities to predict an outcome in individuals [ 82 ]. Even if the relative risk of a variable is statistically significant, it does not provide information about the extent to which this variable is predictive for a particular outcome. The distribution of predictor values, outcome prevalence, and correlations between variables also influences the predictive value of variables within a model [ 83 ]. Effect sizes also provide no information about the amount of variation in outcomes that is explained. In summary: the current quantitative methods seem to be more of an explanatory way of summarizing the available evidence, instead of quantitatively summarizing complete outcome prediction models.
Medline was the only database that was searched for relevant reviews. Our intention was to provide an overview of recently published reviews and not to include all relevant outcome prediction reviews. Within Medline, some eligible reviews may have been missed if their titles and abstracts did not include relevant terms and information. An extensive search strategy was applied and abstracts were screened thoroughly and discussed in case of disagreement. Data-extraction was performed in pairs to prevent reading and interpretation errors. Disagreements mainly occurred when deciding on the objective of a review and the type of primary studies included, due to poor reporting in most of the reviews. This indicates a lack of clarity, explanation and reporting within reviews. Therefore, screening in pairs is a necessity, and standardized criteria should be developed and applied in future studies focusing on such reviews. Consistency in rating on the data-extraction form was enhanced by one review author rating all reviews, with one of the other review authors as second rater. Several items were scored as “no”, but we did not know whether this was a true negative (i.e. leading to bias) or that no information was reported about a particular item. For review authors it is especially difficult to summarize information about primary studies because there may be a lack of information in the studies [ 13 , 14 , 84 ].
Implications
There is still no available methodological procedure for a meta-analysis of regression coefficients of multivariable outcome prediction models. Some authors, such as Riley et al. and Altman [ 81 , 84 ], are of the opinion that it remains practically impossible, due to poor reporting, publication bias, and heterogeneity across studies. However, a considerable number of outcome prediction studies have been published, and it would be useful to integrate this body of evidence into one summary result. Moreover, there is an increase in the number of reviews that are being published. Therefore, there is a need to find the best strategy to integrate the results of primary outcome prediction studies. Consequently, until a method to quantitatively synthesize results has been developed, a sensible qualitative data-synthesis, which takes methodological differences between primary studies into account, is indicated. In summarizing the evidence, differences in methodological items and model-building strategies should be described and taken into account when assessing the overall evidence for outcome prediction. For example, univariable and multivariable results should be described separately, or subgroup analyses should be performed when they are combined. Other items that, in our opinion should be taken into consideration with regard to the data-synthesis are: study quality, variables used for model development, statistical methods used for variable selection procedures, the performance of models, and sufficient cases and non-cases to guarantee adequate study power. Regardless of whether or not these items are taken into consideration in the data-synthesis, we strongly recommend that in reviews they are described for all primary studies included so that readers can also take them into consideration.
In conclusion, poor reporting of relevant information and differences in methodology occur in primary outcome prediction research. Even the predictive ability of the models was rarely reported. This, together with our current inability to pool multivariable outcome prediction models, challenges review authors to make informative reviews of outcome prediction models.
Search strategy: 01-03-2011
Database: MEDLINE
((“systematic review”[tiab] OR “systematic reviews”[tiab] OR “Meta-Analysis as Topic”[Mesh] OR meta-analysis[tiab] OR “Meta-Analysis”[Publication Type]) AND (“2005/11/01”[EDat] : “3000”[EDat]) AND ((“Incidence”[Mesh] OR “Models, Statistical”[Mesh] OR “Mortality”[Mesh] OR “mortality ”[Subheading] OR “Follow-Up Studies”[Mesh] OR “Prognosis”[Mesh:noexp] OR “Disease-Free Survival”[Mesh] OR “Disease Progression”[Mesh:noexp] OR “Natural History”[Mesh] OR “Prospective Studies”[Mesh]) OR ((cohort*[tw] OR course*[tw] OR first episode*[tw] OR predict*[tw] OR predictor*[tw] OR prognos*[tw] OR follow-up stud*[tw] OR inciden*[tw]) NOT medline[sb]))) NOT ((“addresses”[Publication Type] OR “biography”[Publication Type] OR “case reports”[Publication Type] OR “comment”[Publication Type] OR “directory”[Publication Type] OR “editorial”[Publication Type] OR “festschrift”[Publication Type] OR “interview”[Publication Type] OR “lectures”[Publication Type] OR “legal cases”[Publication Type] OR “legislation”[Publication Type] OR “letter”[Publication Type] OR “news”[Publication Type] OR “newspaper article”[Publication Type] OR “patient education handout”[Publication Type] OR “popular works”[Publication Type] OR “congresses”[Publication Type] OR “consensus development conference”[Publication Type] OR “consensus development conference, nih”[Publication Type] OR “practice guideline”[Publication Type]) OR (“Animals”[Mesh] NOT (“Animals”[Mesh] AND “Humans”[Mesh]))).
Items used to assess the characteristics of analyses in outcome prediction primary studies and reviews:
Information about the review:
1. What type of studies are included?
2. Is(/are) the outcome(s) of interest clearly described?
3. Is information about the quality assessment method provided?
a. What method was used?
4. Did the review account for quality?
Information about the analysis of the primary studies:
5. Are the outcome measures clearly described?
6. Is the statistical method used for variable selection described?
7. Is there a description of treatments received provided?
Information about the results of the primary studies:
8. Are crude univariable associations and estimates of dispersion for all the variables of the primary studies presented?
9. Are all variables that were used for model development described?
10. Are the multivariable associations and estimates of dispersions presented?
11. Is model performance assessed and reported?
12. Is the number of predictors relative to the number of outcome events described?
Data-analysis and synthesis of the review:
13. Is the heterogeneity of primary studies described?
14. Is a qualitative synthesis presented?
15. Are methods for quantitative analysis described?
b. Is the statistical heterogeneity assessed?
c. What method is used to assess statistical heterogeneity?
d. If statistical heterogeneity exists, are sources of the heterogeneity investigated?
e. What method is used to investigate potential sources of heterogeneity?
16. Is a graphical presentation of the results provided?
17. Are sensitivity analysis performed?
a. On which level?
Competing interests
All authors report no conflicts of interests.
Authors’ contributions
TvdB, had full access to all of the data in the study and takes responsibility for the integrity of the data and the accuracy of the data analysis. Study concept and design: TvdB, MH, JH, AV, HdV. Acquisition of data: TvdB, MH, SL, DV, AV, HdV Analysis and interpretation of data: TvdB, MH, HdV. Drafting of the manuscript: TvdB, MH, HdV. Critical revision of the manuscript for important intellectual content: TvdB, MH, SL, DV, JH, AV, HdV. Statistical analysis: TvdB Study supervision: MH, HdV. All authors read and approved the final manuscript.
Pre-publication history
The pre-publication history for this paper can be accessed here:
http://www.biomedcentral.com/1471-2288/13/42/prepub
Acknowledgment
We thank Ilse Jansma, MSc, for her contributions as a medical information specialist regarding the Medline search strategy. No compensation was received for her contribution.
No external funding was received for this study.
- Harrell FEJ, Lee KL, Mark DB. Multivariable prognostic models: issues in developing models, evaluating assumptions and adequacy, and measuring and reducing errors. Stat Med. 1996; 15 :361–387. doi: 10.1002/(SICI)1097-0258(19960229)15:4<361::AID-SIM168>3.0.CO;2-4. [ PubMed ] [ CrossRef ] [ Google Scholar ]
- Hemingway H, Riley RD, Altman DG. Ten steps towards improving prognosis research. BMJ. 2009; 339 :b4184. doi: 10.1136/bmj.b4184. [ PubMed ] [ CrossRef ] [ Google Scholar ]
- Moons KGM, Donders AR, Steyerberg EW, Harrell FE. Penalized maximum likelihood estimation to directly adjust diagnostic and prognostic prediction models for overoptimism: a clinical example. J Clin Epidemiol. 2004; 57 :1262–1270. doi: 10.1016/j.jclinepi.2004.01.020. [ PubMed ] [ CrossRef ] [ Google Scholar ]
- Royston P, Altman DG, Sauerbrei W. Dichotomizing continuous predictors in multiple regression: a bad idea. Stat Med. 2006; 25 :127–141. doi: 10.1002/sim.2331. [ PubMed ] [ CrossRef ] [ Google Scholar ]
- Steyerberg EW. Clinical Prediction Models: A Practical Approach to Development, Validation, and Updating. New York: Springer; 2009. [ Google Scholar ]
- Royston P, Moons KGM, Altman DG, Vergouwe Y. Prognosis and prognostic research: Developing a prognostic model. BMJ. 2009; 338 :b604. doi: 10.1136/bmj.b604. [ PubMed ] [ CrossRef ] [ Google Scholar ]
- Moons KGM, Royston P, Vergouwe Y, Grobbee DE, Altman DG. Prognosis and prognostic research: what, why, and how? BMJ. 2009; 338 :b375. doi: 10.1136/bmj.b375. [ PubMed ] [ CrossRef ] [ Google Scholar ]
- Hayden JA, Dunn KM, van der Windt DA, Shaw WS. What is the prognosis of back pain? Best Pract Res Clin Rheumatol. 2010; 24 :167–179. doi: 10.1016/j.berh.2009.12.005. [ PubMed ] [ CrossRef ] [ Google Scholar ]
- Hayden JA, Chou R, Hogg-Johnson S, Bombardier C. Systematic reviews of low back pain prognosis had variable methods and results: guidance for future prognosis reviews. J Clin Epidemiol. 2009; 62 :781–796. doi: 10.1016/j.jclinepi.2008.09.004. [ PubMed ] [ CrossRef ] [ Google Scholar ]
- Krasopoulos G, Brister SJ, Beattie WS, Buchanan MR. Aspirin “resistance” and risk of cardiovascular morbidity: systematic review and meta-analysis. BMJ. 2008; 336 :195–198. doi: 10.1136/bmj.39430.529549.BE. [ PMC free article ] [ PubMed ] [ CrossRef ] [ Google Scholar ]
- Hayden JA, Cote P, Bombardier C. Evaluation of the quality of prognosis studies in systematic reviews. Ann Intern Med. 2006; 144 :427–437. doi: 10.7326/0003-4819-144-6-200603210-00010. [ PubMed ] [ CrossRef ] [ Google Scholar ]
- Mallett S, Timmer A, Sauerbrei W, Altman DG. Reporting of prognostic studies of tumour markers: a review of published articles in relation to REMARK guidelines. Br J Cancer. 2010; 102 :173–180. doi: 10.1038/sj.bjc.6605462. [ PMC free article ] [ PubMed ] [ CrossRef ] [ Google Scholar ]
- Mallett S, Royston P, Waters R, Dutton S, Altman DG. Reporting performance of prognostic models in cancer: a review. BMC Med. 2010; 8 :21. doi: 10.1186/1741-7015-8-21. [ PMC free article ] [ PubMed ] [ CrossRef ] [ Google Scholar ]
- Mallett S, Royston P, Dutton S, Waters R, Altman DG. Reporting methods in studies developing prognostic models in cancer: a review. BMC Med. 2010; 8 :20. doi: 10.1186/1741-7015-8-20. [ PMC free article ] [ PubMed ] [ CrossRef ] [ Google Scholar ]
- Ingui BJ, Rogers MA. Searching for clinical prediction rules in MEDLINE. J Am Med Inform Assoc. 2001; 8 :391–397. doi: 10.1136/jamia.2001.0080391. [ PMC free article ] [ PubMed ] [ CrossRef ] [ Google Scholar ]
- Wilczynski NL. In: PDQ, Evidence-Based Principles and Practice. McKibbon KA, Wilczynski NL, Eady A, Marks S, editor. Shelton, Connecticut: People’s Medical Publishing House; 2009. Natural History and Prognosis. [ Google Scholar ]
- Austin PC, Tu JV. Automated variable selection methods for logistic regression produced unstable models for predicting acute myocardial infarction mortality. J Clin Epidemiol. 2004; 57 :1138–1146. doi: 10.1016/j.jclinepi.2004.04.003. [ PubMed ] [ CrossRef ] [ Google Scholar ]
- Lee M, Chodosh J. Dementia and life expectancy: what do we know? J Am Med Dir Assoc. 2009; 10 :466–471. doi: 10.1016/j.jamda.2009.03.014. [ PubMed ] [ CrossRef ] [ Google Scholar ]
- Gravante G, Garcea G, Ong S. Prediction of Mortality in Acute Pancreatitis: A Systematic Review of the Published Evidence. Pancreatology. 2009; 9 :601–614. doi: 10.1159/000212097. [ PubMed ] [ CrossRef ] [ Google Scholar ]
- Celestin J, Edwards RR, Jamison RN. Pretreatment psychosocial variables as predictors of outcomes following lumbar surgery and spinal cord stimulation: a systematic review and literature synthesis. Pain Med. 2009; 10 :639–653. doi: 10.1111/j.1526-4637.2009.00632.x. [ PubMed ] [ CrossRef ] [ Google Scholar ]
- Wright AA, Cook C, Abbott JH. Variables associated with the progression of hip osteoarthritis: a systematic review. Arthritis Rheum. 2009; 61 :925–936. doi: 10.1002/art.24641. [ PubMed ] [ CrossRef ] [ Google Scholar ]
- Heitz C, Hilfiker R, Bachmann L. Comparison of risk factors predicting return to work between patients with subacute and chronic non-specific low back pain: systematic review. Eur Spine J. 2009; 18 :1829–35. doi: 10.1007/s00586-009-1083-9. [ PMC free article ] [ PubMed ] [ CrossRef ] [ Google Scholar ]
- Sansam K, Neumann V, O’Connor R, Bhakta B. Predicting walking ability following lower limb amputation: a systematic review of the literature. J Rehabil Med. 2009; 41 :593–603. doi: 10.2340/16501977-0393. [ PubMed ] [ CrossRef ] [ Google Scholar ]
- Detaille SI, Heerkens YF, Engels JA, van der Gulden JWJ, van Dijk FJH. Common prognostic factors of work disability among employees with a chronic somatic disease: a systematic review of cohort studies. Scand J Work Environ Health. 2009; 35 :261–281. doi: 10.5271/sjweh.1337. [ PubMed ] [ CrossRef ] [ Google Scholar ]
- Walton DM, Pretty J, MacDermid JC, Teasell RW. Risk factors for persistent problems following whiplash injury: results of a systematic review and meta-analysis. J Orthop Sports Phys Ther. 2009; 39 :334–350. [ PubMed ] [ Google Scholar ]
- van Velzen JM, van Bennekom CAM, Edelaar MJA, Sluiter JK, Frings-Dresen MHW. Prognostic factors of return to work after acquired brain injury: a systematic review. Brain Inj. 2009; 23 :385–395. doi: 10.1080/02699050902838165. [ PubMed ] [ CrossRef ] [ Google Scholar ]
- Borghuis MS, Lucassen PLBJ, van de Laar FA, Speckens AE, van Weel C. olde Hartman TC. Medically unexplained symptoms, somatisation disorder and hypochondriasis: course and prognosis. A systematic review. J Psychosom Res. 2009; 66 :363–377. doi: 10.1016/j.jpsychores.2008.09.018. [ PubMed ] [ CrossRef ] [ Google Scholar ]
- Bramer JAM, van Linge JH, Grimer RJ, Scholten RJPM. Prognostic factors in localized extremity osteosarcoma: a systematic review. Eur J Surg Oncol. 2009; 35 :1030–1036. doi: 10.1016/j.ejso.2009.01.011. [ PubMed ] [ CrossRef ] [ Google Scholar ]
- Tandon P, Garcia-Tsao G. Prognostic indicators in hepatocellular carcinoma: a systematic review of 72 studies. Liver Int. 2009; 29 :502–510. doi: 10.1111/j.1478-3231.2008.01957.x. [ PMC free article ] [ PubMed ] [ CrossRef ] [ Google Scholar ]
- Santaguida PL, Hawker GA, Hudak PL. Patient characteristics affecting the prognosis of total hip and knee joint arthroplasty: a systematic review. Can J Surg. 2008; 51 :428–436. [ PMC free article ] [ PubMed ] [ Google Scholar ]
- Elmunzer BJ, Young SD, Inadomi JM, Schoenfeld P, Laine L. Systematic review of the predictors of recurrent hemorrhage after endoscopic hemostatic therapy for bleeding peptic ulcers. Am J Gastroenterol. 2008; 103 :2625–2632. doi: 10.1111/j.1572-0241.2008.02070.x. [ PubMed ] [ CrossRef ] [ Google Scholar ]
- Adamson SJ, Sellman JD, Frampton CMA. Patient predictors of alcohol treatment outcome: a systematic review. J Subst Abuse Treat. 2009; 36 :75–86. doi: 10.1016/j.jsat.2008.05.007. [ PubMed ] [ CrossRef ] [ Google Scholar ]
- Paez JIG, Costa SF. Risk factors associated with mortality of infections caused by Stenotrophomonas maltophilia: a systematic review. J Hosp Infect. 2008; 70 :101–108. doi: 10.1016/j.jhin.2008.05.020. [ PubMed ] [ CrossRef ] [ Google Scholar ]
- Johnson SR, Swiston JR, Granton JT. Prognostic factors for survival in scleroderma associated pulmonary arterial hypertension. J Rheumatol. 2008; 35 :1584–1590. [ PubMed ] [ Google Scholar ]
- Clarke SA, Eiser C, Skinner R. Health-related quality of life in survivors of BMT for paediatric malignancy: a systematic review of the literature. Bone Marrow Transplant. 2008; 42 :73–82. doi: 10.1038/bmt.2008.156. [ PubMed ] [ CrossRef ] [ Google Scholar ]
- Kok M, Cnossen J, Gravendeel L, van der Post J, Opmeer B, Mol BW. Clinical factors to predict the outcome of external cephalic version: a metaanalysis. Am J Obstet Gynecol. 2008; 199 :630–637. [ PubMed ] [ Google Scholar ]
- Stuart-Harris R, Caldas C, Pinder SE, Pharoah P. Proliferation markers and survival in early breast cancer: a systematic review and meta-analysis of 85 studies in 32,825 patients. Breast. 2008; 17 :323–334. doi: 10.1016/j.breast.2008.02.002. [ PubMed ] [ CrossRef ] [ Google Scholar ]
- Kamper SJ, Rebbeck TJ, Maher CG, McAuley JH, Sterling M. Course and prognostic factors of whiplash: a systematic review and meta-analysis. Pain. 2008; 138 :617–629. doi: 10.1016/j.pain.2008.02.019. [ PubMed ] [ CrossRef ] [ Google Scholar ]
- Nijrolder I, van der Horst H, van der Windt D. Prognosis of fatigue. A systematic review. J Psychosom Res. 2008; 64 :335–349. doi: 10.1016/j.jpsychores.2007.11.001. [ PubMed ] [ CrossRef ] [ Google Scholar ]
- Williams M, Williamson E, Gates S, Lamb S, Cooke M. A systematic literature review of physical prognostic factors for the development of Late Whiplash Syndrome. Spine (Phila Pa 1976) 2007; 32 :E764–E780. doi: 10.1097/BRS.0b013e31815b6565. [ PubMed ] [ CrossRef ] [ Google Scholar ]
- Willemse-van Son AHP, Ribbers GM, Verhagen AP, Stam HJ. Prognostic factors of long-term functioning and productivity after traumatic brain injury: a systematic review of prospective cohort studies. Clin Rehabil. 2007; 21 :1024–1037. doi: 10.1177/0269215507077603. [ PubMed ] [ CrossRef ] [ Google Scholar ]
- Alvarez J, Wilkinson J, Lipshultz S. Outcome Predictors for Pediatric Dilated Cardiomyopathy: A Systematic Review. Prog Pediatr Cardiol. 2007; 23 :25–32. doi: 10.1016/j.ppedcard.2007.05.009. [ PMC free article ] [ PubMed ] [ CrossRef ] [ Google Scholar ]
- Mallen CD, Peat G, Thomas E, Dunn KM, Croft PR. Prognostic factors for musculoskeletal pain in primary care: a systematic review. Br J Gen Pract. 2007; 57 :655–661. [ PMC free article ] [ PubMed ] [ Google Scholar ]
- Stroke Risk in Atrial Fibrillation Working Group. Independent predictors of stroke in patients with atrial fibrillation: a systematic review. Neurology. 2007; 69 :546–554. [ PubMed ] [ Google Scholar ]
- Kent PM, Keating JL. Can we predict poor recovery from recent-onset nonspecific low back pain? A systematic review. Man Ther. 2008; 13 :12–28. doi: 10.1016/j.math.2007.05.009. [ PubMed ] [ CrossRef ] [ Google Scholar ]
- Tjang YS, van Hees Y, Korfer R, Grobbee DE, van der Heijden GJMG. Predictors of mortality after aortic valve replacement. Eur J Cardiothorac Surg. 2007; 32 :469–474. doi: 10.1016/j.ejcts.2007.06.012. [ PubMed ] [ CrossRef ] [ Google Scholar ]
- Pfannschmidt J, Dienemann H, Hoffmann H. Surgical resection of pulmonary metastases from colorectal cancer: a systematic review of published series. Ann Thorac Surg. 2007; 84 :324–338. doi: 10.1016/j.athoracsur.2007.02.093. [ PubMed ] [ CrossRef ] [ Google Scholar ]
- Williamson E, Williams M, Gates S, Lamb SE. A systematic literature review of psychological factors and the development of late whiplash syndrome. Pain. 2008; 135 :20–30. doi: 10.1016/j.pain.2007.04.035. [ PubMed ] [ CrossRef ] [ Google Scholar ]
- Tas U, Verhagen AP, Bierma-Zeinstra SMA, Odding E, Koes BW. Prognostic factors of disability in older people: a systematic review. Br J Gen Pract. 2007; 57 :319–323. [ PMC free article ] [ PubMed ] [ Google Scholar ]
- Rassi AJ, Rassi A, Rassi SG. Predictors of mortality in chronic Chagas disease: a systematic review of observational studies. Circulation. 2007; 115 :1101–1108. doi: 10.1161/CIRCULATIONAHA.106.627265. [ PubMed ] [ CrossRef ] [ Google Scholar ]
- Belo JN, Berger MY, Reijman M, Koes BW, Bierma-Zeinstra SMA. Prognostic factors of progression of osteoarthritis of the knee: a systematic review of observational studies. Arthritis Rheum. 2007; 57 :13–26. doi: 10.1002/art.22475. [ PubMed ] [ CrossRef ] [ Google Scholar ]
- Langer-Gould A, Popat RA, Huang SM. Clinical and demographic predictors of long-term disability in patients with relapsing-remitting multiple sclerosis: a systematic review. Arch Neurol. 2006; 63 :1686–1691. doi: 10.1001/archneur.63.12.1686. [ PubMed ] [ CrossRef ] [ Google Scholar ]
- Lamme B, Mahler CW, van Ruler O, Gouma DJ, Reitsma JB, Boermeester MA. Clinical predictors of ongoing infection in secondary peritonitis: systematic review. World J Surg. 2006; 30 :2170–2181. doi: 10.1007/s00268-005-0333-1. [ PubMed ] [ CrossRef ] [ Google Scholar ]
- van Dijk GM, Dekker J, Veenhof C, van den Ende CHM. Course of functional status and pain in osteoarthritis of the hip or knee: a systematic review of the literature. Arthritis Rheum. 2006; 55 :779–785. doi: 10.1002/art.22244. [ PubMed ] [ CrossRef ] [ Google Scholar ]
- Aalto TJ, Malmivaara A, Kovacs F. Preoperative predictors for postoperative clinical outcome in lumbar spinal stenosis: systematic review. Spine (Phila Pa 1976) 2006; 31 :E648–E663. doi: 10.1097/01.brs.0000231727.88477.da. [ PubMed ] [ CrossRef ] [ Google Scholar ]
- Hauser CA, Stockler MR, Tattersall MHN. Prognostic factors in patients with recently diagnosed incurable cancer: a systematic review. Support Care Cancer. 2006; 14 :999–1011. doi: 10.1007/s00520-006-0079-9. [ PubMed ] [ CrossRef ] [ Google Scholar ]
- Bollen CW, Uiterwaal CSPM, van Vught AJ. Systematic review of determinants of mortality in high frequency oscillatory ventilation in acute respiratory distress syndrome. Crit Care. 2006; 10 :R34. doi: 10.1186/cc4824. [ PMC free article ] [ PubMed ] [ CrossRef ] [ Google Scholar ]
- Steenstra IA, Verbeek JH, Heymans MW, Bongers PM. Prognostic factors for duration of sick leave in patients sick listed with acute low back pain: a systematic review of the literature. Occup Environ Med. 2005; 62 :851–860. doi: 10.1136/oem.2004.015842. [ PMC free article ] [ PubMed ] [ CrossRef ] [ Google Scholar ]
- Bai M, Qi X, Yang Z. Predictors of hepatic encephalopathy after transjugular intrahepatic portosystemic shunt in cirrhotic patients: a systematic review. J Gastroenterol Hepatol. 2011; 26 :943–51. doi: 10.1111/j.1440-1746.2011.06663.x. [ PubMed ] [ CrossRef ] [ Google Scholar ]
- Monteiro-Soares M, Boyko E, Ribeiro J, Ribeiro I, Dinis-Ribeiro M. Risk stratification systems for diabetic foot ulcers: a systematic review. Diabetologia. 2011; 54 :1190–1199. doi: 10.1007/s00125-010-2030-3. [ PubMed ] [ CrossRef ] [ Google Scholar ]
- Lichtman JH, Leifheit-Limson EC, Jones SB. Predictors of hospital readmission after stroke: a systematic review. Stroke. 2010; 41 :2525–2533. doi: 10.1161/STROKEAHA.110.599159. [ PMC free article ] [ PubMed ] [ CrossRef ] [ Google Scholar ]
- Ronden RA, Houben AJ, Kessels AG, Stehouwer CD, de Leeuw PW, Kroon AA. Predictors of clinical outcome after stent placement in atherosclerotic renal artery stenosis: a systematic review and meta-analysis of prospective studies. J Hypertens. 2010; 28 :2370–2377. [ PubMed ] [ Google Scholar ]
- de Jonge RCJ, van Furth AM, Wassenaar M, Gemke RJBJ, Terwee CB. Predicting sequelae and death after bacterial meningitis in childhood: a systematic review of prognostic studies. BMC Infect Dis. 2010; 10 :232. doi: 10.1186/1471-2334-10-232. [ PMC free article ] [ PubMed ] [ CrossRef ] [ Google Scholar ]
- Colohan SM. Predicting prognosis in thermal burns with associated inhalational injury: a systematic review of prognostic factors in adult burn victims. J Burn Care Res. 2010; 31 :529–539. doi: 10.1097/BCR.0b013e3181e4d680. [ PubMed ] [ CrossRef ] [ Google Scholar ]
- Clay FJ, Newstead SV, McClure RJ. A systematic review of early prognostic factors for return to work following acute orthopaedic trauma. Injury. 2010; 41 :787–803. doi: 10.1016/j.injury.2010.04.005. [ PubMed ] [ CrossRef ] [ Google Scholar ]
- Brabrand M, Folkestad L, Clausen NG, Knudsen T, Hallas J. Risk scoring systems for adults admitted to the emergency department: a systematic review. Scand J Trauma Resusc Emerg Med. 2010; 18 :8. doi: 10.1186/1757-7241-18-8. [ PMC free article ] [ PubMed ] [ CrossRef ] [ Google Scholar ]
- Montazeri A. Quality of life data as prognostic indicators of survival in cancer patients: an overview of the literature from 1982 to 2008. Health Qual Life Outcomes. 2009; 7 :102. doi: 10.1186/1477-7525-7-102. [ PMC free article ] [ PubMed ] [ CrossRef ] [ Google Scholar ]
- Copas JB. Prediction and Shrinkage. J R Stat Soc Ser B (methodological) 1983; 45 :311–354. [ Google Scholar ]
- Atkins D, Best D, Briss PA. Grading quality of evidence and strength of recommendations. BMJ. 2004; 328 :1490. [ PMC free article ] [ PubMed ] [ Google Scholar ]
- Deeks JJ, Dinnes J, D’Amico R. Evaluating non-randomised intervention studies. Health Technol Assess. 2003; 7 :iii-173. [ PubMed ] [ Google Scholar ]
- Parekh-Bhurke S, Kwok CS, Pang C. Uptake of methods to deal with publication bias in systematic reviews has increased over time, but there is still much scope for improvement. J Clin Epidemiol. 2011; 64 :349–57. doi: 10.1016/j.jclinepi.2010.04.022. [ PubMed ] [ CrossRef ] [ Google Scholar ]
- Steyerberg EW. Selection of main effects. Clinicical Prediction Models: A Practical Approach to Development, Validation, and Updating. New York: Springer; 2009. [ Google Scholar ]
- Chatfield C. Model Uncertainty, Data Mining and Statistical Inference. J R Stat Soc Ser A. 1995; 158 :419–466. doi: 10.2307/2983440. [ CrossRef ] [ Google Scholar ]
- Steyerberg EW, Eijkemans MJ, Habbema JD. Stepwise selection in small data sets: a simulation study of bias in logistic regression analysis. J Clin Epidemiol. 1999; 52 :935–942. doi: 10.1016/S0895-4356(99)00103-1. [ PubMed ] [ CrossRef ] [ Google Scholar ]
- Altman DG. Systematic reviews of evaluations of prognostic variables. BMJ. 2001; 323 :224–228. doi: 10.1136/bmj.323.7306.224. [ PMC free article ] [ PubMed ] [ CrossRef ] [ Google Scholar ]
- Kyzas PA, Ioannidis JPA. axa-Kyza D. Quality of reporting of cancer prognostic marker studies: association with reported prognostic effect. J Natl Cancer Inst. 2007; 99 :236–243. doi: 10.1093/jnci/djk032. [ PubMed ] [ CrossRef ] [ Google Scholar ]
- Kyzas PA, Ioannidis JPA. axa-Kyza D. Almost all articles on cancer prognostic markers report statistically significant results. Eur J Cancer. 2007; 43 :2559–2579. doi: 10.1016/j.ejca.2007.08.030. [ PubMed ] [ CrossRef ] [ Google Scholar ]
- Rifai N, Altman DG, Bossuyt PM. Reporting bias in diagnostic and prognostic studies: time for action. Clin Chem. 2008; 54 :1101–1103. doi: 10.1373/clinchem.2008.108993. [ PubMed ] [ CrossRef ] [ Google Scholar ]
- Vergouwe Y, Steyerberg EW, Eijkemans MJC, Habbema JD. Validity of prognostic models: when is a model clinically useful? Semin Urol Oncol. 2002; 20 :96–107. doi: 10.1053/suro.2002.32521. [ PubMed ] [ CrossRef ] [ Google Scholar ]
- Altman DG, Vergouwe Y, Royston P, Moons KGM. Prognosis and prognostic research: validating a prognostic model. BMJ. 2009; 338 :b605. doi: 10.1136/bmj.b605. [ PubMed ] [ CrossRef ] [ Google Scholar ]
- Altman DG. In: Systematic Reviews in Health Care. Egger M, Smith GD, Altman DG, editor. London: BMJ Publishing Group; 2001. Systematic reviews of evaluations of prognostic variables; pp. 228–47. [ Google Scholar ]
- Ware JH. The limitations of risk factors as prognostic tools. N Engl J Med. 2006; 355 :2615–2617. doi: 10.1056/NEJMp068249. [ PubMed ] [ CrossRef ] [ Google Scholar ]
- Harrell FE. Multivariable modeling strategies. Regression modeling strategies with applications to linear models, logistic regression, and survival analysis . New York: Springer; 2001. [ Google Scholar ]
- Riley RD, Abrams KR, Sutton AJ. Reporting of prognostic markers: current problems and development of guidelines for evidence-based practice in the future. Br J Cancer. 2003; 88 :1191–1198. doi: 10.1038/sj.bjc.6600886. [ PMC free article ] [ PubMed ] [ CrossRef ] [ Google Scholar ]

Teaching and Research guides
Systematic reviews.
- Starting the review
- About systematic reviews
- Research question
- Plan your search
- Sources to search
- Search example
- Screen and analyse
What is synthesis?
Quantitative synthesis (meta-analysis), qualitative synthesis.
- Guides and software
- Further help
Synthesis is a stage in the systematic review process where extracted data (findings of individual studies) are combined and evaluated. The synthesis part of a systematic review will determine the outcomes of the review.
There are two commonly accepted methods of synthesis in systematic reviews:
- Quantitative data synthesis
- Qualitative data synthesis
The way the data is extracted from your studies and synthesised and presented depends on the type of data being handled.
If you have quantitative information, some of the more common tools used to summarise data include:
- grouping of similar data, i.e. presenting the results in tables
- charts, e.g. pie-charts
- graphical displays such as forest plots
If you have qualitative information, some of the more common tools used to summarise data include:
- textual descriptions, i.e. written words
- thematic or content analysis
Whatever tool/s you use, the general purpose of extracting and synthesising data is to show the outcomes and effects of various studies and identify issues with methodology and quality. This means that your synthesis might reveal a number of elements, including:
- overall level of evidence
- the degree of consistency in the findings
- what the positive effects of a drug or treatment are, and what these effects are based on
- how many studies found a relationship or association between two things
In a quantitative systematic review, data is presented statistically. Typically, this is referred to as a meta-analysis .
The usual method is to combine and evaluate data from multiple studies. This is normally done in order to draw conclusions about outcomes, effects, shortcomings of studies and/or applicability of findings.
Remember, the data you synthesise should relate to your research question and protocol (plan). In the case of quantitative analysis, the data extracted and synthesised will relate to whatever method was used to generate the research question (e.g. PICO method), and whatever quality appraisals were undertaken in the analysis stage.
One way of accurately representing all of your data is in the form of a f orest plot . A forest plot is a way of combining results of multiple clinical trials in order to show point estimates arising from different studies of the same condition or treatment.
It is comprised of a graphical representation and often also a table. The graphical display shows the mean value for each trial and often with a confidence interval (the horizontal bars). Each mean is plotted relative to the vertical line of no difference.
- Forest Plots - Understanding a Meta-Analysis in 5 Minutes or Less (5:38 min) In this video, Dr. Maureen Dobbins, Scientific Director of the National Collaborating Centre for Methods and Tools, uses an example from social health to explain how to construct a forest plot graphic.
- How to interpret a forest plot (5:32 min) In this video, Terry Shaneyfelt, Clinician-educator at UAB School of Medicine, talks about how to interpret information contained in a typical forest plot, including table data.
- An introduction to meta-analysis (13 mins) Dr Christopher J. Carpenter introduces the concept of meta-analysis, a statistical approach to finding patterns and trends among research studies on the same topic. Meta-analysis allows the researcher to weight study results based on size, moderating variables, and other factors.
Journal articles
- Neyeloff, J. L., Fuchs, S. C., & Moreira, L. B. (2012). Meta-analyses and Forest plots using a microsoft excel spreadsheet: step-by-step guide focusing on descriptive data analysis. BMC Research Notes, 5(1), 52-57. https://doi.org/10.1186/1756-0500-5-52 Provides a step-by-step guide on how to use Excel to perform a meta-analysis and generate forest plots.
- Ried, K. (2006). Interpreting and understanding meta-analysis graphs: a practical guide. Australian Family Physician, 35(8), 635- 638. This article provides a practical guide to appraisal of meta-analysis graphs, and has been developed as part of the Primary Health Care Research Evaluation Development (PHCRED) capacity building program for training general practitioners and other primary health care professionals in research methodology.
In a qualitative systematic review, data can be presented in a number of different ways. A typical procedure in the health sciences is thematic analysis .
As explained by James Thomas and Angela Harden (2008) in an article for BMC Medical Research Methodology :
"Thematic synthesis has three stages:
- the coding of text 'line-by-line'
- the development of 'descriptive themes'
- and the generation of 'analytical themes'
While the development of descriptive themes remains 'close' to the primary studies, the analytical themes represent a stage of interpretation whereby the reviewers 'go beyond' the primary studies and generate new interpretive constructs, explanations or hypotheses" (p. 45).
A good example of how to conduct a thematic analysis in a systematic review is the following journal article by Jorgensen et al. (2108) on cancer patients. In it, the authors go through the process of:
(a) identifying and coding information about the selected studies' methodologies and findings on patient care
(b) organising these codes into subheadings and descriptive categories
(c) developing these categories into analytical themes
Jørgensen, C. R., Thomsen, T. G., Ross, L., Dietz, S. M., Therkildsen, S., Groenvold, M., Rasmussen, C. L., & Johnsen, A. T. (2018). What facilitates “patient empowerment” in cancer patients during follow-up: A qualitative systematic review of the literature. Qualitative Health Research, 28(2), 292-304. https://doi.org/10.1177/1049732317721477
Thomas, J., & Harden, A. (2008). Methods for the thematic synthesis of qualitative research in systematic reviews. BMC Medical Research Methodology, 8(1), 45-54. https://doi.org/10.1186/1471-2288-8-45
- << Previous: Screen and analyse
- Next: Write >>

- Last Updated: Apr 12, 2024 1:34 PM
- URL: https://rmit.libguides.com/systematicreviews
Data Extraction and Synthesis in Systematic Reviews of Diagnostic Test Accuracy: A Corpus for Automating and Evaluating the Process
Affiliations.
- 1 LIMSI, CNRS, Université Paris Saclay, F-91405 Orsay.
- 2 Academic Medical Center, University of Amsterdam, Amsterdam, the Netherlands.
- PMID: 30815124
- PMCID: PMC6371350
Background: Systematic reviews are critical for obtaining accurate estimates of diagnostic test accuracy, yet these require extracting information buried in free text articles, an often laborious process.
Objective: We create a dataset describing the data extraction and synthesis processes in 63 DTA systematic reviews, and demonstrate its utility by using it to replicate the data synthesis in the original reviews.
Method: We construct our dataset using a custom automated extraction pipeline complemented with manual extraction, verification, and post-editing. We evaluate using manual assessment by two annotators and by comparing against data extracted from source files.
Results: The constructed dataset contains 5,848 test results for 1,354 diagnostic tests from 1,738 diagnostic studies. We observe an extraction error rate of 0.06-0.3%.
Conclusions: This constitutes the first dataset describing the later stages of the DTA systematic review process, and is intended to be useful for automating or evaluating the process.
Publication types
- Research Support, Non-U.S. Gov't
- Datasets as Topic*
- Diagnostic Tests, Routine*
- Information Storage and Retrieval*
- Systematic Reviews as Topic*
Writing in the Health and Social Sciences: Literature Reviews and Synthesis Tools
- Journal Publishing
- Style and Writing Guides
- Readings about Writing
- Citing in APA Style This link opens in a new window
- Resources for Dissertation Authors
- Citation Management and Formatting Tools
- What are Literature Reviews?
- Conducting & Reporting Systematic Reviews
- Finding Systematic Reviews
- Tutorials & Tools for Literature Reviews
Systematic Literature Reviews: Steps & Resources
These steps for conducting a systematic literature review are listed below .
Also see subpages for more information about:
- The different types of literature reviews, including systematic reviews and other evidence synthesis methods
- Tools & Tutorials
Literature Review & Systematic Review Steps
- Develop a Focused Question
- Scope the Literature (Initial Search)
- Refine & Expand the Search
- Limit the Results
- Download Citations
- Abstract & Analyze
- Create Flow Diagram
- Synthesize & Report Results
1. Develop a Focused Question
Consider the PICO Format: Population/Problem, Intervention, Comparison, Outcome
Focus on defining the Population or Problem and Intervention (don't narrow by Comparison or Outcome just yet!)
"What are the effects of the Pilates method for patients with low back pain?"
Tools & Additional Resources:
- PICO Question Help
- Stillwell, Susan B., DNP, RN, CNE; Fineout-Overholt, Ellen, PhD, RN, FNAP, FAAN; Melnyk, Bernadette Mazurek, PhD, RN, CPNP/PMHNP, FNAP, FAAN; Williamson, Kathleen M., PhD, RN Evidence-Based Practice, Step by Step: Asking the Clinical Question, AJN The American Journal of Nursing : March 2010 - Volume 110 - Issue 3 - p 58-61 doi: 10.1097/01.NAJ.0000368959.11129.79
2. Scope the Literature
A "scoping search" investigates the breadth and/or depth of the initial question or may identify a gap in the literature.
Eligible studies may be located by searching in:
- Background sources (books, point-of-care tools)
- Article databases
- Trial registries
- Grey literature
- Cited references
- Reference lists
When searching, if possible, translate terms to controlled vocabulary of the database. Use text word searching when necessary.
Use Boolean operators to connect search terms:
- Combine separate concepts with AND (resulting in a narrower search)
- Connecting synonyms with OR (resulting in an expanded search)
Search: pilates AND ("low back pain" OR backache )
Video Tutorials - Translating PICO Questions into Search Queries
- Translate Your PICO Into a Search in PubMed (YouTube, Carrie Price, 5:11)
- Translate Your PICO Into a Search in CINAHL (YouTube, Carrie Price, 4:56)
3. Refine & Expand Your Search
Expand your search strategy with synonymous search terms harvested from:
- database thesauri
- reference lists
- relevant studies
Example:
(pilates OR exercise movement techniques) AND ("low back pain" OR backache* OR sciatica OR lumbago OR spondylosis)
As you develop a final, reproducible strategy for each database, save your strategies in a:
- a personal database account (e.g., MyNCBI for PubMed)
- Log in with your NYU credentials
- Open and "Make a Copy" to create your own tracker for your literature search strategies
4. Limit Your Results
Use database filters to limit your results based on your defined inclusion/exclusion criteria. In addition to relying on the databases' categorical filters, you may also need to manually screen results.
- Limit to Article type, e.g.,: "randomized controlled trial" OR multicenter study
- Limit by publication years, age groups, language, etc.
NOTE: Many databases allow you to filter to "Full Text Only". This filter is not recommended . It excludes articles if their full text is not available in that particular database (CINAHL, PubMed, etc), but if the article is relevant, it is important that you are able to read its title and abstract, regardless of 'full text' status. The full text is likely to be accessible through another source (a different database, or Interlibrary Loan).
- Filters in PubMed
- CINAHL Advanced Searching Tutorial
5. Download Citations
Selected citations and/or entire sets of search results can be downloaded from the database into a citation management tool. If you are conducting a systematic review that will require reporting according to PRISMA standards, a citation manager can help you keep track of the number of articles that came from each database, as well as the number of duplicate records.
In Zotero, you can create a Collection for the combined results set, and sub-collections for the results from each database you search. You can then use Zotero's 'Duplicate Items" function to find and merge duplicate records.

- Citation Managers - General Guide
6. Abstract and Analyze
- Migrate citations to data collection/extraction tool
- Screen Title/Abstracts for inclusion/exclusion
- Screen and appraise full text for relevance, methods,
- Resolve disagreements by consensus
Covidence is a web-based tool that enables you to work with a team to screen titles/abstracts and full text for inclusion in your review, as well as extract data from the included studies.

- Covidence Support
- Critical Appraisal Tools
- Data Extraction Tools
7. Create Flow Diagram
The PRISMA (Preferred Reporting Items for Systematic reviews and Meta-Analyses) flow diagram is a visual representation of the flow of records through different phases of a systematic review. It depicts the number of records identified, included and excluded. It is best used in conjunction with the PRISMA checklist .

Example from: Stotz, S. A., McNealy, K., Begay, R. L., DeSanto, K., Manson, S. M., & Moore, K. R. (2021). Multi-level diabetes prevention and treatment interventions for Native people in the USA and Canada: A scoping review. Current Diabetes Reports, 2 (11), 46. https://doi.org/10.1007/s11892-021-01414-3
- PRISMA Flow Diagram Generator (ShinyApp.io, Haddaway et al. )
- PRISMA Diagram Templates (Word and PDF)
- Make a copy of the file to fill out the template
- Image can be downloaded as PDF, PNG, JPG, or SVG
- Covidence generates a PRISMA diagram that is automatically updated as records move through the review phases
8. Synthesize & Report Results
There are a number of reporting guideline available to guide the synthesis and reporting of results in systematic literature reviews.
It is common to organize findings in a matrix, also known as a Table of Evidence (ToE).
- Reporting Guidelines for Systematic Reviews
- Download a sample template of a health sciences review matrix (GoogleSheets)
Steps modified from:
Cook, D. A., & West, C. P. (2012). Conducting systematic reviews in medical education: a stepwise approach. Medical Education , 46 (10), 943–952.
- << Previous: Citation Management and Formatting Tools
- Next: What are Literature Reviews? >>
- Last Updated: Apr 13, 2024 9:10 PM
- URL: https://guides.nyu.edu/healthwriting
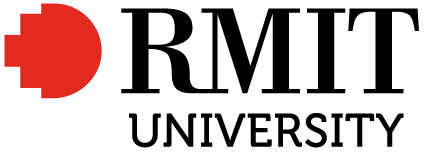
Want to create or adapt books like this? Learn more about how Pressbooks supports open publishing practices.
Synthesising the data
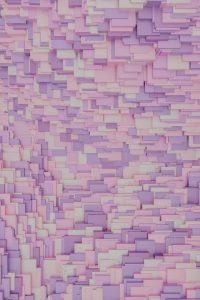
Synthesis is a stage in the systematic review process where extracted data, that is the findings of individual studies, are combined and evaluated.
The general purpose of extracting and synthesising data is to show the outcomes and effects of various studies, and to identify issues with methodology and quality. This means that your synthesis might reveal several elements, including:
- overall level of evidence
- the degree of consistency in the findings
- what the positive effects of a drug or treatment are , and what these effects are based on
- how many studies found a relationship or association between two components, e.g. the impact of disability-assistance animals on the psychological health of workplaces
There are two commonly accepted methods of synthesis in systematic reviews:
Qualitative data synthesis
- Quantitative data synthesis (i.e. meta-analysis)
The way the data is extracted from your studies, then synthesised and presented, depends on the type of data being handled.
In a qualitative systematic review, data can be presented in a number of different ways. A typical procedure in the health sciences is thematic analysis .
Thematic synthesis has three stages:
- the coding of text ‘line-by-line’
- the development of ‘descriptive themes’
- and the generation of ‘analytical themes’
If you have qualitative information, some of the more common tools used to summarise data include:
- textual descriptions, i.e. written words
- thematic or content analysis
Example qualitative systematic review
A good example of how to conduct a thematic analysis in a systematic review is the following journal article on cancer patients. In it, the authors go through the process of:
- identifying and coding information about the selected studies’ methodologies and findings on patient care
- organising these codes into subheadings and descriptive categories
- developing these categories into analytical themes
What Facilitates “Patient Empowerment” in Cancer Patients During Follow-Up: A Qualitative Systematic Review of the Literature
Quantitative data synthesis
In a quantitative systematic review, data is presented statistically. Typically, this is referred to as a meta-analysis .
The usual method is to combine and evaluate data from multiple studies. This is normally done in order to draw conclusions about outcomes, effects, shortcomings of studies and/or applicability of findings.
Remember, the data you synthesise should relate to your research question and protocol (plan). In the case of quantitative analysis, the data extracted and synthesised will relate to whatever method was used to generate the research question (e.g. PICO method), and whatever quality appraisals were undertaken in the analysis stage.
If you have quantitative information, some of the more common tools used to summarise data include:
- grouping of similar data, i.e. presenting the results in tables
- charts, e.g. pie-charts
- graphical displays, i.e. forest plots
Example of a quantitative systematic review
A quantitative systematic review is a combination of qualitative and quantitative, usually referred to as a meta-analysis.
Effectiveness of Acupuncturing at the Sphenopalatine Ganglion Acupoint Alone for Treatment of Allergic Rhinitis: A Systematic Review and Meta-Analysis
About meta-analyses
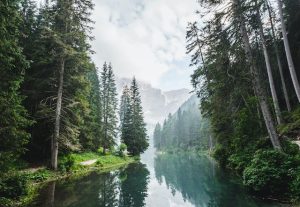
A systematic review may sometimes include a meta-analysis , although it is not a requirement of a systematic review. Whereas, a meta-analysis also includes a systematic review.
A meta-analysis is a statistical analysis that combines data from previous studies to calculate an overall result.
One way of accurately representing all the data is in the form of a forest plot . A forest plot is a way of combining the results of multiple studies in order to show point estimates arising from different studies of the same condition or treatment.
It is comprised of a graphical representation and often also a table. The graphical display shows the mean value for each study and often with a confidence interval (the horizontal bars). Each mean is plotted relative to the vertical line of no difference.
The following is an example of the graphical representation of a forest plot.
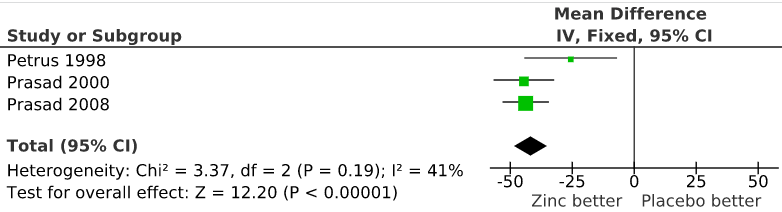
“File:The effect of zinc acetate lozenges on the duration of the common cold.svg” by Harri Hemilä is licensed under CC BY 3.0
Watch the following short video where a social health example is used to explain how to construct a forest plot graphic.
Forest Plots: Understanding a Meta-Analysis in 5 Minutes or Less (5:38 mins)
Forest Plots – Understanding a Meta-Analysis in 5 Minutes or Less (5:38 min) by The NCCMT ( YouTube )
Test your knowledge
Research and Writing Skills for Academic and Graduate Researchers Copyright © 2022 by RMIT University is licensed under a Creative Commons Attribution-NonCommercial 4.0 International License , except where otherwise noted.
Share This Book
Systematic Reviews and Meta-Analyses: Home
- Get Started
- Exploratory Search
- Where to Search
- How to Search
- Grey Literature
- What about errata and retractions?
- Eligibility Screening
- Critical Appraisal
- Data Extraction
- Synthesis & Discussion
- Assess Certainty
- Share & Archive
Welcome! This guide is designed to help novice and experienced review teams navigate the systematic review and/or meta-analysis process.
Guide Sections - Table of Contents
If you're new to this methodology, check out the video and resources below. Each tab above contains more detail about the respective topic.
Table of Contents for this Guide
Get Started | Reporting guidelines and methodological guidance, team formation, finding existing reviews.
Define Scope | F ormation of a clear research question and eligibility criteria; contains subpage for exploratory searching .
Protocol | Introduction to protocol purpose, development, registration.
Comprehensive Search | Contains subpages where to search , how to search , grey literature , and errata/retractions .
Eligibility Screening | Title and abstract screening, full-text review, interrater reliability, and resolving disagreements.
Critical Appraisal | Risk of bias assessment purpose, tools, and presentation.
Data Extraction | Data extraction execution, and presentation.
Synthesis & Discussion | Qualitative synthesis, meta-analysis, and discussion
Assess Certainty | Assessing certainty of evidence using formal methods.
Share & Archive | Repositories to share supplemental material.
Help & Training | Evidence Synthesis Services support and events; additional support outside of the VT Libraries; contains subpage tools .
What is a systematic review and/or meta-analysis?
A review of a clearly formulated question that uses systematic and explicit methods to identify , select , and critically appraise relevant research, and to collect and analyse data from the studies that are included in the review. Statistical methods ( meta-analysis ) may or may not be used to analyse and summarise the results of the included studies.
- Cochrane Collaboration Definition
Considerations before you start.

Has the review already been done or is a review currently underway ? (no need to duplicate if a review exists or is in-progress)
Do you have the resource capacity? (e.g., a team of 3 or more people, time to commit to a months or years long review?)
If a systematic review and/or meta-analysis is not the best option, you may consider alternative evidence synthesis approaches !
Cornerstones
Cornerstones of the systematic review and/or meta-analysis.

According to Wormald & Evans (2018) , the systematic review differs from a subjective, traditional literature review approach in that:
A systematic review is a reproducible piece of observational research and should have a protocol that sets out explicitly objective methods for the conduct of the review, particularly focusing on the control of error , both from bias and the reduction of random error through meta-analysis. Especially important in a systematic review is the objective , methodologically sound and reproducible retrieval of the evidence using...search strategies devised by a trained and experienced information scientist .
Note: This site will continue to evolve and develop through community driven collaboration with information retrieval and evidence synthesis experts across many disciplines. If you've found a broken link or have suggestions for the guide, please reach out to [email protected] .

- Next: Get Started >>
- Last Updated: Apr 12, 2024 12:41 PM
- URL: https://guides.lib.vt.edu/SRMA
- Open access
- Published: 23 April 2024
Designing feedback processes in the workplace-based learning of undergraduate health professions education: a scoping review
- Javiera Fuentes-Cimma 1 , 2 ,
- Dominique Sluijsmans 3 ,
- Arnoldo Riquelme 4 ,
- Ignacio Villagran ORCID: orcid.org/0000-0003-3130-8326 1 ,
- Lorena Isbej ORCID: orcid.org/0000-0002-4272-8484 2 , 5 ,
- María Teresa Olivares-Labbe 6 &
- Sylvia Heeneman 7
BMC Medical Education volume 24 , Article number: 440 ( 2024 ) Cite this article
172 Accesses
Metrics details
Feedback processes are crucial for learning, guiding improvement, and enhancing performance. In workplace-based learning settings, diverse teaching and assessment activities are advocated to be designed and implemented, generating feedback that students use, with proper guidance, to close the gap between current and desired performance levels. Since productive feedback processes rely on observed information regarding a student's performance, it is imperative to establish structured feedback activities within undergraduate workplace-based learning settings. However, these settings are characterized by their unpredictable nature, which can either promote learning or present challenges in offering structured learning opportunities for students. This scoping review maps literature on how feedback processes are organised in undergraduate clinical workplace-based learning settings, providing insight into the design and use of feedback.
A scoping review was conducted. Studies were identified from seven databases and ten relevant journals in medical education. The screening process was performed independently in duplicate with the support of the StArt program. Data were organized in a data chart and analyzed using thematic analysis. The feedback loop with a sociocultural perspective was used as a theoretical framework.
The search yielded 4,877 papers, and 61 were included in the review. Two themes were identified in the qualitative analysis: (1) The organization of the feedback processes in workplace-based learning settings, and (2) Sociocultural factors influencing the organization of feedback processes. The literature describes multiple teaching and assessment activities that generate feedback information. Most papers described experiences and perceptions of diverse teaching and assessment feedback activities. Few studies described how feedback processes improve performance. Sociocultural factors such as establishing a feedback culture, enabling stable and trustworthy relationships, and enhancing student feedback agency are crucial for productive feedback processes.
Conclusions
This review identified concrete ideas regarding how feedback could be organized within the clinical workplace to promote feedback processes. The feedback encounter should be organized to allow follow-up of the feedback, i.e., working on required learning and performance goals at the next occasion. The educational programs should design feedback processes by appropriately planning subsequent tasks and activities. More insight is needed in designing a full-loop feedback process, in which specific attention is needed in effective feedforward practices.
Peer Review reports
The design of effective feedback processes in higher education has been important for educators and researchers and has prompted numerous publications discussing potential mechanisms, theoretical frameworks, and best practice examples over the past few decades. Initially, research on feedback primarily focused more on teachers and feedback delivery, and students were depicted as passive feedback recipients [ 1 , 2 , 3 ]. The feedback conversation has recently evolved to a more dynamic emphasis on interaction, sense-making, outcomes in actions, and engagement with learners [ 2 ]. This shift aligns with utilizing the feedback process as a form of social interaction or dialogue to enhance performance [ 4 ]. Henderson et al. (2019) defined feedback processes as "where the learner makes sense of performance-relevant information to promote their learning." (p. 17). When a student grasps the information concerning their performance in connection to the desired learning outcome and subsequently takes suitable action, a feedback loop is closed so the process can be regarded as successful [ 5 , 6 ].
Hattie and Timperley (2007) proposed a comprehensive perspective on feedback, the so-called feedback loop, to answer three key questions: “Where am I going? “How am I going?” and “Where to next?” [ 7 ]. Each question represents a key dimension of the feedback loop. The first is the feed-up, which consists of setting learning goals and sharing clear objectives of learners' performance expectations. While the concept of the feed-up might not be consistently included in the literature, it is considered to be related to principles of effective feedback and goal setting within educational contexts [ 7 , 8 ]. Goal setting allows students to focus on tasks and learning, and teachers to have clear intended learning outcomes to enable the design of aligned activities and tasks in which feedback processes can be embedded [ 9 ]. Teachers can improve the feed-up dimension by proposing clear, challenging, but achievable goals [ 7 ]. The second dimension of the feedback loop focuses on feedback and aims to answer the second question by obtaining information about students' current performance. Different teaching and assessment activities can be used to obtain feedback information, and it can be provided by a teacher or tutor, a peer, oneself, a patient, or another coworker. The last dimension of the feedback loop is the feedforward, which is specifically associated with using feedback to improve performance or change behaviors [ 10 ]. Feedforward is crucial in closing the loop because it refers to those specific actions students must take to reduce the gap between current and desired performance [ 7 ].
From a sociocultural perspective, feedback processes involve a social practice consisting of intricate relationships within a learning context [ 11 ]. The main feature of this approach is that students learn from feedback only when the feedback encounter includes generating, making sense of, and acting upon the information given [ 11 ]. In the context of workplace-based learning (WBL), actionable feedback plays a crucial role in enabling learners to leverage specific feedback to enhance their performance, skills, and conceptual understandings. The WBL environment provides students with a valuable opportunity to gain hands-on experience in authentic clinical settings, in which students work more independently on real-world tasks, allowing them to develop and exhibit their competencies [ 3 ]. However, WBL settings are characterized by their unpredictable nature, which can either promote self-directed learning or present challenges in offering structured learning opportunities for students [ 12 ]. Consequently, designing purposive feedback opportunities within WBL settings is a significant challenge for clinical teachers and faculty.
In undergraduate clinical education, feedback opportunities are often constrained due to the emphasis on clinical work and the absence of dedicated time for teaching [ 13 ]. Students are expected to perform autonomously under supervision, ideally achieved by giving them space to practice progressively and providing continuous instances of constructive feedback [ 14 ]. However, the hierarchy often present in clinical settings places undergraduate students in a dependent position, below residents and specialists [ 15 ]. Undergraduate or junior students may have different approaches to receiving and using feedback. If their priority is meeting the minimum standards given pass-fail consequences and acting merely as feedback recipients, other incentives may be needed to engage with the feedback processes because they will need more learning support [ 16 , 17 ]. Adequate supervision and feedback have been recognized as vital educational support in encouraging students to adopt a constructive learning approach [ 18 ]. Given that productive feedback processes rely on observed information regarding a student's performance, it is imperative to establish structured teaching and learning feedback activities within undergraduate WBL settings.
Despite the extensive research on feedback, a significant proportion of published studies involve residents or postgraduate students [ 19 , 20 ]. Recent reviews focusing on feedback interventions within medical education have clearly distinguished between undergraduate medical students and residents or fellows [ 21 ]. To gain a comprehensive understanding of initiatives related to actionable feedback in the WBL environment for undergraduate health professions, a scoping review of the existing literature could provide insight into how feedback processes are designed in that context. Accordingly, the present scoping review aims to answer the following research question: How are the feedback processes designed in the undergraduate health professions' workplace-based learning environments?
A scoping review was conducted using the five-step methodological framework proposed by Arksey and O'Malley (2005) [ 22 ], intertwined with the PRISMA checklist extension for scoping reviews to provide reporting guidance for this specific type of knowledge synthesis [ 23 ]. Scoping reviews allow us to study the literature without restricting the methodological quality of the studies found, systematically and comprehensively map the literature, and identify gaps [ 24 ]. Furthermore, a scoping review was used because this topic is not suitable for a systematic review due to the varied approaches described and the large difference in the methodologies used [ 21 ].
Search strategy
With the collaboration of a medical librarian, the authors used the research question to guide the search strategy. An initial meeting was held to define keywords and search resources. The proposed search strategy was reviewed by the research team, and then the study selection was conducted in two steps:
An online database search included Medline/PubMed, Web of Science, CINAHL, Cochrane Library, Embase, ERIC, and PsycINFO.
A directed search of ten relevant journals in the health sciences education field (Academic Medicine, Medical Education, Advances in Health Sciences Education, Medical Teacher, Teaching and Learning in Medicine, Journal of Surgical Education, BMC Medical Education, Medical Education Online, Perspectives on Medical Education and The Clinical Teacher) was performed.
The research team conducted a pilot or initial search before the full search to identify if the topic was susceptible to a scoping review. The full search was conducted in November 2022. One team member (MO) identified the papers in the databases. JF searched in the selected journals. Authors included studies written in English due to feasibility issues, with no time span limitation. After eliminating duplicates, two research team members (JF and IV) independently reviewed all the titles and abstracts using the exclusion and inclusion criteria described in Table 2 and with the support of the screening application StArT [ 25 ]. A third team member (AR) reviewed the titles and abstracts when the first two disagreed. The reviewer team met again at a midpoint and final stage to discuss the challenges related to study selection. Articles included for full-text review were exported to Mendeley. JF independently screened all full-text papers, and AR verified 10% for inclusion. The authors did not analyze study quality or risk of bias during study selection, which is consistent with conducting a scoping review.
The analysis of the results incorporated a descriptive summary and a thematic analysis, which was carried out to clarify and give consistency to the results' reporting [ 22 , 24 , 26 ]. Quantitative data were analyzed to report the characteristics of the studies, populations, settings, methods, and outcomes. Qualitative data were labeled, coded, and categorized into themes by three team members (JF, SH, and DS). The feedback loop framework with a sociocultural perspective was used as the theoretical framework to analyze the results.
The keywords used for the search strategies were as follows:
Clinical clerkship; feedback; formative feedback; health professions; undergraduate medical education; workplace.
Definitions of the keywords used for the present review are available in Appendix 1 .
As an example, we included the search strategy that we used in the Medline/PubMed database when conducting the full search:
("Formative Feedback"[Mesh] OR feedback) AND ("Workplace"[Mesh] OR workplace OR "Clinical Clerkship"[Mesh] OR clerkship) AND (("Education, Medical, Undergraduate"[Mesh] OR undergraduate health profession*) OR (learner* medical education)).
Inclusion and exclusion criteria
The following inclusion and exclusion criteria were used (Table 1 ):
Data extraction
The research group developed a data-charting form to organize the information obtained from the studies. The process was iterative, as the data chart was continuously reviewed and improved as necessary. In addition, following Levac et al.'s recommendation (2010), the three members involved in the charting process (JF, LI, and IV) independently reviewed the first five selected studies to determine whether the data extraction was consistent with the objectives of this scoping review and to ensure consistency. Then, the team met using web-conferencing software (Zoom; CA, USA) to review the results and adjust any details in the chart. The same three members extracted data independently from all the selected studies, considering two members reviewing each paper [ 26 ]. A third team member was consulted if any conflict occurred when extracting data. The data chart identified demographic patterns and facilitated the data synthesis. To organize data, we used a shared Excel spreadsheet, considering the following headings: title, author(s), year of publication, journal/source, country/origin, aim of the study, research question (if any), population/sample size, participants, discipline, setting, methodology, study design, data collection, data analysis, intervention, outcomes, outcomes measure, key findings, and relation of findings to research question.
Additionally, all the included papers were uploaded to AtlasTi v19 to facilitate the qualitative analysis. Three team members (JF, SH, and DS) independently coded the first six papers to create a list of codes to ensure consistency and rigor. The group met several times to discuss and refine the list of codes. Then, one member of the team (JF) used the code list to code all the rest of the papers. Once all papers were coded, the team organized codes into descriptive themes aligned with the research question.
Preliminary results were shared with a number of stakeholders (six clinical teachers, ten students, six medical educators) to elicit their opinions as an opportunity to build on the evidence and offer a greater level of meaning, content expertise, and perspective to the preliminary findings [ 26 ]. No quality appraisal of the studies is considered for this scoping review, which aligns with the frameworks for guiding scoping reviews [ 27 ].
The datasets analyzed during the current study are available from the corresponding author upon request.
A database search resulted in 3,597 papers, and the directed search of the most relevant journals in the health sciences education field yielded 2,096 titles. An example of the results of one database is available in Appendix 2 . Of the titles obtained, 816 duplicates were eliminated, and the team reviewed the titles and abstracts of 4,877 papers. Of these, 120 were selected for full-text review. Finally, 61 papers were included in this scoping review (Fig. 1 ), as listed in Table 2 .
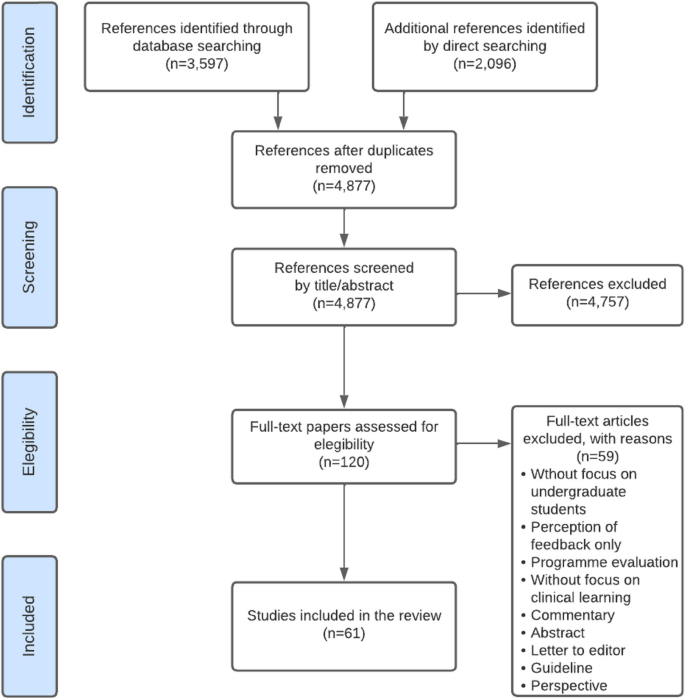
PRISMA flow diagram for included studies, incorporating records identified through the database and direct searching
The selected studies were published between 1986 and 2022, and seventy-five percent (46) were published during the last decade. Of all the articles included in this review, 13% (8) were literature reviews: one integrative review [ 28 ] and four scoping reviews [ 29 , 30 , 31 , 32 ]. Finally, fifty-three (87%) original or empirical papers were included (i.e., studies that answered a research question or achieved a research purpose through qualitative or quantitative methodologies) [ 15 , 33 , 34 , 35 , 36 , 37 , 38 , 39 , 40 , 41 , 42 , 43 , 44 , 45 , 46 , 47 , 48 , 49 , 50 , 51 , 52 , 53 , 54 , 55 , 56 , 57 , 58 , 59 , 60 , 61 , 62 , 63 , 64 , 65 , 66 , 67 , 68 , 69 , 70 , 71 , 72 , 73 , 74 , 75 , 76 , 77 , 78 , 79 , 80 , 81 , 82 , 83 , 84 , 85 ].
Table 2 summarizes the papers included in the present scoping review, and Table 3 describes the characteristics of the included studies.
The thematic analysis resulted in two themes: (1) the organization of feedback processes in WBL settings, and (2) sociocultural factors influencing the organization of feedback processes. Table 4 gives a summary of the themes and subthemes.
Organization of feedback processes in WBL settings.
Setting learning goals (i.e., feed-up dimension).
Feedback that focuses on students' learning needs and is based on known performance standards enhances student response and setting learning goals [ 30 ]. Discussing goals and agreements before starting clinical practice enhances students' feedback-seeking behavior [ 39 ] and responsiveness to feedback [ 83 ]. Farrell et al. (2017) found that teacher-learner co-constructed learning goals enhance feedback interactions and help establish educational alliances, improving the learning experience [ 50 ]. However, Kiger (2020) found that sharing individualized learning plans with teachers aligned feedback with learning goals but did not improve students' perceived use of feedback [ 64 ]
Two papers of this set pointed out the importance of goal-oriented feedback, a dynamic process that depends on discussion of goal setting between teachers and students [ 50 ] and influences how individuals experience, approach, and respond to upcoming learning activities [ 34 ]. Goal-oriented feedback should be embedded in the learning experience of the clinical workplace, as it can enhance students' engagement in safe feedback dialogues [ 50 ]. Ideally, each feedback encounter in the WBL context should conclude, in addition to setting a plan of action to achieve the desired goal, with a reflection on the next goal [ 50 ].
Feedback strategies within the WBL environment. (i.e., feedback dimension)
In undergraduate WBL environments, there are several tasks and feedback opportunities organized in the undergraduate clinical workplace that can enable feedback processes:
Questions from clinical teachers to students are a feedback strategy [ 74 ]. There are different types of questions that the teacher can use, either to clarify concepts, to reach the correct answer, or to facilitate self-correction [ 74 ]. Usually, questions can be used in conjunction with other communication strategies, such as pauses, which enable self-correction by the student [ 74 ]. Students can also ask questions to obtain feedback on their performance [ 54 ]. However, question-and-answer as a feedback strategy usually provides information on either correct or incorrect answers and fewer suggestions for improvement, rendering it less constructive as a feedback strategy [ 82 ].
Direct observation of performance by default is needed to be able to provide information to be used as input in the feedback process [ 33 , 46 , 49 , 86 ]. In the process of observation, teachers can include clarification of objectives (i.e., feed-up dimension) and suggestions for an action plan (i.e., feedforward) [ 50 ]. Accordingly, Schopper et al. (2016) showed that students valued being observed while interviewing patients, as they received feedback that helped them become more efficient and effective as interviewers and communicators [ 33 ]. Moreover, it is widely described that direct observation improves feedback credibility [ 33 , 40 , 84 ]. Ideally, observation should be deliberate [ 33 , 83 ], informal or spontaneous [ 33 ], conducted by a (clinical) expert [ 46 , 86 ], provided immediately after the observation, and clinical teacher if possible, should schedule or be alert on follow-up observations to promote closing the gap between current and desired performance [ 46 ].
Workplace-based assessments (WBAs), by definition, entail direct observation of performance during authentic task demonstration [ 39 , 46 , 56 , 87 ]. WBAs can significantly impact behavioral change in medical students [ 55 ]. Organizing and designing formative WBAs and embedding these in a feedback dialogue is essential for effective learning [ 31 ].
Summative organization of WBAs is a well described barrier for feedback uptake in the clinical workplace [ 35 , 46 ]. If feedback is perceived as summative, or organized as a pass-fail decision, students may be less inclined to use the feedback for future learning [ 52 ]. According to Schopper et al. (2016), using a scale within a WBA makes students shift their focus during the clinical interaction and see it as an assessment with consequences [ 33 ]. Harrison et al. (2016) pointed out that an environment that only contains assessments with a summative purpose will not lead to a culture of learning and improving performance [ 56 ]. The recommendation is to separate the formative and summative WBAs, as feedback in summative instances is often not recognized as a learning opportunity or an instance to seek feedback [ 54 ]. In terms of the design, an organizational format is needed to clarify to students how formative assessments can promote learning from feedback [ 56 ]. Harrison et al. (2016) identified that enabling students to have more control over their assessments, designing authentic assessments, and facilitating long-term mentoring could improve receptivity to formative assessment feedback [ 56 ].
Multiple WBA instruments and systems are reported in the literature. Sox et al. (2014) used a detailed evaluation form to help students improve their clinical case presentation skills. They found that feedback on oral presentations provided by supervisors using a detailed evaluation form improved clerkship students’ oral presentation skills [ 78 ]. Daelmans et al. (2006) suggested that a formal in-training assessment programme composed by 19 assessments that provided structured feedback, could promote observation and verbal feedback opportunities through frequent assessments [ 43 ]. However, in this setting, limited student-staff interactions still hindered feedback follow-up [ 43 ]. Designing frequent WBA improves feedback credibility [ 28 ]. Long et al. (2021) emphasized that students' responsiveness to assessment feedback hinges on its perceived credibility, underlining the importance of credibility for students to effectively engage and improve their performance [ 31 ].
The mini-CEX is one of the most widely described WBA instruments in the literature. Students perceive that the mini-CEX allows them to be observed and encourages the development of interviewing skills [ 33 ]. The mini-CEX can provide feedback that improves students' clinical skills [ 58 , 60 ], as it incorporates a structure for discussing the student's strengths and weaknesses and the design of a written action plan [ 39 , 80 ]. When mini-CEXs are incorporated as part of a system of WBA, such as programmatic assessment, students feel confident in seeking feedback after observation, and being systematic allows for follow-up [ 39 ]. Students suggested separating grading from observation and using the mini-CEX in more informal situations [ 33 ].
Clinical encounter cards allow students to receive weekly feedback and make them request more feedback as the clerkship progresses [ 65 ]. Moreover, encounter cards stimulate that feedback is given by supervisors, and students are more satisfied with the feedback process [ 72 ]. With encounter card feedback, students are responsible for asking a supervisor for feedback before a clinical encounter, and supervisors give students written and verbal comments about their performance after the encounter [ 42 , 72 ]. Encounter cards enhance the use of feedback and add approximately one minute to the length of the clinical encounter, so they are well accepted by students and supervisors [ 72 ]. Bennett (2006) identified that Instant Feedback Cards (IFC) facilitated mid-rotation feedback [ 38 ]. Feedback encounter card comments must be discussed between students and supervisors; otherwise, students may perceive it as impersonal, static, formulaic, and incomplete [ 59 ].
Self-assessments can change students' feedback orientation, transforming them into coproducers of learning [ 68 ]. Self-assessments promote the feedback process [ 68 ]. Some articles emphasize the importance of organizing self-assessments before receiving feedback from supervisors, for example, discussing their appraisal with the supervisor [ 46 , 52 ]. In designing a feedback encounter, starting with a self-assessment as feed-up, discussing with the supervisor, and identifying areas for improvement is recommended, as part of the feedback dialogue [ 68 ].
Peer feedback as an organized activity allows students to develop strategies to observe and give feedback to other peers [ 61 ]. Students can act as the feedback provider or receiver, fostering understanding of critical comments and promoting evaluative judgment for their clinical practice [ 61 ]. Within clerkships, enabling the sharing of feedback information among peers allows for a better understanding and acceptance of feedback [ 52 ]. However, students can find it challenging to take on the peer assessor/feedback provider role, as they prefer to avoid social conflicts [ 28 , 61 ]. Moreover, it has been described that they do not trust the judgment of their peers because they are not experts, although they know the procedures, tasks, and steps well and empathize with their peer status in the learning process [ 61 ].
Bedside-teaching encounters (BTEs) provide timely feedback and are an opportunity for verbal feedback during performance [ 74 ]. Rizan et al. (2014) explored timely feedback delivered within BTEs and determined that it promotes interaction that constructively enhances learner development through various corrective strategies (e.g., question and answers, pauses, etc.). However, if the feedback given during the BTEs was general, unspecific, or open-ended, it could go unnoticed [ 74 ]. Torre et al. (2005) investigated which integrated feedback activities and clinical tasks occurred on clerkship rotations and assessed students' perceived quality in each teaching encounter [ 81 ]. The feedback activities reported were feedback on written clinical history, physical examination, differential diagnosis, oral case presentation, a daily progress note, and bedside feedback. Students considered all these feedback activities high-quality learning opportunities, but they were more likely to receive feedback when teaching was at the bedside than at other teaching locations [ 81 ].
Case presentations are an opportunity for feedback within WBL contexts [ 67 , 73 ]. However, both students and supervisors struggled to identify them as feedback moments, and they often dismissed questions and clarifications around case presentations as feedback [ 73 ]. Joshi (2017) identified case presentations as a way for students to ask for informal or spontaneous supervisor feedback [ 63 ].
Organization of follow-up feedback and action plans (i.e., feedforward dimension).
Feedback that generates use and response from students is characterized by two-way communication and embedded in a dialogue [ 30 ]. Feedback must be future-focused [ 29 ], and a feedback encounter should be followed by planning the next observation [ 46 , 87 ]. Follow-up feedback could be organized as a future self-assessment, reflective practice by the student, and/or a discussion with the supervisor or coach [ 68 ]. The literature describes that a lack of student interaction with teachers makes follow-up difficult [ 43 ]. According to Haffling et al. (2011), follow-up feedback sessions improve students' satisfaction with feedback compared to students who do not have follow-up sessions. In addition, these same authors reported that a second follow-up session allows verification of improved performances or confirmation that the skill was acquired [ 55 ].
Although feedback encounter forms are a recognized way of obtaining information about performance (i.e., feedback dimension), the literature does not provide many clear examples of how they may impact the feedforward phase. For example, Joshi et al. (2016) consider a feedback form with four fields (i.e., what did you do well, advise the student on what could be done to improve performance, indicate the level of proficiency, and personal details of the tutor). In this case, the supervisor highlighted what the student could improve but not how, which is the missing phase of the co-constructed action plan [ 63 ]. Whichever WBA instrument is used in clerkships to provide feedback, it should include a "next steps" box [ 44 ], and it is recommended to organize a long-term use of the WBA instrument so that those involved get used to it and improve interaction and feedback uptake [ 55 ]. RIME-based feedback (Reporting, Interpreting, Managing, Educating) is considered an interesting example, as it is perceived as helpful to students in knowing what they need to improve in their performance [ 44 ]. Hochberg (2017) implemented formative mid-clerkship assessments to enhance face-to-face feedback conversations and co-create an improvement plan [ 59 ]. Apps for structuring and storing feedback improve the amount of verbal and written feedback. In the study of Joshi et al. (2016), a reasonable proportion of students (64%) perceived that these app tools help them improve their performance during rotations [ 63 ].
Several studies indicate that an action plan as part of the follow-up feedback is essential for performance improvement and learning [ 46 , 55 , 60 ]. An action plan corresponds to an agreed-upon strategy for improving, confirming, or correcting performance. Bing-You et al. (2017) determined that only 12% of the articles included in their scoping review incorporated an action plan for learners [ 32 ]. Holmboe et al. (2004) reported that only 11% of the feedback sessions following a mini-CEX included an action plan [ 60 ]. Suhoyo et al. (2017) also reported that only 55% of mini-CEX encounters contained an action plan [ 80 ]. Other authors reported that action plans are not commonly offered during feedback encounters [ 77 ]. Sokol-Hessner et al. (2010) implemented feedback card comments with a space to provide written feedback and a specific action plan. In their results, 96% contained positive comments, and only 5% contained constructive comments [ 77 ]. In summary, although the recommendation is to include a “next step” box in the feedback instruments, evidence shows these items are not often used for constructive comments or action plans.
Sociocultural factors influencing the organization of feedback processes.
Multiple sociocultural factors influence interaction in feedback encounters, promoting or hampering the productivity of the feedback processes.
Clinical learning culture
Context impacts feedback processes [ 30 , 82 ], and there are barriers to incorporating actionable feedback in the clinical learning context. The clinical learning culture is partly determined by the clinical context, which can be unpredictable [ 29 , 46 , 68 ], as the available patients determine learning opportunities. Supervisors are occupied by a high workload, which results in limited time or priority for teaching [ 35 , 46 , 48 , 55 , 68 , 83 ], hindering students’ feedback-seeking behavior [ 54 ], and creating a challenge for the balance between patient care and student mentoring [ 35 ].
Clinical workplace culture does not always purposefully prioritize instances for feedback processes [ 83 , 84 ]. This often leads to limited direct observation [ 55 , 68 ] and the provision of poorly informed feedback. It is also evident that this affects trust between clinical teachers and students [ 52 ]. Supervisors consider feedback a low priority in clinical contexts [ 35 ] due to low compensation and lack of protected time [ 83 ]. In particular, lack of time appears to be the most significant and well-known barrier to frequent observation and workplace feedback [ 35 , 43 , 48 , 62 , 67 , 83 ].
The clinical environment is hierarchical [ 68 , 80 ] and can make students not consider themselves part of the team and feel like a burden to their supervisor [ 68 ]. This hierarchical learning environment can lead to unidirectional feedback, limit dialogue during feedback processes, and hinder the seeking, uptake, and use of feedback [ 67 , 68 ]. In a learning culture where feedback is not supported, learners are less likely to want to seek it and feel motivated and engaged in their learning [ 83 ]. Furthermore, it has been identified that clinical supervisors lack the motivation to teach [ 48 ] and the intention to observe or reobserve performance [ 86 ].
In summary, the clinical context and WBL culture do not fully use the potential of a feedback process aimed at closing learning gaps. However, concrete actions shown in the literature can be taken to improve the effectiveness of feedback by organizing the learning context. For example, McGinness et al. (2022) identified that students felt more receptive to feedback when working in a safe, nonjudgmental environment [ 67 ]. Moreover, supervisors and trainees identified the learning culture as key to establishing an open feedback dialogue [ 73 ]. Students who perceive culture as supportive and formative can feel more comfortable performing tasks and more willing to receive feedback [ 73 ].
Relationships
There is a consensus in the literature that trusting and long-term relationships improve the chances of actionable feedback. However, relationships between supervisors and students in the clinical workplace are often brief and not organized as more longitudinally [ 68 , 83 ], leaving little time to establish a trustful relationship [ 68 ]. Supervisors change continuously, resulting in short interactions that limit the creation of lasting relationships over time [ 50 , 68 , 83 ]. In some contexts, it is common for a student to have several supervisors who have their own standards in the observation of performance [ 46 , 56 , 68 , 83 ]. A lack of stable relationships results in students having little engagement in feedback [ 68 ]. Furthermore, in case of summative assessment programmes, the dual role of supervisors (i.e., assessing and giving feedback) makes feedback interactions perceived as summative and can complicate the relationship [ 83 ].
Repeatedly, the articles considered in this review describe that long-term and stable relationships enable the development of trust and respect [ 35 , 62 ] and foster feedback-seeking behavior [ 35 , 67 ] and feedback-giver behavior [ 39 ]. Moreover, constructive and positive relationships enhance students´ use of and response to feedback [ 30 ]. For example, Longitudinal Integrated Clerkships (LICs) promote stable relationships, thus enhancing the impact of feedback [ 83 ]. In a long-term trusting relationship, feedback can be straightforward and credible [ 87 ], there are more opportunities for student observation, and the likelihood of follow-up and actionable feedback improves [ 83 ]. Johnson et al. (2020) pointed out that within a clinical teacher-student relationship, the focus must be on establishing psychological safety; thus, the feedback conversations might be transformed [ 62 ].
Stable relationships enhance feedback dialogues, which offer an opportunity to co-construct learning and propose and negotiate aspects of the design of learning strategies [ 62 ].
Students as active agents in the feedback processes
The feedback response learners generate depends on the type of feedback information they receive, how credible the source of feedback information is, the relationship between the receiver and the giver, and the relevance of the information delivered [ 49 ]. Garino (2020) noted that students who are most successful in using feedback are those who do not take criticism personally, who understand what they need to improve and know they can do so, who value and feel meaning in criticism, are not surprised to receive it, and who are motivated to seek new feedback and use effective learning strategies [ 52 ]. Successful users of feedback ask others for help, are intentional about their learning, know what resources to use and when to use them, listen to and understand a message, value advice, and use effective learning strategies. They regulate their emotions, find meaning in the message, and are willing to change [ 52 ].
Student self-efficacy influences the understanding and use of feedback in the clinical workplace. McGinness et al. (2022) described various positive examples of self-efficacy regarding feedback processes: planning feedback meetings with teachers, fostering good relationships with the clinical team, demonstrating interest in assigned tasks, persisting in seeking feedback despite the patient workload, and taking advantage of opportunities for feedback, e.g., case presentations [ 67 ].
When students are encouraged to seek feedback aligned with their own learning objectives, they promote feedback information specific to what they want to learn and improve and enhance the use of feedback [ 53 ]. McGinness et al. (2022) identified that the perceived relevance of feedback information influenced the use of feedback because students were more likely to ask for feedback if they perceived that the information was useful to them. For example, if students feel part of the clinical team and participate in patient care, they are more likely to seek feedback [ 17 ].
Learning-oriented students aim to seek feedback to achieve clinical competence at the expected level [ 75 ]; they focus on improving their knowledge and skills and on professional development [ 17 ]. Performance-oriented students aim not to fail and to avoid negative feedback [ 17 , 75 ].
For effective feedback processes, including feed-up, feedback, and feedforward, the student must be feedback-oriented, i.e., active, seeking, listening to, interpreting, and acting on feedback [ 68 ]. The literature shows that feedback-oriented students are coproducers of learning [ 68 ] and are more involved in the feedback process [ 51 ]. Additionally, students who are metacognitively aware of their learning process are more likely to use feedback to reduce gaps in learning and performance [ 52 ]. For this, students must recognize feedback when it occurs and understand it when they receive it. Thus, it is important to organize training and promote feedback literacy so that students understand what feedback is, act on it, and improve the quality of feedback and their learning plans [ 68 ].
Table 5 summarizes those feedback tasks, activities, and key features of organizational aspects that enable each phase of the feedback loop based on the literature review.
The present scoping review identified 61 papers that mapped the literature on feedback processes in the WBL environments of undergraduate health professions. This review explored how feedback processes are organized in these learning contexts using the feedback loop framework. Given the specific characteristics of feedback processes in undergraduate clinical learning, three main findings were identified on how feedback processes are being conducted in the clinical environment and how these processes could be organized to support feedback processes.
First, the literature lacks a balance between the three dimensions of the feedback loop. In this regard, most of the articles in this review focused on reporting experiences or strategies for delivering feedback information (i.e., feedback dimension). Credible and objective feedback information is based on direct observation [ 46 ] and occurs within an interaction or a dialogue [ 62 , 88 ]. However, only having credible and objective information does not ensure that it will be considered, understood, used, and put into practice by the student [ 89 ].
Feedback-supporting actions aligned with goals and priorities facilitate effective feedback processes [ 89 ] because goal-oriented feedback focuses on students' learning needs [ 7 ]. In contrast, this review showed that only a minority of the studies highlighted the importance of aligning learning objectives and feedback (i.e., the feed-up dimension). To overcome this, supervisors and students must establish goals and agreements before starting clinical practice, as it allows students to measure themselves on a defined basis [ 90 , 91 ] and enhances students' feedback-seeking behavior [ 39 , 92 ] and responsiveness to feedback [ 83 ]. In addition, learning goals should be shared, and co-constructed, through a dialogue [ 50 , 88 , 90 , 92 ]. In fact, relationship-based feedback models emphasize setting shared goals and plans as part of the feedback process [ 68 ].
Many of the studies acknowledge the importance of establishing an action plan and promoting the use of feedback (i.e., feedforward). However, there is yet limited insight on how to best implement strategies that support the use of action plans, improve performance and close learning gaps. In this regard, it is described that delivering feedback without perceiving changes, results in no effect or impact on learning [ 88 ]. To determine if a feedback loop is closed, observing a change in the student's response is necessary. In other words, feedback does not work without repeating the same task [ 68 ], so teachers need to observe subsequent tasks to notice changes [ 88 ]. While feedforward is fundamental to long-term performance, it is shown that more research is needed to determine effective actions to be implemented in the WBL environment to close feedback loops.
Second, there is a need for more knowledge about designing feedback activities in the WBL environment that will generate constructive feedback for learning. WBA is the most frequently reported feedback activity in clinical workplace contexts [ 39 , 46 , 56 , 87 ]. Despite the efforts of some authors to use WBAs as a formative assessment and feedback opportunity, in several studies, a summative component of the WBA was presented as a barrier to actionable feedback [ 33 , 56 ]. Students suggest separating grading from observation and using, for example, the mini-CEX in informal situations [ 33 ]. Several authors also recommend disconnecting the summative components of WBAs to avoid generating emotions that can limit the uptake and use of feedback [ 28 , 93 ]. Other literature recommends purposefully designing a system of assessment using low-stakes data points for feedback and learning. Accordingly, programmatic assessment is a framework that combines both the learning and the decision-making function of assessment [ 94 , 95 ]. Programmatic assessment is a practical approach for implementing low-stakes as a continuum, giving opportunities to close the gap between current and desired performance and having the student as an active agent [ 96 ]. This approach enables the incorporation of low-stakes data points that target student learning [ 93 ] and provide performance-relevant information (i.e., meaningful feedback) based on direct observations during authentic professional activities [ 46 ]. Using low-stakes data points, learners make sense of information about their performance and use it to enhance the quality of their work or performance [ 96 , 97 , 98 ]. Implementing multiple instances of feedback is more effective than providing it once because it promotes closing feedback loops by giving the student opportunities to understand the feedback, make changes, and see if those changes were effective [ 89 ].
Third, the support provided by the teacher is fundamental and should be built into a reliable and long-term relationship, where the teacher must take the role of coach rather than assessor, and students should develop feedback agency and be active in seeking and using feedback to improve performance. Although it is recognized that institutional efforts over the past decades have focused on training teachers to deliver feedback, clinical supervisors' lack of teaching skills is still identified as a barrier to workplace feedback [ 99 ]. In particular, research indicates that clinical teachers lack the skills to transform the information obtained from an observation into constructive feedback [ 100 ]. Students are more likely to use feedback if they consider it credible and constructive [ 93 ] and based on stable relationships [ 93 , 99 , 101 ]. In trusting relationships, feedback can be straightforward and credible, and the likelihood of follow-up and actionable feedback improves [ 83 , 88 ]. Coaching strategies can be enhanced by teachers building an educational alliance that allows for trustworthy relationships or having supervisors with an exclusive coaching role [ 14 , 93 , 102 ].
Last, from a sociocultural perspective, individuals are the main actors in the learning process. Therefore, feedback impacts learning only if students engage and interact with it [ 11 ]. Thus, feedback design and student agency appear to be the main features of effective feedback processes. Accordingly, the present review identified that feedback design is a key feature for effective learning in complex environments such as WBL. Feedback in the workplace must ideally be organized and implemented to align learning outcomes, learning activities, and assessments, allowing learners to learn, practice, and close feedback loops [ 88 ]. To guide students toward performances that reflect long-term learning, an intensive formative learning phase is needed, in which multiple feedback processes are included that shape students´ further learning [ 103 ]. This design would promote student uptake of feedback for subsequent performance [ 1 ].
Strengths and limitations
The strengths of this study are (1) the use of an established framework, the Arksey and O'Malley's framework [ 22 ]. We included the step of socializing the results with stakeholders, which allowed the team to better understand the results from another perspective and offer a realistic look. (2) Using the feedback loop as a theoretical framework strengthened the results and gave a more thorough explanation of the literature regarding feedback processes in the WBL context. (3) our team was diverse and included researchers from different disciplines as well as a librarian.
The present scoping review has several limitations. Although we adhered to the recommended protocols and methodologies, some relevant papers may have been omitted. The research team decided to select original studies and reviews of the literature for the present scoping review. This caused some articles, such as guidelines, perspectives, and narrative papers, to be excluded from the current study.
One of the inclusion criteria was a focus on undergraduate students. However, some papers that incorporated undergraduate and postgraduate participants were included, as these supported the results of this review. Most articles involved medical students. Although the authors did not limit the search to medicine, maybe some articles involving students from other health disciplines needed to be included, considering the search in other databases or journals.
The results give insight in how feedback could be organized within the clinical workplace to promote feedback processes. On a small scale, i.e., in the feedback encounter between a supervisor and a learner, feedback should be organized to allow for follow-up feedback, thus working on required learning and performance goals. On a larger level, i.e., in the clerkship programme or a placement rotation, feedback should be organized through appropriate planning of subsequent tasks and activities.
More insight is needed in designing a closed loop feedback process, in which specific attention is needed in effective feedforward practices. The feedback that stimulates further action and learning requires a safe and trustful work and learning environment. Understanding the relationship between an individual and his or her environment is a challenge for determining the impact of feedback and must be further investigated within clinical WBL environments. Aligning the dimensions of feed-up, feedback and feedforward includes careful attention to teachers’ and students’ feedback literacy to assure that students can act on feedback in a constructive way. In this line, how to develop students' feedback agency within these learning environments needs further research.
Boud D, Molloy E. Rethinking models of feedback for learning: The challenge of design. Assess Eval High Educ. 2013;38:698–712.
Article Google Scholar
Henderson M, Ajjawi R, Boud D, Molloy E. Identifying feedback that has impact. In: The Impact of Feedback in Higher Education. Springer International Publishing: Cham; 2019. p. 15–34.
Chapter Google Scholar
Winstone N, Carless D. Designing effective feedback processes in higher education: A learning-focused approach. 1st ed. New York: Routledge; 2020.
Google Scholar
Ajjawi R, Boud D. Assessment & Evaluation in Higher Education Researching feedback dialogue: an interactional analysis approach. 2015. https://doi.org/10.1080/02602938.2015.1102863 .
Carless D. Feedback loops and the longer-term: towards feedback spirals. Assess Eval High Educ. 2019;44:705–14.
Sadler DR. Formative assessment and the design of instructional systems. Instr Sci. 1989;18:119–44.
Hattie J, Timperley H. The Power of Feedback The Meaning of Feedback. Rev Educ Res. 2007;77:81–112.
Zarrinabadi N, Rezazadeh M. Why only feedback? Including feed up and feed forward improves nonlinguistic aspects of L2 writing. Language Teaching Research. 2023;27(3):575–92.
Fisher D, Frey N. Feed up, back, forward. Educ Leadersh. 2009;67:20–5.
Reimann A, Sadler I, Sambell K. What’s in a word? Practices associated with ‘feedforward’ in higher education. Assessment evaluation in higher education. 2019;44:1279–90.
Esterhazy R. Re-conceptualizing Feedback Through a Sociocultural Lens. In: Henderson M, Ajjawi R, Boud D, Molloy E, editors. The Impact of Feedback in Higher Education. Cham: Palgrave Macmillan; 2019. https://doi.org/10.1007/978-3-030-25112-3_5 .
Bransen D, Govaerts MJB, Sluijsmans DMA, Driessen EW. Beyond the self: The role of co-regulation in medical students’ self-regulated learning. Med Educ. 2020;54:234–41.
Ramani S, Könings KD, Ginsburg S, Van Der Vleuten CP. Feedback Redefined: Principles and Practice. J Gen Intern Med. 2019;34:744–53.
Atkinson A, Watling CJ, Brand PL. Feedback and coaching. Eur J Pediatr. 2022;181(2):441–6.
Suhoyo Y, Schonrock-Adema J, Emilia O, Kuks JBM, Cohen-Schotanus JA. Clinical workplace learning: perceived learning value of individual and group feedback in a collectivistic culture. BMC Med Educ. 2018;18:79.
Bowen L, Marshall M, Murdoch-Eaton D. Medical Student Perceptions of Feedback and Feedback Behaviors Within the Context of the “Educational Alliance.” Acad Med. 2017;92:1303–12.
Bok HGJ, Teunissen PW, Spruijt A, Fokkema JPI, van Beukelen P, Jaarsma DADC, et al. Clarifying students’ feedback-seeking behaviour in clinical clerkships. Med Educ. 2013;47:282–91.
Al-Kadri HM, Al-Kadi MT, Van Der Vleuten CPM. Workplace-based assessment and students’ approaches to learning: A qualitative inquiry. Med Teach. 2013;35(SUPPL):1.
Dennis AA, Foy MJ, Monrouxe LV, Rees CE. Exploring trainer and trainee emotional talk in narratives about workplace-based feedback processes. Adv Health Sci Educ. 2018;23:75–93.
Watling C, LaDonna KA, Lingard L, Voyer S, Hatala R. ‘Sometimes the work just needs to be done’: socio-cultural influences on direct observation in medical training. Med Educ. 2016;50:1054–64.
Bing-You R, Hayes V, Varaklis K, Trowbridge R, Kemp H, McKelvy D. Feedback for Learners in Medical Education: What is Known? A Scoping Review Academic Medicine. 2017;92:1346–54.
Arksey H, O’Malley L. Scoping studies: towards a methodological framework. Int J Soc Res Methodol. 2005;8:19–32.
Tricco AC, Lillie E, Zarin W, O’Brien KK, Colquhoun H, Levac D, et al. PRISMA extension for scoping reviews (PRISMA-ScR): Checklist and explanation. Ann Intern Med. 2018;169:467–73.
Colquhoun HL, Levac D, O’brien KK, Straus S, Tricco AC, Perrier L, et al. Scoping reviews: time for clarity in definition methods and reporting. J Clin Epidemiol. 2014;67:1291–4.
StArt - State of Art through Systematic Review. 2013.
Levac D, Colquhoun H, O’Brien KK. Scoping studies: Advancing the methodology. Implementation Science. 2010;5:1–9.
Peters MDJ, BPharm CMGHK, Parker PMD, Soares CB. Guidance for conducting systematic scoping reviews. Int J Evid Based Healthc. 2015;13:141–6.
Bing-You R, Varaklis K, Hayes V, Trowbridge R, Kemp H, McKelvy D, et al. The Feedback Tango: An Integrative Review and Analysis of the Content of the Teacher-Learner Feedback Exchange. Acad Med. 2018;93:657–63.
Ossenberg C, Henderson A, Mitchell M. What attributes guide best practice for effective feedback? A scoping review. Adv Health Sci Educ. 2019;24:383–401.
Spooner M, Duane C, Uygur J, Smyth E, Marron B, Murphy PJ, et al. Self -regulatory learning theory as a lens on how undergraduate and postgraduate learners respond to feedback: A BEME scoping review: BEME Guide No. 66. Med Teach. 2022;44:3–18.
Long S, Rodriguez C, St-Onge C, Tellier PP, Torabi N, Young M. Factors affecting perceived credibility of assessment in medical education: A scoping review. Adv Health Sci Educ. 2022;27:229–62.
Bing-You R, Hayes V, Varaklis K, Trowbridge R, Kemp H, McKelvy D. Feedback for Learners in Medical Education: What is Known? A Scoping Review: Lippincott Williams and Wilkins; 2017.
Schopper H, Rosenbaum M, Axelson R. “I wish someone watched me interview:” medical student insight into observation and feedback as a method for teaching communication skills during the clinical years. BMC Med Educ. 2016;16:286.
Crommelinck M, Anseel F. Understanding and encouraging feedback-seeking behaviour: a literature review. Med Educ. 2013;47:232–41.
Adamson E, Kinga L, Foy L, McLeodb M, Traynor J, Watson W, et al. Feedback in clinical practice: Enhancing the students’ experience through action research. Nurse Educ Pract. 2018;31:48–53.
Al-Mously N, Nabil NM, Al-Babtain SA, et al. Undergraduate medical students’ perceptions on the quality of feedback received during clinical rotations. Med Teach. 2014;36(Supplement 1):S17-23.
Bates J, Konkin J, Suddards C, Dobson S, Pratt D. Student perceptions of assessment and feedback in longitudinal integrated clerkships. Med Educ. 2013;47:362–74.
Bennett AJ, Goldenhar LM, Stanford K. Utilization of a Formative Evaluation Card in a Psychiatry Clerkship. Acad Psychiatry. 2006;30:319–24.
Bok HG, Jaarsma DA, Spruijt A, Van Beukelen P, Van Der Vleuten CP, Teunissen PW, et al. Feedback-giving behaviour in performance evaluations during clinical clerkships. Med Teach. 2016;38:88–95.
Bok HG, Teunissen PW, Spruijt A, Fokkema JP, van Beukelen P, Jaarsma DA, et al. Clarifying students’ feedback-seeking behaviour in clinical clerkships. Med Educ. 2013;47:282–91.
Calleja P, Harvey T, Fox A, Carmichael M, et al. Feedback and clinical practice improvement: A tool to assist workplace supervisors and students. Nurse Educ Pract. 2016;17:167–73.
Carey EG, Wu C, Hur ES, Hasday SJ, Rosculet NP, Kemp MT, et al. Evaluation of Feedback Systems for the Third-Year Surgical Clerkship. J Surg Educ. 2017;74:787–93.
Daelmans HE, Overmeer RM, Van der Hem-Stokroos HH, Scherpbier AJ, Stehouwer CD, van der Vleuten CP. In-training assessment: qualitative study of effects on supervision and feedback in an undergraduate clinical rotation. Medical education. 2006;40(1):51–8.
DeWitt D, Carline J, Paauw D, Pangaro L. Pilot study of a ’RIME’-based tool for giving feedback in a multi-specialty longitudinal clerkship. Med Educ. 2008;42:1205–9.
Dolan BM, O’Brien CL, Green MM. Including Entrustment Language in an Assessment Form May Improve Constructive Feedback for Student Clinical Skills. Med Sci Educ. 2017;27:461–4.
Duijn CC, Welink LS, Mandoki M, Ten Cate OT, Kremer WD, Bok HG. Am I ready for it? Students’ perceptions of meaningful feedback on entrustable professional activities. Perspectives on medical education. 2017;6:256–64.
Elnicki DM, Zalenski D. Integrating medical students’ goals, self-assessment and preceptor feedback in an ambulatory clerkship. Teach Learn Med. 2013;25:285–91.
Embo MP, Driessen EW, Valcke M, Van der Vleuten CP. Assessment and feedback to facilitate self-directed learning in clinical practice of Midwifery students. Medical teacher. 2010;32(7):e263-9.
Eva KW, Armson H, Holmboe E, Lockyer J, Loney E, Mann K, et al. Factors influencing responsiveness to feedback: On the interplay between fear, confidence, and reasoning processes. Adv Health Sci Educ. 2012;17:15–26.
Farrell L, Bourgeois-Law G, Ajjawi R, Regehr G. An autoethnographic exploration of the use of goal oriented feedback to enhance brief clinical teaching encounters. Adv Health Sci Educ. 2017;22:91–104.
Fernando N, Cleland J, McKenzie H, Cassar K. Identifying the factors that determine feedback given to undergraduate medical students following formative mini-CEX assessments. Med Educ. 2008;42:89–95.
Garino A. Ready, willing and able: a model to explain successful use of feedback. Adv Health Sci Educ. 2020;25:337–61.
Garner MS, Gusberg RJ, Kim AW. The positive effect of immediate feedback on medical student education during the surgical clerkship. J Surg Educ. 2014;71:391–7.
Bing-You R, Hayes V, Palka T, Ford M, Trowbridge R. The Art (and Artifice) of Seeking Feedback: Clerkship Students’ Approaches to Asking for Feedback. Acad Med. 2018;93:1218–26.
Haffling AC, Beckman A, Edgren G. Structured feedback to undergraduate medical students: 3 years’ experience of an assessment tool. Medical teacher. 2011;33(7):e349-57.
Harrison CJ, Könings KD, Dannefer EF, Schuwirth LWTT, Wass V, van der Vleuten CPMM. Factors influencing students’ receptivity to formative feedback emerging from different assessment cultures. Perspect Med Educ. 2016;5:276–84.
Harrison CJ, Könings KD, Schuwirth LW, Wass V, Van der Vleuten CP, Konings KD, et al. Changing the culture of assessment: the dominance of the summative assessment paradigm. BMC medical education. 2017;17:1–4.
Harvey P, Radomski N, O’Connor D. Written feedback and continuity of learning in a geographically distributed medical education program. Medical teacher. 2013;35(12):1009–13.
Hochberg M, Berman R, Ogilvie J, Yingling S, Lee S, Pusic M, et al. Midclerkship feedback in the surgical clerkship: the “Professionalism, Reporting, Interpreting, Managing, Educating, and Procedural Skills” application utilizing learner self-assessment. Am J Surg. 2017;213:212–6.
Holmboe ES, Yepes M, Williams F, Huot SJ. Feedback and the mini clinical evaluation exercise. Journal of general internal medicine. 2004;19(5):558–61.
Tai JHM, Canny BJ, Haines TP, Molloy EK. The role of peer-assisted learning in building evaluative judgement: opportunities in clinical medical education. Adv Health Sci Educ. 2016;21:659–76.
Johnson CE, Keating JL, Molloy EK. Psychological safety in feedback: What does it look like and how can educators work with learners to foster it? Med Educ. 2020;54:559–70.
Joshi A, Generalla J, Thompson B, Haidet P. Facilitating the Feedback Process on a Clinical Clerkship Using a Smartphone Application. Acad Psychiatry. 2017;41:651–5.
Kiger ME, Riley C, Stolfi A, Morrison S, Burke A, Lockspeiser T. Use of Individualized Learning Plans to Facilitate Feedback Among Medical Students. Teach Learn Med. 2020;32:399–409.
Kogan J, Shea J. Implementing feedback cards in core clerkships. Med Educ. 2008;42:1071–9.
Lefroy J, Walters B, Molyneux A, Smithson S. Can learning from workplace feedback be enhanced by reflective writing? A realist evaluation in UK undergraduate medical education. Educ Prim Care. 2021;32:326–35.
McGinness HT, Caldwell PHY, Gunasekera H, Scott KM. ‘Every Human Interaction Requires a Bit of Give and Take’: Medical Students’ Approaches to Pursuing Feedback in the Clinical Setting. Teach Learn Med. 2022. https://doi.org/10.1080/10401334.2022.2084401 .
Noble C, Billett S, Armit L, Collier L, Hilder J, Sly C, et al. ``It’s yours to take{’’}: generating learner feedback literacy in the workplace. Adv Health Sci Educ. 2020;25:55–74.
Ogburn T, Espey E. The R-I-M-E method for evaluation of medical students on an obstetrics and gynecology clerkship. Am J Obstet Gynecol. 2003;189:666–9.
Po O, Reznik M, Greenberg L. Improving a medical student feedback with a clinical encounter card. Ambul Pediatr. 2007;7:449–52.
Parkes J, Abercrombie S, McCarty T, Parkes J, Abercrombie S, McCarty T. Feedback sandwiches affect perceptions but not performance. Adv Health Sci Educ. 2013;18:397–407.
Paukert JL, Richards ML, Olney C. An encounter card system for increasing feedback to students. Am J Surg. 2002;183:300–4.
Rassos J, Melvin LJ, Panisko D, Kulasegaram K, Kuper A. Unearthing Faculty and Trainee Perspectives of Feedback in Internal Medicine: the Oral Case Presentation as a Model. J Gen Intern Med. 2019;34:2107–13.
Rizan C, Elsey C, Lemon T, Grant A, Monrouxe L. Feedback in action within bedside teaching encounters: a video ethnographic study. Med Educ. 2014;48:902–20.
Robertson AC, Fowler LC. Medical student perceptions of learner-initiated feedback using a mobile web application. Journal of medical education and curricular development. 2017;4:2382120517746384.
Scheidt PC, Lazoritz S, Ebbeling WL, Figelman AR, Moessner HF, Singer JE. Evaluation of system providing feedback to students on videotaped patient encounters. J Med Educ. 1986;61(7):585–90.
Sokol-Hessner L, Shea J, Kogan J. The open-ended comment space for action plans on core clerkship students’ encounter cards: what gets written? Acad Med. 2010;85:S110–4.
Sox CM, Dell M, Phillipi CA, Cabral HJ, Vargas G, Lewin LO. Feedback on oral presentations during pediatric clerkships: a randomized controlled trial. Pediatrics. 2014;134:965–71.
Spickard A, Gigante J, Stein G, Denny JC. Automatic capture of student notes to augment mentor feedback and student performance on patient write-ups. J Gen Intern Med. 2008;23:979–84.
Suhoyo Y, Van Hell EA, Kerdijk W, Emilia O, Schönrock-Adema J, Kuks JB, et al. nfluence of feedback characteristics on perceived learning value of feedback in clerkships: does culture matter? BMC medical education. 2017;17:1–7.
Torre DM, Simpson D, Sebastian JL, Elnicki DM. Learning/feedback activities and high-quality teaching: perceptions of third-year medical students during an inpatient rotation. Acad Med. 2005;80:950–4.
Urquhart LM, Ker JS, Rees CE. Exploring the influence of context on feedback at medical school: a video-ethnography study. Adv Health Sci Educ. 2018;23:159–86.
Watling C, Driessen E, van der Vleuten C, Lingard L. Learning culture and feedback: an international study of medical athletes and musicians. Med Educ. 2014;48:713–23.
Watling C, Driessen E, van der Vleuten C, Vanstone M, Lingard L. Beyond individualism: Professional culture and its influence on feedback. Med Educ. 2013;47:585–94.
Soemantri D, Dodds A, Mccoll G. Examining the nature of feedback within the Mini Clinical Evaluation Exercise (Mini-CEX): an analysis of 1427 Mini-CEX assessment forms. GMS J Med Educ. 2018;35:Doc47.
Van De Ridder JMMM, Stokking KM, McGaghie WC, ten Cate OTJ, van der Ridder JM, Stokking KM, et al. What is feedback in clinical education? Med Educ. 2008;42:189–97.
van de Ridder JMMM, McGaghie WC, Stokking KM, ten Cate OTJJ. Variables that affect the process and outcome of feedback, relevant for medical training: a meta-review. Med Educ. 2015;49:658–73.
Boud D. Feedback: ensuring that it leads to enhanced learning. Clin Teach. 2015. https://doi.org/10.1111/tct.12345 .
Brehaut J, Colquhoun H, Eva K, Carrol K, Sales A, Michie S, et al. Practice feedback interventions: 15 suggestions for optimizing effectiveness. Ann Intern Med. 2016;164:435–41.
Ende J. Feedback in clinical medical education. J Am Med Assoc. 1983;250:777–81.
Cantillon P, Sargeant J. Giving feedback in clinical settings. Br Med J. 2008;337(7681):1292–4.
Norcini J, Burch V. Workplace-based assessment as an educational tool: AMEE Guide No. 31. Med Teach. 2007;29:855–71.
Watling CJ, Ginsburg S. Assessment, feedback and the alchemy of learning. Med Educ. 2019;53:76–85.
van der Vleuten CPM, Schuwirth LWT, Driessen EW, Dijkstra J, Tigelaar D, Baartman LKJ, et al. A model for programmatic assessment fit for purpose. Med Teach. 2012;34:205–14.
Schuwirth LWT, der Vleuten CPM. Programmatic assessment: from assessment of learning to assessment for learning. Med Teach. 2011;33:478–85.
Schut S, Driessen E, van Tartwijk J, van der Vleuten C, Heeneman S. Stakes in the eye of the beholder: an international study of learners’ perceptions within programmatic assessment. Med Educ. 2018;52:654–63.
Henderson M, Boud D, Molloy E, Dawson P, Phillips M, Ryan T, Mahoney MP. Feedback for learning. Closing the assessment loop. Framework for effective learning. Canberra, Australia: Australian Government, Department for Education and Training; 2018.
Heeneman S, Pool AO, Schuwirth LWT, van der Vleuten CPM, Driessen EW, Oudkerk A, et al. The impact of programmatic assessment on student learning: theory versus practice. Med Educ. 2015;49:487–98.
Lefroy J, Watling C, Teunissen P, Brand P, Watling C. Guidelines: the do’s, don’ts and don’t knows of feedback for clinical education. Perspect Med Educ. 2015;4:284–99.
Ramani S, Krackov SK. Twelve tips for giving feedback effectively in the clinical environment. Med Teach. 2012;34:787–91.
Telio S, Ajjawi R, Regehr G. The, “Educational Alliance” as a Framework for Reconceptualizing Feedback in Medical Education. Acad Med. 2015;90:609–14.
Lockyer J, Armson H, Könings KD, Lee-Krueger RC, des Ordons AR, Ramani S, et al. In-the-Moment Feedback and Coaching: Improving R2C2 for a New Context. J Grad Med Educ. 2020;12:27–35.
Black P, Wiliam D. Developing the theory of formative assessment. Educ Assess Eval Account. 2009;21:5–31.
Download references
Author information
Authors and affiliations.
Department of Health Sciences, Faculty of Medicine, Pontificia Universidad Católica de Chile, Avenida Vicuña Mackenna 4860, Macul, Santiago, Chile
Javiera Fuentes-Cimma & Ignacio Villagran
School of Health Professions Education, Maastricht University, Maastricht, Netherlands
Javiera Fuentes-Cimma & Lorena Isbej
Rotterdam University of Applied Sciences, Rotterdam, Netherlands
Dominique Sluijsmans
Centre for Medical and Health Profession Education, Department of Gastroenterology, Faculty of Medicine, Pontificia Universidad Católica de Chile, Santiago, Chile
Arnoldo Riquelme
School of Dentistry, Faculty of Medicine, Pontificia Universidad Católica de Chile, Santiago, Chile
Lorena Isbej
Sistema de Bibliotecas UC (SIBUC), Pontificia Universidad Católica de Chile, Santiago, Chile
María Teresa Olivares-Labbe
Department of Pathology, Faculty of Health, Medicine and Health Sciences, Maastricht University, Maastricht, Netherlands
Sylvia Heeneman
You can also search for this author in PubMed Google Scholar
Contributions
J.F-C, D.S, and S.H. made substantial contributions to the conception and design of the work. M.O-L contributed to the identification of studies. J.F-C, I.V, A.R, and L.I. made substantial contributions to the screening, reliability, and data analysis. J.F-C. wrote th e main manuscript text. All authors reviewed the manuscript.
Corresponding author
Correspondence to Javiera Fuentes-Cimma .
Ethics declarations
Competing interests.
The authors declare no competing interests.
Additional information
Publisher’s note.
Springer Nature remains neutral with regard to jurisdictional claims in published maps and institutional affiliations.
Supplementary Information
Supplementary material 1, supplementary material 2., rights and permissions.
Open Access This article is licensed under a Creative Commons Attribution 4.0 International License, which permits use, sharing, adaptation, distribution and reproduction in any medium or format, as long as you give appropriate credit to the original author(s) and the source, provide a link to the Creative Commons licence, and indicate if changes were made. The images or other third party material in this article are included in the article's Creative Commons licence, unless indicated otherwise in a credit line to the material. If material is not included in the article's Creative Commons licence and your intended use is not permitted by statutory regulation or exceeds the permitted use, you will need to obtain permission directly from the copyright holder. To view a copy of this licence, visit http://creativecommons.org/licenses/by/4.0/ . The Creative Commons Public Domain Dedication waiver ( http://creativecommons.org/publicdomain/zero/1.0/ ) applies to the data made available in this article, unless otherwise stated in a credit line to the data.
Reprints and permissions
About this article
Cite this article.
Fuentes-Cimma, J., Sluijsmans, D., Riquelme, A. et al. Designing feedback processes in the workplace-based learning of undergraduate health professions education: a scoping review. BMC Med Educ 24 , 440 (2024). https://doi.org/10.1186/s12909-024-05439-6
Download citation
Received : 25 September 2023
Accepted : 17 April 2024
Published : 23 April 2024
DOI : https://doi.org/10.1186/s12909-024-05439-6
Share this article
Anyone you share the following link with will be able to read this content:
Sorry, a shareable link is not currently available for this article.
Provided by the Springer Nature SharedIt content-sharing initiative
- Clinical clerkship
- Feedback processes
- Feedforward
- Formative feedback
- Health professions
- Undergraduate medical education
- Undergraduate healthcare education
- Workplace learning
BMC Medical Education
ISSN: 1472-6920
- Submission enquiries: [email protected]
- General enquiries: [email protected]
REVIEW article
This article is part of the research topic.
Vol II: Person-Centred Rehabilitation – Theory, Practice and Research
Hope as experienced by people with acquired brain injury in a rehabilitationor recovery process: A qualitative systematic review and thematic synthesis Provisionally Accepted
- 1 Aalborg University, Denmark
- 2 Municipality of Copenhagen, Denmark
The final, formatted version of the article will be published soon.
Background: There has been an increasing interest in the concept of hope within the field of brain injury rehabilitation. Existing reviews have nevertheless focused on stroke, leaving out the broad populationgroup of people with acquired brain injury (ABI). Furthermore a just as majority of the included studies in those reviews excluded the subgroup of people with communication difficulties, thus primarily giving voice to a select group of people with ABI. Methods: A qualitative systematic review was conducted with the purpose of systematically reviewing and thematically synthesise findings about hope as experienced by adultspeople with ABI in a rehabilitation or recovery process. The search strategy included peer-reviewed qualitative studies published after 2000 in English or Scandinavian languages. Searches of EBSCO databases incorporating CINAHL, MEDLINE, and PsycINFO were conducted together with SocINDEX, Social Work Abstracts, Eric and Web of Science. Ten qualitative studies were included, and the Critical Appraisal Skills Program (CASP) was used for assessing the quality and relevance of the ten studies. Qualitative findings were synthesized using Thomas and Harden's methodology. data were analysed based on methods for thematic synthesis by Thomas and Harden. Results: Through a thematic synthesis eleven subthemes were identifiedemerged relating to experiences of hope. These were grouped into four analytical themes: (1) Hope a two folded phenomenon; (2) Time and temporality; (3) Progress, goals and visibility and (4) The alliance. Conclusion: This review has shown that even though hope has both a positive and negative side to it, it is necessary as a driving force for people with ABI in terms of supporting them to keep going and not give up. Rehabilitation professionals are advised to embrace the ambiguity of hope, customizing the support of hope to each person with ABI. Attention is needed on how to make progress visible for persons with ABI during their rehabilitation process just as rehabilitation professionals should acknowledge the alliance with the person with ABI as a core component of rehabilitation. This requires a focus on professionals' communication skills if hope promoting relationships between professionals and persons with ABI are to be achieved.
Keywords: hope, acquired brain injury, Rehabilitation, Recovery, literature review, qualitative studies, thematic synthesis
Received: 26 Jan 2024; Accepted: 26 Apr 2024.
Copyright: © 2024 Højgaard Nejst and Glintborg. This is an open-access article distributed under the terms of the Creative Commons Attribution License (CC BY) . The use, distribution or reproduction in other forums is permitted, provided the original author(s) or licensor are credited and that the original publication in this journal is cited, in accordance with accepted academic practice. No use, distribution or reproduction is permitted which does not comply with these terms.
* Correspondence: PhD. Camilla Højgaard Nejst, Aalborg University, Aalborg, Denmark
People also looked at
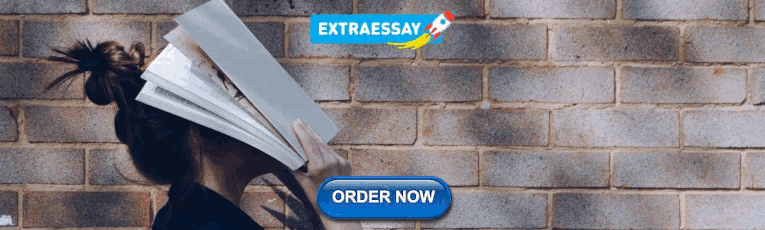
IMAGES
VIDEO
COMMENTS
This article is a practical guide to conducting data analysis in general literature reviews. The general literature review is a synthesis and analysis of published research on a relevant clinical issue, and is a common format for academic theses at the bachelor's and master's levels in nursing, physiotherapy, occupational therapy, public health and other related fields.
The methodology of data synthesis in a review of the latter type of prognosis is comparable to the methodology of aetiological reviews. For that reason, in the present study we only focused on reviews of outcome prediction studies. ... a systematic review and literature synthesis. Pain Med. 2009, 10: 639-653. 10.1111/j.1526-4637.2009.00632.x.
Similarly, within conventional literature synthesis the units of analysis also depend on the research purpose, focus and question as well as on the type of research methods incorporated into the review. ... It is often proposed as an intermediary step to be followed by a more comprehensive review. Data type: The literature is often narrowly ...
Similar to textual narrative synthesis, a scoping review (Arksey and O'Malley 2005) aims to extract as much relevant data from each piece of literature as possible—including methodology, finding, variables, etc.—since the aim of the review is to provide a snapshot of the field and a complete overview of what has been done.
This document aims to assist authors in planning their narrative analysis at protocol stage, and to highlight some issues for authors to consider at review stage. Narrative forms of synthesis are an area of emerging research, and so advice is likely to be adapted as methods develop. This document sits alongside the RevMan templates for ...
Summary tables were used for data extraction and researcher-developed questions-based process for synthesis. Data analysis and synthesis methods were categorized as non-specific, inductive, deductive, and framework-based. Majority of reviews did not explicitly delineate data analysis and synthesis methods (n = 67) or used inductive methods (n ...
1.1. Automated Data Extraction and Synthesis . Systematic reviews are conducted in a multistep process as illustrated in Figure 1, each step following a systematic and highly controlled procedure to ensure close to perfect recall, and a minimum of mistakes.The data extracted from the identified studies is pooled and synthesized, and the conclusions of the review are based on this synthesis.
Develop a table that summarizes the various specific approaches to data analysis and synthesis that you could use to help you select methods in future reviews (you can use those listed in this chapter, those in Chapter 1, and those from other sources). Each row represents a method, such as meta-analysis, realist synthesis, meta-study, etc.
The term literature review has been used to refer to both a review of the literature and a knowledge synthesis (Maggio et al., 2018; Siddaway et al., 2019). For our purposes, we employ the term as commonly used to refer to a knowledge synthesis , which is a formal comprehensive review of the existing body of literature on a topic.
Strategy for Data Synthesis. in Systematic Review. Automate every stage of your literature review to produce evidence-based research faster and more accurately. The purpose of a data extraction table within a systematic review becomes apparent during synthesis, where reviewers collate and evaluate the meaning of the data gathered. Synthesis ...
Synthesis Methods. Narrative summary: is a summary of the review results when meta-analysis is not possible.Narrative summaries describe the results of the review, but some can take a more interpretive approach in summarising the results. [8] These are known as "evidence statements" and can include the results of quality appraisal and weighting processes and provide the ratings of the studies.
Whether or not your Systematic Review includes a full meta-analysis, there is typically some element of data analysis. The quantitative synthesis combines and analyzes the evidence using statistical techniques. This includes comparing methodological similarities and differences and potentially the quality of the studies conducted.
Data synthesis overview. Now that you have extracted your data, the next step is to synthesise the data. Move through the slide deck below to learn about data synthesis. Alternatively, download the PDF document at the bottom of this box. This document is a printable version of the slide deck above.
JBI Sumari (the Joanna Briggs Institute System for the United Management, Assessment and Review of Information) is a systematic review software platform geared toward fields such as health, social sciences, and humanities. Among the other steps of a review project, it facilitates data extraction and data synthesis.
Data extraction is the process of a systematic review that occurs between identifying eligible studies and analysing the data, whether it can be a qualitative synthesis or a quantitative synthesis involving the pooling of data in a meta-analysis. The aims of data extraction are to obtain information about the included studies in terms of the characteristics of each study and its population and ...
According to the Royal Society, 'evidence synthesis' refers to the process of bringing together information from a range of sources and disciplines to inform debates and decisions on specific issues. They generally include a methodical and comprehensive literature synthesis focused on a well-formulated research question.
The key aspects of a scoping review project highlighted in this paper include: •. Ensuring a team-based approach with representative expertize in the topic, methods and literature search requirements; •. Including a pre-planning phase to confirm the methodology is a good fit for the project objectives ( Figure 1 ); •.
The methodology of data synthesis in a review of the latter type of prognosis is comparable to the methodology of aetiological reviews. For that reason, in the present study we only focused on reviews of outcome prediction studies. ... a systematic review and literature synthesis. Pain Med. 2009; 10:639-653. doi: 10.1111/j.1526-4637.2009.00632.x.
The synthesis part of a systematic review will determine the outcomes of the review. There are two commonly accepted methods of synthesis in systematic reviews: Quantitative data synthesis. Qualitative data synthesis. The way the data is extracted from your studies and synthesised and presented depends on the type of data being handled.
This constitutes the first dataset describing the later stages of the DTA systematic review process, and is intended to be useful for automating or evaluating the process. ... Data Extraction and Synthesis in Systematic Reviews of Diagnostic Test Accuracy: A Corpus for Automating and Evaluating the Process AMIA Annu Symp Proc. 2018 Dec 5;2018: ...
2. Scope the Literature. A "scoping search" investigates the breadth and/or depth of the initial question or may identify a gap in the literature. Eligible studies may be located by searching in: Background sources (books, point-of-care tools) Article databases; Trial registries; Grey literature; Cited references; Reference lists
Synthesising the data. Synthesis is a stage in the systematic review process where extracted data, that is the findings of individual studies, are combined and evaluated. The general purpose of extracting and synthesising data is to show the outcomes and effects of various studies, and to identify issues with methodology and quality.
A review of a clearly formulated question that uses systematic and explicit methods to identify, select, and critically appraise relevant research, and to collect and analyse data from the studies that are included in the review. Statistical methods ( meta-analysis) may or may not be used to analyse and summarise the results of the included ...
This article is a practical guide to conducting data analysis in general literature reviews. The general literature review is a synthesis and analysis of published research on a rel-evant clinical issue, and is a common format for academic theses at the bachelor's and master's levels in nursing, physiotherapy, occupational therapy, public ...
This living review will summarize the evidence on the use of ML and AI tools in the conduct of any specific aspects of ESPs commonly produced by EPCs (e.g., abstract screening, data extraction, summary writing). The intended audience includes evidence synthesis practitioners, tool developers, and evidence synthesis methods developers. For the purpose of this review, we define a tool as a ...
This review analyzed over 200 papers published in 2000 to examine various tools to support wastewater management decision-making. We also investigated technical, environmental, economic, and social assessment methodologies to prepare the information needed to inform these decisions. Through this review, the following points were found:
(2) search strategy and article screening; (3) data extraction and critical appraisal; and (4) synthesis and reporting (James et al., 2016; London et al., 2020). In short, literature mapping is the step that aids in the creation of a database of literature for an actual literature review process.
A scoping review was conducted using the five-step methodological framework proposed by Arksey and O'Malley (2005) [], intertwined with the PRISMA checklist extension for scoping reviews to provide reporting guidance for this specific type of knowledge synthesis [].Scoping reviews allow us to study the literature without restricting the methodological quality of the studies found ...
The review team were unable to discern the data, leading to the decision to include these studies in the synthesis because of their relevance to the review question. However, caution in generalizing these results to the broader population of disabled veterans is advised.
Qualitative findings were synthesized using Thomas and Harden's methodology. data were analysed based on methods for thematic synthesis by Thomas and Harden. ... Through a thematic synthesis eleven subthemes were identifiedemerged relating to experiences of hope. ... acquired brain injury, Rehabilitation, Recovery, literature review ...